Table Of Contents
Category
Artificial Intelligence
1. Introduction
The rapid advancement of technology has ushered in an era where the digital and physical worlds are increasingly intertwined. One of the most transformative innovations in this space is the concept of digital twins, particularly those driven by artificial intelligence (AI). Digital twins are virtual replicas of physical entities, systems, or processes that can be used to simulate, predict, and optimize real-world performance. The integration of AI into digital twins has significantly enhanced their capabilities, making them indispensable tools in various industries, from manufacturing and healthcare to urban planning and beyond.
The concept of digital twins is not entirely new; it has its roots in the early days of computer-aided design (CAD) and simulation technologies. However, the advent of AI has revolutionized the way digital twins are created and utilized. AI-driven digital twins leverage machine learning algorithms, data analytics, and real-time data to provide a more accurate and dynamic representation of their physical counterparts. This allows for more precise predictions, better decision-making, and improved efficiency.
In this article, we will delve into the world of AI-driven digital twins, exploring their definition, how they work, and the myriad of applications they have in today's technology-driven landscape. We will also examine the benefits and challenges associated with implementing AI-driven digital twins, providing a comprehensive understanding of this cutting-edge technology.
2. What are AI-Driven Digital Twins?
AI-driven digital twins represent a significant evolution in the field of digital modeling and simulation. At their core, digital twins are virtual models that mirror physical objects, systems, or processes. These models are created using data collected from sensors, IoT devices, and other sources, which are then processed and analyzed to create a digital representation that can be used for various purposes, such as monitoring, simulation, and optimization.
The integration of AI into digital twins enhances their capabilities by enabling them to learn from data, adapt to changing conditions, and make predictions. This is achieved through the use of machine learning algorithms, which can analyze vast amounts of data to identify patterns, trends, and anomalies. By continuously learning from new data, AI-driven digital twins can provide more accurate and up-to-date insights, making them invaluable tools for decision-making and problem-solving.
One of the key advantages of AI-driven digital twins is their ability to simulate and predict future scenarios. For example, in manufacturing, an AI-driven digital twin of a production line can be used to simulate different production schedules, identify potential bottlenecks, and optimize the overall process. In healthcare, a digital twin of a patient can be used to predict the progression of a disease and tailor treatment plans accordingly. The possibilities are virtually endless, and the potential benefits are immense.
2.1. Definition
An AI-driven digital twin is a virtual representation of a physical entity, system, or process that is enhanced with artificial intelligence capabilities. This digital replica is created using data collected from various sources, such as sensors, IoT devices, and historical records. The data is then processed and analyzed using machine learning algorithms to create a dynamic and accurate model that can be used for monitoring, simulation, and optimization.
The defining feature of an AI-driven digital twin is its ability to learn from data and adapt to changing conditions. Unlike traditional digital twins, which are static models, AI-driven digital twins are dynamic and continuously updated with new data. This allows them to provide real-time insights and make predictions based on the latest information. For example, an AI-driven digital twin of a wind turbine can continuously monitor its performance, identify potential issues, and predict when maintenance is needed, thereby reducing downtime and improving efficiency.
In summary, an AI-driven digital twin is a powerful tool that combines the strengths of digital modeling and artificial intelligence. By leveraging data and machine learning, these digital replicas can provide valuable insights, optimize performance, and drive innovation across various industries. As technology continues to advance, the role of AI-driven digital twins is expected to grow, offering new opportunities and challenges in the ever-evolving digital landscape.
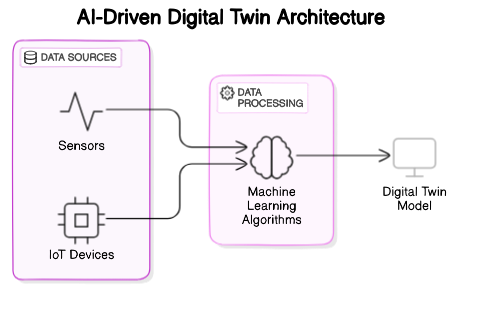
2.2. Key Components
The concept of digital twins has revolutionized various industries by providing a virtual replica of physical assets, systems, or processes. To understand the full potential of digital twins, it is essential to delve into their key components. These components work in unison to create a comprehensive and dynamic model that can be used for monitoring, analysis, and optimization.
The first key component of a digital twin is the physical asset or system itself. This could be anything from a manufacturing machine, a building, a vehicle, or even an entire city. The physical asset is equipped with sensors and other data collection devices that continuously gather information about its condition, performance, and environment. This real-time data is crucial for creating an accurate and up-to-date digital representation.
The second component is the digital model, which is a virtual replica of the physical asset. This model is created using advanced simulation and modeling techniques, often incorporating 3D CAD models, finite element analysis, and other engineering tools. The digital model is designed to mimic the physical asset's behavior and characteristics as closely as possible, allowing for accurate predictions and analysis.
The third key component is the data integration and processing system. This system collects data from the physical asset's sensors and other sources, such as historical records, maintenance logs, and external databases. The data is then processed and integrated into the digital model, ensuring that it remains up-to-date and accurate. Advanced data analytics and machine learning algorithms are often used to analyze the data and identify patterns, trends, and anomalies.
The fourth component is the user interface, which allows stakeholders to interact with the digital twin. This interface can take various forms, such as a web-based dashboard, a mobile app, or a virtual reality environment. The user interface provides access to real-time data, visualizations, and analytical tools, enabling users to monitor the asset's performance, diagnose issues, and make informed decisions.
The fifth key component is the communication and networking infrastructure. This infrastructure ensures that data can be transmitted between the physical asset, the digital model, and the user interface in real-time. It often involves a combination of wired and wireless communication technologies, such as IoT, 5G, and cloud computing. A robust and reliable communication network is essential for maintaining the accuracy and timeliness of the digital twin.
Finally, the sixth component is the security and privacy framework. Given the sensitive nature of the data involved, it is crucial to implement robust security measures to protect against unauthorized access, data breaches, and cyberattacks. This includes encryption, authentication, access control, and regular security audits. Additionally, privacy concerns must be addressed, particularly when dealing with personal or confidential information.
In summary, the key components of a digital twin include the physical asset, the digital model, the data integration and processing system, the user interface, the communication and networking infrastructure, and the security and privacy framework. These components work together to create a dynamic and accurate virtual representation of the physical asset, enabling real-time monitoring, analysis, and optimization.
3. How Do AI-Driven Digital Twins Work?
AI-driven digital twins represent a significant advancement in the field of digital twin technology. By integrating artificial intelligence (AI) and machine learning (ML) capabilities, these digital twins can provide more accurate predictions, deeper insights, and enhanced decision-making capabilities. To understand how AI-driven digital twins work, it is essential to explore the various stages and processes involved.
The first stage in the functioning of an AI-driven digital twin is data collection. This involves gathering real-time data from the physical asset or system using sensors, IoT devices, and other data sources. The data collected can include various parameters such as temperature, pressure, vibration, and operational status. This real-time data is crucial for creating an accurate and up-to-date digital representation of the physical asset.
Once the data is collected, it is processed and integrated into the digital model. This involves cleaning, filtering, and transforming the raw data into a format that can be used by the digital twin. Advanced data processing techniques, such as data normalization and feature extraction, are often employed to ensure the quality and consistency of the data. The processed data is then fed into the digital model, which is designed to mimic the physical asset's behavior and characteristics.
The next stage involves the application of AI and ML algorithms to the digital model. These algorithms analyze the data and identify patterns, trends, and anomalies. For example, machine learning models can be trained to predict equipment failures, optimize maintenance schedules, and improve operational efficiency. AI-driven digital twins can also use predictive analytics to forecast future performance and identify potential issues before they occur.
One of the key advantages of AI-driven digital twins is their ability to learn and adapt over time. As more data is collected and analyzed, the AI algorithms can continuously update and refine the digital model, improving its accuracy and reliability. This iterative learning process enables the digital twin to provide more accurate predictions and insights, leading to better decision-making and optimization.
The user interface plays a crucial role in the functioning of AI-driven digital twins. It allows stakeholders to interact with the digital twin and access real-time data, visualizations, and analytical tools. The user interface can take various forms, such as a web-based dashboard, a mobile app, or a virtual reality environment. It provides users with the ability to monitor the asset's performance, diagnose issues, and make informed decisions based on the insights provided by the AI algorithms.
Communication and networking infrastructure is another critical component of AI-driven digital twins. This infrastructure ensures that data can be transmitted between the physical asset, the digital model, and the user interface in real-time. It often involves a combination of wired and wireless communication technologies, such as IoT, 5G, and cloud computing. A robust and reliable communication network is essential for maintaining the accuracy and timeliness of the digital twin.
Security and privacy are also paramount in the functioning of AI-driven digital twins. Given the sensitive nature of the data involved, it is crucial to implement robust security measures to protect against unauthorized access, data breaches, and cyberattacks. This includes encryption, authentication, access control, and regular security audits. Additionally, privacy concerns must be addressed, particularly when dealing with personal or confidential information.
In summary, AI-driven digital twins work by collecting real-time data from physical assets, processing and integrating the data into a digital model, applying AI and ML algorithms to analyze the data, and providing insights and predictions through a user interface. Communication and networking infrastructure, as well as security and privacy measures, are also critical components of their functioning. By leveraging the power of AI and ML, these digital twins can provide more accurate predictions, deeper insights, and enhanced decision-making capabilities.
3.1. Data Collection
Data collection is the foundational step in the creation and operation of digital twins, particularly AI-driven digital twins. The accuracy, reliability, and effectiveness of a digital twin largely depend on the quality and comprehensiveness of the data collected from the physical asset or system. This process involves several stages and utilizes various technologies to ensure that the data is both relevant and actionable.
The first stage of data collection involves the deployment of sensors and IoT devices on the physical asset. These sensors are designed to monitor various parameters such as temperature, pressure, vibration, humidity, and operational status. The type and number of sensors used depend on the specific requirements of the asset and the objectives of the digital twin. For example, a manufacturing machine may require sensors to monitor its operational speed, temperature, and vibration levels, while a building may need sensors to track energy consumption, occupancy, and environmental conditions.
Once the sensors are in place, they continuously collect real-time data from the physical asset. This data is often transmitted to a central data repository or cloud-based platform using wired or wireless communication technologies. The choice of communication technology depends on factors such as the volume of data, the required transmission speed, and the network infrastructure. Common communication technologies used in data collection include Wi-Fi, Bluetooth, Zigbee, LoRaWAN, and 5G.
The collected data is then subjected to a series of preprocessing steps to ensure its quality and consistency. This involves cleaning the data to remove any noise, errors, or outliers that may affect the accuracy of the digital twin. Data normalization techniques are also applied to standardize the data and make it compatible with the digital model. Additionally, feature extraction methods are used to identify and extract relevant features from the raw data, which can be used for further analysis and modeling.
Data integration is another critical aspect of the data collection process. This involves combining data from multiple sources, such as sensors, historical records, maintenance logs, and external databases, to create a comprehensive dataset. Data integration ensures that the digital twin has access to all relevant information, enabling it to provide accurate and holistic insights. Advanced data integration techniques, such as data fusion and data warehousing, are often employed to achieve this.
Once the data is preprocessed and integrated, it is fed into the digital model. The digital model is a virtual representation of the physical asset, designed to mimic its behavior and characteristics. The model uses the collected data to simulate the asset's performance, identify patterns and trends, and predict future outcomes. In AI-driven digital twins, machine learning algorithms are applied to the data to enhance the model's accuracy and predictive capabilities.
The data collection process is not a one-time activity but a continuous and iterative process. As the physical asset operates, new data is continuously collected and fed into the digital model. This real-time data ensures that the digital twin remains up-to-date and accurately reflects the current state of the asset. Additionally, the continuous flow of data allows the AI algorithms to learn and adapt over time, improving the digital twin's performance and reliability.
In summary, data collection is a critical and foundational step in the creation and operation of digital twins. It involves the deployment of sensors and IoT devices, the transmission of real-time data using communication technologies, and the preprocessing and integration of the collected data. The quality and comprehensiveness of the data collected directly impact the accuracy and effectiveness of the digital twin. By ensuring robust data collection processes, organizations can create digital twins that provide valuable insights, accurate predictions, and enhanced decision-making capabilities.

This diagram includes the following components:
1. Representing the real-world entity being monitored.
2. Collecting real-time data from the physical asset.
3. Handling data collection, preprocessing, and integration.
4. The virtual replica of the physical asset.
5. Analyzing data and providing insights.
6. Dashboards, mobile apps, or VR environments for stakeholder interaction.
7. Ensuring real-time data transmission.
8. This visual representation should help in understanding the complex interactions and components involved in an AI-driven digital twin system.
3.2. Real-Time Analysis
Real-time analysis is a critical component of AI-driven digital twins, enabling the continuous monitoring and assessment of physical assets or systems. This capability is essential for industries that require immediate feedback and decision-making to maintain optimal performance, safety, and efficiency. Real-time analysis involves the collection, processing, and interpretation of data as it is generated, allowing for instant insights and actions.
One of the primary benefits of real-time analysis in digital twins is the ability to detect anomalies and predict potential failures before they occur. By continuously monitoring the operational parameters of a physical asset, the digital twin can identify deviations from normal behavior that may indicate a problem. For example, in the manufacturing industry, real-time analysis can detect unusual vibrations in machinery, signaling potential mechanical issues that need immediate attention. This proactive approach helps prevent costly downtime and extends the lifespan of equipment.
Moreover, real-time analysis enhances decision-making by providing up-to-date information on the current state of the system. In the context of smart cities, for instance, digital twins can analyze traffic patterns in real-time to optimize traffic flow and reduce congestion. This dynamic adjustment can lead to more efficient use of infrastructure and improved quality of life for residents. Similarly, in healthcare, real-time analysis of patient data through digital twins can enable personalized treatment plans and timely interventions, improving patient outcomes.
The integration of advanced technologies such as the Internet of Things (IoT) and edge computing plays a significant role in enabling real-time analysis. IoT devices collect data from various sensors embedded in physical assets, while edge computing processes this data locally, reducing latency and ensuring rapid response times. This combination allows digital twins to operate with high efficiency and accuracy, even in environments with limited connectivity.
Furthermore, real-time analysis supports continuous improvement and optimization. By constantly evaluating performance metrics and comparing them against historical data, digital twins can identify areas for enhancement and implement changes on the fly. In the energy sector, for example, real-time analysis of power grid data can help balance supply and demand, reducing energy waste and lowering costs.
In conclusion, real-time analysis is a cornerstone of AI-driven digital twins, providing the ability to monitor, assess, and respond to changes in physical assets or systems instantaneously. This capability not only enhances operational efficiency and safety but also supports proactive maintenance, informed decision-making, and continuous optimization. As technology continues to advance, the potential applications and benefits of real-time analysis in digital twins will only expand, driving innovation across various industries.
3.3. Simulation and Prediction
Simulation and prediction are fundamental aspects of AI-driven digital twins, enabling the creation of virtual models that replicate the behavior and performance of physical assets or systems. These capabilities allow organizations to test scenarios, forecast outcomes, and make informed decisions without the risks and costs associated with real-world experimentation.
Simulation involves creating a digital replica of a physical asset or system, complete with all its components and interactions. This virtual model can be used to run various scenarios and analyze how the system would respond under different conditions. For example, in the aerospace industry, digital twins of aircraft engines can simulate different flight conditions to assess performance, identify potential issues, and optimize maintenance schedules. This approach not only enhances safety but also improves efficiency and reduces operational costs.
Prediction, on the other hand, leverages historical data and machine learning algorithms to forecast future behavior and outcomes. By analyzing past performance and identifying patterns, digital twins can predict potential failures, maintenance needs, and performance trends. In the context of smart manufacturing, for instance, predictive analytics can forecast equipment failures, allowing for timely maintenance and minimizing downtime. This proactive approach ensures that production processes run smoothly and efficiently.
The combination of simulation and prediction provides a powerful tool for decision-making and optimization. In the energy sector, digital twins of power plants can simulate different operating conditions and predict the impact of various factors such as weather, demand fluctuations, and equipment performance. This information can be used to optimize energy production, reduce emissions, and improve overall efficiency. Similarly, in urban planning, digital twins of cities can simulate the impact of new infrastructure projects, predict traffic patterns, and assess environmental effects, enabling more informed and sustainable development.
Moreover, simulation and prediction support innovation and experimentation. By testing new ideas and technologies in a virtual environment, organizations can explore different approaches and identify the most effective solutions without the risks and costs associated with real-world trials. In the automotive industry, for example, digital twins can simulate the performance of new vehicle designs, assess safety features, and optimize manufacturing processes, accelerating the development of innovative and efficient vehicles.
The integration of advanced technologies such as artificial intelligence (AI) and machine learning (ML) enhances the capabilities of simulation and prediction. AI algorithms can analyze vast amounts of data, identify complex patterns, and make accurate predictions, while ML models can continuously learn and improve over time. This dynamic and adaptive approach ensures that digital twins remain accurate and relevant, providing valuable insights and driving continuous improvement.
In conclusion, simulation and prediction are essential components of AI-driven digital twins, enabling organizations to create virtual models, test scenarios, and forecast outcomes. These capabilities support informed decision-making, proactive maintenance, and continuous optimization, driving efficiency, safety, and innovation across various industries. As technology continues to evolve, the potential applications and benefits of simulation and prediction in digital twins will continue to expand, offering new opportunities for growth and development.
For more on the intersection of AI and industrial simulations, check out Generative AI & Industrial Simulations: Innovate Fast.
4. Types of AI-Driven Digital Twins
AI-driven digital twins come in various forms, each tailored to specific applications and industries. These digital replicas leverage artificial intelligence to simulate, monitor, and optimize the performance of physical assets or systems. Understanding the different types of AI-driven digital twins can help organizations choose the right approach for their needs and maximize the benefits of this technology.
One common type of AI-driven digital twin is the product twin, which focuses on individual products or components. Product twins are used extensively in manufacturing and engineering to monitor the lifecycle of a product, from design and production to operation and maintenance. By creating a digital replica of a product, organizations can simulate different scenarios, predict potential issues, and optimize performance. For example, in the automotive industry, digital twins of engines can simulate various operating conditions, identify potential failures, and optimize maintenance schedules, ensuring reliability and efficiency.
Another type of AI-driven digital twin is the process twin, which models entire processes or workflows. Process twins are used to optimize complex operations, improve efficiency, and reduce costs. In the context of smart manufacturing, process twins can simulate production lines, analyze bottlenecks, and optimize workflows to enhance productivity. Similarly, in the healthcare industry, process twins can model patient care pathways, identify inefficiencies, and improve the delivery of healthcare services.
System twins are another category of AI-driven digital twins, focusing on entire systems or networks. These twins are used to monitor and optimize the performance of interconnected components and subsystems. In the energy sector, for example, digital twins of power grids can simulate different operating conditions, predict demand fluctuations, and optimize energy distribution. This approach ensures a reliable and efficient supply of energy while minimizing costs and environmental impact. Similarly, in the transportation industry, system twins can model entire transportation networks, optimize traffic flow, and improve the efficiency of logistics operations.
Environmental twins are a specialized type of AI-driven digital twin that focuses on natural and built environments. These twins are used to monitor and manage environmental conditions, assess the impact of human activities, and support sustainable development. In urban planning, for example, digital twins of cities can simulate the impact of new infrastructure projects, predict traffic patterns, and assess environmental effects, enabling more informed and sustainable development. Similarly, in agriculture, environmental twins can monitor soil conditions, weather patterns, and crop health, optimizing farming practices and improving yields.
Lastly, human twins are an emerging type of AI-driven digital twin that focuses on individual human beings. These twins are used to monitor and optimize health, performance, and well-being. In the healthcare industry, digital twins of patients can simulate the impact of different treatments, predict health outcomes, and personalize care plans. This approach enables more precise and effective healthcare, improving patient outcomes and reducing costs. Similarly, in sports and fitness, human twins can monitor athletes' performance, optimize training programs, and prevent injuries, enhancing overall performance and well-being.
In conclusion, AI-driven digital twins come in various forms, each tailored to specific applications and industries. Product twins focus on individual products, process twins model entire workflows, system twins optimize interconnected systems, environmental twins monitor natural and built environments, and human twins focus on individual human beings. By understanding the different types of AI-driven digital twins, organizations can choose the right approach for their needs and maximize the benefits of this technology, driving efficiency, innovation, and sustainability across various sectors.
For a deeper understanding of the types and benefits of AI, you can read Understanding AI as a Service (AIaaS): Benefits, Types, and Challenges and AI Agents: Types, Benefits & Real-World Uses.

4.1 Industrial Digital Twins
Industrial digital twins are virtual replicas of physical industrial assets, processes, or systems that are used to simulate, predict, and optimize performance in real-time. These digital twins leverage advanced technologies such as the Internet of Things (IoT), artificial intelligence (AI), machine learning (ML), and big data analytics to create a dynamic and interactive model of the industrial environment. The primary goal of industrial digital twins is to enhance operational efficiency, reduce downtime, and improve decision-making processes.
One of the key applications of industrial digital twins is in predictive maintenance. By continuously monitoring the condition of machinery and equipment through sensors and IoT devices, digital twins can predict potential failures before they occur. This allows maintenance teams to perform timely interventions, thereby reducing unplanned downtime and extending the lifespan of assets. For example, General Electric (GE) uses digital twins to monitor and maintain its fleet of jet engines, resulting in significant cost savings and improved reliability.
Another important application is in process optimization. Digital twins can simulate various scenarios and identify the most efficient ways to operate industrial processes. This is particularly valuable in complex manufacturing environments where small adjustments can lead to significant improvements in productivity and cost savings. For instance, Siemens uses digital twins to optimize the production processes in its factories, leading to increased efficiency and reduced waste.
Digital twins also play a crucial role in the design and development of new products. By creating a virtual prototype, engineers can test and refine their designs before committing to physical production. This not only accelerates the development process but also reduces the risk of costly design errors. Companies like Tesla use digital twins to simulate and test their electric vehicles, ensuring that they meet high standards of performance and safety.
In addition to these applications, industrial digital twins are increasingly being used for supply chain management. By providing real-time visibility into the status of goods and materials, digital twins can help companies optimize their supply chains, reduce lead times, and improve customer satisfaction. For example, DHL uses digital twins to monitor and manage its global logistics network, ensuring that deliveries are made on time and at the lowest possible cost.
The adoption of industrial digital twins is expected to grow rapidly in the coming years, driven by advancements in AI, IoT, and data analytics. According to a report by MarketsandMarkets, the global digital twin market is projected to reach $48.2 billion by 2026, up from $3.8 billion in 2021. This growth is being fueled by the increasing demand for operational efficiency, the need for real-time monitoring and predictive maintenance, and the rising adoption of IoT and AI technologies.
4.2 Human Behavior Modeling Digital Twins
Human behavior modeling digital twins are virtual representations of individuals or groups that simulate their behaviors, actions, and interactions in various environments. These digital twins are created using a combination of data from sensors, wearables, social media, and other sources, along with advanced AI and machine learning algorithms. The primary objective of human behavior modeling digital twins is to understand, predict, and influence human behavior in a wide range of applications, from healthcare and education to marketing and urban planning.
In healthcare, human behavior modeling digital twins can be used to personalize treatment plans and improve patient outcomes. By analyzing data from wearable devices, electronic health records, and other sources, digital twins can create a comprehensive profile of a patient's health and behavior. This allows healthcare providers to tailor interventions to the specific needs and preferences of each patient. For example, digital twins can help manage chronic conditions such as diabetes by predicting blood sugar levels and recommending personalized diet and exercise plans.
In the field of education, human behavior modeling digital twins can enhance the learning experience by providing personalized feedback and support to students. By analyzing data on student performance, engagement, and behavior, digital twins can identify areas where students are struggling and suggest targeted interventions. This can help educators create more effective and inclusive learning environments. For instance, digital twins can be used to simulate different teaching strategies and identify the most effective approaches for individual students.
Marketing is another area where human behavior modeling digital twins can have a significant impact. By analyzing data on consumer behavior, preferences, and interactions, digital twins can help companies create more targeted and effective marketing campaigns. This can lead to increased customer engagement, higher conversion rates, and improved brand loyalty. For example, digital twins can be used to simulate different marketing strategies and predict their impact on consumer behavior, allowing companies to optimize their marketing efforts.
Urban planning is yet another application of human behavior modeling digital twins. By simulating the behavior and interactions of residents in a city, digital twins can help urban planners design more efficient and livable urban environments. This can include optimizing traffic flow, improving public transportation, and enhancing public safety. For instance, digital twins can be used to simulate the impact of new infrastructure projects on traffic patterns and identify potential bottlenecks before they occur.
The development and adoption of human behavior modeling digital twins are being driven by advancements in AI, machine learning, and data analytics. According to a report by Grand View Research, the global digital twin market in the healthcare sector alone is expected to reach $3.1 billion by 2025, up from $1.1 billion in 2020. This growth is being fueled by the increasing demand for personalized healthcare, the rising adoption of wearable devices, and the growing availability of big data.
5. Benefits of AI-Driven Digital Twins
AI-driven digital twins offer a multitude of benefits across various industries by leveraging the power of artificial intelligence to create highly accurate and dynamic virtual models of physical assets, processes, or systems. These benefits include improved operational efficiency, enhanced decision-making, reduced costs, and increased innovation.
One of the primary benefits of AI-driven digital twins is the ability to improve operational efficiency. By continuously monitoring and analyzing data from physical assets and processes, digital twins can identify inefficiencies and recommend corrective actions in real-time. This leads to optimized performance, reduced downtime, and increased productivity. For example, in the manufacturing industry, AI-driven digital twins can monitor the condition of machinery and predict potential failures, allowing maintenance teams to perform timely interventions and avoid costly breakdowns.
Enhanced decision-making is another significant benefit of AI-driven digital twins. By providing real-time insights and predictive analytics, digital twins enable organizations to make more informed and data-driven decisions. This is particularly valuable in complex and dynamic environments where traditional decision-making processes may be insufficient. For instance, in the energy sector, digital twins can simulate different scenarios and predict the impact of various factors on energy production and consumption, helping companies optimize their operations and reduce costs.
Cost reduction is a key advantage of AI-driven digital twins. By enabling predictive maintenance, process optimization, and efficient resource management, digital twins can significantly reduce operational costs. In addition, digital twins can help organizations avoid costly design errors and accelerate the development process by allowing engineers to test and refine their designs in a virtual environment before committing to physical production. This is particularly valuable in industries such as aerospace and automotive, where the cost of design errors can be extremely high.
AI-driven digital twins also foster innovation by providing a platform for experimentation and simulation. By creating virtual prototypes and testing different scenarios, organizations can explore new ideas and approaches without the risk and cost associated with physical experimentation. This can lead to the development of new products, services, and business models. For example, in the healthcare industry, digital twins can be used to simulate the effects of new treatments and interventions, leading to the development of more effective and personalized healthcare solutions.
Furthermore, AI-driven digital twins can enhance collaboration and communication within organizations. By providing a shared and interactive virtual model, digital twins enable different teams and stakeholders to collaborate more effectively and make better-informed decisions. This is particularly valuable in large and complex projects where coordination and communication are critical to success. For instance, in the construction industry, digital twins can be used to create a virtual model of a building project, allowing architects, engineers, and contractors to collaborate and identify potential issues before construction begins.
The adoption of AI-driven digital twins is expected to grow rapidly in the coming years, driven by advancements in AI, IoT, and data analytics. According to a report by Gartner, by 2023, 50% of large industrial companies will use digital twins, resulting in a 10% improvement in effectiveness. This growth is being fueled by the increasing demand for operational efficiency, the need for real-time monitoring and predictive maintenance, and the rising adoption of AI and IoT technologies. As organizations continue to recognize the value of AI-driven digital twins, their adoption is likely to become more widespread, leading to significant improvements in efficiency, decision-making, and innovation across various industries.
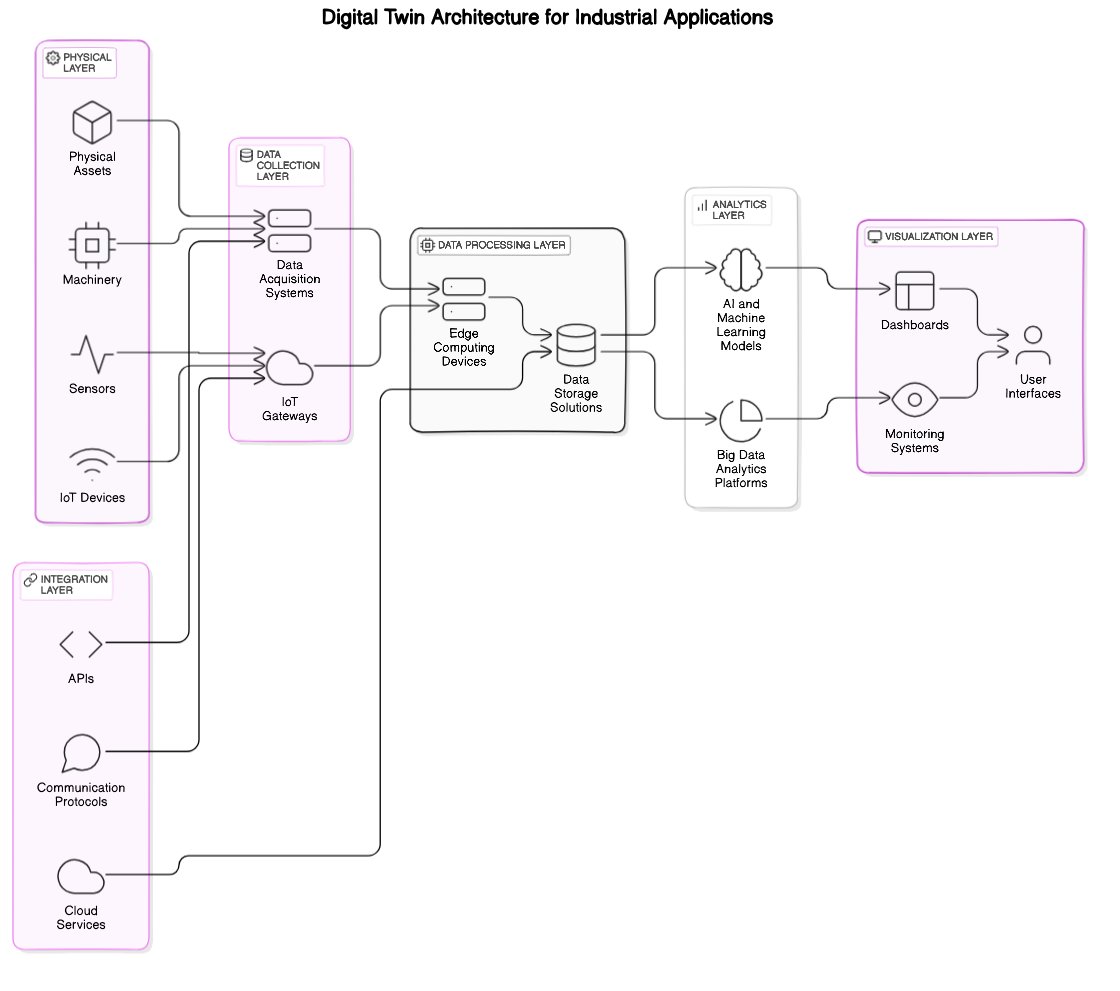
5.1. Enhanced Decision Making
Enhanced decision making is a critical advantage that modern technology and data analytics bring to various sectors, including business, healthcare, and public administration. The ability to make informed decisions based on accurate, real-time data can significantly impact the effectiveness and efficiency of operations.
In the business world, enhanced decision making is often facilitated by advanced analytics and business intelligence tools. These tools collect and analyze vast amounts of data from various sources, providing insights that were previously unattainable. For instance, predictive analytics can forecast market trends, helping companies to anticipate changes and adjust their strategies accordingly. This proactive approach can lead to better resource allocation, improved customer satisfaction, and increased profitability. According to a report by McKinsey, companies that leverage data-driven decision making are 23 times more likely to acquire customers, six times as likely to retain customers, and 19 times as likely to be profitable as a result.
In healthcare, enhanced decision making can lead to better patient outcomes and more efficient use of resources. Electronic Health Records (EHRs) and other health information technologies enable healthcare providers to access comprehensive patient data quickly. This data can include medical history, lab results, and treatment plans, allowing for more accurate diagnoses and personalized treatment plans. Additionally, data analytics can identify patterns and trends in patient data, helping to predict potential health issues before they become critical. For example, predictive models can identify patients at high risk of readmission, enabling healthcare providers to intervene early and reduce hospital readmission rates.
Public administration also benefits from enhanced decision making through the use of data analytics and smart technologies. Governments can use data to improve public services, optimize resource allocation, and enhance transparency and accountability. For example, smart city initiatives use data from various sources, such as traffic sensors and social media, to improve urban planning and management. This can lead to more efficient public transportation systems, better waste management, and improved emergency response times.
For more insights on how AI is revolutionizing decision-making, you can explore Predictive Analytics: AI for High-Stakes Decisions.
In conclusion, enhanced decision making, driven by advanced data analytics and technology, is transforming various sectors by providing valuable insights and enabling more informed, proactive decisions. This leads to improved efficiency, better resource allocation, and ultimately, more successful outcomes.
5.2. Cost Efficiency
Cost efficiency is a fundamental goal for any organization, as it directly impacts profitability and sustainability. Achieving cost efficiency involves optimizing resources, reducing waste, and improving processes to deliver products or services at a lower cost without compromising quality.
One of the primary ways organizations achieve cost efficiency is through automation. Automation technologies, such as robotic process automation (RPA) and artificial intelligence (AI), can perform repetitive and time-consuming tasks more quickly and accurately than humans. This not only reduces labor costs but also minimizes errors and increases productivity. For example, in manufacturing, the use of automated assembly lines can significantly reduce production costs while maintaining high-quality standards. According to a study by Deloitte, companies that implement RPA can achieve cost savings of 20% to 30% on average.
Another key factor in cost efficiency is supply chain optimization. Efficient supply chain management ensures that materials and products are delivered at the right time, in the right quantities, and at the lowest possible cost. This can be achieved through various strategies, such as just-in-time (JIT) inventory management, which reduces inventory holding costs by receiving goods only when they are needed for production. Additionally, advanced analytics can help identify inefficiencies in the supply chain and suggest improvements, such as optimizing transportation routes or consolidating shipments to reduce shipping costs.
Energy efficiency is also a critical component of cost efficiency, particularly for industries with high energy consumption. Implementing energy-efficient technologies and practices can lead to significant cost savings. For example, upgrading to energy-efficient lighting, heating, and cooling systems can reduce energy bills. Additionally, investing in renewable energy sources, such as solar or wind power, can provide long-term cost savings and reduce dependence on fossil fuels.
In the service industry, cost efficiency can be achieved through process improvement and lean management techniques. Lean management focuses on eliminating waste and improving processes to deliver value to customers more efficiently. This can involve streamlining workflows, reducing unnecessary steps, and improving communication and collaboration among team members. For example, in healthcare, lean management techniques can reduce patient wait times, improve the utilization of medical equipment, and enhance overall patient care.
In conclusion, cost efficiency is essential for the success and sustainability of any organization. By leveraging automation, optimizing supply chains, improving energy efficiency, and implementing lean management techniques, organizations can reduce costs, improve productivity, and maintain high-quality standards.
5.3. Improved Safety
Improved safety is a paramount concern across various sectors, including manufacturing, healthcare, transportation, and public safety. Advances in technology and data analytics have significantly enhanced safety measures, reducing the risk of accidents, injuries, and fatalities.
In the manufacturing industry, improved safety is achieved through the implementation of advanced safety protocols and technologies. For example, the use of automation and robotics can reduce the need for human workers to perform dangerous tasks, such as handling hazardous materials or operating heavy machinery. Additionally, wearable technology, such as smart helmets and vests, can monitor workers' vital signs and environmental conditions, alerting them to potential hazards in real-time. According to the Occupational Safety and Health Administration (OSHA), implementing comprehensive safety and health programs can reduce workplace injuries and illnesses by 20% to 40%.
In healthcare, improved safety is critical for both patients and healthcare providers. Electronic Health Records (EHRs) and other health information technologies enhance patient safety by providing accurate and up-to-date information, reducing the risk of medical errors. For example, computerized physician order entry (CPOE) systems can prevent medication errors by ensuring that prescriptions are accurate and checking for potential drug interactions. Additionally, infection control measures, such as hand hygiene protocols and the use of personal protective equipment (PPE), are essential for preventing the spread of infections in healthcare settings.
Transportation safety has also been significantly improved through the use of advanced technologies. For example, autonomous vehicles and advanced driver-assistance systems (ADAS) can reduce the risk of accidents by assisting drivers with tasks such as lane-keeping, adaptive cruise control, and automatic emergency braking. Additionally, data analytics can be used to identify high-risk areas and times for accidents, allowing for targeted interventions, such as increased law enforcement presence or improved road infrastructure.
Public safety is another area where technology has made significant strides. Smart city initiatives use data from various sources, such as surveillance cameras, social media, and emergency response systems, to enhance public safety. For example, predictive policing uses data analytics to identify potential crime hotspots and allocate law enforcement resources more effectively. Additionally, emergency response systems can use real-time data to coordinate responses to natural disasters, fires, and other emergencies, improving the efficiency and effectiveness of rescue efforts.
In conclusion, improved safety is a critical benefit of modern technology and data analytics. By implementing advanced safety protocols and technologies, organizations can reduce the risk of accidents, injuries, and fatalities, ensuring a safer environment for workers, patients, and the general public.
6. Challenges in Implementing AI-Driven Digital Twins
The implementation of AI-driven digital twins, while promising significant advancements in various industries, is fraught with numerous challenges. These challenges can be broadly categorized into data privacy concerns and integration complexity. Each of these aspects presents unique hurdles that need to be addressed to fully leverage the potential of digital twins.
6.1. Data Privacy Concerns
One of the most pressing challenges in implementing AI-driven digital twins is data privacy. Digital twins rely heavily on vast amounts of data to create accurate and functional virtual replicas of physical entities. This data often includes sensitive information, whether it pertains to individuals, businesses, or critical infrastructure. The collection, storage, and processing of such data raise significant privacy concerns.
Firstly, the sheer volume of data required for digital twins can be staggering. For instance, in healthcare, digital twins of patients would necessitate continuous monitoring and collection of personal health data, which is highly sensitive. Ensuring that this data is protected from unauthorized access and breaches is paramount. The General Data Protection Regulation (GDPR) in Europe and the Health Insurance Portability and Accountability Act (HIPAA) in the United States are examples of regulations that impose strict requirements on how personal data should be handled. Non-compliance with these regulations can result in severe penalties and loss of trust.
Moreover, the use of AI in digital twins introduces additional layers of complexity to data privacy. AI algorithms require large datasets to train and improve their accuracy. This often involves sharing data across different platforms and stakeholders, increasing the risk of data exposure. Ensuring that data is anonymized and encrypted can mitigate some risks, but it is not a foolproof solution. There is always the potential for re-identification of anonymized data, especially when combined with other datasets.
Another concern is the ethical use of data. AI-driven digital twins can make decisions or predictions based on the data they process. If the data is biased or incomplete, it can lead to unfair or harmful outcomes. For example, in predictive maintenance for industrial equipment, biased data could result in incorrect predictions, leading to unnecessary downtime or even accidents. Ensuring that the data used is accurate, unbiased, and ethically sourced is crucial.
Finally, there is the issue of data ownership. With multiple stakeholders involved in the creation and use of digital twins, determining who owns the data and who has the right to access and use it can be contentious. Clear policies and agreements need to be established to address these issues and protect the interests of all parties involved.
For more insights on developing privacy-centric AI models, you can refer to Develop Privacy-Centric Language Models: Essential Steps.
6.2. Integration Complexity
Another significant challenge in implementing AI-driven digital twins is integration complexity. Digital twins require the seamless integration of various technologies, systems, and data sources to function effectively. This integration is often complex and fraught with technical and organizational challenges.
One of the primary technical challenges is the interoperability of different systems and technologies. Digital twins often need to integrate data from various sources, such as sensors, IoT devices, enterprise systems, and external databases. These systems may use different protocols, data formats, and standards, making integration difficult. Developing a unified framework that can seamlessly integrate these disparate systems is a significant technical hurdle.
Moreover, the integration of AI algorithms into digital twins adds another layer of complexity. AI models need to be trained, validated, and continuously updated with new data. This requires robust data pipelines and infrastructure to ensure that data flows smoothly between different components of the digital twin. Ensuring that the AI models are accurate and reliable also requires continuous monitoring and validation, which can be resource-intensive.
Organizational challenges also play a significant role in integration complexity. Implementing digital twins often requires collaboration between different departments and stakeholders within an organization. This can lead to issues related to communication, coordination, and alignment of goals. For example, the IT department may be responsible for the technical implementation, while the operations team may be focused on the practical applications of the digital twin. Ensuring that all stakeholders are aligned and working towards a common goal is crucial for successful implementation.
Additionally, there is the challenge of legacy systems. Many organizations have existing systems and infrastructure that may not be compatible with the new technologies required for digital twins. Upgrading or replacing these legacy systems can be costly and time-consuming. Organizations need to carefully plan and manage the transition to ensure minimal disruption to their operations.
Finally, there is the issue of scalability. As digital twins become more complex and incorporate more data and functionalities, ensuring that the underlying infrastructure can scale to meet the increased demands is crucial. This requires careful planning and investment in scalable technologies and infrastructure.
For a comprehensive understanding of integration in AI, you can explore Guide to Generative Integration in AI.
In conclusion, while AI-driven digital twins hold immense potential, their implementation is not without challenges. Addressing data privacy concerns and integration complexity is crucial to unlocking the full potential of digital twins and ensuring their successful adoption across various industries.
6.3. High Initial Costs
The implementation of digital twins, particularly those driven by artificial intelligence (AI), often comes with high initial costs. These costs can be attributed to several factors, including the need for advanced hardware, sophisticated software, and skilled personnel. The hardware required for digital twins includes high-performance computing systems, sensors, and other IoT devices that can collect and process vast amounts of data in real-time. These components are not only expensive to purchase but also to maintain and upgrade over time.
Software development for digital twins is another significant cost driver. Creating a digital twin involves developing complex algorithms and models that can accurately replicate the physical entity or system. This requires specialized software tools and platforms, which can be costly. Additionally, the integration of AI into digital twins necessitates the use of advanced machine learning and data analytics tools, which further adds to the expense.
The human resources required to develop and maintain digital twins also contribute to the high initial costs. Skilled professionals such as data scientists, software engineers, and domain experts are essential for the successful implementation of digital twins. These experts command high salaries due to their specialized knowledge and expertise. Moreover, ongoing training and development are necessary to keep these professionals up-to-date with the latest advancements in the field.
Another factor contributing to the high initial costs is the need for extensive data collection and processing. Digital twins rely on large volumes of data to create accurate and reliable models. Collecting this data often involves deploying numerous sensors and IoT devices, which can be expensive. Additionally, the data must be processed and analyzed, requiring significant computational resources and storage capacity.
Despite the high initial costs, the long-term benefits of digital twins can outweigh the initial investment. Digital twins can lead to improved efficiency, reduced downtime, and better decision-making, ultimately resulting in cost savings and increased profitability. However, organizations must carefully consider the initial costs and weigh them against the potential benefits before embarking on a digital twin implementation.
7. Future of AI-Driven Digital Twins
The future of AI-driven digital twins is promising, with numerous advancements and applications on the horizon. As technology continues to evolve, digital twins are expected to become more sophisticated, accurate, and widely adopted across various industries. One of the key drivers of this growth is the increasing availability of data and the advancements in AI and machine learning technologies.
In the future, AI-driven digital twins are likely to become more autonomous and capable of making real-time decisions without human intervention. This will be made possible by the continuous improvement of AI algorithms and the integration of advanced data analytics techniques. As a result, digital twins will be able to predict and respond to changes in their physical counterparts more effectively, leading to improved performance and reduced downtime.
Another significant development in the future of AI-driven digital twins is the increased use of edge computing. Edge computing involves processing data closer to the source, rather than relying on centralized data centers. This approach can reduce latency and improve the efficiency of digital twins, enabling them to operate in real-time and provide more accurate insights. As edge computing technology advances, it is expected to play a crucial role in the evolution of digital twins.
The future of AI-driven digital twins also includes the expansion of their applications across various industries. While digital twins are already being used in sectors such as manufacturing, healthcare, and smart cities, their adoption is expected to grow in other areas such as agriculture, energy, and transportation. For example, in agriculture, digital twins can be used to monitor crop health, optimize irrigation, and predict yields, leading to more efficient and sustainable farming practices. In the energy sector, digital twins can help optimize the performance of renewable energy systems, such as wind turbines and solar panels, by predicting maintenance needs and improving energy efficiency.
Collaboration and standardization will also play a crucial role in the future of AI-driven digital twins. As more organizations adopt digital twin technology, there will be a growing need for standardized protocols and frameworks to ensure interoperability and data sharing. Industry consortia and standards organizations are expected to play a key role in developing these standards, facilitating the widespread adoption and integration of digital twins. For more on the future of AI-driven digital twins, you can read AI-Driven Digital Twins: Revolutionizing Industries.
7.1. Technological Advancements
Technological advancements are at the core of the evolution and future potential of AI-driven digital twins. One of the most significant advancements is in the field of artificial intelligence and machine learning. These technologies are becoming increasingly sophisticated, enabling digital twins to process and analyze vast amounts of data more efficiently and accurately. Machine learning algorithms can identify patterns and trends in data that may not be immediately apparent to human analysts, leading to more accurate predictions and insights.
Another critical technological advancement is the development of more powerful and efficient computing systems. High-performance computing (HPC) and quantum computing are expected to play a significant role in the future of digital twins. These advanced computing systems can handle the complex calculations and data processing required for creating and maintaining digital twins, enabling them to operate in real-time and provide more accurate and reliable insights.
The Internet of Things (IoT) is another area where technological advancements are driving the evolution of digital twins. The proliferation of IoT devices and sensors is leading to the generation of vast amounts of data that can be used to create more detailed and accurate digital twins. Advances in sensor technology are also enabling the collection of more precise and diverse data, further enhancing the capabilities of digital twins.
Advancements in data storage and management technologies are also crucial for the future of digital twins. As the volume of data generated by IoT devices and other sources continues to grow, there is a need for more efficient and scalable data storage solutions. Technologies such as cloud computing and distributed ledger technology (DLT) are expected to play a significant role in addressing these challenges, providing the necessary infrastructure for storing and managing the vast amounts of data required for digital twins.
Finally, advancements in visualization and user interface technologies are enhancing the usability and accessibility of digital twins. Technologies such as augmented reality (AR) and virtual reality (VR) are enabling more intuitive and immersive interactions with digital twins, allowing users to visualize and interact with complex data and models in a more meaningful way. These advancements are making digital twins more accessible to a broader range of users, from engineers and data scientists to decision-makers and frontline workers.
In conclusion, technological advancements in AI, computing, IoT, data storage, and visualization are driving the evolution and future potential of AI-driven digital twins. These advancements are enabling digital twins to become more sophisticated, accurate, and widely adopted across various industries, leading to improved efficiency, reduced downtime, and better decision-making. As technology continues to evolve, the capabilities and applications of digital twins are expected to expand, unlocking new opportunities and benefits for organizations and society as a whole. For more on the future of AI advancements, you can explore The Future of AI: Generative Integration.
7.2. Broader Applications
The concept of user proxies extends beyond the realm of individual users and can be applied to a variety of broader contexts. One significant application is in the field of artificial intelligence and machine learning. Here, user proxies can be used to simulate user behavior, which is crucial for training algorithms. For instance, in recommendation systems, user proxies can help in generating synthetic data that mimics real user interactions, thereby allowing the system to learn and adapt without the need for extensive real-world data. This is particularly useful in the early stages of development when real user data may be scarce or unavailable.
Another important application is in cybersecurity. User proxies can be employed to detect and prevent fraudulent activities. By creating a baseline of normal user behavior, any deviations can be flagged as potential security threats. This is especially relevant in financial institutions where the stakes are high, and the cost of fraud can be enormous. User proxies can also be used in penetration testing to simulate attacks and identify vulnerabilities in a system, thereby enhancing its security posture.
In the realm of digital marketing, user proxies can be invaluable for A/B testing and personalization. Marketers can use proxies to simulate different user segments and test various marketing strategies to see which ones yield the best results. This can lead to more effective campaigns and a better return on investment. Additionally, user proxies can help in understanding customer journeys and identifying pain points, thereby enabling businesses to improve their products and services.
Healthcare is another sector where user proxies can have a significant impact. In telemedicine, for example, user proxies can be used to simulate patient interactions, helping healthcare providers to better understand patient needs and improve the quality of care. In clinical trials, user proxies can help in simulating patient responses to new treatments, thereby speeding up the research process and reducing costs.
Education is yet another field where user proxies can be beneficial. In online learning platforms, user proxies can be used to simulate student interactions, helping educators to design more effective courses and identify areas where students may struggle. This can lead to a more personalized learning experience and better educational outcomes.
In summary, the broader applications of user proxies are vast and varied, spanning multiple industries and use cases. From artificial intelligence and cybersecurity to digital marketing, healthcare, and education, user proxies offer a powerful tool for simulating user behavior, enhancing security, improving personalization, and driving better outcomes. As technology continues to evolve, the potential applications of user proxies are likely to expand even further, offering new opportunities for innovation and improvement across various sectors. For more insights on AI applications, you can explore AI-Driven Digital Twins: Revolutionizing Industries.
8. Real-World Examples
The concept of user proxies is not just theoretical; it has been implemented in various real-world scenarios with significant success. One notable example is in the realm of e-commerce. Companies like Amazon and eBay use sophisticated algorithms that act as user proxies to recommend products to their customers. These algorithms analyze past behavior, preferences, and even browsing history to make personalized recommendations. This not only enhances the user experience but also drives sales and increases customer loyalty. According to a report by McKinsey, personalized recommendations can increase sales by up to 10% (source: https://www.mckinsey.com/business-functions/marketing-and-sales/our-insights/the-future-of-personalization-and-how-to-get-ready-for-it).
Another real-world example is in the field of social media. Platforms like Facebook and Instagram use user proxies to curate content for their users. These proxies analyze user interactions, such as likes, shares, and comments, to determine what content is most relevant to each user. This ensures that users are more engaged and spend more time on the platform, which in turn increases ad revenue for the company. A study by Hootsuite found that personalized content can increase user engagement by up to 20% (source: https://blog.hootsuite.com/social-media-statistics-for-social-media-managers/).
In the financial sector, user proxies are used for fraud detection and prevention. Banks and financial institutions use machine learning algorithms to create profiles of normal user behavior. Any deviation from this behavior can trigger an alert, helping to prevent fraudulent activities. For example, if a user's account suddenly shows transactions from a different country, the system can flag this as suspicious and take appropriate action. According to a report by the Association of Certified Fraud Examiners, companies that use data analytics for fraud detection can reduce fraud losses by up to 54% (source: https://www.acfe.com/report-to-the-nations/2020/).
Healthcare is another area where user proxies have proven to be beneficial. Telemedicine platforms use user proxies to simulate patient interactions, helping healthcare providers to better understand patient needs and improve the quality of care. For instance, AI-driven chatbots can act as user proxies to triage patients, providing initial consultations and directing them to the appropriate healthcare professional. This not only improves efficiency but also ensures that patients receive timely care.
In summary, the real-world applications of user proxies are diverse and impactful. From e-commerce and social media to finance and healthcare, user proxies are being used to enhance user experience, improve security, and drive better outcomes. As technology continues to advance, the use of user proxies is likely to become even more widespread, offering new opportunities for innovation and improvement across various sectors. For more examples of AI in action, check out A Guide to AI in Insurance.
8.1. Industrial Use Cases
User proxies have found significant applications in various industrial sectors, revolutionizing the way businesses operate and interact with their customers. One prominent use case is in the manufacturing industry. Here, user proxies can be used to simulate the behavior of machines and equipment, helping to predict maintenance needs and prevent downtime. For instance, predictive maintenance systems use user proxies to analyze data from sensors and predict when a machine is likely to fail. This allows companies to perform maintenance proactively, reducing downtime and saving costs. According to a report by Deloitte, predictive maintenance can reduce maintenance costs by up to 25% and downtime by up to 70% (source: https://www2.deloitte.com/us/en/insights/focus/industry-4-0/predictive-maintenance.html).
In the automotive industry, user proxies are used to enhance the development and testing of autonomous vehicles. By simulating various driving scenarios and user behaviors, companies can test the performance and safety of their autonomous systems without the need for extensive real-world testing. This not only speeds up the development process but also ensures that the vehicles are safe and reliable. For example, companies like Tesla and Waymo use user proxies to simulate millions of miles of driving, helping to refine their autonomous systems and improve safety.
The energy sector is another area where user proxies have proven to be valuable. In smart grid systems, user proxies can be used to simulate the behavior of consumers and predict energy demand. This allows utility companies to optimize energy distribution and reduce waste. For instance, by analyzing data from smart meters, user proxies can predict when energy demand is likely to peak and adjust the supply accordingly. This not only improves efficiency but also helps to reduce costs and environmental impact.
In the retail industry, user proxies are used to enhance customer experience and drive sales. For example, retailers can use user proxies to simulate customer behavior and optimize store layouts. By analyzing data on how customers move through the store and interact with products, retailers can design layouts that encourage more purchases and improve the overall shopping experience. Additionally, user proxies can be used to personalize marketing campaigns and promotions, ensuring that customers receive relevant offers and recommendations.
In summary, the industrial use cases of user proxies are diverse and impactful. From manufacturing and automotive to energy and retail, user proxies are being used to enhance efficiency, improve safety, and drive better outcomes. As technology continues to advance, the use of user proxies in industrial applications is likely to become even more widespread, offering new opportunities for innovation and improvement across various sectors. For more on industrial applications of AI, explore Industrial Evolution: Digital Twins and AI.
8.2. Human Behavior Modeling Use Cases
Human behavior modeling is a critical aspect of various fields, including psychology, sociology, marketing, and artificial intelligence. By understanding and predicting human behavior, organizations can make more informed decisions, improve user experiences, and enhance the effectiveness of their strategies. Here are some key use cases of human behavior modeling:
1. Marketing and Advertising
Companies use human behavior modeling to understand consumer preferences and predict purchasing behavior. By analyzing data from social media, online browsing habits, and purchase history, businesses can create personalized marketing campaigns that target specific demographics. This approach increases the likelihood of converting potential customers into actual buyers. For instance, Amazon uses sophisticated algorithms to recommend products based on a user's past behavior, leading to increased sales and customer satisfaction.
2. Healthcare
In the healthcare sector, human behavior modeling can predict patient outcomes and improve treatment plans. By analyzing patient data, such as medical history, lifestyle choices, and genetic information, healthcare providers can identify patterns that indicate the likelihood of developing certain conditions. This predictive capability allows for early intervention and personalized treatment plans, ultimately improving patient outcomes. For example, machine learning models can predict the likelihood of a patient developing diabetes based on their lifestyle and genetic factors, enabling preventive measures to be taken.
3. Urban Planning and Traffic Management
Human behavior modeling is also used in urban planning to design more efficient and livable cities. By analyzing data on how people move and interact within urban environments, planners can optimize public transportation systems, reduce traffic congestion, and improve the overall quality of life for residents. For instance, smart traffic management systems use real-time data to predict traffic patterns and adjust traffic signals accordingly, reducing congestion and improving traffic flow.
4. Education
In the field of education, human behavior modeling can enhance learning experiences and outcomes. By analyzing student data, such as attendance, participation, and performance, educators can identify students who may be at risk of falling behind and provide targeted interventions. Additionally, personalized learning platforms use behavior modeling to adapt educational content to individual students' needs, improving engagement and retention. For example, platforms like Khan Academy use data analytics to recommend personalized learning paths for students based on their performance and learning style.
5. Security and Fraud Detection
Human behavior modeling is crucial in the field of security and fraud detection. By analyzing patterns of behavior, such as login times, transaction history, and online activity, organizations can identify anomalies that may indicate fraudulent activity. This proactive approach allows for the early detection and prevention of security breaches and financial fraud. For instance, banks use machine learning models to detect unusual transaction patterns that may indicate credit card fraud, enabling them to take swift action to protect their customers.
6. Human-Computer Interaction (HCI)
In the realm of HCI, human behavior modeling is used to design more intuitive and user-friendly interfaces. By understanding how users interact with technology, designers can create interfaces that are more responsive to users' needs and preferences. This approach enhances the overall user experience and increases the effectiveness of digital products. For example, voice-activated assistants like Siri and Alexa use natural language processing and behavior modeling to understand and respond to user commands more accurately.
In conclusion, human behavior modeling has a wide range of applications across various fields. By leveraging data and advanced analytics, organizations can gain valuable insights into human behavior, leading to more informed decision-making and improved outcomes. As technology continues to advance, the potential for human behavior modeling to transform industries and improve lives will only grow.
9. In-Depth Explanations
In-depth explanations are essential for understanding complex concepts and processes. They provide detailed information, context, and insights that help individuals grasp the intricacies of a subject. In various fields, from education to technology, in-depth explanations play a crucial role in enhancing knowledge and facilitating informed decision-making. Here are some key aspects of in-depth explanations:
1. Clarity and Detail
In-depth explanations break down complex concepts into simpler, more understandable components. They provide detailed information, including definitions, examples, and step-by-step processes, to ensure that the audience fully comprehends the subject matter. For instance, in a scientific paper, an in-depth explanation of a research methodology would include a detailed description of the experimental design, data collection methods, and statistical analyses used.
2. Context and Background
Providing context and background information is essential for in-depth explanations. This includes historical context, relevant theories, and prior research that has contributed to the current understanding of the topic. By situating the subject within a broader framework, in-depth explanations help the audience appreciate its significance and relevance. For example, an in-depth explanation of climate change would include information on the history of climate science, key discoveries, and the impact of human activities on the environment.
3. Examples and Analogies
Using examples and analogies is an effective way to enhance in-depth explanations. Examples provide concrete instances that illustrate abstract concepts, making them more relatable and easier to understand. Analogies, on the other hand, draw comparisons between unfamiliar concepts and familiar ones, helping the audience grasp new ideas by relating them to known experiences. For instance, explaining the concept of blockchain technology using the analogy of a digital ledger can help individuals understand its decentralized and secure nature.
4. Visual Aids
Visual aids, such as diagrams, charts, and illustrations, are valuable tools for in-depth explanations. They provide a visual representation of complex information, making it easier to comprehend and retain. Visual aids can also highlight key points and relationships between different components of a concept. For example, a flowchart explaining the process of photosynthesis can help students visualize the sequence of events and the interactions between different molecules.
5. Addressing Common Misconceptions
In-depth explanations often address common misconceptions and clarify misunderstandings. By identifying and correcting these misconceptions, they ensure that the audience has an accurate and thorough understanding of the subject. For instance, an in-depth explanation of vaccines would address common myths and misconceptions, such as the false belief that vaccines cause autism, and provide evidence-based information to dispel these myths.
6. Interdisciplinary Connections
In-depth explanations often highlight connections between different fields and disciplines. By showing how a concept or process is related to other areas of knowledge, they provide a more comprehensive understanding and encourage interdisciplinary thinking. For example, an in-depth explanation of artificial intelligence (AI) would explore its applications in various fields, such as healthcare, finance, and transportation, and discuss the ethical and societal implications of AI technologies.
7. Critical Analysis and Evaluation
In-depth explanations involve critical analysis and evaluation of the subject matter. This includes examining different perspectives, identifying strengths and weaknesses, and discussing potential implications and future directions. By encouraging critical thinking, in-depth explanations help the audience develop a more nuanced and informed understanding. For instance, an in-depth explanation of a new medical treatment would include an evaluation of its efficacy, potential side effects, and ethical considerations.
In conclusion, in-depth explanations are essential for understanding complex concepts and processes. They provide clarity, context, and detailed information, helping individuals grasp the intricacies of a subject. By using examples, visual aids, and addressing common misconceptions, in-depth explanations enhance knowledge and facilitate informed decision-making. As the world becomes increasingly complex, the importance of in-depth explanations in education, technology, and other fields will continue to grow.
9.1. Machine Learning Algorithms in Digital Twins
Machine learning algorithms play a pivotal role in the development and functionality of digital twins. Digital twins are virtual replicas of physical systems, processes, or entities that are used to simulate, predict, and optimize real-world operations. By integrating machine learning algorithms, digital twins can analyze vast amounts of data, learn from it, and make informed decisions in real-time. Here are some key aspects of machine learning algorithms in digital twins:
1. Data Integration and Preprocessing
The first step in creating a digital twin involves integrating and preprocessing data from various sources. Machine learning algorithms are used to clean, normalize, and transform raw data into a format suitable for analysis. This process ensures that the data is accurate, consistent, and ready for use in the digital twin model. For example, in a manufacturing setting, data from sensors, production logs, and maintenance records are integrated and preprocessed to create a comprehensive digital twin of the production line.
2. Predictive Modeling
One of the primary applications of machine learning algorithms in digital twins is predictive modeling. By analyzing historical data and identifying patterns, machine learning models can predict future outcomes and behaviors of the physical system. This capability is invaluable for proactive maintenance, demand forecasting, and optimizing operational efficiency. For instance, in the energy sector, digital twins of power plants use machine learning algorithms to predict equipment failures and optimize maintenance schedules, reducing downtime and operational costs.
3. Anomaly Detection
Machine learning algorithms are also used for anomaly detection in digital twins. By continuously monitoring real-time data, these algorithms can identify deviations from normal behavior that may indicate potential issues or failures. Early detection of anomalies allows for timely intervention and prevents costly disruptions. For example, in the aerospace industry, digital twins of aircraft engines use machine learning algorithms to detect anomalies in engine performance, enabling predictive maintenance and ensuring flight safety.
4. Optimization and Control
Digital twins leverage machine learning algorithms to optimize and control physical systems. By simulating different scenarios and evaluating their outcomes, these algorithms can identify the most efficient and effective strategies for achieving desired objectives. This capability is particularly useful in complex systems where multiple variables and constraints need to be considered. For instance, in smart cities, digital twins of traffic systems use machine learning algorithms to optimize traffic flow, reduce congestion, and improve overall transportation efficiency.
5. Real-Time Decision Making
Machine learning algorithms enable digital twins to make real-time decisions based on current data and conditions. By continuously learning from new data, these algorithms can adapt to changing circumstances and provide actionable insights. This real-time decision-making capability is crucial for dynamic environments where timely responses are essential. For example, in autonomous vehicles, digital twins use machine learning algorithms to process sensor data, predict potential hazards, and make real-time driving decisions to ensure safety and efficiency.
6. Personalization and Customization
In consumer-facing applications, machine learning algorithms in digital twins enable personalization and customization of products and services. By analyzing user data and preferences, these algorithms can tailor experiences to individual needs and preferences. This approach enhances user satisfaction and engagement. For instance, in the retail industry, digital twins of customers use machine learning algorithms to recommend personalized products and offers based on their browsing and purchase history.
7. Simulation and Scenario Analysis
Machine learning algorithms enhance the simulation and scenario analysis capabilities of digital twins. By creating virtual models of physical systems, these algorithms can simulate different scenarios and evaluate their potential impacts. This capability is valuable for risk assessment, strategic planning, and decision support. For example, in the healthcare sector, digital twins of patients use machine learning algorithms to simulate the effects of different treatment options, helping doctors make informed decisions about patient care.
In conclusion, machine learning algorithms are integral to the functionality and effectiveness of digital twins. They enable data integration, predictive modeling, anomaly detection, optimization, real-time decision-making, personalization, and simulation. By leveraging these capabilities, digital twins can provide valuable insights, improve operational efficiency, and enhance decision-making across various industries. As technology continues to advance, the integration of machine learning algorithms in digital twins will become increasingly sophisticated, unlocking new possibilities and transforming the way we interact with and manage physical systems.
9.2. IoT and Sensor Integration
The integration of the Internet of Things (IoT) and sensors into various systems has revolutionized the way we collect, analyze, and utilize data. IoT refers to the network of physical objects embedded with sensors, software, and other technologies to connect and exchange data with other devices and systems over the internet. This interconnected web of devices allows for real-time data collection and analysis, leading to more informed decision-making and enhanced operational efficiency.
One of the primary benefits of IoT and sensor integration is the ability to monitor and control environments remotely. For instance, in smart homes, IoT devices such as thermostats, security cameras, and lighting systems can be controlled via smartphones or other internet-enabled devices. This not only provides convenience but also enhances security and energy efficiency. Similarly, in industrial settings, IoT sensors can monitor machinery and equipment, providing real-time data on performance and potential issues. This enables predictive maintenance, reducing downtime and extending the lifespan of equipment.
In healthcare, IoT and sensor integration have led to significant advancements in patient care and monitoring. Wearable devices such as fitness trackers and smartwatches can monitor vital signs, physical activity, and sleep patterns, providing valuable data to both users and healthcare providers. This continuous monitoring can lead to early detection of health issues and more personalized treatment plans. Additionally, IoT-enabled medical devices can transmit data directly to healthcare providers, allowing for remote monitoring and reducing the need for frequent in-person visits.
Agriculture has also benefited from IoT and sensor integration. Smart farming techniques utilize sensors to monitor soil moisture, temperature, and nutrient levels, enabling farmers to optimize irrigation and fertilization. This not only improves crop yields but also conserves resources and reduces environmental impact. Livestock monitoring systems can track the health and location of animals, ensuring timely intervention in case of illness or other issues.
The integration of IoT and sensors is not without challenges. Security and privacy concerns are paramount, as the vast amount of data collected by these devices can be vulnerable to cyberattacks. Ensuring the interoperability of different devices and systems is also a significant challenge, as is managing the sheer volume of data generated. However, advancements in technology and the development of standardized protocols are helping to address these issues.
In conclusion, the integration of IoT and sensors has transformed various industries by enabling real-time data collection and analysis, leading to improved efficiency, enhanced decision-making, and better outcomes. As technology continues to evolve, the potential applications and benefits of IoT and sensor integration are likely to expand even further. For more insights on the future of IoT, check out 22 Tech Trends Shaping 2024.
10. Comparisons & Contrasts
Comparisons and contrasts are essential tools for understanding the similarities and differences between two or more subjects. This analytical approach helps to highlight the unique features, advantages, and disadvantages of each subject, providing a clearer understanding of their relative merits and drawbacks.
In the context of technology, comparisons and contrasts can be particularly useful for evaluating different systems, methodologies, or products. For instance, comparing traditional simulations with AI-driven digital twins can reveal the strengths and weaknesses of each approach, helping stakeholders make informed decisions about which technology to adopt.
Comparisons and contrasts can also be applied to different industries or sectors. For example, comparing the use of IoT and sensor integration in healthcare versus agriculture can highlight how these technologies are adapted to meet the specific needs and challenges of each field. This can provide valuable insights into best practices and potential areas for improvement.
Another important application of comparisons and contrasts is in the evaluation of different business models or strategies. By comparing the approaches of successful companies, organizations can identify key factors that contribute to success and apply these lessons to their own operations. Similarly, contrasting different marketing strategies can help businesses understand which methods are most effective for reaching their target audience.
In education, comparisons and contrasts can be used to evaluate different teaching methods or curricula. By comparing the outcomes of students taught using different approaches, educators can identify the most effective methods for promoting learning and development. This can lead to the adoption of best practices and the improvement of educational outcomes.
Overall, comparisons and contrasts are valuable tools for analyzing and understanding the relative strengths and weaknesses of different subjects. By highlighting the unique features and advantages of each, this approach can provide valuable insights and inform decision-making in a wide range of contexts.
10.1. Traditional Simulations vs. AI-Driven Digital Twins
Traditional simulations and AI-driven digital twins are two distinct approaches to modeling and analyzing systems, each with its own set of advantages and limitations. Understanding the differences between these two methodologies can help organizations choose the most appropriate tool for their specific needs.
Traditional simulations involve creating a mathematical or computational model of a system to study its behavior under various conditions. These models are typically based on a set of predefined rules and equations that describe the relationships between different components of the system. Traditional simulations have been widely used in fields such as engineering, physics, and economics to predict the behavior of complex systems and test different scenarios.
One of the main advantages of traditional simulations is their ability to provide detailed insights into the behavior of a system based on well-established principles and theories. These models can be highly accurate and reliable, especially when the underlying assumptions and parameters are well understood. Traditional simulations are also relatively straightforward to implement and can be run on standard computational hardware.
However, traditional simulations have some limitations. They often require significant expertise to develop and validate, and the accuracy of the results depends heavily on the quality of the input data and assumptions. Additionally, traditional simulations can be computationally intensive, especially for large and complex systems, which can limit their scalability and real-time applicability.
AI-driven digital twins, on the other hand, represent a more advanced and dynamic approach to modeling systems. A digital twin is a virtual replica of a physical system that is continuously updated with real-time data from sensors and other sources. AI algorithms are used to analyze this data and make predictions about the system's behavior, enabling more accurate and timely decision-making.
One of the key advantages of AI-driven digital twins is their ability to provide real-time insights and adapt to changing conditions. By continuously updating the model with real-world data, digital twins can offer a more accurate and up-to-date representation of the system. This makes them particularly useful for applications such as predictive maintenance, where timely and accurate information is critical.
AI-driven digital twins also benefit from the ability to learn and improve over time. Machine learning algorithms can identify patterns and relationships in the data that may not be apparent in traditional simulations, leading to more accurate predictions and better decision-making. Additionally, digital twins can be integrated with other advanced technologies, such as IoT and cloud computing, to enhance their capabilities and scalability.
However, AI-driven digital twins also have some challenges. Developing and maintaining a digital twin requires significant investment in technology and expertise, and the accuracy of the model depends on the quality and availability of real-time data. Ensuring the security and privacy of this data is also a critical concern, as is managing the complexity of integrating multiple data sources and systems.
In conclusion, traditional simulations and AI-driven digital twins each have their own strengths and limitations. Traditional simulations offer detailed and reliable insights based on well-established principles, but may be limited by their computational intensity and reliance on predefined assumptions. AI-driven digital twins provide real-time, adaptive insights and can learn and improve over time, but require significant investment and data management. By understanding these differences, organizations can choose the most appropriate approach for their specific needs and applications. For more on the intersection of AI and simulations, explore Generative AI & Industrial Simulations: Innovate Fast.
10.2. Different Types of Digital Twins
Digital twins are virtual replicas of physical entities, systems, or processes that are used to simulate, predict, and optimize performance in real-time. They are increasingly being adopted across various industries due to their ability to provide valuable insights and improve decision-making. There are several types of digital twins, each serving different purposes and offering unique benefits.
The first type is the Product Digital Twin. This type of digital twin is used to create a virtual model of a physical product. It allows manufacturers to simulate and analyze the product's performance under different conditions, identify potential issues, and make necessary adjustments before the product is actually built. This can significantly reduce the time and cost associated with product development and testing. For example, in the automotive industry, a product digital twin can be used to simulate the performance of a new car model, allowing engineers to optimize its design and improve its safety and efficiency.
The second type is the Process Digital Twin. This type of digital twin is used to model and simulate a specific process, such as a manufacturing process or a supply chain. It allows organizations to analyze the process in real-time, identify bottlenecks, and make data-driven decisions to improve efficiency and reduce costs. For instance, in the manufacturing industry, a process digital twin can be used to simulate the production line, allowing managers to optimize the workflow and reduce downtime.
The third type is the System Digital Twin. This type of digital twin is used to create a virtual model of a complex system, such as a power plant or a smart city. It allows organizations to monitor and analyze the system's performance in real-time, predict potential issues, and make proactive decisions to ensure optimal performance. For example, in the energy sector, a system digital twin can be used to monitor the performance of a power plant, allowing operators to optimize energy production and reduce emissions.
The fourth type is the Human Digital Twin. This type of digital twin is used to create a virtual model of a human being, including their physical and physiological characteristics. It allows healthcare providers to simulate and analyze the impact of different treatments on a patient's health, enabling personalized medicine and improving patient outcomes. For instance, in the healthcare industry, a human digital twin can be used to simulate the impact of a new drug on a patient's body, allowing doctors to tailor the treatment to the patient's specific needs.
In conclusion, digital twins are powerful tools that can be used to simulate, predict, and optimize the performance of physical entities, systems, or processes. By leveraging different types of digital twins, organizations can gain valuable insights, improve decision-making, and drive innovation across various industries.
11. Why Choose Rapid Innovation for Implementation and Development?
In today's fast-paced and highly competitive business environment, organizations need to continuously innovate and adapt to stay ahead of the curve. Rapid innovation, which involves quickly developing and implementing new ideas, products, or processes, is essential for organizations to remain competitive and meet the ever-changing demands of the market. There are several reasons why organizations should choose rapid innovation for implementation and development.
Firstly, rapid innovation allows organizations to quickly respond to market changes and customer needs. In a dynamic market, customer preferences and demands can change rapidly, and organizations need to be able to adapt quickly to stay relevant. By adopting rapid innovation, organizations can quickly develop and launch new products or services that meet the evolving needs of their customers, ensuring they remain competitive and maintain customer loyalty.
Secondly, rapid innovation can help organizations reduce time-to-market. In many industries, being the first to market with a new product or service can provide a significant competitive advantage. By leveraging rapid innovation, organizations can streamline their development processes, reduce the time it takes to bring new products or services to market, and gain a competitive edge over their rivals.
Thirdly, rapid innovation can drive cost savings and efficiency. Traditional development processes can be time-consuming and costly, often involving multiple iterations and extensive testing. Rapid innovation, on the other hand, focuses on quickly developing and testing new ideas, allowing organizations to identify and address potential issues early in the development process. This can help reduce development costs, minimize waste, and improve overall efficiency.
Moreover, rapid innovation fosters a culture of continuous improvement and experimentation. By encouraging employees to think creatively and experiment with new ideas, organizations can drive innovation and stay ahead of the competition. This culture of continuous improvement can also lead to increased employee engagement and satisfaction, as employees feel empowered to contribute to the organization's success.
Finally, rapid innovation can help organizations mitigate risks. In a rapidly changing business environment, organizations face various risks, including technological disruptions, changing regulations, and economic uncertainties. By adopting rapid innovation, organizations can quickly adapt to these changes, identify potential risks early, and develop strategies to mitigate them. This proactive approach can help organizations navigate uncertainties and ensure long-term success.
In conclusion, rapid innovation is essential for organizations to remain competitive, reduce time-to-market, drive cost savings and efficiency, foster a culture of continuous improvement, and mitigate risks. By choosing rapid innovation for implementation and development, organizations can stay ahead of the curve and achieve sustainable growth in today's dynamic business environment.
11.1. Expertise in AI and Blockchain
Artificial Intelligence (AI) and Blockchain are two of the most transformative technologies of the 21st century, and organizations that possess expertise in these areas are well-positioned to drive innovation and achieve a competitive advantage. Expertise in AI and Blockchain can provide organizations with the tools and capabilities needed to develop cutting-edge solutions, improve operational efficiency, and create new business opportunities.
AI, which involves the development of algorithms and systems that can perform tasks that typically require human intelligence, such as learning, reasoning, and decision-making, has the potential to revolutionize various industries. Organizations with expertise in AI can leverage this technology to automate routine tasks, analyze large volumes of data, and make data-driven decisions. For example, in the healthcare industry, AI can be used to analyze medical images, predict patient outcomes, and personalize treatment plans, improving patient care and outcomes. In the financial sector, AI can be used to detect fraudulent transactions, optimize investment strategies, and provide personalized financial advice to customers.
Blockchain, on the other hand, is a decentralized and distributed ledger technology that enables secure and transparent transactions. Organizations with expertise in Blockchain can leverage this technology to enhance security, improve transparency, and streamline processes. For instance, in the supply chain industry, Blockchain can be used to track the movement of goods, verify the authenticity of products, and ensure compliance with regulations. In the financial sector, Blockchain can be used to facilitate secure and transparent transactions, reduce the risk of fraud, and improve the efficiency of cross-border payments.
Moreover, the combination of AI and Blockchain can create powerful synergies and unlock new possibilities. For example, AI can be used to analyze data stored on a Blockchain, providing valuable insights and enabling more informed decision-making. Conversely, Blockchain can enhance the security and transparency of AI systems, ensuring the integrity and trustworthiness of the data used for AI training and decision-making.
Organizations with expertise in AI and Blockchain can also drive innovation by developing new business models and creating new revenue streams. For example, AI-powered platforms can be used to offer personalized products and services, while Blockchain-based solutions can enable new forms of digital transactions and collaborations. Additionally, organizations can leverage their expertise in these technologies to develop and offer innovative solutions to their customers, creating a competitive advantage and driving business growth.
In conclusion, expertise in AI and Blockchain is essential for organizations to drive innovation, improve operational efficiency, and create new business opportunities. By leveraging these transformative technologies, organizations can develop cutting-edge solutions, enhance security and transparency, and achieve a competitive advantage in today's rapidly evolving business landscape. For more insights on how AI and Blockchain are transforming industries, you can explore Rapid Innovation: AI & Blockchain Transforming Industries.
11.2. Customized Solutions
In today's fast-paced and highly competitive business environment, the one-size-fits-all approach is no longer viable. Customized solutions have become a cornerstone for businesses aiming to meet specific needs and achieve unique goals. These tailored solutions are designed to address the particular challenges and opportunities that a business faces, ensuring that the strategies and tools employed are perfectly aligned with the company's objectives.
Customized solutions begin with a thorough understanding of the client's business model, market environment, and specific requirements. This involves a detailed analysis of the company's strengths, weaknesses, opportunities, and threats (SWOT analysis). By gaining a deep insight into the client's operations and market position, service providers can develop bespoke strategies that are more effective than generic solutions.
One of the primary benefits of customized solutions is their ability to provide a competitive edge. When solutions are tailored to the unique needs of a business, they can address specific pain points more effectively, leading to improved efficiency, productivity, and profitability. For instance, a customized customer relationship management (CRM) system can be designed to cater to the unique sales processes and customer interactions of a business, resulting in better customer service and higher sales conversion rates.
Moreover, customized solutions are scalable and flexible, allowing businesses to adapt to changing market conditions and evolving business needs. This adaptability is crucial in today's dynamic business landscape, where companies must be agile and responsive to stay ahead of the competition. Customized solutions can be easily modified and upgraded to accommodate new requirements, ensuring that businesses remain competitive and relevant.
Another significant advantage of customized solutions is the enhanced user experience they offer. When solutions are designed with the end-user in mind, they are more intuitive and user-friendly, leading to higher adoption rates and better overall performance. For example, a customized software application can be developed with a user interface that aligns with the specific workflows and preferences of the users, resulting in a more efficient and satisfying user experience.
Furthermore, customized solutions often lead to better data management and decision-making. By integrating various data sources and providing tailored analytics and reporting tools, customized solutions enable businesses to gain valuable insights and make informed decisions. This data-driven approach can lead to more effective strategies and better business outcomes.
In conclusion, customized solutions are essential for businesses looking to achieve their unique goals and stay competitive in today's market. By providing tailored strategies and tools that address specific needs, customized solutions offer numerous benefits, including improved efficiency, scalability, user experience, and data management. As businesses continue to face new challenges and opportunities, the demand for customized solutions will only grow, making them a critical component of modern business strategy.
For more information on customized AI solutions, you can explore AI & Blockchain Solutions for Fintech & Banking Industry and Effective Strategies for Evaluating and Optimizing Enterprise AI Solutions.
11.3. Proven Methodologies
Proven methodologies are systematic, repeatable processes that have been tested and validated over time to deliver consistent and reliable results. These methodologies are essential for businesses and organizations seeking to achieve their goals efficiently and effectively. By leveraging established best practices and frameworks, companies can minimize risks, optimize resources, and ensure successful project outcomes.
One of the most widely recognized proven methodologies is the Project Management Institute's (PMI) Project Management Body of Knowledge (PMBOK). The PMBOK provides a comprehensive framework for managing projects, including processes, tools, and techniques that have been proven to work across various industries and project types. By following the PMBOK guidelines, project managers can ensure that their projects are completed on time, within budget, and to the desired quality standards.
Another well-known methodology is Agile, which is particularly popular in software development and other fast-paced industries. Agile emphasizes iterative development, collaboration, and flexibility, allowing teams to respond quickly to changing requirements and deliver value to customers more rapidly. The Agile methodology has been proven to improve project outcomes by fostering better communication, increasing transparency, and promoting continuous improvement.
Lean Six Sigma is another proven methodology that combines the principles of Lean manufacturing and Six Sigma to improve process efficiency and quality. Lean focuses on eliminating waste and optimizing workflows, while Six Sigma aims to reduce variability and defects. By integrating these two approaches, Lean Six Sigma provides a powerful framework for achieving operational excellence and driving continuous improvement.
In addition to these well-established methodologies, there are numerous industry-specific frameworks and best practices that have been proven to deliver results. For example, the Information Technology Infrastructure Library (ITIL) is a widely adopted framework for IT service management that provides best practices for delivering high-quality IT services. Similarly, Capability Maturity Model Integration (CMMI) is a process improvement framework that helps organizations improve their software development and other business processes.
The use of proven methodologies offers several key benefits. First, they provide a structured approach to problem-solving and decision-making, ensuring that all aspects of a project or process are considered and addressed. This structured approach helps to minimize risks and avoid common pitfalls, leading to more successful outcomes.
Second, proven methodologies promote consistency and standardization, which are essential for achieving repeatable and reliable results. By following established best practices, organizations can ensure that their processes are efficient, effective, and aligned with industry standards.
Third, proven methodologies facilitate continuous improvement by providing a framework for measuring performance, identifying areas for improvement, and implementing changes. This focus on continuous improvement helps organizations to stay competitive and adapt to changing market conditions.
In conclusion, proven methodologies are invaluable tools for businesses and organizations seeking to achieve their goals efficiently and effectively. By leveraging established best practices and frameworks, companies can minimize risks, optimize resources, and ensure successful project outcomes. As the business landscape continues to evolve, the importance of proven methodologies will only grow, making them a critical component of modern business strategy.
12. Conclusion
In conclusion, the modern business landscape is characterized by rapid change, intense competition, and increasing complexity. To navigate these challenges and achieve their goals, businesses must adopt strategies and solutions that are both effective and adaptable. Customized solutions and proven methodologies are two critical components of a successful business strategy, offering numerous benefits that can help organizations stay competitive and achieve long-term success.
Customized solutions provide tailored strategies and tools that address the unique needs and challenges of a business. By gaining a deep understanding of the client's operations and market environment, service providers can develop bespoke solutions that are more effective than generic approaches. These tailored solutions offer several advantages, including improved efficiency, scalability, user experience, and data management. As businesses continue to face new challenges and opportunities, the demand for customized solutions will only grow, making them an essential component of modern business strategy.
Proven methodologies, on the other hand, offer a structured and systematic approach to problem-solving and decision-making. By leveraging established best practices and frameworks, organizations can minimize risks, optimize resources, and ensure successful project outcomes. Proven methodologies promote consistency, standardization, and continuous improvement, helping businesses to stay competitive and adapt to changing market conditions. As the business landscape continues to evolve, the importance of proven methodologies will only increase, making them a critical component of modern business strategy.
In summary, the combination of customized solutions and proven methodologies provides a powerful framework for achieving business success. By addressing the unique needs of a business and leveraging established best practices, organizations can navigate the complexities of the modern business environment and achieve their goals efficiently and effectively. As businesses continue to face new challenges and opportunities, the adoption of customized solutions and proven methodologies will be essential for staying competitive and achieving long-term success.