Table Of Contents
Category
FinTech
Artificial Intelligence
Blockchain
1. What is Machine Learning's Role in Modern FinTech?
Machine learning (ML) is revolutionizing the financial technology (FinTech) sector by enabling companies to analyze vast amounts of data quickly and efficiently. This technology allows for the automation of processes, improved decision-making, and enhanced customer experiences.
- Predictive analytics: ML algorithms can analyze historical data to predict future trends, helping financial institutions make informed decisions. For instance, Rapid Innovation can implement predictive models that assist banks in forecasting loan defaults, thereby optimizing their lending strategies. This is a key aspect of machine learning in fintech.
- Fraud detection: Machine learning models can identify unusual patterns in transactions, significantly reducing the risk of fraud. Our solutions can help financial institutions deploy real-time fraud detection systems that adapt and learn from new data, ensuring robust security. This is crucial for fintech and machine learning applications.
- Personalized services: By analyzing customer data, ML can help tailor financial products and services to individual needs, enhancing customer satisfaction. Rapid Innovation can develop personalized recommendation engines that improve customer engagement and retention, showcasing the importance of machine learning and fintech.
- Risk management: ML can assess credit risk more accurately by analyzing a broader range of data points, leading to better lending decisions. Our expertise allows us to create advanced risk assessment tools that provide deeper insights into borrower profiles, a vital component of machine learning fintech companies.
1.1. How Big is the ML FinTech Market?
The machine learning market within the FinTech sector is experiencing rapid growth. According to various reports, the market is projected to reach significant figures in the coming years.
- Growth Rate: The global machine learning market in FinTech is expected to grow at a compound annual growth rate (CAGR) of around 30% from 2021 to 2028.
- Market Size: By 2025, the market size is anticipated to exceed $10 billion, driven by increasing demand for automation and data-driven decision-making.
- Investment: Major players in the FinTech space are investing heavily in ML technologies to stay competitive and meet evolving customer expectations, particularly in areas like deep learning in fintech.
1.2. What Impact is ML Having on Financial Services?
Machine learning is having a profound impact on various aspects of financial services, transforming how institutions operate and interact with customers.
- Enhanced customer experience: ML algorithms analyze customer behavior to provide personalized recommendations, improving user engagement and satisfaction. Rapid Innovation can help implement these algorithms to create a seamless customer journey, a key benefit of machine learning applications in fintech.
- Improved operational efficiency: Automation of routine tasks through ML reduces human error and operational costs, allowing financial institutions to focus on strategic initiatives. Our solutions can streamline back-office operations, leading to significant cost savings.
- Advanced risk assessment: ML models can evaluate creditworthiness more accurately by considering non-traditional data sources, leading to better lending practices. We can assist in developing models that incorporate diverse data sets for more comprehensive risk evaluations, which is essential for fintech and machine learning.
- Regulatory compliance: ML can help financial institutions comply with regulations by automating reporting processes and monitoring transactions for suspicious activities. Rapid Innovation can create compliance solutions that leverage ML to ensure adherence to regulatory standards.
- Investment strategies: ML is used in algorithmic trading to analyze market trends and execute trades at optimal times, maximizing returns for investors. Our expertise in developing trading algorithms can help clients achieve superior investment performance, highlighting the role of AI and machine learning in fintech.
The integration of machine learning in financial services is not just a trend; it is becoming a necessity for companies aiming to thrive in a competitive landscape. Rapid Innovation is committed to helping clients leverage ML technologies to achieve greater ROI and drive business success, particularly through innovative fintech machine learning applications. For more information on how we can assist you, check out our MLOps consulting services and learn about the top 10 use cases to rely on ML model engineering services.
Refer to the image for a visual representation of Machine Learning's role in Modern FinTech:

1.3. Key Technology Drivers and Trends
The financial technology (FinTech) sector is rapidly evolving, driven by several key technology trends and innovations. These drivers are reshaping how financial services are delivered and consumed.
- Artificial Intelligence (AI) and Machine Learning (ML): AI and ML are at the forefront of FinTech innovation. They enable predictive analytics, risk assessment, and personalized customer experiences. According to a report, the global AI in FinTech market is expected to reach $22.6 billion by 2025. Rapid Innovation leverages AI and ML to help clients optimize their operations, enhance customer engagement, and achieve greater ROI through data-driven decision-making.
- Blockchain Technology: Blockchain offers enhanced security and transparency in transactions. It is being used for everything from cross-border payments to smart contracts, reducing fraud and operational costs. Rapid Innovation specializes in blockchain solutions that empower clients to streamline their processes and build trust with their customers.
- Regulatory Technology (RegTech): As regulations become more complex, RegTech solutions help companies comply efficiently. These technologies automate compliance processes, reducing the risk of human error and ensuring adherence to regulations. Rapid Innovation provides consulting and development services to implement RegTech solutions that minimize compliance risks and enhance operational efficiency.
- Digital Payments: The shift towards cashless transactions is accelerating. Mobile wallets, contactless payments, and cryptocurrencies are becoming mainstream, driven by consumer demand for convenience and speed. Rapid Innovation assists clients in adopting digital payment solutions that cater to evolving consumer preferences, ultimately driving revenue growth.
- Cloud Computing: Cloud technology allows FinTech companies to scale operations quickly and cost-effectively. It provides the infrastructure needed for data storage, processing, and analytics, enabling firms to innovate rapidly. Rapid Innovation helps clients migrate to cloud-based solutions, enhancing their agility and reducing operational costs.
- Cybersecurity: With the rise of digital transactions, cybersecurity has become paramount. Advanced security measures, including biometric authentication and encryption, are essential to protect sensitive financial data. Rapid Innovation offers robust cybersecurity solutions that safeguard client data and build customer trust.
- Open Banking: Open banking initiatives encourage collaboration between banks and third-party providers. This trend fosters innovation and competition, leading to better services for consumers. Rapid Innovation supports clients in navigating the open banking landscape, enabling them to create innovative financial products and services.
- FinTech Trends: The landscape of financial technology is continuously evolving, with emerging fintech trends shaping the future of the industry. Current fintech trends highlight the importance of adapting to new technologies and consumer demands, while gartner fintech trends provide insights into the strategic direction of the sector. Emerging trends in financial technology emphasize the need for innovation and agility in response to market changes.
2. What Are the Key Use Cases of ML in FinTech?
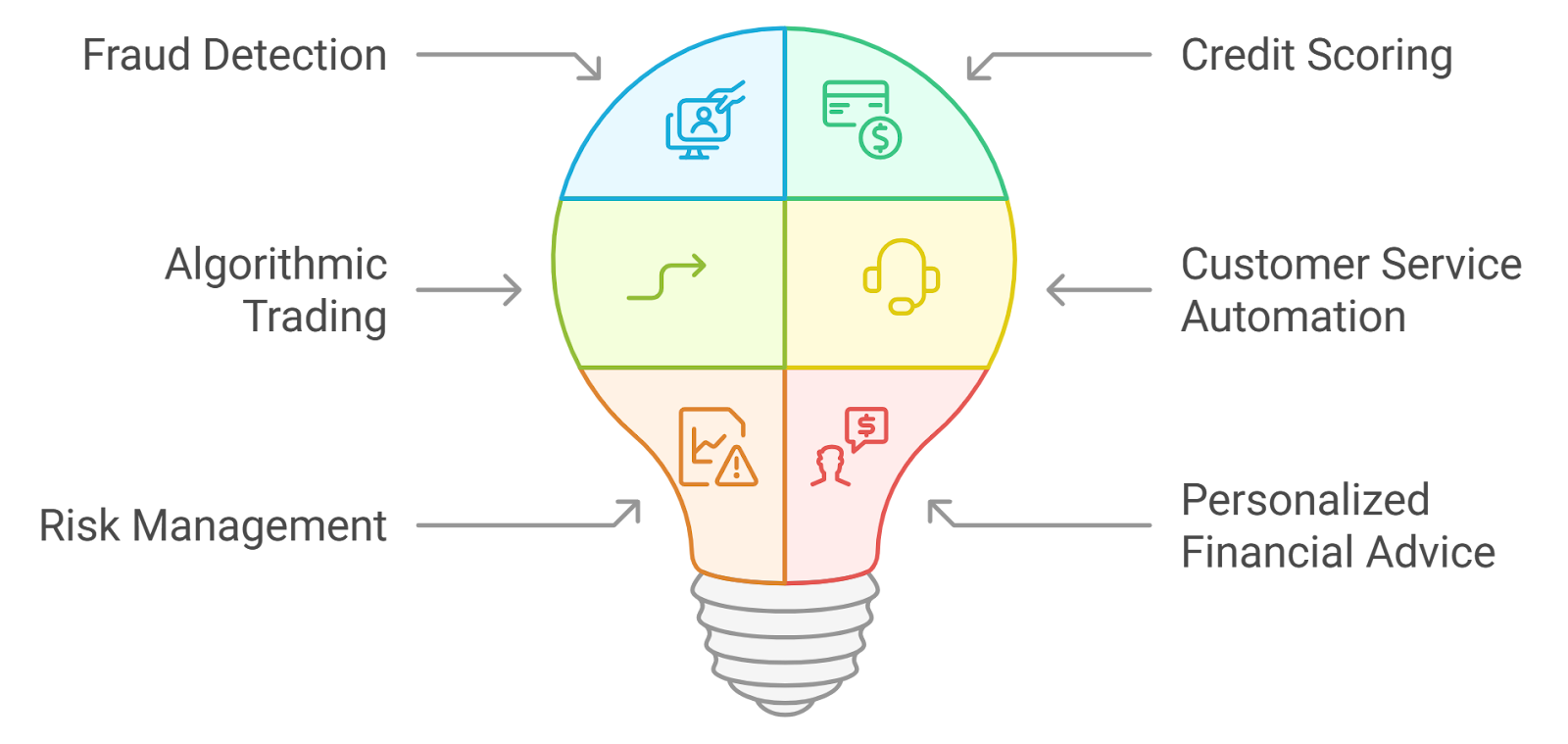
Machine Learning (ML) is transforming the FinTech landscape by enabling more efficient processes and better decision-making. Here are some of the key use cases of ML in this sector:
- Fraud Detection and Prevention: ML algorithms analyze transaction patterns to identify anomalies that may indicate fraudulent activity. By learning from historical data, these systems can adapt and improve over time, significantly reducing false positives. Rapid Innovation implements ML-driven fraud detection systems that enhance security and reduce losses for clients.
- Credit Scoring: Traditional credit scoring models often overlook valuable data. ML models can incorporate a wider range of data points, including social media activity and transaction history, to provide a more accurate assessment of creditworthiness. Rapid Innovation helps clients develop advanced credit scoring models that improve lending decisions and expand customer access to credit.
- Algorithmic Trading: ML is used to develop trading algorithms that analyze market data in real-time. These algorithms can execute trades at optimal times, maximizing returns and minimizing risks. Rapid Innovation collaborates with clients to create tailored algorithmic trading solutions that enhance investment strategies.
- Customer Service Automation: Chatbots powered by ML can handle customer inquiries efficiently, providing instant responses and freeing up human agents for more complex issues. This enhances customer satisfaction and reduces operational costs. Rapid Innovation designs and implements AI-driven customer service solutions that improve client interactions and operational efficiency.
- Risk Management: ML models can predict potential risks by analyzing various factors, including market trends and economic indicators. This allows financial institutions to make informed decisions and mitigate risks effectively. Rapid Innovation assists clients in developing risk management frameworks that leverage ML for better decision-making.
- Personalized Financial Advice: ML can analyze individual spending habits and financial goals to offer tailored advice. This personalization enhances customer engagement and loyalty. Rapid Innovation helps clients create personalized financial advisory services that drive customer satisfaction and retention.
2.1. Understanding the Application Landscape
The application landscape of ML in FinTech is diverse, encompassing various sectors and functionalities. Understanding this landscape is crucial for stakeholders looking to leverage ML technologies effectively.
- Payments: ML enhances payment processing by detecting fraud and optimizing transaction flows. It helps in real-time risk assessment, ensuring secure and efficient transactions. Rapid Innovation provides ML solutions that improve payment security and efficiency for clients.
- Lending: In the lending sector, ML models assess borrower risk more accurately. They analyze a broader range of data, enabling lenders to make better-informed decisions and offer competitive rates. Rapid Innovation supports clients in developing ML-driven lending solutions that enhance risk assessment and customer experience.
- Insurance: ML is revolutionizing underwriting processes in insurance. By analyzing vast amounts of data, insurers can assess risk more accurately and tailor policies to individual customers. Rapid Innovation collaborates with insurance clients to implement ML solutions that optimize underwriting and claims processing.
- Investment Management: ML algorithms analyze market trends and investor behavior to optimize portfolio management. They can identify investment opportunities and automate trading strategies. Rapid Innovation partners with investment firms to develop ML-driven tools that enhance portfolio performance.
- Regulatory Compliance: ML tools assist in monitoring transactions for compliance with regulations. They can flag suspicious activities and ensure adherence to legal requirements, reducing the burden on compliance teams. Rapid Innovation offers RegTech solutions that streamline compliance processes for clients.
- Customer Insights: Financial institutions use ML to gain insights into customer behavior. This data-driven approach helps in developing targeted marketing strategies and improving customer retention. Rapid Innovation helps clients harness customer data to drive marketing effectiveness and enhance customer loyalty.
- Financial Forecasting: ML models can predict market trends and economic shifts, aiding financial institutions in strategic planning and investment decisions. Rapid Innovation provides forecasting solutions that empower clients to make informed strategic choices.
By understanding these applications, FinTech companies can harness the power of ML to drive innovation, improve efficiency, and enhance customer experiences. Rapid Innovation is committed to helping clients navigate this landscape, ensuring they achieve their business goals efficiently and effectively.
Refer to the image for a visual representation of the key technology drivers and trends in the FinTech sector.
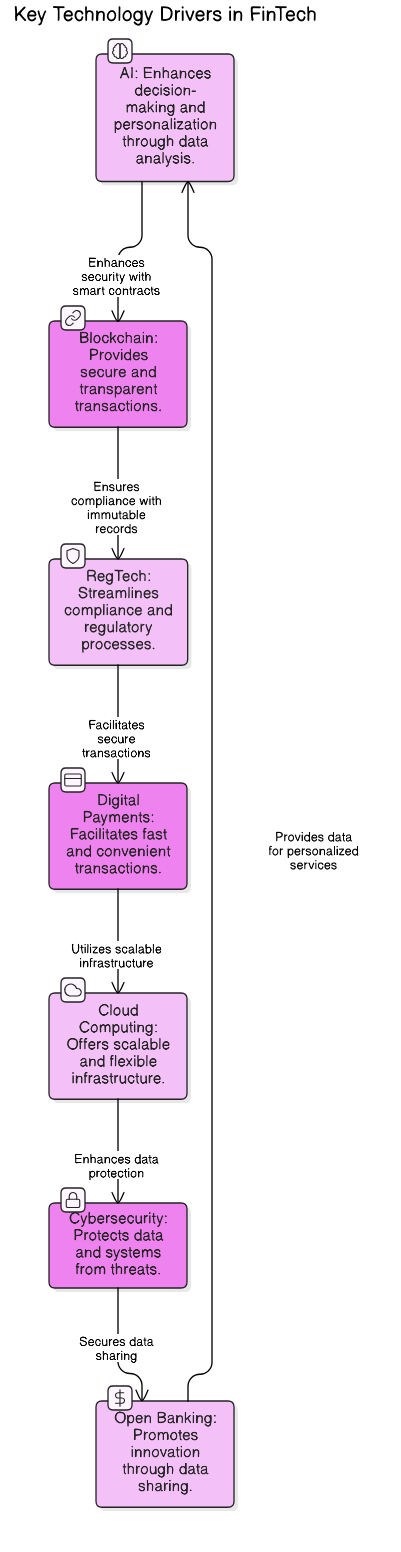
2.2. Which Industries Lead in ML Implementation?
Machine Learning (ML) is transforming various sectors by enhancing efficiency, accuracy, and decision-making processes. The following industries are at the forefront of ML implementation:
- Healthcare: ML algorithms analyze vast amounts of medical data to assist in diagnostics and treatment recommendations. Predictive analytics help in patient management and resource allocation, enabling healthcare providers to deliver personalized care and improve patient outcomes.
- Finance: Financial institutions use ML for credit scoring, risk assessment, and fraud detection. Algorithms analyze transaction patterns to identify anomalies and prevent fraudulent activities, thereby safeguarding assets and enhancing customer trust.
- Retail: Retailers leverage ML for personalized marketing, inventory management, and customer service. Recommendation systems enhance user experience by suggesting products based on past behavior, leading to increased sales and customer loyalty.
- Manufacturing: Predictive maintenance powered by ML reduces downtime and operational costs. Quality control processes are improved through real-time data analysis, ensuring higher product quality and efficiency in production lines.
- Transportation: ML optimizes route planning and logistics, improving delivery times and reducing costs. Autonomous vehicles rely heavily on ML for navigation and safety, paving the way for innovative transportation solutions.
- Telecommunications: Companies use ML for network optimization and predictive maintenance. Customer service chatbots enhance user experience and reduce operational costs, allowing for more efficient customer interactions.
These industries are leading the charge in ML implementation, showcasing the technology's versatility and potential to drive innovation. Additionally, various organizations are exploring specific implementations such as knn implementation python, knn classifier implementation python, and implementing machine learning algorithms from scratch to enhance their capabilities. For expert guidance in these areas, consider partnering with an AI consulting company like Rapid Innovation.
2.3. Implementation Success Factors
Successful implementation of Machine Learning requires careful consideration of several key factors:
- Data Quality: High-quality, relevant data is crucial for training effective ML models. Organizations must ensure data is clean, structured, and representative of real-world scenarios.
- Skilled Workforce: A team with expertise in data science, statistics, and domain knowledge is essential. Continuous training and upskilling help keep the workforce updated with the latest ML trends, including knowledge of knn algorithm implementation in python and gradient boosting implementation python.
- Clear Objectives: Defining clear goals and use cases for ML projects helps in aligning efforts and measuring success. Organizations should focus on specific problems that ML can solve effectively, such as implementing knn in python or naive bayes implementation in python.
- Infrastructure: Robust IT infrastructure is necessary to support data storage, processing, and model deployment. Cloud computing solutions can provide scalable resources for ML applications, facilitating implementations like random forest implementation and gradient descent implementation.
- Collaboration: Cross-departmental collaboration fosters a culture of innovation and knowledge sharing. Engaging stakeholders from various functions ensures that ML initiatives align with business objectives.
- Iterative Approach: An iterative development process allows for continuous improvement and adaptation of ML models. Regular feedback loops help refine algorithms based on real-world performance, which is essential for successful machine learning implementation.
By focusing on these success factors, organizations can enhance their chances of effectively implementing Machine Learning solutions, including various algorithms and techniques.
3. Fraud Detection and Prevention Systems
Fraud detection and prevention systems are critical in safeguarding businesses and consumers from financial losses. Machine Learning plays a pivotal role in enhancing these systems through various methodologies:
- Anomaly Detection: ML algorithms analyze transaction patterns to identify unusual behavior that may indicate fraud. Techniques such as clustering and classification help in distinguishing between legitimate and fraudulent activities.
- Predictive Modeling: Predictive models assess the likelihood of fraud based on historical data. These models can adapt over time, improving their accuracy as new data becomes available.
- Real-time Monitoring: ML systems can process transactions in real-time, allowing for immediate alerts and actions. This capability is crucial for minimizing losses and preventing fraudulent transactions before they occur.
- Natural Language Processing (NLP): NLP techniques analyze unstructured data, such as customer communications, to detect potential fraud indicators. Sentiment analysis can also help identify suspicious behavior in customer interactions.
- Behavioral Analytics: ML models track user behavior to establish a baseline and detect deviations that may signal fraud. This approach enhances the accuracy of fraud detection by considering individual user patterns.
- Integration with Existing Systems: Effective fraud detection systems must integrate seamlessly with existing financial and operational systems. This integration ensures a holistic view of transactions and enhances the overall security framework.
By leveraging Machine Learning in fraud detection and prevention systems, organizations can significantly reduce the risk of financial fraud and enhance their security measures. At Rapid Innovation, we specialize in developing tailored ML solutions that align with your business goals, ensuring greater ROI and operational efficiency, including implementations like federated learning implementation and mlops implementation.
Refer to the image for a visual representation of the industries leading in Machine Learning implementation.

3.1. Real-time Transaction Monitoring
Real-time transaction monitoring is a critical component in the fight against financial crime. It involves the continuous analysis of transactions as they occur, allowing financial institutions to detect suspicious activities immediately. This system enables an immediate response to potential fraud or money laundering activities, utilizing advanced technologies such as machine learning and artificial intelligence to analyze transaction data. By ensuring compliance with regulatory requirements, it effectively reduces the risk of penalties. Additionally, it provides a comprehensive view of customer behavior, enhancing risk assessment and facilitating the identification of trends and patterns that may indicate illicit activities.
At Rapid Innovation, we leverage our expertise in AI to implement robust real-time transaction monitoring systems tailored to the specific needs of financial institutions. This proactive approach not only protects the institution but also safeguards customers from potential fraud, ultimately leading to greater ROI through reduced losses and enhanced customer trust. For more information on our services, visit our AI banking solutions and learn more about generative AI in finance and banking applications.
3.2. Pattern Recognition in Financial Crime
Pattern recognition plays a vital role in identifying and preventing financial crime. By analyzing historical data, institutions can uncover patterns that may indicate fraudulent behavior. This process involves the use of algorithms to detect recurring behaviors associated with financial crimes, helping to distinguish between legitimate transactions and those that are potentially harmful. It can also identify complex schemes, such as Ponzi or pyramid schemes, by recognizing unusual transaction patterns. Furthermore, it enhances the ability to predict future fraudulent activities based on past behaviors and supports the development of risk profiles for customers, allowing for more targeted monitoring.
At Rapid Innovation, we harness the power of AI-driven pattern recognition technologies to enable financial organizations to process vast amounts of data quickly. This capability allows institutions to stay ahead of criminals, significantly reducing their exposure to risk and enhancing their overall operational efficiency.
3.3. Anomaly Detection Algorithms
Anomaly detection algorithms are essential tools in the realm of financial crime prevention. These algorithms are designed to identify deviations from expected behavior, which can signal potential fraud or other illicit activities. They operate by establishing a baseline of normal transaction behavior for individuals or entities and flagging transactions that fall outside this baseline for further investigation. These algorithms utilize various techniques, including statistical analysis, machine learning, and deep learning, to enhance detection capabilities. They can adapt over time, learning from new data to improve accuracy and reduce false positives, and provide insights that help in refining risk management strategies and compliance efforts.
The implementation of anomaly detection algorithms at Rapid Innovation allows financial institutions to maintain a robust defense against evolving threats. By continuously monitoring and analyzing transaction data, these algorithms help ensure that suspicious activities are identified and addressed promptly, ultimately contributing to a more secure financial environment and improved ROI for our clients.
Refer to the image for a visual representation of real-time transaction monitoring and its components.

3.4. What Cost Benefits Does ML Security Provide?
Machine Learning (ML) security offers numerous cost benefits that can significantly enhance an organization's financial efficiency. By leveraging advanced algorithms and data analysis, businesses can reduce expenses related to security breaches and improve overall operational efficiency.
- Reduced Incident Response Costs: ML security systems can detect threats in real-time, allowing for quicker responses to potential breaches. This minimizes the financial impact of incidents, which can be substantial. According to a report, the average cost of a data breach is around $4.24 million.
- Lower False Positive Rates: Traditional security systems often generate numerous false positives, leading to wasted resources and time. ML algorithms can learn from past data, improving their accuracy and reducing the number of false alarms. This efficiency translates to lower operational costs.
- Automated Threat Detection: By automating the threat detection process, organizations can reduce the need for extensive human resources dedicated to monitoring and responding to security threats. This not only cuts labor costs but also allows skilled personnel to focus on more strategic tasks.
- Enhanced Risk Management: ML security tools can analyze vast amounts of data to identify vulnerabilities and predict potential threats. This proactive approach helps organizations allocate resources more effectively, reducing the likelihood of costly breaches.
- Scalability: As businesses grow, their security needs evolve. ML security solutions can easily scale to accommodate increased data and user activity without a proportional increase in costs, making them a cost-effective choice for expanding organizations. For more information on how to implement these solutions, check out our AI Business Automation Solutions and learn about algorithmic trading leveraging AI and ML in finance.
4. Trading and Portfolio Management
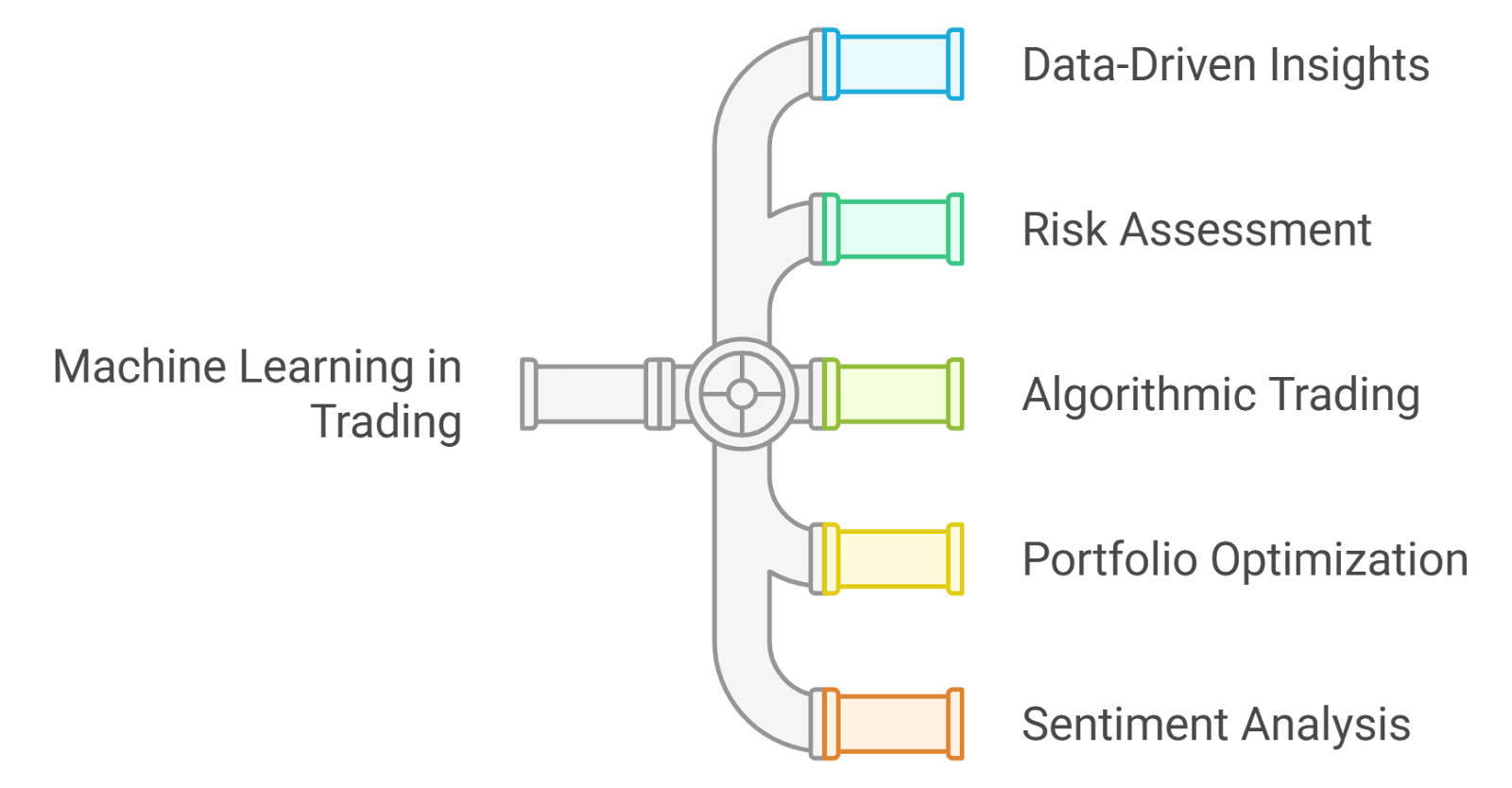
Trading and portfolio management have been revolutionized by the integration of technology, particularly through the use of machine learning. These advancements enable traders and investors to make more informed decisions, optimize their strategies, and manage risks effectively.
- Data-Driven Insights: ML algorithms analyze vast datasets to uncover patterns and trends that human traders might miss. This data-driven approach allows for more accurate predictions and better-informed trading decisions.
- Risk Assessment: Machine learning models can assess the risk associated with various investment options by analyzing historical data and market conditions. This helps portfolio managers to make informed choices that align with their risk tolerance.
- Algorithmic Trading: The use of algorithms in trading has become increasingly popular. ML enhances these algorithms by allowing them to adapt to changing market conditions, improving their performance over time.
- Portfolio Optimization: ML techniques can optimize asset allocation by analyzing correlations between different assets. This leads to more balanced portfolios that can withstand market volatility.
- Sentiment Analysis: ML can analyze news articles, social media, and other sources to gauge market sentiment. This information can be invaluable for traders looking to anticipate market movements.
4.1. High-Frequency Trading Systems
High-frequency trading (HFT) systems utilize advanced algorithms to execute a large number of orders at extremely high speeds. Machine learning plays a crucial role in enhancing the effectiveness of these systems.
- Speed and Efficiency: HFT systems rely on speed to capitalize on market inefficiencies. ML algorithms can process vast amounts of data in milliseconds, allowing traders to make split-second decisions that can lead to significant profits.
- Predictive Analytics: Machine learning models can predict short-term price movements by analyzing historical data and identifying patterns. This predictive capability is essential for HFT strategies that depend on rapid execution.
- Market Microstructure Analysis: ML can analyze the microstructure of markets, including order book dynamics and trade execution patterns. This analysis helps traders understand market behavior and optimize their trading strategies accordingly.
- Risk Management: HFT systems face unique risks, including market volatility and execution risks. ML can help identify and mitigate these risks by continuously monitoring market conditions and adjusting trading strategies in real-time.
- Adaptive Algorithms: One of the key advantages of ML in HFT is the ability to create adaptive algorithms that learn from market changes. These algorithms can adjust their strategies based on new data, improving their performance over time.
- Cost Efficiency: By automating trading processes and reducing the need for human intervention, HFT systems can lower operational costs. This efficiency is crucial in a competitive trading environment where margins are often thin.
At Rapid Innovation, we harness the power of ML and blockchain technologies to provide tailored solutions that help organizations achieve their business goals efficiently and effectively. By integrating these advanced technologies, we enable our clients to enhance their security measures, optimize trading strategies, and ultimately achieve greater ROI.
4.2. Market Prediction Models
Market prediction models are essential tools used by investors and analysts to forecast future market trends and price movements. These models leverage historical data, statistical techniques, and machine learning algorithms to provide insights into potential market behavior.
- Types of Models:
- Time Series Analysis: This involves analyzing historical price data to identify patterns and trends. Techniques like ARIMA (AutoRegressive Integrated Moving Average) and ARIMA for stock price prediction are commonly used.
- Regression Analysis: This method examines the relationship between different variables, such as economic indicators and stock prices, to predict future movements.
- Machine Learning Models: Advanced algorithms, including neural networks, convolutional neural networks for stock prediction, and decision trees, are increasingly used to analyze vast datasets and improve prediction accuracy.
At Rapid Innovation, we harness the power of AI-driven market prediction models, such as lstm stock price prediction and rnn stock market prediction, to help our clients make informed investment decisions. By utilizing machine learning algorithms, we can analyze extensive datasets, enabling our clients to identify trends and opportunities that may not be immediately apparent. This leads to more accurate forecasts and ultimately greater ROI. For more insights on predictive analytics in finance, visit this link.
- Key Factors Influencing Predictions:
- Economic Indicators: GDP growth, unemployment rates, and inflation can significantly impact market trends.
- Market Sentiment: Investor sentiment, often gauged through surveys and social media analysis, can influence market movements.
- Global Events: Political instability, natural disasters, and other global events can lead to sudden market shifts.
- Limitations:
- Data Quality: The accuracy of predictions heavily relies on the quality and relevance of the data used.
- Market Volatility: Sudden changes in market conditions can render models ineffective.
- Overfitting: Complex models, such as deep neural network stock prediction, may perform well on historical data but fail to predict future trends accurately.
4.3. Risk Assessment Frameworks
Risk assessment frameworks are structured approaches used to identify, analyze, and mitigate risks associated with investments. These frameworks help investors make informed decisions by evaluating potential threats to their portfolios.
- Components of Risk Assessment:
- Risk Identification: Recognizing potential risks, including market risk, credit risk, operational risk, and liquidity risk.
- Risk Analysis: Evaluating the likelihood and impact of identified risks. This can involve quantitative methods, such as Value at Risk (VaR), and qualitative assessments.
- Risk Mitigation: Developing strategies to minimize or eliminate risks. This may include diversification, hedging, and insurance.
At Rapid Innovation, we implement robust risk assessment frameworks that integrate AI and blockchain technologies. By utilizing AI for predictive analytics, we can identify potential risks more accurately, while blockchain ensures data integrity and transparency in risk management processes.
- Common Frameworks:
- COSO Framework: Focuses on enterprise risk management and integrates risk management with organizational strategy.
- ISO 31000: Provides guidelines for risk management principles and processes applicable to any organization.
- Basel Accords: A set of international banking regulations that establish risk management standards for financial institutions.
- Importance of Risk Assessment:
- Informed Decision-Making: Helps investors understand potential downsides and make better investment choices.
- Regulatory Compliance: Ensures adherence to legal and regulatory requirements, reducing the risk of penalties.
- Enhanced Resilience: Organizations that effectively assess risks are better equipped to withstand market fluctuations and crises.
4.4. Portfolio Optimization Strategies
Portfolio optimization strategies aim to maximize returns while minimizing risk through the strategic allocation of assets. These strategies are crucial for investors seeking to achieve their financial goals.
- Key Concepts:
- Modern Portfolio Theory (MPT): Developed by Harry Markowitz, MPT emphasizes the importance of diversification to reduce risk. It suggests that a well-diversified portfolio can achieve higher returns for a given level of risk.
- Efficient Frontier: A graphical representation of optimal portfolios that offer the highest expected return for a defined level of risk.
- Asset Allocation: The process of dividing investments among different asset categories, such as stocks, bonds, and real estate, to balance risk and reward.
- Optimization Techniques:
- Mean-Variance Optimization: A mathematical approach that seeks to find the best possible portfolio by maximizing expected returns for a given level of risk.
- Black-Litterman Model: Combines market equilibrium with investor views to create a more customized asset allocation.
- Dynamic Asset Allocation: Adjusts the portfolio in response to changing market conditions, allowing for greater flexibility and responsiveness.
At Rapid Innovation, we employ advanced portfolio optimization techniques powered by AI, including artificial neural network stock prediction and lstm to predict stock prices, to enhance our clients' investment strategies. By analyzing market data and trends, we help clients achieve a well-balanced portfolio that aligns with their financial objectives and risk tolerance.
- Benefits of Portfolio Optimization:
- Risk Management: Helps in identifying the optimal mix of assets to minimize risk exposure.
- Performance Enhancement: A well-optimized portfolio can lead to improved returns over time.
- Goal Alignment: Ensures that the investment strategy aligns with the investor's financial objectives and risk tolerance.
5. Credit Scoring and Risk Assessment
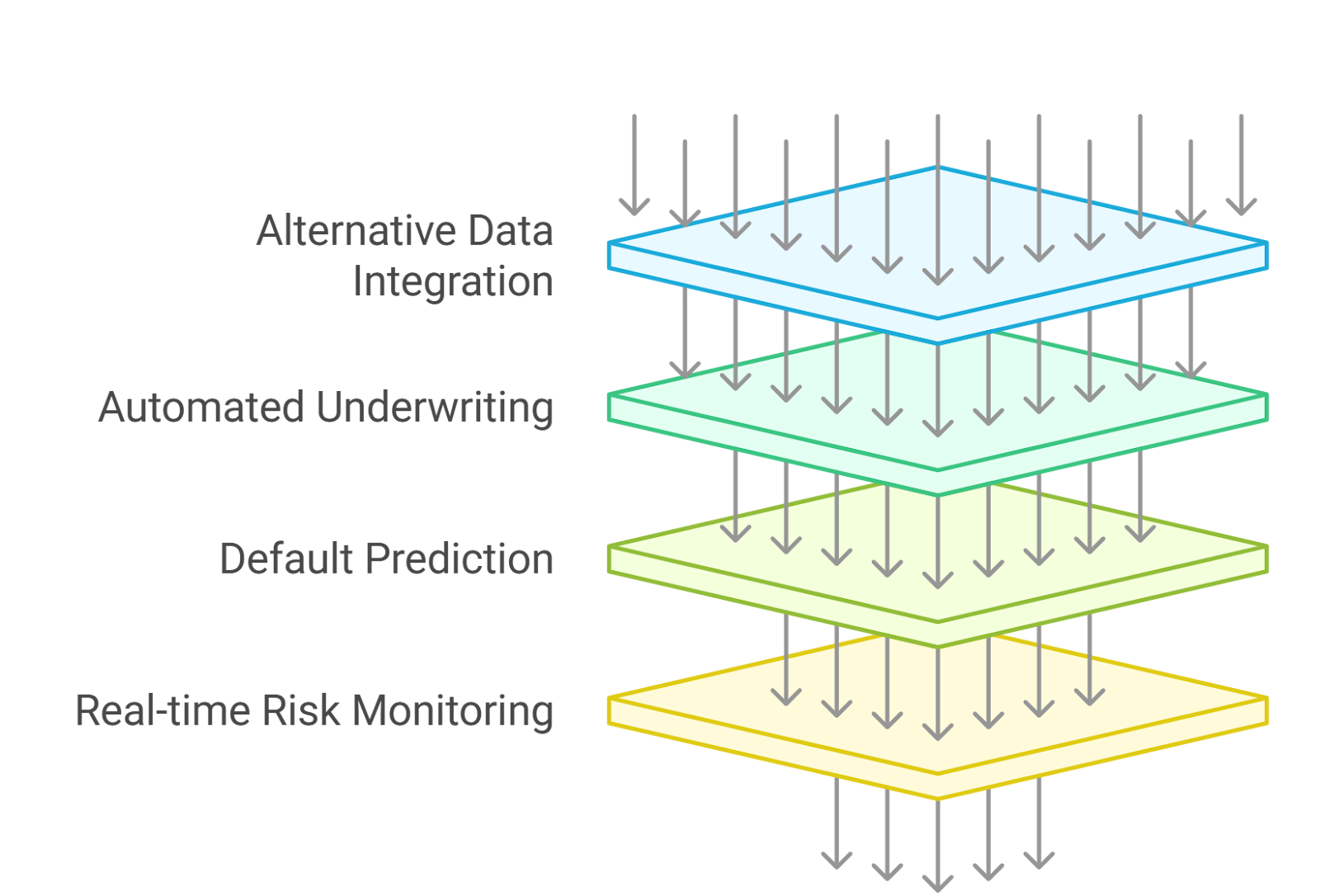
Credit scoring and risk assessment are critical components of the lending process. They help lenders evaluate the creditworthiness of potential borrowers, ensuring that they make informed decisions. The traditional credit scoring models primarily rely on historical credit data, but the landscape is evolving with the introduction of alternative data and automated underwriting processes.
5.1. Alternative Data in Credit Decisions
Alternative data refers to non-traditional information used to assess a borrower's creditworthiness. This data can provide a more comprehensive view of an individual's financial behavior, especially for those with limited credit histories.
- Types of alternative data include:
- Utility and rent payment histories
- Bank transaction data
- Employment and income information
- Social media activity
- Education and professional background
- Benefits of using alternative data:
- Expands access to credit for underbanked populations
- Provides a more accurate risk assessment by capturing real-time financial behavior
- Reduces reliance on traditional credit scores, which may not reflect current financial situations
- Challenges associated with alternative data:
- Privacy concerns regarding data collection and usage
- Potential biases in data interpretation
- Regulatory hurdles in integrating alternative data into credit scoring models
The use of alternative data is gaining traction among lenders, as it allows them to make more informed decisions and reach a broader audience. According to a report by the Consumer Financial Protection Bureau, incorporating alternative data can lead to a 20% increase in credit access for consumers with thin credit files.
At Rapid Innovation, we leverage our expertise in AI to help clients integrate alternative data into their credit scoring models. By utilizing machine learning algorithms, we can assist lenders in analyzing diverse data sources, leading to more accurate assessments and improved ROI.
5.2. Automated Underwriting Processes
Automated underwriting processes leverage technology to streamline the credit assessment and approval process. These systems use algorithms and machine learning to evaluate borrower information quickly and efficiently.
- Key features of automated underwriting:
- Real-time data analysis to assess creditworthiness
- Integration of alternative data sources for a holistic view
- Reduced processing time, often providing instant decisions
- Consistency in decision-making, minimizing human error
- Advantages of automated underwriting:
- Increased efficiency in loan processing, leading to faster approvals
- Enhanced accuracy in risk assessment through data-driven insights
- Lower operational costs for lenders, allowing for competitive interest rates
- Improved customer experience with quicker responses
- Considerations for automated underwriting:
- The need for robust data security measures to protect sensitive information
- Ensuring transparency in decision-making processes to build consumer trust
- Continuous monitoring and updating of algorithms to avoid biases
Automated underwriting is transforming the lending landscape, making it more accessible and efficient. As technology continues to advance, lenders are increasingly adopting these systems to enhance their credit scoring and risk assessment processes. Rapid Innovation is at the forefront of this transformation, providing tailored solutions that harness the power of AI to optimize underwriting processes, ultimately driving greater ROI for our clients.
In addition, the d&b supplier evaluation risk rating plays a crucial role in assessing the overall risk associated with potential borrowers, while credit worthiness evaluation further refines the understanding of a borrower's financial reliability. Together, these elements contribute to a more robust credit scoring and risk assessment framework. For more information on how we can assist with blockchain banking solutions, visit our Blockchain Banking Solutions and learn more about AI-based credit scoring use cases, types, and benefits.
5.3. Default Prediction Models
Default prediction models are essential tools in the financial sector, particularly for banks and lending institutions. These models help predict the likelihood of a borrower defaulting on a loan, enabling organizations to make informed lending decisions. One common approach is credit card default prediction using logistic regression, which estimates the probability of default based on various borrower characteristics.
- Types of Models:
- Logistic Regression: A statistical method that estimates the probability of default based on various borrower characteristics.
- Decision Trees: These models use a tree-like graph of decisions to classify borrowers based on their risk levels.
- Machine Learning Algorithms: Advanced techniques like Random Forests and Neural Networks can analyze large datasets to improve prediction accuracy.
- Key Factors Considered:
- Credit History: Past borrowing behavior is a strong indicator of future performance.
- Income Level: Higher income often correlates with a lower risk of default.
- Employment Stability: Job security can significantly impact a borrower's ability to repay.
- Benefits of Default Prediction Models:
- Improved Risk Assessment: Organizations can better identify high-risk borrowers.
- Enhanced Profitability: By reducing defaults, lenders can increase their overall profitability.
- Regulatory Compliance: Accurate models help institutions meet regulatory requirements regarding risk management.
At Rapid Innovation, we leverage our expertise in AI to develop sophisticated default prediction models tailored to your specific needs. By utilizing machine learning algorithms, we can enhance the accuracy of your risk assessments, ultimately leading to a greater return on investment (ROI) for your lending operations. Our focus on predicting default risk allows us to create models that are both effective and efficient. For more insights on personalized risk evaluation in insurance with AI agents, visit this link.
5.4. Real-time Risk Monitoring
Real-time risk monitoring is a critical component of modern financial management. It involves continuously assessing the risk levels associated with various financial activities and investments.
- Importance of Real-time Monitoring:
- Immediate Response: Organizations can react quickly to emerging risks, minimizing potential losses.
- Enhanced Decision-Making: Real-time data provides insights that lead to better strategic decisions.
- Regulatory Compliance: Continuous monitoring helps institutions adhere to regulatory standards.
- Tools and Technologies:
- Data Analytics Platforms: These tools analyze vast amounts of data in real-time to identify risk patterns.
- Artificial Intelligence: AI algorithms can detect anomalies and predict potential risks before they escalate.
- Dashboards: Visual representations of risk metrics allow for quick assessments and informed decision-making.
- Key Components of Real-time Risk Monitoring:
- Risk Indicators: Establishing key performance indicators (KPIs) to track risk levels.
- Alerts and Notifications: Automated systems that notify stakeholders of significant risk changes.
- Integration with Other Systems: Ensuring that risk monitoring tools work seamlessly with existing financial systems.
At Rapid Innovation, we implement cutting-edge AI technologies to facilitate real-time risk monitoring, ensuring that your organization can swiftly adapt to changing market conditions and maintain compliance with regulatory standards.
6. Customer Service and Experience Enhancement
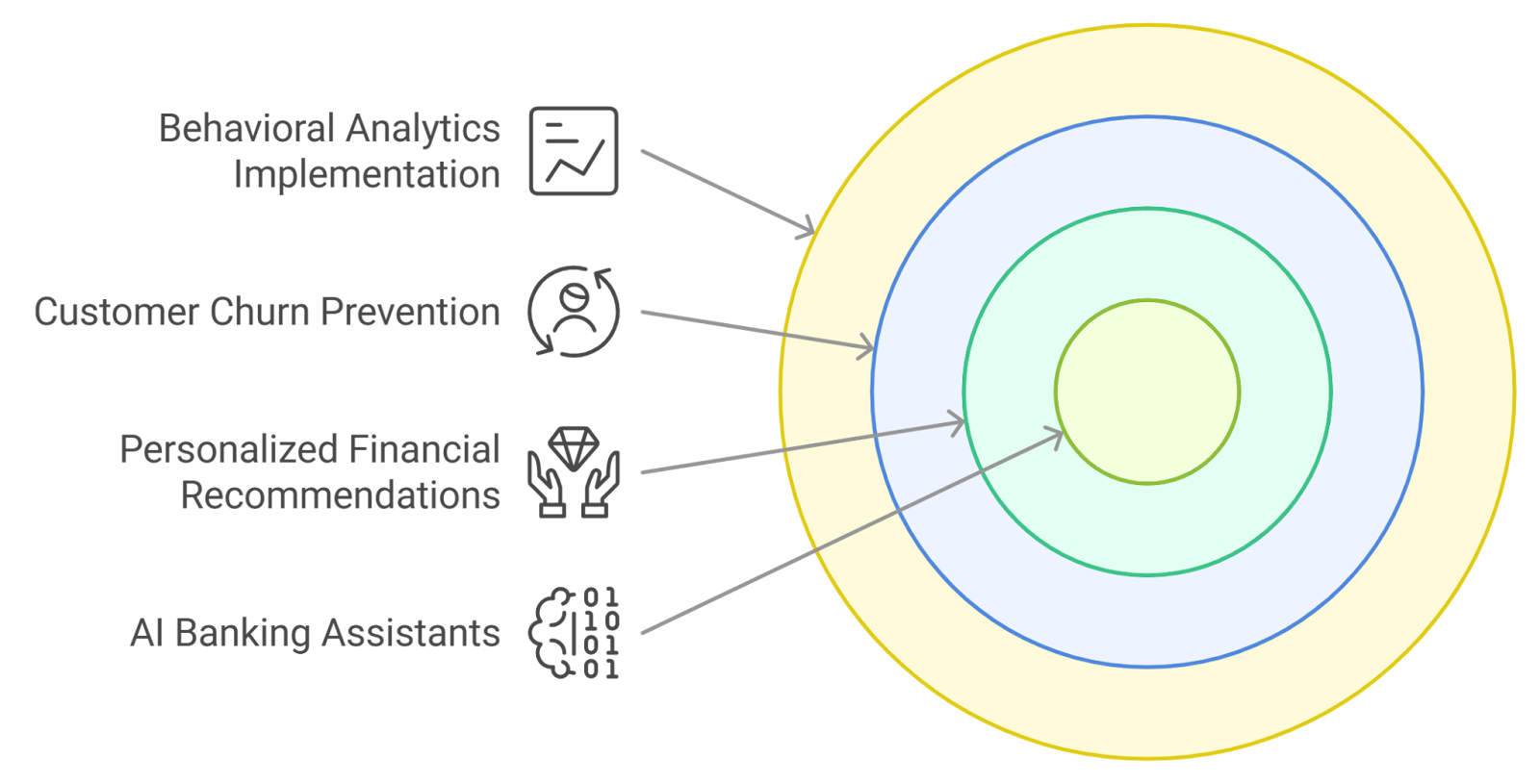
Enhancing customer service and experience is vital for businesses looking to retain clients and attract new ones. In the financial sector, this is particularly important due to the competitive landscape.
- Strategies for Improvement:
- Personalization: Tailoring services and communications to meet individual customer needs.
- Omnichannel Support: Providing consistent support across various channels, including phone, email, and social media.
- Proactive Communication: Regularly updating customers about their accounts and any changes that may affect them.
- Technology's Role:
- Chatbots: AI-driven chatbots can provide instant responses to customer inquiries, improving response times.
- Customer Relationship Management (CRM) Systems: These systems help track customer interactions and preferences, enabling personalized service.
- Mobile Apps: User-friendly apps enhance customer engagement and provide easy access to services.
- Measuring Customer Experience:
- Customer Feedback: Regular surveys and feedback mechanisms help gauge customer satisfaction.
- Net Promoter Score (NPS): This metric assesses customer loyalty and likelihood to recommend services.
- Customer Retention Rates: Monitoring retention rates can indicate the effectiveness of customer service strategies.
By focusing on default prediction models, including credit default prediction and predicting default risk, real-time risk monitoring, and enhancing customer service, financial institutions can significantly improve their operational efficiency and customer satisfaction. Rapid Innovation is committed to helping you achieve these goals through our tailored AI and Blockchain solutions, ensuring a greater ROI for your business.
6.1. What Can AI Banking Assistants Do?
AI banking assistants, such as the bank of america erica ai, are revolutionizing the financial services industry by providing a range of functionalities that enhance customer experience and operational efficiency. These virtual assistants leverage artificial intelligence to perform various tasks, including:
- 24/7 Customer Support: AI banking assistants are available around the clock, providing instant responses to customer inquiries, which improves customer satisfaction and reduces operational costs.
- Transaction Monitoring: They can track and analyze transactions in real-time, alerting customers to any suspicious activities or potential fraud, thereby enhancing security and trust.
- Account Management: Users can check balances, transfer funds, and manage their accounts through simple voice commands or chat interfaces, streamlining user interactions and reducing the need for human intervention.
- Budgeting Tools: AI assistants can help users set budgets and track spending habits, offering insights into their financial health and promoting better financial decision-making.
- Loan Assistance: They can guide customers through the loan application process, providing information on eligibility and required documentation, which simplifies the borrowing experience.
- Personalized Alerts: AI can send notifications for bill payments, low balances, or unusual spending patterns, helping customers stay on top of their finances and avoid unnecessary fees.
6.2. Personalized Financial Recommendations
One of the standout features of AI banking assistants is their ability to provide personalized financial recommendations. By analyzing user data and behavior, these assistants can offer tailored advice that meets individual financial needs. Key aspects include:
- Spending Analysis: AI can categorize spending patterns, helping users understand where their money goes and suggesting areas for potential savings, ultimately leading to improved financial health.
- Investment Suggestions: Based on risk tolerance and financial goals, AI can recommend investment opportunities, including stocks, bonds, or mutual funds, enabling users to make informed investment decisions.
- Savings Goals: AI assistants can help users set and track savings goals, providing strategies to reach them faster, which can enhance overall financial stability.
- Credit Score Monitoring: They can offer insights into credit scores and suggest actions to improve them, such as paying down debt or correcting inaccuracies, thereby empowering users to take control of their credit health.
- Tailored Product Offers: By analyzing customer profiles, AI can suggest relevant banking products, such as credit cards or savings accounts, that align with user needs, increasing customer satisfaction and loyalty.
6.3. Customer Churn Prevention
Preventing customer churn is crucial for banks, and AI banking assistants play a significant role in this area. By utilizing data analytics and machine learning, these assistants can identify at-risk customers and implement strategies to retain them. Important strategies include:
- Predictive Analytics: AI can analyze customer behavior to predict potential churn, allowing banks to intervene before customers decide to leave, thus preserving valuable relationships.
- Personalized Engagement: By offering tailored communication and solutions, AI can enhance customer relationships, making clients feel valued and understood, which is essential for retention.
- Feedback Collection: AI assistants can gather customer feedback in real-time, helping banks address issues promptly and improve service quality, leading to higher customer satisfaction.
- Incentive Programs: They can suggest personalized loyalty programs or rewards that resonate with individual customers, encouraging them to stay and engage more deeply with the bank.
- Proactive Problem Resolution: AI can identify common pain points and proactively offer solutions, reducing frustration and increasing customer satisfaction, ultimately driving greater loyalty and retention.
At Rapid Innovation, we harness the power of AI and blockchain technologies to implement these advanced banking solutions, including AI customer service agents, ensuring our clients achieve greater ROI through enhanced customer engagement, operational efficiency, and data-driven decision-making. Our expertise in AI and blockchain allows us to tailor solutions that not only meet but exceed the evolving needs of the financial services industry.
6.4. Behavioral Analytics Implementation
Behavioral analytics is a powerful tool that helps organizations understand customer behavior through data analysis. Implementing behavioral analytics implementation can significantly enhance decision-making processes and improve customer experiences in various sectors, especially in finance and banking. By analyzing data from various touchpoints, organizations can identify patterns in customer behavior, preferences, and needs. Additionally, behavioral analytics can help in detecting fraudulent activities by recognizing unusual patterns that deviate from typical customer behavior. With insights gained from behavioral analytics, businesses can tailor their marketing strategies to target specific customer segments effectively. Understanding why customers leave or stay allows organizations to implement strategies to enhance customer satisfaction and loyalty. Furthermore, behavioral analytics provides actionable insights that can guide strategic decisions, leading to better outcomes.
At Rapid Innovation, we leverage advanced AI algorithms to implement behavioral analytics solutions that empower our clients to make data-driven decisions. Our expertise in AI allows us to create customized analytics frameworks that not only identify customer trends but also predict future behaviors, enabling proactive engagement strategies. The implementation of behavioral analytics requires a robust data infrastructure, skilled personnel, and a clear strategy to ensure that insights are effectively translated into actions. For organizations looking to enhance their offerings, our AI insurance solutions can be a game-changer. Additionally, you can explore AI innovations in banking to discover the top applications that can further enhance your strategies.
7. Process Automation in Banking Operations

Process automation in banking operations refers to the use of technology to automate repetitive tasks, streamline workflows, and enhance operational efficiency. This transformation is crucial for banks looking to reduce costs and improve service delivery. Automation reduces the time taken to complete tasks, allowing banks to serve customers faster and more effectively. By minimizing manual processes, banks can significantly lower operational costs associated with labor and errors. Automated systems reduce the likelihood of human error, leading to more accurate data processing and reporting. Additionally, automation helps banks adhere to regulatory requirements by ensuring that processes are consistently followed and documented. With faster processing times and fewer errors, customers enjoy a smoother banking experience.
To successfully implement process automation, banks must assess their current workflows, identify areas for improvement, and invest in the right technology solutions.
7.1. Document Processing Systems
Document processing systems are essential tools in the banking sector, enabling organizations to manage and process large volumes of documents efficiently. These systems utilize advanced technologies such as Optical Character Recognition (OCR) and machine learning to automate document handling. Document processing systems automate the capture, classification, and extraction of data from documents, reducing manual intervention. By using OCR and machine learning, these systems can significantly improve the accuracy of data extraction, minimizing errors associated with manual data entry. Automated document processing allows banks to handle documents quickly, leading to faster decision-making and improved customer service. Reducing the need for manual document handling can lead to significant cost savings in labor and operational expenses. Furthermore, document processing systems can help banks maintain compliance with regulations by ensuring that documents are stored securely and processed according to established protocols.
At Rapid Innovation, we specialize in implementing document processing systems that integrate seamlessly with existing banking workflows. Our solutions not only enhance operational efficiency but also ensure compliance and security, ultimately driving greater ROI for our clients. Implementing a document processing system requires careful planning, including selecting the right technology, training staff, and integrating the system with existing workflows to maximize its benefits.
7.2. Regulatory Compliance Automation
Regulatory compliance automation refers to the use of technology to streamline and manage compliance processes within organizations. This is particularly crucial in industries such as finance, healthcare, and manufacturing, where regulations are stringent and constantly evolving. The concept of automated regulatory compliance is gaining traction as organizations seek to enhance their compliance frameworks.
- Enhances accuracy: Automation reduces human error, ensuring that compliance reports and documentation are accurate and up-to-date.
- Saves time: Automated systems can quickly gather and analyze data, allowing organizations to respond to regulatory changes more efficiently.
- Improves reporting: Automated compliance tools can generate reports that meet regulatory standards, making audits smoother and less time-consuming.
- Facilitates real-time monitoring: Organizations can continuously monitor compliance status, enabling proactive management of potential issues.
- Reduces manual workload: By automating repetitive tasks, employees can focus on more strategic initiatives rather than mundane compliance checks.
At Rapid Innovation, we leverage advanced AI algorithms and blockchain technology to implement regulatory compliance automation tailored to your specific industry needs. By integrating these technologies, we help organizations achieve significant improvements in operational efficiency and risk management. Companies that invest in regulatory compliance automation often find themselves better equipped to handle regulatory challenges and maintain a competitive edge. The integration of AI and machine learning for regulatory compliance further enhances these capabilities, allowing for seamless automation of compliance tasks.
7.3. Back-office Operation Optimization
Back-office operation optimization involves improving the efficiency and effectiveness of internal processes that support front-line operations. This includes functions such as accounting, human resources, IT, and supply chain management.
- Streamlines processes: By analyzing workflows, organizations can identify bottlenecks and eliminate unnecessary steps, leading to faster turnaround times.
- Enhances data management: Optimized back-office operations often involve better data integration and management, allowing for more informed decision-making.
- Increases employee productivity: With streamlined processes, employees can focus on higher-value tasks rather than getting bogged down in administrative duties.
- Leverages technology: Implementing software solutions such as Enterprise Resource Planning (ERP) systems can automate many back-office functions, leading to improved accuracy and efficiency.
- Supports scalability: Optimized operations can easily adapt to growth, ensuring that back-office functions can handle increased demand without a proportional increase in costs.
By focusing on back-office operation optimization, organizations can create a more agile and responsive business model, ultimately enhancing customer satisfaction and profitability. Rapid Innovation specializes in deploying AI-driven solutions that enhance data management and streamline processes, ensuring your back-office operations are not only efficient but also scalable.
7.4. Cost Reduction Strategies
Cost reduction strategies are essential for organizations looking to improve their bottom line without sacrificing quality or service. These strategies can take various forms, from operational efficiencies to renegotiating supplier contracts.
- Process improvement: Streamlining operations can lead to significant cost savings. Techniques such as Lean and Six Sigma can help identify waste and inefficiencies.
- Outsourcing: By outsourcing non-core functions, companies can reduce labor costs and focus on their primary business objectives.
- Technology investment: Investing in automation and technology can lead to long-term savings by reducing labor costs and increasing productivity.
- Supplier negotiation: Regularly reviewing and renegotiating contracts with suppliers can lead to better pricing and terms, reducing overall procurement costs.
- Energy efficiency: Implementing energy-saving measures can significantly lower utility bills, contributing to overall cost reduction.
Organizations that adopt effective cost reduction strategies can enhance their financial health and position themselves for sustainable growth in a competitive marketplace. At Rapid Innovation, we assist clients in identifying and implementing technology-driven cost reduction strategies that align with their business goals, ensuring a greater return on investment.
8. Personalized Banking Solutions
Personalized banking solutions are transforming the financial services landscape by tailoring products and services to meet individual customer needs. This approach enhances customer satisfaction, loyalty, and overall engagement. By leveraging data analytics and technology, banks can create customized experiences that resonate with their clients, such as internet banking platforms and services like Bank of America merchant services log in.
- Enhanced customer experience
- Increased customer loyalty
- Improved product offerings
- Greater operational efficiency
8.1. Customer Segmentation Models

Customer segmentation models are essential for banks to understand their diverse clientele better. By categorizing customers based on various criteria, banks can tailor their services and marketing strategies effectively.
- Demographic Segmentation: This involves dividing customers based on age, gender, income, and education level. For instance, younger customers may prefer mobile banking solutions, while older clients might value personalized service.
- Behavioral Segmentation: This model focuses on customer behavior, such as spending habits, transaction frequency, and product usage. Banks can identify high-value customers and target them with specific offers, including first bank merchant service options.
- Psychographic Segmentation: This approach considers customers' lifestyles, values, and interests. Understanding these factors allows banks to create marketing messages that resonate on a personal level.
- Geographic Segmentation: Banks can also segment customers based on their location. This is particularly useful for tailoring services to meet regional needs, such as local investment opportunities or community-focused products, like those offered by equity bank merchant services.
By implementing these segmentation models, banks can develop targeted marketing campaigns, enhance customer engagement, and improve customer retention rates.
8.2. Product Recommendation Engines
Product recommendation engines utilize advanced algorithms and machine learning to suggest financial products that align with individual customer profiles. These engines analyze vast amounts of data to provide personalized recommendations, enhancing the customer experience.
- Data-Driven Insights: By analyzing customer data, banks can identify patterns and preferences, allowing them to recommend products that meet specific needs. For example, if a customer frequently travels, the engine might suggest a travel rewards credit card or services from TD credit card merchant services.
- Real-Time Recommendations: Product recommendation engines can provide suggestions in real-time, whether through mobile apps or online banking platforms, such as first merchants business online banking. This immediacy can lead to higher conversion rates as customers are presented with relevant options at the right moment.
- Cross-Selling Opportunities: These engines can identify opportunities for cross-selling by suggesting complementary products. For instance, if a customer opens a savings account, the engine might recommend a high-yield savings option or a financial planning service, similar to those offered by Bank of America business merchant services.
- Enhanced Customer Engagement: Personalized recommendations foster a sense of connection between the bank and the customer. When clients feel understood and valued, they are more likely to engage with the bank's offerings, including services like signature bank remote deposit capture.
By leveraging product recommendation engines, banks can increase sales and revenue, improve customer satisfaction, and foster long-term relationships with clients. At Rapid Innovation, we specialize in developing and implementing these advanced AI-driven solutions, ensuring that our clients achieve greater ROI through enhanced customer experiences and operational efficiencies, including those related to Bank of America merchant services headquarters and logo.
8.3. Targeted Marketing Campaigns
Targeted marketing campaigns, such as targeted email marketing and targeted marketing emails, are essential for businesses looking to maximize their return on investment (ROI) and engage effectively with their audience. By focusing on specific segments of the market, companies can tailor their messaging and offers to meet the unique needs of different customer groups.
- Segmentation: Divide your audience based on demographics, behavior, interests, or purchasing history. This allows for more personalized marketing efforts, including targeted marketing campaigns like geo fencing campaigns and targeted advertising campaigns.
- Data Utilization: Leverage customer data to understand preferences and trends. Tools like CRM systems can help gather and analyze this information, enabling Rapid Innovation to provide insights that drive effective marketing strategies, including targeted digital marketing campaigns.
- Personalization: Create customized content and offers that resonate with each segment. Personalized emails, targeted ads, and tailored promotions can significantly increase engagement rates, leading to higher conversion rates and improved ROI. Examples of targeted marketing campaigns include targeted email marketing campaigns and targeted SMS marketing.
- Multi-Channel Approach: Utilize various platforms such as social media, email, and search engines to reach your audience where they are most active. Rapid Innovation can assist in developing a cohesive strategy that integrates these channels for maximum impact, including display campaign targeting.
- A/B Testing: Experiment with different messages, visuals, and offers to determine what resonates best with each segment. This helps refine your campaigns for better performance, ensuring that marketing budgets are spent efficiently. Best targeted marketing campaigns often utilize A/B testing to optimize results.
- Performance Tracking: Monitor the success of your campaigns through metrics like click-through rates, conversion rates, and customer feedback. Adjust strategies based on these insights, allowing Rapid Innovation to optimize your marketing efforts continuously. Understanding the campaign target audience and campaign target market is crucial for effective tracking.
By implementing targeted marketing campaigns, businesses can enhance customer engagement, improve conversion rates, and ultimately drive sales growth, resulting in a greater ROI. For instance, understanding the dove target market and dove men care target market can lead to more effective targeted marketing campaigns. For more insights on leveraging AI in marketing applications, check out this resource.
8.4. Customer Lifetime Value Analysis
Customer Lifetime Value (CLV) analysis is a crucial metric that helps businesses understand the total worth of a customer over the entire duration of their relationship. This analysis informs marketing strategies, customer service improvements, and product development.
- Definition of CLV: CLV is the predicted revenue a customer will generate during their lifetime as a paying customer. It helps businesses assess the long-term value of acquiring and retaining customers.
- Importance of CLV: Understanding CLV allows businesses to allocate marketing budgets more effectively, identify high-value customers for targeted retention strategies, and improve customer acquisition strategies by focusing on channels that yield high CLV.
- Calculating CLV: The basic formula for calculating CLV is:
language="language-plaintext"CLV = (Average Purchase Value) x (Average Purchase Frequency) x (Customer Lifespan)
- Factors Influencing CLV:
- Customer retention rates: Higher retention leads to increased CLV.
- Purchase frequency: More frequent purchases contribute to a higher CLV.
- Average order value: Increasing the average order value can significantly boost CLV.
- Using CLV for Decision Making: Businesses can use CLV analysis to tailor marketing strategies to focus on high-value customers, develop loyalty programs that encourage repeat purchases, and optimize product offerings based on customer preferences and behaviors.
By conducting a thorough CLV analysis, businesses can make informed decisions that enhance customer relationships and drive profitability.
9. What Are the Best Practices for ML Implementation?
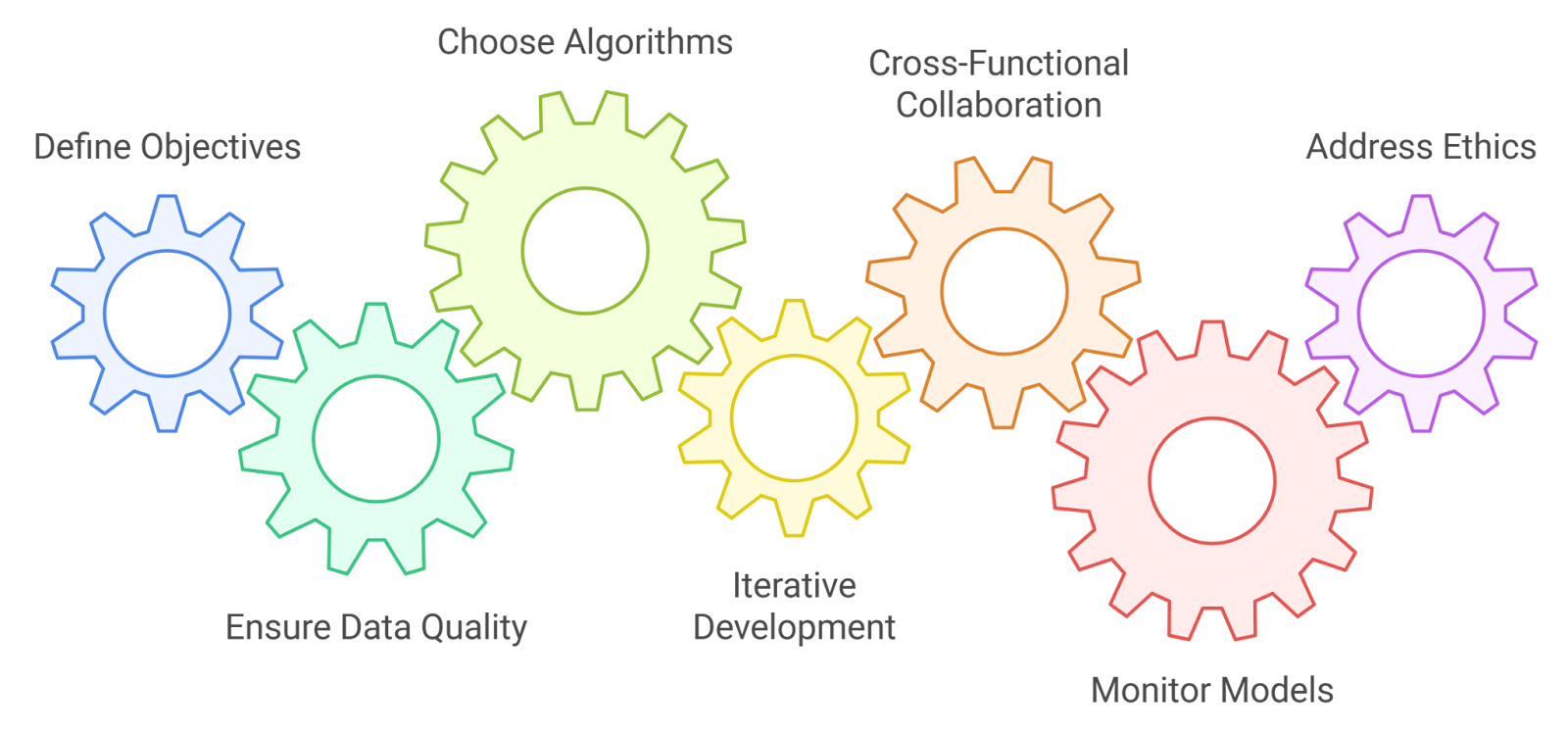
Implementing machine learning (ML) in business processes can lead to significant improvements in efficiency, decision-making, and customer satisfaction. However, successful ML implementation requires careful planning and execution. Here are some best practices to consider:
- Define Clear Objectives: Establish specific goals for what you want to achieve with ML. This could include improving customer service, automating processes, or enhancing product recommendations.
- Data Quality and Quantity: Ensure you have access to high-quality, relevant data. The success of ML models heavily relies on the data used for training. Clean, well-structured data leads to better outcomes.
- Choose the Right Algorithms: Select algorithms that align with your objectives and the nature of your data. Different problems may require different approaches, such as supervised learning for classification tasks or unsupervised learning for clustering.
- Iterative Development: Adopt an iterative approach to model development. Start with a simple model, evaluate its performance, and gradually refine it based on feedback and results.
- Cross-Functional Collaboration: Involve stakeholders from various departments, including IT, marketing, and operations. This ensures that the ML solution aligns with business needs and has the necessary support for implementation.
- Monitor and Maintain Models: Once deployed, continuously monitor the performance of ML models. Regular updates and retraining may be necessary to adapt to changing data patterns and business environments.
- Ethical Considerations: Address ethical concerns related to data privacy and algorithmic bias. Ensure compliance with regulations and maintain transparency in how ML models make decisions.
By following these best practices, businesses can effectively implement machine learning solutions that drive innovation and improve operational efficiency. Rapid Innovation is here to guide you through this process, ensuring that your ML initiatives yield the desired results.
9.1. Technical Infrastructure Requirements
A robust technical infrastructure is essential for any organization aiming to leverage technology effectively. This infrastructure encompasses hardware, software, networks, and services that support the organization's operations. Key components include:
- Hardware: Servers, computers, and networking devices must be capable of handling the organization's workload. Considerations include:
- Processing power: Ensuring that systems can handle AI computations and blockchain transactions efficiently.
- Storage capacity: Adequate storage for large datasets, especially relevant for AI training and blockchain data.
- Scalability for future growth: Infrastructure should be designed to scale as business needs evolve, particularly in rapidly changing tech landscapes.
- Software: The right software solutions are crucial for operational efficiency. This includes:
- Operating systems: Optimized for performance in AI and blockchain applications.
- Application software tailored to business needs: Custom solutions that integrate AI and blockchain functionalities to enhance business processes.
- Cloud services for flexibility and scalability: Utilizing cloud platforms to support AI workloads and blockchain networks.
- Network: A reliable network infrastructure ensures seamless communication and data transfer. Important aspects include:
- High-speed internet connectivity: Essential for real-time data processing in AI and blockchain applications.
- Secure and efficient local area networks (LAN): Critical for internal communications and data sharing.
- Virtual private networks (VPN) for remote access: Ensuring secure access to systems for remote teams.
- Support Services: Technical support and maintenance are vital for minimizing downtime. This includes:
- IT support teams: Specialized in AI and blockchain technologies to address specific challenges.
- Regular system updates and backups: Ensuring that systems are up-to-date and data is secure.
- Disaster recovery plans: Preparedness for potential disruptions, particularly important in blockchain environments.
Investing in a solid technical infrastructure not only enhances productivity but also supports innovation and growth, enabling organizations to harness the full potential of technical infrastructure for AI and blockchain technologies and learning from real-world AI implementations.
9.2. Data Security and Compliance
Data security and compliance are critical in today’s digital landscape, where data breaches and regulatory requirements are prevalent. Organizations must prioritize the following:
- Data Protection: Implementing measures to safeguard sensitive information is essential. Key strategies include:
- Encryption of data at rest and in transit: Vital for protecting sensitive AI training data and blockchain transactions.
- Regular security audits and vulnerability assessments: Ensuring that systems are secure against emerging threats.
- Access controls to limit data exposure: Implementing strict access protocols to safeguard sensitive information.
- Compliance: Adhering to legal and regulatory standards is non-negotiable. Important regulations include:
- General Data Protection Regulation (GDPR): Ensuring compliance in AI data usage and blockchain applications.
- Health Insurance Portability and Accountability Act (HIPAA): Relevant for organizations handling healthcare data.
- Payment Card Industry Data Security Standard (PCI DSS): Critical for businesses processing payment information.
- Incident Response: Having a robust incident response plan is crucial for mitigating damage in case of a data breach. This includes:
- Immediate identification and containment of the breach: Quick action to minimize impact.
- Notification procedures for affected parties: Transparency in communication during incidents.
- Post-incident analysis to prevent future occurrences: Learning from incidents to strengthen security measures.
- Employee Training: Regular training on data security best practices helps create a culture of security awareness. Topics should cover:
- Recognizing phishing attempts: Educating employees on common threats.
- Safe data handling procedures: Best practices for managing sensitive information.
- Reporting security incidents: Encouraging prompt reporting to mitigate risks.
By prioritizing data security and compliance, organizations can protect their assets and maintain customer trust, which is essential for leveraging AI and blockchain technologies effectively.
9.3. ROI Measurement Frameworks
Measuring return on investment (ROI) is vital for assessing the effectiveness of business initiatives and technology investments. A structured ROI measurement framework can help organizations make informed decisions. Key components include:
- Defining Objectives: Clearly outline the goals of the investment. This could involve:
- Increasing revenue: Through enhanced AI-driven insights and blockchain efficiencies.
- Reducing costs: By streamlining operations with AI automation and blockchain transparency.
- Improving customer satisfaction: Leveraging AI for personalized experiences and blockchain for trust.
- Identifying Metrics: Establish relevant metrics to evaluate performance. Common metrics include:
- Financial metrics (e.g., net profit, cost savings): Directly linked to technology investments.
- Operational metrics (e.g., efficiency improvements, time savings): Measuring the impact of AI and blockchain on processes.
- Customer metrics (e.g., retention rates, satisfaction scores): Assessing the effectiveness of technology in enhancing customer experiences.
- Data Collection: Gather data systematically to ensure accurate analysis. This involves:
- Utilizing analytics tools to track performance: Essential for AI and blockchain data insights.
- Conducting surveys and feedback sessions: Gathering qualitative data on customer experiences.
- Monitoring key performance indicators (KPIs): Regularly assessing progress against objectives.
- Analysis and Reporting: Analyze the collected data to assess ROI. This includes:
- Comparing actual performance against projected outcomes: Evaluating the success of technology investments.
- Identifying areas for improvement: Using data to refine strategies.
- Reporting findings to stakeholders for transparency: Ensuring accountability in decision-making.
- Continuous Improvement: Use the insights gained from ROI analysis to refine strategies and investments. This could involve:
- Adjusting resource allocation: Ensuring optimal use of resources in AI and blockchain projects.
- Implementing new technologies: Staying ahead of the curve with emerging solutions.
- Enhancing training programs: Ensuring teams are equipped to leverage new technologies effectively.
By establishing a comprehensive ROI measurement framework, organizations can ensure that their investments yield tangible benefits and drive growth, particularly in the dynamic fields of AI and blockchain.
9.4. Team Structure and Expertise
A well-defined team structure and the right expertise are crucial for the successful implementation of machine learning (ML) in FinTech. The complexity of financial data and the need for compliance with regulations necessitate a diverse skill set within the team.
- Data Scientists: These professionals are responsible for developing algorithms and models that can analyze vast amounts of financial data. They must possess strong statistical knowledge and programming skills, particularly in languages like Python and R. Their work often involves machine learning applications in fintech to derive insights from data.
- Data Engineers: They focus on the architecture and infrastructure needed to collect, store, and process data efficiently. Their expertise in database management and big data technologies is essential for handling the large datasets typical in fintech and machine learning.
- Domain Experts: Financial analysts and industry specialists provide insights into market trends, regulatory requirements, and customer behavior. Their knowledge helps ensure that ML models are relevant and compliant with financial regulations, particularly in areas like ai and machine learning in fintech.
- Software Developers: These team members are responsible for integrating ML models into existing systems and ensuring that applications are user-friendly. They need to be proficient in software development practices and agile methodologies, especially when working on fintech machine learning applications.
- Compliance Officers: Given the highly regulated nature of the financial industry, compliance officers ensure that all ML applications adhere to legal standards. Their role is vital in mitigating risks associated with data privacy and security, particularly in the context of machine learning and fintech.
- Project Managers: They oversee the project lifecycle, ensuring that timelines are met and resources are allocated efficiently. Their leadership skills are essential for coordinating between different teams and stakeholders, especially in projects involving machine learning fintech companies.
At Rapid Innovation, we understand that a balanced team structure that combines these roles can significantly enhance the effectiveness of ML initiatives in fintech, leading to better decision-making and improved customer experiences. Our team is equipped with the necessary expertise to guide clients through the complexities of ML implementation, ensuring that they achieve their business goals efficiently and effectively. We also offer custom AI model development services to further support your needs. Additionally, we explore the role of AI in business process automation to enhance operational efficiency.
10. Future of ML in FinTech
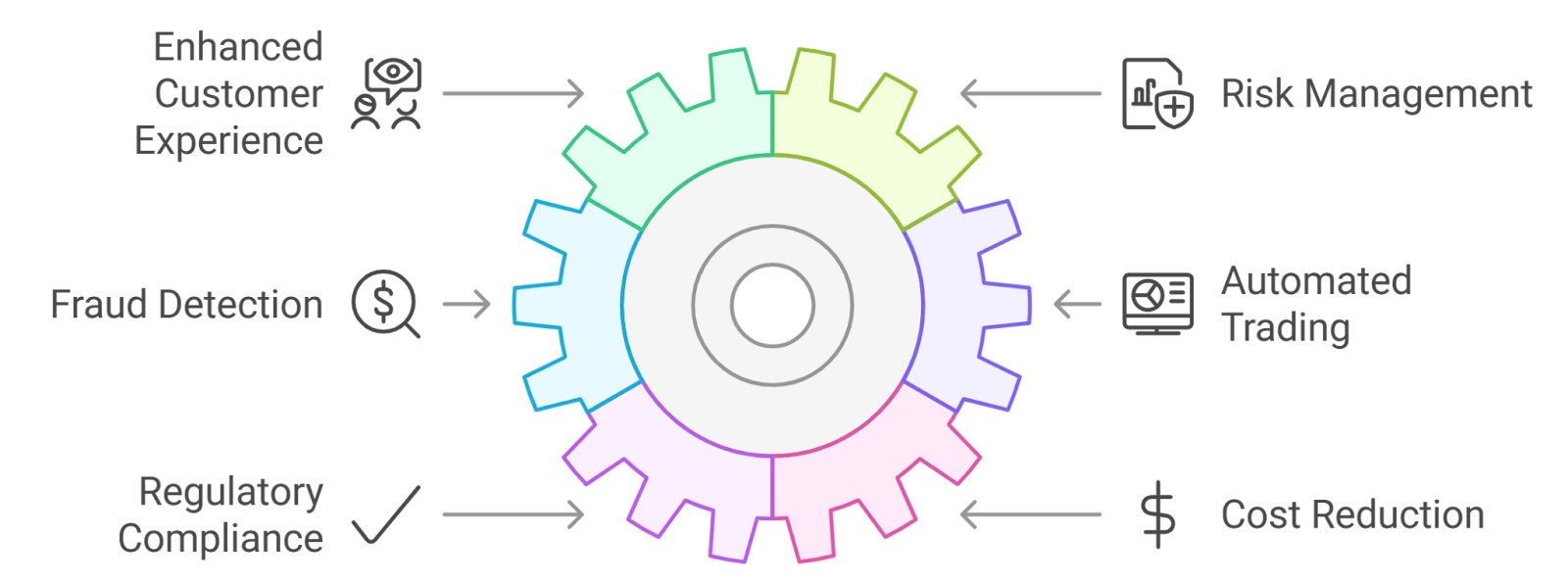
The future of machine learning in fintech is promising, with numerous opportunities for innovation and growth. As technology continues to evolve, ML is expected to play an increasingly pivotal role in transforming financial services.
- Enhanced Customer Experience: ML algorithms can analyze customer data to provide personalized financial advice, improving customer satisfaction and loyalty.
- Risk Management: Advanced ML models can predict potential risks by analyzing historical data and market trends, allowing financial institutions to make informed decisions.
- Fraud Detection: Machine learning can identify unusual patterns in transactions, enabling quicker detection of fraudulent activities and reducing financial losses.
- Automated Trading: Algorithms can analyze market conditions in real-time, allowing for automated trading strategies that can capitalize on market fluctuations.
- Regulatory Compliance: ML can assist in monitoring transactions and ensuring compliance with regulations, reducing the burden on compliance teams.
- Cost Reduction: By automating various processes, ML can help financial institutions reduce operational costs and improve efficiency.
As these trends continue to develop, the integration of ML in fintech will likely lead to more innovative products and services, reshaping the financial landscape.
10.1. Emerging Technologies and Trends
Several emerging technologies and trends are shaping the future of machine learning in fintech. These advancements are not only enhancing existing services but also paving the way for new business models.
- Artificial Intelligence (AI): The integration of AI with ML is creating more sophisticated systems capable of understanding and predicting customer behavior. This synergy is crucial for developing intelligent financial advisors and chatbots.
- Blockchain Technology: Blockchain can enhance the security and transparency of financial transactions. When combined with ML, it can improve fraud detection and risk assessment by providing immutable records of transactions.
- Natural Language Processing (NLP): NLP enables machines to understand and interpret human language. In fintech, it can be used for sentiment analysis, customer service automation, and compliance monitoring.
- Robotic Process Automation (RPA): RPA can automate repetitive tasks, allowing financial institutions to focus on more strategic initiatives. When integrated with ML, RPA can learn from data and improve its processes over time.
- Cloud Computing: The shift to cloud-based solutions allows for scalable data storage and processing capabilities. This flexibility is essential for deploying ML models that require significant computational resources.
- Edge Computing: As financial transactions become more instantaneous, edge computing allows data processing closer to the source, reducing latency and improving real-time decision-making.
- Open Banking: The trend towards open banking is fostering innovation by allowing third-party developers to create applications that interact with financial institutions. ML can enhance these applications by providing insights based on customer data, particularly in the context of machine learning in fintech.
These emerging technologies and trends are set to revolutionize the fintech landscape, making it more efficient, secure, and customer-centric. As organizations continue to adopt these innovations, the role of machine learning will become increasingly integral to their success. At Rapid Innovation, we are committed to helping our clients navigate these advancements, ensuring they leverage the full potential of ML and related technologies to achieve greater ROI.
10.2. Predicted Market Evolution
The market landscape is continuously evolving, influenced by various factors such as technological advancements, consumer behavior, and economic conditions. Understanding these trends is crucial for businesses aiming to stay competitive.
- Technological Advancements: Innovations in technology are reshaping industries. For instance, artificial intelligence (AI) and machine learning are becoming integral in data analysis, customer service, and product development. Companies that leverage these technologies can enhance efficiency and improve customer experiences. At Rapid Innovation, we specialize in implementing AI solutions that optimize operations and drive significant ROI for our clients through market evolution strategies.
- Consumer Behavior Shifts: Today's consumers are more informed and connected than ever. They demand personalized experiences and are increasingly concerned about sustainability. Businesses must adapt their strategies to meet these changing preferences, focusing on transparency and ethical practices. Our blockchain solutions can help organizations ensure transparency in their supply chains, thereby building trust with consumers, which is essential in the context of market evolution strategies.
- Economic Factors: Global economic conditions, such as inflation rates and employment levels, significantly impact market dynamics. Organizations need to be agile, adjusting their strategies in response to economic fluctuations to maintain profitability. Rapid Innovation assists clients in developing adaptive strategies that leverage AI and blockchain technologies to navigate these economic challenges effectively, aligning with market evolution strategies.
- Regulatory Changes: Governments worldwide are implementing new regulations that affect various industries. Staying informed about these changes is essential for compliance and can also present opportunities for innovation. Our consulting services help businesses understand and adapt to regulatory changes, ensuring compliance while fostering innovation, which is a key aspect of market evolution strategies.
- Globalization: The interconnectedness of markets means that businesses must consider international competition and opportunities. Expanding into new markets can drive growth, but it requires careful research and strategic planning. Rapid Innovation provides the necessary tools and insights to help clients successfully enter and thrive in new markets through advanced AI analytics and blockchain solutions, supporting their market evolution strategies. Additionally, our expertise in crypto arbitrage bot development can further enhance your competitive edge in the evolving market landscape. For more insights on how AI can drive retail growth, check out AI: The Key to Unlocking Retail Growth.
10.3. Preparation Strategies for Organizations
To navigate the predicted market evolution successfully, organizations must adopt proactive preparation strategies. These strategies can help businesses remain resilient and adaptable in a rapidly changing environment.
- Market Research: Conducting thorough market research is vital. Understanding industry trends, customer preferences, and competitor strategies can provide valuable insights for decision-making. Our AI-driven market analysis tools can provide real-time insights that empower organizations to make informed decisions, which is crucial for effective market evolution strategies.
- Agility and Flexibility: Organizations should foster a culture of agility, allowing them to pivot quickly in response to market changes. This can involve adopting flexible business models and encouraging innovative thinking among employees. Rapid Innovation promotes agile methodologies in project management, ensuring that our clients can adapt swiftly to market demands, a fundamental component of market evolution strategies.
- Investment in Technology: Embracing new technologies can streamline operations and enhance customer engagement. Investing in digital tools, such as CRM systems and data analytics platforms, can provide a competitive edge. Our expertise in AI and blockchain allows us to guide clients in selecting and implementing the right technologies for their needs, aligning with their market evolution strategies.
- Employee Training and Development: Continuous learning is essential for keeping the workforce skilled and knowledgeable. Organizations should invest in training programs that equip employees with the skills needed to adapt to new technologies and market demands. Rapid Innovation offers tailored training programs focused on AI and blockchain technologies to empower your workforce, supporting their market evolution strategies.
- Sustainability Initiatives: As consumers increasingly prioritize sustainability, organizations should integrate eco-friendly practices into their operations. This can enhance brand reputation and attract environmentally conscious customers. Our blockchain solutions can help track and verify sustainable practices, providing transparency that resonates with today's consumers, which is integral to market evolution strategies.
- Strategic Partnerships: Collaborating with other businesses can open new avenues for growth. Strategic partnerships can provide access to new markets, technologies, and expertise. Rapid Innovation fosters partnerships that leverage our AI and blockchain capabilities, enabling clients to expand their reach and enhance their offerings, which is a vital aspect of market evolution strategies.
11. Frequently Asked Questions
Understanding common queries can help clarify the complexities of market evolution and preparation strategies. Here are some frequently asked questions:
- What are the key factors driving market evolution?
Technological advancements, changing consumer behaviors, economic conditions, regulatory changes, and globalization are primary drivers of market evolution. - How can organizations stay competitive in a changing market?
Organizations can stay competitive by conducting market research, being agile, investing in technology, training employees, adopting sustainability practices, and forming strategic partnerships. - What role does technology play in market evolution?
Technology plays a crucial role by enabling businesses to analyze data, improve customer interactions, and streamline operations. Companies that embrace technology can better respond to market changes and consumer needs. - Why is sustainability important for businesses today?
Sustainability is increasingly important as consumers demand ethical practices. Companies that prioritize sustainability can enhance their brand image and attract a loyal customer base. - How can businesses prepare for economic fluctuations?
Businesses can prepare for economic fluctuations by maintaining financial flexibility, diversifying their offerings, and staying informed about market trends and economic indicators.
By addressing these aspects, organizations can better position themselves for success in an evolving market landscape. Rapid Innovation is here to support you in leveraging AI and blockchain technologies to achieve your business goals efficiently and effectively through effective market evolution strategies.
11.1. What Are the Biggest Implementation Challenges for ML in FinTech?
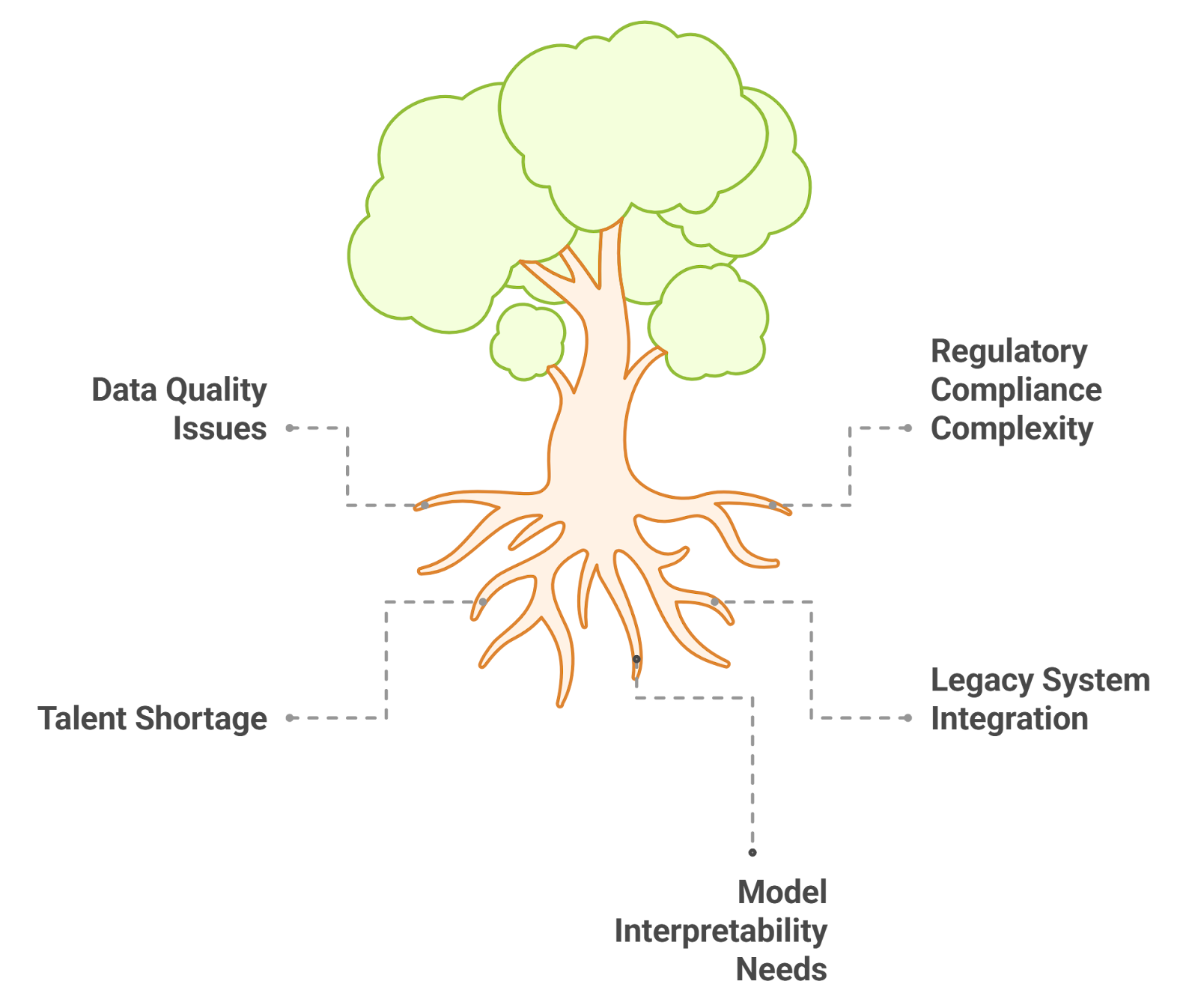
Implementing machine learning (ML) in the FinTech sector presents several challenges that organizations must navigate to achieve successful outcomes. These challenges include:
- Data Quality and Availability: Financial institutions often deal with vast amounts of data, but not all of it is clean or structured. Poor data quality can lead to inaccurate models. Access to high-quality, relevant data is crucial for training effective ML algorithms. At Rapid Innovation, we assist clients in establishing robust data management practices to ensure data integrity and availability, enabling them to build more accurate ML models. This is particularly important when addressing machine learning challenges in fintech.
- Regulatory Compliance: The FinTech industry is heavily regulated, and ensuring that ML models comply with regulations can be complex. Organizations must navigate various laws and guidelines, such as GDPR and PCI DSS, which can impact data usage and model transparency. Our team at Rapid Innovation provides consulting services to help clients understand and implement compliance measures, ensuring that their ML initiatives align with regulatory requirements. This is a significant aspect of the machine learning challenges in fintech.
- Talent Shortage: There is a significant demand for skilled data scientists and ML engineers in the FinTech sector. The shortage of qualified professionals can hinder the development and implementation of ML solutions. Rapid Innovation offers training and development programs to upskill existing teams, helping organizations bridge the talent gap and effectively leverage ML technologies. Addressing this talent shortage is crucial to overcoming machine learning challenges in fintech.
- Integration with Legacy Systems: Many financial institutions rely on outdated technology, making it difficult to integrate new ML systems. Legacy systems may not support the data processing needs of modern ML applications. We specialize in creating seamless integration solutions that allow clients to modernize their technology stack while incorporating advanced ML capabilities. This integration is vital to tackling machine learning challenges in fintech.
- Model Interpretability: Financial institutions require transparency in their decision-making processes, which can be challenging with complex ML models. Ensuring that models are interpretable and explainable is essential for gaining stakeholder trust. Rapid Innovation employs advanced techniques to enhance model interpretability, ensuring that our clients can provide clear explanations for their ML-driven decisions. This focus on interpretability addresses one of the key machine learning challenges in fintech. For more insights on building effective ML platforms, check out how to build an end-to-end ML platform.
11.2. How to Calculate ROI for ML in FinTech?
Calculating the return on investment (ROI) for machine learning initiatives in FinTech involves several steps to ensure a comprehensive evaluation. Key considerations include:
- Define Clear Objectives: Establish specific goals for the ML project, such as reducing fraud, improving customer service, or enhancing risk assessment. Clear objectives help in measuring the success of the implementation.
- Identify Costs: Consider all costs associated with the ML project, including development and deployment expenses, infrastructure costs (cloud services, hardware), ongoing maintenance and support, and training and hiring of personnel.
- Measure Benefits: Quantify the benefits derived from the ML implementation, which may include increased revenue from improved customer targeting, cost savings from automation and efficiency gains, and reduction in losses due to fraud detection. Rapid Innovation assists clients in identifying and measuring these benefits, ensuring a clear understanding of the value generated by their ML initiatives.
- Calculate ROI: Use the formula:
language="language-plaintext"``` -a1b2c3- ROI = (Net Profit / Total Investment) x 100
Net Profit is calculated by subtracting total costs from total benefits.
- Monitor and Adjust: Continuously track the performance of the ML initiative against the defined objectives. Be prepared to adjust strategies based on performance data to maximize ROI. Our team provides ongoing support to help clients refine their ML strategies and optimize their ROI over time.
11.3. How to Ensure ML Compliance?
Ensuring compliance in machine learning applications within the FinTech sector is critical to maintaining trust and adhering to regulations. Key strategies include:
- Understand Regulatory Requirements: Familiarize yourself with relevant regulations that impact ML, such as Anti-Money Laundering (AML) laws, Know Your Customer (KYC) regulations, and data protection laws like GDPR.
- Implement Robust Data Governance: Establish data governance frameworks to ensure data integrity, security, and compliance. Regularly audit data sources and usage to maintain compliance with regulations. Rapid Innovation helps clients develop and implement effective data governance strategies tailored to their specific needs.
- Ensure Model Transparency: Develop models that are interpretable and can provide explanations for their predictions. Use techniques such as LIME or SHAP to enhance model transparency.
- Conduct Regular Audits: Perform regular audits of ML models to ensure they comply with regulatory standards and internal policies. Document the decision-making processes and model performance for accountability.
- Engage with Legal and Compliance Teams: Collaborate with legal and compliance experts during the development and deployment of ML solutions. Ensure that all aspects of the ML project align with regulatory requirements and best practices. At Rapid Innovation, we facilitate collaboration between technical teams and compliance experts to ensure that our clients' ML initiatives are both innovative and compliant.
11.4. Can ML Work with Legacy Systems?
Machine Learning (ML) has become a transformative technology across various industries, but its integration with machine learning legacy systems poses unique challenges and opportunities. Legacy systems are older software or hardware that are still in use, often due to their critical role in business operations. Here’s how ML can work with these systems:
- Data Accessibility: Legacy systems often store valuable data that can be leveraged for ML models. Extracting data from these systems may require custom APIs or data extraction tools. Ensuring data quality and consistency is crucial for effective ML training.
- Interoperability: ML solutions can be designed to interface with legacy systems through middleware. This allows for real-time data exchange and processing without overhauling existing infrastructure. Using microservices architecture can facilitate smoother integration.
- Incremental Adoption: Organizations can implement ML in phases, starting with less critical functions. This approach minimizes risk and allows for gradual adaptation of ML technologies. Pilot projects can demonstrate value before full-scale implementation.
- Enhancing Legacy Systems: ML can enhance the functionality of legacy systems by adding predictive analytics capabilities. For example, predictive maintenance can be applied to machinery controlled by legacy systems, leading to reduced downtime and improved operational efficiency.
- Cost Considerations: Integrating ML with legacy systems can be cost-effective compared to complete system replacements. Organizations can maximize their existing investments while modernizing their capabilities. However, initial integration costs and ongoing maintenance should be considered.
- Skill Requirements: Teams may need training to work with both ML technologies and legacy systems. Bridging the knowledge gap between traditional IT and data science is essential. Collaboration between data scientists and legacy system experts can drive successful integration.
- Security and Compliance: Legacy systems may have outdated security protocols, posing risks when integrating ML. Ensuring compliance with data protection regulations is critical during integration. Regular audits and updates can help maintain security standards.
- Scalability: ML models can be designed to scale independently of legacy systems, allowing organizations to expand their ML capabilities without being limited by older technology. Cloud-based solutions can provide the necessary infrastructure for scalability.
- Real-World Examples: Many organizations have successfully integrated ML with legacy systems. For instance, financial institutions use ML to analyze transaction data from legacy banking systems, while manufacturing companies apply ML for quality control, leveraging data from older machinery.
- Future-Proofing: Integrating ML with legacy systems can serve as a stepping stone toward modernization. Organizations can gradually transition to more advanced systems while still utilizing existing resources, helping to align with future technological advancements.
In conclusion, while integrating ML with legacy systems presents challenges, it also offers significant opportunities for enhancing business processes and decision-making. By carefully planning the integration and addressing potential issues, organizations can harness the power of ML without discarding their valuable machine learning legacy systems. At Rapid Innovation, we specialize in guiding organizations through this integration process, ensuring that they achieve greater ROI while leveraging their existing infrastructure effectively. For more information on our services, visit our large language model development page and learn about the importance of blockchain integration with legacy systems.