Table Of Contents
Category
Artificial Intelligence
Healthcare & Medicine
1. Introduction to Computer Vision in Healthcare
Computer vision is a field of artificial intelligence that enables machines to interpret and understand visual information from the world. In healthcare, computer vision plays a transformative role by enhancing diagnostic accuracy, improving patient outcomes, and streamlining workflows. The application of computer vision in healthcare is becoming increasingly prevalent as it offers innovative solutions to complex medical challenges.
- It involves the use of algorithms and models to analyze images and videos.
- The technology can process vast amounts of data quickly, which is crucial in medical settings.
- Rest development in Computer vision systems can assist healthcare professionals by providing insights that may not be immediately apparent.
The integration of computer vision in healthcare is driven by the need for efficiency and precision in medical practices. As the volume of medical data continues to grow, the ability to analyze this data effectively becomes increasingly important. The rise of computer vision applications in healthcare is a testament to its potential in revolutionizing patient care.
2. Applications of Computer Vision in Healthcare
Computer vision has a wide range of applications in healthcare, significantly impacting various aspects of patient care and medical research. The application of computer vision in the medical field is diverse, ranging from diagnostics to treatment planning.
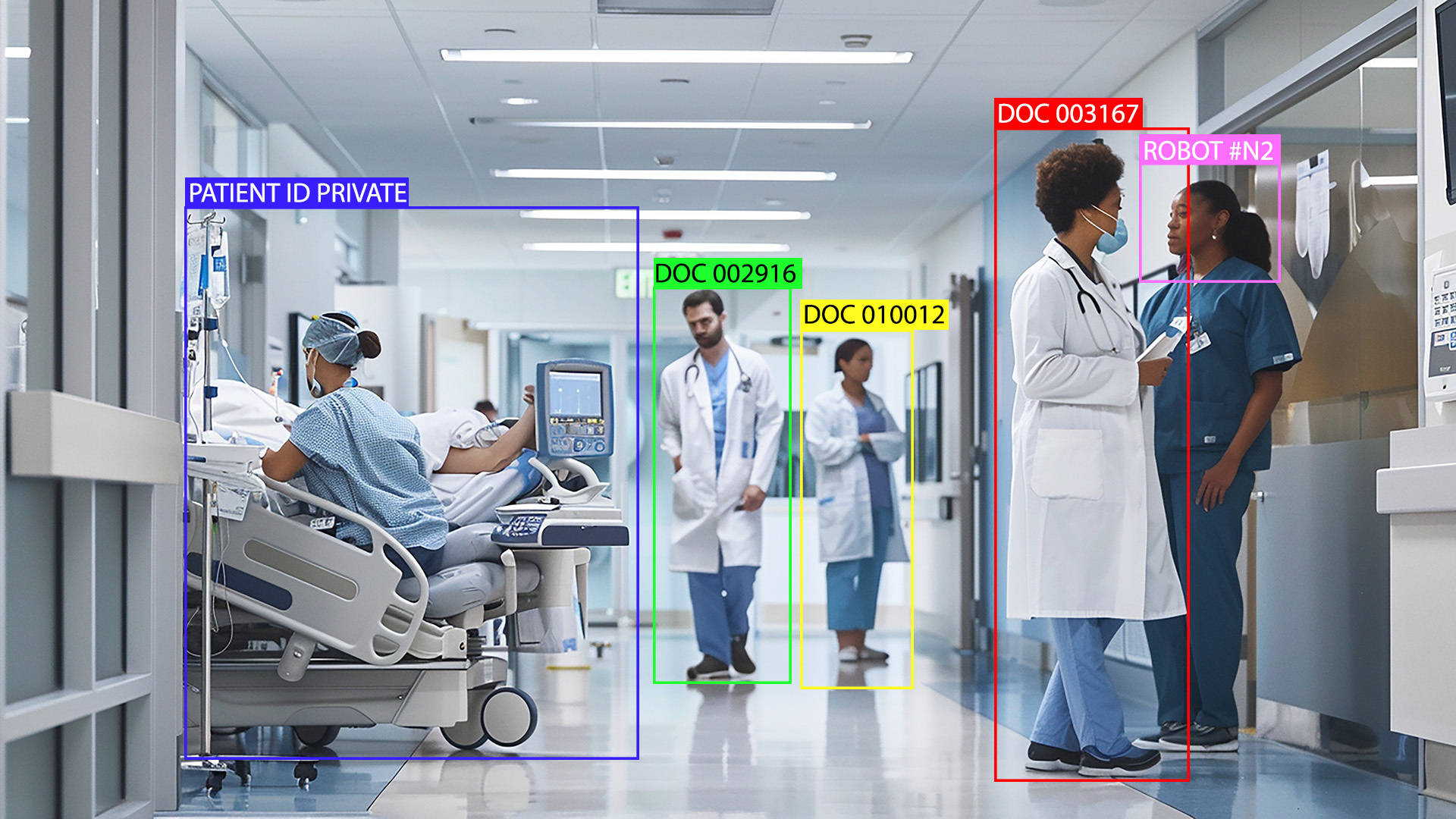
- It enhances diagnostic processes by providing detailed analysis of medical images.
- It aids in monitoring patient conditions through real-time image analysis.
- It supports surgical procedures by providing augmented reality overlays.
The applications of computer vision in healthcare can be categorized into several key areas, each contributing to improved healthcare delivery.
2.1. Medical Imaging Analysis
Medical imaging analysis is one of the most prominent applications of computer vision in healthcare. It involves the use of advanced algorithms to interpret images obtained from various imaging modalities, showcasing the importance of computer vision in healthcare diagnostics.
Types of Medical Imaging:
- X-rays
- CT scans
- MRIs
- Ultrasounds
Key Benefits:
- Increased accuracy in detecting abnormalities such as tumors, fractures, and other conditions.
- Reduction in the time required for image interpretation, allowing for faster diagnosis.
- Enhanced ability to track disease progression over time through comparative analysis.
Techniques Used:
- Deep learning algorithms, particularly convolutional neural networks (CNNs), are commonly employed for image classification and segmentation.
- Image enhancement techniques improve the quality of images for better analysis.
- Automated systems can flag potential issues for further review by radiologists.
Impact on Radiology:
- Computer vision tools assist radiologists by providing second opinions and reducing the likelihood of human error.
- They can prioritize cases based on urgency, ensuring that critical conditions are addressed promptly.
Research and Development:
- Ongoing research focuses on improving the accuracy and reliability of computer vision systems in medical imaging.
- Collaborative efforts between tech companies and healthcare institutions are driving innovation in this field, leading to new computer vision use cases in healthcare.
The integration of computer vision in medical imaging analysis not only enhances diagnostic capabilities but also supports healthcare professionals in delivering high-quality patient care. The application of computer vision for healthcare is paving the way for more efficient and effective medical practices.
At Rapid Innovation, we understand the complexities of implementing such advanced technologies in healthcare settings. Our expertise in Computer Vision Development allows us to tailor solutions that meet the unique needs of our clients. By partnering with us, healthcare organizations can expect to achieve greater ROI through improved operational efficiency, enhanced patient outcomes, and reduced costs associated with diagnostic errors. Our commitment to innovation ensures that you stay ahead in a rapidly evolving industry, ultimately leading to a more effective healthcare delivery system.
2.1.1. X-ray Analysis
X-ray analysis is a crucial diagnostic tool in medicine, allowing healthcare professionals to visualize the internal structures of the body. It is particularly useful for identifying fractures, infections, and tumors.
- X-rays work by passing radiation through the body, which is absorbed differently by various tissues.
- Dense tissues, like bones, appear white on the X-ray film, while softer tissues appear darker.
- Radiologists interpret X-ray images by looking for abnormalities in size, shape, and position of structures.
- Common conditions diagnosed through X-ray include: Â
- Bone fractures
- Arthritis
- Pneumonia
- X-ray analysis is quick and non-invasive, making it a first-line imaging technique in many cases.
- Limitations include exposure to radiation and difficulty in visualizing soft tissues.
- X-ray imaging techniques are among the most common medical imaging techniques used in diagnostic imaging methods.
2.1.2. MRI Interpretation
Magnetic Resonance Imaging (MRI) is a powerful imaging technique that uses strong magnetic fields and radio waves to generate detailed images of organs and tissues.
- MRI is particularly effective for imaging soft tissues, such as the brain, muscles, and ligaments.
- The images produced can be in various planes, providing a comprehensive view of the area being examined.
- Radiologists interpret MRI scans by assessing: Â
- Signal intensity differences between various tissues
- The presence of lesions or abnormalities
- Anatomical structures and their relationships
- Common uses of MRI include: Â
- Diagnosing brain tumors
- Evaluating joint injuries
- Assessing spinal cord conditions
- MRI is non-invasive and does not involve ionizing radiation, making it safer for repeated use.
- However, it can be time-consuming and may not be suitable for patients with certain implants or claustrophobia.
- MRI imaging techniques are part of the broader category of medical imaging methods and are essential in diagnostic imaging technologies.
2.1.3. CT Scan Processing
Computed Tomography (CT) scans combine X-ray images taken from different angles and use computer processing to create cross-sectional images of the body.
- CT scans provide more detailed images than standard X-rays, particularly for complex structures.
- The process involves: Â
- Rotating X-ray equipment around the patient
- Capturing multiple images from various angles
- Using computer algorithms to reconstruct these images into a 3D format
- CT scans are particularly useful for: Â
- Detecting tumors and cancers
- Evaluating internal injuries and bleeding
- Guiding biopsies and other procedures
- The speed of CT scans makes them ideal for emergency situations.
- However, they involve higher radiation exposure compared to standard X-rays, which is a consideration in patient safety.
- CT scans can also be enhanced with contrast agents to improve the visibility of certain structures, and they are a key component of advanced imaging technologies in medical imaging.
- Â
- 2.1.4. Ultrasound Image Analysis
Ultrasound image analysis is a critical component in medical diagnostics, particularly in obstetrics, cardiology, and musculoskeletal imaging. This technique utilizes sound waves to create images of internal body structures, allowing for real-time visualization.
Key aspects of ultrasound image analysis include:
- Image Acquisition: The process begins with the generation of sound waves by a transducer, which are then reflected back from tissues to create images.
- Image Interpretation: Radiologists or sonographers analyze the images for abnormalities, such as tumors, cysts, or organ malformations.
- Quantitative Analysis: Advanced software tools can measure dimensions, volumes, and flow rates, providing quantitative data to support clinical decisions.
- Artificial Intelligence (AI): AI algorithms are increasingly being integrated into ultrasound analysis to enhance image interpretation, reduce human error, and improve diagnostic accuracy.
- 3D and 4D Imaging: Modern ultrasound technology allows for three-dimensional and four-dimensional imaging, providing more detailed views of structures and movements, particularly in fetal imaging.
- Spectral Analysis Ultrasound: This technique enhances the evaluation of blood flow and tissue characteristics, providing additional insights during ultrasound image analysis.
- Ultrasound Image Analysis: The integration of advanced algorithms allows for improved detection of subtle changes in tissue, enhancing diagnostic capabilities.
2.2. Pathology and Histology
Pathology and histology are essential fields in medicine that focus on the study of diseases and the microscopic examination of tissues, respectively. They play a vital role in diagnosing conditions and understanding disease mechanisms.
Important elements of pathology and histology include:
- Tissue Examination: Pathologists examine tissue samples (biopsies) to identify disease processes, such as cancer or infections.
- Histological Techniques: Techniques like staining and sectioning are used to prepare tissue samples for microscopic analysis, highlighting specific cellular structures.
- Diagnostic Pathology: This branch focuses on diagnosing diseases based on histological findings, guiding treatment decisions.
- Molecular Pathology: This emerging field combines traditional pathology with molecular biology to understand the genetic and molecular basis of diseases.
- Clinical Relevance: Accurate pathology and histology are crucial for effective patient management, influencing treatment plans and prognoses.
2.2.1. Digital Pathology
Digital pathology is the practice of converting glass slides into digital images that can be analyzed, shared, and stored electronically. This innovation is transforming the field of pathology by enhancing workflow efficiency and diagnostic accuracy.
Key features of digital pathology include:
- Whole Slide Imaging (WSI): This technology captures high-resolution images of entire tissue slides, allowing for comprehensive analysis.
- Remote Access: Pathologists can access digital slides from anywhere, facilitating collaboration and second opinions across geographical boundaries.
- Image Analysis Tools: Advanced software can assist in quantifying features, detecting patterns, and even identifying specific cell types, improving diagnostic precision.
- Integration with AI: AI algorithms can analyze digital pathology images to assist in diagnosis, predict outcomes, and streamline workflows.
- Regulatory Approval: Digital pathology systems are increasingly receiving regulatory approvals, making them viable options for clinical use in many regions.
Digital pathology is paving the way for more efficient and accurate diagnostic processes, ultimately improving patient care.
At Rapid Innovation, we understand the complexities and challenges faced by healthcare providers in implementing advanced technologies like ultrasound image analysis, ultrasound report analysis, and digital pathology. Our expertise in AI and blockchain development allows us to create tailored solutions that enhance diagnostic accuracy and operational efficiency.
By partnering with us, clients can expect:
- Increased ROI: Our AI-driven solutions streamline workflows, reduce diagnostic errors, and enhance patient outcomes, leading to higher returns on investment.
- Enhanced Collaboration: Our digital solutions facilitate remote access and collaboration among healthcare professionals, ensuring timely and accurate diagnoses.
- Regulatory Compliance: We help clients navigate the regulatory landscape, ensuring that their digital pathology systems meet necessary standards for clinical use.
- Custom Solutions: We work closely with clients to develop bespoke software tools that meet their specific needs, whether in ultrasound analysis or pathology.
- Ongoing Support: Our commitment to client success extends beyond implementation; we provide continuous support and updates to ensure that our solutions evolve with the changing healthcare landscape.
Let Rapid Innovation be your partner in achieving greater efficiency and effectiveness in your medical diagnostics. Together, we can transform the way healthcare is delivered, ultimately improving patient care and outcomes.
2.2.2. Cell Counting and Classification
Cell counting and classification are essential processes in various fields, including biology, medicine, and biotechnology. These processes help researchers and clinicians understand cellular behavior, disease progression, and treatment efficacy.
- Cell Counting: Â
- Involves determining the number of cells in a given sample.
- Techniques include manual counting using a hemocytometer, automated cell counters, and flow cytometry.
- Accurate cell counting is crucial for experiments, diagnostics, and therapeutic applications.
- Classification of Cells: Â
- Refers to categorizing cells based on specific characteristics such as size, shape, and surface markers.
- Common methods include microscopy, immunofluorescence, and molecular profiling.
- Classification aids in identifying cell types, such as stem cells, cancer cells, and immune cells.
- Applications: Â
- In cancer research, cell counting and classification help in assessing tumor growth and response to treatment.
- In immunology, understanding different immune cell types is vital for vaccine development and disease management.
- In biotechnology, cell classification is essential for developing cell-based therapies and regenerative medicine.
2.3. Surgical Assistance
Surgical assistance encompasses various technologies and techniques designed to support surgeons during procedures. These advancements aim to enhance precision, reduce complications, and improve patient outcomes.
- Types of Surgical Assistance: Â
- Robotic systems that provide enhanced dexterity and visualization.
- Image-guided surgery that uses imaging technologies to improve accuracy.
- Augmented reality systems that overlay digital information onto the surgical field.
- Benefits: Â
- Increased precision in surgical procedures, leading to fewer complications.
- Reduced recovery times for patients due to minimally invasive techniques.
- Enhanced training opportunities for surgeons through simulation and real-time feedback.
- Challenges: Â
- High costs associated with advanced surgical technologies.
- The need for specialized training for surgical teams to operate new systems effectively.
- Potential for technical malfunctions during procedures, which can pose risks.
2.3.1. Robotic Surgery Guidance
Robotic surgery guidance refers to the use of robotic systems to assist surgeons in performing complex surgical procedures. These systems enhance the surgeon's capabilities and improve surgical outcomes.
- Key Features of Robotic Surgery: Â
- High-definition 3D visualization that provides a magnified view of the surgical site.
- Articulated robotic arms that can maneuver with greater precision than human hands.
- Enhanced dexterity that allows for complex movements in tight spaces.
- Applications: Â
- Commonly used in urology, gynecology, and general surgery.
- Procedures such as prostatectomies, hysterectomies, and cardiac surgeries benefit from robotic assistance.
- Robotic systems can also be used in minimally invasive surgeries, reducing patient trauma.
- Advantages: Â
- Reduced blood loss and shorter hospital stays for patients.
- Lower risk of infection due to smaller incisions.
- Improved surgeon ergonomics, leading to less fatigue during long procedures.
- Considerations: Â
- The cost of robotic systems can be a barrier for some healthcare facilities.
- Ongoing training and skill development are necessary for surgical teams.
- Patient selection is crucial to ensure the best outcomes with robotic-assisted procedures.
- Â
- 2.3.2. Augmented Reality in Surgery
Augmented Reality (AR) is revolutionizing the surgical landscape by enhancing visualization and improving precision during procedures.
- AR overlays digital information onto the real-world environment, allowing surgeons to see critical data without diverting their attention from the surgical field.
- It can provide 3D models of patient anatomy, helping surgeons plan and execute complex procedures with greater accuracy.
- AR systems can integrate imaging data, such as CT or MRI scans, directly into the surgical view, facilitating better decision-making.
- Surgeons can visualize blood vessels, tumors, and other structures in real-time, reducing the risk of complications.
- Training and education for medical professionals can be enhanced through AR simulations, allowing for hands-on practice in a controlled environment.
- Studies have shown that AR can reduce surgery time and improve outcomes, leading to shorter recovery periods for patients.
- Companies like Microsoft with HoloLens and other startups are pioneering AR applications in surgery, demonstrating its potential in various specialties, including orthopedics and neurosurgery.
- Surgical augmented reality is being utilized in procedures such as augmented reality spine surgery and augmented reality knee replacement, showcasing its versatility.
- Augmedics Xvision and Medivis surgical AR are examples of innovative technologies that are enhancing surgical precision and visualization.
- The integration of AR guided surgery and mixed reality surgery is paving the way for more advanced surgical techniques.
2.4. Remote Patient Monitoring
Remote Patient Monitoring (RPM) is a technology-driven approach that allows healthcare providers to monitor patients' health data outside traditional clinical settings.
- RPM utilizes devices such as wearables, mobile apps, and sensors to collect health metrics like heart rate, blood pressure, and glucose levels.
- This continuous monitoring enables timely interventions, reducing hospital readmissions and improving chronic disease management.
- Patients benefit from increased convenience and comfort, as they can receive care from home while maintaining regular communication with their healthcare providers.
- RPM can enhance patient engagement, as individuals become more aware of their health status and can take proactive steps in their care.
- Data collected through RPM can be analyzed to identify trends, allowing for personalized treatment plans and better health outcomes.
- The COVID-19 pandemic accelerated the adoption of RPM, highlighting its importance in maintaining care continuity during crises.
2.4.1. Fall Detection
Fall detection is a critical component of remote patient monitoring, particularly for elderly individuals or those with mobility issues.
- Fall detection systems use sensors, wearables, or cameras to identify when a fall occurs, triggering alerts to caregivers or emergency services.
- These systems can significantly reduce response times, ensuring that help is provided quickly to prevent further injury.
- Advanced algorithms analyze movement patterns to distinguish between regular activities and falls, minimizing false alarms.
- Many fall detection devices are designed to be user-friendly, encouraging adoption among older adults who may be hesitant to use technology.
- Integration with telehealth services allows for immediate assessment and intervention, enhancing patient safety.
- Studies indicate that effective fall detection systems can lead to a decrease in fall-related injuries and hospitalizations, improving overall quality of life for at-risk populations.
At Rapid Innovation, we leverage cutting-edge technologies like AR and RPM to help healthcare providers enhance patient care and operational efficiency. By partnering with us, clients can expect greater ROI through improved patient outcomes, reduced operational costs, and enhanced engagement. Our expertise in AI and Blockchain development ensures that we deliver tailored solutions that meet the unique needs of each client, driving innovation and success in their healthcare initiatives.
2.4.2. Medication Adherence
Medication adherence refers to the extent to which patients take their medications as prescribed by their healthcare providers. It is a critical factor in the effectiveness of treatment plans and overall health outcomes.
- Importance of Medication Adherence: Â
- Improves health outcomes and reduces the risk of complications.
- Decreases healthcare costs by minimizing hospitalizations and emergency visits.
- Enhances the effectiveness of chronic disease management.
- Factors Influencing Adherence: Â
- Patient-related factors: Â
- Understanding of the disease and treatment.
- Beliefs about medication and its necessity.
- Mental health issues, such as depression or anxiety.
- Treatment-related factors: Â
- Complexity of the medication regimen.
- Side effects experienced from medications.
- Healthcare system factors: Â
- Accessibility of medications.
- Quality of communication between healthcare providers and patients.
- Patient-related factors: Â
- Strategies to Improve Adherence: Â
- Simplifying medication regimens to reduce complexity.
- Utilizing reminders, such as alarms or pill organizers.
- Providing education about the importance of adherence and potential consequences of non-adherence.
- Engaging family members or caregivers in the treatment process.
2.5. Dermatology
Dermatology is the branch of medicine that focuses on the diagnosis and treatment of skin disorders. It encompasses a wide range of conditions, from cosmetic issues to serious diseases.
- Key Areas of Dermatology: Â
- Skin cancer detection and treatment.
- Management of chronic skin conditions like psoriasis and eczema.
- Cosmetic dermatology, including procedures like Botox and fillers.
- Pediatric dermatology, addressing skin issues in children.
- Importance of Dermatology: Â
- Skin is the largest organ and serves as a barrier to protect the body.
- Early detection of skin conditions can lead to better treatment outcomes.
- Dermatological health is closely linked to overall health and well-being.
- Common Dermatological Procedures: Â
- Biopsies to diagnose skin conditions.
- Cryotherapy for wart and lesion removal.
- Laser treatments for various skin issues, including scars and pigmentation.
2.5.1. Skin Lesion Classification
Skin lesions are abnormal changes in the skin's appearance or texture. Classifying these lesions is essential for diagnosis and treatment.
- Types of Skin Lesions: Â
- Primary lesions: Â
- Macules: flat, discolored spots.
- Papules: small, raised bumps.
- Vesicles: fluid-filled blisters.
- Secondary lesions: Â
- Crusts: dried exudate on the skin.
- Scales: flakes of skin.
- Ulcers: open sores.
- Primary lesions: Â
- Classification Systems: Â
- The ABCDE rule for melanoma: Â
- Asymmetry: one half of the mole does not match the other.
- Border: irregular or scalloped edges.
- Color: varied shades of brown, black, or other colors.
- Diameter: larger than 6mm (about the size of a pencil eraser).
- Evolving: changes in size, shape, or color over time.
- The ABCDE rule for melanoma: Â
- Importance of Accurate Classification: Â
- Helps in determining the nature of the lesion (benign vs. malignant).
- Guides treatment decisions and follow-up care.
- Aids in research and understanding of skin diseases.
- Tools for Classification: Â
- Dermatoscopy: a non-invasive tool that allows for detailed examination of skin lesions.
- Histopathological examination: biopsy samples analyzed under a microscope for definitive diagnosis.
At Rapid Innovation, we understand the complexities of healthcare technology and the importance of medication adherence, including medication compliance and non-adherence, and dermatological health. Our AI and Blockchain solutions can streamline patient management systems, enhance communication between healthcare providers and patients, and ultimately improve adherence rates, including prescription adherence and adherence with medication. By partnering with us, clients can expect greater ROI through reduced healthcare costs, improved patient outcomes, and efficient management of chronic conditions, addressing issues like drug non-adherence and health adherence. Let us help you achieve your goals effectively and efficiently.
2.5.2. Wound Assessment
Wound assessment is a critical component in the management and treatment of wounds. It involves a systematic evaluation of the wound's characteristics to determine the appropriate care plan.
- Types of Wounds: Â
- Acute wounds (e.g., surgical, traumatic)
- Chronic wounds (e.g., diabetic ulcers, pressure ulcers)
- Assessment Parameters: Â
- Size: Length, width, and depth measurements
- Appearance: Color, texture, and presence of necrotic tissue
- Exudate: Amount, color, and odor of drainage
- Surrounding Skin: Condition of the skin around the wound, including signs of infection
- Tools for Assessment: Â
- Visual inspection
- Measurement tools (rulers, digital imaging)
- Assessment scales (e.g., Bates-Jensen Wound Assessment Tool)
- Smartphone based wound assessment system for patients with diabetes
- Importance of Regular Assessment: Â
- Tracks healing progress
- Identifies complications early
- Guides treatment decisions
- Documentation: Â
- Accurate records are essential for continuity of care
- Helps in evaluating the effectiveness of interventions
3. Technologies and Algorithms
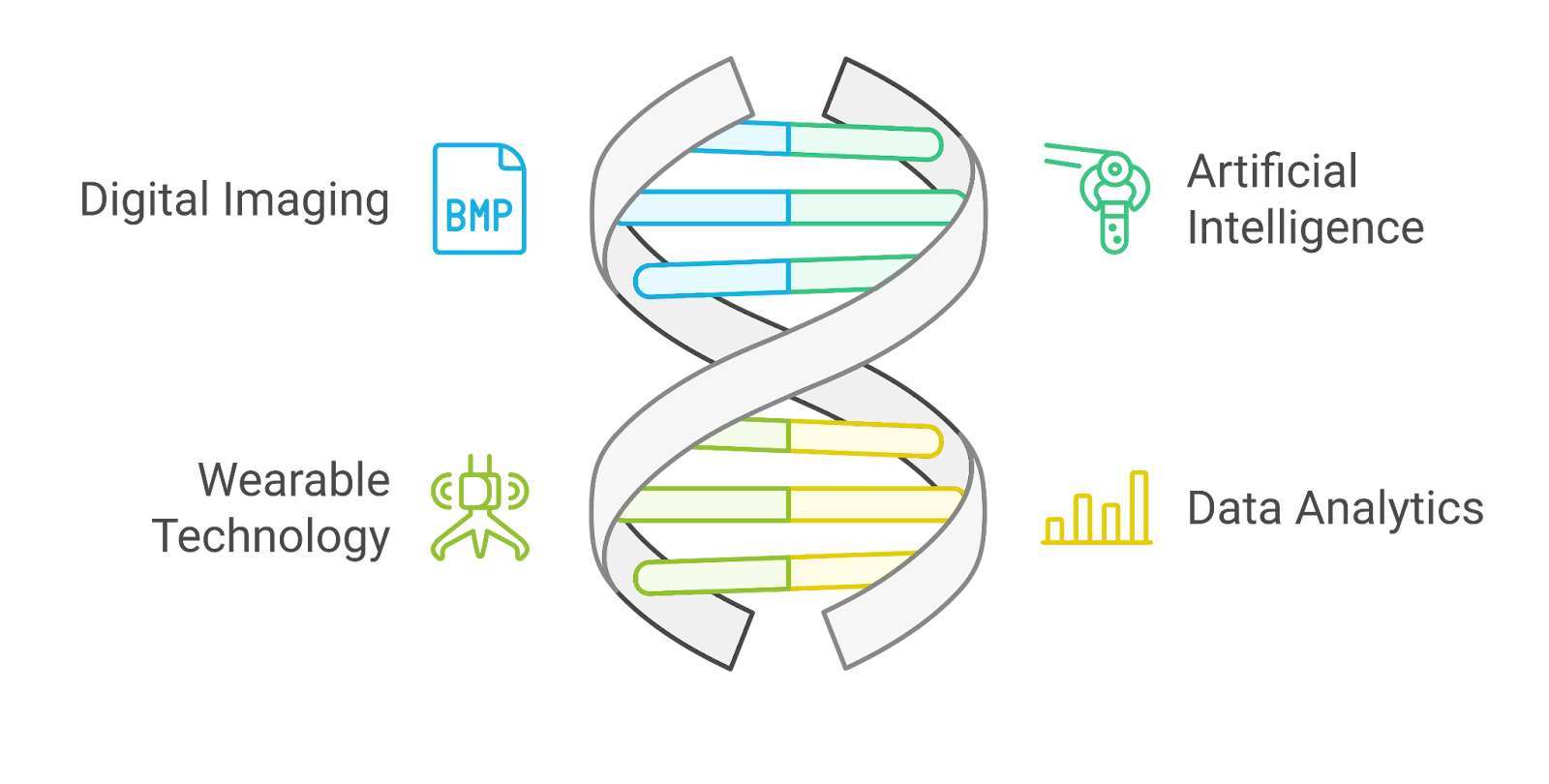
‍
Technological advancements have significantly impacted various fields, including healthcare. In wound care, technologies and algorithms play a vital role in improving assessment and treatment outcomes.
- Digital Imaging: Â
- High-resolution cameras and imaging software allow for detailed wound documentation.
- Enables remote consultations and telemedicine.
- Artificial Intelligence (AI): Â
- AI algorithms can analyze wound images to assess healing progress.
- Machine learning models can predict wound healing outcomes based on historical data.
- Wearable Technology: Â
- Smart bandages equipped with sensors can monitor wound conditions in real-time.
- Data collected can be transmitted to healthcare providers for timely interventions.
- Data Analytics: Â
- Big data analytics can identify trends and patterns in wound healing.
- Helps in developing personalized treatment plans based on patient data.
- Wound assessment technology can enhance the accuracy of these analytics.
3.1. Deep Learning in Medical Image Analysis
Deep learning, a subset of machine learning, has revolutionized medical image analysis, including wound assessment. It utilizes neural networks to process and analyze complex data.
- Image Recognition: Â
- Deep learning algorithms can identify and classify wound types with high accuracy.
- Reduces the need for manual assessment, minimizing human error.
- Segmentation: Â
- Algorithms can segment wound areas from surrounding tissues in images.
- Facilitates precise measurement and monitoring of wound size and depth.
- Predictive Analytics: Â
- Deep learning models can predict healing trajectories based on initial wound characteristics.
- Assists clinicians in making informed decisions regarding treatment options.
- Integration with Electronic Health Records (EHR): Â
- Deep learning systems can be integrated with EHRs to provide comprehensive patient data.
- Enhances clinical decision-making by providing context-specific insights.
- Research and Development: Â
- Ongoing research is focused on improving the accuracy and efficiency of deep learning models.
- Collaboration between tech companies and healthcare institutions is essential for innovation.
- Challenges: Â
- Data quality and availability can impact model performance.
- Ethical considerations regarding patient data privacy and algorithm transparency must be addressed.
At Rapid Innovation, we leverage these advanced technologies and methodologies to help healthcare providers enhance their wound assessment processes. By integrating AI and deep learning into your wound care protocols, we can help you achieve greater efficiency, reduce costs, and ultimately improve patient outcomes. Partnering with us means you can expect increased ROI through streamlined operations, enhanced decision-making capabilities, and improved patient satisfaction. Let us guide you in transforming your wound care management with our cutting-edge solutions.
3.2. Convolutional Neural Networks (CNNs)
Convolutional Neural Networks (CNNs) represent a powerful class of deep learning algorithms specifically designed for processing structured grid data, such as images. These networks are adept at automatically and adaptively learning spatial hierarchies of features from input images, making them invaluable in various applications, including image enhancement and image preprocessing.
Key components of CNNs:
- Convolutional Layers: These layers perform convolution operations on the input, utilizing filters to extract essential features like edges, textures, and patterns, which are crucial for tasks such as edge detection image processing and sobel edge detection.
- Activation Functions: Non-linear functions, such as ReLU (Rectified Linear Unit), are employed to introduce non-linearity into the model, enabling it to learn complex patterns effectively.
- Pooling Layers: These layers reduce the spatial dimensions of the feature maps, which helps decrease computational load and mitigate overfitting. Max pooling is a commonly used technique.
- Fully Connected Layers: At the conclusion of the network, fully connected layers integrate the features learned by previous layers to generate final predictions.
Advantages of CNNs:
- Parameter Sharing: Filters are reused across the entire image, which reduces the number of parameters and enhances efficiency.
- Translation Invariance: CNNs possess the ability to recognize objects regardless of their position within the image.
- Feature Hierarchy: They learn features at multiple levels, ranging from simple edges to complex objects.
Applications of CNNs:
- Image classification
- Facial recognition
- Medical image analysis, including medical image segmentation
- Autonomous vehicles
3.3. Object Detection and Segmentation
Object detection and segmentation are pivotal tasks in computer vision that focus on identifying and localizing objects within images.
Object Detection:
- This process entails identifying instances of objects within an image and drawing bounding boxes around them.
- Popular algorithms include:
- YOLO (You Only Look Once): A real-time object detection system that predicts bounding boxes and class probabilities directly from full images.
- Faster R-CNN: This algorithm combines region proposal networks with CNNs for precise object detection.
Applications:
- Surveillance systems
- Autonomous driving
- Retail analytics
Image Segmentation:
- Segmentation advances the process by classifying each pixel in the image, allowing for precise localization of objects.
Types of segmentation:
- Semantic Segmentation: Assigns a class label to each pixel, treating all instances of a class uniformly.
- Instance Segmentation: Differentiates between separate instances of the same class, providing a more detailed understanding of the scene.
Popular frameworks:
- U-Net: Widely utilized in biomedical image segmentation, particularly in medical image segmentation.
- Mask R-CNN: An extension of Faster R-CNN that performs instance segmentation.
Applications:
- Medical imaging (tumor detection)
- Autonomous navigation
- Augmented reality
- Image segmentation images are often used to improve the accuracy of these applications.
3.4. Image Registration and Fusion
Image registration and fusion are advanced techniques employed to align and combine multiple images into a single coherent representation.
Image Registration:
- This process involves aligning two or more images of the same scene captured at different times, from various viewpoints, or by different sensors.
Steps in image registration:
- Feature Detection: Identifying key points or features within the images.
- Feature Matching: Establishing correspondences between features in different images.
- Transformation Estimation: Calculating the transformation required to align the images.
- Image Resampling: Adjusting pixel values to create a single aligned image.
Applications:
- Medical imaging (aligning scans from different modalities)
- Remote sensing (combining satellite images)
- Robotics (localization and mapping)
Image Fusion:
- This technique merges multiple images to create a single image that contains more information than any of the individual images.
Types of image fusion:
- Pixel-level Fusion: Combines pixel values from different images, often utilized in multi-spectral imaging.
- Feature-level Fusion: Merges features extracted from different images, beneficial in object recognition tasks.
- Decision-level Fusion: Combines decisions made by different classifiers based on the images.
Applications:
- Enhancing image quality in low-light conditions through techniques like unsharp masking
- Improving object detection accuracy
- Creating high-resolution images from low-resolution inputs, often involving image preprocessing in Python and OpenCV image preprocessing.
These techniques are essential across various fields, including medical imaging, remote sensing, and computer vision, enabling more accurate analysis and interpretation of visual data. By partnering with Rapid Innovation, clients can leverage these advanced methodologies to achieve greater efficiency and effectiveness in their projects, ultimately leading to enhanced ROI and competitive advantage.
4. Challenges and Limitations

‍
The rapid advancement of technology and data analytics has brought numerous benefits, but it also presents significant challenges and limitations. Understanding these issues is crucial for organizations looking to leverage data effectively.
4.1. Data Privacy and Security
Data privacy and security are paramount concerns in today's digital landscape. Organizations must navigate various challenges to protect sensitive information.
- Increasing data breaches: Cyberattacks are becoming more sophisticated, leading to a rise in data breaches. In 2021, there were over 1,500 reported data breaches in the U.S. alone, exposing millions of records.
- User consent: Obtaining explicit consent from users for data collection and processing is essential. Many users are unaware of how their data is used, leading to distrust and potential backlash against organizations.
- Data storage vulnerabilities: Storing large volumes of data increases the risk of unauthorized access. Organizations must implement robust security measures to protect against hacking and data theft.
- Compliance with privacy laws: Regulations like GDPR and CCPA impose strict requirements on how organizations handle personal data. Non-compliance can result in hefty fines and reputational damage. The GDPR protected data framework emphasizes the importance of protecting personal information.
- Balancing data utility and privacy: Organizations often struggle to find the right balance between utilizing data for insights and ensuring user privacy. This tension can hinder innovation and data-driven decision-making, particularly in the context of data privacy and security.
4.2. Regulatory Compliance
Regulatory compliance is a critical aspect of managing data and technology. Organizations face various challenges in adhering to legal requirements.
- Complex regulatory landscape: The regulatory environment is constantly evolving, with new laws and guidelines emerging regularly. Organizations must stay informed about changes to ensure compliance, especially with the GDPR general data protection regulations.
- Resource allocation: Ensuring compliance often requires significant resources, including time, personnel, and financial investment. Smaller organizations may struggle to allocate sufficient resources for compliance efforts.
- Risk of non-compliance: Failing to comply with regulations can lead to severe consequences, including fines, legal action, and damage to reputation. Organizations must prioritize compliance to mitigate these risks, particularly in relation to personal data protection.
- Training and awareness: Employees must be trained on compliance requirements and best practices. Lack of awareness can lead to unintentional violations and increased vulnerability to regulatory scrutiny.
- Global compliance challenges: For organizations operating internationally, navigating different regulatory frameworks can be particularly challenging. Each country may have its own set of rules, complicating compliance efforts, especially regarding data privacy and security.
At Rapid Innovation, we understand these challenges and are committed to helping organizations navigate the complexities of data privacy, security, and regulatory compliance. Our expertise in AI and Blockchain technology allows us to provide tailored solutions that not only address these issues but also enhance operational efficiency.
By partnering with us, clients can expect:
- Enhanced Security Measures: We implement cutting-edge security protocols to safeguard sensitive data, reducing the risk of breaches and ensuring compliance with privacy laws, including database privacy and security.
- Streamlined Compliance Processes: Our consulting services help organizations stay ahead of regulatory changes, ensuring that compliance efforts are efficient and effective, particularly in the context of data protection data security.
- Increased ROI: By leveraging our innovative solutions, clients can optimize their data utilization while maintaining user privacy, leading to better decision-making and increased profitability.
- Expert Guidance: Our team of experts provides ongoing support and training, ensuring that your organization is well-equipped to handle compliance challenges and data management effectively.
In a rapidly evolving technological landscape, let Rapid Innovation be your trusted partner in achieving your goals efficiently and effectively.
4.3. Integration with Existing Healthcare Systems
Seamless integration is crucial for the successful implementation of new technologies in healthcare. Existing healthcare systems often include Electronic Health Records (EHR), laboratory information systems, and imaging systems. Interoperability is a key challenge; systems must communicate effectively to share data.
Integration can enhance patient care by:
- Providing a comprehensive view of patient history.
- Reducing duplication of tests and procedures.
- Streamlining workflows for healthcare providers.
Technologies such as APIs (Application Programming Interfaces) can facilitate integration, including medical device integration and medical device integration software. Data security and privacy must be prioritized during integration to comply with regulations like HIPAA. Training staff on new systems is essential to ensure smooth adoption and usage. Successful case studies demonstrate that integrated systems, such as healthcare integration software and capsule medical device integration, can lead to improved patient outcomes and operational efficiency.
4.4. Interpretability and Explainability
Interpretability refers to the ability to understand how a model makes decisions. Explainability is the degree to which a human can comprehend the reasons behind a model's output. Both are critical in healthcare, where decisions can significantly impact patient lives.
Key reasons for prioritizing interpretability and explainability include:
- Building trust among healthcare professionals and patients.
- Ensuring compliance with regulatory standards.
- Facilitating better clinical decision-making.
Techniques to enhance interpretability include:
- Using simpler models that are inherently easier to understand.
- Implementing visualization tools to illustrate model predictions.
- Providing clear documentation and rationale for model decisions.
Challenges include balancing complexity and performance; more complex models may yield better results but are harder to interpret. Ongoing research is focused on developing frameworks that enhance both interpretability and performance in healthcare applications.
5. Future Trends and Opportunities
The healthcare landscape is rapidly evolving, driven by technological advancements and changing patient needs. Key trends shaping the future include:
- Increased use of artificial intelligence (AI) and machine learning for diagnostics and treatment planning.
- Growth of telemedicine and remote patient monitoring, especially post-pandemic.
- Emphasis on personalized medicine, tailoring treatments to individual patient profiles.
Opportunities for innovation include:
- Developing predictive analytics tools to anticipate patient needs and outcomes.
- Enhancing patient engagement through mobile health applications and wearable devices.
- Leveraging big data to improve population health management and resource allocation.
Collaboration between tech companies and healthcare providers is essential for the successful implementation of new solutions, including healthcare technology integration and medical device integration with EMR. Regulatory frameworks will need to adapt to keep pace with technological advancements while ensuring patient safety and data security. The focus on value-based care will drive the demand for solutions that demonstrate measurable outcomes and cost-effectiveness. For insights on how AI-driven digital twins can revolutionize industries, see Revolutionizing Industries with AI-Driven Digital Twins.
At Rapid Innovation, we understand the complexities of integrating new technologies into existing healthcare systems. Our expertise in AI and blockchain development allows us to provide tailored solutions that not only enhance operational efficiency but also improve patient outcomes. By partnering with us, clients can expect greater ROI through streamlined processes, enhanced data security, and improved patient engagement. Let us help you navigate the future of healthcare technology with confidence and clarity. For more on the intersection of AI and blockchain in healthcare, explore AI and Blockchain: Revolutionizing Industries.
5.1. AI-Powered Diagnostic Tools
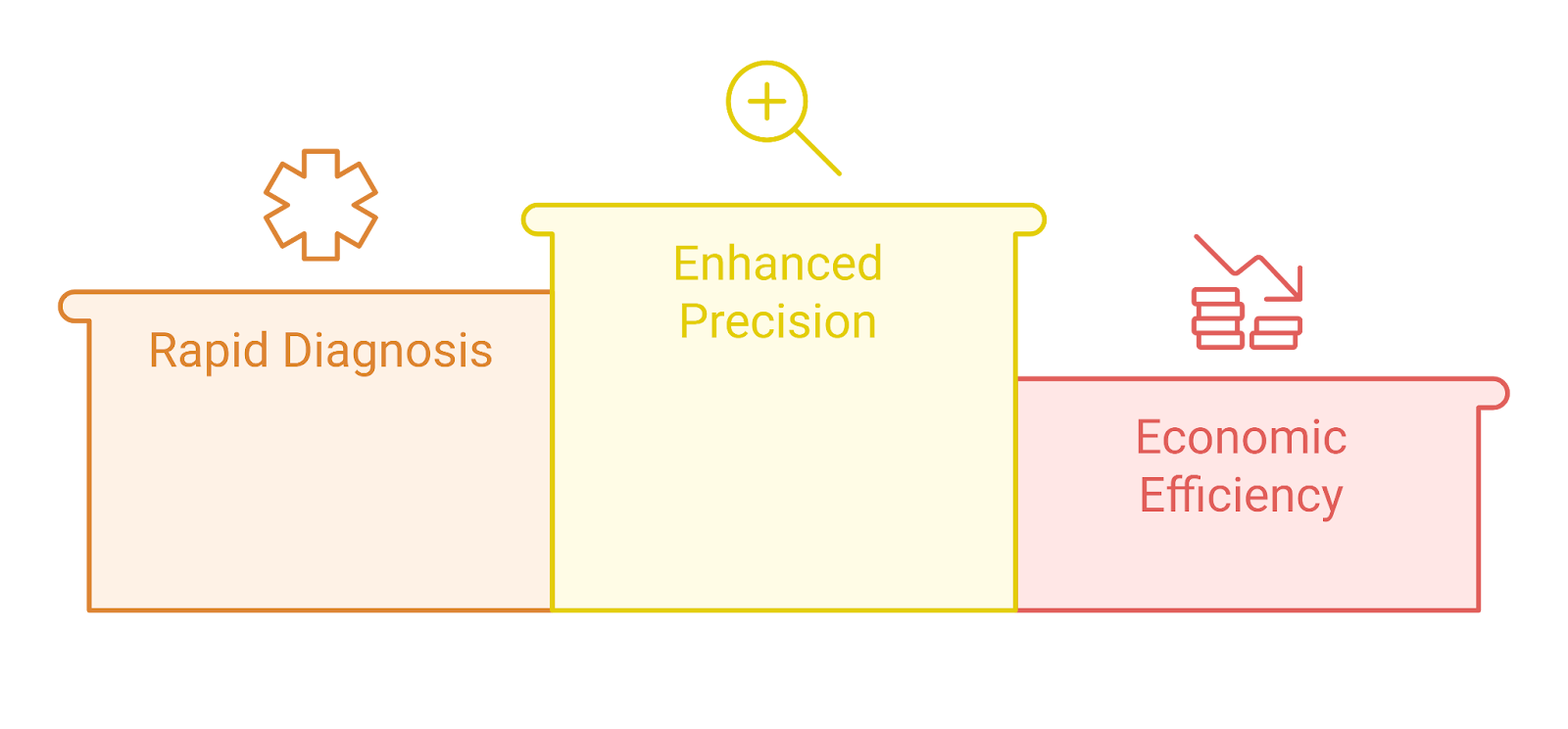
‍
At Rapid Innovation, we understand that AI-powered diagnostic tools, including ai diagnostic tools, are revolutionizing the healthcare landscape by enhancing the accuracy and speed of disease detection. These tools leverage machine learning algorithms and vast datasets to analyze medical images, lab results, and patient histories, ultimately leading to improved patient outcomes.
- Improved accuracy: Our AI systems can identify patterns in data that may be missed by human eyes, leading to earlier and more accurate diagnoses. This precision not only enhances patient care but also reduces the risk of misdiagnosis.
- Speed: Automated analysis can significantly reduce the time required for diagnosis, allowing for quicker treatment initiation. This efficiency translates to better resource management and increased patient throughput for healthcare providers.
- Cost-effectiveness: By streamlining the diagnostic process, our AI tools can help reduce healthcare costs associated with prolonged diagnostic procedures. This cost-saving aspect is crucial for healthcare organizations aiming to maximize their ROI.
- Examples: AI algorithms are being used in radiology to detect conditions like tumors in X-rays and MRIs, and in pathology to analyze biopsy samples. Our firm has successfully implemented these solutions for various clients, resulting in enhanced diagnostic capabilities.
- Integration: Many healthcare providers are integrating our AI tools into their existing systems, enhancing the capabilities of healthcare professionals. This seamless integration ensures that clients can leverage their current infrastructure while benefiting from cutting-edge technology.
5.2. Personalized Treatment Planning
Personalized treatment planning is a cornerstone of modern healthcare, and at Rapid Innovation, we specialize in tailoring medical care to the individual characteristics of each patient. This approach is made possible through the use of AI and data analytics, which can analyze genetic, environmental, and lifestyle factors.
- Precision medicine: Our AI solutions can help identify the most effective treatments based on a patient’s unique genetic makeup, leading to better outcomes. This targeted approach not only improves patient satisfaction but also enhances the overall effectiveness of treatment protocols.
- Data analysis: By analyzing large datasets, our AI can uncover insights about which treatments work best for specific patient profiles. This data-driven approach empowers healthcare providers to make informed decisions.
- Patient engagement: Personalized plans often involve patients more actively in their care, improving adherence to treatment protocols. Our solutions facilitate better communication between patients and providers, fostering a collaborative care environment.
- Predictive analytics: Our AI can predict how patients will respond to certain treatments, allowing for adjustments before side effects occur. This proactive approach minimizes complications and enhances patient safety.
- Examples: Oncology is a leading field for personalized treatment, where our AI helps in selecting targeted therapies based on tumor genetics. Clients who have adopted our solutions have reported significant improvements in treatment outcomes.
5.3. Real-time Disease Monitoring
Real-time disease monitoring is essential for effective healthcare management, and Rapid Innovation is at the forefront of utilizing technology to track patient health continuously. This capability allows for timely interventions and better management of chronic conditions.
- Wearable devices: Our solutions incorporate smartwatches and fitness trackers that can monitor vital signs, activity levels, and other health metrics in real-time. This data is invaluable for both patients and healthcare providers.
- Remote patient monitoring: Telehealth solutions enable healthcare providers to keep track of patients’ health data remotely, facilitating timely adjustments to treatment plans. This flexibility is particularly beneficial in today’s fast-paced healthcare environment.
- Alerts and notifications: Our AI systems can analyze data from monitoring devices and send alerts to healthcare providers or patients when concerning trends are detected. This proactive monitoring can prevent complications and improve patient outcomes.
- Chronic disease management: Real-time monitoring is particularly beneficial for managing conditions like diabetes, hypertension, and heart disease, where timely interventions can prevent complications. Our clients have seen a marked improvement in patient management through our solutions.
- Data integration: These systems can integrate with electronic health records (EHRs), providing a comprehensive view of a patient’s health status. This holistic approach ensures that healthcare providers have all the information they need to make informed decisions.
By partnering with Rapid Innovation, clients can expect to achieve greater ROI through enhanced efficiency, improved patient outcomes, and reduced operational costs. Our expertise in AI and blockchain development positions us as a trusted advisor in the healthcare sector, ready to help you navigate the complexities of modern medical technology, including AI in Healthcare for Advanced Image Analysis.
5.4. Telemedicine and Remote Diagnostics
Telemedicine has revolutionized healthcare delivery by allowing patients to receive medical consultations and diagnostics remotely. This approach has gained significant traction, especially during the COVID-19 pandemic, as it minimizes the need for in-person visits.
- Increased Access to Care Â
- Patients in rural or underserved areas can access specialists without traveling long distances.
- Telemedicine can reduce wait times for appointments, improving overall patient satisfaction.
- Cost-Effectiveness Â
- Remote consultations can lower healthcare costs for both providers and patients.
- Patients save on travel expenses and time off work, while providers can reduce overhead costs.
- Enhanced Monitoring Â
- Remote diagnostics enable continuous monitoring of chronic conditions through wearable devices.
- Patients can share real-time data with healthcare providers, leading to timely interventions.
- Technology Integration Â
- Telemedicine platforms often integrate with electronic health records (EHR), streamlining patient data management.
- Video conferencing tools and mobile health apps facilitate communication between patients and providers.
- Challenges and Limitations Â
- Not all medical conditions can be diagnosed or treated remotely; some require physical examinations.
- Technical issues, such as poor internet connectivity, can hinder effective communication.
6. Ethical Considerations
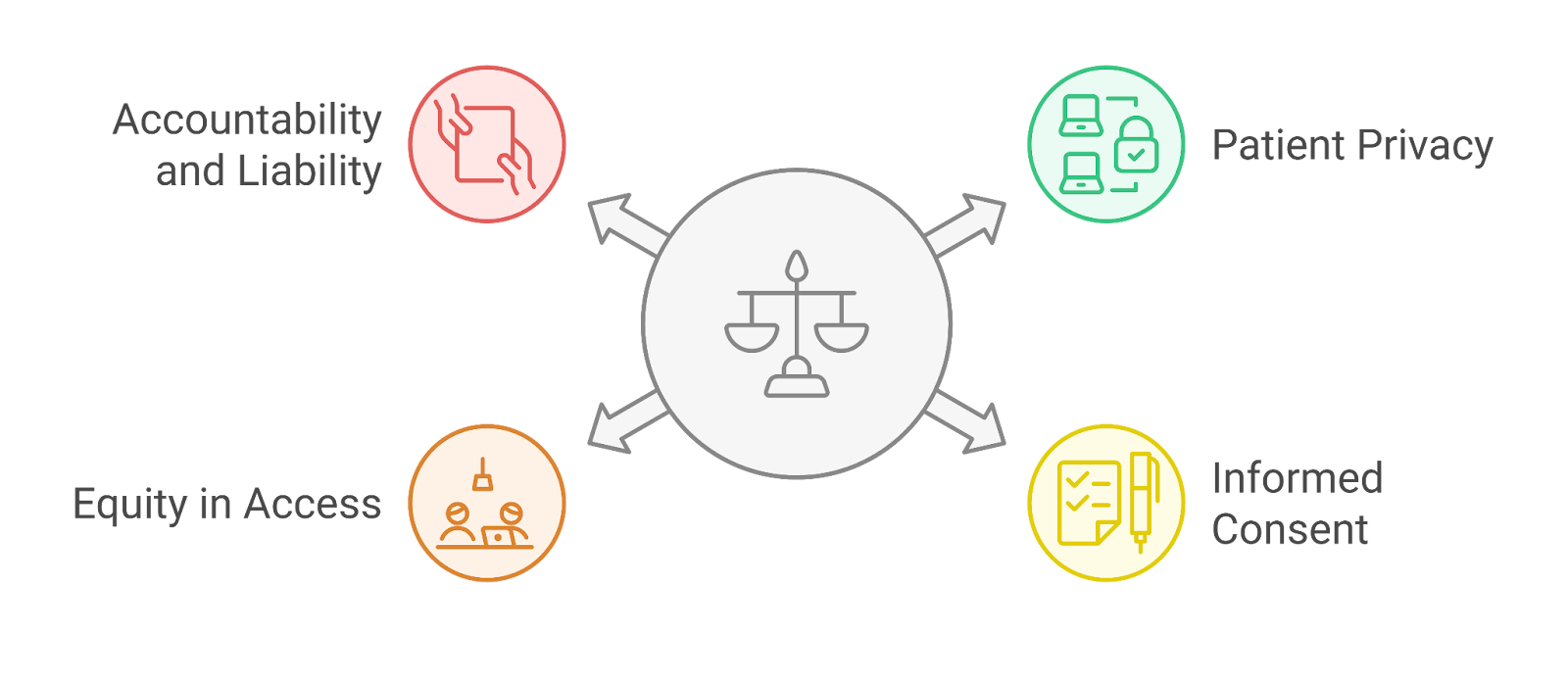
‍
As telemedicine and AI technologies become more prevalent in healthcare, ethical considerations must be addressed to ensure patient safety and trust.
- Patient Privacy and Data Security Â
- Protecting patient information is paramount, as telemedicine involves sharing sensitive health data.
- Healthcare providers must comply with regulations like HIPAA to safeguard patient privacy.
- Informed Consent Â
- Patients should be fully informed about the nature of telemedicine services and any associated risks.
- Clear communication about data usage and sharing practices is essential for informed consent.
- Equity in Access Â
- Disparities in access to technology can lead to unequal healthcare delivery.
- Efforts must be made to ensure that all patients, regardless of socioeconomic status, can benefit from telemedicine.
- Accountability and Liability Â
- Determining liability in cases of misdiagnosis or inadequate care in a remote setting can be complex.
- Clear guidelines are needed to establish accountability among healthcare providers using telemedicine.
6.1. Bias in AI Algorithms
AI algorithms are increasingly used in healthcare for diagnostics, treatment recommendations, and patient management. However, bias in these algorithms can lead to significant ethical concerns.
- Sources of Bias Â
- AI systems can inherit biases from the data they are trained on, which may not represent diverse populations.
- Historical inequalities in healthcare data can perpetuate disparities in treatment recommendations.
- Impact on Patient Care Â
- Biased algorithms can result in misdiagnosis or inappropriate treatment for certain demographic groups.
- Patients from underrepresented backgrounds may receive lower-quality care due to algorithmic bias.
- Mitigation Strategies Â
- Diverse datasets should be used to train AI algorithms to ensure they are representative of the entire population.
- Continuous monitoring and evaluation of AI systems are necessary to identify and correct biases.
- Ethical Responsibility Â
- Developers and healthcare organizations must prioritize ethical considerations in AI deployment.
- Transparency in how algorithms are developed and used can help build trust among patients and providers.
- Regulatory Oversight Â
- Regulatory bodies should establish guidelines for the ethical use of AI in healthcare.
- Ensuring accountability for biased outcomes is crucial for maintaining public trust in AI technologies.
At Rapid Innovation, we understand the complexities of telemedicine and AI integration in healthcare. Our expertise in AI and blockchain development allows us to create tailored solutions that enhance patient care while addressing ethical considerations. By partnering with us, clients can expect increased efficiency, improved patient outcomes, and a greater return on investment. We are committed to helping healthcare organizations navigate the challenges of modern technology while ensuring compliance and ethical standards are met. Let us help you achieve your goals effectively and efficiently with our telemedicine services, telehealth appointments, and telehealth urgent care solutions.
6.2. Patient Consent and Data Ownership
- Patient consent is a critical aspect of healthcare, particularly in the context of patient consent and data ownership.
- Patients must be informed about how their data will be used, stored, and shared.
Key considerations include:
- Transparency: Patients should understand what data is collected and for what purpose.
- Control: Patients should have the ability to opt-in or opt-out of data sharing.
- Revocation: Patients should be able to withdraw consent at any time.
- Data ownership is increasingly being recognized as a patient right.
- Patients often feel a sense of ownership over their health data, leading to demands for more control.
- Legal frameworks, such as HIPAA in the U.S., govern how patient data can be used and shared.
- Ethical considerations also play a role in patient consent, emphasizing respect for patient autonomy.
- The rise of digital health technologies complicates consent processes, as data can be collected from various sources.
- Organizations must develop clear policies and practices to ensure compliance with consent requirements.
- Engaging patients in discussions about data ownership can foster trust and improve healthcare outcomes.
6.3. Human-AI Collaboration in Healthcare
- Human-AI collaboration is transforming the healthcare landscape, enhancing decision-making and patient care.
- AI technologies can analyze vast amounts of data quickly, providing insights that support clinical decisions.
Key benefits of human-AI collaboration include:
- Improved diagnostics: AI can assist in identifying diseases earlier and more accurately.
- Personalized treatment: AI algorithms can analyze patient data to recommend tailored treatment plans.
- Efficiency: AI can automate routine tasks, allowing healthcare professionals to focus on patient care.
Successful collaboration requires:
- Training: Healthcare professionals need to be trained to work effectively with AI tools.
- Trust: Building trust in AI systems is essential for their acceptance in clinical settings.
- Ethical considerations: Ensuring that AI systems are designed and used ethically is crucial.
Challenges include:
- Data quality: AI systems rely on high-quality data; poor data can lead to inaccurate results.
- Integration: Seamlessly integrating AI into existing healthcare workflows can be complex.
- Accountability: Determining who is responsible for decisions made with AI assistance is a key concern.
- The future of healthcare will likely see more sophisticated AI tools that enhance human capabilities rather than replace them.
7. Conclusion and Future Outlook
- The integration of technology in healthcare is reshaping patient care and data management.
- Patient consent and data ownership are becoming increasingly important as digital health solutions proliferate.
- Human-AI collaboration is poised to enhance clinical decision-making and operational efficiency.
Future trends may include:
- Greater emphasis on patient engagement in data sharing and consent processes.
- Continued advancements in AI technologies, leading to more personalized and effective treatments.
- Ongoing discussions about ethical implications and regulatory frameworks governing AI in healthcare.
- The healthcare industry must adapt to these changes to improve patient outcomes and maintain trust.
- Collaboration among stakeholders, including patients, healthcare providers, and technology developers, will be essential for navigating the evolving landscape.
At Rapid Innovation, we understand the complexities of these challenges and are committed to providing tailored solutions that empower healthcare organizations. By leveraging our expertise in AI and blockchain technology, we can help you navigate the intricacies of patient consent and data ownership, ensuring compliance while enhancing patient trust. Our innovative approaches to human-AI collaboration can streamline your operations, improve diagnostic accuracy, and ultimately lead to greater ROI. Partner with us to transform your healthcare delivery and achieve your goals efficiently and effectively.