Table Of Contents
Category
Artificial Intelligence
Retail & Ecommerce
Supply Chain & Logistics
Marketing
CRM
1. Introduction to AI-Driven Demand Forecasting
Demand forecasting is a critical aspect of business strategy that involves predicting future customer demand for products or services. With the rise of artificial intelligence (AI), traditional methods of demand forecasting are being transformed, leading to more accurate predictions and better decision-making.
1.1. What is Demand Forecasting? Why Does It Matter for Businesses?
- Demand forecasting is the process of estimating future customer demand based on historical data, market trends, and other relevant factors.
- It helps businesses: Â
- Optimize inventory levels to reduce holding costs and avoid stockouts.
- Improve production planning and scheduling to meet customer needs efficiently.
- Enhance financial planning by predicting revenue and cash flow.
- Make informed marketing and sales strategies based on anticipated demand.
- Accurate demand forecasting is crucial because: Â
- It minimizes waste and maximizes resource utilization.
- It supports better customer satisfaction by ensuring product availability.
- It aids in strategic decision-making, allowing businesses to respond proactively to market changes.
- According to a study, companies that implement effective demand forecasting can reduce inventory costs by up to 20%.
1.2. How AI is Revolutionizing Traditional Demand Forecasting
- AI is changing the landscape of demand forecasting by introducing advanced algorithms and machine learning techniques that enhance accuracy and efficiency. This includes the use of AI sales forecasting software and sales forecasting using AI.
- Key ways AI is revolutionizing demand forecasting include: Â
- Data Processing: AI can analyze vast amounts of data from various sources, including sales history, market trends, and social media, to identify patterns and correlations that traditional methods might miss. This is particularly relevant in AI demand forecasting and AI in demand forecasting.
- Predictive Analytics: Machine learning models can predict future demand by learning from historical data and continuously improving their accuracy over time. AI for forecasting and AI in forecasting are essential components of this process.
- Real-Time Insights: AI systems can provide real-time data analysis, allowing businesses to adjust their forecasts based on current market conditions and consumer behavior. This is a key advantage of AI sales forecasting and demand forecasting AI.
- Benefits of AI-driven demand forecasting: Â
- Increased accuracy in predictions, leading to better inventory management.
- Enhanced agility in responding to market fluctuations and consumer trends.
- Reduction in forecasting errors, which can lead to significant cost savings. AI for demand forecasting and AI-based demand forecasting are critical in achieving these benefits.
- A report indicates that businesses using AI for demand forecasting can achieve up to a 50% improvement in forecast accuracy.
At Rapid Innovation, we specialize in leveraging AI to help manufacturing businesses like yours achieve greater ROI through enhanced demand forecasting solutions. By partnering with us, you can expect improved operational efficiency, reduced costs, and a more strategic approach to meeting customer needs. Our expertise in AI-driven solutions ensures that you stay ahead of market trends and make informed decisions that drive growth, particularly through AI in demand planning and AI demand planning.
Refer to the image for a visual representation of AI-driven demand forecasting concepts:

1.3. The Role of AI in Modern Business Planning and Decision Making
AI has transformed how businesses approach planning and decision-making by enabling organizations to analyze vast amounts of data quickly and accurately. Key benefits include enhanced predictive analytics for better forecasting, improved efficiency in operations and resource allocation, and data-driven insights that support strategic initiatives. AI tools can identify patterns and trends that may not be visible to human analysts.
Businesses can leverage AI for market analysis to understand consumer behavior, risk assessment to mitigate potential challenges, and financial modeling to optimize budgets and investments. AI facilitates real-time decision-making, allowing companies to respond swiftly to market changes. Companies using AI in decision-making report increased agility and competitiveness.
Examples of AI applications in business planning include chatbots for customer service to gather feedback, machine learning algorithms for sales predictions, and natural language processing for sentiment analysis in marketing. Additionally, AI sales forecasting software and AI demand forecasting tools are becoming essential for accurate sales predictions and demand planning.
2. What is AI-Enabled Demand Forecasting? (Explained Simply)
AI-enabled demand forecasting uses artificial intelligence to predict future customer demand by combining historical data with advanced algorithms to generate accurate forecasts. Key components include data collection from various sources (sales, market trends, etc.), machine learning models that learn from data patterns, and continuous improvement through feedback loops.
The benefits of AI-enabled demand forecasting include increased accuracy in predictions, reducing stockouts and overstock situations, enhanced ability to respond to market fluctuations, and improved inventory management and supply chain efficiency. AI can analyze complex variables that affect demand, such as seasonal trends, economic indicators, and consumer preferences. Businesses can use these forecasts to make informed decisions about production, staffing, and marketing strategies, utilizing AI for forecasting to enhance their operational capabilities.
2.1. Definition of AI-Powered Demand Forecasting and Why It’s Crucial
AI-powered demand forecasting refers to the use of AI technologies to predict future demand for products or services. It is crucial for several reasons: it helps businesses align their supply with customer demand, minimizing waste; supports strategic planning by providing insights into future sales trends; and enhances customer satisfaction by ensuring product availability.
Key features of AI-powered demand forecasting include utilizing machine learning algorithms to analyze historical data, incorporating real-time data for more accurate predictions, and adapting to changing market conditions through continuous learning. The importance of accurate demand forecasting lies in its ability to reduce costs associated with excess inventory, improve cash flow management by optimizing stock levels, and enable better resource allocation and workforce planning. AI in demand forecasting and AI-based demand forecasting are vital for achieving these objectives.
Companies that implement AI-powered demand forecasting can gain a competitive edge by making proactive decisions based on reliable data, responding quickly to changes in consumer behavior, and enhancing overall operational efficiency. AI demand planning and AI in demand planning are essential components of this process.
At Rapid Innovation, we specialize in harnessing the power of AI and blockchain technologies to help our clients achieve their business goals efficiently and effectively. By partnering with us, you can expect increased ROI through improved decision-making processes, enhanced operational efficiency, and data-driven strategies that align with market demands. Our expertise in AI-enabled solutions ensures that your business remains agile and competitive in an ever-evolving landscape.
Refer to the image based on the role of AI in modern business planning and decision-making.
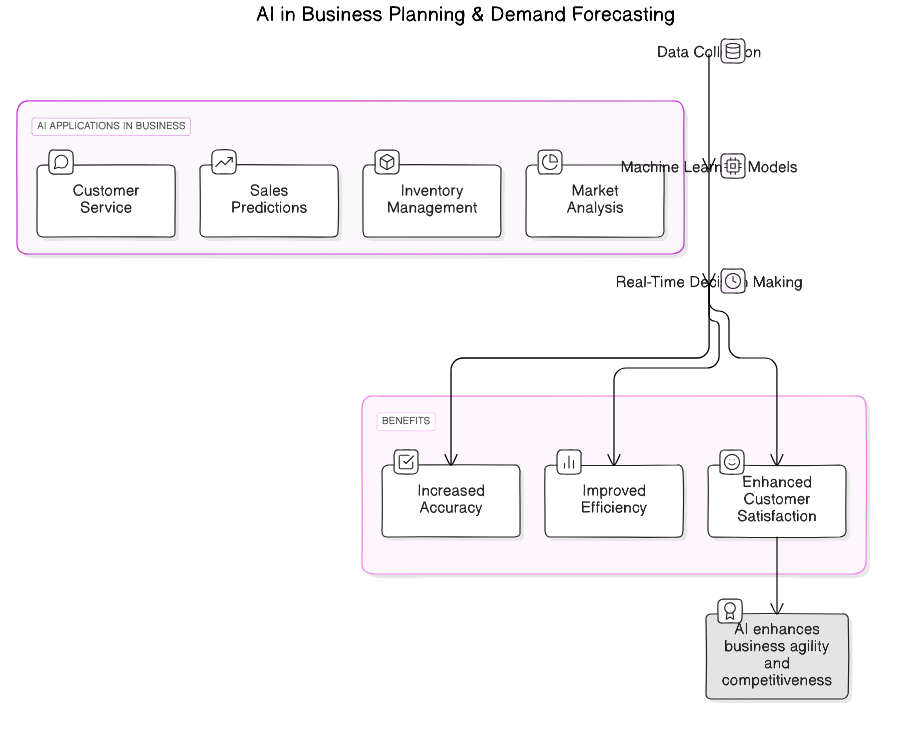
2.2. Key Components and Technologies Behind AI-Driven Predictions
AI-driven demand forecasting relies on several key components and technologies that enable machines to analyze data and make informed forecasts. These components include:
- Machine Learning Algorithms: Â
- Supervised learning: Uses labeled data to train models for predictions.
- Unsupervised learning: Identifies patterns in unlabeled data.
- Reinforcement learning: Learns optimal actions through trial and error.
- Data Processing Techniques: Â
- Data cleaning: Ensures accuracy by removing errors and inconsistencies.
- Data normalization: Standardizes data to improve model performance.
- Feature engineering: Selects and transforms variables to enhance predictive power.
- Big Data Technologies: Â
- Distributed computing frameworks (e.g., Hadoop, Spark): Allow processing of large datasets.
- Cloud computing: Provides scalable resources for data storage and processing.
- Natural Language Processing (NLP): Â
- Text analysis: Analyzes text data from sources like social media and customer reviews to extract insights.
- Sentiment analysis: Enables the gauging of public opinion and trends.
- Neural Networks: Â
- Deep learning models: Mimic human brain functions to recognize complex patterns.
- Applications: Useful in image and speech recognition, as well as time-series forecasting.
- Predictive Analytics Tools: Â
- Software platforms (e.g., Tableau, SAS): Integrate AI algorithms for data visualization and analysis.
- Decision-making: Facilitate decision-making by providing actionable insights.
2.3. How Does AI Forecast Demand?
AI forecasts demand by leveraging advanced algorithms and vast amounts of data to predict future customer behavior and market trends. The process typically involves several steps:
- Data Analysis: Â
- AI systems analyze historical sales data, market trends, and external factors (e.g., economic indicators) to identify patterns and correlations that inform future demand.
- Model Training: Â
- Machine learning models are trained on historical data to recognize patterns and make predictions. Continuous learning allows models to adapt to new data and changing market conditions.
- Scenario Simulation: Â
- AI can simulate various scenarios to assess potential impacts on demand, helping businesses prepare for different market conditions and customer behaviors.
- Real-Time Adjustments: Â
- AI systems can adjust forecasts in real-time based on new data inputs, ensuring that predictions remain relevant and accurate.
- Collaboration with Human Insights: Â
- AI complements human expertise by providing data-driven insights, while human intuition and experience can refine AI predictions.
2.3.1. Data Collection and Integration from Multiple Sources
Data collection and integration are critical for accurate AI-driven demand forecasting. This process involves gathering data from various sources and ensuring it is usable for analysis. Key aspects include:
- Diverse Data Sources: Â
- Internal data: Sales records, inventory levels, and customer databases.
- External data: Market trends, competitor analysis, and economic indicators.
- Social media: Customer sentiment and engagement metrics.
- Data Integration Techniques: Â
- ETL (Extract, Transform, Load): A process that extracts data from different sources, transforms it into a suitable format, and loads it into a data warehouse.
- APIs (Application Programming Interfaces): Facilitate real-time data exchange between systems.
- Data Quality Assurance: Â
- Regular audits ensure data accuracy and consistency, while implementing validation rules helps catch errors during data entry.
- Regular audits ensure data accuracy and consistency, while implementing validation rules helps catch errors during data entry.
- Data Storage Solutions: Â
- Cloud storage: Offers scalability and accessibility for large datasets.
- Data lakes: Store raw data in its native format, allowing for flexible analysis.
- Data Privacy and Security: Â
- Compliance with regulations (e.g., GDPR) protects customer data, and implementing security measures safeguards sensitive information.
- Collaboration Tools: Â
- Platforms that enable teams to share insights and collaborate on data analysis enhance communication and decision-making across departments.
At Rapid Innovation, we leverage these advanced technologies and methodologies to help our clients achieve their goals efficiently and effectively. By partnering with us, clients can expect enhanced ROI through improved decision-making, optimized operations, and a deeper understanding of market dynamics. Our expertise in AI-driven demand forecasting and blockchain development ensures that we provide tailored solutions that drive innovation and growth, ultimately leading to greater success in their respective industries. For more information on AI applications, check out our article on AI Agent for Marketing Applications: Use Cases, Capabilities, Best Practices, Benefits.
Refer to the image for a visual representation of the key components and technologies behind AI-driven predictions:

At Rapid Innovation, we understand that in today's data-driven world, the ability to harness and analyze vast amounts of information is crucial for achieving business goals. Our expertise in advanced data processing and AI-driven analytics empowers organizations to make informed decisions, enhance customer experiences, and ultimately drive greater ROI.
2.3.2. Advanced Data Processing and AI-Driven Analytics
- Advanced data processing involves the use of sophisticated algorithms and technologies to analyze large volumes of data.
- AI-driven analytics enhances traditional data analysis by incorporating machine learning and artificial intelligence techniques.
- Key components of advanced data processing include: Â
- Data collection: Gathering data from various sources, including social media, IoT devices, and transaction records.
- Data cleaning: Removing inaccuracies and inconsistencies to ensure high-quality data for analysis.
- Data integration: Combining data from different sources to create a unified view.
- AI algorithms can process data at unprecedented speeds, enabling real-time analysis and insights.
- Benefits of AI-driven analytics: Â
- Improved decision-making: Organizations can make informed decisions based on data-driven insights.
- Enhanced customer experiences: Businesses can tailor their offerings based on consumer preferences and behaviors.
- Predictive capabilities: AI can identify trends and patterns that help forecast future outcomes.
- Industries leveraging advanced data processing and AI-driven analytics include finance, healthcare, retail, and marketing.
By partnering with us, clients can expect to see significant improvements in their operational efficiency and customer engagement, leading to a higher return on investment.
2.3.3. Pattern Recognition: How AI Learns Consumer Behavior
- Pattern recognition is a core function of AI that enables it to identify trends and behaviors in consumer data.
- AI systems analyze historical data to detect patterns that inform predictions about future consumer actions.
- Key aspects of pattern recognition in consumer behavior include: Â
- Data segmentation: Dividing consumers into groups based on shared characteristics or behaviors.
- Behavioral analysis: Understanding how consumers interact with products and services over time.
- Sentiment analysis: Evaluating consumer opinions and emotions through text and social media data.
- Machine learning models, such as neural networks, are often used for pattern recognition due to their ability to learn from vast datasets.
- Benefits of understanding consumer behavior through pattern recognition: Â
- Personalized marketing: Tailoring advertisements and promotions to individual preferences.
- Improved product development: Creating products that meet the specific needs of target audiences.
- Enhanced customer retention: Identifying at-risk customers and implementing strategies to retain them.
- Companies like Amazon and Netflix utilize pattern recognition to recommend products and content based on user behavior.
Our clients can leverage these insights to create targeted marketing strategies that resonate with their audience, leading to increased sales and customer loyalty.
2.3.4. Generating Accurate Predictions with AI
- AI excels in generating accurate predictions by analyzing historical data and identifying underlying patterns.
- Predictive analytics involves using statistical algorithms and machine learning techniques to forecast future events.
- Key elements of generating accurate predictions with AI include: Â
- Data input: Feeding the AI system with relevant historical data to train predictive models.
- Model selection: Choosing the appropriate machine learning model based on the nature of the data and the prediction task.
- Continuous learning: AI systems can adapt and improve their predictions over time as new data becomes available.
- Applications of AI-generated predictions span various industries: Â
- Retail: Forecasting inventory needs and consumer demand to optimize stock levels.
- Finance: Predicting stock market trends and assessing credit risk for loan approvals.
- Healthcare: Anticipating patient outcomes and optimizing treatment plans based on historical data.
- Benefits of accurate predictions include: Â
- Increased efficiency: Organizations can allocate resources more effectively based on predicted needs.
- Risk management: Identifying potential risks and implementing strategies to mitigate them.
- Competitive advantage: Businesses can stay ahead of market trends and consumer demands through informed decision-making.
By collaborating with Rapid Innovation, clients can harness the power of AI-driven analytics to not only predict future trends but also to strategically position themselves in the market, ensuring sustained growth and profitability. Our commitment to delivering tailored solutions ensures that your organization can achieve its goals efficiently and effectively.
3. Industry-Specific Applications and Real-World Use Cases of AI Forecasting
3.1. AI Forecasting in Retail Industry
The retail industry has been significantly transformed by the integration of artificial intelligence (AI) forecasting. Retailers leverage AI to enhance decision-making processes, improve customer experiences, and optimize operations. AI forecasting helps retailers predict demand, manage inventory, and tailor marketing strategies effectively.
3.1.1. How AI Optimizes Inventory Management in Retail
AI plays a crucial role in optimizing inventory management, which is vital for maintaining profitability and customer satisfaction in the retail sector. Here are some key ways AI achieves this:
- Demand Forecasting: AI algorithms analyze historical sales data, seasonal trends, and external factors (like economic indicators) to predict future demand. This helps retailers stock the right amount of products, reducing the risk of overstocking or stockouts.
- Real-Time Inventory Tracking: AI systems can monitor inventory levels in real-time, providing accurate data on stock availability. This allows retailers to make informed decisions about restocking and reallocating inventory across different locations.
- Automated Replenishment: AI can automate the replenishment process by triggering orders based on predictive analytics. This ensures that popular items are always in stock, enhancing customer satisfaction and sales.
- Dynamic Pricing Strategies: AI analyzes market trends and competitor pricing to suggest optimal pricing strategies. This can help retailers adjust prices in real-time to maximize sales and minimize excess inventory.
- Supplier Management: AI tools can evaluate supplier performance and predict lead times, helping retailers choose the best suppliers for their needs. This improves the efficiency of the supply chain and reduces delays in inventory replenishment.
- Customer Behavior Analysis: AI can analyze customer purchasing patterns and preferences, allowing retailers to tailor their inventory to meet specific customer needs. This personalization can lead to increased sales and customer loyalty.
- Inventory Optimization Models: AI uses advanced algorithms to create optimization models that balance inventory levels with service levels. This helps retailers minimize holding costs while ensuring product availability.
- Scenario Planning: AI can simulate various market scenarios to help retailers prepare for unexpected changes in demand. This proactive approach allows retailers to adjust their inventory strategies accordingly.
- Reduction of Waste: By accurately predicting demand, AI helps retailers reduce waste, especially in perishable goods. This not only saves costs but also contributes to sustainability efforts.
- Enhanced Decision-Making: AI provides actionable insights and data-driven recommendations, empowering retail managers to make better inventory decisions. This leads to improved operational efficiency and profitability.
In conclusion, AI forecasting is revolutionizing inventory management in the retail industry by providing tools and insights that enhance efficiency, reduce costs, and improve customer satisfaction. Retailers that adopt AI-driven inventory management strategies, such as ai inventory management, ai for inventory management, and ai in inventory management, are better positioned to thrive in a competitive market.
At Rapid Innovation, we specialize in implementing these AI solutions tailored to your specific needs, ensuring that you achieve greater ROI and operational excellence. Partnering with us means you can expect enhanced decision-making capabilities, reduced operational costs, and improved customer satisfaction, ultimately driving your business success. Our expertise includes ai inventory management software, ai inventory optimization, and the use of ai in inventory management systems, ensuring comprehensive support for your inventory needs.
3.1.2. Using AI for Accurate Promotional Planning and Price Optimization
- AI technologies can analyze vast amounts of data to identify patterns and trends in consumer behavior.
- Machine learning algorithms can predict the effectiveness of various promotional strategies by evaluating historical sales data.
- AI can optimize pricing strategies by considering factors such as: Â
- Competitor pricing
- Customer demand
- Inventory levels
- Dynamic pricing models can be implemented, allowing businesses to adjust prices in real-time based on market conditions.
- AI tools can segment customers to tailor promotions, ensuring that marketing efforts are more targeted and effective.
- Predictive analytics can help businesses forecast the impact of promotions on sales, enabling better planning and resource allocation.
- By using AI promotional planning, companies can reduce the risk of overstocking or understocking during promotional periods, leading to improved profitability.
3.1.3. Seasonal Demand Trends and AI's Role in Store-Level Forecasting
- Seasonal demand trends can significantly impact inventory management and sales strategies.
- AI can analyze historical sales data to identify seasonal patterns and predict future demand fluctuations.
- Key benefits of AI in store-level forecasting include: Â
- Enhanced accuracy in predicting seasonal spikes or drops in demand.
- Improved inventory turnover rates by aligning stock levels with anticipated demand.
- Ability to account for external factors such as weather, holidays, and local events that influence buying behavior.
- AI-driven forecasting models can integrate real-time data, allowing for adjustments based on current market conditions.
- Retailers can optimize staffing and resource allocation based on accurate demand forecasts, improving customer service and operational efficiency.
- By leveraging AI, businesses can minimize waste and markdowns associated with unsold seasonal inventory.
3.1.4. AI’s Impact on Supply Chain and Stock Management
- AI technologies streamline supply chain operations by enhancing visibility and efficiency.
- Key impacts of AI on supply chain and stock management include: Â
- Predictive analytics that forecast demand, allowing for proactive inventory management.
- Automation of routine tasks, reducing human error and increasing operational speed.
- Real-time tracking of inventory levels, enabling timely replenishment and reducing stockouts.
- AI can optimize logistics by analyzing transportation routes and delivery schedules, leading to cost savings and improved delivery times.
- Machine learning algorithms can identify potential disruptions in the supply chain, allowing businesses to develop contingency plans.
- Enhanced data analytics can improve supplier relationship management by evaluating supplier performance and reliability.
- Overall, AI contributes to a more agile and responsive supply chain, enabling businesses to adapt quickly to changing market conditions.
At Rapid Innovation, we leverage these advanced AI capabilities to help our clients achieve greater ROI through enhanced decision-making, optimized operations, and improved customer engagement. By partnering with us, businesses can expect increased efficiency, reduced costs, and a significant competitive edge in their respective markets.
3.2. AIÂ Demand Forecasting in Supply Chain and Logistics
The supply chain and logistics sector is undergoing a significant transformation due to advancements in technology, particularly artificial intelligence (AI). AI is enhancing efficiency, reducing costs, and improving decision-making processes across various supply chain functions, including artificial intelligence in logistics and artificial intelligence for logistics.
3.2.1. AI-Driven Transportation and Warehouse Planning
AI-driven transportation and warehouse planning is revolutionizing how businesses manage their logistics operations. By leveraging AI technologies, companies can optimize routes, manage inventory, and enhance overall operational efficiency in logistics and artificial intelligence.
- Route Optimization: AI algorithms analyze traffic patterns, weather conditions, and delivery schedules to determine the most efficient routes. This reduces fuel consumption and delivery times, leading to cost savings and improved customer satisfaction.
- Inventory Management: AI systems predict demand trends using historical data and market analysis, allowing for better inventory control. Businesses can minimize overstock and stockouts, ensuring that products are available when needed without tying up excess capital in inventory.
- Warehouse Automation: AI technologies, such as robotics and machine learning, streamline warehouse operations by automating picking, packing, and sorting processes. This increases accuracy and speed, reducing labor costs and human error.
- Predictive Maintenance: AI can monitor equipment and vehicles in real-time, predicting when maintenance is needed before a failure occurs. This proactive approach minimizes downtime and extends the lifespan of assets.
- Data-Driven Decision Making: AI tools provide insights through data analytics, enabling managers to make informed decisions based on real-time information. This leads to improved strategic planning and resource allocation.
3.2.2. Using AI for Efficient Order Fulfillment and Supplier Management
AI is also playing a crucial role in enhancing order fulfillment and supplier management processes. By automating and optimizing these functions, businesses can achieve greater efficiency and responsiveness in ai in logistics and supply chain.
- Order Processing Automation: AI systems can automate order entry, processing, and tracking, reducing the time taken to fulfill customer orders. This leads to faster delivery times and improved customer satisfaction.
- Demand Forecasting: AI analyzes historical sales data and market trends to forecast future demand accurately. This helps businesses align their inventory levels with expected sales, reducing excess stock and minimizing waste.
- Supplier Selection and Evaluation: AI tools can assess supplier performance based on various metrics, such as delivery times, quality, and pricing. This enables companies to make data-driven decisions when selecting and managing suppliers.
- Risk Management: AI can identify potential risks in the supply chain, such as supplier disruptions or geopolitical issues, allowing businesses to develop contingency plans. This proactive approach helps maintain continuity and reduces the impact of unforeseen events.
- Enhanced Communication: AI-powered chatbots and virtual assistants facilitate communication between suppliers, logistics providers, and customers. This improves transparency and responsiveness, leading to better relationships and collaboration.
- Cost Reduction: By optimizing order fulfillment processes and supplier management, AI helps reduce operational costs. This can lead to increased profitability and a competitive edge in the market.
At Rapid Innovation, we understand the complexities of the supply chain and logistics landscape, including the applications of artificial intelligence in logistics and supply chain management. Our expertise in AI and blockchain technology allows us to provide tailored solutions that drive efficiency and enhance decision-making. By partnering with us, clients can expect improved operational performance, reduced costs, and a significant return on investment. Our commitment to innovation ensures that your business stays ahead of the competition, leveraging the latest technologies to achieve your goals effectively and efficiently in the logistics industry. For more information, visit the role of AI agents in supply chain and logistics.
3.2.3. How AI Helps with Risk Mitigation in Logistics
Artificial Intelligence (AI) plays a crucial role in enhancing risk mitigation strategies within the logistics sector. By leveraging advanced algorithms and data analytics, AI helps organizations identify, assess, and manage potential risks effectively.
- Predictive Analytics: AI systems analyze historical data to predict potential disruptions, forecasting issues such as supply chain delays, equipment failures, or demand fluctuations. This proactive approach allows companies to prepare for challenges before they arise.
- Real-time Monitoring: AI tools provide real-time tracking of shipments and inventory, allowing for immediate responses to unexpected events, such as weather disruptions or traffic delays. This capability ensures that logistics operations remain agile and responsive.
- Enhanced Decision-Making: AI algorithms can evaluate multiple scenarios and suggest optimal responses, helping logistics managers make informed decisions quickly and reducing the impact of risks. This leads to more strategic planning and execution.
- Improved Supplier Management: AI can assess supplier reliability and performance. By identifying weak links in the supply chain, companies can proactively seek alternatives or negotiate better terms, thereby strengthening their supply chain resilience.
- Risk Assessment Models: AI-driven models can quantify risks associated with various logistics operations, enabling organizations to prioritize risk management efforts based on potential impact. This targeted approach enhances overall risk management strategies.
- Cost Reduction: By minimizing disruptions and optimizing operations, AI contributes to significant cost savings, allowing companies to avoid losses associated with delays and inefficiencies. This ultimately leads to a higher return on investment (ROI) through effective logistics risk mitigation.
3.3. AI Demand Forecasting in Manufacturing Sector
The manufacturing sector is increasingly adopting AI technologies to enhance efficiency, reduce costs, and improve product quality. AI applications in manufacturing streamline processes and enable smarter decision-making.
- Automation of Processes: AI facilitates the automation of repetitive tasks, reducing human error. This leads to increased productivity and allows workers to focus on more complex tasks, driving innovation and efficiency.
- Quality Control: AI systems can monitor production lines in real-time to detect defects, ensuring that only high-quality products reach the market and reducing waste and rework. This commitment to quality enhances brand reputation and customer satisfaction.
- Supply Chain Optimization: AI analyzes data from various sources to optimize inventory levels and production schedules, helping manufacturers respond quickly to market demands and reduce excess stock. This agility is crucial in today’s fast-paced market.
- Predictive Maintenance: AI tools predict equipment failures before they occur, allowing for timely maintenance. This minimizes downtime and extends the lifespan of machinery, leading to significant cost savings and improved operational efficiency.
- Data-Driven Insights: AI provides actionable insights from vast amounts of production data, enabling manufacturers to refine processes and improve overall efficiency. This data-centric approach fosters continuous improvement and innovation.
3.3.1. Optimizing Production Planning with AI Forecasting Tools
AI forecasting tools are transforming production planning in the manufacturing sector by providing accurate demand predictions and optimizing resource allocation.
- Demand Forecasting: AI algorithms analyze historical sales data, market trends, and external factors to predict future demand, helping manufacturers align production schedules with actual market needs. This alignment reduces waste and enhances profitability.
- Resource Allocation: AI tools optimize the allocation of resources, including labor, materials, and machinery, ensuring that production runs smoothly without overcommitting resources. This efficiency translates into cost savings and improved margins.
- Scenario Planning: AI enables manufacturers to simulate various production scenarios based on different demand forecasts, allowing for better preparedness and flexibility in production planning. This capability enhances strategic decision-making.
- Inventory Management: AI forecasting tools help maintain optimal inventory levels by predicting when to reorder materials, reducing carrying costs and minimizing the risk of stockouts. This proactive inventory management supports operational efficiency.
- Continuous Improvement: AI systems learn from past production data to improve future forecasts. This iterative process leads to increasingly accurate predictions over time, fostering a culture of continuous improvement.
- Integration with Supply Chain: AI forecasting tools can integrate with supply chain management systems, ensuring that all stakeholders are aligned and can respond to changes in demand effectively. This integration enhances collaboration and operational synergy.
By partnering with Rapid Innovation, clients can leverage these advanced AI and blockchain solutions to achieve greater ROI, streamline operations, and enhance their competitive edge in the market. Our expertise in these domains ensures that we deliver tailored solutions that meet the unique needs of each client, driving efficiency and effectiveness in their operations.
3.3.2. Predicting Raw Material Needs and Scheduling Maintenance
Accurate prediction of raw material needs is crucial for maintaining production flow and minimizing costs. AI algorithms analyze historical data, market trends, and supplier performance to forecast material requirements, significantly enhancing production efficiency.
Benefits of AI in predicting raw material needs include: - Reduced waste through optimized inventory levels. - Enhanced supplier relationship management by anticipating demand. - Improved cash flow management by aligning purchases with production schedules.
Scheduling maintenance is equally important to avoid unexpected downtimes. AI can predict equipment failures by analyzing sensor data and maintenance history, which is vital for maintaining production efficiency.
Key advantages of AI in maintenance scheduling include: - Proactive maintenance reduces the risk of costly breakdowns. - Optimized maintenance schedules minimize production interruptions. - Data-driven insights help prioritize maintenance tasks based on urgency and impact.
3.3.3. Capacity Planning: AI’s Role in Managing Production Efficiency
Capacity planning ensures that production capabilities meet demand without overextending resources. AI enhances capacity planning by providing real-time data analysis and predictive modeling, which is essential for improving production efficiency.
Key contributions of AI in capacity planning include: - Demand forecasting: AI analyzes market trends and customer behavior to predict future demand. - Resource allocation: AI optimizes the distribution of labor and machinery based on production needs. - Scenario simulation: AI can simulate various production scenarios to identify the most efficient strategies.
Benefits of AI in managing production efficiency include: - Increased flexibility in responding to market changes. - Improved utilization of resources, leading to cost savings. - Enhanced decision-making through data-driven insights.
Companies leveraging AI for capacity planning report significant improvements in production efficiency and reduced lead times.
3.4. AI Demand Forecasting in Financial Services
The financial services sector is increasingly adopting AI technologies to enhance operations and customer experiences.
Key applications of AI in financial services include: - Fraud detection: AI algorithms analyze transaction patterns to identify suspicious activities in real-time. - Risk assessment: AI models evaluate creditworthiness and investment risks more accurately than traditional methods. - Customer service: AI-powered chatbots provide instant support and personalized financial advice.
Benefits of AI in financial services include: - Increased efficiency through automation of routine tasks. - Enhanced accuracy in data analysis, leading to better decision-making. - Improved customer satisfaction with faster response times and tailored services.
Regulatory compliance is another area where AI plays a vital role.
Key aspects of AI in regulatory compliance include: - Monitoring transactions for compliance with regulations, reducing the risk of penalties. - Automated reporting tools that streamline compliance processes, saving time and resources.
The integration of AI in financial services is expected to grow, with many institutions investing in AI technologies to stay competitive.
At Rapid Innovation, we understand the complexities of modern business challenges and are committed to helping our clients achieve their goals efficiently and effectively. By leveraging our expertise in AI and Blockchain technologies, we empower organizations to enhance their operational capabilities, optimize resource management, and ultimately achieve greater ROI.
For instance, our AI-driven solutions in predicting raw material needs and scheduling maintenance not only minimize costs but also enhance production flow, ensuring that our clients can meet market demands without unnecessary delays. Similarly, our capacity planning tools enable businesses to adapt swiftly to changing market conditions, leading to improved resource utilization and significant cost savings.
In the financial services sector, our AI applications enhance operational efficiency and customer satisfaction, allowing institutions to stay ahead of the competition. By automating routine tasks and providing data-driven insights, we help our clients make informed decisions that drive growth and profitability.
Partnering with Rapid Innovation means gaining access to cutting-edge technology solutions tailored to your specific needs. Our clients can expect increased efficiency, enhanced accuracy, and improved customer experiences, all contributing to a stronger bottom line. Let us help you navigate the future of technology and achieve your business objectives with confidence. For more information, visit our AI in Financial Contract Analysis. At Rapid Innovation, we understand that leveraging advanced technologies like AI for business intelligence can significantly enhance your business operations and drive greater ROI. Our expertise in AI and Blockchain development allows us to provide tailored solutions that help you achieve your goals efficiently and effectively. For more insights, check out the potential of business AI engineering best practices.
3.4.1. How AI Enhances Cash Flow Forecasting and Resource Planning
- AI technologies improve cash flow forecasting by analyzing vast amounts of historical data, enabling you to make informed financial decisions.
- Machine learning algorithms can identify patterns and trends that human analysts might overlook, ensuring you capitalize on every opportunity.
- Predictive analytics allows businesses to anticipate cash flow fluctuations, enabling proactive decision-making that can safeguard your financial health.
- AI can integrate real-time data from various sources, such as sales, expenses, and market conditions, to provide more accurate forecasts, reducing the risk of financial missteps.
- Automated tools can generate forecasts with minimal human intervention, reducing errors and saving time, allowing your team to focus on strategic initiatives.
- AI-driven simulations can model different scenarios, helping businesses prepare for potential cash flow challenges and ensuring you are always one step ahead.
- Enhanced resource planning is achieved through AI's ability to optimize inventory levels and manage supply chain logistics, leading to cost savings and improved efficiency.
- Companies can allocate resources more effectively, ensuring that they meet demand without overextending their budgets, ultimately enhancing profitability.
3.4.2. AI's Role in Predicting Product Demand and Managing Risk
- AI algorithms analyze historical sales data to predict future product demand with greater accuracy, allowing you to align production with market needs.
- By considering factors like seasonality, market trends, and consumer behavior, AI can refine demand forecasts, reducing the risk of overproduction or stockouts.
- Businesses can adjust production schedules and inventory levels based on AI-generated insights, reducing excess stock and minimizing waste, which directly impacts your bottom line.
- AI can also assess external factors, such as economic indicators and competitor actions, to provide a comprehensive view of potential risks, enabling you to navigate uncertainties effectively.
- Risk management is enhanced through AI's ability to identify anomalies and potential disruptions in the supply chain, allowing you to take corrective actions before issues escalate.
- Companies can implement AI-driven risk assessment models to evaluate the likelihood of various scenarios impacting product demand, ensuring you are prepared for any eventuality.
- This proactive approach allows businesses to develop contingency plans and mitigate risks before they materialize, safeguarding your investments.
- AI tools can continuously learn from new data, improving their predictive capabilities over time, ensuring that your strategies remain relevant and effective.
3.4.3. AI for Optimizing Branch Operations and Staffing
AI can streamline branch operations by automating routine tasks, such as scheduling and inventory management, freeing up valuable resources for more strategic activities. Intelligent systems analyze foot traffic and customer behavior to optimize staffing levels during peak and off-peak hours, ensuring you provide excellent service without overspending on labor.
AI-driven analytics identify inefficiencies in branch operations, allowing managers to make data-informed decisions that enhance productivity. Predictive maintenance powered by AI helps prevent equipment failures, ensuring that branches operate smoothly and reducing downtime.
AI enhances customer service by providing staff with real-time insights into customer preferences and needs, enabling personalized interactions that drive customer loyalty. Chatbots and virtual assistants handle basic inquiries, freeing up staff to focus on more complex customer interactions, ultimately improving customer satisfaction.
By analyzing performance metrics, AI helps identify training needs and improve employee productivity, fostering a culture of continuous improvement. Overall, AI contributes to a more efficient and responsive branch environment, enhancing both employee and customer satisfaction.
Partnering with Rapid Innovation means you can expect a strategic ally dedicated to helping you harness the power of AI business intelligence and AI solutions for business. Together, we can drive innovation, optimize operations, and achieve your business objectives with greater efficiency and effectiveness.
3.5. AI Demand Forecasting in Automotive Industry
The automotive industry is undergoing a significant transformation driven by advancements in technology, particularly artificial intelligence (AI). AI is being integrated into various aspects of the automotive sector, including ai automotive, ai in automotive, and automotive artificial intelligence, enhancing efficiency, productivity, and customer satisfaction.
3.5.1. AI Solutions for Predicting Parts Inventory and Production Scheduling
AI solutions are revolutionizing how automotive manufacturers manage parts inventory and production scheduling. By leveraging data analytics and machine learning algorithms, companies can optimize their supply chain processes.
- Predictive analytics: AI analyzes historical data to forecast future demand for parts, helping in maintaining optimal inventory levels and reducing excess stock and shortages.
- Real-time data processing: AI systems can process data from various sources, including sales trends, market conditions, and production capabilities, enabling manufacturers to adjust production schedules dynamically based on real-time insights.
- Improved efficiency: AI reduces lead times and enhances the overall production process while minimizing downtime by ensuring that necessary parts are available when needed.
- Cost savings: Optimized inventory management leads to reduced holding costs, and efficient production scheduling can lower operational costs and improve profit margins.
- Enhanced decision-making: AI provides actionable insights that help managers make informed decisions and facilitates better collaboration between departments, such as procurement and production.
3.5.2. AI-Powered Sales and Service Forecasting in the Automotive Sector
AI is also playing a crucial role in sales and service forecasting within the automotive industry. By analyzing customer data and market trends, AI tools can provide valuable insights that drive sales strategies and improve customer service.
- Customer behavior analysis: AI algorithms analyze customer preferences and buying patterns, helping in identifying potential sales opportunities and tailoring marketing efforts.
- Demand forecasting: AI predicts future sales trends based on historical data and market analysis, assisting dealerships in managing inventory and aligning sales strategies with customer demand.
- Service optimization: AI tools can forecast service needs based on vehicle usage patterns and maintenance history, enabling service departments to proactively schedule maintenance and repairs, thereby improving customer satisfaction.
- Enhanced customer experience: Personalized recommendations based on customer data lead to better engagement, while AI chatbots and virtual assistants provide 24/7 support, answering customer queries and scheduling appointments.
- Competitive advantage: Companies leveraging AI for sales and service forecasting can respond more quickly to market changes. Improved accuracy in forecasting leads to better resource allocation and strategic planning.
In conclusion, the integration of AI in the automotive industry is transforming inventory management, production scheduling, sales forecasting, and customer service. By harnessing the power of AI, automotive companies can enhance operational efficiency, reduce costs, and improve customer satisfaction. The benefits of AI in the automotive industry are becoming increasingly clear, with companies focusing on ai in automotive industry and ai in automobile industry to stay competitive. At Rapid Innovation, we are committed to helping our manufacturing clients navigate this AI transformation, ensuring they achieve greater ROI through tailored AI and blockchain solutions that drive efficiency and effectiveness in their operations. Partnering with us means gaining access to cutting-edge technology and expert guidance that can propel your business forward in this rapidly evolving landscape, including insights from artificial intelligence in automotive industry ppt and artificial intelligence in automotive industry pdf.
3.6. AI Demand Forecasting in Healthcare Sector
3.6.1. Predicting Patient Volume with AI for Better Resource Allocation
- AI technologies are increasingly being utilized to predict patient volume in healthcare settings, including ai healthcare forecasting.
- Accurate predictions help hospitals and clinics manage resources more effectively, ensuring that they can meet patient needs without overstaffing or underutilizing equipment.
- Machine learning algorithms analyze historical patient data, seasonal trends, and external factors (like flu seasons or pandemics) to forecast patient inflow.
- Benefits of AI in predicting patient volume include: Â
- Improved patient care through timely access to services.
- Enhanced operational efficiency by optimizing staff schedules and resource allocation.
- Reduction in wait times, leading to higher patient satisfaction.
- Hospitals using AI-driven models have reported a significant decrease in patient overflow and better management of emergency departments.
- A study indicated that AI can improve forecasting accuracy by up to 30%, allowing for more informed decision-making in healthcare management.
3.6.2. AI-Powered Forecasting for Medical Supplies and Staffing
- AI is revolutionizing how healthcare facilities forecast their needs for medical supplies and staffing.
- By analyzing data from various sources, including patient volume predictions, historical usage patterns, and supply chain dynamics, AI can provide accurate forecasts.
- Key advantages of AI-powered forecasting include: Â
- Reduction in waste by ensuring that supplies are ordered based on actual needs rather than estimates.
- Improved inventory management, leading to cost savings and better resource utilization.
- Enhanced staffing models that align workforce availability with patient care demands.
- AI systems can also adapt to real-time changes, such as sudden spikes in patient numbers due to outbreaks or emergencies.
- Facilities that have implemented AI forecasting tools have seen improvements in their supply chain efficiency and a decrease in stockouts of critical medical supplies.
- Research shows that AI can reduce supply chain costs by up to 20% while improving service levels.
At Rapid Innovation, we leverage our expertise in AI and blockchain to help healthcare organizations optimize their operations, enhance patient care, and achieve greater ROI. By partnering with us, clients can expect tailored solutions that not only meet their immediate needs but also position them for long-term success in an ever-evolving industry.
3.6.3. Using AI for Emergency Planning and Drug Demand Forecasting
- AI technologies are increasingly being utilized in emergency planning to enhance preparedness and response strategies. Predictive analytics powered by AI can analyze historical data and real-time information to forecast potential emergencies, such as natural disasters or public health crises. Machine learning algorithms can identify patterns in data, helping to predict the likelihood of events and their potential impact on communities.
- AI can assist in resource allocation by optimizing the distribution of emergency supplies and personnel based on predicted needs. In drug demand forecasting, AI models can analyze various factors, including seasonal trends, demographic data, and health trends, to predict the demand for medications. This forecasting helps pharmaceutical companies and healthcare providers ensure that they have adequate supplies to meet patient needs, reducing shortages and waste.
- AI can also monitor social media and news reports to gauge public sentiment and potential spikes in drug demand during crises. By integrating AI into emergency planning and drug demand forecasting, organizations can improve their responsiveness and efficiency, ultimately saving lives and resources. For more insights on AI applications, you can refer to this guide.
3.7. AI Demand Forecasting in Travel and Hospitality Industry
- The travel and hospitality industry is undergoing a transformation with the integration of AI technologies. AI is being used to enhance customer experiences, streamline operations, and improve decision-making processes. Key applications of AI in this sector include personalized recommendations, chatbots for customer service, and dynamic pricing strategies.
- AI can analyze vast amounts of data from various sources, including customer reviews, social media, and booking patterns, to provide insights into traveler preferences. This data-driven approach allows businesses to tailor their offerings and marketing strategies to meet the specific needs of their customers. Additionally, AI can help in managing operational efficiencies, such as optimizing staff schedules and inventory management.
- The use of AI in the travel and hospitality industry is expected to grow, with companies investing in technology to stay competitive and enhance customer satisfaction.
3.7.1. AI for Predicting Booking Patterns and Revenue Management
- AI plays a crucial role in predicting booking patterns, enabling travel and hospitality businesses to make informed decisions. Machine learning algorithms can analyze historical booking data to identify trends and seasonal fluctuations. This analysis helps businesses anticipate demand, allowing them to adjust pricing and availability accordingly.
- AI can also consider external factors, such as local events, holidays, and economic indicators, to refine predictions. Revenue management systems powered by AI can optimize pricing strategies in real-time, maximizing revenue while remaining competitive. By implementing dynamic pricing models, businesses can adjust rates based on demand, occupancy levels, and competitor pricing.
- AI can also enhance customer segmentation, allowing businesses to target specific groups with tailored offers and promotions. The integration of AI in booking pattern prediction and revenue management leads to improved profitability and customer satisfaction, as businesses can better meet the needs of their clients.
At Rapid Innovation, we leverage these advanced AI capabilities to help our clients in both emergency planning and the travel and hospitality sectors achieve greater efficiency and effectiveness. By partnering with us, organizations can expect enhanced decision-making, optimized resource allocation, and improved customer experiences, ultimately leading to a higher return on investment. Our expertise in AI and blockchain development ensures that our clients are equipped with the tools they need to thrive in an increasingly competitive landscape.
3.7.2. Using AI to Optimize Staff Scheduling and Maintenance Planning
AI can analyze historical data to predict staffing needs based on various factors such as seasonal trends, customer demand fluctuations, and employee availability. This is similar to how ai sales forecasting software operates, utilizing data to enhance decision-making.
Benefits of AI in staff scheduling:
- Improved efficiency: AI algorithms can create optimal schedules that minimize labor costs while ensuring adequate coverage, much like sales forecasting using ai.
- Enhanced employee satisfaction: By considering employee preferences and availability, AI can lead to more balanced workloads, akin to ai demand forecasting.
- Real-time adjustments: AI systems can adapt schedules dynamically in response to unexpected changes, such as employee absences or sudden spikes in demand, similar to ai in demand forecasting.
AI in maintenance planning:
- Predictive maintenance: AI can analyze equipment data to predict failures before they occur, reducing downtime and maintenance costs, much like ai for forecasting.
- Resource allocation: AI can optimize the scheduling of maintenance tasks, ensuring that resources are used effectively and that maintenance is performed at the most convenient times, similar to demand forecasting ai.
- Data-driven insights: AI can provide insights into equipment performance trends, helping organizations make informed decisions about upgrades or replacements, akin to ai powered demand forecasting tools.
4. Step-by-Step Guide to Implementing AI-Based Demand Forecasting
Understand the basics of demand forecasting:
Demand forecasting involves predicting future customer demand for products or services. Accurate forecasting helps businesses manage inventory, optimize production, and improve customer satisfaction, similar to ai for demand forecasting.
Identify the right AI tools:
Research various AI tools and platforms that specialize in demand forecasting, such as ai based demand forecasting. Consider factors such as ease of use, integration capabilities, and scalability.
Gather and prepare data:
Collect historical sales data, market trends, and external factors that may influence demand. Clean and preprocess the data to ensure accuracy and consistency, similar to demand forecasting using ai.
Choose the appropriate forecasting model:
Select a model that aligns with your business needs, such as time series analysis, machine learning algorithms, or regression analysis, akin to ai ml demand forecasting.
Train the AI model:
Use the prepared data to train the chosen model. Monitor the training process to ensure the model learns effectively, similar to ai ml based demand forecasting tool.
Validate and test the model:
Split the data into training and testing sets to evaluate the model's performance. Adjust parameters as needed to improve accuracy, akin to ai in demand planning.
Implement the model in your operations:
Integrate the AI model into your existing systems for real-time forecasting. Ensure that relevant stakeholders are trained to use the new system effectively, similar to ai in forecasting.
Monitor and refine the model:
Continuously track the model's performance and make adjustments based on new data and changing market conditions. Regularly update the model to maintain accuracy over time, akin to intelligent demand forecasting.
4.1. How to Implement AI in Demand Forecasting (Beginner’s Guide)
Start with a clear understanding of your goals:
Define what you want to achieve with AI in demand forecasting, such as reducing stockouts or improving inventory turnover, similar to ai demand planning.
Educate yourself on AI concepts:
Familiarize yourself with basic AI and machine learning concepts to better understand how they apply to demand forecasting.
Assess your current forecasting methods:
Evaluate the effectiveness of your existing forecasting processes and identify areas for improvement, similar to sales forecasting ai.
Choose the right data sources:
Identify internal and external data sources that can enhance your forecasting accuracy, including sales history, market research, and economic indicators.
Select an AI tool or platform:
Research user-friendly AI tools that cater to beginners and offer robust support and resources, similar to ai powered demand planning software.
Start small with pilot projects:
Implement AI forecasting on a smaller scale to test its effectiveness before rolling it out across the organization.
Collaborate with stakeholders:
Involve key stakeholders from various departments to ensure buy-in and gather diverse insights.
Measure success and iterate:
Establish key performance indicators (KPIs) to measure the success of your AI implementation. Use feedback and performance data to refine your approach continuously.
At Rapid Innovation, we specialize in leveraging AI and blockchain technologies to help businesses like yours achieve greater efficiency and ROI. By partnering with us, you can expect tailored solutions that not only meet your unique needs but also drive significant improvements in operational performance and customer satisfaction. Our expertise ensures that you stay ahead of the competition while maximizing your investment in technology.
4.2. Initial Assessment: Is Your Business Ready for AI Forecasting?
- Evaluate your current processes: Â
- Identify existing forecasting methods and their effectiveness.
- Assess the accuracy of current demand predictions.
- Determine organizational readiness: Â
- Ensure leadership support for AI initiatives.
- Foster a culture that embraces data-driven decision-making.
- Analyze data infrastructure: Â
- Check if you have the necessary data storage and processing capabilities.
- Evaluate the quality and accessibility of your data.
- Identify key performance indicators (KPIs): Â
- Define what success looks like for your AI forecasting efforts.
- Establish metrics to measure the impact of AI on demand forecasting.
- Consider industry-specific factors: Â
- Understand how AI forecasting can address unique challenges in your sector.
- Research competitors' use of AI in forecasting to gauge market trends.
4.3. Preparing Your Data: Best Practices for Data Collection
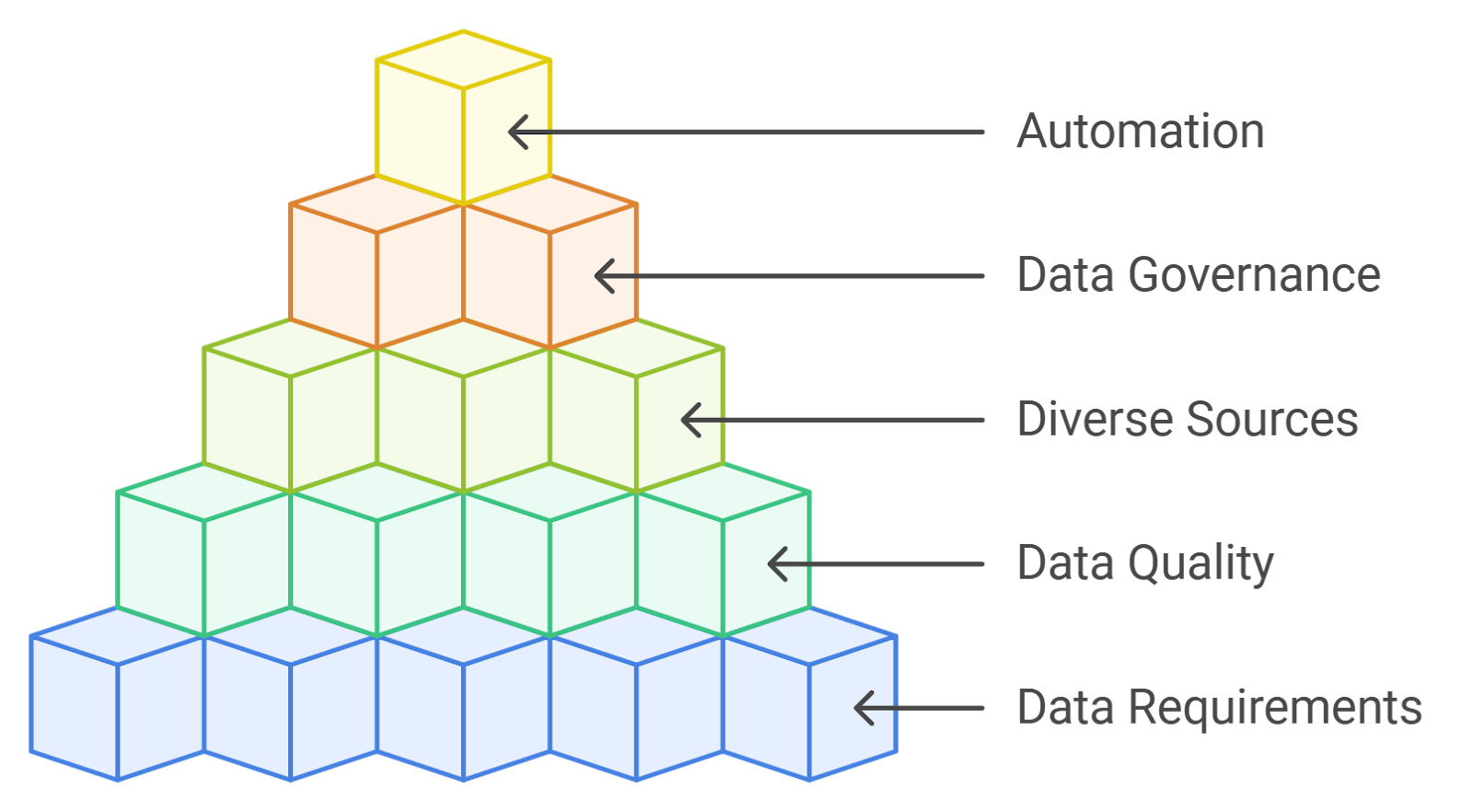
‍
- Define data requirements: Â
- Identify the types of data needed for accurate forecasting (historical sales, market trends, etc.).
- Determine the frequency and granularity of data collection.
- Ensure data quality: Â
- Implement processes for data cleaning to remove inaccuracies and inconsistencies.
- Regularly audit data sources to maintain high-quality inputs.
- Utilize diverse data sources: Â
- Combine internal data (sales, inventory) with external data (market trends, economic indicators).
- Explore unstructured data (social media, customer reviews) for additional insights.
- Establish a data governance framework: Â
- Define roles and responsibilities for data management within your organization.
- Create policies for data access, security, and compliance.
- Automate data collection processes: Â
- Use tools and software to streamline data gathering and reduce manual errors.
- Implement real-time data feeds where possible to keep information current.
4.4. Building and Training AI Models for Demand Prediction
- Choose the right algorithms: Â
- Evaluate different machine learning algorithms (e.g., regression, time series analysis) based on your data characteristics.
- Consider ensemble methods for improved accuracy.
- Split data into training and testing sets: Â
- Use a portion of your data to train the model and another to validate its performance.
- Ensure that the testing set is representative of future demand scenarios.
- Feature engineering: Â
- Identify and create relevant features that can enhance model performance.
- Consider seasonal trends, promotions, and other factors that influence demand.
- Train the model iteratively: Â
- Continuously refine the model by adjusting parameters and incorporating new data.
- Monitor performance metrics to ensure the model is improving over time.
- Validate and test the model: Â
- Use cross-validation techniques to assess model robustness.
- Compare predictions against actual outcomes to evaluate accuracy.
- Implement feedback loops: Â
- Establish mechanisms for ongoing learning and adaptation of the model.
- Use real-time data to update the model and improve its predictive capabilities.
At Rapid Innovation, we understand that the journey to effective AI forecasting readiness begins with a thorough assessment of your current capabilities and readiness. By partnering with us, you can expect a structured approach that not only enhances your forecasting accuracy but also drives greater ROI through informed decision-making and optimized resource allocation. Our expertise in AI and blockchain development ensures that you are equipped with the best tools and strategies to stay ahead in your industry.
4.5. How to Integrate AI Forecasting into Your Existing Systems
Integrating AI forecasting integration into existing systems can significantly enhance decision-making and improve operational efficiency. Here are key steps to consider:
- Assess Current Systems Â
- Evaluate existing forecasting methods and tools.
- Identify gaps and areas for improvement.
- Choose the Right AI Tools Â
- Select AI forecasting tools that align with your business needs.
- Consider platforms that offer easy integration with current systems.
- Data Preparation Â
- Ensure data quality by cleaning and organizing historical data.
- Integrate data from various sources for a comprehensive view.
- API Integration Â
- Utilize APIs to connect AI forecasting tools with existing software.
- Ensure seamless data flow between systems for real-time updates.
- Train the AI Model Â
- Use historical data to train the AI model for accurate predictions.
- Continuously refine the model based on new data and feedback.
- Monitor Performance Â
- Regularly assess the accuracy of AI forecasts.
- Adjust parameters and retrain models as necessary.
- User Training Â
- Provide training for staff on how to use the new AI tools.
- Encourage feedback to improve the integration process.
4.6. Optimizing AI Models Over Time for Better Forecasting
Optimizing AI models is crucial for maintaining accuracy and relevance in forecasting. Here are strategies to enhance model performance:
- Continuous Learning Â
- Implement mechanisms for the model to learn from new data.
- Use techniques like reinforcement learning to adapt to changing conditions.
- Regular Updates Â
- Schedule regular updates to the model with fresh data.
- Monitor trends and seasonality to adjust forecasting methods accordingly.
- Feature Engineering Â
- Identify and create new features that can improve model accuracy.
- Experiment with different variables to see their impact on forecasts.
- Hyperparameter Tuning Â
- Adjust hyperparameters to find the optimal settings for the model.
- Use techniques like grid search or random search for effective tuning.
- Performance Metrics Â
- Establish clear metrics to evaluate model performance.
- Use metrics like Mean Absolute Error (MAE) or Root Mean Square Error (RMSE) for assessment.
- Stakeholder Feedback Â
- Gather input from stakeholders to understand forecasting needs.
- Use feedback to refine models and improve user satisfaction.
- A/B Testing Â
- Conduct A/B tests to compare different model versions.
- Use results to determine which model performs better in real-world scenarios.
5. Key Success Factors for AI Demand Forecasting
Successful AI demand forecasting relies on several critical factors. Here are the key elements to consider:
- Data Quality Â
- High-quality, accurate data is essential for reliable forecasts.
- Regularly clean and validate data to maintain integrity.
- Cross-Functional Collaboration Â
- Foster collaboration between departments such as sales, marketing, and supply chain.
- Share insights and data to create a unified forecasting approach.
- Clear Objectives Â
- Define clear goals for what the forecasting should achieve.
- Align forecasting objectives with overall business strategy.
- Technology Infrastructure Â
- Invest in robust technology that supports AI capabilities.
- Ensure systems can handle large volumes of data and complex algorithms.
- Skilled Personnel Â
- Employ data scientists and analysts with expertise in AI and forecasting.
- Provide ongoing training to keep skills up to date.
- Change Management Â
- Prepare the organization for changes brought by AI integration.
- Communicate the benefits and involve employees in the transition process.
- Continuous Improvement Â
- Establish a culture of continuous improvement in forecasting practices.
- Regularly review and refine processes based on performance and feedback.
By partnering with Rapid Innovation, clients can leverage our expertise in AI forecasting integration and Blockchain to seamlessly integrate these advanced technologies into their existing systems. This not only enhances operational efficiency but also drives greater ROI through improved forecasting accuracy and decision-making capabilities. Our tailored solutions ensure that businesses can adapt to market changes swiftly, ultimately leading to increased profitability and sustained growth.
5.1. Factors that Influence Forecast Accuracy (And How to Improve It)
Forecast accuracy is crucial for effective decision-making in businesses. Several factors can influence the accuracy of forecasts:
- Data Quality: Inaccurate or incomplete data can lead to poor forecasts. Regularly clean and validate data to ensure reliability. For more insights on the importance of data quality, check out the critical role of data quality in AI implementations.
- Model Selection: Different forecasting models have varying levels of accuracy depending on the context. Experiment with multiple models to find the best fit for your data.
- External Variables: Economic conditions, market trends, and competitor actions can impact forecasts. Incorporate external data sources to enhance predictive capabilities.
- Time Horizon: Short-term forecasts tend to be more accurate than long-term ones. Adjust forecasting methods based on the time frame being analyzed.
- Human Factors: Biases in judgment can skew forecasts. Use collaborative forecasting techniques to mitigate individual biases.
To improve forecast accuracy:
- Implement advanced analytics and machine learning techniques, including improving forecast accuracy with machine learning.
- Regularly review and update forecasting models based on new data to increase forecast accuracy.
- Foster a culture of continuous improvement in forecasting practices, exploring ways to improve forecasting accuracy.
5.2. Technical Considerations for Implementing AI Forecasting Models
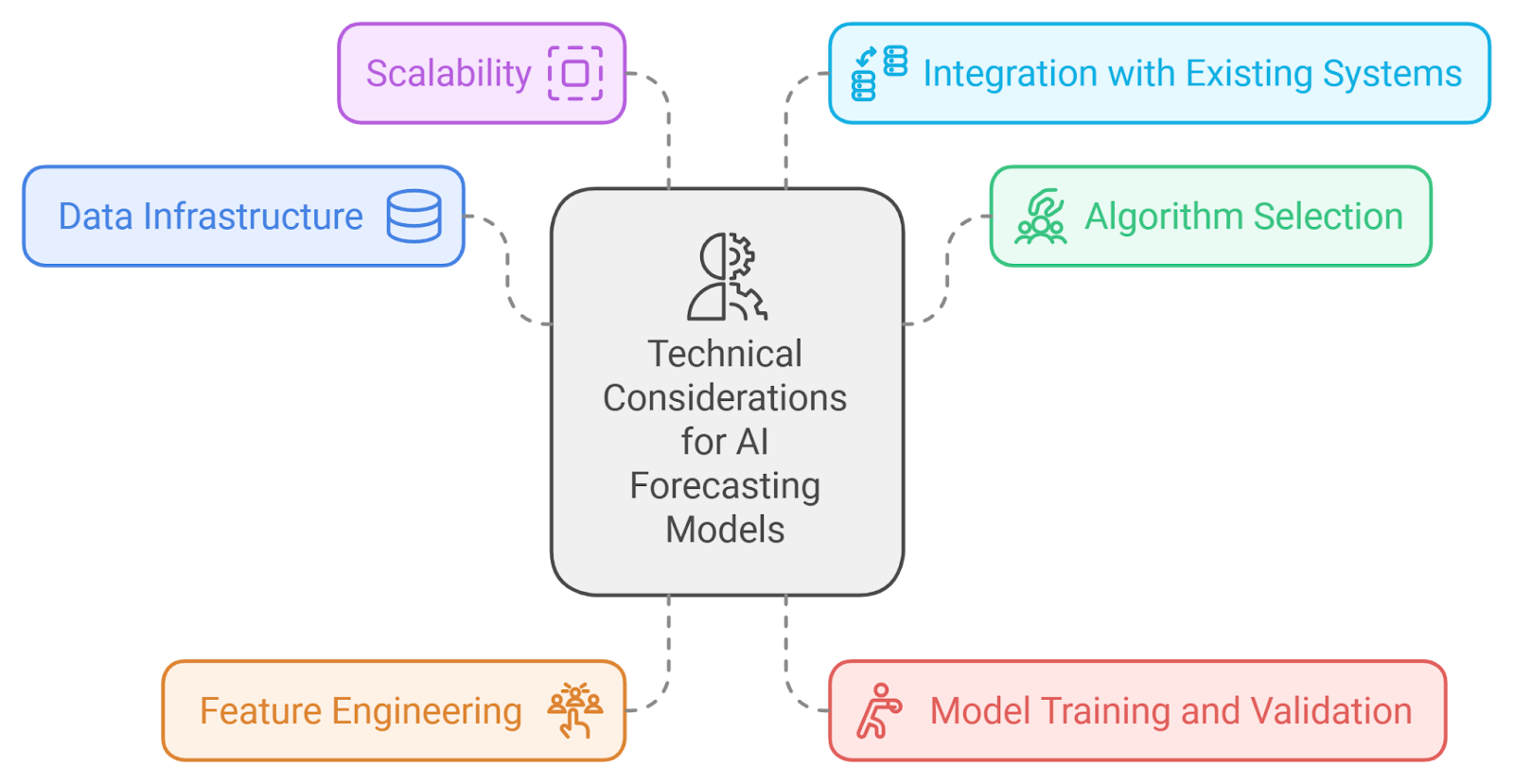
‍
Implementing AI forecasting models involves several technical considerations that can significantly impact their effectiveness:
- Data Infrastructure: Ensure you have a robust data infrastructure to support AI models. Invest in data storage and processing capabilities to handle large datasets.
- Algorithm Selection: Choose algorithms that are suitable for your specific forecasting needs. Consider using ensemble methods that combine multiple algorithms for better accuracy.
- Feature Engineering: Identify and create relevant features that can improve model performance. Use domain knowledge to select features that are most predictive.
- Model Training and Validation: Split data into training and validation sets to assess model performance. Use cross-validation techniques to ensure models generalize well to unseen data.
- Scalability: Design models that can scale with increasing data volumes. Consider cloud-based solutions for flexibility and scalability.
- Integration with Existing Systems: Ensure that AI models can be integrated with existing business systems. Use APIs to facilitate data exchange between systems.
5.3. Business Considerations: Aligning AI Forecasting with Business Goals
Aligning AI forecasting with business goals is essential for maximizing its value. Key considerations include:
- Understanding Business Objectives: Clearly define what the business aims to achieve with forecasting. Align forecasting efforts with strategic goals, such as revenue growth or cost reduction.
- Stakeholder Engagement: Involve key stakeholders in the forecasting process to ensure buy-in. Regularly communicate insights and updates to relevant teams.
- Customization of Forecasting Models: Tailor forecasting models to meet specific business needs and contexts. Consider industry-specific factors that may influence forecasts, including forecast accuracy in the supply chain.
- Performance Metrics: Establish clear metrics to evaluate the success of forecasting efforts. Use KPIs that reflect business objectives, such as accuracy, timeliness, and impact on decision-making.
- Change Management: Prepare the organization for changes that AI forecasting may bring. Provide training and resources to help teams adapt to new forecasting tools and processes.
- Continuous Feedback Loop: Create a system for ongoing feedback to refine forecasting models. Use insights from actual performance to improve future forecasts.
At Rapid Innovation, we understand that effective forecasting is not just about numbers; it's about making informed decisions that drive business success. By leveraging our expertise in AI and blockchain technologies, we can help you enhance your forecasting accuracy, leading to greater ROI. Our tailored solutions ensure that your forecasting models align with your specific business goals, enabling you to navigate market complexities with confidence. Partnering with us means you can expect improved data quality, advanced analytics, and a collaborative approach that fosters continuous improvement, ultimately positioning your business for sustained growth and success.
5.4. Managing Risk When Using AI for Business Forecasting
- Understanding the inherent risks of AI in forecasting is crucial for businesses.
- Key risks include: Â
- Data quality issues: Poor data can lead to inaccurate forecasts.
- Model bias: AI models can perpetuate existing biases in data, leading to skewed predictions.
- Overfitting: AI models may perform well on historical data but fail to generalize to new data.
- Strategies for managing these risks: Â
- Regularly audit data sources to ensure accuracy and relevance.
- Implement bias detection mechanisms to identify and mitigate bias in AI models.
- Use cross-validation techniques to test model performance on unseen data.
- Incorporate AI forecasting strategies that focus on continuous improvement and adaptation to changing data environments.
- Establish a robust governance framework: Â
- Define clear roles and responsibilities for AI oversight.
- Create protocols for model updates and maintenance.
- Foster a culture of transparency: Â
- Encourage open discussions about AI decision-making processes.
- Provide stakeholders with insights into how forecasts are generated.
- Continuous monitoring and evaluation: Â
- Regularly assess the performance of AI forecasting models.
- Adjust models based on changing market conditions and new data.
6. AI-Powered Forecasting vs Traditional Methods
- AI-powered forecasting leverages advanced algorithms and machine learning techniques.
- Traditional methods often rely on historical data and statistical techniques.
- Key differences include: Â
- Data handling:
- AI can process vast amounts of structured and unstructured data.
- Traditional methods typically focus on structured data.
- Speed and efficiency:
- AI models can generate forecasts in real-time.
- Traditional methods may require more time for data analysis and model adjustments.
- Adaptability:
- AI can quickly adapt to new patterns and trends in data.
- Traditional methods may struggle to adjust to sudden market changes.
- Data handling:
- Benefits of AI-powered forecasting: Â
- Improved accuracy through complex pattern recognition.
- Enhanced predictive capabilities by incorporating diverse data sources.
- Automation of repetitive tasks, freeing up human resources for strategic decision-making.
- Limitations of AI: Â
- Requires significant data and computational resources.
- May lack interpretability, making it difficult for stakeholders to understand predictions.
6.1. Comparing AI Forecasting to Traditional Approaches: What’s Better?
- The choice between AI forecasting and traditional methods depends on various factors: Â
- Business needs:
- Companies with complex data environments may benefit more from AI.
- Simpler forecasting needs may be adequately met by traditional methods.
- Resource availability:
- AI requires investment in technology and skilled personnel.
- Traditional methods may be more accessible for smaller businesses with limited resources.
- Performance comparison:
- Studies show that AI forecasting can outperform traditional methods in accuracy.
- AI can identify non-linear relationships in data that traditional methods may miss.
- Considerations for implementation:
- Organizations should assess their readiness for AI adoption.
- Training and change management are essential for successful integration.
- Hybrid approaches:
- Some businesses may find value in combining both methods.
- Using AI for initial forecasts and traditional methods for validation can enhance reliability.
- Business needs:
- Ultimately, the best approach is context-dependent, requiring careful evaluation of specific business goals and data capabilities.
At Rapid Innovation, we understand the complexities of AI and blockchain technologies and how they can be leveraged to enhance your business operations. By partnering with us, you can expect to achieve greater ROI through tailored solutions that address your unique challenges. Our expertise in managing risks associated with AI forecasting ensures that you can make informed decisions while maximizing the accuracy and reliability of your forecasts. Let us help you navigate the future of business forecasting with confidence.
6.2. How AI-Powered Forecasting Outperforms Traditional Methods

‍
AI-powered forecasting utilizes advanced algorithms and machine learning techniques to analyze vast amounts of data, leading to more accurate predictions compared to traditional methods.
- Enhanced Data Processing
AI can process large datasets quickly, identifying patterns that may not be visible through manual analysis. Traditional methods often rely on historical data and simple statistical techniques, which can overlook complex relationships. - Real-Time Analysis
AI systems can analyze data in real-time, allowing businesses to adjust forecasts based on the latest information. In contrast, traditional forecasting methods typically use static data, which can become outdated quickly. - Improved Accuracy
Studies show that AI-driven forecasts can reduce errors by up to 30% compared to traditional methods. Machine learning models continuously learn and adapt, improving their accuracy over time. - Incorporation of Diverse Data Sources
AI can integrate various data types, including social media trends, weather patterns, and economic indicators. Traditional methods often rely on limited datasets, which can lead to biased or incomplete forecasts. - Automation and Efficiency
AI automates the forecasting process, reducing the time and resources needed for manual analysis. This allows teams to focus on strategic decision-making rather than data crunching.
6.3. Cost-Benefit Analysis: Is AI-Driven Demand Forecasting Worth It?
Implementing AI-driven demand forecasting involves costs, but the potential benefits often outweigh these expenses.
- Initial Investment
Costs include software acquisition, infrastructure upgrades, and training personnel. Businesses may need to invest in data management systems to support AI tools. - Long-Term Savings
AI forecasting can lead to significant cost reductions in inventory management and supply chain operations. Improved accuracy can minimize overstock and stockouts, reducing waste and lost sales. - Increased Revenue
More accurate forecasts can enhance customer satisfaction by ensuring product availability. Businesses can capitalize on market trends and consumer behavior, leading to increased sales. - Competitive Advantage
Companies using AI-driven forecasting can respond more quickly to market changes, gaining an edge over competitors. Enhanced decision-making capabilities can lead to better strategic planning and resource allocation. - Return on Investment (ROI)
Many organizations report a positive ROI within the first year of implementing AI forecasting solutions. The long-term benefits often include improved operational efficiency and higher profit margins.
6.4. Key Metrics for Evaluating AI Forecast Performance
To assess the effectiveness of AI-driven forecasting, businesses should track specific performance metrics.
- Mean Absolute Error (MAE)
MAE measures the average magnitude of errors in a set of forecasts, providing insight into accuracy. A lower MAE indicates better forecasting performance. - Mean Absolute Percentage Error (MAPE)
MAPE expresses forecast accuracy as a percentage, making it easier to compare across different products or time periods. A lower MAPE signifies more reliable forecasts. - Forecast Bias
This metric assesses whether forecasts are consistently overestimating or underestimating demand. Identifying bias helps in adjusting models for improved accuracy. - R-squared Value
This statistic indicates how well the forecast model explains the variability of the data. A higher R-squared value suggests a better fit between the model and actual outcomes. - Customer Satisfaction Metrics
Tracking customer feedback and satisfaction can provide indirect insights into the effectiveness of demand forecasting. Improved availability and reduced stockouts often correlate with higher customer satisfaction scores. - Inventory Turnover Ratio
This ratio measures how often inventory is sold and replaced over a period. A higher turnover indicates effective demand forecasting and inventory management. - Cost of Goods Sold (COGS)
Monitoring COGS can help evaluate the financial impact of forecasting accuracy on overall profitability. Reducing COGS through better forecasting can lead to improved margins.
By partnering with Rapid Innovation, clients can leverage these advanced AI-driven forecasting capabilities to enhance their operational efficiency, reduce costs, and ultimately achieve a greater return on investment. Our expertise in AI and blockchain development ensures that your business is equipped with the tools necessary to thrive in a competitive landscape.
7. Benefits and ROI of AI Demand Forecasting
AI demand forecasting leverages advanced algorithms and machine learning to predict customer demand more accurately. This technology offers numerous benefits that can significantly enhance a business's return on investment (ROI):
- Enhanced accuracy in demand predictions.
- Reduction in excess inventory and stockouts.
- Improved customer satisfaction and loyalty.
- Better resource allocation and planning.
- Increased competitiveness in the market.
7.1. How AI Improves Business Efficiency
AI demand forecasting streamlines various business processes, leading to improved efficiency across the board. By utilizing AI, companies can make data-driven decisions that optimize their operations:
- Real-time data analysis allows for quick adjustments to demand changes.
- Predictive analytics helps in anticipating market trends.
- Automation of routine tasks frees up human resources for strategic initiatives.
- Enhanced collaboration across departments through shared insights.
7.1.1. Operational Efficiency: Reduced Costs and Improved Productivity
AI demand forecasting directly impacts operational efficiency by minimizing costs and boosting productivity. Here are some key aspects:
- Cost Reduction: It decreases the need for excess inventory, reducing storage costs, lowers the risk of markdowns and waste due to overproduction, and optimizes supply chain management, leading to lower transportation and logistics costs.
- Improved Productivity: AI automates repetitive tasks, allowing employees to focus on higher-value activities. It enhances decision-making speed with real-time insights, leading to quicker responses to market changes, and streamlines communication between teams, reducing delays and improving project timelines.
- Data-Driven Decisions: AI utilizes historical data and market trends to inform production schedules and inventory levels. It enables proactive rather than reactive strategies, minimizing disruptions in operations, and facilitates better forecasting accuracy, which leads to more effective resource allocation.
- Scalability: AI systems can easily adapt to growing data volumes, making it easier for businesses to scale operations. They also support expansion into new markets by providing insights into local demand patterns.
By implementing AI demand forecasting, businesses can achieve significant operational efficiencies that translate into cost savings and enhanced productivity. Solutions like ai sales forecasting software and sales forecasting using ai can further enhance these capabilities. Partnering with Rapid Innovation allows you to harness these benefits, ensuring that your organization not only meets but exceeds its operational goals, ultimately leading to a greater ROI. Our expertise in AI and blockchain development positions us as a valuable ally in your journey toward enhanced efficiency and competitiveness in the market. With tools such as ai demand forecasting and ai for forecasting, businesses can stay ahead of the curve. For more information on the benefits of AI-driven efficiency and cost savings, visit this guide.
7.1.2. Strategic Benefits: Smarter Business Decisions and Better Planning
- AI forecasting enhances decision-making by providing data-driven insights that empower organizations to make informed choices.
- Businesses can analyze vast amounts of data quickly, identifying trends and patterns that may not be visible through traditional methods, thus gaining a competitive edge.
- Improved accuracy in predictions leads to better resource allocation, reducing waste and optimizing inventory levels, which directly contributes to cost savings.
- Companies can anticipate market changes, allowing them to adapt strategies proactively rather than reactively, ensuring they stay ahead of the competition.
- AI tools can simulate various scenarios, helping businesses evaluate potential outcomes and make informed choices that align with their strategic goals.
- Enhanced customer insights enable personalized marketing strategies, improving customer engagement and satisfaction, which ultimately drives loyalty and repeat business.
- Overall, AI forecasting supports strategic planning by aligning business objectives with market realities, ensuring that organizations are well-prepared for future challenges.
7.2. Measurable Outcomes: Real Examples of Business Success with AI Forecasting
- Companies leveraging AI forecasting have reported significant improvements in operational efficiency and profitability, showcasing the value of this technology.
- For instance, a retail chain using AI forecasting for business saw a 20% reduction in stockouts, leading to increased sales and enhanced customer satisfaction.
- A major airline implemented AI to predict passenger demand, resulting in a 15% increase in revenue through optimized flight schedules and pricing strategies, demonstrating the financial impact of accurate forecasting.
- In the manufacturing sector, a company that adopted AI forecasting reduced production costs by 10% by accurately predicting material needs and minimizing excess inventory, showcasing the operational benefits.
- These measurable outcomes demonstrate the tangible benefits of integrating AI forecasting into business operations, highlighting the potential for increased ROI.
7.3. Case Studies: Companies That Increased Revenue with AI Forecasting
- Numerous companies have successfully utilized AI forecasting to boost their revenue and enhance their market position.
- A well-known e-commerce platform employed AI algorithms to analyze customer behavior, leading to a 30% increase in conversion rates through targeted recommendations, illustrating the power of data-driven marketing.
- A global beverage company used AI to forecast demand for new product launches, resulting in a 25% increase in sales during the first quarter of the launch, demonstrating the effectiveness of predictive analytics.
- A financial services firm implemented AI forecasting to enhance risk assessment, which improved their investment strategies and led to a 40% increase in portfolio returns, showcasing the financial advantages of AI integration.
- These case studies illustrate how AI forecasting not only drives revenue growth but also enhances overall business performance, making it a valuable investment for organizations looking to thrive in a competitive landscape.
By partnering with Rapid Innovation, clients can leverage our expertise in AI and Blockchain to achieve these outcomes, ensuring they maximize their return on investment while navigating the complexities of today's business environment.
8. Future Trends in AI-Driven Demand Forecasting (2024-2027)
The landscape of demand forecasting is rapidly evolving, driven by advancements in artificial intelligence (AI). As businesses seek to enhance their predictive capabilities, several trends are emerging that will shape the future of AI-driven demand forecasting from 2024 to 2027.
8.1. Emerging Technologies in AI Forecasting (What’s Next?)
- Machine Learning Enhancements: Continuous improvements in machine learning algorithms will lead to more accurate predictions. Techniques like deep learning will enable models to analyze complex datasets more effectively.
- Natural Language Processing (NLP): NLP will allow businesses to analyze unstructured data, such as customer reviews and social media sentiment, providing insights into consumer preferences and trends that traditional data sources may miss.
- Internet of Things (IoT) Integration: IoT devices will generate vast amounts of real-time data, which can be leveraged for more dynamic forecasting. Businesses can track inventory levels, customer behavior, and environmental factors to refine their demand predictions.
- Cloud Computing: The shift to cloud-based solutions will facilitate easier access to advanced AI tools and data analytics. Companies can scale their forecasting capabilities without significant upfront investments in infrastructure.
- Blockchain Technology: Blockchain can enhance data integrity and transparency in the supply chain, leading to more reliable forecasting. Smart contracts can automate inventory management based on real-time demand signals.
- Augmented Analytics: Tools that combine AI with data analytics will empower non-technical users to generate insights. This democratization of data will enable more stakeholders to contribute to demand forecasting efforts.
8.2. The Future of Demand Forecasting: AI-Driven Innovations

‍
- Predictive Analytics: AI will enhance predictive analytics, allowing businesses to anticipate demand fluctuations with greater precision. Companies will be able to model various scenarios and assess the impact of external factors on demand.
- Real-Time Forecasting: The ability to update forecasts in real-time will become standard practice, enabling businesses to respond more swiftly to changes in consumer behavior and market conditions.
- Personalized Demand Forecasting: AI will enable hyper-personalized forecasting based on individual customer preferences and behaviors, helping businesses tailor their offerings and inventory to meet specific customer needs.
- Collaborative Forecasting: AI tools will facilitate collaboration between different departments, such as sales, marketing, and supply chain. Shared insights will lead to more cohesive and accurate demand forecasts.
- Sustainability Considerations: Future demand forecasting will increasingly incorporate sustainability metrics, analyzing how consumer preferences for sustainable products impact demand.
- Enhanced Visualization Tools: Advanced visualization tools will help stakeholders understand complex data and forecasts easily. Interactive dashboards will allow users to explore different forecasting scenarios and outcomes.
- Ethical AI Practices: As AI becomes more integral to demand forecasting, ethical considerations will gain prominence. Businesses will need to ensure that their AI models are fair, transparent, and free from bias.
- Integration with Supply Chain Management: AI-driven demand forecasting will be closely integrated with supply chain management systems, enabling businesses to optimize inventory levels and reduce waste based on accurate demand predictions.
- Focus on Customer Experience: Demand forecasting will increasingly prioritize enhancing the customer experience, with an emphasis on understanding customer needs to drive product development and inventory decisions.
- Adoption of Autonomous Systems: The future may see the rise of autonomous systems that can independently manage inventory and supply chain logistics based on AI forecasts, potentially leading to significant efficiency gains and cost reductions.
As AI continues to evolve, the future of demand forecasting will be characterized by greater accuracy, real-time insights, and a focus on customer-centric strategies. Businesses that embrace these trends, including AI demand forecasting trends, will be better positioned to navigate the complexities of the market and meet consumer demands effectively.
At Rapid Innovation, we are committed to helping our clients leverage these emerging trends in AI-driven demand forecasting. By partnering with us, you can expect enhanced predictive capabilities, improved ROI, and a streamlined approach to meeting your business goals. Our expertise in AI and blockchain technology ensures that you stay ahead of the curve, enabling you to make informed decisions that drive growth and efficiency. Let us help you transform your demand forecasting processes and achieve greater success in your market.
8.3. Industry-Specific Trends: How AI Will Shape Different Sectors
AI is transforming various industries by enhancing efficiency, improving decision-making, and driving innovation. Here are some key trends across different sectors:
- Healthcare: AI is used for predictive analytics to forecast patient admissions and optimize resource allocation. Machine learning algorithms analyze medical images for faster and more accurate diagnoses. Personalized medicine is on the rise, with AI helping to tailor treatments based on individual patient data.
- Retail: AI-driven demand forecasting tools, such as ai sales forecasting software and sales forecasting using ai, analyze consumer behavior and market trends to optimize inventory levels. Chatbots and virtual assistants enhance customer service, providing personalized shopping experiences. Dynamic pricing models use AI to adjust prices in real-time based on demand fluctuations.
- Manufacturing: Predictive maintenance powered by AI reduces downtime by forecasting equipment failures before they occur. AI optimizes supply chain management, improving logistics and reducing costs. Robotics and automation streamline production processes, increasing efficiency and output.
- Finance: AI algorithms detect fraudulent transactions by analyzing patterns and anomalies in real-time. Robo-advisors provide personalized investment advice based on individual financial goals and risk tolerance. Credit scoring models are enhanced with AI, allowing for more accurate assessments of borrower risk.
- Transportation: AI is integral to the development of autonomous vehicles, improving safety and efficiency on the roads. Traffic management systems use AI to optimize flow and reduce congestion in urban areas. Predictive analytics in logistics helps companies forecast delivery times and optimize routes.
8.4. The Role of Multi-Modal AI in the Future of Demand Forecasting
Multi-modal AI refers to the integration of various data types—such as text, images, and numerical data—to improve decision-making processes. In demand forecasting, this approach offers several advantages:
- Enhanced Data Integration: This combines diverse data sources, including social media sentiment, sales data, and weather patterns, providing a holistic view of factors influencing demand, leading to more accurate forecasts. AI demand forecasting and ai in demand forecasting are examples of how this integration can be applied.
- Improved Predictive Accuracy: Multi-modal models can capture complex relationships between different data types, leading to better predictions by considering various influencing factors simultaneously. AI for forecasting and ai based demand forecasting are key methodologies in this area.
- Real-Time Insights: AI systems can process and analyze data in real-time, allowing businesses to respond quickly to changing market conditions. This agility is crucial for maintaining a competitive advantage in fast-paced industries. AI powered demand forecasting tools and ai ml demand forecasting are essential for achieving this.
- Customer Behavior Analysis: By analyzing customer interactions across multiple channels, businesses can better understand preferences and trends. This insight helps in tailoring marketing strategies and inventory management, particularly through ai for demand forecasting and ai in demand planning.
- Scenario Planning: Multi-modal AI enables businesses to simulate different scenarios based on varying inputs. This capability aids in strategic planning and risk management, allowing companies to prepare for potential market shifts. Demand forecasting using ai and demand planning ai are critical components of this process.
9. Overcoming Challenges When Implementing AI for Demand Forecasting

‍
Implementing AI for demand forecasting presents several challenges that organizations must address to ensure success:
- Data Quality and Availability: High-quality, relevant data is essential for accurate forecasting. Organizations must invest in data cleaning and integration processes to ensure data reliability.
- Skill Gaps: There is often a shortage of skilled professionals who can develop and manage AI systems. Companies may need to invest in training programs or hire external experts to bridge this gap.
- Integration with Existing Systems: Integrating AI solutions with legacy systems can be complex and time-consuming. A clear strategy for integration is necessary to avoid disruptions in operations.
- Change Management: Employees may resist adopting new technologies due to fear of job displacement or lack of understanding. Effective change management strategies, including training and communication, are crucial for smooth transitions.
- Regulatory and Ethical Considerations: Organizations must navigate regulatory frameworks governing data use and AI applications. Ethical considerations, such as bias in AI algorithms, must be addressed to maintain trust and compliance.
- Cost of Implementation: The initial investment in AI technology can be significant, posing a barrier for some organizations. A clear ROI analysis can help justify the costs and guide decision-making.
- Continuous Monitoring and Improvement: AI systems require ongoing monitoring to ensure they remain effective and relevant. Organizations should establish feedback loops to continuously improve forecasting models based on new data and insights.
At Rapid Innovation, we understand these challenges and are committed to helping our clients navigate them effectively. By leveraging our expertise in AI and blockchain technology, we provide tailored solutions that enhance operational efficiency, drive innovation, and ultimately lead to greater ROI. Partnering with us means gaining access to cutting-edge technology, expert guidance, and a collaborative approach that aligns with your business goals. Together, we can transform your operations and position your organization for success in an increasingly competitive landscape.
9.1. Common Challenges in AI Forecasting (And How to Overcome Them)
- Data Quality and Availability: AI forecasting relies heavily on high-quality data. Inaccurate or incomplete data can lead to poor predictions. At Rapid Innovation, we help clients implement robust data collection and cleaning processes, ensuring that they regularly audit data sources to maintain accuracy. This proactive approach significantly enhances the reliability of forecasting outcomes, ultimately leading to greater ROI.
- Model Complexity: Many AI models can become overly complex, making them difficult to interpret and manage. Our team at Rapid Innovation simplifies models where possible and employs techniques like feature selection to focus on the most relevant data points. This not only streamlines the forecasting process but also empowers clients to make informed decisions based on clear insights.
- Overfitting: This occurs when a model learns noise in the training data rather than the underlying pattern, leading to poor performance on new data. We utilize techniques such as cross-validation and regularization to mitigate overfitting, ensuring that our clients' models remain robust and effective in real-world applications.
- Changing Environments: AI models can become outdated as market conditions or consumer behaviors change. Rapid Innovation emphasizes the importance of regularly updating models with new data and retraining them to adapt to evolving circumstances. This agility allows our clients to stay ahead of the competition and maximize their investment.
- Integration with Existing Systems: Implementing AI forecasting can be challenging if it does not integrate well with existing business processes and systems. We work closely with our clients to develop a clear strategy for integration, involving stakeholders from different departments early in the process. This collaborative approach ensures a seamless transition and enhances overall operational efficiency.
9.2. Data Privacy and Security Concerns in AI-Enabled Forecasting
- Sensitive Data Handling: AI forecasting often requires access to sensitive personal or business data, raising privacy concerns. Rapid Innovation helps clients implement strict data governance policies and ensures compliance with regulations like GDPR or CCPA. This commitment to data privacy not only protects clients but also builds trust with their customers.
- Data Breaches: The risk of data breaches increases with the use of AI, potentially exposing sensitive information. We advise clients to use encryption, secure access controls, and conduct regular security audits to protect their data. By prioritizing security, clients can safeguard their assets and maintain their reputation.
- Anonymization Techniques: While anonymizing data can help protect privacy, it may also reduce the data's usefulness for forecasting. Our experts at Rapid Innovation balance the need for privacy with data utility by employing advanced anonymization techniques that retain essential data characteristics, ensuring that clients can still derive valuable insights.
- User Consent: Obtaining user consent for data collection and usage is crucial but can be challenging. We guide clients in clearly communicating how data will be used and ensuring that consent mechanisms are user-friendly and transparent. This approach fosters a positive relationship with users and enhances data collection efforts.
- Transparency in Data Usage: Users may be unaware of how their data is being used in AI forecasting models. Rapid Innovation emphasizes the importance of providing clear information about data usage and the benefits of AI forecasting to build trust with users. This transparency not only enhances user confidence but also contributes to better data quality.
9.3. Ensuring Ethical Use of AI: Bias, Transparency, and Accountability
- Bias in AI Models: AI models can inadvertently perpetuate or amplify biases present in training data, leading to unfair outcomes. Rapid Innovation regularly audits models for bias and uses diverse datasets to train AI systems, minimizing bias and ensuring fair outcomes for all stakeholders.
- Transparency in Algorithms: Many AI algorithms operate as "black boxes," making it difficult to understand how decisions are made. We strive for transparency by using interpretable models and providing explanations for AI-driven decisions. This commitment to clarity helps clients build trust with their users and stakeholders.
- Accountability Mechanisms: Establishing accountability for AI decisions is essential to ensure responsible use. Rapid Innovation assists clients in creating clear guidelines and frameworks for accountability, including assigning responsibility for AI outcomes to specific individuals or teams. This structured approach fosters a culture of responsibility and ethical AI use.
- Stakeholder Engagement: Engaging stakeholders in the development and deployment of AI systems can help identify ethical concerns early. We encourage our clients to involve diverse groups, including ethicists, community representatives, and industry experts, in discussions about AI use. This collaborative effort ensures that ethical considerations are prioritized throughout the process.
- Continuous Monitoring and Evaluation: The ethical implications of AI can evolve over time, necessitating ongoing monitoring. Rapid Innovation implements regular evaluations of AI systems to assess their impact and make adjustments as needed to uphold ethical standards. This commitment to continuous improvement ensures that our clients remain at the forefront of ethical AI practices.
By partnering with Rapid Innovation, clients can expect not only to overcome ai forecasting challenges but also to achieve greater ROI through enhanced efficiency, security, and ethical practices in their AI and blockchain initiatives. For more information on ethical AI practices, visit our Ethical AI Development Guide.
10. How to Get Started with AI Demand Forecasting (Practical Guide)
AI demand forecasting is a powerful tool that helps businesses predict future demand for their products or services. By leveraging data and machine learning algorithms, companies can make informed decisions about inventory management, production planning, and resource allocation. Here’s a practical guide to getting started with AI demand forecasting.
10.1. Resources and Tools for AI Forecasting
To effectively implement AI demand forecasting, businesses need access to various resources and tools. Here are some essential components:
- Data Collection: Gather historical sales data, customer behavior data, and market trends from various sources, including CRM systems, ERP systems, and external market research.
- Data Cleaning and Preparation: Ensure data quality by removing duplicates, correcting errors, and filling in missing values. Normalize data to ensure consistency across different datasets.
- Machine Learning Frameworks: Utilize frameworks like TensorFlow, PyTorch, or Scikit-learn for building forecasting models. These frameworks provide libraries and tools for developing machine learning algorithms.
- Statistical Analysis Tools: Use tools like R or Python for statistical analysis and visualization, which help in understanding data patterns and trends.
- Cloud Computing Services: Leverage cloud platforms like AWS, Google Cloud, or Microsoft Azure for scalable computing resources. Cloud services can handle large datasets and complex computations efficiently.
- Collaboration Tools: Implement project management and collaboration tools like Trello or Asana to streamline team efforts. These tools help in tracking progress and ensuring effective communication among team members.
10.2. Top AI Forecasting Tools and Software for Businesses
Several AI forecasting tools and software can help businesses enhance their demand forecasting capabilities. Here are some of the top options:
- Forecast Pro: A user-friendly forecasting software that offers a range of statistical methods, ideal for businesses looking for a straightforward solution to demand forecasting.
- SAP Integrated Business Planning (IBP): A comprehensive solution that integrates demand planning with supply chain management, offering advanced analytics and machine learning capabilities for accurate forecasting.
- Microsoft Azure Machine Learning: A cloud-based platform that provides tools for building, training, and deploying machine learning models. It supports various algorithms and can be integrated with other Microsoft services.
- IBM Watson Studio: A powerful platform for data scientists and developers to build and train AI models, offering tools for data preparation, model building, and deployment.
- Google Cloud AI Platform: Provides a suite of machine learning tools and services for building custom forecasting models, integrating seamlessly with other Google Cloud services for data storage and processing.
- Tableau: A data visualization tool that helps in analyzing and presenting forecasting data, allowing users to create interactive dashboards for better decision-making.
- Anaplan: A cloud-based planning platform that supports demand forecasting and supply chain management, offering real-time data analysis and collaboration features.
- Demand Works Smoothie: A demand planning software that uses advanced algorithms for accurate forecasting, suitable for businesses of all sizes looking to improve their demand planning processes.
- Katanacuts: A tool designed for inventory management and demand forecasting, helping businesses optimize stock levels based on predicted demand.
- DataRobot: An automated machine learning platform that simplifies the model-building process, ideal for businesses that want to leverage AI without extensive data science expertise.
By utilizing the right resources and tools, including demand planning artificial intelligence software and ai demand forecasting tools, businesses can effectively implement AI demand forecasting, leading to improved accuracy in predicting customer demand and optimizing operations.
At Rapid Innovation, we specialize in guiding businesses through the complexities of AI demand forecasting. Our expertise in AI and blockchain development allows us to tailor solutions that align with your specific needs, ensuring you achieve greater ROI. By partnering with us, you can expect enhanced operational efficiency, reduced costs, and improved decision-making capabilities. Let us help you harness the power of AI to transform your demand forecasting processes and drive your business forward.
10.3. How to Choose the Right AI Forecasting Platform for Your Business
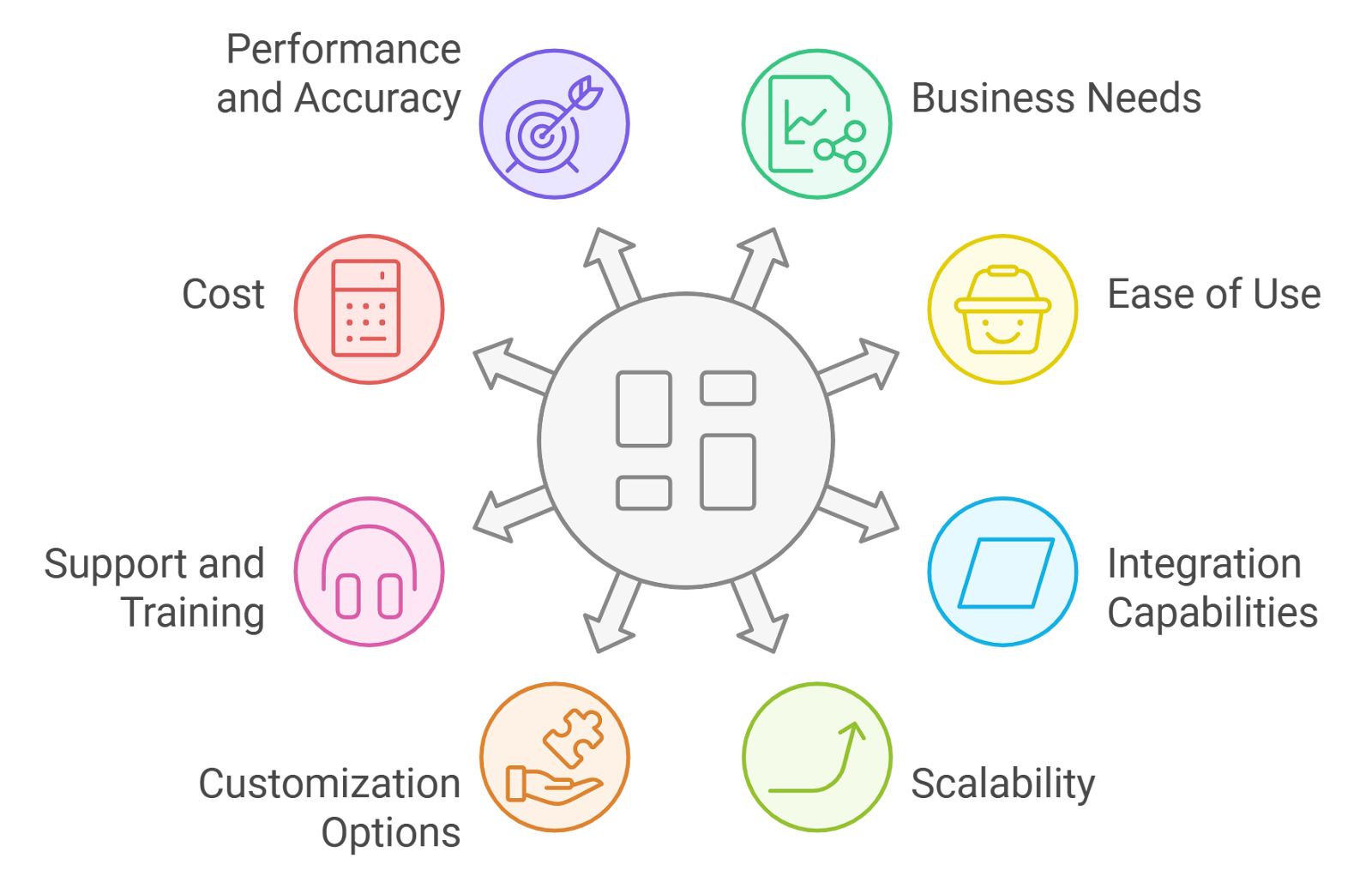
‍
Selecting the right AI forecasting platform, such as datarobot forecasting, is crucial for maximizing the benefits of AI in your business. Here are key factors to consider:
- Business Needs: Identify specific forecasting requirements and determine the types of data you will be analyzing (e.g., sales, inventory, customer behavior).
- Ease of Use: Look for user-friendly interfaces and ensure that the platform offers intuitive navigation and clear documentation.
- Integration Capabilities: Check if the platform can easily integrate with existing systems (e.g., ERP, CRM) and assess compatibility with various data sources and formats.
- Scalability: Choose a platform that can grow with your business and ensure it can handle increasing data volumes and complexity over time.
- Customization Options: Evaluate the ability to tailor the platform to your specific needs and look for features that allow for custom algorithms and models.
- Support and Training: Investigate the level of customer support provided and consider platforms that offer training resources and ongoing assistance.
- Cost: Analyze pricing structures, including subscription fees and additional costs for features, and compare the total cost of ownership against expected ROI.
- Performance and Accuracy: Research the platform’s forecasting accuracy and reliability, and look for case studies or testimonials from other businesses in your industry, especially those using an AI forecasting platform.
10.4. AI Consultation Services: Getting Expert Help for AI Implementation
Implementing AI can be complex, and consulting services can provide valuable expertise. Here’s how to approach AI consultation:
- Identify Your Goals: Clearly define what you want to achieve with AI and set measurable objectives to guide the consultation process.
- Research Potential Consultants: Look for firms with a proven track record in AI implementation and check their experience in your industry and with similar projects.
- Evaluate Expertise: Assess the qualifications and backgrounds of the consulting team to ensure they have a mix of technical skills and business acumen.
- Discuss Methodologies: Inquire about their approach to AI implementation and understand their strategies for data collection, model training, and deployment.
- Request Case Studies: Ask for examples of previous successful AI projects and evaluate the outcomes and how they align with your goals.
- Consider Ongoing Support: Determine if the consultant offers post-implementation support and look for services that include monitoring, maintenance, and updates.
- Budgeting: Discuss pricing structures and ensure they fit within your budget while considering the potential ROI when evaluating costs.
10.5. Best Practices for Successful AI Forecasting Deployment
Deploying AI forecasting effectively requires adherence to best practices. Here are essential guidelines:
- Data Quality: Ensure data is accurate, complete, and relevant. Regularly clean and update datasets to maintain integrity.
- Define Clear Objectives: Set specific, measurable goals for your forecasting efforts and align objectives with overall business strategy.
- Involve Stakeholders: Engage key stakeholders from various departments early in the process and foster collaboration to ensure buy-in and gather diverse insights.
- Start Small: Begin with pilot projects to test the AI forecasting model and use initial results to refine processes before scaling up.
- Monitor Performance: Continuously track the accuracy and effectiveness of forecasts and adjust models and strategies based on performance metrics.
- Iterate and Improve: Treat AI forecasting as an ongoing process and regularly update models and incorporate new data to enhance accuracy.
- Educate Your Team: Provide training for employees on how to use the AI forecasting tools and foster a culture of data-driven decision-making within the organization.
- Document Processes: Keep detailed records of methodologies, data sources, and model adjustments to ensure transparency and facilitate knowledge transfer within the team.
At Rapid Innovation, we understand that choosing the right AI forecasting platform, such as an ai forecasting platform, and implementing it effectively can significantly enhance your business operations. Our expertise in AI and Blockchain development allows us to guide you through this process, ensuring that you achieve greater ROI and operational efficiency. By partnering with us, you can expect tailored solutions, ongoing support, and a commitment to helping you reach your business goals effectively and efficiently.
11. Conclusion: Transforming Your Business with AI-Enabled Forecasting
AI-enabled forecasting is revolutionizing how businesses plan and make decisions. By leveraging advanced algorithms and data analytics, organizations can gain deeper insights into market trends, customer behavior, and operational efficiencies. This transformation is not just about adopting new technology; it’s about fundamentally changing how businesses operate and strategize for the future.
11.1. Key Takeaways: Why AI is Essential for Modern Business Planning
- Enhanced Accuracy: AI algorithms can analyze vast amounts of data to produce more accurate forecasts than traditional methods, leading to better decision-making and resource allocation.
- Real-Time Insights: AI systems can process data in real-time, allowing businesses to respond quickly to market changes and customer needs.
- Predictive Analytics: AI can identify patterns and trends that may not be immediately obvious, enabling proactive rather than reactive strategies.
- Cost Efficiency: By optimizing inventory and resource management, AI can help reduce operational costs and improve profit margins.
- Competitive Advantage: Companies that adopt AI forecasting can stay ahead of competitors by anticipating market shifts and customer preferences.
- Scalability: AI solutions can easily scale with business growth, accommodating increasing data volumes and complexity without a significant increase in resources.
- Improved Collaboration: AI tools can facilitate better communication and collaboration across departments by providing a unified view of data and forecasts.
11.2. Next Steps: How to Begin Your AI Forecasting Journey
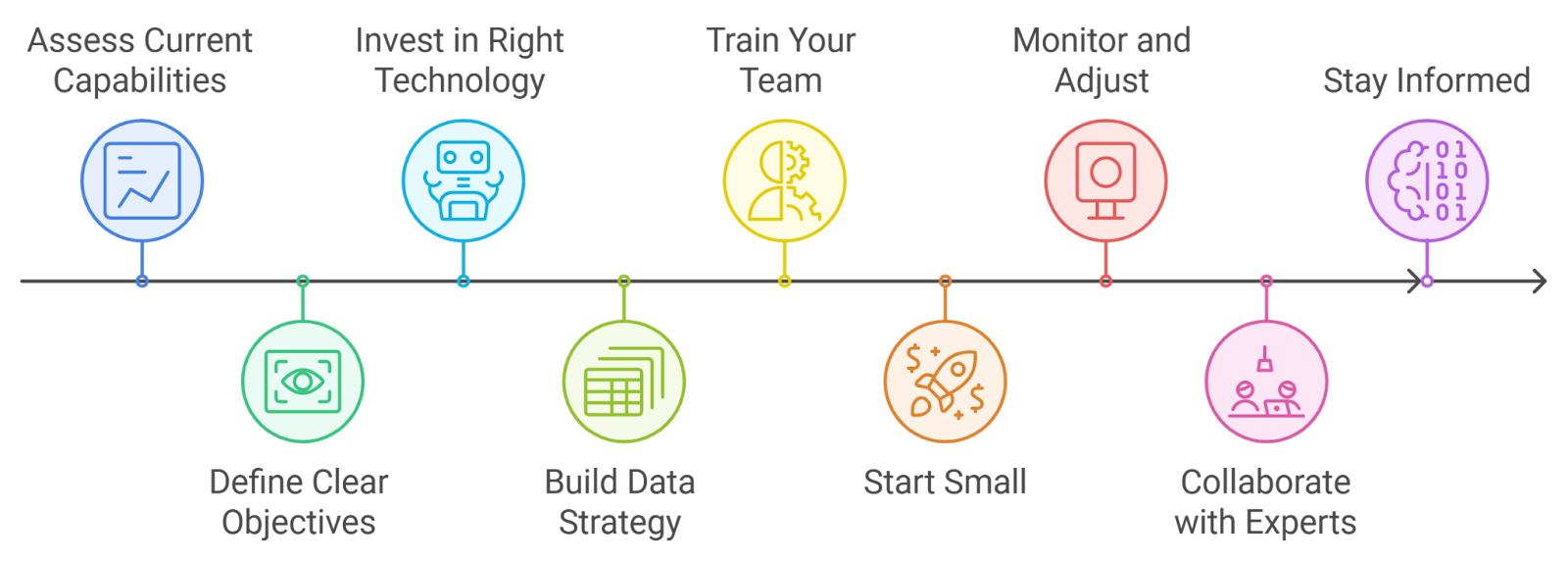
‍
- Assess Your Current Capabilities: Evaluate your existing data infrastructure and forecasting methods to identify gaps and areas for improvement.
- Define Clear Objectives: Establish what you want to achieve with AI forecasting for business, such as improved accuracy, faster decision-making, or enhanced customer insights.
- Invest in the Right Technology: Research and select AI tools that align with your business needs. Consider platforms that offer user-friendly interfaces and integration capabilities.
- Build a Data Strategy: Ensure you have access to high-quality data. This may involve cleaning existing data, integrating new data sources, and establishing data governance practices.
- Train Your Team: Provide training for your staff on how to use AI tools effectively. This will help them understand the technology and leverage it for better outcomes.
- Start Small: Begin with pilot projects to test AI forecasting in specific areas of your business. This allows you to learn and adjust before a full-scale implementation.
- Monitor and Adjust: Continuously evaluate the performance of your AI forecasting models. Use feedback and results to refine your approach and improve accuracy over time.
- Collaborate with Experts: Consider partnering with AI specialists or consultants who can provide insights and guidance throughout your journey.
- Stay Informed: Keep up with the latest trends and advancements in AI technology to ensure your forecasting methods remain relevant and effective.
At Rapid Innovation, we understand the complexities of integrating AI into your business processes. Our team of experts is dedicated to helping you navigate this journey, ensuring that you achieve greater ROI through tailored solutions that meet your specific needs. By partnering with us, you can expect enhanced operational efficiencies, improved decision-making capabilities, and a significant competitive edge in your industry. Let us help you transform your business with AI-enabled forecasting and unlock your full potential.
11.3. Call to Action: Unlock the Power of AI for Better Business Outcomes
- Embrace AI technology to enhance decision-making processes, including AI for business intelligence.
- Leverage AI tools to analyze large datasets for actionable insights, utilizing business intelligence and AI.
- Implement AI-driven solutions to improve operational efficiency, such as AI solutions for business.
- Foster a culture of innovation by encouraging teams to explore AI applications, including AI platforms for business.
- Invest in training and development to equip employees with AI skills, focusing on AI business solutions.
- Collaborate with AI experts and consultants to tailor solutions to specific business needs, including custom AI development company partnerships.
- Monitor and evaluate AI initiatives to measure their impact on business performance, particularly in business intelligence using AI.
- Stay updated on AI trends and advancements to remain competitive in the market, including insights from AI analytics companies.
12. Additional Resources and Case Studies
- Explore various platforms and publications that provide insights into AI applications in business, such as business intelligence artificial intelligence resources.
- Utilize online courses and webinars to gain a deeper understanding of AI technologies, including dynamics 365 AI.
- Read industry reports and whitepapers to stay informed about AI trends and best practices, particularly in automated business intelligence.
- Join professional networks and forums to connect with other businesses leveraging AI, including those focused on conversational business intelligence.
- Access case studies that showcase successful AI implementations across different sectors, including AI powered business intelligence examples.
- Follow thought leaders and organizations in the AI space for the latest developments and strategies, especially in AI retail companies.
12.1. Detailed Case Studies on AI Demand Forecasting
- Case Study 1: Retail Industry
A major retail chain implemented AI algorithms to analyze customer purchasing patterns, resulting in a 20% increase in inventory turnover and a 15% reduction in stockouts. They utilized machine learning models to predict seasonal demand fluctuations. - Case Study 2: Manufacturing Sector
A manufacturing company adopted AI for demand forecasting to optimize production schedules, achieving a 30% reduction in excess inventory and improved production efficiency. They integrated AI with existing ERP systems for real-time data analysis. - Case Study 3: E-commerce Platform
An e-commerce platform used AI to personalize product recommendations based on user behavior, leading to a 25% increase in conversion rates and enhanced customer satisfaction. They employed predictive analytics to forecast demand for new product launches.
At Rapid Innovation, we are committed to helping our clients harness the power of AI and Blockchain technologies to achieve their business goals efficiently and effectively. By partnering with us, you can expect greater ROI through tailored solutions that drive innovation and operational excellence. Let us guide you on your journey to unlock the full potential of AI for better business outcomes.
12.2. White Papers and eBooks on Implementing AI in Business Forecasting
At Rapid Innovation, we recognize that white papers and eBooks serve as invaluable resources for businesses looking to implement AI in business forecasting. These documents provide in-depth analysis, case studies, and practical guidance on leveraging AI technologies to enhance forecasting accuracy and efficiency.
- Definition and Purpose
White papers are authoritative reports that present a problem and propose a solution, often backed by research and data. eBooks are more comprehensive and can cover a broader range of topics, often including step-by-step guides and best practices. Both formats aim to educate stakeholders about the benefits and challenges of integrating AI into business forecasting. - Key Topics Covered  Â
- Overview of AI technologies relevant to forecasting, such as machine learning, neural networks, and natural language processing. Â
- Case studies showcasing successful AI implementations in various industries, highlighting real-world applications and outcomes. Â
- Best practices for data collection, preparation, and management to ensure high-quality inputs for AI models. Â
- Strategies for selecting the right AI tools and platforms that align with business needs and objectives. Â
- Discussion of ethical considerations and data privacy issues when using AI in forecasting.
- Benefits of Using White Papers and eBooks  Â
- Structured approach to understanding complex AI concepts and their applications in forecasting. Â
- Insights from industry experts and thought leaders, enhancing credibility and trust in the information presented. Â
- Reference for decision-makers when evaluating AI solutions and justifying investments in technology.
- How to Utilize These Resources  Â
- Identify specific forecasting challenges your business faces and seek white papers or eBooks that address those issues. Â
- Use the insights gained to develop a strategic plan for AI implementation, including timelines and resource allocation. Â
- Share findings with relevant teams to foster collaboration and ensure alignment on AI initiatives.
- Examples of Notable White Papers and eBooks  Â
- "The Future of Business Forecasting: AI and Machine Learning" - A comprehensive guide on how AI is transforming forecasting practices. Â
- "Implementing AI in Supply Chain Forecasting" - Focuses on practical steps for integrating AI into supply chain management. Â
- "Data-Driven Decision Making: Leveraging AI for Accurate Forecasting" - Discusses the importance of data quality and analytics in AI forecasting.
- Challenges and Considerations  Â
- Understanding the limitations of AI and the importance of human oversight in the forecasting process. Â
- Addressing potential resistance to change within the organization and ensuring buy-in from all stakeholders. Â
- Keeping up with the rapidly evolving AI landscape and continuously updating knowledge and skills.
- Conclusion
White papers and eBooks are essential tools for businesses aiming to implement AI in business forecasting. They provide valuable insights, practical guidance, and a roadmap for successful integration of AI technologies. By leveraging these resources, organizations can enhance their forecasting capabilities and drive better business outcomes.
At Rapid Innovation, we are committed to helping our clients navigate these resources effectively, ensuring that they achieve greater ROI through informed decision-making and strategic implementation of AI technologies, including our adaptive AI development. Partnering with us means gaining access to expert insights, tailored solutions, and a collaborative approach that drives efficiency and effectiveness in your forecasting processes. For more information on how to implement AI in your business, check out our article on adaptive artificial intelligence in business.