Table Of Contents
Category
Artificial Intelligence
AIML
IoT
Computer Vision
CRM
Security
Marketing
FinTech
1. Introduction to AI in Loan Processing
Artificial Intelligence (AI) is revolutionizing various sectors, and the financial services industry is no exception. In loan processing, AI in loan processing is transforming traditional methods, making them more efficient, accurate, and customer-friendly. AI technologies, such as machine learning and natural language processing, are being integrated into loan processing systems. These technologies help in automating repetitive tasks, reducing human error, and speeding up the decision-making process. Additionally, AI agents can analyze vast amounts of data quickly, providing insights that were previously difficult to obtain.
The adoption of AI in loan processing is driven by the need for improved customer experiences and operational efficiency. Financial institutions are increasingly recognizing the potential of AI to streamline their processes and enhance service delivery. AI can assist in credit scoring by evaluating a borrower's creditworthiness more comprehensively, allowing lenders to make informed decisions that minimize risk. It can also help in fraud detection by identifying unusual patterns in loan applications, thereby safeguarding financial institutions against potential losses. The use of AI can lead to faster loan approvals, which is a significant advantage for both lenders and borrowers, ultimately resulting in a higher return on investment (ROI) for financial organizations.
At Rapid Innovation, we leverage our expertise in AI to help clients implement these transformative technologies effectively. By partnering with us, financial institutions can harness the power of AI to enhance their loan processing capabilities, improve customer satisfaction, and achieve greater operational efficiency. As the financial landscape continues to evolve, the integration of AI in loan processing is expected to grow, leading to more innovative solutions and improved financial services.
Refer to the image for a visual representation of AI's impact on loan processing:
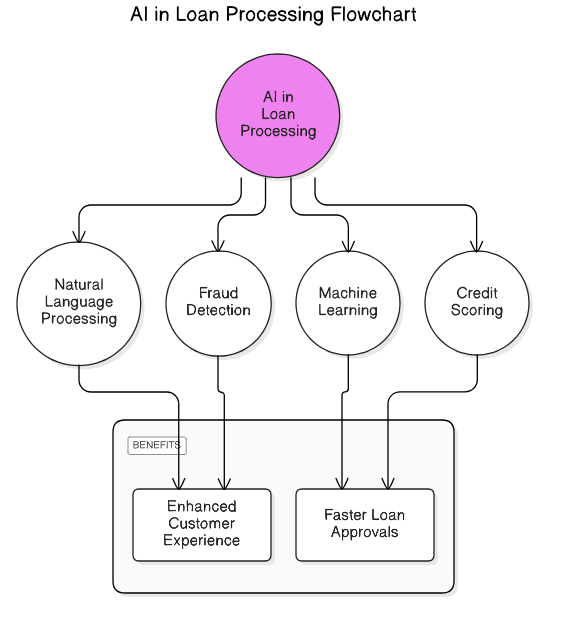
1.1. Evolution of Loan Processing Systems
The evolution of loan processing systems has been a significant journey, reflecting advancements in technology and changing consumer expectations.
- Early loan processing was manual, involving extensive paperwork and face-to-face interactions.
- The introduction of computers in the 1980s began to streamline processes, allowing for faster data entry and retrieval.
- In the 1990s, the rise of the internet enabled online applications, making it easier for borrowers to apply for loans from anywhere.
- The 2000s saw the emergence of automated underwriting systems, such as automated underwriting system mortgage and automated loan origination system, which used algorithms to assess creditworthiness, reducing the time taken for approvals.
- Today, loan processing systems, including automated loan processing system and digital loan processing system, are increasingly integrated with artificial intelligence (AI) and machine learning, allowing for real-time data analysis and improved decision-making.
- Modern systems also focus on user experience, offering mobile applications and online portals that enhance accessibility for borrowers.
The evolution of these systems has not only improved efficiency but also transformed the way lenders interact with customers, making the loan process more transparent and user-friendly. At Rapid Innovation, we leverage these advancements to help our clients implement cutting-edge loan processing solutions, such as lending origination system and loan processing software, that drive greater ROI and enhance customer satisfaction. Additionally, we offer AI insurance solutions that can further enhance the capabilities of loan processing systems. For more insights on how AI agents are revolutionizing loan approvals, check out our article on AI agents for loan approval.
1.2. The Need for AI Automation
The need for AI automation in loan processing is driven by several factors that highlight the limitations of traditional methods.
- Increased volume of loan applications: With the rise of digital banking, lenders face a surge in applications, necessitating faster processing times.
- Enhanced accuracy: AI can analyze vast amounts of data quickly, reducing human error in credit assessments and risk evaluations, which is crucial for systems like automated loan approval system and automated underwriting mortgage.
- Cost efficiency: Automating repetitive tasks can significantly lower operational costs for lenders, allowing them to allocate resources more effectively.
- Improved customer experience: AI-driven chatbots and virtual assistants can provide instant support to borrowers, enhancing satisfaction and engagement.
- Regulatory compliance: AI can help ensure adherence to complex regulations by automating compliance checks and reporting, which is essential for systems like mortgage lead management system and mortgage application tracking system.
As the financial landscape continues to evolve, the integration of AI automation in loan processing is becoming essential for lenders to remain competitive and meet customer expectations. Rapid Innovation specializes in developing AI-driven solutions that streamline loan processing, enabling our clients to achieve significant cost savings and improved operational efficiency.
1.3. Current Industry Challenges
Despite advancements in loan processing systems and the integration of AI, the industry faces several challenges that hinder optimal performance.
- Data security: With the increasing digitization of loan applications, protecting sensitive customer information from cyber threats is a top priority for lenders.
- Integration issues: Many financial institutions still rely on legacy systems that are not easily compatible with modern technologies, creating inefficiencies, particularly in systems like loan processing systems and types of automated underwriting system.
- Regulatory compliance: The financial industry is heavily regulated, and staying compliant with changing laws can be a daunting task for lenders.
- Customer trust: Building and maintaining trust with borrowers is crucial, especially in an era where data breaches and fraud are prevalent.
- Talent acquisition: The demand for skilled professionals who can manage and interpret AI systems is growing, but there is a shortage of qualified candidates in the market.
Addressing these challenges is vital for the continued evolution of loan processing systems and the successful implementation of AI automation in the industry. At Rapid Innovation, we provide expert consulting and development services to help our clients navigate these challenges, ensuring they can leverage AI and blockchain technologies effectively to enhance their loan processing capabilities, including automated loan origination system and loan application processing system, and achieve greater ROI.
Refer to the image for a visual representation of the evolution of loan processing systems.

1.4. Regulatory Landscape Overview
The regulatory landscape for artificial intelligence (AI) in financial services is evolving rapidly. Governments and regulatory bodies are increasingly focusing on how AI technologies are implemented, particularly in sensitive areas like loan processing.
- Regulatory bodies are concerned about issues such as data privacy, algorithmic bias, and transparency.
- The European Union has proposed the AI Act, which aims to create a legal framework for AI applications, categorizing them based on risk levels.
- In the United States, agencies like the Consumer Financial Protection Bureau (CFPB) are scrutinizing AI use in lending to ensure compliance with fair lending laws.
- Compliance with regulations such as the General Data Protection Regulation (GDPR) is crucial for financial institutions using AI, as it governs data protection and privacy.
- Financial institutions must also consider sector-specific regulations, such as the Fair Credit Reporting Act (FCRA), which impacts how credit data is used in AI models.
Understanding the regulatory landscape is essential for financial institutions to mitigate risks and ensure compliance while leveraging AI technologies, including applications of AI in finance and artificial intelligence in banking. At Rapid Innovation, we provide expert consulting services to help clients navigate these complex regulations, ensuring that their AI implementations are compliant and aligned with industry standards. For more information on the use cases and applications of AI in banking and finance.
1.5. Impact on Financial Institutions
The integration of AI in financial institutions is transforming operations, particularly in loan processing. The impact can be seen in several key areas:
- Enhanced Efficiency: AI automates repetitive tasks, reducing the time required for loan approvals and processing.
- Improved Decision-Making: AI algorithms analyze vast amounts of data, providing insights that help in making informed lending decisions.
- Risk Management: AI tools can identify potential risks and fraud patterns, allowing institutions to take proactive measures.
- Customer Experience: AI-driven chatbots and virtual assistants improve customer service by providing instant responses to inquiries.
- Cost Reduction: By automating processes, financial institutions can lower operational costs and allocate resources more effectively.
However, the adoption of AI also presents challenges, including the need for skilled personnel and the potential for algorithmic bias, which can affect lending decisions. Rapid Innovation offers tailored solutions to address these challenges, ensuring that our clients can harness the full potential of AI in financial services while minimizing risks.
2. Core AI Technologies in Loan Processing
AI technologies are revolutionizing loan processing by enhancing efficiency, accuracy, and customer experience. Key technologies include:
- Machine Learning (ML): ML algorithms analyze historical data to predict borrower behavior and assess creditworthiness. This technology helps in automating the underwriting process.
- Natural Language Processing (NLP): NLP enables systems to understand and interpret human language, allowing for better customer interactions through chatbots and automated responses.
- Robotic Process Automation (RPA): RPA automates routine tasks such as data entry and document verification, streamlining the loan application process.
- Predictive Analytics: This technology uses statistical algorithms and machine learning techniques to identify the likelihood of future outcomes based on historical data, aiding in risk assessment.
- Computer Vision: Used for document verification, computer vision can analyze images of documents to extract relevant information, reducing manual errors.
These core AI technologies not only improve the efficiency of loan processing but also enhance the overall customer experience, making the lending process faster and more reliable. At Rapid Innovation, we leverage these technologies to deliver innovative solutions that drive greater ROI for our clients in the financial sector, including applications of artificial intelligence in financial services and wealth management artificial intelligence.
Refer to the image for a visual representation of the regulatory landscape for AI in financial services.
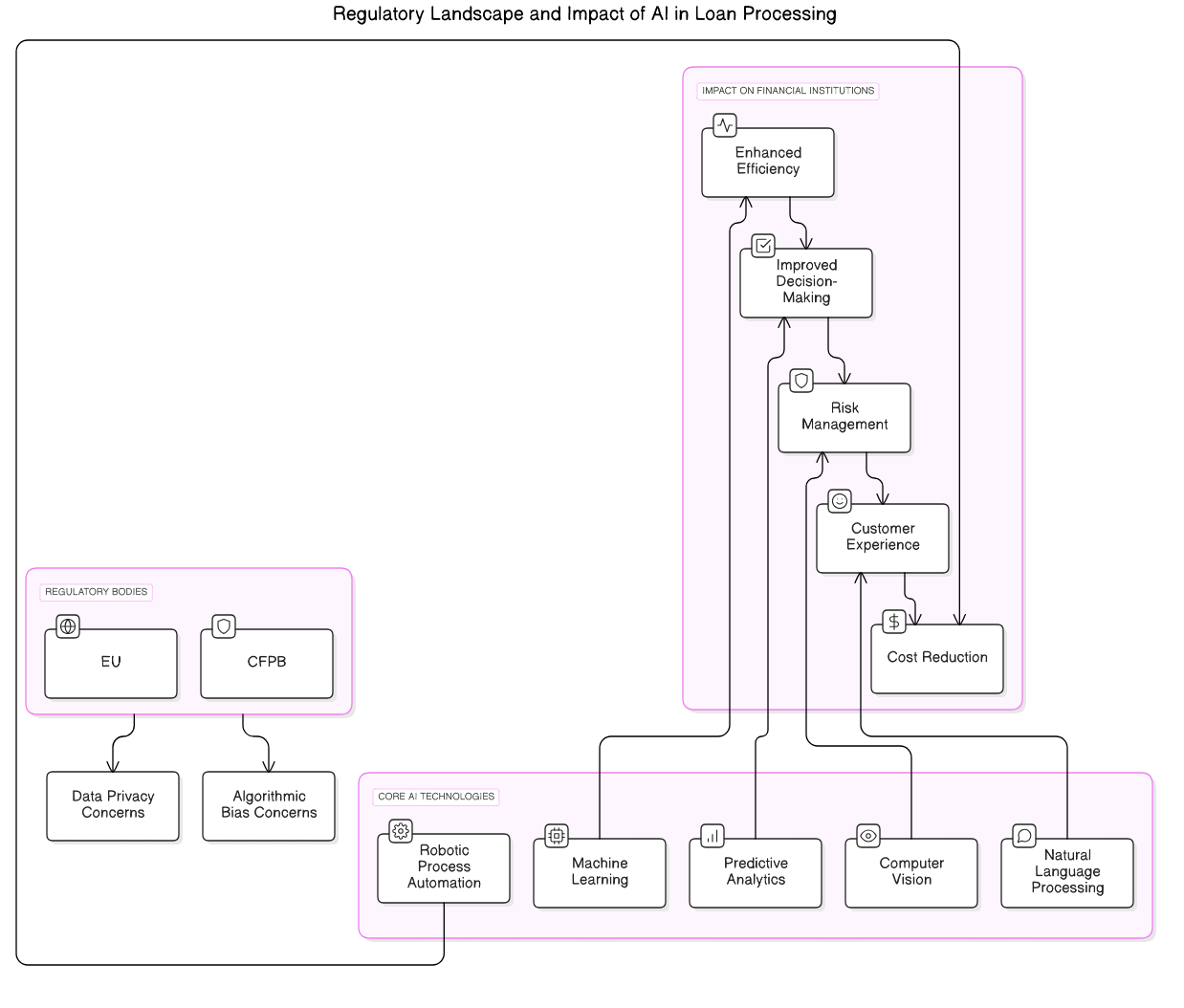
2.1. Machine Learning Foundations
Machine learning (ML) is a subset of artificial intelligence (AI) that enables systems to learn from data, identify patterns, and make decisions with minimal human intervention. The foundations of machine learning are built on various algorithms and statistical models that allow computers to process and analyze vast amounts of data. Understanding these foundations is crucial for anyone looking to delve into the field of machine learning, including designing machine learning systems and applying machine learning in various domains.
- Key components of machine learning include:
- Data: The raw information used for training models.
- Algorithms: The mathematical procedures that process data.
- Models: The output of algorithms after training on data.
- Features: The individual measurable properties or characteristics of the data.
2.1.1. Supervised Learning Models
Supervised learning is a type of machine learning where the model is trained on labeled data. This means that the input data is paired with the correct output, allowing the model to learn the relationship between the two. Supervised learning is widely used for classification and regression tasks, including machine learning applications in healthcare and business intelligence.
- Common supervised learning algorithms include: Â
- Linear Regression: Used for predicting continuous values.
- Logistic Regression: Used for binary classification problems.
- Decision Trees: A flowchart-like structure that makes decisions based on feature values.
- Support Vector Machines (SVM): Effective for high-dimensional spaces and classification tasks.
- Neural Networks: Complex models that mimic the human brain, suitable for various tasks, including deep learning applications.
- Applications of supervised learning: Â
- Email filtering: Classifying emails as spam or not spam.
- Image recognition: Identifying objects within images, such as in machine learning image classification.
- Medical diagnosis: Predicting diseases based on patient data, including machine learning in drug discovery.
At Rapid Innovation, we leverage supervised learning to help clients enhance their decision-making processes. For instance, by implementing predictive analytics in healthcare, we enable organizations to forecast patient outcomes, thereby improving treatment plans and operational efficiency, ultimately leading to a greater return on investment (ROI).
Supervised learning requires a significant amount of labeled data, which can be time-consuming and expensive to obtain. However, its effectiveness in providing accurate predictions makes it a popular choice in many industries, including machine learning for systems and applications.
2.1.2. Unsupervised Learning Applications
Unsupervised learning, in contrast to supervised learning, deals with unlabeled data. The model attempts to learn the underlying structure of the data without any explicit guidance on what the output should be. This type of learning is particularly useful for discovering hidden patterns or groupings within data, such as in manifold learning.
- Common unsupervised learning techniques include: Â
- Clustering: Grouping similar data points together (e.g., K-means, hierarchical clustering).
- Dimensionality Reduction: Reducing the number of features while preserving essential information (e.g., Principal Component Analysis, t-SNE).
- Anomaly Detection: Identifying unusual data points that do not fit the expected pattern.
- Applications of unsupervised learning: Â
- Market segmentation: Identifying distinct customer groups for targeted marketing, which can be enhanced by machine learning and business intelligence.
- Recommendation systems: Suggesting products based on user behavior and preferences.
- Image compression: Reducing the size of images while maintaining quality, relevant in image processing machine learning.
At Rapid Innovation, we utilize unsupervised learning techniques to help businesses uncover valuable insights from their data. For example, by employing clustering algorithms, we assist retail clients in segmenting their customer base, enabling them to tailor marketing strategies that resonate with specific groups, thus maximizing their marketing ROI.
Unsupervised learning is particularly valuable in scenarios where labeled data is scarce or unavailable. It allows organizations to gain insights from their data without the need for extensive labeling efforts, making it applicable in various fields, including signal processing for machine learning and machine learning in embedded systems.
Refer to the image for a visual representation of the concepts discussed in the Machine Learning Foundations.

2.1.3. Reinforcement Learning Use Cases
Reinforcement Learning (RL) is a subset of machine learning where an agent learns to make decisions by taking actions in an environment to maximize cumulative rewards. This approach has numerous practical applications across various industries, and Rapid Innovation leverages RL to help clients achieve their business goals efficiently and effectively.
- Gaming: RL has been successfully applied in video games, where agents learn to play and improve their strategies over time. Notable examples include AlphaGo, which defeated a world champion in the game of Go, and OpenAI's Dota 2 bot, which learned to play the complex multiplayer game at a high level. Rapid Innovation can assist gaming companies in developing advanced AI agents that enhance user engagement and retention.
- Robotics: In robotics, RL is used to train robots to perform tasks such as walking, grasping objects, and navigating environments. By simulating different scenarios, robots can learn optimal movements and strategies to complete tasks efficiently. Rapid Innovation can provide tailored RL solutions to improve automation processes in manufacturing and logistics, leading to significant cost savings.
- Finance: In the financial sector, RL algorithms are employed for algorithmic trading, portfolio management, and risk assessment. These systems can adapt to market changes and optimize trading strategies based on historical data and real-time information. Rapid Innovation's expertise in RL can help financial institutions enhance their trading algorithms, resulting in greater ROI and reduced risk exposure.
- Healthcare: RL is being explored for personalized treatment plans, optimizing drug dosages, and managing patient care pathways. By analyzing patient data, RL can help in making better clinical decisions. Rapid Innovation can collaborate with healthcare providers to implement RL solutions that improve patient outcomes and operational efficiency.
- Autonomous Vehicles: Self-driving cars utilize RL to make real-time decisions based on their surroundings. The vehicles learn to navigate complex environments, avoid obstacles, and follow traffic rules through continuous feedback. Rapid Innovation can support automotive companies in developing robust RL systems that enhance the safety and reliability of autonomous driving technologies.
- Multi-Agent Systems: The application of multi-agent reinforcement learning is gaining traction in various domains, where multiple agents interact and learn from each other. This approach can be particularly useful in scenarios such as traffic management, resource allocation, and collaborative robotics. Rapid Innovation can help organizations implement multi-agent RL solutions that optimize performance in complex environments.
- Real-World Applications: Reinforcement learning applications extend to various real-world scenarios, including fraud detection, where RL can adaptively learn to identify fraudulent activities based on patterns in data. Rapid Innovation can assist businesses in deploying RL for fraud detection, enhancing security measures and reducing financial losses.
- Deep Reinforcement Learning: The integration of deep learning with reinforcement learning has led to significant advancements in various applications. Rapid Innovation can leverage deep reinforcement learning algorithms to tackle complex problems in fields such as computer vision and natural language processing, providing innovative solutions for clients.
- Learning to Communicate: Research in learning to communicate with deep multi-agent reinforcement learning has shown promising results in improving cooperation among agents. Rapid Innovation can explore these techniques to enhance collaborative systems in industries such as logistics and supply chain management.
- Soft Actor-Critic Algorithms: The use of soft actor-critic algorithms and their applications in various domains is an area of interest. Rapid Innovation can implement these advanced RL techniques to improve decision-making processes in dynamic environments.
- Reinforcement Learning Use Cases: The versatility of reinforcement learning use cases spans across different sectors, including robotics, finance, healthcare, and gaming. Rapid Innovation is committed to exploring and implementing these use cases to drive innovation and efficiency for its clients.
2.4. Predictive Analytics
Predictive analytics is a branch of advanced analytics that uses historical data, statistical algorithms, and machine learning techniques to identify the likelihood of future outcomes. In various industries, predictive analytics plays a crucial role in decision-making processes.
- Data Utilization: Predictive analytics relies heavily on data from various sources, including customer behavior, market trends, and historical performance. This data is analyzed to forecast future events.
- Techniques Used: Common techniques include regression analysis, time series analysis, and machine learning algorithms. These methods help in identifying patterns and making predictions.
- Applications: Â
- In finance, predictive analytics can assess credit risk and predict loan defaults.
- In marketing, it helps in customer segmentation and targeting.
- In healthcare, it can predict patient outcomes and optimize treatment plans.
- Examples of predictive analytics applications include hr predictive analytics tools and applications of predictive modeling.
- Benefits: Â
- Improved accuracy in forecasting.
- Enhanced decision-making capabilities.
- Increased operational efficiency.
- Challenges: Â
- Data quality and availability can impact predictions.
- The complexity of models may require specialized skills.
- Ethical considerations regarding data privacy and bias.
At Rapid Innovation, we leverage predictive analytics to empower our clients to make informed decisions that drive business growth. By utilizing advanced machine learning techniques, we help organizations enhance their forecasting accuracy, leading to better resource allocation and increased ROI. Our work includes the application of predictive analysis in healthcare and the business application of predictive analytics.
2.5. Decision Support Systems
Decision Support Systems (DSS) are computer-based information systems that support business or organizational decision-making activities. They combine data, sophisticated analytical models, and user-friendly software to help decision-makers analyze complex data.
- Components of DSS: Â
- Data Management: A DSS integrates data from various sources, ensuring that decision-makers have access to relevant information.
- Model Management: It includes mathematical and analytical models that help in evaluating different scenarios and outcomes.
- User Interface: A user-friendly interface allows decision-makers to interact with the system easily, facilitating better understanding and analysis.
- Types of DSS: Â
- Data-driven DSS: Focuses on the analysis of large volumes of data.
- Model-driven DSS: Utilizes mathematical models to analyze data and support decision-making.
- Knowledge-driven DSS: Provides specialized knowledge to help in decision-making processes.
- Applications: Â
- In finance, DSS can assist in portfolio management and risk assessment.
- In healthcare, it can support clinical decision-making and resource allocation.
- In supply chain management, it helps optimize inventory levels and logistics.
- Applications of predictive analysis are also relevant in this context.
- Benefits: Â
- Enhanced decision quality and speed.
- Improved collaboration among team members.
- Better resource allocation and risk management.
- Challenges: Â
- High implementation costs and complexity.
- Resistance to change from users accustomed to traditional decision-making methods.
- The need for continuous updates and maintenance to ensure data relevance.
Rapid Innovation specializes in developing tailored Decision Support Systems that enable organizations to make data-driven decisions efficiently. Our solutions enhance collaboration and improve decision quality, ultimately leading to better business outcomes.
3. AI Agent Architecture for Loan Processing
AI agent architecture for loan processing refers to the structured framework that enables artificial intelligence systems to automate and optimize the loan approval process. This architecture integrates various components to enhance efficiency, accuracy, and customer experience.
- Core Components: Â
- Data Ingestion: AI agents collect and process data from multiple sources, including credit reports, financial statements, and customer applications.
- Machine Learning Models: These models analyze historical data to assess creditworthiness and predict loan defaults. They continuously learn from new data to improve accuracy.
- Decision Engine: This component evaluates the processed data against predefined criteria to make real-time lending decisions.
- Workflow Automation: Â
- AI agents streamline the loan processing workflow by automating repetitive tasks such as data entry, document verification, and compliance checks. This reduces processing time and minimizes human error.
- Customer Interaction: Â
- AI chatbots and virtual assistants enhance customer service by providing instant responses to inquiries and guiding applicants through the loan process. They can also gather additional information needed for loan approval.
- Risk Assessment: Â
- AI agents utilize predictive analytics to assess risks associated with loan applications, enabling lenders to make informed decisions. They can identify potential fraud by analyzing patterns and anomalies in data, similar to crm predictive modeling techniques.
- Benefits: Â
- Faster loan processing times lead to improved customer satisfaction.
- Enhanced accuracy in credit assessments reduces the risk of defaults.
- Cost savings through automation and reduced manual intervention.
- Challenges: Â
- Ensuring data privacy and compliance with regulations.
- The need for transparency in AI decision-making processes.
- Addressing biases in algorithms to ensure fair lending practices.
At Rapid Innovation, we are at the forefront of revolutionizing the financial industry through our AI agent architecture for loan processing. Our solutions not only enhance efficiency and accuracy but also significantly improve the customer experience, ultimately driving greater ROI for our clients.
3.1. System Components
In any data-driven architecture, understanding the system components is crucial for effective data management and analysis. The system components typically include the data ingestion layer, processing engine, storage solutions, and visualization tools. Each of these components plays a vital role in ensuring that data flows seamlessly from its source to actionable insights.
- Data ingestion layer: This is the entry point for data into the system. It is responsible for collecting and importing data from various sources, which can include databases, APIs, and real-time data streams. The ingestion layer ensures that data is captured in a timely manner and is prepared for further processing.
- Processing engine: Once data is ingested, it needs to be processed to extract meaningful insights. The processing engine performs various operations such as data transformation, aggregation, and analysis. This component is essential for turning raw data into structured information that can be easily understood and utilized.
3.1.1. Data Ingestion Layer
The data ingestion layer is a critical component of any data architecture. It serves as the bridge between data sources and the processing engine. This layer can handle various types of data, including structured, semi-structured, and unstructured data.
- Types of data ingestion: Â
- Batch ingestion: Data is collected and processed in large batches at scheduled intervals. This method is suitable for scenarios where real-time data is not critical.
- Real-time ingestion: Data is ingested continuously as it is generated. This approach is essential for applications that require immediate insights, such as fraud detection or monitoring systems.
- Key functions of the data ingestion layer: Â
- Data collection: Gathers data from multiple sources, ensuring that all relevant information is captured.
- Data validation: Checks the quality and integrity of the data before it moves to the processing engine.
- Data transformation: Prepares data for analysis by converting it into a suitable format.
- Tools and technologies: Various tools are available for data ingestion, including Apache Kafka, Apache NiFi, AWS Glue, and open source data ingestion tools. These tools facilitate the efficient collection and movement of data across different systems, including data ingestion into data lakes and cloud data ingestion.
3.1.2. Processing Engine
The processing engine is the heart of the data architecture, where the actual data manipulation and analysis occur. It takes the ingested data and applies various algorithms and processes to derive insights.
- Types of processing: Â
- Batch processing: Involves processing large volumes of data at once. This method is efficient for tasks that do not require immediate results, such as generating reports or performing complex calculations.
- Stream processing: Handles data in real-time, allowing for immediate analysis and response. This is crucial for applications that need to react to data as it arrives, such as in real-time data ingestion scenarios.
- Key functions of the processing engine: Â
- Data transformation: Converts raw data into a structured format that is easier to analyze.
- Data aggregation: Combines data from multiple sources to provide a comprehensive view.
- Data analysis: Applies statistical and machine learning techniques to extract insights and patterns from the data.
- Tools and technologies: Popular processing engines include Apache Spark, Apache Flink, and Google Cloud Dataflow. These tools provide powerful capabilities for both batch and stream processing, enabling organizations to handle large datasets efficiently.
In summary, the data ingestion layer and processing engine are fundamental components of a data architecture. They work together to ensure that data is collected, validated, transformed, and analyzed effectively, enabling organizations to make data-driven decisions. At Rapid Innovation, we leverage these components to help our clients optimize their data strategies, ensuring they achieve greater ROI through efficient data management and insightful analytics, including automated data ingestion and AI as a Service solutions tailored for big data environments, including revolutionizing data processing.
3.1.3. Decision Layer
The decision layer is a critical component in various systems, particularly in artificial intelligence and machine learning frameworks. This layer is responsible for analyzing data inputs and making informed decisions based on predefined algorithms and models.
- Functions of the Decision Layer: Â
- Processes data from the input layer.
- Applies algorithms to derive insights and predictions.
- Utilizes machine learning models to enhance decision-making accuracy.
- Incorporates business rules and logic to align decisions with organizational goals.
- Importance of the Decision Layer: Â
- Enhances operational efficiency by automating decision-making processes.
- Reduces human error by relying on data-driven insights.
- Facilitates real-time decision-making, which is crucial in dynamic environments.
- Supports predictive analytics, allowing organizations to anticipate future trends.
- Technologies Involved: Â
- Machine learning frameworks (e.g., TensorFlow, PyTorch).
- Decision trees and neural networks for complex decision-making.
- Rule-based systems for straightforward decision processes.
At Rapid Innovation, we leverage the decision layer in AI to help clients optimize their operations. For instance, by implementing machine learning models tailored to specific business needs, we enable organizations to automate routine decisions, thereby increasing efficiency and reducing costs. This approach not only enhances productivity but also leads to a significant return on investment (ROI) as businesses can allocate resources more effectively. For more insights on best practices, check out the potential of business AI engineering.
3.1.4. Output Generation
Output generation is the final stage in a data processing system where the results of the decision layer are transformed into actionable insights or deliverables. This stage is essential for communicating findings to stakeholders and enabling further actions.
- Key Aspects of Output Generation: Â
- Formats outputs in user-friendly ways, such as reports, dashboards, or visualizations.
- Ensures that the output is relevant and tailored to the audience's needs.
- Provides clear and concise summaries of the data analysis and decision-making processes.
- Types of Outputs: Â
- Visual representations (charts, graphs) for quick comprehension.
- Detailed reports for in-depth analysis.
- Alerts and notifications for immediate action.
- Importance of Effective Output Generation: Â
- Enhances decision-making by providing clear insights.
- Facilitates communication between technical teams and stakeholders.
- Supports strategic planning by presenting data in a digestible format.
At Rapid Innovation, we understand that effective output generation is key to driving business success. By providing clients with tailored dashboards and reports, we ensure that decision-makers have access to the insights they need to make informed choices. This capability not only streamlines operations but also enhances strategic planning, ultimately leading to improved ROI.
3.2. Integration Points
Integration points refer to the various interfaces and connections between different components of a system, allowing for seamless data flow and communication. In the context of software architecture, these points are crucial for ensuring that different modules work together effectively.
- Types of Integration Points: Â
- APIs (Application Programming Interfaces) for data exchange between systems.
- Middleware solutions that facilitate communication between disparate applications.
- Data pipelines that ensure smooth data transfer and processing.
- Importance of Integration Points: Â
- Promotes interoperability among different systems and applications.
- Enhances data consistency and accuracy across platforms.
- Reduces redundancy by allowing systems to share resources and information.
- Best Practices for Integration: Â
- Use standardized protocols (e.g., REST, SOAP) for API development.
- Implement robust error handling to manage integration failures.
- Regularly monitor and maintain integration points to ensure optimal performance.
By focusing on these components—decision layer in AI, output generation, and integration points—organizations can build more efficient and effective systems that leverage data for strategic advantage. At Rapid Innovation, we specialize in creating integrated solutions that not only enhance operational efficiency but also drive significant ROI for our clients.
3.3. Security Framework
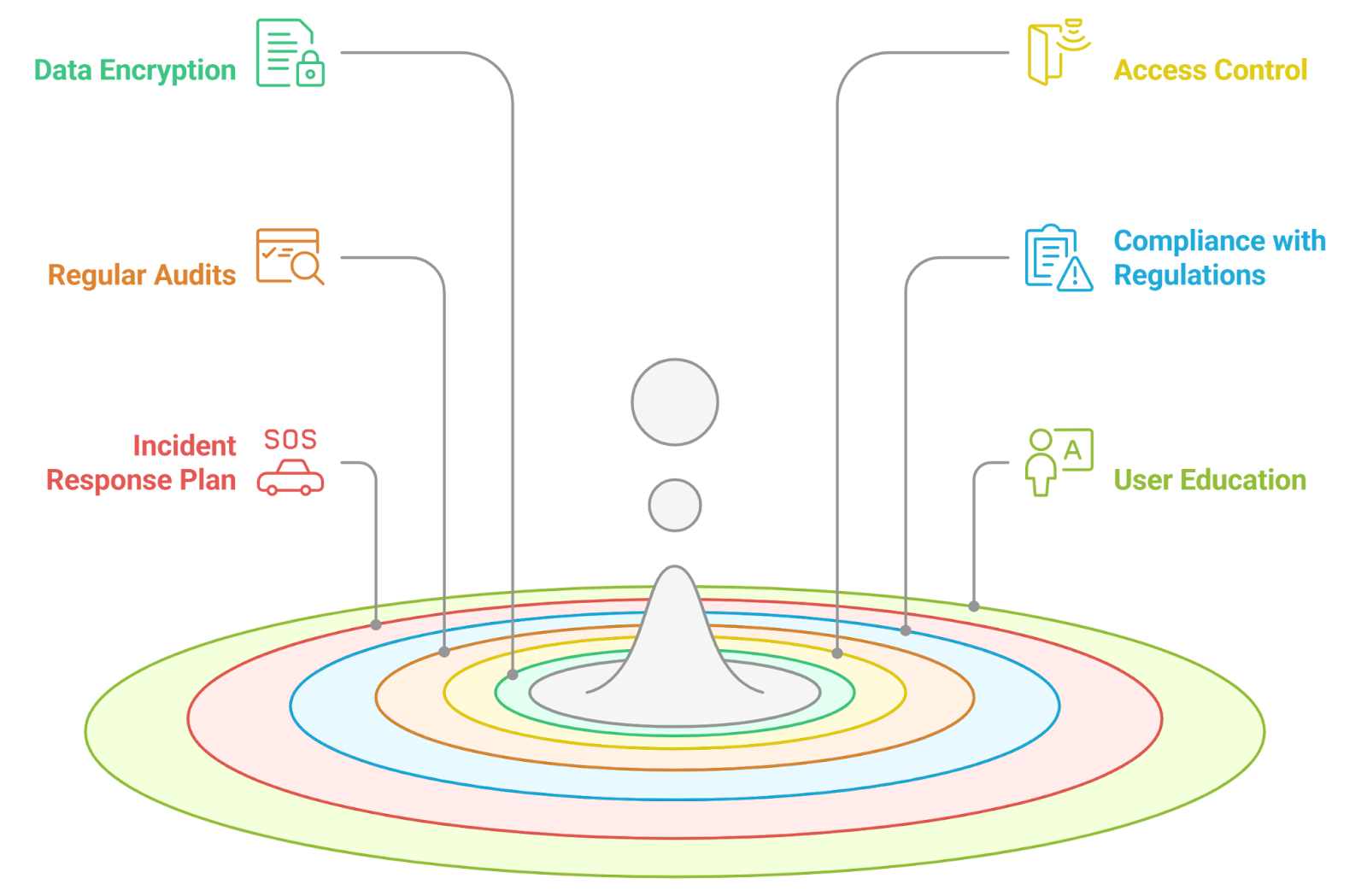
‍
A robust security framework is essential for any financial institution, particularly in the context of loan processing security. This framework encompasses various strategies and technologies designed to protect sensitive data and ensure compliance with regulations.
- Data Encryption: Encrypting data both at rest and in transit is crucial. This ensures that sensitive information, such as personal identification and financial details, is safeguarded against unauthorized access.
- Access Control: Implementing strict access controls helps limit who can view or manipulate sensitive data. Role-based access control (RBAC) is often employed to ensure that only authorized personnel can access specific information.
- Regular Audits: Conducting regular security audits and vulnerability assessments helps identify potential weaknesses in the system. This proactive approach allows organizations to address issues before they can be exploited.
- Compliance with Regulations: Adhering to regulations such as GDPR, CCPA, and PCI DSS is vital. These regulations set standards for data protection and privacy, ensuring that organizations handle customer information responsibly.
- Incident Response Plan: Having a well-defined incident response plan is essential for quickly addressing security breaches. This plan should outline the steps to take in the event of a data breach, including communication strategies and remediation efforts.
- User Education: Training employees on security best practices can significantly reduce the risk of human error, which is often a leading cause of security breaches.
3.4. Scalability Considerations
Scalability is a critical factor in the design and implementation of loan processing systems. As demand for loans fluctuates, the system must be able to adapt without compromising performance or user experience.
- Cloud Infrastructure: Utilizing cloud services allows for flexible resource allocation. Organizations can scale up or down based on demand, ensuring that they only pay for what they use.
- Microservices Architecture: Adopting a microservices architecture enables different components of the loan processing system to scale independently. This approach allows for more efficient resource management and faster deployment of new features.
- Load Balancing: Implementing load balancing helps distribute incoming traffic evenly across servers. This ensures that no single server becomes a bottleneck, maintaining optimal performance during peak times.
- Database Scalability: Choosing a database solution that supports horizontal scaling is essential. This allows organizations to add more servers to handle increased data loads without significant downtime.
- Performance Monitoring: Regularly monitoring system performance helps identify potential scalability issues before they impact users. Tools that provide real-time analytics can help organizations make informed decisions about scaling.
- Future-Proofing: Planning for future growth is essential. This includes anticipating changes in technology and user behavior, ensuring that the system can adapt to new demands.
4. Loan Processing Workflow Components
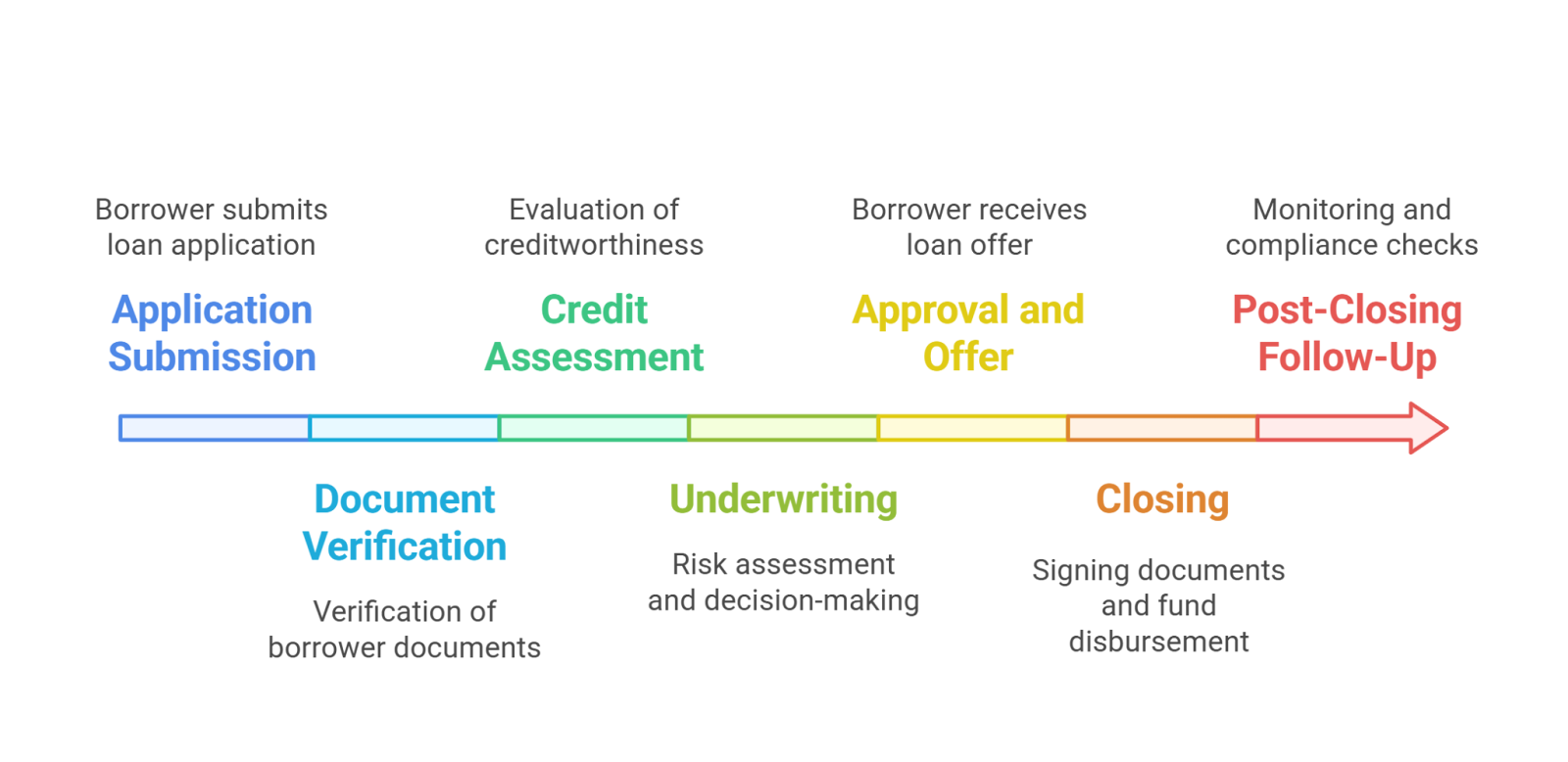
‍
The loan processing workflow consists of several key components that work together to ensure a smooth and efficient process. Understanding these components is vital for optimizing the overall workflow.
- Application Submission: The process begins with the borrower submitting a loan application. This can be done online or in person, and it typically includes personal and financial information.
- Document Verification: Once the application is submitted, the next step involves verifying the documents provided by the borrower. This may include income statements, credit reports, and identification documents.
- Credit Assessment: A thorough credit assessment is conducted to evaluate the borrower's creditworthiness. This involves analyzing credit scores, debt-to-income ratios, and other financial indicators.
- Underwriting: The underwriting process involves assessing the risk associated with the loan. Underwriters review the application and supporting documents to determine whether to approve or deny the loan.
- Approval and Offer: If the loan is approved, the borrower receives a loan offer detailing the terms and conditions. This includes interest rates, repayment schedules, and any fees associated with the loan.
- Closing: The final step in the loan processing workflow is closing the loan. This involves signing the necessary documents and disbursing the funds to the borrower.
- Post-Closing Follow-Up: After the loan is closed, follow-up procedures are essential. This includes monitoring payments, managing customer service inquiries, and ensuring compliance with regulatory requirements.
By understanding and optimizing each component of the loan processing workflow, financial institutions can enhance efficiency, reduce processing times, and improve customer satisfaction. Rapid Innovation is committed to leveraging AI and Blockchain technologies to streamline these processes, ensuring that our clients achieve greater ROI through enhanced security, scalability, and operational efficiency. For more information on our services, visit our AI banking solutions.
4.1. Application Intake and Verification
Application intake and verification is a critical process in various sectors, including finance, healthcare, and education. This stage ensures that all submitted applications are complete, accurate, and meet the necessary criteria for further processing. The efficiency of this process can significantly impact the overall workflow and customer satisfaction. Streamlined processes help reduce the time taken for application approval, while effective verification minimizes the risk of fraud and errors. Additionally, automation tools can enhance accuracy and speed in processing applications, ultimately leading to greater ROI for organizations.
4.1.1. Document Authentication
Document authentication is a vital step in the application intake verification process. It involves verifying the legitimacy of the documents submitted by applicants, which is crucial to ensure that the information provided is accurate and trustworthy. Various methods can be employed for document authentication, including manual verification by trained personnel, the use of advanced technology such as Optical Character Recognition (OCR) to scan and verify documents, and cross-referencing with official databases to confirm the authenticity of documents.
Common documents that require authentication include identification cards (e.g., passports, driver's licenses), financial statements (e.g., bank statements, tax returns), and educational certificates (e.g., diplomas, transcripts). The consequences of inadequate document authentication can be severe, leading to an increased risk of identity theft and fraud, legal repercussions for organizations that fail to verify documents properly, and damage to reputation and loss of customer trust. Rapid Innovation leverages blockchain technology to create immutable records of document verification, enhancing trust and transparency in the process.
4.1.2. Information Extraction
Information extraction is the process of retrieving relevant data from the authenticated documents. This step is essential for transforming unstructured data into structured formats that can be easily analyzed and processed. Key aspects of information extraction include identifying and isolating critical data points, such as names, dates, and financial figures, utilizing machine learning algorithms to improve the accuracy of data extraction over time, and implementing data validation checks to ensure the extracted information is correct.
Techniques used in information extraction can include Natural Language Processing (NLP) to understand and interpret text, data mining to uncover patterns and insights from the extracted information, and integration with databases to store and manage the extracted data efficiently. The benefits of effective information extraction are significant, including enhanced decision-making capabilities through accurate data analysis, improved operational efficiency by reducing manual data entry tasks, and better compliance with regulatory requirements by maintaining accurate records. Rapid Innovation's AI-driven solutions can automate these processes, leading to faster turnaround times and increased productivity.
In conclusion, application intake verification, along with document authentication and information extraction, are essential components of a robust application processing system. By focusing on these areas, organizations can enhance their operational efficiency, reduce risks, and improve customer satisfaction, ultimately achieving greater ROI through the innovative solutions offered by Rapid Innovation.
4.1.3. Fraud Detection
Fraud detection is a critical component of financial services, retail, and online transactions. It involves identifying and preventing fraudulent activities that can lead to significant financial losses. With the rise of digital transactions, the need for robust fraud detection mechanisms, such as insurance fraud detection software and ai fraud detection, has become more pronounced.
- Machine Learning Algorithms: At Rapid Innovation, we leverage advanced machine learning algorithms that analyze transaction patterns and identify anomalies indicative of fraud. These algorithms continuously learn and adapt to new fraud tactics over time, significantly improving their accuracy and effectiveness. Our fraud detection software is designed to integrate seamlessly with existing systems, enhancing overall fraud detection and prevention.
- Real-Time Monitoring: Our solutions include continuous monitoring of transactions, enabling immediate detection of suspicious activities. This includes flagging transactions that deviate from a user's typical behavior, allowing businesses to act swiftly and mitigate potential losses. Payment fraud detection tools are essential in this process, ensuring that fraudulent transactions are identified in real-time.
- Multi-Factor Authentication: We recommend implementing multi-factor authentication as an additional layer of security. This approach helps prevent unauthorized access and reduces the risk of fraud, ensuring that only legitimate users can complete transactions. Fraud prevention solutions are critical in safeguarding sensitive information.
- Data Analytics: Utilizing our data analytics capabilities, businesses can analyze large datasets to uncover trends and patterns that may indicate fraudulent behavior. By examining user behavior, transaction history, and geographic locations, we help clients make informed decisions to enhance their fraud detection strategies. Our fraud analytics software provides insights that are crucial for effective fraud management.
- Collaboration with Financial Institutions: We facilitate collaboration with financial institutions to share information about known fraud patterns and tactics. This collective intelligence enhances the overall effectiveness of fraud detection efforts, providing a more comprehensive defense against fraudulent activities. Our fraud detection services are designed to work in tandem with these institutions to improve outcomes, including the use of AI agents for credit limit recommendations.
4.2. Credit Assessment
Credit assessment is the process of evaluating an individual's or business's creditworthiness. This evaluation helps lenders make informed decisions about extending credit. A thorough credit assessment can mitigate risks and ensure that loans are granted to reliable borrowers.
- Credit History Review: Lenders examine an applicant's credit history, including past loans, payment history, and any defaults. This information is crucial in determining the likelihood of repayment.
- Income Verification: Assessing an applicant's income helps lenders understand their ability to repay the loan, which can include reviewing pay stubs, tax returns, and other financial documents.
- Debt-to-Income Ratio: This ratio compares an applicant's total monthly debt payments to their gross monthly income. A lower ratio indicates a better ability to manage additional debt.
- Employment Stability: A stable employment history can indicate reliability and the potential for consistent income, which is favorable for credit assessment.
- Use of Credit Scoring Models: Credit scoring models provide a standardized way to evaluate creditworthiness based on various factors, making the assessment process more efficient.
4.2.1. Credit Scoring Models
Credit scoring models are statistical tools used to predict the likelihood of a borrower defaulting on a loan. These models analyze various factors to generate a credit score, which lenders use to make lending decisions.
- FICO Score: One of the most widely used credit scoring models, the FICO score ranges from 300 to 850. It considers payment history, amounts owed, length of credit history, new credit, and types of credit used.
- VantageScore: Developed by the three major credit bureaus, VantageScore also ranges from 300 to 850. It uses similar factors as the FICO score but may weigh them differently, providing an alternative assessment of creditworthiness.
- Alternative Data: Some credit scoring models incorporate alternative data, such as utility payments and rental history, to assess individuals with limited credit histories. This can help expand access to credit for underserved populations.
- Machine Learning Models: Advanced credit scoring models utilize machine learning techniques to analyze vast amounts of data, improving predictive accuracy. These models can adapt to changing economic conditions and borrower behaviors.
- Regulatory Compliance: Credit scoring models must comply with regulations such as the Fair Credit Reporting Act (FCRA) to ensure fairness and transparency in lending practices.
By understanding these components of fraud detection, credit assessment, and credit scoring models, businesses can enhance their risk management strategies and improve their lending processes. At Rapid Innovation, we are committed to providing tailored solutions that help our clients achieve greater ROI through effective risk management and credit evaluation strategies, including fraud detection tools and fraud management solutions.
4.2.2. Risk Analysis
Risk analysis is a critical component in various industries, particularly in finance and insurance. It involves identifying, assessing, and prioritizing risks to minimize their impact on an organization. Effective risk analysis can lead to better decision-making and resource allocation.
- Identify potential risks: This includes both internal and external factors that could affect the organization. Common risks include market volatility, regulatory changes, and operational failures.
- Assess the likelihood and impact: Each identified risk should be evaluated based on its probability of occurrence and potential consequences. This helps in prioritizing which risks need immediate attention.
- Develop mitigation strategies: Once risks are assessed, organizations can create strategies to minimize their impact. This may involve diversifying investments, implementing stricter compliance measures, or enhancing operational processes.
- Monitor and review: Risk analysis is not a one-time process. Continuous monitoring and periodic reviews are essential to adapt to changing circumstances and emerging risks.
At Rapid Innovation, we leverage advanced AI algorithms to enhance risk analysis processes, including automated risk assessment tools. By utilizing machine learning models, we can identify patterns and predict potential risks more accurately, allowing organizations to allocate resources effectively and mitigate risks proactively. Our automated risk assessment tools information security ensures that organizations can assess their security posture efficiently.
4.2.3. Alternative Data Processing
Alternative data processing refers to the use of non-traditional data sources to gain insights and make informed decisions. This approach is increasingly popular in sectors like finance, marketing, and insurance, where conventional data may not provide a complete picture.
- Types of alternative data: This can include social media activity, satellite imagery, transaction data, and web scraping. These data sources can reveal consumer behavior and market trends that traditional data might miss.
- Enhanced decision-making: By integrating alternative data, organizations can improve their predictive analytics, leading to better risk assessment and customer targeting.
- Challenges in processing: While alternative data offers valuable insights, it also presents challenges such as data quality, privacy concerns, and the need for advanced analytical tools.
- Regulatory considerations: Organizations must navigate the legal landscape surrounding data usage, ensuring compliance with data protection regulations.
Rapid Innovation assists clients in harnessing alternative data through our AI-driven analytics platforms. By processing and analyzing diverse data sets, we empower organizations to make data-driven decisions that enhance their competitive edge, including tools to automate risk assessment.
4.3. Underwriting Automation
Underwriting automation streamlines the process of evaluating risk and determining insurance premiums. By leveraging technology, organizations can enhance efficiency, reduce costs, and improve accuracy in underwriting decisions.
- Speed and efficiency: Automation significantly reduces the time required to process applications. This allows insurers to respond to customers more quickly, improving customer satisfaction.
- Data-driven decisions: Automated underwriting systems utilize algorithms and machine learning to analyze vast amounts of data, leading to more accurate risk assessments.
- Cost reduction: By minimizing manual intervention, organizations can lower operational costs associated with underwriting. This can lead to more competitive pricing for consumers.
- Enhanced accuracy: Automation reduces human error, ensuring that underwriting decisions are based on consistent criteria and comprehensive data analysis.
- Scalability: Automated systems can easily scale to handle increased application volumes, making them ideal for growing businesses.
At Rapid Innovation, we implement blockchain technology to enhance the transparency and security of underwriting processes. By creating immutable records of transactions and decisions, we help organizations build trust with their clients while streamlining operations.
In conclusion, risk analysis, alternative data processing, and underwriting automation are interconnected elements that can significantly enhance an organization's operational efficiency and decision-making capabilities. By embracing these practices, including automating security risk assessments and utilizing risk analysis and automation, businesses can better navigate the complexities of their respective industries, ultimately achieving greater ROI with the support of Rapid Innovation's expertise in AI and blockchain solutions.
4.4. Approval and Decision Making
In the loan processing landscape, approval and decision-making are critical stages that determine whether a loan application is accepted or rejected. This process has evolved significantly with the integration of technology, particularly artificial intelligence (AI) in loan processing. AI algorithms analyze vast amounts of data to assess creditworthiness, while machine learning models can predict the likelihood of default based on historical data. Automated systems streamline the decision-making process, reducing the time taken to approve loans.
The traditional method of manual underwriting is often slow and prone to human error. AI enhances this by providing faster processing times, often reducing approval times from days to minutes. It also ensures consistency in decision-making, minimizing biases that can occur with human evaluators, and enhances risk assessment through predictive analytics, allowing lenders to make informed decisions.
Moreover, AI can help in identifying potential fraud by flagging unusual patterns in applications. This not only protects lenders but also ensures that genuine applicants are not unfairly denied loans. The use of AI in approval and decision-making is transforming the lending landscape, making it more efficient and reliable.
4.5. Loan Servicing and Monitoring
Loan servicing and monitoring are essential components of the loan lifecycle, ensuring that loans are managed effectively after approval. This stage involves ongoing communication with borrowers and the management of loan repayments. AI-driven systems can automate payment reminders, reducing the risk of late payments. Predictive analytics can identify borrowers who may be at risk of default, allowing lenders to intervene early. Additionally, chatbots and virtual assistants provide 24/7 support to borrowers, answering queries and assisting with payment processes.
Effective loan servicing is crucial for maintaining a healthy loan portfolio. Key aspects include regular monitoring of borrower behavior to ensure compliance with loan terms, adjusting repayment plans based on the borrower’s financial situation to improve repayment rates, and utilizing data analytics to assess the performance of loans and make necessary adjustments to lending strategies.
By leveraging technology in loan servicing and monitoring, lenders can enhance customer satisfaction and reduce operational costs. This proactive approach not only benefits the lender but also supports borrowers in managing their loans effectively.
5. Types of AI Agents in Loan Processing

‍
The integration of AI in loan processing has led to the development of various AI agents that streamline operations and enhance decision-making. These agents can be categorized based on their functions and capabilities.
- Chatbots: These AI agents provide instant support to borrowers, answering questions about loan products, application processes, and repayment options. They can handle multiple inquiries simultaneously, improving customer service efficiency.
- Predictive Analytics Agents: These agents analyze historical data to forecast borrower behavior and assess credit risk. By identifying patterns, they help lenders make informed decisions regarding loan approvals and terms.
- Fraud Detection Agents: Utilizing machine learning algorithms, these agents monitor transactions and application data to identify potential fraudulent activities. They flag suspicious behavior, allowing lenders to take preventive measures.
- Document Processing Agents: These AI systems automate the extraction and verification of information from loan applications and supporting documents. This reduces manual effort and speeds up the processing time.
- Risk Assessment Agents: These agents evaluate the risk associated with lending to specific borrowers by analyzing various factors, including credit scores, income levels, and economic conditions. They provide insights that help lenders tailor their offerings.
- Loan Management Agents: These AI tools assist in managing the loan lifecycle, from disbursement to repayment. They track payment schedules, send reminders, and provide insights into borrower behavior.
The use of these AI agents in loan processing not only enhances efficiency but also improves the overall customer experience. By automating routine tasks and providing valuable insights, lenders can focus on strategic decision-making and relationship building with borrowers.
At Rapid Innovation, we specialize in implementing these AI-driven solutions to help our clients achieve greater ROI by optimizing their loan processing operations. Our expertise in AI and blockchain technology ensures that your lending processes are not only efficient but also secure and scalable, allowing you to meet your business goals effectively.
5.1. Document Processing Agents
Document processing agents are specialized software tools designed to automate the handling and management of documents. These agents utilize advanced technologies such as Optical Character Recognition (OCR), Natural Language Processing (NLP), and machine learning to streamline the processing of various types of documents.
- Automate data extraction from documents like invoices, contracts, and forms. Â
- Enhance accuracy by reducing human error in data entry. Â
- Improve efficiency by processing large volumes of documents quickly. Â
- Enable easy categorization and indexing of documents for better retrieval. Â
- Support compliance by ensuring that documents are processed according to regulatory standards. Â
These document processing agents are particularly beneficial in industries such as finance, healthcare, and legal, where document management is critical. By implementing document processing agents, organizations can significantly reduce operational costs and improve productivity. At Rapid Innovation, we leverage our expertise in AI to develop tailored document processing solutions that align with your business objectives, ultimately driving greater ROI. For more information on how AI can enhance credit scoring and its various use cases.
5.2. Credit Analysis Agents
Credit analysis agents are tools that assist financial institutions in evaluating the creditworthiness of individuals or businesses. These agents analyze various data points to provide insights into the likelihood of default on loans or credit obligations.
- Assess credit scores and histories to determine risk levels. Â
- Analyze financial statements and cash flow to evaluate business health. Â
- Utilize predictive analytics to forecast future credit behavior. Â
- Integrate with external databases for comprehensive credit assessments. Â
- Generate reports that help lenders make informed decisions. Â
The use of credit analysis agents can lead to more accurate lending decisions, reduced default rates, and improved customer satisfaction. By leveraging these credit analysis agents, financial institutions can enhance their risk management strategies and streamline the credit approval process. Rapid Innovation's expertise in AI allows us to create sophisticated credit analysis solutions that not only improve decision-making but also enhance profitability.
5.3. Risk Assessment Agents
Risk assessment agents are tools designed to identify, evaluate, and prioritize risks associated with various business activities. These agents employ quantitative and qualitative methods to assess potential risks and their impacts on an organization.
- Identify potential risks in areas such as finance, operations, and compliance. Â
- Evaluate the likelihood and impact of identified risks. Â
- Prioritize risks based on their severity and potential consequences. Â
- Provide recommendations for risk mitigation strategies. Â
- Monitor ongoing risks and adjust assessments as necessary. Â
Organizations that utilize risk assessment agents can enhance their decision-making processes and improve overall risk management. By proactively identifying and addressing risks, businesses can safeguard their assets and ensure long-term sustainability. At Rapid Innovation, we harness the power of AI and blockchain technology to develop robust risk assessment solutions that empower organizations to navigate uncertainties effectively, thereby maximizing their return on investment.
5.4. Customer Service Agents
Customer service agents play a crucial role in maintaining customer satisfaction and loyalty. They are the frontline representatives of a company, responsible for addressing customer inquiries, resolving issues, and providing information about products and services.
- Key Responsibilities: Â
- Responding to customer queries via phone, email, or chat.
- Assisting customers with product selection and troubleshooting.
- Handling complaints and providing solutions to enhance customer experience.
- Documenting customer interactions and feedback for future reference.
- Skills Required: Â
- Excellent communication skills to convey information clearly.
- Strong problem-solving abilities to address customer concerns effectively.
- Empathy and patience to understand customer needs and frustrations.
- Familiarity with customer relationship management (CRM) software.
- Importance in Business: Â
- Customer service agents directly impact customer retention rates.
- They gather valuable feedback that can inform product development and service improvements.
- A positive customer service experience can lead to word-of-mouth referrals and increased sales.
At Rapid Innovation, we leverage AI-driven chatbots and customer service automation tools to enhance the efficiency of customer service agents. By integrating AI solutions, we enable businesses to provide 24/7 support, reduce response times, and improve overall customer satisfaction, ultimately leading to a greater return on investment (ROI). For more insights on the role of AI in enhancing customer service, you can read about the advantages and future of generative AI in finance and banking applications.
For those interested in pursuing a career in this field, there are various customer care service jobs available, including customer service representative roles. Job seekers can explore customer care jobs near me or look for customer service representative jobs that offer remote opportunities. Additionally, positions such as live chat agent jobs are becoming increasingly popular, allowing for flexible work arrangements.
5.5. Compliance Monitoring Agents
Compliance monitoring agents are essential for ensuring that a company adheres to legal standards and internal policies. They help mitigate risks associated with non-compliance, which can lead to financial penalties and reputational damage.
- Key Responsibilities: Â
- Monitoring business operations to ensure compliance with regulations.
- Conducting audits and assessments to identify potential compliance issues.
- Developing and implementing compliance training programs for employees.
- Reporting findings to management and recommending corrective actions.
- Skills Required: Â
- Strong analytical skills to assess compliance risks and issues.
- Attention to detail to identify discrepancies in processes and documentation.
- Knowledge of relevant laws and regulations applicable to the industry.
- Ability to communicate compliance requirements effectively to staff.
- Importance in Business: Â
- Compliance monitoring agents help protect the organization from legal repercussions.
- They foster a culture of accountability and ethical behavior within the company.
- Effective compliance monitoring can enhance a company's reputation and build trust with stakeholders.
Rapid Innovation employs blockchain technology to create transparent and immutable records of compliance activities. This not only streamlines the compliance process but also enhances accountability, thereby reducing the risk of non-compliance and associated penalties.
5.6. Fraud Detection Agents
Fraud detection agents are tasked with identifying and preventing fraudulent activities within an organization. Their role is critical in safeguarding company assets and maintaining customer trust.
- Key Responsibilities: Â
- Analyzing transactions and customer behavior to detect suspicious activities.
- Investigating potential fraud cases and gathering evidence.
- Collaborating with law enforcement and regulatory agencies when necessary.
- Implementing fraud prevention strategies and technologies.
- Skills Required: Â
- Strong analytical and critical thinking skills to assess risk factors.
- Familiarity with fraud detection software and tools.
- Excellent attention to detail to spot anomalies in data.
- Effective communication skills to report findings and collaborate with teams.
- Importance in Business: Â
- Fraud detection agents help minimize financial losses due to fraudulent activities.
- They protect the company’s reputation by ensuring a secure environment for customers.
- Their work contributes to the overall integrity of the financial system, fostering trust among consumers.
At Rapid Innovation, we utilize advanced AI algorithms and machine learning techniques to enhance fraud detection capabilities. By analyzing vast amounts of transaction data in real-time, we empower organizations to identify and mitigate fraudulent activities swiftly, thereby safeguarding their assets and enhancing customer trust. This proactive approach not only reduces financial losses but also contributes to a stronger bottom line.
6. Implementation Strategy
An effective implementation strategy is crucial for the successful execution of any project or initiative. It outlines the steps necessary to achieve the desired outcomes while ensuring that resources are utilized efficiently. A well-structured implementation strategy includes assessment and planning, which are foundational to understanding the current state and determining the best path forward.
6.1 Assessment and Planning
Assessment and planning are the first steps in the implementation strategy. This phase involves evaluating the existing processes, identifying gaps, and setting clear objectives. It is essential to establish clear goals and objectives for the implementation, identify stakeholders and their roles in the process, gather data to understand the current state of operations, analyze potential risks and challenges that may arise during implementation, and develop a timeline for the implementation process. This phase is crucial for ensuring that the implementation aligns with the overall goals of the organization, helps in allocating resources effectively, and sets realistic expectations.
6.1.1 Current Process Analysis
Current process analysis is a critical component of the assessment phase. It involves a thorough examination of existing workflows, systems, and practices to identify inefficiencies and areas for improvement. The analysis includes the following steps:
- Data Collection: Gather quantitative and qualitative data on current processes, which may include performance metrics, employee feedback, and customer satisfaction surveys.
- Process Mapping: Create visual representations of current workflows to identify bottlenecks and redundancies, helping to understand how tasks are currently performed and where improvements can be made.
- Stakeholder Interviews: Conduct interviews with employees and stakeholders to gain insights into their experiences with current processes, revealing pain points and areas that require immediate attention.
- Benchmarking: Compare current processes against industry standards or best practices to identify gaps and set performance targets.
- SWOT Analysis: Perform a SWOT analysis (Strengths, Weaknesses, Opportunities, Threats) to evaluate the current processes, providing a comprehensive view of internal and external factors affecting performance.
By conducting a thorough current process analysis, organizations can make informed decisions about the changes needed to enhance efficiency and effectiveness. This analysis serves as a foundation for developing targeted strategies that address specific issues and align with the overall goals of the organization. At Rapid Innovation, we leverage our expertise in AI and Blockchain to provide actionable insights and innovative solutions that drive greater ROI for our clients, ensuring that their implementation strategies are not only effective but also transformative. This includes developing a strategy implementation plan and utilizing change implementation strategies to facilitate smooth transitions. Additionally, we focus on strategy formulation and implementation to ensure that our clients have a comprehensive approach to their projects, including AI in anomaly detection for businesses.
6.1.2. Gap Identification
Gap identification is a crucial step in any project or strategic planning process. It involves recognizing the discrepancies between the current state and the desired state of a project, organization, or system. This curriculum gap identification process helps in pinpointing areas that require improvement or additional resources.
- Assess current performance metrics to establish a baseline.
- Compare these metrics against industry standards or best practices.
- Identify specific areas where performance falls short, such as:
- Operational inefficiencies
- Skill shortages within the team
- Technology limitations
- Utilize tools like SWOT analysis (Strengths, Weaknesses, Opportunities, Threats) to gain a comprehensive view of the gaps.
- Engage stakeholders to gather insights and perspectives on perceived gaps.
- Document findings to create a clear action plan for addressing identified gaps.
By systematically identifying gaps, organizations can prioritize their efforts and allocate resources effectively to bridge these gaps, ultimately leading to improved performance and outcomes. At Rapid Innovation, we leverage our expertise in AI and Blockchain to help clients identify these gaps and implement tailored solutions that enhance operational efficiency and drive greater ROI.
6.1.3. Resource Requirements
Understanding resource requirements is essential for successful project execution. This involves determining the necessary resources—human, financial, and technological—to achieve project goals.
- Identify the types of resources needed, including:
- Personnel with specific skills and expertise
- Financial resources for budgeting and funding
- Technological tools and software
- Assess the availability of current resources and identify any shortfalls.
- Create a detailed resource allocation plan that includes:
- Roles and responsibilities of team members
- Budget estimates for each phase of the project
- Timeline for resource acquisition and deployment
- Consider potential risks associated with resource shortages and develop contingency plans.
- Regularly review and adjust resource requirements as the project progresses to ensure alignment with goals.
By clearly defining resource requirements, organizations can enhance their ability to meet project objectives and minimize delays or budget overruns. Rapid Innovation assists clients in optimizing their resource allocation through advanced analytics and strategic planning, ensuring that every project is equipped for success.
6.2. Data Preparation
Data preparation is a fundamental step in data analysis and decision-making processes. It involves cleaning, organizing, and transforming raw data into a usable format for analysis.
- Begin with data collection from various sources, ensuring data quality and relevance.
- Clean the data by:
- Removing duplicates
- Correcting errors or inconsistencies
- Handling missing values appropriately
- Organize the data into a structured format, such as:
- Spreadsheets
- Databases
- Data warehouses
- Transform the data to meet analysis requirements, which may include:
- Normalization or standardization
- Aggregation of data points
- Creating new calculated fields
- Document the data preparation process to ensure transparency and reproducibility.
- Validate the prepared data to confirm its accuracy and readiness for analysis.
Effective data preparation is vital for ensuring that the analysis yields reliable and actionable insights, ultimately supporting informed decision-making. At Rapid Innovation, we employ cutting-edge AI techniques to streamline data preparation processes, enabling our clients to derive meaningful insights that drive strategic initiatives and enhance overall business performance.
6.3. Model Training and Validation
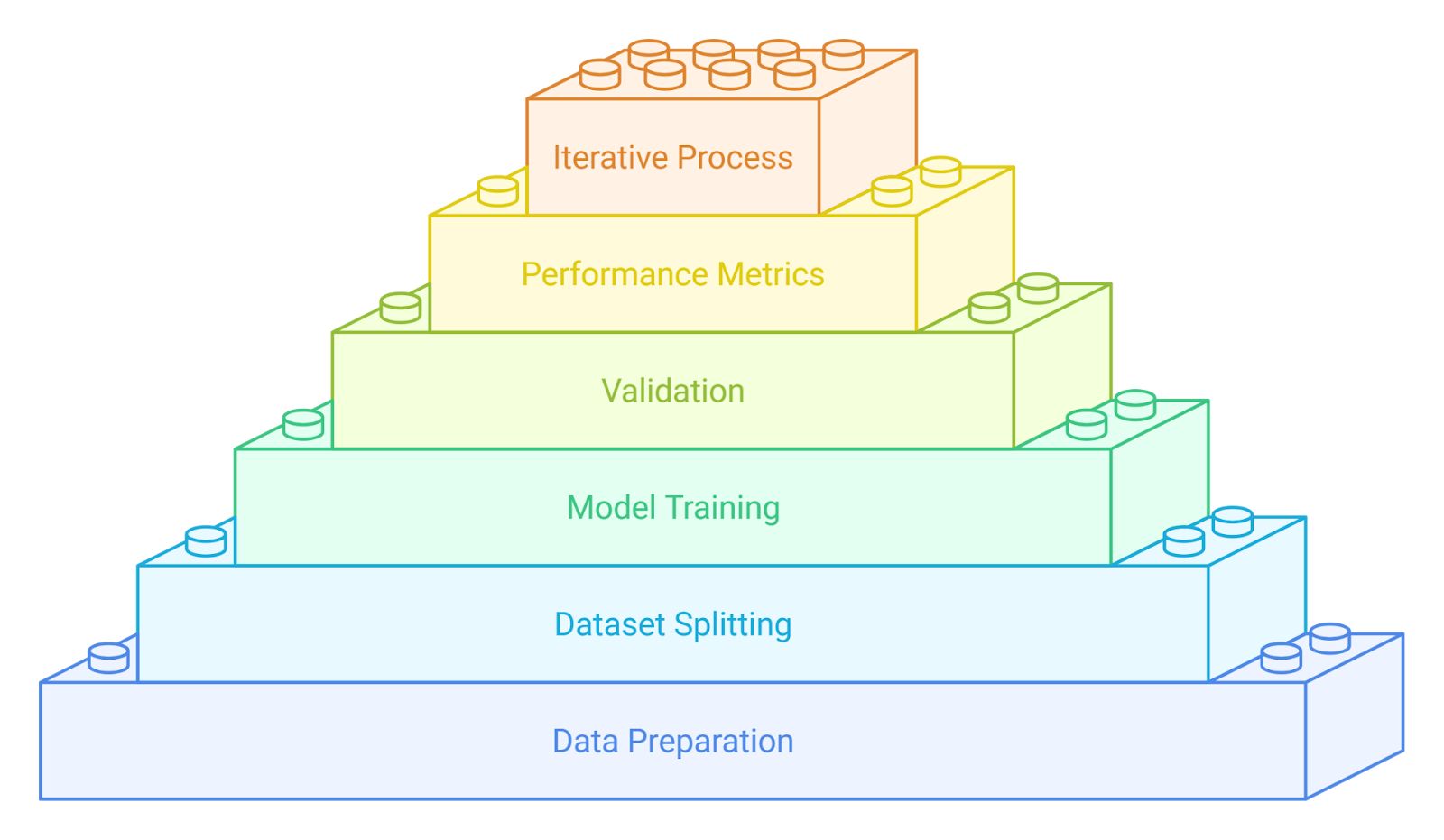
‍
Model training and validation are critical steps in the machine learning lifecycle. This phase involves teaching the model to recognize patterns in data and ensuring that it performs well on unseen data.
- Data Preparation: Before training, data must be cleaned and preprocessed. This includes handling missing values, normalizing data, and encoding categorical variables. At Rapid Innovation, we emphasize the importance of high-quality data preparation to enhance model accuracy and reliability.
- Splitting the Dataset: The dataset is typically divided into training, validation, and test sets. A common split is 70% for training, 15% for validation, and 15% for testing. This ensures that the model is trained on one subset and validated on another to avoid overfitting. Our expertise in data management ensures that this process is executed efficiently, maximizing the model's potential. This includes approaches like training set validation set and test set, as well as holdout dataset strategies.
- Training the Model: During training, the model learns from the training data by adjusting its parameters to minimize the error. Various algorithms can be used, such as linear regression, decision trees, or neural networks. Rapid Innovation leverages advanced algorithms tailored to specific business needs, ensuring optimal performance and ROI. This phase often involves training test validation set methodologies to ensure comprehensive learning.
- Validation: After training, the model is validated using the validation set. This step helps in tuning hyperparameters and selecting the best model. Techniques like k-fold cross-validation can be employed to ensure robustness. Our team utilizes these techniques to refine models, ensuring they are well-suited for real-world applications. This includes the use of training validation and test sets to ensure thorough evaluation.
- Performance Metrics: Metrics such as accuracy, precision, recall, and F1 score are used to evaluate the model's performance. These metrics help in understanding how well the model is likely to perform on unseen data. We provide comprehensive performance analysis to our clients, enabling informed decision-making. This analysis is crucial in machine learning train test validation scenarios.
- Iterative Process: Model training and validation are iterative. Based on validation results, adjustments may be made to the model architecture or training process to improve performance. Rapid Innovation's iterative approach ensures continuous improvement, leading to enhanced model effectiveness. This process often involves training and validation sets to refine the model further.
6.4. Deployment Phases
Deployment is the process of making a trained model available for use in a production environment. This phase is crucial for ensuring that the model can deliver value in real-world applications.
- Model Packaging: The trained model is packaged along with its dependencies. This can include libraries, configuration files, and any necessary scripts to ensure it runs smoothly in the target environment.
- Environment Setup: A suitable environment must be established for the model to run. This could be on-premises servers, cloud platforms, or edge devices, depending on the application requirements. Rapid Innovation assists clients in selecting the most appropriate deployment environment to optimize performance and cost.
- Integration: The model needs to be integrated into existing systems or applications. This may involve creating APIs or embedding the model within software applications to facilitate interaction. Our integration services ensure seamless functionality, enhancing user experience.
- Testing: Before full deployment, the model should be tested in a staging environment. This helps identify any issues that may arise when the model interacts with real-world data.
- Rollout Strategy: A rollout strategy should be defined, which could include phased deployment, canary releases, or blue-green deployments. This minimizes risks by allowing for gradual exposure of the model to users.
- User Training: If necessary, users should be trained on how to interact with the model. This ensures that they understand its capabilities and limitations.
6.5. Performance Monitoring
Performance monitoring is essential to ensure that the deployed model continues to perform well over time. This phase involves tracking the model's performance and making adjustments as needed.
- Real-Time Monitoring: Implementing real-time monitoring allows for immediate detection of performance issues. Metrics such as response time, throughput, and error rates should be tracked continuously. Rapid Innovation provides robust monitoring solutions to ensure optimal model performance.
- Drift Detection: Over time, the data the model encounters may change, leading to concept drift. Monitoring for drift helps identify when the model's performance begins to degrade due to changes in the underlying data distribution.
- Periodic Evaluation: Regularly evaluating the model against the test set or a new validation set is crucial. This helps in assessing whether the model still meets performance expectations.
- Feedback Loops: Establishing feedback loops allows for continuous improvement. User feedback and performance data can be used to retrain the model or adjust its parameters.
- Alerts and Notifications: Setting up alerts for significant performance drops or anomalies ensures that issues are addressed promptly. This can involve automated notifications to the development team.
- Documentation: Keeping detailed records of model performance, changes made, and the rationale behind those changes is important for transparency and future reference.
By focusing on these aspects of model training, deployment, and performance monitoring, organizations can ensure that their machine learning models deliver consistent and reliable results. Rapid Innovation is committed to helping clients achieve their business goals efficiently and effectively through our comprehensive AI and Blockchain solutions.
6.6. Continuous Improvement
Continuous improvement is a systematic, ongoing effort to enhance products, services, or processes. It is a fundamental aspect of quality management and is essential for organizations aiming to remain competitive in today's fast-paced market.
- Focus on incremental changes rather than radical shifts.
- Utilize methodologies such as Plan-Do-Check-Act (PDCA) or Six Sigma to guide improvement efforts.
- Encourage a culture of feedback where employees at all levels can contribute ideas for improvement.
- Regularly assess performance metrics to identify areas needing enhancement.
- Implement training programs to equip employees with skills necessary for improvement initiatives.
- Foster collaboration across departments to share best practices and innovative solutions.
- Use customer feedback to drive improvements in service delivery and product quality.
- Document changes and their impacts to create a knowledge base for future reference.
At Rapid Innovation, we leverage AI and Blockchain technologies to facilitate continuous improvement. For instance, AI-driven analytics can provide insights into operational inefficiencies, allowing organizations to make data-informed decisions that enhance productivity. Additionally, Blockchain can ensure transparency and traceability in processes, enabling organizations to identify areas for improvement more effectively. You can learn more about how AI and machine learning contribute to regulatory compliance.
Continuous improvement not only enhances operational efficiency but also boosts employee morale and customer satisfaction. Organizations that embrace this philosophy are better positioned to adapt to changes in the market and meet evolving customer needs.
7. Regulatory Compliance and Risk Management
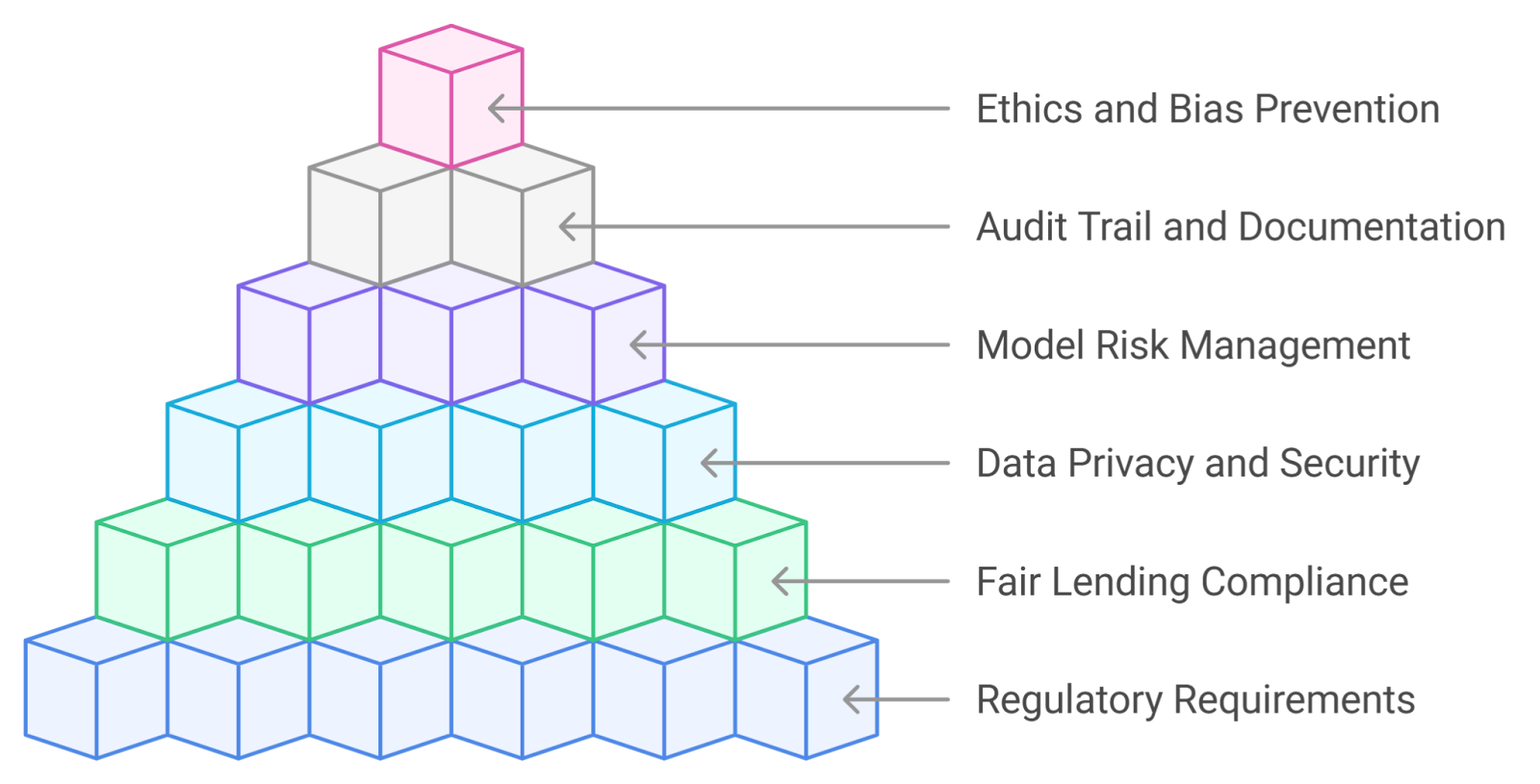
‍
Regulatory compliance and risk management are critical components of any organization’s operational framework. They ensure that businesses adhere to laws and regulations while effectively managing potential risks that could impact their operations.
- Regulatory compliance involves understanding and adhering to laws, regulations, and guidelines relevant to the industry, including managing regulatory change and ensuring regulatory compliance and risk management.
- Risk management focuses on identifying, assessing, and mitigating risks that could hinder an organization’s objectives, including regulatory compliance risk and regulatory risk management.
- Both elements are interconnected; non-compliance can lead to significant risks, including financial penalties and reputational damage.
Organizations must develop robust compliance programs that include:
- Regular training for employees on compliance requirements and ethical standards, including legal compliance risk management.
- Establishing clear policies and procedures to guide compliance efforts, particularly in areas like governance risk and compliance regulations.
- Conducting regular audits to assess compliance status and identify areas for improvement, such as regulatory compliance risk examples.
- Engaging with legal and regulatory experts to stay updated on changes in laws and regulations, including those related to governance risk & regulation.
Effective risk management strategies include:
- Conducting risk assessments to identify potential threats and vulnerabilities, including those related to regulatory compliance and risk management in appraisal.
- Developing a risk management plan that outlines strategies for mitigating identified risks, such as legal compliance and risk management.
- Implementing monitoring systems to track risk factors and compliance status, including grc regulatory compliance.
- Creating a crisis management plan to address potential incidents swiftly and effectively.
7.1. Regulatory Requirements
Regulatory requirements vary by industry and region, but they generally encompass a range of laws and standards that organizations must follow to operate legally and ethically. Understanding these requirements is crucial for maintaining compliance and avoiding legal repercussions.
- Organizations must identify applicable regulations, which may include: Â
- Environmental regulations
- Health and safety standards
- Financial reporting requirements
- Data protection laws (e.g., GDPR, HIPAA)
- Key steps to ensure compliance with regulatory requirements include: Â
- Conducting a thorough analysis of relevant regulations affecting the organization, including bank regulation risk management and compliance.
- Developing a compliance framework that outlines roles, responsibilities, and processes.
- Regularly reviewing and updating compliance policies to reflect changes in regulations, including legal compliance risk.
- Engaging with regulatory bodies to clarify requirements and seek guidance when needed.
- Non-compliance can lead to: Â
- Legal penalties, including fines and sanctions.
- Loss of business licenses or permits.
- Damage to reputation and loss of customer trust.
By prioritizing regulatory compliance, organizations can not only avoid legal issues but also enhance their credibility and foster a culture of integrity within the workplace. Rapid Innovation is committed to helping clients navigate these complexities through tailored solutions that integrate AI and Blockchain technologies, ensuring compliance while optimizing operational efficiency.
7.2. Fair Lending Compliance
Fair lending compliance is essential for financial institutions to ensure that they provide equal access to credit and financial services without discrimination. This compliance is governed by various laws and regulations, including the Equal Credit Opportunity Act (ECOA) and the Fair Housing Act (FHA). Financial institutions must regularly review their lending practices to ensure they do not discriminate based on race, color, religion, national origin, sex, marital status, age, or because a person receives public assistance. Institutions should implement robust training programs for employees to understand fair lending laws and the importance of compliance. Regular audits and assessments can help identify potential discriminatory practices and rectify them before they lead to legal issues. Data collection and analysis are crucial for monitoring lending patterns and ensuring compliance with fair lending regulations. Additionally, institutions should have a clear complaint resolution process for customers who believe they have been discriminated against in lending practices.
- Regular reviews of lending practices to prevent discrimination.
- Robust training programs for employees on fair lending laws.
- Regular audits and assessments to identify and rectify discriminatory practices.
- Data collection and analysis for monitoring compliance with fair lending regulations.
- Clear complaint resolution process for customers alleging discrimination.
At Rapid Innovation, we leverage AI-driven analytics to enhance data collection and analysis, enabling financial institutions to identify and address potential biases in their lending practices. Our solutions can automate the review process, ensuring compliance with fair lending regulations while minimizing the risk of human error.
7.3. Data Privacy and Security
Data privacy and security are critical components of modern financial services, especially with the increasing reliance on technology and digital transactions. Financial institutions must protect sensitive customer information to maintain trust and comply with regulations such as the Gramm-Leach-Bliley Act (GLBA) and the General Data Protection Regulation (GDPR). Institutions should implement strong encryption methods to protect data both in transit and at rest. Regular security assessments and penetration testing can help identify vulnerabilities in systems and processes. Employee training on data privacy policies and security best practices is essential to minimize human error, which is often a significant risk factor. Establishing a clear data breach response plan can help institutions respond quickly and effectively in the event of a data breach. Compliance with data privacy regulations not only protects customers but also enhances the institution's reputation and trustworthiness.
- Strong encryption methods to protect data in transit and at rest.
- Regular security assessments and penetration testing to identify vulnerabilities.
- Employee training on data privacy policies and security best practices.
- Clear data breach response plan for quick and effective responses.
- Compliance with data privacy regulations to protect customers and enhance reputation.
Rapid Innovation employs blockchain technology to enhance data security and privacy. By utilizing decentralized ledgers, we ensure that sensitive customer information is securely stored and accessed, significantly reducing the risk of data breaches and enhancing compliance with privacy regulations.
7.4. Model Risk Management
Model risk management is a critical aspect of financial institutions' operations, particularly in the context of risk assessment and decision-making. Models are used for various purposes, including credit risk assessment, market risk analysis, and operational risk management. Institutions should establish a comprehensive model risk management framework that includes model development, validation, and monitoring processes. Regular validation of models is necessary to ensure their accuracy and reliability, especially in changing market conditions. Documentation of model assumptions, methodologies, and limitations is crucial for transparency and regulatory compliance. Institutions should have a governance structure in place to oversee model risk management activities, ensuring accountability and adherence to best practices. Continuous training and development for staff involved in model risk management can enhance the institution's ability to manage model-related risks effectively.
- Comprehensive model risk management framework including development, validation, and monitoring.
- Regular validation of models to ensure accuracy and reliability.
- Documentation of model assumptions, methodologies, and limitations for transparency.
- Governance structure to oversee model risk management activities.
- Continuous training and development for staff involved in model risk management.
At Rapid Innovation, we integrate AI technologies to enhance model validation processes, ensuring that financial institutions can adapt to changing market conditions with confidence. Our solutions provide real-time insights and predictive analytics, enabling better decision-making and risk management.
Fair Lending Risk Assessment
In addition to the above practices, conducting a fair lending risk assessment is vital for identifying potential areas of concern within lending operations. This assessment helps institutions proactively address any compliance gaps and mitigate risks associated with fair lending violations.
Fair Lending Compliance Program
To ensure ongoing adherence to fair lending laws, institutions should develop a comprehensive fair lending compliance program. This program should include policies, procedures, and monitoring mechanisms to promote fair lending practices and ensure compliance with the Fair Lending Act.
7.5. Audit Trail and Documentation
An audit trail is a crucial component in any system that involves data handling, especially in fields like finance, healthcare, and technology. It refers to the chronological record of all activities and transactions that have occurred within a system. Proper documentation and audit trails ensure accountability, transparency, and traceability.
- Importance of Audit Trails: Â
- Provides a clear history of data access and modifications.
- Helps in identifying unauthorized access or data breaches.
- Facilitates compliance with regulatory requirements, such as GDPR or HIPAA.
- Key Elements of Effective Documentation: Â
- Detailed logs of user activities, including timestamps and actions taken.
- Clear version control for documents and data sets.
- Regular reviews and updates to ensure accuracy and relevance.
- Best Practices: Â
- Implement automated logging systems to reduce human error.
- Ensure logs are stored securely and are tamper-proof.
- Regularly audit the logs to identify any anomalies or suspicious activities, such as through audit trail reports or laserfiche audit trail systems.
In addition, maintaining comprehensive audit trail documentation is essential. This includes creating an audit trail example pdf for reference and ensuring that all audit trail files collected are organized and accessible. For specific systems, such as bill com audit trail or check audit trail oracle, tailored documentation practices should be followed. Salesforce field audit trail implementation guide can also provide valuable insights into effective practices. For more information on best practices regarding AI and data privacy.
7.6. Ethics and Bias Prevention
Ethics and bias prevention are critical in any data-driven environment, particularly in artificial intelligence and machine learning. Ethical considerations ensure that systems are designed and operated in a manner that respects user rights and promotes fairness.
- Understanding Bias: Â
- Bias can occur at various stages, including data collection, algorithm design, and output interpretation. It can lead to unfair treatment of individuals or groups, particularly marginalized communities.
- Strategies for Bias Prevention: Â
- Diverse Data Sets: Use diverse and representative data to train algorithms, minimizing the risk of bias.
- Regular Audits: Conduct regular audits of algorithms to identify and mitigate bias.
- Ethical Guidelines: Establish clear ethical guidelines for data usage and algorithm development.
- Importance of Ethics: Â
- Builds trust with users and stakeholders.
- Enhances the credibility of the organization.
- Reduces the risk of legal repercussions and reputational damage.
8. Performance Metrics and Optimization

‍
Performance metrics are essential for evaluating the effectiveness of systems and processes. They provide quantifiable measures that help organizations understand how well they are achieving their goals.
- Key Performance Metrics: Â
- Accuracy: Measures how often the system produces correct results.
- Efficiency: Assesses the speed and resource usage of the system.
- User Satisfaction: Gauges user experience and satisfaction levels.
- Optimization Techniques: Â
- Continuous Monitoring: Regularly track performance metrics to identify areas for improvement.
- A/B Testing: Experiment with different approaches to determine which yields better results.
- Feedback Loops: Incorporate user feedback to refine and enhance system performance.
- Benefits of Optimization: Â
- Improved user experience and satisfaction.
- Increased operational efficiency and reduced costs.
- Enhanced decision-making capabilities based on data-driven insights.
At Rapid Innovation, we leverage our expertise in AI and Blockchain to implement robust audit trails and ethical frameworks that not only enhance compliance but also build trust with your stakeholders. By integrating advanced performance metrics and optimization techniques, we help you achieve greater ROI and operational excellence. Our tailored solutions ensure that your systems are not only efficient but also fair and transparent, aligning with your business goals.
8.1. Key Performance Indicators
Key Performance Indicators (KPIs) are essential metrics that help organizations measure their success in achieving specific objectives. They provide a clear framework for assessing performance and making informed decisions.
- KPIs should be specific, measurable, achievable, relevant, and time-bound (SMART).
- Common KPIs include: Â
- Revenue growth rate
- Customer acquisition cost
- Net promoter score (NPS)
- KPIs can vary by industry, so it’s crucial to select those that align with your business goals. At Rapid Innovation, we leverage AI analytics to tailor KPIs that resonate with your unique business landscape, ensuring that you focus on metrics that drive growth.
- Regularly reviewing KPIs allows organizations to identify trends, areas for improvement, and opportunities for growth. Our blockchain solutions can provide immutable records of KPI performance, enhancing transparency and trust in your data.
- Effective communication of KPIs across teams fosters accountability and encourages a performance-driven culture. We assist in establishing dashboards that integrate AI insights, making KPI tracking intuitive and accessible for all stakeholders.
- Defining KPIs is crucial for understanding their meaning and impact. Key performance indicators examples can include metrics like customer satisfaction scores and employee productivity rates.
- Understanding what a KPI is and how it functions within your organization can lead to better strategic decisions. Key point indicators can help in pinpointing specific areas that require attention.
8.2. Quality Assurance Measures
Quality assurance (QA) measures are systematic processes designed to ensure that products or services meet specified requirements and standards. Implementing robust QA measures is vital for maintaining customer satisfaction and operational efficiency.
- QA measures typically include: Â
- Regular audits and inspections
- Testing and validation processes
- Employee training and development programs
- Establishing clear quality standards helps in setting expectations for performance. Rapid Innovation employs AI-driven testing frameworks that enhance the accuracy and speed of quality assessments.
- Continuous feedback loops from customers can enhance quality assurance efforts. Our blockchain technology can facilitate secure and transparent feedback mechanisms, ensuring that customer insights are effectively integrated into your QA processes.
- Utilizing technology, such as automated testing tools, can streamline QA processes and reduce human error. We provide tailored automation solutions that leverage AI to optimize your QA workflows.
- A strong QA culture promotes a proactive approach to identifying and resolving issues before they escalate. Our consulting services can help instill a quality-first mindset across your organization.
8.3. Efficiency Metrics
Efficiency metrics are quantitative measures that assess how effectively resources are utilized to achieve desired outcomes. These metrics are crucial for optimizing operations and maximizing productivity.
- Key efficiency metrics include: Â
- Overall equipment effectiveness (OEE)
- Cycle time
- Cost per unit
- Analyzing efficiency metrics helps organizations identify bottlenecks and areas for improvement. Rapid Innovation utilizes AI algorithms to analyze operational data, providing actionable insights that drive efficiency.
- Implementing lean methodologies can enhance efficiency by eliminating waste and streamlining processes. Our blockchain solutions can enhance traceability in your supply chain, ensuring that lean practices are effectively applied.
- Regularly tracking efficiency metrics allows for timely adjustments and informed decision-making. We offer real-time monitoring solutions that integrate AI and blockchain, enabling you to make data-driven decisions swiftly.
- Employee engagement in efficiency initiatives can lead to innovative solutions and improved morale. Our workshops and training programs empower your team to embrace efficiency as a core value, fostering a culture of continuous improvement.
- Key performance metrics examples can further illustrate how efficiency can be measured and improved within your organization. Understanding KPI indicators and their implications can lead to better resource allocation and strategic planning.
8.4. Cost Analysis
Cost analysis is a critical component of financial management that helps organizations understand their expenses and optimize their budgets. It involves evaluating all costs associated with a project, product, or service to identify areas for improvement and cost-saving opportunities.
- Types of Costs: Â
- Fixed Costs: These are expenses that do not change with the level of production or sales, such as rent and salaries.
- Variable Costs: These costs fluctuate based on production volume, including materials and labor.
- Semi-Variable Costs: These have both fixed and variable components, like utility bills that have a base charge plus usage fees.
- Importance of Cost Analysis: Â
- Identifies inefficiencies: Helps pinpoint areas where costs can be reduced without sacrificing quality, enabling organizations to allocate resources more effectively.
- Informs pricing strategies: Understanding costs allows businesses to set competitive prices while ensuring profitability, which is essential for maintaining a strong market position.
- Aids in budgeting: Provides a clear picture of where money is being spent, facilitating better financial planning and resource allocation.
- Techniques for Cost Analysis: Â
- Break-even analysis: Determines the sales volume at which total revenues equal total costs, helping businesses understand the minimum performance required to avoid losses.
- Activity-based costing: Allocates overhead costs based on actual activities, providing a more accurate cost per product or service, which can lead to more informed pricing and investment decisions.
- Variance analysis: Compares actual costs to budgeted costs to identify discrepancies and areas for improvement, allowing organizations to adjust their strategies proactively.
- Cost price analysis: This technique focuses on evaluating the cost structure of products or services to ensure that pricing strategies are aligned with overall business objectives.
- Cost analysis techniques: Various methodologies can be employed to assess costs, including direct and indirect cost analysis, which helps in understanding the full financial impact of business operations.
- Cost and price analysis techniques: These combined approaches allow organizations to evaluate both the costs incurred and the prices set for products or services, ensuring that profitability is maintained.
- Cost price analysis techniques: This involves a detailed examination of the relationship between costs and pricing, helping businesses to optimize their pricing strategies based on cost structures.
At Rapid Innovation, we leverage advanced AI and Blockchain technologies to enhance your cost analysis, customer satisfaction metrics, and ROI measurement processes. By integrating AI-driven analytics, we help you uncover hidden inefficiencies and optimize your budget allocation. Our Blockchain solutions ensure transparency and traceability in your financial transactions, further enhancing your ability to measure and improve ROI. Let us partner with you to achieve greater efficiency and effectiveness in reaching your business goals.
8.5. Customer Satisfaction Metrics
Customer satisfaction metrics are essential for measuring how well a company meets or exceeds customer expectations. These metrics provide insights into customer experiences and help businesses improve their products and services.
- Key Metrics: Â
- Net Promoter Score (NPS): Measures customer loyalty by asking how likely customers are to recommend a company to others.
- Customer Satisfaction Score (CSAT): A straightforward metric that gauges customer satisfaction with a specific interaction or overall experience.
- Customer Effort Score (CES): Assesses how easy it is for customers to interact with a company, focusing on the effort required to resolve issues.
- Importance of Customer Satisfaction Metrics: Â
- Enhances customer retention: Satisfied customers are more likely to return and make repeat purchases, which is crucial for long-term business success.
- Drives referrals: Happy customers often share their positive experiences, leading to new business opportunities and organic growth.
- Informs product development: Feedback from satisfaction metrics can guide improvements and innovations, ensuring that offerings align with customer needs.
- Methods for Collecting Data: Â
- Surveys: Online or in-person surveys can gather direct feedback from customers.
- Social media monitoring: Analyzing customer comments and reviews on social platforms provides real-time insights.
- Focus groups: Engaging with a small group of customers can yield in-depth qualitative data.
8.6. ROI Measurement
Return on Investment (ROI) measurement is a vital financial metric that evaluates the profitability of an investment relative to its cost. It helps businesses assess the effectiveness of their investments and make informed decisions.
- Calculating ROI: Â
- The basic formula for ROI is:
language="language-plaintext"ROI = (Net Profit / Cost of Investment) x 100
- Net Profit is calculated as total revenue generated from the investment minus the total costs associated with it. Â
- Importance of ROI Measurement:
- Evaluates performance: Provides a clear picture of how well an investment is performing, allowing organizations to make data-driven decisions.
- Aids in decision-making: Helps businesses determine which projects or initiatives are worth pursuing based on their potential returns, ensuring that resources are allocated effectively.
- Justifies expenditures: Demonstrates the value of investments to stakeholders and can secure funding for future projects. Â
- Factors Influencing ROI:
- Timeframe: The duration over which the investment is measured can significantly impact ROI calculations.
- Market conditions: Economic factors and market trends can affect revenue generation and costs.
- Quality of data: Accurate and comprehensive data is essential for reliable ROI assessments. Â
- Best Practices for ROI Measurement:
- Set clear objectives: Define what success looks like for each investment to measure against.
- Monitor continuously: Regularly track performance to identify trends and make adjustments as needed.
- Compare with benchmarks: Use industry standards to evaluate the effectiveness of your investments relative to competitors.
9. Integration with Existing Systems
Integration with existing systems is crucial for businesses looking to enhance their operational efficiency and improve customer experiences. By ensuring that new technologies work seamlessly with established systems, organizations can leverage their existing investments while minimizing disruptions.
9.1 Core Banking Systems

‍
Core banking systems are the backbone of financial institutions, enabling them to manage accounts, transactions, and customer data effectively. Integrating new technologies with core banking systems can lead to significant benefits, including enhanced customer experience, operational efficiency, data consistency, scalability, regulatory compliance, and improved risk management.
- Enhanced Customer Experience: Integration allows for real-time access to customer data, enabling personalized services and faster response times. Customers can enjoy seamless transactions and improved service delivery.
- Operational Efficiency: By connecting new applications with core banking systems, banks can automate processes, reduce manual errors, and streamline operations, leading to cost savings and improved productivity.
- Data Consistency: Integration ensures that data across various platforms is consistent and up-to-date, which is vital for compliance and reporting purposes, as discrepancies can lead to regulatory issues.
- Scalability: As banks grow, their systems need to scale accordingly. Integrating with core banking systems allows for the addition of new functionalities without overhauling existing infrastructure.
- Regulatory Compliance: Many financial institutions face stringent regulations. Integration helps in maintaining compliance by ensuring that all systems adhere to the necessary standards and protocols.
- Improved Risk Management: By integrating risk management tools with core banking systems, institutions can better assess and mitigate risks associated with lending and investment activities.
9.2 CRM Integration
Customer Relationship Management (CRM) systems are essential for managing interactions with customers and prospects. Integrating CRM with other business systems can significantly enhance customer engagement and operational efficiency. The benefits of CRM integration include a unified customer view, streamlined communication, sales and marketing alignment, automated workflows, enhanced reporting and analytics, and improved customer retention.
- Unified Customer View: Integration allows businesses to create a 360-degree view of the customer by consolidating data from various sources, helping in understanding customer behavior and preferences.
- Streamlined Communication: By connecting CRM with communication tools like ringcentral, businesses can ensure that all customer interactions are logged and accessible, leading to more effective follow-ups and improved customer satisfaction.
- Sales and Marketing Alignment: Integrating CRM with sales and marketing platforms enables better collaboration between teams, ensuring that marketing campaigns are aligned with sales strategies, which leads to higher conversion rates. For instance, integrating sap business one with salesforce can enhance this alignment.
- Automated Workflows: Integration can automate repetitive tasks, such as data entry and lead assignment, freeing up time for employees to focus on more strategic activities. This can be particularly beneficial when integrating with business process integration software.
- Enhanced Reporting and Analytics: By integrating CRM with analytics tools, businesses can gain deeper insights into customer behavior and campaign performance, helping in making informed decisions. For example, integrating netsuite power bi can provide valuable analytics.
- Improved Customer Retention: With integrated systems, businesses can proactively address customer issues and provide personalized experiences, leading to higher retention rates. Utilizing tools like xero inventory software can also enhance customer satisfaction through better inventory management.
In conclusion, the integration of core banking systems and CRM with existing systems is essential for organizations aiming to enhance their operational capabilities and customer engagement. By leveraging these integrations, businesses can achieve greater efficiency, compliance, and customer satisfaction. At Rapid Innovation, we specialize in facilitating these integrations, ensuring that our clients can maximize their ROI through streamlined operations and enhanced customer experiences. Our expertise in AI and Blockchain technologies further empowers organizations to innovate and stay ahead in a competitive landscape. Additionally, we offer solutions for integrating platforms such as sap magento integration, b2b edi integration, and nextiva crm integration to meet diverse business needs. For more insights on the role of AI in retail and e-commerce, check out our article on the growth of AI agents in retail and e-commerce.
9.3. Document Management Systems
Document Management Systems (DMS) are essential tools for organizations looking to streamline their document handling processes. These systems help in the creation, storage, management, and tracking of electronic documents and images of paper-based information. DMS provides a centralized repository for all documents, making it easier to access and manage files. Users can track changes and maintain different versions of documents, ensuring that the most current information is always available. Additionally, DMS often includes security features such as user authentication, access controls, and encryption to protect sensitive information. Teams can collaborate on documents in real-time, facilitating better communication and productivity. Many DMS solutions also offer features that help organizations comply with regulations by maintaining audit trails of document access and modifications.
At Rapid Innovation, we leverage AI technologies to enhance DMS capabilities, enabling intelligent document classification and automated workflows. This not only reduces manual effort but also increases accuracy, leading to significant time savings and improved ROI. Popular DMS solutions include SharePoint, M-Files, and DocuWare, which cater to various business needs and sizes. Other notable systems include google documentum, output management system, and edms document management, which provide additional functionalities for document management. The integration of document archiving systems and document handling systems further enhances the efficiency of DMS, ensuring that organizations can manage their documents effectively. If you're looking to enhance your document management systems, consider hiring Action Transformer Developers to help you implement advanced solutions. For insights on how AI can transform financial reporting, check out our article on generative AI and automated financial reporting applications.
9.4. Payment Processing Systems
Payment Processing Systems are crucial for businesses that handle transactions, whether online or in-person. These systems facilitate the acceptance, processing, and management of payments, ensuring a smooth transaction experience for both businesses and customers. Payment processing systems support various payment methods, including credit cards, debit cards, digital wallets, and bank transfers, catering to diverse customer preferences. These systems employ encryption and tokenization to protect sensitive payment information, reducing the risk of fraud and data breaches. Many payment processors can integrate with existing accounting and inventory management systems, streamlining financial operations. Payment processing systems often provide real-time transaction processing, allowing businesses to receive funds quickly and efficiently. Additionally, these systems typically offer reporting tools that help businesses analyze sales trends, customer behavior, and payment performance.
Rapid Innovation can assist in implementing blockchain-based payment processing systems, enhancing security and transparency in transactions. This innovative approach not only mitigates fraud risks but also fosters trust among customers, ultimately driving greater ROI. Popular payment processing systems include PayPal, Stripe, and Square, each offering unique features tailored to different business models.
9.5. Reporting and Analytics Platforms
Reporting and Analytics Platforms are vital for organizations seeking to make data-driven decisions. These platforms enable businesses to collect, analyze, and visualize data, providing insights that can drive strategic initiatives. Reporting platforms often include tools for creating charts, graphs, and dashboards, making complex data easier to understand and interpret. Users can generate customized reports based on specific metrics, timeframes, and data sources, allowing for tailored insights. Many analytics platforms provide real-time data access, enabling organizations to make timely decisions based on the latest information. Advanced reporting tools often include predictive analytics capabilities, helping businesses forecast trends and outcomes based on historical data. These platforms typically allow for sharing reports and insights across teams, fostering collaboration and informed decision-making.
At Rapid Innovation, we utilize AI-driven analytics to enhance reporting capabilities, enabling organizations to uncover hidden patterns and insights that can lead to more informed strategic decisions. Popular reporting and analytics platforms include Tableau, Microsoft Power BI, and Google Data Studio, each offering unique functionalities to meet various analytical needs.
10. Customer Experience Enhancement
Enhancing customer experience is crucial for businesses aiming to build loyalty and increase satisfaction. By focusing on digital applications and real-time updates, companies can significantly improve how customers interact with their services, leading to improving customer experience and enhancing customer service.
10.1 Digital Application Interface

‍
A well-designed digital application interface is essential for providing a seamless user experience. The interface serves as the primary point of interaction between the customer and the service, making it vital for businesses to prioritize its design and functionality.
- User-Friendly Design: A clean, intuitive layout helps users navigate the application effortlessly. This includes clear menus and navigation paths, consistent color schemes and fonts, and responsive design for various devices.
- Accessibility Features: Ensuring that the application is accessible to all users, including those with disabilities, is crucial. This can involve screen reader compatibility, text resizing options, and high-contrast color settings.
- Personalization Options: Allowing users to customize their experience can enhance satisfaction. Features may include personalized dashboards, tailored content recommendations, and user-specific settings and preferences.
- Feedback Mechanisms: Incorporating ways for users to provide feedback directly through the application can help businesses improve customer service. This can include in-app surveys, rating systems for features, and direct communication channels with support teams.
- Integration with Other Services: A digital application that integrates seamlessly with other platforms can enhance user experience. This can involve linking to social media accounts, integration with payment gateways, and compatibility with third-party applications.
10.2 Real-time Status Updates
Real-time status updates are essential for keeping customers informed and engaged. Providing timely information can significantly enhance the overall customer experience.
- Instant Notifications: Sending push notifications or alerts about important updates can keep users informed. This includes order confirmations, shipping updates, and service interruptions or maintenance alerts.
- Live Tracking Features: For services that involve delivery or appointments, live tracking can provide peace of mind. This can involve GPS tracking for deliveries, real-time updates on service technician arrival times, and interactive maps showing service progress.
- Transparent Communication: Keeping customers in the loop about any changes or delays is crucial. This can include regular updates via email or SMS, clear explanations for any issues encountered, and proactive communication about potential delays.
- User Control: Allowing customers to manage their preferences for updates can enhance their experience. This can involve options to choose preferred communication channels, customizable notification settings, and the ability to pause or resume updates as needed.
- Data Security: Ensuring that real-time updates are secure is vital for maintaining customer trust. This can involve encryption of sensitive information, regular security audits, and compliance with data protection regulations.
By focusing on these aspects of digital application interfaces and real-time status updates, businesses can significantly enhance customer experience, leading to increased satisfaction and loyalty. Strategies to improve customer service and enhance client experience are essential for achieving these goals. At Rapid Innovation, we leverage our expertise in AI and Blockchain to develop tailored solutions that not only improve user engagement but also ensure data integrity and security, ultimately driving greater ROI for our clients. Additionally, we explore various AI in customer support use cases to further enhance service delivery and customer satisfaction.
10.3. Automated Communications
Automated customer communication is essential in today's fast-paced digital landscape. They streamline interactions between businesses and customers, ensuring timely responses and efficient service delivery. Rapid Innovation leverages advanced AI technologies to implement automated communication systems that can significantly enhance your business operations.
- Enhances customer engagement by providing instant replies to inquiries through AI-driven chatbots. Â
- Reduces the workload on customer service teams, allowing them to focus on complex issues that require human intervention. Â
- Utilizes chatbots and automated email responses to handle common queries, ensuring that customers receive immediate assistance. Â
- Increases operational efficiency by automating routine tasks, such as appointment reminders and follow-ups, which can lead to cost savings. Â
- Provides 24/7 support, ensuring customers can receive assistance at any time, thus improving overall service availability. Â
- Improves customer satisfaction through quick resolutions and consistent communication, ultimately leading to higher retention rates. Â
According to a report by Gartner, 85% of customer interactions will be managed without a human by 2025, highlighting the growing importance of automated customer communication in customer service strategies.
10.4. Self-service Options
Self-service options empower customers to find solutions independently, enhancing their overall experience. This approach not only saves time but also fosters a sense of control among users. Rapid Innovation can help you implement self-service solutions that align with your business goals.
- Offers FAQs, knowledge bases, and instructional videos for easy access to information, reducing the need for direct support. Â
- Enables customers to manage their accounts, track orders, and update personal information without needing assistance, enhancing user autonomy. Â
- Reduces the volume of inquiries directed to customer service, allowing teams to focus on more complex issues that require specialized knowledge. Â
- Increases customer satisfaction by providing immediate access to solutions, which can lead to improved brand loyalty. Â
- Encourages user engagement through interactive tools, such as chatbots and virtual assistants, which can enhance the customer journey. Â
- Supports various platforms, including mobile apps and websites, ensuring accessibility and convenience for all users. Â
Research indicates that 70% of customers prefer to use self-service options to address their issues, demonstrating the effectiveness of this approach in enhancing customer experience.
10.5. Personalization Features
Personalization features are crucial for creating tailored experiences that resonate with individual customers. By leveraging data and insights, Rapid Innovation can help businesses enhance customer loyalty and satisfaction through personalized solutions.
- Utilizes customer data to deliver personalized recommendations and content, ensuring that marketing efforts are relevant and effective. Â
- Enhances marketing efforts through targeted campaigns based on user behavior and preferences, leading to higher engagement rates. Â
- Creates a more engaging user experience by addressing customers by name and acknowledging their past interactions, fostering a sense of connection. Â
- Increases conversion rates by presenting relevant products or services that align with customer interests, ultimately driving sales. Â
- Fosters long-term relationships by making customers feel valued and understood, which is essential for brand loyalty. Â
- Adapts communication styles and channels based on customer preferences, ensuring a seamless experience across all touchpoints. Â
According to a study by Epsilon, 80% of consumers are more likely to make a purchase when brands offer personalized experiences, underscoring the importance of personalization in driving sales and customer loyalty. Rapid Innovation's expertise in AI and data analytics can help you harness the power of personalization to achieve greater ROI.
11. Advanced Features and Future Trends
The financial landscape is rapidly evolving, driven by technological advancements and changing consumer expectations. Two significant trends shaping the future of finance are blockchain integration and open banking APIs. These innovations promise to enhance security, transparency, and customer experience in the financial sector.
11.1 Blockchain Integration

‍
Blockchain technology is revolutionizing various industries, including finance. Its decentralized nature offers numerous advantages that can transform traditional banking systems.
- Enhanced Security: Blockchain provides a secure way to record transactions. Each transaction is encrypted and linked to the previous one, making it nearly impossible to alter past records. This feature significantly reduces the risk of fraud and cyberattacks.
- Transparency and Trust: With blockchain, all participants in a transaction can access the same information in real-time. This transparency fosters trust among users, as they can verify transactions independently without relying on a central authority.
- Cost Efficiency: By eliminating intermediaries, blockchain can reduce transaction costs. Financial institutions can streamline processes, leading to faster and cheaper transactions for consumers. This is particularly relevant in applications of blockchain in finance, where efficiency is paramount.
- Smart Contracts: These self-executing contracts, with the terms of the agreement directly written into code, can automate various processes, such as loan approvals and insurance claims. This automation reduces the need for manual intervention, speeding up transactions and minimizing errors. Blockchain financial applications often utilize smart contracts for enhanced functionality.
- Cross-Border Transactions: Blockchain can facilitate faster and cheaper cross-border payments. Traditional methods often involve multiple banks and high fees, but blockchain can simplify this process, making it more accessible for individuals and businesses. Blockchain for remittances is a growing area of interest.
- Tokenization of Assets: Blockchain allows for the tokenization of physical assets, such as real estate or art. This process can democratize investment opportunities, enabling fractional ownership and increasing liquidity in traditionally illiquid markets. Blockchain corporate finance is an emerging field that leverages this capability.
As blockchain technology continues to mature, its integration into financial services is expected to grow, offering innovative solutions that enhance security, efficiency, and user experience. At Rapid Innovation, we specialize in implementing blockchain solutions that align with your business goals, ensuring you achieve greater ROI through enhanced operational efficiency and reduced costs. The benefits of blockchain in financial services are becoming increasingly recognized, leading to a surge in blockchain finance companies.
11.2 Open Banking APIs
Open banking is another trend reshaping the financial services landscape. It allows third-party developers to build applications and services around financial institutions, fostering innovation and competition.
- Consumer Empowerment: Open banking APIs give consumers greater control over their financial data. They can choose to share their information with third-party providers, enabling personalized financial services tailored to their needs.
- Increased Competition: By allowing new entrants into the market, open banking promotes competition among financial institutions. This competition can lead to better services, lower fees, and improved customer experiences.
- Innovative Financial Products: Open banking encourages the development of innovative financial products and services. Fintech companies can leverage APIs to create solutions that address specific consumer needs, such as budgeting tools, investment platforms, and payment solutions.
- Seamless User Experience: Open banking APIs enable seamless integration between different financial services. Consumers can access multiple services through a single platform, simplifying their financial management.
- Data-Driven Insights: Financial institutions can use data from open banking to gain insights into consumer behavior. This information can help them tailor their offerings and improve customer engagement.
- Regulatory Compliance: Open banking is often driven by regulatory initiatives aimed at increasing competition and protecting consumer rights. Institutions must comply with regulations, ensuring that consumer data is handled securely and transparently.
As open banking continues to evolve, it is expected to drive innovation in the financial sector, leading to enhanced services and improved customer satisfaction. Rapid Innovation is at the forefront of this transformation, providing consulting and development services that help financial institutions leverage open banking APIs to create tailored solutions that meet the needs of their customers.
In conclusion, both blockchain integration and open banking APIs are pivotal in shaping the future of finance. These advanced features not only enhance security and transparency but also empower consumers and foster innovation in financial services. By partnering with Rapid Innovation, you can harness these technologies to achieve your business objectives efficiently and effectively, ultimately driving greater ROI. The applications of blockchain technology in banking and financial sector are vast, and the future looks promising for blockchain solutions for financial services.
11.3. Enhanced Biometric Verification
Enhanced biometric verification is revolutionizing the way financial institutions authenticate users. This technology leverages unique biological traits to ensure secure access to accounts and services.
- Fingerprint recognition: Widely used in smartphones, this method allows users to unlock devices and authorize transactions with a simple touch.
- Facial recognition: This technology scans and analyzes facial features, providing a quick and secure way to verify identity. This includes advanced systems like facial recognition identity verification and facial biometric verification.
- Voice recognition: By analyzing voice patterns, financial institutions can authenticate users over the phone or through voice-activated services.
- Iris scanning: This highly secure method uses the unique patterns in the iris to confirm identity, making it difficult to forge.
- Behavioral biometrics: This innovative approach monitors user behavior, such as typing speed and mouse movements, to detect anomalies and prevent fraud.
The adoption of enhanced biometric verification not only improves security but also enhances user experience by streamlining the authentication process. As cyber threats continue to evolve, financial institutions are increasingly turning to biometric solutions, such as biometric identity verification systems and biometric verification technology, to safeguard sensitive information. At Rapid Innovation, we specialize in integrating advanced biometric technologies into existing systems, ensuring that our clients can provide secure and seamless user experiences while significantly reducing the risk of fraud through biometric identity checks and biometric verification machines. Additionally, we offer predictive analytics solutions that can further enhance security measures.
11.4. Real-time Risk Assessment
Real-time risk assessment is a critical component of modern financial services, enabling institutions to evaluate potential risks as they occur. This proactive approach helps in making informed decisions and mitigating potential losses.
- Data analytics: By analyzing vast amounts of data in real-time, financial institutions can identify trends and patterns that indicate potential risks.
- Machine learning algorithms: These algorithms continuously learn from new data, improving their ability to predict and assess risks over time.
- Instant alerts: Real-time risk assessment systems can send immediate notifications to relevant stakeholders when a potential risk is detected, allowing for swift action.
- Customer behavior analysis: Monitoring customer transactions and behaviors in real-time helps identify unusual activities that may indicate fraud or other risks.
- Regulatory compliance: Real-time assessments ensure that financial institutions remain compliant with regulations by identifying risks associated with non-compliance.
By implementing real-time risk assessment, financial institutions can enhance their risk management strategies, protect their assets, and maintain customer trust. Rapid Innovation offers tailored solutions that leverage AI-driven analytics to provide our clients with the tools necessary for effective risk management, ultimately leading to improved ROI and operational efficiency.
11.5. Predictive Loan Servicing
Predictive loan servicing utilizes advanced analytics and machine learning to anticipate borrower behavior and improve loan management. This approach allows financial institutions to proactively address potential issues and enhance customer satisfaction.
- Early warning systems: By analyzing historical data, predictive models can identify borrowers at risk of default, enabling timely intervention.
- Personalized communication: Financial institutions can tailor their outreach efforts based on predicted borrower behavior, improving engagement and retention.
- Optimized repayment plans: Predictive analytics can help create customized repayment plans that align with a borrower’s financial situation, increasing the likelihood of successful repayment.
- Enhanced customer support: By anticipating borrower needs, financial institutions can provide proactive support, addressing issues before they escalate.
- Improved portfolio management: Predictive loan servicing allows institutions to better manage their loan portfolios by identifying trends and adjusting strategies accordingly.
The integration of predictive loan servicing not only enhances operational efficiency but also fosters stronger relationships between financial institutions and their customers. At Rapid Innovation, we empower our clients with predictive analytics solutions that drive better decision-making and customer engagement, ultimately leading to higher returns on investment.
12. Case Studies and Best Practices
Case studies and best practices provide valuable insights into successful strategies and methodologies that organizations have employed. They serve as a guide for others looking to implement similar initiatives, showcasing what works and what doesn’t.
12.1 Implementation Success Stories
Implementation success stories highlight real-world examples where organizations have effectively executed strategies, leading to significant improvements and outcomes. These stories often illustrate the practical application of theories and concepts, making them relatable and actionable.
- Company A: Digital Transformation
A leading retail company adopted a comprehensive digital transformation strategy, integrating e-commerce with brick-and-mortar operations, which resulted in a 30% increase in online sales within the first year. The initiative also enhanced customer experience through personalized marketing and streamlined logistics, showcasing how Rapid Innovation's AI-driven analytics can optimize customer engagement and sales strategies. - Company B: Sustainability Initiatives
A manufacturing firm implemented sustainable practices by reducing waste and optimizing energy use. This approach achieved a 25% reduction in operational costs while improving brand reputation, ultimately gaining recognition as an industry leader in sustainability and attracting eco-conscious consumers. Rapid Innovation's blockchain solutions facilitated transparent supply chain management, ensuring accountability and sustainability. - Company C: Employee Engagement Programs
A tech company launched an employee engagement program focused on mental health and work-life balance. This initiative increased employee satisfaction scores by 40% and significantly reduced turnover rates. It also fostered a culture of innovation and collaboration, leading to improved productivity. Rapid Innovation's AI tools helped analyze employee feedback, enabling the company to tailor programs that resonate with their workforce.
These success stories not only demonstrate the effectiveness of various strategies but also inspire other organizations to adopt similar approaches. They emphasize the importance of aligning initiatives with organizational goals and the need for continuous evaluation and adaptation.
12.2 Lessons Learned
Lessons learned from case studies provide critical insights into the challenges faced during implementation and the strategies that helped overcome them. Understanding these lessons can help organizations avoid common pitfalls and enhance their chances of success.
- Importance of Stakeholder Engagement
Engaging stakeholders early in the process is crucial for buy-in and support. Regular communication helps address concerns and fosters a collaborative environment. - Flexibility and Adaptability
Organizations must remain flexible and willing to adapt their strategies based on feedback and changing circumstances. Successful implementations often involve iterative processes that allow for adjustments along the way. - Data-Driven Decision Making
Utilizing data analytics to inform decisions can lead to more effective strategies. Organizations that leverage data often see improved outcomes and can better measure success. Rapid Innovation emphasizes the importance of AI-driven insights to guide strategic decisions. - Training and Development
Investing in employee training ensures that staff are equipped with the necessary skills to implement new initiatives. Continuous learning opportunities can enhance employee engagement and retention. - Clear Objectives and Metrics
Establishing clear objectives and measurable outcomes is essential for tracking progress. Organizations should regularly review these metrics to assess effectiveness and make necessary adjustments.
By analyzing these lessons learned, organizations can refine their approaches and increase the likelihood of successful implementation. The combination of success stories and lessons learned creates a comprehensive understanding of best practices for case studies, guiding future initiatives. Rapid Innovation stands ready to assist organizations in leveraging AI and blockchain technologies to achieve their business goals efficiently and effectively.
12.3. Industry Benchmarks
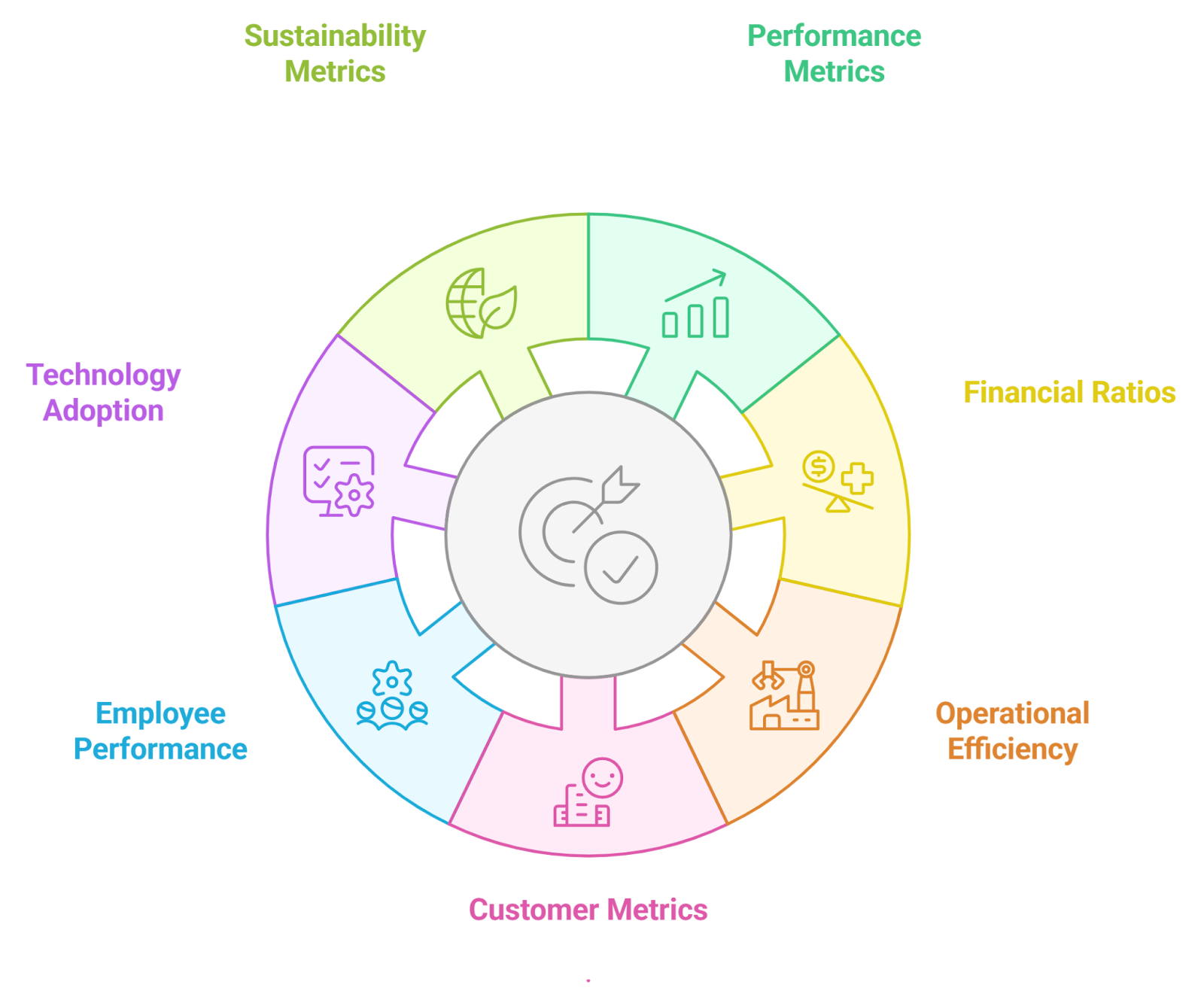
‍
Industry benchmarks are critical for organizations to measure their performance against established standards within their sector. These benchmarks provide a reference point that helps businesses understand their position relative to competitors and the industry as a whole.
- Performance Metrics: Common metrics include revenue growth, profit margins, customer satisfaction scores, and employee turnover rates. These metrics help organizations gauge their operational efficiency and effectiveness.
- Financial Ratios: Key financial ratios such as return on investment (ROI), debt-to-equity ratio, and current ratio are essential for assessing financial health. Comparing these ratios with industry averages can highlight areas for improvement. At Rapid Innovation, we leverage AI-driven analytics to provide clients with insights that enhance their ROI, ensuring they are not only meeting but exceeding industry standards.
- Operational Efficiency: Benchmarks related to production costs, supply chain efficiency, and inventory turnover can help organizations identify inefficiencies. For instance, a company may find that its production costs are significantly higher than the industry average, prompting a review of its processes. Our blockchain solutions can streamline supply chain operations, reducing costs and improving transparency. Additionally, industry benchmarks such as inventory turnover benchmark by industry can provide valuable insights.
- Customer Metrics: Customer-related benchmarks, such as net promoter score (NPS) and customer acquisition cost (CAC), provide insights into customer loyalty and marketing effectiveness. Understanding these metrics can help businesses refine their customer engagement strategies. Rapid Innovation employs AI tools to analyze customer data, enabling businesses to tailor their marketing efforts for better engagement and retention. For example, benchmarking in healthcare can reveal important trends in patient satisfaction.
- Employee Performance: Employee productivity and engagement benchmarks can inform HR practices. Organizations can compare their employee satisfaction scores with industry standards to identify areas for improvement in workplace culture. Our consulting services can help implement AI-driven performance management systems that enhance employee engagement and productivity.
- Technology Adoption: In today’s digital age, benchmarks related to technology usage, such as the percentage of automated processes or digital marketing effectiveness, are increasingly important. Companies can assess their technological readiness against industry leaders. Rapid Innovation assists clients in adopting cutting-edge AI and blockchain technologies, ensuring they remain competitive. Technology benchmarks can help organizations understand their position in the market.
- Sustainability Metrics: As sustainability becomes a priority, benchmarks related to carbon footprint, waste management, and resource efficiency are gaining traction. Organizations can evaluate their environmental impact compared to industry standards. We help clients integrate sustainable practices through innovative technology solutions, enhancing their market reputation.
Utilizing industry benchmarks allows organizations to set realistic goals, identify best practices, and drive continuous improvement. By regularly reviewing these benchmarks, businesses can adapt to changing market conditions and maintain a competitive edge. For instance, average bounce rate by industry and google analytics benchmarks by industry can provide insights into online performance. Additionally, for organizations looking to enhance their blockchain capabilities, our zk-rollup development services can provide a significant advantage. Furthermore, learning from real-world AI implementations can offer valuable lessons and strategies for organizations aiming to improve their performance and competitiveness.
12.4. Best Practice Guidelines
Best practice guidelines are essential frameworks that organizations can adopt to enhance their operations, improve efficiency, and achieve strategic objectives. These guidelines are often based on proven methods and successful case studies within the industry.
- Standard Operating Procedures (SOPs): Developing clear SOPs ensures consistency in operations. These documents outline step-by-step processes for various tasks, reducing errors and improving efficiency.
- Continuous Improvement: Embracing a culture of continuous improvement, such as Lean or Six Sigma methodologies, helps organizations identify waste and enhance processes. Regular training and workshops can foster this mindset among employees.
- Data-Driven Decision Making: Utilizing data analytics to inform decision-making processes is crucial. Organizations should invest in tools that allow them to collect, analyze, and interpret data effectively, leading to informed strategies. Rapid Innovation specializes in AI solutions that empower businesses to make data-driven decisions, enhancing their strategic planning.
- Employee Training and Development: Providing ongoing training opportunities for employees ensures they remain skilled and knowledgeable. This investment in human capital can lead to higher productivity and job satisfaction.
- Customer-Centric Approach: Adopting a customer-centric approach involves understanding customer needs and preferences. Organizations should regularly gather feedback and adjust their offerings accordingly to enhance customer satisfaction.
- Risk Management: Implementing robust risk management practices helps organizations identify potential threats and develop mitigation strategies. Regular risk assessments and contingency planning are vital components of this process.
- Compliance and Regulatory Adherence: Staying compliant with industry regulations is essential for avoiding legal issues. Organizations should regularly review their practices to ensure they meet all necessary standards.
- Collaboration and Communication: Encouraging open communication and collaboration among teams fosters innovation and problem-solving. Utilizing collaborative tools can enhance teamwork and project management.
- Technology Integration: Leveraging technology to streamline operations is a best practice that can lead to significant efficiency gains. Organizations should assess their current technology stack and explore new solutions that align with their goals. Rapid Innovation offers tailored AI and blockchain solutions that facilitate seamless technology integration.
- Performance Monitoring: Regularly monitoring performance against established KPIs allows organizations to track progress and make necessary adjustments. This practice ensures that strategic objectives are met effectively.
By implementing best practice guidelines, organizations can enhance their operational effectiveness, improve employee engagement, and ultimately drive better business outcomes. These guidelines serve as a roadmap for achieving excellence in various aspects of the organization, including b2b benchmarks and financial benchmarks by industry.