Table Of Contents
Category
Artificial Intelligence
1. Introduction
Artificial Intelligence (AI) has permeated various sectors, revolutionizing how we interact with technology and make decisions in business, healthcare, and everyday life. This book delves into the practical applications of AI, providing readers with a comprehensive understanding of its impact and potential. By exploring real-world case studies, the book aims to bridge the gap between theoretical AI concepts and their practical implementations.
1.1. The Importance of Real-World AI Case Studies
Real-world AI case studies are crucial as they provide tangible examples of how AI technologies are being utilized across different industries. These case studies not only illustrate the capabilities and limitations of AI but also offer insights into the ethical considerations and the societal impact of deploying AI solutions. By examining specific instances where AI has been applied, readers can better appreciate the nuances and complexities involved in integrating these technologies into real-world scenarios. For more detailed examples, you can explore AI Innovations in the NFT Ecosystem: Enhancing Art, Security, and Market Dynamics and Revolutionizing Legal Practices with AI: Applications and Benefits.
1.2. Overview of the Book's Structure
The structure of the book is designed to guide readers through a logical progression of AI applications, from basic concepts to more complex implementations. It begins with an introduction to fundamental AI principles, followed by chapters that each focus on different sectors such as healthcare, finance, automotive, and more. Each chapter presents case studies that highlight specific AI applications, followed by discussions on the outcomes, challenges, and future prospects. This structured approach helps readers build a comprehensive understanding of how AI is shaping various industries. For a broader perspective, consider exploring Top 15 AI Agent Use Cases in Business.
Implementing AI in any organization or system requires a solid foundation that not only involves understanding the key technologies and concepts but also addressing the ethical considerations associated with its deployment. This ensures that the AI systems are not only effective but also operate within accepted moral and ethical boundaries.
2. Foundations of AI Implementation
2.1 Key AI Technologies and Concepts
AI technologies encompass a broad range of tools and methodologies that enable machines to mimic human intelligence. These include machine learning (ML), natural language processing (NLP), robotics, and computer vision, among others. Machine learning, for instance, uses algorithms to parse data, learn from it, and then make a determination or prediction about something in the world. Natural language processing allows machines to understand and interpret human language, enabling applications like chatbots and virtual assistants.
Each of these technologies relies on data to learn and improve over time. As such, data management becomes a crucial aspect of AI implementation. This involves data collection, storage, processing, and analysis, all of which must be handled securely and efficiently to build effective AI systems.
2.2 Ethical Considerations in AI Deployment
The deployment of AI systems brings with it a host of ethical considerations that must be addressed to maintain trust and fairness. One major concern is the potential for AI to perpetuate existing biases found in the data it is trained on. This can lead to unfair outcomes, particularly in sensitive areas such as hiring, law enforcement, and loan approvals.
Privacy is another significant ethical issue. AI systems often require vast amounts of data, which can include personal information. Ensuring that this data is collected, stored, and used in compliance with privacy laws and standards is crucial to protecting individual rights.
Finally, there is the issue of accountability. It must be clear who is responsible for the decisions made by AI systems, especially when these decisions have significant impacts on people’s lives. Establishing clear guidelines and accountability frameworks can help address these concerns, ensuring that AI systems are used responsibly and ethically. For a deeper understanding, consider exploring Revolutionizing Legal Practices with AI: Applications and Benefits.
2.3. AI Project Lifecycle and Management
Understanding the Lifecycle
The lifecycle of an AI project encompasses several stages, starting from defining the problem and data collection, to model training, deployment, and ongoing management. Each phase requires careful planning and execution to ensure the project meets its intended goals.
Key Management Practices
Effective management is crucial in navigating the AI project lifecycle. This involves setting clear objectives, ensuring data quality, selecting the right tools and technologies, and continuously monitoring the system’s performance post-deployment. Stakeholder engagement and cross-functional collaboration are also vital to address ethical considerations and align the project outcomes with business objectives.
3. AI in Healthcare
Revolutionizing Patient Care
AI in healthcare is transforming patient care by enhancing diagnostic accuracy, personalizing treatment plans, and improving operational efficiencies. By analyzing vast amounts of healthcare data, AI can identify patterns that are imperceptible to humans, leading to earlier and more accurate diagnoses.
Challenges and Opportunities
While AI presents significant opportunities in healthcare, it also poses challenges such as data privacy concerns, the need for large annotated datasets, and the integration of AI tools into existing healthcare systems. Addressing these challenges is essential for the successful adoption of AI technologies in healthcare settings.
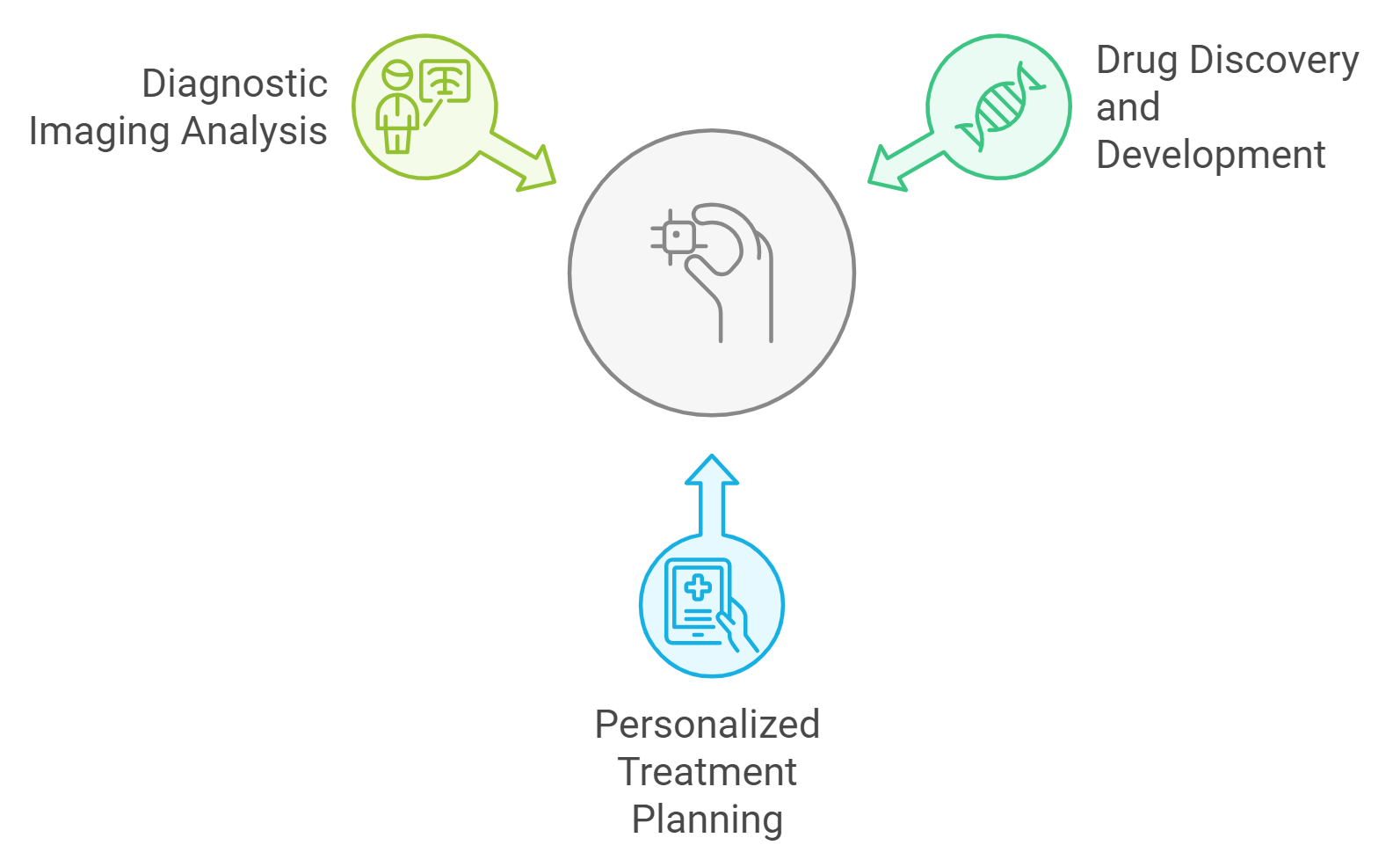
3.1. Diagnostic Imaging Analysis
Enhancing Diagnostic Accuracy
AI technologies, particularly deep learning, have shown remarkable capabilities in analyzing medical images such as X-rays, CT scans, and MRIs. These AI systems can detect abnormalities with high accuracy, often matching or surpassing expert radiologists in speed and precision.
Impact on Radiology
The integration of AI into diagnostic imaging is not only improving diagnostic accuracy but also reducing the workload on radiologists by automating routine tasks. This allows healthcare professionals to focus more on patient care and complex cases, thereby enhancing the overall efficiency of healthcare services.
3.2 Drug Discovery and Development
Accelerating Research with AI
Artificial Intelligence (AI) significantly accelerates the process of drug discovery and development. By analyzing vast datasets, AI can identify potential drug candidates much faster than traditional methods. This not only speeds up the research phase but also reduces the costs associated with it.
Enhancing Precision and Efficiency
AI algorithms can predict how different chemicals will interact with the body, which improves the precision of drug development. This capability allows pharmaceutical companies to focus on the most promising compounds, streamlining the development process and increasing the likelihood of success in clinical trials.
3.3 Personalized Treatment Planning
Tailoring Medical Treatments
Personalized treatment planning utilizes AI to analyze individual patient data, including genetic information, lifestyle, and previous health records. This analysis helps healthcare providers develop tailored treatment plans that are significantly more effective than one-size-fits-all approaches. Personalized treatments can lead to better patient outcomes and more efficient use of healthcare resources.
Predictive Analytics in Healthcare
AI-driven predictive analytics can anticipate the progression of a disease in a patient, allowing for early intervention and more effective management. This proactive approach in healthcare not only improves the quality of life for patients but also reduces the overall cost of healthcare by preventing severe disease phases.
3.4 Case Study: IBM Watson Health
Revolutionizing Healthcare with Cognitive Computing
IBM Watson Health represents a pioneering example of how AI can be applied in the field of healthcare. Watson's cognitive computing ability enables it to process and analyze vast amounts of unstructured data, including medical journals, research papers, and clinical trial data, which can be used to inform treatment decisions and health policies.
Challenges and Achievements
Despite facing challenges such as data privacy concerns and the initial skepticism of healthcare professionals, IBM Watson Health has achieved notable successes. It has been used in oncology to assist in diagnosing and treating cancer, offering recommendations that are informed by the latest research and clinical practices. Watson's ability to keep up-to-date with medical literature and clinical studies supports healthcare providers in delivering evidence-based treatment plans.
4. AI in Finance
The integration of Artificial Intelligence (AI) in finance is revolutionizing the industry by enhancing efficiency, accuracy, and profitability. AI technologies are being employed in various aspects of financial services, from algorithmic trading to fraud detection and risk management, fundamentally changing how institutions operate and deliver services to their customers.

4.1 Algorithmic Trading
Algorithmic trading uses AI to make high-speed trading decisions to capitalize on market opportunities. By analyzing vast amounts of market data and executing trades at optimal times, AI algorithms can outperform human traders in terms of both speed and precision. These systems learn from market patterns and changes, continuously improving their trading strategies over time. Learn more about
The use of AI in algorithmic trading has led to increased market efficiency. However, it also raises concerns such as potential market manipulation and systemic risks due to interconnected AI systems. Regulatory bodies are thus keenly observing these developments to ensure a stable trading environment.
4.2 Fraud Detection and Risk Assessment
AI significantly improves the ability of financial institutions to detect and prevent fraudulent activities. By analyzing patterns and trends in data that may indicate fraudulent behavior, AI systems can identify irregularities faster and more accurately than ever before. This capability not only helps in minimizing losses but also in improving customer trust and compliance with regulatory requirements.
In risk assessment, AI algorithms assess the risk levels associated with loans, insurance policies, and other financial activities by considering a multitude of factors, including market conditions, customer behavior, and economic indicators. This holistic view helps financial institutions make more informed decisions, tailor services to individual risk profiles, and mitigate potential losses effectively. Discover more about
AI's role in finance is expanding, and as it does, it will continue to transform the landscape of the industry. By leveraging AI, financial services can not only enhance operational efficiencies but also offer more secure, personalized, and innovative services to their clients.
4.3 Robo-advisors and Personal Finance
Robo-advisors are digital platforms that provide automated, algorithm-driven financial planning services with minimal human supervision. They analyze user data to offer financial advice and automatically manage investments. These platforms are accessible, cost-effective, and are becoming increasingly popular among tech-savvy investors.
Robo-advisors offer several advantages, including lower fees compared to traditional financial advisors, 24/7 availability, and the ability to handle complex calculations effortlessly. They are particularly appealing to younger investors who prefer digital interactions and have straightforward financial planning needs.
4.4 Case Study: JPMorgan's COIN (Contract Intelligence) Platform
JPMorgan Chase introduced the COIN platform to streamline the labor-intensive process of interpreting commercial loan agreements. By utilizing Natural Language Processing (NLP), COIN automates the analysis of legal documents, significantly reducing the time and errors associated with manual review.
The implementation of COIN has revolutionized how JPMorgan handles complex documentation. It has cut down the time required to review documents from 360,000 hours to mere seconds per document, enhancing efficiency and reducing costs. This case study exemplifies how AI can transform traditional banking operations.
5. AI in Manufacturing
Artificial Intelligence is reshaping the manufacturing sector by optimizing production lines, improving supply chain efficiency, and enhancing product quality. AI technologies like machine learning models predict equipment failures, automate quality control, and streamline inventory management.
The integration of AI in manufacturing leads to significant cost reductions, increased productivity, and higher customer satisfaction. However, it also presents challenges such as the need for skilled workers to manage AI systems and concerns over job displacement due to automation. Despite these challenges, the potential benefits make AI a critical advancement in the manufacturing industry.
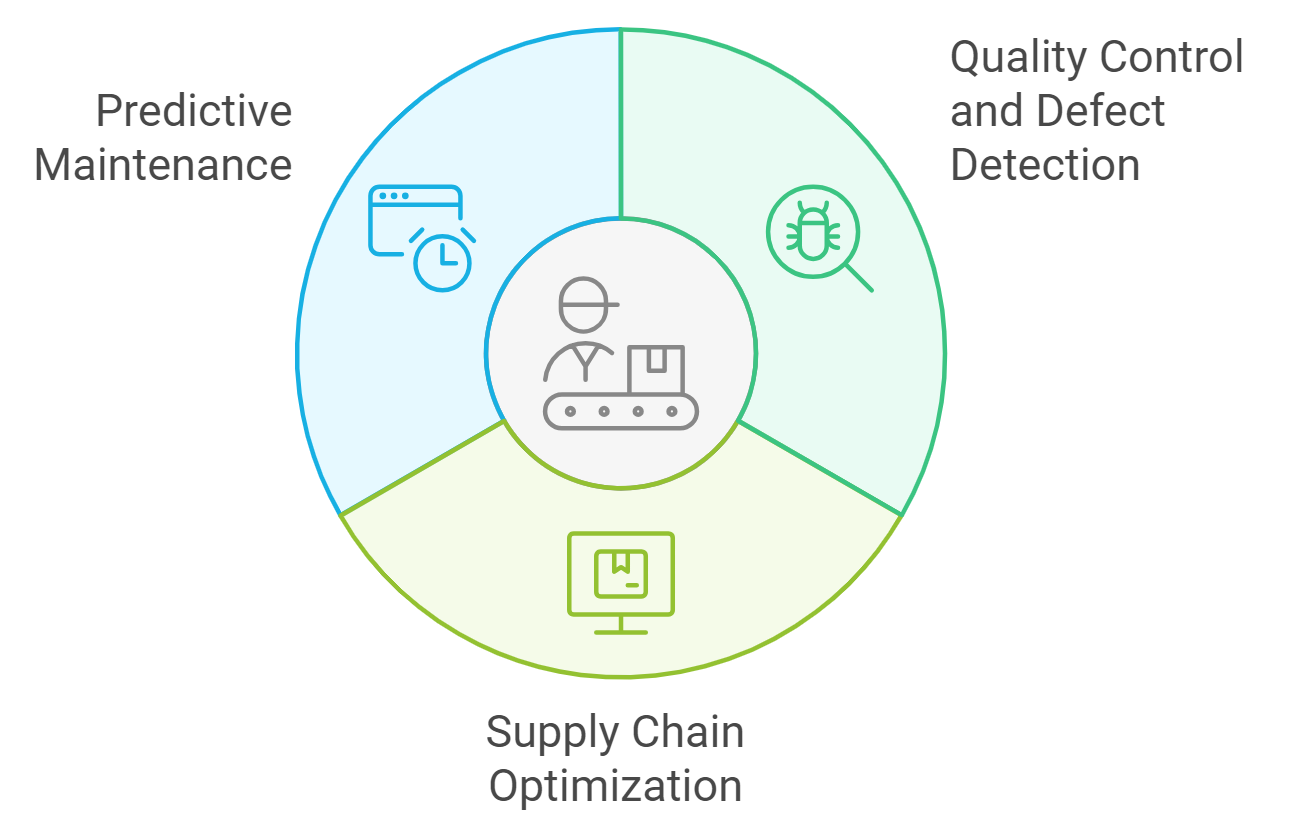
5.1 Predictive Maintenance
Predictive maintenance refers to the technique of using data analysis tools and techniques to detect anomalies in the operation and possible defects in equipment and processes before they fail. This approach uses data from various sources including historical performance, sensors, and real-time inputs to predict equipment failures before they happen, thereby reducing maintenance costs and downtime.
5.2 Quality Control and Defect Detection
Quality control and defect detection are critical components in manufacturing, ensuring that the products meet strict standards and specifications. Advanced technologies like machine learning, computer vision, and automated inspection systems play a pivotal role in identifying and quantifying defects. These technologies can analyze vast amounts of data from production lines in real-time to detect anomalies that might indicate defects.
The integration of these technologies leads to higher consistency in product quality and compliance with international standards. Automated systems provide a more objective assessment of quality compared to manual inspections, which can be subjective and inconsistent. By detecting defects early in the manufacturing process, companies can save on rework costs and prevent faulty products from reaching the consumer, thereby protecting the brand reputation.
5.3 Supply Chain Optimization
Supply chain optimization involves streamlining operations to enhance efficiency and reduce costs. This includes automating inventory management, improving logistics and distribution strategies, and using predictive analytics to forecast demand and adjust supply levels accordingly. Effective supply chain management ensures that products are delivered on time, inventory levels are kept optimal, and the production schedules are aligned with market demand.
The use of advanced analytics and IoT devices in supply chain management allows for real-time monitoring and decision-making. These tools help in identifying bottlenecks, predicting possible disruptions, and facilitating quick responses to changing market conditions. By optimizing the supply chain, companies can not only reduce operational costs but also improve customer satisfaction by delivering products faster and more reliably.
5.4 Case Study: Siemens' AI-driven Factory Automation
Siemens has been at the forefront of integrating artificial intelligence into its factory automation processes. Their approach focuses on enhancing efficiency, reducing downtime, and customizing production processes through advanced AI technologies.
In one of their flagship projects, Siemens implemented AI-driven systems that analyze machine data in real time. This allows for predictive maintenance, where the system can forecast equipment failures before they occur, significantly reducing unplanned downtime. Moreover, AI algorithms optimize production processes by adjusting operations dynamically based on various input factors, leading to higher productivity and reduced waste.
Siemens continues to invest in AI to further automate its production lines. The integration of AI not only streamlines manufacturing but also enables Siemens to offer new services such as detailed data analysis and consultancy for factory design, which adds a new revenue stream while enhancing customer satisfaction.

6. AI in Retail and E-commerce
AI technology has revolutionized the retail and e-commerce sectors by personalizing the shopping experience and optimizing business operations. From personalized marketing to inventory management, AI's role in retail extends across the entire value chain.
AI-powered chatbots and virtual assistants help businesses provide personalized customer service at scale. These tools can handle a multitude of customer queries simultaneously, providing quick responses that help in improving customer satisfaction and loyalty.
AI is also used to streamline operations in retail. For instance, AI algorithms predict optimal stock levels, automate replenishments, and even assist in managing supply chains more efficiently. This reduces overhead costs and improves the speed and accuracy of service delivery.
6.1 Recommendation Systems
AI-driven recommendation systems are crucial in e-commerce, enhancing user experience by suggesting products based on browsing history, purchase behavior, and user preferences. These systems use complex algorithms to analyze large datasets, ensuring that the recommendations are highly relevant and timely.
Effective recommendation systems can significantly boost sales by increasing the average order value and enhancing customer retention. They make shopping experiences more convenient and tailored, which encourages customers to make additional purchases.
While these systems are powerful, they also pose challenges such as privacy concerns and the need for high-quality data. However, the continuous improvement in AI algorithms offers opportunities for even more accurate and insightful product recommendations in the future.
6.2 Inventory Management and Demand Forecasting
Inventory management is crucial for businesses to ensure they have the right products available at the right time. AI enhances this process by predicting future product demands based on historical sales data, trends, and external factors such as weather or economic conditions. This precision helps companies reduce overstock and stockouts, optimizing their inventory levels and reducing costs.
AI algorithms can analyze vast amounts of data to forecast demand more accurately. This capability allows businesses to plan better and allocate resources more efficiently, ensuring they meet customer demand without excessive investment in inventory. Improved forecasting can lead to better customer satisfaction by having products available when needed while also increasing operational efficiency.
6.3 Customer Service Chatbots
Chatbots powered by AI are revolutionizing customer service by providing quick responses to customer inquiries without human intervention. These chatbots can handle a wide range of tasks from answering FAQs to more complex queries like order tracking or product recommendations. This not only enhances customer experience by providing instant support but also allows human agents to focus on more complex customer service issues.
AI chatbots learn from each interaction and continuously improve their responses. They can analyze customer sentiment and adapt their interactions accordingly, making them more effective over time. This adaptive learning helps businesses maintain a high level of customer service with minimal human oversight, reducing operational costs and improving customer satisfaction.
6.4 Case Study: Amazon's AI-powered Operations
Amazon utilizes AI across its operations, particularly in managing its vast logistics network. AI algorithms help optimize delivery routes and manage inventory in real-time, significantly reducing shipping times and costs. This efficiency is a key factor in Amazon's ability to offer services like same-day delivery, which has set a new standard in consumer expectations.
Amazon's AI also personalizes the shopping experience for millions of users. By analyzing individual customer behavior, previous purchases, and search histories, AI provides tailored product recommendations. This not only enhances the user experience but also increases the likelihood of purchases, driving Amazon's revenue growth.
Amazon's integration of AI into its operations provides a clear example of how AI can transform business processes, improve customer satisfaction, and drive economic efficiency.
7. AI in Transportation
The integration of Artificial Intelligence (AI) in transportation is revolutionizing how we commute, manage traffic, and maintain infrastructure. AI technologies are being leveraged to enhance efficiency, safety, and sustainability in various aspects of transportation.

7.1 Autonomous Vehicles
Self-Driving Technology
Autonomous vehicles (AVs) are perhaps the most well-known application of AI in transportation. These vehicles use AI algorithms to interpret sensor data, enabling them to navigate roads, recognize obstacles, and make real-time decisions without human intervention. This technology aims to reduce human error, which is a major cause of accidents, and to increase road safety.
Impact on Society
The widespread adoption of autonomous vehicles could significantly alter transportation landscapes by reducing traffic congestion and lowering emissions. Additionally, AVs hold the potential to provide new mobility options for the elderly and disabled, thus enhancing their quality of life.
7.2 Traffic Management and Optimization
Smart Traffic Control Systems
AI is also transforming traffic management systems. By analyzing data from traffic cameras, sensors, and GPS signals, AI algorithms can optimize traffic flow and signal timings. This helps in reducing congestion and minimizing waiting times at intersections, thereby improving the overall efficiency of the transportation network.
Predictive Traffic Modeling
AI-driven predictive models can forecast traffic conditions based on historical data and real-time information. This allows city planners and traffic management centers to proactively adjust signals and inform drivers about the best possible routes, thus preventing potential bottlenecks and enhancing the commuting experience.
7.3 Logistics and Route Planning
Importance of Efficient Logistics
In the realm of logistics, efficiency and timing are paramount. Companies strive to deliver goods in the most cost-effective and timely manner. Advanced route planning facilitated by AI significantly enhances these aspects by optimizing delivery routes and predicting potential delays.
AI Enhancements in Route Planning
AI technologies utilize vast amounts of data from various sources, including traffic patterns, weather conditions, and vehicle performance histories, to optimize route planning. This not only helps in reducing fuel consumption and delivery times but also increases overall operational efficiency. AI-driven tools can dynamically alter routes in real-time to avoid unexpected obstacles, ensuring that logistics operations are more adaptable and resilient. For more insights, read about the Impact of AI Agents on Supply Chain Efficiency and Logistics Management.
7.4 Case Study: Waymo's Self-Driving Technology
Waymo's Impact on Transportation
Waymo, a subsidiary of Alphabet Inc., is at the forefront of developing self-driving technology. Their autonomous vehicles are designed to improve safety and reduce human error on the roads by using sophisticated machine learning algorithms that enable them to navigate complex traffic environments.
Technological Innovations by Waymo
Waymo's vehicles are equipped with high-resolution cameras, radar, and lidar systems that create a detailed 3D map of their environment. This technology allows the vehicles to make split-second decisions that are crucial for safe navigation. Waymo's continuous improvements in their self-driving algorithms have shown promising results in reducing traffic accidents and enhancing mobility for everyone, including those unable to drive.
8. AI in Energy and Utilities
AI's Role in Modernizing Energy Systems
The energy sector is undergoing a significant transformation with the integration of AI technologies. AI is used to predict energy demand, optimize power generation, and enhance the efficiency of the electrical grid. This not only helps in reducing operational costs but also plays a crucial role in advancing sustainable energy practices.
Improving Renewable Energy Efficiency
AI algorithms are particularly effective in managing renewable energy sources such as wind and solar power, which are inherently intermittent and unpredictable. By accurately predicting weather conditions and energy production, AI enables utilities to better integrate renewable energy into the grid, thus increasing the reliability and stability of energy supply.
Smart Grid Technology
Smart grids, enhanced with AI, are another revolutionary aspect in this sector. They use real-time data analytics to automatically adjust the flow of electricity to where it's most needed, improving the efficiency and reliability of power distribution. This not only helps in reducing energy waste but also ensures that consumers receive a consistent and reliable energy supply, even during peak times or in adverse weather conditions.
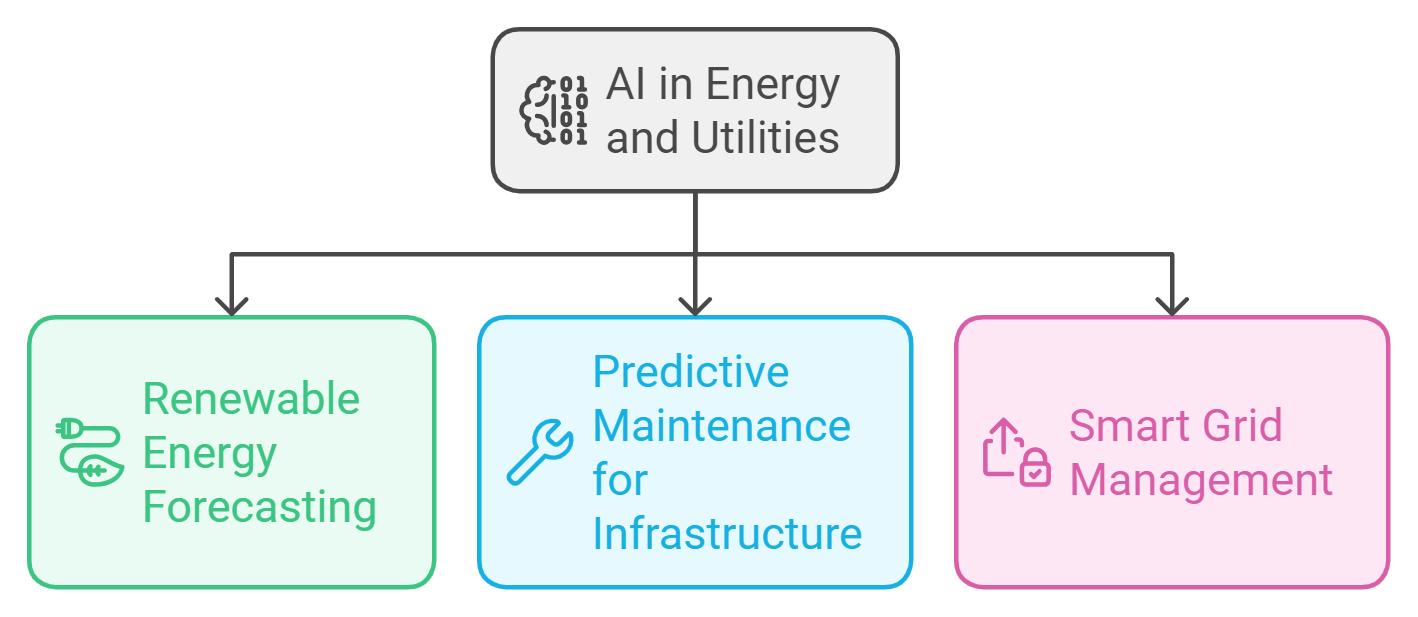
8.1 Smart Grid Management
Smart grid management utilizes advanced technologies like the Internet of Things (IoT) and Artificial Intelligence (AI) to enhance the efficiency and reliability of electricity distribution. By integrating these technologies, utilities can dynamically manage energy flow, respond to changes in demand in real-time, and improve system resilience against outages.
The use of real-time data analytics in smart grid management allows for the optimization of energy distribution and consumption. This not only helps in reducing energy waste but also aids in maintaining the balance between supply and demand. Moreover, it supports the integration of renewable energy sources into the grid, making energy systems more sustainable.
8.2 Renewable Energy Forecasting
Renewable energy forecasting is crucial for the effective integration of sources like solar and wind into the power grid. Accurate predictions help in planning the energy load and in making informed decisions about energy storage and distribution, ensuring that the variability of renewable sources does not affect the grid stability.
Advancements in weather prediction models and machine learning algorithms have significantly improved the accuracy of renewable energy forecasts. These technologies can analyze vast amounts of historical weather data to predict energy production levels, thereby optimizing the operation of renewable energy systems.
8.3 Predictive Maintenance for Infrastructure
Predictive maintenance for infrastructure involves the use of sensors and data analytics to monitor the condition of equipment and predict when maintenance should be performed. This approach helps in preventing unexpected equipment failures and reducing downtime, which in turn lowers maintenance costs and extends the lifespan of the equipment.
In the energy sector, predictive maintenance can be particularly beneficial for maintaining high-cost assets like turbines and transformers. Continuous monitoring and data analysis can detect potential failures before they occur, allowing for timely interventions that prevent costly repairs and potential safety hazards.
8.4 Case Study: DeepMind's AI for Google Data Center Cooling
DeepMind Technologies, a subsidiary of Alphabet Inc., has developed an AI system that optimizes the cooling processes in Google's data centers. This AI system uses a series of neural networks to predict the temperature and pressure conditions within the data centers and adjusts the cooling systems accordingly to improve efficiency.
The implementation of DeepMind's AI has led to a significant reduction in energy consumption. By optimizing cooling systems, Google has reported a 40% reduction in the amount of energy used for cooling, which translates to a 15% reduction in overall energy overhead. This not only supports sustainability efforts but also results in substantial cost savings for the company.
9. Challenges and Lessons Learned
Integrating AI into existing systems presents a myriad of challenges, from technical hurdles to organizational resistance. Companies often face difficulties in data integration, algorithm training, and system compatibility. Moreover, the shift to AI-driven processes requires a change in the workforce dynamics and operational procedures, which can meet resistance from employees accustomed to traditional methods.
9.1 Common Pitfalls in AI Implementation
One of the most common pitfalls in AI implementation is the underestimation of the importance of data quality and quantity. AI systems require large volumes of high-quality data to train effectively. Poor data can lead to inaccurate models and unreliable outputs, which can diminish the benefits of AI.
AI systems are not set-and-forget solutions. They need continuous monitoring, maintenance, and training to adapt to new data and changing conditions. Failure to provide ongoing support can lead to AI systems becoming obsolete or less effective over time.
While AI can significantly enhance efficiency and decision-making, it is crucial to maintain human oversight. Relying too heavily on AI without adequate checks and balances can lead to errors and oversight. Human expertise is essential to interpret AI outputs and make final decisions, especially in critical applications.
9.2 Overcoming Data Quality and Availability Issues
Data quality is crucial for the success of AI systems. Poor data quality can lead to inaccurate models and unreliable outcomes. To overcome these issues, organizations must implement robust data governance practices. This includes regular audits to ensure data accuracy, completeness, and consistency. Additionally, using advanced data cleaning tools and techniques can help in identifying and correcting errors before the data is used for training AI models.
Data availability is another critical factor. In many cases, the necessary data may not be readily available, or it may be fragmented across different systems. To address this, organizations can adopt data integration techniques, which consolidate data sources and provide a unified view that is more useful for AI applications. Furthermore, partnerships and data-sharing agreements can also be beneficial in enhancing the availability of high-quality data.
9.3 Addressing Bias and Fairness Concerns
AI systems can inadvertently perpetuate or even amplify existing biases if not carefully managed. To address bias and fairness concerns, it is essential to develop AI with diverse datasets that reflect the real-world distribution and demographics. Techniques such as bias detection algorithms can identify potential biases in AI behavior. Moreover, involving domain experts and ethicists in the development process can help in understanding and mitigating these biases effectively.
Ensuring fairness involves more than just adjusting data; it also requires continuous monitoring and updating of AI systems to adapt to new data and contexts. Implementing fairness metrics and regularly assessing AI decisions against these metrics can help ensure that AI systems operate equitably. Additionally, transparency in AI processes and decisions can build trust and allow stakeholders to understand and rectify any issues of fairness.
9.4 Scaling AI Solutions
Scaling AI solutions requires a robust infrastructure that can handle increased computational demands and data volumes. Cloud-based AI services offer scalable infrastructure solutions that can dynamically adjust resources based on the needs of the AI applications. This flexibility is crucial for efficiently managing the cost and performance of AI systems as they scale.
Modular design in AI development can facilitate scaling by allowing different parts of an AI system to be improved or replaced independently. This approach not only speeds up the development process but also makes it easier to integrate new technologies and methodologies. Furthermore, modular designs support the reuse of components across different AI projects, reducing time and cost.
By addressing these critical areas, organizations can enhance the effectiveness and reach of their AI initiatives, leading to more innovative and impactful outcomes.
10. Future Trends and Opportunities
The landscape of technology and business is perpetually evolving, with artificial intelligence (AI) at the forefront of creating transformative changes across various sectors. As we look to the future, several trends and opportunities in AI are poised to redefine industries, enhance operational efficiencies, and open new avenues for innovation.
10.1 Emerging AI Technologies
The development of more advanced machine learning models promises significant enhancements in AI capabilities. These models are becoming increasingly efficient at processing large datasets, leading to more accurate predictions and automation in various fields such as healthcare, finance, and autonomous vehicles.
Quantum computing, when combined with AI, is set to revolutionize problem-solving capacities. Quantum AI involves using quantum computing to improve machine learning algorithms. This technology is still in its infancy but has the potential to process information at speeds unattainable by traditional computers, which could drastically reduce the time needed for data-heavy tasks like drug discovery and traffic optimization. Learn more about the
The integration of AI with the Internet of Things (IoT) is another exciting frontier. This convergence enables smarter IoT devices that can analyze data and make decisions in real-time, improving everything from energy management in smart homes to predictive maintenance in manufacturing.
10.2 Cross-industry AI Applications
In healthcare, AI is being used to personalize patient care, improve diagnostic accuracy, and streamline administrative processes. AI-powered tools can analyze medical images, predict patient outcomes, and even assist in robotic surgeries, leading to faster and more effective patient care.
The financial sector benefits from AI in areas such as fraud detection, risk management, and customer service. AI algorithms can analyze transaction patterns to detect fraudulent activity more quickly than traditional methods. Additionally, AI-driven chatbots and automated advisors are transforming customer interactions, providing 24/7 service and personalized financial advice. Discover more about
AI is transforming the retail industry by enhancing customer experience and optimizing supply chain operations. Through AI, retailers can offer personalized shopping experiences, recommend products based on consumer behavior, and manage inventory more efficiently. AI-driven analytics also help in predicting market trends, which can be crucial for staying competitive. Explore how AI is reshaping retail through
As AI continues to evolve, its applications across different industries are expected to expand, leading to more innovative solutions and opportunities. The future of AI offers a promising horizon filled with potential for growth, efficiency, and groundbreaking advancements in technology and business practices.
10.3 The Role of AI in Addressing Global Challenges
Artificial Intelligence (AI) is revolutionizing healthcare by improving diagnostics and treatment options. AI algorithms can analyze complex medical data much faster than human capabilities, leading to earlier and more accurate diagnoses. For instance, AI-driven tools are used in radiology to detect anomalies in X-rays and MRIs with high precision.
AI also plays a crucial role in environmental conservation. It helps in monitoring deforestation, wildlife movements, and climate change impacts using satellite images and data analytics. AI-driven systems can predict natural disasters, such as floods and hurricanes, with greater accuracy, allowing for better preparedness and resource management.
In the field of education, AI enhances learning experiences through personalized learning. Systems powered by AI adapt to the individual learning pace and style of students, making education more accessible and effective. AI tools also help in automating administrative tasks, allowing educators to focus more on teaching and less on paperwork.
11. Conclusion
As we explore the vast capabilities of AI, it's clear that this technology holds significant potential to solve some of the most pressing global challenges. From healthcare and environmental conservation to education, AI is not just a tool of convenience but a pivotal force in driving positive change.
11.1 Key Takeaways from Real-World AI Implementations
Real-world implementations of AI in healthcare have shown promising results. AI applications in disease detection and personalized treatment plans have led to improvements in patient outcomes and healthcare efficiencies. These advancements underscore AI's potential to transform healthcare systems globally.
AI's role in environmental conservation has led to more effective monitoring and management of natural resources. AI-driven initiatives have successfully tracked illegal deforestation activities and have been instrumental in wildlife conservation efforts, showcasing the technology's ability to support sustainability.
In education, AI has facilitated the shift towards more personalized learning environments. Schools and institutions adopting AI technologies report higher engagement rates and improved learning outcomes, highlighting AI's capacity to redefine traditional educational paradigms.
In conclusion, AI's integration into various sectors illustrates its versatility and effectiveness in addressing diverse global challenges. As technology continues to evolve, its potential to foster significant improvements across different fields remains vast and largely untapped.
11.2 Best Practices for Successful AI Adoption
Before implementing AI, it's crucial to have a clear understanding of the business needs and goals. Identify specific problems that AI can solve and set measurable objectives. This alignment ensures that the AI solution adds value and enhances productivity.
Selecting the appropriate AI technology and partners is vital for successful adoption. Research and compare different AI tools and platforms to find the best fit for your specific needs. Partnering with reputable AI providers can also offer additional support and expertise.
AI systems require high-quality data to function effectively. Invest in robust data management practices to ensure data accuracy, availability, and security. Clean, well-organized data is a critical component of successful AI implementation.
AI adoption often requires changes in existing processes and roles. Provide comprehensive training to staff to help them adapt to new technologies and workflows. Effective change management practices can ease the transition and maximize the benefits of AI.
After implementing AI, continuously monitor its performance against the set goals. Use insights from performance data to make iterative improvements. Regular updates and adjustments help in refining the AI system and enhancing its effectiveness over time.
12. Appendices
A. Glossary of AI Terms
AI refers to the simulation of human intelligence in machines that are programmed to think and learn like humans. It encompasses a variety of technologies that can perform tasks requiring human intelligence.
A subset of AI, machine learning involves algorithms that allow computers to learn from and make decisions based on data. ML systems improve their performance over time without being explicitly programmed to do so.
Deep learning is a type of machine learning that uses neural networks with many layers. It is particularly effective for tasks such as speech recognition, image recognition, and natural language processing.
NLP is a branch of AI that gives machines the ability to read, understand, and derive meaning from human languages. It is widely used in applications like chatbots and translation services.
Neural networks are algorithms modeled loosely after the human brain that are designed to recognize patterns. They interpret sensory data through a kind of machine perception, labeling, or clustering raw input.
In the context of AI, an algorithm is a set of rules or instructions given to an AI program to help it learn on its own. Algorithms are what make it possible for AI to perform tasks without needing step-by-step directions from a human operator.
Data mining is the process of discovering patterns and knowledge from large amounts of data. The process is often used in machine learning to find insights and relationships in data sets.
Robotics is a branch of technology that deals with the design, construction, operation, and use of robots. In AI, robotics is used to develop machines that can perform tasks automatically.
Cognitive computing involves creating AI systems that simulate human thought processes in a computerized model. It involves self-learning systems that use data mining, pattern recognition, and natural language processing to mimic the way the human brain works.
Supervised learning is a type of machine learning where the model is trained on a labeled dataset. The system learns to make predictions based on the input-output pairs provided.
In unsupervised learning, the AI system is given data without explicit instructions on what to do with it. The system tries to identify patterns and relationships in the data on its own.
Reinforcement learning is a type of machine learning where an AI agent learns to make decisions by performing certain actions and receiving rewards or penalties.
This glossary provides a foundational understanding of key AI terms that are crucial for anyone looking to delve deeper into the field of artificial intelligence.
1. Online Courses and Tutorials
For those looking to deepen their understanding of a subject, online courses offer structured learning paths often designed by experts in the field. Platforms like Coursera, Udemy, and Khan Academy provide a wide range of courses covering various topics. These courses often include video lectures, quizzes, and hands-on projects that help consolidate learning.
2. Books and eBooks
Books remain one of the most valuable resources for comprehensive learning. Whether it's a textbook on a technical subject or a non-fiction book covering historical events, books provide in-depth knowledge and insights. For convenience, eBooks offer a digital alternative, accessible on devices like Kindle, which can make learning portable and more accessible.
3. Academic and Research Journals
For those interested in academic or scientific topics, journals are indispensable. They provide the latest research findings and expert analyses in specific fields. Websites like Google Scholar and JSTOR give access to a plethora of peer-reviewed journals. Access might require a subscription, but many institutions offer free access to students and faculty members.
4. Educational Websites and Blogs
Many educational websites and blogs offer free content designed to supplement more formal learning. Sites like TED Talks, National Geographic, and BBC Future provide articles, videos, and interviews with experts that can broaden one’s understanding of a topic. These resources are particularly useful for gaining diverse perspectives and staying updated with the latest developments.
5. Workshops and Webinars
Participating in workshops and webinars can be a highly interactive way to learn. These platforms often offer live sessions with experts, providing the opportunity to ask questions and engage in discussions. Many professional organizations and educational institutions host webinars that are either free or available at a nominal fee.
6. Libraries and Archives
Don’t underestimate the value of your local library or an archive. Libraries not only provide free access to books, magazines, and papers, but many also offer access to online resources and databases. Archives are especially useful for research in history and other fields where original documents and records are necessary for deep dives into specific topics.
7. Learning Communities and Forums
Joining a learning community or a forum can enhance your educational journey. Platforms like Reddit, Stack Exchange, and specialized online forums provide spaces where individuals can share resources, exchange ideas, and solve problems collectively. These communities can be particularly beneficial for complex subjects such as programming, science, or languages.
8. Podcasts and Audiobooks
For auditory learners, podcasts and audiobooks are excellent resources. They make it possible to learn about new topics or catch up on developments in various fields while commuting, exercising, or during other activities. Platforms like Audible for audiobooks and Spotify for podcasts host a vast array of content in numerous genres and subjects.
9. Educational Apps
Mobile apps can provide interactive and portable learning experiences. Language learning apps like Duolingo, or coding apps like Grasshopper, use gamification to teach skills in an engaging way. Many apps are designed to allow learning in short, manageable segments, ideal for busy schedules.
10. Social Media Groups
Social media platforms like Facebook, LinkedIn, and even Instagram offer groups and pages dedicated to education and learning. These can be great places to find information, ask questions, and connect with others who have similar learning interests. They often share valuable resources, advice, and encouragement.
Each of these resources offers unique benefits and can be used in combination to create a comprehensive and engaging learning experience. Whether you prefer self-paced learning through books and apps or interactive sessions via webinars and workshops, there’s a wealth of resources available to support your educational goals.
For more insights and services related to Artificial Intelligence, visit our AI Services Page or explore our Main Page for a full range of offerings.