Table Of Contents
Category
Artificial Intelligence
1. Introduction to Artificial Intelligence (AI)
Artificial Intelligence (AI) has become a transformative technology, impacting various industries and aspects of daily life. This section provides a foundational understanding of AI, including its definition and historical background.
1.1. Definition of AI
Artificial Intelligence (AI) refers to the simulation of human intelligence in machines that are programmed to think and learn like humans. These intelligent systems can perform tasks typically requiring human intelligence, such as visual perception, speech recognition, decision-making, and language translation. The ultimate aim of AI is to create systems that can function intelligently and independently, adapting to new information and scenarios in ways similar to human cognitive processes.
Key Characteristics:
- Learning: The ability to learn from data and experiences, improving over time.
- Reasoning: The capability to make decisions and solve problems based on available information.
- Perception: Interpreting and understanding sensory inputs, such as vision and sound.
- Natural Language Processing (NLP): Understanding and generating human language.
1.2. Brief History of AI
The history of AI dates back to the mid-20th century when the concept of "thinking machines" began to take shape. Here are some key milestones in the evolution of AI:
- Early Concepts (1940s-1950s): The idea of artificial intelligence was first explored by mathematicians and computer scientists such as Alan Turing, who proposed the Turing Test to measure a machine's ability to exhibit intelligent behavior.
- Dartmouth Conference (1956): The term "Artificial Intelligence" was coined during a seminal conference at Dartmouth College, organized by John McCarthy, Marvin Minsky, Nathaniel Rochester, and Claude Shannon. This event is often regarded as the birth of AI as a field of study.
- Early Development (1950s-1970s): Initial research focused on developing algorithms and programming languages, such as LISP, to facilitate AI experimentation. Early AI systems included simple problem-solving programs and rule-based systems.
- AI Winter (1970s-1980s): During this period, enthusiasm for AI waned due to unmet expectations and limited computational power. Funding and interest declined, leading to what is known as the "AI Winter."
- Renewed Interest (1980s-1990s): Advances in machine learning, neural networks, and expert systems reignited interest in AI. The development of more powerful computers and the availability of large datasets contributed to this resurgence.
- Modern AI (2000s-Present): The advent of big data, deep learning, and advanced algorithms has propelled AI to new heights. Significant achievements include natural language processing systems like GPT-3, advanced computer vision models, and autonomous systems.
2. Types of Artificial Intelligence
AI can be categorized based on its capabilities and functionalities. Understanding these types helps in grasping the current state and future potential of AI technologies.
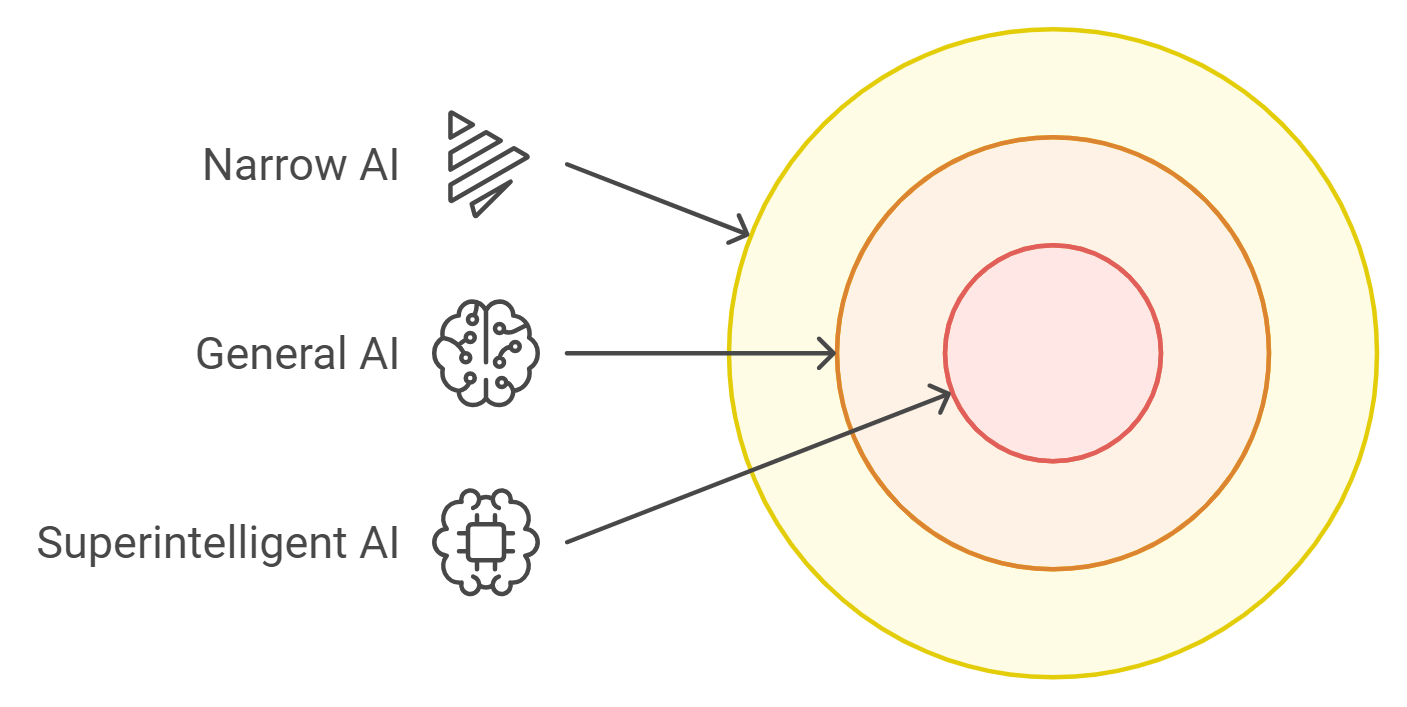
‍
2.1. Narrow AI (ANI)
Narrow AI, also known as Weak AI, is designed to perform a specific task or a narrow set of tasks. It operates under a limited set of constraints and capabilities, making it highly efficient for particular applications. Examples of Narrow AI include:
- Facial Recognition: Used in security systems and social media platforms to identify individuals.
- Internet Searches: Algorithms that provide relevant search results based on user queries.
- Self-Driving Cars: Systems that navigate and operate vehicles autonomously.
Narrow AI is commonly used today because it excels in specific tasks within predefined parameters.
2.2. General AI (AGI)
General AI, or Strong AI, refers to systems with the ability to understand, learn, and apply intelligence across a broad range of tasks, mimicking human cognitive abilities. Unlike Narrow AI, AGI is not limited to specific tasks and can perform various intellectual tasks with a high degree of proficiency.
2.2.1. Differences between ANI and AGI
The primary difference between Narrow AI (ANI) and General AI (AGI) lies in their capabilities:
- ANI: Excels in specific tasks, operates within a set framework, and lacks the ability to generalize beyond its training.
- AGI: Can perform any intellectual task that a human can, with the ability to learn, understand, and apply knowledge across different domains.
2.2.2. Current Research and Development in AGI
Research in AGI is focused on creating systems that can reason, solve problems, and plan autonomously. Â For instance, projects like OpenAI and DeepMind are at the forefront of developing AGI technologies. Notable projects and organizations at the forefront of AGI development include:
- OpenAI: A research organization dedicated to advancing digital intelligence in a way that benefits humanity as a whole.
- DeepMind: An AI company known for developing systems like AlphaGo, which demonstrated significant advances in AGI capabilities.
These efforts aim to push the boundaries of what AI can achieve, moving closer to human-like intelligence.
2.3. Superintelligent AI (ASI)
Superintelligent AI refers to a hypothetical form of AI that surpasses human intelligence across all aspects, including creativity, general wisdom, and problem-solving. This type of AI is still theoretical and represents a future where AI can outperform humans in virtually every cognitive task.
- Potential Capabilities: ASI would possess superior problem-solving skills, advanced decision-making abilities, and the capability to innovate beyond human limitations.
- Theoretical Nature: While ASI is an intriguing concept, it remains speculative and poses significant ethical, safety, and control challenges.
3. Benefits of Artificial Intelligence
AI offers numerous benefits across various sectors, enhancing efficiency, decision-making, and personalization.
3.1. Efficiency and Automation
AI significantly contributes to efficiency and automation in various industries. By automating routine tasks, AI systems operate at higher speeds than human workers, often with greater accuracy and less downtime. This leads to increased productivity and cost savings.
Key Points:
- Speed: AI systems can process large volumes of data quickly.
- Accuracy: AI reduces human error in repetitive tasks.
- 24/7 Operation: AI can work continuously without fatigue.
3.2. Enhanced Decision Making
AI enhances decision-making capabilities by quickly processing large amounts of data and providing insights that are not easily visible to human analysts. This is particularly valuable in fields like healthcare and finance.
3.2.1. Examples in Healthcare
In healthcare, AI tools assist in diagnosing diseases more accurately and predicting patient outcomes based on historical data. AI applications also personalize treatment plans and manage healthcare resources more efficiently.
Examples:
- Disease Diagnosis: AI algorithms can detect diseases such as cancer from medical images with high accuracy.
- Predictive Analytics: AI predicts patient outcomes and potential complications, allowing for proactive treatment plans.
- Resource Management: AI optimizes the allocation of medical resources, such as staff and equipment, improving overall efficiency.
3.2.2. Examples in Finance
In finance, AI is utilized for algorithmic trading, fraud detection, and risk management. AI systems analyze market data to make investment decisions or identify unusual patterns that may indicate fraudulent activity.
Examples:
- Algorithmic Trading: AI models execute trades at optimal times based on market analysis, maximizing returns.
- Fraud Detection: AI detects irregularities and potential fraud by analyzing transaction patterns.
- Risk Management: AI assesses and mitigates financial risks by evaluating market trends and financial data.
3.3. Personalization in Service Industries
AI plays a crucial role in personalizing customer service, enhancing user experiences by tailoring products and services to individual preferences. This is particularly evident in online retail, where AI algorithms recommend products based on browsing and purchase history, significantly improving customer satisfaction and loyalty.
Examples:
- Retail: AI recommends products to customers based on their previous purchases and browsing history, increasing sales and customer satisfaction.
- Entertainment: Streaming services use AI to suggest movies and TV shows based on viewing habits.
- Customer Service: AI-powered chatbots provide personalized assistance and support, improving customer engagement and satisfaction.
4. AI Tools and Technologies
Artificial Intelligence (AI) encompasses a wide array of tools and technologies designed to facilitate the development, deployment, and management of AI applications. This section explores some of the most prominent frameworks, platforms, and hardware that power AI solutions.
4.1. Machine Learning Frameworks
Machine learning frameworks are essential for developing AI models. They provide the necessary tools and libraries to create, train, and deploy machine learning algorithms.
4.1.1. TensorFlow
TensorFlow, developed by Google, is a widely-used open-source library for numerical computation and machine learning. It offers a flexible and comprehensive toolkit that enables developers to build and deploy AI-powered applications efficiently. TensorFlow supports various machine learning tasks, including deep learning, making it suitable for both research and production environments.
4.1.2. PyTorch
PyTorch, created by Facebook's AI Research lab, is renowned for its simplicity and ease of use, particularly in the research community. It provides strong support for deep learning and neural networks, featuring a dynamic computational graph that allows for more flexible model development. PyTorch's efficient memory usage and extensive library of pre-built functions make it a favorite among AI developers.
4.2. AI Platforms
AI platforms offer integrated environments for building, training, and deploying machine learning models. These platforms provide various services that streamline the AI development process.
4.2.1. Google AI Platform
Google AI Platform is a suite of services designed to help developers quickly build, train, and deploy machine learning models. It offers a range of tools that simplify the integration of AI capabilities into applications, including managed services for data labeling, model training, and model deployment.
4.2.2. Microsoft Azure AI
Microsoft Azure AI is a comprehensive cloud platform offering a wide range of AI services, including machine learning, knowledge mining, and cognitive services. Azure AI helps businesses scale and improve their operations by integrating AI solutions into their workflows, providing tools for data analysis, predictive modeling, and natural language processing.
4.3. AI-Enabled Chips
AI-enabled chips are specialized hardware designed to accelerate AI computations, particularly in tasks that require substantial processing power.
4.3.1. GPUs for AI Processing
Graphics Processing Units (GPUs) are critical for AI processing, especially in training deep learning models. GPUs provide parallel processing capabilities, which significantly speed up the computation time required for handling large and complex datasets. This parallelism is essential for tasks such as image recognition, natural language processing, and neural network training.
4.3.2. Custom AI Chips like Google's TPU
Custom AI chips, such as Google's Tensor Processing Unit (TPU), are designed specifically for high-speed machine learning operations. TPUs are optimized for processing large amounts of data and performing high-volume computations efficiently. These custom chips enhance the performance of AI applications, making them ideal for intensive machine learning tasks.
5. Use Cases of AI in Various Industries
AI is transforming various industries by providing innovative solutions that enhance efficiency, accuracy, and customer satisfaction. This section highlights some key use cases of AI across different sectors.
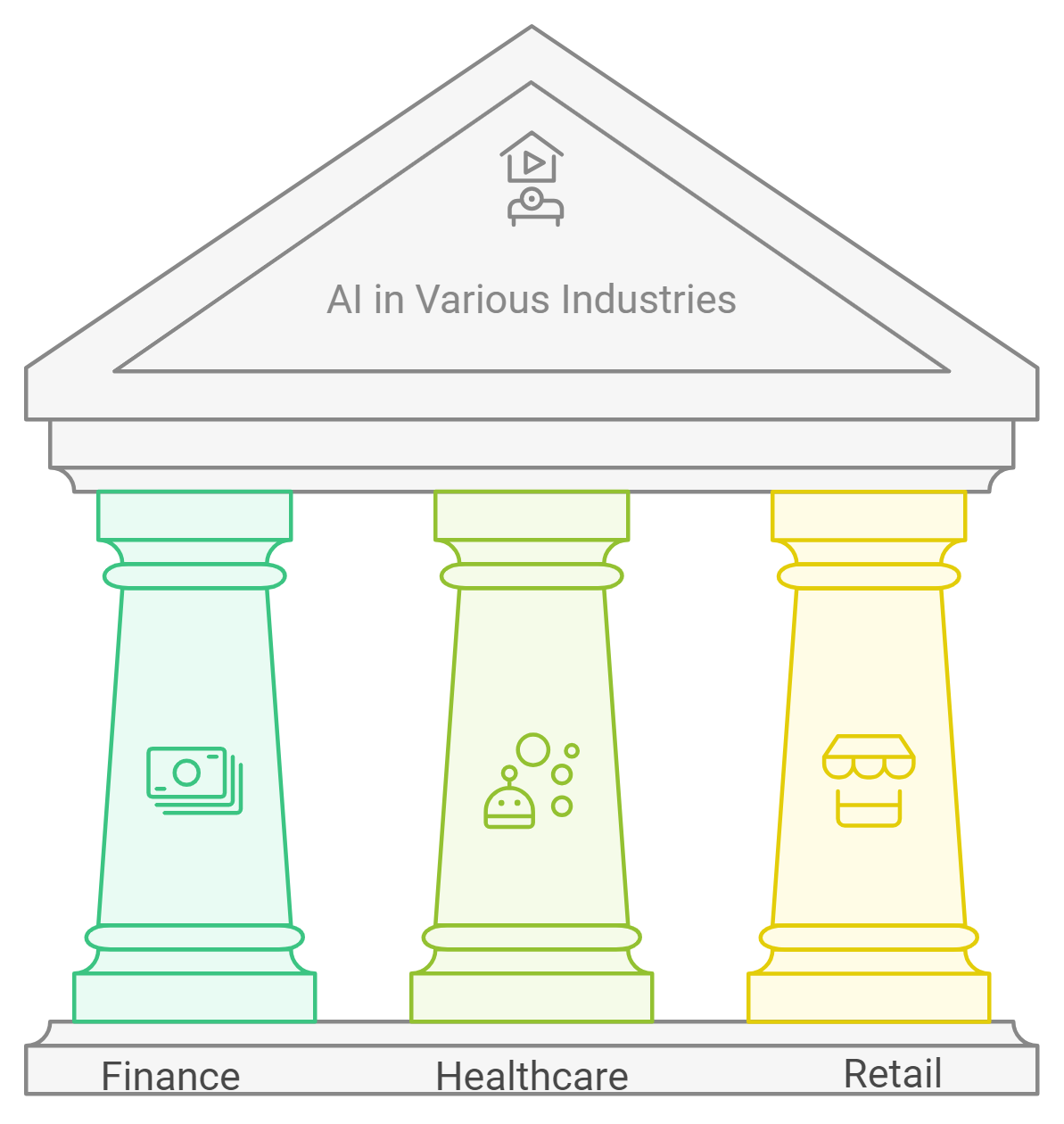
‍
5.1. Healthcare
5.1.1. Predictive Analytics
In healthcare, predictive analytics uses AI to analyze medical data and predict trends and patient outcomes. This helps in early diagnosis and preventive healthcare, significantly improving patient care and reducing costs.
5.1.2. Robot-Assisted Surgery
Robot-assisted surgery integrates AI to enhance the precision and effectiveness of surgical procedures. These robots can perform complex surgeries with minimal invasiveness, which reduces recovery time and improves surgical outcomes.
5.2. Finance
5.2.1. Algorithmic Trading
AI drives algorithmic trading by using complex mathematical models to make high-speed trading decisions. This technology enables traders to achieve better market performance and risk management. Learn more about Algorithmic Trading: Leveraging AI & ML in Finance.
5.2.2. Fraud Detection
AI enhances fraud detection in finance by analyzing transaction patterns and identifying anomalies that may indicate fraudulent activities. This proactive approach helps in minimizing losses and improving the security of financial transactions.
5.3. Retail
5.3.1. Customer Recommendation Systems
AI-powered recommendation systems in retail analyze customer data and shopping behavior to suggest products that are more likely to be of interest to the customer. This personalization enhances the shopping experience and boosts sales.
5.3.2. Inventory Management
AI in inventory management helps retailers maintain optimal stock levels by predicting demand trends and automating restocking processes. This reduces the risk of overstocking or stockouts, ensuring efficient operations.
6. Challenges in AI Implementation
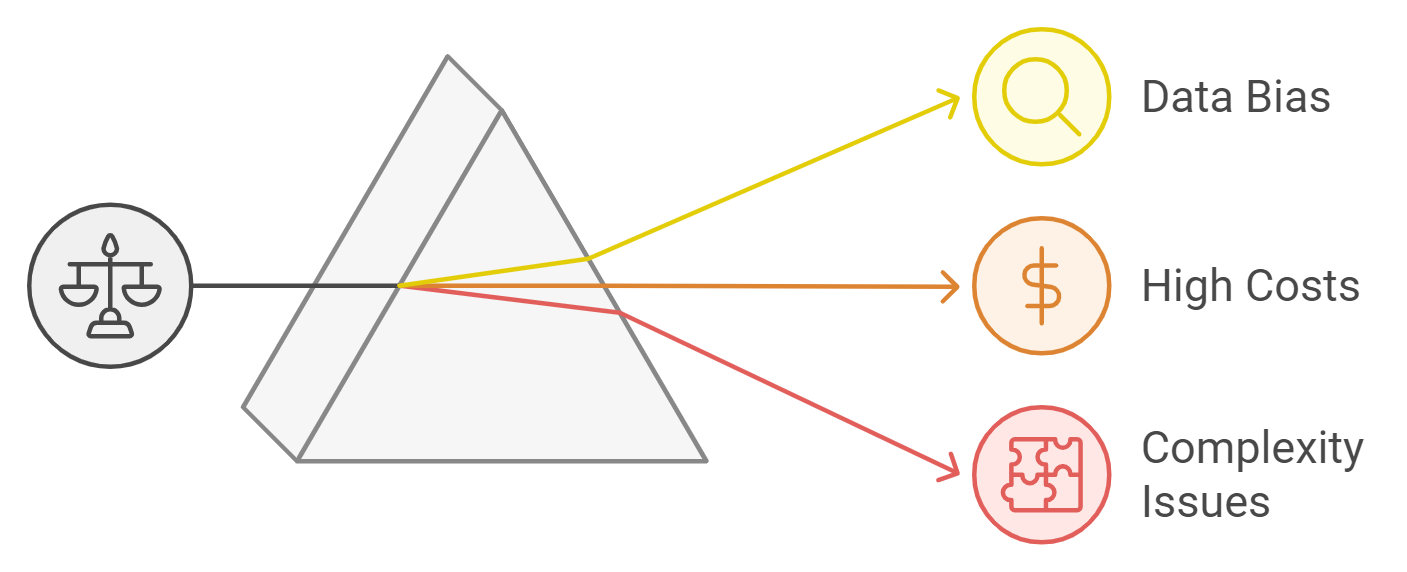
‍
6.1. Ethical and Privacy Concerns
The implementation of AI raises significant ethical and privacy concerns, including the potential for surveillance and the misuse of personal data. Ensuring transparency and adhering to ethical guidelines is crucial for maintaining public trust in AI technologies.
6.2. Data Bias and Quality Issues
AI systems are only as good as the data they are trained on. Data bias and quality issues can lead to inaccurate or unfair AI outcomes, highlighting the need for diverse and high-quality training datasets.
6.3. High Costs and Complexity of AI Systems
The high costs and complexity associated with developing and maintaining AI systems can be a barrier for many organizations. This includes the need for specialized hardware, software, and skilled personnel to manage AI operations.
7. The Future of AI
7.1. Trends and Predictions
The future of AI is poised for exponential growth with advancements in computing power and data availability. Predictions suggest that AI will become more integrated into daily life, enhancing personal and professional tasks with greater efficiency and precision. Trends indicate a shift towards more ethical AI, focusing on transparency and fairness to mitigate biases inherent in training data. For more insights, explore The Future of AI: Generative Integration.
7.2. Potential for New AI Applications
AI's potential extends into virtually every sector, including healthcare, finance, and education. In healthcare, AI could revolutionize patient care through personalized medicine and early diagnosis. In finance, AI applications in algorithmic trading and fraud detection are becoming more sophisticated. Education will see AI personalize learning experiences, adapting to individual student needs and pace. Discover more about AI in education.
8. Real-World Examples of AI
8.1. AI in Autonomous Vehicles
AI plays a critical role in the development of autonomous vehicles. Through machine learning algorithms, these vehicles can interpret sensory data, enabling them to understand their surroundings and make real-time decisions. This technology promises to reduce accidents, improve traffic efficiency, and decrease carbon emissions.
8.2. AI in Smart Home Devices
Smart home devices use AI to learn from users' habits and preferences to automate tasks. Devices like smart thermostats adjust the temperature based on your routine, while smart speakers learn to recognize different voices and provide personalized responses. These devices not only offer convenience but also enhance energy efficiency and security.
9. In-depth Explanations
9.1. How AI Learns: An Overview of Machine Learning Algorithms
AI learns through machine learning algorithms, which allow it to improve its performance over time without being explicitly programmed. These algorithms can be categorized into supervised learning, where the model learns from labeled data; unsupervised learning, which finds patterns in data without labels; and reinforcement learning, where an agent learns to make decisions by receiving rewards or penalties.
9.2. The Role of Big Data in AI
Big data is crucial for the effectiveness of AI. The vast amounts of data generated daily provide the raw material for AI systems to learn and make informed decisions. Big data enables the identification of trends, patterns, and correlations that would be impossible for humans to detect, thereby enhancing the accuracy and applicability of AI solutions.
10. Comparisons & Contrasts
10.1. AI vs. Human Intelligence
Artificial Intelligence (AI) and human intelligence differ fundamentally in their operations. AI excels in processing large volumes of data quickly and performing tasks with consistent precision. In contrast, human intelligence offers the ability to think abstractly, apply emotional intelligence, and perform complex problem-solving that involves ethical considerations and moral judgments. For more insights, explore AI Ethics and Multimodal Learning: Future Integration.
10.2. AI in Different Sectors: Comparing Use Cases and Impact
AI's application varies significantly across different sectors, each demonstrating unique impacts and challenges. In healthcare, AI is used to predict patient diagnoses and outcomes, significantly improving treatment accuracy and patient care. In contrast, the finance sector utilizes AI for risk assessment, fraud detection, and customer service optimization, enhancing efficiency and security. Each sector benefits differently from AI technologies, tailored to specific needs and objectives. Learn more about AI & Blockchain Development Services for Healthcare Industry.
11. Why Choose Rapid Innovation for AI Implementation and Development
11.1. Expertise and Experience
Choosing a provider like Rapid Innovation for AI implementation leverages years of expertise and deep industry experience. This ensures that AI solutions are not only state-of-the-art but also aligned with current industry standards and future trends. Experienced professionals can navigate complex AI landscapes and deliver solutions that are both innovative and effective.
11.2. Custom AI Solutions Tailored to Client Needs
Rapid Innovation focuses on developing custom AI solutions that are specifically tailored to meet the unique needs of each client. This personalized approach ensures that the AI systems integrate seamlessly with existing processes and truly enhance business operations, rather than offering a one-size-fits-all solution.
11.3. Comprehensive Support and Maintenance
Post-implementation support and maintenance are crucial for the success of AI systems. Rapid Innovation provides comprehensive support and ongoing maintenance to ensure that AI solutions continue to operate efficiently and evolve with changing business needs and technological advancements.
12. Conclusion
12.1. Summary of AI's Impact and Potential
AI has revolutionized numerous industries by enhancing efficiency, accuracy, and productivity. Its potential to further transform businesses and everyday life remains vast, with ongoing advancements promising even greater impacts. AI's ability to analyze data and automate complex processes continues to be its strongest asset in various sectors.
12.2. Final Thoughts on the Evolution of AI
The evolution of AI is an ongoing journey marked by continuous improvement and innovation. As AI technologies become more sophisticated and accessible, their integration into various aspects of life and business will likely deepen, making AI an indispensable part of modern society. The future of AI holds exciting possibilities for further advancements that will continue to reshape how we live and work. Explore more about AI in Business: Automation & Innovation.
For more insights and services related to Artificial Intelligence, visit our AI Services Page or explore our Main Page for a full range of offerings.