Table Of Contents
Category
Artificial Intelligence
AIML
1. Introduction: The AI Revolution in Healthcare
The integration of artificial intelligence (AI) in healthcare is transforming the industry, enhancing patient care, and streamlining operations. Solutions developed on AI are being utilized to analyze vast amounts of data, improve diagnostic accuracy, and personalize treatment plans. This revolution is not just a trend; it represents a fundamental shift in how healthcare is delivered and managed. AI is being used in various applications, including diagnostics, treatment recommendations, patient monitoring, and administrative tasks. The technology is helping healthcare professionals make more informed decisions, ultimately leading to better patient outcomes. Additionally, AI's ability to process and analyze data at unprecedented speeds is enabling healthcare providers to identify patterns and trends that were previously undetectable.
1.1. Current State of Healthcare AI and Its Impact
The current state of AI in healthcare is characterized by rapid advancements and increasing adoption across various sectors. Healthcare organizations are recognizing the potential of AI to enhance efficiency and improve patient care. AI is being implemented in areas such as radiology, pathology, and genomics, where it assists in interpreting medical images and analyzing genetic data. Virtual health assistants and chatbots are improving patient engagement and providing immediate responses to inquiries. Furthermore, predictive analytics powered by AI is helping in early disease detection and management, leading to proactive healthcare.
The impact of AI on healthcare is profound, with benefits including improved diagnostic accuracy and speed, enhanced patient engagement and satisfaction, and reduced operational costs alongside increased efficiency. At Rapid Innovation, we leverage our expertise in AI to help healthcare organizations implement tailored solutions that drive better patient outcomes and optimize operational workflows, ultimately leading to greater ROI.
1.1.1. Market Size and Growth Projections
The market for AI in healthcare is experiencing significant growth, driven by technological advancements and increasing demand for efficient healthcare solutions. According to a report by MarketsandMarkets, the global AI in healthcare market is projected to reach $45.2 billion by 2026, growing at a compound annual growth rate (CAGR) of 44.9% from 2021 to 2026. The increasing volume of healthcare data and the need for advanced analytics are key factors fueling this growth. Additionally, investments in AI startups and collaborations between tech companies and healthcare providers are contributing to market expansion.
Key growth drivers include:
- Rising demand for personalized medicine and treatment plans, including AI for health and artificial intelligence in health.
- The need for cost-effective healthcare solutions, such as medical coding AI and AI for medical.
- Advancements in machine learning and natural language processing technologies, including machine learning in healthcare and generative AI in healthcare.
As the healthcare industry continues to embrace AI, the potential for innovation and improvement in patient care is immense. Rapid Innovation is committed to guiding organizations through this transformative journey, ensuring they harness the full potential of AI to achieve their business goals efficiently and effectively. This includes exploring applications like AI medical diagnosis, artificial intelligence in medical imaging, and AI in the medical field.
1.1.2. Key Stakeholders and Decision Makers in AI Healthcare
- Healthcare Providers: Hospitals and clinics that implement AI healthcare stakeholders to improve patient care, along with physicians and nurses who utilize AI tools for diagnostics and treatment planning. Rapid Innovation collaborates with these stakeholders to integrate AI solutions that enhance patient outcomes and streamline operations.
- Technology Companies: Firms that develop AI algorithms and software tailored for healthcare applications, including startups focusing on innovative AI solutions for specific medical challenges. Rapid Innovation partners with these companies to leverage cutting-edge technologies, ensuring our clients benefit from the latest advancements in AI.
- Regulatory Bodies: Organizations like the FDA that oversee the approval and regulation of AI technologies in healthcare, as well as policymakers who create guidelines to ensure the safe and ethical use of AI in medical settings. Rapid Innovation stays abreast of regulatory changes, helping clients navigate compliance and implement AI solutions that meet industry standards.
- Patients: Individuals who benefit from AI-driven healthcare solutions, influencing demand and acceptance, along with patient advocacy groups that voice concerns and preferences regarding AI applications. By focusing on patient-centric solutions, Rapid Innovation ensures that our AI implementations align with patient needs and improve overall satisfaction.
- Researchers and Academics: Institutions conducting studies on the efficacy and safety of AI in healthcare, and experts who contribute to the development of best practices and ethical standards. Rapid Innovation engages with researchers to validate our AI solutions, ensuring they are grounded in scientific evidence and best practices.
- Insurance Companies: Payers that assess the cost-effectiveness of AI technologies and their impact on healthcare costs, as well as insurers that may influence the adoption of AI by determining coverage policies. Rapid Innovation assists clients in demonstrating the ROI of AI solutions to insurance companies, facilitating broader adoption and reimbursement.
1.1.3. Challenges and Opportunities in AI-Driven Healthcare
- Challenges: Â
- Data Privacy and Security: Concerns about patient data breaches and unauthorized access to sensitive information, along with compliance with regulations like HIPAA to protect patient confidentiality. Rapid Innovation prioritizes data security in our AI solutions, implementing robust measures to safeguard patient information.
- Integration with Existing Systems: Difficulty in incorporating AI solutions into legacy healthcare systems and the need for interoperability between different technologies and platforms. Rapid Innovation specializes in seamless integration, ensuring that our AI tools work harmoniously with existing systems to enhance functionality.
- Bias and Fairness: The risk of AI algorithms perpetuating existing biases in healthcare data, highlighting the importance of ensuring equitable access and treatment across diverse populations. Rapid Innovation is committed to developing fair and unbiased AI solutions, conducting thorough testing to mitigate these risks.
- Regulatory Hurdles: Complex approval processes for new AI technologies can delay implementation, necessitating clear guidelines on the use of AI in clinical settings. Rapid Innovation provides guidance on navigating regulatory landscapes, helping clients expedite the deployment of AI technologies.
- Opportunities: Â
- Improved Diagnostics: AI can enhance the accuracy of disease detection and diagnosis, with the potential to analyze vast amounts of data quickly, leading to earlier interventions. Rapid Innovation harnesses AI's capabilities to develop diagnostic tools that empower healthcare providers with actionable insights.
- Personalized Medicine: AI enables tailored treatment plans based on individual patient data and offers the opportunity to predict patient responses to specific therapies. Rapid Innovation works with clients to create personalized medicine solutions that optimize treatment efficacy and patient outcomes.
- Operational Efficiency: Automation of administrative tasks can reduce costs and improve workflow, while AI can assist in resource allocation and patient management. Rapid Innovation's AI solutions streamline operations, allowing healthcare providers to focus on delivering quality care.
- Enhanced Patient Engagement: AI-driven tools can facilitate better communication between patients and providers, creating opportunities for remote monitoring and telehealth services. Rapid Innovation develops patient engagement platforms that enhance interaction and improve health management.
1.2. The Benefits and Risks of Implementing AI in Healthcare
- Benefits: Â
- Increased Efficiency: AI can streamline processes, reducing the time healthcare professionals spend on routine tasks. Automation of data entry and analysis can lead to faster decision-making. Rapid Innovation's AI solutions are designed to enhance operational efficiency, resulting in significant time savings for healthcare teams.
- Enhanced Accuracy: AI algorithms can analyze medical images and data with high precision, improving diagnostic accuracy and reducing human error through data-driven insights. Rapid Innovation focuses on developing highly accurate AI models that support clinical decision-making.
- Cost Reduction: There is potential to lower healthcare costs by optimizing resource use and reducing unnecessary procedures. AI can help identify cost-effective treatment options. Rapid Innovation assists clients in realizing cost savings through our AI-driven solutions, ultimately improving their ROI.
- Better Patient Outcomes: AI can lead to improved treatment plans and better management of chronic diseases, with enhanced monitoring capabilities resulting in timely interventions. Rapid Innovation's commitment to patient outcomes drives our development of AI tools that support proactive healthcare management.
- Risks: Â
- Dependence on Technology: Over-reliance on AI may lead to diminished clinical skills among healthcare providers, along with the risk of technology failures impacting patient care. Rapid Innovation emphasizes the importance of maintaining a balance between technology and human expertise in our AI implementations.
- Ethical Concerns: Issues related to informed consent and the use of patient data for AI training arise, as well as the potential for AI to make decisions without human oversight, raising ethical dilemmas. Rapid Innovation is dedicated to ethical AI practices, ensuring transparency and accountability in our solutions.
- Job Displacement: Automation may lead to job losses in certain administrative roles, highlighting the need for workforce retraining to adapt to new technologies. Rapid Innovation advocates for workforce development, providing training and support to help staff transition to new roles in an AI-enhanced environment.
- Data Quality Issues: AI's effectiveness is contingent on the quality of data used for training, and poor data quality can lead to inaccurate predictions and outcomes. Rapid Innovation prioritizes data quality in our AI projects, implementing rigorous data management practices to ensure reliable results.
Refer to the image based on the Key Stakeholders and Decision Makers in AI Healthcare.

1.2.1. Potential Benefits to Patient Outcomes and Costs
- Improved Diagnosis Accuracy: AI algorithms can analyze medical data more efficiently than traditional methods, leading to more accurate diagnoses. For instance, AI in healthcare can identify patterns in imaging data that may be missed by human eyes, thereby enhancing the diagnostic capabilities of healthcare providers.
- Personalized Treatment Plans: AI can help tailor treatment plans to individual patients by analyzing their unique genetic makeup, lifestyle, and medical history. This personalized approach can enhance treatment effectiveness and reduce adverse effects, ultimately leading to better patient outcomes.
- Enhanced Predictive Analytics: AI can predict patient outcomes by analyzing historical data, allowing healthcare providers to intervene earlier in the treatment process. This proactive approach can lead to better management of chronic diseases and reduced hospital readmissions, contributing to overall cost savings.
- Cost Reduction: By streamlining administrative tasks and optimizing resource allocation, AI can significantly lower operational costs in healthcare settings. For example, AI can automate scheduling and billing processes, freeing up staff to focus on patient care and improving the overall efficiency of healthcare operations.
- Increased Efficiency: AI can assist in triaging patients, managing workflows, and reducing wait times. This efficiency can lead to improved patient satisfaction and better overall care, which is essential for maintaining a competitive edge in the healthcare market.
- Access to Care: AI technologies can extend healthcare access to underserved populations through telemedicine and remote monitoring, ensuring that more patients receive timely care. This can help bridge the gap in healthcare disparities and improve community health outcomes.
1.2.2. Risks, Ethical Concerns, and Regulatory Barriers
- Data Privacy and Security: The use of AI in healthcare often involves handling sensitive patient data. There is a risk of data breaches, which can compromise patient confidentiality and trust, necessitating robust security measures.
- Algorithmic Bias: AI systems can perpetuate existing biases in healthcare if they are trained on non-representative datasets. This can lead to disparities in care and outcomes for different demographic groups, highlighting the need for diverse data sources in AI training.
- Lack of Transparency: Many AI algorithms operate as "black boxes," making it difficult for healthcare providers to understand how decisions are made. This lack of transparency can hinder trust in AI-driven recommendations, emphasizing the importance of explainable AI.
- Ethical Dilemmas: The use of AI raises ethical questions about accountability in decision-making. If an AI system makes a mistake, it can be unclear who is responsible—the healthcare provider, the developer, or the institution, necessitating clear guidelines and accountability frameworks.
- Regulatory Challenges: The rapid development of AI technologies often outpaces existing regulations. There is a need for clear guidelines to ensure the safe and effective use of AI in healthcare, which can be a barrier to implementation.
- Integration with Existing Systems: Incorporating AI into current healthcare practices can be challenging due to compatibility issues with existing technologies and workflows. This can lead to resistance from healthcare professionals, making change management a critical aspect of successful AI implementation.
2. Core AI Technologies in Healthcare
- Machine Learning: This subset of AI involves algorithms that learn from data to make predictions or decisions. In healthcare, machine learning is used for tasks such as disease prediction, patient risk assessment, and treatment optimization, enabling healthcare providers to make data-driven decisions.
- Natural Language Processing (NLP): NLP enables machines to understand and interpret human language. In healthcare, NLP can be used to analyze clinical notes, extract relevant information from unstructured data, and improve patient-provider communication, enhancing the overall patient experience.
- Computer Vision: This technology allows computers to interpret and process visual information. In healthcare, computer vision is applied in radiology for image analysis, helping to detect abnormalities in X-rays, MRIs, and CT scans, thereby improving diagnostic accuracy.
- Robotics: AI-powered robots are increasingly used in surgical procedures, rehabilitation, and patient care. They can assist surgeons with precision tasks, provide companionship to patients, and help with mobility, enhancing the quality of care provided.
- Predictive Analytics: This technology uses historical data to forecast future events. In healthcare, predictive analytics can identify patients at risk for certain conditions, enabling proactive interventions that can lead to better health outcomes.
- Wearable Technology: Devices equipped with AI can monitor patients' vital signs and health metrics in real-time. This data can be used for early detection of health issues and to promote preventive care, ultimately contributing to improved patient health and reduced healthcare costs.
At Rapid Innovation, we leverage these core AI technologies, including machine learning and natural language processing, to help our clients achieve their business goals efficiently and effectively, ensuring a greater return on investment (ROI) through enhanced patient outcomes and streamlined operations. Our expertise in AI and Blockchain development positions us as a trusted partner in navigating the complexities of modern healthcare technology, including artificial intelligence in medical diagnosis and AI for health.
Refer to the image for a visual representation of the potential benefits of AI in healthcare outcomes and costs.
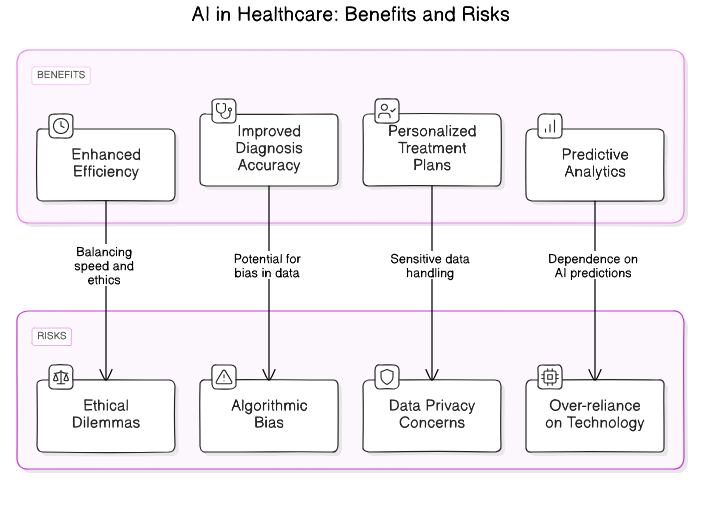
2.1. Machine Learning and Deep Learning: Foundations of AI in Medicine
Machine learning (ML) and deep learning (DL) are subsets of artificial intelligence (AI) that have transformed various fields, including medicine. These technologies enable computers to learn from data, identify patterns, and make decisions with minimal human intervention.
- Machine Learning: Â
- Involves algorithms that improve automatically through experience.
- Utilizes structured data to make predictions or classifications.
- Common algorithms include decision trees, support vector machines, and neural networks.
- Deep Learning: Â
- A specialized form of machine learning that uses neural networks with many layers.
- Particularly effective for unstructured data like images, audio, and text.
- Examples include convolutional neural networks (CNNs) and recurrent neural networks (RNNs).
The integration of ML and DL in medicine has led to significant advancements in diagnostics, treatment planning, and patient care, including applications in machine learning in medicine, machine learning for medical imaging, and deep learning in medical imaging.
2.1.1. Predictive Analytics for Disease Trends and Patient Outcomes
Predictive analytics leverages historical data and statistical algorithms to forecast future outcomes. In the medical field, this approach is crucial for understanding disease trends and improving patient outcomes.
- Disease Trend Analysis: Â
- Identifies patterns in disease prevalence and incidence.
- Helps public health officials allocate resources effectively.
- Can predict outbreaks and inform preventive measures.
- Patient Outcome Prediction: Â
- Utilizes patient data (demographics, medical history, etc.) to predict health outcomes.
- Assists healthcare providers in making informed decisions about treatment plans.
- Enhances personalized medicine by tailoring interventions to individual patients, aligning with the principles of precision medicine machine learning.
- Tools and Techniques: Â
- Machine learning models analyze large datasets to uncover insights.
- Techniques like regression analysis, clustering, and classification are commonly used.
- Real-time data integration from electronic health records (EHRs) improves accuracy.
- Impact on Healthcare: Â
- Reduces hospital readmission rates by identifying at-risk patients.
- Improves chronic disease management through proactive interventions.
- Enhances clinical trial design by identifying suitable patient populations, as seen in machine learning medical diagnosis.
2.1.2. Deep Learning Applications in Medical Research
Deep learning has opened new avenues in medical research, enabling breakthroughs in various domains.
- Medical Imaging: Â
- Deep learning algorithms analyze medical images (X-rays, MRIs, CT scans) for diagnosis.
- CNNs excel in image recognition tasks, detecting anomalies like tumors or fractures.
- Studies show that DL models can match or exceed human radiologists in accuracy, particularly in deep learning in medical image analysis.
- Genomics and Personalized Medicine: Â
- Deep learning aids in analyzing genomic data to identify genetic markers for diseases.
- Facilitates the development of personalized treatment plans based on genetic profiles.
- Enables researchers to uncover complex relationships between genes and diseases, contributing to machine learning in precision medicine.
- Drug Discovery: Â
- Accelerates the drug discovery process by predicting molecular interactions.
- Deep learning models can analyze vast chemical libraries to identify potential drug candidates.
- Reduces the time and cost associated with bringing new drugs to market.
- Clinical Decision Support: Â
- DL systems assist clinicians by providing evidence-based recommendations.
- Analyzes patient data and medical literature to support diagnosis and treatment decisions.
- Enhances the efficiency of healthcare delivery by reducing cognitive load on providers, as seen in artificial intelligence and machine learning in healthcare.
- Challenges and Considerations: Â
- Data privacy and security concerns must be addressed.
- The need for high-quality, annotated datasets for training models.
- Ensuring transparency and interpretability of deep learning models in clinical settings.
At Rapid Innovation, we leverage our expertise in AI and blockchain to help healthcare organizations implement these advanced technologies effectively. By utilizing machine learning and deep learning, we assist clients in enhancing patient care, optimizing operational efficiency, and ultimately achieving greater ROI. Our tailored solutions ensure that healthcare providers can harness the power of data-driven insights to make informed decisions, improve patient outcomes, and streamline processes, including the use of machine learning in healthcare and machine learning in the medical field.
Refer to the image for a visual representation of the concepts discussed in 2.1 Machine Learning and Deep Learning: Foundations of AI in Medicine.
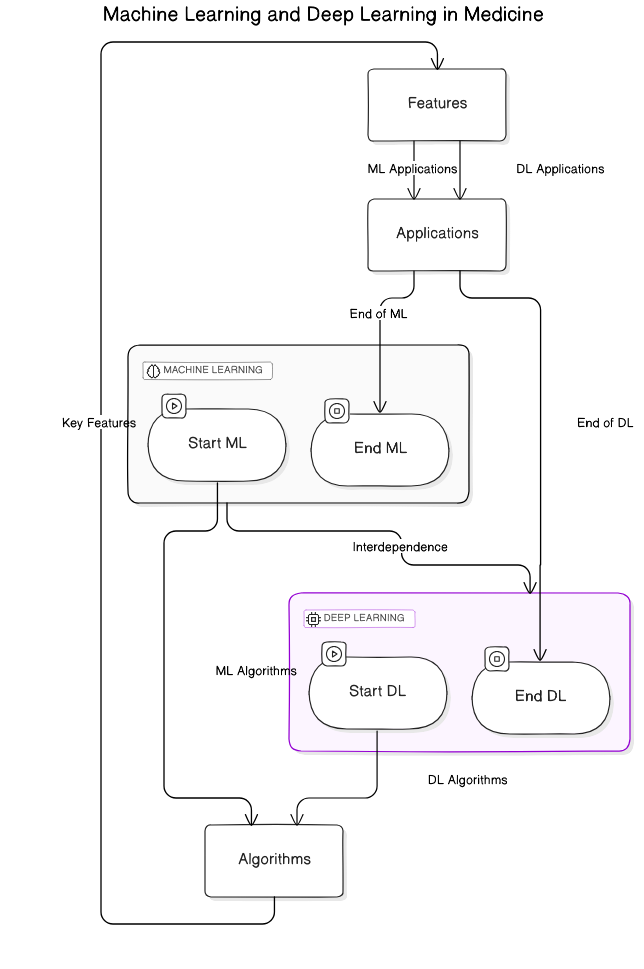
2.2. Natural Language Processing (NLP) in Healthcare
Natural Language Processing (NLP) is a branch of artificial intelligence that focuses on the interaction between computers and human language. In healthcare, NLP is transforming how medical professionals manage and analyze vast amounts of data. By enabling machines to understand and interpret human language, NLP enhances patient care, streamlines operations, and improves clinical outcomes.
2.2.1. Using NLP for Patient Record Analysis
NLP plays a crucial role in analyzing patient records, which are often unstructured and complex. This technology helps healthcare providers extract meaningful insights from electronic health records (EHRs) and other documentation.
- Data Extraction: NLP algorithms can sift through large volumes of patient records to identify key information such as diagnoses, medications, and treatment plans. This extraction helps in creating comprehensive patient profiles that can inform clinical decisions.
- Sentiment Analysis: NLP can analyze patient feedback and notes to gauge sentiment, helping providers understand patient satisfaction and areas needing improvement. This analysis can lead to better patient engagement and tailored care strategies.
- Predictive Analytics: By analyzing historical patient data, NLP can help predict future health outcomes, enabling proactive interventions. For instance, identifying patients at risk for readmission can lead to targeted follow-up care.
- Clinical Research: NLP facilitates the mining of clinical data for research purposes, allowing researchers to identify trends and correlations in patient outcomes. This can accelerate the development of new treatments and improve existing protocols.
- Regulatory Compliance: NLP can assist in ensuring that patient records meet regulatory standards by flagging incomplete or non-compliant documentation. This reduces the risk of legal issues and enhances the quality of care.
2.2.2. Automating Clinical Documentation
Automating clinical documentation is another significant application of NLP in healthcare. This process reduces the administrative burden on healthcare providers, allowing them to focus more on patient care.
- Speech Recognition: NLP-powered speech recognition tools enable clinicians to dictate notes directly into EHRs, streamlining the documentation process. This technology can significantly reduce the time spent on paperwork, allowing for more patient interaction.
- Template Generation: NLP can automatically generate documentation templates based on the context of the patient visit, ensuring that all necessary information is captured. This standardization improves consistency and accuracy in clinical records.
- Error Reduction: By automating documentation, NLP minimizes human errors that can occur during manual data entry. This leads to more reliable patient records and enhances overall patient safety.
- Real-time Updates: NLP systems can update patient records in real-time during consultations, ensuring that information is current and accessible. This immediacy supports better decision-making and care coordination.
- Billing and Coding: NLP can assist in automating the coding process for billing, ensuring that services rendered are accurately documented and billed. This reduces the administrative workload and improves revenue cycle management.
In conclusion, NLP is revolutionizing healthcare by enhancing patient record analysis and automating clinical documentation. These advancements not only improve efficiency but also contribute to better patient outcomes and satisfaction. At Rapid Innovation, we leverage our expertise in NLP in healthcare to help healthcare organizations optimize their operations, ultimately leading to greater ROI and improved patient care. By integrating advanced NLP solutions, such as medical natural language processing and healthcare natural language processing, we empower our clients to harness the full potential of their data, ensuring they stay ahead in a rapidly evolving healthcare landscape. Additionally, we explore various applications of NLP in healthcare, including sentiment analysis, predictive analytics, and the use of NLP for medical records, to provide comprehensive solutions tailored to the needs of healthcare providers. For more information on our services, visit our AI Healthcare Management page.
2.3. Computer Vision and Medical Imaging
Computer vision is a field of artificial intelligence that enables machines to interpret and understand visual information from the world. In medical imaging, computer vision plays a crucial role in enhancing diagnostic accuracy and efficiency. By analyzing images from various imaging modalities, AI can assist healthcare professionals in making informed decisions.
2.3.1. AI in Analyzing X-rays, MRIs, and CT Scans
AI technologies are increasingly being integrated into the analysis of medical images such as X-rays, MRIs, and CT scans. These technologies can significantly improve the speed and accuracy of diagnoses.
- X-ray Analysis: AI algorithms can detect abnormalities such as fractures, tumors, and infections. Machine learning models are trained on large datasets to recognize patterns indicative of specific conditions.
- MRI Interpretation: AI can assist in segmenting brain structures and identifying lesions. Deep learning techniques enhance the ability to differentiate between healthy and pathological tissues. For instance, artificial intelligence in MRI can improve the accuracy of identifying lesions.
- CT Scan Evaluation: AI systems can quickly analyze cross-sectional images to identify issues like pulmonary embolisms or cancers. Automated detection tools reduce the workload on radiologists, allowing them to focus on complex cases. AI in CT scan applications has shown significant promise in enhancing diagnostic capabilities.
The integration of AI in these imaging modalities has shown promising results, with studies indicating that AI can match or even exceed human performance in certain diagnostic tasks. For instance, a study found that AI systems could achieve an accuracy rate of over 90% in detecting pneumonia from chest X-rays.
2.3.2. Early Detection through Image Analysis
Early detection of diseases is critical for effective treatment and improved patient outcomes. AI-driven image analysis enhances the ability to identify conditions at their nascent stages.
- Cancer Detection: AI algorithms can analyze mammograms to detect breast cancer earlier than traditional methods. Studies have shown that AI can reduce false positives and negatives, leading to more accurate screenings. The use of AI in medical imaging for cancer detection is becoming increasingly prevalent.
- Neurological Disorders: AI can identify early signs of conditions like Alzheimer's disease by analyzing MRI scans for subtle changes in brain structure. Early intervention can significantly slow disease progression and improve quality of life. AI in medical imaging is crucial for detecting such neurological disorders.
- Cardiovascular Issues: AI tools can analyze cardiac imaging to detect abnormalities in heart function and structure. Early identification of conditions such as coronary artery disease can lead to timely treatment and better outcomes. The integration of AI in medical imaging for cardiovascular issues is a growing area of research.
The use of AI in early detection is supported by research indicating that machine learning models can improve diagnostic accuracy by up to 20% compared to traditional methods. This capability not only enhances patient care but also reduces healthcare costs by minimizing the need for advanced treatments that may arise from late-stage diagnoses.
At Rapid Innovation, we leverage our expertise in AI and blockchain to provide tailored solutions that enhance the capabilities of healthcare providers. By integrating advanced AI algorithms into medical imaging processes, including AI medical image analysis and AI imaging healthcare, we help our clients achieve greater ROI through improved diagnostic accuracy, reduced operational costs, and enhanced patient outcomes. Our commitment to innovation ensures that healthcare organizations can harness the full potential of AI technologies, ultimately leading to more efficient and effective healthcare delivery. For more information on our services, visit our AI consulting company.
2.4. Robotics and Automation
Robotics and automation are transforming the healthcare landscape, enhancing precision, efficiency, and patient outcomes. These technologies are being integrated into various aspects of healthcare, from surgical procedures to administrative tasks, including the use of medical robots and robotics in healthcare.
2.4.1. Robotics in Surgery and Interventions
Robotic systems are increasingly being utilized in surgical settings, offering numerous advantages over traditional methods. These advantages include:
- Enhanced Precision: Robotic-assisted surgeries allow for greater accuracy in procedures, minimizing the risk of human error.
- Minimally Invasive Techniques: Many robotic surgeries are performed using smaller incisions, leading to reduced recovery times and less postoperative pain for patients.
- Improved Visualization: Robotic systems often come equipped with high-definition cameras and 3D visualization, providing surgeons with a better view of the surgical site.
- Versatility: Robotics can be applied in various surgical specialties, including: Â
- Urology
- Gynecology
- Orthopedics
- Cardiac surgery
- Training and Simulation: Robotics also play a role in training new surgeons, allowing them to practice techniques in a controlled environment before performing on patients.
- Cost Considerations: While the initial investment in robotic systems can be high, studies suggest that they may lead to cost savings in the long run due to shorter hospital stays and fewer complications.
2.4.2. AI-Powered Automation in Healthcare Workflows
Artificial intelligence (AI) is revolutionizing healthcare workflows by automating routine tasks and improving decision-making processes. Key benefits of AI in healthcare include:
- Streamlined Administrative Tasks: AI can automate scheduling, billing, and patient record management, freeing up healthcare professionals to focus on patient care.
- Enhanced Data Analysis: AI algorithms can analyze vast amounts of patient data quickly, identifying patterns and trends that may not be apparent to human analysts.
- Predictive Analytics: AI can help predict patient outcomes and potential complications, allowing for proactive interventions.
- Improved Patient Engagement: Chatbots and virtual assistants powered by AI can provide patients with information and support, improving their overall experience.
- Resource Allocation: AI can optimize resource management, ensuring that staff and equipment are used efficiently, which is particularly important in high-demand situations.
- Clinical Decision Support: AI tools can assist healthcare providers in making informed decisions by providing evidence-based recommendations.
- Cost Efficiency: By reducing administrative burdens and improving operational efficiency, AI-powered automation can lead to significant cost savings for healthcare organizations.
At Rapid Innovation, we leverage our expertise in AI and robotics to help healthcare organizations implement these advanced technologies effectively. By partnering with us, clients can achieve greater ROI through improved operational efficiencies, enhanced patient care, and reduced costs associated with traditional healthcare practices. Our tailored solutions ensure that healthcare providers can navigate the complexities of integrating robotics and automation, including medical device robotics and healthcare robotics companies, into their workflows, ultimately leading to better patient outcomes and a more sustainable business model. The integration of robots in the medical field, such as home health care robots and robot medical assistants, exemplifies the potential of robotics in healthcare.
3. Clinical Applications and Patient Care
3.1. Diagnosis and Early Disease Detection
3.1.1. AI-Driven Diagnostics Tools: Digital Stethoscopes, Diagnostic Apps
AI-driven diagnostic tools are revolutionizing the way healthcare professionals diagnose and monitor diseases. These tools leverage advanced algorithms and machine learning to enhance traditional diagnostic methods, leading to improved patient outcomes.
- Digital Stethoscopes: Digital stethoscopes are equipped with sensors that capture heart and lung sounds with high fidelity. They often include features like noise cancellation, which allows for clearer auscultation in noisy environments. Many digital stethoscopes can connect to smartphones or tablets, enabling real-time data sharing and analysis. Some models incorporate AI-driven diagnostic tools that can analyze heart sounds and detect abnormalities, such as murmurs or arrhythmias, with high accuracy. These devices can store and transmit data, facilitating remote consultations and second opinions.
- Diagnostic Apps: Diagnostic apps utilize AI to assist healthcare providers in making clinical decisions. They can analyze patient symptoms and medical history to suggest possible diagnoses. Many apps are designed to be user-friendly, allowing both healthcare professionals and patients to input data easily. Some diagnostic apps use image recognition technology to analyze medical images, such as X-rays or skin lesions, for signs of disease. These AI-driven diagnostic tools can help in triaging patients, ensuring that those who need immediate care receive it promptly.
- Benefits of AI-Driven Tools: Â
- Enhanced accuracy: AI algorithms can process vast amounts of data, leading to more accurate diagnoses.
- Early detection: By identifying potential health issues sooner, these tools can facilitate timely interventions.
- Increased efficiency: Healthcare providers can save time on routine diagnostics, allowing them to focus on patient care.
- Improved patient engagement: Patients can use apps to monitor their health, leading to better adherence to treatment plans.
- Challenges and Considerations: Â
- Data privacy: The use of digital tools raises concerns about the security of patient information.
- Integration with existing systems: Healthcare facilities may face challenges in incorporating new technologies into their workflows.
- Dependence on technology: Over-reliance on AI tools may lead to diminished clinical skills among healthcare providers.
At Rapid Innovation, we understand the transformative potential of AI-driven diagnostic tools in enhancing patient care. By integrating these advanced technologies into healthcare systems, we help our clients achieve greater efficiency and accuracy in their diagnostic processes. Our expertise in AI development ensures that healthcare providers can leverage these tools effectively, ultimately leading to improved patient outcomes and a higher return on investment. AI-driven diagnostic tools, including digital stethoscopes and diagnostic apps, are not just innovations; they are essential components of a modern healthcare strategy that can significantly enhance the quality of care delivered to patients. For more insights on the role of AI in healthcare, check out our article on computer vision in healthcare.
3.1.2. Early Detection for Chronic Diseases and Cancer
Early detection of chronic diseases and cancer is crucial for improving patient outcomes and reducing mortality rates. Advances in technology and medical research have significantly enhanced our ability to identify these conditions at an earlier stage.
- Screening Programs: Regular screening programs for diseases like breast cancer, cervical cancer, and colorectal cancer have been shown to reduce mortality rates. For instance, mammograms can detect early stage breast cancer up to three years before it becomes palpable. Additionally, the galleri test is emerging as a promising tool for early detection of multiple cancers.
- Biomarkers: The identification of specific biomarkers in blood tests, such as the galleri blood test, can indicate the presence of certain cancers or chronic diseases. For example, prostate-specific antigen (PSA) tests are used to screen for prostate cancer.
- Imaging Techniques: Advanced imaging technologies, such as MRI, CT scans, and PET scans, allow for the visualization of internal organs and tissues, aiding in the early detection of tumors and other abnormalities. This is particularly important for conditions like skin cancer early detection and early detection of lung cancer.
- Genetic Testing: Genetic tests can identify individuals at high risk for certain cancers, enabling proactive monitoring and preventive measures. For example, BRCA1 and BRCA2 gene mutations significantly increase the risk of breast and ovarian cancers. The grail cancer test and grail cancer screening are also notable advancements in genetic testing for early detection.
- Artificial Intelligence: AI algorithms are increasingly being used to analyze medical data and imaging results, improving the accuracy of early detection. Studies have shown that AI can outperform human radiologists in identifying certain cancers in imaging scans, including early detection of pancreatic cancer and early detection of colon cancer.
3.2. Personalized AI Solutions for Medicine and Genomics
Personalized medicine is an innovative approach that tailors medical treatment to the individual characteristics of each patient. This method relies heavily on genomics, the study of genes and their functions.
- Genetic Profiling: By analyzing a patient's genetic makeup, healthcare providers can identify specific mutations that may influence disease risk and treatment response. This is particularly relevant for conditions like early stage ovarian cancer ultrasound and early detection of prostate cancer.
- Targeted Therapies: Personalized medicine often involves the use of targeted therapies that specifically attack cancer cells based on their genetic profile. For example, drugs like trastuzumab (Herceptin) are effective in treating HER2-positive breast cancer.
- Pharmacogenomics: This field studies how genes affect a person's response to drugs, allowing for the customization of medication types and dosages. For instance, patients with certain genetic variants may metabolize drugs differently, necessitating dosage adjustments.
- Improved Outcomes: Personalized medicine has been associated with better patient outcomes, including higher response rates to treatment and fewer side effects. Studies indicate that patients receiving personalized treatments often experience improved survival rates, particularly in cases like first stages of oral cancer and mouth cancer first stage.
- Cost-Effectiveness: Although personalized medicine can be expensive, it may ultimately reduce healthcare costs by minimizing ineffective treatments and hospitalizations.
3.2.1. AI in Personalized Treatment Plans Based on Genetics
Artificial intelligence (AI) is revolutionizing the development of personalized treatment plans by leveraging genetic information to optimize patient care.
- Data Analysis: AI can analyze vast amounts of genetic data quickly, identifying patterns and correlations that may not be apparent to human researchers. This capability enhances the understanding of how genetic variations influence disease and treatment responses.
- Predictive Modeling: AI algorithms can create predictive models that forecast how a patient will respond to specific treatments based on their genetic profile. This allows for more informed decision-making in treatment planning.
- Real-Time Adjustments: AI systems can continuously learn from new data, enabling real-time adjustments to treatment plans as more information becomes available. This adaptability is crucial in managing complex diseases like cancer.
- Integration with Electronic Health Records: AI can integrate genetic data with electronic health records (EHRs), providing healthcare providers with a comprehensive view of a patient's health history and genetic predispositions. This integration supports more personalized and effective treatment strategies.
- Clinical Trials: AI can identify suitable candidates for clinical trials based on genetic markers, facilitating the development of new therapies tailored to specific patient populations. This approach accelerates the discovery of effective treatments.
By harnessing the power of AI and genomics, healthcare providers can create more effective, personalized treatment plans that improve patient outcomes and enhance the overall quality of care.
At Rapid Innovation, we leverage our expertise in AI and blockchain technology to help healthcare organizations implement these advanced solutions. By integrating AI-driven analytics and blockchain for secure data management, we enable our clients to enhance early detection capabilities, such as through the galleri tests, and personalize treatment plans, ultimately leading to improved patient outcomes and greater ROI.
3.2.2. Genomic Data Analysis for Precision Medicine
Genomic data analysis plays a crucial role in the advancement of precision medicine, which tailors medical treatment to the individual characteristics of each patient. This approach relies on understanding the genetic makeup of patients to predict disease risk, treatment responses, and outcomes.
- Personalized Treatment Plans: Genomic data allows healthcare providers to create customized treatment plans based on a patient's genetic profile. This can lead to more effective therapies with fewer side effects, ultimately enhancing patient satisfaction and outcomes. Techniques such as dna methylation data analysis and whole genome sequencing data analysis are essential in this process.
- Disease Prediction: By analyzing genomic data, clinicians can identify genetic predispositions to certain diseases, enabling early intervention and preventive measures. This proactive approach can significantly reduce long-term healthcare costs. Tools for genome sequence analysis and targeted genomic sequence analysis are vital for accurate predictions.
- Targeted Therapies: Certain cancers and genetic disorders can be treated more effectively with targeted therapies that specifically address the genetic mutations present in a patient’s tumor or condition. This precision not only improves treatment efficacy but also optimizes resource allocation in healthcare settings. Whole exome sequencing data analysis can provide insights into these mutations.
- Pharmacogenomics: This field studies how genes affect a person's response to drugs, helping to determine the most effective medications and dosages for individual patients. By leveraging pharmacogenomics, healthcare providers can minimize trial-and-error prescribing, leading to faster recovery times and reduced healthcare expenditures. Analyzing genetic data through methods like microarray gene expression analysis can enhance pharmacogenomic strategies.
- Data Integration: Combining genomic data with other health information (like electronic health records) enhances the understanding of complex diseases and improves patient outcomes. Rapid Innovation can assist in developing integrated systems that streamline data flow, ensuring that healthcare providers have access to comprehensive patient information, including whole genome sequencing results and tcga data analysis.
- Ethical Considerations: The use of genomic data raises ethical issues, including privacy concerns and the potential for genetic discrimination. Rapid Innovation emphasizes the importance of implementing robust security measures and compliance protocols to protect sensitive patient information.
3.3. AI for Patient Monitoring and Management
Patient monitoring and management are essential components of modern healthcare, focusing on tracking patient health and ensuring timely interventions. This approach enhances patient outcomes and optimizes healthcare resources.
- Continuous Monitoring: Technologies enable real-time tracking of vital signs and health metrics, allowing for immediate response to any changes in a patient's condition. Rapid Innovation can develop AI-driven solutions that enhance monitoring capabilities, ensuring timely interventions.
- Chronic Disease Management: Effective monitoring systems are crucial for managing chronic diseases such as diabetes, hypertension, and heart disease, helping patients maintain their health and avoid complications. Our expertise in AI can help create predictive models that alert healthcare providers to potential issues before they escalate.
- Telehealth Integration: Remote monitoring tools can be integrated with telehealth services, allowing healthcare providers to consult with patients without the need for in-person visits. Rapid Innovation can facilitate seamless integration, improving access to care and patient satisfaction.
- Data-Driven Decisions: Analyzing collected data helps healthcare providers make informed decisions regarding treatment adjustments and care plans. Our AI solutions can enhance data analytics capabilities, providing actionable insights that lead to better patient outcomes.
- Patient Engagement: Empowering patients with tools to monitor their health encourages active participation in their care, leading to better adherence to treatment plans. Rapid Innovation can develop user-friendly applications that enhance patient engagement and education.
- Cost-Effectiveness: Efficient patient monitoring can reduce hospital readmissions and emergency visits, ultimately lowering healthcare costs. By implementing our innovative solutions, healthcare organizations can achieve significant cost savings while improving care quality.
3.3.1. AI for Health Monitoring and Wearable Tech
Artificial Intelligence (AI) is revolutionizing health monitoring through the integration of wearable technology, providing innovative AI solutions for tracking health metrics and enhancing patient care.
- Wearable Devices: Devices like smartwatches and fitness trackers monitor various health parameters, including heart rate, sleep patterns, and physical activity levels. Rapid Innovation can assist in developing advanced wearable technologies that provide real-time health insights.
- Real-Time Data Analysis: AI algorithms analyze data collected from wearables to detect anomalies and provide insights into a user’s health status. Our expertise in AI can enhance the accuracy and reliability of these analyses, leading to better health outcomes.
- Predictive Analytics: AI can predict potential health issues by analyzing trends in the data, allowing for proactive interventions before conditions worsen. Rapid Innovation can create predictive models that empower healthcare providers to act swiftly and effectively.
- Personalized Feedback: Wearable tech equipped with AI can offer tailored health recommendations based on individual data, promoting healthier lifestyle choices. Our solutions can help healthcare providers deliver personalized care that resonates with patients.
- Integration with Healthcare Systems: Wearable devices can sync with electronic health records, providing healthcare providers with comprehensive data for better patient management. Rapid Innovation specializes in creating interoperable systems that enhance data sharing and collaboration.
- Remote Patient Monitoring: AI-powered wearables facilitate remote monitoring, enabling healthcare professionals to keep track of patients' health without requiring them to visit a clinic. This capability is essential for improving access to care, especially in underserved areas.
- Enhanced Research Opportunities: The data collected from wearables can be used for research purposes, helping to identify patterns and correlations in health data across diverse populations. Rapid Innovation can support research initiatives by providing the necessary tools and infrastructure for data analysis, including raw genome data analysis and wgs data analysis.
- Â
- 3.3.2. Enhancing Patient Engagement and Compliance
- Patient engagement refers to the involvement of patients in their own healthcare decisions and management.
- AI technologies can facilitate better communication between healthcare providers and patients, ensuring that patients are well-informed and actively participating in their care.
- Personalized health information can be delivered through AI-driven platforms, making it easier for patients to understand their conditions and treatment options, ultimately leading to more informed decision-making.
- AI can analyze patient data to provide tailored reminders for medication and appointments, significantly improving adherence to treatment plans and enhancing overall health outcomes.
- Interactive chatbots can answer patient queries in real-time, providing support and information outside of regular office hours, thus increasing accessibility and patient satisfaction.
- Gamification techniques can be integrated into health apps, including patient engagement mobile apps, to motivate patients to engage in their health management actively, fostering a sense of ownership over their health journey.
- Studies show that engaged patients are more likely to follow treatment plans, leading to better health outcomes.
- Enhanced patient engagement, supported by digital patient engagement strategies, can lead to reduced hospital readmissions and lower healthcare costs, ultimately contributing to a more efficient healthcare system.
- The use of patient portals and patient engagement technology can further enhance communication and access to health information.
3.3.3. Reducing Medical Errors with AI-Based Checks
- Medical errors can occur due to miscommunication, incorrect data entry, or oversight in patient care.
- AI systems can analyze vast amounts of patient data to identify potential errors before they occur, thereby enhancing the safety and reliability of healthcare delivery.
- Automated checks can flag discrepancies in medication dosages, allergies, and patient histories, alerting healthcare providers to potential issues and allowing for timely interventions.
- Natural language processing (NLP) can be used to extract relevant information from unstructured data, such as clinical notes, to ensure accurate patient records and improve clinical decision-making.
- AI can assist in diagnostic processes by cross-referencing symptoms with a database of conditions, reducing the likelihood of misdiagnosis and improving patient outcomes.
- Machine learning algorithms can continuously learn from past errors, improving their predictive capabilities over time and further enhancing patient safety.
- Research indicates that AI can reduce diagnostic errors by up to 20% in certain medical fields.
- Implementing AI-based checks can enhance patient safety and trust in healthcare systems, ultimately leading to a more effective healthcare environment.
4. Specialized Care AI Applications
- Specialized care applications focus on specific medical fields or conditions, providing tailored solutions for patients and healthcare providers.
- Telemedicine platforms enable specialists to consult with patients remotely, increasing access to care for those in underserved areas and improving overall healthcare accessibility.
- AI-driven diagnostic tools can assist specialists in identifying rare diseases or conditions that may be overlooked in general practice, ensuring timely and accurate diagnoses.
- Mobile health applications, including patient engagement mobile health apps, can help manage chronic conditions, such as diabetes or hypertension, by providing real-time monitoring and feedback, empowering patients to take control of their health.
- Virtual reality (VR) and augmented reality (AR) technologies can be used in specialized training for healthcare professionals, enhancing their skills in complex procedures and improving the quality of care.
- Personalized treatment plans can be developed using AI algorithms that analyze genetic information and patient history, leading to more effective therapies tailored to individual needs.
- Specialized care applications can improve patient outcomes by ensuring that individuals receive the most appropriate and timely interventions.
- The integration of AI in specialized care has the potential to streamline workflows, reduce costs, and enhance the overall quality of care, ultimately driving greater ROI for healthcare organizations.
At Rapid Innovation, we leverage our expertise in AI and Blockchain to help healthcare providers implement these advanced technologies, ensuring they achieve their business goals efficiently and effectively. By enhancing patient engagement through patient engagement technology and telehealth patient engagement, reducing medical errors, and developing specialized care applications, we empower our clients to deliver superior healthcare services while maximizing their return on investment.
4.1. AI for Mental and Elderly Care
4.1.1. AI for Mental Health Support: Diagnosis and Treatment
Artificial Intelligence (AI) is increasingly being integrated into mental health care, providing innovative solutions for diagnosis and treatment.
- Enhanced Diagnosis: Â
- AI algorithms can analyze vast amounts of data from various sources, including social media, electronic health records, and patient surveys.
- Machine learning models can identify patterns and predict mental health issues, such as depression or anxiety, often before a human clinician can.
- Tools like chatbots can conduct preliminary assessments, helping to triage patients based on their symptoms.
- Personalized Treatment: Â
- AI can tailor treatment plans to individual needs by analyzing patient data and treatment responses.
- Predictive analytics can help clinicians understand which therapies are likely to be most effective for specific patients.
- Virtual reality (VR) and AI-driven applications can provide immersive therapy experiences, such as exposure therapy for phobias.
- Accessibility and Support: Â
- AI-powered apps can offer 24/7 support, making mental health resources more accessible to those in need.
- These tools can provide coping strategies, mindfulness exercises, and mood tracking, empowering users to manage their mental health proactively.
- AI can also facilitate remote therapy sessions, connecting patients with therapists through telehealth platforms.
- Platforms like wysa mental health support and ai mental health support are examples of how technology can enhance accessibility and provide immediate assistance.
4.1.2. AI Solutions for Elderly Care and Assisted Living
AI technologies are transforming elderly care and assisted living, enhancing the quality of life for seniors and supporting caregivers.
- Monitoring and Safety: Â
- AI systems can monitor seniors' daily activities and detect anomalies, such as falls or changes in routine, alerting caregivers or emergency services when necessary.
- Wearable devices equipped with AI can track vital signs and health metrics, providing real-time data to healthcare providers.
- Smart home technologies can automate tasks, such as adjusting lighting or temperature, ensuring a comfortable living environment.
- Companionship and Engagement: Â
- AI-powered robots and virtual assistants can provide companionship, reducing feelings of loneliness and isolation among seniors.
- These technologies can engage users in conversation, play games, or remind them to take medications, promoting cognitive engagement.
- Interactive platforms can facilitate social connections, allowing seniors to communicate with family and friends easily.
- Caregiver Support: Â
- AI can assist caregivers by providing insights into the health and well-being of their elderly clients, helping them make informed decisions.
- Predictive analytics can help anticipate care needs, allowing caregivers to plan and allocate resources more effectively.
- Training programs powered by AI can enhance caregiver skills, ensuring they are equipped to handle various challenges in elderly care.
At Rapid Innovation, we leverage our expertise in AI to develop tailored solutions that enhance mental health support and elderly care. By integrating advanced AI technologies, we help our clients achieve greater ROI through improved patient outcomes, increased operational efficiency, and enhanced user engagement. Our commitment to innovation ensures that we remain at the forefront of these transformative fields, empowering organizations to meet their business goals effectively and efficiently.
4.1.3. Conversational AI and Health Chatbots for Patient Support
Conversational AI and health chatbots, including medical AI chatbots and healthcare chatbots, are transforming the way patients interact with healthcare systems. These technologies provide immediate assistance and support, enhancing patient experience and engagement. Health chatbots can operate around the clock, offering patients access to information and support at any time. Many chatbots, such as AI chatbots for healthcare, can assess symptoms and provide preliminary advice, helping patients determine whether they need to seek further medical attention. Additionally, chatbots can facilitate the scheduling of appointments, reducing the administrative burden on healthcare staff. They can also send reminders for medication adherence, improving patient compliance and health outcomes. Furthermore, conversational AI can provide mental health resources and support, offering a safe space for patients to discuss their feelings. These tools can gather patient data, which can be used to improve care and personalize treatment plans. Finally, implementing chatbots, including healthcare conversational AI, can reduce operational costs for healthcare providers while maintaining high levels of patient support.
At Rapid Innovation, we leverage our expertise in AI to develop customized conversational AI solutions that align with your healthcare objectives. By integrating these advanced technologies, we help healthcare providers enhance patient engagement, streamline operations, and ultimately achieve greater ROI. For more information on healthcare chatbot development, visit our healthcare chatbot development page.
4.2. Emergency and Population Health
Emergency and population health are critical areas where AI can make a significant impact. The integration of AI technologies can enhance response times, improve patient outcomes, and optimize resource allocation. AI can analyze data to predict outbreaks and identify at-risk populations, allowing for proactive measures. It can also help in efficiently distributing resources during emergencies, ensuring that the most affected areas receive timely assistance. AI systems can process vast amounts of data in real-time, providing insights that can guide emergency response efforts. Additionally, AI can track health trends and monitor population health metrics, aiding in the identification of public health issues. AI tools can facilitate communication between health authorities and the public, improving awareness and response to health crises.
4.2.1. AI in Emergency Response and Medical Triage
AI plays a crucial role in emergency response and medical triage, streamlining processes and improving patient care during critical situations. AI algorithms can quickly assess patient conditions based on symptoms and vital signs, prioritizing those who need immediate care. AI can assist healthcare professionals in making informed decisions by providing evidence-based recommendations during emergencies. It can also optimize the use of medical supplies and personnel, ensuring that resources are allocated where they are most needed. Furthermore, AI can enhance telemedicine services, allowing for remote assessments and consultations during emergencies. AI can be used to create realistic training scenarios for emergency responders, improving their preparedness for real-life situations. Finally, AI can analyze past emergency responses to identify areas for improvement, leading to better preparedness for future incidents.
At Rapid Innovation, we harness the power of AI to develop solutions that enhance emergency response capabilities, ensuring that healthcare providers can deliver timely and effective care when it matters most. Our expertise in AI-driven analytics and real-time data processing enables organizations to optimize their resources and improve patient outcomes, ultimately driving greater ROI.
4.2.2. AI in Population Health Management for Community Health
Artificial Intelligence (AI) is transforming population health management by enabling healthcare providers to analyze vast amounts of data to improve community health outcomes.
- Predictive Analytics: AI algorithms can analyze historical health data to predict future health trends and outbreaks. This allows for proactive measures to be taken, such as vaccination drives or health education campaigns, ultimately leading to improved community health and reduced healthcare costs.
- Risk Stratification: AI can identify high-risk populations by analyzing various factors, including demographics, socioeconomic status, and medical history. This targeted approach helps allocate resources more effectively and tailor interventions to specific community needs, enhancing the overall efficiency of healthcare delivery.
- Personalized Interventions: AI can help design personalized health programs based on individual risk profiles, leading to better adherence to treatment plans and improved health outcomes. This not only benefits patients but also optimizes resource utilization for healthcare providers.
- Data Integration: AI systems can integrate data from multiple sources, including electronic health records (EHRs), social determinants of health, and community resources. This holistic view enables healthcare providers to address the root causes of health disparities, fostering a more equitable healthcare environment.
- Community Engagement: AI tools can facilitate communication between healthcare providers and community members. Engaging the community in health initiatives can lead to increased participation and better health outcomes, ultimately driving greater ROI for healthcare organizations.
5. Healthcare Operations and Administration
Healthcare operations and administration encompass the management of healthcare facilities, ensuring that they run efficiently and effectively.
- Resource Management: Efficient allocation of resources, including staff, equipment, and facilities, is crucial for optimal operations. AI can assist in forecasting demand and managing inventory, reducing waste and costs, which directly contributes to improved financial performance.
- Workflow Optimization: Streamlining processes, such as patient scheduling and billing, can enhance operational efficiency. AI-driven tools can automate routine tasks, allowing staff to focus on patient care, thereby increasing productivity and patient satisfaction.
- Quality Improvement: Continuous quality improvement initiatives are essential for maintaining high standards of care. Data analytics can identify areas for improvement and track the effectiveness of interventions, ensuring that healthcare organizations meet regulatory standards and enhance patient outcomes.
- Compliance and Regulation: Healthcare organizations must adhere to various regulations and standards. AI can help monitor compliance and flag potential issues before they become significant problems, reducing the risk of costly penalties and enhancing operational integrity.
- Financial Management: Effective financial management is critical for the sustainability of healthcare organizations. AI can analyze financial data to identify trends, optimize billing processes, and improve revenue cycle management, ultimately leading to greater profitability. This includes the implementation of AI in revenue cycle management and artificial intelligence in healthcare revenue cycle.
5.1. Clinical Operations and Workforce Management
Clinical operations and workforce management are vital components of healthcare administration, focusing on the delivery of patient care and the management of healthcare personnel.
- Staffing Optimization: AI can analyze patient volume and staff availability to create optimal staffing schedules, ensuring that healthcare facilities are adequately staffed during peak times, which improves patient care and operational efficiency.
- Training and Development: Continuous education and training are essential for healthcare professionals. AI can identify skill gaps and recommend training programs tailored to individual needs, fostering a more competent workforce.
- Performance Monitoring: Regular assessment of staff performance is crucial for maintaining high-quality care. AI tools can provide real-time feedback and analytics on staff performance metrics, enabling timely interventions and continuous improvement.
- Patient Flow Management: Efficient patient flow is essential for reducing wait times and improving patient satisfaction. AI can predict patient arrivals and optimize resource allocation accordingly, enhancing the overall patient experience.
- Telehealth Integration: The rise of telehealth has changed the landscape of clinical operations. AI can facilitate the integration of telehealth services, ensuring that patients receive timely care regardless of location, thus expanding access to healthcare and improving outcomes.
At Rapid Innovation, we leverage our expertise in AI and Blockchain to help healthcare organizations implement these advanced solutions, driving efficiency, enhancing patient care, and ultimately achieving greater ROI. This includes the application of machine learning in revenue cycle management and proactive healthcare management AI.
5.1.1. AI for Medical Records Management and EMR Optimization
AI technologies are transforming how medical records are managed and optimized. Electronic Medical Records (EMR) systems benefit from AI through improved data entry accuracy by using natural language processing (NLP) to convert speech to text, automated data extraction from various sources that reduces manual input and errors, and predictive analytics that help identify patient trends and potential health risks.
AI can streamline workflows by automating routine tasks such as appointment scheduling and reminders, as well as enhancing interoperability between different EMR systems, allowing for seamless data sharing. The use of AI in EMR optimization can lead to increased efficiency in healthcare delivery and better patient outcomes through timely access to accurate information. According to a study, AI can reduce the time spent on administrative tasks by up to 30%. The integration of AI in revenue cycle management also plays a crucial role in optimizing financial processes within healthcare organizations.
5.1.2. Healthcare Workforce Management: Staffing and Scheduling
Effective workforce management is crucial for healthcare organizations to ensure optimal patient care. AI tools can enhance staffing and scheduling by analyzing patient volume and staff availability to create efficient schedules, as well as predicting staffing needs based on historical data and trends, ensuring adequate coverage during peak times.
Benefits of AI in workforce management include reduced overtime costs by optimizing staff allocation, improved employee satisfaction through fair and balanced scheduling, and enhanced patient care by ensuring the right staff is available at the right time. AI can also assist in identifying skill gaps within the workforce and suggesting training programs, as well as monitoring staff performance and providing insights for improvement. A report indicates that AI-driven scheduling can improve staff productivity by 20%. The application of AI in healthcare administration further supports these efforts by streamlining operational processes.
5.1.3. Clinical Trials Optimization through AI for Efficiency
AI is revolutionizing the clinical trial process, making it more efficient and effective. Key areas where AI contributes to clinical trials include patient recruitment by analyzing large datasets to identify suitable candidates quickly, and monitoring patient data in real-time, allowing for timely adjustments to trial protocols.
AI can enhance trial design by utilizing machine learning algorithms to predict outcomes and optimize trial parameters, as well as simulating various scenarios to identify the most effective trial designs. Benefits of AI in clinical trials include reduced time to market for new drugs and therapies, lower costs associated with trial management and patient recruitment, and increased likelihood of trial success through better patient matching and data analysis. Research shows that AI can reduce the time required for patient recruitment by up to 50%. The role of artificial intelligence in healthcare management is becoming increasingly vital in ensuring the success of these trials.
At Rapid Innovation, we leverage these AI capabilities to help healthcare organizations achieve their business goals efficiently and effectively, ultimately leading to greater ROI through enhanced operational performance and improved patient care outcomes. The integration of AI in health information management and proactive healthcare management further enhances our approach to delivering value in the healthcare sector.
5.2. Data Analytics and Insights
Data analytics and insights play a crucial role in transforming raw data into actionable information. Organizations leverage data analytics to enhance decision-making processes, improve operational efficiency, and drive strategic initiatives. The integration of advanced technologies in data analytics allows for deeper insights and more informed decisions.
5.2.1. Advanced Predictive Analytics for Informed Decision Making
Predictive analytics involves using statistical algorithms and machine learning techniques to identify the likelihood of future outcomes based on historical data. This approach is increasingly being adopted across various sectors, including healthcare, finance, and marketing. Organizations utilize predictive analytics tools and predictive analytics solutions to enhance their capabilities.
- Enhances decision-making: Provides data-driven insights that help organizations anticipate trends and make informed choices, while also reducing uncertainty by quantifying risks and opportunities.
- Key components: Â
- Data collection: Gathering relevant historical data from various sources.
- Data processing: Cleaning and organizing data to ensure accuracy.
- Model building: Developing predictive models using algorithms to analyze data patterns.
- Validation: Testing models to ensure reliability and accuracy.
- Applications in various industries: Â
- Healthcare: Predicting patient outcomes, readmission rates, and disease outbreaks using predictive analytics tools for healthcare.
- Retail: Forecasting sales trends and inventory needs with predictive retail analytics.
- Finance: Assessing credit risk and detecting fraudulent activities through predictive sales analytics.
- Benefits: Â
- Improved operational efficiency by optimizing resource allocation.
- Enhanced customer satisfaction through personalized services and products.
- Increased profitability by identifying new market opportunities.
Organizations that effectively implement predictive analytics can gain a competitive edge by making proactive decisions rather than reactive ones. Rapid Innovation specializes in developing tailored predictive analytics solutions that empower clients to harness their data effectively, leading to improved decision-making and a potential increase in ROI. The use of cloud-based predictive analytics and predictive analytics as a service further enhances these capabilities. For more information on the use cases, advantages, and development of predictive analytics, visit our site.
5.2.2. NLP for Data Extraction and Healthcare Documentation Automation
Natural Language Processing (NLP) is a branch of artificial intelligence that focuses on the interaction between computers and human language. In the context of data extraction and healthcare documentation, NLP can significantly streamline processes and improve accuracy.
- Key functionalities of NLP: Â
- Text analysis: Extracting relevant information from unstructured data sources such as clinical notes, research papers, and patient records.
- Sentiment analysis: Understanding patient sentiments and experiences through their feedback and reviews.
- Language translation: Facilitating communication in multilingual healthcare settings.
- Benefits in healthcare documentation: Â
- Automation of routine tasks: Reduces the burden on healthcare professionals by automating documentation processes.
- Improved accuracy: Minimizes human errors in data entry and documentation.
- Enhanced patient care: Allows healthcare providers to focus more on patient interaction rather than administrative tasks.
- Applications of NLP in healthcare: Â
- Clinical decision support: Analyzing patient data to provide recommendations for treatment.
- Research: Extracting insights from vast amounts of medical literature to support evidence-based practices.
- Patient engagement: Analyzing patient feedback to improve services and address concerns.
- Challenges: Â
- Data privacy: Ensuring compliance with regulations such as HIPAA when processing sensitive patient information.
- Language nuances: Addressing the complexities of human language, including slang and idioms, which can affect data interpretation.
NLP technologies can significantly enhance the efficiency of healthcare documentation processes. Rapid Innovation offers advanced NLP solutions that can automate data extraction and documentation, leading to improved patient outcomes and streamlined operations. Studies indicate that implementing NLP can reduce documentation time by up to 50%. By leveraging these technologies, healthcare providers can focus on delivering quality care while maximizing operational efficiency. The integration of predictive analytics dynamics 365 and other predictive analytics platforms can further support these efforts.
6. Pharmaceutical and Research Applications
The pharmaceutical industry is increasingly leveraging advanced technologies to enhance research and development processes. Artificial Intelligence (AI) plays a pivotal role in transforming how drugs are discovered, developed, and brought to market.
6.1. AI in Drug Discovery and Development
Drug discovery and development is a complex, time-consuming, and costly process. Traditional methods can take over a decade and cost billions of dollars. AI is revolutionizing this landscape by streamlining various stages of drug development, from initial discovery to clinical trials. AI algorithms can analyze vast datasets to identify potential drug candidates, while machine learning models can predict how different compounds will interact with biological targets. Additionally, AI can optimize the design of clinical trials, improving patient selection and trial efficiency.
6.1.1. AI in Accelerating Drug Discovery Processes
AI technologies are significantly accelerating the drug discovery process, making it more efficient and cost-effective. Here are some key ways AI is contributing:
- Data Analysis: AI can process and analyze large volumes of biological and chemical data much faster than traditional methods. This capability allows researchers to identify patterns and correlations that may not be immediately apparent.
- Predictive Modeling: Machine learning algorithms can predict the efficacy and safety of drug candidates by simulating their interactions with biological systems. This predictive capability helps in prioritizing compounds for further testing.
- Target Identification: AI can assist in identifying new drug targets by analyzing genomic and proteomic data, leading to the discovery of novel therapeutic pathways.
- Virtual Screening: AI enables virtual screening of compound libraries, allowing researchers to evaluate thousands of compounds quickly. This reduces the time and resources spent on synthesizing and testing compounds in the lab.
- Optimization of Lead Compounds: Once potential drug candidates are identified, AI can help optimize their chemical structures to enhance their efficacy and reduce side effects. This process, known as lead optimization, is crucial for developing successful drugs.
- Clinical Trial Design: AI can improve the design of clinical trials by identifying suitable patient populations and predicting outcomes, leading to more successful trials and faster approvals.
- Real-World Data Utilization: AI can analyze real-world data from electronic health records and other sources to gain insights into drug performance in diverse populations. This information can inform drug development and post-marketing surveillance.
- Cost Reduction: By streamlining the drug discovery process, AI can significantly reduce the costs associated with bringing a new drug to market. Estimates suggest that AI could save the pharmaceutical industry billions of dollars annually.
- Case Studies: Several pharmaceutical companies have successfully integrated AI into their drug discovery processes. For example, Atomwise uses AI to predict how different molecules will interact, leading to the discovery of potential treatments for diseases like Ebola and multiple sclerosis. Other companies, such as insitro and DeepMind, are also making strides in AI-driven drug discovery.
At Rapid Innovation, we harness the power of AI to help pharmaceutical companies navigate these complexities of AI in drug development, ensuring they achieve greater ROI through enhanced efficiency and reduced time-to-market. Our expertise in AI-driven solutions positions us as a valuable partner in the pharmaceutical sector, enabling clients to leverage cutting-edge technology for transformative results.
AI's integration into drug discovery and development is not just a trend; it represents a fundamental shift in how new therapies are developed. As technology continues to evolve, the potential for AI to enhance pharmaceutical research will only grow, leading to faster, safer, and more effective drug development. The rise of AI in drug discovery, including applications like generative AI drug discovery and AI-powered drug discovery, is paving the way for innovative solutions in the pharmaceutical landscape.
6.1.2. Clinical Research and Experimentation with AI
Artificial Intelligence (AI) is transforming clinical research and experimentation by enhancing efficiency, accuracy, and speed in various processes. AI algorithms can analyze vast amounts of clinical data quickly, identifying patterns and correlations that may not be apparent to human researchers. This capability accelerates the discovery of potential drug candidates and treatment protocols. Additionally, AI tools can streamline the patient recruitment process by analyzing electronic health records (EHRs) to identify suitable candidates for clinical trials, thereby reducing the time and cost associated with finding participants. The use of AI in clinical trials is becoming increasingly prevalent, with companies focusing on AI clinical trials to improve outcomes.
Moreover, AI can predict patient responses to treatments based on historical data, helping researchers design more effective trials and leading to more personalized medicine approaches. AI systems can also monitor patient adherence to trial protocols in real-time, ensuring compliance and improving data integrity, which can result in more reliable outcomes and faster regulatory approvals. Furthermore, Natural Language Processing (NLP) can be utilized to analyze unstructured data from clinical notes, publications, and other sources, extracting valuable insights that can inform research directions. The role of artificial intelligence in clinical trials is crucial for enhancing the overall research process.
At Rapid Innovation, we leverage our expertise in AI to provide tailored solutions that enhance clinical research processes. By implementing advanced AI algorithms, we help clients reduce operational costs and improve the speed of drug development, ultimately leading to a greater return on investment (ROI). Our focus on artificial intelligence for clinical trial design ensures that our clients are at the forefront of innovation. For more information on the benefits and implementation strategies of clinical decision support software, visit our site.
6.2. AI in Biopharma Manufacturing
The biopharmaceutical manufacturing sector is increasingly adopting AI technologies to enhance production processes, improve quality control, and reduce costs. AI can optimize manufacturing processes by analyzing data from production lines to identify inefficiencies and suggest improvements, leading to increased yield and reduced waste. AI systems can monitor production in real-time, using machine learning algorithms to detect anomalies and ensure product quality, which minimizes the risk of defects and recalls.
Additionally, AI can enhance supply chain efficiency by predicting demand, optimizing inventory levels, and improving logistics, ensuring that raw materials are available when needed and reducing downtime. AI can also predict equipment failures before they occur, allowing for timely maintenance and reducing unplanned downtime, which increases overall operational efficiency. Furthermore, AI can assist in maintaining compliance with regulatory standards by automating documentation and reporting processes, ensuring that all necessary records are accurate and up-to-date.
6.2.1. AI in Biopharma Production and Manufacturing
AI is playing a crucial role in revolutionizing biopharma production and manufacturing, leading to more efficient and cost-effective processes. AI-driven automation can handle repetitive tasks in biopharma manufacturing, such as mixing, filling, and packaging, which reduces human error and increases throughput. AI systems can continuously monitor production parameters, such as temperature and pressure, ensuring that they remain within optimal ranges, which helps maintain product quality.
Moreover, AI can assist in the formulation development process by predicting how different ingredients will interact, leading to more effective and stable drug formulations. AI technologies can facilitate the scaling up of production processes from laboratory to commercial scale, ensuring that the transition is smooth and efficient. By optimizing processes and reducing waste, AI can significantly lower production costs, making biopharmaceuticals more accessible to patients and healthcare systems.
At Rapid Innovation, we are committed to helping biopharma clients harness the power of AI to streamline their manufacturing processes. Our solutions not only enhance operational efficiency but also contribute to significant cost savings, ultimately driving higher ROI.
In conclusion, the integration of AI in clinical research and biopharma manufacturing is paving the way for more efficient, accurate, and cost-effective healthcare solutions. The ongoing advancements in AI technology promise to further enhance these sectors, ultimately benefiting patients and healthcare providers alike. Rapid Innovation stands ready to partner with organizations in these fields to unlock the full potential of AI and achieve their business goals effectively. The use of artificial intelligence in clinical trials and the role of machine learning in clinical research are just a few examples of how AI is reshaping the landscape of healthcare.
6.2.2. Quality Control and Predictive Maintenance in Biopharma
Quality control (QC) and predictive maintenance are critical components in the biopharmaceutical industry, ensuring that products meet stringent safety and efficacy standards.
- Quality Control: Â
- Involves systematic processes to ensure that biopharmaceutical products are manufactured to the required specifications.
- Utilizes various testing methods, including:
- Analytical testing for potency and purity.
- Microbial testing to ensure sterility.
- Stability testing to assess product shelf life.
- Regulatory compliance is paramount, with organizations like the FDA and EMA setting guidelines that must be followed.
- Advanced technologies, such as real-time monitoring and data analytics, enhance biopharma quality control processes by providing immediate feedback on production quality.
- Predictive Maintenance: Â
- Focuses on anticipating equipment failures before they occur, minimizing downtime and ensuring continuous production.
- Employs data analytics and machine learning algorithms to analyze historical data and predict when maintenance should be performed.
- Benefits include:
- Reduced operational costs by preventing unexpected breakdowns.
- Increased equipment lifespan through timely maintenance.
- Enhanced production efficiency, leading to better product availability.
- The integration of IoT devices allows for real-time monitoring of equipment health, providing valuable insights for maintenance planning.
At Rapid Innovation, we leverage our expertise in AI and blockchain technologies to enhance biopharma quality control and predictive maintenance processes in the biopharmaceutical sector. By implementing advanced data analytics and machine learning solutions, we help clients achieve greater ROI through improved operational efficiency and compliance with regulatory standards. Our tailored solutions ensure that biopharmaceutical companies can maintain high-quality production while minimizing costs and maximizing equipment lifespan.
7.1.1. Infrastructure Needs for AI in Healthcare
- Robust computing power is essential for processing large datasets and running complex algorithms. Rapid Innovation can assist healthcare organizations in selecting and implementing high-performance computing solutions tailored to their specific needs, ensuring efficient data processing and analysis.
- Cloud computing solutions provide scalable resources, enabling healthcare organizations to manage fluctuating workloads. Our expertise in cloud architecture allows us to design and deploy cloud-based systems that enhance flexibility and reduce operational costs.
- High-speed internet connectivity is crucial for real-time data access and communication between devices. We can help organizations assess their connectivity needs and implement solutions that ensure seamless data flow across all platforms.
- Data storage solutions must be secure and capable of handling vast amounts of patient data, including images and genomic information. Rapid Innovation specializes in developing secure data storage architectures that comply with industry standards, ensuring patient data is both accessible and protected.
- Interoperability between different systems is necessary to ensure seamless data exchange and integration. Our team can facilitate the integration of disparate systems, enabling healthcare providers to share information efficiently and improve patient outcomes.
- Investment in AI-specific hardware, such as GPUs, can significantly enhance processing capabilities. We provide consulting services to help organizations identify the right hardware investments that align with their AI initiatives, maximizing their return on investment.
- Organizations need to establish a dedicated IT team with expertise in AI technologies to maintain and optimize infrastructure. Rapid Innovation offers training and support to help organizations build and sustain a skilled IT workforce capable of managing AI systems effectively.
- Regular updates and maintenance of software and hardware are vital to keep up with technological advancements. We provide ongoing support and maintenance services to ensure that healthcare organizations remain at the forefront of AI technology.
7.1.2. Data Privacy, Security, and Compliance with Regulations
- Healthcare organizations must comply with regulations such as HIPAA in the U.S. to protect patient information. Rapid Innovation can guide organizations through the compliance landscape, ensuring that all AI implementations adhere to necessary regulations.
- Implementing strong encryption methods is essential for safeguarding sensitive data during transmission and storage. Our team can design and implement robust encryption strategies that protect patient data from unauthorized access.
- Access controls should be established to ensure that only authorized personnel can access patient data. We assist organizations in developing comprehensive access control policies that enhance data security.
- Regular audits and assessments can help identify vulnerabilities and ensure compliance with data protection regulations. Rapid Innovation offers audit services to evaluate existing systems and recommend improvements to enhance security and compliance.
- Organizations should adopt a data minimization approach, collecting only the necessary information for AI applications. We help clients develop data governance frameworks that prioritize data minimization while still enabling effective AI utilization.
- Training staff on data privacy and security best practices is crucial to prevent human errors that could lead to breaches. Our training programs equip healthcare professionals with the knowledge needed to safeguard patient information.
- Utilizing anonymization techniques can help protect patient identities while still allowing for valuable data analysis. We provide expertise in data anonymization methods that comply with legal standards while enabling meaningful insights.
- Collaborating with legal experts can ensure that AI implementations adhere to all relevant laws and regulations. Rapid Innovation can connect organizations with legal professionals who specialize in healthcare compliance, ensuring that all AI initiatives are legally sound.
7.1.3. Integrating AI into Existing Healthcare Systems
- A clear strategy is needed to align AI initiatives with organizational goals and patient care objectives. Rapid Innovation works with clients to develop tailored AI strategies that support their specific business objectives.
- Stakeholder engagement is critical; involving clinicians, IT staff, and administrators can facilitate smoother integration. We facilitate workshops and discussions to ensure all stakeholders are aligned and engaged in the AI integration process.
- Conducting a thorough assessment of existing systems helps identify compatibility issues and integration challenges. Our team conducts comprehensive system assessments to pinpoint potential integration hurdles and recommend solutions.
- Pilot programs can be implemented to test AI solutions on a smaller scale before full deployment. We assist organizations in designing and executing pilot programs that allow for controlled testing of AI technologies.
- Training healthcare professionals on how to use AI tools effectively is essential for maximizing their benefits. Rapid Innovation offers customized training sessions to ensure that healthcare staff are proficient in utilizing AI tools.
- Continuous monitoring and evaluation of AI systems can help identify areas for improvement and ensure they meet clinical needs. We provide ongoing monitoring services to assess AI performance and recommend enhancements.
- Establishing feedback loops allows for ongoing adjustments based on user experiences and outcomes. Our approach includes creating feedback mechanisms that facilitate continuous improvement of AI systems.
- Collaboration with technology vendors can provide insights and support for successful integration efforts. Rapid Innovation leverages its network of technology partners to ensure that clients receive the best solutions for their AI integration needs.
- Â
- 7.1.4. Performance Monitoring and Continuous Optimization
Performance monitoring and continuous optimization are critical components in ensuring the success of any AI implementation. This process involves regularly assessing the performance of AI systems and making necessary adjustments to improve outcomes.
- Establish Key Performance Indicators (KPIs): Â
- Define specific metrics to evaluate AI performance.
- Common KPIs include accuracy, speed, and user satisfaction.
- Implement Real-Time Monitoring: Â
- Use dashboards to track AI performance continuously.
- Identify anomalies or performance dips promptly.
- Conduct Regular Audits: Â
- Schedule periodic reviews of AI systems to ensure compliance and effectiveness.
- Assess whether the AI is meeting the established KPIs.
- Gather User Feedback: Â
- Collect insights from end-users to understand their experiences.
- Use surveys and interviews to gather qualitative data.
- Optimize Algorithms: Â
- Regularly update and refine algorithms based on performance data.
- Incorporate new data to enhance learning and adaptability.
- Foster a Culture of Continuous Improvement: Â
- Encourage teams to seek innovative solutions and improvements.
- Promote knowledge sharing and collaboration among departments.
- Leverage Advanced Analytics: Â
- Utilize data analytics tools to gain deeper insights into performance.
- Identify trends and patterns that can inform optimization strategies.
7.2. Organizational Readiness for AI Adoption
Organizational readiness is essential for successful AI adoption. It encompasses the culture, infrastructure, and processes that support the integration of AI technologies.
- Assess Current Capabilities: Â
- Evaluate existing technology infrastructure and data management practices.
- Identify gaps in skills and resources that need to be addressed.
- Foster a Supportive Culture: Â
- Create an environment that embraces innovation and change.
- Encourage open communication about AI initiatives and their benefits.
- Develop a Strategic Vision: Â
- Outline clear goals for AI adoption aligned with organizational objectives.
- Ensure leadership commitment to drive the AI agenda.
- Invest in Training and Development: Â
- Provide training programs to upskill employees on AI technologies.
- Promote cross-functional teams to enhance collaboration.
- Establish Governance Frameworks: Â
- Create policies and guidelines for AI usage and data management.
- Ensure compliance with ethical standards and regulations.
- Engage Stakeholders: Â
- Involve key stakeholders in the planning and implementation process.
- Address concerns and expectations to build trust and support.
- Monitor Progress and Adapt: Â
- Regularly review the AI adoption process and make adjustments as needed.
- Use feedback to refine strategies and improve readiness.
7.2.1. Change Management for AI Implementation in Healthcare
Change management is crucial for the successful implementation of AI in healthcare settings. It involves preparing, supporting, and helping individuals and teams to adopt new technologies effectively.
- Communicate the Vision: Â
- Clearly articulate the purpose and benefits of AI in healthcare.
- Use multiple channels to reach all stakeholders, including staff and patients.
- Involve Key Stakeholders Early: Â
- Engage healthcare professionals in the planning process.
- Gather input from clinicians, administrators, and IT staff to ensure buy-in.
- Provide Comprehensive Training: Â
- Offer training sessions tailored to different user groups.
- Include hands-on practice with AI tools to build confidence.
- Address Resistance to Change: Â
- Identify potential sources of resistance and address concerns proactively.
- Use change champions to advocate for AI adoption among peers.
- Create a Support System: Â
- Establish a helpdesk or support team to assist users during the transition.
- Provide resources such as FAQs and user manuals.
- Monitor and Evaluate: Â
- Track the implementation process and gather feedback from users.
- Adjust strategies based on user experiences and outcomes.
- Celebrate Successes: Â
- Recognize and reward teams and individuals who contribute to successful AI adoption.
- Share success stories to motivate others and reinforce the benefits of change.
At Rapid Innovation, we understand that effective performance monitoring and continuous optimization are essential for maximizing the return on investment (ROI) from AI initiatives, including ai performance monitoring. By implementing robust performance metrics and fostering a culture of continuous improvement, we help our clients achieve their business goals efficiently and effectively. Our expertise in AI and blockchain technologies enables us to provide tailored solutions that drive innovation and enhance operational efficiency, ultimately leading to greater ROI for our clients.
7.2.2. Staff Training and Development for AI Proficiency
- Importance of Training
As AI technologies evolve, continuous training is essential for staff to stay updated. Proficient staff can leverage AI tools effectively, leading to improved productivity and innovation. At Rapid Innovation, we emphasize the importance of equipping your team with the necessary skills to harness AI's full potential, ultimately driving your business towards greater efficiency and success. This includes comprehensive ai staff training to ensure that all employees are well-prepared for the integration of AI into their workflows. - Types of Training Programs  Â
- Technical Training: Focuses on the technical aspects of AI, including programming, data analysis, and machine learning. Our tailored programs ensure that your team is well-versed in the latest AI technologies, enabling them to implement solutions that align with your business goals. Â
- Soft Skills Development: Enhances skills such as critical thinking, problem-solving, and communication, which are crucial for working alongside AI systems. We believe that a well-rounded skill set is vital for maximizing the benefits of AI integration. Â
- Hands-on Workshops: Practical sessions where employees can experiment with AI tools and applications. These workshops foster a culture of innovation and experimentation, allowing your team to explore new possibilities.
- Training Delivery Methods  Â
- Online Courses: Flexible learning options that allow staff to learn at their own pace. This approach ensures that your team can balance their training with their daily responsibilities. Â
- In-person Workshops: Facilitated sessions that encourage collaboration and direct interaction with experts. These workshops provide an opportunity for your team to engage deeply with the material and ask questions in real-time. Â
- Mentorship Programs: Pairing less experienced staff with AI specialists to foster knowledge transfer. This hands-on approach accelerates learning and builds a strong foundation for future AI initiatives.
- Measuring Training Effectiveness  Â
- Pre- and post-training assessments to evaluate knowledge gains. This allows us to quantify the impact of our training programs on your team's capabilities. Â
- Feedback surveys to gather participant insights on training relevance and applicability. We value your team's input to continuously improve our offerings. Â
- Tracking performance improvements in AI-related tasks post-training. By monitoring these metrics, we can demonstrate the tangible benefits of our training initiatives.
7.2.3. ROI Assessment and Tracking AI Impact
- Understanding ROI in AI
ROI (Return on Investment) measures the financial return generated from AI initiatives compared to the costs incurred. It helps organizations justify AI investments and make informed decisions about future projects. At Rapid Innovation, we assist clients in establishing clear ROI metrics to ensure that their AI investments yield significant returns. - Key Metrics for Assessment  Â
- Cost Savings: Reduction in operational costs due to automation and efficiency improvements. Our AI solutions are designed to streamline processes, leading to substantial cost reductions. Â
- Revenue Growth: Increased sales or market share attributed to AI-driven insights and innovations. We help clients leverage AI to uncover new market opportunities and enhance customer engagement. Â
- Productivity Gains: Enhanced employee output and reduced time spent on repetitive tasks. Our AI implementations are focused on augmenting human capabilities, allowing your team to focus on higher-value activities.
- Tracking AI Impact  Â
- Establishing Baselines: Measure performance metrics before AI implementation to compare against post-implementation results. This baseline assessment is crucial for understanding the true impact of AI initiatives. Â
- Continuous Monitoring: Regularly assess AI systems' performance and their impact on business objectives. We provide ongoing support to ensure that your AI solutions remain aligned with your evolving business needs. Â
- Reporting: Create comprehensive reports that detail findings, insights, and recommendations for stakeholders. Our transparent reporting process keeps all stakeholders informed and engaged.
- Tools for ROI Assessment  Â
- Analytics Software: Utilize tools that can analyze data and provide insights into AI performance. We recommend the best tools tailored to your specific needs, ensuring you have the right resources at your disposal. Â
- Dashboards: Visual representations of key metrics that allow for quick assessments of AI impact. Our custom dashboards provide real-time insights into your AI initiatives. Â
- Surveys and Feedback: Collect qualitative data from employees and customers to gauge satisfaction and effectiveness. This feedback loop is essential for continuous improvement.
8. Regulatory and Ethical Framework
- Importance of a Regulatory Framework
Establishes guidelines for the responsible use of AI technologies and ensures compliance with laws and regulations, reducing the risk of legal issues. Rapid Innovation is committed to helping clients navigate the complex regulatory landscape surrounding AI. - Key Components of the Framework  Â
- Data Privacy: Regulations that protect personal data and ensure ethical data usage. We assist clients in implementing robust data governance practices to safeguard sensitive information. Â
- Transparency: Requirements for organizations to disclose how AI systems make decisions. Our solutions prioritize transparency, enabling clients to build trust with their stakeholders. Â
- Accountability: Mechanisms to hold organizations responsible for AI outcomes and impacts. We guide clients in establishing accountability frameworks to ensure responsible AI usage.
- Ethical Considerations  Â
- Bias Mitigation: Strategies to identify and reduce biases in AI algorithms to ensure fair treatment of all individuals. Our team works with clients to develop ethical AI models that promote fairness and inclusivity. Â
- Human Oversight: Ensuring that human judgment remains integral in critical decision-making processes involving AI. We advocate for a balanced approach that combines AI efficiency with human insight. Â
- Social Impact: Assessing the broader implications of AI technologies on society, including job displacement and inequality. We help clients understand and address the social ramifications of their AI initiatives.
- Global Regulatory Landscape
Different countries have varying regulations regarding AI, necessitating organizations to stay informed about local laws. Rapid Innovation provides clients with the knowledge and resources needed to remain compliant in a dynamic regulatory environment. International collaborations may emerge to create unified standards for AI ethics and regulation. - Best Practices for Compliance  Â
- Regular Audits: Conduct audits to ensure adherence to regulatory requirements and ethical standards. We assist clients in establishing audit processes that promote accountability. Â
- Training on Compliance: Educate staff about relevant laws and ethical considerations in AI usage. Our training programs include compliance education to empower your team. Â
- Stakeholder Engagement: Involve diverse stakeholders in discussions about AI policies to ensure a comprehensive approach. We facilitate stakeholder engagement to foster collaboration and shared understanding.
8.1. Ethical Implications of AI in Healthcare
The integration of artificial intelligence (AI) in healthcare brings numerous benefits, such as enhanced diagnostic accuracy and treatment personalization. However, it also raises significant ethical concerns regarding patient privacy, consent, and data security. Additionally, the potential for bias in AI algorithms can lead to unequal treatment outcomes. These implications must be carefully considered to ensure that AI technologies are used responsibly and equitably, particularly in the context of the ethics of AI in healthcare.
8.1.1. Addressing Ethical Concerns and Biases in AI Systems
Bias in AI systems can stem from various sources, including data selection, algorithm design, and human oversight. Addressing these biases is crucial for equitable healthcare delivery, especially when considering the ethical issues with AI in healthcare.
Data Quality: AI systems rely on large datasets for training. If these datasets are not representative of diverse populations, the AI may produce biased results, raising ethical implications of AI in healthcare.
Algorithmic Bias: Algorithms can inadvertently favor certain demographics over others, leading to disparities in care. For example, a study found that some AI systems were less accurate in diagnosing conditions in minority populations, highlighting the ethical concerns of AI in healthcare.
Human Oversight: Continuous monitoring and evaluation of AI systems are necessary to identify and mitigate biases. Healthcare professionals must be involved in the development and deployment of AI tools to ensure ethical standards are upheld, addressing the ethical issues of artificial intelligence in healthcare.
Strategies to address these concerns include:
- Implementing diverse datasets that reflect the population's demographics.
- Regularly auditing AI systems for bias and performance across different groups.
- Engaging stakeholders, including patients and advocacy groups, in the development process to ensure diverse perspectives are considered, which is essential for ethical machine learning in health care.
8.1.2. The Role of Transparency and Explainability in Healthcare AI
Transparency and explainability are critical components in building trust in AI systems used in healthcare. Patients and healthcare providers need to understand how AI systems make decisions, which is a key aspect of AI ethics in healthcare.
Transparency: This involves clear communication about how AI algorithms work, including the data used and the decision-making processes. Transparency helps demystify AI and fosters trust among users, addressing ethical concerns of AI in healthcare.
Explainability: AI systems should provide understandable explanations for their recommendations or decisions. This is particularly important in healthcare, where decisions can significantly impact patient outcomes, emphasizing the need for ethical issues of artificial intelligence in healthcare.
Key aspects of transparency and explainability include:
- Providing clear documentation of AI algorithms and their intended use.
- Developing user-friendly interfaces that allow healthcare providers to interact with AI systems easily.
- Offering training for healthcare professionals on interpreting AI outputs and integrating them into clinical decision-making, which is vital for addressing ethical issues in AI in healthcare.
By prioritizing transparency and explainability, healthcare organizations can enhance the ethical use of AI, ensuring that it serves as a valuable tool rather than a source of confusion or mistrust. At Rapid Innovation, we are committed to helping healthcare organizations navigate these ethical challenges, including the ethical and legal challenges of artificial intelligence-driven healthcare, by providing tailored AI solutions that prioritize data integrity, transparency, and stakeholder engagement, ultimately driving greater ROI and improved patient outcomes.
8.2. Data Privacy and Patient Rights
In the realm of healthcare, data privacy and patient rights are paramount, especially with the increasing integration of artificial intelligence (AI) in medical applications. Ensuring that patient data is handled responsibly and ethically is crucial for maintaining trust and compliance with regulations, particularly in light of healthcare data privacy laws and the importance of data privacy in healthcare.
8.2.1. Ensuring Data Privacy and Security in AI Applications
The use of AI in healthcare brings significant benefits, but it also raises concerns about data privacy and security. Key considerations include:
- Data Encryption: Implementing strong encryption methods to protect patient data both at rest and in transit, which helps prevent unauthorized access and data breaches.
- Access Controls: Establishing strict access controls to ensure that only authorized personnel can access sensitive patient information. Role-based access can limit exposure to data based on job responsibilities.
- Anonymization Techniques: Utilizing data anonymization and pseudonymization techniques to protect patient identities while still allowing for valuable data analysis, which can help in research without compromising individual privacy.
- Regular Audits: Conducting regular security audits and assessments to identify vulnerabilities in AI systems. This proactive approach can help mitigate risks before they lead to data breaches.
- Compliance with Regulations: Adhering to regulations such as the Health Insurance Portability and Accountability Act (HIPAA) in the U.S. and the General Data Protection Regulation (GDPR) in Europe, which set standards for data protection and patient privacy, including the data protection act healthcare.
- Incident Response Plans: Developing and maintaining incident response plans to address potential data breaches swiftly, including notifying affected patients and regulatory bodies as required by law.
- Transparency: Providing clear information to patients about how their data will be used, stored, and protected. Transparency fosters trust and encourages patients to engage with AI-driven healthcare solutions, which is essential for protecting patient health information.
8.2.2. Obtaining Patient Consent for AI-Driven Healthcare
Obtaining informed consent from patients is a critical aspect of integrating AI into healthcare. This process ensures that patients understand how their data will be used and the implications of AI technologies. Important elements include:
- Clear Communication: Providing patients with clear, concise information about AI applications, including their purpose, benefits, and potential risks, which helps patients make informed decisions.
- Comprehensive Consent Forms: Designing consent forms that cover all aspects of data usage, including data sharing with third parties, data retention policies, and the use of AI for diagnosis or treatment recommendations, particularly in relation to selling medical data to third parties.
- Ongoing Consent: Recognizing that consent is not a one-time event. Patients should be informed about any changes in data usage or AI applications, allowing them to withdraw consent if they choose.
- Education and Awareness: Offering educational resources to help patients understand AI technologies and their implications for healthcare, which can include workshops, informational brochures, or online resources.
- Cultural Sensitivity: Being aware of cultural differences in attitudes toward data privacy and consent. Tailoring consent processes to respect these differences can enhance patient engagement and trust.
- Feedback Mechanisms: Implementing feedback mechanisms that allow patients to express concerns or ask questions about AI applications, helping healthcare providers address issues and improve the consent process.
- Legal Considerations: Ensuring that consent processes comply with local laws and regulations regarding patient rights and data protection, including understanding the legal implications of using AI in healthcare settings and the importance of medical data privacy laws.
By prioritizing data privacy and patient rights, healthcare providers can harness the power of AI while maintaining the trust and confidence of their patients. At Rapid Innovation, we specialize in developing AI solutions that not only enhance operational efficiency but also ensure compliance with data privacy regulations, ultimately leading to greater ROI for healthcare organizations. Our expertise in AI and blockchain technology allows us to implement robust security measures and transparent data handling practices, ensuring that patient rights are respected while leveraging the full potential of AI in healthcare, including the critical aspects of electronic health records privacy and the general data protection regulation healthcare.
8.3. Compliance with Healthcare Regulations
Compliance with healthcare regulations is crucial for the successful integration of artificial intelligence (AI) healthcare compliance in the healthcare sector. Regulatory bodies ensure that AI technologies meet safety, efficacy, and ethical standards before they can be deployed in clinical settings. This compliance not only protects patients but also fosters trust in AI solutions among healthcare providers and stakeholders.
8.3.1. Regulatory Requirements for AI in Healthcare
AI technologies in healthcare must adhere to various regulatory requirements to ensure they are safe and effective. Key aspects include:
- FDA Approval: In the United States, the Food and Drug Administration (FDA) regulates medical devices, including AI-based solutions. AI systems intended for diagnostic or therapeutic purposes may require premarket approval or clearance.
- Data Privacy Regulations: Compliance with laws such as the Health Insurance Portability and Accountability Act (HIPAA) is essential. These regulations govern the handling of patient data, ensuring that AI systems protect sensitive information.
- International Standards: Different countries have their own regulatory frameworks. For instance, the European Union has the Medical Device Regulation (MDR) and In Vitro Diagnostic Regulation (IVDR) that apply to AI technologies in healthcare.
- Transparency and Explainability: Regulatory bodies are increasingly emphasizing the need for AI systems to be transparent. This includes providing clear explanations of how AI algorithms make decisions, which is vital for clinical acceptance.
- Post-Market Surveillance: After deployment, AI systems must undergo continuous monitoring to ensure they remain compliant with safety and efficacy standards. This includes reporting adverse events and updating algorithms as necessary.
8.3.2. Clinical Validation and Safety Standards for AI Solutions
Clinical validation is a critical step in ensuring that AI solutions are safe and effective for patient care. This process involves rigorous testing and adherence to safety standards, which include:
- Robust Testing Protocols: AI systems must undergo extensive testing in clinical settings to validate their performance. This includes randomized controlled trials (RCTs) to compare AI solutions against standard care practices.
- Performance Metrics: Key performance indicators (KPIs) such as sensitivity, specificity, and accuracy must be established and met. These metrics help determine the reliability of AI systems in diagnosing or treating conditions.
- Risk Assessment: A thorough risk assessment is necessary to identify potential hazards associated with AI use. This includes evaluating the consequences of false positives or negatives in clinical decision-making.
- User Training and Guidelines: Healthcare professionals must be adequately trained to use AI tools. Clear guidelines should be provided to ensure that AI solutions are integrated into clinical workflows effectively.
- Ethical Considerations: Ethical standards must be upheld throughout the development and deployment of AI in healthcare. This includes ensuring that AI does not perpetuate biases or inequalities in patient care.
- Regulatory Compliance Documentation: Developers must maintain comprehensive documentation demonstrating compliance with regulatory requirements. This includes records of clinical trials, risk assessments, and post-market surveillance activities.
At Rapid Innovation, we understand the complexities of navigating these regulatory landscapes. Our expertise in AI healthcare compliance and blockchain technology allows us to assist healthcare organizations in achieving compliance efficiently. By leveraging our development and consulting solutions, clients can ensure that their AI systems not only meet regulatory standards but also enhance patient care and operational efficiency, ultimately leading to greater ROI.
9. The Future of AI in Healthcare: Trends and Innovations
The integration of artificial intelligence (AI) in healthcare is rapidly evolving, with numerous trends and innovations shaping the future of medical practices. AI is poised to enhance patient care, streamline operations, and improve health outcomes.
9.1. Emerging Technologies and Innovations in AI for Healthcare
AI technologies are continuously advancing, leading to innovative applications in healthcare. Some key areas of focus include:
- Predictive analytics for early disease detection
- Natural language processing for better patient communication
- Machine learning algorithms for personalized treatment plans
- Robotics for surgical assistance and rehabilitation
- AI-driven imaging analysis for more accurate diagnostics
These innovations are not only improving efficiency but also enhancing the quality of care provided to patients.
9.1.1. Multimodal AI for Combining Health, Behavioral, and Environmental Data
Multimodal AI refers to the integration of various data types—health, behavioral, and environmental—to create a comprehensive understanding of patient health. This approach offers several advantages:
- Holistic view of patient health: By combining diverse data sources, healthcare providers can gain insights into how different factors influence health outcomes.
- Improved predictive capabilities: Multimodal AI can identify patterns and correlations that single-modality data might miss, leading to better predictions of disease risk and progression.
- Enhanced personalized care: Tailoring treatment plans based on a broader range of data allows for more effective interventions that consider individual patient circumstances.
Key components of multimodal AI in healthcare include:
- Health data: Electronic health records (EHRs), lab results, and medical imaging provide essential clinical information.
- Behavioral data: Information from wearable devices, mobile health apps, and patient-reported outcomes can reveal lifestyle factors affecting health.
- Environmental data: Data on social determinants of health, such as socioeconomic status, living conditions, and access to healthcare, can inform treatment strategies.
Challenges in implementing multimodal AI include:
- Data integration: Combining data from various sources requires sophisticated algorithms and infrastructure.
- Privacy concerns: Ensuring patient data security and compliance with regulations is critical.
- Interoperability: Different healthcare systems must be able to communicate effectively to share data seamlessly.
Despite these challenges, the potential benefits of multimodal AI in healthcare are significant. By leveraging a comprehensive dataset, healthcare providers can:
- Identify at-risk populations more accurately
- Develop targeted prevention strategies
- Monitor treatment effectiveness in real-time
- Enhance patient engagement through personalized communication
At Rapid Innovation, we specialize in harnessing the power of AI in healthcare to help organizations navigate these complexities. Our expertise in developing tailored AI solutions enables clients to implement multimodal AI strategies effectively, ensuring they can achieve greater ROI through improved patient outcomes and operational efficiencies. As the field of artificial intelligence in healthcare continues to evolve, multimodal approaches will likely play a crucial role in shaping the future of healthcare, leading to improved patient outcomes and more efficient healthcare systems. The rise of healthcare AI companies and advancements in medical AI will further drive these innovations, making AI and healthcare an integral part of modern medicine.
9.1.2. Federated Learning for Personalized Patient Models
- Federated learning is a decentralized approach to machine learning that allows multiple institutions to collaborate on model training without sharing sensitive patient data. This method enhances patient privacy and data security, as the data remains on local devices or servers.
- Key benefits include: Â
- Improved personalization of healthcare models by utilizing diverse datasets from various institutions.
- Reduction in data transfer costs and compliance risks associated with data sharing.
- Ability to create models that are more representative of different populations, leading to better health outcomes.
- Applications in healthcare include: Â
- Predictive analytics for patient outcomes.
- Personalized treatment recommendations based on individual patient data.
- Enhanced diagnostic tools that learn from a broader range of cases without compromising privacy, including applications of ai in healthcare and artificial intelligence in medical diagnosis.
- Challenges include: Â
- Technical complexities in coordinating model updates across different sites.
- Ensuring model accuracy and consistency despite variations in local data.
- Addressing regulatory and ethical concerns related to data use and patient consent.
At Rapid Innovation, we leverage federated learning to help healthcare organizations enhance their AI capabilities while maintaining patient privacy. By implementing federated learning solutions, we enable our clients to develop personalized patient models that improve treatment outcomes and operational efficiency, ultimately leading to a greater return on investment (ROI).
9.2. Market Predictions and Growth Opportunities
- The healthcare AI market is projected to grow significantly, driven by advancements in technology and increasing demand for efficient healthcare solutions, particularly in areas like ai for health and artificial intelligence in health.
- Key growth factors include: Â
- Rising healthcare costs prompting the need for cost-effective solutions.
- Increased adoption of electronic health records (EHRs) and digital health technologies.
- Growing emphasis on personalized medicine and patient-centric care, including the integration of machine learning in healthcare.
- Market predictions suggest: Â
- The global AI in healthcare market could reach $45.2 billion by 2026, growing at a CAGR of 44.9% from 2021 to 2026.
- Significant investments are expected in AI-driven diagnostics, treatment planning, and operational efficiency, with a focus on medical ai and artificial intelligence in healthcare.
- Opportunities for innovation include: Â
- Development of AI tools for remote patient monitoring and telehealth services.
- Integration of AI with wearable devices for real-time health tracking.
- Expansion of AI applications in drug discovery and clinical trials, including generative ai in healthcare.
9.2.1. Investment Trends in AI-Driven Healthcare
- Investment in AI-driven healthcare has surged, reflecting confidence in the technology's potential to transform the industry.
- Key trends include: Â
- Increased venture capital funding for AI startups focused on healthcare solutions, particularly those specializing in ai medical imaging and medical artificial intelligence.
- Strategic partnerships between tech companies and healthcare providers to develop AI applications.
- Government initiatives and grants aimed at promoting AI research in healthcare.
- Notable statistics: Â
- In 2021, global investment in digital health reached $29.1 billion, with a significant portion directed towards AI technologies.
- The number of AI healthcare startups has grown exponentially, with over 2,000 companies operating in this space as of 2023, including healthcare ai companies and ai companies in healthcare.
- Areas attracting investment include: Â
- AI-powered diagnostic tools that enhance accuracy and speed, such as those used in medical coding ai and ai for medical diagnosis.
- Predictive analytics platforms that improve patient outcomes and operational efficiency.
- Natural language processing (NLP) applications for better data management and patient interaction.
- Challenges in investment include: Â
- Regulatory hurdles that can delay product development and market entry.
- The need for robust validation of AI models to ensure safety and efficacy.
- Competition among startups, making it challenging to secure funding and market share.
At Rapid Innovation, we are committed to guiding our clients through these investment trends and helping them capitalize on the growth opportunities in the AI-driven healthcare market. Our expertise in AI and blockchain technologies positions us to deliver innovative solutions that enhance operational efficiency and drive significant ROI for our clients.
9.2.2. Emerging Research Directions in AI Applications for Medicine
Artificial Intelligence (AI) is rapidly transforming the field of medicine, leading to innovative research directions that promise to enhance patient care, streamline operations, and improve health outcomes. Here are some key areas of focus:
- Predictive Analytics: AI algorithms are being developed to analyze vast amounts of patient data to predict health outcomes. These models can identify patients at risk for diseases such as diabetes or heart disease before symptoms appear. Predictive analytics can also optimize treatment plans by forecasting how patients will respond to specific therapies. At Rapid Innovation, we leverage predictive analytics to help healthcare providers make data-driven decisions, ultimately leading to improved patient outcomes and greater ROI.
- Personalized Medicine: AI is enabling the customization of medical treatments based on individual patient characteristics. Machine learning models can analyze genetic information, lifestyle factors, and environmental influences to tailor therapies. This approach aims to improve efficacy and reduce adverse effects by ensuring that treatments are suited to each patient's unique profile. Our expertise in AI allows us to assist clients in developing personalized medicine solutions that enhance patient satisfaction and treatment effectiveness.
- Medical Imaging: AI applications in medical imaging are advancing rapidly, with deep learning techniques enhancing image analysis. Algorithms can detect anomalies in X-rays, MRIs, and CT scans with high accuracy, often surpassing human radiologists. This technology can lead to earlier diagnosis of conditions such as cancer, improving patient outcomes. Rapid Innovation can help healthcare organizations implement AI-driven imaging solutions that increase diagnostic accuracy and operational efficiency.
- Natural Language Processing (NLP): NLP is being utilized to extract valuable insights from unstructured clinical data, such as physician notes and patient records. AI systems can analyze this data to identify trends, improve documentation, and enhance clinical decision-making. This research direction aims to streamline workflows and reduce the administrative burden on healthcare providers. Our NLP solutions can help clients unlock the potential of their data, leading to better patient care and reduced costs.
- Robotic Surgery: AI is playing a significant role in the development of robotic surgical systems that enhance precision and control. These systems can assist surgeons in performing complex procedures with minimal invasiveness, leading to quicker recovery times. Ongoing research focuses on improving the autonomy of robotic systems, allowing them to adapt to real-time surgical conditions. Rapid Innovation is at the forefront of developing AI technologies that support robotic surgery, enhancing surgical outcomes and patient safety.
- Telemedicine and Remote Monitoring: The integration of AI in telemedicine is facilitating remote patient monitoring and virtual consultations. AI algorithms can analyze data from wearable devices to monitor vital signs and detect potential health issues. This research direction is particularly relevant in managing chronic diseases and providing care in underserved areas. Our telemedicine solutions empower healthcare providers to deliver high-quality care remotely, improving access and patient engagement.
- Drug Discovery and Development: AI is revolutionizing the drug discovery process by predicting how different compounds will interact with biological targets. Machine learning models can analyze existing research and clinical trial data to identify promising drug candidates more efficiently. This approach can significantly reduce the time and cost associated with bringing new medications to market. Rapid Innovation's expertise in AI can accelerate drug development timelines, leading to faster market entry and increased ROI for pharmaceutical companies.
- Clinical Decision Support Systems (CDSS): AI-driven CDSS are being developed to assist healthcare professionals in making informed clinical decisions. These systems can provide evidence-based recommendations, flag potential drug interactions, and suggest diagnostic tests. Research is focused on improving the accuracy and reliability of these systems to enhance patient safety. Our CDSS solutions can help healthcare organizations improve clinical outcomes while optimizing resource utilization.
- Mental Health Applications: AI is being explored for its potential in mental health care, including chatbots and virtual therapists. These tools can provide immediate support and resources for individuals experiencing mental health issues. Ongoing research aims to validate the effectiveness of AI interventions in improving mental health outcomes. Rapid Innovation is committed to developing AI solutions that enhance mental health support, ensuring that individuals receive timely and effective care.
- Health Equity and Access: AI research is increasingly addressing health disparities by developing tools that improve access to care for marginalized populations. Algorithms can identify social determinants of health and tailor interventions to meet the needs of diverse communities. This direction aims to ensure that AI applications benefit all patients, regardless of their background. Our initiatives focus on creating equitable healthcare solutions that enhance access and improve health outcomes for all.
- Ethics and Regulation: As AI applications in medicine expand, research is focusing on ethical considerations and regulatory frameworks. Issues such as data privacy, algorithmic bias, and accountability are critical areas of investigation. Developing guidelines and standards will be essential to ensure the responsible use of AI in healthcare. Rapid Innovation prioritizes ethical AI development, ensuring that our solutions comply with regulatory standards and promote patient trust.
These emerging research directions highlight the transformative potential of AI in medicine, paving the way for innovations that can significantly improve patient care and health outcomes. As the field continues to evolve, collaboration among researchers, clinicians, and policymakers will be crucial to harnessing the full benefits of AI technologies. At Rapid Innovation, we are dedicated to partnering with healthcare organizations to drive these advancements and achieve greater ROI through our AI and blockchain solutions. This includes the use of artificial intelligence in medical diagnosis, applications of artificial intelligence in medicine, and the use of AI in the medical field, among other areas. Our commitment extends to exploring the applications of AI in healthcare, ensuring that we remain at the forefront of this transformative journey.
‍