Table Of Contents
Category
1. Introduction
The rapid advancement of technology has brought about significant changes in various sectors, from healthcare to manufacturing, and from entertainment to finance. Among the most transformative technologies are Generative AI and Digital Twins. These technologies are not only revolutionizing how we interact with digital systems but are also paving the way for new innovations and efficiencies. This document aims to provide a comprehensive overview of these two groundbreaking technologies, exploring their definitions, functionalities, and potential applications.
1.1. Overview of Generative AI
Generative AI refers to a subset of artificial intelligence that focuses on creating new content, whether it be text, images, audio, or even complex data structures. Unlike traditional AI, which is primarily concerned with analyzing and interpreting existing data, Generative AI is designed to produce new data that is often indistinguishable from human-created content. This capability is achieved through advanced algorithms and models, such as Generative Adversarial Networks (GANs) and Variational Autoencoders (VAEs).
Generative AI has a wide range of applications. In the creative industries, it is used to generate art, music, and even literature. For instance, AI-generated art has been sold at prestigious auction houses, fetching prices comparable to works by human artists. In the field of natural language processing, models like OpenAI's GPT-3 can generate coherent and contextually relevant text, making them useful for tasks such as content creation, customer service, and even coding.
In healthcare, Generative AI is being used to create synthetic medical data, which can be invaluable for training machine learning models without compromising patient privacy. It is also being explored for drug discovery, where it can generate potential molecular structures for new medications. In the realm of cybersecurity, Generative AI can be used to simulate cyber-attacks, helping organizations to better prepare and defend against real-world threats.
The technology is not without its challenges. Ethical considerations, such as the potential for misuse in creating deepfakes or generating misleading information, are significant concerns. Additionally, the computational resources required for training generative models are substantial, often necessitating specialized hardware and significant energy consumption.
1.2. Overview of Digital Twins
Digital Twins are virtual replicas of physical entities, systems, or processes. These digital counterparts are designed to mirror their real-world counterparts in real-time, providing a dynamic and interactive model that can be used for analysis, simulation, and optimization. The concept of Digital Twins has been around for several decades, but it has gained significant traction in recent years due to advancements in IoT (Internet of Things), data analytics, and cloud computing.
The primary function of a Digital Twin is to provide a comprehensive and real-time view of a physical asset or system. This is achieved by integrating data from various sensors and sources, which is then processed and visualized in a digital format. For example, in manufacturing, a Digital Twin of a production line can provide real-time insights into machine performance, identify potential bottlenecks, and predict maintenance needs. This can lead to increased efficiency, reduced downtime, and significant cost savings.
In the realm of urban planning, Digital Twins are being used to create virtual models of entire cities. These models can simulate various scenarios, such as traffic flow, energy consumption, and emergency response, allowing planners to make data-driven decisions that improve the quality of life for residents. In healthcare, Digital Twins of patients can be used to simulate treatment plans, predict outcomes, and personalize medical care.
The benefits of Digital Twins are numerous, but there are also challenges to consider. The creation and maintenance of a Digital Twin require significant data collection and processing capabilities. Ensuring the accuracy and reliability of the data is crucial, as any discrepancies between the digital and physical entities can lead to incorrect conclusions and decisions. Additionally, issues related to data security and privacy must be addressed, particularly when dealing with sensitive information.
represent significant advancements in the field of technology. While they serve different purposes, their potential to transform industries and improve efficiencies is immense. As these technologies continue to evolve, they will undoubtedly play a crucial role in shaping the future of various sectors.
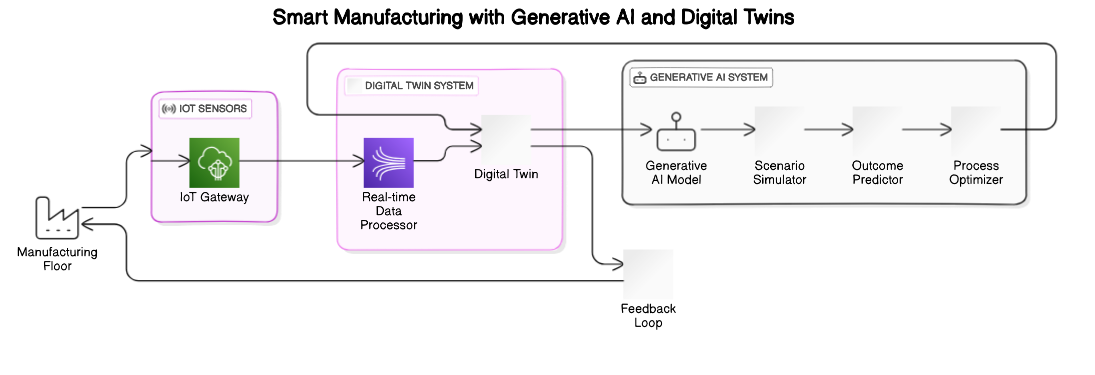
1.3. Importance of Predictive Analytics
Predictive analytics is a branch of advanced analytics that uses historical data, statistical algorithms, and machine learning techniques to identify the likelihood of future outcomes based on historical data. The importance of predictive analytics cannot be overstated in today's data-driven world. It provides businesses with actionable insights that can lead to more informed decision-making, improved operational efficiency, and a competitive edge in the market.
One of the primary benefits of predictive analytics is its ability to forecast future trends and behaviors. For instance, in the retail industry, predictive analytics can help businesses anticipate customer demand, optimize inventory levels, and personalize marketing campaigns. By analyzing past purchasing patterns and customer behavior, retailers can predict which products are likely to be popular in the future and adjust their strategies accordingly. This not only helps in reducing stockouts and overstock situations but also enhances customer satisfaction by ensuring that the right products are available at the right time.
In the healthcare sector, predictive analytics plays a crucial role in improving patient outcomes and reducing costs. By analyzing patient data, healthcare providers can identify individuals at high risk of developing chronic conditions, such as diabetes or heart disease, and intervene early with preventive measures. Predictive models can also help in optimizing hospital resource allocation, such as predicting patient admission rates and ensuring that adequate staff and equipment are available to meet patient needs.
Financial institutions also benefit significantly from predictive analytics. By analyzing transaction data, banks and credit card companies can detect fraudulent activities in real-time, reducing the risk of financial losses. Additionally, predictive models can help in assessing credit risk, enabling lenders to make more informed decisions about loan approvals and interest rates. This not only minimizes the risk of defaults but also ensures that credit is extended to individuals and businesses that are most likely to repay.
Predictive analytics is also transforming the manufacturing industry by enabling predictive maintenance. By analyzing data from sensors and machinery, manufacturers can predict when equipment is likely to fail and schedule maintenance before a breakdown occurs. This not only reduces downtime and maintenance costs but also extends the lifespan of machinery and improves overall productivity.
Moreover, predictive analytics is essential for enhancing customer experience across various industries. By analyzing customer data, businesses can gain insights into customer preferences, behaviors, and pain points. This enables them to tailor their products, services, and interactions to meet customer needs more effectively. For example, in the telecommunications industry, predictive analytics can help in identifying customers who are likely to churn and implementing retention strategies to keep them engaged.
In conclusion, the importance of predictive analytics lies in its ability to transform data into valuable insights that drive better decision-making, optimize operations, and enhance customer experience. As businesses continue to generate vast amounts of data, the role of predictive analytics will only become more critical in helping them stay competitive and achieve their strategic goals.
1.4. Purpose of the Blog
The purpose of this blog is to provide readers with a comprehensive understanding of predictive analytics, its significance, and how it can be effectively integrated into various business processes. In today's data-driven world, organizations across different industries are increasingly recognizing the value of leveraging data to gain insights and make informed decisions. However, the concept of predictive analytics can be complex and overwhelming for those who are not familiar with it. This blog aims to demystify predictive analytics by breaking down its key components, explaining its benefits, and providing practical examples of its applications.
One of the primary objectives of this blog is to educate readers about the fundamental principles of predictive analytics. This includes an overview of the different techniques and algorithms used in predictive modeling, such as regression analysis, decision trees, and machine learning. By understanding these concepts, readers will gain a better appreciation of how predictive analytics works and how it can be applied to solve real-world problems.
Another important purpose of this blog is to highlight the various use cases of predictive analytics across different industries. By showcasing real-life examples, readers will be able to see how predictive analytics is being used to drive business value in areas such as marketing, finance, healthcare, and manufacturing. This will not only help readers understand the practical applications of predictive analytics but also inspire them to explore how it can be implemented in their own organizations.
Additionally, this blog aims to provide readers with insights into the challenges and best practices associated with implementing predictive analytics. While the benefits of predictive analytics are significant, there are also several challenges that organizations may face, such as data quality issues, lack of skilled personnel, and integration with existing systems. By addressing these challenges and offering practical tips and strategies, this blog will help readers navigate the complexities of predictive analytics and maximize its potential.
Furthermore, this blog seeks to keep readers informed about the latest trends and advancements in the field of predictive analytics. As technology continues to evolve, new tools and techniques are being developed that can enhance the accuracy and efficiency of predictive models. By staying up-to-date with these developments, readers will be better equipped to leverage the latest innovations and stay ahead of the competition.
In summary, the purpose of this blog is to provide readers with a thorough understanding of predictive analytics, its applications, and best practices for implementation. By offering valuable insights and practical examples, this blog aims to empower readers to harness the power of predictive analytics to drive business success and achieve their strategic objectives.
2. How Does Integration Work?
Integration in the context of predictive analytics refers to the process of incorporating predictive models and insights into existing business processes and systems. This is a critical step in ensuring that the insights generated from predictive analytics are actionable and can drive meaningful business outcomes. The integration process involves several key steps, including data integration, model deployment, and operationalization.
The first step in the integration process is data integration. Predictive analytics relies on large volumes of data from various sources, such as transactional databases, customer relationship management (CRM) systems, and external data providers. To build accurate predictive models, it is essential to integrate and consolidate this data into a single, unified dataset. This often involves data cleaning, transformation, and normalization to ensure that the data is of high quality and suitable for analysis. Data integration tools and platforms, such as ETL (Extract, Transform, Load) tools, can help automate this process and streamline data preparation.
Once the data is integrated, the next step is model deployment. This involves taking the predictive models developed during the analytics phase and deploying them into the production environment where they can be used to generate predictions in real-time. Model deployment can be done using various methods, such as embedding the models into existing applications, using APIs (Application Programming Interfaces) to integrate with other systems, or deploying the models on cloud-based platforms. The choice of deployment method depends on the specific requirements and infrastructure of the organization.
After the models are deployed, the final step is operationalization. This involves integrating the predictive insights into business processes and decision-making workflows. For example, in a retail setting, predictive models can be integrated into inventory management systems to optimize stock levels based on predicted demand. In a marketing context, predictive insights can be used to personalize customer interactions and target marketing campaigns more effectively. Operationalization also involves setting up monitoring and feedback mechanisms to track the performance of the predictive models and make necessary adjustments over time.
One of the key challenges in the integration process is ensuring that the predictive models are scalable and can handle large volumes of data and high transaction rates. This requires robust infrastructure and efficient algorithms that can process data quickly and generate predictions in real-time. Additionally, it is important to ensure that the predictive models are interpretable and transparent so that business users can understand and trust the insights generated.
Another important aspect of integration is collaboration between different teams within the organization. Successful integration of predictive analytics requires close collaboration between data scientists, IT professionals, and business stakeholders. Data scientists are responsible for developing and validating the predictive models, while IT professionals handle the technical aspects of data integration and model deployment. Business stakeholders, on the other hand, provide domain expertise and ensure that the predictive insights are aligned with business objectives and can be effectively used in decision-making.
.

2.1. Understanding Generative AI
Generative AI refers to a subset of artificial intelligence that focuses on creating new content, data, or solutions rather than merely analyzing or acting upon existing information. This type of AI leverages advanced algorithms, particularly those based on deep learning and neural networks, to generate outputs that can range from text and images to music and even complex designs. One of the most well-known examples of generative AI is the Generative Adversarial Network (GAN), which consists of two neural networks: a generator and a discriminator. The generator creates new data instances, while the discriminator evaluates them for authenticity, leading to the creation of highly realistic outputs.
Generative AI has a wide array of applications across various industries. In the creative arts, it can be used to generate new pieces of music, art, or literature, providing artists with novel ideas and inspiration. In the field of design and manufacturing, generative AI can optimize product designs by exploring a vast space of potential configurations and selecting the most efficient or innovative ones. For instance, companies like Autodesk use generative design to create lightweight yet strong components for aerospace and automotive industries.
In healthcare, generative AI can assist in drug discovery by generating potential molecular structures that could serve as new medications. This accelerates the research process and reduces costs. Additionally, generative AI can be used to create synthetic medical data, which can be invaluable for training machine learning models without compromising patient privacy.
The technology also has significant implications for natural language processing (NLP). Models like OpenAI's GPT-3 can generate human-like text, enabling applications such as chatbots, automated content creation, and even coding assistance. These models are trained on vast datasets and can understand and generate text in a way that is contextually relevant and coherent.
However, the power of generative AI also comes with challenges and ethical considerations. The ability to create highly realistic fake content, such as deepfakes, poses risks for misinformation and privacy. Ensuring the responsible use of generative AI involves implementing robust ethical guidelines and developing technologies to detect and mitigate malicious uses.
In summary, generative AI represents a transformative technology with the potential to revolutionize various sectors by creating new content and solutions. Its applications are vast, ranging from creative arts and design to healthcare and NLP. However, the ethical implications and potential risks associated with generative AI necessitate careful consideration and responsible implementation.
2.2. Understanding Digital Twins
Digital twins are virtual replicas of physical entities, systems, or processes that are used to simulate, analyze, and optimize their real-world counterparts. This concept leverages advanced technologies such as the Internet of Things (IoT), artificial intelligence, and data analytics to create a dynamic, real-time digital representation of a physical object or system. The digital twin continuously receives data from sensors and other sources, allowing it to mirror the state and behavior of its physical counterpart accurately.
The origins of digital twins can be traced back to the aerospace industry, where they were initially used to create virtual models of aircraft and spacecraft for testing and maintenance purposes. Today, the application of digital twins has expanded across various industries, including manufacturing, healthcare, urban planning, and energy management.
In manufacturing, digital twins are used to optimize production processes, improve product quality, and reduce downtime. By creating a digital replica of a production line, manufacturers can simulate different scenarios, identify potential bottlenecks, and implement improvements without disrupting actual operations. This leads to increased efficiency and cost savings.
In healthcare, digital twins can be used to create personalized models of patients, enabling more accurate diagnoses and tailored treatment plans. For example, a digital twin of a patient's heart can be used to simulate different surgical procedures and predict their outcomes, helping doctors make more informed decisions.
Urban planners and city managers use digital twins to design and manage smart cities. By creating a digital replica of a city, they can simulate traffic patterns, energy consumption, and environmental impacts, allowing for more efficient and sustainable urban development. Digital twins also play a crucial role in disaster management by simulating the effects of natural disasters and planning effective response strategies.
In the energy sector, digital twins are used to monitor and optimize the performance of power plants, wind turbines, and other energy assets. By analyzing real-time data from these assets, operators can predict maintenance needs, optimize energy production, and reduce operational costs.
The implementation of digital twins involves several key components: data collection, data integration, modeling, and simulation. Sensors and IoT devices collect data from the physical entity, which is then integrated into the digital twin model. Advanced algorithms and machine learning techniques are used to analyze the data and simulate the behavior of the physical entity. The insights gained from these simulations can be used to make informed decisions and optimize performance.
Despite their numerous benefits, digital twins also present challenges, particularly in terms of data security and privacy. The continuous flow of data between the physical entity and its digital twin creates potential vulnerabilities that need to be addressed through robust cybersecurity measures.
In conclusion, digital twins represent a powerful tool for simulating, analyzing, and optimizing physical entities and systems. Their applications span various industries, offering significant benefits in terms of efficiency, cost savings, and improved decision-making. However, the successful implementation of digital twins requires careful consideration of data security and privacy concerns.
2.3. The Integration Process
The integration process of generative AI and digital twins involves combining the capabilities of both technologies to create more advanced and intelligent systems. This integration can lead to significant improvements in various applications, from manufacturing and healthcare to urban planning and energy management.
The first step in the integration process is data collection and management. Both generative AI and digital twins rely on large amounts of data to function effectively. Sensors and IoT devices collect real-time data from physical entities, which is then fed into the digital twin model. This data serves as the foundation for the generative AI algorithms, enabling them to create new content, solutions, or optimizations based on the current state and behavior of the physical entity.
Next, the data needs to be integrated and processed. This involves cleaning, organizing, and structuring the data to ensure it is suitable for analysis and simulation. Advanced data integration platforms and tools can help streamline this process, ensuring that the data is accurate, consistent, and up-to-date.
Once the data is prepared, the digital twin model is created. This involves developing a virtual replica of the physical entity, system, or process using advanced modeling techniques. The digital twin continuously receives data from the physical entity, allowing it to mirror its state and behavior in real-time. This dynamic representation enables more accurate simulations and analyses.
With the digital twin in place, generative AI algorithms can be applied to the model. These algorithms use the data from the digital twin to generate new content, solutions, or optimizations. For example, in a manufacturing setting, generative AI can analyze the digital twin of a production line to identify potential improvements, such as optimizing the layout or adjusting process parameters to increase efficiency and reduce waste.
The integration of generative AI and digital twins also involves continuous monitoring and feedback. As the physical entity operates, the digital twin and generative AI algorithms continuously receive and analyze new data. This enables real-time adjustments and optimizations, ensuring that the system remains efficient and effective.
One of the key benefits of integrating generative AI and digital twins is the ability to create more intelligent and adaptive systems. By combining the predictive and generative capabilities of AI with the real-time simulation and analysis provided by digital twins, organizations can develop systems that can autonomously adapt to changing conditions and optimize performance.
However, the integration process also presents challenges. Ensuring data security and privacy is crucial, as the continuous flow of data between the physical entity, digital twin, and generative AI algorithms creates potential vulnerabilities. Robust cybersecurity measures and data governance policies are essential to protect sensitive information and maintain the integrity of the system.
In conclusion, the integration of generative AI and digital twins offers significant potential for creating more advanced and intelligent systems across various industries. The process involves data collection and management, data integration and processing, digital twin modeling, and the application of generative AI algorithms. Continuous monitoring and feedback ensure real-time adjustments and optimizations, leading to more efficient and adaptive systems. However, addressing data security and privacy concerns is crucial for the successful implementation of these integrated technologies.
.

2.4 Tools and Technologies Involved
The realm of User Proxy involves a myriad of tools and technologies that facilitate the seamless interaction between users and systems. These tools and technologies are designed to enhance user experience, ensure security, and provide efficient data management. One of the primary tools in this domain is the proxy server itself. A proxy server acts as an intermediary between the user's device and the internet, allowing for various functionalities such as anonymity, security, and content filtering. Proxy servers can be categorized into different types, including forward proxies, reverse proxies, and transparent proxies, each serving distinct purposes.
Forward proxies are commonly used to provide anonymity and security for users by masking their IP addresses and routing their requests through the proxy server. This helps in protecting user privacy and preventing unauthorized access to sensitive information. Reverse proxies, on the other hand, are used to manage and distribute incoming traffic to multiple servers, ensuring load balancing and improving the performance of web applications. Transparent proxies, as the name suggests, operate without the user's knowledge and are often used by organizations to monitor and control internet usage.
Another crucial technology in the User Proxy domain is Virtual Private Network (VPN) software. VPNs create a secure and encrypted connection between the user's device and the internet, ensuring that data transmitted over the network is protected from eavesdropping and cyber threats. VPNs are widely used by individuals and organizations to safeguard their online activities and access restricted content.
In addition to proxy servers and VPNs, firewalls play a significant role in the User Proxy ecosystem. Firewalls are network security devices that monitor and control incoming and outgoing network traffic based on predetermined security rules. They act as a barrier between trusted internal networks and untrusted external networks, preventing unauthorized access and protecting against cyberattacks.
Content Delivery Networks (CDNs) are another essential technology in this space. CDNs are distributed networks of servers that deliver web content to users based on their geographic location. By caching content on multiple servers worldwide, CDNs reduce latency and improve the speed and reliability of content delivery. This is particularly important for websites and applications with a global user base.
Moreover, encryption technologies such as Secure Sockets Layer (SSL) and Transport Layer Security (TLS) are vital for ensuring secure communication between users and servers. SSL and TLS protocols encrypt data transmitted over the internet, preventing unauthorized access and ensuring data integrity.
Lastly, monitoring and analytics tools are indispensable for managing and optimizing User Proxy systems. These tools provide insights into user behavior, network performance, and security threats, enabling administrators to make informed decisions and take proactive measures to enhance the overall user experience.
In conclusion, the tools and technologies involved in the User Proxy domain are diverse and multifaceted, encompassing proxy servers, VPNs, firewalls, CDNs, encryption protocols, and monitoring tools. Each of these components plays a crucial role in ensuring secure, efficient, and user-friendly interactions between users and systems.
3. What is Generative AI?
Generative AI refers to a subset of artificial intelligence that focuses on creating new content, data, or solutions by learning patterns from existing data. Unlike traditional AI, which primarily focuses on analyzing and interpreting data, generative AI aims to generate new and original outputs that mimic the characteristics of the input data. This technology leverages advanced machine learning techniques, particularly deep learning, to achieve its goals.
One of the most well-known applications of generative AI is in the field of natural language processing (NLP). Generative AI models, such as OpenAI's GPT-3, are capable of generating human-like text based on a given prompt. These models are trained on vast amounts of text data and can produce coherent and contextually relevant responses, making them useful for tasks such as content creation, chatbots, and language translation.
Generative AI is also widely used in the field of computer vision. Generative Adversarial Networks (GANs) are a popular technique in this domain. GANs consist of two neural networks, a generator and a discriminator, that work together to create realistic images. The generator creates new images, while the discriminator evaluates their authenticity. Through this adversarial process, GANs can generate highly realistic images that are indistinguishable from real ones. This technology has applications in areas such as image synthesis, video generation, and even art creation.
Another significant application of generative AI is in the field of music and audio generation. AI models can be trained on large datasets of music to generate new compositions that mimic the style and structure of the input data. This has led to the development of AI-powered music composition tools that assist musicians and composers in creating new pieces of music.
Generative AI also has applications in the field of healthcare. For instance, it can be used to generate synthetic medical data for research and training purposes. This is particularly useful in scenarios where real medical data is scarce or difficult to obtain. Additionally, generative AI can assist in drug discovery by generating new molecular structures with desired properties, potentially accelerating the development of new medications.
In summary, generative AI is a powerful and versatile technology that has the potential to revolutionize various industries by creating new and original content, data, and solutions. Its applications span across natural language processing, computer vision, music and audio generation, and healthcare, among others. By leveraging advanced machine learning techniques, generative AI is pushing the boundaries of what is possible with artificial intelligence.
3.1 Definition and Explanation
Generative AI, at its core, is a branch of artificial intelligence that focuses on generating new data or content that is similar to the input data it has been trained on. This is achieved through the use of advanced machine learning algorithms, particularly deep learning models. The primary goal of generative AI is to create outputs that are not only novel but also coherent and contextually relevant.
One of the fundamental techniques used in generative AI is the Generative Adversarial Network (GAN). GANs consist of two neural networks, a generator and a discriminator, that work in tandem to produce realistic outputs. The generator creates new data samples, while the discriminator evaluates their authenticity. The two networks are trained simultaneously in a process known as adversarial training, where the generator aims to produce data that can fool the discriminator, and the discriminator aims to accurately distinguish between real and generated data. This adversarial process continues until the generator produces data that is indistinguishable from real data.
Another important technique in generative AI is the Variational Autoencoder (VAE). VAEs are a type of neural network that learns to encode input data into a lower-dimensional latent space and then decode it back into the original data space. By sampling from the latent space, VAEs can generate new data samples that are similar to the input data. VAEs are particularly useful for generating continuous data, such as images and audio.
Generative AI models are typically trained on large datasets to learn the underlying patterns and structures of the input data. For example, a generative AI model trained on a dataset of images can learn to generate new images that resemble the training data. Similarly, a model trained on a dataset of text can generate new text that mimics the style and content of the input data.
One of the key advantages of generative AI is its ability to create new and original content without explicit programming. This makes it a valuable tool for creative industries, such as art, music, and literature. For instance, AI-generated art has gained significant attention in recent years, with some pieces even being sold at prestigious art auctions.
Generative AI also has practical applications in various fields. In healthcare, it can be used to generate synthetic medical data for research and training purposes. In the field of drug discovery, generative AI can assist in designing new molecular structures with desired properties. In the gaming industry, generative AI can be used to create realistic and immersive virtual environments.
.

3.2. Key Features
Digital twins are virtual replicas of physical entities, systems, or processes that are used to simulate, predict, and optimize performance in real-time. One of the key features of digital twins is their ability to integrate data from various sources, including sensors, IoT devices, and historical records. This integration allows for a comprehensive and dynamic representation of the physical counterpart, enabling real-time monitoring and analysis. The data collected is continuously updated, ensuring that the digital twin remains an accurate reflection of the current state of the physical entity.
Another significant feature is the predictive analytics capability. Digital twins leverage advanced algorithms and machine learning models to predict future states and behaviors. This predictive power is crucial for proactive maintenance, risk management, and decision-making. For instance, in manufacturing, digital twins can predict equipment failures before they occur, allowing for timely interventions and minimizing downtime.
Interoperability is also a key feature of digital twins. They are designed to work seamlessly with various software and hardware systems, ensuring that they can be integrated into existing workflows and processes. This interoperability extends to different platforms and devices, making digital twins versatile tools that can be used across multiple industries and applications.
Scalability is another important feature. Digital twins can be scaled up or down depending on the complexity and size of the physical entity they represent. This scalability ensures that digital twins can be used for both small-scale applications, such as individual machines, and large-scale systems, such as entire factories or cities.
Visualization is a critical aspect of digital twins. They provide intuitive and interactive visual representations of the physical entity, making it easier for users to understand and analyze data. Advanced visualization tools, such as 3D models and augmented reality, enhance the user experience and provide deeper insights into the system's performance.
Lastly, digital twins offer enhanced collaboration capabilities. They enable multiple stakeholders to access and interact with the same virtual model, facilitating better communication and coordination. This collaborative environment is particularly beneficial in complex projects where different teams need to work together to achieve common goals.
3.3. Applications in Various Industries
Digital twins have found applications in a wide range of industries, each leveraging the technology to address specific challenges and improve efficiency. In the manufacturing sector, digital twins are used to optimize production processes, monitor equipment health, and enhance product quality. By creating virtual replicas of production lines, manufacturers can simulate different scenarios, identify bottlenecks, and implement improvements without disrupting actual operations. This leads to increased productivity, reduced downtime, and cost savings.
In the healthcare industry, digital twins are revolutionizing patient care and medical research. They are used to create personalized models of patients, allowing doctors to simulate treatments and predict outcomes. This personalized approach enhances the accuracy of diagnoses and treatment plans, leading to better patient outcomes. Additionally, digital twins are used in the development of medical devices, enabling manufacturers to test and refine designs before physical prototypes are created.
The automotive industry is another sector where digital twins are making a significant impact. They are used to design and test vehicles, optimize manufacturing processes, and monitor the performance of individual components. For example, digital twins of engines can simulate different operating conditions, helping engineers to identify potential issues and improve efficiency. In the realm of autonomous vehicles, digital twins are used to simulate real-world driving scenarios, enhancing the safety and reliability of self-driving cars.
In the energy sector, digital twins are used to optimize the performance of power plants, wind turbines, and other energy assets. They enable operators to monitor equipment in real-time, predict maintenance needs, and optimize energy production. This leads to increased efficiency, reduced operational costs, and improved sustainability. For instance, digital twins of wind turbines can simulate different wind conditions, helping operators to maximize energy output and minimize wear and tear.
The construction industry is also benefiting from digital twins. They are used to create virtual models of buildings and infrastructure projects, enabling architects, engineers, and contractors to collaborate more effectively. Digital twins provide a comprehensive view of the project, allowing stakeholders to identify potential issues, optimize designs, and streamline construction processes. This results in faster project completion, reduced costs, and improved quality.
In the aerospace industry, digital twins are used to design and test aircraft, monitor the health of critical components, and optimize maintenance schedules. By simulating different flight conditions, engineers can identify potential issues and improve the performance and safety of aircraft. Digital twins also enable airlines to monitor the health of their fleets in real-time, predict maintenance needs, and minimize downtime.
4. What are Digital Twins?
Digital twins are virtual representations of physical entities, systems, or processes that are used to simulate, predict, and optimize performance in real-time. The concept of digital twins originated from the field of product lifecycle management (PLM) and has since evolved to encompass a wide range of applications across various industries. At its core, a digital twin is a dynamic, data-driven model that mirrors the physical counterpart, providing a comprehensive and real-time view of its state and behavior.
The creation of a digital twin involves several key steps. First, data is collected from the physical entity using sensors, IoT devices, and other data sources. This data includes information about the entity's current state, historical performance, and environmental conditions. Next, this data is integrated and processed to create a virtual model that accurately represents the physical entity. Advanced algorithms and machine learning models are then used to analyze the data, predict future states, and optimize performance.
One of the primary benefits of digital twins is their ability to provide real-time monitoring and analysis. By continuously updating the virtual model with real-time data, digital twins enable operators to monitor the performance of the physical entity, identify potential issues, and make informed decisions. This real-time visibility is particularly valuable in industries where downtime and failures can have significant consequences, such as manufacturing, energy, and healthcare.
Another key advantage of digital twins is their predictive capabilities. By leveraging advanced analytics and machine learning, digital twins can predict future states and behaviors, allowing operators to take proactive measures. For example, in the manufacturing industry, digital twins can predict equipment failures before they occur, enabling timely maintenance and reducing downtime. In the healthcare industry, digital twins can simulate the effects of different treatments on a patient, helping doctors to develop personalized treatment plans.
Digital twins also facilitate better collaboration and communication among stakeholders. By providing a shared virtual model, digital twins enable different teams to work together more effectively, share insights, and make coordinated decisions. This collaborative environment is particularly beneficial in complex projects where multiple stakeholders need to align their efforts to achieve common goals.
4.1. Definition and Explanation
A user proxy, often referred to simply as a proxy, is an intermediary server that separates end users from the websites they browse. Proxies provide varying levels of functionality, security, and privacy depending on your needs, company policies, or privacy concerns. When a user connects to a proxy server, the server makes requests to websites, servers, and services on behalf of the user. The proxy then returns the requested information to the user, effectively masking the user's IP address and other identifying information.
The primary purpose of a user proxy is to act as a gateway between the user and the internet. This can be beneficial for several reasons, including enhancing security, improving privacy, and enabling access to restricted content. For instance, in a corporate environment, proxies can be used to monitor and control employee internet usage, ensuring that company policies are adhered to. In a personal context, proxies can help users access content that may be restricted in their geographical location, such as streaming services or news websites.
Proxies can be categorized into several types, including forward proxies, reverse proxies, transparent proxies, and anonymous proxies. A forward proxy is the most common type and is used to retrieve data from a wide range of sources on behalf of the user. A reverse proxy, on the other hand, is used to retrieve resources on behalf of a server, often to distribute the load and improve performance. Transparent proxies do not modify the request or response beyond what is required for proxy authentication and identification, while anonymous proxies hide the user's IP address but may still reveal that a proxy is being used.
4.2. Key Features
User proxies come with a variety of features that make them indispensable tools for both individuals and organizations. One of the most significant features is anonymity. By masking the user's IP address, proxies can help protect the user's identity and location, making it more difficult for websites and online services to track their activities. This is particularly useful for users who are concerned about privacy and want to avoid targeted advertising or surveillance.
Another key feature is content filtering. Proxies can be configured to block access to specific websites or types of content, making them useful for parental controls or corporate environments where internet usage needs to be regulated. This can help prevent access to inappropriate or harmful content, ensuring a safer online experience.
Proxies also offer caching capabilities, which can significantly improve browsing speed and reduce bandwidth usage. By storing copies of frequently accessed web pages, proxies can serve these pages to users more quickly than if they had to be retrieved from the original server each time. This can be particularly beneficial in environments with limited bandwidth or high traffic volumes.
Security is another crucial feature of user proxies. They can act as a barrier between the user and potential threats, such as malware or phishing attacks. By filtering out malicious content and blocking access to known harmful websites, proxies can help protect users from online threats. Additionally, proxies can be used to enforce security policies, such as requiring authentication before allowing access to certain resources.
Finally, user proxies can provide access to geo-restricted content. By routing traffic through servers located in different regions, proxies can make it appear as though the user is accessing the internet from a different location. This can be useful for bypassing regional restrictions on content, such as streaming services or news websites that are only available in certain countries.
4.3. Applications in Various Industries
User proxies have a wide range of applications across various industries, each leveraging the unique features of proxies to meet specific needs and challenges. In the corporate world, proxies are often used to enhance security and control internet usage. By routing all internet traffic through a proxy server, companies can monitor and regulate employee internet activity, ensuring compliance with company policies and preventing access to inappropriate or non-work-related content. This can help improve productivity and protect the company from potential security threats.
In the education sector, proxies are commonly used to provide a safe and controlled online environment for students. Schools and universities can use proxies to block access to harmful or distracting content, ensuring that students can focus on their studies. Additionally, proxies can help protect students' privacy by masking their IP addresses and preventing tracking by third-party websites.
The healthcare industry also benefits from the use of proxies. With the increasing digitization of medical records and the growing use of telemedicine, protecting patient data has become a top priority. Proxies can help secure sensitive information by acting as a barrier between healthcare providers and potential cyber threats. They can also be used to control access to online resources, ensuring that only authorized personnel can access patient data.
In the world of e-commerce, proxies are used to gather competitive intelligence and monitor market trends. By using proxies to access competitors' websites, businesses can collect data on pricing, product availability, and customer reviews without revealing their identity. This information can be used to make informed business decisions and stay ahead of the competition.
Finally, in the realm of digital marketing, proxies are used to manage multiple social media accounts and conduct web scraping. By routing traffic through different IP addresses, marketers can avoid detection and prevent their accounts from being flagged or banned. This allows them to gather valuable data and engage with a broader audience more effectively.
5. Types of Integrations
In the realm of modern technology, integration plays a pivotal role in ensuring that various systems and technologies work seamlessly together. Integration can be defined as the process of combining different subsystems or components into a single, unified system that functions cohesively. This is particularly important in the context of digital transformation, where businesses and organizations are increasingly relying on a multitude of technologies to drive efficiency, innovation, and competitiveness. Among the various types of integrations, two emerging and highly impactful areas are Generative AI-Driven Digital Twins and Digital Twin-Enhanced Generative AI. These integrations are revolutionizing industries by providing advanced capabilities for simulation, prediction, and optimization.
5.1. Generative AI-Driven Digital Twins
Generative AI-Driven Digital Twins represent a cutting-edge integration where generative artificial intelligence (AI) is used to create and enhance digital twins. A digital twin is a virtual replica of a physical object, system, or process that is used to simulate, analyze, and optimize its real-world counterpart. By integrating generative AI with digital twins, organizations can achieve unprecedented levels of accuracy and efficiency in their operations.
Generative AI refers to a subset of artificial intelligence that focuses on creating new content, such as images, text, or even entire virtual environments, based on existing data. When applied to digital twins, generative AI can be used to generate highly detailed and realistic models of physical assets. These models can then be used to simulate various scenarios, predict outcomes, and optimize performance.
One of the key benefits of generative AI-driven digital twins is their ability to continuously learn and adapt. As new data is collected from the physical asset, the digital twin can be updated in real-time, ensuring that it remains an accurate representation of the real-world object. This continuous learning capability allows organizations to make more informed decisions, reduce downtime, and improve overall efficiency.
For example, in the manufacturing industry, generative AI-driven digital twins can be used to optimize production processes. By simulating different production scenarios, manufacturers can identify potential bottlenecks, predict equipment failures, and optimize resource allocation. This not only improves productivity but also reduces costs and enhances product quality. Revolutionizing Industries with AI-Driven Digital Twins
In the healthcare sector, generative AI-driven digital twins can be used to create personalized treatment plans for patients. By simulating the effects of different treatments on a virtual model of the patient's body, healthcare providers can identify the most effective treatment options and minimize potential side effects. This personalized approach to healthcare can lead to better patient outcomes and more efficient use of medical resources.
5.2. Digital Twin-Enhanced Generative AI
Digital Twin-Enhanced Generative AI represents the reverse integration, where digital twins are used to enhance the capabilities of generative AI. In this integration, digital twins provide a rich source of data and context that can be used to train and improve generative AI models.
Generative AI models rely on large amounts of data to learn and generate new content. Digital twins, with their detailed and accurate representations of physical assets, provide an ideal source of data for training these models. By incorporating data from digital twins, generative AI models can achieve higher levels of accuracy and realism in their outputs.
One of the key applications of digital twin-enhanced generative AI is in the field of design and engineering. For example, in the automotive industry, digital twins of vehicles can be used to train generative AI models to create new car designs. By analyzing the data from digital twins, generative AI can identify design patterns, optimize aerodynamics, and improve overall vehicle performance. This integration allows automotive manufacturers to accelerate the design process, reduce development costs, and create more innovative and efficient vehicles.
In the field of urban planning, digital twin-enhanced generative AI can be used to create realistic simulations of city environments. By incorporating data from digital twins of buildings, infrastructure, and transportation systems, generative AI can generate detailed and accurate models of urban areas. These models can be used to simulate the impact of different urban planning decisions, such as the construction of new buildings or the implementation of new transportation routes. This allows urban planners to make more informed decisions and create more sustainable and efficient cities.
5.3. Hybrid Models
Hybrid models in the context of data science and machine learning refer to the combination of different types of models to leverage the strengths of each and mitigate their weaknesses. These models can integrate various machine learning techniques, such as combining supervised and unsupervised learning, or blending traditional statistical methods with modern deep learning approaches. The primary goal of hybrid models is to improve predictive accuracy, robustness, and generalizability of the results.
One common example of a hybrid model is the ensemble method, which combines multiple learning algorithms to obtain better predictive performance than could be obtained from any of the constituent models alone. Techniques like bagging, boosting, and stacking are popular ensemble methods. Bagging, or Bootstrap Aggregating, involves training multiple versions of a model on different subsets of the data and then averaging their predictions. Boosting, on the other hand, sequentially trains models, each trying to correct the errors of its predecessor. Stacking involves training a meta-model to combine the predictions of several base models.
Another example of hybrid models is the integration of rule-based systems with machine learning models. Rule-based systems are excellent for incorporating domain knowledge and handling specific scenarios with clear rules, while machine learning models excel at identifying patterns in large datasets. By combining these approaches, hybrid models can provide more accurate and interpretable results.
Hybrid models are also used in time series forecasting, where traditional statistical methods like ARIMA (AutoRegressive Integrated Moving Average) are combined with machine learning models like neural networks. This combination can capture both linear and non-linear patterns in the data, leading to more accurate forecasts.
In the realm of natural language processing (NLP), hybrid models can combine rule-based methods for tasks like part-of-speech tagging with machine learning models for tasks like sentiment analysis. This approach can improve the overall performance of NLP systems by leveraging the strengths of both methods.
The development and deployment of hybrid models require careful consideration of the strengths and weaknesses of each component model, as well as the interactions between them. Proper validation and testing are crucial to ensure that the hybrid model performs well on unseen data and does not overfit to the training data.
6. Benefits of Integration
Integration in the context of data science and machine learning refers to the process of combining different data sources, tools, and techniques to create a cohesive and efficient system. The benefits of integration are manifold and can significantly enhance the capabilities and performance of data-driven applications.
One of the primary benefits of integration is the ability to leverage diverse data sources. By integrating data from various sources, organizations can gain a more comprehensive view of their operations, customers, and market trends. This holistic perspective enables better decision-making and more accurate predictions. For example, integrating customer data from CRM systems with social media data can provide deeper insights into customer behavior and preferences.
Integration also facilitates the seamless flow of data across different systems and departments within an organization. This interoperability ensures that data is readily available to all stakeholders, reducing data silos and improving collaboration. For instance, integrating sales data with inventory management systems can help optimize stock levels and reduce costs.
Another significant benefit of integration is the enhancement of data quality. By combining data from multiple sources, organizations can cross-verify and validate the information, leading to more accurate and reliable datasets. This improved data quality is crucial for building robust machine learning models and making informed business decisions.
Integration also enables the automation of data processing and analysis workflows. By connecting various tools and platforms, organizations can streamline their data pipelines, reducing manual intervention and minimizing the risk of errors. This automation not only saves time and resources but also ensures that data is processed consistently and efficiently.
Furthermore, integration can enhance the scalability and flexibility of data systems. By using modular and interoperable components, organizations can easily adapt to changing requirements and scale their systems as needed. This flexibility is particularly important in the rapidly evolving field of data science, where new tools and techniques are constantly emerging.
6.1. Enhanced Predictive Analytics
Enhanced predictive analytics refers to the improvement of predictive models and techniques to provide more accurate and actionable insights. This enhancement can be achieved through various means, including the integration of diverse data sources, the use of advanced machine learning algorithms, and the implementation of hybrid models.
One of the key factors contributing to enhanced predictive analytics is the availability of diverse and high-quality data. By integrating data from multiple sources, organizations can create richer datasets that capture a broader range of variables and patterns. This comprehensive data enables the development of more accurate and robust predictive models. For example, in the healthcare industry, integrating patient data from electronic health records with genomic data and lifestyle information can lead to more precise predictions of disease risk and treatment outcomes.
The use of advanced machine learning algorithms is another crucial aspect of enhanced predictive analytics. Techniques such as deep learning, reinforcement learning, and ensemble methods have shown significant improvements in predictive performance across various domains. Deep learning, for instance, has revolutionized fields like image recognition and natural language processing by enabling the extraction of complex patterns and features from large datasets. Reinforcement learning, on the other hand, has been successfully applied to areas like robotics and game playing, where it learns optimal strategies through trial and error.
Hybrid models, as discussed earlier, also play a vital role in enhancing predictive analytics. By combining different types of models, hybrid approaches can leverage the strengths of each and mitigate their weaknesses, leading to more accurate and reliable predictions. For instance, in financial forecasting, combining traditional econometric models with machine learning techniques can capture both linear and non-linear relationships in the data, resulting in better forecasts.
Enhanced predictive analytics also benefits from the continuous improvement of computational power and data storage capabilities. With the advent of cloud computing and distributed systems, organizations can now process and analyze vast amounts of data in real-time, enabling more timely and actionable insights. This scalability and speed are particularly important in industries like finance and e-commerce, where rapid decision-making is crucial.
In conclusion, enhanced predictive analytics is achieved through the integration of diverse data sources, the use of advanced machine learning algorithms, and the implementation of hybrid models. These improvements lead to more accurate and actionable insights, enabling organizations to make better decisions and achieve their goals more effectively.
6.2 Improved Decision-Making
Improved decision-making is one of the most significant advantages brought about by advancements in technology and data analytics. In today's fast-paced business environment, the ability to make informed decisions quickly can be the difference between success and failure. Data analytics tools and technologies provide businesses with the insights they need to make better decisions. These tools can process vast amounts of data in real-time, allowing decision-makers to identify trends, patterns, and anomalies that would be impossible to detect manually.
For instance, predictive analytics can help businesses forecast future trends based on historical data. This can be particularly useful in industries such as retail, where understanding consumer behavior can lead to more effective marketing strategies and inventory management. By analyzing past sales data, businesses can predict which products are likely to be in high demand and adjust their stock levels accordingly. This not only helps in meeting customer demand but also in reducing excess inventory and associated costs.
Moreover, improved decision-making is not limited to the business sector. In healthcare, data analytics can help in diagnosing diseases more accurately and in developing personalized treatment plans. By analyzing patient data, healthcare providers can identify risk factors and recommend preventive measures, thereby improving patient outcomes. Similarly, in the public sector, data analytics can help in policy-making by providing insights into the effectiveness of various programs and initiatives.
Another critical aspect of improved decision-making is the ability to respond to changes quickly. In a rapidly changing market, businesses need to be agile and adaptable. Data analytics provides the real-time insights needed to make quick decisions. For example, if a company notices a sudden drop in sales, it can quickly analyze the data to identify the cause and take corrective action. This could involve adjusting pricing strategies, launching targeted marketing campaigns, or even re-evaluating product offerings.
Furthermore, improved decision-making also involves risk management. By analyzing data, businesses can identify potential risks and take proactive measures to mitigate them. This can include anything from financial risks, such as credit defaults, to operational risks, such as supply chain disruptions. By having a clear understanding of the risks involved, businesses can develop strategies to manage them effectively.
In conclusion, improved decision-making is a multifaceted benefit of data analytics and technology. It enables businesses and other organizations to make informed decisions quickly, respond to changes in the market, and manage risks effectively. As technology continues to evolve, the ability to make data-driven decisions will become increasingly important, providing a competitive edge to those who can harness its power effectively.
6.3 Cost Efficiency
Cost efficiency is a critical factor for the success of any business. It involves minimizing costs while maximizing output and maintaining quality. Advances in technology and data analytics have significantly contributed to achieving cost efficiency in various ways. One of the primary ways technology aids in cost efficiency is through automation. Automation of repetitive and time-consuming tasks can lead to significant cost savings. For example, in manufacturing, the use of robotics and automated assembly lines can reduce labor costs and increase production speed. Similarly, in the service industry, chatbots and automated customer service systems can handle routine inquiries, freeing up human resources for more complex tasks.
Another way technology contributes to cost efficiency is through improved resource management. Data analytics can provide insights into how resources are being used and identify areas where waste can be reduced. For instance, in the energy sector, smart grids and IoT devices can monitor energy consumption in real-time, allowing for more efficient energy use and reducing costs. In logistics, route optimization algorithms can help in planning the most efficient delivery routes, saving fuel and reducing transportation costs.
Moreover, technology can also help in reducing operational costs through better maintenance practices. Predictive maintenance, powered by data analytics, can predict when equipment is likely to fail and schedule maintenance before a breakdown occurs. This not only reduces downtime but also extends the lifespan of the equipment, leading to cost savings. For example, in the aviation industry, predictive maintenance can help in identifying potential issues with aircraft components, allowing for timely repairs and reducing the risk of costly delays and cancellations.
In addition to operational efficiencies, technology can also help in reducing costs related to inventory management. By using data analytics to forecast demand accurately, businesses can maintain optimal inventory levels, reducing the costs associated with overstocking or stockouts. This is particularly important in industries with perishable goods, such as food and pharmaceuticals, where excess inventory can lead to significant losses.
Furthermore, technology can also contribute to cost efficiency through improved procurement practices. E-procurement systems can streamline the purchasing process, reduce administrative costs, and provide better visibility into spending. By analyzing procurement data, businesses can identify opportunities for cost savings, such as bulk purchasing or negotiating better terms with suppliers.
In conclusion, cost efficiency is a crucial aspect of business success, and technology plays a vital role in achieving it. Through automation, improved resource management, predictive maintenance, optimized inventory management, and better procurement practices, technology can help businesses reduce costs while maintaining or even improving quality and output. As technology continues to advance, the potential for achieving greater cost efficiency will only increase, providing businesses with a competitive edge in the market.
6.4 Real-Time Monitoring and Feedback
Real-time monitoring and feedback are essential components of modern business operations, enabled by advancements in technology and data analytics. These capabilities allow businesses to track performance, identify issues, and make adjustments on the fly, leading to improved efficiency and effectiveness. One of the primary benefits of real-time monitoring is the ability to detect and address issues as they arise. In manufacturing, for example, real-time monitoring systems can track the performance of machinery and equipment, identifying any deviations from normal operating conditions. This allows for immediate corrective action, reducing downtime and preventing costly breakdowns. Similarly, in the IT sector, real-time monitoring of network performance can help in identifying and resolving issues before they impact users, ensuring smooth and uninterrupted service.
Real-time feedback is also crucial in customer service and support. With the help of chatbots and automated systems, businesses can provide instant responses to customer inquiries, improving customer satisfaction and loyalty. Additionally, real-time feedback from customers can help businesses identify areas for improvement and make necessary adjustments to their products or services. For instance, in the retail industry, real-time feedback from customers can help in optimizing store layouts, product placements, and promotional strategies, leading to increased sales and customer satisfaction.
Moreover, real-time monitoring and feedback are essential in the healthcare sector. Remote patient monitoring systems can track vital signs and other health metrics in real-time, allowing healthcare providers to intervene promptly in case of any abnormalities. This can be particularly beneficial for patients with chronic conditions, as it enables continuous monitoring and timely intervention, improving patient outcomes and reducing hospital readmissions.
In the financial sector, real-time monitoring of transactions can help in detecting and preventing fraudulent activities. By analyzing transaction data in real-time, financial institutions can identify suspicious patterns and take immediate action to prevent fraud. This not only protects the institution but also enhances customer trust and confidence.
Furthermore, real-time monitoring and feedback are critical in the field of environmental management. IoT sensors and data analytics can monitor environmental conditions such as air and water quality, providing real-time data to regulatory authorities and enabling timely interventions to address any issues. This can help in preventing environmental disasters and ensuring compliance with regulations.
In conclusion, real-time monitoring and feedback are indispensable in today's fast-paced and dynamic business environment. They enable businesses to detect and address issues promptly, optimize operations, and improve customer satisfaction. As technology continues to evolve, the capabilities for real-time monitoring and feedback will only expand, providing businesses with even greater opportunities to enhance their performance and achieve their goals.
7. Challenges in Integration
Integrating various systems, applications, and technologies within an organization is a complex process that often presents numerous challenges. These challenges can be broadly categorized into technical challenges and issues related to data privacy and security. Each of these categories encompasses a range of specific problems that organizations must address to achieve seamless integration.
7.1. Technical Challenges
Technical challenges are among the most significant obstacles to successful integration. These challenges can arise from a variety of sources, including differences in technology stacks, legacy systems, and the need for real-time data synchronization.
One of the primary technical challenges is the compatibility of different systems. Organizations often use a mix of legacy systems and modern applications, each with its own set of protocols, data formats, and communication methods. Ensuring that these disparate systems can communicate effectively requires extensive customization and the development of middleware solutions. Middleware acts as a bridge between different systems, translating data and commands so that they can be understood by each system involved. However, developing and maintaining middleware can be resource-intensive and requires specialized expertise.
Another significant technical challenge is data synchronization. In an integrated environment, data must be consistent and up-to-date across all systems. This requires real-time data synchronization, which can be difficult to achieve, especially when dealing with large volumes of data or systems that operate on different schedules. Inconsistent data can lead to errors, inefficiencies, and poor decision-making, undermining the benefits of integration.
Scalability is also a critical technical challenge. As organizations grow and their needs evolve, their integrated systems must be able to scale accordingly. This can be particularly challenging when dealing with legacy systems that were not designed with scalability in mind. Upgrading or replacing these systems can be costly and disruptive, but it is often necessary to ensure that the integrated environment can support the organization's long-term goals.
Finally, technical challenges can also arise from the need for continuous monitoring and maintenance. Integrated systems are complex and require ongoing oversight to ensure that they continue to function correctly. This includes monitoring for performance issues, identifying and resolving errors, and applying updates and patches as needed. Without proper maintenance, integrated systems can become unreliable and prone to failure.
For more insights on overcoming technical challenges in integration, you can explore Revolutionizing Industries with AI-Driven Digital Twins.
7.2. Data Privacy and Security
Data privacy and security are critical concerns in any integration project. As organizations integrate their systems and share data across different platforms, they must ensure that sensitive information is protected from unauthorized access and breaches.
One of the primary data privacy challenges is compliance with regulations. Different regions and industries have their own data privacy laws and regulations, such as the General Data Protection Regulation (GDPR) in the European Union and the Health Insurance Portability and Accountability Act (HIPAA) in the United States. Organizations must ensure that their integrated systems comply with all relevant regulations, which can be a complex and time-consuming process. Non-compliance can result in significant fines and damage to the organization's reputation.
Another significant challenge is securing data in transit and at rest. When data is transmitted between systems, it is vulnerable to interception and tampering. Organizations must implement robust encryption methods to protect data in transit and ensure that only authorized systems and users can access it. Similarly, data at rest must be stored securely, with access controls and encryption to prevent unauthorized access.
Access control is another critical aspect of data privacy and security. In an integrated environment, multiple systems and users may need access to the same data. Organizations must implement strict access controls to ensure that only authorized users can access sensitive information. This includes using authentication methods such as multi-factor authentication (MFA) and role-based access control (RBAC) to limit access based on the user's role and responsibilities.
Finally, organizations must also be prepared to respond to data breaches and security incidents. This includes having an incident response plan in place, regularly testing and updating the plan, and ensuring that all employees are trained on how to respond to a security incident. A swift and effective response can help minimize the impact of a data breach and protect the organization's reputation.
For more information on data privacy and security in integration, you can read  AI and Blockchain: Digital Security & Efficiency 2024.
In conclusion, while integration offers numerous benefits, it also presents significant challenges. Organizations must address both technical challenges and data privacy and security concerns to achieve successful integration. By doing so, they can create a seamless and secure integrated environment that supports their business goals and protects sensitive information.
7.3. Integration Complexity
Integrating generative AI with digital twins presents a multifaceted challenge that encompasses technical, operational, and strategic dimensions. At the technical level, one of the primary complexities arises from the need to harmonize disparate data sources. Digital twins rely on real-time data from various sensors, IoT devices, and other data streams to create a virtual replica of physical assets. Generative AI, on the other hand, requires vast amounts of data to train its models effectively. Ensuring that these data sources are compatible, accurate, and timely is a significant hurdle. Data integration tools and middleware can help, but they often require customization to handle the specific requirements of both digital twins and generative AI systems.
Another layer of complexity is the interoperability between different software platforms. Digital twins and generative AI systems are often developed using different programming languages, frameworks, and protocols. Achieving seamless communication between these systems necessitates the development of APIs, middleware, or other integration solutions. This can be particularly challenging in environments where legacy systems are in use, as these older systems may not support modern integration techniques.
Operationally, the integration of generative AI with digital twins requires a robust infrastructure capable of handling the computational demands of both technologies. Digital twins need to process real-time data continuously, while generative AI models require significant computational power for training and inference. This necessitates a scalable and resilient IT infrastructure, often involving cloud computing resources, edge computing, and high-performance data storage solutions. Ensuring that this infrastructure is both cost-effective and capable of meeting performance requirements adds another layer of complexity.
Strategically, organizations must consider the alignment of their integration efforts with broader business objectives. The integration of generative AI with digital twins should not be an end in itself but should serve to enhance business outcomes, whether through improved operational efficiency, better decision-making, or the creation of new revenue streams. This requires a clear understanding of the potential benefits and limitations of both technologies, as well as a well-defined roadmap for their integration. Stakeholder buy-in is crucial, as the integration process often involves significant investment and changes to existing workflows and processes.
Security and compliance are also critical considerations. The integration of generative AI with digital twins involves the handling of sensitive data, which must be protected against unauthorized access and breaches. Compliance with data protection regulations, such as GDPR or CCPA, adds another layer of complexity. Organizations must implement robust security measures, including encryption, access controls, and regular security audits, to ensure that their integrated systems are secure and compliant.
In summary, the integration of generative AI with digital twins is a complex endeavor that requires careful planning and execution. It involves technical challenges related to data harmonization and system interoperability, operational challenges related to infrastructure and performance, and strategic challenges related to alignment with business objectives and stakeholder buy-in. Security and compliance considerations further add to the complexity. However, with the right approach and resources, organizations can successfully navigate these challenges and unlock the significant benefits that the integration of these two powerful technologies can offer.
For more insights on integrating AI-driven digital twins, you can explore Revolutionizing Industries with AI-Driven Digital Twins.
7.4. Skill Gaps
The integration of generative AI with digital twins necessitates a diverse set of skills that are often in short supply. One of the most significant skill gaps is in the area of data science and machine learning. Generative AI models, such as Generative Adversarial Networks (GANs) and Variational Autoencoders (VAEs), require specialized knowledge to develop, train, and fine-tune. Data scientists with expertise in these areas are highly sought after, and their scarcity can be a significant bottleneck for organizations looking to leverage generative AI.
In addition to data science expertise, the integration of generative AI with digital twins requires skills in software engineering and system integration. Digital twins are complex systems that involve the integration of various data sources, real-time processing, and simulation capabilities. Software engineers with experience in developing and integrating such systems are essential for successful implementation. This includes knowledge of programming languages, such as Python and Java, as well as experience with integration tools and middleware.
Another critical skill gap is in the area of domain-specific knowledge. Digital twins are often used in specialized industries, such as manufacturing, healthcare, and energy. Understanding the specific requirements and challenges of these industries is crucial for developing effective digital twin solutions. This requires a combination of technical skills and industry-specific knowledge, which can be difficult to find. For example, developing a digital twin for a manufacturing plant requires not only expertise in digital twin technology but also a deep understanding of manufacturing processes and equipment.
Cybersecurity is another area where skill gaps can pose a significant challenge. The integration of generative AI with digital twins involves the handling of sensitive data, which must be protected against cyber threats. Cybersecurity professionals with expertise in securing complex, integrated systems are essential to ensure that the integrated solution is secure and compliant with data protection regulations. This includes knowledge of encryption, access controls, and security auditing.
Project management and strategic planning skills are also crucial for the successful integration of generative AI with digital twins. This involves coordinating the efforts of various teams, managing timelines and budgets, and ensuring that the integration aligns with broader business objectives. Project managers with experience in managing complex technology projects are essential to navigate the various challenges and ensure successful implementation.
To address these skill gaps, organizations can take several approaches. One option is to invest in training and development programs to upskill existing employees. This can involve formal training courses, workshops, and on-the-job training. Another option is to partner with external experts or consultants who have the necessary skills and experience. This can provide immediate access to the required expertise, although it may be more costly in the short term. Finally, organizations can look to hire new employees with the necessary skills, although this can be challenging given the high demand for these skills in the job market.
In conclusion, the integration of generative AI with digital twins requires a diverse set of skills that are often in short supply. Addressing these skill gaps is crucial for successful implementation and requires a combination of training and development, external partnerships, and strategic hiring. By taking a proactive approach to addressing these skill gaps, organizations can position themselves to successfully leverage the powerful combination of generative AI and digital twins.
For more information on hiring the right talent for AI projects, you can read The Essential Guide to Hiring a Prompt Engineer for AI Optimization.
8. Future of Integrating Generative AI with Digital Twins
The future of integrating generative AI with digital twins holds immense potential and promises to revolutionize various industries by enhancing predictive capabilities, optimizing operations, and enabling more sophisticated simulations. As both technologies continue to evolve, their convergence is expected to unlock new levels of innovation and efficiency.
One of the most significant future trends is the advancement of real-time, adaptive digital twins. Currently, digital twins are primarily used for monitoring and simulation purposes. However, with the integration of generative AI, these digital replicas can become more dynamic and adaptive. Generative AI can continuously learn from new data, enabling digital twins to update and evolve in real-time. This will allow for more accurate predictions and more responsive systems. For example, in manufacturing, an adaptive digital twin could optimize production processes on-the-fly, reducing downtime and improving efficiency.
Another promising development is the use of generative AI to enhance the decision-making capabilities of digital twins. By leveraging advanced machine learning algorithms, generative AI can analyze vast amounts of data and generate insights that would be difficult or impossible for humans to discern. This can lead to more informed and effective decision-making. For instance, in healthcare, a digital twin of a patient could use generative AI to predict the progression of a disease and recommend personalized treatment plans, improving patient outcomes.
The integration of generative AI with digital twins is also expected to drive innovation in the field of autonomous systems. Digital twins can serve as virtual testing grounds for autonomous vehicles, drones, and robots, allowing for the safe and efficient development of these technologies. Generative AI can enhance this process by generating realistic scenarios and environments for testing, accelerating the development and deployment of autonomous systems. This has the potential to revolutionize industries such as transportation, logistics, and agriculture.
Furthermore, the future of integrating generative AI with digital twins will likely see increased collaboration and data sharing across organizations and industries. As digital twins become more sophisticated and generative AI models become more powerful, the value of shared data and insights will grow. This can lead to the development of industry-wide digital twin ecosystems, where organizations collaborate to create more comprehensive and accurate digital replicas. For example, in the energy sector, companies could share data to create a digital twin of an entire power grid, enabling more efficient energy management and reducing the risk of blackouts.
The ethical and regulatory landscape will also play a crucial role in shaping the future of integrating generative AI with digital twins. As these technologies become more pervasive, there will be increased scrutiny on issues such as data privacy, security, and algorithmic bias. Organizations will need to navigate these challenges carefully, ensuring that their use of generative AI and digital twins is ethical and compliant with regulations. This will require ongoing collaboration with regulators, as well as the development of robust governance frameworks.
In conclusion, the future of integrating generative AI with digital twins is bright and full of potential. Advancements in real-time, adaptive digital twins, enhanced decision-making capabilities, and the development of autonomous systems are just a few of the exciting possibilities. Increased collaboration and data sharing, along with careful attention to ethical and regulatory considerations, will be essential to realizing the full potential of these technologies. As generative AI and digital twins continue to evolve, their integration is set to drive significant innovation and transformation across various industries.
8.1. Emerging Trends
Emerging trends in technology and business are shaping the future in unprecedented ways. One of the most significant trends is the rise of artificial intelligence (AI) and machine learning. These technologies are being integrated into various sectors, from healthcare to finance, to improve efficiency and decision-making processes. AI-driven analytics are enabling companies to predict market trends, personalize customer experiences, and automate routine tasks, thereby reducing operational costs and increasing productivity. Revolutionizing Industries with AI-Driven Digital Twins
Another emerging trend is the proliferation of the Internet of Things (IoT). IoT devices are becoming more prevalent in both consumer and industrial applications. In smart homes, IoT devices like thermostats, security cameras, and smart speakers are enhancing convenience and security. In industrial settings, IoT sensors are being used to monitor equipment health, optimize supply chains, and improve energy efficiency. The data generated by these devices is invaluable for predictive maintenance and operational optimization.
Blockchain technology is also gaining traction beyond its initial application in cryptocurrencies. Industries such as supply chain management, healthcare, and finance are exploring blockchain for its potential to enhance transparency, security, and traceability. For instance, blockchain can be used to track the provenance of goods in a supply chain, ensuring authenticity and reducing fraud.
The trend towards remote work and digital collaboration tools has been accelerated by the COVID-19 pandemic. Companies are increasingly adopting cloud-based solutions to facilitate remote work, leading to a surge in demand for collaboration platforms like Zoom, Microsoft Teams, and Slack. This shift is not only changing the way we work but also influencing real estate markets and urban planning as the need for physical office spaces diminishes.
Sustainability is another critical trend. Businesses are under increasing pressure to adopt sustainable practices and reduce their carbon footprint. This is driving innovation in renewable energy, electric vehicles, and sustainable supply chain practices. Companies are investing in green technologies and adopting circular economy principles to minimize waste and promote resource efficiency.
Lastly, the rise of 5G technology is set to revolutionize various industries by providing faster and more reliable internet connectivity. This will enable advancements in areas such as autonomous vehicles, smart cities, and augmented reality. The increased bandwidth and lower latency of 5G networks will support the deployment of more sophisticated IoT devices and enhance real-time data processing capabilities.
8.2. Potential Innovations
The landscape of potential innovations is vast and continually evolving, driven by advancements in technology and changing societal needs. One area ripe for innovation is healthcare. Personalized medicine, powered by genomics and AI, promises to revolutionize patient care by tailoring treatments to individual genetic profiles. This approach can improve the efficacy of treatments and reduce adverse effects. Additionally, telemedicine and remote monitoring technologies are making healthcare more accessible, especially in underserved areas. Wearable devices that track vital signs and health metrics are enabling proactive health management and early detection of diseases. Advanced AI in Healthcare 2024: Predictive Analytics
In the realm of energy, innovations in renewable energy sources and storage solutions are critical for addressing climate change. Advances in solar and wind energy technologies are making these sources more efficient and cost-effective. Energy storage solutions, such as advanced batteries and supercapacitors, are essential for managing the intermittent nature of renewable energy. Innovations in grid management and smart grids are also crucial for integrating renewable energy into existing infrastructure and ensuring reliable power supply.
Transportation is another sector poised for significant innovation. The development of electric and autonomous vehicles is set to transform the way we travel. Electric vehicles (EVs) are becoming more affordable and have longer ranges, making them a viable alternative to traditional internal combustion engine vehicles. Autonomous vehicles, powered by AI and advanced sensors, have the potential to reduce traffic accidents, improve traffic flow, and provide mobility solutions for those unable to drive.
In the field of agriculture, precision farming technologies are enabling more efficient and sustainable farming practices. Drones, IoT sensors, and AI-driven analytics are being used to monitor crop health, optimize irrigation, and manage pests. These technologies can increase crop yields, reduce resource usage, and minimize environmental impact.
The financial sector is also experiencing a wave of innovation, particularly with the rise of fintech. Blockchain and cryptocurrencies are challenging traditional banking systems and offering new ways to conduct transactions. Decentralized finance (DeFi) platforms are providing financial services without intermediaries, potentially increasing financial inclusion and reducing costs. AI and machine learning are being used to detect fraud, assess credit risk, and provide personalized financial advice. AI-Powered Fintech: Redefining Banking
Lastly, the field of education is undergoing a transformation with the advent of digital learning platforms and AI-driven personalized learning. These technologies are making education more accessible and tailored to individual learning styles and paces. Virtual and augmented reality are being used to create immersive learning experiences, enhancing engagement and retention.
8.3. Long-Term Impact on Industries
The long-term impact of emerging trends and potential innovations on industries is profound and multifaceted. In the healthcare industry, the integration of AI, genomics, and telemedicine is expected to lead to more personalized and efficient patient care. This will not only improve health outcomes but also reduce healthcare costs by enabling early diagnosis and preventive care. The shift towards remote monitoring and telehealth will make healthcare more accessible, particularly in rural and underserved areas, potentially reducing health disparities. Transforming Healthcare with AI
In the energy sector, the transition to renewable energy sources and the development of advanced energy storage solutions will play a crucial role in combating climate change. This shift will reduce dependence on fossil fuels, decrease greenhouse gas emissions, and promote energy security. The adoption of smart grids and grid management technologies will enhance the reliability and efficiency of power supply, supporting the integration of renewable energy into the grid.
The transportation industry will be significantly impacted by the rise of electric and autonomous vehicles. Electric vehicles will reduce carbon emissions and dependence on oil, contributing to environmental sustainability. Autonomous vehicles have the potential to improve road safety, reduce traffic congestion, and provide mobility solutions for the elderly and disabled. The widespread adoption of these technologies will also create new business opportunities and disrupt traditional automotive and transportation industries.
In agriculture, precision farming technologies will lead to more sustainable and efficient farming practices. This will increase food production to meet the demands of a growing global population while minimizing environmental impact. The use of drones, IoT sensors, and AI-driven analytics will optimize resource usage, reduce waste, and improve crop yields. These advancements will also support the development of sustainable supply chains and reduce the carbon footprint of agricultural practices.
The financial industry will undergo significant changes with the rise of fintech, blockchain, and decentralized finance. These technologies will increase financial inclusion by providing access to financial services for unbanked and underbanked populations. They will also reduce transaction costs, enhance security, and increase transparency. The adoption of AI and machine learning in finance will improve risk management, fraud detection, and personalized financial services, leading to more efficient and customer-centric financial systems. How AI is Changing Banking
In the education sector, digital learning platforms and AI-driven personalized learning will make education more accessible and tailored to individual needs. This will enhance learning outcomes and provide lifelong learning opportunities. The use of virtual and augmented reality in education will create immersive and engaging learning experiences, improving knowledge retention and application. These advancements will also support the development of a skilled workforce, ready to meet the demands of a rapidly changing job market.
Overall, the long-term impact of these trends and innovations will be transformative, driving economic growth, improving quality of life, and addressing global challenges such as climate change, healthcare access, and financial inclusion. Industries that embrace these changes and invest in innovation will be well-positioned to thrive in the future.
9. Real-World Examples
Real-world examples provide tangible evidence of how theoretical concepts and strategies are applied in practical scenarios. They help bridge the gap between abstract ideas and their implementation, offering insights into the challenges and successes experienced by different industries. By examining real-world examples, we can better understand the impact of various approaches and technologies, and how they can be adapted to suit specific needs. In this section, we will explore two case studies from the manufacturing and healthcare sectors to illustrate the application of innovative solutions in these fields.
9.1. Case Study 1: Manufacturing
In the manufacturing sector, the adoption of advanced technologies and innovative practices has led to significant improvements in efficiency, productivity, and quality. One notable example is the implementation of Industry 4.0 principles by a leading automotive manufacturer. Industry 4.0, also known as the fourth industrial revolution, involves the integration of cyber-physical systems, the Internet of Things (IoT), and big data analytics to create smart factories.
The automotive manufacturer in question faced several challenges, including high production costs, frequent equipment breakdowns, and inconsistent product quality. To address these issues, the company decided to implement a comprehensive Industry 4.0 strategy. This involved the deployment of IoT sensors across the production line to monitor equipment performance in real-time. The data collected from these sensors was then analyzed using advanced analytics and machine learning algorithms to predict potential equipment failures and schedule preventive maintenance.
As a result of this implementation, the company experienced a significant reduction in unplanned downtime, leading to increased production efficiency and lower maintenance costs. Additionally, the use of data analytics enabled the company to identify and address quality issues more quickly, resulting in higher product quality and customer satisfaction. The integration of cyber-physical systems also allowed for greater flexibility in the production process, enabling the company to respond more rapidly to changes in market demand.
This case study demonstrates the transformative potential of Industry 4.0 technologies in the manufacturing sector. By leveraging IoT, big data analytics, and machine learning, manufacturers can optimize their operations, reduce costs, and improve product quality. The success of this automotive manufacturer serves as a compelling example for other companies looking to adopt similar strategies to enhance their competitiveness in the market. For more insights on how AI-driven digital twins are revolutionizing industries, you can read Revolutionizing Industries with AI-Driven Digital Twins.
9.2. Case Study 2: Healthcare
The healthcare sector has also seen significant advancements through the adoption of innovative technologies and practices. One compelling example is the use of telemedicine and remote patient monitoring by a large healthcare provider to improve patient outcomes and reduce healthcare costs.
The healthcare provider faced several challenges, including a growing patient population, limited access to healthcare services in rural areas, and rising healthcare costs. To address these issues, the provider implemented a telemedicine program that allowed patients to consult with healthcare professionals remotely using video conferencing technology. Additionally, the provider deployed remote patient monitoring devices to track the health status of patients with chronic conditions, such as diabetes and heart disease, in real-time.
The telemedicine program enabled patients to receive timely medical consultations without the need to travel long distances, improving access to healthcare services, particularly for those in rural areas. This not only enhanced patient satisfaction but also reduced the burden on healthcare facilities, allowing them to focus on more critical cases. The remote patient monitoring devices provided healthcare professionals with continuous data on patients' health status, enabling early detection of potential health issues and timely intervention.
As a result of these initiatives, the healthcare provider observed a significant improvement in patient outcomes, with reduced hospital readmission rates and better management of chronic conditions. The use of telemedicine and remote patient monitoring also led to cost savings for both the provider and patients, as it reduced the need for in-person visits and hospitalizations.
9.3. Case Study 3: Smart Cities
Smart cities represent a transformative approach to urban living, leveraging technology to enhance the quality of life for residents, improve the efficiency of city services, and promote sustainability. The concept of smart cities encompasses a wide range of applications, from intelligent transportation systems and energy-efficient buildings to advanced waste management and public safety solutions. This case study will explore the implementation of smart city initiatives in various global cities, highlighting the benefits, challenges, and future prospects of this innovative urban model.
One of the most prominent examples of a smart city is Barcelona, Spain. Barcelona has implemented a comprehensive smart city strategy that includes a variety of interconnected projects aimed at improving urban living. The city has deployed a network of sensors and IoT devices to monitor and manage resources such as water, energy, and waste. For instance, smart water meters provide real-time data on water usage, helping to detect leaks and reduce consumption. Similarly, smart street lighting systems adjust brightness based on pedestrian and vehicular traffic, resulting in significant energy savings.
Another key aspect of Barcelona's smart city initiative is its focus on mobility. The city has introduced a range of smart transportation solutions, including a bike-sharing program, electric vehicle charging stations, and an integrated public transportation system. These efforts have not only reduced traffic congestion but also lowered carbon emissions, contributing to a cleaner and more sustainable urban environment.
Singapore is another leading example of a smart city. The city-state has invested heavily in digital infrastructure and data analytics to enhance urban living. One of the flagship projects is the Smart Nation initiative, which aims to harness technology to improve various aspects of daily life. For example, the Smart Health initiative leverages telemedicine and wearable devices to provide remote healthcare services, making it easier for residents to access medical care. Additionally, the Smart Mobility program uses real-time data to optimize traffic flow and reduce travel times.
Despite the numerous benefits, the implementation of smart city initiatives also presents several challenges. One of the primary concerns is data privacy and security. The extensive use of sensors and IoT devices generates vast amounts of data, raising questions about how this data is collected, stored, and used. Ensuring the privacy and security of residents' data is crucial to maintaining public trust and preventing potential misuse.
Another challenge is the digital divide. While smart city technologies can significantly improve urban living, they may also exacerbate existing inequalities if not implemented inclusively. Ensuring that all residents have access to the benefits of smart city initiatives, regardless of their socioeconomic status, is essential for creating equitable and inclusive urban environments.
Looking ahead, the future of smart cities appears promising. Advances in technology, such as 5G connectivity, artificial intelligence, and blockchain, are expected to further enhance the capabilities of smart city solutions. For instance, 5G networks will enable faster and more reliable communication between devices, supporting the deployment of more sophisticated IoT applications. Similarly, AI can be used to analyze large datasets and provide actionable insights for city planners and policymakers.
In conclusion, smart cities represent a forward-thinking approach to urban living, leveraging technology to create more efficient, sustainable, and livable environments. While there are challenges to overcome, the potential benefits of smart city initiatives are immense. By addressing issues such as data privacy, security, and inclusivity, cities can harness the power of technology to improve the quality of life for all residents.
10. In-Depth Explanations
In-depth explanations are essential for understanding complex topics and concepts. They provide detailed information, context, and insights that help readers grasp the nuances and intricacies of a subject. In-depth explanations can be particularly valuable in fields such as science, technology, medicine, and economics, where a thorough understanding of the underlying principles is crucial for making informed decisions and advancing knowledge.
One of the key elements of an in-depth explanation is clarity. It is important to present information in a clear and concise manner, avoiding jargon and technical terms that may be unfamiliar to the reader. When technical terms are necessary, they should be defined and explained in a way that is easy to understand. Using analogies and examples can also help to illustrate complex concepts and make them more relatable.
Another important aspect of in-depth explanations is context. Providing background information and historical context can help readers understand how a particular concept or technology has evolved over time. This can also highlight the significance of recent developments and innovations, showing how they build on previous knowledge and address existing challenges.
In-depth explanations should also be comprehensive, covering all relevant aspects of a topic. This includes discussing different perspectives and viewpoints, as well as potential implications and future directions. For example, when explaining a new medical treatment, it is important to discuss not only how it works but also its potential side effects, limitations, and the conditions under which it is most effective.
Visual aids such as diagrams, charts, and graphs can also enhance in-depth explanations by providing a visual representation of complex information. These tools can help to break down complicated processes and data, making them easier to understand and interpret. For instance, a flowchart can illustrate the steps involved in a manufacturing process, while a graph can show trends and patterns in data over time.
In addition to clarity, context, and comprehensiveness, in-depth explanations should also be accurate and well-researched. This involves citing credible sources and providing evidence to support claims and statements. Inaccurate or misleading information can undermine the credibility of the explanation and lead to misunderstandings or incorrect conclusions.
Engaging the reader is another important consideration. In-depth explanations should be written in an engaging and accessible style, capturing the reader's interest and encouraging them to explore the topic further. This can be achieved by using a conversational tone, posing questions, and highlighting interesting facts and anecdotes.
In conclusion, in-depth explanations play a crucial role in enhancing our understanding of complex topics and concepts. By providing clear, comprehensive, and well-researched information, they help readers grasp the nuances and intricacies of a subject. Whether in science, technology, medicine, or economics, in-depth explanations are essential for making informed decisions, advancing knowledge, and fostering a deeper appreciation of the world around us.
10.1. Technical Architecture
Technical architecture refers to the structured design and organization of a system's components and their interactions. It serves as a blueprint for the development, deployment, and maintenance of software and hardware systems, ensuring that they meet the desired requirements and performance standards. Technical architecture encompasses various aspects, including system components, data flow, integration, scalability, security, and maintainability.
One of the fundamental elements of technical architecture is the identification and definition of system components. These components can include hardware devices, software applications, databases, and network infrastructure. Each component has a specific role and function within the system, and their interactions must be carefully designed to ensure seamless operation. For example, in a web application, the components may include the web server, application server, database server, and client devices. The technical architecture defines how these components communicate and work together to deliver the desired functionality.
Data flow is another critical aspect of technical architecture. It involves the movement of data between different components and processes within the system. Understanding data flow is essential for designing efficient and effective systems, as it helps to identify potential bottlenecks and optimize performance. Data flow diagrams and models can be used to visualize the flow of data, showing how information is collected, processed, stored, and transmitted. For instance, in an e-commerce system, data flow diagrams can illustrate how customer orders are processed, from the initial order placement to payment processing and order fulfillment.
Integration is a key consideration in technical architecture, particularly in complex systems that involve multiple components and technologies. Integration ensures that different parts of the system can work together seamlessly, exchanging data and functionality as needed. This can involve the use of APIs (Application Programming Interfaces), middleware, and other integration tools and techniques. For example, in a healthcare system, integration may involve connecting electronic health record (EHR) systems with laboratory information systems (LIS) and medical imaging systems to provide a comprehensive view of patient data.
Scalability is another important aspect of technical architecture. It refers to the system's ability to handle increased load and demand without compromising performance. Scalability can be achieved through various means, such as load balancing, horizontal and vertical scaling, and distributed computing. For example, a cloud-based application can be designed to scale horizontally by adding more servers to handle increased traffic, or vertically by upgrading existing servers with more powerful hardware.
Security is a critical consideration in technical architecture, as it involves protecting the system and its data from unauthorized access, breaches, and other threats. Security measures can include encryption, authentication, access control, and intrusion detection and prevention systems. For example, in a financial system, security measures may involve encrypting sensitive data, implementing multi-factor authentication for user access, and monitoring for suspicious activity.
Maintainability is also an important aspect of technical architecture, as it involves designing systems that are easy to update, modify, and troubleshoot. This can include using modular and reusable components, following coding standards and best practices, and implementing comprehensive documentation and testing procedures. For example, a software application can be designed with a modular architecture, allowing individual components to be updated or replaced without affecting the entire system.
10.2 Data Flow and Management
Data flow and management are critical components in any data-driven system, ensuring that data is efficiently collected, processed, stored, and utilized. The process begins with data collection, where raw data is gathered from various sources such as sensors, databases, user inputs, or external APIs. This data is often heterogeneous, coming in different formats and structures, which necessitates a robust data ingestion mechanism. Tools like Apache Kafka or AWS Kinesis are commonly used for real-time data streaming, while batch processing can be handled by systems like Apache Hadoop.
Once the data is collected, it undergoes a series of preprocessing steps to clean and transform it into a usable format. This involves removing duplicates, handling missing values, normalizing data, and converting it into a consistent format. Data preprocessing is crucial as it directly impacts the quality of the insights derived from the data. Techniques such as ETL (Extract, Transform, Load) are employed to automate this process, with tools like Talend, Informatica, and Apache NiFi being popular choices.
After preprocessing, the data is stored in a data warehouse or data lake, depending on the use case. Data warehouses like Amazon Redshift, Google BigQuery, and Snowflake are optimized for structured data and complex queries, making them ideal for business intelligence and reporting. On the other hand, data lakes such as Amazon S3 and Azure Data Lake are designed to handle large volumes of unstructured and semi-structured data, providing flexibility for data scientists and analysts to explore and analyze the data.
Data management also involves ensuring data quality, security, and governance. Data quality management includes continuous monitoring and validation to ensure the data remains accurate, complete, and consistent. Security measures such as encryption, access controls, and auditing are implemented to protect sensitive data from unauthorized access and breaches. Data governance frameworks establish policies and procedures for data usage, ensuring compliance with regulations like GDPR and CCPA.
Effective data flow and management enable organizations to harness the full potential of their data, driving informed decision-making and innovation. By implementing best practices and leveraging advanced tools, businesses can create a seamless data pipeline that supports their analytical and operational needs.
10.3 Algorithms and Models Used
Algorithms and models are the backbone of data-driven systems, enabling the extraction of valuable insights and predictions from data. These algorithms can be broadly categorized into supervised learning, unsupervised learning, and reinforcement learning, each serving different purposes and applications.
Supervised learning algorithms are used when the goal is to predict a target variable based on input features. These algorithms learn from labeled training data, where the correct output is provided for each input. Common supervised learning algorithms include linear regression, logistic regression, decision trees, random forests, support vector machines, and neural networks. For example, linear regression is used for predicting continuous variables, while logistic regression is used for binary classification tasks. Decision trees and random forests are popular for their interpretability and ability to handle complex, non-linear relationships. Neural networks, particularly deep learning models, have gained prominence for their ability to handle large-scale, high-dimensional data, making them suitable for tasks like image and speech recognition.
Unsupervised learning algorithms, on the other hand, are used when the data does not have labeled outputs. These algorithms aim to identify patterns and structures within the data. Common unsupervised learning techniques include clustering, dimensionality reduction, and anomaly detection. Clustering algorithms like K-means and hierarchical clustering group similar data points together, which can be useful for customer segmentation or identifying similar items. Dimensionality reduction techniques like Principal Component Analysis (PCA) and t-Distributed Stochastic Neighbor Embedding (t-SNE) reduce the number of features while preserving the underlying structure, making it easier to visualize and analyze high-dimensional data. Anomaly detection algorithms identify outliers or unusual patterns, which can be critical for fraud detection or monitoring system health.
Reinforcement learning is a type of machine learning where an agent learns to make decisions by interacting with an environment. The agent receives rewards or penalties based on its actions and learns to maximize cumulative rewards over time. Reinforcement learning has been successfully applied in areas such as robotics, game playing, and autonomous driving. Algorithms like Q-learning, Deep Q-Networks (DQN), and Proximal Policy Optimization (PPO) are commonly used in reinforcement learning applications.
In addition to these traditional machine learning algorithms, advanced models like ensemble methods and hybrid models are also widely used. Ensemble methods, such as boosting and bagging, combine multiple models to improve overall performance and robustness. Hybrid models integrate different types of algorithms to leverage their strengths and mitigate their weaknesses, providing more accurate and reliable predictions.
The choice of algorithm and model depends on the specific problem, the nature of the data, and the desired outcome. By selecting the appropriate algorithms and fine-tuning their parameters, data scientists can build powerful models that drive actionable insights and support data-driven decision-making.
Comparisons and contrasts are essential for understanding the relative strengths and weaknesses of different approaches, technologies, or methodologies. In the context of data flow and management, and algorithms and models, several key comparisons and contrasts can be drawn.
Firstly, comparing data warehouses and data lakes highlights their distinct use cases and advantages. Data warehouses are optimized for structured data and complex queries, making them ideal for business intelligence and reporting. They provide high performance and reliability for analytical workloads but can be expensive and less flexible for handling unstructured data. In contrast, data lakes offer a more flexible and cost-effective solution for storing large volumes of unstructured and semi-structured data. They support a wide range of data types and formats, making them suitable for data exploration and advanced analytics. However, data lakes may require more effort to ensure data quality and governance.
When comparing supervised and unsupervised learning algorithms, the primary distinction lies in the presence of labeled data. Supervised learning algorithms require labeled training data and are used for tasks where the goal is to predict a specific outcome. They tend to perform well when there is a clear relationship between input features and the target variable. Unsupervised learning algorithms, on the other hand, do not require labeled data and are used to identify patterns and structures within the data. They are useful for exploratory data analysis and tasks like clustering and anomaly detection but may not provide as clear or interpretable results as supervised learning algorithms.
Reinforcement learning differs from both supervised and unsupervised learning in its approach to learning from interaction with an environment. While supervised and unsupervised learning rely on static datasets, reinforcement learning involves an agent making decisions and receiving feedback in the form of rewards or penalties. This dynamic learning process allows reinforcement learning to excel in tasks that require sequential decision-making and adaptation to changing conditions, such as robotics and game playing.
Another important comparison is between traditional machine learning algorithms and deep learning models. Traditional algorithms like linear regression, decision trees, and support vector machines are often easier to interpret and require less computational power. They perform well on smaller datasets and problems with well-defined features. Deep learning models, particularly neural networks, are capable of handling large-scale, high-dimensional data and can automatically learn complex feature representations. They have achieved state-of-the-art performance in tasks like image and speech recognition but require significant computational resources and large amounts of labeled data for training.
Ensemble methods and hybrid models offer a contrast to single-model approaches by combining multiple algorithms to improve performance and robustness. Ensemble methods like boosting and bagging aggregate the predictions of several models to reduce variance and bias, leading to more accurate and reliable results. Hybrid models integrate different types of algorithms to leverage their complementary strengths, providing a more comprehensive solution to complex problems.
In summary, comparisons and contrasts in data flow and management, and algorithms and models, provide valuable insights into their respective strengths and limitations. By understanding these differences, organizations can make informed decisions about the best approaches and technologies to adopt for their specific needs, ultimately driving more effective and efficient data-driven solutions.
11.1. Traditional Predictive Analytics vs. AI-Driven Digital Twins
Traditional predictive analytics and AI-driven digital twins represent two distinct approaches to forecasting and decision-making in various industries. Traditional predictive analytics relies on historical data and statistical algorithms to predict future outcomes. This method typically involves the use of regression models, time series analysis, and machine learning techniques to identify patterns and trends in past data. The primary goal is to make informed predictions about future events based on these historical patterns. Traditional predictive analytics is widely used in fields such as finance, marketing, and healthcare to forecast sales, customer behavior, and disease outbreaks, among other things.
On the other hand, AI-driven digital twins take predictive analytics to a whole new level by creating virtual replicas of physical assets, processes, or systems. These digital twins are dynamic, real-time models that continuously receive data from their physical counterparts through sensors and IoT devices. AI algorithms then analyze this data to provide insights, predictions, and recommendations. Unlike traditional predictive analytics, which relies heavily on historical data, AI-driven digital twins leverage real-time data to offer a more accurate and up-to-date representation of the physical world. This real-time capability allows for more precise predictions and the ability to simulate various scenarios to optimize performance and decision-making.
One of the key advantages of AI-driven digital twins is their ability to provide a holistic view of complex systems. For example, in manufacturing, a digital twin can monitor the entire production process, from raw materials to finished products, and identify potential bottlenecks or inefficiencies. This level of insight is challenging to achieve with traditional predictive analytics alone. Additionally, AI-driven digital twins can be used for predictive maintenance, where they continuously monitor the condition of machinery and predict when maintenance is needed, reducing downtime and costs.
In summary, while traditional predictive analytics relies on historical data and statistical models to make predictions, AI-driven digital twins offer a more advanced and real-time approach by creating virtual replicas of physical assets and systems. This allows for more accurate predictions, real-time monitoring, and the ability to simulate various scenarios for optimization. As technology continues to advance, the use of AI-driven digital twins is expected to become increasingly prevalent across various industries, providing a powerful tool for decision-making and performance optimization.
For more information, you can read about Revolutionizing Industries with AI-Driven Digital Twins and AI-Driven Digital Twins: Transforming Industries.
11.2. Generative AI vs. Other AI Models
Generative AI and other AI models represent different approaches to artificial intelligence, each with its own strengths and applications. Generative AI, as the name suggests, focuses on generating new content, such as images, text, music, or even entire virtual environments. This type of AI is designed to create data that is similar to the input data it has been trained on. One of the most well-known examples of generative AI is the Generative Adversarial Network (GAN), which consists of two neural networks: a generator and a discriminator. The generator creates new data, while the discriminator evaluates the authenticity of the generated data. Through this adversarial process, the generator improves its ability to produce realistic content.
Generative AI has a wide range of applications, from creating realistic images and videos to generating human-like text and even designing new drugs. For instance, in the field of art and entertainment, generative AI can be used to create original artwork, music compositions, and even entire virtual worlds for video games. In healthcare, generative AI can assist in drug discovery by generating new molecular structures that have the potential to become effective medications. The ability of generative AI to create new and innovative content makes it a powerful tool in various creative and scientific fields.
In contrast, other AI models, such as discriminative models, focus on classification and prediction tasks. Discriminative models are designed to distinguish between different classes or categories based on input data. For example, a discriminative model might be used to classify emails as spam or not spam, or to predict whether a customer will churn based on their behavior. These models are typically trained using supervised learning techniques, where they learn to map input data to specific output labels. Common examples of discriminative models include support vector machines (SVMs), decision trees, and convolutional neural networks (CNNs).
While generative AI excels at creating new content, discriminative models are more suited for tasks that involve classification, prediction, and decision-making. Both types of AI models have their own unique strengths and are often used in combination to achieve more comprehensive solutions. For example, in natural language processing (NLP), generative models can be used to generate human-like text, while discriminative models can be used to classify the sentiment of the text or identify named entities.
In summary, generative AI focuses on creating new content and has applications in fields such as art, entertainment, and drug discovery. Other AI models, such as discriminative models, are designed for classification and prediction tasks and are commonly used in applications like spam detection and customer churn prediction. Both types of AI models have their own strengths and can be used together to achieve more comprehensive and effective solutions in various domains.
11.3. Digital Twins vs. Simulation Models
Digital twins and simulation models are both powerful tools used to understand, analyze, and optimize complex systems, but they differ in their approach and capabilities. A digital twin is a virtual replica of a physical asset, process, or system that is continuously updated with real-time data from its physical counterpart. This real-time data integration allows digital twins to provide an accurate and up-to-date representation of the physical world. Digital twins are dynamic and can simulate various scenarios, predict outcomes, and provide insights for decision-making and optimization. They are widely used in industries such as manufacturing, healthcare, and smart cities to monitor and optimize performance, predict maintenance needs, and improve overall efficiency.
One of the key advantages of AI-driven digital twins is their ability to provide a holistic view of complex systems. For example, in a smart city, a digital twin can integrate data from various sources, such as traffic sensors, weather stations, and energy grids, to provide a comprehensive view of the city's operations. This allows city planners to make informed decisions, optimize resource allocation, and improve the quality of life for residents. In manufacturing, digital twins can monitor the entire production process, identify potential bottlenecks, and optimize production schedules to increase efficiency and reduce costs.
In contrast, simulation models are mathematical or computational representations of a system that are used to study its behavior under different conditions. Simulation models are typically used to analyze specific scenarios or test hypotheses by running simulations with different input parameters. Unlike digital twins, simulation models do not continuously receive real-time data from the physical world. Instead, they rely on predefined data and assumptions to simulate the behavior of the system. Simulation models are widely used in fields such as engineering, finance, and environmental science to study the impact of different variables on system performance and to make predictions about future outcomes.
While both digital twins and simulation models are valuable tools for understanding and optimizing complex systems, AI-driven digital twins offer several advantages due to their real-time data integration and dynamic nature. Digital twins provide a more accurate and up-to-date representation of the physical world, allowing for more precise predictions and real-time decision-making. Additionally, AI-driven digital twins can simulate various scenarios and provide insights for optimization, making them a powerful tool for industries that require continuous monitoring and optimization of their operations.
In summary, digital twins and simulation models are both used to analyze and optimize complex systems, but they differ in their approach and capabilities. Digital twins are dynamic, real-time virtual replicas of physical assets or systems that continuously receive data from their physical counterparts, providing a more accurate and up-to-date representation of the physical world. Simulation models, on the other hand, are mathematical or computational representations used to study specific scenarios or test hypotheses based on predefined data and assumptions. Both tools have their own strengths and are used in various industries to improve performance, optimize operations, and make informed decisions.
For further reading, check out Predictive Analytics: AI for High-Stakes Decisions and Generative AI & Industrial Simulations: Innovate Fast.
12. Why Choose Rapid Innovation for Implementation and Development
In the fast-paced world of technology, businesses are constantly seeking ways to stay ahead of the curve. Rapid innovation in AI and Blockchain has emerged as a key strategy for companies looking to implement and develop cutting-edge solutions quickly and efficiently. This approach is particularly valuable in the realms of Artificial Intelligence (AI) and Blockchain, where the landscape is continually evolving. Rapid Innovation allows businesses to adapt to changes swiftly, ensuring they remain competitive and relevant. By leveraging the expertise in AI and Blockchain, and offering customized solutions, companies can address specific needs and challenges, driving growth and success.
12.1. Expertise in AI and Blockchain
Artificial Intelligence and Blockchain are two of the most transformative technologies of our time. AI has the potential to revolutionize industries by automating processes, enhancing decision-making, and providing insights through data analysis. Blockchain, on the other hand, offers a decentralized and secure way to record transactions, making it invaluable for industries such as finance, supply chain, and healthcare. Rapid innovation in AI and Blockchain requires a deep understanding of the technologies and their applications.
Companies that specialize in AI and Blockchain bring a wealth of knowledge and experience to the table. They are well-versed in the latest advancements and best practices, ensuring that the solutions they develop are not only cutting-edge but also practical and scalable. For instance, AI experts can design algorithms that improve over time, learning from data to provide more accurate predictions and recommendations. Blockchain specialists can create secure and transparent systems that enhance trust and efficiency in transactions.
Moreover, these experts are adept at identifying the most suitable technologies and tools for a given project. They can assess the specific needs of a business and recommend the best AI models or Blockchain frameworks to achieve the desired outcomes. This level of expertise is crucial for successful implementation and development, as it ensures that the solutions are robust, efficient, and aligned with the business objectives.
12.2. Customized Solutions
One of the key advantages of rapid innovation in AI and Blockchain is the ability to provide customized solutions tailored to the unique needs of each business. Off-the-shelf products often fall short in addressing specific challenges or requirements, leading to suboptimal performance and limited value. Customized solutions, on the other hand, are designed to fit seamlessly into the existing workflows and processes, maximizing their impact and effectiveness.
Customization begins with a thorough understanding of the business and its goals. This involves close collaboration between the development team and the stakeholders to identify pain points, opportunities, and desired outcomes. By gaining a deep insight into the business, the development team can design solutions that address the specific needs and challenges, ensuring a perfect fit.
For example, in the case of AI, a customized solution might involve developing a machine learning model that is trained on the company’s own data, rather than relying on generic datasets. This ensures that the model is highly relevant and accurate, providing insights and predictions that are directly applicable to the business. Similarly, a customized Blockchain solution might involve creating a private blockchain network that is tailored to the company’s specific requirements, such as the need for high transaction throughput or enhanced privacy.
Furthermore, customized solutions offer greater flexibility and scalability. As the business grows and evolves, the solutions can be adapted and expanded to meet new demands and challenges. This ensures that the investment in technology continues to deliver value over time, supporting long-term growth and success.
is a powerful strategy for businesses looking to implement and develop advanced technologies such as AI and Blockchain. By leveraging the expertise of specialists and offering customized solutions, companies can address their specific needs and challenges, driving growth and success. This approach ensures that the solutions are not only cutting-edge but also practical, scalable, and aligned with the business objectives, providing a solid foundation for future innovation and development.
12.3. Proven Methodologies
Proven methodologies are systematic, repeatable processes that have been tested and validated to achieve consistent and reliable results. These methodologies are essential in various fields, including project management, software development, healthcare, and education, among others. They provide a structured approach to problem-solving and decision-making, ensuring that tasks are completed efficiently and effectively.
One of the most well-known proven methodologies in project management is the Project Management Institute's (PMI) Project Management Body of Knowledge (PMBOK). The PMBOK provides a comprehensive framework for managing projects, including processes, best practices, and guidelines. It covers various aspects of project management, such as scope, time, cost, quality, human resources, communication, risk, and procurement. By following the PMBOK methodology, project managers can ensure that their projects are completed on time, within budget, and to the required quality standards.
In software development, Agile and Scrum are two widely adopted proven methodologies. Agile is an iterative approach that emphasizes flexibility, collaboration, and customer feedback. It allows development teams to respond quickly to changes and deliver high-quality software in shorter cycles. Scrum, a subset of Agile, provides a specific framework for managing and completing complex projects. It involves roles such as Scrum Master, Product Owner, and Development Team, and practices like sprints, daily stand-ups, and sprint reviews. These methodologies have been proven to enhance productivity, improve communication, and deliver better software products.
In healthcare, evidence-based practice (EBP) is a proven methodology that integrates clinical expertise, patient values, and the best available evidence to make informed decisions about patient care. EBP involves a systematic process of reviewing and appraising research evidence, applying clinical judgment, and considering patient preferences. This approach has been shown to improve patient outcomes, enhance the quality of care, and reduce healthcare costs.
In education, the Universal Design for Learning (UDL) is a proven methodology that aims to create inclusive learning environments that accommodate the diverse needs of all students. UDL provides a framework for designing curriculum, instruction, and assessment that are flexible and accessible to all learners. It involves three main principles: providing multiple means of representation, multiple means of action and expression, and multiple means of engagement. By implementing UDL, educators can ensure that all students have equal opportunities to succeed.
Proven methodologies are not static; they evolve over time as new research, technologies, and best practices emerge. Organizations and professionals must stay updated with the latest developments in their respective fields to ensure that they are using the most effective methodologies. Continuous learning, training, and professional development are essential to maintaining and improving the effectiveness of these methodologies.
In conclusion, proven methodologies provide a structured and reliable approach to achieving desired outcomes in various fields. They are based on systematic processes, best practices, and evidence, ensuring that tasks are completed efficiently and effectively. By adopting and continuously improving these methodologies, organizations and professionals can enhance their performance, achieve their goals, and deliver high-quality results.
12.4. Client Success Stories
Client success stories are powerful testimonials that showcase the positive impact of a company's products or services on its customers. These stories highlight real-life examples of how clients have benefited from the solutions provided, demonstrating the value and effectiveness of the company's offerings. Client success stories serve as compelling evidence of a company's capabilities and can significantly influence potential customers' purchasing decisions.
One of the key elements of a compelling client success story is a clear and detailed description of the client's challenges before using the company's products or services. This context helps potential customers understand the specific problems that the company can solve. For example, a software company might describe how a client was struggling with inefficient workflows, data silos, and communication breakdowns before implementing their software solution. By providing this background, the company sets the stage for showcasing the transformative impact of their product.
The next crucial element is a detailed account of how the company's products or services addressed the client's challenges. This section should highlight the specific features, functionalities, and benefits that made a difference for the client. For instance, the software company might explain how their solution streamlined workflows, integrated data from multiple sources, and improved team communication. Providing concrete examples and quantifiable results, such as increased productivity, reduced costs, or higher customer satisfaction, adds credibility and impact to the story.
Client success stories should also include testimonials or quotes from the clients themselves. These firsthand accounts add authenticity and trustworthiness to the narrative. Clients can share their experiences, insights, and satisfaction with the company's products or services. For example, a client might say, "Since implementing the software, our team has become more efficient, and we've seen a 30% increase in productivity." Such testimonials provide social proof and help potential customers relate to the experiences of others.
Another important aspect of client success stories is highlighting the ongoing support and partnership provided by the company. This demonstrates the company's commitment to customer success and long-term relationships. For instance, the software company might describe how they provided training, regular updates, and responsive customer support to ensure the client's continued success. This reassures potential customers that they will receive the necessary support and assistance throughout their journey.
Client success stories can be presented in various formats, including written case studies, video testimonials, and interactive web pages. Each format has its advantages and can be used strategically to reach different audiences. Written case studies provide detailed and in-depth accounts, while video testimonials offer a more personal and engaging experience. Interactive web pages can combine text, images, and videos to create a dynamic and immersive storytelling experience.
In conclusion, client success stories are powerful tools for showcasing the value and effectiveness of a company's products or services. They provide real-life examples of how clients have benefited, highlighting the challenges faced, the solutions provided, and the positive outcomes achieved. By including testimonials, quantifiable results, and demonstrating ongoing support, client success stories build trust and credibility, influencing potential customers' purchasing decisions. Companies should leverage these stories in various formats to reach and engage their target audience effectively.
13. Conclusion
In conclusion, the importance of proven methodologies and client success stories cannot be overstated in today's competitive business landscape. Proven methodologies provide a structured and reliable approach to achieving desired outcomes across various fields, including project management, software development, healthcare, and education. These methodologies are based on systematic processes, best practices, and evidence, ensuring that tasks are completed efficiently and effectively. By adopting and continuously improving these methodologies, organizations and professionals can enhance their performance, achieve their goals, and deliver high-quality results.
Client success stories, on the other hand, serve as powerful testimonials that showcase the positive impact of a company's products or services on its customers. These stories highlight real-life examples of how clients have benefited from the solutions provided, demonstrating the value and effectiveness of the company's offerings. By including detailed descriptions of the client's challenges, the solutions provided, and the positive outcomes achieved, client success stories build trust and credibility. Testimonials and quantifiable results add authenticity and impact, influencing potential customers' purchasing decisions.
Together, proven methodologies and client success stories create a compelling narrative that can significantly enhance a company's reputation and market position. Proven methodologies provide the foundation for delivering consistent and reliable results, while client success stories offer tangible evidence of the company's capabilities and the value of its products or services. By leveraging both, companies can effectively communicate their expertise, build trust with potential customers, and differentiate themselves from competitors.
In today's fast-paced and ever-evolving business environment, staying updated with the latest developments and continuously improving methodologies is essential. Organizations and professionals must invest in continuous learning, training, and professional development to maintain and enhance the effectiveness of their methodologies. Additionally, companies should actively seek and document client success stories to showcase their impact and build a strong portfolio of testimonials.
In summary, proven methodologies and client success stories are invaluable assets for any organization. They provide a structured approach to achieving desired outcomes and offer compelling evidence of a company's capabilities and the value of its products or services. By adopting and continuously improving proven methodologies and leveraging client success stories, companies can enhance their performance, build trust with potential customers, and achieve long-term success in their respective fields.
13.1. Recap of Key Points
In this section, we will revisit the key points discussed throughout the document to ensure a comprehensive understanding of the subject matter. The primary objective of this recap is to consolidate the essential information and insights that have been covered, providing a clear and concise summary for the reader.
Firstly, we explored the foundational concepts and definitions pertinent to the topic. This included an in-depth examination of the core principles, terminologies, and theoretical frameworks that underpin the subject. By establishing a solid foundation, we were able to build upon these concepts in subsequent sections, ensuring a coherent and logical progression of ideas.
Next, we delved into the historical context and evolution of the topic. This involved tracing its origins, significant milestones, and key developments over time. Understanding the historical trajectory allowed us to appreciate the current state of the field and anticipate future trends and challenges.
We then examined the various methodologies and approaches employed in the study and application of the topic. This included a detailed analysis of different research methods, experimental designs, and analytical techniques. By comparing and contrasting these approaches, we were able to identify their respective strengths and limitations, providing valuable insights for future research and practice.
Furthermore, we discussed the practical implications and real-world applications of the topic. This involved exploring how the theoretical concepts and methodologies are translated into practice, and the impact they have on various industries and sectors. By highlighting specific case studies and examples, we were able to illustrate the tangible benefits and challenges associated with the topic.
In addition, we addressed the ethical considerations and societal implications of the topic. This included a critical examination of the potential risks, benefits, and ethical dilemmas that arise in the context of its application. By engaging with these complex issues, we aimed to foster a more nuanced and responsible approach to the topic.
Finally, we identified the key challenges and future directions for the field. This involved highlighting the current gaps in knowledge, emerging trends, and potential areas for further research and development. By outlining these future directions, we aimed to provide a roadmap for advancing the field and addressing the pressing issues that lie ahead.
In summary, this recap of key points serves as a comprehensive overview of the main themes and insights discussed throughout the document. By revisiting these essential elements, we aim to reinforce the reader's understanding and appreciation of the topic, setting the stage for the final thoughts and future outlook sections.
13.2. Final Thoughts
As we reach the conclusion of this document, it is important to reflect on the overarching themes and insights that have emerged from our exploration of the topic. The journey through the various sections has provided a rich tapestry of information, analysis, and critical reflection, culminating in a deeper understanding of the subject matter.
One of the key takeaways from our discussion is the importance of a multidisciplinary approach to the topic. The complexity and multifaceted nature of the subject necessitate drawing on diverse perspectives and expertise. By integrating insights from different fields, we can develop a more holistic and nuanced understanding, which is essential for addressing the challenges and opportunities that lie ahead.
Another significant insight is the dynamic and evolving nature of the field. The rapid pace of technological advancements, coupled with shifting societal and economic landscapes, means that the topic is in a constant state of flux. This underscores the need for continuous learning, adaptability, and innovation. Staying abreast of the latest developments and being open to new ideas and approaches will be crucial for navigating the future.
Ethical considerations have also emerged as a central theme in our discussion. The application of the topic has far-reaching implications for individuals, communities, and society at large. It is imperative that we approach the subject with a strong ethical compass, ensuring that our actions and decisions are guided by principles of fairness, justice, and respect for human dignity. This involves not only adhering to established ethical standards but also engaging in ongoing dialogue and reflection to address emerging ethical dilemmas.
Furthermore, the practical applications and real-world impact of the topic cannot be overstated. The insights and knowledge gained from our exploration have the potential to drive significant advancements and improvements across various sectors. Whether it is in healthcare, education, business, or public policy, the application of the topic can lead to tangible benefits and positive change. However, this also requires a concerted effort to bridge the gap between theory and practice, ensuring that the insights are effectively translated into actionable strategies and solutions.
In conclusion, the final thoughts section serves as a synthesis of the key themes and insights that have emerged from our exploration of the topic. It highlights the importance of a multidisciplinary approach, the dynamic nature of the field, the centrality of ethical considerations, and the practical applications and impact of the topic. As we move forward, it is essential to carry these insights with us, guiding our actions and decisions in the pursuit of knowledge, innovation, and positive change.
13.3. Future Outlook
Looking ahead, the future outlook for the topic is both promising and challenging. The rapid pace of technological advancements, coupled with evolving societal and economic landscapes, presents a unique set of opportunities and challenges that will shape the trajectory of the field.
One of the most significant trends that will influence the future of the topic is the continued advancement of technology. Innovations in areas such as artificial intelligence, machine learning, and data analytics are poised to revolutionize the field, offering new tools and capabilities for research, analysis, and application. These technological advancements have the potential to drive significant improvements in efficiency, accuracy, and scalability, opening up new possibilities for the field.
However, the integration of these technologies also presents a set of challenges. Ensuring that these technologies are used ethically and responsibly will be paramount. This involves addressing issues such as data privacy, algorithmic bias, and the potential for misuse. Developing robust ethical frameworks and guidelines, as well as fostering a culture of ethical awareness and accountability, will be crucial for navigating these challenges.
Another key trend that will shape the future of the topic is the increasing emphasis on interdisciplinary collaboration. The complexity and multifaceted nature of the field necessitate drawing on diverse perspectives and expertise. By fostering collaboration across different disciplines, we can develop more holistic and nuanced solutions to the challenges we face. This will require breaking down silos, promoting open communication, and creating opportunities for cross-disciplinary engagement.
The future outlook also involves addressing the current gaps in knowledge and research. There are still many unanswered questions and areas that require further exploration. Identifying these gaps and prioritizing research efforts will be essential for advancing the field. This includes not only conducting rigorous and innovative research but also ensuring that the findings are effectively disseminated and translated into practice.
Furthermore, the future of the topic will be shaped by the evolving societal and economic landscapes. Issues such as globalization, demographic shifts, and changing economic conditions will have a significant impact on the field. Understanding and anticipating these trends will be crucial for developing strategies and solutions that are relevant and effective in the future context.
In conclusion, the future outlook for the topic is characterized by a dynamic interplay of opportunities and challenges. Technological advancements, interdisciplinary collaboration, addressing research gaps, and understanding evolving societal and economic trends will be key factors shaping the future of the field. By staying abreast of these trends and proactively addressing the associated challenges, we can harness the potential of the topic to drive positive change and innovation in the years to come.