Table Of Contents
Category
CRM
FinTech
Healthcare & Medicine
Supply Chain & Logistics
Manufacturing
Artificial Intelligence
Blockchain
Security
1. What is Multi-Agent AI? A Strategic Business Overview
Multi-Agent AI refers to a system where multiple intelligent agents interact with each other to achieve specific goals. These agents can be software programs, robots, or any autonomous entities capable of perceiving their environment and making decisions. The strategic business overview of Multi-Agent AI highlights its potential to enhance efficiency, improve decision-making, and foster innovation across various industries.
- Multi-Agent AI solutions can solve complex problems that are difficult for a single agent to tackle.
- They are designed to work collaboratively, sharing information and resources.
- Industries such as logistics, finance, healthcare, and smart cities are increasingly adopting Multi-Agent AI for optimization and automation.
The rise of Multi-Agent AI is driven by advancements in machine learning, natural language processing, and distributed computing. Businesses are leveraging these technologies to create more adaptive and resilient systems that can respond to dynamic environments.
At Rapid Innovation, we specialize in developing tailored Multi-Agent AI solutions that help our clients achieve greater ROI by streamlining operations, enhancing decision-making processes, and fostering innovation. For instance, in the logistics sector, our Multi-Agent AI solutions can optimize supply chain management by enabling real-time communication and coordination among various agents, leading to reduced costs and improved delivery times.
1.1. How Do Multi-Agent AI Systems Work?
Multi-Agent AI systems operate through a combination of communication, coordination, and negotiation among agents. Each agent has its own set of goals and capabilities, allowing them to work independently while contributing to a collective objective.
- Agents perceive their environment through sensors and gather data.
- They communicate with each other using predefined protocols to share information.
- Coordination mechanisms help agents align their actions to avoid conflicts and optimize outcomes.
- Agents may negotiate to resolve differences and reach agreements on resource allocation or task execution.
The architecture of Multi-Agent AI systems can vary, but they typically include:
- Agent Communication Language (ACL): A standardized way for agents to communicate.
- Environment Model: A representation of the external environment that agents interact with.
- Decision-Making Algorithms: Techniques that enable agents to make informed choices based on their goals and the information they receive.
These systems can adapt to changes in the environment, making them suitable for dynamic and complex scenarios. Rapid Innovation's expertise in AI development ensures that our clients can harness the full potential of Multi-Agent AI solutions to drive efficiency and innovation.
1.2. What's the Difference Between Single-Agent and Multi-Agent Systems?
The primary distinction between single-agent and multi-agent systems lies in the number of autonomous entities involved and their interaction dynamics.
- Single-Agent Systems:
- Operate with one agent making decisions based on its own perceptions and goals.
- Simpler in design and implementation, often used for straightforward tasks.
- Limited in handling complex problems that require collaboration or competition.
- Multi-Agent Systems:
- Comprise multiple agents that can work together or compete to achieve their objectives.
- More complex, allowing for richer interactions and problem-solving capabilities.
- Suitable for scenarios where tasks can be distributed among agents, leading to improved efficiency and effectiveness.
Key differences include:
- Collaboration: Multi-agent systems can leverage the strengths of multiple agents, while single-agent systems rely solely on one entity.
- Scalability: Multi-agent systems can scale more effectively to handle larger and more complex tasks.
- Robustness: The failure of one agent in a multi-agent system does not necessarily compromise the entire system, unlike in single-agent systems.
Understanding these differences is crucial for businesses looking to implement AI solutions tailored to their specific needs and challenges. At Rapid Innovation, we guide our clients in selecting the right approach—whether single-agent or multi-agent systems—to maximize their operational efficiency and achieve their business objectives. For more information on the differences between these systems, you can read our detailed article on Multi-Agent Systems vs. Single Agents.
Refer to the image for a visual representation of Multi-Agent AI systems and their components:
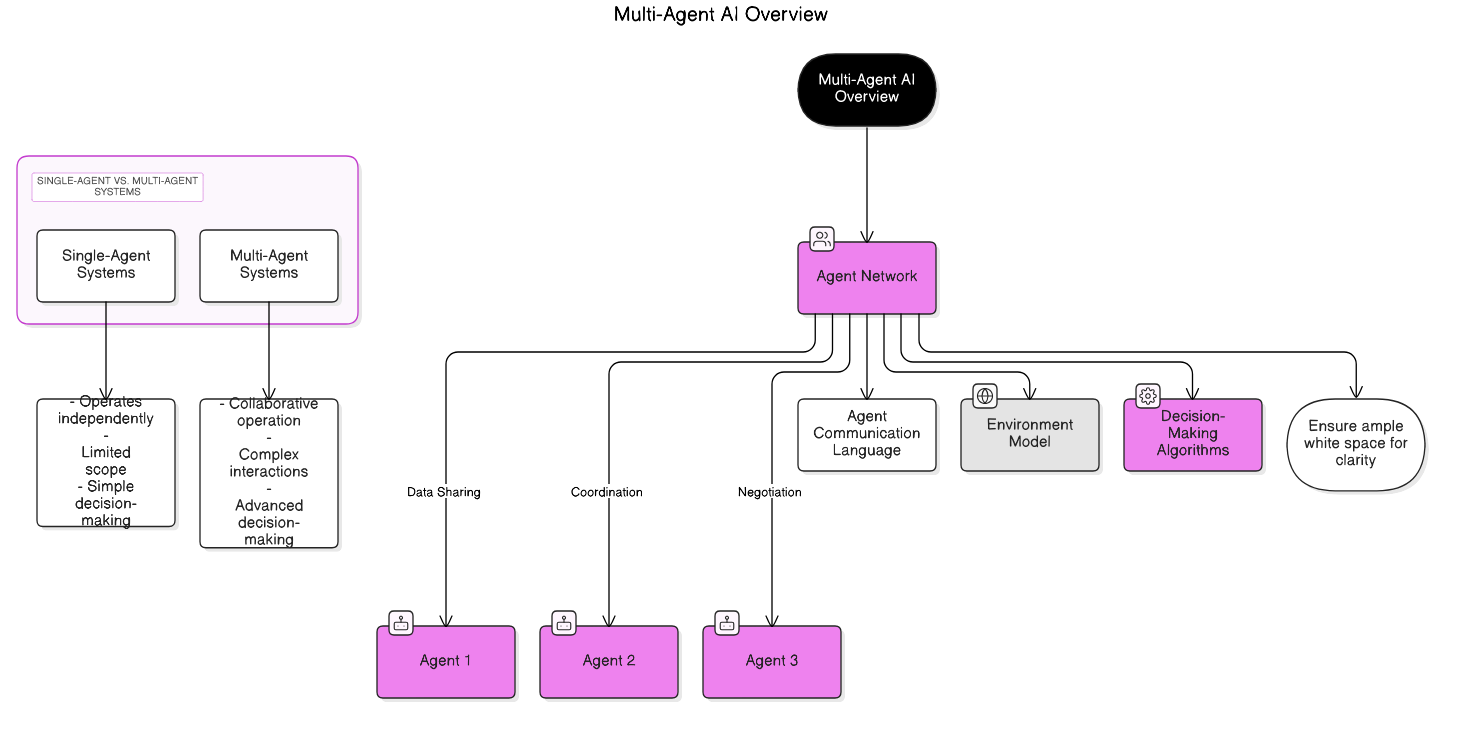
1.3. Where Are Multi-Agent Systems Being Used Today?
Multi-agent systems (MAS) are increasingly being integrated into various sectors due to their ability to solve complex problems through collaboration among multiple agents. These systems are designed to work autonomously and interact with one another to achieve specific goals. Here are some key areas where multi-agent systems are being utilized:
- Healthcare: Multi-agent systems are used for patient monitoring, resource allocation, and managing healthcare workflows. They can facilitate communication between medical devices and healthcare professionals, improving patient outcomes.
- Transportation: In traffic management, MAS can optimize traffic flow by coordinating traffic signals and managing public transportation systems. They help reduce congestion and improve overall efficiency.
- Robotics: Multi-agent systems are essential in robotics, particularly in swarm robotics, where multiple robots work together to complete tasks such as search and rescue operations or environmental monitoring. This includes applications in multi agent robotic systems and multi agent robot technologies.
- Smart Grids: In energy management, MAS can optimize the distribution of electricity by coordinating between various energy sources and consumers, enhancing the efficiency of smart grids.
- Finance: In the financial sector, multi-agent systems are used for algorithmic trading, fraud detection, and risk management. They can analyze vast amounts of data and make real-time decisions.
- Gaming: In video games, MAS are employed to create intelligent non-player characters (NPCs) that can adapt to player behavior, enhancing the gaming experience.
- Supply Chain Management: MAS can optimize logistics and inventory management by enabling real-time communication between suppliers, manufacturers, and retailers. This is particularly relevant in multi agent systems examples within supply chain contexts.
2. What Are the Business Benefits of Multi-Agent AI?
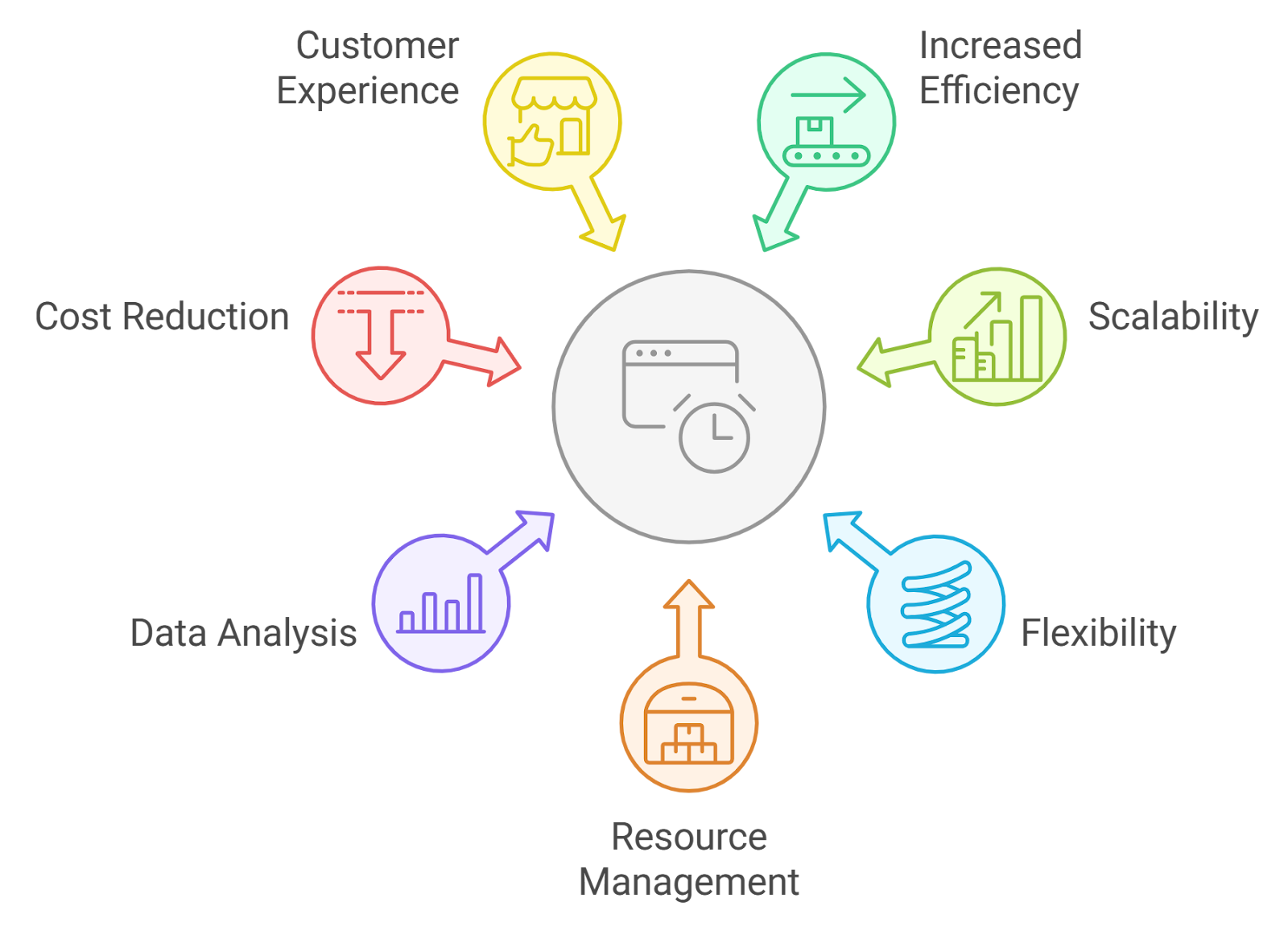
Multi-agent AI offers numerous advantages for businesses looking to enhance their operations and decision-making processes. The following benefits highlight why organizations are increasingly adopting multi-agent systems:
- Increased Efficiency: By automating tasks and enabling agents to work collaboratively, businesses can streamline operations and reduce the time required to complete complex processes.
- Scalability: Multi-agent systems can easily scale to accommodate growing business needs. As demand increases, additional agents can be deployed without significant changes to the existing infrastructure.
- Enhanced Flexibility: MAS can adapt to changing environments and requirements, allowing businesses to respond quickly to market fluctuations or operational challenges.
- Improved Resource Management: Multi-agent systems can optimize the allocation of resources, ensuring that assets are used effectively and reducing waste.
- Better Data Analysis: With the ability to process and analyze large datasets, multi-agent AI can provide valuable insights that inform strategic decision-making.
- Cost Reduction: By automating processes and improving efficiency, businesses can significantly reduce operational costs, leading to higher profit margins.
- Enhanced Customer Experience: Multi-agent systems can personalize customer interactions and improve service delivery, leading to increased customer satisfaction and loyalty.
2.1. How Does Multi-Agent AI Improve Decision-Making?
Multi-agent AI enhances decision-making in several ways, making it a valuable tool for organizations. Here are some key aspects of how it contributes to better decision-making:
- Distributed Intelligence: Multi-agent systems leverage the collective intelligence of multiple agents, allowing for a more comprehensive analysis of situations. This distributed approach leads to more informed decisions.
- Real-Time Data Processing: MAS can process data in real-time, enabling organizations to make timely decisions based on the most current information available.
- Collaboration and Negotiation: Agents can communicate and negotiate with one another, simulating human-like interactions. This collaboration can lead to more effective solutions and consensus-building.
- Scenario Simulation: Multi-agent systems can simulate various scenarios and outcomes, helping decision-makers understand potential risks and benefits before implementing changes.
- Adaptive Learning: Many multi-agent systems incorporate machine learning algorithms, allowing them to learn from past experiences and improve their decision-making capabilities over time.
- Handling Complexity: MAS can manage complex systems with numerous variables, making them ideal for environments where traditional decision-making processes may falter.
- Enhanced Predictive Analytics: By analyzing patterns and trends, multi-agent AI can provide predictive insights that help organizations anticipate future challenges and opportunities.
In conclusion, multi-agent systems are transforming various industries by improving efficiency, decision-making, and overall business performance. Their ability to work collaboratively and adapt to changing environments makes them a powerful tool for organizations looking to thrive in today's competitive landscape. At Rapid Innovation, we leverage our expertise in AI and blockchain to implement multi-agent systems that align with your business goals, ensuring you achieve greater ROI and operational excellence. This includes the application of multi agent systems in artificial intelligence and the exploration of types of multi agent systems to meet diverse needs. For more information on the impact of multi-agent reinforcement learning.
Refer to the image for a visual representation of the various sectors where multi-agent systems are being utilized.
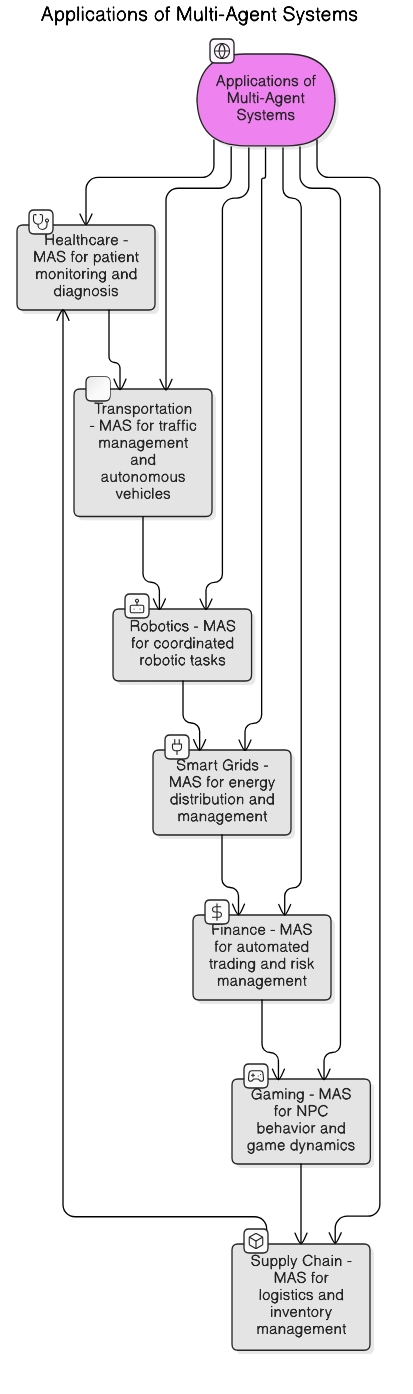
2.2. Why is Multi-Agent AI More Scalable Than Traditional Systems?
Multi-agent AI systems are designed to handle complex tasks by distributing responsibilities among multiple agents. This architecture offers several advantages over traditional systems, particularly in terms of scalability.
- Decentralization: Multi-agent systems operate on a decentralized model, allowing agents to work independently. This reduces bottlenecks that often occur in centralized systems, enabling easier scaling as more agents can be added without overloading a single point of control.
- Parallel Processing: Each agent can process information and make decisions simultaneously. This parallelism significantly enhances the system's ability to handle large datasets and complex computations, making it more efficient than traditional systems that often rely on sequential processing.
- Dynamic Adaptability: Multi-agent systems can adapt to changing environments and requirements. As new agents are introduced, they can learn from existing agents and integrate seamlessly, allowing the system to grow organically without extensive reconfiguration.
- Resource Optimization: By distributing tasks among agents, multi-agent systems can optimize resource usage. Agents can be assigned specific roles based on their strengths, leading to more efficient operations and reduced waste.
- Robustness: The failure of one agent does not compromise the entire system. This redundancy enhances reliability and allows for continuous operation, which is crucial for scaling operations in dynamic business environments. For more insights on building effective multi-agent environments, check out this framework and tools.
2.3. How Do Multi-Agent Systems Reduce Business Risk?
Implementing multi-agent systems can significantly mitigate various business risks. These systems provide a framework that enhances decision-making, operational efficiency, and adaptability.
- Improved Decision-Making: Multi-agent systems can analyze vast amounts of data from different sources, leading to more informed decisions. This capability reduces the risk of errors that can arise from relying on a single data source or decision-maker.
- Enhanced Flexibility: Businesses often face unpredictable changes in market conditions. Multi-agent systems can quickly adapt to these changes by reallocating resources or adjusting strategies, minimizing the risk of operational disruptions.
- Fault Tolerance: The decentralized nature of multi-agent systems means that if one agent fails, others can continue to operate. This fault tolerance reduces the risk of complete system failure, ensuring business continuity.
- Proactive Risk Management: Multi-agent systems can monitor and analyze potential risks in real-time. By identifying threats early, businesses can take proactive measures to mitigate risks before they escalate.
- Cost Efficiency: By optimizing processes and resource allocation, multi-agent systems can lead to significant cost savings. This financial stability reduces the risk associated with budget overruns and resource mismanagement.
3. Multi-Agent AI Architecture and Implementation
The architecture of multi-agent AI systems is crucial for their functionality and effectiveness. Understanding this architecture is essential for successful implementation.
- Agent Design: Each agent is designed with specific capabilities and functions. This includes defining the agent's goals, behaviors, and communication protocols. Agents can be reactive, proactive, or hybrid, depending on the tasks they need to perform.
- Communication Framework: Agents must communicate effectively to coordinate their actions. This can involve direct communication, shared knowledge bases, or negotiation protocols. A robust communication framework ensures that agents can collaborate efficiently.
- Environment Representation: Multi-agent systems operate within a defined environment. This environment can be physical, virtual, or a combination of both. Proper representation of the environment is essential for agents to understand their context and make informed decisions.
- Coordination Mechanisms: To achieve common goals, agents need coordination mechanisms. This can include task allocation, scheduling, and conflict resolution strategies. Effective coordination minimizes redundancy and enhances overall system performance.
- Learning and Adaptation: Multi-agent systems often incorporate machine learning algorithms that allow agents to learn from their experiences. This capability enables agents to adapt to new situations and improve their performance over time.
- Implementation Challenges: While multi-agent systems offer numerous benefits, implementing them can be complex. Challenges include ensuring interoperability between agents, managing communication overhead, and addressing security concerns. Careful planning and testing are essential to overcome these challenges.
- Deployment Strategies: Successful deployment of multi-agent systems requires a clear strategy. This includes pilot testing, gradual scaling, and continuous monitoring to ensure that the system meets business objectives and adapts to changing needs.
At Rapid Innovation, we leverage our expertise in AI and blockchain technologies to help businesses implement multi-agent systems in artificial intelligence effectively. By doing so, we enable our clients to achieve greater ROI through enhanced scalability, reduced risks, and optimized resource management. Our tailored solutions ensure that your organization can navigate the complexities of modern business environments with confidence and agility, utilizing multi-agent systems in AI to their fullest potential. If you're looking to enhance your capabilities, consider our Action Transformer Developers for specialized support.
Refer to the image for a visual representation of the scalability advantages of multi-agent AI systems over traditional systems:

3.1. What Are the Core Components of Multi-Agent Systems?
Multi-agent systems (MAS) consist of several key components that work together to achieve complex tasks. Understanding these components is essential for designing and implementing effective MAS.
- Agents: The fundamental units in a multi-agent system. Each agent is an autonomous entity capable of perceiving its environment, making decisions, and taking actions. Agents can be software-based or physical robots, such as multi agent robots.
- Environment: The context in which agents operate. The environment can be static or dynamic, and it can be fully observable or partially observable. The environment influences agent behavior and decision-making.
- Communication: Agents need to share information to coordinate their actions. Communication can occur through various protocols and languages, enabling agents to exchange messages, share knowledge, and negotiate. This is particularly important in multiagent systems in artificial intelligence.
- Coordination: This involves managing the interactions between agents to achieve common goals. Coordination mechanisms can include task allocation, scheduling, and conflict resolution, which are essential in multi agent systems examples.
- Learning: Many agents incorporate learning algorithms to adapt to changing environments or improve their performance over time. This can involve reinforcement learning, supervised learning, or other machine learning techniques, which are often utilized in multi agent optimization.
- Goals and Objectives: Each agent typically has specific goals or objectives it aims to achieve. These goals guide the agent's actions and decision-making processes, which can be seen in multi agent systems ai.
- Decision-Making: Agents must make decisions based on their perceptions and the information they receive from other agents. Decision-making can be based on predefined rules, algorithms, or learning processes, as seen in types of multi agent systems. For more insights on the key components of modern AI agent architecture.
3.2. How Do AI Agents Communicate With Each Other?
Effective communication among AI agents is crucial for the success of multi-agent systems. The methods and protocols used for communication can significantly impact the system's overall performance.
- Message Passing: Agents communicate by sending and receiving messages. This can include simple text messages, structured data, or complex objects. Message passing allows agents to share information and coordinate actions, which is vital in multi agent systems in ai.
- Communication Protocols: Various protocols govern how agents communicate. Common protocols include FIPA (Foundation for Intelligent Physical Agents) and KQML (Knowledge Query and Manipulation Language). These protocols define the syntax and semantics of messages exchanged between agents.
- Shared Language: Agents often use a common language or ontology to ensure that they understand each other. This shared language helps in reducing ambiguity and improving the clarity of communication.
- Negotiation: In scenarios where agents have conflicting goals, negotiation becomes essential. Agents can negotiate terms, share resources, or reach agreements through dialogue, enhancing collaboration, which is a key aspect of autonomous agents and multi agent systems.
- Broadcasting: Agents can broadcast messages to all other agents in the system. This is useful for disseminating information quickly, especially in dynamic environments where rapid updates are necessary.
- Direct Communication: Agents can also communicate directly with specific agents. This targeted communication can be more efficient for resolving specific issues or coordinating particular tasks.
3.3. How to Integrate Multi-Agent AI With Existing Systems?
Integrating multi-agent AI into existing systems can enhance functionality and improve efficiency. However, this process requires careful planning and execution.
- Assessment of Existing Systems: Before integration, it’s crucial to evaluate the current system architecture, workflows, and technologies. Understanding the existing infrastructure helps identify compatibility issues and integration points.
- Define Objectives: Clearly outline the goals of integrating multi-agent systems. This could include improving automation, enhancing decision-making, or increasing system responsiveness.
- Choose the Right Framework: Select a suitable multi-agent framework or platform that aligns with the existing system. Popular frameworks include JADE (Java Agent Development Framework) and NetLogo, which provide tools for building and managing agents, including multi agent platforms.
- Interfacing: Develop interfaces that allow agents to interact with existing components. This may involve creating APIs or middleware that facilitate communication between agents and legacy systems.
- Data Sharing: Ensure that data can be shared seamlessly between agents and existing systems. This may require data transformation or mapping to ensure compatibility.
- Testing and Validation: Conduct thorough testing to ensure that the integrated system functions as intended. This includes validating agent behavior, communication, and overall system performance.
- Monitoring and Maintenance: After integration, continuous monitoring is essential to ensure that the multi-agent system operates effectively. Regular maintenance and updates may be necessary to adapt to changing requirements or environments.
At Rapid Innovation, we leverage our expertise in AI and blockchain technologies to help clients implement multi-agent systems that drive efficiency and enhance decision-making. By integrating these systems into your existing infrastructure, we can help you achieve greater ROI through improved automation and streamlined processes. Our tailored solutions ensure that your business can adapt to the evolving technological landscape while maximizing the value of your investments, including applications in multi agent system application and multi agency system.
Refer to the image for a visual representation of the core components of multi-agent systems.

4. Industry-Specific Applications and Success Stories
In today's competitive landscape, businesses are increasingly leveraging technology to enhance their operations. Industry-specific applications have emerged as vital tools for optimizing processes, improving customer interactions, and driving overall success. Below, we explore two significant areas where these applications have made a substantial impact: Enterprise Resource Planning (ERP) and Supply Chain Optimization, as well as Customer Service and Sales Process.
Enterprise Resource Planning (ERP) and Supply Chain Optimization
- Streamlined Operations: ERP systems integrate various business processes, allowing for seamless data flow and improved efficiency. Rapid Innovation specializes in developing tailored enterprise resource planning systems that align with your unique business needs, ensuring that your operations run smoothly and effectively.
- Real-Time Data Access: Businesses can access real-time data, enabling informed decision-making and quicker responses to market changes. Our AI-driven analytics tools provide actionable insights, empowering your team to make data-backed decisions swiftly.
- Cost Reduction: By optimizing resource allocation and reducing waste, companies can significantly lower operational costs. With our blockchain solutions, we enhance transparency and traceability in supply chains, leading to reduced fraud and operational inefficiencies.
- Enhanced Collaboration: ERP systems foster better communication and collaboration across departments, leading to improved project outcomes. Rapid Innovation's expertise in integrating AI and blockchain technologies ensures that your teams can collaborate seamlessly, driving innovation and productivity.
Customer Service and Sales Process
- Improved Customer Interactions: Technology enables personalized communication, enhancing customer satisfaction and loyalty. Our AI solutions can analyze customer behavior, allowing for tailored interactions that resonate with your audience.
- Data-Driven Insights: Businesses can analyze customer data to identify trends and preferences, allowing for targeted marketing strategies. Rapid Innovation leverages advanced machine learning algorithms to provide deep insights into customer preferences, helping you refine your marketing efforts.
- Automation of Sales Processes: Automating routine tasks frees up sales teams to focus on building relationships and closing deals. Our AI-driven automation tools streamline sales processes, ensuring your team can dedicate more time to strategic initiatives.
- 24/7 Support Availability: Implementing chatbots and AI-driven solutions ensures customers receive assistance at any time, improving overall service quality. Rapid Innovation's AI chatbots are designed to provide instant support, enhancing customer experience and satisfaction around the clock. You can learn more about our AI customer service agent solutions here.
By partnering with Rapid Innovation, businesses can harness the power of enterprise resource management and ERP enterprise resource planning to achieve greater ROI and drive sustainable growth.
4.3. Financial Services and Risk Management Solutions
Financial services and risk management solutions, including financial risk management and financial risk management certification, are critical components of the modern financial landscape. These solutions leverage technology to enhance decision-making, improve efficiency, and mitigate risks. Financial institutions utilize advanced data analytics to assess risk and make informed decisions. By analyzing historical data, they can identify trends and predict future market movements.
- Data Analytics: Financial institutions utilize advanced data analytics to assess risk and make informed decisions. By analyzing historical data, they can identify trends and predict future market movements, ultimately leading to improved ROI.
- Regulatory Compliance: With increasing regulations, financial services must ensure compliance. Solutions like automated reporting tools help institutions adhere to regulations efficiently, reducing the risk of penalties and associated costs.
- Fraud Detection: AI and machine learning algorithms are employed to detect fraudulent activities in real-time. These systems analyze transaction patterns and flag anomalies, helping to protect both the institution and its customers, thereby reducing financial losses.
- Credit Risk Assessment: Financial services use sophisticated models to evaluate the creditworthiness of borrowers. By analyzing various data points, these models provide a more accurate assessment of risk, leading to better lending decisions and reduced default rates.
- Portfolio Management: Risk management solutions assist in optimizing investment portfolios. They provide insights into asset allocation and risk exposure, enabling better investment strategies that enhance returns. This includes risk investment management and market risk management.
- Insurance Underwriting: In the insurance sector, AI-driven solutions streamline the underwriting process. They assess risk factors more accurately, leading to better pricing and reduced claims, which ultimately improves profitability.
- Market Volatility Management: Financial services utilize predictive analytics to manage market volatility. By forecasting potential downturns, institutions can adjust their strategies accordingly, minimizing losses and maximizing gains.
- Customer Insights: Understanding customer behavior is crucial for financial services. Advanced analytics provide insights into customer preferences, enabling personalized services and improved customer satisfaction, which can lead to increased customer loyalty and revenue. This is particularly relevant in wealth management risk management and financial services risk management. For more information on AI applications in banking, check out this article on AI in banking: from risk assessment to personalization.
5. Emerging Trends in Multi-Agent AI Development
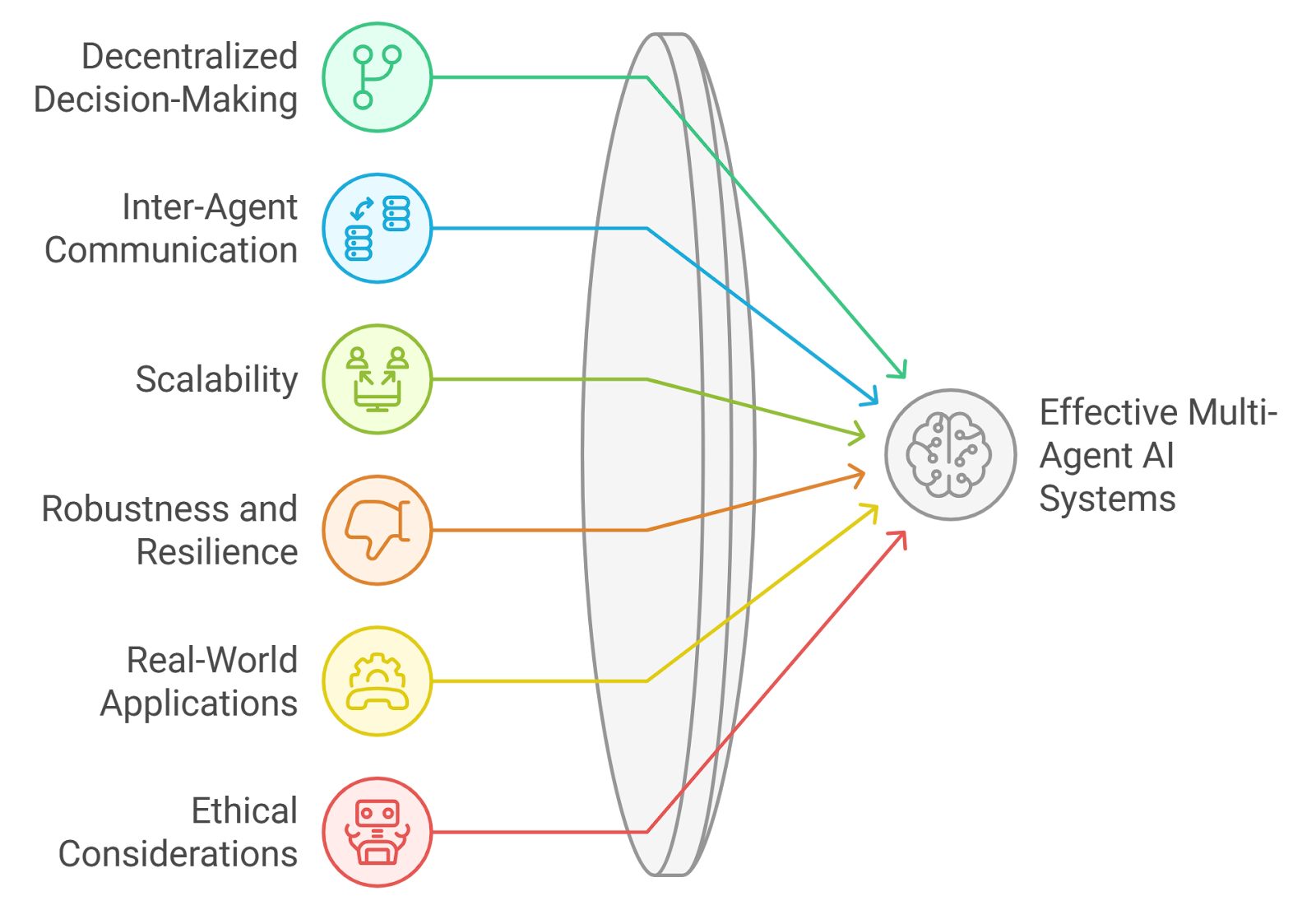
Multi-agent AI systems are becoming increasingly prevalent, driven by advancements in technology and the need for more sophisticated solutions. These systems consist of multiple intelligent agents that can collaborate and communicate to solve complex problems.
- Decentralized Decision-Making: Multi-agent systems allow for decentralized decision-making, where agents operate independently yet collaboratively. This leads to more efficient problem-solving and resource allocation.
- Inter-Agent Communication: Effective communication between agents is essential for the success of multi-agent systems. Emerging protocols and frameworks facilitate seamless interaction, enhancing overall system performance.
- Scalability: As the demand for AI solutions grows, multi-agent systems offer scalability. They can easily adapt to increased workloads by adding more agents without compromising performance.
- Robustness and Resilience: Multi-agent systems are inherently more robust. If one agent fails, others can continue functioning, ensuring system resilience and reliability.
- Real-World Applications: These systems are being applied in various fields, including logistics, healthcare, and finance. For instance, in logistics, agents can optimize supply chain operations by coordinating deliveries and inventory management.
- Ethical Considerations: As multi-agent AI systems evolve, ethical considerations become paramount. Ensuring fairness, transparency, and accountability in decision-making processes is crucial.
5.1. Advanced Collaborative Learning Mechanisms
Advanced collaborative learning mechanisms are at the forefront of multi-agent AI development. These mechanisms enable agents to learn from each other, enhancing their capabilities and improving overall system performance.
- Knowledge Sharing: Agents can share knowledge and experiences, leading to collective learning. This process accelerates the learning curve and enhances the agents' ability to adapt to new situations.
- Distributed Learning: Collaborative learning allows agents to learn in a distributed manner. Each agent can process information locally while contributing to a shared knowledge base, improving efficiency.
- Adaptive Algorithms: Advanced algorithms enable agents to adapt their learning strategies based on the performance of their peers. This adaptability fosters a more dynamic learning environment.
- Peer-to-Peer Learning: Agents can engage in peer-to-peer learning, where they teach and learn from each other. This method enhances the overall intelligence of the system.
- Real-Time Feedback: Collaborative learning mechanisms often incorporate real-time feedback, allowing agents to adjust their strategies promptly. This responsiveness is crucial in dynamic environments.
- Enhanced Problem Solving: By collaborating, agents can tackle complex problems more effectively. They can pool their resources and knowledge, leading to innovative solutions.
- Application in Various Domains: Advanced collaborative learning mechanisms are being utilized in various domains, including robotics, autonomous vehicles, and smart grids. These applications demonstrate the versatility and effectiveness of multi-agent systems.
- Future Prospects: As technology continues to evolve, the potential for advanced collaborative learning mechanisms in multi-agent AI systems is vast. Innovations in machine learning and communication protocols will further enhance their capabilities.
At Rapid Innovation, we harness these advanced technologies to help our clients in the financial sector achieve greater ROI through efficient risk management and decision-making processes, including financial planning risk assessment and financial risk advisory. Our expertise in AI and blockchain ensures that we deliver tailored solutions that meet the unique needs of each client, driving their success in a competitive landscape.
5.2. Natural Language Processing in Agent Communication
Natural Language Processing (NLP) plays a crucial role in enhancing communication between agents in multi-agent systems. By enabling agents to understand, interpret, and generate human language, NLP facilitates more effective interactions and collaboration among agents in multi-agent AI.
- Improved Understanding: NLP allows agents to comprehend user queries and commands in natural language, making it easier for users to interact with them.
- Context Awareness: Through techniques like sentiment analysis and entity recognition, agents can grasp the context of conversations, leading to more relevant responses.
- Dialogue Management: NLP techniques help manage conversations, ensuring that agents can maintain context over multiple exchanges and provide coherent responses.
- Language Generation: Agents can generate human-like responses, making interactions feel more natural and engaging for users.
- Cross-Language Communication: NLP enables agents to communicate across different languages, broadening their usability in diverse environments.
The integration of NLP in agent communication not only enhances user experience but also improves the efficiency of multi-agent systems in artificial intelligence by allowing agents to collaborate more effectively. At Rapid Innovation, we leverage NLP to develop intelligent agents that can significantly enhance customer engagement and streamline operations, ultimately driving greater ROI for our clients.
5.3. Edge Computing and Distributed AI Systems
Edge computing and distributed AI systems are transforming how data is processed and analyzed, particularly in environments where real-time decision-making is critical. By bringing computation closer to the data source, these technologies reduce latency and improve performance.
- Reduced Latency: Edge computing minimizes the time it takes for data to travel to a centralized server, allowing for quicker responses and actions.
- Bandwidth Efficiency: By processing data locally, edge computing reduces the amount of data that needs to be transmitted to the cloud, conserving bandwidth and lowering costs.
- Enhanced Privacy: Sensitive data can be processed on-site, reducing the risk of exposure during transmission and enhancing privacy.
- Scalability: Distributed AI systems can easily scale by adding more edge devices, allowing for flexible and efficient resource management.
- Real-Time Analytics: With edge computing, data can be analyzed in real-time, enabling immediate insights and actions, which is crucial for applications like autonomous vehicles and smart cities.
The combination of edge computing and distributed AI systems is paving the way for more responsive and efficient applications across various industries. Rapid Innovation harnesses these technologies to create solutions that not only meet the demands of modern businesses but also enhance operational efficiency and profitability.
6. How to Implement Multi-Agent AI Successfully
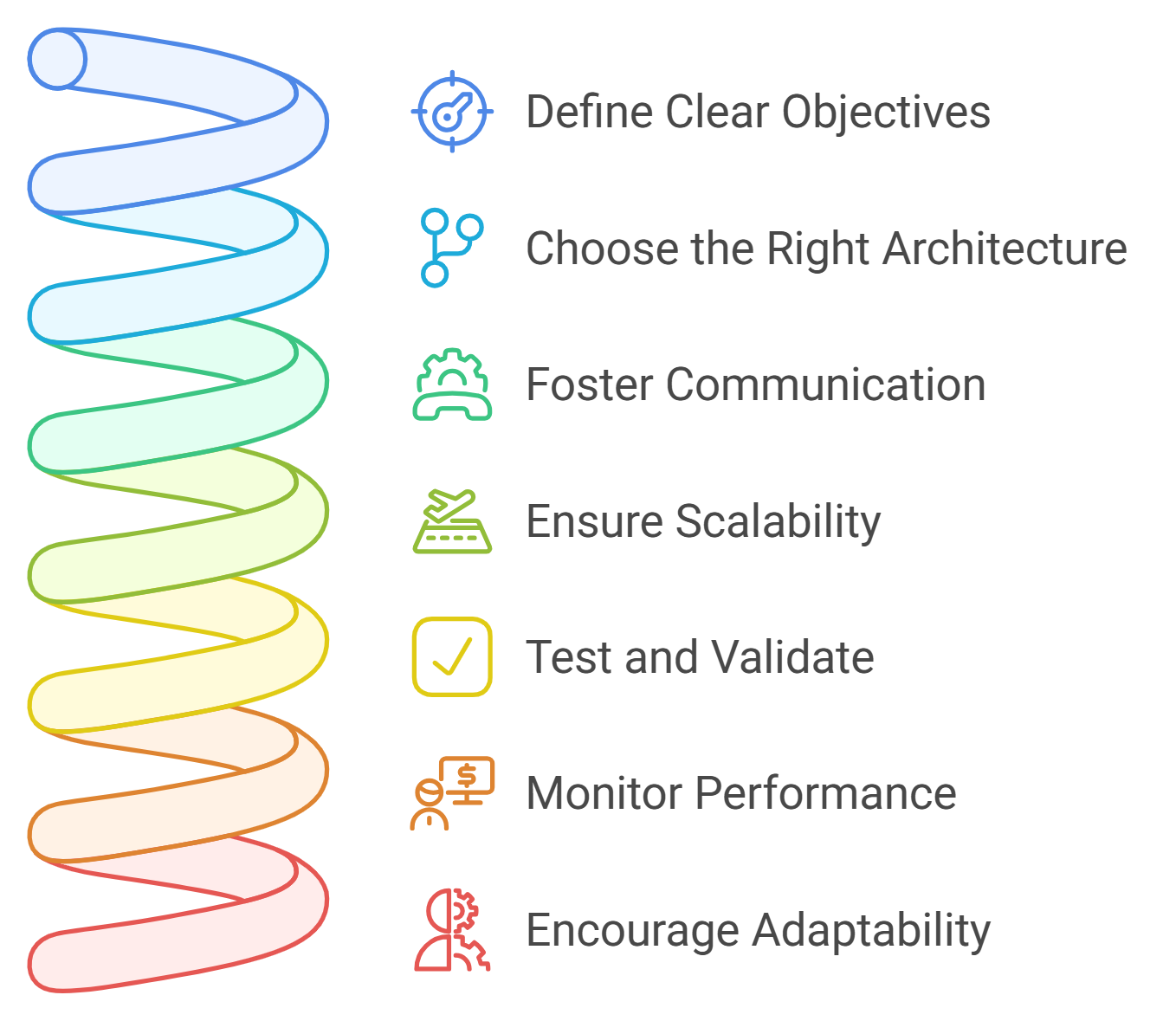
Implementing multi-agent AI systems requires careful planning and execution to ensure that the agents work harmoniously and achieve the desired outcomes. Here are key steps to consider:
- Define Clear Objectives: Establish specific goals for the multi-agent system, ensuring that each agent has a defined role and purpose.
- Choose the Right Architecture: Select an appropriate architecture that supports communication and collaboration among agents, such as centralized, decentralized, or hybrid models.
- Foster Communication: Implement robust communication protocols that allow agents to share information and coordinate their actions effectively.
- Ensure Scalability: Design the system to accommodate future growth, allowing for the addition of new agents without significant reconfiguration.
- Test and Validate: Conduct thorough testing to identify potential issues and validate that the agents are functioning as intended in various scenarios.
- Monitor Performance: Continuously monitor the performance of the multi-agent system, using metrics to assess efficiency, effectiveness, and areas for improvement.
- Encourage Adaptability: Equip agents with the ability to learn and adapt to changing environments and user needs, enhancing their long-term effectiveness.
By following these steps, organizations can successfully implement multi-agent systems in AI that are efficient, scalable, and capable of meeting complex challenges. At Rapid Innovation, we guide our clients through each phase of implementation, ensuring that their multi-agent systems are optimized for success and aligned with their business objectives.
6.1. Assessment and Planning Guidelines
Assessment and planning are critical steps in any project or initiative. They ensure that objectives are clearly defined and that resources are allocated efficiently. Here are some key guidelines to follow:
- Define clear objectives: Establish what you want to achieve, including specific goals, timelines, and deliverables. For instance, if you're looking to implement an AI-driven solution, clarify the expected outcomes such as increased efficiency or reduced costs.
- Conduct a needs assessment: Identify the needs of stakeholders and the resources available to understand gaps and opportunities. This is particularly important in AI and Blockchain projects, where understanding user requirements can lead to more tailored solutions.
- Gather data: Use qualitative and quantitative methods to collect relevant data. Surveys, interviews, and existing reports can provide valuable insights. For example, analyzing user behavior data can inform the development of a more effective AI model.
- Analyze the current state: Evaluate existing processes, systems, and resources to identify strengths and weaknesses. This analysis can reveal how Blockchain technology can enhance transparency and security in your operations.
- Develop a strategic plan: Create a roadmap that outlines the steps needed to achieve your objectives, including timelines, responsibilities, and resource allocation. This plan should incorporate milestones for AI model training or Blockchain integration phases. Consider using a risk assessment plan example to guide this process.
- Engage stakeholders: Involve key stakeholders in the planning process to ensure buy-in and gather diverse perspectives. Engaging with technical teams can provide insights into the feasibility of AI and Blockchain implementations.
- Review and adjust: Regularly revisit the plan to make necessary adjustments based on feedback and changing circumstances. This iterative approach is essential in the fast-evolving fields of AI and Blockchain.
6.2. Risk Management and Security Considerations
Risk management is essential for safeguarding assets and ensuring project success. It involves identifying, assessing, and mitigating risks. Here are some considerations:
- Identify potential risks: Conduct a thorough risk assessment to identify possible threats, including financial, operational, and reputational risks. In Blockchain projects, consider risks related to smart contract vulnerabilities. A risk management table example can be useful in this phase.
- Assess risk impact: Evaluate the potential impact of each risk on the project to prioritize which risks need immediate attention. For AI projects, this could involve assessing the implications of biased data on model outcomes.
- Develop a risk management plan: Create a plan that outlines strategies for mitigating identified risks, which may include contingency plans and risk transfer options. This is crucial for ensuring the integrity of Blockchain transactions.
- Implement security measures: Ensure that appropriate security protocols are in place to protect sensitive information and assets, including cybersecurity measures and physical security. For AI, this may involve securing data used for training models.
- Monitor risks continuously: Regularly review and update the risk management plan to address new risks as they arise. This proactive approach helps minimize potential disruptions, especially in dynamic environments like AI and Blockchain.
- Train staff: Provide training on risk awareness and security protocols to ensure that all team members understand their roles in managing risks. This is particularly important for teams working with sensitive AI algorithms or Blockchain networks.
6.3. Performance Monitoring and Optimization Techniques
Performance monitoring is crucial for evaluating the effectiveness of a project and making necessary adjustments. Here are some techniques to consider:
- Establish key performance indicators (KPIs): Define measurable indicators that align with your objectives to help track progress and identify areas for improvement. For AI projects, KPIs might include model accuracy or processing speed.
- Use data analytics: Leverage data analytics tools to gather insights on performance metrics, which can help identify trends and make informed decisions. In Blockchain, analytics can reveal transaction patterns and network performance.
- Conduct regular reviews: Schedule periodic performance reviews to assess progress against KPIs, allowing for timely adjustments to strategies and tactics. This is essential for ensuring that AI models remain effective over time. Examples of monitoring and evaluation plan can guide this process.
- Gather feedback: Solicit feedback from stakeholders and team members to gain insights into performance and areas needing improvement. This feedback loop is vital for refining AI algorithms and Blockchain applications.
- Implement continuous improvement processes: Adopt methodologies such as Lean or Six Sigma to streamline processes and enhance efficiency. These methodologies can be applied to optimize AI workflows or Blockchain transaction processes.
- Utilize technology: Invest in performance management software to automate tracking and reporting, saving time and improving accuracy. This technology can provide real-time insights into AI performance and Blockchain network health.
- Benchmark against industry standards: Compare your performance metrics with industry benchmarks to identify gaps and opportunities for optimization. This benchmarking can help ensure that your AI and Blockchain initiatives are competitive and effective. Consider creating a project assessment plan to facilitate this comparison.
7. ROI and Performance Metrics
Understanding Return on Investment (ROI) and performance metrics is crucial for businesses aiming to evaluate the effectiveness of their strategies and initiatives. These metrics provide insights into financial performance, operational efficiency, and overall business health.
7.1. Measuring Business Impact and Value Creation
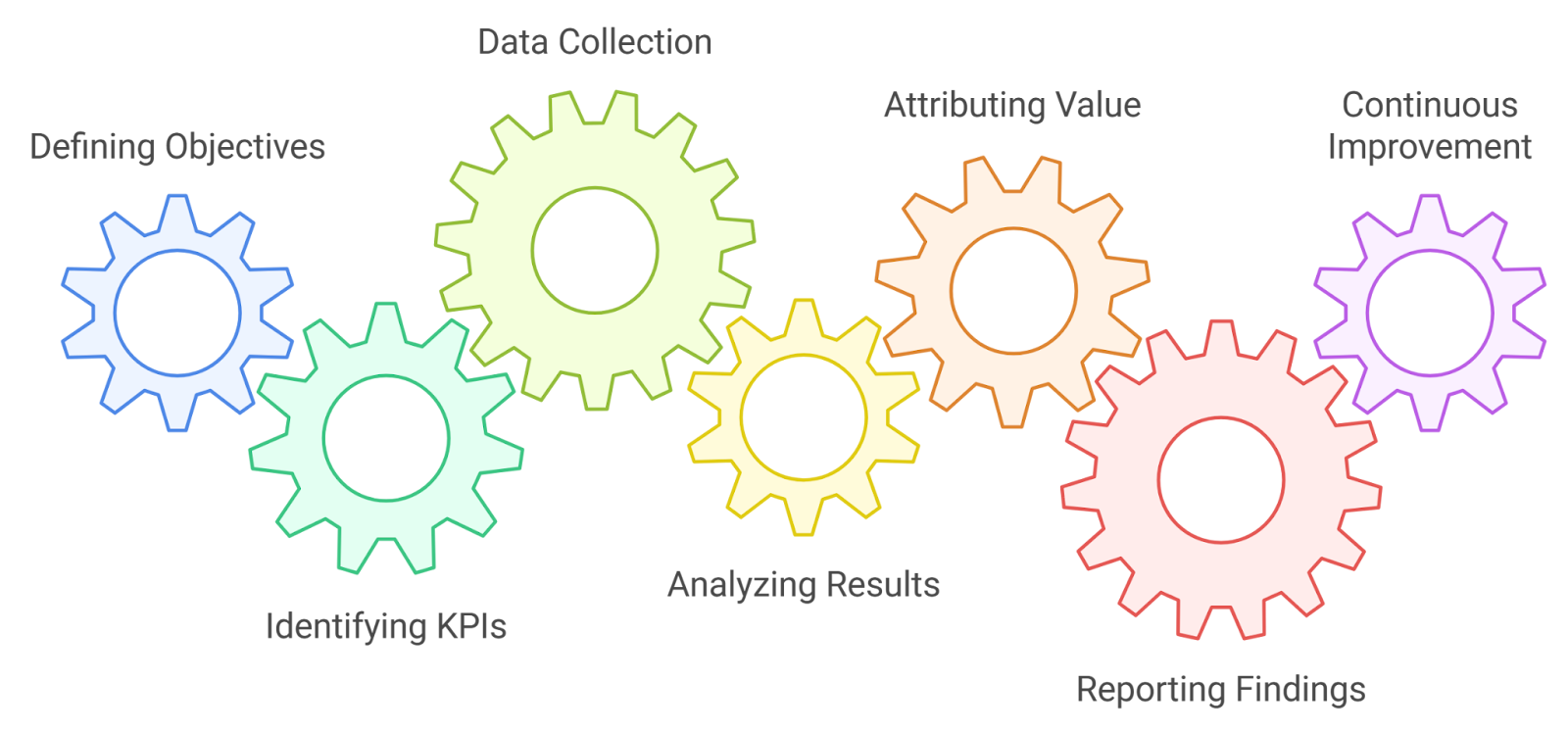
Measuring business impact and value creation involves assessing how various initiatives contribute to the organization's goals. This process can be broken down into several key components:
- Defining Objectives: Clearly outline what success looks like for your business. This could include revenue growth, customer satisfaction, or market share expansion.
- Identifying Key Performance Indicators (KPIs): Select relevant KPIs that align with your objectives. Common KPIs include revenue growth rate, customer acquisition cost, and net promoter score (NPS). This may also include business performance metrics, financial kpis, and key performance indicators in ecommerce.
- Data Collection: Gather data from various sources, such as sales reports, customer feedback, and market analysis. This data will help in evaluating performance against the defined KPIs, including business metrics and company kpis.
- Analyzing Results: Use statistical methods and analytical tools to interpret the data. Look for trends, patterns, and correlations that indicate the impact of specific initiatives, such as retail store kpis and kpi for construction company.
- Attributing Value: Determine how much value each initiative has created. This can be done through incremental revenue analysis, cost savings evaluation, and customer lifetime value (CLV) assessment, which may involve human resources manager kpis and accounting kpis.
- Reporting Findings: Present the results in a clear and concise manner. Use visual aids like charts and graphs to illustrate the impact effectively, focusing on key business metrics and kpi of business.
- Continuous Improvement: Use the insights gained to refine strategies and improve future initiatives. Regularly revisit your objectives and KPIs to ensure they remain relevant, including key performance indicators for accounts payable and kpi of company.
7.2. Cost-Benefit Analysis Framework
A Cost-Benefit Analysis (CBA) framework is essential for evaluating the financial viability of projects and initiatives. This systematic approach helps businesses make informed decisions by comparing the costs and benefits associated with a particular action.
- Identifying Costs: List all potential costs involved in the initiative, including direct costs (e.g., materials, labor), indirect costs (e.g., overhead, administrative expenses), and opportunity costs (e.g., potential revenue lost from alternative investments).
- Estimating Benefits: Quantify the expected benefits, which may include increased revenue, cost savings, and enhanced customer satisfaction leading to repeat business. This could also involve restaurant kpis and kpi for a restaurant.
- Time Frame: Determine the time frame for the analysis. A longer time frame may provide a more comprehensive view of benefits but can also introduce uncertainty.
- Discount Rate: Apply a discount rate to future cash flows to account for the time value of money. This helps in comparing costs and benefits that occur at different times.
- Net Present Value (NPV): Calculate the NPV by subtracting total costs from total benefits, adjusted for the discount rate. A positive NPV indicates that the benefits outweigh the costs.
- Sensitivity Analysis: Conduct sensitivity analysis to understand how changes in assumptions (e.g., cost estimates, benefit projections) affect the outcome. This helps in assessing the robustness of the analysis.
- Decision Making: Use the results of the CBA to guide decision-making. If the benefits significantly exceed the costs, the initiative may be worth pursuing.
- Documentation: Keep thorough documentation of the analysis process, assumptions made, and results obtained. This transparency is crucial for future reference and accountability.
By effectively measuring business impact and employing a robust cost-benefit analysis framework, organizations can enhance their decision-making processes, optimize resource allocation, and ultimately drive growth and profitability. At Rapid Innovation, we leverage our expertise in AI and Blockchain to provide tailored solutions that not only improve your ROI but also ensure that your initiatives align with your strategic objectives, leading to sustainable business success.
7.3. Long-term Scalability and Growth Potential
Long-term scalability is crucial for any business aiming to thrive in a competitive landscape. It refers to the ability of a company to grow and manage increased demand without compromising performance or losing revenue potential. Key factors that contribute to long-term scalability include:
- Infrastructure: A robust infrastructure is essential for scalability. This includes technology, human resources, and operational processes that can adapt to growth. Rapid Innovation specializes in developing scalable AI and blockchain solutions that ensure your infrastructure can handle increased demand seamlessly.
- Market Demand: Understanding market trends and consumer behavior can help businesses anticipate growth opportunities. Companies that can pivot quickly to meet changing demands are more likely to succeed. Our data analytics capabilities empower clients to gain insights into market trends, enabling them to make informed decisions.
- Financial Resources: Access to capital is vital for scaling operations. Businesses should explore various funding options, including venture capital, loans, and reinvestment of profits. Rapid Innovation can assist in identifying funding opportunities and optimizing financial strategies to support growth.
- Talent Acquisition: As a company grows, attracting and retaining skilled employees becomes increasingly important. A strong company culture and competitive compensation packages can aid in this effort. We provide consulting services to help organizations build a talent acquisition strategy that aligns with their growth objectives.
- Technology Adoption: Leveraging technology can streamline operations and enhance productivity. Automation and data analytics can provide insights that drive strategic decisions. Rapid Innovation offers tailored AI solutions that automate processes, allowing businesses to focus on core activities while improving efficiency.
- Partnerships and Collaborations: Forming strategic alliances can open new markets and enhance service offerings, contributing to long-term growth. Our expertise in blockchain technology facilitates secure and transparent partnerships, enabling businesses to collaborate effectively and expand their reach.
8. Future Outlook and Strategic Considerations
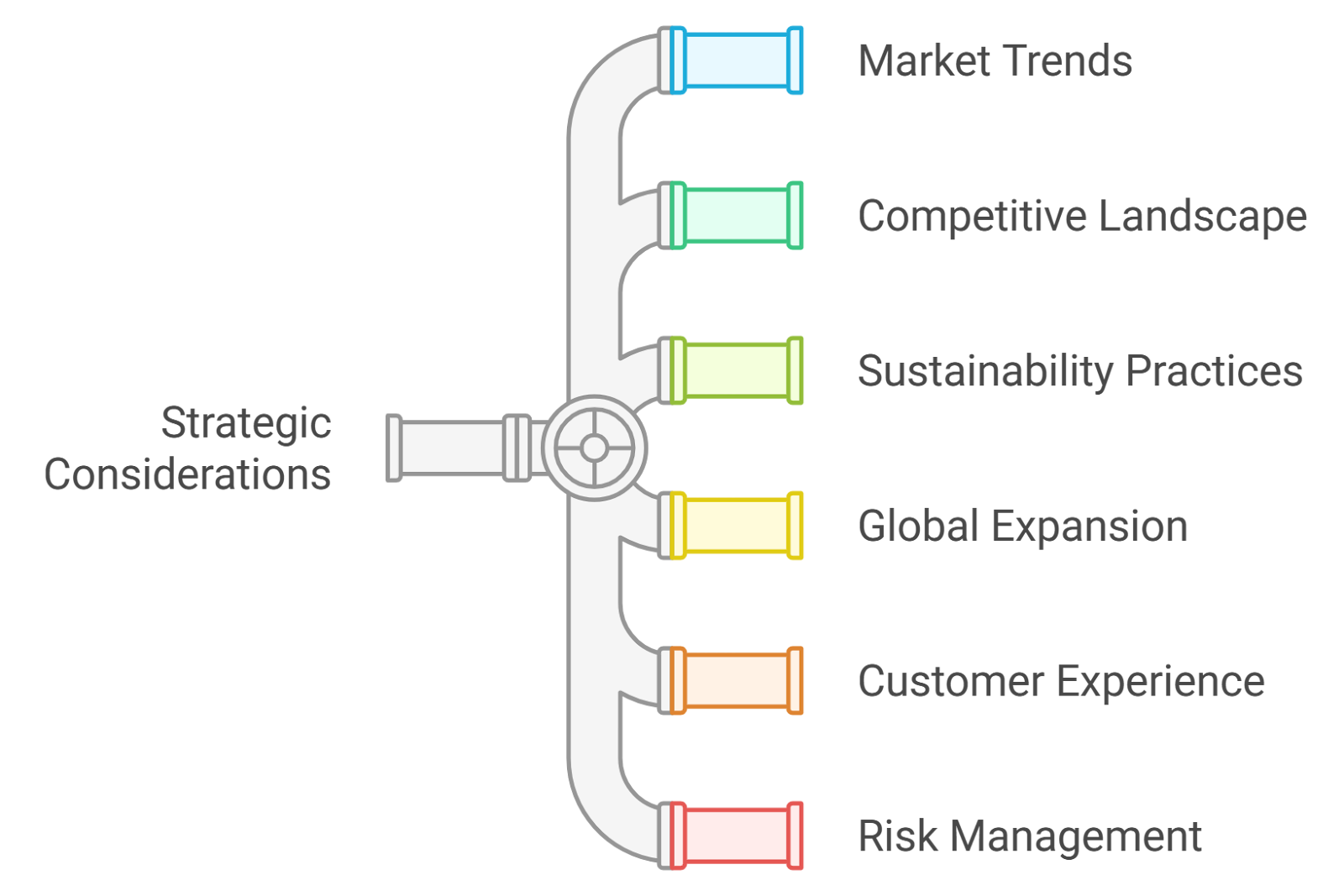
The future outlook for businesses is shaped by various strategic considerations that can influence their trajectory. Important aspects to consider include:
- Market Trends: Keeping an eye on emerging trends can help businesses stay ahead of the curve. This includes shifts in consumer preferences, technological advancements, and regulatory changes.
- Competitive Landscape: Understanding competitors' strategies and market positioning is essential. Companies should conduct regular SWOT analyses to identify their strengths, weaknesses, opportunities, and threats.
- Sustainability Practices: Increasingly, consumers are favoring brands that prioritize sustainability. Implementing eco-friendly practices can enhance brand reputation and customer loyalty.
- Global Expansion: Exploring international markets can provide new revenue streams. However, businesses must consider cultural differences, legal requirements, and market entry strategies.
- Customer Experience: Focusing on customer satisfaction can lead to repeat business and referrals. Companies should invest in customer service training and feedback mechanisms to improve the overall experience.
- Risk Management: Identifying potential risks and developing mitigation strategies is crucial for long-term success. This includes financial, operational, and reputational risks.
8.1. Emerging Technologies and Integration Opportunities
Emerging technologies present significant opportunities for businesses to innovate and improve efficiency. Key technologies to consider include:
- Artificial Intelligence (AI): AI can enhance decision-making processes, automate routine tasks, and provide personalized customer experiences. Companies should explore AI applications relevant to their industry. Rapid Innovation offers AI-driven solutions that can transform your business operations and customer interactions.
- Blockchain: This technology offers transparency and security in transactions. Businesses can leverage blockchain for supply chain management, contract execution, and data integrity. Our blockchain solutions ensure that your transactions are secure and verifiable, enhancing trust with stakeholders.
- Internet of Things (IoT): IoT devices can collect valuable data that can inform business strategies. Companies can use this data to optimize operations and enhance product offerings.
- Cloud Computing: Cloud solutions provide flexibility and scalability, allowing businesses to adjust resources based on demand. This can lead to cost savings and improved collaboration.
- Augmented Reality (AR) and Virtual Reality (VR): These technologies can transform customer engagement and training processes. Businesses can create immersive experiences that enhance brand interaction.
- Cybersecurity: As technology evolves, so do cyber threats. Investing in robust cybersecurity measures is essential to protect sensitive data and maintain customer trust.
By focusing on these areas, businesses can position themselves for sustainable growth and success in an ever-evolving market landscape. Rapid Innovation is committed to helping clients navigate these challenges and leverage technology for maximum ROI.
In this context, understanding business scalability is essential. Companies should define scalable business models that allow for growth without sacrificing quality. Exploring scalable business ideas can lead to innovative solutions that meet market demands. The best scalable businesses often have a clear scalable business definition that guides their strategies. Examples of scalability in business can be seen in companies that have successfully built a scalable business, demonstrating the importance of scalability in achieving long-term success.
8.2. Regulatory Landscape and Compliance Requirements
Understanding the regulatory landscape is crucial for businesses operating in any industry. Compliance requirements can vary significantly based on the sector, geographical location, and specific business activities. Regulatory bodies often set standards to ensure safety, fairness, and transparency in business operations. Businesses must stay updated on local, national, and international regulations that may impact their operations. Key areas of compliance include data protection, environmental regulations, labor laws, and financial reporting standards.
For instance, the General Data Protection Regulation (GDPR) in Europe imposes strict rules on data handling and privacy, affecting how companies manage customer information. Non-compliance can lead to hefty fines and reputational damage. Companies should conduct regular audits to ensure adherence to regulations. Training employees on compliance matters is essential to foster a culture of accountability. Engaging with legal experts can help navigate complex regulatory environments.
At Rapid Innovation, we leverage our expertise in AI and Blockchain to help businesses not only understand but also comply with these regulations efficiently. For example, our AI-driven compliance solutions can automate the monitoring of regulatory changes, ensuring that businesses are always aligned with the latest requirements. Additionally, our Blockchain technology can enhance transparency and traceability in data handling, significantly reducing the risk of non-compliance. We also offer regulatory compliance software and regulatory compliance management software to streamline these processes, including our enterprise AI development services and AI agents for compliance monitoring.
8.3. Strategic Roadmap for Business Implementation
A strategic roadmap is essential for guiding businesses through the implementation of new initiatives or technologies. It provides a clear framework for achieving goals and aligning resources effectively.
- Define clear objectives: Establish what the business aims to achieve with the new implementation.
- Assess current capabilities: Evaluate existing resources, skills, and technologies to identify gaps.
- Develop a phased approach: Break down the implementation into manageable stages to facilitate smoother transitions.
Key components of a strategic roadmap include:
- Stakeholder engagement: Involve key stakeholders early in the process to gain insights and foster buy-in.
- Resource allocation: Determine the budget, personnel, and technology needed for successful implementation, including regulatory compliance software solutions.
- Performance metrics: Establish KPIs to measure progress and success throughout the implementation process.
Regularly reviewing and adjusting the roadmap based on feedback and changing circumstances is vital for long-term success. Rapid Innovation can assist in crafting a tailored strategic roadmap that integrates AI and Blockchain technologies, ensuring that your business not only meets its objectives but also maximizes ROI through innovative solutions, including regulatory compliance tracking software.
9. Case Studies and Real-World Applications
Case studies provide valuable insights into how businesses successfully implement strategies and navigate challenges. They serve as practical examples for others in similar situations.
- Company A: A tech startup implemented a new customer relationship management (CRM) system, resulting in a 30% increase in customer retention rates. By training staff and integrating the system with existing tools, they streamlined operations and improved customer satisfaction.
- Company B: A manufacturing firm adopted sustainable practices, reducing waste by 40% and cutting costs by 15%. By investing in eco-friendly technologies and engaging employees in sustainability initiatives, they enhanced their brand reputation and attracted environmentally conscious consumers.
- Company C: A retail chain utilized data analytics to optimize inventory management, leading to a 20% reduction in stockouts. By analyzing customer purchasing patterns, they improved supply chain efficiency and increased sales.
These real-world applications highlight the importance of strategic planning, compliance, and adaptability in achieving business success. At Rapid Innovation, we are committed to helping our clients navigate these challenges through our advanced AI and Blockchain solutions, ultimately driving greater ROI and sustainable growth, while ensuring adherence to regulatory compliance management systems.
9.1. Enterprise Digital Transformation Success Stories
Digital transformation has become a crucial strategy for enterprises aiming to enhance efficiency, improve customer experience, and stay competitive. Here are some notable success stories:
- General Electric (GE): GE embraced digital transformation by integrating the Industrial Internet of Things (IIoT) into its operations. The company developed Predix, a cloud-based platform that connects machines and analyzes data to optimize performance. This initiative led to significant cost savings and improved operational efficiency, showcasing how Rapid Innovation can assist clients in leveraging IIoT for enhanced operational capabilities. This is one of the many digital transformation success stories that highlight the impact of technology on traditional industries.
- Nike: Nike leveraged digital transformation to enhance its supply chain and customer engagement. By implementing data analytics and personalized marketing strategies, Nike improved its online sales and customer loyalty, reporting a 30% increase in digital sales in a single quarter. Rapid Innovation can help businesses like Nike harness AI-driven analytics to tailor customer experiences and drive sales growth. This is a prime example of success stories of digital transformation that illustrate the benefits of data-driven decision-making.
- Domino's Pizza: Domino's transformed its business model by adopting digital technologies for ordering and delivery. The introduction of an AI-powered chatbot and a user-friendly mobile app streamlined the ordering process, resulting in a 20% increase in sales. Rapid Innovation specializes in developing AI solutions that can optimize customer interactions and improve service delivery. This transformation is among the success stories of digital transformation companies that have effectively utilized technology to enhance customer experience.
9.2. Industry-Specific Implementation Examples
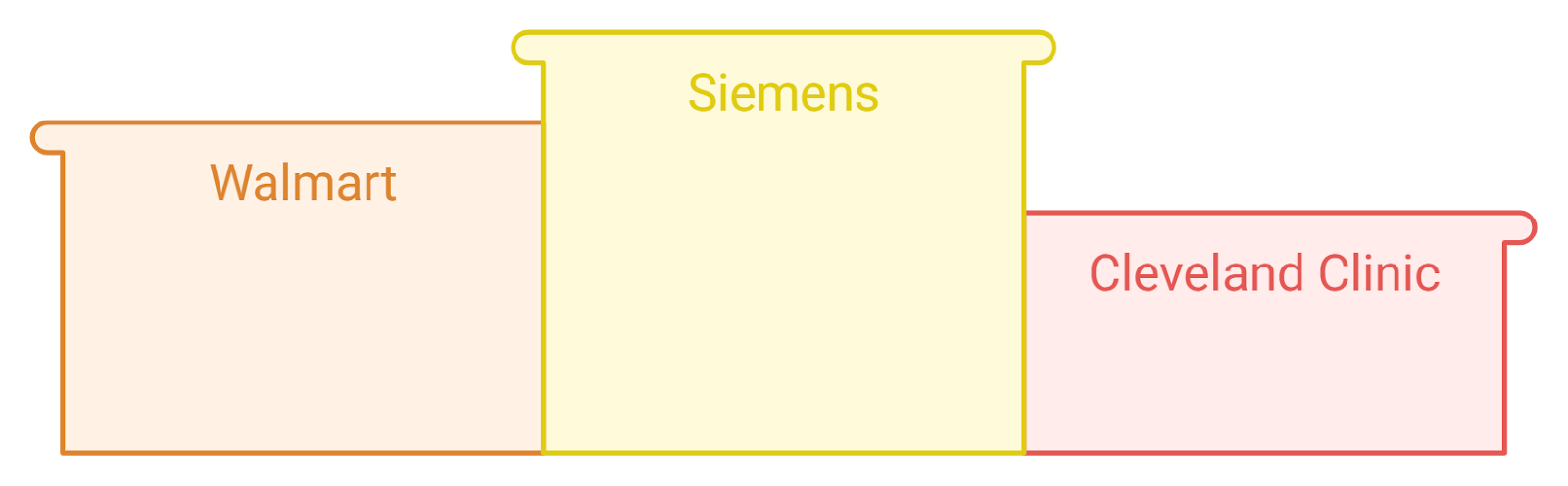
Different industries have unique challenges and opportunities when it comes to digital transformation. Here are some industry-specific examples:
- Healthcare: The Cleveland Clinic implemented a digital health platform that allows patients to access their medical records, schedule appointments, and communicate with healthcare providers online. This initiative improved patient engagement and streamlined administrative processes. Rapid Innovation can support healthcare organizations in developing secure, user-friendly platforms that enhance patient care through blockchain technology. This is a notable example of success stories of business using digital transformation in the healthcare sector.
- Retail: Walmart adopted a digital transformation strategy by utilizing big data analytics to optimize inventory management and enhance customer experience. The company implemented machine learning algorithms to predict demand, resulting in reduced stockouts and improved sales. Rapid Innovation can assist retailers in deploying AI solutions that enhance inventory accuracy and customer satisfaction. This case reflects the broader trend of transformation success stories in the retail industry.
- Manufacturing: Siemens implemented digital twin technology in its manufacturing processes. By creating virtual replicas of physical assets, Siemens improved product design and operational efficiency, leading to a 30% reduction in time-to-market for new products. Rapid Innovation can help manufacturers implement similar digital twin solutions to streamline operations and accelerate product development. This is one of the many business transformation success stories that demonstrate the power of digital tools in manufacturing.
9.3. Lessons Learned and Best Practices
As enterprises embark on their digital transformation journeys, several lessons and best practices have emerged:
- Start with a Clear Vision: Establish a clear digital transformation strategy aligned with business goals. This vision should guide all initiatives and help prioritize projects.
- Engage Stakeholders: Involve employees, customers, and other stakeholders in the transformation process. Their insights can provide valuable feedback and foster a culture of innovation.
- Invest in Training: Equip employees with the necessary skills to adapt to new technologies. Continuous training and development are essential for successful implementation.
- Leverage Data Analytics: Utilize data analytics to drive decision-making. Analyzing customer behavior and operational data can uncover insights that lead to improved strategies.
- Iterate and Adapt: Digital transformation is an ongoing process. Be prepared to iterate on strategies and adapt to changing market conditions and technological advancements.
- Measure Success: Establish key performance indicators (KPIs) to measure the success of digital transformation initiatives. Regularly review these metrics to assess progress and make necessary adjustments.
By partnering with Rapid Innovation, enterprises can effectively navigate their digital transformation journeys, leveraging AI and blockchain technologies to achieve greater ROI and operational excellence. The success stories of digital transformation serve as a testament to the potential of technology in driving business growth and innovation.
10. How to Get Started With Multi-Agent AI?
Getting started with Multi-Agent AI implementation involves understanding the fundamental concepts, identifying the right tools, and developing a clear strategy for implementation. Multi-Agent AI refers to systems where multiple agents interact, collaborate, or compete to achieve specific goals. Here’s how to embark on this journey:
- Understand the basics of Multi-Agent Systems (MAS).
- Identify the specific use cases relevant to your organization.
- Research existing frameworks and platforms that support Multi-Agent AI implementation.
- Assemble a team with expertise in AI, machine learning, and system integration.
- Develop a clear project plan that outlines objectives, resources, and timelines.
10.1. Evaluation and Selection Criteria?
When evaluating and selecting a Multi-Agent AI implementation system, several criteria should be considered to ensure the chosen solution meets your needs effectively:
- Scalability: The system should handle an increasing number of agents without performance degradation.
- Interoperability: Ensure compatibility with existing systems and technologies.
- Flexibility: The ability to adapt to changing requirements and environments is crucial.
- Performance Metrics: Evaluate based on response time, accuracy, and resource consumption.
- Community and Support: A strong user community and support resources can be invaluable for troubleshooting and enhancements.
- Cost: Consider both initial investment and ongoing operational costs.
10.2. Implementation Roadmap and Timeline?
Creating an implementation roadmap for Multi-Agent AI is essential for a structured approach. Here’s a suggested timeline and key phases:
- Phase 1: Research and Planning (1-2 months): Conduct a needs assessment, identify potential use cases and objectives, and research available technologies and frameworks.
- Phase 2: Design and Prototyping (2-3 months): Develop a system architecture, create prototypes to test concepts and functionalities, and gather feedback from stakeholders.
- Phase 3: Development (3-6 months): Build the Multi-Agent AI implementation system based on the design, implement necessary integrations with existing systems, and conduct iterative testing and refinement.
- Phase 4: Deployment (1-2 months): Roll out the system in a controlled environment, monitor performance and gather user feedback, and make necessary adjustments based on real-world usage.
- Phase 5: Maintenance and Scaling (Ongoing): Regularly update the system to incorporate new features and improvements, and scale the system as needed based on user demand and performance metrics.
By following these steps, organizations can effectively implement Multi-Agent AI systems that enhance operational efficiency and drive innovation. At Rapid Innovation, we specialize in guiding businesses through this process, ensuring that your Multi-Agent AI implementation aligns with your strategic goals and delivers a significant return on investment. Our expertise in AI and system integration allows us to tailor solutions that not only meet your current needs but also adapt to future challenges, maximizing your operational capabilities.
10.3. Resource Planning and Team Development
Resource planning and team development are critical components of project management and organizational success. Effective resource planning ensures that the right resources are allocated to the right tasks, while team development focuses on building a cohesive and skilled workforce. Here’s a detailed look at both aspects.
Resource Planning
Resource planning involves identifying, allocating, and managing resources effectively to achieve project goals. This includes human resources, financial resources, and physical assets.
- Identifying Resources: Assess the types of resources needed for the project, considering both tangible (equipment, materials) and intangible (skills, knowledge) resources. At Rapid Innovation, we leverage AI-driven analytics to accurately forecast resource needs based on project scope and historical data.
- Resource Allocation: Assign resources based on project requirements and team members' strengths. Use tools like Gantt charts or resource allocation software to visualize resource distribution. Our blockchain solutions can enhance transparency in resource allocation, ensuring accountability and traceability.
- Budgeting: Develop a budget that outlines the costs associated with each resource and monitor expenses to ensure the project stays within budget. By utilizing AI algorithms, we can predict budget variances and optimize spending in real-time.
- Resource Optimization: Analyze resource usage to identify areas for improvement and implement strategies to maximize efficiency, such as cross-training team members. Our AI tools can provide insights into resource utilization patterns, enabling better decision-making.
- Risk Management: Identify potential resource-related risks and develop mitigation strategies. Regularly review resource allocation to adapt to changing project needs. Our blockchain technology can help in tracking risks and ensuring compliance, thus minimizing potential disruptions.
Team Development
Team development focuses on enhancing the skills, collaboration, and overall performance of team members. A well-developed team can significantly impact project success.
- Skill Assessment: Evaluate the current skills of team members to identify gaps. Use assessments or surveys to gather information on individual strengths and weaknesses. Rapid Innovation employs AI-driven assessments to provide a comprehensive view of team capabilities.
- Training and Development: Provide training programs to enhance skills and knowledge, and encourage continuous learning through workshops, online courses, or mentorship. Our AI platforms can personalize training programs based on individual learning styles and career goals, including career preparation worksheets developed by writing team members.
- Team Building Activities: Organize team-building exercises to foster collaboration and trust. Activities can range from problem-solving tasks to social events. We integrate gamification techniques into team-building activities to enhance engagement and effectiveness.
- Clear Communication: Establish open lines of communication within the team. Use tools like Slack or Microsoft Teams to facilitate discussions and updates. Our solutions can integrate communication tools with project management systems for seamless information flow.
- Setting Goals and Expectations: Define clear, measurable goals for the team and ensure that each team member understands their role and responsibilities. We utilize AI to track progress against goals and provide actionable insights for improvement.
- Feedback and Recognition: Implement a system for regular feedback to help team members improve, and recognize and reward achievements to boost morale and motivation. Our platforms can automate feedback collection and recognition processes, ensuring timely and meaningful interactions.
- Conflict Resolution: Develop strategies for addressing conflicts within the team and encourage open dialogue to resolve issues before they escalate. We provide AI-driven mediation tools that can analyze communication patterns and suggest resolutions.
- Diversity and Inclusion: Promote a diverse team to bring different perspectives and ideas, and foster an inclusive environment where all team members feel valued. Our commitment to diversity is reflected in our hiring practices and team-building initiatives.
Effective resource planning and team development are interlinked. A well-planned resource allocation can enhance team performance, while a skilled team can optimize resource usage. By focusing on both aspects, organizations can improve project outcomes and drive success. At Rapid Innovation, we are dedicated to helping our clients achieve greater ROI through strategic resource management and team development initiatives, including our project estimation services.