Table Of Contents
Category
Artificial Intelligence
AIML
IoT
Blockchain
Customer Service
Retail & Ecommerce
Security
1. What are Enterprise AI Copilots and How Do They Work?
Enterprise AI Copilots are advanced artificial intelligence systems designed to assist organizations in various tasks, enhancing productivity and decision-making. They leverage machine learning, natural language processing, and data analytics to provide real-time insights and recommendations tailored to specific business needs. By integrating with existing enterprise software, these enterprise AI copilots analyze vast amounts of data and learn from user interactions. They can automate repetitive tasks, generate reports, and even assist in customer service by providing instant responses to queries. Utilizing predictive analytics, they can forecast trends and suggest actions, enabling businesses to stay ahead of the competition.
1.1. What Makes an AI Copilot Different from Traditional Enterprise Software?
AI Copilots differ significantly from traditional enterprise software in several key aspects:
- Intelligence and Adaptability: Unlike traditional software that follows predefined rules, AI copilots learn from user behavior and adapt their responses accordingly. This allows for a more personalized experience.
- Real-Time Insights: AI copilots provide real-time data analysis and insights, whereas traditional software often requires manual data processing and reporting.
- Automation of Complex Tasks: While traditional software can automate simple tasks, AI copilots can handle complex workflows, making them more efficient in managing business processes.
- User Interaction: AI copilots utilize natural language processing to interact with users in a conversational manner, making it easier for non-technical users to engage with the system.
1.2. What are the Core Components of Enterprise-Grade AI Copilots?
The effectiveness of enterprise AI copilots relies on several core components:
- Natural Language Processing (NLP): This allows the AI to understand and respond to user queries in a human-like manner, facilitating seamless communication.
- Machine Learning Algorithms: These algorithms enable the AI to learn from data and improve its performance over time, ensuring that it remains relevant and effective.
- Data Integration: AI copilots must integrate with various data sources, including CRM systems, databases, and cloud services, to provide comprehensive insights.
- User Interface (UI): A user-friendly interface is crucial for ensuring that users can easily interact with the AI copilot, making it accessible to all employees, regardless of their technical expertise.
- Security and Compliance: Given the sensitive nature of enterprise data, AI copilots must adhere to strict security protocols and compliance regulations to protect information.
To implement an enterprise AI copilot, organizations can follow these steps:
- Identify specific business needs and areas where AI can add value.
- Choose the right AI copilot solution that aligns with organizational goals.
- Integrate the AI copilot with existing systems and data sources.
- Train employees on how to effectively use the AI copilot.
- Continuously monitor and evaluate the AI copilot's performance, making adjustments as necessary.
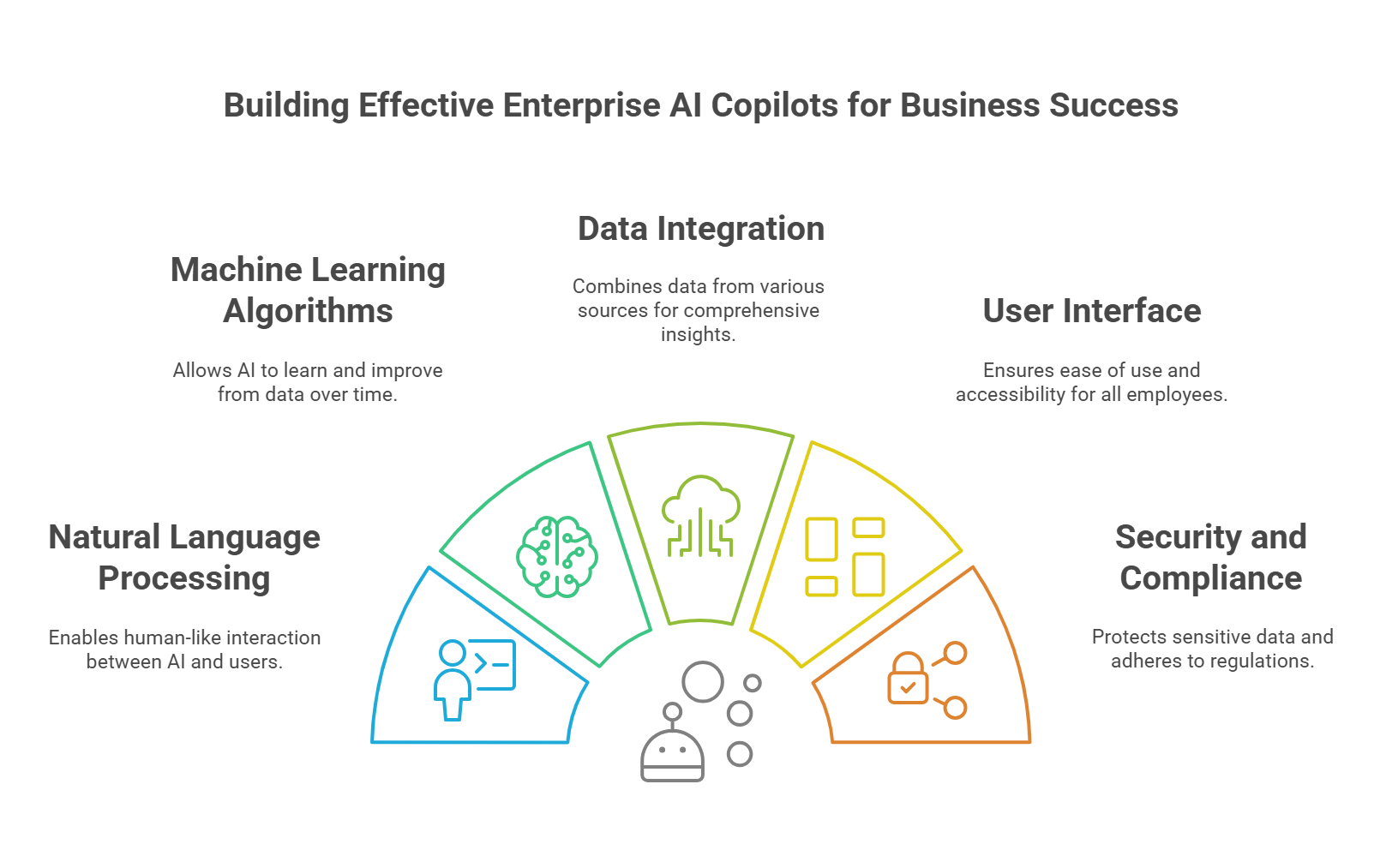
By understanding the capabilities and components of enterprise AI copilots, organizations can leverage this technology to enhance their operations and drive innovation. At Rapid Innovation, we specialize in developing and implementing AI copilot solutions tailored to your unique business needs, ensuring you achieve greater ROI and operational efficiency.
1.3. ROI Impact of AI Copilots on Business Operations
The integration of AI copilots into business operations can significantly enhance return on investment (ROI). AI copilots streamline processes, improve decision-making, and increase productivity, leading to measurable financial benefits.
- Increased Efficiency: AI copilots automate repetitive tasks, allowing employees to focus on higher-value activities. This can lead to a reduction in operational costs. For instance, companies that implement AI integration solutions can see a productivity increase of up to 40% (source: McKinsey).
- Enhanced Decision-Making: AI copilots analyze vast amounts of data quickly, providing insights that help businesses make informed decisions. This can lead to better resource allocation and strategic planning, ultimately driving revenue growth.
- Improved Customer Experience: By utilizing AI copilots, businesses can offer personalized services and faster response times, enhancing customer satisfaction. Satisfied customers are more likely to return, increasing lifetime value.
- Scalability: AI copilots can easily scale operations without a proportional increase in costs. This flexibility allows businesses to adapt to market changes swiftly.
- Data-Driven Insights: AI copilots provide analytics that can identify trends and opportunities, enabling businesses to pivot strategies effectively. Companies leveraging data analytics can achieve a 5-6% increase in productivity (source: Deloitte).
2. Planning Your Enterprise AI Copilot Strategy
Developing a robust AI copilot strategy is essential for maximizing the benefits of AI in business operations. A well-planned strategy ensures alignment with organizational goals and effective implementation.

- Define Objectives: Clearly outline what you aim to achieve with AI copilots. This could include improving efficiency, enhancing customer service, or driving innovation.
- Identify Use Cases: Determine specific areas within your organization where AI copilots can add value. Common use cases include customer support, data analysis, and project management.
- Engage Stakeholders: Involve key stakeholders from various departments to gather insights and ensure buy-in. This collaborative approach fosters a culture of innovation.
- Choose the Right Technology: Evaluate different AI technologies and platforms to find the best fit for your organization’s needs. Consider factors such as scalability, integration capabilities, and user-friendliness.
- Develop a Roadmap: Create a detailed implementation plan that outlines timelines, resources, and milestones. This roadmap will guide your organization through the AI adoption process.
- Monitor and Adjust: Continuously assess the performance of AI copilots and make necessary adjustments. Regular feedback loops will help optimize their effectiveness.
2.1. Assessing Organizational AI Readiness
Before implementing AI copilots, it’s crucial to assess your organization’s readiness for AI adoption. This evaluation helps identify potential challenges and areas for improvement.
- Evaluate Current Infrastructure: Assess your existing technology stack to determine if it can support AI initiatives. This includes hardware, software, and data management systems.
- Analyze Data Quality: High-quality data is essential for effective AI performance. Evaluate the accuracy, completeness, and relevance of your data sources.
- Assess Skills and Expertise: Identify gaps in your team’s AI knowledge and skills. Consider investing in training programs or hiring experts to bridge these gaps.
- Cultural Readiness: Gauge your organization’s openness to change and innovation. A culture that embraces technology will facilitate smoother AI adoption.
- Regulatory Compliance: Ensure that your organization is aware of and compliant with relevant regulations regarding data privacy and AI usage.
- Pilot Programs: Consider running pilot programs to test AI copilots in a controlled environment. This allows you to gather insights and make necessary adjustments before a full-scale rollout.
By carefully planning and assessing readiness, organizations can effectively leverage AI copilots to enhance business operations and drive ROI. Rapid Innovation is here to assist you in this journey, providing tailored AI copilot strategies that align with your business objectives and maximize your investment.
2.2. Which Use Cases are Best Suited for AI Implementation?
AI implementation can significantly enhance various business processes. Here are some of the most suitable use cases:
- Customer Support Automation: AI customer support chatbots can handle customer inquiries 24/7, providing instant responses and reducing the workload on human agents. This leads to improved customer satisfaction and operational efficiency, ultimately driving higher retention rates and loyalty.
- Predictive Analytics: Businesses can leverage AI to analyze historical data and predict future trends. This is particularly useful in sectors like retail, where understanding customer behavior can drive sales strategies, leading to increased revenue and market share.
- Fraud Detection: Financial institutions utilize AI algorithms to detect unusual patterns in transactions, helping to identify and prevent fraudulent activities in real-time. This not only protects assets but also enhances customer trust and reduces financial losses.
- Personalization: AI can analyze user data to deliver personalized recommendations, enhancing user experience in e-commerce and content platforms. This tailored approach can significantly boost conversion rates and customer satisfaction.
- Supply Chain Optimization: AI can forecast demand, optimize inventory levels, and streamline logistics, leading to cost savings and improved service delivery. Efficient supply chain management can result in reduced operational costs and increased profitability.
- Healthcare Diagnostics: AI systems can analyze medical images and patient data to assist in diagnosing diseases, improving accuracy and speed in healthcare delivery. This can lead to better patient outcomes and reduced healthcare costs.
2.3. How to Measure AI Copilot Success?
Measuring the success of AI copilots involves several key performance indicators (KPIs) that reflect their impact on business operations:
- User Adoption Rate: Track how many users are actively engaging with the AI copilot. A high adoption rate indicates that users find the tool valuable.
- Task Completion Time: Measure the time taken to complete tasks with and without the AI copilot. A reduction in time signifies improved efficiency.
- Error Rate: Monitor the frequency of errors in tasks performed with the AI copilot. A decrease in errors suggests that the AI is enhancing accuracy.
- User Satisfaction Scores: Conduct surveys to gauge user satisfaction with the AI copilot. Positive feedback can indicate successful implementation.
- Return on Investment (ROI): Calculate the financial benefits gained from using the AI copilot against the costs incurred. A positive ROI demonstrates the tool's value to the organization.
- Business Impact Metrics: Assess broader business metrics such as revenue growth, customer retention, and operational costs to understand the AI copilot's overall impact.
3. Technical Architecture of Enterprise AI Copilots
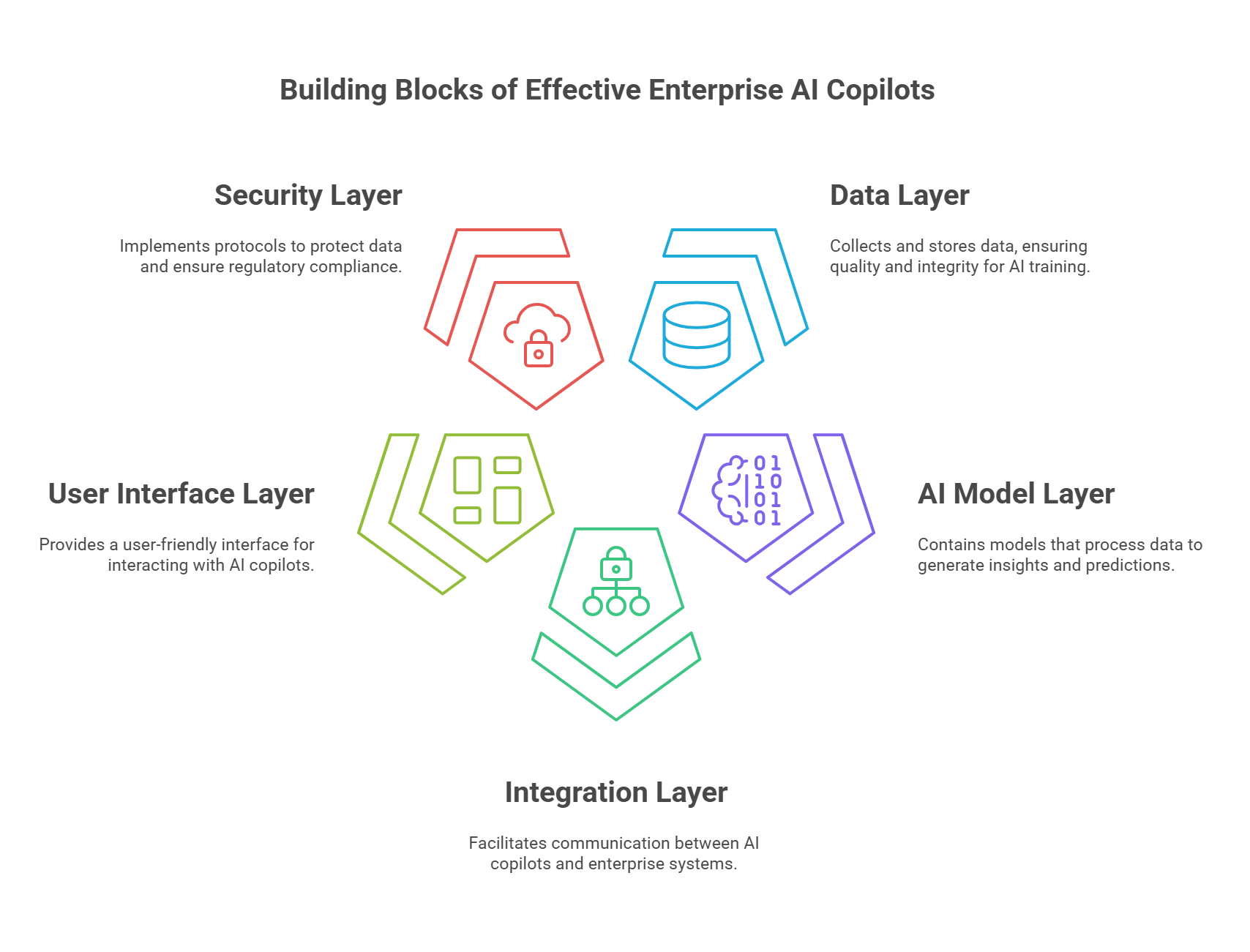
The technical architecture of enterprise AI copilots is crucial for their effective deployment and operation. Here’s a breakdown of the components involved:
- Data Layer: This layer collects and stores data from various sources, including databases, APIs, and user interactions. It ensures data quality and integrity for accurate AI model training.
- AI Model Layer: This layer contains machine learning and deep learning models that process data and generate insights. Models are trained on historical data to improve their predictive capabilities.
- Integration Layer: This layer facilitates communication between the AI copilot and existing enterprise systems (e.g., CRM, ERP). It ensures seamless data flow and interoperability.
- User Interface Layer: This layer provides a user-friendly interface for interaction with the AI copilot. It can include chat interfaces, dashboards, or voice-activated systems.
- Feedback Loop: This component incorporates user feedback to continuously improve AI models and allows for real-time adjustments based on user interactions and outcomes.
- Security Layer: This layer implements security protocols to protect sensitive data and ensure compliance with regulations. It includes authentication, encryption, and access controls.
By understanding these components, organizations can effectively design and implement AI copilots that enhance productivity and decision-making. At Rapid Innovation, we specialize in guiding businesses through the AI implementation process, ensuring that they achieve their goals efficiently and effectively while maximizing their return on investment.
3.1. Foundation Models and Large Language Model Integration
Foundation models are large-scale machine learning models trained on vast amounts of data, enabling them to perform a variety of tasks. Large Language Models (LLMs) are a subset of foundation models specifically designed for understanding and generating human language. Integrating these models into applications can significantly enhance their capabilities, driving efficiency and effectiveness in achieving business goals.
- Understanding Foundation Models: They are pre-trained on diverse datasets, allowing them to generalize across multiple tasks. Examples include GPT-3, BERT, and T5, which can perform tasks like translation, summarization, and question-answering. Rapid Innovation leverages these models to tailor solutions that meet specific client needs, ensuring a higher return on investment (ROI).
- Benefits of LLM Integration:
- Improved natural language understanding and generation, leading to more accurate and relevant outputs.
- Ability to handle context and nuances in human language, which enhances communication and interaction.
- Enhanced user experience through conversational interfaces, making applications more intuitive and user-friendly.
- Integration Steps:
- Identify the specific use case for the salesforce copilot, aligning it with business objectives.
- Choose an appropriate foundation model based on the task requirements, ensuring it fits the operational context.
- Fine-tune the model on domain-specific data to improve performance, maximizing the effectiveness of the solution.
- Implement the model using APIs or SDKs provided by platforms like OpenAI or Hugging Face, facilitating seamless integration into existing systems.
3.2. What Security Framework Does Your AI Copilot Need?
As AI copilots become integral to business operations, ensuring their security is paramount. A robust security framework protects sensitive data and maintains user trust, which is essential for long-term success.
- Key Components of a Security Framework:
- Data Privacy: Implement measures to protect user data, ensuring compliance with regulations like GDPR and CCPA, which is critical for maintaining customer trust.
- Access Control: Use role-based access control (RBAC) to limit who can interact with the AI copilot and what data they can access, safeguarding sensitive information.
- Model Security: Protect the integrity of the AI model against adversarial attacks and ensure it cannot be easily reverse-engineered, preserving the competitive advantage of your solutions.
- Best Practices:
- Regularly update and patch systems to mitigate vulnerabilities, ensuring ongoing protection against emerging threats.
- Conduct security audits and penetration testing to identify weaknesses, allowing for proactive risk management.
- Implement encryption for data at rest and in transit, reinforcing the security posture of your AI applications.
3.3. How to Connect AI Copilots with Existing Systems?
Integrating AI copilots with existing systems is crucial for maximizing their utility and ensuring seamless workflows. This process involves several technical steps that Rapid Innovation can expertly navigate.
- Assessment of Existing Systems:
- Evaluate current infrastructure and identify integration points, ensuring compatibility and efficiency.
- Determine the data flow between the AI copilot and existing systems, facilitating smooth interactions and data exchange.
- Integration Steps:
- API Development: Create APIs that allow the AI copilot to communicate with existing applications, enabling interoperability and functionality.
- Data Synchronization: Ensure that data is consistently updated between the AI copilot and other systems, maintaining accuracy and relevance.
- Testing: Conduct thorough testing to ensure that the integration works as intended and does not disrupt existing workflows, safeguarding operational continuity.
- Tools and Technologies:
- Use middleware solutions like Apache Kafka or RabbitMQ for real-time data processing, enhancing responsiveness and performance.
- Leverage cloud services (e.g., AWS, Azure) for scalable integration solutions, allowing for flexibility and growth.
By focusing on these areas, organizations can effectively leverage foundation models and LLMs, implement necessary security frameworks, and ensure smooth integration with existing systems. Rapid Innovation is committed to helping clients enhance the functionality and reliability of AI copilot development, ultimately driving greater ROI and achieving business goals efficiently and effectively.
4. How to Implement an AI Copilot Step by Step?
Implementing an AI copilot involves a systematic approach that includes building a robust knowledge base, creating a training dataset, and developing context-aware response systems. Here’s a detailed breakdown of the steps involved.
4.1. Building the Knowledge Base and Training Dataset
A well-structured knowledge base is crucial for the effectiveness of an AI copilot implementation. It serves as the foundation for the AI's understanding and response capabilities.
- Identify the domain: Determine the specific area or industry your AI copilot will serve, such as healthcare, finance, or customer support.
- Gather data: Collect relevant data from various sources, including:
- Internal documents: manuals, FAQs
- External resources: websites, research papers
- User interactions: chat logs, feedback
- Organize the data: Structure the collected data into a coherent format. This may involve:
- Categorizing information by topics
- Creating a taxonomy for easy navigation
- Ensuring data is up-to-date and relevant
- Clean the dataset: Remove any irrelevant or duplicate information to enhance the quality of the training dataset. This can include:
- Filtering out noise
- Standardizing formats
- Validating data accuracy
- Annotate the data: Label the data to provide context for the AI. This can involve:
- Tagging entities: names, dates, locations
- Classifying intents: questions, commands
- Highlighting relationships between data points
- Train the AI model: Use the cleaned and annotated dataset to train your AI model. This involves:
- Selecting appropriate algorithms: e.g., supervised learning, reinforcement learning
- Splitting the dataset into training, validation, and test sets
- Iteratively refining the model based on performance metrics
4.2. How to Develop Context-Aware Response Systems?
Context-aware response systems enhance the AI copilot's ability to provide relevant and timely responses based on user interactions and situational context.
- Define context parameters: Identify the key factors that influence context, such as:
- User history: previous interactions, preferences
- Current environment: location, device
- Temporal factors: time of day, urgency
- Implement natural language processing (NLP): Utilize NLP techniques to understand user inputs better. This includes:
- Tokenization: breaking down sentences into words or phrases
- Named entity recognition: identifying and classifying key information
- Sentiment analysis: understanding the emotional tone of user messages
- Create a context management system: Develop a system that tracks and manages context throughout the interaction. This can involve:
- Storing user profiles and interaction history
- Updating context dynamically based on new inputs
- Retrieving relevant information from the knowledge base
- Design response generation mechanisms: Ensure the AI can generate contextually appropriate responses. This may include:
- Using templates for common queries
- Implementing machine learning models for dynamic response generation
- Incorporating fallback strategies for ambiguous queries
- Test and refine: Continuously evaluate the performance of the context-aware response system. This can involve:
- Conducting user testing to gather feedback
- Analyzing response accuracy and relevance
- Iterating on the design based on insights gained

By following these steps, you can effectively implement an AI copilot that is both knowledgeable and context-aware, ultimately enhancing user experience and operational efficiency. At Rapid Innovation, we specialize in guiding organizations through this AI copilot implementation process, ensuring that your AI copilot is tailored to meet your specific business needs and objectives, thereby maximizing your return on investment.
4.3. How to Create Custom Enterprise Workflows?
Creating custom enterprise workflows is essential for optimizing business processes and enhancing productivity. A well-defined workflow can streamline operations, reduce errors, and improve collaboration among teams. Here’s how to create custom enterprise workflows effectively:
- Identify Objectives: Determine the specific goals you want to achieve with the workflow. This could include reducing processing time, improving communication, or increasing compliance.
- Map Existing Processes: Analyze current workflows to identify bottlenecks and inefficiencies. Use flowcharts or diagrams to visualize the steps involved.
- Engage Stakeholders: Involve team members who will be using the workflow. Gather their input to ensure the new process meets their needs and addresses pain points.
- Select Workflow Tools: Choose appropriate software or tools that support workflow automation. Popular options include Microsoft Power Automate, Asana, and Trello. At Rapid Innovation, we can assist in selecting and integrating the right tools tailored to your specific business needs, ensuring a seamless transition to automated custom enterprise workflows.
- Design the Workflow: Create a detailed design of the workflow, including all tasks, decision points, and responsible parties. Ensure clarity in roles and responsibilities.
- Implement the Workflow: Roll out the new workflow in phases, if possible. This allows for adjustments based on feedback and minimizes disruption. Our team at Rapid Innovation can guide you through this implementation phase, leveraging our expertise in AI and Blockchain to enhance the efficiency of your custom enterprise workflows.
- Train Users: Provide training sessions for all users to familiarize them with the new workflow. Ensure they understand how to use the tools effectively.
- Monitor and Optimize: After implementation, continuously monitor the workflow’s performance. Use metrics to assess efficiency and make necessary adjustments. Rapid Innovation can help you set up monitoring systems that utilize AI analytics to provide insights into workflow performance, enabling data-driven optimizations. For more information on ensuring data privacy in these workflows, check out our best practices for AI data privacy.
5. Security and Compliance Considerations
In today’s digital landscape, security and compliance are paramount for enterprises, especially when dealing with sensitive data. Organizations must adopt robust measures to protect information and adhere to regulations. Here are key considerations:
- Data Encryption: Implement encryption protocols for data at rest and in transit. This ensures that sensitive information is protected from unauthorized access.
- Access Controls: Establish strict access controls to limit who can view or modify sensitive data. Use role-based access control (RBAC) to enforce these restrictions.
- Regular Audits: Conduct regular security audits and compliance checks to identify vulnerabilities and ensure adherence to regulations such as GDPR or HIPAA.
- Incident Response Plan: Develop a comprehensive incident response plan to address potential data breaches or security incidents swiftly.
- Employee Training: Provide ongoing training for employees on security best practices and compliance requirements. This helps create a culture of security awareness.
5.1. How to Ensure Data Privacy in AI Copilots?
As AI copilots become integral to custom enterprise workflows, ensuring data privacy is crucial. Here are strategies to maintain data privacy while leveraging AI technology:
- Data Minimization: Collect only the data necessary for the AI to function effectively. Avoid excessive data collection that could lead to privacy concerns.
- Anonymization Techniques: Use anonymization or pseudonymization techniques to protect personal data. This reduces the risk of identifying individuals from the data used by AI systems.
- User Consent: Obtain explicit consent from users before collecting or processing their data. Clearly communicate how their data will be used and stored.
- Regular Privacy Assessments: Conduct regular assessments of AI systems to evaluate their compliance with data privacy regulations. Adjust practices as necessary to align with evolving laws.
- Transparency: Maintain transparency with users regarding data usage. Provide clear information about how AI copilots operate and the data they access.
By following these guidelines, organizations can create effective custom enterprise workflows while ensuring security and compliance, particularly in the context of AI copilots. Rapid Innovation is here to support you in implementing these strategies, ensuring that your workflows are not only efficient but also secure and compliant with industry standards.
5.2. Regulatory Compliance and Industry Standards
Regulatory compliance and adherence to industry standards are critical for organizations, especially in sectors like finance, healthcare, and technology. Compliance ensures that businesses operate within legal frameworks, protecting them from penalties and enhancing their reputation.
- Understanding Regulations: Familiarize yourself with relevant regulations such as GDPR, HIPAA, and PCI-DSS. Each regulation has specific requirements that organizations must meet to ensure data protection and privacy.
- Implementing Policies: Develop and implement internal policies that align with regulatory requirements, including data handling, storage, and sharing protocols.
- Regular Audits: Conduct regular audits to assess compliance with industry standards, helping to identify gaps and areas for improvement.
- Training and Awareness: Provide training for employees on compliance requirements and best practices to ensure that everyone understands their role in maintaining regulatory compliance and access control.
- Documentation: Maintain thorough documentation of compliance efforts, including policies, training records, and audit results, which is essential for demonstrating compliance during inspections.
- Utilizing Technology: Leverage compliance management software to automate tracking and reporting, streamlining processes and reducing the risk of human error. Rapid Innovation can assist in implementing tailored compliance solutions that integrate seamlessly with your existing systems, ensuring that you meet regulatory requirements efficiently. For more insights on the advantages and future of generative AI in finance and banking applications, visit this link.
5.3. Access Control and User Authentication Systems
Access control and user authentication systems are vital for safeguarding sensitive information and ensuring that only authorized personnel can access specific resources.
- Role-Based Access Control (RBAC): Implement RBAC to assign permissions based on user roles, minimizing the risk of unauthorized access by ensuring users only have access to the information necessary for their job functions.
- Multi-Factor Authentication (MFA): Use MFA to enhance security, requiring users to provide two or more verification factors, such as a password and a fingerprint, before gaining access.
- Regular Access Reviews: Conduct periodic reviews of user access rights to ensure that permissions are up-to-date and reflect current job responsibilities.
- Logging and Monitoring: Implement logging and monitoring systems to track access attempts and identify suspicious activities, helping to quickly respond to potential security breaches.
- User Education: Educate users about the importance of strong passwords and secure authentication practices, encouraging the use of password managers to help manage complex passwords.
- Integration with Identity Management Systems: Consider integrating access control systems with identity management solutions to streamline user provisioning and de-provisioning processes.
6. How to Integrate AI Copilots with Enterprise Systems

Integrating AI copilots with enterprise systems can enhance productivity and streamline workflows. Here’s how to achieve this integration effectively:
- Identify Use Cases: Determine specific use cases where AI copilots can add value, such as automating repetitive tasks, providing data insights, or enhancing customer support.
- Choose the Right AI Copilot: Select an AI copilot that aligns with your enterprise needs, considering factors like compatibility with existing systems, scalability, and user-friendliness.
- API Integration: Utilize APIs to connect the AI copilot with your enterprise systems, allowing for seamless data exchange and functionality.
- Data Preparation: Ensure that the data used by the AI copilot is clean, structured, and relevant, enhancing the accuracy and effectiveness of the AI's outputs.
- User Training: Provide training for employees on how to effectively use the AI copilot, including understanding its capabilities and limitations.
- Monitor Performance: Continuously monitor the performance of the AI copilot to assess its impact on productivity and identify areas for improvement.
- Feedback Loop: Establish a feedback loop to gather user input on the AI copilot’s performance, using this feedback to make necessary adjustments and enhancements.
By focusing on regulatory compliance and access control, as well as the integration of AI copilots, organizations can create a secure and efficient operational environment. Rapid Innovation is committed to helping clients navigate these complexities, ensuring that they achieve their business goals effectively and efficiently while maximizing their return on investment.
6.1. Seamless Integration with Existing Enterprise Tools
Integrating AI solutions into existing enterprise tools, such as ai system integration and ai integration solutions, is crucial for maximizing productivity and ensuring a smooth transition. Seamless integration allows organizations to leverage their current systems while enhancing functionality with AI capabilities.
- Identify existing tools: Assess the current software and platforms in use, such as CRM, ERP, or project management tools.
- Evaluate compatibility: Check if the AI solution can easily connect with these tools through APIs or plugins.
- Implement middleware: Use middleware solutions to bridge gaps between systems, ensuring data flows smoothly.
- Conduct user training: Provide training sessions for employees to familiarize them with the integrated system.
- Monitor performance: Regularly assess the integration's effectiveness and make adjustments as needed.
By ensuring seamless AI integration, organizations can enhance user experience and improve overall efficiency, ultimately leading to a greater return on investment (ROI).
6.2. Custom API Development and Documentation
Custom API development is essential for tailoring AI solutions to meet specific business needs. Well-documented APIs facilitate easier integration and usage, allowing developers to understand and implement the functionalities effectively.
- Define requirements: Gather input from stakeholders to determine the necessary features and functionalities of the API.
- Design the API: Create a blueprint that outlines endpoints, data formats, and authentication methods.
- Develop the API: Use programming languages like
Python
orJavaScript
to build the API, ensuring it meets the defined requirements. - Write comprehensive documentation: Include clear instructions, code examples, and use cases to help developers understand how to use the API effectively.
- Test the API: Conduct thorough testing to identify and fix any bugs or performance issues before deployment.
Custom APIs not only enhance the functionality of AI solutions but also ensure that they align with the unique needs of the organization, thereby driving efficiency and maximizing ROI.
6.3. How to Scale Your AI Copilot Performance?
Scaling AI copilot performance is vital for organizations looking to enhance their operational efficiency and user experience. Here are some strategies to achieve this:
- Optimize algorithms: Regularly update and refine the algorithms used by the AI copilot to improve accuracy and response times.
- Increase data input: Feed the AI copilot with more diverse and extensive datasets to enhance its learning capabilities.
- Implement feedback loops: Create mechanisms for users to provide feedback, allowing the AI to learn from real-world interactions.
- Utilize cloud infrastructure: Leverage cloud services to ensure that the AI copilot can handle increased workloads and user demands.
- Monitor performance metrics: Track key performance indicators (KPIs) to identify areas for improvement and ensure the AI copilot meets user expectations.
By focusing on these strategies, organizations can effectively scale their AI copilot performance, leading to improved productivity and user satisfaction, which translates into a higher ROI for their investments in AI technology.
7. How to Test and Verify AI Copilot Performance?
Testing and verifying the performance of AI Copilots is crucial to ensure they meet user expectations and function effectively in real-world scenarios. This process involves various methodologies and frameworks tailored to assess the unique characteristics of AI systems, including ai copilot testing frameworks.
7.1. Which Testing Frameworks Work Best for AI Systems?
Selecting the right testing framework is essential for evaluating AI systems. Here are some of the most effective frameworks:
- TensorFlow Extended (TFX): Designed for production ML pipelines, it supports model validation, data validation, and model serving.
- MLflow: An open-source platform for managing the ML lifecycle, it facilitates tracking experiments, packaging code, and sharing models.
- Pytest: A testing framework for Python that can be adapted for AI applications, allowing for writing simple as well as scalable test cases.
- Apache Airflow: Useful for orchestrating complex workflows in AI projects, it helps in scheduling and monitoring tasks.
- KubeFlow: A Kubernetes-native platform for deploying ML workflows, it supports testing and scaling of AI models in cloud environments.
When choosing a framework, consider factors such as ease of integration, community support, and specific features that align with your AI Copilot's requirements. At Rapid Innovation, we assist clients in selecting and implementing the most suitable ai copilot testing frameworks to enhance their AI capabilities, ultimately driving greater ROI.
7.2. User Acceptance Testing Methodologies
User Acceptance Testing (UAT) is vital for ensuring that the AI Copilot meets user needs and expectations. Here are some methodologies to consider:
- Alpha Testing: Conducted by internal teams before releasing the product to external users, it focuses on identifying bugs and usability issues.
- Beta Testing: Involves a select group of external users who test the AI Copilot in real-world scenarios, providing valuable feedback on performance and user experience.
- A/B Testing: Compares two versions of the AI Copilot to determine which performs better, helping in making data-driven decisions based on user interactions.
- Surveys and Feedback Forms: Collect qualitative and quantitative data from users regarding their experience, which can be integrated into the AI Copilot interface for real-time feedback.
- Focus Groups: Gather a diverse group of users to discuss their experiences and expectations, providing insights into user perceptions and potential improvements.
To effectively implement UAT methodologies, follow these steps:
- Define clear objectives for the testing phase.
- Select a representative group of users for testing.
- Develop test cases that cover various scenarios and use cases.
- Collect and analyze feedback systematically.
- Iterate on the AI Copilot based on user insights.
By employing the right testing frameworks and UAT methodologies, organizations can ensure that their AI Copilot performs optimally and meets user expectations. This not only enhances user satisfaction but also contributes to the overall success of the AI system. Rapid Innovation is committed to guiding clients through this process, ensuring that their AI solutions are robust, user-friendly, and aligned with business goals, ultimately leading to improved ROI.
7.3. Performance Benchmarking and Optimization
Performance benchmarking and optimization are critical for ensuring that AI systems, such as AI copilot deployment, operate efficiently and effectively. This process involves measuring the performance of the AI system against established standards and making necessary adjustments to enhance its capabilities.
- Identify Key Performance Indicators (KPIs): Define what success looks like for your AI copilot. Common KPIs include response time, accuracy, user satisfaction, and resource utilization.
- Conduct Benchmark Tests: Use standardized datasets to evaluate the AI's performance. This can include load testing to assess how the system performs under heavy usage and stress testing to determine the system's limits.
- Analyze Results: Compare the results against industry standards or previous versions of the AI copilot. Look for areas of improvement, such as latency issues and inaccurate predictions or responses.
- Optimize Algorithms: Fine-tune the AI algorithms based on the analysis. Techniques may include adjusting hyperparameters and implementing more efficient data processing methods.
- Monitor Performance Continuously: Set up a monitoring system to track performance in real-time. This allows for quick identification of issues and ongoing optimization.
- User Feedback Loop: Incorporate user feedback to understand real-world performance. This can help identify usability issues that may not be apparent in testing.
8. How to Deploy an AI Copilot Successfully?

Deploying an AI copilot requires careful planning and execution to ensure that it meets user needs and integrates seamlessly into existing workflows. Here are key steps to consider:
- Define Objectives and Use Cases: Clearly outline the goals of the AI copilot. Identify specific use cases where it can add value, such as automating repetitive tasks and providing real-time insights.
- Engage Stakeholders Early: Involve key stakeholders from various departments to gather input and ensure buy-in. This can include IT teams, end-users, and management.
- Develop a Comprehensive Training Plan: Provide training sessions for users to familiarize them with the AI copilot's features and functionalities. Consider hands-on workshops and online tutorials.
- Implement Robust Testing: Before full deployment, conduct extensive testing to identify and resolve any issues. This includes user acceptance testing (UAT) and pilot programs with select user groups.
- Establish Support Mechanisms: Set up a support system to assist users post-deployment. This can include help desks and online resources and FAQs.
- Measure Success Post-Deployment: After deployment, continuously measure the AI copilot's performance against the defined KPIs. Use this data to make iterative improvements.
8.1. Phased Roll-out Strategy for Enterprise Adoption
A phased roll-out strategy can facilitate smoother adoption of an AI copilot deployment within an enterprise. This approach allows for gradual integration, minimizing disruption and enabling adjustments based on feedback.
- Phase 1: Pilot Program: Select a small group of users to test the AI copilot in a controlled environment. Gather feedback and make necessary adjustments.
- Phase 2: Expand to Early Adopters: Roll out the AI copilot to a larger group of enthusiastic users who can provide valuable insights and advocate for its benefits.
- Phase 3: Full Deployment: Once the AI copilot has been refined based on earlier phases, deploy it across the organization. Ensure that all users have access to training and support.
- Phase 4: Continuous Improvement: After full deployment, maintain a focus on continuous improvement. Regularly solicit user feedback and monitor performance to identify areas for enhancement.
By following these steps, organizations can effectively benchmark, optimize, and deploy AI copilots, ensuring they deliver maximum value and enhance productivity. Rapid Innovation is committed to guiding clients through this process, leveraging our expertise in AI and Blockchain to help you achieve greater ROI and operational efficiency.
8.2. Employee Training and Onboarding Programs
Effective employee training and onboarding programs, such as management training for managers and supervisor training, are crucial for integrating new hires into an organization, especially when implementing advanced technologies like AI copilots. A well-structured program can enhance productivity, reduce turnover, and ensure that employees are equipped to leverage AI tools effectively.
- Define Objectives: Clearly outline what the training aims to achieve. This could include understanding AI functionalities, improving workflow efficiency, or enhancing customer interactions.
- Develop Training Materials: Create comprehensive resources, including manuals, video tutorials, and interactive modules that cover:
- AI copilot features
- Best practices for usage
- Troubleshooting common issues
- Training plans for employees and corporate training programs for employees
- Utilize Hands-On Training: Incorporate practical sessions where employees can interact with the AI copilot in real-time. This could involve:
- Simulated tasks
- Role-playing scenarios
- Group exercises to foster collaboration
- Line worker training and employee training programs
- Feedback Mechanism: Establish a system for collecting feedback from participants to continuously improve the training program. This can include:
- Surveys post-training
- One-on-one interviews
- Regular check-ins to assess comfort levels with the AI tools
- Employee development training and employee training development program
- Ongoing Support: Provide continuous learning opportunities through:
- Refresher courses
- Access to an online knowledge base
- Regular updates on new features or changes in the AI system
- Online training courses for employees and online staff training courses
- Adaptive AI development to tailor training programs to specific organizational needs. For example, incorporating insights from computer vision in sports training can enhance training effectiveness.
8.3. Measuring and Monitoring System Performance
To ensure that your AI copilot is functioning optimally, it is essential to measure and monitor its performance regularly. This helps identify areas for improvement and ensures that the system meets organizational goals.
- Establish Key Performance Indicators (KPIs): Define metrics that will help assess the AI copilot's effectiveness. Common KPIs include:
- User engagement rates
- Task completion times
- Error rates in AI-generated outputs
- Utilize Analytics Tools: Implement analytics software to track performance data. This can include:
- User interaction logs
- System response times
- Feedback from users on AI suggestions
- Conduct Regular Audits: Schedule periodic reviews of the AI copilot's performance to ensure it aligns with business objectives. This can involve:
- Comparing performance against benchmarks
- Identifying trends in user behavior
- Assessing the impact on overall productivity
- Iterate Based on Findings: Use the data collected to make informed decisions about system updates or training needs. This could involve:
- Adjusting algorithms based on user feedback
- Enhancing training programs to address identified gaps
- Implementing new features that align with user needs
9. How to Maintain and Improve Your AI Copilot?
Maintaining and improving your AI copilot is an ongoing process that requires attention to detail and a proactive approach. Here are some strategies to ensure your AI system remains effective and relevant.
- Regular Updates: Keep the AI copilot updated with the latest algorithms and features. This can involve:
- Scheduling routine software updates
- Monitoring industry trends for new capabilities
- Ensuring compatibility with other systems
- User Training Refreshers: Conduct periodic training sessions to keep users informed about new features and best practices. This can include:
- Workshops on advanced functionalities
- Q&A sessions to address user concerns
- Sharing success stories to motivate users
- HR manager training and development and manager training & development
- Feedback Loop: Create a continuous feedback loop with users to gather insights on their experiences. This can involve:
- Regular surveys to assess satisfaction
- Open forums for discussing challenges
- Incentives for users who provide constructive feedback
- Performance Reviews: Regularly review the AI copilot's performance metrics to identify areas for improvement. This can include:
- Analyzing user engagement data
- Reviewing error rates and user complaints
- Adjusting strategies based on performance trends
By implementing these strategies, organizations can ensure their AI copilot remains a valuable asset, driving efficiency and enhancing user experience. Rapid Innovation is committed to helping businesses achieve these goals through tailored training and performance monitoring solutions, ensuring that your investment in AI technology yields maximum ROI.
9.1. When and How to Update AI Models?
Updating AI models is crucial for maintaining their accuracy and relevance. The timing and method of updates can significantly impact performance.
When to Update:
- Data Drift: If the input data changes over time, it may lead to a decline in model performance. Regularly monitor for data drift to ensure your model remains aligned with current trends and patterns.
- Performance Degradation: If the model's accuracy drops below a certain threshold, it’s time to consider an update. Rapid Innovation employs advanced monitoring tools to detect such declines early, allowing for timely interventions.
- New Data Availability: Incorporating new data can enhance the model's learning and adaptability. Our team can assist in identifying relevant data sources and integrating them effectively.
- Technological Advancements: New algorithms or techniques may offer better performance, necessitating an update. Rapid Innovation stays at the forefront of AI research to ensure our clients benefit from the latest advancements.
How to Update:
- Retraining: Use the latest data to retrain the model. This can be done periodically or triggered by specific events. Our automated solutions streamline this process, reducing downtime and enhancing efficiency.
- Transfer Learning: Leverage pre-trained models and fine-tune them with new data to save time and resources. This approach can significantly reduce costs while maintaining high performance.
- Version Control: Implement version control for models to track changes and revert if necessary. Rapid Innovation emphasizes best practices in model management to ensure transparency and accountability.
- Automated Pipelines: Set up automated pipelines for continuous integration and deployment (CI/CD) of AI models. Our expertise in DevOps practices ensures seamless updates and minimal disruption to business operations.
9.2. How to Track AI Copilot Performance?
Tracking the performance of AI copilots is essential for ensuring they meet user needs and expectations.
Key Metrics to Monitor:
- Accuracy: Measure how often the AI copilot provides correct suggestions or actions. This metric is vital for assessing the effectiveness of the AI in real-world applications.
- Response Time: Track the time taken for the AI to respond to user queries. Rapid response times are crucial for user satisfaction and engagement.
- User Engagement: Analyze how frequently users interact with the AI copilot and the duration of these interactions. Understanding user behavior helps in refining the AI's capabilities.
- Error Rate: Monitor the frequency of incorrect suggestions or actions taken by the AI. A low error rate is essential for building trust in AI systems.
Methods to Track Performance:
- User Feedback: Collect qualitative feedback from users to understand their experiences and areas for improvement. Rapid Innovation can help design effective feedback mechanisms tailored to your organization.
- A/B Testing: Implement A/B testing to compare different versions of the AI copilot and determine which performs better. This data-driven approach allows for informed decision-making.
- Analytics Tools: Utilize analytics platforms to gather data on user interactions and performance metrics. Our team can assist in selecting and integrating the right tools for your needs.
- Dashboards: Create real-time dashboards to visualize performance metrics and trends over time. This enables stakeholders to make timely adjustments based on insights.
9.3. Feedback Loop Implementation and Iteration
Implementing a feedback loop is vital for continuous improvement of AI models and copilots.
Steps for Implementation:
- Collect Feedback: Gather user feedback through surveys, ratings, or direct input after interactions. Rapid Innovation can help design user-friendly feedback systems that encourage participation.
- Analyze Data: Use data analytics to identify patterns and insights from the feedback collected. Our data scientists specialize in extracting actionable insights from complex datasets.
- Iterate on Model: Based on the analysis, make necessary adjustments to the AI model or copilot functionality. Continuous iteration is key to maintaining relevance and effectiveness.
- Test Changes: Before full deployment, test the changes in a controlled environment to ensure they improve performance. Our rigorous testing protocols minimize risks associated with updates.
- Deploy Updates: Once validated, deploy the updated model or features to all users. Rapid Innovation ensures that deployment is smooth and does not disrupt ongoing operations.
By establishing a robust feedback loop, organizations can ensure their AI systems remain effective and aligned with user needs. This iterative process fosters a culture of continuous improvement, ultimately leading to better user satisfaction and enhanced performance. Rapid Innovation is committed to guiding clients through this journey, leveraging our expertise in AI model updates and Blockchain to drive greater ROI and business success.
10. How to Future-Proof Your AI Copilot Investment?
Investing in AI copilot technology is a strategic move for businesses looking to enhance productivity and streamline operations. However, to ensure that this investment remains relevant and beneficial in the long term, it is essential to future-proof it. Here are some key strategies to consider:
- Continuous Learning and Adaptation: AI technologies evolve rapidly. Regularly update your AI copilot systems to incorporate the latest advancements in machine learning and natural language processing. This ensures that your investment remains competitive and effective.
- User Feedback Mechanisms: Implement systems to gather user feedback on the AI copilot's performance. This data can guide improvements and adaptations, ensuring that the technology meets the evolving needs of your workforce.
- Scalability Considerations: Design your AI copilot infrastructure with scalability in mind. This allows for easy expansion as your business grows or as new features become available.
10.1. How to Plan for AI Copilot Scaling?
Scaling your AI copilot investment requires careful planning and execution. Here are steps to effectively plan for scaling:
- Assess Current Infrastructure: Evaluate your existing IT infrastructure to determine if it can support the increased load that comes with scaling. Consider cloud solutions for flexibility and scalability.
- Define Clear Objectives: Establish specific goals for scaling your AI copilot. This could include increasing user adoption, enhancing functionality, or expanding to new departments.
- Invest in Training: Ensure that your team is well-trained in using the AI copilot. This includes understanding its capabilities and limitations, which can help maximize its effectiveness.
- Monitor Performance Metrics: Set up key performance indicators (KPIs) to track the success of your scaling efforts. Regularly review these metrics to identify areas for improvement.
- Iterate and Optimize: Use the data collected from performance metrics and user feedback to make iterative improvements to the AI copilot. This ensures that it continues to meet the needs of your organization as it scales.
10.2. Emerging Technology Integration
Integrating emerging technologies with your AI copilot can enhance its capabilities and future-proof your investment. Here are some technologies to consider:
- Natural Language Processing (NLP): Incorporating advanced NLP can improve the AI copilot's ability to understand and respond to user queries more effectively.
- Machine Learning (ML): Utilize machine learning algorithms to enable the AI copilot to learn from user interactions, improving its responses over time.
- Robotic Process Automation (RPA): Integrating RPA can automate repetitive tasks, allowing the AI copilot to focus on more complex queries and enhancing overall productivity.
- Cloud Computing: Leverage cloud solutions for scalability and flexibility. This allows for easy updates and access to the latest AI technologies without significant infrastructure investments.
- Data Analytics: Implement data analytics tools to gain insights from user interactions with the AI copilot. This data can inform future enhancements and ensure that the technology evolves with user needs.
By focusing on these strategies, businesses can effectively future-proof their AI copilot investments, ensuring they remain valuable assets in an ever-changing technological landscape. At Rapid Innovation, we specialize in guiding organizations through this process, leveraging our expertise in AI copilot investment and blockchain to help you achieve greater ROI and operational efficiency. Additionally, understanding the implications of copilot stock can provide further insights into the market dynamics surrounding AI technologies. If you're looking to enhance your capabilities, consider hiring generative AI engineers to support your initiatives.
10.3. How to Keep Your AI Copilot Updated?
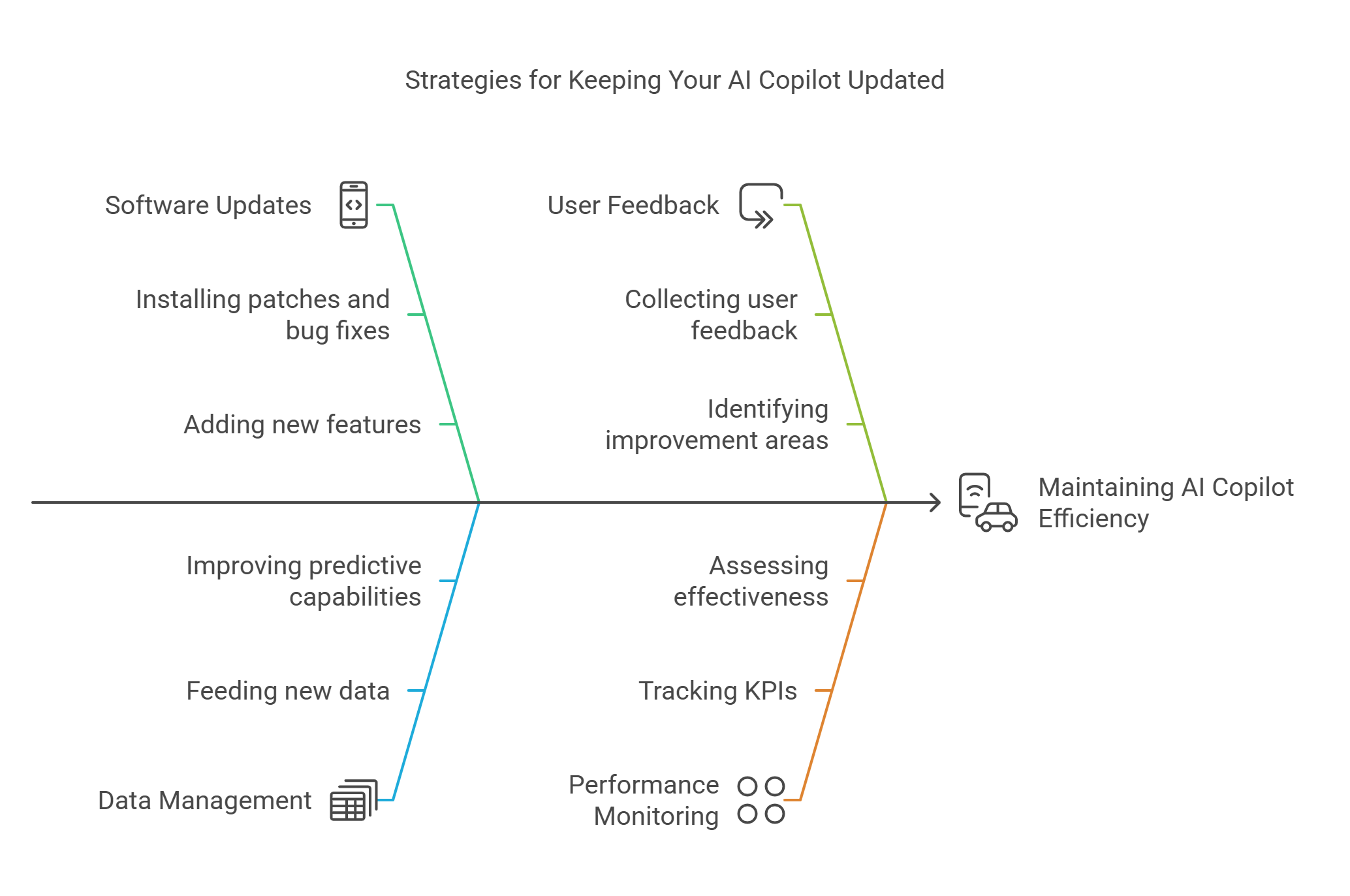
Keeping your AI copilot updated is crucial for maintaining its efficiency and effectiveness. Regular updates ensure that the AI system can adapt to new data, improve its algorithms, and incorporate user feedback. Here are some strategies to keep your AI copilot updated:
- Regular Software Updates: Ensure that the AI copilot software is updated regularly. This includes installing patches, bug fixes, and new features released by the developers, such as ai copilot updates.
- Data Refresh: Continuously feed new data into the AI system. This helps the AI learn from recent trends and user behaviors, improving its predictive capabilities.
- User Feedback Integration: Collect feedback from users regularly. This feedback can help identify areas where the AI copilot may need improvement or adjustment.
- Monitor Performance Metrics: Keep track of key performance indicators (KPIs) to assess the AI copilot's effectiveness. If performance dips, it may indicate a need for updates or retraining.
- Stay Informed on AI Trends: Follow industry news and research to stay updated on the latest advancements in AI technology. This knowledge can guide you in implementing new features or methodologies.
- Collaborate with Developers: Maintain a close relationship with the AI developers. They can provide insights into upcoming updates and best practices for keeping the system current.
11. What are the Costs and ROI of AI Copilots?
Understanding the costs and return on investment (ROI) of AI copilots is essential for businesses considering their implementation. The costs can vary widely based on several factors, including the complexity of the AI system, the scale of deployment, and ongoing maintenance needs.
- Initial Investment: This includes the cost of software licenses, hardware, and any necessary infrastructure. According to a report, companies can expect to invest between $10,000 to $500,000 for initial setup, depending on the scale and complexity of the AI solution.
- Operational Costs: Ongoing costs include maintenance, updates, and support. These can range from 15% to 20% of the initial investment annually.
- Training Costs: Employees may require training to effectively use the AI copilot. This can involve both time and financial resources.
- Opportunity Costs: Consider the potential revenue lost during the implementation phase when the AI copilot is being integrated into existing workflows.
- ROI Calculation: To calculate ROI, consider the benefits gained from increased efficiency, reduced errors, and improved decision-making. A well-implemented AI copilot can lead to significant cost savings and revenue generation.
- Long-term Benefits: Over time, the benefits of AI copilots can outweigh the initial and ongoing costs. Companies often report improved productivity and enhanced customer satisfaction, leading to higher profits.
11.1. Total Cost of Ownership Calculation
Calculating the Total Cost of Ownership (TCO) for an AI copilot involves assessing all costs associated with the system over its entire lifecycle. This includes:
- Acquisition Costs: Initial purchase price, installation, and setup costs.
- Operational Costs: Ongoing expenses such as maintenance, support, and updates.
- Training Costs: Expenses related to training staff to use the AI copilot effectively.
- Infrastructure Costs: Any additional hardware or software needed to support the AI system.
- Downtime Costs: Potential losses incurred during the implementation phase or due to system failures.
- Disposal Costs: Costs associated with decommissioning the AI system at the end of its lifecycle.
By calculating TCO, businesses can make informed decisions about the financial viability of implementing an AI copilot, ensuring that they understand both the short-term and long-term financial implications. At Rapid Innovation, we specialize in guiding clients through this process, leveraging our expertise in AI and Blockchain to help you achieve greater ROI and streamline your operations effectively.
11.2. What ROI Can You Expect from AI Copilots?
The return on investment (ROI) from AI copilots can be significant, depending on how they are integrated into your business processes. Here are some key factors to consider:
- Increased Productivity: AI copilots can automate repetitive tasks, allowing employees to focus on higher-value work. Studies show that automation can increase productivity by up to 40% in certain sectors.
- Cost Savings: By streamlining operations and reducing the need for manual intervention, businesses can save on labor costs. For instance, companies that implement AI copilot solutions can expect to reduce operational costs by 20-30%.
- Enhanced Decision-Making: AI copilots provide data-driven insights that can lead to better decision-making. This can result in improved sales and customer satisfaction, ultimately boosting revenue.
- Faster Time to Market: With AI copilots assisting in development and project management, companies can accelerate their time to market, which can lead to increased market share and profitability.
To calculate ROI, consider the following formula:
language="language-plaintext"ROI = (Net Profit / Cost of Investment) x 100
- Net Profit: Total revenue generated from AI copilot implementation minus total costs.
- Cost of Investment: Initial setup costs, ongoing maintenance, and operational costs.
11.3. How to Budget for AI Copilot Implementation?
Budgeting for AI copilot implementation requires careful planning and consideration of various factors. Here are steps to create an effective budget:
- Define Objectives: Clearly outline what you aim to achieve with AI copilots. This could include improving efficiency, reducing costs, or enhancing customer experience.
- Assess Current Infrastructure: Evaluate your existing technology stack to determine what upgrades or integrations are necessary for AI implementation.
- Estimate Costs: Consider the following cost components:
- Software Licensing: Costs associated with purchasing or subscribing to AI copilot tools.
- Hardware Upgrades: Any necessary upgrades to servers or workstations.
- Training: Budget for training employees to effectively use AI copilots.
- Maintenance and Support: Ongoing costs for technical support and system maintenance.
- Calculate Potential ROI: Use the ROI formula mentioned earlier to estimate the financial benefits of implementing AI copilots.
- Create a Contingency Fund: Set aside a percentage of your budget for unexpected expenses that may arise during implementation.
- Review and Adjust: Regularly review your budget against actual spending and adjust as necessary to stay on track.
12. What Challenges Will You Face with AI Copilots?
While AI copilots offer numerous benefits, there are challenges that organizations may encounter during implementation:
- Integration Issues: Integrating AI copilots with existing systems can be complex and may require significant technical expertise.
- Data Quality: AI systems rely on high-quality data. Poor data quality can lead to inaccurate insights and hinder decision-making.
- Change Management: Employees may resist adopting new technologies. Effective change management strategies are essential to ensure smooth transitions.
- Cost Overruns: Initial estimates for AI copilot implementation may not account for all costs, leading to budget overruns.
- Ethical Considerations: The use of AI raises ethical questions regarding data privacy and bias. Organizations must navigate these issues carefully to maintain trust.
- Skill Gaps: There may be a lack of skilled personnel to manage and operate AI systems, necessitating additional training or hiring.
By understanding these challenges, organizations can better prepare for the successful implementation of AI copilots, maximizing their potential benefits. At Rapid Innovation, we specialize in guiding businesses through these complexities, ensuring that your investment in AI copilot technology yields the highest possible returns. Our expertise in AI and Blockchain development allows us to tailor solutions that align with your specific business goals, ultimately driving efficiency and profitability.
12.1. How to Overcome Technical Integration Challenges?
Technical integration challenges often arise when merging different systems, software, or platforms. These challenges can hinder productivity and lead to data silos. Here are some strategies to overcome these issues:
- Assess Compatibility: Before integration, evaluate the compatibility of existing systems, including checking APIs, data formats, and protocols. Rapid Innovation can assist in this assessment, ensuring that your systems are primed for seamless integration.
- Use Middleware Solutions: Middleware can act as a bridge between different applications, facilitating communication and data exchange. Consider using tools like
Apache Camel
orMuleSoft
, which our team can implement to streamline your integration processes. - Standardize Data Formats: Ensure that all systems use standardized data formats (e.g.,
JSON
,XML
) to minimize conversion issues. Our expertise in data management can help you establish these standards effectively. - Implement Robust APIs: Develop and utilize well-documented APIs to allow seamless interaction between systems. This can simplify integration and reduce errors, a service Rapid Innovation specializes in to enhance your operational capabilities.
- Conduct Thorough Testing: Before going live, conduct extensive testing to identify and resolve any integration issues. This includes unit testing, system testing, and user acceptance testing, all of which we can facilitate to ensure a smooth transition.
- Provide Training: Ensure that your team is well-trained on the new systems and integration processes to minimize errors and improve efficiency. Rapid Innovation offers tailored training programs to empower your staff with technical integration solutions.
12.2. User Adoption Barriers
User adoption barriers can significantly impact the success of new technologies or systems. Understanding these barriers is crucial for effective implementation. Here are some common barriers and how to address them:
- Lack of Awareness: Users may not understand the benefits of the new system. To combat this, provide clear communication about the advantages and features of the new technology. Rapid Innovation can help craft effective communication strategies.
- Resistance to Change: Change can be daunting. Engage users early in the process and involve them in decision-making to foster a sense of ownership. Our consultants can guide you in change management practices to ease this transition.
- Insufficient Training: Users may feel overwhelmed if they are not adequately trained. Offer comprehensive training sessions and resources, such as tutorials and FAQs, which we can develop to suit your specific needs.
- Poor User Experience: If the new system is not user-friendly, adoption will suffer. Focus on intuitive design and gather user feedback to make necessary adjustments. Rapid Innovation excels in user experience design, ensuring that your systems are both functional and user-friendly.
- Inadequate Support: Users need ongoing support during the transition. Establish a helpdesk or support team to assist with any issues that arise. We can help set up a robust support framework tailored to your organization.
- Cultural Resistance: Organizational culture can impact adoption. Promote a culture of innovation and flexibility to encourage users to embrace new technologies. Our team can assist in fostering an innovative culture within your organization.
12.3. How to Solve Performance Issues?
Performance issues can arise from various factors, including system overload, inefficient code, or inadequate resources. Here are steps to identify and resolve these issues:
- Monitor System Performance: Use monitoring tools like
New Relic
orDatadog
to track system performance metrics and identify bottlenecks. Rapid Innovation can implement these tools to provide you with real-time insights. - Optimize Code: Review and optimize code to improve efficiency. This may involve refactoring, reducing complexity, or eliminating redundant processes. Our development team specializes in code optimization to enhance system performance.
- Scale Resources: If the system is under heavy load, consider scaling resources. This can include upgrading hardware, increasing server capacity, or utilizing cloud services for flexibility, all of which we can assist you with.
- Implement Caching: Use caching mechanisms to store frequently accessed data, reducing the load on databases and improving response times. Our expertise in system architecture can help you implement effective caching strategies.
- Conduct Load Testing: Perform load testing to simulate high traffic and identify how the system performs under stress. Tools like
JMeter
can be useful for this purpose, and we can facilitate this testing to ensure your systems are resilient. - Regular Maintenance: Schedule regular maintenance to ensure systems are running optimally. This includes updating software, clearing out unnecessary data, and checking for security vulnerabilities. Rapid Innovation offers ongoing maintenance services to keep your systems in peak condition.
By addressing these technical integration challenges, user adoption barriers, and performance issues, organizations can enhance their operational efficiency and ensure successful technology implementation. Rapid Innovation is here to support you in achieving these goals effectively and efficiently, ultimately leading to greater ROI for your business through technical integration solutions. AI copilots for business are transforming the way businesses operate by enhancing productivity, improving decision-making, and streamlining workflows. As organizations increasingly adopt AI technologies, understanding the results they can expect from AI copilots becomes crucial.
13. What Results Can You Expect from AI Copilots?
AI copilots can deliver a range of results that significantly impact business operations. Here are some key outcomes:
- Increased Efficiency: AI copilots automate repetitive tasks, allowing employees to focus on higher-value activities. This leads to faster project completion and reduced operational costs.
- Enhanced Decision-Making: By analyzing vast amounts of data, AI copilots provide insights that help teams make informed decisions quickly. This can lead to better strategic planning and risk management.
- Improved Collaboration: AI copilots facilitate communication and collaboration among team members by providing real-time data and insights, ensuring everyone is on the same page.
- Personalized Customer Experiences: AI copilots can analyze customer data to tailor interactions, leading to improved customer satisfaction and loyalty.
- Scalability: As businesses grow, AI copilots can easily scale operations without the need for significant additional resources, making them a cost-effective solution.
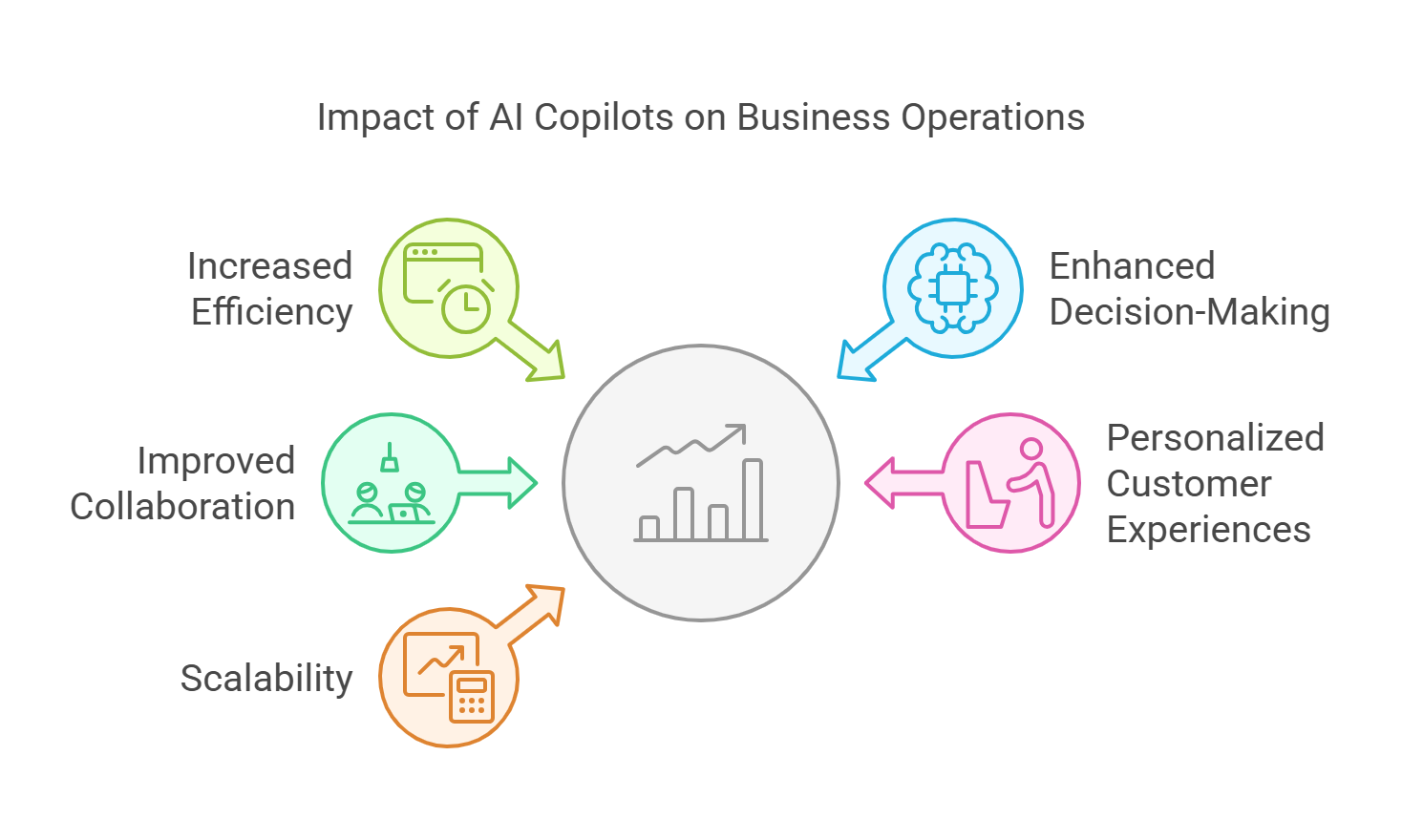
13.1. Which Companies Have Successfully Implemented AI Copilots?
Several companies across various industries have successfully integrated AI copilots for business into their operations. Notable examples include:
- Microsoft: With its Copilot feature in Microsoft 365, the company has enhanced productivity tools like Word and Excel, allowing users to generate content and analyze data more efficiently.
- Salesforce: The introduction of Einstein AI has enabled Salesforce to provide personalized customer relationship management (CRM) solutions, improving sales and customer service outcomes.
- IBM: IBM's Watson has been utilized in healthcare to assist doctors in diagnosing diseases and recommending treatment plans, showcasing the potential of AI copilots in critical decision-making environments.
These companies demonstrate the versatility and effectiveness of AI copilots in enhancing business processes.
13.2. Measurable Business Impact Results
The measurable business impact of AI copilots for business can be significant. Here are some key metrics that organizations have reported:
- Productivity Gains: Companies have reported productivity increases of up to 40% after implementing AI copilots, as they automate routine tasks and streamline workflows.
- Cost Reduction: Organizations have seen operational cost reductions of 20-30% by leveraging AI to optimize processes and reduce manual labor.
- Customer Satisfaction: Businesses utilizing AI copilots for customer interactions have experienced a 15% increase in customer satisfaction scores, thanks to personalized and timely responses.
To achieve these results, organizations can follow these steps:
- Identify Key Areas for AI Implementation: Assess which processes can benefit most from AI copilots for business, such as customer service, data analysis, or project management.
- Choose the Right AI Tools: Select AI copilots that align with your business needs and integrate seamlessly with existing systems.
- Train Employees: Provide training for employees to effectively use AI copilots, ensuring they understand how to leverage the technology for maximum benefit.
- Monitor and Measure Impact: Establish key performance indicators (KPIs) to track the effectiveness of AI copilots and make adjustments as necessary.
- Iterate and Improve: Continuously refine AI implementations based on feedback and performance data to enhance results over time.
At Rapid Innovation, we specialize in helping organizations navigate the complexities of AI implementation. Our expertise in AI and Blockchain development allows us to tailor solutions that align with your specific business goals. By leveraging our services, you can expect to achieve greater ROI through enhanced operational efficiency, improved decision-making, and innovative customer engagement strategies.
By understanding the potential results and following a structured approach, businesses can effectively harness the power of AI copilots for business to drive growth and innovation.
13.3. Lessons Learned and Best Practices
Implementing AI copilot implementation in various sectors has provided valuable insights and best practices that can enhance future projects. Here are some key lessons learned:
- User-Centric Design: Prioritize the needs and preferences of end-users. Engaging users during the design phase ensures that the AI copilot is intuitive and meets their requirements effectively.
- Iterative Development: Adopt an agile approach to development. Continuous feedback loops allow for rapid adjustments and improvements, ensuring the AI copilot evolves based on real-world usage.
- Data Quality Matters: The effectiveness of AI copilots heavily relies on the quality of data used for training. Ensure that data is clean, relevant, and representative of the tasks the AI will perform.
- Transparency and Explainability: Users are more likely to trust AI systems that provide clear explanations of their decision-making processes. Implement features that allow users to understand how the AI arrives at its conclusions.
- Integration with Existing Systems: Seamless integration with current workflows and tools is crucial. This minimizes disruption and enhances user adoption rates.
- Continuous Learning: AI systems should be designed to learn from new data and user interactions. This adaptability ensures that the copilot remains relevant and effective over time.
- Ethical Considerations: Address ethical concerns proactively. Ensure that the AI copilot adheres to guidelines that prevent bias and promote fairness in decision-making.
14. How to Get Started with AI Copilots?
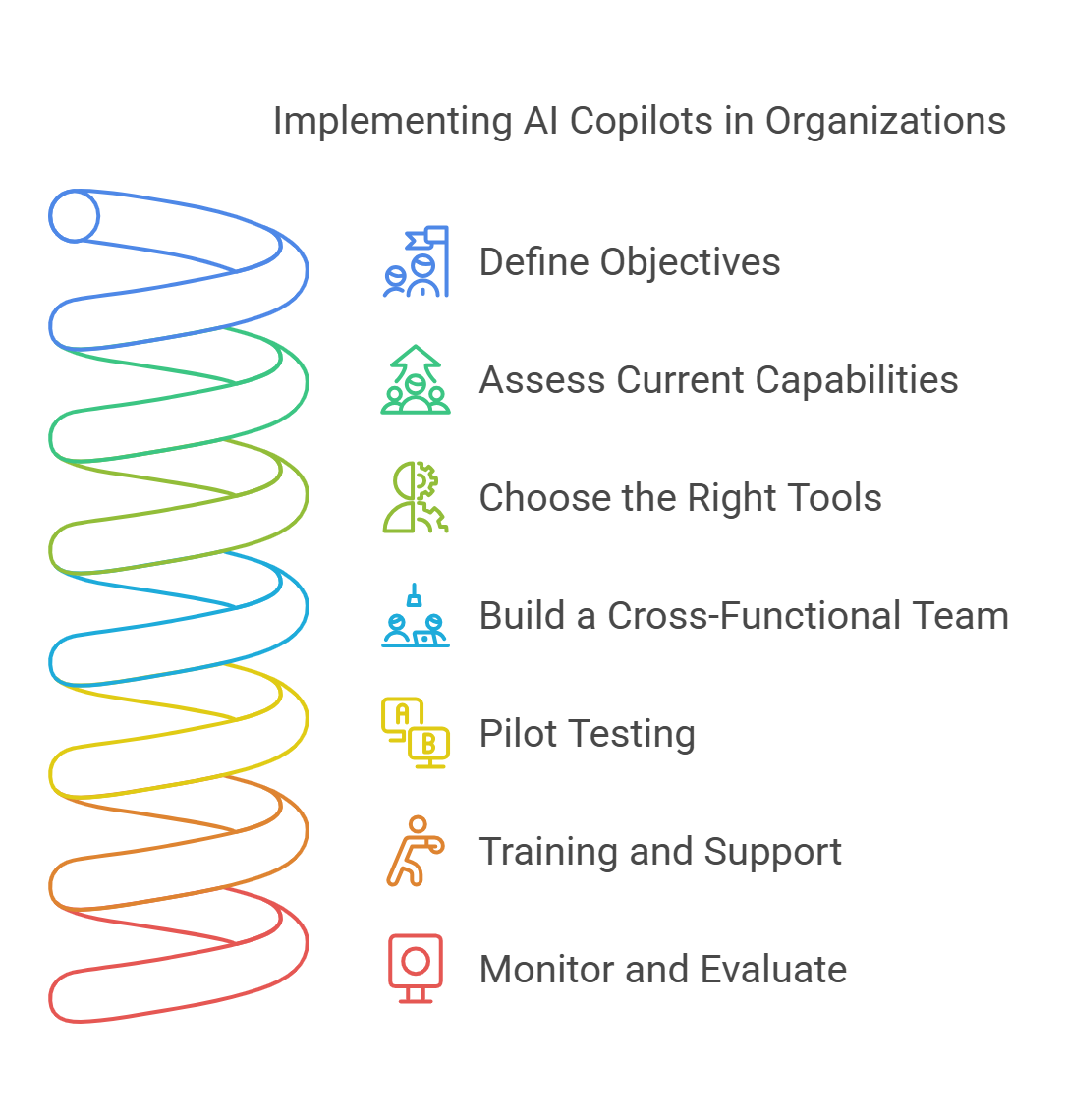
Getting started with AI copilots involves several strategic steps to ensure successful implementation. Here’s a guide to help organizations embark on this journey:
- Define Objectives: Clearly outline the goals you want to achieve with the AI copilot. Whether it’s improving productivity, enhancing customer service, or streamlining operations, having specific objectives will guide the development process.
- Assess Current Capabilities: Evaluate your existing technology infrastructure and data resources. Understanding your current capabilities will help identify gaps that need to be addressed before implementing an AI copilot.
- Choose the Right Tools: Select AI tools and platforms that align with your objectives. Consider factors such as scalability, ease of integration, and support for machine learning algorithms.
- Build a Cross-Functional Team: Assemble a team that includes data scientists, software engineers, and domain experts. Collaboration among diverse skill sets will enhance the development process and ensure the AI copilot meets user needs.
- Pilot Testing: Before full-scale deployment, conduct pilot tests to gather feedback and identify potential issues. This step allows for adjustments based on real-world performance.
- Training and Support: Provide comprehensive training for users to ensure they understand how to interact with the AI copilot effectively. Ongoing support is also essential to address any challenges that arise post-implementation.
- Monitor and Evaluate: After deployment, continuously monitor the AI copilot’s performance. Use metrics to evaluate its impact on productivity and user satisfaction, making adjustments as necessary.
14.1. Implementation Checklist
An implementation checklist can streamline the process of integrating AI copilots into your organization. Here are essential items to consider:
- Objective Setting
- Define clear goals for the AI copilot.
- Identify key performance indicators (KPIs) for success.
- Technology Assessment
- Evaluate existing infrastructure and data quality.
- Determine necessary upgrades or new tools.
- Team Formation
- Assemble a cross-functional team.
- Assign roles and responsibilities.
- Tool Selection
- Research and select appropriate AI platforms.
- Ensure compatibility with existing systems.
- Pilot Testing
- Develop a pilot program to test the AI copilot implementation.
- Gather user feedback and make necessary adjustments.
- Training Programs
- Create training materials for users.
- Schedule training sessions and provide ongoing support.
- Monitoring Plan
- Establish a system for tracking performance metrics.
- Schedule regular evaluations to assess effectiveness.
By following these steps and best practices, organizations can effectively implement AI copilots, enhancing productivity and user experience while minimizing potential challenges. At Rapid Innovation, we leverage our expertise in AI and Blockchain to guide clients through this process, ensuring they achieve greater ROI and operational efficiency.
14.2. Resource Planning Guide
Effective resource planning is crucial for the successful implementation of any project, especially when integrating advanced technologies like AI Copilot. A well-structured resource planning guide, such as the strategic planning guide for nonprofits or the field guide to nonprofit strategic planning and facilitation, can help organizations allocate their resources efficiently, ensuring that all aspects of the project are covered.
Key Components of a Resource Planning Guide
- Identify Resources Needed: Determine the types of resources required, including human resources, technology, and financial investments.
- Assess Current Resources: Evaluate existing resources to identify gaps and areas that need enhancement.
- Create a Resource Allocation Plan: Develop a detailed plan that outlines how resources will be distributed across various tasks and phases of the project.
- Set Priorities: Establish priorities for resource allocation based on project timelines and critical tasks.
- Monitor and Adjust: Implement a system for tracking resource usage and make adjustments as necessary to stay on track.
Tools for Resource Planning
- Project Management Software: Utilize tools like Asana, Trello, or Microsoft Project to visualize resource allocation and project timelines.
- Resource Management Tools: Consider specialized software like Resource Guru or Float for real-time resource tracking.
- Collaboration Platforms: Use platforms like Slack or Microsoft Teams to facilitate communication among team members regarding resource needs and updates.
Best Practices for Resource Planning
- Involve Stakeholders: Engage all relevant stakeholders in the planning process to ensure that their needs and insights are considered.
- Regular Reviews: Schedule regular reviews of the resource plan to adapt to any changes in project scope or resource availability.
- Documentation: Keep thorough documentation of resource allocation decisions and changes to maintain transparency and accountability.
14.3. How Long Does AI Copilot Implementation Take?
The timeline for implementing AI Copilot can vary significantly based on several factors, including the complexity of the project, the size of the organization, and the existing infrastructure.
Factors Influencing Implementation Time
- Project Scope: Larger projects with more extensive requirements will naturally take longer to implement.
- Integration Complexity: The ease of integrating AI Copilot with existing systems can affect the timeline. More complex integrations may require additional time for testing and adjustments.
- Team Expertise: The skill level of the team involved in the implementation can speed up or slow down the process. Experienced teams may navigate challenges more efficiently.
Typical Implementation Timeline
- Initial Planning: 2-4 weeks for defining project goals, resource allocation, and stakeholder engagement.
- Development Phase: 4-12 weeks depending on the complexity of the AI Copilot features being implemented.
- Testing and Feedback: 2-6 weeks for thorough testing, gathering user feedback, and making necessary adjustments.
- Deployment: 1-2 weeks for final deployment and training sessions for end-users.
Steps to Achieve Successful Implementation
- Define Objectives: Clearly outline what you aim to achieve with AI Copilot.
- Assemble a Team: Gather a team with the necessary skills and experience.
- Develop a Timeline: Create a realistic timeline that accounts for all phases of the project.
- Conduct Training: Ensure that all users are adequately trained on how to use AI Copilot effectively.
- Monitor Progress: Regularly check in on the implementation process to address any issues promptly.
By following a structured resource planning guide, such as the strategic planning handbook or the undp handbook on planning monitoring and evaluating for development results, and understanding the timeline for AI Copilot implementation, organizations can enhance their chances of a successful integration, ultimately leading to improved productivity and efficiency. At Rapid Innovation, we leverage our expertise in AI and Blockchain to ensure that your resource planning aligns with your business goals, maximizing your return on investment and driving sustainable growth.