Table Of Contents
Category
Artificial Intelligence
1. Introduction to Industry 4.0
Industry 4.0 represents the fourth industrial revolution, characterized by the integration of digital technologies into manufacturing processes. This transformative phase follows the initial mechanization through water and steam power, mass production with the aid of electricity, and the digital revolution that introduced automated production. Today, Industry 4.0 is reshaping the manufacturing landscape by enhancing connectivity, automation, machine learning, and real-time data.
Industry 4.0 merges physical production and operations with smart digital technology, machine learning, and big data to create a more holistic and better-connected ecosystem for companies that focus on manufacturing and supply chain management. This integration significantly improves visibility, boosts operational efficiency, and increases production output while reducing costs. As a result, businesses are better equipped to respond to the demands and challenges of the modern market.
1.1. Definition and Importance
Industry 4.0 is defined as the ongoing automation of traditional manufacturing and industrial practices, using modern smart technology. The primary importance of Industry 4.0 lies in its ability to empower manufacturers to better control and understand every aspect of their operations, and to leapfrog to new levels of efficiency and productivity. This revolution is not just about enhancing the technological processes but also about revolutionizing the way those processes impact the entire business ecosystem.
The importance of Industry 4.0 extends beyond simple productivity gains. It represents a significant shift in how industries operate, leading to greater operational flexibility, better quality products, and improved workplace safety. Companies that embrace Industry 4.0 technologies are positioned to respond more quickly to market changes and customer needs, which is crucial in a globally competitive market. This adaptability is vital for business sustainability and growth in the digital age.
1.2. Key Technologies Driving Industry 4.0
Several key technologies are at the forefront of driving Industry 4.0. These include the Internet of Things (IoT), Artificial Intelligence (AI), robotics, cloud computing, and cybersecurity. IoT is pivotal as it allows for a network of devices to communicate and collaborate, enhancing the efficiency of industrial processes. AI contributes by enabling machines to make decisions based on real-time data, leading to increased automation and improved decision-making processes.
Robotics technology has also seen significant advancements, with robots now capable of performing complex tasks that are either too dangerous or repetitive for human workers. Cloud computing facilitates the seamless sharing and storage of vast amounts of data, which is crucial for real-time monitoring and management of industrial operations. Lastly, as more devices become interconnected, cybersecurity becomes increasingly critical in protecting sensitive industrial data and maintaining the integrity of automated processes.
Together, these technologies not only drive the efficiency and productivity of manufacturing processes but also enhance the agility of businesses to adapt to new challenges and opportunities in the market.
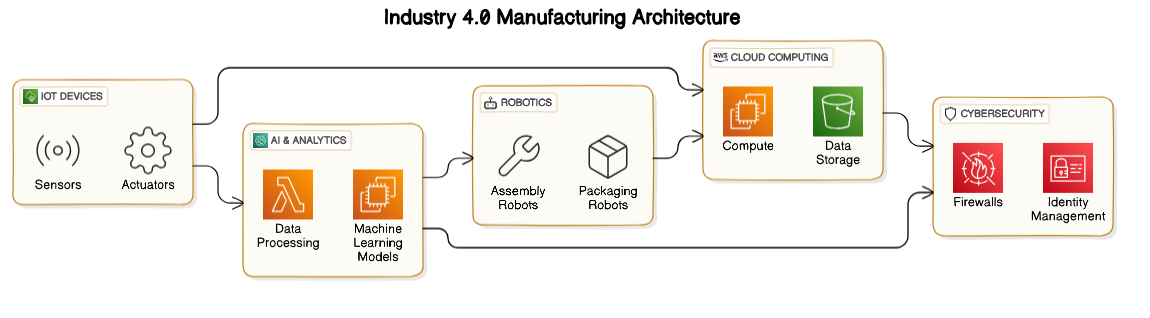
This architectural diagram visually represents the integration and interaction between the key technologies driving Industry 4.0, providing a clearer understanding of how they function within a manufacturing environment.
1.3. The Role of Digital Twins
Digital twins are virtual replicas of physical devices that data scientists and IT pros can use to run simulations before actual devices are built and deployed. They are used in various industries, including manufacturing, healthcare, and urban planning. The concept of digital twins goes beyond merely simulating the physical characteristics of an object; it also involves simulating the dynamics and how they interact with their environment over time.
For instance, in manufacturing, digital twins are used to optimize the manufacturing process, reduce downtime, and improve predictive maintenance. By creating a digital twin of a manufacturing system, engineers can test changes and predict outcomes with high accuracy, thus minimizing risk and potentially costly physical trials. Siemens offers extensive insights into how digital twins have revolutionized industries by enhancing efficiency and innovation.
In the realm of healthcare, digital twins can simulate the human body or parts of it for medical research, surgical preparation, and training. This technology can lead to personalized medicine and improved healthcare outcomes. Philips, for example, explores the use of digital twins in healthcare, enhancing patient care and treatment outcomes.
Urban planning also benefits from digital twins. Cities can create models to improve infrastructure, plan urban developments, and enhance sustainability practices. The concept helps in managing city resources more effectively and responding to changes in a dynamic environment. Bentley Systems provides a detailed exploration of how digital twins are transforming city planning and management.
Generative AI refers to the subset of artificial intelligence focused on creating new content, whether it be text, images, audio, or video, based on the training data it has been fed. This type of AI learns from a vast amount of data inputs and tries to generate outputs that are indistinguishable from the original data. It has applications across various fields, from creative arts to scientific research.
One of the most talked-about examples of generative AI is in the field of art and design, where tools like DALL-E from OpenAI can create images from textual descriptions, showcasing the creative potential of AI (DALL-E). This not only opens up new avenues for creativity but also assists designers in rapid prototyping and ideation processes.
In the realm of text, generative AI models like GPT-3 have demonstrated the ability to write coherent and contextually relevant paragraphs, simulate conversations, and even generate code. These capabilities make generative AI a valuable tool for content creation, customer service, and more, as detailed by OpenAI (GPT-3).
Moreover, in scientific research, generative AI can help in drug discovery by predicting molecular structures that could lead to new pharmaceuticals, significantly speeding up what is traditionally a long and costly process. The potential of AI in accelerating drug discovery is explored in depth by organizations like DeepMind (DeepMind).
2. Generative AI Applications and Benefits
2.1. What is Generative AI?
Generative AI is a branch of artificial intelligence that focuses on creating new data instances that resemble the training data. Essentially, it learns from existing data to generate new content that can be text, images, audio, or video. This technology relies heavily on machine learning models, particularly on neural networks that are structured to understand and replicate the complexities of the data they are trained on.
The power of generative AI lies in its ability to automate creative processes and generate solutions to problems by learning from existing patterns and data. For instance, in the field of natural language processing, GPT-3 by OpenAI is a prime example of generative AI that can compose text that feels natural to human readers, which has vast implications for writing, customer support, and even programming (GPT-3).
In the visual arts, generative AI can create new artworks based on historical styles or completely new aesthetics, as demonstrated by AI programs like Artbreeder, which blends and evolves images to create new visuals (Artbreeder).
Furthermore, in the music industry, tools like Jukebox from OpenAI demonstrate how generative AI can compose music in various styles, creating new melodies and harmonies that mimic known artists and genres (Jukebox). This capability shows the potential of AI not just in mimicking human creativity but also in pushing its boundaries.

This diagram illustrates the architecture of a generative AI system, showing the flow from data input to output generation across different media types.
2.2. Applications in Various Industries
Generative AI has found applications across a wide range of industries, revolutionizing how businesses operate and innovate. In the healthcare sector, for example, generative AI is used to create synthetic data for training machine learning models without compromising patient privacy. This technology can generate realistic, anonymized medical images used for training diagnostic algorithms, potentially improving diagnostic accuracy and patient outcomes. More details on this application can be found on HealthITAnalytics (HealthITAnalytics).
In the automotive industry, generative AI assists in designing vehicles. AI algorithms can generate thousands of potential vehicle designs by inputting specific parameters such as weight, material strength, and cost constraints, significantly speeding up the design process and enhancing innovation. This application is well-documented in a report by McKinsey (McKinsey).
The entertainment industry also benefits from generative AI, particularly in content creation. AI tools are now capable of generating music, writing scripts, and even creating video game environments, which can help reduce the time and cost associated with creative processes. An interesting discussion on AI in entertainment is available on Variety (Variety).
These examples illustrate just a few of the ways generative AI is being applied across different sectors, driving efficiency, creativity, and innovation.
2.3. Benefits of Generative AI in Simulation
Generative AI significantly enhances the capabilities of simulation technologies, providing substantial benefits across various fields. In engineering, for instance, AI-driven simulations can predict the outcomes of experiments and design processes under different conditions, which helps in optimizing designs and reducing the need for physical prototypes. This not only saves time and resources but also accelerates the development of new technologies.
In environmental science, generative AI models simulate complex ecological systems to predict changes due to various factors like climate change or human activity. These simulations can be crucial for planning and decision-making in conservation efforts. The Nature Conservancy discusses some of these applications in detail (The Nature Conservancy).
Furthermore, in the field of economics, generative AI can simulate market scenarios, helping businesses and policymakers understand potential economic outcomes under various global events or policy changes. This can guide strategy and policy formulation, making them more adaptive to future uncertainties.
Overall, the integration of generative AI into simulation tools not only makes the simulations more accurate and faster but also allows for the exploration of scenarios that would be impossible or impractical to test in reality.
3. Exploring Multimodal Learning
Multimodal learning is an area of artificial intelligence that involves integrating and processing multiple types of data input, such as text, audio, and visual information, to improve learning accuracy and performance. This approach is particularly effective because it mirrors human learning processes, where multiple senses are used to understand and interact with the world.
One of the key applications of multimodal learning is in the development of more sophisticated AI assistants that can understand context better and interact in a more human-like manner. For example, by analyzing both voice intonation and facial expressions, an AI system can understand a user's mood and the nuances of the conversation to provide more empathetic and accurate responses.
Another significant application is in autonomous vehicles. These vehicles rely on multimodal learning to process data from various sensors simultaneously, including visual, radar, and lidar inputs, to make real-time navigation decisions. This comprehensive sensory input is crucial for safe and efficient operation.
The potential of multimodal learning is vast, and its exploration is still in the early stages. As AI continues to evolve, the integration of multimodal techniques is expected to lead to breakthroughs in AI capabilities and applications. More insights into multimodal learning can be found on the NVIDIA Developer blog (NVIDIA Developer).

This architectural diagram illustrates the integration of various data inputs in a multimodal learning framework, highlighting the flow and interaction between different components such as data preprocessors, AI models, and output modules.
3.1. Definition of Multimodal Learning
Multimodal learning is an educational approach that integrates multiple modes of learning inputs and outputs, such as text, audio, visual, and kinesthetic, to enhance the learning experience and improve information retention. This approach is based on the understanding that different people have different learning preferences and that combining various methods can cater to a broader range of learners. Multimodal learning can occur in both physical and digital environments, making it a versatile option for education in today’s diverse educational landscape.
The concept of multimodal learning is rooted in the theory of multimodal communication, which suggests that people understand and retain information better when it is presented through multiple channels. By engaging various senses, multimodal learning creates more neural connections in the brain, which can lead to deeper understanding and recall of the information. For more insights into the definition and theoretical background of multimodal learning, resources such as the Cambridge Dictionary or educational websites like Edutopia provide comprehensive explanations and examples.
3.2. How It Works
Multimodal learning works by leveraging different sensory modalities to deliver content in various formats. For instance, a lesson might include reading materials (textual), videos (visual), podcasts (audio), and interactive simulations (kinesthetic). This variety ensures that learners are not only reading or listening but also seeing and doing, which can help to reinforce learning through multiple pathways in the brain.
In practice, educators might use tools like digital whiteboards, multimedia presentations, and virtual or augmented reality experiences to create a rich, immersive learning environment. Technology plays a crucial role in facilitating multimodal learning, especially in online settings, where digital tools can simulate hands-on activities or provide visual and auditory supplements to traditional texts. Websites like Khan Academy or platforms like Coursera are examples of how digital tools are used to implement multimodal strategies effectively.
Here is an architectural diagram illustrating the integration of various modalities in a multimodal learning system:
3.3. Advantages Over Traditional Models
Multimodal learning offers several advantages over traditional, single-mode educational models. Firstly, it accommodates diverse learning styles, which can increase engagement and motivation among students. By providing multiple ways to access and engage with content, learners can choose the method that best suits their personal learning style, whether they are visual, auditory, reading/writing, or kinesthetic learners.
Secondly, multimodal learning can lead to improved comprehension and retention of information. The use of multiple sensory inputs helps to reinforce memory storage and recall by activating different areas of the brain. Research has shown that when information is presented in varied formats, it can enhance the learning process and lead to better academic outcomes. For further reading on the effectiveness of multimodal learning, scholarly articles and research studies available on educational research journals like JSTOR or ERIC can provide detailed empirical evidence.
Lastly, this approach is particularly well-suited to the modern digital world, where digital literacy is crucial. Multimodal learning often incorporates digital tools and media, preparing students for the technological demands of the contemporary workplace and society. This integration of technology in education not only supports learning but also develops essential digital skills among learners.
The integration of Generative AI with digital twins represents a transformative leap in how industries manage and optimize complex systems. Digital twins are virtual replicas of physical devices or systems that can be used for simulation and analysis. By incorporating Generative AI, these models not only replicate but also predict and evolve based on incoming data, leading to more dynamic and responsive systems.
Generative AI algorithms can generate new data scenarios based on historical data, which enhances the predictive capabilities of digital twins. This integration is particularly beneficial in sectors like manufacturing, automotive, and healthcare, where being able to predict outcomes with high accuracy can significantly impact efficiency and safety. For instance, in manufacturing, a digital twin of a production line integrated with Generative AI can simulate and optimize production processes under various conditions without the need to physically test each scenario.
4.1. Enhancing Simulation Accuracy
The accuracy of simulations is critical, especially in fields like aerospace and medicine, where precise outcomes are necessary. Generative AI contributes by creating highly accurate predictive models that feed into digital twins, enhancing the reliability of the simulations. These AI-enhanced models can predict the wear and tear on machinery, the impact of environmental factors on materials, and even human responses to different medical treatments.
This capability not only improves the quality and safety of products and services but also reduces costs by minimizing the need for physical prototypes and trials. For example, in the aerospace sector, digital twins enhanced with Generative AI can simulate aircraft performance under various atmospheric conditions, leading to safer and more efficient aircraft designs. More about the impact of AI on simulation accuracy can be found on websites like ScienceDirect.
4.2. Real-time Data Utilization
Real-time data utilization is another significant advantage of integrating Generative AI with digital twins. This integration allows for the continuous updating of the digital twin models as new data is collected, ensuring that the models always reflect the current state of the physical counterpart. This is crucial for industries where conditions change rapidly, such as energy and utilities.
For example, in the energy sector, a digital twin of a wind turbine integrated with Generative AI can adjust its operations in real-time based on weather data to maximize efficiency and reduce wear and tear. This not only enhances performance but also extends the lifespan of the equipment. Real-time data utilization also supports predictive maintenance, which can preemptively address potential failures, thereby avoiding downtime and expensive repairs.
Further insights into real-time data utilization can be explored through resources like TechTarget and Gartner. These platforms provide in-depth analyses and case studies on how real-time data is transforming various industries through advanced AI integration.
This architectural diagram illustrates the dynamic interaction between Generative AI and digital twins, highlighting the data flow and feedback mechanisms that enhance operational efficiency and simulation accuracy.
4.3. Predictive Maintenance and Anomaly Detection
Predictive maintenance and anomaly detection are critical components of modern industrial operations, leveraging data analytics and machine learning to anticipate equipment failures and optimize maintenance schedules. This approach contrasts with traditional maintenance strategies, which often rely on scheduled or reactive maintenance practices. By predicting when equipment will fail, companies can reduce downtime, save on repair costs, and extend the lifespan of their machinery.
Predictive maintenance utilizes various data sources such as historical performance data, real-time monitoring, and environmental conditions. Machine learning algorithms analyze this data to identify patterns or anomalies that precede equipment failures. For instance, vibration analysis, temperature monitoring, and acoustic inspections can provide early warnings of mechanical issues. This proactive maintenance strategy is particularly beneficial in industries where equipment failure can result in significant operational disruptions and safety hazards.
Several platforms and tools facilitate predictive maintenance and anomaly detection. IBM’s Maximo is a notable example, offering advanced analytics capabilities to monitor asset health and predict potential failures (source: IBM Maximo). Similarly, Microsoft Azure provides solutions that integrate machine learning and IoT technologies to enhance maintenance strategies (source: Microsoft Azure). These technologies not only improve reliability and efficiency but also contribute to substantial cost savings over time.
Here is an architectural diagram illustrating a Predictive Maintenance System:

5. Case Studies
5.1. Automotive Industry
The automotive industry has been at the forefront of adopting advanced technologies like artificial intelligence, IoT, and big data analytics to revolutionize various aspects of its operations. One significant application is in enhancing manufacturing processes and vehicle maintenance through predictive analytics.
Automakers use predictive maintenance to foresee and prevent vehicle malfunctions and part failures, thereby improving the reliability and safety of vehicles. For example, Tesla has integrated sophisticated diagnostic capabilities into its vehicles, which continuously collect data to predict maintenance needs and automatically schedule service appointments when necessary (source: Tesla). This not only enhances customer satisfaction but also reduces warranty costs and improves vehicle longevity.
Another case study involves BMW’s use of AI to predict and streamline its manufacturing processes. BMW employs AI algorithms to analyze production data and detect potential anomalies or inefficiencies in real-time. This proactive approach allows for immediate corrections, minimizing downtime and production delays. By integrating these technologies, BMW ensures a higher quality of production and more efficient operations, setting a benchmark in the automotive industry.
These examples illustrate how predictive maintenance and anomaly detection are not just theoretical concepts but are actively transforming industries by enabling smarter, more efficient, and customer-oriented practices.
5.2. Manufacturing Sector
The manufacturing sector is undergoing a significant transformation, driven by advancements in technology and changes in consumer demand. The integration of digital technologies, often referred to as Industry 4.0, is revolutionizing the way products are designed, produced, and distributed. Technologies such as the Internet of Things (IoT), artificial intelligence (AI), and robotics are at the forefront of this transformation, enabling smarter, more efficient production processes.
For instance, IoT devices allow for real-time monitoring and control of manufacturing equipment, leading to improved efficiency and reduced downtime. AI algorithms can predict maintenance needs and optimize production schedules, further enhancing productivity. Robotics, particularly collaborative robots (cobots), are being increasingly adopted for tasks that require precision and consistency, helping to reduce human error and increase safety.
The impact of these technologies is profound, offering manufacturers the ability to significantly reduce costs, improve product quality, and increase flexibility in production. As a result, companies that embrace these technologies are likely to see a competitive advantage in the global market. More insights on the impact of digital transformation in manufacturing can be found on websites like Manufacturing Global (https://www.manufacturingglobal.com/) and Industry Week (https://www.industryweek.com/).
Here is an architectural diagram illustrating the Industry 4.0 ecosystem in the manufacturing sector:
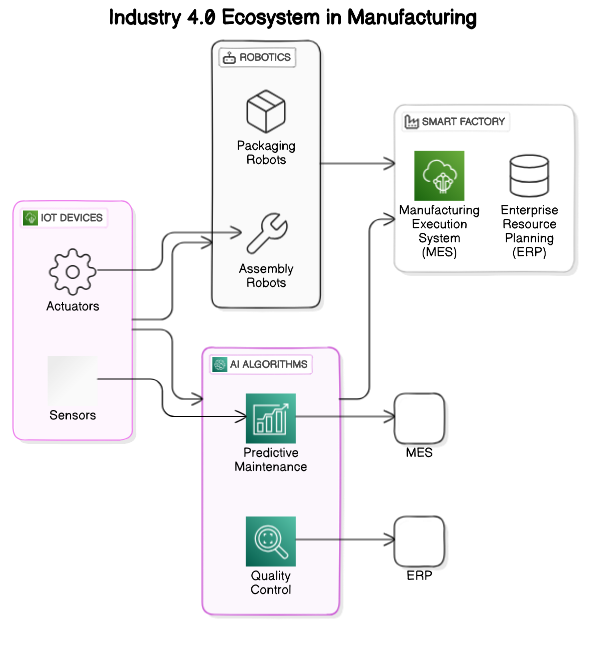
5.3. Energy Management
Energy management in the modern era is critical not only for cost reduction but also for environmental sustainability. Businesses and governments are increasingly focusing on implementing more efficient energy use practices and integrating renewable energy sources. Smart grids, energy storage systems, and energy management software play pivotal roles in optimizing energy use and reducing waste.
Smart grids enable better demand response and distribution of electricity, which can significantly enhance the efficiency of power systems. Energy storage technologies, such as batteries and thermal storage, allow for the buffering of energy, making renewable sources like solar and wind more viable and reliable. Furthermore, energy management systems (EMS) provide detailed insights into energy consumption patterns and can automate the control of energy resources to maximize efficiency.
The push towards greener energy solutions is not only a response to increasing environmental concerns but also to economic incentives. Governments around the world are offering various subsidies and tax incentives for energy efficiency projects, which can be explored further on platforms like the International Energy Agency (https://www.iea.org/) and Energy Manager Today (https://www.energymanagertoday.com/).
Looking ahead, several key trends are expected to shape various industries and global markets. Technological advancements such as AI, machine learning, and continued innovations in IoT are set to further disrupt traditional business models. In healthcare, personalized medicine and telehealth are anticipated to become more prevalent, driven by advancements in genomic sequencing and wearable health technology.
In the realm of transportation, electric and autonomous vehicles are expected to gain significant traction, altering not just how people commute but also impacting the global oil demand and automotive industry structures. Urbanization and smart city projects are likely to prioritize sustainability, leading to increased investments in green infrastructure and technologies.
The future also holds potential for significant advancements in quantum computing, which could revolutionize fields ranging from cryptography to materials science. As these technologies mature, they will likely lead to new products and services that we can hardly imagine today. For more detailed predictions on future trends, resources like Forbes Technology and TechCrunch (https://techcrunch.com/) offer extensive coverage and insights.
Each of these points reflects the dynamic nature of technological and economic landscapes, indicating a future that is increasingly interconnected and driven by innovation. For further insights into future trends in technology, you can explore articles like AI on the Job: Powering Business Operations Through Automation and Innovation which discusses the role of AI in powering business operations through automation and innovation, potentially highlighting its applications, benefits, and future trends.
6.1. Evolving Capabilities of AI and Digital Twins
The integration of Artificial Intelligence (AI) with digital twins represents a significant leap in the capabilities of technology to simulate, predict, and manage real-world systems. A digital twin is a virtual model designed to accurately reflect a physical object. AI enhances these models through its ability to analyze data and learn from it, thereby improving the accuracy and functionality of the twins. For instance, in industries like manufacturing, AI-driven digital twins can predict equipment failures before they occur, schedule timely maintenance, and optimize production processes for increased efficiency.
The evolution of AI capabilities has also expanded the scope of digital twins beyond traditional sectors. In healthcare, digital twins can simulate the human body or organs to predict how they would respond to treatments without direct experimentation on the patient. This capability could revolutionize personalized medicine and treatment plans tailored specifically to individual genetic profiles and health conditions.
Here is an architectural diagram illustrating the integration of AI with digital twins in various sectors such as manufacturing and healthcare:

Further reading on the advancements in AI and digital twins can be found on websites like Forbes and TechCrunch, which regularly feature articles on the latest developments in these technologies. For example, Forbes discusses how AI-driven digital twins are transforming industries, while TechCrunch often explores the intersection of AI and healthcare (TechCrunch). Additionally, you can explore detailed use cases and insights on AI in various applications at Rapid Innovation (Rapid Innovation).
6.2. Impact on Workforce and Skill Requirements
The rapid advancement of technology, particularly in AI and automation, is significantly altering workforce dynamics and skill requirements across various industries. As machines take over more routine and repetitive tasks, there is a growing demand for skills that are uniquely human, such as creative problem-solving, emotional intelligence, and strategic thinking. This shift necessitates a reevaluation of educational systems and training programs to prepare the future workforce adequately.
Moreover, the rise of AI and automation could lead to job displacement, but it also creates opportunities for new job roles. For instance, as AI systems become more prevalent, there will be an increased need for AI specialists, data scientists, and machine learning engineers. Additionally, as digital platforms become the norm, there will be a higher demand for cybersecurity experts to protect sensitive information from digital threats.
Organizations like the World Economic Forum and McKinsey & Company provide insights and reports on how AI impacts employment and skills. The World Economic Forum’s "Future of Jobs Report" (World Economic Forum) and McKinsey’s analyses (McKinsey & Company) are excellent resources for understanding these trends.
6.3. Regulatory and Ethical Considerations
As AI technologies become more sophisticated and integrated into daily life, regulatory and ethical considerations are increasingly important. The ability of AI systems to process and analyze vast amounts of personal data raises significant privacy concerns. There is a need for robust data protection laws that govern the use of AI and ensure that individuals' privacy is not compromised. Additionally, as AI systems make more decisions, questions about accountability and transparency arise. Who is responsible when an AI system makes a mistake?
Ethical considerations are also paramount, especially in terms of bias and fairness. AI systems are only as unbiased as the data they are trained on, and if this data is skewed, the AI's decisions will be too. This can perpetuate existing inequalities in society unless proactive measures are taken to ensure fairness in AI algorithms.
Organizations such as the European Union have been at the forefront of establishing regulations for AI, with proposals for new legal frameworks aimed at safeguarding fundamental rights and ensuring safety (European Commission). Similarly, ethical guidelines and frameworks are being developed by academic and professional bodies to guide the responsible development and deployment of AI technologies. For more insights into AI and its regulatory landscape, you can visit Rapid Innovation’s detailed discussion on AI regulations (Rapid Innovation).
‍