Table Of Contents
Category
Artificial Intelligence
AIML
IoT
Blockchain
1. Introduction to AI in Underwriting
Artificial Intelligence (AI) is transforming various sectors, and the insurance industry is no exception. Underwriting, the process of evaluating risk and determining policy terms, is increasingly being enhanced by AI technologies. This shift is driven by the need for efficiency, accuracy, and improved customer experiences. AI tools can analyze vast amounts of data quickly, allowing underwriters to make informed decisions faster than traditional methods. AI can automate repetitive tasks, freeing up underwriters to focus on more complex cases. Machine learning algorithms can identify patterns in data that may not be visible to human underwriters. Additionally, enhanced data analytics can lead to more personalized insurance products, such as those offered through AI underwriting.
1.1. The Rise of AI in Insurance Underwriting
The adoption of AI in insurance underwriting has seen significant growth in recent years. Several factors contribute to this trend:
- Increased data availability: The rise of big data has provided underwriters with access to a wealth of information, including social media, IoT devices, and historical claims data.
- Improved technology: Advances in machine learning and natural language processing have made it easier to develop AI systems that can analyze and interpret complex data sets.
- Competitive pressure: Insurers are adopting AI to stay competitive, as those who leverage technology can offer better pricing and faster service.
Key developments include:
- Predictive analytics: AI can forecast future claims based on historical data, helping insurers set premiums more accurately.
- Risk assessment: AI tools can evaluate risk factors more comprehensively, leading to better underwriting decisions, particularly in areas like insurance underwriting AI.
- Enhanced customer experience: AI chatbots and virtual assistants can streamline the application process, making it more user-friendly, which is crucial for AI in insurance underwriting.
1.2. Economic Impacts of AI on the Insurance Industry
The economic implications of AI in the insurance sector are profound and multifaceted. As AI continues to evolve, its impact on the industry can be observed in several areas:
- Cost reduction: Automation of underwriting processes can significantly lower operational costs for insurers, potentially leading to reduced premiums for consumers.
- Increased efficiency: AI can process applications and claims faster, improving turnaround times and customer satisfaction.
- Enhanced profitability: By improving risk assessment and pricing accuracy, insurers can reduce losses and increase profitability, particularly through artificial intelligence underwriting.
Some statistics highlight the economic impact:
- According to a report, AI could save the insurance industry up to $1.1 trillion by 2030 through improved efficiencies and reduced fraud.
- A study found that AI-driven underwriting can reduce the time taken to assess risk by up to 90%.
- The global AI in insurance market is projected to reach $10.14 billion by 2025, growing at a CAGR of 25.4%.
Overall, the integration of AI in underwriting is not just a technological advancement; it represents a fundamental shift in how insurance companies operate, impacting their economic viability and customer engagement strategies. At Rapid Innovation, we leverage our expertise in AI to help clients optimize their underwriting processes, ensuring they achieve greater ROI through enhanced efficiency and accuracy.
Refer to the image for a visual representation of the key concepts discussed in the introduction to AI in underwriting:

1.3. Overcoming Traditional Underwriting Challenges with AI: Rapid Innovation’s AI Agent Solutions for Insurance Underwriting
Traditional underwriting processes often face several challenges that can hinder efficiency and accuracy. These challenges include time-consuming processes, human error, limited data utilization, and inconsistent decision-making. Traditional underwriting can take weeks or even months due to manual data collection and analysis. Additionally, manual processes are prone to errors, which can lead to incorrect risk assessments and financial losses. Underwriters often rely on historical data and subjective judgment, which may not provide a comprehensive view of risk. Furthermore, different underwriters may interpret data differently, leading to inconsistencies in policy approvals and pricing.
AI underwriting solutions can address these challenges by automating data collection, enhancing accuracy, utilizing big data, and standardizing decisions. AI can quickly gather and analyze vast amounts of data from various sources, significantly reducing the time needed for underwriting. Machine learning algorithms can identify patterns and correlations in data that humans might overlook, leading to more accurate risk assessments. AI can process and analyze large datasets, including unstructured data, to provide a more holistic view of an applicant's risk profile. Additionally, AI systems can apply consistent criteria across all applications, reducing variability in decision-making.
Rapid Innovation’s AI agent solutions are transforming the underwriting process by addressing traditional challenges with innovative, data-driven automation. Our AI-powered systems leverage machine learning, predictive analytics, and natural language processing (NLP) to enhance decision-making, optimize risk assessment, and streamline underwriting workflows. By automating routine tasks such as data collection and analysis, our solution accelerates the underwriting process, improving accuracy and efficiency while minimizing human bias. Rapid Innovation’s AI agents provide real-time insights into policyholder risk, enabling underwriters to make faster, more informed decisions. Whether for life, health, auto, or property insurance, our technology helps insurers adapt to evolving market demands, reduce costs, and maintain a competitive edge in the industry.

By integrating AI underwriting solutions into underwriting processes, insurers can improve efficiency, reduce costs, and enhance the overall customer experience, ultimately leading to greater ROI. For more insights on how AI agents are shaping personalized risk evaluation in insurance, check out our website.
2. Use Cases of AI in Underwriting
AI is transforming underwriting across various insurance sectors. Some notable use cases include predictive analytics, fraud detection, personalized pricing, streamlined application processes, and risk assessment. AI models can predict future claims based on historical data, helping underwriters assess risk more accurately. Machine learning algorithms can identify unusual patterns that may indicate fraudulent activity, allowing insurers to take preventive measures. AI can analyze individual customer data to offer personalized premiums, improving customer satisfaction and retention. AI chatbots and virtual assistants can guide applicants through the underwriting process, making it more user-friendly and efficient. Furthermore, AI can evaluate a wide range of factors, including lifestyle choices and social media activity, to provide a more comprehensive risk assessment.
These use cases demonstrate how AI can enhance underwriting processes, making them more efficient and effective.
2.1. Life Insurance Underwriting Innovations
Life insurance underwriting is experiencing significant innovations due to AI technology. Key advancements include automated underwriting, wearable technology integration, telemedicine, behavioral analytics, and enhanced customer experience. AI systems can automate the underwriting process, allowing for quicker approvals and reducing the need for extensive medical exams. Insurers are using data from wearable devices to assess an applicant's health and lifestyle, leading to more accurate risk evaluations. Virtual health assessments can provide underwriters with real-time health data, streamlining the underwriting process and improving accuracy. AI can analyze behavioral data to predict health risks, enabling insurers to offer tailored policies based on individual lifestyles. Additionally, AI-driven platforms can provide instant feedback and policy recommendations, improving the overall customer journey.
These innovations are reshaping the life insurance underwriting landscape, making it more efficient and customer-centric, and ultimately driving better business outcomes for insurers.
Refer to the image for a visual representation of how AI can overcome traditional underwriting challenges.
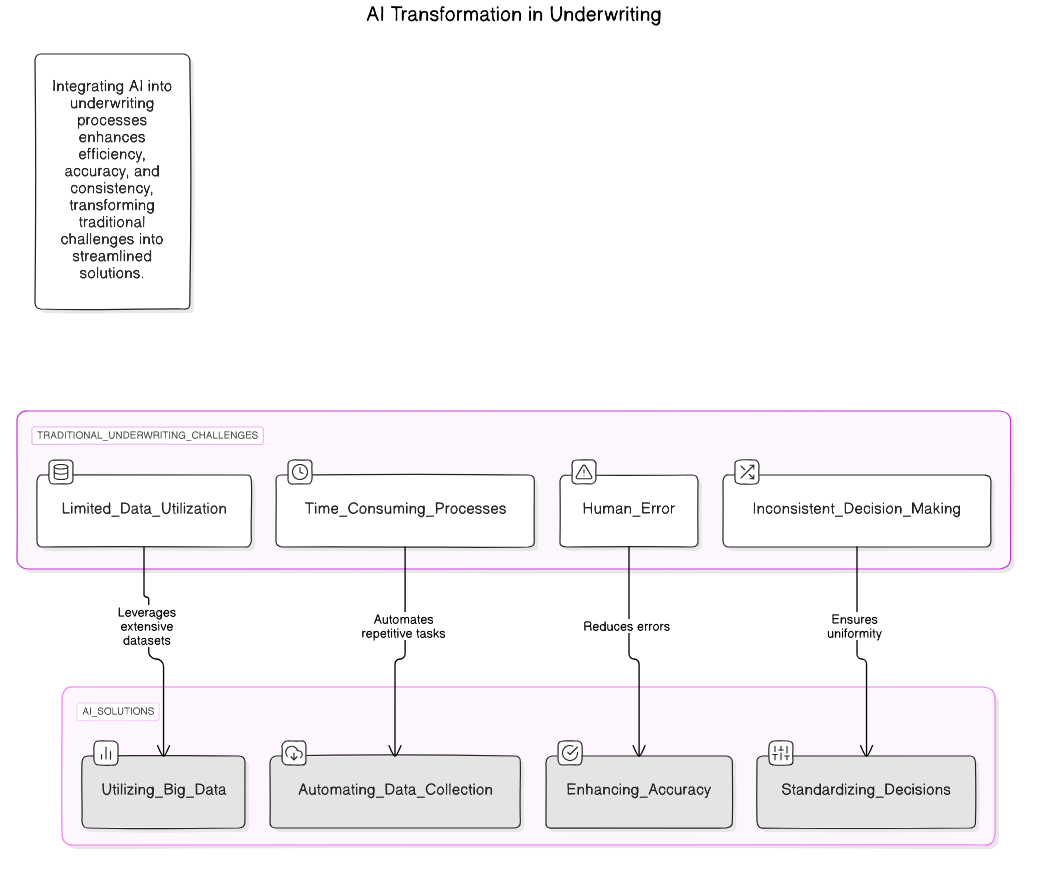
2.2. Property and Casualty Insurance Enhancements
Property and casualty insurance (P&C) has seen significant enhancements in recent years, driven by technological advancements and changing consumer needs. These improvements aim to provide better coverage, streamline processes, and enhance customer experience.
- Advanced Data Analytics: Insurers are leveraging big data to assess risk more accurately. This includes analyzing historical claims data, weather patterns, and even social media activity to predict potential losses. At Rapid Innovation, we utilize AI-driven analytics to help insurers make data-informed decisions, ultimately leading to improved risk management and higher ROI.
- Usage-Based Insurance: Many companies now offer pay-as-you-go models, allowing customers to pay premiums based on actual usage rather than estimates. This is particularly popular in auto and home insurance. Our blockchain solutions can facilitate secure and transparent transactions, ensuring that customers are billed accurately based on their usage.
- Enhanced Customer Experience: Digital platforms and mobile apps enable policyholders to manage their insurance needs easily. Features like instant claims processing and 24/7 customer support are becoming standard. Rapid Innovation can develop customized digital solutions that enhance user engagement and streamline customer interactions, leading to increased customer satisfaction and retention.
- Cyber Insurance Growth: With the rise of cyber threats, P&C insurers are expanding their offerings to include cyber liability coverage, protecting businesses from data breaches and other cyber risks. Our expertise in blockchain technology can help insurers create secure and immutable records of transactions, enhancing trust and security in cyber insurance offerings.
- Regulatory Changes: Insurers are adapting to new regulations that require more transparency and consumer protection, leading to more tailored policies and better customer service. Rapid Innovation can assist in developing compliance solutions that leverage AI to monitor regulatory changes and ensure adherence, minimizing risks and enhancing operational efficiency. For more information on AI insurance solutions, visit Rapid Innovation.
2.3. Health Insurance Underwriting Advances
Health insurance underwriting has evolved significantly, focusing on improving risk assessment and ensuring fair pricing for policyholders. These advances are crucial in a rapidly changing healthcare landscape.
- Predictive Analytics: Insurers are using predictive modeling to assess the health risks of applicants more accurately. This involves analyzing a wide range of data, including medical history, lifestyle choices, and genetic information. Rapid Innovation employs AI algorithms to enhance predictive analytics, enabling insurers to make more informed underwriting decisions and improve profitability.
- Telemedicine Integration: The rise of telehealth services has influenced underwriting practices. Insurers are considering telemedicine usage in their risk assessments, recognizing its role in preventive care and chronic disease management. Our solutions can integrate telemedicine data into underwriting processes, providing a more comprehensive view of applicant health.
- Personalized Health Plans: Advances in underwriting allow for more personalized health insurance plans. Insurers can tailor coverage options based on individual health profiles, leading to better health outcomes and customer satisfaction. Rapid Innovation can help design AI-driven platforms that facilitate personalized health plan offerings, enhancing customer engagement.
- Streamlined Application Processes: Technology has enabled insurers to simplify the application process, reducing the time it takes to underwrite policies. Automated systems can quickly evaluate risk factors and provide instant quotes. Our expertise in automation can help insurers reduce operational costs and improve turnaround times.
- Focus on Wellness Programs: Many insurers are now incorporating wellness initiatives into their underwriting criteria. By incentivizing healthy behaviors, they aim to reduce overall healthcare costs and improve policyholder health. Rapid Innovation can assist in developing AI-driven wellness programs that track and reward healthy behaviors, ultimately leading to lower claims costs.
2.4. Auto Insurance Risk Assessment Improvements
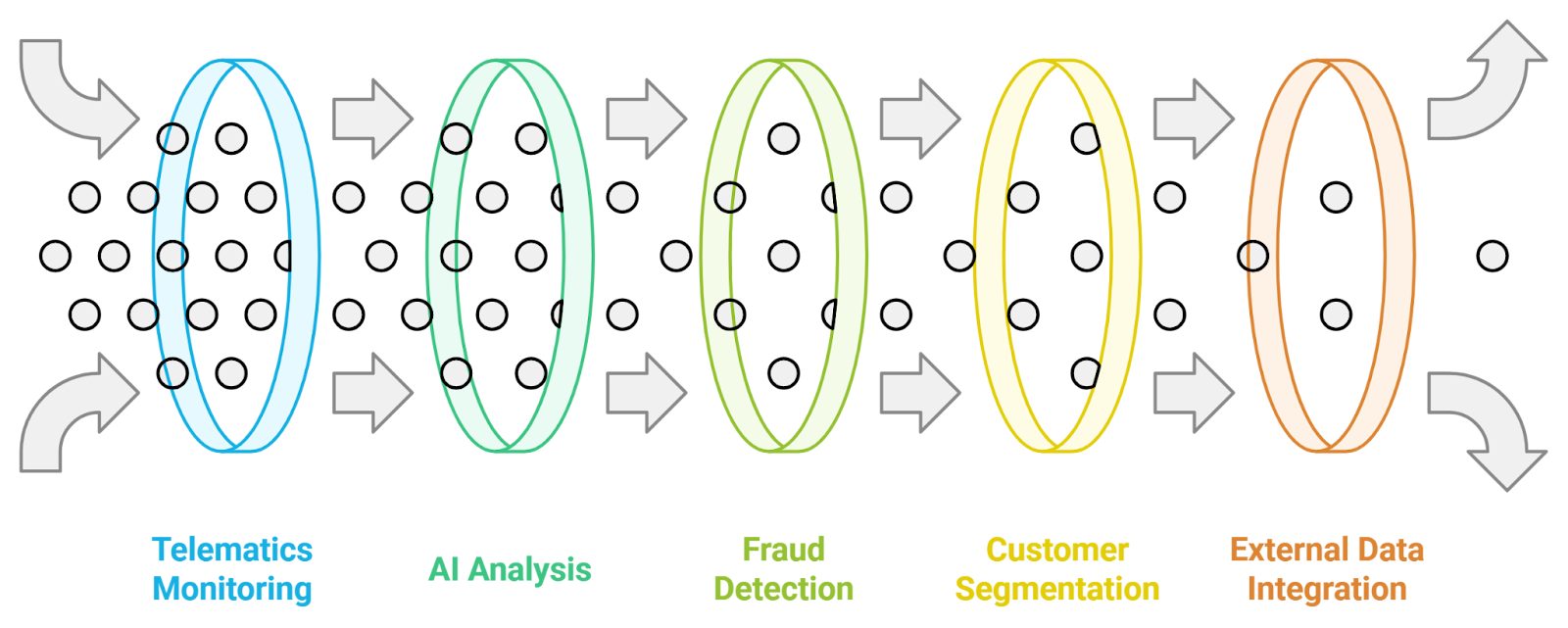
‍
The auto insurance industry has made significant strides in risk assessment, utilizing technology and data analytics to enhance underwriting processes and improve pricing accuracy.
- Telematics Technology: Insurers are increasingly using telematics devices to monitor driving behavior. This data helps assess risk based on factors like speed, braking patterns, and time of day, allowing for more personalized premiums. Rapid Innovation can develop advanced telematics solutions that provide real-time data analysis, enhancing risk assessment accuracy.
- AI and Machine Learning: Artificial intelligence is being employed to analyze vast amounts of data, identifying patterns and trends that can predict future claims. This leads to more accurate risk assessments and pricing models. Our AI solutions can help insurers leverage machine learning to refine their underwriting processes, resulting in improved profitability.
- Enhanced Fraud Detection: Advanced algorithms are being used to detect fraudulent claims more effectively. By analyzing claim patterns and comparing them to historical data, insurers can identify suspicious activities early. Rapid Innovation's AI-driven fraud detection systems can significantly reduce losses due to fraud, enhancing overall financial performance.
- Improved Customer Segmentation: Insurers are refining their customer segmentation strategies, allowing for more targeted marketing and tailored coverage options. This helps in offering competitive rates to low-risk drivers while adequately pricing higher-risk individuals. Our data analytics capabilities can assist insurers in developing more effective segmentation strategies, leading to better customer acquisition and retention.
- Integration of External Data Sources: Insurers are incorporating external data sources, such as credit scores and public records, into their risk assessment models. This holistic approach provides a more comprehensive view of an applicant's risk profile. Rapid Innovation can facilitate the integration of diverse data sources, enhancing the accuracy of risk assessments and improving underwriting outcomes.
2.5. Commercial Insurance and Risk Evaluation
Commercial insurance is designed to protect businesses from various risks, including property damage, liability, and employee-related risks. Risk evaluation is a critical component of underwriting in commercial insurance, as it helps insurers determine the likelihood of a claim and set appropriate premiums.
- Risk Assessment: Insurers evaluate the potential risks associated with a business by analyzing various factors, including industry type, location, and operational practices. Tools such as risk matrices and loss history analysis are commonly used to quantify risks.
- Data Utilization: Insurers leverage big data analytics to gather insights from multiple sources, including financial records, market trends, and historical claims data. Advanced analytics can identify patterns and predict future risks, allowing for more accurate pricing. At Rapid Innovation, we utilize AI-driven analytics to enhance data utilization, enabling our clients to make informed decisions that lead to greater ROI. For more information on how artificial intelligence is reshaping price optimization.
- Regulatory Compliance: Insurers must adhere to regulations that govern commercial insurance risk evaluation processes, ensuring transparency and fairness in premium calculations. Compliance with standards helps maintain trust and credibility in the insurance market. Our consulting services can guide clients through the complexities of regulatory compliance, ensuring they meet industry standards while optimizing their operations.
- Technology Integration: The use of artificial intelligence (AI) and machine learning enhances commercial insurance risk evaluation by automating data analysis and improving accuracy. Predictive modeling techniques can forecast potential losses, enabling insurers to adjust their coverage options accordingly. Rapid Innovation specializes in integrating AI solutions that streamline risk evaluation processes, ultimately leading to improved efficiency and reduced costs for our clients.
2.6. Travel Insurance and Dynamic Pricing Models
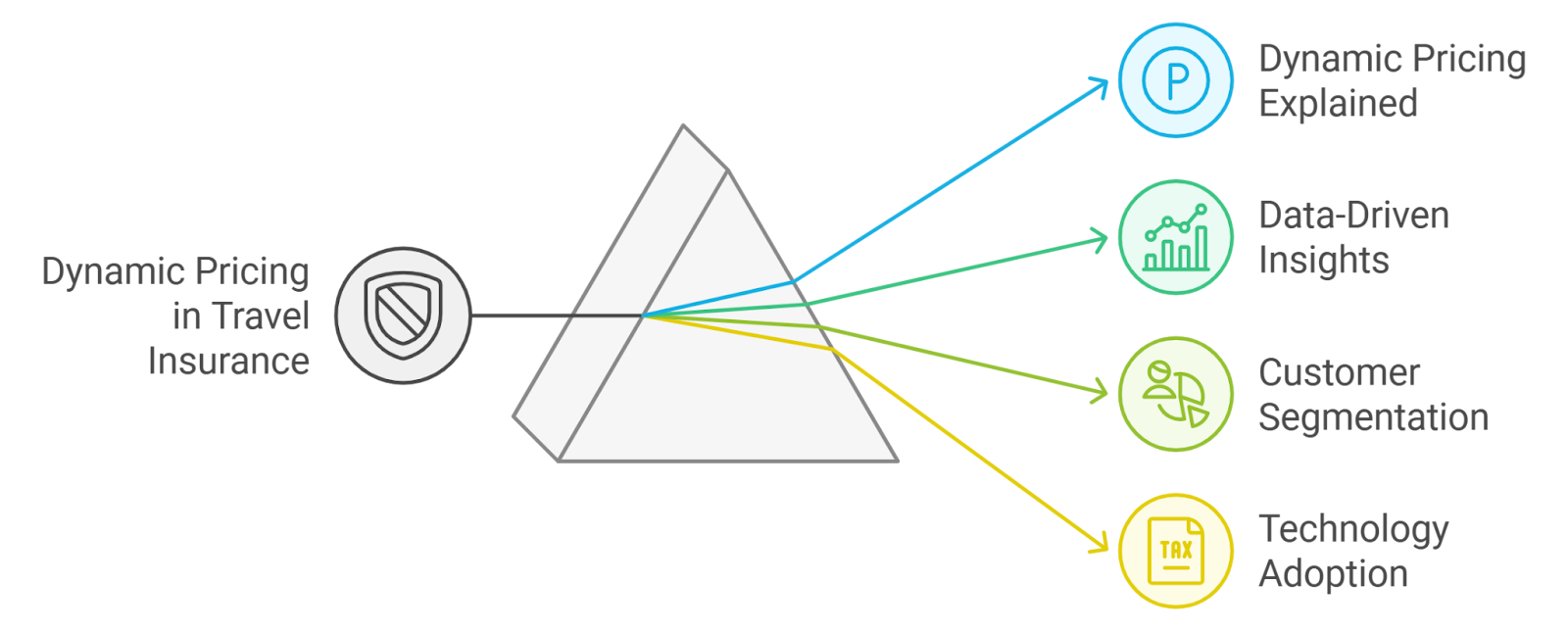
‍
Travel insurance provides coverage for unexpected events that may occur during travel, such as trip cancellations, medical emergencies, and lost luggage. Dynamic pricing models are increasingly being used in the travel insurance sector to optimize pricing strategies based on real-time data.
- Dynamic Pricing Explained: Dynamic pricing involves adjusting insurance premiums based on various factors, including demand, customer behavior, and market conditions. This model allows insurers to offer competitive rates while maximizing profitability.
- Data-Driven Insights: Insurers analyze data from travel booking platforms, social media, and customer feedback to gauge demand and adjust prices accordingly. Real-time data helps insurers respond quickly to changes in travel trends and customer preferences. At Rapid Innovation, we empower clients with AI tools that provide actionable insights, enabling them to adapt their pricing strategies effectively.
- Customer Segmentation: Dynamic pricing models enable insurers to segment customers based on risk profiles, travel habits, and purchasing behavior. Tailored pricing strategies can attract different customer segments, enhancing market reach. Our expertise in machine learning allows us to develop sophisticated segmentation models that help clients target their offerings more precisely.
- Technology Adoption: Advanced algorithms and machine learning techniques are employed to predict customer behavior and optimize pricing. Insurers can implement automated pricing adjustments, ensuring that premiums reflect current market conditions. Rapid Innovation's solutions facilitate the adoption of these technologies, driving efficiency and profitability for our clients.
3. Core Technologies Driving AI in Underwriting
Artificial intelligence (AI) is revolutionizing the underwriting process in the insurance industry by enhancing efficiency, accuracy, and decision-making. Several core technologies are driving this transformation.
- Machine Learning: Machine learning algorithms analyze vast amounts of data to identify patterns and trends that inform underwriting decisions. These algorithms can continuously learn from new data, improving their predictive capabilities over time. Rapid Innovation leverages machine learning to enhance underwriting processes, resulting in better risk assessment and increased ROI for our clients.
- Natural Language Processing (NLP): NLP enables computers to understand and interpret human language, allowing insurers to analyze unstructured data from documents, emails, and customer interactions. This technology helps streamline the underwriting process by extracting relevant information quickly. Our NLP solutions can significantly reduce processing times, enhancing overall operational efficiency.
- Predictive Analytics: Predictive analytics uses historical data to forecast future outcomes, helping underwriters assess risk more accurately. Insurers can leverage predictive models to identify high-risk applicants and adjust their underwriting criteria accordingly. Rapid Innovation's predictive analytics capabilities empower clients to make data-driven decisions that enhance profitability.
- Robotic Process Automation (RPA): RPA automates repetitive tasks in the underwriting process, such as data entry and document verification. This technology reduces manual errors and speeds up the overall underwriting workflow. Our RPA solutions help clients streamline their operations, allowing them to focus on strategic initiatives.
- Blockchain: Blockchain technology enhances data security and transparency in underwriting by providing a decentralized ledger for storing and sharing information. This can improve trust between insurers and clients, as well as streamline the claims process. Rapid Innovation's blockchain expertise enables clients to implement secure and efficient systems that foster trust and transparency.
- Cloud Computing: Cloud-based solutions facilitate the storage and processing of large datasets, enabling insurers to access real-time information for underwriting decisions. This technology supports collaboration among teams and enhances scalability for insurance companies. Our cloud solutions ensure that clients can scale their operations seamlessly while maintaining access to critical data.
Refer to the image for a visual representation of the concepts discussed in 2.5 Commercial Insurance and Risk Evaluation:

3.1. Machine Learning for Risk Assessment
Machine learning (ML) has become a pivotal tool in risk assessment across various industries, particularly in finance and insurance. By leveraging algorithms and statistical models, organizations can analyze vast amounts of data to identify potential risks and make informed decisions. Rapid Innovation specializes in implementing ML solutions that enhance risk management strategies for our clients, including machine learning risk assessment and credit risk analysis using machine learning.
- Enhanced accuracy: ML algorithms can process large datasets more efficiently than traditional methods, leading to more accurate risk predictions. This precision translates into better decision-making and reduced financial exposure for businesses, particularly in credit risk assessment machine learning.
- Real-time analysis: Machine learning enables continuous monitoring of risk factors, allowing organizations to respond quickly to emerging threats. Rapid Innovation's solutions ensure that clients can stay ahead of potential risks in a dynamic market environment, particularly through risk assessment using machine learning.
- Pattern recognition: ML can identify complex patterns in data that may not be apparent to human analysts, improving risk identification. Our expertise in ML allows us to develop tailored models that uncover hidden insights specific to our clients' needs, enhancing risk analysis machine learning.
- Automation: Automating risk assessment processes reduces human error and increases efficiency, allowing teams to focus on strategic decision-making. Rapid Innovation helps organizations streamline their operations, leading to significant cost savings in risk analysis using machine learning.
- Customization: ML models can be tailored to specific industries or organizations, providing more relevant insights. We work closely with clients to develop bespoke solutions that align with their unique business objectives, including machine learning for risk analysis and Natural Language Processing (NLP).
3.2. Predictive Analytics and Data Modeling
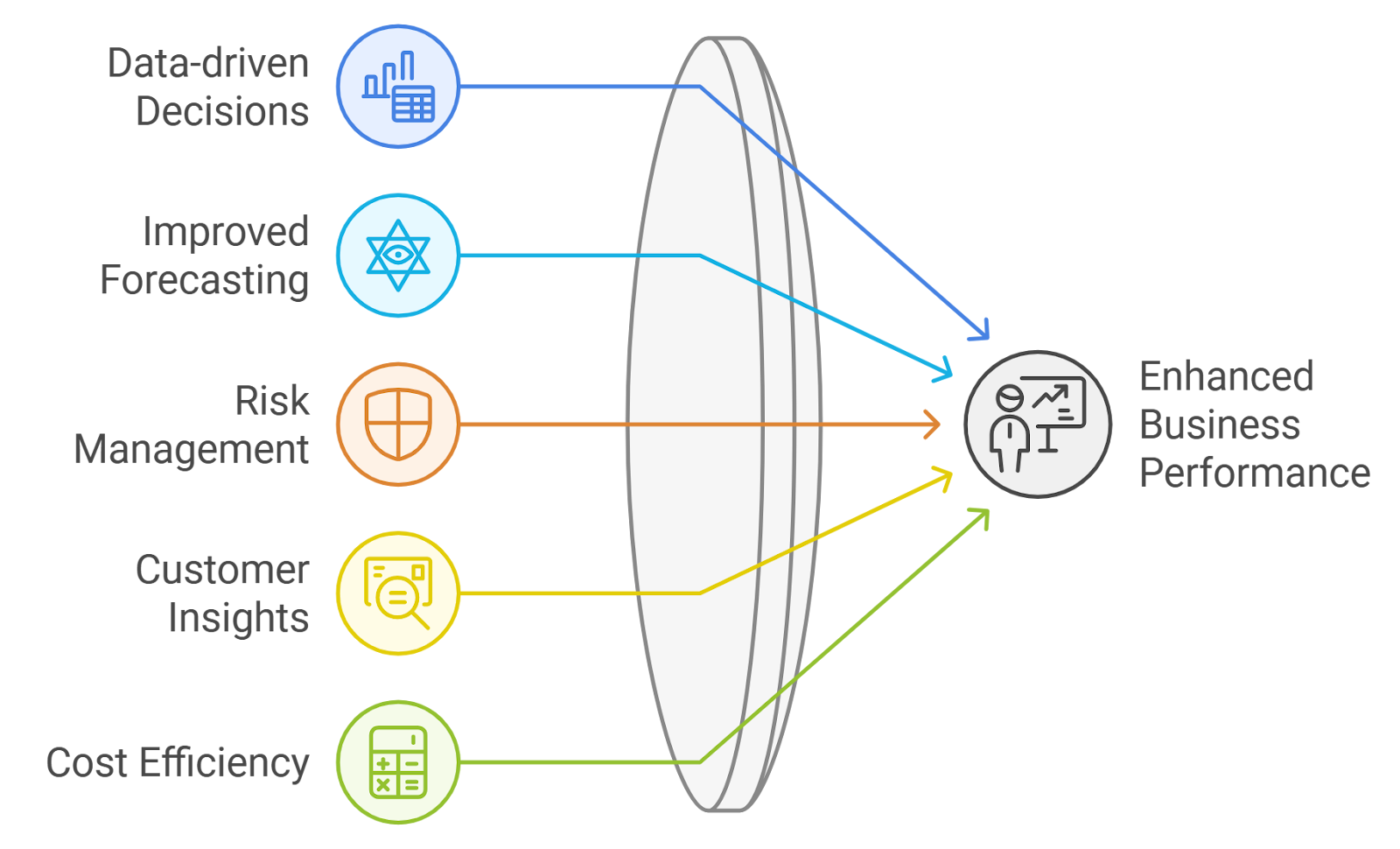
‍
Predictive analytics involves using historical data to forecast future outcomes, making it a valuable tool for decision-making in various sectors. Data modeling is a crucial component of predictive analytics, as it helps in structuring and analyzing data to derive insights. Rapid Innovation empowers organizations to harness the power of predictive analytics for enhanced business performance.
- Data-driven decisions: Predictive analytics allows organizations to make informed decisions based on data rather than intuition. Our solutions enable clients to leverage data effectively, leading to improved outcomes.
- Improved forecasting: By analyzing historical trends, organizations can predict future events, such as customer behavior or market changes. Rapid Innovation's predictive models help clients anticipate shifts in their industries, allowing for proactive strategies.
- Risk management: Predictive models can identify potential risks and opportunities, enabling proactive measures to mitigate losses. We assist clients in developing robust risk management frameworks that enhance resilience.
- Customer insights: Businesses can better understand customer preferences and behaviors, leading to improved marketing strategies and product offerings. Our analytics solutions provide actionable insights that drive customer engagement and loyalty.
- Cost efficiency: By anticipating future trends, organizations can allocate resources more effectively, reducing waste and increasing profitability. Rapid Innovation's expertise in data modeling ensures that clients achieve optimal resource utilization.
3.3. Natural Language Processing (NLP) in Underwriting
Natural Language Processing (NLP) is a branch of artificial intelligence that focuses on the interaction between computers and human language. In underwriting, NLP can streamline processes and enhance decision-making. Rapid Innovation leverages NLP technologies to transform underwriting practices for our clients.
- Automated document analysis: NLP can quickly analyze large volumes of text, such as insurance applications and claims, identifying relevant information and reducing processing time. Our solutions help clients expedite their underwriting processes, improving operational efficiency.
- Sentiment analysis: By assessing the tone and sentiment of written communication, NLP can help underwriters gauge customer satisfaction and risk levels. Rapid Innovation's NLP tools provide valuable insights that enhance client relationships.
- Enhanced communication: NLP tools can facilitate better communication between underwriters and clients, ensuring that all parties understand the terms and conditions clearly. We focus on improving transparency and clarity in the underwriting process.
- Fraud detection: NLP can identify inconsistencies or unusual patterns in text data, aiding in the detection of fraudulent claims. Our advanced NLP solutions bolster clients' fraud prevention efforts, safeguarding their interests.
- Improved compliance: NLP can assist in ensuring that underwriting processes adhere to regulatory requirements by analyzing documentation for compliance-related language. Rapid Innovation helps clients navigate complex regulatory landscapes with confidence.
4. Data Sources and Management for AI Underwriting
In the realm of AI underwriting, effective data sources and management are crucial for enhancing decision-making processes. The integration of diverse data sources and the utilization of real-time data can significantly improve the accuracy and efficiency of underwriting practices, particularly through AI underwriting data integration.
4.1. Integrating Diverse Data Sources
Integrating various data sources is essential for creating a comprehensive view of applicants. This integration allows underwriters to assess risk more accurately and make informed decisions.
- Types of Data Sources:
- Traditional data: Includes credit scores, financial statements, and historical claims data.
- Alternative data: Encompasses social media activity, online behavior, and payment histories.
- Public records: Involves property records, court records, and business registrations.
- Benefits of Integration:
- Enhanced risk assessment: A broader data pool leads to more accurate risk evaluations.
- Improved customer insights: Understanding customer behavior and preferences can tailor offerings.
- Streamlined processes: Automated data integration reduces manual entry and errors.
- Challenges:
- Data quality: Ensuring the accuracy and reliability of diverse data sources can be difficult. Learn more about the critical role of data quality in AI implementations.
- Compliance: Adhering to regulations regarding data usage and privacy is essential.
- Technical integration: Merging different data systems requires robust technology solutions.
4.2. The Importance of Real-Time Data in Underwriting
Real-time data plays a pivotal role in modern underwriting processes, allowing for timely and informed decision-making.
- Advantages of Real-Time Data:
- Immediate insights: Access to up-to-date information enables underwriters to respond quickly to changes in an applicant's situation.
- Dynamic risk assessment: Continuous monitoring of data allows for adjustments in risk profiles as new information becomes available.
- Enhanced customer experience: Faster underwriting processes lead to improved customer satisfaction and retention.
- Applications of Real-Time Data:
- Fraud detection: Real-time analytics can identify suspicious activities or inconsistencies in applications.
- Market trends: Monitoring industry trends and economic indicators helps underwriters adjust their strategies accordingly.
- Behavioral analytics: Understanding customer behavior in real-time can inform personalized underwriting decisions.
- Challenges:
- Data overload: Managing and analyzing vast amounts of real-time data can be overwhelming.
- Integration with legacy systems: Ensuring that real-time data can be effectively utilized alongside existing systems is crucial.
- Security concerns: Protecting sensitive real-time data from breaches is a top priority.
At Rapid Innovation, we leverage our expertise in AI underwriting data integration and blockchain technology to help clients navigate these challenges effectively. By implementing advanced data integration solutions and real-time analytics, we empower underwriters to make more informed decisions, ultimately leading to greater ROI and enhanced operational efficiency. Our tailored consulting services ensure that your organization can harness the full potential of diverse data sources while maintaining compliance and security.
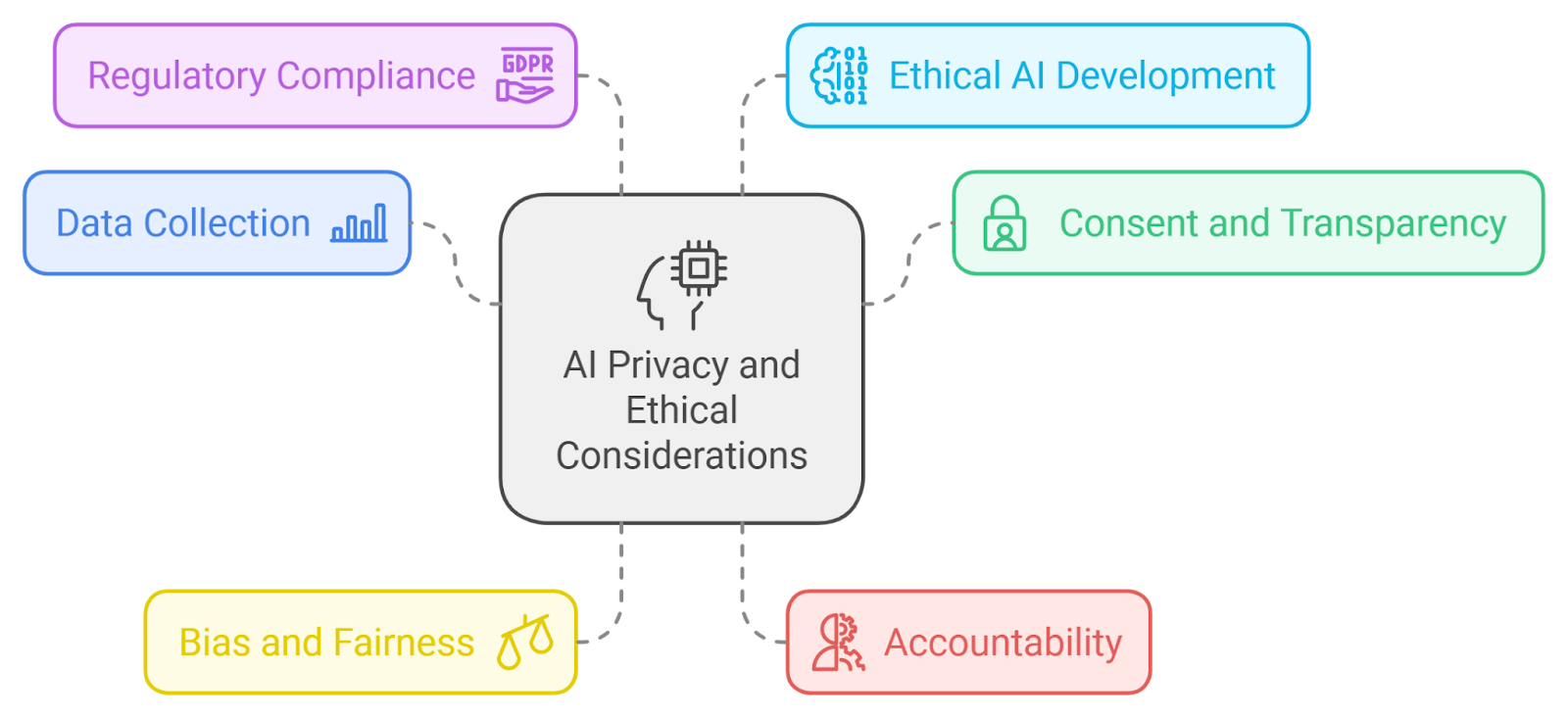
‍
The rise of AI in various sectors, including finance and healthcare, has raised significant privacy and ethical concerns, particularly in the context of AI privacy ethics.
- Data Collection: Organizations often collect vast amounts of personal data to train AI models. This data can include sensitive information, leading to potential misuse or breaches.
- Consent and Transparency: Users should be informed about what data is collected and how it will be used. Clear consent mechanisms are essential to ensure users are aware of their rights.
- Bias and Fairness: AI systems can inadvertently perpetuate biases present in training data. It is crucial to regularly audit AI models to identify and mitigate bias, ensuring that the solutions provided by Rapid Innovation are fair and equitable.
- Accountability: Organizations must establish clear accountability for AI decisions. This includes understanding who is responsible for errors or negative outcomes resulting from AI use, a principle that Rapid Innovation emphasizes in its development processes.
- Regulatory Compliance: Adhering to regulations such as GDPR or CCPA is vital for protecting user privacy. Organizations should implement robust data governance frameworks to ensure compliance, a service that Rapid Innovation can assist with through its consulting solutions.
- Ethical AI Development: Developers should prioritize ethical considerations in AI design and deployment, including AI privacy and ethics. Engaging diverse stakeholders can help identify potential ethical issues early in the process, aligning with Rapid Innovation's commitment to responsible AI practices. For more information on how to implement ethical AI solutions, consider exploring our ethical AI development guide and our adaptive AI development services.
5. Advanced AI Techniques in Underwriting
The underwriting process has evolved significantly with the integration of advanced AI techniques.
- Enhanced Risk Assessment: AI can analyze vast datasets to identify risk factors more accurately, leading to more precise underwriting decisions and improved risk management.
- Predictive Analytics: Machine learning algorithms can predict future claims based on historical data, allowing underwriters to make informed decisions and adjust policies accordingly.
- Automation of Routine Tasks: AI can automate repetitive tasks, freeing up underwriters to focus on complex cases. This increases efficiency and reduces the time taken to process applications.
- Improved Customer Insights: AI tools can analyze customer behavior and preferences, helping in tailoring products and services to meet specific customer needs.
- Fraud Detection: Advanced algorithms can identify patterns indicative of fraudulent activity. Early detection of fraud can save organizations significant costs.
- Continuous Learning: AI systems can continuously learn from new data, improving their accuracy over time. This adaptability is crucial in a rapidly changing market environment.
5.1. Machine Learning Models for Enhanced Decision Making
Machine learning (ML) models play a pivotal role in enhancing decision-making processes in underwriting.
- Types of Models:
- Supervised Learning: Utilizes labeled data to train models for predicting outcomes.
- Unsupervised Learning: Identifies patterns in data without predefined labels, useful for clustering similar risks.
- Feature Engineering: Selecting relevant features is critical for model performance. Effective feature engineering can significantly improve the accuracy of predictions.
- Model Evaluation: Regular evaluation of ML models is necessary to ensure they remain effective. Metrics such as accuracy, precision, and recall help assess model performance.
- Integration with Existing Systems: ML models should be integrated seamlessly with existing underwriting systems, ensuring that underwriters can leverage AI insights without disrupting workflows.
- Real-time Decision Making: ML models can process data in real-time, enabling quicker decision-making. This is particularly beneficial in fast-paced environments where timely responses are crucial.
- Ethical Considerations: It is essential to ensure that ML models are developed and used ethically. Regular audits can help identify and mitigate any biases in the decision-making process, reinforcing Rapid Innovation's dedication to ethical AI practices.
5.2. Predictive Analytics for Proactive Risk Management
Predictive analytics involves using historical data, statistical algorithms, and machine learning techniques to identify the likelihood of future outcomes. In the context of risk management, it plays a crucial role in helping organizations anticipate potential risks before they materialize.
- Enhances decision-making:
- Provides insights into risk patterns and trends.
- Supports data-driven decisions rather than relying solely on intuition.
- Identifies high-risk areas:
- Analyzes customer behavior and transaction history.
- Flags anomalies that may indicate fraudulent activities.
- Improves resource allocation:
- Helps prioritize risk management efforts based on predicted impact.
- Allocates resources more efficiently to mitigate identified risks.
- Facilitates real-time monitoring:
- Enables continuous assessment of risk factors.
- Allows organizations to respond swiftly to emerging threats.
- Supports regulatory compliance:
- Assists in identifying compliance risks before they escalate.
- Helps maintain adherence to industry regulations and standards.
- Case studies and applications:
- Many industries, including finance and insurance, utilize predictive analytics for risk assessment.
- Companies like Progressive Insurance use predictive models to assess risk and set premiums accordingly, showcasing the effectiveness of predictive risk management.
5.3. Future of Natural Language Processing in Underwriting
Natural Language Processing (NLP) is a branch of artificial intelligence that focuses on the interaction between computers and human language. In underwriting, NLP has the potential to revolutionize how data is processed and analyzed.
- Streamlines data processing:
- Automates the extraction of relevant information from unstructured data sources.
- Reduces the time spent on manual data entry and analysis.
- Enhances risk assessment:
- Analyzes customer communications, such as emails and chat transcripts, for insights.
- Identifies potential risks based on language patterns and sentiment analysis.
- Improves customer experience:
- Enables personalized communication through chatbots and virtual assistants.
- Provides quick responses to customer inquiries, enhancing satisfaction.
- Facilitates regulatory compliance:
- Monitors communications for compliance with industry regulations.
- Flags potential issues in real-time, reducing the risk of non-compliance.
- Future trends:
- Increased integration of NLP with machine learning for more accurate predictions.
- Development of more sophisticated algorithms to understand context and nuances in language.
- Industry examples:
- Companies like Lemonade are leveraging NLP to streamline their underwriting processes.
- Traditional insurers are beginning to adopt NLP tools to enhance efficiency and accuracy.
6. Implementation Strategies for AI Underwriting
Implementing AI in underwriting requires a strategic approach to ensure successful integration and maximize benefits.
- Assess organizational readiness:
- Evaluate existing technology infrastructure and data quality.
- Identify gaps in skills and knowledge among staff.
- Define clear objectives:
- Establish specific goals for AI implementation, such as reducing processing time or improving accuracy.
- Align AI initiatives with overall business strategy.
- Invest in technology:
- Choose the right AI tools and platforms that fit organizational needs.
- Ensure scalability to accommodate future growth and changes.
- Foster a data-driven culture:
- Encourage data sharing and collaboration across departments.
- Provide training to staff on data analytics and AI technologies.
- Pilot projects:
- Start with small-scale pilot projects to test AI applications in underwriting.
- Gather feedback and make necessary adjustments before full-scale implementation.
- Monitor and evaluate performance:
- Establish key performance indicators (KPIs) to measure the success of AI initiatives.
- Continuously assess the impact of AI on underwriting processes and outcomes.
- Address ethical considerations:
- Ensure transparency in AI decision-making processes.
- Mitigate biases in algorithms to promote fairness and equity in underwriting.
- Collaborate with stakeholders:
- Engage with technology partners, regulators, and industry experts.
- Share insights and best practices to enhance AI implementation efforts.
At Rapid Innovation, we leverage our expertise in AI and blockchain to help organizations implement these strategies effectively, ensuring that they achieve their business goals while maximizing ROI. By integrating predictive analytics in financial risk management and NLP into your risk management and underwriting processes, we can help you stay ahead of potential risks and enhance operational efficiency.
6.1. Building an AI-Ready Underwriting Infrastructure
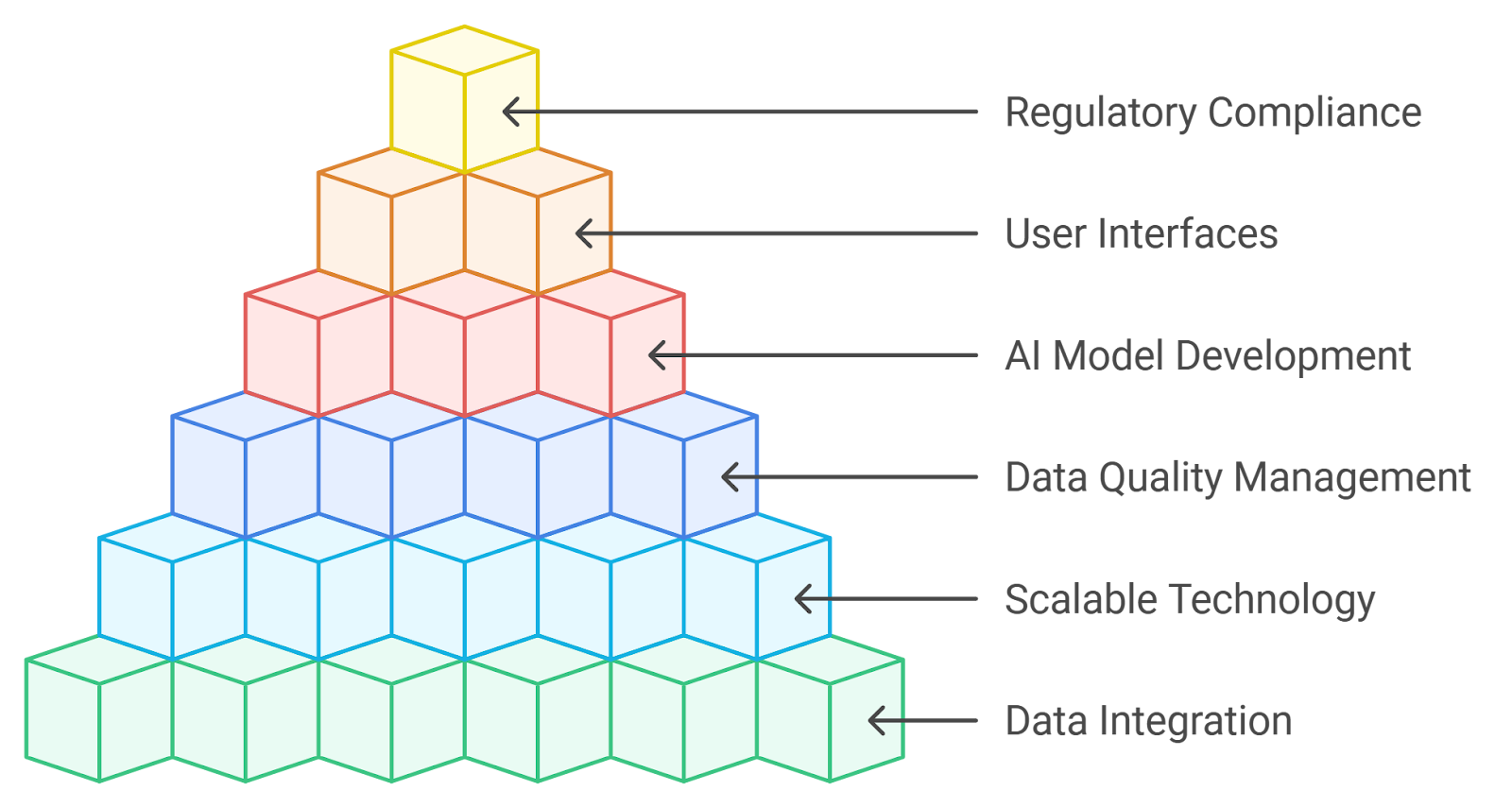
‍
- Data Integration: Centralize data from various sources, including customer information, historical claims, and market trends. Rapid Innovation utilizes APIs to connect disparate systems for seamless data flow, ensuring that all relevant data is accessible for informed decision-making, particularly in ai underwriting solutions.
- Scalable Technology: Invest in cloud-based solutions to handle large volumes of data and support AI algorithms. Rapid Innovation ensures that the infrastructure can scale as the volume of data and complexity of models increase, allowing clients to adapt to changing market demands without disruption.
- Data Quality Management: Implement processes for data cleansing and validation to ensure accuracy and reliability. Rapid Innovation regularly audits data sources to maintain high-quality datasets, which is crucial for effective AI model performance.
- AI Model Development: Collaborate with data scientists to develop machine learning models tailored to underwriting needs. Rapid Innovation employs iterative testing and validation to refine models before full deployment, ensuring that clients benefit from robust and reliable ai underwriting solutions.
- User-Friendly Interfaces: Design intuitive dashboards for underwriters to interact with AI insights easily. Rapid Innovation provides training for staff to effectively use AI tools in their decision-making processes, enhancing user adoption and maximizing the value of AI investments.
- Regulatory Compliance: Stay updated on regulations affecting data usage and AI in underwriting. Rapid Innovation implements compliance checks within the infrastructure to avoid legal issues, ensuring that clients operate within the bounds of industry standards. For more insights on integrating ethical practices in AI.
6.2. Ensuring Ethical AI Practices in Underwriting
- Bias Mitigation: Regularly assess AI models for biases that may affect underwriting decisions. Rapid Innovation emphasizes the use of diverse datasets to train models, ensuring fair treatment of all applicants and promoting ethical practices.
- Transparency: Maintain clear documentation of AI algorithms and decision-making processes. Rapid Innovation provides explanations for automated decisions to stakeholders and customers, fostering trust and accountability in AI-driven underwriting.
- Accountability: Establish a governance framework to oversee AI practices in underwriting. Rapid Innovation designates responsible individuals or teams to monitor AI outcomes and address ethical concerns, ensuring that clients uphold high ethical standards.
- Stakeholder Engagement: Involve diverse stakeholders in discussions about AI ethics, including customers, regulators, and advocacy groups. Rapid Innovation gathers feedback to improve AI practices and address community concerns, promoting a collaborative approach to ethical AI.
- Continuous Monitoring: Implement systems for ongoing evaluation of AI performance and ethical implications. Rapid Innovation adjusts models and practices based on findings to ensure alignment with ethical standards, helping clients maintain responsible AI usage.
6.3. Measuring Success: Key Performance Indicators (KPIs)
- Accuracy of Predictions: Track the accuracy of AI-driven underwriting decisions compared to traditional methods. Rapid Innovation measures the percentage of correct risk assessments and claims predictions, providing clients with insights into the effectiveness of their ai underwriting solutions.
- Operational Efficiency: Monitor the time taken to process applications before and after AI implementation. Rapid Innovation evaluates reductions in manual workload for underwriters due to AI assistance, demonstrating the operational benefits of AI integration.
- Customer Satisfaction: Conduct surveys to gauge customer satisfaction with the underwriting process. Rapid Innovation analyzes feedback on the speed and fairness of decisions made using AI, helping clients enhance their customer experience.
- Loss Ratios: Measure the ratio of claims paid to premiums earned to assess the effectiveness of underwriting decisions. Rapid Innovation compares loss ratios before and after AI integration to determine impact, providing clients with a clear view of their financial performance.
- Regulatory Compliance Rates: Track compliance with industry regulations and standards in AI practices. Rapid Innovation monitors the number of compliance issues or audits related to ai underwriting solutions, ensuring that clients remain compliant and mitigate risks.
- Return on Investment (ROI): Calculate the financial benefits gained from AI implementation against the costs incurred. Rapid Innovation assesses improvements in profitability and market share attributed to enhanced underwriting processes, helping clients understand the value of their ai underwriting solutions.
7. The Future of AI in Underwriting
The underwriting process is undergoing a significant transformation due to advancements in artificial intelligence (AI). As the industry adapts to these changes, understanding the future of AI in underwriting becomes crucial for organizations aiming to stay competitive.
7.1. Emerging Trends Shaping AI-Driven Underwriting

‍
AI is reshaping underwriting through various emerging trends that enhance efficiency, accuracy, and decision-making.
- Data Utilization: AI systems can analyze vast amounts of data from diverse sources, including social media, IoT devices, and public records. This data-driven approach allows for more accurate risk assessments and personalized insurance products, ultimately leading to greater ROI for organizations. The integration of AI in insurance underwriting is pivotal in this data utilization.
- Machine Learning Algorithms: Machine learning models continuously improve by learning from historical data and outcomes. These algorithms can identify patterns and predict risks more effectively than traditional methods, enabling underwriters to make informed decisions that enhance profitability. The use of machine learning in insurance underwriting is becoming increasingly prevalent.
- Automation of Processes: Routine tasks such as data entry and document verification are increasingly automated. This automation reduces human error and speeds up the underwriting process, allowing underwriters to focus on more complex cases, thereby increasing operational efficiency. AI for underwriting is a key driver of this automation.
- Enhanced Customer Experience: AI-driven underwriting can lead to faster policy approvals and more tailored insurance solutions. Improved customer interactions through chatbots and virtual assistants enhance service delivery, fostering customer loyalty and retention. The role of AI in insurance underwriting is crucial for enhancing customer experience.
- Predictive Analytics: Predictive analytics tools help underwriters forecast future risks based on historical data. This capability enables proactive risk management and better pricing strategies, ultimately driving higher returns on investment. AI credit underwriting is an example of how predictive analytics can be applied.
- Regulatory Compliance: AI can assist in ensuring compliance with ever-evolving regulations by monitoring changes and automating reporting processes. This reduces the risk of non-compliance penalties and enhances operational efficiency, safeguarding the organization's financial health. AI in underwriting can streamline compliance efforts.
- Integration with Other Technologies: AI is increasingly integrated with blockchain, big data, and cloud computing. This integration enhances data security, transparency, and accessibility, further improving underwriting processes and building trust with stakeholders. The collaboration of AI and underwriting with other technologies is essential for future advancements.
7.2. Preparing Organizations for the AI Revolution in Underwriting
To successfully navigate the AI revolution in underwriting, organizations must take proactive steps to prepare.
- Invest in Technology: Organizations should invest in AI technologies and tools that align with their underwriting processes, including adopting machine learning platforms and data analytics solutions. Rapid Innovation can assist in identifying and implementing the right technologies to maximize ROI.
- Upskill Workforce: Training programs should be implemented to equip underwriters with the necessary skills to work alongside AI tools. Emphasizing data literacy and analytical skills will be crucial for the workforce, ensuring they can leverage AI effectively. AI in life insurance underwriting is an area where upskilling can be particularly beneficial.
- Foster a Culture of Innovation: Encouraging a culture that embraces change and innovation will help organizations adapt to new technologies. Leadership should promote experimentation and support teams in exploring AI applications, driving continuous improvement. AI and underwriting should be seen as a partnership for innovation.
- Collaborate with Tech Partners: Partnering with technology firms specializing in AI can provide organizations with the expertise needed for successful implementation. Collaboration can also lead to the development of customized solutions tailored to specific underwriting needs, enhancing overall effectiveness. AI-based underwriting solutions can emerge from such partnerships.
- Focus on Data Governance: Establishing robust data governance frameworks is essential to ensure data quality and compliance. Organizations must prioritize data privacy and security to build trust with customers, which is vital for long-term success. The importance of data governance in AI underwriting cannot be overstated.
- Monitor Industry Trends: Staying informed about industry trends and advancements in AI will help organizations remain competitive. Regularly attending conferences, webinars, and workshops can provide valuable insights, enabling organizations to adapt swiftly. Keeping an eye on trends like machine learning in underwriting is essential.
- Pilot Programs: Implementing pilot programs allows organizations to test AI solutions on a smaller scale before full deployment. This approach helps identify potential challenges and refine strategies for broader implementation, minimizing risks. AI for underwriting can be effectively tested through pilot programs.
- Evaluate Performance Metrics: Organizations should establish clear performance metrics to assess the effectiveness of AI-driven underwriting. Regular evaluations will help in making data-driven decisions and optimizing processes, ultimately leading to improved ROI. Metrics for AI credit underwriting can provide valuable insights.
By embracing these emerging trends and preparing adequately, organizations can harness the full potential of AI in underwriting, leading to improved efficiency, better risk management, and enhanced customer satisfaction. Rapid Innovation is here to guide you through this AI insurance transformative journey, ensuring you achieve your business goals effectively and efficiently.
8. Challenges in AI Underwriting Implementation
The integration of artificial intelligence (AI) into underwriting processes presents numerous challenges that organizations must navigate. These challenges can be broadly categorized into technical and organizational issues.
8.1. Technical Challenges to Address
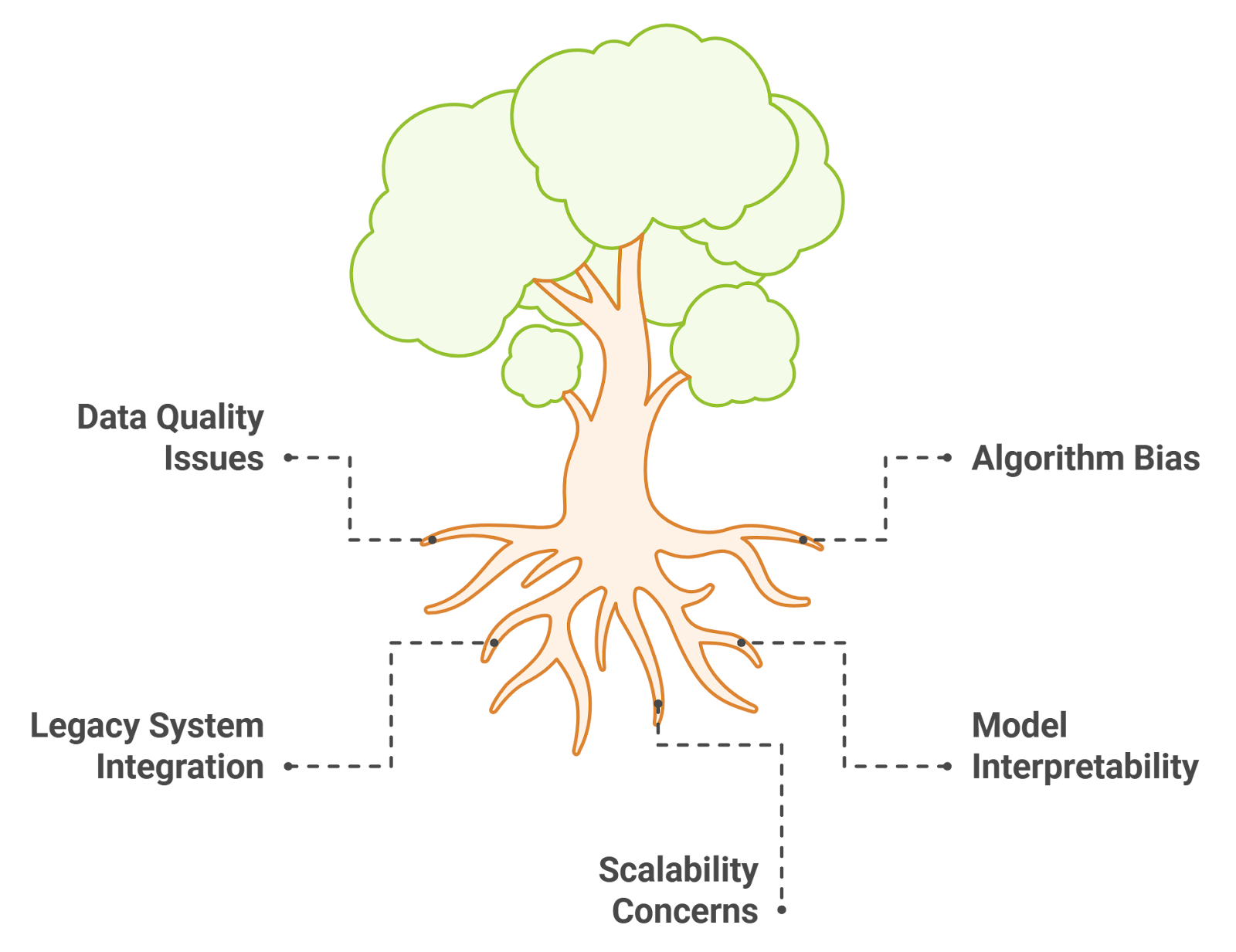
‍
AI underwriting relies on complex algorithms and vast amounts of data, which can lead to several technical hurdles:
- Data Quality and Availability: Inconsistent data formats can hinder effective analysis, while insufficient historical data may limit the model's predictive capabilities. Additionally, data privacy regulations can restrict access to necessary information.
- Algorithm Bias: AI models can inadvertently perpetuate existing biases present in training data. Biased algorithms can lead to unfair underwriting decisions, impacting customer trust. Continuous monitoring and adjustment of algorithms are necessary to mitigate bias.
- Integration with Legacy Systems: Many organizations still rely on outdated systems that may not support AI technologies. Integrating AI solutions with existing infrastructure can be complex and costly, and a phased approach to integration may be required to minimize disruption.
- Model Interpretability: AI models, particularly deep learning algorithms, can be difficult to interpret. The lack of transparency can lead to challenges in explaining decisions to stakeholders. Developing interpretable models is crucial for regulatory compliance and customer trust.
- Scalability: As organizations grow, their AI systems must be able to scale accordingly. Ensuring that AI solutions can handle increased data volumes and complexity is essential, and regular updates and maintenance are necessary to keep systems efficient.
8.2. Organizational Challenges and Solutions
Implementing AI in underwriting also involves various organizational challenges that require strategic solutions:
- Change Management: Resistance to change from employees can hinder AI adoption. Clear communication about the benefits of AI can help alleviate concerns, and training programs can equip staff with the necessary skills to work alongside AI.
- Skill Gaps: A shortage of skilled professionals in AI and data science can impede implementation. Organizations may need to invest in training or hire new talent to bridge these gaps. Collaborating with educational institutions can help develop a pipeline of skilled workers.
- Cultural Resistance: An organizational culture that is not open to innovation can stifle AI initiatives. Leadership must foster a culture that embraces technology and data-driven decision-making. Encouraging collaboration between departments can promote a more innovative environment.
- Regulatory Compliance: Navigating the regulatory landscape can be challenging for AI underwriting. Organizations must stay informed about relevant regulations and ensure compliance. Engaging legal and compliance teams early in the process can help mitigate risks.
- Resource Allocation: Implementing AI requires significant investment in technology and talent. Organizations must carefully allocate resources to ensure successful implementation. Developing a clear business case for AI can help secure necessary funding and support.
At Rapid Innovation, we understand these AI underwriting challenges and are equipped to assist organizations in overcoming them. Our expertise in AI and blockchain development allows us to provide tailored solutions that enhance underwriting processes, ensuring greater efficiency and improved ROI. By leveraging our advanced technologies, clients can navigate the complexities of AI implementation while maximizing their investment in innovation.
9. Conclusion: The Strategic Importance of AI in Underwriting
The integration of Artificial Intelligence (AI) in underwriting processes is transforming the insurance industry. As companies strive to enhance efficiency, accuracy, and customer satisfaction, AI emerges as a pivotal tool. The strategic importance of AI in underwriting cannot be overstated, as it not only streamlines operations but also provides a competitive edge in a rapidly evolving market.
9.1. Leveraging AI for Competitive Advantage in Insurance
AI offers numerous advantages that can significantly enhance an insurance company's competitive position.
- Improved Risk Assessment: AI algorithms analyze vast amounts of data to identify patterns and predict risks more accurately than traditional methods. This leads to better pricing strategies and reduced losses, particularly in areas like ai underwriting and underwriting ai.
- Enhanced Customer Experience: AI-driven chatbots and virtual assistants provide 24/7 support, answering queries and guiding customers through the underwriting process, which improves customer satisfaction and retention. This is especially relevant in ai in insurance underwriting.
- Faster Decision-Making: Automated underwriting processes powered by AI can drastically reduce the time taken to assess applications, allowing insurers to respond to customers more quickly and efficiently. This is a key benefit of artificial intelligence underwriting.
- Cost Reduction: By automating routine tasks, AI reduces operational costs, enabling insurers to allocate resources more effectively and invest in innovation. This is crucial for companies looking to implement ai for underwriting.
- Data-Driven Insights: AI tools can analyze customer data to uncover insights that inform product development and marketing strategies, helping insurers tailor their offerings to meet market demands. This is particularly important in the context of ai credit underwriting and ai in life insurance underwriting.
9.2. Actionable Steps to Achieve AI Excellence in Underwriting
To harness the full potential of AI in underwriting, insurance companies should consider the following actionable steps:
- Invest in Technology: Allocate budget for AI technologies and tools that can enhance underwriting processes. This includes machine learning platforms, data analytics software, and customer relationship management systems, which are essential for insurance underwriting ai.
- Build a Skilled Workforce: Train existing staff and hire new talent with expertise in AI and data science. A skilled workforce is essential for effectively implementing and managing AI solutions, particularly in ai for insurance underwriting.
- Collaborate with Tech Partners: Form partnerships with technology firms specializing in AI and data analytics. Collaborating with experts can accelerate the adoption of AI and provide access to cutting-edge solutions, including underwriting machine learning.
- Focus on Data Quality: Ensure that the data used for AI training is accurate, comprehensive, and up-to-date. High-quality data is crucial for the effectiveness of AI algorithms, especially in ai and insurance underwriting.
- Implement Pilot Programs: Start with pilot projects to test AI applications in underwriting. This allows companies to evaluate the effectiveness of AI solutions before full-scale implementation, particularly in ai underwriting insurance.
- Monitor and Evaluate Performance: Continuously assess the performance of AI systems and make adjustments as necessary. Regular evaluation helps in identifying areas for improvement and ensuring that AI initiatives align with business goals.
- Foster a Culture of Innovation: Encourage a culture that embraces technological advancements and innovation. This mindset will facilitate the adoption of AI and other emerging technologies in underwriting processes.
At Rapid Innovation, we specialize in guiding insurance companies through the integration of AI into their underwriting processes. Our expertise in AI development and consulting ensures that our clients can leverage these technologies effectively, ultimately achieving greater ROI and enhancing their competitive advantage in the market. For more insights on AI in underwriting, check out our post on AI in loan underwriting.