Table Of Contents
Category
Machine Learning (ML)
Artificial Intelligence (AI)
Decentralized Finance (DeFi)
Manufacturing
1. Introduction to AI in Production Planning
Artificial Intelligence (AI) is revolutionizing various industries, and production planning is no exception. The integration of AI in production planning and scheduling processes enhances efficiency, reduces costs, and improves decision-making. AI technologies, such as machine learning and predictive analytics, enable manufacturers to analyze vast amounts of data quickly. These technologies help in forecasting demand, optimizing inventory levels, and streamlining production processes. Additionally, AI can adapt to changing market conditions, allowing for more agile production planning.
The traditional methods of production planning often rely on manual processes and historical data, which can lead to inefficiencies. AI addresses these challenges by providing real-time insights and automating routine tasks. Enhanced data analysis capabilities allow for better demand forecasting. AI algorithms can identify patterns and trends that human planners might overlook. Furthermore, automation of scheduling tasks reduces the time spent on manual adjustments.
At Rapid Innovation, we specialize in implementing AI for production planning solutions tailored to your needs. By leveraging our expertise, clients have experienced significant improvements in their operational efficiency and overall productivity. For instance, one of our clients in the manufacturing sector saw a 30% reduction in inventory costs and a 25% increase in production throughput after integrating our AI-driven solutions.
Incorporating AI into production planning not only improves operational efficiency but also enhances overall productivity. As businesses strive to remain competitive, leveraging AI in production planning becomes essential for optimizing production processes. Rapid Innovation is here to guide you through this transformation, ensuring you achieve greater ROI and meet your business goals effectively.
Refer to the image for a visual representation of how AI enhances production planning processes.

1.1. Defining Production Planning and Scheduling
Production planning and scheduling are critical components of manufacturing operations. They involve the strategic organization of resources, processes, and timelines to ensure that products are produced efficiently and meet customer demand.
- Production Planning: This is the process of determining what to produce, how much to produce, and when to produce it. It includes forecasting demand to align production with market needs, allocating resources such as labor, materials, and machinery, and setting production goals and timelines. Advanced planning and scheduling techniques are often employed to enhance this process.
- Scheduling: This refers to the detailed timetable for production activities. It includes assigning specific tasks to workers and machines, determining the sequence of operations to optimize workflow, and adjusting schedules based on real-time data and changes in demand. Production scheduling software can assist in creating and managing these schedules effectively.
Effective production planning and scheduling can lead to reduced lead times, lower inventory costs, and improved customer satisfaction. Tools such as advanced planning systems and manufacturing scheduling software are essential for achieving these outcomes. Additionally, our AI for production planning can further enhance these processes, ensuring that your operations are optimized for success.
1.2. Traditional Challenges in Manufacturing
Manufacturing faces several traditional challenges that can hinder efficiency and productivity. These challenges often stem from outdated practices and lack of integration in processes.
- Inefficient Resource Utilization: Many manufacturers struggle with underutilized machinery and labor, leading to increased costs and wasted time. Production scheduling programs can help optimize resource allocation.
- Demand Variability: Fluctuations in customer demand can create challenges in maintaining optimal inventory levels, resulting in either stockouts or excess inventory. Advanced planning and scheduling can mitigate these issues.
- Communication Gaps: Poor communication between departments can lead to misalignment in production goals and delays in response to market changes. Manufacturing planning software can facilitate better communication and coordination.
- Complex Supply Chains: Managing multiple suppliers and logistics can complicate production schedules and increase the risk of disruptions. Manufacturing scheduling software for small businesses can simplify these complexities.
- Data Silos: Lack of integrated data systems can prevent manufacturers from gaining insights into production performance, making it difficult to make informed decisions. Implementing production planning software can help break down these silos.
Addressing these challenges is essential for manufacturers to remain competitive in a rapidly changing market.
1.3. AI as a Transformative Technology
Artificial Intelligence (AI) is revolutionizing the manufacturing sector by providing innovative solutions to traditional challenges. Its ability to analyze vast amounts of data and learn from patterns makes it a powerful tool for enhancing production planning and scheduling.
- Predictive Analytics: AI can forecast demand more accurately by analyzing historical data and market trends. This leads to better inventory management and reduced stockouts and overproduction, which is crucial for effective production scheduling.
- Real-time Monitoring: AI systems can monitor production processes in real-time, allowing for immediate adjustments to schedules and resource allocation. Benefits include increased operational efficiency and reduced downtime.
- Automation: AI-driven automation can streamline repetitive tasks, freeing up human resources for more complex activities. This results in higher productivity and improved quality control, particularly in manufacturing scheduling.
- Enhanced Decision-Making: AI can provide insights and recommendations based on data analysis, enabling manufacturers to make informed decisions quickly regarding production planning and scheduling.
- Supply Chain Optimization: AI can analyze supply chain data to identify bottlenecks and inefficiencies, leading to improved logistics and reduced costs.
At Rapid Innovation, we leverage AI technologies to help manufacturers overcome these challenges and achieve greater ROI. By integrating AI into production planning and scheduling, we empower our clients to transform their operations, making them more agile and responsive to market demands. Our expertise in AI development and consulting ensures that your manufacturing processes are optimized for efficiency and effectiveness, ultimately driving business success.
Refer to the image for a visual representation of production planning and scheduling concepts.

1.4. Evolution of Scheduling Methodologies
Scheduling methodologies have undergone significant transformations over the years, adapting to the changing needs of industries and advancements in technology.
- Early scheduling methods were primarily manual, relying on paper-based systems and human judgment.
- The introduction of computers in the 1960s marked a pivotal moment, allowing for more complex calculations and the development of algorithms to optimize scheduling.
- The 1980s saw the rise of project management software, which automated many scheduling tasks and introduced Gantt charts and critical path methods.
- In the 1990s, the focus shifted towards resource allocation and optimization, leading to the development of more sophisticated scheduling algorithms that could handle multiple constraints and variables.
- The 2000s brought about the integration of artificial intelligence (AI) and machine learning (ML) into scheduling, enabling systems to learn from past data and improve future scheduling decisions. This period also saw the emergence of agile project scheduling and critical chain scheduling methodologies, which focused on enhancing efficiency and flexibility in project management.
- Today, scheduling methodologies are increasingly dynamic, utilizing real-time data and analytics to adapt to changing conditions and demands. Approaches such as critical chain project scheduling and lean scheduling methodology are now prevalent, emphasizing the importance of resource management and minimizing waste.
The evolution of scheduling methodologies reflects a broader trend towards automation and efficiency, driven by technological advancements and the need for organizations to respond quickly to market changes. At Rapid Innovation, we leverage these advancements to help our clients implement AI-driven scheduling solutions that enhance operational efficiency and maximize return on investment (ROI).
1.5. The Paradigm Shift: From Static to Dynamic Planning
The shift from static to dynamic planning represents a fundamental change in how organizations approach scheduling and resource management.
- Static planning involves fixed schedules that do not adapt to changes in the environment or operational conditions. This approach can lead to inefficiencies and missed opportunities.
- Dynamic planning, on the other hand, allows for real-time adjustments based on current data and conditions. This flexibility enables organizations to respond quickly to unexpected events, such as supply chain disruptions or changes in customer demand.
Key benefits of dynamic planning include: - Improved resource utilization - Enhanced responsiveness to market changes - Increased collaboration among teams - Better risk management through proactive adjustments
Technologies such as AI and big data analytics play a crucial role in enabling dynamic planning. These tools provide insights that help organizations make informed decisions and optimize their scheduling processes. At Rapid Innovation, we specialize in implementing AI solutions that facilitate dynamic planning, ensuring our clients can adapt swiftly to market fluctuations and operational challenges. As businesses continue to embrace digital transformation, the shift towards dynamic planning is expected to accelerate, leading to more agile and resilient operations.
2. Foundational AI Technologies
Artificial intelligence (AI) is built on several foundational technologies that enable machines to perform tasks that typically require human intelligence.
- Machine Learning (ML): A subset of AI that focuses on the development of algorithms that allow computers to learn from and make predictions based on data. ML is widely used in various applications, including predictive analytics and recommendation systems.
- Natural Language Processing (NLP): This technology enables machines to understand and interpret human language. NLP is essential for applications such as chatbots, virtual assistants, and sentiment analysis.
- Computer Vision: This field of AI focuses on enabling machines to interpret and understand visual information from the world. Computer vision is used in applications like facial recognition, autonomous vehicles, and image analysis.
- Robotics: AI technologies are increasingly integrated into robotics, allowing machines to perform complex tasks in various environments. This includes everything from manufacturing robots to drones used in delivery services.
- Neural Networks: Inspired by the human brain, neural networks are a key component of deep learning, a subset of ML. They are used for tasks such as image and speech recognition, enabling more sophisticated AI applications.
- Data Analytics: AI relies heavily on data analytics to extract insights from large datasets. This technology helps organizations make data-driven decisions and improve operational efficiency.
These foundational AI technologies are driving innovation across industries, enabling organizations to automate processes, enhance decision-making, and improve customer experiences. At Rapid Innovation, we harness these technologies to deliver tailored AI solutions that empower our clients to achieve their business goals efficiently and effectively. As AI continues to evolve, its impact on various sectors will only grow, leading to new opportunities and challenges.
Refer to the image for a visual representation of the evolution of scheduling methodologies.
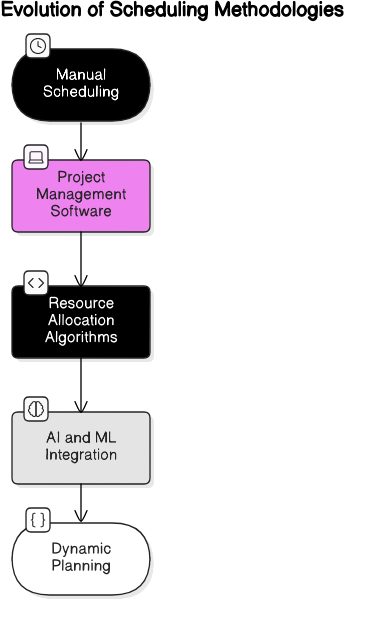
2.1. Machine Learning Fundamentals
Machine learning (ML) is a subset of artificial intelligence (AI) that focuses on the development of algorithms that allow computers to learn from and make predictions based on data. Understanding the fundamentals of machine learning is crucial for anyone looking to delve into this field, and at Rapid Innovation, we leverage these principles to help our clients achieve their business goals efficiently and effectively.
- Types of Machine Learning: Â
- Supervised Learning: Involves training a model on labeled data, where the outcome is known. Common algorithms include linear regression, decision trees, and support vector machines. Rapid Innovation utilizes supervised learning to enhance predictive accuracy in various applications, such as customer behavior forecasting, leading to improved ROI for our clients.
- Unsupervised Learning: Deals with unlabeled data, aiming to find hidden patterns or intrinsic structures. Examples include clustering algorithms like K-means and hierarchical clustering. By employing unsupervised learning, we help clients uncover valuable insights from their data, enabling better strategic decisions.
- Reinforcement Learning: Involves training models to make sequences of decisions by rewarding desired behaviors and punishing undesired ones. This is often used in robotics and game playing. Our expertise in reinforcement learning allows us to optimize processes and enhance operational efficiency for our clients.
- Key Concepts: Â
- Features and Labels: Features are the input variables used for prediction, while labels are the output variables. Understanding these concepts is essential for effective model development.
- Training and Testing: The dataset is typically split into training and testing sets to evaluate the model's performance. Rapid Innovation emphasizes rigorous testing to ensure our models deliver reliable results.
- Overfitting and Underfitting: Overfitting occurs when a model learns noise in the training data, while underfitting happens when a model is too simple to capture the underlying trend. We implement strategies to mitigate these issues, ensuring robust model performance.
- Applications: Â
- Image recognition
- Natural language processing
- Fraud detection
- machine learning fundamentals
- deep learning fundamentals
- ai ml basics
- ml fundamentals
- ai and machine learning basics
- fundamentals of ai and ml
- artificial intelligence and machine learning fundamentals
- machine learning fundamentals course
- learn tensorflow and deep learning fundamentals with python
- mlops fundamentals
- fundamentals of ml
- machine learning fundamentals and algorithms
- computational fundamentals for machine learning
- datacamp machine learning fundamentals with python
- deep learning fundamentals with keras
- fundamental of python for machine learning
- fundamentals of machine learning and deep learning
- fundamentals of reinforcement learning coursera
- fundamentals of reinforcement learning coursera github
- fundamentals of statistical learning
- learn unity ml agents fundamentals of unity machine learning
- machine learning fundamentals edx
- mastering data science and machine learning fundamentals
- microsoft azure essentials azure machine learning
- python ai basics
- fundamental of deep learning in practice
2.2. Optimization Algorithms
Optimization algorithms are essential in machine learning as they help in minimizing or maximizing a function, often related to the loss or cost function in predictive models. These algorithms are crucial for training models effectively, and at Rapid Innovation, we apply these techniques to enhance our clients' model performance.
- Gradient Descent: A widely used optimization algorithm that iteratively adjusts parameters to minimize the loss function. Variants include: Â
- Stochastic Gradient Descent (SGD): Updates parameters using a single training example, which can lead to faster convergence. We utilize SGD to accelerate model training, providing quicker insights for our clients.
- Mini-batch Gradient Descent: Combines the benefits of both batch and stochastic methods by using a small subset of data for each update.
- Advanced Optimization Techniques: Â
- Adam (Adaptive Moment Estimation): Combines the advantages of two other extensions of stochastic gradient descent, namely AdaGrad and RMSProp, to provide an efficient optimization method. Our use of Adam ensures that our models are trained effectively, maximizing their predictive capabilities.
- RMSProp: Adapts the learning rate for each parameter, which helps in dealing with non-stationary objectives.
- Importance of Optimization: Â
- A well-optimized model can significantly improve accuracy and performance. Rapid Innovation focuses on optimization to ensure our clients achieve the best possible outcomes from their AI initiatives.
- Helps in avoiding local minima, ensuring that the model converges to the best possible solution.
2.3. Predictive Analytics
Predictive analytics involves using statistical algorithms and machine learning techniques to identify the likelihood of future outcomes based on historical data. It is a powerful tool for decision-making across various industries, and Rapid Innovation harnesses this capability to drive business success for our clients.
- Key Components: Â
- Data Collection: Gathering relevant data from various sources, including databases, APIs, and real-time data streams. We assist clients in establishing robust data collection frameworks to support their analytics needs.
- Data Preparation: Cleaning and transforming data to ensure it is suitable for analysis. This may involve handling missing values, normalizing data, and feature selection. Our expertise in data preparation ensures that our clients' models are built on high-quality data.
- Model Building: Selecting appropriate algorithms and techniques to create predictive models. This can include regression analysis, classification, and time series forecasting. Rapid Innovation customizes model building to align with our clients' specific business objectives.
- Applications: Â
- Finance: Credit scoring, risk assessment, and fraud detection.
- Healthcare: Predicting patient outcomes, disease outbreaks, and treatment effectiveness.
- Marketing: Customer segmentation, sales forecasting, and personalized recommendations.
- Benefits: Â
- Enhances decision-making by providing insights into future trends. Our predictive analytics solutions empower clients to make informed decisions that drive growth.
- Increases efficiency by allowing organizations to allocate resources more effectively. We help clients optimize their operations through data-driven insights.
- Improves customer satisfaction through personalized experiences and targeted marketing strategies. By leveraging predictive analytics, Rapid Innovation enables clients to enhance their customer engagement and loyalty.
Refer to the image for a visual representation of the concepts discussed in the Machine Learning Fundamentals.
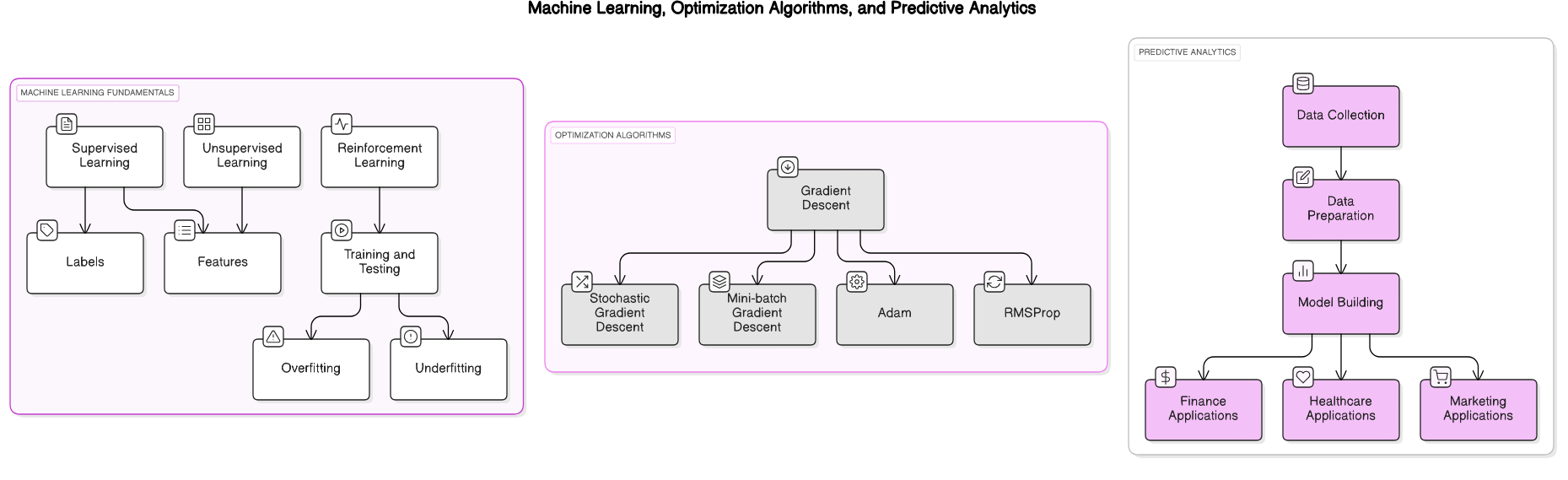
2.4. Constraint Satisfaction Techniques
Constraint Satisfaction Techniques (CST) are essential in solving problems where a set of constraints must be satisfied. These techniques are widely used in various fields, including artificial intelligence, operations research, and computer science.
- Definition: CST involves finding values for variables that meet specific constraints.
- Applications: Common applications include scheduling, resource allocation, and configuration problems.
- Types of Constraints: Â
- Hard Constraints: Must be strictly satisfied (e.g., a person cannot be in two places at once).
- Soft Constraints: Preferable but not mandatory (e.g., minimizing travel time).
- Techniques Used: Â
- Backtracking: A systematic way of searching for a solution by exploring possible variable assignments.
- Constraint Propagation: Reduces the search space by inferring variable values based on constraints.
- Local Search: Involves iteratively improving a solution by making small changes.
- Benefits: Â
- Efficiently narrows down potential solutions.
- Provides a structured approach to complex problems.
- Challenges: Â
- The complexity of constraints can lead to increased computational time.
- Finding optimal solutions can be difficult in large-scale problems.
At Rapid Innovation, we leverage constraint satisfaction techniques (CST) to help clients streamline their operations and enhance decision-making processes. For instance, in resource allocation scenarios, our solutions can optimize the distribution of resources across various projects, ensuring that hard constraints are met while also considering soft constraints to maximize overall efficiency.
2.5. Multi-Agent Systems
Multi-Agent Systems (MAS) consist of multiple interacting intelligent agents that can work together to solve problems or achieve goals. These systems are increasingly relevant in various domains, including robotics, telecommunications, and distributed computing.
- Definition: MAS is a system where multiple agents operate autonomously but can communicate and collaborate.
- Characteristics of Agents: Â
- Autonomy: Agents operate independently.
- Social Ability: Agents can communicate with one another.
- Reactivity: Agents respond to changes in their environment.
- Proactiveness: Agents can take initiative to achieve their goals.
- Applications: Â
- Robotics: Coordinating multiple robots for tasks like exploration or assembly.
- Traffic Management: Managing traffic flow through intelligent systems.
- E-commerce: Facilitating negotiations and transactions between buyers and sellers.
- Benefits: Â
- Scalability: MAS can easily scale to accommodate more agents.
- Flexibility: Agents can adapt to new tasks or environments.
- Robustness: The failure of one agent does not necessarily compromise the entire system.
- Challenges: Â
- Coordination: Ensuring agents work together effectively can be complex.
- Communication: Agents must have a common language or protocol to interact.
- Security: Protecting against malicious agents is crucial.
At Rapid Innovation, we implement MAS to enhance collaborative efforts in various sectors. For example, in e-commerce, our systems can facilitate real-time negotiations between buyers and sellers, leading to improved transaction efficiency and customer satisfaction.
2.6. Reinforcement Learning in Production Environments
Reinforcement Learning (RL) is a type of machine learning where agents learn to make decisions by taking actions in an environment to maximize cumulative rewards. In production environments, RL can optimize processes and improve efficiency.
- Definition: RL involves agents learning from the consequences of their actions rather than from explicit instructions.
- Key Components: Â
- Agent: The learner or decision-maker.
- Environment: The context in which the agent operates.
- Actions: Choices made by the agent.
- Rewards: Feedback received from the environment based on actions taken.
- Applications in Production: Â
- Supply Chain Management: Optimizing inventory levels and logistics.
- Manufacturing: Enhancing production schedules and machine operations.
- Quality Control: Adjusting processes in real-time to maintain product quality.
- Benefits: Â
- Adaptability: RL can adjust to changing conditions in production environments.
- Efficiency: Can lead to significant cost savings and improved resource utilization.
- Continuous Improvement: Agents can learn and improve over time, leading to better decision-making.
- Challenges: Â
- Sample Efficiency: RL often requires a large amount of data to learn effectively.
- Exploration vs. Exploitation: Balancing the need to explore new strategies while exploiting known successful ones can be difficult.
- Implementation Complexity: Integrating RL into existing production systems can be challenging.
At Rapid Innovation, we harness the power of RL to drive efficiency in production environments. For instance, our RL solutions can optimize supply chain management by dynamically adjusting inventory levels based on real-time data, ultimately leading to reduced costs and improved service levels.
3. Technical Architecture of AI Scheduling Systems
AI scheduling systems, including job shop scheduling in artificial intelligence, are designed to optimize the allocation of resources and time management through advanced algorithms and data processing techniques. The technical architecture of these systems is crucial for their effectiveness and efficiency.
3.1 Data Integration Frameworks
Data integration frameworks are essential for AI scheduling systems as they enable the seamless collection, processing, and utilization of data from various sources. These frameworks ensure that the scheduling system has access to accurate and timely information, which is vital for making informed decisions.
- Types of Data Sources: Â
- Internal databases: e.g., employee schedules, project timelines
- External APIs: e.g., weather data, traffic information
- IoT devices: e.g., sensors for resource availability
- Integration Techniques: Â
- ETL (Extract, Transform, Load): This traditional method involves extracting data from different sources, transforming it into a suitable format, and loading it into a central repository.
- Real-time Data Streaming: Technologies like Apache Kafka or AWS Kinesis allow for continuous data flow, enabling the scheduling system to react to changes instantly.
- API Integration: RESTful APIs facilitate the connection between various software applications, allowing for real-time data exchange.
- Data Quality Management: Ensuring data accuracy and consistency is critical. Techniques such as data validation, cleansing, and deduplication are employed to maintain high data quality. Regular audits and monitoring help identify and rectify data discrepancies.
- Scalability: The framework should be scalable to accommodate growing data volumes and additional data sources without compromising performance. Cloud-based solutions often provide the flexibility needed for scaling.
- Security and Compliance: Data integration frameworks must adhere to regulations such as GDPR or HIPAA, ensuring that sensitive information is protected. Implementing encryption and access controls is essential for safeguarding data integrity.
3.2 Real-time Data Processing
Real-time data processing is a critical component of AI scheduling systems, allowing them to make instantaneous decisions based on the latest information. This capability enhances the system's responsiveness and adaptability to changing conditions.
- Importance of Real-time Processing: Â
- Enables immediate adjustments to schedules based on unforeseen events (e.g., employee absences, equipment failures).
- Improves resource utilization by dynamically reallocating resources as needed.
- Technologies Used: Â
- Stream Processing Frameworks: Tools like Apache Flink, Apache Storm, and Spark Streaming are commonly used for processing data in real-time.
- Event-Driven Architecture: This approach allows the system to react to events as they occur, facilitating timely decision-making.
- Data Ingestion: Real-time data ingestion techniques, such as message queues (e.g., RabbitMQ, Apache Kafka), ensure that data is captured and processed without delays. Batch processing is less effective in dynamic environments where immediate responses are required.
- Analytics and Machine Learning: Real-time analytics provide insights that can influence scheduling decisions, such as predicting demand or identifying bottlenecks. Machine learning algorithms can be trained on historical data to improve the accuracy of predictions and recommendations.
- User Interface and Experience: A user-friendly interface is essential for displaying real-time data and insights to users, allowing them to make informed decisions quickly. Dashboards that visualize data trends and alerts can enhance user engagement and responsiveness.
- Challenges: Handling large volumes of data in real-time can strain system resources, necessitating efficient architecture and resource management. Ensuring low latency is crucial for maintaining the effectiveness of real-time processing.
In conclusion, the technical architecture of AI scheduling systems, particularly through data integration frameworks and real-time data processing, plays a pivotal role in their functionality. By leveraging these components, organizations can enhance their scheduling efficiency, improve resource allocation, and respond swiftly to changing conditions. At Rapid Innovation, we specialize in developing tailored AI scheduling solutions that integrate these advanced architectures, helping our clients achieve greater ROI through improved operational efficiency and resource management.
3.3. Sensor and IoT Integration
Sensor and IoT integration is a critical component in the development of smart systems and applications. This integration allows for the collection, transmission, and analysis of data from various sensors, enabling real-time monitoring and decision-making. Sensors are devices that detect and respond to physical stimuli such as temperature, light, motion, and pressure. IoT (Internet of Things) refers to the network of interconnected devices that communicate and exchange data over the internet. The combination of sensor and IoT integration creates a robust framework for smart cities, healthcare, agriculture, and industrial automation.
At Rapid Innovation, we leverage sensor and IoT integration to help our clients achieve greater operational efficiency and enhanced decision-making capabilities. For instance, in the healthcare sector, we have implemented IoT solutions that allow for real-time patient monitoring, leading to timely interventions and improved patient outcomes.
Key benefits of sensor and IoT integration include:
- Enhanced data collection: Sensors provide accurate and real-time data, which can be analyzed for insights.
- Improved efficiency: Automated systems can optimize processes, reducing waste and increasing productivity.
- Remote monitoring: Users can access data from anywhere, allowing for timely interventions and decision-making.
Challenges in sensor and IoT integration include:
- Data security: Protecting sensitive information from cyber threats is crucial.
- Interoperability: Ensuring that different devices and systems can communicate effectively is essential for seamless integration.
- Scalability: As the number of devices increases, managing and processing the data becomes more complex.
3.4. Cloud and Edge Computing Approaches
Cloud and edge computing are two complementary approaches that enhance data processing and storage capabilities in IoT systems. Each has its unique advantages and use cases. Cloud computing involves storing and processing data on remote servers accessed via the internet, offering scalability, flexibility, and cost-effectiveness. Edge computing, on the other hand, processes data closer to the source, reducing latency and bandwidth usage, which is particularly beneficial for applications requiring real-time responses.
At Rapid Innovation, we help clients navigate the complexities of cloud and edge computing to maximize their ROI. For example, we have assisted manufacturing clients in implementing edge computing solutions that reduce latency in their production lines, resulting in faster decision-making and improved operational efficiency.
Benefits of cloud computing include:
- Centralized data management: Easy access to data from multiple devices and locations.
- High storage capacity: Cloud services can accommodate vast amounts of data without the need for physical infrastructure.
- Advanced analytics: Cloud platforms often provide powerful tools for data analysis and machine learning.
Benefits of edge computing include:
- Reduced latency: Processing data locally allows for faster response times, which is critical for applications like autonomous vehicles and industrial automation.
- Bandwidth efficiency: By processing data at the edge, only essential information is sent to the cloud, reducing data transmission costs.
- Enhanced privacy: Sensitive data can be processed locally, minimizing exposure to potential breaches.
Challenges faced by cloud and edge computing include:
- Data synchronization: Ensuring consistency between cloud and edge data can be complex.
- Security concerns: Both approaches require robust security measures to protect data integrity and privacy.
- Resource management: Balancing workloads between cloud and edge environments can be challenging.
3.5. Simulation and Digital Twin Technologies
Simulation and digital twin technologies are revolutionizing how industries design, test, and optimize their processes and products. These technologies create virtual representations of physical systems, allowing for enhanced analysis and decision-making. Simulation involves creating a model of a system to study its behavior under various conditions, helping in understanding complex systems without the need for physical prototypes. Digital twins are real-time digital replicas of physical assets, processes, or systems, integrating data from sensors and IoT devices to provide insights into performance and potential issues.
At Rapid Innovation, we utilize simulation and digital twin technologies to help clients reduce risks and costs associated with product development. For example, in the manufacturing sector, our digital twin solutions enable predictive maintenance, which minimizes downtime and enhances productivity.
Key benefits of simulation and digital twin technologies include:
- Risk reduction: Testing scenarios in a virtual environment minimizes the risks associated with physical trials.
- Cost savings: Reducing the need for physical prototypes and tests can significantly lower development costs.
- Improved performance: Continuous monitoring and analysis of digital twins can lead to optimized operations and maintenance.
Applications of simulation and digital twin technologies span various industries:
- Manufacturing: Digital twins can monitor equipment health and predict maintenance needs, reducing downtime.
- Healthcare: Simulations can model patient outcomes and optimize treatment plans.
- Urban planning: Digital twins of cities can help in traffic management, resource allocation, and infrastructure development.
Challenges in implementing simulation and digital twin technologies include:
- Data integration: Combining data from various sources can be complex and time-consuming.
- High initial investment: Developing accurate simulations and digital twins may require significant upfront costs.
- Skill gaps: Organizations may need to invest in training personnel to effectively utilize these technologies.
By partnering with Rapid Innovation, clients can effectively harness these advanced technologies to achieve their business goals efficiently and effectively, ultimately leading to greater ROI. Additionally, you can learn more about how AI-driven digital twins.
4. Key AI Capabilities in Production Planning
AI technologies are revolutionizing production planning by enhancing efficiency, accuracy, and responsiveness. The integration of AI capabilities allows businesses to streamline operations, reduce costs, and improve overall productivity. Two critical AI capabilities in production planning are demand forecasting and resource allocation optimization.
4.1 Demand Forecasting
Demand forecasting is the process of predicting future customer demand for products or services. Accurate demand forecasting is essential for effective production planning, as it helps businesses align their production schedules with market needs.
- AI algorithms analyze historical sales data, market trends, and external factors to generate precise demand forecasts.
- Machine learning models can identify patterns and correlations in data that traditional methods may overlook.
- Real-time data integration allows businesses to adjust forecasts based on current market conditions, improving responsiveness.
Benefits of AI-driven demand forecasting include enhanced accuracy, reduced inventory costs, and improved customer satisfaction.
- Enhanced accuracy: AI can improve forecast accuracy by up to 30% compared to traditional methods.
- Reduced inventory costs: By predicting demand more accurately, businesses can minimize excess inventory and associated holding costs.
- Improved customer satisfaction: Meeting customer demand promptly leads to higher satisfaction and loyalty.
AI tools for demand forecasting often utilize techniques such as time series analysis, regression analysis, and neural networks. These methods enable businesses to create dynamic forecasts that adapt to changing market conditions. Companies leveraging AI in production planning for demand forecasting can gain a competitive edge by being more agile and responsive to customer needs. For more insights on AI-driven demand forecasting, check out this predictive demand forecaster.
4.2 Resource Allocation Optimization
Resource allocation optimization involves efficiently distributing resources—such as labor, materials, and machinery—across production processes to maximize output and minimize waste. AI plays a crucial role in optimizing resource allocation by analyzing complex datasets and providing actionable insights.
- AI algorithms can evaluate multiple variables, including production capacity, lead times, and resource availability, to determine the best allocation strategies.
- Predictive analytics can forecast potential bottlenecks and suggest preemptive measures to mitigate disruptions.
- Machine learning models continuously learn from past performance, refining resource allocation strategies over time.
Key advantages of AI in resource allocation optimization include increased efficiency, enhanced flexibility, and improved decision-making.
- Increased efficiency: AI can identify the most efficient use of resources, leading to reduced operational costs.
- Enhanced flexibility: Businesses can quickly adapt to changes in demand or resource availability, ensuring optimal production flow.
- Improved decision-making: AI provides data-driven insights that support informed decision-making regarding resource allocation.
AI techniques used for resource allocation optimization include linear programming, genetic algorithms, and simulation modeling. By implementing AI-driven resource allocation strategies, companies can achieve higher productivity levels and better align their resources with production goals. This not only enhances operational efficiency but also contributes to overall business growth and sustainability.
At Rapid Innovation, we specialize in harnessing these AI capabilities to help our clients achieve their business goals efficiently and effectively. By integrating advanced demand forecasting and resource allocation optimization into your production planning processes, we can help you realize greater ROI and drive sustainable growth. Our expertise in AI for production planning ensures that your organization remains competitive in an ever-evolving market landscape.
4.3. Capacity Planning
Capacity planning is a critical process that ensures an organization can meet future demand for its products or services. It involves assessing current resources and forecasting future needs to optimize production efficiency.
- Understanding Demand: Analyze historical data and market trends to predict future demand, which helps in making informed decisions about resource allocation. Rapid Innovation leverages advanced AI algorithms to enhance demand forecasting accuracy, enabling clients to align their resources effectively.
- Resource Assessment: Evaluate current capacity, including workforce, machinery, and technology, and identify any gaps between current capabilities and future requirements. Our AI-driven analytics tools provide insights that help organizations understand their resource utilization and identify areas for improvement, particularly in workforce capacity planning.
- Flexibility: Implement flexible capacity strategies, such as scalable workforce solutions or adjustable production schedules, to adapt to changing market conditions. Rapid Innovation assists clients in developing adaptive strategies that can respond to fluctuations in demand, ensuring operational resilience through effective capacity management.
- Cost Management: Balance capacity with cost efficiency, as overcapacity can lead to increased costs while undercapacity can result in lost sales opportunities. Our consulting services focus on optimizing resource allocation to minimize costs while maximizing output, including making informed capacity decisions.
- Technology Integration: Utilize software tools for capacity planning that can simulate different scenarios and provide insights into optimal resource allocation. Rapid Innovation integrates cutting-edge AI technologies to enhance decision-making processes in capacity planning, including it capacity management solutions.
- Continuous Monitoring: Regularly review capacity plans and adjust them based on real-time data and changing market dynamics. Our solutions include real-time monitoring systems that provide actionable insights, allowing organizations to stay ahead of demand shifts, particularly in resource and capacity planning.
4.4. Inventory Management
Inventory management is the process of overseeing and controlling the ordering, storage, and use of a company's inventory. Effective inventory management ensures that a business has the right products in the right quantities at the right time.
- Stock Levels: Maintain optimal stock levels to prevent overstocking or stockouts by setting reorder points and safety stock levels based on demand forecasts. Rapid Innovation employs AI-driven analytics to optimize stock levels, reducing excess inventory costs.
- Inventory Tracking: Implement inventory tracking systems, such as barcode scanning or RFID technology, to monitor stock levels in real-time. Our solutions enhance visibility across the supply chain, ensuring accurate inventory management.
- Supplier Relationships: Build strong relationships with suppliers to ensure timely deliveries and negotiate better terms, which can help in managing lead times and reducing costs. We provide strategic consulting to strengthen supplier partnerships, enhancing supply chain efficiency.
- Inventory Valuation: Use appropriate inventory valuation methods (FIFO, LIFO, or weighted average) to accurately assess the value of inventory on hand. Our expertise in data analytics helps clients choose the best valuation methods for their specific needs.
- Demand Forecasting: Utilize data analytics to predict future inventory needs based on sales trends, seasonality, and market changes. Rapid Innovation's predictive analytics capabilities empower clients to make informed inventory decisions.
- Regular Audits: Conduct regular inventory audits to identify discrepancies and ensure accuracy in stock levels. Our automated auditing solutions streamline the auditing process, improving accuracy and efficiency.
4.5. Predictive Maintenance Scheduling
Predictive maintenance scheduling is a proactive approach to maintenance that uses data analysis to predict when equipment failures might occur. This strategy helps organizations minimize downtime and extend the lifespan of their assets.
- Data Collection: Gather data from equipment sensors, historical maintenance records, and operational logs to identify patterns and potential failure points. Rapid Innovation utilizes IoT and AI technologies to enhance data collection processes, providing deeper insights into equipment performance.
- Analytical Tools: Use predictive analytics tools to analyze data and forecast maintenance needs, with machine learning algorithms enhancing the accuracy of predictions. Our advanced analytics solutions enable clients to anticipate maintenance requirements, reducing unexpected failures.
- Maintenance Planning: Schedule maintenance activities based on predictive insights rather than reactive measures, leading to more efficient use of resources and reduced operational disruptions. We assist organizations in developing tailored maintenance schedules that optimize resource allocation.
- Cost Savings: By preventing unexpected breakdowns, organizations can save on repair costs and avoid production losses. Our predictive maintenance solutions have consistently demonstrated significant ROI for clients by minimizing downtime.
- Asset Management: Implement a comprehensive asset management system that integrates predictive maintenance with overall asset lifecycle management. Rapid Innovation offers integrated solutions that enhance asset visibility and management throughout their lifecycle.
- Continuous Improvement: Regularly review and refine predictive maintenance strategies based on new data and technological advancements to enhance effectiveness. Our commitment to innovation ensures that clients benefit from the latest advancements in predictive maintenance technologies.
4.6. Supply Chain Coordination
Supply chain coordination is essential for ensuring that all components of the supply chain work together efficiently. Effective coordination can lead to reduced costs, improved service levels, and enhanced overall performance. This includes aspects such as supply chain coordination with contracts and inter functional coordination in supply chain management.
- Collaboration among stakeholders: Involves manufacturers, suppliers, distributors, and retailers. This collaboration encourages the sharing of information and resources, which is crucial for optimizing supply chain operations. Global supply chain coordination is vital in this context, as it allows for better integration across different regions.
- Information sharing: Real-time data exchange helps in demand forecasting and inventory management. Technologies like ERP (Enterprise Resource Planning) systems facilitate seamless communication, enabling stakeholders to make informed decisions quickly. Coordination of supply chain activities is enhanced through effective information sharing.
- Joint planning and forecasting: Collaborative planning helps in aligning production schedules with market demand, which reduces the risk of stockouts and overstock situations. By leveraging AI-driven analytics, businesses can enhance their forecasting accuracy, leading to better inventory management. This is a key aspect of coordinated product and supply chain design.
- Performance metrics: Establishing KPIs (Key Performance Indicators) to measure supply chain efficiency is crucial. Metrics can include order fulfillment rates, lead times, and inventory turnover. Rapid Innovation can assist in developing tailored metrics that align with your specific business goals, ensuring that supply chain coordination is effectively monitored.
- Risk management: Identifying potential disruptions in the supply chain and developing contingency plans is essential. Coordination helps in mitigating risks associated with supply chain vulnerabilities, ensuring business continuity. Understanding the meaning of supply chain coordination can aid in better risk management strategies. For more insights on how AI is used to forecast shortages and improve risk management in supply chains, visit this link.
- Technology integration: Utilizing advanced technologies like IoT (Internet of Things) and AI (Artificial Intelligence) for better visibility and control is important. Automation can streamline processes and reduce human error, ultimately enhancing operational efficiency. This technology integration is a critical component of coordinated supply chain management.
5. Implementation Strategies
Implementing effective strategies is crucial for the success of any project or initiative. A well-structured approach can lead to better outcomes and ensure that objectives are met.
- Define clear objectives: Establish specific, measurable, achievable, relevant, and time-bound (SMART) goals. Clear objectives guide the implementation process and help in tracking progress.
- Develop a detailed plan: Create a roadmap outlining the steps needed to achieve the objectives. Include timelines, resources required, and responsible parties.
- Engage stakeholders: Involve all relevant parties early in the process to gain buy-in and support. Regular communication keeps stakeholders informed and engaged.
- Allocate resources: Ensure that adequate resources, including budget, personnel, and technology, are available. Proper resource allocation is critical for smooth implementation.
- Monitor progress: Regularly review the implementation process against the established plan. Use performance metrics to assess effectiveness and make necessary adjustments.
- Training and support: Provide training for staff to ensure they are equipped to handle new processes or technologies. Ongoing support helps in addressing challenges that may arise during implementation.
5.1. Assessment and Readiness Evaluation
Before implementing any new strategy or system, conducting an assessment and readiness evaluation is vital. This process helps identify strengths, weaknesses, and areas that require improvement.
- Current state analysis: Evaluate existing processes, systems, and resources to identify gaps and areas for enhancement.
- Stakeholder feedback: Gather input from employees, customers, and partners to understand their perspectives. Feedback can highlight potential challenges and opportunities.
- Resource availability: Assess whether the necessary resources are in place for successful implementation, considering financial, human, and technological resources.
- Risk assessment: Identify potential risks associated with the new strategy or system and develop strategies to mitigate these risks.
- Cultural readiness: Evaluate the organizational culture to determine if it supports change. A culture that embraces innovation and flexibility is more likely to succeed.
- Benchmarking: Compare current performance against industry standards or best practices. Benchmarking can provide insights into areas for improvement.
- Pilot testing: Conduct small-scale trials to test the feasibility of the new strategy. Gather data and feedback to refine the approach before full-scale implementation.
By leveraging Rapid Innovation's expertise in AI and technology integration, businesses can enhance their supply chain coordination, leading to improved efficiency and greater ROI. Our tailored solutions ensure that your organization is well-equipped to navigate the complexities of modern supply chains, including examples of supply chain coordination and the role of supply chain coordination ltd.
5.2. Data Preparation and Normalization
Data preparation and normalization are critical steps in the data science workflow. They ensure that the data is clean, consistent, and ready for analysis or modeling, ultimately leading to improved business outcomes.
- Data Cleaning: This involves removing inaccuracies, duplicates, and irrelevant information from the dataset. Techniques include handling missing values through imputation or removal and correcting inconsistencies in data formats (e.g., date formats). Rapid Innovation employs advanced data cleaning techniques to ensure that our clients' datasets are reliable, which is essential for making informed business decisions.
- Data Transformation: This step modifies the data to make it suitable for analysis. Common techniques include encoding categorical variables into numerical formats (e.g., one-hot encoding) and scaling numerical features to a standard range (e.g., Min-Max scaling or Z-score normalization). By transforming data effectively, we help clients derive actionable insights that drive ROI.
- Normalization: This process adjusts the values in the dataset to a common scale without distorting differences in the ranges of values. Key methods include: Â
- Min-Max Normalization: Rescales the data to a fixed range, typically [0, 1].
- Z-score Normalization: Centers the data around the mean with a standard deviation of 1.
- Feature Selection: Identifying the most relevant features for the model can improve performance and reduce overfitting. Techniques include Recursive Feature Elimination (RFE) and using algorithms like Random Forest for feature importance. Rapid Innovation utilizes these techniques to ensure that our clients' models are both efficient and effective, maximizing their return on investment.
- Data Splitting: Dividing the dataset into training, validation, and test sets is essential for evaluating model performance. Common splits include 70% training, 15% validation, and 15% testing. This structured approach allows us to provide our clients with robust models that perform well in real-world scenarios.
Effective data preparation and normalization can significantly enhance the accuracy and efficiency of machine learning models, ultimately leading to better business outcomes for our clients. For more insights on enhancing AI and machine learning models, check out data annotation services.
5.3. Model Training Methodologies
Model training methodologies refer to the various approaches used to train machine learning models. Selecting the right methodology is crucial for achieving optimal performance and ensuring that our clients meet their business objectives.
- Supervised Learning: This approach uses labeled data to train models. It includes: Â
- Regression: Predicting continuous outcomes (e.g., house prices).
- Classification: Assigning labels to data points (e.g., spam detection). Rapid Innovation leverages supervised learning to help clients make accurate predictions that drive strategic decisions.
- Unsupervised Learning: This methodology deals with unlabeled data, focusing on discovering patterns. Techniques include: Â
- Clustering: Grouping similar data points (e.g., customer segmentation).
- Dimensionality Reduction: Reducing the number of features while retaining essential information (e.g., PCA). By employing these techniques, we assist clients in uncovering hidden insights that can lead to new business opportunities.
- Semi-Supervised Learning: This combines labeled and unlabeled data, often yielding better results than using only one type. It is particularly useful when labeled data is scarce, allowing our clients to maximize the value of their existing data.
- Reinforcement Learning: This methodology involves training models through trial and error, using feedback from actions taken in an environment. It is widely used in robotics and game playing, and Rapid Innovation applies it to optimize processes for our clients.
- Transfer Learning: This technique leverages knowledge gained from one task to improve performance on a related task. It is especially beneficial in deep learning, where pre-trained models can be fine-tuned for specific applications, enabling our clients to achieve faster results.
- Cross-Validation: This technique assesses how the results of a statistical analysis will generalize to an independent dataset. Common methods include k-fold cross-validation, which divides the dataset into k subsets. This rigorous evaluation process ensures that our clients receive reliable and robust models.
Choosing the right model training methodology is essential for building robust and accurate machine learning models that align with our clients' business goals.
5.4. Deployment Models
Deployment models refer to the various ways machine learning models can be implemented in real-world applications. The choice of deployment model can impact scalability, accessibility, and performance, which are critical for our clients' success.
- On-Premises Deployment: This model involves hosting the machine learning model on local servers. Key features include greater control over data security and compliance, as well as lower latency for internal applications. Rapid Innovation supports clients who require stringent data governance.
- Cloud Deployment: Utilizing cloud services (e.g., AWS, Azure, Google Cloud) for hosting models offers several advantages, including: Â
- Scalability: Easily adjust resources based on demand.
- Cost-Effectiveness: Pay-as-you-go pricing models reduce upfront costs. We help clients leverage cloud deployment to enhance their operational efficiency.
- Hybrid Deployment: This model combines on-premises and cloud solutions, allowing organizations to balance control and scalability. It is suitable for businesses with sensitive data that require local processing, ensuring that our clients can meet their unique needs.
- Edge Deployment: In this model, machine learning models are deployed on edge devices (e.g., IoT devices, mobile phones). Benefits include reduced latency by processing data closer to the source and lower bandwidth usage since less data needs to be sent to the cloud. Rapid Innovation assists clients in implementing edge solutions for real-time analytics.
- API-Based Deployment: This approach exposes the model as an API, allowing other applications to interact with it. Key advantages include easy integration with existing systems and flexibility to update the model without disrupting services, which enhances our clients' operational agility.
- Containerization: Using technologies like Docker, models can be packaged with their dependencies, ensuring consistency across different environments. This simplifies deployment and scaling, allowing our clients to focus on their core business activities.
Selecting the appropriate deployment model is crucial for ensuring that machine learning solutions are effective, efficient, and aligned with business needs, ultimately driving greater ROI for our clients.
5.4.1. On-Premise Solutions
On-premise solutions refer to software and hardware that are installed and run on the user's own servers and infrastructure. This traditional model has been widely used by organizations for many years, including on premise contact center solutions and on premise platforms.
- Control: Organizations have complete control over their data and systems, which can enhance security and compliance. This is particularly beneficial for industries with stringent regulatory requirements, where data governance is paramount.
- Customization: On-premise solutions can be tailored to meet specific business needs, allowing for greater flexibility in functionality. Rapid Innovation can assist in developing bespoke applications that align with your unique operational requirements, including on premise as a service options.
- Performance: Running applications locally can lead to faster performance, as there is no reliance on internet connectivity. This is crucial for businesses that require real-time processing and low latency, especially for on premise business operations.
- Upfront Costs: Typically, on-premise solutions require significant upfront investment in hardware and software licenses. However, Rapid Innovation can help you assess the long-term ROI to ensure that this investment aligns with your strategic goals, including evaluating on premise cost and pricing.
- Maintenance: Organizations are responsible for ongoing maintenance, updates, and support, which can require dedicated IT resources. Rapid Innovation offers consulting services to optimize your IT management, ensuring that your systems remain efficient and up-to-date.
While on-premise solutions offer advantages, they may not be suitable for every organization, especially those looking for scalability and lower initial costs. For more information on how AI can enhance your on-premise solutions, check out AI as a Service.
5.4.2. Cloud-Based Implementations
Cloud-based implementations involve hosting applications and data on remote servers accessed via the internet. This model has gained popularity due to its numerous benefits.
- Scalability: Cloud solutions can easily scale up or down based on business needs, allowing organizations to pay only for what they use. Rapid Innovation can help you design a cloud strategy that aligns with your growth trajectory.
- Cost-Effectiveness: Lower upfront costs and a subscription-based pricing model make cloud solutions more accessible for many businesses. This can lead to improved cash flow and resource allocation.
- Accessibility: Users can access cloud applications from anywhere with an internet connection, promoting remote work and collaboration. Rapid Innovation can facilitate the transition to a cloud-based environment, enhancing team productivity.
- Automatic Updates: Cloud providers handle software updates and maintenance, reducing the burden on internal IT teams. This allows your organization to focus on core business activities while ensuring that your technology remains current.
- Security: Many cloud providers invest heavily in security measures, often providing better protection than smaller organizations can achieve on their own. Rapid Innovation can guide you in selecting a cloud provider that meets your security and compliance needs.
Despite these advantages, organizations must consider potential challenges, such as data privacy concerns and reliance on internet connectivity.
5.4.3. Hybrid Approaches
Hybrid approaches combine elements of both on-premise and cloud-based solutions, allowing organizations to leverage the benefits of both models.
- Flexibility: Organizations can choose which applications and data to keep on-premise and which to move to the cloud, providing tailored solutions. Rapid Innovation can assist in developing a hybrid strategy that maximizes your operational efficiency.
- Cost Management: A hybrid model can help manage costs by allowing businesses to maintain critical applications on-premise while utilizing cloud resources for less sensitive operations. This approach can lead to significant savings and improved resource allocation.
- Enhanced Security: Sensitive data can remain on-premise, while less critical data can be stored in the cloud, balancing security and accessibility. Rapid Innovation can help you implement robust security protocols to protect your data across both environments.
- Disaster Recovery: Hybrid solutions can enhance disaster recovery strategies by allowing data to be backed up in the cloud while maintaining local copies. Rapid Innovation can design a comprehensive disaster recovery plan tailored to your business needs.
- Gradual Transition: Organizations can gradually migrate to the cloud without a complete overhaul of their existing systems, reducing disruption. Rapid Innovation provides expert guidance throughout this transition, ensuring a smooth and efficient process.
Hybrid approaches offer a balanced solution for organizations looking to modernize their IT infrastructure while maintaining control over critical data and applications. By leveraging Rapid Innovation's expertise, you can achieve greater ROI and align your technology strategy with your business goals, including exploring onpremise and cloud solutions.
5.5. Continuous Learning and Adaptation
Continuous learning and adaptation are essential components for organizations aiming to thrive in today's fast-paced and ever-evolving business landscape. This approach enables companies to remain competitive, innovate, and respond effectively to market changes.
- Emphasizes the importance of a growth mindset within the organization. Â
- Encourages employees to seek new knowledge and skills regularly. Â
- Utilizes feedback loops to assess performance and identify areas for improvement. Â
- Incorporates training programs and workshops to enhance employee capabilities. Â
- Leverages technology, such as e-learning platforms, to facilitate ongoing education. Â
- Promotes a culture of experimentation, where failure is viewed as a learning opportunity. Â
- Adapts strategies based on market trends, customer feedback, and technological advancements. Â
- Engages in cross-functional collaboration to share insights and best practices. Â
Organizations that prioritize continuous learning and adaptation are better equipped to navigate challenges and seize opportunities. By fostering an environment that values knowledge and flexibility, businesses can enhance their resilience and drive sustainable growth. At Rapid Innovation, we assist clients in implementing AI-driven solutions that facilitate continuous learning and adaptation, ensuring that their teams are always equipped with the latest skills and insights to maximize efficiency and effectiveness.
6. Use Cases and Scenarios
Use cases and scenarios are practical applications that illustrate how a particular technology, process, or strategy can be implemented in real-world situations. They help organizations understand the potential benefits and challenges associated with various approaches.
- Provide concrete examples to clarify complex concepts. Â
- Help stakeholders visualize the impact of decisions. Â
- Serve as a basis for developing strategies and solutions. Â
- Facilitate communication among team members and departments. Â
- Allow for the identification of best practices and lessons learned. Â
By analyzing use cases and scenarios, organizations can make informed decisions that align with their goals and objectives. Rapid Innovation specializes in crafting tailored use cases that demonstrate the tangible benefits of AI technologies, enabling clients to visualize their potential ROI and strategically plan their implementation.
6.1. Discrete Manufacturing
Discrete manufacturing refers to the production of distinct items that can be counted, touched, and seen. This type of manufacturing is characterized by the assembly of individual parts or components into finished products.
- Commonly found in industries such as automotive, electronics, and consumer goods. Â
- Involves processes like assembly, machining, and fabrication. Â
- Products are typically produced in batches or made to order. Â
- Requires precise inventory management and quality control measures. Â
- Utilizes technologies such as CAD (Computer-Aided Design) and CAM (Computer-Aided Manufacturing) for design and production. Â
Key benefits of discrete manufacturing include:
- Flexibility to customize products based on customer specifications. Â
- Ability to implement lean manufacturing principles to reduce waste. Â
- Enhanced tracking of production processes and inventory levels. Â
- Improved collaboration between design and production teams. Â
Discrete manufacturing plays a crucial role in meeting consumer demands and driving innovation in various sectors. By leveraging advanced technologies and methodologies, companies can optimize their production processes and deliver high-quality products efficiently. Rapid Innovation supports discrete manufacturers by integrating AI solutions that enhance operational efficiency, reduce costs, and ultimately drive greater ROI.
6.2. Process Manufacturing
Process manufacturing refers to the production of goods by combining supplies, ingredients, or raw materials using a specific formula or recipe. This method is prevalent in industries where the end product is a chemical, food, or beverage.
- Key characteristics of process manufacturing include: Â
- Continuous production: Often operates on a continuous flow basis, where materials are processed without interruption.
- Batch production: In some cases, products are made in batches, allowing for variations in the output.
- Quality control: Strict quality control measures are essential to ensure consistency and safety in the final product.
- Common industries involved in process manufacturing: Â
- Food and beverage: Production of items like bread, beer, and dairy products.
- Chemicals: Manufacturing of fertilizers, plastics, and pharmaceuticals.
- Oil and gas: Refining crude oil into usable products like gasoline and diesel.
- Challenges faced in process manufacturing: Â
- Regulatory compliance: Adhering to safety and environmental regulations can be complex.
- Equipment maintenance: Continuous operation requires regular maintenance to prevent downtime.
- Supply chain management: Ensuring a steady supply of raw materials is crucial for uninterrupted production.
At Rapid Innovation, we leverage AI technologies to enhance process manufacturing efficiency. For instance, our predictive maintenance solutions can analyze equipment performance data to forecast potential failures, thereby minimizing downtime and maintenance costs. Additionally, our AI-driven quality control systems can monitor production processes in real-time, ensuring that products meet safety and quality standards, ultimately leading to greater ROI for our clients. Techniques such as electro discharge machining and laser cutting machine are also utilized to improve precision in manufacturing processes.
6.3. Automotive Industry
The automotive industry encompasses the design, development, manufacturing, marketing, and selling of motor vehicles. This sector is a significant contributor to the global economy and employs millions of people worldwide.
- Key components of the automotive industry include: Â
- Vehicle manufacturing: Involves assembling various parts to create cars, trucks, and motorcycles.
- Supply chain: A complex network of suppliers providing components like engines, tires, and electronics.
- Aftermarket services: Includes maintenance, repair, and parts replacement for vehicles post-sale.
- Trends shaping the automotive industry: Â
- Electric vehicles (EVs): Growing demand for sustainable transportation options is driving innovation in EV technology.
- Autonomous driving: Advancements in AI and sensor technology are paving the way for self-driving cars.
- Connectivity: Integration of smart technology in vehicles for enhanced user experience and safety.
- Challenges in the automotive industry: Â
- Regulatory pressures: Stricter emissions standards and safety regulations require constant adaptation.
- Global competition: Manufacturers face competition from both established brands and new entrants.
- Supply chain disruptions: Events like the COVID-19 pandemic have highlighted vulnerabilities in global supply chains.
Rapid Innovation supports automotive clients by implementing AI solutions that optimize supply chain logistics and enhance vehicle design processes. For example, our machine learning algorithms can analyze consumer data to predict market trends, allowing manufacturers to align production with demand, thus maximizing profitability. The adoption of the Toyota production system has also been instrumental in streamlining operations.
6.4. Electronics and High-Tech Manufacturing
Electronics and high-tech manufacturing involve the production of electronic devices and components, including semiconductors, circuit boards, and consumer electronics. This sector is characterized by rapid innovation and technological advancements.
- Key aspects of electronics and high-tech manufacturing: Â
- Research and development: Continuous investment in R&D is crucial for staying competitive in this fast-paced industry.
- Precision manufacturing: High levels of accuracy and quality control are necessary to produce reliable electronic components.
- Global supply chains: Sourcing materials and components from various countries is common, making logistics critical.
- Major sectors within electronics and high-tech manufacturing: Â
- Consumer electronics: Production of devices like smartphones, tablets, and laptops.
- Industrial electronics: Manufacturing of equipment used in automation, robotics, and telecommunications.
- Medical devices: Development of electronic devices for healthcare, such as diagnostic equipment and monitoring systems.
- Challenges faced in electronics and high-tech manufacturing: Â
- Rapid technological change: Keeping up with the latest advancements requires constant innovation.
- Intellectual property concerns: Protecting patents and proprietary technology is essential to maintain a competitive edge.
- Environmental regulations: Compliance with regulations regarding e-waste and sustainable practices is increasingly important.
At Rapid Innovation, we assist electronics manufacturers by integrating AI into their R&D processes, enabling faster product development cycles and improved product quality. Our data analytics solutions can also help companies identify market opportunities and optimize their supply chains, leading to enhanced operational efficiency and increased ROI. Techniques such as plastic injection molding and 3D printing metals are increasingly being adopted to enhance production capabilities. Additionally, methods like selective laser melting and fused deposition modeling are revolutionizing the way components are manufactured.
6.5. Pharmaceutical Production
Pharmaceutical production is a critical sector that involves the development, manufacturing, and distribution of medications. This industry is highly regulated to ensure the safety and efficacy of drugs. Advanced technologies and methodologies are increasingly being integrated into pharmaceutical production processes to enhance efficiency and compliance.
- Automation: The use of automated systems in pharmaceutical production helps streamline operations, reduce human error, and improve consistency in drug manufacturing. Robotics and AI-driven systems can manage repetitive tasks, allowing human workers to focus on more complex activities. Rapid Innovation can assist clients in implementing these automated solutions, including pharmaceutical robotics, leading to increased throughput and reduced operational costs.
- Quality Control: Implementing rigorous quality control measures is essential in pharmaceutical production. Techniques such as real-time monitoring and data analytics help identify potential issues early in the production process, ensuring that products meet regulatory standards. Our expertise in AI can enhance these quality control systems, providing clients with predictive insights that minimize risks and ensure compliance.
- Supply Chain Management: Effective supply chain management is crucial for the timely delivery of raw materials and finished products. Advanced forecasting tools and AI algorithms can optimize inventory levels, reducing waste and ensuring that production schedules are met. Rapid Innovation's solutions can help clients achieve greater ROI by improving supply chain efficiency and reducing costs associated with excess inventory. Additionally, blockchain technology can enhance supply chain safety and product quality, providing transparency and traceability.
- Regulatory Compliance: The pharmaceutical industry is subject to strict regulations from agencies like the FDA and EMA. Companies must adhere to Good Manufacturing Practices (GMP) to ensure product safety and quality. Regular audits and inspections are part of maintaining compliance. Our consulting services can guide clients through the complexities of regulatory compliance, ensuring they meet all necessary standards while minimizing disruptions.
- Sustainability: There is a growing emphasis on sustainable practices in pharmaceutical production. Companies are exploring eco-friendly manufacturing processes and waste reduction strategies to minimize their environmental impact. Rapid Innovation can support clients in adopting sustainable technologies that not only comply with regulations but also enhance their brand reputation and appeal to environmentally conscious consumers.
6.6. Food and Beverage Sector
The food and beverage sector is a vital part of the global economy, encompassing everything from agriculture to retail. This industry faces unique challenges, including food safety, supply chain complexities, and changing consumer preferences. Innovations in technology are transforming how food and beverages are produced, processed, and distributed.
- Food Safety: Ensuring food safety is paramount in the food and beverage sector. Implementing Hazard Analysis and Critical Control Points (HACCP) systems helps identify and mitigate risks throughout the production process. Regular testing and monitoring are essential to maintain high safety standards. Rapid Innovation can assist clients in integrating AI-driven monitoring systems that enhance food safety protocols.
- Traceability: Consumers are increasingly concerned about the origins of their food. Advanced tracking systems, such as blockchain technology, enable companies to provide transparency in their supply chains, allowing consumers to trace the journey of their food from farm to table. Our expertise in blockchain can help clients implement robust traceability solutions that build consumer trust and loyalty.
- Automation and Robotics: Automation is revolutionizing food production and processing. Robotics can handle repetitive tasks, such as packaging and sorting, improving efficiency and reducing labor costs. This technology also helps maintain consistent product quality. Rapid Innovation can guide clients in adopting these technologies, leading to improved operational efficiency and cost savings.
- Health Trends: The food and beverage sector is adapting to changing consumer preferences, including a growing demand for healthier options. Companies are reformulating products to reduce sugar, salt, and unhealthy fats while incorporating more natural ingredients. Our consulting services can help clients navigate these trends, ensuring they meet consumer demands while maintaining profitability.
- Sustainability: Sustainability is becoming a key focus in the food and beverage industry. Companies are adopting practices such as reducing food waste, using sustainable packaging, and sourcing ingredients responsibly to meet consumer demand for environmentally friendly products. Rapid Innovation can assist clients in developing sustainable practices that not only comply with regulations but also enhance their market position.
7. Advanced AI Scheduling Techniques
Advanced AI scheduling techniques are transforming how businesses manage their resources and optimize operations. These techniques leverage artificial intelligence to create efficient schedules that maximize productivity while minimizing costs.
- Predictive Analytics: AI-driven predictive analytics can forecast demand and resource needs, allowing businesses to create schedules that align with anticipated workloads. This helps in reducing downtime and ensuring that resources are utilized effectively. Rapid Innovation can implement these analytics solutions, enabling clients to make data-driven scheduling decisions that enhance productivity.
- Dynamic Scheduling: Unlike traditional scheduling methods, dynamic scheduling adjusts in real-time based on changing conditions. AI algorithms can analyze data from various sources, such as employee availability and project deadlines, to create optimal schedules that adapt to new information. Our expertise in AI can help clients develop dynamic scheduling systems that improve operational flexibility.
- Resource Allocation: Advanced AI scheduling techniques enable better resource allocation by analyzing historical data and current trends. This ensures that the right resources are assigned to the right tasks at the right time, improving overall efficiency. Rapid Innovation can assist clients in optimizing their resource allocation strategies, leading to enhanced performance and reduced costs.
- Employee Satisfaction: Effective scheduling can enhance employee satisfaction by considering individual preferences and availability. AI can help create schedules that balance workload and personal commitments, leading to higher morale and productivity. Our solutions can help clients foster a positive work environment, ultimately contributing to better business outcomes.
- Integration with Other Systems: AI scheduling tools can integrate with other business systems, such as project management and HR software. This integration allows for seamless communication and data sharing, further enhancing scheduling efficiency. Rapid Innovation can support clients in implementing integrated systems that streamline operations and improve collaboration.
- Cost Reduction: By optimizing schedules, businesses can reduce operational costs associated with overtime, underutilization of resources, and missed deadlines. AI scheduling techniques help identify inefficiencies and suggest improvements, leading to significant cost savings. Our consulting services can guide clients in leveraging AI scheduling to achieve substantial ROI and operational excellence.
7.1. Multi-Objective Optimization
Multi-objective optimization (MOO) is a crucial area in optimization problems where multiple conflicting objectives need to be satisfied simultaneously. This approach is particularly relevant in fields such as engineering, economics, and logistics, where decision-makers often face trade-offs between competing goals. MOO aims to find a set of optimal solutions, known as Pareto optimal solutions, where no objective can be improved without worsening another.
Common applications include: - Resource allocation - Portfolio optimization - Scheduling problems
Techniques used in MOO include: - Weighted sum method - ε-constraint method - Pareto-based methods
MOO can be applied in various industries, such as: - Manufacturing, to optimize production schedules while minimizing costs and maximizing quality. - Transportation, to balance travel time and fuel consumption.
At Rapid Innovation, we leverage advanced algorithms to enhance the effectiveness of multiobjective optimization, enabling our clients to efficiently explore the solution space and achieve high-quality outcomes. By implementing multi objective optimisation strategies, we help businesses align their objectives with operational capabilities, ultimately driving greater ROI. Our expertise in multiobjective optimisation and multi objective algorithms allows us to tackle complex problems effectively. We also utilize multi objective optimization algorithms to refine our approaches, ensuring optimal results for our clients. Additionally, our services extend to areas like AI healthcare management, where we apply MOO techniques to improve patient outcomes and operational efficiency.
7.2. Genetic Algorithms in Scheduling
Genetic algorithms (GAs) are a class of optimization techniques inspired by the process of natural selection. They are particularly effective in solving complex scheduling problems, where traditional methods may struggle. GAs operate through a cycle of selection, crossover, and mutation to evolve solutions over generations.
Key features of GAs in scheduling include: - Population-based approach, allowing exploration of multiple solutions simultaneously. - Adaptability to various types of scheduling problems, such as job-shop scheduling, flow-shop scheduling, and resource allocation.
Benefits of using GAs for scheduling: - Ability to handle large and complex search spaces. - Flexibility in incorporating various constraints and objectives. - Robustness against local optima, often leading to better overall solutions.
Applications of GAs in scheduling can be found in: - Manufacturing, where they optimize production lines. - Transportation, for route optimization. - Project management, to allocate resources effectively.
At Rapid Innovation, we harness the power of GAs to deliver efficient scheduling solutions tailored to our clients' unique needs. By optimizing resource allocation and improving operational efficiency, we help organizations achieve significant cost savings and enhanced productivity.
7.3. Neural Network-Based Scheduling
Neural networks (NNs) are a subset of machine learning techniques that can model complex relationships in data. They have gained traction in scheduling due to their ability to learn from historical data and make predictions. NNs can be used to predict task durations, resource availability, and other critical factors that influence scheduling.
Key advantages of neural network-based scheduling include: - Ability to process large datasets and identify patterns that traditional methods may overlook. - Flexibility in adapting to changes in scheduling requirements or constraints. - Capability to improve over time as more data becomes available, leading to increasingly accurate predictions.
Common applications of NNs in scheduling involve: - Dynamic job scheduling in manufacturing environments. - Predictive maintenance scheduling based on equipment performance data. - Workforce scheduling, optimizing employee shifts based on demand forecasts.
At Rapid Innovation, we utilize neural networks to enhance the efficiency and effectiveness of scheduling processes. By integrating predictive analytics into scheduling, we empower our clients to make informed decisions that lead to improved operational performance and increased ROI. Our experience with multiobjective optimization and multi objective bayesian optimization further strengthens our capabilities in delivering innovative solutions.
7.4. Constraint Programming
Constraint programming (CP) is a powerful paradigm used in artificial intelligence and operations research for solving combinatorial problems. It focuses on defining a set of constraints that must be satisfied, allowing for efficient problem-solving in various domains.
- Definition: CP involves specifying a problem in terms of variables, domains, and constraints. Variables represent the unknowns, domains are the possible values for these variables, and constraints define the relationships between them.
- Applications: Â
- Scheduling: CP is widely used in scheduling tasks, such as employee shifts or project timelines, where various constraints must be met. Rapid Innovation can implement CP solutions to optimize workforce management, ensuring that resources are utilized effectively while adhering to labor regulations.
- Resource Allocation: It helps in optimizing the allocation of limited resources in manufacturing or logistics. By leveraging CP, Rapid Innovation can assist clients in maximizing output while minimizing costs, leading to a higher return on investment (ROI).
- Configuration Problems: CP is effective in product configuration, where different components must fit together according to specific rules. Rapid Innovation can develop tailored solutions that streamline product design processes, enhancing customer satisfaction and reducing time-to-market.
- Advantages: Â
- Flexibility: CP can handle a wide range of problems without needing to change the underlying algorithm, making it adaptable to various business needs.
- Efficiency: Many CP solvers use advanced techniques like backtracking and constraint propagation to prune the search space, leading to faster solutions. This efficiency translates into cost savings and improved operational performance for our clients.
- Popular Tools: Some well-known CP tools include Google OR-Tools, Choco, and Gecode, which provide robust frameworks for implementing constraint programming solutions. Rapid Innovation utilizes these tools to deliver high-quality, customized solutions that align with client objectives.
7.5. Hybrid Intelligence Approaches
Hybrid intelligence approaches combine multiple artificial intelligence techniques to leverage their strengths and mitigate their weaknesses. This integration can lead to more robust and effective solutions in complex problem domains.
- Definition: Hybrid intelligence typically merges symbolic AI (like rule-based systems) with sub-symbolic AI (like neural networks) to create systems that can reason and learn simultaneously.
- Applications: Â
- Robotics: Hybrid systems can enhance robot perception and decision-making by combining sensory data processing with logical reasoning. Rapid Innovation can develop intelligent robotic systems that improve operational efficiency in manufacturing and logistics.
- Healthcare: In medical diagnosis, hybrid models can integrate expert knowledge with machine learning to improve accuracy and reliability. Our solutions can assist healthcare providers in making better-informed decisions, ultimately leading to improved patient outcomes.
- Natural Language Processing: Combining rule-based parsing with deep learning can enhance understanding and generation of human language. Rapid Innovation can create advanced chatbots and virtual assistants that provide exceptional customer service, driving engagement and satisfaction.
- Advantages: Â
- Improved Performance: By leveraging the strengths of different AI methods, hybrid systems can achieve better performance than single-method approaches, resulting in higher efficiency and effectiveness for our clients.
- Greater Flexibility: Hybrid systems can adapt to various tasks and environments, making them suitable for diverse applications across industries.
- Enhanced Interpretability: Combining symbolic reasoning with machine learning can provide more interpretable models, which is crucial in fields like healthcare and finance, where transparency is essential.
- Examples: Notable examples of hybrid intelligence include IBM Watson, which combines natural language processing with machine learning, and autonomous vehicles that integrate sensor data with decision-making algorithms. Rapid Innovation draws inspiration from these examples to develop innovative solutions tailored to client needs.
8. Performance Metrics and Evaluation
Performance metrics and evaluation are critical components in assessing the effectiveness of AI models and systems. They provide quantitative measures to determine how well a model performs in various tasks.
- Definition: Performance metrics are standardized measures used to evaluate the accuracy, efficiency, and overall effectiveness of AI models. They help in comparing different models and understanding their strengths and weaknesses.
- Common Metrics: Â
- Accuracy: The proportion of correct predictions made by the model compared to the total predictions.
- Precision and Recall: Precision measures the accuracy of positive predictions, while recall assesses the model's ability to identify all relevant instances.
- F1 Score: The harmonic mean of precision and recall, providing a balance between the two metrics.
- AUC-ROC: The area under the receiver operating characteristic curve, which evaluates the trade-off between true positive and false positive rates.
- Evaluation Techniques: Â
- Cross-Validation: A technique that involves partitioning the dataset into subsets to ensure that the model is tested on different data points, reducing overfitting.
- Confusion Matrix: A table used to visualize the performance of a classification model, showing true positives, false positives, true negatives, and false negatives.
- Benchmarking: Comparing the performance of a model against established standards or other models to assess its relative effectiveness.
- Importance: Â
- Model Selection: Performance metrics guide the selection of the best model for a specific task based on empirical evidence.
- Continuous Improvement: Regular evaluation helps in identifying areas for improvement, leading to iterative enhancements in model performance.
- Stakeholder Communication: Clear metrics facilitate communication with stakeholders, providing a transparent view of model capabilities and limitations. Rapid Innovation emphasizes the importance of these metrics to ensure that our clients achieve their business goals efficiently and effectively.
8.1. Key Performance Indicators (KPIs)
Key Performance Indicators (KPIs) are essential metrics that help organizations measure their performance against strategic goals. They provide a clear framework for assessing progress and making informed decisions. The term "kpi meaning" refers to the significance of these indicators in evaluating success.
- KPIs can be categorized into different types: Â
- Financial KPIs: Measure profitability, revenue growth, and cost management.
- Operational KPIs: Focus on efficiency, productivity, and process improvements.
- Customer KPIs: Assess customer satisfaction, retention, and engagement.
- Employee KPIs: Evaluate workforce performance, satisfaction, and turnover rates.
- Setting effective KPIs involves: Â
- Aligning them with business objectives to ensure relevance.
- Making them Specific, Measurable, Achievable, Relevant, and Time-bound (SMART).
- Regularly reviewing and adjusting KPIs based on changing business conditions.
- Examples of common KPIs include: Â
- Net Profit Margin: Indicates profitability relative to revenue.
- Customer Acquisition Cost: Measures the cost of acquiring a new customer.
- Employee Turnover Rate: Assesses workforce stability and satisfaction.
Utilizing KPIs effectively can lead to improved decision-making and enhanced organizational performance. At Rapid Innovation, we leverage AI-driven analytics to help clients define and track KPIs that align with their unique business objectives, ensuring they can measure success accurately and make data-informed decisions. This includes defining KPIs and understanding key performance indicators meaning.
8.2. Efficiency Measurement
Efficiency measurement is the process of evaluating how well resources are utilized to achieve desired outcomes. It is crucial for identifying areas for improvement and optimizing operations.
- Key aspects of efficiency measurement include: Â
- Input vs. Output: Analyzing the ratio of resources used (input) to the results achieved (output).
- Time Management: Assessing how time is spent on various tasks and identifying bottlenecks.
- Resource Utilization: Evaluating how effectively materials, labor, and technology are used.
- Techniques for measuring efficiency: Â
- Benchmarking: Comparing performance against industry standards or competitors.
- Process Mapping: Visualizing workflows to identify inefficiencies and redundancies.
- Performance Analytics: Using data analysis tools to track and measure efficiency metrics.
- Benefits of efficiency measurement: Â
- Cost savings through optimized resource allocation.
- Enhanced productivity leading to higher output.
- Improved quality of products or services by streamlining processes.
By focusing on efficiency measurement, organizations can drive continuous improvement and achieve better results. Rapid Innovation employs advanced AI algorithms to analyze operational data, helping clients identify inefficiencies and implement solutions that enhance productivity and reduce costs.
8.3. Cost Reduction Analysis
Cost reduction analysis involves identifying and implementing strategies to lower expenses without compromising quality or performance. It is a critical component of financial management and operational efficiency.
- Key steps in conducting a cost reduction analysis: Â
- Identify Cost Drivers: Determine the main factors contributing to costs in various areas of the business.
- Analyze Spending Patterns: Review historical spending data to identify trends and areas for potential savings.
- Evaluate Alternatives: Consider different approaches, such as outsourcing, renegotiating contracts, or adopting new technologies.
- Common strategies for cost reduction: Â
- Process Improvement: Streamlining operations to eliminate waste and enhance efficiency.
- Supplier Negotiation: Working with suppliers to secure better pricing or terms.
- Energy Efficiency: Implementing energy-saving measures to reduce utility costs.
- Benefits of cost reduction analysis: Â
- Increased profitability through lower operational costs.
- Enhanced competitiveness by allowing for more flexible pricing strategies.
- Improved cash flow, enabling reinvestment in growth opportunities.
By conducting thorough cost reduction analysis, organizations can achieve sustainable financial health and long-term success. Rapid Innovation assists clients in leveraging AI technologies to uncover hidden cost-saving opportunities, ensuring they can maintain quality while optimizing their financial performance. This includes understanding key performance metrics examples and kpi examples to guide their strategies.
8.4. Flexibility and Responsiveness
Flexibility and responsiveness are critical components in today's fast-paced business environment. Organizations must adapt quickly to changes in market conditions, customer preferences, and technological advancements. Business flexibility refers to the ability of a business to adjust its operations, strategies, and processes in response to external and internal changes, while responsiveness is the speed at which a company reacts to these changes, ensuring that it meets customer needs and stays competitive.
Key aspects of flexibility and responsiveness include:
- Agile methodologies: Implementing agile practices allows teams to pivot quickly, enhancing their ability to respond to market demands. At Rapid Innovation, we leverage AI-driven agile frameworks that enable our clients to streamline their development processes, ensuring they can adapt to changes swiftly.
- Cross-functional teams: Encouraging collaboration across departments fosters a culture of adaptability, enabling quicker decision-making. Our consulting services help organizations build cross-functional teams that utilize AI tools for enhanced communication and collaboration.
- Real-time data analysis: Utilizing data analytics tools helps organizations monitor trends and customer feedback, allowing for timely adjustments to products or services. Rapid Innovation provides advanced AI analytics solutions that empower businesses to make data-driven decisions in real-time.
Companies that prioritize business flexibility and responsiveness often experience:
- Improved customer satisfaction: By quickly addressing customer needs, businesses can enhance loyalty and retention. Our AI solutions help clients personalize customer interactions, leading to higher satisfaction rates.
- Competitive advantage: Organizations that adapt faster than their competitors can capture market share and drive growth. Rapid Innovation's AI technologies enable clients to stay ahead of market trends, ensuring they remain competitive.
- Increased innovation: A flexible approach encourages experimentation and creativity, leading to new ideas and solutions. We assist clients in fostering a culture of innovation through AI-driven insights and tools.
For more insights on how AI can enhance business flexibility and responsiveness, check out business AI engineering best practices.
8.5. Comparative Benchmarking
Comparative benchmarking is a strategic process that involves evaluating an organization's performance against industry standards or competitors. This practice helps businesses identify areas for improvement and set realistic performance goals. Benchmarking can be categorized into several types:
- Internal benchmarking: Comparing performance metrics within different departments of the same organization.
- Competitive benchmarking: Analyzing direct competitors to understand their strengths and weaknesses.
- Functional benchmarking: Looking at best practices from different industries to gain insights.
Benefits of comparative benchmarking include:
- Identifying performance gaps: By comparing metrics, organizations can pinpoint areas where they lag behind competitors. Rapid Innovation employs AI tools to facilitate this analysis, providing clients with actionable insights.
- Setting performance targets: Benchmarking provides a framework for establishing realistic and achievable goals. Our consulting services help organizations define KPIs that align with their strategic objectives.
- Enhancing operational efficiency: Understanding best practices can lead to process improvements and cost reductions. We assist clients in implementing AI solutions that optimize their operations based on benchmarking results.
To effectively implement comparative benchmarking, organizations should:
- Select relevant metrics: Choose key performance indicators (KPIs) that align with business objectives.
- Gather data: Collect information from reliable sources, including industry reports and competitor analysis.
- Analyze results: Evaluate the data to identify trends and areas for improvement.
9. Challenges and Limitations
While flexibility, responsiveness, and comparative benchmarking offer significant advantages, organizations may face several challenges and limitations in their implementation. These include:
- Resistance to change: Employees may be hesitant to adopt new processes or technologies, hindering flexibility and responsiveness. Rapid Innovation provides change management consulting to help organizations navigate this transition smoothly.
- Resource constraints: Limited budgets and personnel can restrict an organization's ability to adapt quickly or invest in benchmarking initiatives. We help clients identify cost-effective AI solutions that maximize their resources.
- Data quality issues: Inaccurate or outdated data can lead to misguided decisions, undermining the effectiveness of benchmarking efforts. Our AI-driven data management solutions ensure that organizations maintain high-quality data for informed decision-making.
Additional challenges include:
- Overemphasis on competition: Focusing too much on competitors can lead to a reactive rather than proactive approach, stifling innovation. We guide clients in developing a balanced strategy that fosters both competitive awareness and innovation.
- Complexity of implementation: Establishing a benchmarking process can be time-consuming and require significant effort to gather and analyze data. Rapid Innovation streamlines this process through automated AI tools that simplify data collection and analysis.
- Maintaining consistency: Ensuring that benchmarking practices are consistently applied across the organization can be difficult, especially in larger companies. We assist organizations in developing standardized benchmarking frameworks that promote consistency.
To overcome these challenges, organizations can:
- Foster a culture of change: Encourage open communication and provide training to help employees embrace new practices.
- Allocate resources strategically: Prioritize investments in areas that will yield the highest returns in flexibility and responsiveness.
- Ensure data integrity: Implement robust data management practices to maintain accurate and reliable information for benchmarking.
By addressing these challenges, organizations can better leverage flexibility, responsiveness, and comparative benchmarking to drive success in a competitive landscape. Rapid Innovation is committed to helping clients navigate these complexities, ensuring they achieve their business goals efficiently and effectively. For those looking to enhance their development capabilities, consider our Action Transformer Developers services.
9.1. Data Quality and Reliability
Data quality and reliability are critical components in any data-driven organization. High-quality data ensures that decisions are based on accurate and relevant information, which can significantly impact business outcomes.
- Accuracy: Data must be correct and free from errors. Inaccurate data can lead to misguided strategies and poor decision-making.
- Completeness: Data should be comprehensive, containing all necessary information. Missing data can skew analysis and lead to incomplete insights.
- Consistency: Data should be uniform across different systems and platforms. Inconsistent data can create confusion and undermine trust in the data.
- Timeliness: Data must be up-to-date to be relevant. Outdated information can lead to decisions based on obsolete trends.
- Reliability: Data should be dependable and sourced from credible origins. Reliable data enhances the overall integrity of the analysis.
At Rapid Innovation, we understand that organizations can improve data quality by implementing robust data governance frameworks, conducting regular audits, and utilizing advanced data cleansing tools. By leveraging our AI-driven solutions, clients can automate data validation processes, ensuring that their data remains accurate and reliable. According to a study, poor data quality can cost organizations up to $15 million annually (source: IBM). Our expertise in AI can help mitigate these costs, ultimately leading to greater ROI. The trustworthiness of data and data quality refers to the assurance that the data is both accurate and reliable, which is essential for effective decision-making.
9.2. Integration Complexities
Integration complexities arise when organizations attempt to combine data from various sources, systems, or platforms. This process can be challenging due to several factors.
- Diverse Data Sources: Organizations often use multiple systems (CRM, ERP, etc.) that may not be compatible, making integration difficult.
- Data Silos: Different departments may store data in isolated systems, leading to a lack of visibility and collaboration.
- Varied Data Formats: Data may come in different formats (structured, unstructured), requiring transformation for effective integration.
- Real-time vs. Batch Processing: Organizations must decide whether to integrate data in real-time or through batch processing, each with its own set of challenges.
- Scalability Issues: As organizations grow, their data integration needs may evolve, necessitating scalable solutions that can adapt to changing requirements.
To address integration complexities, organizations can adopt middleware solutions, utilize APIs, and invest in data integration platforms. At Rapid Innovation, we specialize in creating tailored integration strategies that enhance data accessibility and improve overall operational efficiency. Our AI solutions can streamline the integration process, allowing clients to focus on leveraging their data for strategic decision-making. Ensuring data quality reliability is crucial during this integration phase to maintain the integrity of the combined datasets.
9.3. Change Management
Change management is essential for successfully implementing new processes, technologies, or systems within an organization. Effective change management ensures that transitions are smooth and that employees are engaged throughout the process.
- Communication: Clear communication about the changes is vital. Employees should understand the reasons behind the change and how it will affect their roles.
- Training and Support: Providing adequate training helps employees adapt to new systems or processes. Ongoing support can alleviate concerns and build confidence.
- Stakeholder Involvement: Engaging stakeholders early in the process fosters buy-in and reduces resistance. Their feedback can also provide valuable insights.
- Monitoring and Feedback: Continuous monitoring of the change process allows organizations to identify issues early. Gathering feedback from employees can help refine the approach.
- Cultural Considerations: Understanding the organizational culture is crucial. Change initiatives should align with the company’s values and practices to ensure acceptance.
Implementing a structured change management framework can significantly enhance the likelihood of successful transitions. At Rapid Innovation, we guide organizations through this process, ensuring that our AI solutions are seamlessly integrated into their operations. According to research, organizations with effective change management practices are 6 times more likely to achieve their objectives (source: Prosci). By partnering with us, clients can navigate change confidently and achieve their business goals efficiently and effectively. Reliability in data quality is a key aspect that organizations must focus on during change management to ensure that new processes are built on a solid foundation of trustworthy data.
9.4. Computational Overhead
Computational overhead refers to the additional resources required to perform a task beyond the basic processing needs. In the context of machine learning and artificial intelligence, this can significantly impact performance and efficiency.
- Increased resource consumption: Algorithms may require more CPU, memory, or storage, leading to higher operational costs. Rapid Innovation helps clients optimize their algorithms to minimize resource consumption, ensuring that they achieve maximum efficiency without incurring unnecessary expenses. Techniques such as optimization for machine learning and using optimizers in deep learning can be particularly effective.
- Time delays: Complex models can result in longer training and inference times, affecting real-time applications. Our team at Rapid Innovation employs advanced techniques to streamline model performance, reducing time delays and enhancing responsiveness for critical applications. For instance, mini batch gradient descent can be utilized to improve training efficiency.
- Scalability issues: As data volume grows, the computational demands can escalate, making it challenging to maintain performance. We assist clients in designing scalable solutions that can adapt to increasing data loads, ensuring sustained performance as their business grows. This includes strategies for optimization in deep learning to handle larger datasets effectively.
- Energy consumption: High computational overhead can lead to increased energy usage, raising environmental concerns and operational costs. Rapid Innovation focuses on developing energy-efficient models that not only reduce costs but also align with sustainability goals. Implementing machine learning optimization techniques can help in achieving these energy efficiencies.
Addressing computational overhead is crucial for optimizing machine learning systems. Techniques such as model pruning, quantization, and using more efficient algorithms can help mitigate these issues, and our expertise ensures that clients can implement these strategies effectively. For successful AI integration strategies, check out our AI integration strategies.
9.5. Interpretability and Explainability
Interpretability and explainability are essential aspects of machine learning models, particularly in sensitive applications like healthcare, finance, and law enforcement. These concepts refer to the ability to understand and trust the decisions made by AI systems.
- Importance of transparency: Stakeholders need to comprehend how models arrive at decisions to ensure accountability and trust. Rapid Innovation emphasizes the importance of transparency in AI solutions, helping clients communicate model decisions clearly to their stakeholders. This is especially relevant when discussing hyperparameter in machine learning and its impact on model outcomes.
- Regulatory compliance: Many industries are subject to regulations that require clear explanations of automated decisions. Our consulting services guide clients through compliance requirements, ensuring that their AI systems meet necessary standards.
- User acceptance: Users are more likely to adopt AI solutions if they can understand the rationale behind the outcomes. We work with clients to enhance user experience by making AI outputs more interpretable, fostering greater acceptance and utilization. Techniques such as learning to optimize can be beneficial in this context.
- Techniques for enhancing interpretability: Â
- Feature importance analysis helps identify which variables influence model predictions.
- Visualization tools can illustrate how models make decisions.
- Simplifying models can improve interpretability without sacrificing performance.
Investing in interpretability and explainability not only builds trust but also enhances the overall effectiveness of AI systems, a core focus of Rapid Innovation's development approach.
10. Economic and Strategic Implications
The economic and strategic implications of AI and machine learning are profound, influencing various sectors and reshaping business landscapes.
- Increased productivity: Automation of routine tasks allows employees to focus on higher-value activities, leading to greater efficiency. Rapid Innovation helps clients implement AI solutions that drive productivity gains across their operations, including the use of deep learning for optimization.
- Cost reduction: AI can optimize operations, reduce waste, and lower labor costs, contributing to improved profit margins. Our tailored solutions are designed to identify cost-saving opportunities, ensuring clients achieve a strong return on investment through strategies like price optimization machine learning.
- Competitive advantage: Companies leveraging AI technologies can gain insights that drive innovation and market differentiation. Rapid Innovation empowers clients to harness AI for strategic insights, enabling them to stay ahead of the competition by utilizing advanced optimization techniques.
- Job displacement: While AI creates new opportunities, it can also lead to job losses in certain sectors, necessitating workforce retraining. We provide guidance on workforce transition strategies, helping clients navigate the challenges of AI implementation.
- Investment in AI: Organizations are increasingly allocating budgets to AI research and development, recognizing its potential to transform business models. Rapid Innovation supports clients in maximizing their AI investments, ensuring they realize the full potential of their initiatives, including the adoption of deep learning optimizers.
Understanding these economic and strategic implications is vital for businesses aiming to navigate the evolving landscape shaped by AI and machine learning technologies. Rapid Innovation is committed to guiding clients through this transformation, ensuring they achieve their business goals efficiently and effectively.
10.1. Cost-Benefit Analysis
Cost-benefit analysis (CBA) is a systematic approach to evaluating the economic pros and cons of a project or decision. It helps organizations determine whether the benefits of a project outweigh its costs, making it a crucial tool in decision-making.
- Definition: CBA quantifies the total expected costs against the total expected benefits of a project, often referred to as cost and benefit analysis.
- Purpose: The primary goal is to assess the feasibility and efficiency of a project, which is also known as the benefit of cost benefit analysis.
- Steps involved: Â
- Identify costs: Direct costs, indirect costs, and opportunity costs.
- Identify benefits: Tangible benefits (e.g., increased revenue) and intangible benefits (e.g., improved customer satisfaction).
- Calculate net present value (NPV): Discount future cash flows to present value to compare costs and benefits.
- Sensitivity analysis: Assess how changes in assumptions affect outcomes.
CBA is widely used in various sectors, including healthcare, infrastructure, and environmental projects. It provides a clear framework for stakeholders to make informed decisions based on quantitative data. At Rapid Innovation, we leverage CBA to help clients evaluate the potential return on investment (ROI) of AI projects, ensuring that resources are allocated efficiently and effectively. For example, a cost benefit assessment can illustrate the financial implications of different project options. Additionally, a cost benefit analysis example can help clarify the process for stakeholders unfamiliar with the methodology. You can learn more about how AI technology is changing the construction landscape.
10.2. Competitive Advantage Potential
Competitive advantage potential refers to the unique attributes or capabilities that allow a company to outperform its competitors. Understanding and leveraging these advantages is essential for long-term success in any industry.
- Types of competitive advantages: Â
- Cost leadership: Offering products or services at lower prices than competitors.
- Differentiation: Providing unique features or superior quality that justify a higher price.
- Focus: Targeting a specific market segment more effectively than competitors.
- Factors influencing competitive advantage: Â
- Innovation: Continuous improvement and adaptation to market changes.
- Brand reputation: Building trust and loyalty among customers.
- Operational efficiency: Streamlining processes to reduce costs and improve service delivery.
At Rapid Innovation, we assist clients in identifying and enhancing their competitive advantage potential through AI-driven insights and solutions. By analyzing market trends and customer behavior, we empower organizations to make informed strategic decisions that position them ahead of their competitors.
10.3. Investment Strategies
Investment strategies are plans designed to achieve specific financial goals through the allocation of resources. A well-defined investment strategy can help individuals and organizations maximize returns while managing risk.
- Types of investment strategies: Â
- Growth investing: Focusing on stocks or assets expected to grow at an above-average rate.
- Value investing: Identifying undervalued stocks with strong fundamentals.
- Income investing: Prioritizing investments that generate regular income, such as dividends or interest.
- Key considerations for developing an investment strategy: Â
- Risk tolerance: Understanding how much risk an investor is willing to take.
- Time horizon: Determining the length of time an investment will be held.
- Diversification: Spreading investments across various asset classes to reduce risk.
- Monitoring and adjusting: Regularly reviewing the performance of investments and making necessary adjustments based on market conditions and personal financial goals.
A successful investment strategy requires thorough research, continuous learning, and adaptability to changing market dynamics. Rapid Innovation supports clients in developing AI-focused investment strategies that align with their financial objectives, ensuring they capitalize on emerging opportunities while managing associated risks effectively. An example of cost and benefit analysis can be instrumental in evaluating the effectiveness of different investment strategies.
10.4. Long-Term Value Creation
Long-term value creation is essential for sustainable business growth and involves strategies that focus on generating consistent returns over time, including of long term value and wealth creation from equity investing. Companies that prioritize long-term value creation often outperform their competitors in the market. Key strategies include:
- Focus on sustainable practices: Implementing environmentally friendly practices can enhance brand reputation and customer loyalty. Rapid Innovation can assist in integrating AI-driven sustainability solutions that optimize resource usage and reduce waste.
- Invest in employee development: Training and upskilling employees leads to higher productivity and innovation. Our AI solutions can personalize learning experiences, ensuring that employees acquire the skills necessary to drive business success.
- Foster customer relationships: Building strong relationships with customers can lead to repeat business and referrals. Utilizing AI analytics, Rapid Innovation helps businesses understand customer behavior and preferences, enabling tailored marketing strategies.
- Embrace innovation: Continuously investing in research and development can help companies stay ahead of market trends and meet evolving consumer needs. Rapid Innovation provides cutting-edge AI technologies that facilitate innovation and streamline product development processes.
- Financial discipline: Maintaining a strong balance sheet and prudent financial management ensures resources are available for future investments. Our AI tools can enhance financial forecasting and budgeting, allowing for more informed decision-making.
According to a study by McKinsey, companies that focus on long-term value creation can achieve up to 50% higher returns than those that prioritize short-term gains (source: McKinsey). Additionally, implementing longterm value creation strategies can further enhance these outcomes. For instance, leveraging AI-enabled customer experience software can significantly improve customer engagement and satisfaction, contributing to long-term value.
10.5. Risk Mitigation Approaches
Risk mitigation is crucial for businesses to protect their assets and ensure long-term sustainability. Effective risk management strategies can help organizations identify, assess, and minimize potential risks. Important approaches include:
- Conduct regular risk assessments: Identifying potential risks early allows companies to develop strategies to mitigate them. Rapid Innovation offers AI-driven risk assessment tools that provide real-time insights into potential vulnerabilities.
- Diversify investments: Spreading investments across various sectors can reduce exposure to market volatility. Our consulting services can guide businesses in identifying diverse investment opportunities supported by data analytics.
- Implement robust cybersecurity measures: Protecting sensitive data from cyber threats is essential in today’s digital landscape. Rapid Innovation specializes in AI-enhanced cybersecurity solutions that proactively detect and respond to threats.
- Develop contingency plans: Having a plan in place for unexpected events can minimize disruptions and ensure business continuity. We assist organizations in creating AI-supported contingency strategies that adapt to changing circumstances.
- Foster a risk-aware culture: Encouraging employees to recognize and report risks can lead to proactive management. Our training programs emphasize the importance of risk awareness and equip employees with the tools to identify potential issues.
According to a report by Deloitte, organizations with strong risk management practices can reduce their risk exposure by up to 30% (source: Deloitte).
11. Future Trends and Emerging Technologies
The business landscape is constantly evolving, driven by technological advancements and changing consumer behaviors. Staying ahead of future trends and emerging technologies is vital for companies aiming to maintain a competitive edge. Key trends include:
- Artificial Intelligence (AI): AI is transforming industries by automating processes, enhancing decision-making, and improving customer experiences. Rapid Innovation is at the forefront of AI development, providing tailored solutions that drive efficiency and innovation.
- Internet of Things (IoT): IoT devices are enabling real-time data collection and analysis, leading to more informed business strategies. Our expertise in IoT integration allows businesses to harness data for actionable insights.
- Blockchain technology: This technology is revolutionizing supply chain management and enhancing transparency in transactions. Rapid Innovation offers blockchain solutions that improve traceability and security in business operations.
- Remote work technologies: The rise of remote work has led to increased demand for collaboration tools and digital communication platforms. We provide AI-driven tools that enhance remote collaboration and productivity.
- Sustainability technologies: Innovations in renewable energy and waste management are becoming essential as businesses strive for sustainability. Our consulting services help organizations implement sustainable technologies that align with their long-term goals.
According to Gartner, by 2025, 75% of organizations will shift from piloting to operationalizing AI (source: Gartner).
By understanding and adapting to these trends, businesses can position themselves for success in an ever-changing environment. Rapid Innovation is committed to helping clients navigate these challenges and achieve their business goals efficiently and effectively. For more information on our services, visit our AI technology consulting.
11.1. Quantum Computing in Scheduling
Quantum computing is revolutionizing various fields, including scheduling, by leveraging the principles of quantum mechanics. Traditional computing struggles with complex scheduling problems due to the vast number of possible combinations. Quantum computers, however, can process this information exponentially faster.
- Quantum algorithms can optimize scheduling tasks in real-time, significantly reducing the time required to find optimal solutions.
- They can handle large datasets and complex constraints, making them ideal for industries like logistics, manufacturing, and telecommunications.
- Quantum annealing, a specific type of quantum computing, is particularly effective for solving optimization problems, including scheduling.
Recent studies suggest that quantum computing could outperform classical methods in specific scheduling scenarios, leading to increased efficiency and reduced operational costs. For instance, companies like D-Wave are already exploring quantum solutions for scheduling challenges in various sectors. At Rapid Innovation, we harness these advancements in quantum computing scheduling to help our clients streamline their scheduling processes, ultimately driving greater ROI through enhanced operational efficiency. Our expertise also extends to computer vision software development, ensuring comprehensive solutions for our clients. Additionally, we are exploring how quantum computing can transform blockchain security and scalability in 2024.
11.2. Edge AI and Distributed Intelligence
Edge AI refers to the deployment of artificial intelligence algorithms on local devices rather than relying on centralized cloud servers. This approach enhances the efficiency and speed of data processing, particularly in real-time applications.
- Edge AI reduces latency, allowing for quicker decision-making in critical situations, such as autonomous vehicles or industrial automation.
- It minimizes bandwidth usage by processing data locally, which is essential for IoT devices that generate massive amounts of data.
- Distributed intelligence enables devices to collaborate and share insights, leading to more informed decision-making across networks.
The integration of Edge AI and distributed intelligence is transforming industries by enabling smarter, more responsive systems. For example, in smart cities, traffic management systems can analyze data from various sensors in real-time, optimizing traffic flow and reducing congestion. Rapid Innovation leverages Edge AI to empower our clients with faster, more efficient solutions that enhance their operational capabilities and improve customer experiences.
11.3. Autonomous Planning Systems
Autonomous planning systems are designed to automate the decision-making process in various applications, from logistics to robotics. These systems utilize advanced algorithms and machine learning techniques to create efficient plans without human intervention.
- They can analyze vast amounts of data to identify optimal paths, schedules, and resource allocations.
- Autonomous planning systems are particularly beneficial in dynamic environments where conditions change rapidly, such as supply chain management.
- They enhance operational efficiency by reducing human error and increasing the speed of decision-making.
Industries are increasingly adopting autonomous planning systems to streamline operations. For instance, in manufacturing, these systems can optimize production schedules based on real-time demand and resource availability, leading to significant cost savings and improved productivity. At Rapid Innovation, we implement these advanced systems to help our clients achieve their business goals more effectively, ensuring they remain competitive in an ever-evolving market landscape.
11.4. Blockchain Integration
Blockchain technology is revolutionizing various industries by providing a decentralized and secure method for recording transactions. Integrating blockchain into existing systems, such as through blockchain and ERP integration or blockchain API integration, can enhance transparency, security, and efficiency.
- Decentralization: Unlike traditional databases, blockchain operates on a decentralized network, reducing the risk of data tampering and fraud.
- Transparency: Every transaction on a blockchain is recorded on a public ledger, allowing all participants to verify and audit transactions easily.
- Smart Contracts: These self-executing contracts, with the terms of the agreement directly written into code, can automate processes and reduce the need for intermediaries.
- Supply Chain Management: Blockchain can track products from origin to consumer, ensuring authenticity and reducing counterfeiting.
- Data Security: The cryptographic nature of blockchain enhances data security, making it difficult for unauthorized users to access sensitive information.
At Rapid Innovation, we specialize in helping businesses integrate blockchain technology into their operations, including blockchain integration with ERP and integrating blockchain with IoT. By leveraging our expertise, clients can achieve significant cost savings and improved operational efficiency. Companies that adopt this technology can gain a competitive edge by streamlining processes and enhancing trust among stakeholders.
11.5. Advanced Predictive Capabilities
Advanced predictive capabilities utilize data analytics and machine learning to forecast future trends and behaviors. This technology is essential for businesses looking to make informed decisions based on data-driven insights.
- Data Analysis: By analyzing historical data, businesses can identify patterns and trends that inform future strategies.
- Machine Learning Algorithms: These algorithms can learn from data and improve their predictions over time, leading to more accurate forecasts.
- Real-time Insights: Advanced predictive analytics can provide real-time insights, allowing businesses to respond quickly to changing market conditions.
- Risk Management: Predictive capabilities can help identify potential risks and opportunities, enabling proactive decision-making.
- Customer Behavior Prediction: Understanding customer preferences and behaviors can lead to more targeted marketing strategies and improved customer satisfaction.
Implementing advanced predictive capabilities can significantly enhance a company's ability to anticipate market changes and customer needs, ultimately driving growth and profitability. At Rapid Innovation, we guide our clients through the process of adopting these technologies, ensuring they maximize their return on investment.
12. Implementation Roadmap
An implementation roadmap is a strategic plan that outlines the steps necessary to integrate new technologies or processes within an organization. A well-defined roadmap ensures a smooth transition and maximizes the benefits of the new system.
- Assessment Phase: Evaluate current systems and processes to identify areas for improvement and determine the feasibility of new technology integration, such as blockchain integration services.
- Goal Setting: Define clear objectives and key performance indicators (KPIs) to measure the success of the implementation.
- Stakeholder Engagement: Involve key stakeholders from various departments to ensure buy-in and gather diverse perspectives on the implementation process.
- Resource Allocation: Identify the necessary resources, including budget, personnel, and technology, to support the implementation.
- Pilot Testing: Conduct a pilot test of the new system in a controlled environment to identify potential issues and gather feedback.
- Full-scale Implementation: Roll out the new technology across the organization, ensuring that all employees are trained and equipped to use the system effectively.
- Monitoring and Evaluation: Continuously monitor the implementation process and evaluate its success against the established KPIs, making adjustments as necessary.
A comprehensive implementation roadmap is crucial for minimizing disruptions and ensuring that the organization can fully leverage the benefits of new technologies, including blockchain technology integration. By following these steps, businesses can achieve a successful integration that enhances efficiency and drives innovation, with Rapid Innovation as their trusted partner in this journey.
12.1. Initial Assessment Framework
The Initial Assessment Framework is a crucial step in evaluating the feasibility and potential impact of a project or program. This framework helps organizations identify key metrics and criteria that will guide the assessment process.
- Define objectives: Clearly outline the goals of the assessment, such as understanding community needs or evaluating existing resources. At Rapid Innovation, we assist clients in articulating these objectives to align with their strategic business goals.
- Identify stakeholders: Engage with relevant parties, including community members, local organizations, and government agencies, to gather diverse perspectives. Our expertise in stakeholder engagement ensures that all voices are heard, leading to more comprehensive insights.
- Develop assessment criteria: Establish specific metrics to evaluate the program's effectiveness, such as cost, reach, and sustainability. We leverage AI-driven analytics to create tailored assessment criteria that reflect the unique needs of each project, including frameworks like the organizational project management maturity model and the project assessment framework.
- Collect baseline data: Gather initial data to understand the current situation and establish a reference point for future evaluations. Our data collection methodologies are designed to ensure accuracy and relevance, providing a solid foundation for analysis.
- Analyze data: Use qualitative and quantitative methods to assess the information collected, ensuring a comprehensive understanding of the context. Rapid Innovation employs advanced AI algorithms to derive actionable insights from complex datasets.
- Report findings: Share the results with stakeholders to inform decision-making and foster transparency. Our reporting tools facilitate clear communication of findings, enhancing stakeholder engagement and trust.
The Initial Assessment Framework sets the stage for informed decision-making and helps ensure that subsequent phases of the project are grounded in a solid understanding of the environment and needs, utilizing models such as Kerzner's project management maturity model and the PMI project management maturity model. For accurate project estimation, consider partnering with a project estimation company.
12.2. Pilot Program Design
Pilot Program Design is essential for testing the viability of a project before full-scale implementation. A well-structured pilot can provide valuable insights and help refine the program.
- Define the scope: Clearly outline the objectives, target population, and specific activities to be included in the pilot. Rapid Innovation collaborates with clients to ensure that the pilot's scope aligns with their strategic vision.
- Develop a timeline: Create a realistic schedule that includes key milestones and deadlines for each phase of the pilot. Our project management tools help clients visualize timelines and track progress effectively.
- Allocate resources: Identify the necessary resources, including funding, personnel, and materials, to support the pilot program. We assist in resource allocation to maximize efficiency and minimize costs.
- Establish evaluation metrics: Determine how success will be measured, using both qualitative and quantitative indicators. Our AI capabilities enable the development of robust evaluation metrics tailored to specific project goals, including those outlined in the feasibility assessment framework.
- Engage stakeholders: Involve community members and other stakeholders in the design process to ensure the pilot meets their needs and expectations. We facilitate stakeholder workshops to gather input and foster collaboration.
- Implement the pilot: Execute the program according to the established plan, ensuring that all team members are trained and informed. Rapid Innovation provides training and support to ensure smooth implementation.
- Monitor progress: Continuously track the pilot's implementation, making adjustments as needed based on feedback and observations. Our real-time monitoring tools allow for agile responses to emerging challenges.
A well-designed pilot program can help identify potential challenges and opportunities, allowing for adjustments before scaling up, informed by frameworks such as the project risk identification framework.
12.3. Scalability Considerations
Scalability Considerations are vital for determining whether a program can be expanded effectively after a successful pilot. Understanding scalability ensures that the program can grow without compromising quality or impact.
- Assess demand: Evaluate the need for the program in other areas or populations to determine potential for expansion. Rapid Innovation utilizes market analysis tools to gauge demand accurately.
- Analyze resources: Consider whether the necessary resources, including funding, personnel, and infrastructure, are available to support scaling. We help clients identify gaps and strategize resource acquisition.
- Evaluate processes: Review the program's processes to identify any bottlenecks or inefficiencies that could hinder scalability. Our process optimization techniques ensure that scaling is both efficient and effective, drawing on insights from the project management maturity model examples.
- Develop partnerships: Establish collaborations with other organizations or stakeholders that can provide additional support and resources for expansion. Rapid Innovation leverages its network to facilitate strategic partnerships.
- Plan for training: Ensure that there is a strategy in place for training new staff or volunteers to maintain program quality as it scales. We provide comprehensive training programs to equip teams for growth.
- Monitor and adapt: Continuously assess the program's performance and make necessary adjustments to ensure it remains effective as it grows. Our adaptive management approach ensures that programs evolve in response to changing needs, utilizing the OPM3 organizational project management maturity model as a guiding framework.
By addressing scalability considerations, organizations can better prepare for successful expansion, ensuring that the program continues to meet the needs of the community effectively. Rapid Innovation is committed to guiding clients through this process, maximizing their return on investment and achieving sustainable growth.
12.4. Organizational Change Management
Organizational Change Management (OCM) is a structured approach to managing the transition of individuals, teams, and organizations from a current state to a desired future state. It is essential for ensuring that changes are implemented smoothly and effectively, minimizing resistance and maximizing engagement.
- Importance of OCM: Â
- Facilitates smoother transitions during changes.
- Reduces employee resistance and anxiety.
- Enhances communication and collaboration across teams.
- Key Components of OCM: Â
- Leadership Support: Strong leadership is crucial for driving change. Leaders must communicate the vision and benefits of the change clearly.
- Stakeholder Engagement: Involving stakeholders early in the process helps in understanding their concerns and gaining their support.
- Training and Development: Providing training ensures that employees have the necessary skills to adapt to new processes or technologies.
- Steps in OCM: Â
- Assessment: Evaluate the current state and identify the need for change.
- Planning: Develop a comprehensive change management plan that outlines objectives, timelines, and resources.
- Implementation: Execute the change plan while maintaining open lines of communication.
- Monitoring and Evaluation: Continuously assess the impact of the change and make adjustments as necessary.
- Challenges in OCM: Â
- Resistance to change from employees.
- Lack of clear communication.
- Insufficient training and support.
- Best Practices: Â
- Foster a culture of openness and trust.
- Use feedback mechanisms to gauge employee sentiment.
- Celebrate small wins to build momentum.
At Rapid Innovation, we understand that effective OCM, including managing change management and organizational change management, is critical for the successful implementation of AI solutions. By leveraging our expertise, we help organizations navigate the complexities of change, ensuring that employees are equipped and engaged throughout the transition. This not only minimizes disruption but also enhances the overall return on investment (ROI) from AI initiatives. For more insights on AI development, check out our AI Development Guide for Businesses.
12.5. Continuous Improvement Strategy
A Continuous Improvement Strategy (CIS) is a systematic approach to enhancing processes, products, or services over time. It focuses on incremental improvements rather than large-scale changes, fostering a culture of ongoing enhancement within an organization.
- Importance of Continuous Improvement: Â
- Drives efficiency and productivity.
- Enhances customer satisfaction by delivering better quality products and services.
- Encourages innovation and adaptability in a competitive market.
- Key Principles of Continuous Improvement: Â
- Customer Focus: Understanding customer needs and expectations is vital for driving improvements.
- Employee Involvement: Engaging employees at all levels encourages ownership and accountability for improvements.
- Data-Driven Decision Making: Utilizing data and metrics to identify areas for improvement ensures that changes are based on evidence rather than assumptions.
- Common Methodologies: Â
- Kaizen: A Japanese term meaning "change for better," focusing on small, continuous improvements.
- Lean: A methodology aimed at reducing waste and improving efficiency.
- Six Sigma: A data-driven approach that seeks to improve quality by identifying and removing causes of defects.
- Steps in Implementing a Continuous Improvement Strategy: Â
- Identify Opportunities: Use data analysis to pinpoint areas needing improvement.
- Set Goals: Establish clear, measurable objectives for improvement.
- Implement Changes: Execute the improvement initiatives while ensuring employee involvement.
- Review and Adjust: Regularly assess the effectiveness of changes and make necessary adjustments.
- Challenges in Continuous Improvement: Â
- Resistance to change from employees.
- Lack of management support.
- Insufficient training on improvement methodologies.
- Best Practices: Â
- Foster a culture that encourages experimentation and learning from failures.
- Regularly communicate the benefits of continuous improvement to all employees.
- Recognize and reward contributions to improvement efforts.
At Rapid Innovation, we emphasize the importance of a Continuous Improvement Strategy in the context of AI development. By continuously refining our processes and solutions, including managing change organization and organizational change and change management, we ensure that our clients achieve greater efficiency and effectiveness, ultimately leading to a higher ROI. Our data-driven approach allows us to identify opportunities for enhancement, ensuring that our clients remain competitive in an ever-evolving market.