Table Of Contents
Category
Customer Service
CRM
FinTech
1. What is NLP in Customer Service?
Natural Language Processing (NLP) is a branch of artificial intelligence that focuses on the interaction between computers and humans through natural language. In customer service, NLP enables machines to understand, interpret, and respond to human language in a way that is both meaningful and useful. This technology is increasingly being integrated into customer support systems, such as nlp in customer service and customer support nlp, to enhance user experience and streamline operations.
- Enhances communication between customers and support agents.
- Automates responses to common inquiries, reducing wait times.
- Analyzes customer sentiment to improve service quality.
- Provides insights into customer behavior and preferences.
1.1. How Does Natural Language Processing (NLP) Work in Customer Service?
%2520Work%2520in%2520Customer%2520Service_%2520-%2520visual%2520selection.png)
NLP in customer service operates through several key processes that allow machines to understand and respond to human language effectively.
- Text Analysis: NLP algorithms analyze text data from customer inquiries, identifying keywords and phrases to determine intent.
- Sentiment Analysis: This process evaluates the emotional tone behind a series of words, helping businesses gauge customer satisfaction and urgency.
- Entity Recognition: NLP identifies specific entities within the text, such as product names or dates, to provide relevant responses.
- Response Generation: Based on the analysis, NLP systems can generate appropriate responses, either through pre-defined templates or by using machine learning to create unique replies.
These processes work together to create a seamless interaction between customers and automated systems, improving efficiency and satisfaction.
1.2. How Has NLP Technology Evolved in Customer Support?
NLP technology has undergone significant advancements over the years, transforming the landscape of customer support.
- Early Chatbots: Initially, chatbots relied on simple keyword matching, which often led to misunderstandings and frustration for users.
- Machine Learning Integration: The introduction of machine learning algorithms allowed NLP systems to learn from interactions, improving their ability to understand context and intent over time.
- Voice Recognition: With the rise of voice-activated assistants, NLP has expanded to include voice recognition, enabling customers to interact with support systems through spoken language.
- Multilingual Support: Modern NLP systems can now process multiple languages, making customer support more accessible to a global audience.
- Real-time Analytics: Advanced NLP tools provide real-time analytics, allowing businesses to monitor customer interactions and adjust strategies accordingly.
These developments have made NLP an essential component of modern customer service, enhancing both efficiency and customer satisfaction. At Rapid Innovation, we leverage these advancements in customer support natural language processing to help our clients optimize their customer service operations, ultimately leading to greater ROI and improved customer loyalty. By integrating tailored NLP solutions, such as nlp for support tickets and nlp help center, we empower businesses to respond to customer needs swiftly and accurately, ensuring a competitive edge in the market.
1.3. Key Components of NLP-Powered Customer Service
Natural Language Processing (NLP) is a branch of artificial intelligence that enables machines to understand, interpret, and respond to human language. In customer service, NLP can significantly enhance interactions between businesses and customers. The key components of NLP-powered customer service include:
- Text Analysis: This involves breaking down customer inquiries into understandable segments. Text analysis helps in identifying the intent behind customer messages, allowing for more accurate responses.
- Sentiment Analysis: NLP can assess the emotional tone of customer communications. By analyzing sentiment, businesses can gauge customer satisfaction and adjust their responses accordingly.
- Chatbots and Virtual Assistants: These AI-driven tools utilize NLP to engage with customers in real-time. They can handle common queries, provide information, and escalate issues to human agents when necessary.
- Speech Recognition: NLP enables systems to convert spoken language into text. This is particularly useful in call centers, where voice interactions are prevalent, especially in nlp customer service.
- Language Translation: NLP can facilitate communication across different languages, allowing businesses to serve a global customer base effectively.
- Contextual Understanding: Advanced NLP systems can understand context, which helps in providing relevant responses based on previous interactions or specific customer needs.
2. What Are the Business Benefits of NLP in Customer Service?
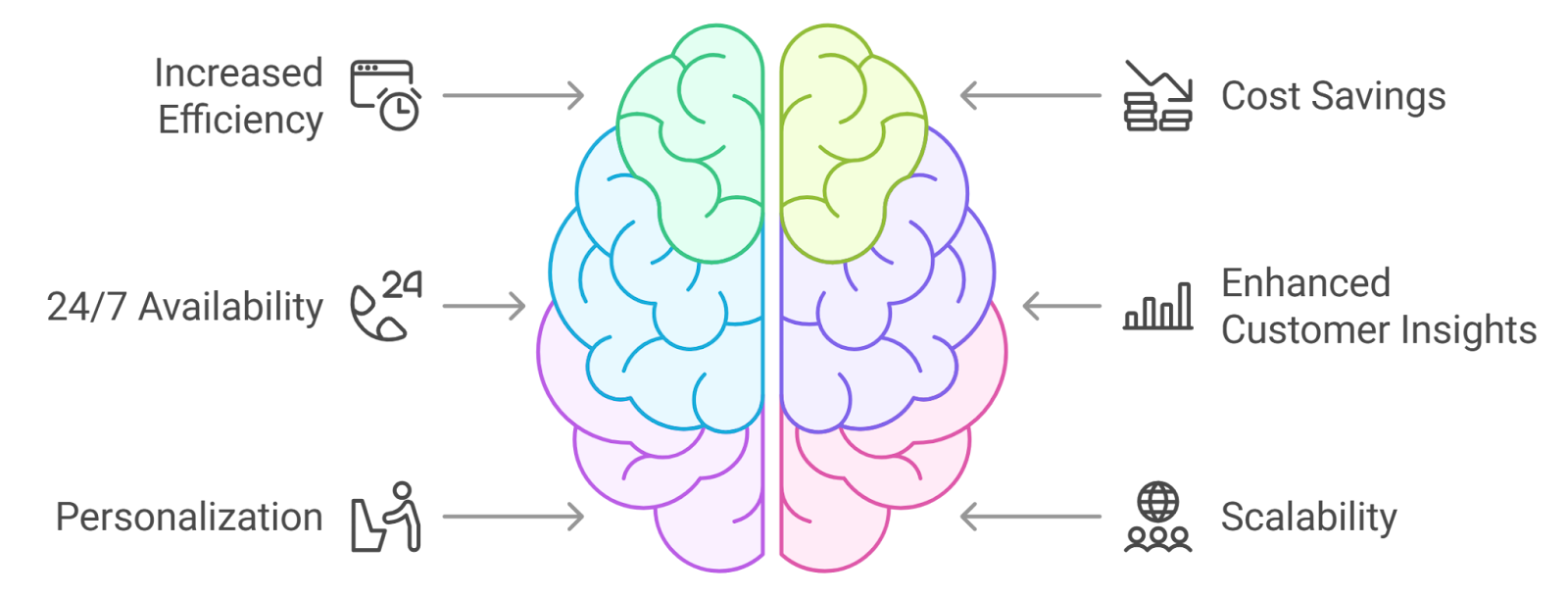
Implementing NLP in customer service offers numerous advantages for businesses, including:
- Increased Efficiency: NLP automates routine inquiries, allowing human agents to focus on more complex issues. This leads to faster resolution times and improved productivity, particularly in call center nlp environments.
- Cost Savings: By reducing the need for extensive human resources, businesses can lower operational costs. Automated systems can handle a high volume of inquiries without additional staffing.
- 24/7 Availability: NLP-powered chatbots and virtual assistants can operate around the clock, providing customers with immediate assistance regardless of time zones.
- Enhanced Customer Insights: Analyzing customer interactions through NLP can reveal trends and patterns, helping businesses understand customer preferences and pain points, especially in customer support natural language processing.
- Personalization: NLP allows for tailored responses based on customer history and behavior, leading to a more personalized experience that can increase customer loyalty.
- Scalability: As businesses grow, NLP systems can easily scale to handle increased customer interactions without a significant increase in costs.
2.1. How Does NLP Improve Customer Experience?
NLP significantly enhances customer experience in various ways:
- Faster Response Times: With NLP, customer inquiries can be processed and responded to almost instantly, reducing wait times and improving satisfaction.
- Accurate Understanding of Queries: NLP systems can accurately interpret customer questions, leading to more relevant and helpful responses.
- Consistent Service Quality: Automated systems provide consistent answers to common questions, ensuring that all customers receive the same level of service.
- Proactive Engagement: NLP can analyze customer behavior and trigger proactive outreach, such as follow-up messages or recommendations, enhancing the overall experience.
- Multichannel Support: NLP can be integrated across various platforms, including social media, email, and chat, providing a seamless experience for customers regardless of how they choose to communicate.
- Feedback Collection: NLP tools can analyze customer feedback and sentiment, allowing businesses to make informed decisions to improve their services, particularly in nlp for support tickets.
By leveraging these components and benefits, Rapid Innovation empowers businesses to create a more efficient, responsive, and customer-centric service model that meets the evolving needs of their clientele. Our expertise in AI and NLP ensures that clients can achieve greater ROI through enhanced customer interactions and streamlined operations, including nlp help center solutions. For a more in-depth understanding, check out this comprehensive guide on Natural Language Processing in AI agents.
2.2. How Much Can NLP Reduce Customer Service Costs?
Natural Language Processing (NLP) has emerged as a transformative technology in customer service, significantly reducing operational costs. By automating various tasks, NLP can streamline processes and enhance efficiency. Here are some key ways NLP contributes to cost reduction:
- Automation of Routine Queries: NLP-powered chatbots can handle a large volume of customer inquiries without human intervention, reducing the need for extensive customer service teams. This allows businesses to allocate resources more effectively, focusing on complex issues that require human expertise.
- 24/7 Availability: Unlike human agents, NLP systems can operate around the clock, ensuring that customer queries are addressed at any time. This capability can lead to higher customer satisfaction and retention, as customers receive immediate assistance regardless of the hour.
- Reduced Training Costs: With NLP systems, the need for extensive training programs for customer service representatives diminishes. The technology can be updated and improved without the need for retraining staff, allowing organizations to maintain a leaner workforce while still providing high-quality service.
- Improved Response Times: NLP can analyze and respond to customer inquiries in real-time, leading to faster resolution of issues and reducing the time agents spend on each call. This efficiency not only enhances customer satisfaction but also allows businesses to handle a higher volume of inquiries.
- Scalability: As businesses grow, NLP systems can easily scale to handle increased customer interactions without a proportional increase in costs. This flexibility is crucial for companies looking to expand their operations without compromising service quality.
According to a report by McKinsey, companies that implement AI technologies, including NLP customer service cost reduction, can reduce customer service costs by up to 30%.
2.3. What Customer Insights Can You Get from NLP Analytics?
NLP analytics provides businesses with valuable insights into customer behavior, preferences, and sentiments. By analyzing customer interactions, companies can gain a deeper understanding of their audience. Here are some insights that can be derived from NLP analytics:
- Sentiment Analysis: NLP can assess customer emotions expressed in text, helping businesses understand how customers feel about their products or services. This understanding can inform marketing strategies and product development.
- Trend Identification: By analyzing large volumes of customer feedback, NLP can identify emerging trends and topics of interest, allowing businesses to adapt their strategies accordingly and stay ahead of the competition.
- Customer Segmentation: NLP can categorize customers based on their interactions, preferences, and behaviors, enabling targeted marketing and personalized service. This segmentation can lead to more effective campaigns and improved customer engagement.
- Feedback Analysis: Businesses can analyze customer feedback to identify common pain points and areas for improvement, leading to enhanced product development and service delivery. This proactive approach can significantly boost customer loyalty.
- Predictive Insights: NLP can help predict future customer behavior based on historical data, allowing businesses to proactively address potential issues and tailor their offerings to meet customer needs.
These insights can lead to improved customer experiences and more informed business decisions, ultimately driving growth and profitability.
3. Top NLP Applications in Modern Customer Service
NLP technology is being widely adopted in various applications within customer service, enhancing efficiency and customer satisfaction. Here are some of the top NLP applications:
- Chatbots and Virtual Assistants: These AI-driven tools can handle customer inquiries, provide information, and assist with transactions, all while learning from interactions to improve over time. This continuous learning process ensures that the service becomes more effective and personalized.
- Voice Recognition Systems: NLP enables voice-activated customer service solutions, allowing customers to interact with systems using natural language, making the experience more intuitive and user-friendly.
- Email and Ticketing Automation: NLP can analyze incoming emails and support tickets, categorizing and prioritizing them for human agents, which streamlines the workflow and ensures that urgent issues are addressed promptly.
- Customer Feedback Analysis: Businesses can use NLP to analyze customer reviews, surveys, and social media comments to gauge sentiment and identify areas for improvement. This analysis can inform strategic decisions and enhance brand reputation.
- Knowledge Management: NLP can help in organizing and retrieving information from large databases, making it easier for customer service agents to find answers quickly. This efficiency not only improves response times but also enhances the overall customer experience.
These applications not only improve operational efficiency but also enhance the overall customer experience, making them essential tools in modern customer service strategies. At Rapid Innovation, we leverage these NLP capabilities to help our clients achieve their business goals effectively and efficiently, ultimately driving greater ROI.
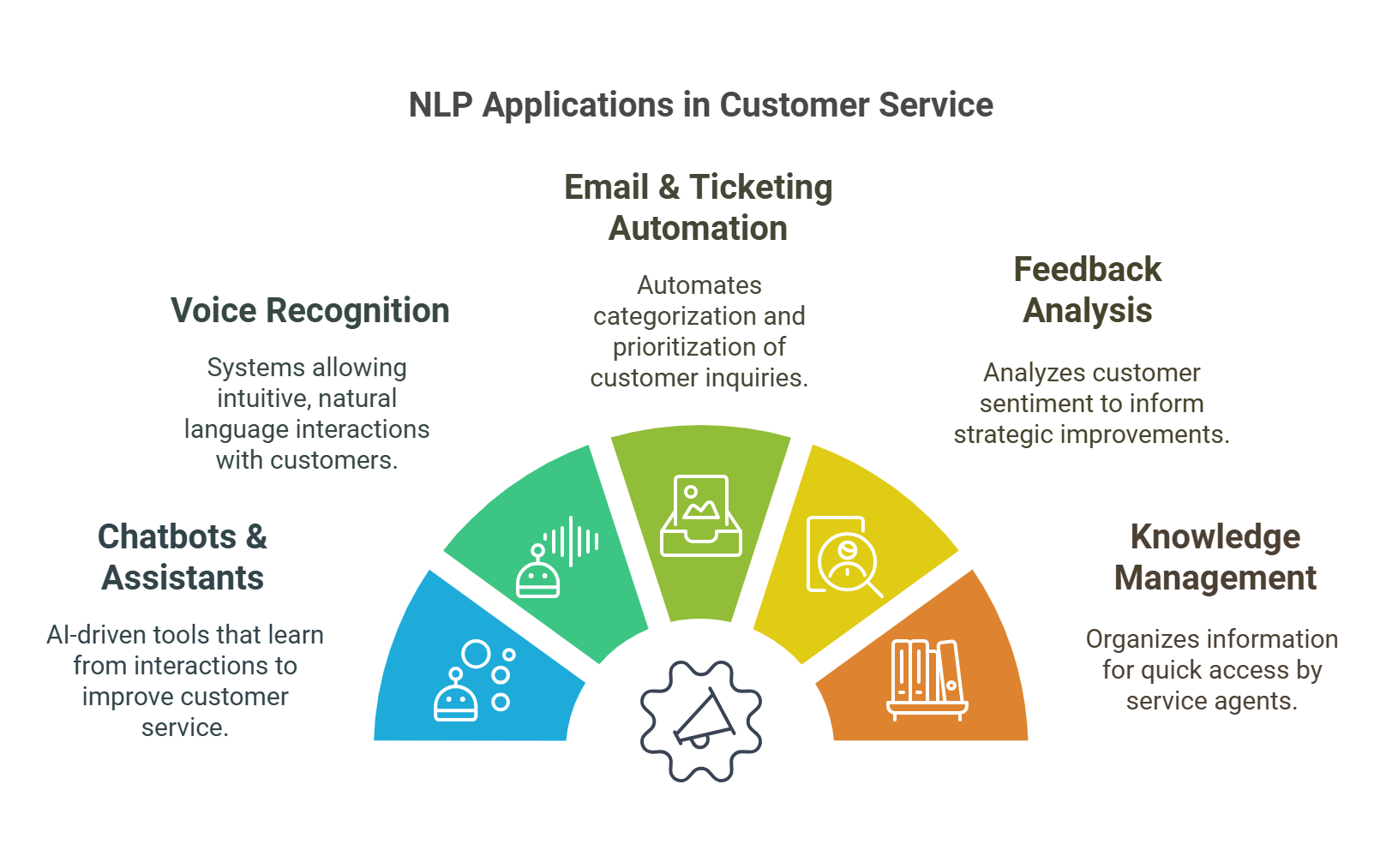
3.1. How Do AI Chatbots Use NLP for Customer Support?
AI chatbots leverage Natural Language Processing (NLP) to enhance customer support by understanding and responding to user inquiries in a human-like manner. NLP enables chatbots to interpret the nuances of human language, making interactions more efficient and effective.
- Understanding User Queries: NLP algorithms analyze the text input from customers, breaking down sentences to identify keywords and phrases. This helps chatbots understand the context and intent behind the queries, allowing Rapid Innovation to develop tailored solutions that meet specific client needs, such as conversational ai for customer support.
- Contextual Responses: By utilizing NLP, chatbots can maintain context throughout a conversation. They can remember previous interactions and provide relevant responses, improving the overall customer experience. This capability can lead to increased customer satisfaction and retention, ultimately driving greater ROI for businesses.
- Multilingual Support: NLP allows chatbots to communicate in multiple languages, catering to a diverse customer base. This is crucial for global businesses aiming to provide support in various regions, enabling them to expand their market reach effectively.
- 24/7 Availability: AI chatbots powered by NLP can operate around the clock, providing instant responses to customer inquiries without the need for human intervention. This ensures that customers receive timely assistance at any hour, enhancing operational efficiency and reducing support costs.
3.2. How Does NLP Detect Customer Intent and Sentiment?
NLP plays a vital role in detecting customer intent and sentiment, which is essential for tailoring responses and improving customer satisfaction.
- Intent Recognition: NLP algorithms classify user inputs to determine the underlying intent. For example, if a customer types "I want to return my order," the system recognizes the intent to initiate a return process. This capability allows Rapid Innovation to create systems that streamline customer interactions and improve service delivery.
- Sentiment Analysis: NLP can analyze the emotional tone of customer messages. By identifying positive, negative, or neutral sentiments, businesses can gauge customer satisfaction and respond appropriately. This insight can inform strategic decisions, enhancing customer engagement and loyalty.
- Keyword Extraction: NLP techniques extract important keywords from customer interactions, helping to identify specific issues or requests. This aids in prioritizing responses based on urgency or importance, allowing businesses to allocate resources more effectively.
- Contextual Understanding: Advanced NLP models can understand the context of conversations, allowing for more accurate intent detection. This is particularly useful in complex queries where the intent may not be immediately clear, ensuring that customer needs are met promptly.
3.3. Can NLP Automate Ticket Classification?
NLP can significantly streamline the ticket classification process in customer support systems, enhancing efficiency and reducing response times.
- Automated Categorization: NLP algorithms can automatically categorize incoming support tickets based on their content. This reduces the need for manual sorting and ensures that tickets are directed to the appropriate department or agent, optimizing workflow and resource allocation.
- Improved Accuracy: By analyzing the text of support tickets, NLP can improve the accuracy of classification. This minimizes the chances of misclassification, ensuring that customer issues are addressed promptly and effectively.
- Prioritization of Tickets: NLP can assess the urgency of tickets by analyzing keywords and sentiment. High-priority issues can be flagged for immediate attention, improving overall response times and enhancing customer satisfaction.
- Integration with Helpdesk Systems: Many helpdesk platforms now incorporate NLP capabilities, allowing for seamless integration into existing workflows. This enhances the overall efficiency of customer support operations, enabling businesses to achieve greater ROI through improved service delivery and customer engagement, including the use of ai chatbots for customer support.
At Rapid Innovation, we harness the power of AI and NLP to provide our clients with innovative solutions that drive efficiency, enhance customer experiences, and ultimately lead to increased profitability.
3.4. How to Provide Multilingual Support with NLP?

Providing multilingual support using Natural Language Processing (NLP) is essential for businesses operating in diverse markets. NLP can help bridge language barriers, ensuring effective communication with customers across different regions. Here are some strategies to implement multilingual support:
- Language Detection: Utilize NLP algorithms to automatically detect the language of incoming customer queries. This allows for immediate routing to the appropriate language support team or automated response system, enhancing operational efficiency.
- Translation Services: Integrate machine translation tools that leverage NLP to convert customer messages into the preferred language of the support agent. This integration can significantly enhance response accuracy and speed, leading to improved customer satisfaction.
- Sentiment Analysis: Implement sentiment analysis in multiple languages to gauge customer emotions and satisfaction levels. This capability helps tailor responses based on the customer's emotional state, regardless of language, thereby fostering a more personalized customer experience.
- Training Multilingual Models: Develop and train NLP models on diverse datasets that encompass various languages. This ensures that the models can effectively understand and process different linguistic nuances, which is crucial for accurate communication.
- User Interface Localization: Ensure that your customer service platforms are localized, meaning that not only the language but also cultural references and idioms are adapted for different regions. This approach enhances user experience and engagement.
- Feedback Loop: Establish a feedback mechanism to continuously improve the multilingual support system. Collecting data on customer interactions allows for the refinement of language models and translation accuracy, ensuring that the support system evolves with customer needs.
4. How to Implement NLP Solutions in Customer Service?
Implementing NLP solutions in customer service can significantly enhance efficiency and customer satisfaction. Here are key steps to consider:
- Identify Use Cases: Determine specific areas where NLP can add value, such as chatbots, automated ticketing systems, or sentiment analysis tools. This targeted approach ensures that resources are allocated effectively.
- Choose the Right Tools: Select NLP tools and platforms that align with your business needs. Consider factors like ease of integration, scalability, and language support to ensure a smooth implementation process.
- Data Collection: Gather historical customer interaction data to train your NLP models. This data is crucial for improving the accuracy and relevance of responses, ultimately leading to better customer experiences.
- Integration with Existing Systems: Ensure that the NLP solution integrates seamlessly with your existing customer service platforms, such as CRM systems or helpdesk software. This integration is vital for maintaining operational continuity.
- Pilot Testing: Conduct pilot tests to evaluate the effectiveness of the NLP solution. Monitor performance metrics like response time, customer satisfaction, and resolution rates to assess the impact of the implementation.
- Training and Support: Provide training for your customer service team on how to use the NLP tools effectively. Continuous support is essential for maximizing the benefits of the technology and ensuring that staff are well-equipped to handle customer inquiries.
- Monitor and Optimize: Regularly analyze the performance of your NLP solutions. Use customer feedback and interaction data to make necessary adjustments and improvements, ensuring that the system remains responsive to evolving customer needs.
4.1. Assessing Your Organization's NLP Readiness
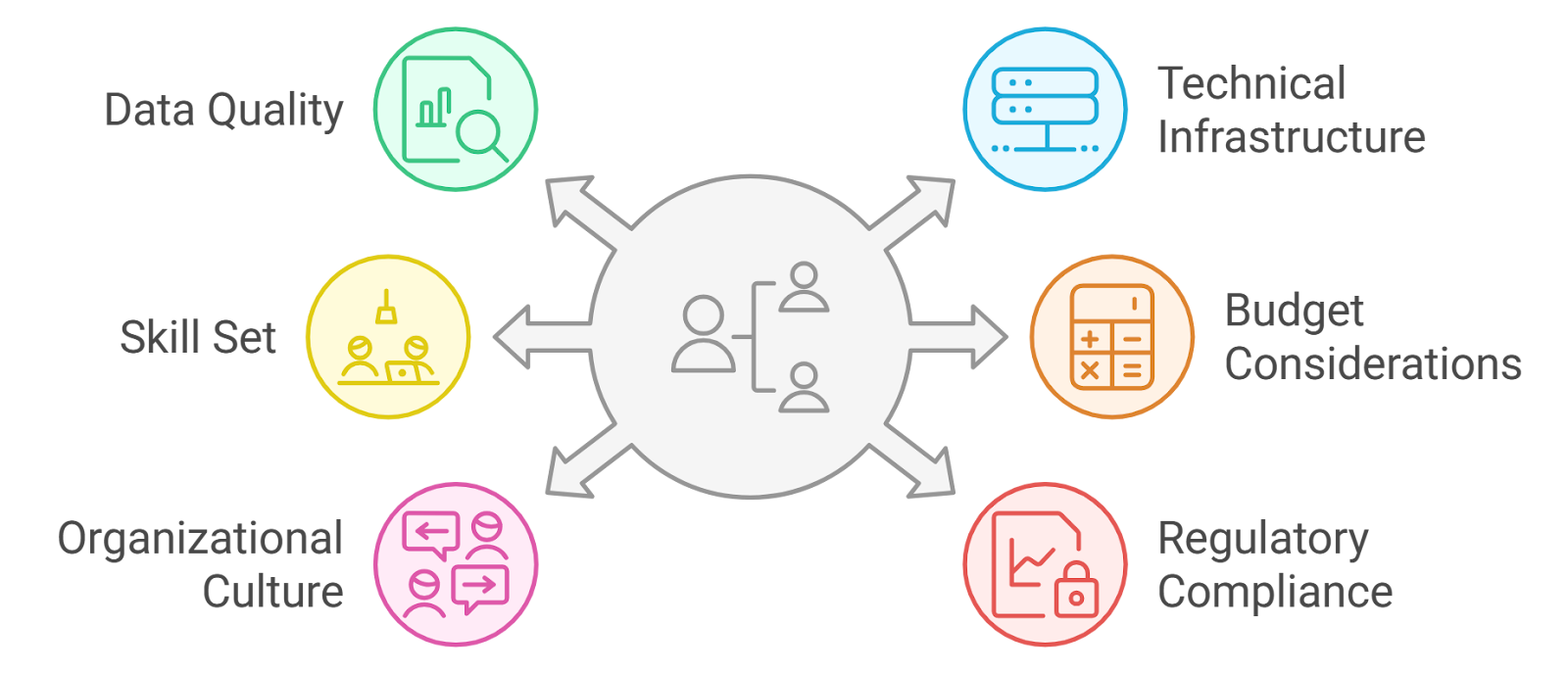
Before implementing NLP solutions, it’s crucial to assess your organization’s readiness. This evaluation can help identify potential challenges and areas for improvement. Here are some factors to consider:
- Data Quality: Evaluate the quality and quantity of your existing customer interaction data. High-quality data is essential for training effective NLP models, which in turn drives better customer engagement.
- Technical Infrastructure: Assess your current technology stack to determine if it can support NLP tools. Consider factors like processing power, storage, and integration capabilities to ensure a robust implementation.
- Skill Set: Analyze the skill set of your team. Ensure that you have personnel with expertise in data science, machine learning, and NLP to manage and optimize the solutions effectively.
- Budget Considerations: Determine the budget available for implementing NLP solutions. This includes costs for software, training, and ongoing maintenance, which are critical for long-term success.
- Organizational Culture: Consider the willingness of your organization to adopt new technologies. A culture that embraces innovation will facilitate smoother implementation and greater acceptance of NLP solutions.
- Regulatory Compliance: Ensure that your NLP solutions comply with relevant regulations, especially regarding data privacy and security. This compliance is essential for maintaining customer trust and safeguarding sensitive information.
- Customer Expectations: Understand your customers' expectations regarding service quality and response times. This insight will guide the implementation of NLP solutions to meet or exceed these expectations, ultimately driving greater customer loyalty and satisfaction.
At Rapid Innovation, we leverage our expertise in AI and NLP, including multilingual support nlp, to help businesses implement these strategies effectively, ensuring that you achieve your business goals efficiently and effectively while maximizing your return on investment.
4.2. Choosing the Right NLP Tools and Platforms
Selecting the appropriate Natural Language Processing (NLP) tools for customer service is crucial for enhancing customer service. The right tools can streamline operations, improve customer interactions, and provide valuable insights. Here are key considerations when choosing NLP tools:
- Functionality: Assess the specific features offered by the NLP tool. Look for capabilities such as sentiment analysis, entity recognition, and language translation. Ensure the tool aligns with your customer service goals.
- Ease of Integration: Choose platforms that can easily integrate with your existing systems, such as CRM software or chatbots. This will minimize disruption and allow for a smoother transition.
- Scalability: Opt for tools that can grow with your business. As your customer base expands, your NLP needs may evolve, so select a platform that can accommodate increased demand.
- User-Friendliness: The tool should be intuitive for your team to use. A user-friendly interface can reduce training time and improve adoption rates among staff.
- Cost: Evaluate the pricing structure of the NLP tools. Consider both upfront costs and ongoing expenses. Look for solutions that offer a good balance between features and affordability.
- Support and Community: Check for available customer support and community resources. A strong support system can help troubleshoot issues and provide guidance on best practices.
- Performance Metrics: Investigate how the tool measures performance. Look for analytics features that provide insights into customer interactions and overall effectiveness.
4.3. How to Integrate NLP with Existing Customer Service Systems?

Integrating NLP with your current customer service systems can significantly enhance efficiency and customer satisfaction. Here are steps to ensure a successful integration:
- Assess Current Systems: Begin by evaluating your existing customer service platforms. Identify areas where NLP can add value, such as automating responses or analyzing customer feedback.
- Choose the Right NLP Solution: Select an NLP tool that aligns with your identified needs. Ensure it can seamlessly connect with your current systems, whether through APIs or other integration methods.
- Develop a Clear Integration Plan: Create a detailed plan outlining the integration process. Include timelines, responsibilities, and key milestones to keep the project on track.
- Test the Integration: Before full deployment, conduct thorough testing. This will help identify any issues and ensure that the NLP tool functions as intended within your existing systems.
- Train Your Team: Provide training for your customer service team on how to use the new NLP features. This will help them leverage the technology effectively and improve customer interactions.
- Monitor Performance: After integration, continuously monitor the performance of the NLP system. Use analytics to assess its impact on customer service metrics and make adjustments as needed.
- Gather Feedback: Solicit feedback from both customers and staff regarding the new system. This can provide insights into areas for improvement and help refine the integration.
5. Best Practices for NLP-Driven Customer Service

Implementing NLP in customer service can lead to significant improvements, but following best practices is essential for maximizing its benefits. Here are some key practices to consider:
- Understand Customer Intent: Use NLP to analyze customer inquiries and understand their intent. This can help in providing more accurate and relevant responses.
- Personalize Interactions: Leverage NLP to personalize customer interactions. Tailoring responses based on previous interactions can enhance customer satisfaction and loyalty.
- Utilize Chatbots Effectively: Deploy chatbots powered by NLP tools for customer service to handle routine inquiries. This can free up human agents to focus on more complex issues, improving overall efficiency.
- Continuously Train the Model: Regularly update and train your NLP models with new data. This ensures that the system remains accurate and relevant as language and customer needs evolve.
- Monitor and Analyze Performance: Use analytics to track the performance of your NLP-driven customer service. Identify trends, measure success, and make data-driven decisions for improvements.
- Encourage Human Oversight: While NLP can automate many tasks, human oversight is still crucial. Ensure that complex or sensitive issues are escalated to human agents for resolution.
- Stay Updated on Trends: Keep abreast of the latest developments in NLP technology. Staying informed can help you adapt and implement new features that enhance customer service.
- Focus on Security and Privacy: Ensure that your NLP systems comply with data protection regulations. Safeguarding customer data is essential for maintaining trust and credibility.
By following these best practices, businesses can effectively leverage NLP to enhance their customer service operations, leading to improved customer experiences and increased satisfaction.
At Rapid Innovation, we specialize in helping businesses select and integrate the right NLP tools for customer service tailored to their unique needs. Our expertise ensures that you not only choose the best solutions but also implement them effectively, maximizing your return on investment and enhancing customer engagement.
5.1. Training and Fine-tuning NLP Models
Training and fine-tuning Natural Language Processing (NLP) models is crucial for achieving high performance in various applications, such as chatbots, sentiment analysis, and language translation. At Rapid Innovation, we leverage our expertise in AI to guide clients through this process, ensuring they achieve optimal results and greater ROI. The process involves several key steps:
- Data Collection: Gather a diverse dataset that represents the language and context in which the model will operate. This can include text from social media, customer reviews, or domain-specific documents. Our team assists clients in identifying and sourcing high-quality data tailored to their specific needs.
- Preprocessing: Clean and preprocess the data to remove noise. This includes tokenization, stemming, and removing stop words. Proper preprocessing ensures that the model learns from relevant information, which we optimize to enhance model performance.
- Model Selection: Choose an appropriate NLP model architecture. Options include traditional models like Bag of Words and advanced models like Transformers (e.g., BERT, GPT). The choice depends on the complexity of the task and the available computational resources. We help clients select the best model based on their unique requirements and constraints.
- Training: Train the model using the prepared dataset. This involves feeding the data into the model and adjusting the weights based on the loss function. Techniques like transfer learning can be employed to leverage pre-trained language models, reducing training time and improving performance. Our expertise ensures that clients benefit from the latest advancements in AI, including pretrained language models and self training improves pre training for natural language understanding.
- Fine-tuning: After initial training, fine-tune the model on a smaller, task-specific dataset. This step helps the model adapt to the nuances of the specific application, enhancing its accuracy and relevance. We provide tailored fine-tuning strategies that align with our clients' business objectives, including fine tuned language models for text classification.
- Evaluation: Assess the model's performance using metrics like accuracy, precision, recall, and F1 score. This evaluation helps identify areas for improvement. Our team conducts thorough evaluations to ensure that the models meet the desired performance benchmarks, including nlp pre trained models and nlp pretrained models.
- Iteration: Based on evaluation results, iterate on the model by adjusting hyperparameters, adding more data, or refining preprocessing steps. We emphasize a continuous improvement approach, enabling clients to achieve sustained performance enhancements, particularly in nlp model training and adapting prompt-based nlp with adaprompt adaptive model training for prompt based nlp and best practices for transformer model development.
5.2. How to Balance Human and AI Customer Service?

Balancing human and AI customer service is essential for providing an optimal customer experience. At Rapid Innovation, we implement strategies that help our clients achieve this balance effectively:
- Define Roles: Clearly outline the roles of AI and human agents. AI can handle routine inquiries, while human agents can manage complex issues requiring empathy and nuanced understanding. We assist clients in defining these roles to maximize efficiency.
- Use AI for Initial Contact: Implement AI chatbots for first-line support. They can quickly address common questions, freeing human agents to focus on more complicated cases. Our solutions are designed to enhance customer engagement while reducing operational costs.
- Seamless Handover: Ensure a smooth transition from AI to human agents when necessary. Customers should be able to escalate their issues easily without repeating information. We develop systems that facilitate this seamless interaction, improving customer satisfaction.
- Monitor Performance: Regularly assess the performance of both AI and human agents. Use metrics like response time, resolution rate, and customer satisfaction scores to identify areas for improvement. Our analytics tools provide actionable insights for continuous enhancement.
- Training and Development: Invest in training for human agents to enhance their skills in areas where AI falls short, such as emotional intelligence and problem-solving. We offer training programs that empower human agents to excel in their roles.
- Feedback Loop: Create a feedback mechanism where customers can share their experiences with both AI and human interactions. Use this data to refine both systems. Our approach ensures that client services evolve based on real customer feedback.
5.3. How to Ensure NLP Data Privacy and Security?
Ensuring data privacy and security in NLP applications is paramount, especially when handling sensitive information. Rapid Innovation emphasizes best practices to safeguard client data:
- Data Anonymization: Remove personally identifiable information (PII) from datasets before training models. Techniques like tokenization and hashing can help protect user identities. We implement robust anonymization techniques to ensure compliance with privacy standards.
- Access Control: Implement strict access controls to limit who can view and manipulate data. Use role-based access to ensure that only authorized personnel can access sensitive information. Our security protocols are designed to protect client data integrity.
- Encryption: Use encryption methods to protect data both at rest and in transit. This ensures that even if data is intercepted, it remains unreadable without the proper decryption keys. We integrate advanced encryption technologies to enhance data security.
- Regular Audits: Conduct regular security audits and vulnerability assessments to identify and address potential weaknesses in your data handling processes. Our proactive approach helps clients maintain compliance and security.
- Compliance with Regulations: Stay informed about data protection regulations such as GDPR and CCPA. Ensure that your NLP applications comply with these laws to avoid legal repercussions. We guide clients through the compliance landscape, ensuring adherence to relevant regulations.
- User Consent: Obtain explicit consent from users before collecting and processing their data. Clearly communicate how their data will be used and stored. Our solutions prioritize transparency and user trust.
- Incident Response Plan: Develop a robust incident response plan to address potential data breaches. This plan should outline steps for containment, investigation, and notification of affected users. We assist clients in creating comprehensive incident response strategies to mitigate risks effectively.
6. Measuring Success with NLP in Customer Service
Natural Language Processing (NLP) has transformed customer service by enabling businesses to understand and respond to customer inquiries more effectively. Measuring the success of NLP implementations is crucial for ensuring that these technologies deliver the desired outcomes.
6.1. Key Performance Indicators (KPIs) for NLP Implementation

To evaluate the effectiveness of NLP in customer service, businesses should focus on specific Key Performance Indicators (KPIs). These metrics help in assessing how well NLP tools are performing and their impact on customer satisfaction and operational efficiency.
- Customer Satisfaction Score (CSAT): This metric gauges customer satisfaction with the service received. A higher CSAT indicates that NLP tools are effectively addressing customer needs.
- First Response Time (FRT): This measures the time taken to respond to a customer inquiry. NLP can significantly reduce FRT by automating responses, leading to quicker resolutions.
- Resolution Rate: This KPI tracks the percentage of customer issues resolved on the first contact. An increase in resolution rates often indicates that NLP is effectively understanding and addressing customer queries.
- Volume of Automated Interactions: This metric assesses how many customer interactions are handled by NLP systems without human intervention. A higher volume suggests successful implementation of NLP technologies, such as nlp in customer service and customer support nlp.
- Sentiment Analysis: By analyzing customer feedback and interactions, businesses can gauge overall sentiment towards their service. Positive sentiment trends can indicate effective NLP usage, particularly in customer support natural language processing.
- Cost per Interaction: This measures the cost associated with handling customer inquiries. NLP can lower this cost by automating responses and reducing the need for human agents.
- Agent Productivity: This KPI evaluates how much work customer service agents can handle. With NLP tools assisting in routine inquiries, agents can focus on more complex issues, improving overall productivity, especially in scenarios like nlp for support tickets.
6.2. How to Calculate ROI for NLP in Customer Service?
Calculating the Return on Investment (ROI) for NLP in customer service involves assessing both the financial benefits and the costs associated with implementing these technologies. A clear understanding of ROI helps businesses justify their investments in NLP solutions.
- Identify Costs: Start by calculating the total costs associated with NLP implementation. This includes:
- Software and licensing fees
- Training costs for staff
- Maintenance and support expenses
- Integration costs with existing systems
- Measure Benefits: Next, quantify the benefits gained from NLP. This can include:
- Increased customer satisfaction leading to higher retention rates
- Reduction in operational costs due to automation
- Decreased average handling time (AHT) for customer inquiries
- Increased sales from improved customer engagement, particularly through natural language processing customer support initiatives
- Calculate ROI: Use the following formula to calculate ROI:
language="language-plaintext"``` -a1b2c3- ROI = (Net Benefits / Total Costs) x 100
- Net Benefits: This is the total benefits minus the total costs. A positive ROI indicates that the benefits of NLP implementation outweigh the costs, making it a worthwhile investment.
- Monitor Over Time: ROI should not be a one-time calculation. Continuously monitor the KPIs and adjust the ROI calculation as the business evolves and as more data becomes available.
- Consider Intangible Benefits: While financial metrics are crucial, also consider intangible benefits such as improved brand reputation, enhanced customer loyalty, and better employee satisfaction. These factors can significantly contribute to long-term success but may not be easily quantifiable.
By focusing on these KPIs and calculating ROI effectively, businesses can measure the success of their NLP initiatives in customer service, ensuring they maximize the benefits of this powerful technology. At Rapid Innovation, we leverage our expertise in AI to help clients implement NLP solutions that not only enhance customer service but also drive significant ROI, aligning with their business goals efficiently and effectively, including strategies for nlp help center optimization.
6.3. Continuous Improvement Strategies
Continuous improvement strategies are essential for enhancing the effectiveness of Natural Language Processing (NLP) in customer service improvement. These strategies focus on refining processes, technologies, and customer interactions to ensure optimal performance and satisfaction.
- Regular Training and Updates:
- Implement ongoing training programs for NLP models to adapt to new language patterns and customer preferences.
- Update algorithms regularly to incorporate the latest advancements in machine learning and AI.
- Feedback Loops:
- Establish mechanisms for collecting customer feedback on NLP interactions.
- Use this feedback to identify areas for improvement and adjust NLP systems accordingly.
- Performance Metrics:
- Monitor key performance indicators (KPIs) such as response time, accuracy, and customer satisfaction scores.
- Analyze data to identify trends and areas needing enhancement.
- Cross-Functional Collaboration:
- Foster collaboration between customer service teams, data scientists, and IT departments.
- Share insights and best practices to drive improvements in NLP applications.
- A/B Testing:
- Conduct A/B testing to evaluate different NLP models or approaches.
- Use results to determine the most effective strategies for customer interactions.
- Scalability Considerations:
- Ensure that NLP systems can scale with growing customer demands.
- Regularly assess infrastructure and resources to support increased usage.
- Innovation Adoption:
- Stay updated on emerging technologies and methodologies in NLP.
- Integrate innovative solutions that can enhance customer service improvement capabilities, such as AI customer service agents.
7. Future Trends in NLP Customer Service
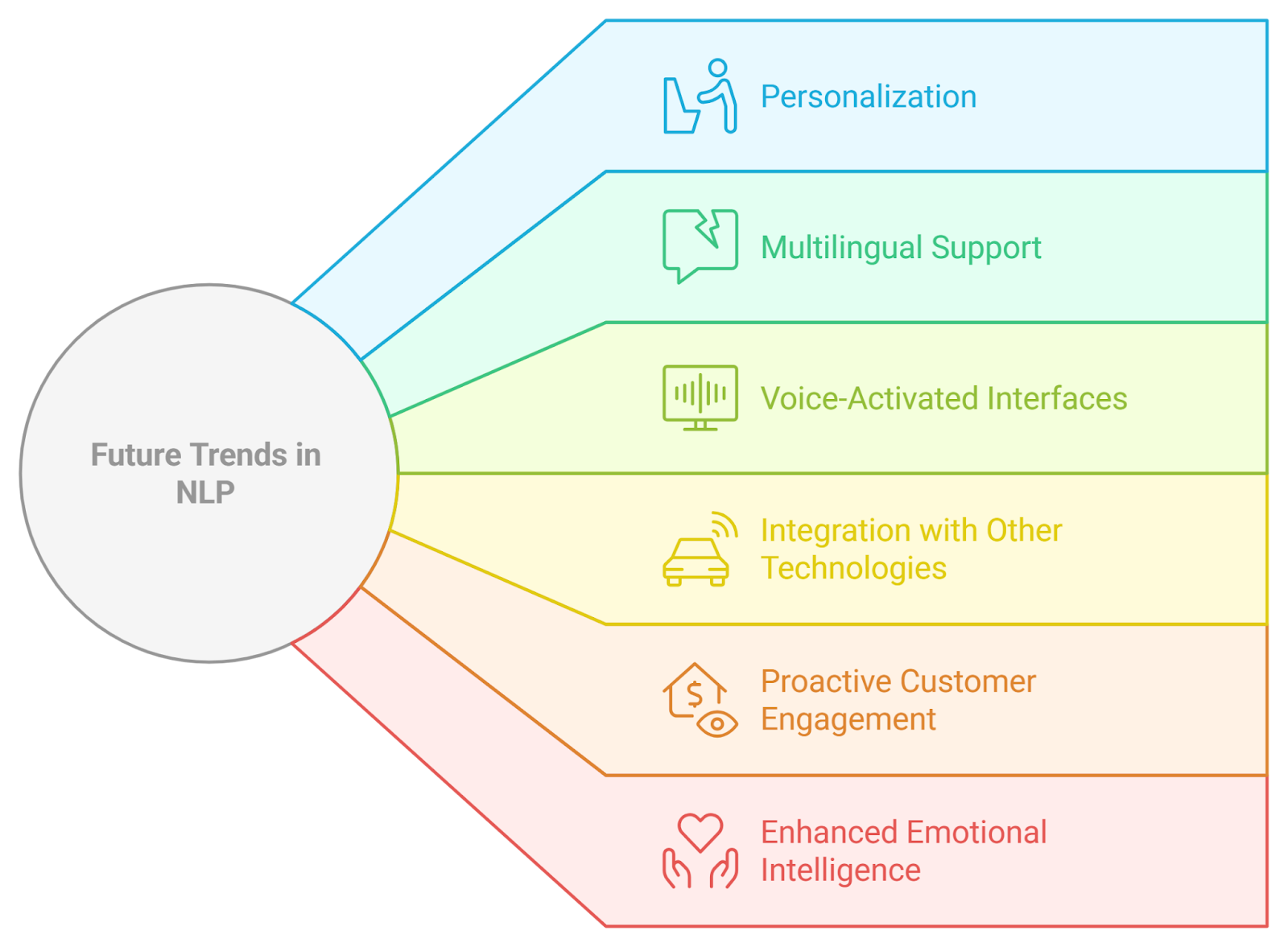
The future of NLP in customer service is poised for significant transformation, driven by advancements in technology and changing customer expectations. Understanding these trends can help businesses stay competitive and improve customer experiences.
- Personalization:
- Increasingly, NLP systems will leverage data to provide personalized responses and recommendations, enhancing customer satisfaction and loyalty.
- Multilingual Support:
- As businesses expand globally, NLP will evolve to support multiple languages and dialects, enabling companies to serve diverse customer bases effectively.
- Voice-Activated Interfaces:
- The rise of voice-activated devices will lead to more voice-based customer service interactions, with NLP playing a crucial role in understanding and processing spoken language.
- Integration with Other Technologies:
- NLP will increasingly integrate with other technologies like chatbots, machine learning, and analytics, creating more seamless and efficient customer service experiences.
- Proactive Customer Engagement:
- Future NLP systems will focus on proactive engagement, anticipating customer needs before they arise, which can lead to improved customer satisfaction and reduced service inquiries.
- Enhanced Emotional Intelligence:
- Advances in sentiment analysis will enable NLP systems to better understand customer emotions, allowing for more empathetic and effective interactions.
7.1. Emerging NLP Technologies and Innovations
Emerging NLP technologies and innovations are reshaping the landscape of customer service, offering new opportunities for businesses to enhance their operations and customer interactions.
- Transformer Models:
- Transformer architectures, such as BERT and GPT, are revolutionizing NLP by improving context understanding and response generation, allowing these models to handle complex queries and provide more accurate responses.
- Contextual Understanding:
- Innovations in contextual understanding allow NLP systems to grasp the nuances of customer inquiries, leading to more relevant and precise answers, which enhances the overall customer experience.
- Real-Time Language Translation:
- Emerging technologies enable real-time translation of customer interactions across different languages, breaking down language barriers and facilitating global customer support.
- Sentiment Analysis Tools:
- Advanced sentiment analysis tools are being developed to gauge customer emotions during interactions, helping businesses tailor their responses based on customer sentiment.
- Automated Content Generation:
- Innovations in automated content generation allow for the creation of personalized responses and FAQs, significantly reducing response times and improving customer engagement.
- AI-Powered Analytics:
- AI-driven analytics tools are emerging to analyze customer interactions and derive actionable insights, informing strategies for improving customer service and NLP performance.
- Ethical AI Practices:
- As NLP technologies evolve, there is a growing emphasis on ethical AI practices, with businesses focusing on transparency, fairness, and accountability in their NLP applications.
At Rapid Innovation, we leverage these continuous improvement strategies and emerging technologies to help our clients enhance their customer service improvement capabilities. By implementing tailored NLP solutions, we enable businesses to achieve greater ROI through improved customer satisfaction, operational efficiency, and data-driven decision-making. Our expertise in AI and blockchain ensures that our clients stay ahead of the curve in an ever-evolving digital landscape.
7.2. Predictive Customer Service Models
Predictive customer service models leverage data analytics and machine learning to anticipate customer needs and behaviors. These models analyze historical data to identify patterns and trends, enabling businesses to proactively address customer issues before they escalate.
- Key components of predictive customer service models include:
- Data Collection: Gathering data from various sources such as customer interactions, purchase history, and feedback.
- Data Analysis: Utilizing algorithms to analyze the data and identify trends that can predict future customer behavior.
- Proactive Engagement: Implementing strategies to reach out to customers based on predictions, such as offering support before a problem arises.
- Benefits of predictive customer service models:
- Improved Customer Satisfaction: By anticipating needs, businesses can provide timely solutions, enhancing the overall customer experience.
- Increased Efficiency: Reducing the volume of incoming inquiries by addressing potential issues proactively.
- Cost Savings: Minimizing the resources spent on reactive customer service by focusing on prevention.
Predictive customer service models can also help in segmenting customers based on their likelihood to churn, allowing businesses to tailor their retention strategies effectively. According to a report by McKinsey, companies that effectively use predictive analytics can improve their customer satisfaction scores by up to 20%.
At Rapid Innovation, we specialize in developing and implementing predictive customer service models tailored to your business needs. By harnessing the power of AI and machine learning, we can help you achieve greater ROI through enhanced customer engagement and satisfaction. Additionally, our expertise in Natural Language Processing solutions can further enhance your predictive models. For a more comprehensive understanding of how AI can transform customer service, check out our complete guide on AI in Customer Service.
7.3. The Role of Large Language Models in Customer Support
Large Language Models (LLMs) have revolutionized customer support by enabling more natural and efficient interactions between businesses and customers. These AI-driven models can understand and generate human-like text, making them invaluable in various customer service applications.
- Key roles of LLMs in customer support include:
- Chatbots and Virtual Assistants: LLMs power chatbots that can handle a wide range of customer inquiries, providing instant responses and support.
- Sentiment Analysis: Analyzing customer interactions to gauge sentiment, allowing businesses to respond appropriately to customer emotions.
- Personalization: Tailoring responses based on customer data, enhancing the relevance of interactions.
- Advantages of using LLMs in customer support:
- 24/7 Availability: LLMs can provide support around the clock, ensuring customers receive assistance whenever they need it.
- Scalability: Businesses can handle a larger volume of inquiries without a proportional increase in staff.
- Cost Efficiency: Reducing the need for extensive human resources while maintaining high-quality service.
Research indicates that companies using AI in customer service can reduce operational costs by up to 30%. The integration of LLMs not only streamlines processes but also enhances the overall customer experience by providing quick and accurate responses.
At Rapid Innovation, we leverage LLMs to create intelligent customer support solutions that drive efficiency and improve customer satisfaction, ultimately leading to a higher return on investment for your business.
8. Case Studies: NLP Success Stories in Customer Service
Natural Language Processing (NLP) has been successfully implemented in various organizations, transforming their customer service operations. Here are a few notable case studies that highlight the effectiveness of NLP in enhancing customer support.
- Zendesk: This customer service platform integrated NLP to analyze customer tickets and categorize them automatically. By doing so, they improved response times and reduced the workload on support agents. The implementation of NLP led to a 20% increase in ticket resolution speed.
- H&M: The fashion retailer utilized NLP to enhance its chatbot capabilities. By analyzing customer queries, the chatbot could provide personalized recommendations and style advice. This resulted in a significant increase in customer engagement and satisfaction, with a reported 30% boost in online sales attributed to the chatbot's effectiveness.
- American Express: The financial services company employed NLP to analyze customer feedback from various channels, including social media and surveys. By understanding customer sentiment, they were able to identify areas for improvement in their services. This proactive approach led to a 15% increase in customer retention rates.
These case studies illustrate the transformative power of NLP in customer service, showcasing how businesses can leverage technology to improve efficiency, enhance customer satisfaction, and drive revenue growth. At Rapid Innovation, we are committed to helping you implement similar solutions that align with your business objectives and maximize your ROI.
8.1. How Do Enterprises Implement NLP Successfully?
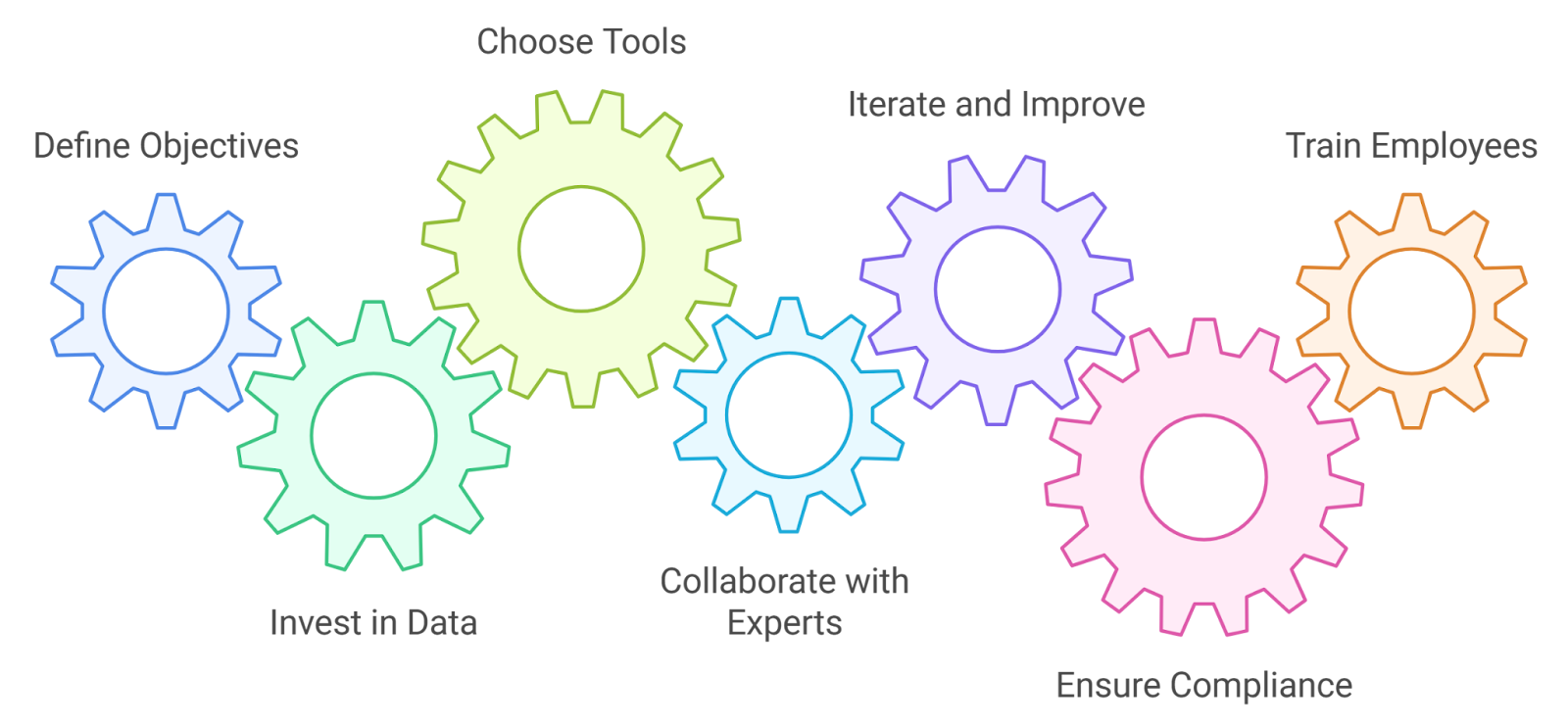
Enterprises can successfully implement Natural Language Processing (NLP) by following a structured approach that aligns with their business goals. Here are key strategies:
- Define Clear Objectives: Establish specific goals for NLP applications, such as improving customer service, automating data entry, or enhancing sentiment analysis.
- Invest in Quality Data: High-quality, relevant data is crucial for training NLP models. Enterprises should focus on collecting and curating datasets that reflect their industry and customer interactions.
- Choose the Right Tools and Technologies: Select appropriate NLP frameworks and libraries, such as
TensorFlow
,spaCy
, orNLTK
, that suit the enterprise's technical capabilities and project requirements. - Collaborate with Experts: Engage data scientists and NLP specialists to guide the implementation process. Their expertise can help in model selection, tuning, and deployment.
- Iterate and Improve: NLP models require continuous refinement. Enterprises should regularly evaluate model performance and make adjustments based on user feedback and changing data patterns.
- Ensure Compliance and Ethics: Address privacy concerns and ensure compliance with regulations like GDPR when handling customer data. Ethical considerations should be integrated into the NLP strategy.
- Train Employees: Provide training for staff to understand and leverage NLP tools effectively. This can enhance user adoption and maximize the benefits of NLP solutions.
8.2. How Can Small Businesses Benefit from NLP?
Small businesses can leverage NLP to enhance operations, improve customer engagement, and drive growth. Here are some benefits:
- Cost-Effective Customer Support: Implementing chatbots powered by NLP can provide 24/7 customer support, reducing the need for extensive customer service teams.
- Enhanced Marketing Strategies: NLP can analyze customer feedback and social media sentiment, helping small businesses tailor their marketing campaigns to better meet customer needs.
- Streamlined Operations: Automating routine tasks such as data entry and document processing can save time and reduce human error, allowing small businesses to focus on core activities.
- Improved Decision-Making: By analyzing large volumes of unstructured data, NLP can provide insights that inform strategic decisions, helping small businesses stay competitive.
- Personalized Customer Experiences: NLP can help small businesses understand customer preferences and behaviors, enabling them to offer personalized recommendations and services.
- Market Research: Small businesses can use NLP to analyze trends and consumer sentiment, providing valuable insights for product development and market positioning.
8.3. Industry-Specific Applications
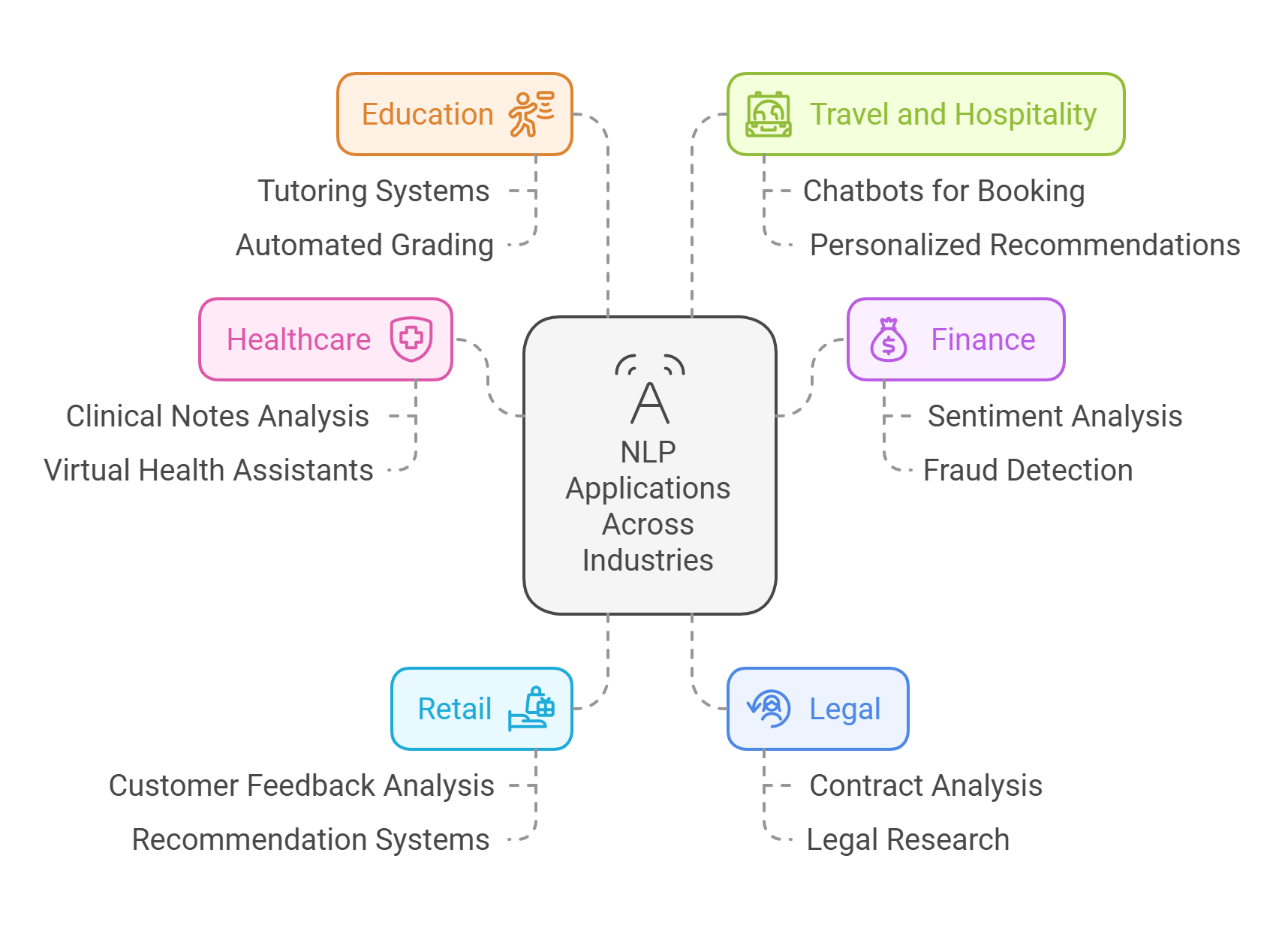
NLP has diverse applications across various industries, each tailored to meet specific needs. Here are some industry-specific examples:
- Healthcare: NLP can analyze clinical notes and patient records to extract relevant information, aiding in diagnosis and treatment recommendations. It can also power virtual health assistants to provide patient support.
- Finance: In the finance sector, NLP is used for sentiment analysis of market trends, fraud detection through anomaly detection in transaction data, and automating customer inquiries via chatbots.
- Retail: Retailers utilize NLP for inventory management by analyzing customer reviews and feedback to optimize product offerings. It also helps in personalizing shopping experiences through recommendation systems.
- Legal: NLP can assist in document review and contract analysis, significantly reducing the time lawyers spend on these tasks. It can also help in legal research by quickly identifying relevant case law.
- Education: In the education sector, NLP can enhance learning experiences through personalized tutoring systems and automated grading of written assignments, providing instant feedback to students.
- Travel and Hospitality: NLP applications in this industry include chatbots for booking assistance, sentiment analysis of customer reviews, and personalized travel recommendations based on user preferences.
At Rapid Innovation, we specialize in implementing these NLP implementation strategies and solutions tailored to your specific industry needs. Our expertise in AI and Blockchain development ensures that you achieve greater ROI through efficient and effective technology integration. By leveraging our services, enterprises can not only enhance their operational capabilities but also drive significant growth and customer satisfaction.
9. How to Get Started with NLP in Customer Service?
Natural Language Processing (NLP) is revolutionizing customer service by enabling businesses to understand and respond to customer inquiries more effectively. Implementing NLP can enhance customer satisfaction, streamline operations, and reduce response times. Here’s how to get started.
9.1. Step-by-Step Implementation Guide
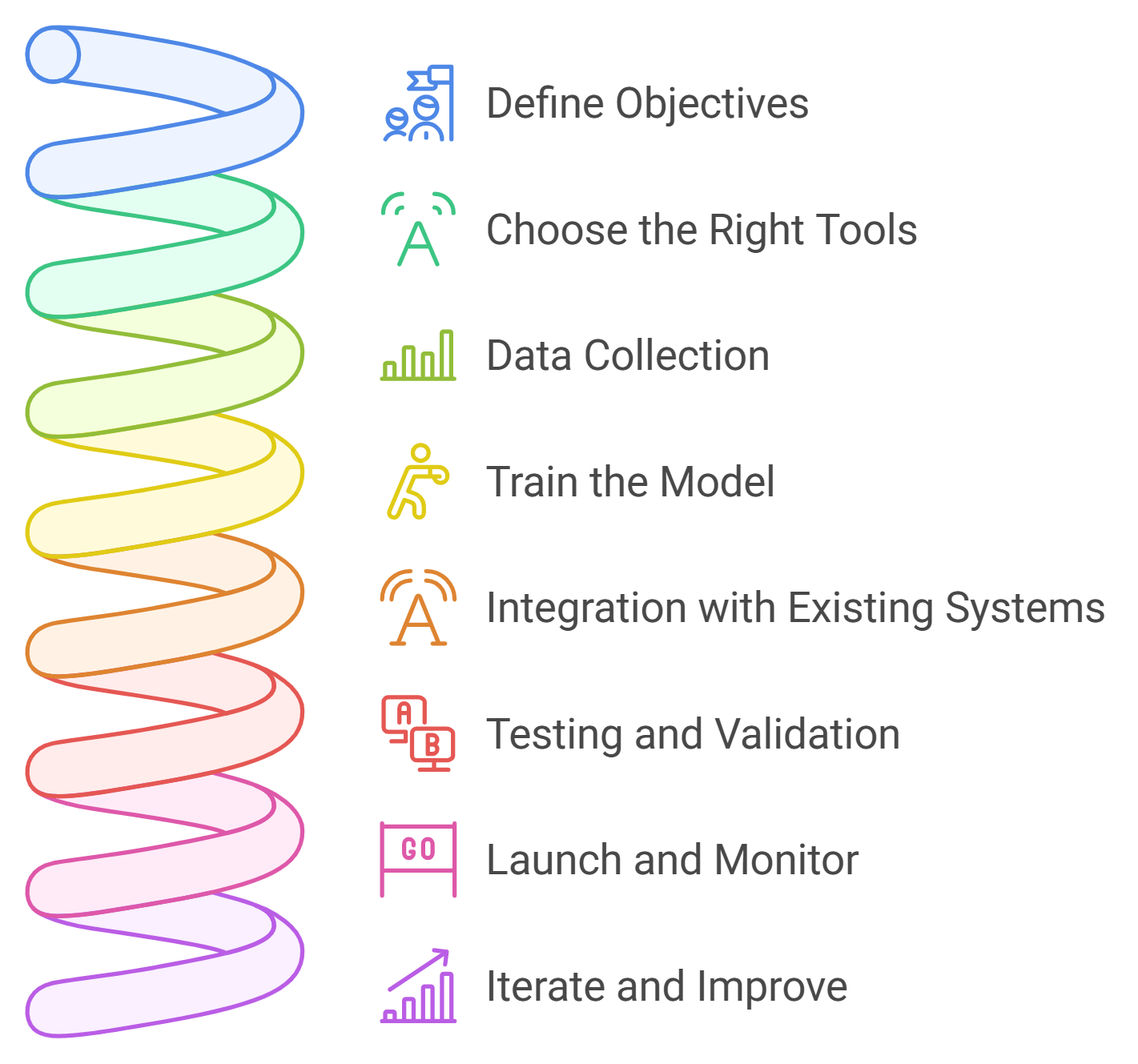
- Define Objectives: Identify specific goals for using NLP in customer service, such as improving response times, automating FAQs, or enhancing customer insights. Set measurable KPIs to track progress.
- Choose the Right Tools: Research and select NLP tools that fit your business needs. Popular options include Google Cloud Natural Language, IBM Watson, and Microsoft Azure Text Analytics. Consider factors like ease of integration, scalability, and cost.
- Data Collection: Gather historical customer interaction data, including emails, chat logs, and social media messages. Ensure data is clean and well-organized for effective analysis.
- Train the Model: Use the collected data to train your NLP model. This involves teaching the model to recognize patterns, intents, and sentiments in customer communications. Regularly update the model with new data to improve accuracy.
- Integration with Existing Systems: Integrate the NLP solution with your existing customer service platforms, such as CRM systems or chatbots. Ensure seamless communication between the NLP tool and your customer service representatives.
- Testing and Validation: Conduct thorough testing to validate the NLP model’s performance. Use real-world scenarios to assess its effectiveness and gather feedback from customer service agents to identify areas for improvement.
- Launch and Monitor: Roll out the NLP solution to your customer service team. Provide training to ensure they understand how to leverage the technology. Continuously monitor performance metrics and customer feedback to make necessary adjustments.
- Iterate and Improve: Regularly review the system’s performance and make iterative improvements based on user feedback and evolving customer needs. Stay updated with advancements in NLP technology to enhance your customer service capabilities.
9.2. Common Challenges and Solutions
- Data Privacy Concerns:
- Challenge: Handling sensitive customer data can raise privacy issues.
- Solution: Implement robust data protection measures and comply with regulations like GDPR. Anonymize data where possible to protect customer identities.
- Understanding Context:
- Challenge: NLP models may struggle with understanding context, leading to misinterpretations.
- Solution: Use advanced NLP techniques like contextual embeddings (e.g., BERT) to improve understanding. Regularly train the model with diverse data to enhance its contextual awareness.
- Integration Issues:
- Challenge: Integrating NLP tools with existing systems can be complex.
- Solution: Work with experienced developers or consultants who specialize in NLP integration. Choose tools that offer APIs for easier integration.
- User Resistance:
- Challenge: Employees may resist adopting new technology.
- Solution: Provide comprehensive training and demonstrate the benefits of NLP in easing their workload. Encourage feedback to foster a sense of ownership.
- High Initial Costs:
- Challenge: The initial investment in NLP technology can be significant.
- Solution: Start with a pilot project to demonstrate ROI before scaling up. Look for cost-effective solutions that offer essential features without unnecessary complexity.
- Maintaining Accuracy:
- Challenge: NLP models can become outdated or less accurate over time.
- Solution: Regularly update the model with new data and retrain it to maintain accuracy. Monitor performance metrics to identify when updates are needed.
- Limited Language Support:
- Challenge: Some NLP tools may not support all languages or dialects.
- Solution: Choose NLP solutions that offer multilingual support or consider developing custom models for specific languages.
By following this guide and addressing common challenges, businesses can effectively implement NLP in customer service, such as nlp in customer service, customer support natural language processing, and nlp for support tickets, leading to improved customer experiences and operational efficiency. At Rapid Innovation, we specialize in providing tailored NLP solutions that align with your business objectives, ensuring you achieve greater ROI and enhanced customer engagement. For more information, visit our AI-driven customer support page.
9.3. Resource Planning and Budget Considerations
Resource planning and budget considerations are critical components of any project or business strategy. Effective resource planning ensures that the right resources are allocated efficiently, while budget considerations help in managing financial constraints.
- Identify resource needs:
- Assess the types of resources required, including human, technological, and material resources.
- Determine the quantity and quality of resources needed to meet project goals.
- Develop a budget:
- Create a detailed budget that outlines all expected costs, including labor, materials, and overhead. Utilize a personal financial budget template or an excel budget worksheet to streamline this process.
- Include contingency funds to address unexpected expenses, using a free budget workbook for better tracking.
- Monitor resource allocation:
- Regularly review resource usage to ensure alignment with the project timeline and budget.
- Adjust allocations as necessary to optimize performance and minimize waste, potentially using a budget worksheet template excel.
- Utilize technology:
- Implement project management software to track resources and budgets in real-time, such as a free downloadable budget template.
- Use data analytics to forecast future resource needs and budget adjustments, leveraging a financial planning excel template.
- Engage stakeholders:
- Involve key stakeholders in the planning process to gain insights and ensure buy-in.
- Communicate budget constraints and resource limitations clearly to all team members, possibly using a personal budget worksheet excel.
- Evaluate and adjust:
- Conduct regular evaluations of resource utilization and budget adherence.
- Be prepared to make adjustments based on project progress and changing circumstances, utilizing a monthly budget plan template.
At Rapid Innovation, we leverage advanced AI and Blockchain technologies to enhance resource planning and budgeting processes. For instance, our AI-driven analytics tools can provide predictive insights into resource needs, allowing businesses to allocate resources more effectively and avoid over-commitment. Additionally, our Blockchain solutions can ensure transparency in budget tracking, reducing the risk of fraud and misallocation of funds. To learn more about how we can assist with your project estimation needs, visit our AI project estimation services or our project estimation company in the USA.
10. Expert Insights and FAQs
Expert insights provide valuable perspectives on industry trends, best practices, and common challenges. Frequently asked questions (FAQs) can help clarify complex topics and guide decision-making.
- Importance of expert insights:
- Leverage the knowledge of industry leaders to stay ahead of market trends.
- Gain practical advice on overcoming common obstacles in resource planning and budgeting.
- Common FAQs:
- What are the best practices for resource allocation?
- How can I effectively manage a project budget using tools like a budget planning worksheet or a budget planning tool?
- What tools are available for tracking resources and expenses, such as examples of budget templates?
- Sources of expert insights:
- Industry reports and whitepapers often contain valuable data and recommendations.
- Webinars and conferences provide opportunities to learn from experts and network with peers.
10.1. Industry Expert Recommendations
Industry experts often share recommendations that can enhance resource planning and budgeting processes. These insights can help organizations optimize their operations and achieve better outcomes.
- Emphasize strategic alignment:
- Ensure that resource planning aligns with overall business objectives.
- Regularly review and adjust plans to reflect changes in strategy.
- Foster a culture of accountability:
- Encourage team members to take ownership of their resource usage and budget adherence.
- Implement performance metrics to track progress and hold individuals accountable.
- Invest in training and development:
- Provide ongoing training for staff to improve their resource management skills.
- Encourage cross-functional collaboration to enhance resource sharing and efficiency, possibly using a budget for an event template.
- Leverage data-driven decision-making:
- Use data analytics to inform resource allocation and budgeting decisions.
- Regularly analyze performance metrics to identify areas for improvement.
- Stay adaptable:
- Be prepared to pivot and adjust plans based on market changes or project developments.
- Foster a flexible mindset within the team to encourage innovative solutions.
By focusing on these key areas, organizations can enhance their resource planning and budgeting processes, leading to improved project outcomes and overall business success. Rapid Innovation is committed to helping clients achieve these goals through our tailored AI and Blockchain solutions, ensuring greater ROI and operational efficiency.
10.2. Frequently Asked Questions
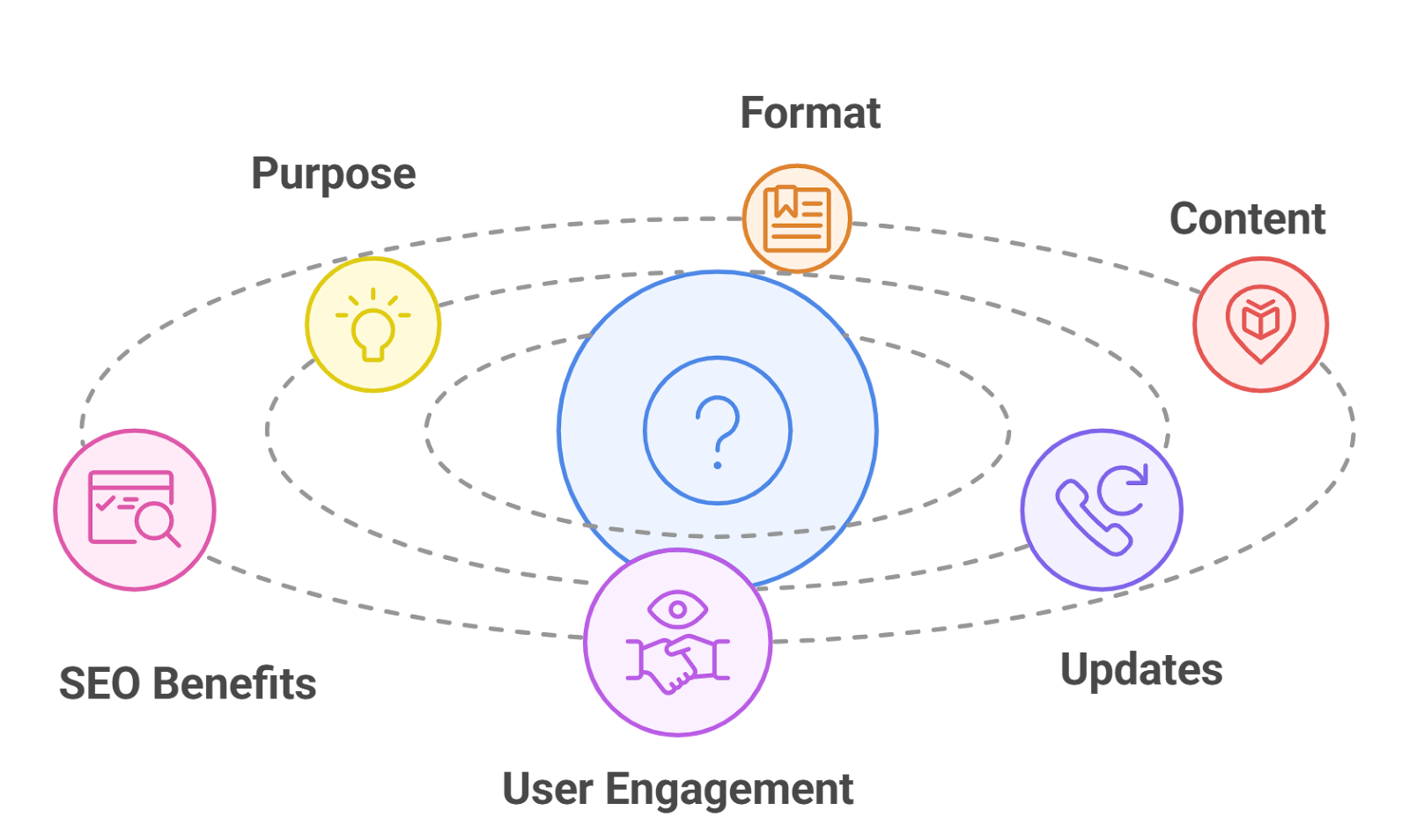
Frequently Asked Questions (faqs for user experience) serve as a valuable resource for individuals seeking quick answers to common queries. They can enhance user experience by providing immediate information and reducing the need for direct customer support. Here are some key aspects of FAQs:
- Purpose: FAQs aim to address common concerns, clarify doubts, and provide essential information about a product, service, or topic.
- Format: Typically presented in a question-and-answer format, FAQs are easy to navigate and understand.
- Content: Effective FAQs cover a range of topics, including product features and specifications, pricing and payment options, shipping and delivery information, return and refund policies, and technical support and troubleshooting.
- SEO Benefits: Well-structured FAQs can improve search engine optimization (SEO) by incorporating relevant keywords and phrases that potential customers might use in their searches.
- User Engagement: By addressing common questions, FAQs can engage users and encourage them to explore further, potentially leading to conversions.
- Updates: Regularly updating FAQs ensures that the information remains relevant and accurate, reflecting any changes in policies or offerings.
10.3. Additional Resources and Tools
Additional resources and tools can significantly enhance the user experience and provide further assistance beyond the basic information. These resources can help users make informed decisions and improve their overall understanding of a subject. Here are some examples:
- Guides and Tutorials: Comprehensive guides and step-by-step tutorials can help users navigate complex topics or products, particularly in AI and Blockchain, where understanding the technology is crucial.
- Webinars and Workshops: Live or recorded sessions can provide in-depth knowledge about AI and Blockchain applications, allowing users to ask questions in real-time and gain insights from industry experts.
- Community Forums: Online forums or discussion boards enable users to connect with others, share experiences, and seek advice, fostering a collaborative environment for learning and problem-solving.
- Blogs and Articles: Regularly updated blogs can offer insights, tips, and industry news related to AI and Blockchain, keeping users informed and engaged with the latest trends and innovations.
- Tools and Calculators: Interactive tools, such as ROI calculators or assessment quizzes, can help users evaluate their needs or make decisions based on specific criteria relevant to their business goals.
- Customer Support: Access to customer support channels, such as live chat, email, or phone support, ensures that users can get personalized assistance when needed, particularly for complex AI and Blockchain projects.
- Social Media: Engaging with users on social media platforms can provide additional support and foster a sense of community, allowing for the sharing of knowledge and experiences in the rapidly evolving fields of AI and Blockchain.
By providing a comprehensive set of FAQs and additional resources, businesses can enhance user satisfaction, build trust, and ultimately drive conversions. For those interested in advanced solutions, consider exploring our continuous AI education resources and adaptive AI development services.