Table Of Contents
Category
Artificial Intelligence
IoT
Manufacturing
Security
Supply Chain & Logistics
1. Introduction to Predictive Maintenance
Predictive maintenance (PdM) is a proactive approach to maintenance that leverages data analysis and advanced technologies to predict when equipment failures might occur. This strategy aims to optimize maintenance schedules, reduce downtime, and extend the lifespan of machinery. By utilizing various data sources, including sensors and historical performance data, organizations can make informed decisions about when to perform maintenance tasks.
- Focuses on predicting equipment failures before they happen.
- Utilizes data analytics, machine learning, and IoT technologies.
- Aims to minimize unplanned downtime and maintenance costs.
1.1. Understanding Predictive Maintenance: Definition and Key Concepts
Predictive maintenance is defined as a maintenance strategy that uses data-driven insights to forecast equipment failures. The key concepts associated with predictive maintenance include:
- Data Collection: Gathering data from various sources, such as sensors, historical records, and operational logs.
- Data Analysis: Employing statistical methods and machine learning algorithms to analyze the collected data and identify patterns.
- Condition Monitoring: Continuously monitoring the health of equipment to detect anomalies and predict potential failures.
- Maintenance Scheduling: Planning maintenance activities based on predictive insights rather than fixed schedules or reactive measures.
The benefits of predictive maintenance include increased equipment reliability and availability, reduced maintenance costs by addressing issues before they escalate, and enhanced safety by minimizing the risk of equipment failure during operation.
1.2. The Shift from Traditional to AI-Enabled Maintenance Strategies
The transition from traditional maintenance strategies to AI-enabled predictive maintenance represents a significant evolution in how organizations manage their assets. Traditional maintenance approaches often rely on:
- Time-based maintenance: Performing maintenance at regular intervals, regardless of equipment condition.
- Reactive maintenance: Addressing equipment failures only after they occur, leading to unplanned downtime and higher costs.
In contrast, AI-enabled maintenance strategies offer several advantages:
- Data-Driven Insights: AI algorithms can analyze vast amounts of data to identify trends and predict failures more accurately than traditional methods.
- Real-Time Monitoring: IoT devices enable continuous monitoring of equipment, allowing for immediate detection of anomalies.
- Improved Decision-Making: AI tools provide actionable insights that help maintenance teams prioritize tasks and allocate resources effectively.
The shift to AI-enabled predictive maintenance is driven by advancements in technology, the need for operational efficiency, and competitive pressure. Organizations are increasingly seeking ways to reduce costs and improve productivity, and companies that adopt predictive maintenance can gain a competitive edge by minimizing downtime and optimizing asset performance.
At Rapid Innovation, we specialize in implementing predictive maintenance solutions, including IoT predictive maintenance and industrial predictive maintenance, tailored to your specific needs. By partnering with us, you can expect enhanced AI operational efficiency in industrial sector, reduced maintenance costs, and improved asset reliability. Our expertise in AWS predictive maintenance and SAP predictive maintenance ensures that you receive cutting-edge solutions that drive greater ROI and help you achieve your business goals effectively and efficiently. Whether you are looking for predictive maintenance platforms or specific predictive maintenance companies, we have the right solutions for you. For more information on AI in predictive maintenance, visit this link.
1.3. The Importance of Proactive Maintenance in Modern Operations

Proactive maintenance is a strategy that focuses on preventing equipment failures before they occur, rather than responding to them after the fact. This approach is increasingly vital in modern operations due to several factors:
- Cost Efficiency: Proactive maintenance can significantly reduce operational costs by minimizing downtime and extending the lifespan of equipment. According to a study, companies that implement proactive maintenance can save up to 30% on maintenance costs.
- Increased Productivity: By preventing unexpected breakdowns, organizations can maintain a steady workflow. This leads to higher productivity levels as employees can focus on their tasks without interruptions.
- Safety Improvements: Regular maintenance checks help identify potential hazards, reducing the risk of accidents. A safer work environment not only protects employees but also enhances overall morale.
- Data-Driven Decisions: Proactive maintenance often involves the use of technology and data analytics. This allows organizations to make informed decisions based on real-time data, improving the effectiveness of maintenance strategies.
- Regulatory Compliance: Many industries are subject to strict regulations regarding equipment safety and maintenance. Proactive maintenance helps ensure compliance, avoiding potential fines and legal issues.
- Customer Satisfaction: For businesses that rely on equipment to deliver products or services, maintaining operational efficiency directly impacts customer satisfaction. Fewer delays and higher quality output lead to better customer experiences.
2. Challenges of Traditional Maintenance Approaches
Traditional maintenance approaches, such as reactive and preventive maintenance, face several challenges that can hinder operational efficiency:
- Reactive Maintenance: This approach involves fixing equipment only after it fails. While it may seem cost-effective, it often leads to increased downtime, higher repair costs, and unplanned disruptions in operations.
- Preventive Maintenance: Although more proactive than reactive maintenance, preventive maintenance has its own set of challenges. Over-scheduling can lead to unnecessary downtime and wasted resources. Incomplete assessments may not address all potential failure points, leaving some equipment vulnerable. Additionally, it can be difficult to allocate the right resources for preventive maintenance, leading to inefficiencies.
- Lack of Real-Time Data: Traditional methods often rely on historical data, which may not accurately reflect current equipment conditions. This can result in missed opportunities for timely interventions.
- Resistance to Change: Organizations may be hesitant to adopt new maintenance strategies due to a lack of understanding or fear of the unknown. This resistance can slow down the transition to more effective maintenance practices.
- Skill Gaps: As technology evolves, there is often a skills gap in the workforce. Employees may not be trained to handle advanced maintenance technologies, leading to ineffective maintenance practices.
2.1. Common Issues with Reactive and Preventive Maintenance
Both reactive and preventive maintenance approaches have common issues that can impact their effectiveness:
- Unpredictable Costs: Reactive maintenance can lead to unexpected expenses due to emergency repairs and the need for expedited parts. This unpredictability can strain budgets.
- Inconsistent Performance: Preventive maintenance schedules may not align with actual equipment needs, leading to inconsistent performance and potential failures.
- Limited Visibility: Without real-time monitoring, organizations may lack visibility into equipment health, making it difficult to predict failures accurately.
- Inefficient Resource Use: Both approaches can lead to inefficient use of labor and materials. Reactive maintenance often requires urgent resource allocation, while preventive maintenance may waste resources on unnecessary tasks.
- Short-Term Focus: Reactive maintenance often prioritizes immediate fixes over long-term solutions, which can lead to recurring issues and a cycle of constant repairs.
- Data Overload: Preventive maintenance can generate a large amount of data, which can be overwhelming. Without proper analysis, this data may not provide actionable insights.
- Employee Burnout: The pressure to respond to unexpected failures in reactive maintenance can lead to employee burnout, affecting overall morale and productivity.
By understanding these challenges and issues, organizations can better appreciate the need for a shift towards more proactive maintenance strategies, such as preventive predictive and proactive maintenance, that leverage technology and data analytics for improved operational efficiency. At Rapid Innovation, we specialize in implementing these advanced strategies, including proactive maintenance strategies, ensuring that our clients not only overcome these challenges but also achieve greater ROI through enhanced operational efficiency and reduced costs. Partnering with us means gaining access to cutting-edge solutions that drive productivity, safety, and customer satisfaction, including predictive and proactive maintenance approaches. For more information on the costs associated with building AI agents that can enhance these strategies, visit this link.
Refer to the image for a visual representation of the importance of proactive maintenance in modern operations.

2.2. The Cost of Unplanned Downtime and Inefficiencies
Unplanned downtime can have significant financial and operational impacts on businesses. The costs associated with unexpected equipment failures can be staggering.
- Direct Costs: Loss of production time can lead to immediate revenue loss, and repair costs can escalate quickly, especially if emergency services are required.
- Indirect Costs: Customer dissatisfaction due to delayed deliveries can harm reputation, and increased labor costs may arise as employees need to work overtime to catch up.
- Statistics: According to a study, unplanned downtime can cost manufacturers up to $260,000 per hour. A report indicated that 82% of companies experience unplanned downtime, with an average of 2.5 hours lost per incident.
- Operational Inefficiencies: Inefficient processes can lead to wasted resources and time. Poor inventory management can result in excess stock or shortages, further complicating operations.
- Long-term Impacts: Repeated downtime can lead to a loss of market share, and companies may face increased insurance premiums due to higher risk profiles. For more insights on how to mitigate these issues, consider exploring AI-driven digital twins integrated with IoT for next-generation smart manufacturing.
2.3. Why AI Solutions are Needed in Today's Industrial Landscape
The industrial landscape is evolving rapidly, and AI solutions are becoming essential for maintaining competitiveness and efficiency.
- Data Explosion: The amount of data generated in industrial settings is growing exponentially. AI can analyze vast datasets quickly, providing actionable insights.
- Complexity of Operations: Modern manufacturing processes are increasingly complex, requiring advanced solutions for optimization. AI can help streamline operations by identifying bottlenecks and inefficiencies.
- Need for Real-time Decision Making: Businesses require real-time data to make informed decisions. AI can process data in real-time, enabling quicker responses to issues.
- Cost Reduction: Implementing AI can lead to significant cost savings by optimizing resource allocation. Predictive analytics can reduce maintenance costs by anticipating equipment failures before they occur, which is a key aspect of predictive maintenance solutions.
- Competitive Advantage: Companies that adopt AI technologies can outperform competitors who do not. AI can enhance product quality and reduce time-to-market, giving businesses an edge, especially in areas like industrial predictive maintenance.
3. The AI Advantage in Predictive Maintenance
Predictive maintenance is one of the most impactful applications of AI in industrial settings, offering numerous benefits.
- Proactive Approach: AI enables a shift from reactive to proactive maintenance strategies. By predicting failures before they happen, companies can schedule maintenance at optimal times, which is crucial for IoT predictive maintenance.
- Improved Equipment Lifespan: Regularly scheduled maintenance based on AI insights can extend the life of machinery, reducing the frequency of costly replacements and repairs.
- Data-Driven Insights: AI algorithms analyze historical data to identify patterns and predict future failures, leading to more accurate maintenance schedules and reduced downtime, a significant advantage of predictive maintenance platforms.
- Cost Efficiency: Predictive maintenance can lower maintenance costs by up to 30%. It minimizes the need for unnecessary routine maintenance, saving time and resources, which is a primary goal of predictive maintenance companies.
- Enhanced Safety: By predicting equipment failures, AI can help prevent accidents and injuries. A safer work environment can lead to lower insurance costs and improved employee morale.
- Integration with IoT: AI works seamlessly with IoT devices to gather real-time data from machinery, enhancing the accuracy of predictive maintenance models, particularly in the context of IoT for predictive maintenance.
- Scalability: AI solutions can be scaled across multiple facilities, providing consistent maintenance strategies. This allows companies to maintain high operational standards across all locations, which is essential for industrial IoT predictive maintenance.
At Rapid Innovation, we understand the challenges businesses face in today's fast-paced industrial environment. By leveraging our expertise in AI and blockchain technologies, we can help you minimize unplanned downtime, enhance operational efficiency, and ultimately achieve greater ROI. Partnering with us means you can expect tailored solutions that not only address your immediate needs but also position your business for long-term success, including advanced solutions like AWS predictive maintenance and SAP predictive maintenance.
Refer to the image for a visual representation of the costs associated with unplanned downtime and inefficiencies in industrial settings:

At Rapid Innovation, we understand that the integration of AI technologies into maintenance practices, such as ai predictive maintenance and predictive maintenance ai, is not just a trend; it is a transformative approach that can significantly enhance operational efficiency and drive greater ROI for our clients.
3.1. How AI Transforms Maintenance Practices
AI technologies are revolutionizing maintenance practices across various industries. By implementing predictive maintenance, we utilize AI algorithms to analyze data from machinery and equipment, allowing our clients to stay ahead of potential issues. For instance, our machine learning models can identify patterns and predict failures before they occur, enabling proactive measures that minimize downtime. This is particularly relevant in applications of ai for predictive maintenance.
Moreover, AI can automate routine maintenance tasks, reducing human error and increasing efficiency. With real-time monitoring through AI systems, our clients can respond immediately to potential issues, ensuring that operations run smoothly. AI-driven maintenance optimizes scheduling, ensuring that maintenance is performed only when necessary, which translates to significant cost savings. This is where ai based predictive maintenance comes into play.
Enhanced decision-making is another key benefit we provide. Our AI solutions offer insights that help prioritize maintenance tasks, allowing organizations to allocate resources effectively. Additionally, AI can integrate seamlessly with IoT devices, creating a connected ecosystem that improves overall maintenance strategies, including ai preventive maintenance.
3.2. The Role of Data in AI-Driven Maintenance
Data is the backbone of AI-driven maintenance strategies. At Rapid Innovation, we emphasize the importance of large volumes of data from sensors, historical maintenance records, and operational metrics. We ensure that data quality is paramount; accurate and relevant data leads to better predictive models. Our AI algorithms require continuous data input to improve their accuracy over time, and we leverage data analytics to identify trends and anomalies that may indicate potential failures, which is essential for predictive maintenance using ai.
The integration of structured and unstructured data enhances the AI's ability to make informed predictions. We also utilize data visualization tools to present insights in an easily digestible format for maintenance teams, empowering them to make informed decisions. Organizations must invest in robust data management systems, and we are here to guide them through this process effectively.
3.3. Key Benefits of AI-Powered Predictive Maintenance

When partnering with Rapid Innovation, our clients can expect a multitude of benefits from AI-powered predictive maintenance:
- Reduced downtime: Our AI solutions predict equipment failures, allowing for timely interventions that keep operations running smoothly, which is a key aspect of ai maintenance.
- Cost savings: By preventing unexpected breakdowns, organizations can save on repair costs and lost productivity, leading to a healthier bottom line. This is particularly relevant for predictive maintenance ml.
- Increased equipment lifespan: Regular, data-driven maintenance extends the life of machinery, maximizing asset utilization, a crucial factor in ai predictive maintenance manufacturing.
- Enhanced safety: Predictive maintenance reduces the risk of accidents caused by equipment failure, fostering a safer work environment.
- Improved resource allocation: Maintenance teams can focus on high-priority tasks based on AI insights, optimizing workforce efficiency.
- Better inventory management: Our AI can forecast parts needs, reducing excess inventory and associated costs, which streamlines operations.
- Higher operational efficiency: Streamlined maintenance processes lead to smoother operations, allowing organizations to focus on their core business objectives.
- Competitive advantage: Organizations leveraging ai in maintenance can outperform competitors who rely on traditional methods, positioning themselves as leaders in their respective industries, especially in the context of c3 ai predictive maintenance.
By choosing Rapid Innovation as your partner in AI and blockchain development, you are not just investing in technology; you are investing in a future where your organization can achieve its goals efficiently and effectively. Let us help you unlock the full potential of AI-driven maintenance strategies, including applications of ai for predictive maintenance, and drive greater ROI for your business. For more insights, check out our article on AI-driven digital twins revolutionizing industries.
3.3.1. Cost Reduction and Increased ROI
- Predictive maintenance leverages data analytics to anticipate equipment failures before they occur, allowing organizations to take proactive measures.
- By identifying potential issues early, organizations can schedule maintenance during non-peak hours, effectively minimizing downtime and maximizing productivity.
- This proactive approach significantly reduces the frequency of emergency repairs, which are often more costly and disruptive to operations.
- Companies can save significantly on labor costs by optimizing maintenance schedules, ensuring that resources are allocated efficiently.
- Reduced equipment failure not only leads to lower replacement costs but also extends the lifespan of machinery, providing long-term financial benefits.
- According to a study, predictive maintenance can reduce maintenance costs by 10-40% and increase ROI by up to 25% (source: Deloitte), demonstrating the tangible financial advantages of this strategy.
- Improved operational efficiency translates to better resource allocation and increased productivity, allowing organizations to focus on their core business objectives.
- The integration of IoT predictive maintenance solutions enhances the ability to monitor equipment health in real-time, further driving cost reduction and increased ROI.
3.3.2. Improved Equipment Reliability and Lifespan
- Predictive maintenance enhances equipment reliability by continuously monitoring performance metrics, ensuring that machinery operates at peak efficiency.
- Advanced algorithms analyze data from sensors to detect anomalies that may indicate potential failures, allowing for timely interventions.
- Early detection of issues prevents catastrophic breakdowns, safeguarding both equipment and personnel.
- Regular maintenance based on predictive insights ensures that equipment operates at optimal levels, further enhancing reliability.
- This approach not only improves reliability but also extends the lifespan of machinery, leading to significant cost savings over time.
- Equipment that is well-maintained can operate more efficiently, resulting in energy savings and reduced operational costs.
- Studies show that organizations implementing predictive maintenance can see a 20-25% increase in equipment lifespan (source: McKinsey), highlighting the long-term benefits of this strategy.
- Enhanced reliability fosters a safer work environment, reducing the risk of accidents caused by equipment failure and promoting employee well-being.
4. How AI-Powered Predictive Maintenance Works
- AI-powered predictive maintenance utilizes machine learning algorithms to analyze historical and real-time data, providing organizations with actionable insights.
- Data is collected from various sources, including sensors, maintenance logs, and operational metrics, creating a comprehensive view of equipment health.
- The AI system identifies patterns and trends that indicate the health of equipment, enabling organizations to make informed decisions.
- Predictive models are developed to forecast when maintenance should be performed based on the likelihood of failure, ensuring timely interventions.
- These models continuously learn and improve as more data is fed into the system, increasing accuracy over time and enhancing decision-making capabilities.
- Alerts and notifications are generated to inform maintenance teams of potential issues before they escalate, allowing for proactive management.
- Integration with existing maintenance management systems, such as IBM Maximo predictive maintenance and SAP predictive maintenance, streamlines workflows and enhances decision-making, making it easier for organizations to implement predictive maintenance strategies.
- The use of AI allows for more sophisticated analysis, enabling organizations to transition from reactive to proactive maintenance strategies, ultimately leading to greater efficiency and ROI.
By partnering with Rapid Innovation, clients can leverage our expertise in AI and blockchain technology to implement predictive maintenance solutions, including IoT for predictive maintenance, that drive cost reduction, improve equipment reliability, and enhance overall operational efficiency. Our tailored approach ensures that organizations achieve their goals effectively and efficiently, resulting in a significant return on investment.
4.1. Data Collection: Essential Sources and Technologies
Data collection is a critical component of any data-driven strategy. It involves gathering information from various sources to inform decision-making, improve processes, and enhance customer experiences. The effectiveness of data collection relies on the technologies and methods employed to gather and analyze data, including various data collection strategies.
4.1.1. Utilizing IoT Sensors for Real-Time Insights
The Internet of Things (IoT) has revolutionized data collection by enabling real-time monitoring and analysis. IoT sensors are devices that collect data from their environment and transmit it for processing.
- Real-time data acquisition: IoT sensors provide immediate insights, allowing organizations to respond quickly to changes or anomalies.
- Diverse applications: These sensors can be used in various sectors, including agriculture (monitoring soil moisture and crop health), manufacturing (tracking equipment performance), and healthcare (monitoring patient vitals).
- Enhanced decision-making: With real-time data, businesses can make informed decisions that improve operational efficiency and customer satisfaction.
- Cost-effectiveness: IoT sensors can reduce the need for manual data collection, saving time and labor costs.
- Scalability: Organizations can easily scale their IoT networks to include more sensors as their data needs grow.
4.1.2. Analyzing Historical Data for Predictive Insights
Historical data analysis involves examining past data to identify trends, patterns, and correlations that can inform future predictions. This method is essential for organizations looking to anticipate market changes and customer behavior.
- Trend identification: By analyzing historical data, businesses can identify long-term trends that may influence future performance.
- Predictive analytics: Techniques such as regression analysis and machine learning can be applied to historical data to forecast future outcomes. For more information on predictive analytics, visit Predictive Analytics.
- Risk management: Historical data can help organizations assess risks and develop strategies to mitigate them.
- Customer behavior insights: Analyzing past customer interactions can reveal preferences and behaviors, allowing for targeted marketing and improved customer service.
- Performance benchmarking: Historical data provides a baseline for measuring current performance against past results, helping organizations set realistic goals.
Incorporating both IoT sensors for real-time insights and historical data analysis creates a comprehensive data collection strategy that enhances decision-making and drives business success.
At Rapid Innovation, we leverage these advanced data collection techniques, including market research data collection methods and customer data collection strategy, to help our clients achieve greater ROI. By implementing IoT solutions, we enable businesses to gain immediate insights that lead to faster decision-making and improved operational efficiency. Our expertise in historical data analysis allows clients to anticipate market trends and customer behaviors, ensuring they stay ahead of the competition.
When you partner with us, you can expect enhanced data-driven strategies, cost savings through automation, and a scalable approach that grows with your business needs. Let us help you transform your data collection processes into a powerful tool for success, utilizing effective data gathering strategies and data collection tactics.
4.2. AI and Machine Learning in Maintenance Analytics
AI and machine learning (ML) are transforming maintenance analytics by enabling organizations to predict failures, optimize maintenance schedules, and enhance overall operational efficiency. These technologies analyze vast amounts of data to identify patterns and anomalies that human analysts might miss. The benefits include:
- Improved decision-making through data-driven insights.
- Enhanced predictive capabilities leading to reduced downtime.
- Cost savings by optimizing maintenance resources.
4.2.1. Pattern Detection and Failure Prediction
Pattern detection involves analyzing historical data to identify trends and correlations that can indicate potential failures. Machine learning algorithms can learn from past incidents and predict future equipment behavior. Key aspects include:
- Historical data analysis: This utilizes data from sensors, maintenance logs, and operational records to identify recurring issues and their root causes.
- Predictive modeling: Algorithms like regression analysis and neural networks forecast potential failures. Models can be trained on specific equipment types or operational conditions, such as those used in sap predictive maintenance and azure predictive maintenance.
- Benefits of pattern detection: Early identification of potential failures allows for proactive maintenance, leading to a reduction in unplanned downtime and associated costs, as well as improved asset lifespan through timely interventions. Companies like IBM predictive maintenance and Oracle predictive maintenance are at the forefront of these advancements.
- Real-world applications: Industries such as manufacturing, aviation, and energy are leveraging these techniques. Companies report up to a 30% reduction in maintenance costs through predictive analytics, with top predictive maintenance companies like Deloitte and Aveva predictive maintenance leading the way.
4.2.2. Real-Time Anomaly Detection and Alerts
Real-time anomaly detection focuses on continuously monitoring equipment and systems to identify deviations from normal operating conditions. This capability allows organizations to respond swiftly to potential issues before they escalate. Important components include:
- Continuous monitoring: This utilizes IoT sensors and data streams to track equipment performance and analyzes data in real-time to detect unusual patterns or behaviors, similar to the approaches used in splunk predictive maintenance.
- Machine learning algorithms: These employ techniques like clustering and classification to identify anomalies. Algorithms can adapt and improve over time as they learn from new data, as seen in uptake predictive maintenance and dataiku predictive maintenance.
- Alert systems: Automated alerts notify maintenance teams of detected anomalies, which can be prioritized based on severity, enabling efficient resource allocation.
- Advantages of real-time detection: Immediate response to potential failures minimizes downtime, enhances safety by identifying hazardous conditions before they lead to accidents, and improves operational efficiency through timely interventions.
- Industry impact: Companies using real-time anomaly detection have reported up to a 50% reduction in equipment failures, leading to enhanced customer satisfaction due to improved service reliability and performance.
In conclusion, AI and machine learning are revolutionizing maintenance analytics through pattern detection and real-time anomaly detection. These technologies empower organizations to make informed decisions, reduce costs, and enhance operational efficiency. By partnering with Rapid Innovation, clients can leverage these advanced solutions to achieve greater ROI and drive their business success. Our expertise in AI and blockchain development ensures that you receive tailored solutions that meet your specific needs, ultimately leading to improved operational performance and strategic growth. For more information on real-time anomaly detection, visit this link.
4.3. Key Components of an AI-Enabled Predictive Maintenance System
An AI-enabled predictive maintenance system leverages advanced technologies to anticipate equipment failures and optimize maintenance schedules. The key components of such a system include data collection, machine learning algorithms, visualization tools, and integration with existing processes.
4.3.1. Visualization Tools for Enhanced Decision-Making
Visualization tools play a crucial role in predictive maintenance by transforming complex data into understandable formats. These tools help stakeholders make informed decisions based on real-time insights. Effective visualization tools not only improve understanding but also foster collaboration among teams, leading to quicker and more accurate decision-making.
- Dashboards: Provide a comprehensive view of equipment health and performance metrics, allowing users to monitor key performance indicators (KPIs) at a glance.
- Graphs and Charts: Illustrate trends over time, such as equipment wear and tear, and help identify patterns that may indicate potential failures.
- Heat Maps: Visualize areas of concern within machinery or systems, highlighting components that require immediate attention based on their condition.
- Alerts and Notifications: Enable proactive responses to potential issues and ensure that maintenance teams are informed of critical changes in equipment status.
- User-Friendly Interfaces: Simplify the interaction with complex data sets and enhance accessibility for non-technical users, allowing broader team involvement in decision-making.
4.3.2. Integration with Existing Maintenance Processes
Integrating predictive maintenance systems with existing maintenance processes is essential for maximizing their effectiveness. This integration ensures that predictive insights are seamlessly incorporated into daily operations.
- Data Synchronization: Aligns data from various sources, such as sensors and historical maintenance records, ensuring that all stakeholders have access to the same information.
- Workflow Automation: Streamlines maintenance tasks based on predictive insights and reduces manual intervention, minimizing the risk of human error.
- Collaboration with Maintenance Teams: Encourages communication between predictive maintenance systems and on-ground teams, facilitating the sharing of insights and recommendations for maintenance actions.
- Feedback Loops: Incorporates feedback from maintenance activities back into the predictive model, enhancing the accuracy of predictions over time by learning from past actions.
- Training and Support: Provides necessary training for staff to adapt to new tools and processes, ensuring that teams are equipped to leverage predictive maintenance effectively.
Integrating predictive maintenance with existing processes not only enhances operational efficiency but also fosters a culture of continuous improvement within organizations.
At Rapid Innovation, we understand the importance of these components in driving greater ROI for our clients. By implementing AI-enabled predictive maintenance systems, including solutions like cmms predictive maintenance, predictive maintenance software system, and predictive maintenance platform, we help organizations reduce downtime, lower maintenance costs, and improve asset longevity. Our expertise in AI and blockchain technology ensures that our clients receive tailored solutions that align with their specific operational needs, such as sap predictive maintenance and oracle predictive maintenance. Partnering with us means gaining access to cutting-edge tools and strategies, including predictive maintenance using IoT and IBM Maximo predictive maintenance, that empower your teams to make data-driven decisions, ultimately leading to enhanced productivity and profitability. For more insights on how AI is transforming industries, check out our article on revolutionizing industries with AI-driven digital twins and multimodal learning in 2024.
5. Implementing AI-Enabled Predictive Maintenance: A Step-by-Step Guide
5.1. Phase 1: Assessment and Planning
5.1.1. Evaluating Business Needs and Current Maintenance Practices
- Understand the objectives of predictive maintenance implementation: The primary goals are to reduce downtime and maintenance costs, improve equipment reliability and lifespan, and enhance overall operational efficiency. By aligning these objectives with your business goals, we can help you achieve a greater return on investment (ROI).
- Conduct a thorough assessment of current maintenance practices: This involves identifying existing maintenance strategies (reactive, preventive, predictive), analyzing historical maintenance data to determine patterns and trends, and evaluating the effectiveness of current practices in meeting business goals. Our expertise in data analytics allows us to uncover insights that can lead to more effective maintenance strategies.
- Engage stakeholders to gather insights: Involve maintenance teams, operators, and management in discussions to collect feedback on pain points and areas for improvement. Ensuring alignment of predictive maintenance implementation goals with overall business objectives is crucial, and our consulting services can facilitate these discussions to ensure all voices are heard.
- Identify critical assets and failure modes: Determine which equipment is vital to operations and has the highest failure rates. Use failure mode and effects analysis (FMEA) to prioritize assets based on risk, focusing on those that significantly impact production and safety. Our team can assist in identifying these critical assets, ensuring that your resources are allocated effectively.
- Assess data availability and quality: Review existing data sources, such as sensors, logs, and maintenance records. Evaluate the quality and completeness of the data for predictive analytics, and identify gaps in data collection that may hinder predictive maintenance implementation efforts. Our expertise in AI and data management ensures that you have the right data to drive your predictive maintenance initiatives.
- Define key performance indicators (KPIs): Establish metrics to measure the success of predictive maintenance implementation initiatives. Common KPIs include mean time between failures (MTBF), maintenance costs, and equipment availability. We can help you define KPIs that align with your business objectives and ensure they can be tracked over time for continuous improvement.
- Develop a roadmap for implementation: Outline the steps needed to transition from current practices to AI-enabled predictive maintenance implementation. Set realistic timelines and milestones for each phase of the implementation, and allocate resources, including budget and personnel, to support the initiative. Our structured approach to implementation ensures that you stay on track and achieve your goals efficiently.
- Consider potential challenges and risks: Identify barriers to implementation, such as resistance to change or lack of expertise. Develop strategies to mitigate risks, including training and change management, and prepare for potential technical challenges related to data integration and AI model development. Our team is equipped to guide you through these challenges, ensuring a smooth transition.
- Research industry best practices and case studies: Look for successful implementations of predictive maintenance in similar industries. Analyze lessons learned and strategies that contributed to their success, using this information to inform your own implementation strategy. Leveraging our extensive knowledge of industry best practices can help you avoid common pitfalls and accelerate your success.
- Create a communication plan: Develop a strategy for communicating the benefits and progress of predictive maintenance implementation to all stakeholders. Ensure transparency and encourage feedback throughout the implementation process, highlighting success stories and quick wins to build momentum and support for the initiative. Our communication strategies can help foster a culture of collaboration and innovation within your organization.
By partnering with Rapid Innovation, you can expect to achieve greater ROI through enhanced operational efficiency, reduced maintenance costs, and improved equipment reliability. Our expertise in AI and blockchain development ensures that you have the tools and strategies needed to succeed in today's competitive landscape. Let us help you transform your maintenance practices and drive your business forward. For more information on maintaining best practices, visit this link.
5.2. Phase 2: Technology Selection and Infrastructure Setup
In this phase, organizations focus on selecting the appropriate technologies and setting up the necessary infrastructure to support their IoT and AI initiatives. This involves careful consideration of various components that will ensure the system operates efficiently and effectively.
- Assess organizational needs and goals.
- Evaluate existing infrastructure for compatibility.
- Identify potential technology partners and vendors.
- Consider scalability and future growth.
- Ensure compliance with industry standards and regulations.
5.2.1. Choosing the Right IoT Sensors and AI Platforms
Selecting the right IoT sensors and AI platforms is crucial for the success of any IoT project. The choice of sensors and platforms can significantly impact data quality, processing capabilities, and overall system performance.
- Identify the specific use case and requirements.
- Evaluate sensor types based on:
- Accuracy
- Range
- Power consumption
- Cost
- Consider the integration capabilities of sensors with existing systems.
- Research AI platforms that offer:
- Robust data processing capabilities
- Machine learning algorithms suitable for the application
- User-friendly interfaces for model training and deployment.
- Look for platforms that support:
- Real-time data analytics
- Scalability to handle increasing data volumes
- Security features to protect sensitive information.
5.3. Phase 3: AI Model Development and Deployment
Once the technology and infrastructure are in place, the next step is to develop and deploy AI models that will analyze the data collected from IoT sensors. This phase is critical for transforming raw data into actionable insights.
- Define the objectives of the AI model.
- Collect and preprocess data from IoT sensors.
- Choose appropriate machine learning algorithms based on:
- Data characteristics
- Desired outcomes
- Train the model using historical data.
- Validate the model to ensure accuracy and reliability.
- Deploy the model in a production environment.
- Monitor model performance and make adjustments as necessary.
- Implement feedback loops to continuously improve the model based on new data and insights.
By following these structured phases, organizations can effectively leverage IoT technology selection and AI technologies to enhance their operations and achieve their strategic goals. At Rapid Innovation, we specialize in guiding our clients through these phases, ensuring that they not only select the right technologies but also maximize their return on investment. Our expertise in AI and blockchain development allows us to tailor solutions that align with your specific business objectives, ultimately driving efficiency and effectiveness in your operations. Partnering with us means you can expect enhanced data-driven decision-making, improved operational efficiency, and a competitive edge in your industry.
5.3.1. Training and Validating AI Models for Predictive Maintenance
- Data Collection: Gather historical data from machinery and equipment, including sensor data, maintenance logs, and operational parameters. Ensure data is clean, relevant, and comprehensive. This data is crucial for ai predictive maintenance and ai for predictive maintenance applications.
- Feature Engineering: Identify key features that influence equipment performance and create new variables that can enhance model accuracy. Use domain knowledge to select features that matter most, particularly those relevant to ai based predictive maintenance.
- Model Selection: Choose appropriate algorithms based on the problem type (e.g., regression, classification). Common models include decision trees, random forests, and neural networks. Consider ensemble methods for improved performance, especially in ai predictive maintenance manufacturing.
- Training the Model: Split data into training and validation sets. Use the training set to teach the model patterns in the data and monitor performance metrics like accuracy, precision, and recall. This is essential for effective predictive maintenance using ai.
- Validation Techniques: Employ cross-validation to ensure model robustness. Use techniques like k-fold cross-validation to assess performance on different data subsets and validate against unseen data to test generalization, which is vital for ai and predictive maintenance.
- Hyperparameter Tuning: Optimize model parameters to enhance performance using grid search or random search methods for tuning. Evaluate the impact of changes on model accuracy, particularly in the context of machine learning and predictive maintenance.
- Performance Evaluation: Assess model performance using metrics such as F1 score, ROC-AUC, and confusion matrix. Compare results against baseline models to ensure improvements and iterate on model design based on evaluation results, focusing on ai preventive maintenance.
- Deployment Considerations: Prepare the model for integration into existing systems, ensuring compatibility with data pipelines and operational workflows. Plan for ongoing monitoring and maintenance of the model, which is crucial for ai maintenance.
5.4. Phase 4: Continuous Improvement and Scaling AI Solutions

- Feedback Loops: Establish mechanisms for continuous feedback from users and systems. Use feedback to refine models and improve predictions, regularly updating the model with new data to enhance accuracy, especially in applications of ai for predictive maintenance.
- Performance Monitoring: Continuously track model performance in real-time. Set up alerts for significant deviations from expected outcomes and use dashboards to visualize key performance indicators (KPIs), which is essential for predictive maintenance ml.
- Scalability Planning: Assess infrastructure needs for scaling AI solutions. Consider cloud-based solutions for flexibility and resource management, ensuring that the architecture can handle increased data loads and user demands, particularly in c3 ai predictive maintenance.
- Cross-Department Collaboration: Foster collaboration between IT, operations, and maintenance teams. Share insights and data across departments to enhance predictive capabilities and encourage a culture of innovation and experimentation, leveraging ai in predictive maintenance.
- Training and Development: Invest in training programs for staff to understand AI tools and methodologies. Encourage continuous learning to keep up with advancements in AI technology and promote knowledge sharing within the organization, particularly in the context of artificial intelligence maintenance.
- Iterative Development: Adopt agile methodologies for ongoing development and improvement. Regularly revisit and refine AI models based on new insights and data, implementing a cycle of testing, learning, and adapting, which is crucial for ai helps manufacturing with quality checks and predicting maintenance costs.
- Integration with Other Technologies: Explore synergies with IoT, big data, and cloud computing. Leverage these technologies to enhance data collection and analysis, creating a holistic approach to predictive maintenance, including predictive maintenance edge ai.
6. Real-World Applications of AI-Powered Predictive Maintenance
- Manufacturing: AI models predict equipment failures, reducing downtime. Companies like Siemens use AI to optimize maintenance schedules, and predictive analytics helps in managing supply chains effectively, showcasing the benefits of ai predictive maintenance.
- Energy Sector: Utilities employ AI to monitor and maintain power generation equipment. Predictive maintenance reduces outages and improves reliability, with companies like GE using AI to analyze turbine performance data, demonstrating the effectiveness of ai for predictive maintenance.
- Transportation: Airlines utilize AI to predict aircraft maintenance needs, helping in scheduling repairs and minimizing delays. Rail companies use AI to monitor track conditions and prevent accidents, highlighting the role of ai in maintenance.
- Healthcare: Hospitals apply AI to maintain medical equipment and devices, ensuring critical equipment is always operational. AI also helps in scheduling preventive maintenance for imaging machines, illustrating the applications of ai preventive maintenance.
- Automotive: Car manufacturers use AI to monitor vehicle performance in real-time, alerting drivers to potential issues before they escalate. Companies like Tesla leverage AI for over-the-air updates and diagnostics, showcasing the impact of ai predictive maintenance manufacturing.
- Oil and Gas: AI models predict equipment failures in drilling and extraction processes, enhancing safety and operational efficiency. Companies like BP use AI to analyze sensor data from offshore rigs, demonstrating the importance of predictive maintenance using ai.
- Smart Buildings: AI systems monitor HVAC and electrical systems for maintenance needs, improving energy efficiency and occupant comfort. Building management systems utilize AI to optimize operations, reflecting the benefits of ai based predictive maintenance.
At Rapid Innovation, we understand that the integration of AI and blockchain technologies can significantly enhance operational efficiency and drive greater ROI for our clients. By leveraging our expertise in training and validating AI models for predictive maintenance, we empower businesses to minimize downtime, optimize resource allocation, and ultimately achieve their strategic goals.
When you partner with us, you can expect:
- Tailored Solutions: We customize our AI and blockchain solutions to meet your specific business needs, ensuring that you receive the most relevant and effective tools for your operations.
- Expert Guidance: Our team of experienced professionals provides ongoing support and consultation, helping you navigate the complexities of AI and blockchain technologies.
- Increased Efficiency: By implementing predictive maintenance strategies, we help you reduce operational costs and improve equipment reliability, leading to enhanced productivity.
- Scalability: Our solutions are designed with scalability in mind, allowing your business to grow and adapt to changing market conditions without compromising performance.
- Continuous Improvement: We emphasize a culture of continuous learning and improvement, ensuring that your AI models evolve with your business and the industry landscape.
By choosing Rapid Innovation, you are not just investing in technology; you are investing in a partnership that prioritizes your success and drives measurable results. Let us help you unlock the full potential of AI and blockchain to achieve your business objectives efficiently and effectively. For more information on building AI applications, check out this step-by-step guide.
6.1. Manufacturing: Optimizing Production Lines and Reducing Waste
- Lean Manufacturing Principles: At Rapid Innovation, we help clients implement lean principles that identify and eliminate waste in production processes. By reducing excess inventory, minimizing waiting times, and streamlining workflows, our clients can achieve significant cost savings and improved overall equipment efficiency.
- Automation and Technology: Our expertise in automation technologies, including robotics and AI, enables manufacturers to enhance production efficiency. These technologies perform repetitive tasks faster and with greater precision, leading to reduced cycle times and lower error rates, ultimately driving greater ROI.
- Data Analytics: We leverage data analytics to empower manufacturers to monitor production lines in real-time. By analyzing data, our clients can identify bottlenecks, predict maintenance needs, and optimize resource allocation, resulting in improved operational performance and manufacturing efficiency.
- Continuous Improvement: We foster a culture of continuous improvement within organizations, encouraging employees to suggest enhancements to processes. By employing techniques like Six Sigma, we systematically reduce defects and improve quality, leading to higher customer satisfaction and loyalty, as well as manufacturing efficiency improvement.
- Sustainable Practices: Our commitment to sustainable manufacturing practices not only reduces waste but also enhances brand reputation. We guide clients in recycling materials, reducing energy consumption, and minimizing emissions, which can lead to cost savings and a positive public image, contributing to energy efficiency in manufacturing.
6.2. Energy and Utilities: Enhancing Grid Reliability and Efficiency
- Smart Grid Technology: Rapid Innovation specializes in integrating smart grid technology, allowing for real-time monitoring and management of energy distribution. This leads to improved reliability and the ability to quickly respond to outages, ensuring a more stable energy supply for our clients.
- Demand Response Programs: We assist utilities in implementing demand response programs that incentivize consumers to reduce or shift their energy usage during peak periods. This helps balance supply and demand, ultimately enhancing grid efficiency and reducing operational costs.
- Renewable Energy Integration: Our team supports the incorporation of renewable energy sources, such as solar and wind, into the grid. This transition not only improves sustainability but also enhances energy security, providing clients with a competitive edge in the market.
- Energy Storage Solutions: We offer advanced energy storage technologies, like batteries, that enable the storage of excess energy generated during low demand periods. This stored energy can be utilized during peak demand, improving overall grid reliability and efficiency.
- Predictive Maintenance: Utilizing predictive analytics, we help utilities anticipate equipment failures before they occur. This proactive approach minimizes downtime and ensures a more reliable energy supply, ultimately leading to cost savings and improved service delivery.
6.3. Transportation and Logistics: Improving Fleet Management

- Route Optimization: Our route optimization software significantly reduces fuel consumption and travel time for clients. By analyzing traffic patterns and road conditions, we determine the most efficient routes, leading to lower operational costs and increased profitability.
- Fleet Tracking Systems: We provide real-time tracking systems that offer visibility into fleet operations. This allows managers to monitor vehicle locations, driver behavior, and maintenance needs, leading to improved efficiency and reduced costs.
- Preventive Maintenance: We help clients establish a preventive maintenance schedule that identifies potential issues before they escalate. Regular maintenance reduces the risk of breakdowns and extends the lifespan of vehicles, resulting in lower total cost of ownership.
- Driver Training Programs: Investing in our driver training programs enhances safety and fuel efficiency. Educated drivers are more likely to adopt eco-friendly driving habits, which can lead to reduced operational costs and improved company reputation.
- Sustainability Initiatives: We assist clients in implementing green logistics practices, such as using alternative fuels and optimizing load capacities. This not only reduces the carbon footprint of transportation operations but also enhances the company's image as a responsible corporate citizen.
By partnering with Rapid Innovation, clients can expect to achieve greater ROI through enhanced efficiency, reduced costs, and improved sustainability across their operations. Our expertise in AI and blockchain development ensures that we provide tailored solutions that align with your business goals, including improving efficiency in manufacturing and increasing manufacturing efficiency.
6.4. Oil and Gas: Ensuring Safety and Compliance in Operations
- The oil and gas industry operates in a high-risk environment, making oil and gas safety compliance critical. At Rapid Innovation, we understand the complexities of this sector and offer tailored solutions that enhance safety protocols and ensure compliance with regulatory frameworks such as OSHA and EPA.
- Regulatory frameworks, such as OSHA and EPA, set standards for safety practices and environmental protection. Our consulting services help clients navigate these regulations effectively, ensuring that they not only meet but exceed compliance requirements.
- Companies must conduct regular risk assessments to identify potential hazards and implement mitigation strategies. We provide advanced risk assessment tools powered by AI, enabling companies to proactively identify and address potential risks, thereby reducing incidents and enhancing operational efficiency.
- Training programs for employees are essential to ensure they understand safety protocols and emergency procedures. We design and implement comprehensive training programs that equip employees with the knowledge and skills necessary to maintain a safe working environment.
- Advanced technologies, such as drones and IoT sensors, are increasingly used for monitoring equipment and detecting leaks. Our expertise in AI and blockchain allows us to integrate these technologies seamlessly, providing real-time monitoring and data analytics that enhance safety measures.
- Incident reporting systems help track safety violations and near-misses, fostering a culture of continuous improvement. We develop customized incident reporting systems that not only track violations but also analyze data to identify trends and areas for improvement.
- Compliance audits are necessary to ensure adherence to regulations and internal policies. Our team conducts thorough compliance audits, providing actionable insights that help organizations maintain high standards of safety and compliance.
- Collaboration with local communities and stakeholders can enhance safety measures and environmental stewardship. We facilitate partnerships that promote transparency and collaboration, ensuring that safety measures are aligned with community expectations.
- Investing in safety technologies can lead to reduced accidents and lower operational costs in the long run. By partnering with Rapid Innovation, clients can expect a significant return on investment through reduced incidents and enhanced operational efficiency. For more information on how we are revolutionising pipeline integrity, visit this link.
6.5. Healthcare: Maintaining Medical Equipment Reliability
- Medical equipment reliability is crucial for patient safety and effective healthcare delivery. Our solutions focus on ensuring that healthcare providers can rely on their equipment, ultimately improving patient outcomes.
- Regular maintenance schedules are essential to ensure that equipment functions correctly and meets regulatory standards. We implement automated maintenance scheduling systems that streamline the process and ensure compliance with industry standards.
- Predictive maintenance techniques can help anticipate equipment failures before they occur, minimizing downtime. Our AI-driven predictive maintenance solutions allow healthcare organizations to forecast potential failures, reducing downtime and associated costs.
- Staff training on proper equipment use can prevent misuse and extend the lifespan of medical devices. We offer specialized training programs that empower staff to use medical equipment effectively, ensuring longevity and reliability.
- Compliance with standards set by organizations like the FDA and ISO is necessary for maintaining equipment reliability. Our consulting services ensure that healthcare providers meet and exceed these standards, enhancing their reputation and operational efficiency.
- Implementing a robust inventory management system can help track equipment usage and maintenance history. We develop customized inventory management solutions that provide real-time insights into equipment status and maintenance needs.
- Engaging with manufacturers for support and updates can enhance the reliability of medical devices. Our team facilitates communication with manufacturers, ensuring that healthcare providers receive timely updates and support.
- Utilizing data analytics can provide insights into equipment performance and inform maintenance strategies. We leverage advanced data analytics to help organizations make informed decisions about their equipment maintenance strategies.
- Establishing a culture of accountability among staff can improve adherence to maintenance protocols. We work with organizations to foster a culture of accountability, ensuring that maintenance protocols are followed diligently.
7. Measuring the Impact of AI-Powered Predictive Maintenance
- AI-powered predictive maintenance leverages data analytics to forecast equipment failures and optimize maintenance schedules. Our solutions empower organizations to harness the power of AI, leading to significant improvements in operational efficiency.
- Key performance indicators (KPIs) can be established to measure the effectiveness of predictive maintenance initiatives. We help clients define and track KPIs that align with their business goals, ensuring that predictive maintenance initiatives deliver measurable results.
- Metrics such as equipment uptime, maintenance costs, and mean time to repair (MTTR) can provide insights into performance. Our analytics tools provide real-time insights into these metrics, enabling organizations to make data-driven decisions.
- Case studies have shown that companies implementing AI-driven solutions can reduce maintenance costs by up to 30%. By partnering with Rapid Innovation, clients can expect similar results, enhancing their bottom line.
- The integration of machine learning algorithms allows for continuous improvement in predictive accuracy over time. Our solutions evolve with your organization, ensuring that predictive maintenance strategies remain effective and relevant.
- Employee training on AI tools is essential to maximize the benefits of predictive maintenance technologies. We provide comprehensive training programs that equip staff with the skills needed to leverage AI tools effectively.
- Feedback loops can be established to refine predictive models based on real-world performance data. Our approach includes continuous feedback mechanisms that enhance the accuracy of predictive models over time.
- Collaboration between IT and operational teams can enhance the implementation of AI solutions. We facilitate collaboration between teams, ensuring that AI solutions are integrated seamlessly into existing workflows.
- Regular reviews of predictive maintenance outcomes can help organizations adjust strategies and improve overall efficiency. Our ongoing support includes regular reviews and strategy adjustments, ensuring that clients achieve their operational goals efficiently and effectively.
By partnering with Rapid Innovation, clients can expect not only enhanced oil and gas safety compliance but also improved operational efficiency and a greater return on investment. Our expertise in AI and blockchain technology positions us as a leader in delivering innovative solutions tailored to the unique challenges of the oil and gas and healthcare industries.
7.1. Financial Benefits: Quantifying Cost Savings and ROI
- Cost savings can be realized through various avenues, including:
- Reduced operational costs due to improved processes.
- Lower maintenance expenses from more reliable systems.
- Decreased labor costs through automation and efficiency improvements.
- Return on Investment (ROI) is a critical metric for evaluating financial benefits:
- ROI can be calculated by comparing the net profit from an investment to its cost.
- A positive ROI indicates that the benefits outweigh the costs, making the investment worthwhile.
- Organizations can quantify cost savings and ROI by:
- Conducting a thorough analysis of current expenses and identifying areas for improvement.
- Implementing tracking systems to monitor savings over time.
- Utilizing financial modeling to project future savings based on current data.
- Real-world examples show significant financial benefits:
- Companies that adopt automation technologies often report up to a 30% reduction in operational costs (source: McKinsey).
- Businesses that invest in energy-efficient systems can save up to 25% on energy bills (source: U.S. Department of Energy).
- Regularly reviewing financial metrics helps organizations stay on track:
- Establishing key performance indicators (KPIs) related to cost savings and ROI.
- Adjusting strategies based on performance data to maximize financial benefits.
7.2. Operational Benefits: Enhancing Efficiency and Reliability
- Operational efficiency is crucial for maximizing productivity:
- Streamlined processes reduce waste and improve workflow.
- Automation minimizes human error, leading to more reliable outcomes.
- Reliability in operations leads to:
- Consistent product quality, which enhances customer satisfaction.
- Fewer disruptions in service, resulting in better overall performance.
- Key strategies for enhancing operational efficiency include:
- Implementing lean management principles to eliminate inefficiencies.
- Utilizing technology to automate repetitive tasks and improve accuracy.
- Training and development of staff can also contribute to operational benefits:
- Skilled employees are more capable of identifying and solving problems quickly.
- Continuous training ensures that staff are up-to-date with the latest tools and techniques.
- Metrics to measure operational benefits include:
- Cycle time reduction, which indicates faster production or service delivery.
- Increased output per labor hour, reflecting improved productivity.
- Organizations that focus on operational efficiency often see significant improvements:
- Companies that adopt lean practices can achieve up to a 50% reduction in lead times (source: Lean Enterprise Institute).
- Businesses that invest in employee training report a 24% increase in productivity (source: Association for Talent Development).
7.3. Strategic Advantages: Staying Competitive in the Market
- Strategic advantages are essential for long-term success:
- Companies that innovate and adapt are better positioned to respond to market changes.
- A strong competitive edge can lead to increased market share and profitability.
- Key factors contributing to strategic advantages include:
- Differentiation through unique products or services that meet customer needs.
- Agility in operations, allowing for quick responses to market demands.
- Investing in research and development (R&D) is vital for maintaining competitiveness:
- R&D enables companies to innovate and stay ahead of industry trends.
- Organizations that prioritize R&D often see higher growth rates compared to their competitors.
- Building strong relationships with customers can also provide strategic benefits:
- Understanding customer preferences leads to better product offerings.
- Engaging with customers fosters loyalty and repeat business.
- Monitoring competitors and market trends is crucial:
- Conducting regular market analysis helps identify opportunities and threats.
- Adapting strategies based on competitive intelligence can enhance market positioning.
- Companies that leverage strategic advantages often achieve remarkable results:
- Firms that focus on innovation can experience up to a 20% higher revenue growth (source: PwC).
- Businesses with strong customer engagement strategies see a 10-15% increase in customer retention rates (source: Bain & Company).
At Rapid Innovation, we are committed to helping our clients harness these financial, operational, and strategic benefits through our AI and Blockchain development solutions. By partnering with us, you can expect enhanced efficiency, reduced costs, and a stronger competitive position in the market, ultimately leading to greater cost savings and ROI and long-term success.
8. Overcoming Challenges in AI-Powered Predictive Maintenance Implementation
Implementing AI-powered predictive maintenance can significantly enhance operational efficiency and reduce downtime. However, organizations often face various challenges during this process. Understanding these challenges and how to overcome them is crucial for successful implementation.
8.1. Identifying Common Barriers to Adoption

Several barriers can hinder the adoption of AI-powered predictive maintenance:
- Lack of Skilled Workforce: Many organizations struggle to find personnel with the necessary skills in AI, data analytics, and machine learning. Training existing staff or hiring new talent can be costly and time-consuming.
- Data Silos: Data may be stored in different systems or departments, making it difficult to access and analyze. Integration of disparate data sources is essential for effective predictive maintenance.
- High Initial Costs: The upfront investment in AI technologies, sensors, and infrastructure can be significant. Organizations may hesitate to allocate budget without guaranteed ROI.
- Resistance to Change: Employees may be resistant to adopting new technologies due to fear of job loss or unfamiliarity. Change management strategies are necessary to facilitate a smooth transition.
- Insufficient Data: Predictive maintenance relies heavily on data; insufficient historical data can limit the effectiveness of AI models. Organizations need to ensure they have enough quality data to train their models.
- Regulatory Compliance: Adhering to industry regulations and standards can complicate the implementation of AI solutions. Organizations must ensure that their predictive maintenance strategies comply with relevant laws.
8.2. Best Practices for Ensuring Data Quality and Security
To successfully implement AI-powered predictive maintenance, organizations should focus on data quality and security:
- Establish Data Governance: Create a framework for data management that includes policies for data collection, storage, and usage. Assign roles and responsibilities to ensure accountability in data handling.
- Invest in Data Cleaning: Regularly clean and preprocess data to remove inaccuracies and inconsistencies. Implement automated tools to streamline the data cleaning process.
- Utilize Advanced Analytics: Employ advanced analytics techniques to enhance data quality and derive actionable insights. Use machine learning algorithms to identify patterns and anomalies in data.
- Implement Robust Security Measures: Protect sensitive data with encryption, access controls, and regular security audits. Ensure compliance with data protection regulations, such as GDPR or HIPAA.
- Foster a Culture of Data Literacy: Train employees on the importance of data quality and security. Encourage a culture where data-driven decision-making is valued.
- Regularly Monitor and Update Systems: Continuously monitor data quality and security protocols to identify potential issues. Update systems and processes as needed to adapt to changing technologies and threats.
By addressing these barriers and following best practices, organizations can enhance their chances of successfully implementing AI-powered predictive maintenance, leading to improved operational efficiency and reduced costs.
At Rapid Innovation, we specialize in guiding organizations through these challenges, ensuring that your investment in AI-powered predictive maintenance yields significant returns. Our expertise in AI and blockchain development allows us to tailor solutions that not only meet your specific needs but also drive greater ROI. Partnering with us means you can expect enhanced operational efficiency, reduced downtime, and a smoother transition to advanced technologies. Let us help you navigate the complexities of AI implementation and unlock the full potential of your operations with AI predictive maintenance, predictive maintenance AI, and AI for predictive maintenance.
8.3. Building Cross-Functional Teams for Successful Implementation
At Rapid Innovation, we understand that cross-functional teams are essential for driving successful implementation of AI and blockchain solutions. By bringing together diverse expertise from various departments, these cross-functional teams enhance problem-solving capabilities and foster innovation. Key roles in these teams may include:
- Engineers: Provide technical insights and feasibility assessments.
- Data Analysts: Analyze data trends and predictive models.
- Operations Managers: Ensure alignment with operational goals and processes.
- IT Specialists: Manage technology integration and data security.
The benefits of cross-functional teams include:
- Improved communication: Breaks down silos between departments, fostering collaboration and ensuring that all voices are heard.
- Enhanced innovation: Diverse perspectives lead to creative solutions that can address complex challenges.
- Faster decision-making: Teams can address issues more quickly with varied expertise, leading to timely project execution.
To build effective cross-functional teams, consider the following strategies:
- Clearly define roles and responsibilities to avoid confusion and ensure accountability.
- Foster a culture of trust and open communication to encourage collaboration and idea sharing.
- Set common goals to align team efforts and measure success, ensuring everyone is working towards the same objectives.
However, there are challenges to consider:
- Potential conflicts may arise due to differing priorities and perspectives, which can be mitigated through effective communication.
- Time constraints as team members balance their primary responsibilities, necessitating careful planning and resource allocation.
Successful examples of cross-functional teams can be found in companies like General Electric and Siemens, which have effectively implemented predictive maintenance strategies, showcasing the power of collaboration in achieving business goals. For more insights on successful implementation strategies, check out our successful AI integration strategies.
9. Best Practices for Maximizing Predictive Maintenance Success

To maximize the success of your predictive maintenance program, follow these best practices:
- Establish clear objectives:
- Define what success looks like for your predictive maintenance program.
- Align objectives with overall business goals to ensure relevance and impact.
- Invest in the right technology:
- Utilize advanced analytics and machine learning tools to enhance predictive capabilities.
- Ensure that the technology integrates seamlessly with existing systems to avoid disruptions.
- Focus on data quality:
- Collect accurate and relevant data to improve predictive models and outcomes.
- Regularly clean and validate data to maintain its integrity and reliability.
- Train staff effectively:
- Provide training on new technologies and processes to ensure team members are equipped to utilize predictive maintenance tools.
- Encourage a culture of continuous learning and adaptation to keep pace with industry advancements.
- Monitor and adjust:
- Regularly review the performance of predictive maintenance initiatives to identify areas for improvement.
- Be prepared to adjust strategies based on feedback and results to optimize outcomes.
- Collaborate with external experts:
- Engage with consultants or industry experts to gain insights and best practices that can enhance your initiatives.
- Participate in industry forums to stay updated on trends and innovations that can inform your strategies.
9.1. Starting Small: Pilot Projects for Immediate Impact
Pilot projects allow organizations to test predictive maintenance strategies on a smaller scale before full implementation. The benefits of starting small include:
- Reduced risk: Limits exposure to potential failures and allows for adjustments based on initial findings.
- Quick wins: Demonstrates value and builds support for broader initiatives, showcasing the effectiveness of your approach.
- Easier resource allocation: Requires fewer resources and less investment initially, making it a manageable starting point.
To implement a pilot project, follow these steps:
- Identify a specific asset or system to focus on, ensuring it has enough data for analysis to yield meaningful insights.
- Set clear objectives and success metrics to evaluate the pilot's effectiveness and impact on operations.
- Engage a small, cross-functional team to oversee the project, ensuring diverse expertise is applied.
Monitor results closely by:
- Collecting data throughout the pilot to assess performance against established metrics and identify trends.
- Using findings to refine predictive models and strategies for future initiatives.
Communicate successes by:
- Sharing results with stakeholders to build momentum for larger-scale implementation and demonstrate the value of your efforts.
- Highlighting lessons learned to improve future projects and foster a culture of continuous improvement.
Examples of successful pilot projects can be found in various industries, showcasing how small-scale initiatives can lead to significant improvements in maintenance practices and overall operational efficiency. By partnering with Rapid Innovation, you can leverage our expertise to implement these strategies effectively, ensuring a greater return on investment and achieving your business goals efficiently.
9.2. Ensuring Continuous Training and Development for Staff
- Continuous learning and development are essential for maintaining a skilled workforce in any organization. Regular training helps employees stay updated with the latest technologies and methodologies, fostering a culture of ongoing training and development, which is crucial in fast-paced industries.
- Key components of effective training programs include:
- Onboarding Programs: Introduce new employees to company culture and processes.
- Skill Development Workshops: Focus on specific skills relevant to current job roles.
- Leadership Training: Prepare employees for future leadership roles within the organization.
- Utilizing various training methods can enhance learning:
- E-Learning Platforms: Offer flexibility and accessibility for employees to learn at their own pace.
- Mentorship Programs: Pair less experienced staff with seasoned professionals for guidance.
- Cross-Training: Encourage employees to learn different roles within the organization to promote versatility.
- Regular assessments and feedback mechanisms are vital:
- Performance Reviews: Identify areas for improvement and tailor training accordingly.
- Surveys and Feedback: Gather employee input on training effectiveness and areas of interest.
- Investing in continuous training and development can lead to:
- Increased employee satisfaction and retention.
- Enhanced productivity and efficiency.
- A more innovative and competitive workforce.
- The benefits of continuous training of employees include improved skills and knowledge, which contribute to overall organizational success. For more insights on effective training strategies, you can refer to learning from real-world AI implementations.
9.3. Leveraging AI Insights for Ongoing Process Improvement
- AI Data-driven insights are crucial for identifying areas of improvement within an organization. Analyzing performance metrics can reveal inefficiencies and opportunities for enhancement.
- Key strategies for leveraging insights include:
- Regular Data Analysis: Use analytics tools to monitor key performance indicators (KPIs).
- Feedback Loops: Implement systems for collecting feedback from employees and customers to inform improvements.
- Benchmarking: Compare performance against industry standards to identify gaps.
- Collaboration across departments can enhance insights:
- Cross-Functional Teams: Encourage collaboration between different departments to share insights and best practices.
- Knowledge Sharing Platforms: Create forums for employees to discuss challenges and solutions.
- Continuous improvement methodologies can be applied:
- Lean Management: Focus on reducing waste and improving efficiency.
- Six Sigma: Utilize data-driven approaches to eliminate defects and improve quality.
- The benefits of leveraging AI insights for process improvement include:
- Enhanced operational efficiency.
- Improved customer satisfaction through better service delivery.
- Increased profitability by optimizing resource allocation.
10. Future Trends in AI-Powered Predictive Maintenance
- Predictive maintenance is evolving rapidly, driven by advancements in artificial intelligence (AI) and machine learning. The future of predictive maintenance will likely include several key trends:
- Increased Use of IoT Devices:
- More organizations will adopt Internet of Things (IoT) sensors to collect real-time data from equipment. This data will be analyzed to predict failures before they occur.
- Advanced Analytics and Machine Learning:
- AI algorithms will become more sophisticated, enabling better predictions based on historical data. Machine learning models will continuously improve as they process more data over time.
- Integration with Augmented Reality (AR):
- AR tools will assist technicians in performing maintenance tasks by overlaying digital information onto physical equipment. This can enhance training and improve the accuracy of maintenance procedures.
- Cloud Computing and Big Data:
- Cloud platforms will facilitate the storage and analysis of vast amounts of data generated by predictive maintenance systems. Big data analytics will enable organizations to identify patterns and trends that were previously undetectable.
- Focus on Sustainability:
- Predictive maintenance will increasingly be aligned with sustainability goals, helping organizations reduce waste and energy consumption. By optimizing maintenance schedules, companies can extend the lifespan of equipment and reduce their environmental footprint.
- Enhanced Collaboration and Communication:
- Predictive maintenance solutions will promote better communication between maintenance teams and other departments. Real-time data sharing will enable quicker decision-making and more effective resource allocation.
- The impact of these trends will likely include:
- Reduced downtime and maintenance costs.
- Improved asset reliability and performance.
- Greater overall efficiency in operations.
10.1. Emerging Technologies: The Role of Digital Twins and Edge Computing
- Digital Twins:
- A digital twin is a virtual representation of a physical object or system, allowing for real-time monitoring and analysis. They enable organizations to simulate, predict, and optimize performance by mirroring real-world conditions. Applications include manufacturing, healthcare, and urban planning, where they can enhance decision-making and operational efficiency. According to a report by Gartner, by 2023, more than 50% of large industrial companies will use digital twins.
- Edge Computing:
- Edge computing processes data closer to the source rather than relying solely on centralized data centers. This reduces latency, enhances speed, and improves the efficiency of data handling. It is particularly beneficial for IoT devices, which generate vast amounts of data that need immediate processing. The combination of digital twins and edge computing allows for real-time data analysis, leading to quicker responses to changes in the physical environment.
- Synergy of Digital Twins and Edge Computing:
- Together, they facilitate advanced analytics and machine learning applications. They support predictive maintenance by analyzing data from equipment and predicting failures before they occur. This integration can lead to significant cost savings and improved operational efficiency.
10.2. How 5G Will Revolutionize Real-Time Monitoring
- Enhanced Speed and Capacity:
- 5G technology offers significantly higher data transfer speeds compared to previous generations, enabling real-time data transmission. It can support a larger number of connected devices, making it ideal for IoT applications in various industries.
- Low Latency:
- 5G networks have ultra-low latency, which is crucial for applications requiring immediate feedback, such as autonomous vehicles and remote surgeries. This capability allows for instantaneous communication between devices, enhancing operational efficiency.
- Improved Connectivity:
- 5G can connect devices in remote or challenging environments, expanding the possibilities for real-time monitoring. Industries such as agriculture, mining, and logistics can benefit from enhanced connectivity, leading to better resource management.
- Impact on Industries:
- In manufacturing, 5G can enable real-time monitoring of production lines, leading to quicker adjustments and reduced downtime. In healthcare, it can facilitate remote patient monitoring and telemedicine, improving patient outcomes. The logistics sector can utilize 5G for tracking shipments in real-time, optimizing supply chain management.
10.3. Predictions for AI-Driven Maintenance in Industry 4.0
- Predictive Maintenance:
- AI algorithms can analyze data from machinery to predict when maintenance is needed, reducing unexpected breakdowns. This approach can lead to a significant reduction in maintenance costs and downtime.
- Enhanced Decision-Making:
- AI can process vast amounts of data from various sources, providing insights that help in making informed maintenance decisions. It can identify patterns and anomalies that human operators might miss, leading to more proactive maintenance strategies.
- Automation of Maintenance Tasks:
- AI-driven systems can automate routine maintenance tasks, freeing up human resources for more complex issues. This can lead to increased efficiency and reduced labor costs.
- Integration with IoT:
- The combination of AI and IoT devices allows for continuous monitoring of equipment health. This integration can provide real-time alerts and recommendations for maintenance actions.
- Future Trends:
- By 2025, it is predicted that AI-driven maintenance will become a standard practice in many industries, with a focus on sustainability and efficiency. Companies that adopt AI-driven maintenance strategies are likely to gain a competitive edge in the market.
At Rapid Innovation, we leverage these emerging technologies to help our clients achieve their goals efficiently and effectively. By integrating digital twins and edge computing, we enable organizations to optimize their operations, leading to greater ROI. Our expertise in 5G technology enhances real-time monitoring capabilities, allowing businesses to make informed decisions swiftly. Furthermore, our AI-driven maintenance solutions reduce downtime and maintenance costs, ensuring that our clients remain competitive in their respective industries. Partnering with us means gaining access to cutting-edge solutions that drive innovation and operational excellence.
11. Getting Started with AI-Powered Predictive Maintenance
AI-powered predictive maintenance leverages data analytics and machine learning to predict equipment failures before they occur. This proactive approach can significantly reduce downtime and maintenance costs. To successfully implement AI-powered predictive maintenance, organizations must assess their readiness and develop a clear roadmap.
11.1. Assessing Your Organization’s Readiness for AI Solutions
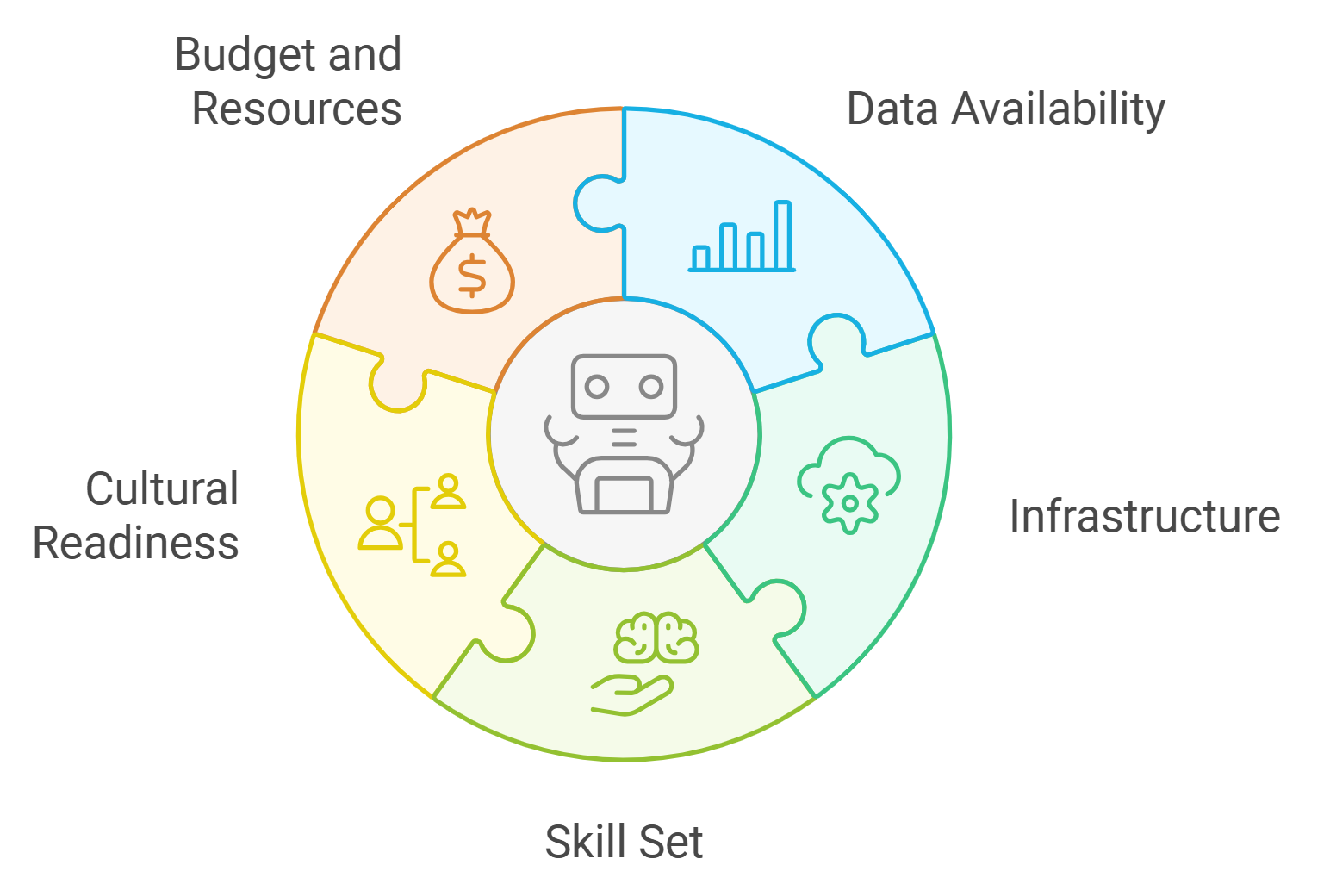
Before diving into AI-powered predictive maintenance, it’s crucial to evaluate your organization’s current capabilities and readiness. This assessment can help identify gaps and areas for improvement.
- Data Availability: Ensure you have access to historical and real-time data from your equipment. Assess the quality of the data; it should be accurate, complete, and relevant.
- Infrastructure: Evaluate your existing IT infrastructure to support AI-powered predictive maintenance solutions. Consider whether you need to upgrade hardware or software to handle data processing and storage.
- Skill Set: Identify the skills available within your team. Determine if you need to hire new talent or provide training for existing employees in data science and machine learning.
- Cultural Readiness: Assess the organizational culture regarding technology adoption. Ensure that leadership is supportive of AI initiatives and that there is a willingness to embrace change.
- Budget and Resources: Analyze your budget to determine if you can allocate funds for AI-powered predictive maintenance tools and training. Consider the resources required for implementation, including time and personnel.
11.2. Developing a Roadmap for Implementation
Once you have assessed your organization’s readiness, the next step is to create a detailed roadmap for implementing AI-powered predictive maintenance. This roadmap will guide your efforts and help ensure a successful deployment.
- Define Objectives: Clearly outline the goals you want to achieve with AI-powered predictive maintenance, such as reducing downtime, lowering maintenance costs, or improving equipment lifespan.
- Select Use Cases: Identify specific use cases where AI-powered predictive maintenance can be applied. Focus on high-impact areas that can deliver quick wins and demonstrate value.
- Choose the Right Tools: Research and select AI tools and platforms that align with your objectives and use cases. Consider factors such as ease of integration, scalability, and support.
- Pilot Program: Start with a pilot program to test the AI-powered predictive maintenance solution on a smaller scale. Monitor performance and gather feedback to make necessary adjustments before full-scale implementation.
- Data Strategy: Develop a strategy for data collection, storage, and analysis. Ensure that data governance practices are in place to maintain data integrity and security.
- Training and Change Management: Plan for training sessions to equip your team with the necessary skills to use AI-powered predictive maintenance tools effectively. Implement change management strategies to facilitate a smooth transition and encourage adoption.
- Monitor and Iterate: Establish metrics to evaluate the success of your AI-powered predictive maintenance efforts. Continuously monitor performance and be prepared to iterate on your approach based on insights and feedback.
By following these steps, organizations can effectively prepare for and implement AI-powered predictive maintenance, leading to improved operational efficiency and reduced costs.
At Rapid Innovation, we specialize in guiding organizations through this transformative journey. Our expertise in AI and blockchain development ensures that you not only achieve your AI-powered predictive maintenance goals but also maximize your return on investment. By partnering with us, you can expect enhanced operational efficiency, reduced downtime, and significant cost savings, all while leveraging cutting-edge technology tailored to your specific needs. Let us help you unlock the full potential of your operations through AI-powered solutions.
11.3. Partnering with Experts to Accelerate Your Journey
- Collaborating with industry experts can significantly enhance your predictive maintenance strategy. Experts bring specialized knowledge and experience that can help identify potential pitfalls and best practices. They can assist in:
- Developing a tailored predictive maintenance strategy that aligns with your business goals.
- Implementing advanced analytics and machine learning models to improve accuracy.
- Training your team on new technologies and methodologies.
- Consider partnering with:
- Consulting firms specializing in predictive maintenance.
- Technology vendors who offer AI and IoT solutions.
- Academic institutions for research and development collaborations.
- Establish clear communication channels to ensure alignment on objectives and expectations.
- Regularly review progress and adapt strategies based on expert feedback and industry trends.
11.4. Recommended Tools and Technologies for Predictive Maintenance
- Selecting the right tools and technologies is crucial for effective predictive maintenance. Key categories of tools include:
- Data Analytics Platforms: These platforms analyze historical and real-time data to predict equipment failures.
- IoT Sensors: Sensors collect data on equipment performance, enabling real-time monitoring.
- Machine Learning Algorithms: These algorithms identify patterns in data that indicate potential issues.
- Recommended tools include:
- IBM Maximo: A comprehensive asset management solution that integrates predictive maintenance capabilities.
- Uptake: Offers AI-driven insights for industrial equipment maintenance.
- PTC ThingWorx: A platform for developing IoT applications that support predictive maintenance.
- Ensure that the tools you choose can integrate with your existing systems for seamless data flow.
- Consider scalability and ease of use when selecting technologies to accommodate future growth.
11.5. Next Steps: Launching Your AI-Powered Maintenance Program
- Launching an AI-powered maintenance program requires careful planning and execution. Key steps to consider include:
- Define Objectives: Clearly outline what you aim to achieve with predictive maintenance, such as reducing downtime or extending equipment life.
- Assess Current Capabilities: Evaluate your existing maintenance processes and technologies to identify gaps.
- Develop a Roadmap: Create a detailed plan that includes timelines, milestones, and resource allocation.
- Engage stakeholders across the organization to ensure buy-in and support for the initiative.
- Start with a pilot program to test the effectiveness of your predictive maintenance strategy before full-scale implementation.
- Monitor performance metrics to evaluate the success of the program and make necessary adjustments.
- Continuously update your approach based on new data, technological advancements, and feedback from your team.
At Rapid Innovation, we understand that the journey to effective predictive maintenance can be complex. By partnering with us, you gain access to our expertise in AI and blockchain technologies, enabling you to implement solutions that not only enhance operational efficiency but also drive greater ROI. Our tailored strategies, including preventive predictive and proactive maintenance, predictive based maintenance, and condition based maintenance and predictive maintenance, along with advanced analytics capabilities ensure that you are well-equipped to meet your business goals while minimizing risks and maximizing returns. Let us help you navigate this journey effectively and efficiently.