Table Of Contents
Category
Artificial Intelligence
AIML
IoT
Blockchain
1. Introduction to AI Content Creation
AI content creation refers to the use of artificial intelligence technologies to generate written, visual, or audio content. This innovative approach leverages machine learning algorithms, natural language processing (NLP), and deep learning to produce high-quality content efficiently.
- AI tools for content creation can analyze vast amounts of data to understand context, tone, and style, allowing them to create content that resonates with target audiences.
- The technology can generate various types of content, including articles, blog posts, social media updates, marketing copy, and even video scripts.
- AI content creation is becoming increasingly popular due to its ability to save time, reduce costs, and enhance creativity.
The rise of AI content creation is transforming the way businesses and individuals approach content marketing and communication. By automating the content generation process, organizations can focus on strategy and engagement while ensuring a steady flow of relevant content.
At Rapid Innovation, we harness the power of AI content creators to help our clients achieve their business goals efficiently and effectively. By implementing tailored AI solutions, we enable organizations to maximize their return on investment (ROI) through enhanced content strategies that drive engagement and conversion.
AI content creation tools are designed to assist writers, marketers, and content creators in various ways, from brainstorming ideas to drafting complete articles. As the technology continues to evolve, it is essential to understand its capabilities, limitations, and potential applications across different industries. Rapid Innovation is committed to guiding clients through this landscape, ensuring they leverage automated content creation to its fullest potential for sustainable growth and success. For more information on how to get started, check out AI for Content Creation: How to Get Started.
1.1. Defining AI Content Creation
AI content creation refers to the use of artificial intelligence technologies to generate written, visual, or audio content. This process leverages algorithms and machine learning models to produce material that can mimic human creativity and style. AI content creation tools can generate articles, blogs, social media posts, and even scripts for videos. These tools analyze vast amounts of data to understand language patterns, tone, and context, allowing them to create coherent and relevant content. The primary goal is to enhance productivity, reduce costs, and maintain quality in content production.
AI content creation is increasingly popular among businesses and marketers, as it allows for:
- Rapid content generation: By automating the content creation process, businesses can produce large volumes of material in a fraction of the time it would take human writers, leading to faster project completion and quicker time-to-market.
- Personalization at scale: AI tools can analyze customer data to create tailored content that resonates with specific audience segments, enhancing engagement and conversion rates.
- Consistency in messaging: AI ensures that the tone and style of content remain uniform across various platforms, reinforcing brand identity and trust.
1.2. Historical Evolution of Content Generation Technologies
The evolution of content generation technologies has been a gradual process, marked by significant milestones that have shaped how content is created and consumed.
- Early Automation: The journey began with simple automation tools in the 1950s and 1960s, which could generate basic text based on predefined templates.
- Natural Language Processing (NLP): In the 1980s, advancements in NLP allowed computers to understand and generate human language more effectively. This laid the groundwork for more sophisticated content generation.
- Machine Learning: The 2000s saw the rise of machine learning, enabling systems to learn from data and improve their content generation capabilities over time.
- Deep Learning: The introduction of deep learning in the 2010s revolutionized AI content creation. Models like GPT-3 can generate human-like text, making it possible to create high-quality content with minimal human intervention.
The historical evolution has led to:
- Increased efficiency in content production: Businesses can now produce content faster and at a lower cost, leading to a higher return on investment (ROI).
- Enhanced creativity through AI-generated ideas: AI can suggest innovative concepts and topics, helping businesses stay ahead of trends and engage their audiences effectively.
- Greater accessibility to content creation tools for individuals and businesses: With the democratization of AI tools for content creation, even small businesses can leverage advanced content generation technologies to compete in the market.
1.3. Fundamental Technological Foundations
The technological foundations of AI content creation are built on several key components that enable machines to generate content effectively.
- Natural Language Processing (NLP): NLP is crucial for understanding and generating human language. It involves parsing text, understanding context, and generating coherent sentences.
- Machine Learning Algorithms: These algorithms allow AI systems to learn from data, improving their ability to generate relevant and engaging content over time.
- Deep Learning Models: Advanced models, such as neural networks, are used to process large datasets and generate high-quality content. These models can capture complex patterns in language and style.
- Data Analytics: Analyzing user data helps AI systems tailor content to specific audiences, enhancing engagement and relevance.
The combination of these technologies results in:
- Improved accuracy in content generation: AI can produce content that closely aligns with user expectations and preferences, leading to higher satisfaction rates.
- Enhanced ability to mimic human writing styles: This allows businesses to maintain a consistent voice across all content, which is essential for brand recognition.
- Greater adaptability to different content formats and platforms: AI can generate content suitable for various channels, ensuring that businesses can reach their audiences wherever they are.
At Rapid Innovation, we harness these technological foundations to help our clients achieve their business goals efficiently and effectively, ultimately driving greater ROI through innovative AI solutions, including automated content creation and AI-powered content creation tools.
1.4. Paradigm Shift in Content Production
The landscape of content production has undergone a significant transformation in recent years, driven by advancements in technology and changing consumer behaviors. This paradigm shift is characterized by several key trends:
- Increased Accessibility: With the rise of digital platforms, content creation has become more accessible to individuals and small businesses. Tools like Canva and Adobe Spark allow anyone to create professional-quality graphics and videos without extensive training.
- User-Generated Content (UGC): Brands are increasingly leveraging UGC to enhance authenticity and engagement. Consumers trust content created by their peers more than traditional advertising, leading to a shift in how brands approach marketing.
- Real-Time Content Creation: The demand for timely and relevant content has led to a focus on real-time production. Social media platforms like Twitter and Instagram encourage brands to create and share content instantly, responding to current events and trends.
- Data-Driven Strategies: Content production is now heavily influenced by data analytics. Marketers use insights from consumer behavior to tailor content that resonates with their target audience, optimizing engagement and conversion rates.
- Diverse Formats: The shift towards multimedia content, including podcasts, videos, and interactive content, reflects changing consumer preferences. Brands are diversifying their content strategies to include various formats that cater to different audience segments, including the latest video production trends and social media video trends 2023.
- Collaboration and Co-Creation: The rise of collaborative tools has enabled teams to work together seamlessly, regardless of location. This fosters creativity and innovation in content production, allowing for a more dynamic approach.
- Sustainability and Ethical Considerations: As consumers become more conscious of environmental and social issues, brands are shifting towards sustainable content production practices. This includes using eco-friendly materials and promoting social responsibility in their messaging.
2. Technological Foundations
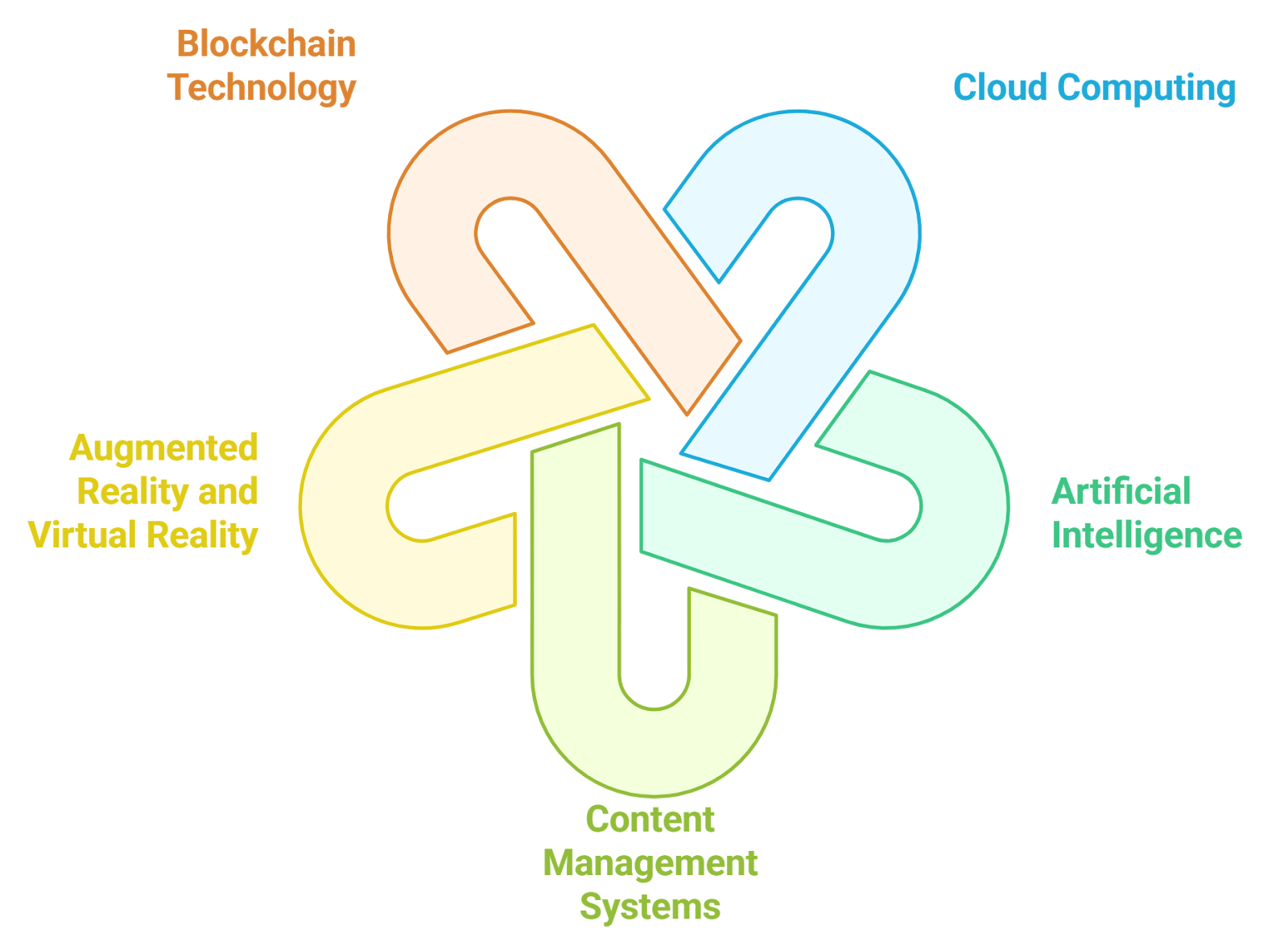
‍
The technological foundations of modern content production are critical to understanding the current landscape. These technologies not only enhance the efficiency of content creation but also improve the quality and relevance of the output. Key components include:
- Cloud Computing: Cloud-based platforms allow for easy storage, sharing, and collaboration on content. This technology enables teams to access files from anywhere, facilitating remote work and real-time updates.
- Artificial Intelligence (AI): AI tools are revolutionizing content production by automating repetitive tasks, such as editing and formatting. AI-driven analytics also provide insights into audience preferences, helping creators tailor their content more effectively.
- Content Management Systems (CMS): Modern CMS platforms streamline the process of content creation, management, and distribution. They offer features like SEO optimization, analytics tracking, and user-friendly interfaces that simplify the workflow.
- Augmented Reality (AR) and Virtual Reality (VR): These immersive technologies are changing how content is consumed. Brands are using AR and VR to create engaging experiences that captivate audiences and enhance storytelling.
- Blockchain Technology: Blockchain is emerging as a solution for content ownership and copyright issues. It provides a transparent and secure way to track content usage and ensure creators are compensated fairly.
2.1. Machine Learning Architectures
Machine learning (ML) architectures are at the forefront of technological advancements in content production. These architectures enable systems to learn from data and improve their performance over time. Key aspects include:
- Natural Language Processing (NLP): NLP allows machines to understand and generate human language. This technology is used in chatbots, content generation, and sentiment analysis, enhancing user interaction and engagement.
- Recommendation Systems: ML algorithms power recommendation engines that suggest content based on user behavior. Platforms like Netflix and Spotify utilize these systems to personalize user experiences, increasing retention and satisfaction.
- Image and Video Recognition: Machine learning models can analyze visual content, identifying objects, scenes, and even emotions. This capability is essential for content tagging, automated editing, and enhancing user-generated content.
- Predictive Analytics: By analyzing historical data, ML architectures can predict future trends and consumer behavior. This insight helps marketers create proactive content strategies that align with audience interests.
- Automated Content Creation: Some ML models can generate written content, such as news articles or product descriptions, based on data inputs. This automation can save time and resources while maintaining quality.
- A/B Testing and Optimization: Machine learning can optimize content by analyzing the performance of different versions. This allows marketers to refine their strategies based on real-time feedback and improve overall effectiveness.
- Enhanced User Experience: By leveraging ML, brands can create more personalized and engaging experiences for their audiences. This includes tailored content recommendations, dynamic content delivery, and improved customer service through AI-driven chatbots.
At Rapid Innovation, we harness these technological advancements to help our clients achieve their business goals efficiently and effectively. By integrating AI and machine learning into content production processes, we enable businesses to optimize their strategies, enhance user engagement, and ultimately achieve greater ROI. Our expertise in these domains ensures that our clients stay ahead of the curve in an ever-evolving digital landscape.
2.1.1. Neural Networks
Neural networks are a subset of machine learning models inspired by the human brain's structure and function. They consist of interconnected nodes or "neurons" that process data in layers. The primary goal of neural networks is to recognize patterns and make predictions based on input data.
- Structure: Neural networks typically have three types of layers: Â
- Input layer: Receives the initial data.
- Hidden layers: Perform computations and feature extraction.
- Output layer: Produces the final prediction or classification.
- Learning Process: Neural networks learn through a process called backpropagation, where the model adjusts its weights based on the error of its predictions. This iterative process continues until the model achieves an acceptable level of accuracy.
- Applications: Neural networks are widely used in various fields, including: Â
- Image recognition (e.g., convolutional neural networks application, convolutional neural network for image classification, convolutional neural networks image classification)
- Speech recognition
- Financial forecasting
- Medical diagnosis (e.g., neural network imaging, neural network applications)
Neural networks have revolutionized many industries by enabling machines to perform complex tasks that were previously thought to require human intelligence. At Rapid Innovation, we leverage neural networks to help our clients enhance their data-driven decision-making processes, leading to improved operational efficiency and greater ROI. Our work includes neural computing and applications, neural computing & applications, and application for neural network solutions.
2.1.2. Transformer Models
Transformer models are a type of neural network architecture that has gained immense popularity, particularly in the field of natural language processing (NLP). Introduced in the paper "Attention is All You Need" by Vaswani et al. in 2017, transformers have transformed how machines understand and generate human language.
- Key Features: Â
- Attention Mechanism: Transformers utilize self-attention mechanisms that allow the model to weigh the importance of different words in a sentence, regardless of their position. This enables better context understanding.
- Parallelization: Unlike traditional recurrent neural networks (RNNs), transformers can process data in parallel, significantly speeding up training times.
- Components: Â
- Encoder: Processes the input data and generates a representation.
- Decoder: Takes the encoder's output and generates the final output, such as translated text or responses.
- Applications: Transformer models have been instrumental in various NLP tasks, including: Â
- Machine translation
- Text summarization
- Sentiment analysis
- Chatbots and conversational agents
The introduction of transformer models has led to significant advancements in the capabilities of AI systems, making them more efficient and effective in understanding human language. Rapid Innovation employs transformer models to develop sophisticated NLP solutions that enhance customer engagement and streamline communication, ultimately driving higher returns on investment for our clients.
2.1.3. Natural Language Processing (NLP)
Natural Language Processing (NLP) is a field of artificial intelligence that focuses on the interaction between computers and human language. It encompasses a range of techniques and technologies that enable machines to understand, interpret, and generate human language in a meaningful way.
- Core Components: Â
- Tokenization: Breaking down text into smaller units, such as words or phrases, to facilitate analysis.
- Part-of-Speech Tagging: Identifying the grammatical categories of words in a sentence, such as nouns, verbs, and adjectives.
- Named Entity Recognition: Detecting and classifying entities in text, such as names, dates, and locations.
- Techniques: Â
- Machine Learning: Utilizing algorithms to learn from data and improve language understanding.
- Deep Learning: Employing neural networks, particularly transformer models, to enhance NLP capabilities.
- Applications: NLP has a wide range of applications, including: Â
- Virtual assistants (e.g., Siri, Alexa)
- Sentiment analysis for social media monitoring
- Automated customer support through chatbots
- Content recommendation systems (e.g., neural net applications, neural network and application)
NLP continues to evolve, driven by advancements in machine learning and deep learning, making it an essential component of modern AI applications. At Rapid Innovation, we harness the power of NLP to create tailored solutions that not only meet our clients' specific needs but also maximize their operational efficiency and profitability.
2.2. Large Language Models (LLMs)
Large Language Models (LLMs) are a subset of artificial intelligence that focuses on understanding and generating human language. These models are designed to process vast amounts of text data, enabling them to perform a variety of language-related tasks.
- LLMs utilize deep learning techniques, particularly neural networks, to analyze and generate text.
- They are trained on diverse datasets, which can include books, articles, websites, and other written content, allowing them to learn context, grammar, and even nuances of language.
- Popular examples of large language models include OpenAI's GPT-3 and Google's BERT, which have set benchmarks in natural language processing (NLP).
- LLMs can perform tasks such as translation, summarization, question-answering, and even creative writing.
- The scale of these models is significant, with some containing billions of parameters, which are the weights and biases that the model learns during training.
- The ability of LLMs to generate coherent and contextually relevant text has made them valuable in various applications, from llm chatbots to content creation.
At Rapid Innovation, we leverage large language models to help our clients enhance customer engagement through intelligent chatbots and personalized content generation, ultimately driving greater ROI. If you're looking to enhance your projects with cutting-edge technology, consider hiring generative AI engineers to bring your ideas to life. For more information on LLMs, check out this article on large language models.
2.3. Generative AI Technologies
Generative AI technologies refer to systems that can create new content, whether it be text, images, music, or other forms of media. These technologies leverage advanced algorithms to produce outputs that mimic human creativity.
- Generative AI encompasses various techniques, including Generative Adversarial Networks (GANs) and Variational Autoencoders (VAEs).
- GANs consist of two neural networks, a generator and a discriminator, that work together to create realistic outputs. The generator creates new data, while the discriminator evaluates its authenticity.
- Applications of generative AI are vast and include:
- Art and design: AI-generated artwork and graphic design.
- Music: Composition of original music tracks.
- Text: Content generation for articles, blogs, and marketing materials.
- Generative AI can also be used in gaming, where it creates dynamic environments and characters.
- The technology has raised ethical concerns, particularly regarding copyright and the potential for misuse in creating deepfakes or misleading information.
At Rapid Innovation, we harness generative AI to create unique marketing content and innovative designs, enabling our clients to stand out in competitive markets and achieve higher returns on their investments.
2.4. Training Methodologies
Training methodologies for LLMs and generative AI technologies are crucial for their performance and effectiveness. These methodologies determine how models learn from data and improve over time.
- Supervised learning: This involves training models on labeled datasets, where the input data is paired with the correct output. It is commonly used for tasks like classification and regression.
- Unsupervised learning: In this approach, models learn from unlabeled data, identifying patterns and structures without explicit guidance. This is useful for clustering and dimensionality reduction.
- Reinforcement learning: This method involves training models through trial and error, where they receive feedback based on their actions. It is often used in applications like game playing and robotics.
- Transfer learning: This technique allows models to leverage knowledge gained from one task to improve performance on another related task. It is particularly effective in NLP, where pre-trained models can be fine-tuned for specific applications.
- Data augmentation: This involves artificially increasing the size of the training dataset by creating modified versions of existing data. This helps improve model robustness and generalization.
- Continuous learning: This methodology enables models to adapt and learn from new data over time, ensuring they remain relevant and effective in dynamic environments.
By employing these training methodologies, developers can enhance the capabilities of large language models and generative AI technologies, leading to more accurate and versatile applications. At Rapid Innovation, we implement these methodologies to ensure our AI solutions are not only effective but also continuously evolving to meet our clients' needs, thereby maximizing their ROI.
2.4.1. Supervised Learning
Supervised learning is a type of machine learning where the model is trained on a labeled dataset. This means that each training example is paired with an output label, allowing the algorithm to learn the relationship between the input data and the corresponding output.
- Key characteristics: Â
- Requires a labeled dataset for training.
- The model makes predictions based on the learned relationships.
- Commonly used for classification and regression tasks.
- Common algorithms: Â
- Linear Regression
- Logistic Regression
- Decision Trees
- Support Vector Machines (SVM)
- Neural Networks
- Applications: Â
- Email spam detection: Classifying emails as spam or not spam.
- Image recognition: Identifying objects within images.
- Medical diagnosis: Predicting diseases based on patient data.
At Rapid Innovation, we leverage supervised learning to help our clients enhance their decision-making processes and operational efficiencies. For instance, by implementing predictive models for medical diagnosis, healthcare providers can improve patient outcomes while reducing costs. Supervised learning is widely used due to its effectiveness in various applications, including machine learning applications in business intelligence and machine learning for drug discovery. According to a report by McKinsey, companies that leverage machine learning can increase their profitability by 5-10%.
2.4.2. Unsupervised Learning
Unsupervised learning is a type of machine learning where the model is trained on data without labeled responses. The goal is to identify patterns, groupings, or structures within the data.
- Key characteristics: Â
- Does not require labeled data.
- Focuses on discovering hidden patterns or intrinsic structures.
- Often used for clustering and association tasks.
- Common algorithms: Â
- K-Means Clustering
- Hierarchical Clustering
- Principal Component Analysis (PCA)
- t-Distributed Stochastic Neighbor Embedding (t-SNE)
- Applications: Â
- Customer segmentation: Grouping customers based on purchasing behavior.
- Anomaly detection: Identifying unusual patterns in data, such as fraud detection.
- Market basket analysis: Discovering associations between products purchased together.
At Rapid Innovation, we utilize unsupervised learning techniques to help businesses uncover valuable insights from their data. For example, customer segmentation can lead to more targeted marketing strategies, ultimately driving higher conversion rates. Unsupervised learning is particularly useful in exploratory data analysis, including applications of machine learning in signal processing. According to a study by Gartner, 70% of organizations are investing in AI and machine learning technologies, with unsupervised learning being a key area of focus.
2.4.3. Reinforcement Learning
Reinforcement learning (RL) is a type of machine learning where an agent learns to make decisions by taking actions in an environment to maximize cumulative rewards. The agent receives feedback in the form of rewards or penalties based on its actions, allowing it to learn optimal strategies over time.
- Key characteristics: Â
- Involves an agent, environment, actions, and rewards.
- The learning process is trial-and-error based.
- Focuses on long-term reward maximization.
- Common algorithms: Â
- Q-Learning
- Deep Q-Networks (DQN)
- Proximal Policy Optimization (PPO)
- Actor-Critic methods
- Applications: Â
- Game playing: Training agents to play games like chess or Go.
- Robotics: Teaching robots to navigate and perform tasks in real-world environments.
- Autonomous vehicles: Enabling self-driving cars to make decisions based on their surroundings.
At Rapid Innovation, we are at the forefront of applying reinforcement learning to complex decision-making scenarios, such as optimizing logistics and supply chain management. Reinforcement learning has gained significant attention due to its success in complex tasks, including deep learning applications in computer vision. A report by OpenAI highlights that RL has been instrumental in achieving breakthroughs in various fields, including gaming and robotics. By integrating these advanced techniques, we help our clients achieve greater ROI and operational excellence, particularly in machine learning for embedded systems and mobile applications.
3. How AI Content Creation Works
AI content creation involves a series of sophisticated processes that enable machines to generate human-like text. This technology leverages vast amounts of data and advanced algorithms to produce content that can be informative, engaging, and relevant. Understanding how AI content creation works can help businesses and individuals harness its potential effectively.
3.1. Data Collection and Preprocessing
Data collection is the foundational step in AI content creation. It involves gathering a diverse range of text data from various sources to train AI models. The quality and quantity of this data significantly influence the performance of the AI.
- Sources of Data: Â
- Websites, blogs, and articles
- Social media platforms
- Books and academic papers
- User-generated content
- Preprocessing Steps: Â
- Cleaning: Removing irrelevant information, such as HTML tags, special characters, and duplicates.
- Tokenization: Breaking down text into smaller units, such as words or phrases, to facilitate analysis.
- Normalization: Converting text to a standard format, including lowercasing and stemming, to ensure consistency.
- Annotation: Labeling data for supervised learning, which helps the model understand context and meaning.
The preprocessing stage is crucial as it ensures that the data fed into the AI model is clean and structured. This step enhances the model's ability to learn patterns and generate coherent content, ultimately leading to more effective and efficient content generation for businesses, including automated content creation and ai tools for content creation.
3.2. Model Training Processes
Once the data is collected and preprocessed, the next step is model training. This process involves using machine learning algorithms to teach the AI how to generate text based on the input data.
- Types of Models: Â
- Natural Language Processing (NLP) Models: These models focus on understanding and generating human language. Examples include GPT (Generative Pre-trained Transformer) and BERT (Bidirectional Encoder Representations from Transformers).
- Deep Learning Models: These models utilize neural networks to learn complex patterns in data. They are particularly effective for tasks like language translation and sentiment analysis.
- Training Techniques: Â
- Supervised Learning: The model is trained on labeled data, where the correct output is provided. This helps the model learn the relationship between input and output.
- Unsupervised Learning: The model learns from unlabeled data, identifying patterns and structures without explicit guidance.
- Reinforcement Learning: The model learns through trial and error, receiving feedback based on its performance. This technique is often used in conversational AI.
- Evaluation and Fine-tuning: Â
- After training, the model is evaluated using metrics such as accuracy, precision, and recall. Fine-tuning may be necessary to improve performance, which involves adjusting parameters and retraining the model on specific datasets.
The model training process is iterative, requiring multiple cycles of training and evaluation to achieve optimal results. The end goal is to create an AI that can generate high-quality content that meets user needs and expectations. By leveraging these advanced processes, including ai content creation tools and ai content creator tools, Rapid Innovation empowers clients to enhance their content strategies, ultimately driving greater ROI through improved engagement and efficiency. Additionally, our expertise in data annotation services allows us to tailor solutions that meet specific business needs.
3.3. Prompt Engineering

‍
Prompt engineering is a crucial aspect of working with AI language models. It involves crafting specific inputs or prompts that guide the model to produce desired outputs. Effective prompt engineering can significantly enhance the quality and relevance of the generated content, especially in areas like ai content generation and ai content creation.
- Understanding the model's capabilities is essential. Different models have varying strengths and weaknesses, so knowing what your model can do will help in formulating effective prompts, particularly when using ai tools for content creation.
- Clarity is key. A well-defined prompt reduces ambiguity and helps the model understand the context better. For example, instead of asking, "Tell me about dogs," a more specific prompt like, "What are the top five dog breeds for families?" yields better results, similar to how one might create content with ai.
- Experimentation is vital. Testing multiple prompts can reveal which formulations produce the best responses. Iterative testing allows users to refine their prompts based on the outputs received, which is particularly useful in automated content creation.
- Contextual cues can enhance responses. Including relevant background information or specific instructions in the prompt can lead to more accurate and contextually appropriate outputs, especially when utilizing ai content generation tools.
- Length matters. Short prompts may lead to vague responses, while overly long prompts can confuse the model. Finding the right balance is essential for effective communication, particularly in the context of ai content creators.
3.4. Content Generation Mechanisms
Content generation mechanisms refer to the various methods and techniques used by AI models to create text. Understanding these mechanisms can help users leverage AI more effectively for their content needs, including ai for content creation.
- Natural Language Processing (NLP) is at the core of content generation. NLP techniques enable models to understand and generate human-like text by analyzing language patterns and structures, which is fundamental in creating content with ai.
- Machine Learning (ML) algorithms play a significant role. These algorithms learn from vast datasets, allowing models to generate content that mimics human writing styles and tones, similar to what one might expect from ai powered content creation.
- Transformer architecture is a key innovation in AI. This architecture allows models to process and generate text more efficiently by focusing on the relationships between words in a sentence, enhancing the capabilities of ai content creator tools.
- Pre-training and fine-tuning are essential steps. Models are initially pre-trained on large datasets and then fine-tuned on specific tasks or domains to improve their performance in generating relevant content, such as automated content generation.
- User input can guide content generation. By providing specific keywords or themes, users can influence the direction and focus of the generated text, making it more aligned with their needs, particularly in the realm of creating content with ai.
3.5. Quality Control and Refinement
Quality control and refinement are critical in ensuring that the generated content meets the desired standards. Implementing effective quality control measures can enhance the reliability and accuracy of AI-generated text, especially when using tools like a free ai writing app.
- Review processes are essential. Regularly reviewing generated content helps identify errors, inconsistencies, or areas for improvement. This can involve human oversight or automated checks, ensuring that the output from ai content generation tools is reliable.
- Feedback loops can enhance quality. Incorporating user feedback into the content generation process allows for continuous improvement. Users can provide insights on what works and what doesn’t, guiding future iterations, particularly in the context of ai in content creation.
- Plagiarism checks are important. Ensuring that the generated content is original and not copied from existing sources is crucial for maintaining credibility and avoiding legal issues, especially when using automated content creation.
- Consistency in tone and style is vital. Establishing guidelines for tone, style, and formatting helps maintain a cohesive voice across all generated content, which is essential for ai generated facebook post.
- Iterative refinement can lead to better outcomes. Continuously tweaking prompts and adjusting parameters based on previous outputs can significantly improve the quality of the generated text over time, particularly when utilizing best free ai writer tools.
At Rapid Innovation, we leverage these principles of prompt engineering, content generation mechanisms, and quality control to help our clients achieve their business goals efficiently and effectively. By utilizing our expertise in AI, we ensure that the content generated aligns with your strategic objectives, ultimately leading to greater ROI.
3.6. Ethical Considerations in Generation
Ethical considerations in AI content generation are crucial as they shape the way technology interacts with society. As AI systems become more sophisticated, the implications of their use in content creation raise several ethical questions.
- Bias and Fairness: AI models can inadvertently perpetuate biases present in their training data, leading to the generation of content that is discriminatory or offensive. Ensuring fairness in AI-generated content is essential to avoid reinforcing stereotypes, especially in applications like ai content generation and ai content creation.
- Intellectual Property: The ownership of AI-generated content is a complex issue. Questions arise about who owns the rights to content created by AI—whether it’s the developer, the user, or the AI itself. This ambiguity can lead to legal disputes and challenges in content attribution, particularly with tools like ai content creation tools and ai content creator tools.
- Misinformation: AI can generate highly convincing but false information, posing a risk of spreading misinformation. This can have serious consequences in areas like politics, health, and public safety. Ethical guidelines must be established to mitigate the risks associated with AI-generated misinformation, especially when using ai tools for content creation.
- Transparency: Users should be aware when they are interacting with AI-generated content. Transparency about the use of AI in content creation can help build trust and allow users to make informed decisions about the information they consume, particularly in contexts like creating content with ai and automated content creation.
- Accountability: Determining accountability for AI-generated content is challenging. If harmful content is produced, it is essential to identify who is responsible—the developers, the users, or the AI itself. Establishing clear accountability frameworks is vital for ethical AI use, especially in the realm of ai content generation tools and ai powered content creation.
4. Types of AI Content Creation
AI content creation encompasses various forms of media, each with unique characteristics and applications. Understanding these types can help users leverage AI effectively for their needs.
- Text Generation: This involves the use of AI to create written content, ranging from articles and blogs to poetry and stories. Text generation models, like GPT-3, utilize vast datasets to produce coherent and contextually relevant text, which is essential for applications like ai content creators and ai content creation software.
- Image Generation: AI can create images based on textual descriptions or existing images. Tools like DALL-E and Midjourney allow users to generate unique visuals, which can be used in marketing, art, and design, similar to the capabilities of ai content generation.
- Video Generation: AI technologies can also generate video content, including animations and deepfakes. These tools can create engaging visual narratives but also raise ethical concerns regarding authenticity and misinformation, particularly in the context of using ai for content creation.
- Audio Generation: AI can produce music, voiceovers, and sound effects. This technology is used in various applications, from creating background music for videos to generating realistic voice synthesis for virtual assistants, enhancing the overall content creation process.
4.1. Text Generation
Text generation is one of the most prominent applications of AI in content creation. It involves using algorithms to produce human-like text based on input prompts. This technology has transformed how content is created across various industries, including automated content generation and ai driven content creation.
- Natural Language Processing (NLP): Text generation relies heavily on NLP techniques, which enable machines to understand and generate human language. This includes parsing sentences, understanding context, and generating coherent responses.
- Applications: Â
- Blogging and Articles: AI can assist writers by generating ideas, outlines, or even full articles, saving time and enhancing creativity, particularly with tools like best free ai writer and jasper ai writing.
- Social Media Content: Businesses use AI to create engaging posts, captions, and responses, helping maintain an active online presence, such as with facebook post generator ai.
- Customer Support: AI-generated responses can streamline customer service by providing instant replies to common inquiries.
- Benefits: Â
- Efficiency: AI can produce content at a much faster rate than humans, allowing for quicker turnaround times.
- Cost-Effectiveness: Automating content generation can reduce labor costs, making it an attractive option for businesses.
- Scalability: AI can generate large volumes of content, making it easier for companies to scale their marketing efforts, especially with the help of best ai content creation tools.
- Challenges: Â
- Quality Control: While AI can generate text quickly, the quality may vary. Human oversight is often necessary to ensure accuracy and relevance.
- Creativity Limitations: AI lacks true creativity and may produce generic content. It often requires human input to add unique perspectives and insights.
- Ethical Concerns: As mentioned earlier, issues like bias and misinformation must be addressed to ensure responsible use of AI in text generation.
In conclusion, text generation is a powerful tool that can enhance content creation across various sectors. However, it is essential to navigate the ethical landscape and ensure that AI-generated content is used responsibly and effectively.
At Rapid Innovation, we understand the importance of these ethical considerations and work closely with our clients to implement AI solutions that not only drive efficiency and effectiveness but also adhere to ethical standards. By prioritizing transparency, accountability, and fairness, we help businesses achieve greater ROI while fostering trust and integrity in their AI applications.
4.1.1. Articles and Blog Posts
Articles and blog posts are essential tools for content marketing, providing valuable information to readers while enhancing a brand's online presence. They serve multiple purposes, including educating the audience, driving traffic to a website, and improving search engine rankings.
- Engaging Content: Articles should be well-researched and engaging, capturing the reader's attention from the start. Use compelling headlines and subheadings to break up text and make it easier to skim. For instance, Rapid Innovation can create insightful articles on AI trends that not only inform but also position your brand as a thought leader in the industry. This can include marketing articles, social media marketing articles, and articles related to marketing.
- SEO Optimization: Incorporate relevant keywords and phrases naturally throughout the content. This helps search engines understand the topic and improves visibility in search results. By optimizing your content for AI-related keywords, Rapid Innovation can help you attract a targeted audience interested in your services, including digital marketing articles and strategic marketing articles.
- Consistent Posting: Regularly updating a blog with fresh content keeps the audience engaged and encourages return visits. Aim for a consistent posting schedule, whether weekly, bi-weekly, or monthly. Rapid Innovation can assist in developing a content calendar that aligns with your business goals, featuring recent articles about marketing and current marketing articles.
- Call to Action: Each article should include a clear call to action (CTA), guiding readers on what to do next, whether it’s subscribing to a newsletter, downloading a resource, or exploring products. Effective CTAs can significantly enhance conversion rates, driving greater ROI for your business.
- Shareability: Create content that is easily shareable on social media platforms. Including share buttons and crafting catchy snippets can increase reach and engagement. Rapid Innovation can help you design shareable content that resonates with your audience, amplifying your brand's message. This can include promotion articles and influencer marketing articles. For more insights on AI capabilities, check out this GPT-4 Vision.
4.1.2. Marketing Copy
Marketing copy is the text used in promotional materials to persuade potential customers to take action. It plays a crucial role in branding and sales strategies, making it essential for businesses to master this skill.
- Clear Messaging: The copy should clearly convey the benefits of a product or service. Avoid jargon and focus on how the offering solves a problem or fulfills a need. Rapid Innovation can help you articulate the unique value of your AI solutions, making it easier for potential clients to understand their benefits, similar to business marketing articles and articles about content marketing.
- Emotional Appeal: Effective marketing copy often taps into the emotions of the audience. Use storytelling techniques to create a connection and resonate with readers on a personal level. By sharing success stories of clients who have achieved significant ROI through AI implementations, Rapid Innovation can enhance your marketing efforts.
- Strong Headlines: Headlines are the first impression and should be attention-grabbing. Use power words and numbers to create intrigue and encourage further reading. Rapid Innovation can assist in crafting compelling headlines that draw in your target audience, potentially inspired by marketing newspaper articles and article marketing service.
- Conciseness: Keep the copy concise and to the point. Use short sentences and bullet points to enhance readability and ensure key messages stand out. This approach can help potential clients quickly grasp the advantages of your offerings.
- A/B Testing: Experiment with different versions of marketing copy to see which performs better. A/B testing can provide insights into what resonates with the audience and drives conversions. Rapid Innovation can guide you through this process, ensuring you optimize your marketing strategies for maximum impact.
4.1.3. Technical Documentation
Technical documentation is crucial for products and services that require detailed explanations, such as software, machinery, or complex systems. It provides users with the necessary information to understand and effectively use a product.
- Clarity and Precision: Technical documents should be clear and precise, avoiding ambiguity. Use straightforward language and define any technical terms to ensure comprehension. Rapid Innovation can help create user-friendly documentation that demystifies complex AI technologies for your clients.
- Structured Format: Organize the documentation in a logical structure, using headings, subheadings, and bullet points. This makes it easier for users to find the information they need quickly. A well-structured document can significantly enhance user experience and satisfaction.
- Visual Aids: Incorporate diagrams, screenshots, and flowcharts to complement the text. Visual aids can enhance understanding and retention of complex information. Rapid Innovation can assist in creating visually appealing documentation that simplifies the learning process for users.
- User-Centric Approach: Consider the target audience when creating technical documentation. Tailor the content to their level of expertise, whether they are beginners or advanced users. This approach ensures that your documentation meets the needs of all users, ultimately leading to better product adoption.
- Regular Updates: Keep technical documentation up to date with the latest product features and changes. Regular reviews ensure that users have access to accurate and relevant information. Rapid Innovation can help you establish a process for maintaining your documentation, ensuring it remains a valuable resource for your clients.
4.2. Visual Content Creation
Visual content creation is a crucial aspect of digital marketing and communication strategies. It involves producing images, videos, infographics, and other visual elements that engage audiences and convey messages effectively. The rise of social media and digital platforms has made visual content more important than ever, as it can significantly enhance user engagement and retention.
- Visual content is processed 60,000 times faster than text. Â
- Posts with images receive 94% more views than those without. Â
- 65% of people are visual learners. Â
4.2.1. Image Generation
Image generation refers to the process of creating images using various tools and techniques, including photography, digital art, and AI-generated images. The importance of high-quality images cannot be overstated, as they play a vital role in attracting and retaining audience attention.
- Photography: Capturing high-quality photographs can enhance brand identity and storytelling. Professional images can convey emotions and messages that resonate with the audience. Â
- Digital Art: This includes illustrations, paintings, and other forms of art created digitally. Digital art can be tailored to fit specific brand aesthetics and can be used in various marketing materials. Â
- AI-Generated Images: With advancements in technology, AI tools can now generate images based on specific parameters. These tools can create unique visuals quickly and efficiently, making them a valuable resource for marketers. Rapid Innovation leverages AI-driven image generation to help clients produce tailored visuals that align with their brand identity, ultimately enhancing their marketing efforts and driving greater ROI.
When creating images, consider the following:
- Consistency: Ensure that the images align with your brand's visual identity. Â
- Relevance: Use images that are relevant to your content and audience. Â
- Quality: High-resolution images are essential for maintaining professionalism. Â
4.2.2. Graphic Design
Graphic design is the art of combining text and visuals to communicate ideas effectively. It encompasses a wide range of applications, from branding and advertising to web design and social media graphics. Good graphic design can significantly impact how a brand is perceived and can enhance user experience.
- Branding: Graphic design plays a crucial role in establishing a brand's identity. Logos, color schemes, and typography all contribute to how a brand is recognized and remembered. Â
- Marketing Materials: Brochures, flyers, and posters are all examples of graphic design in marketing. Effective design can capture attention and convey messages clearly. Â
- User Interface (UI) Design: In the digital realm, graphic design is essential for creating user-friendly interfaces. A well-designed UI can improve user experience and increase engagement. Rapid Innovation employs cutting-edge graphic design techniques to ensure that our clients' marketing materials are not only visually appealing but also strategically aligned with their business objectives, leading to improved customer engagement and higher conversion rates.
Key elements of effective graphic design include:
- Balance: Ensure that visual elements are evenly distributed to create a harmonious layout. Â
- Contrast: Use contrasting colors and fonts to highlight important information and create visual interest. Â
- Alignment: Proper alignment of text and images can enhance readability and organization. Â
In conclusion, both image generation and graphic design are integral components of visual content creation. By leveraging these elements effectively, brands can enhance their communication strategies and engage their audiences more effectively. Rapid Innovation is committed to helping clients harness the power of visual content creation tools to achieve their business goals efficiently and effectively. Additionally, the use of online tools for visual content creation can streamline the process and improve overall productivity. Whether through audio visual content creation or traditional graphic design, the importance of visual content creators cannot be overstated in today's digital landscape.
4.2.3. Video Content
Video content has become a dominant form of media consumption, making it essential for brands and creators to leverage its potential. The rise of platforms like YouTube, TikTok, and Instagram Reels has transformed how audiences engage with content.
- Engaging storytelling: Video allows for dynamic storytelling, combining visuals, sound, and motion to create a compelling narrative that can effectively communicate your brand's message. This is particularly important for video marketing videos and video advertising.
- Increased retention: Studies show that viewers retain 95% of a message when they watch it in a video compared to just 10% when reading text. This highlights the importance of video in conveying complex information succinctly and memorably, especially in marketing videos for business.
- Versatile formats: Video content can take various forms, including tutorials, vlogs, interviews, and live streams, catering to different audience preferences and enhancing user engagement. This versatility is crucial for video marketing companies and video marketing agencies.
- SEO benefits: Videos can improve search engine rankings, as they often lead to higher engagement rates and longer time spent on pages, ultimately driving more traffic to your website. This is particularly relevant for video content marketing and video marketing in real estate.
- Shareability: Video content is highly shareable, increasing the potential for virality and broader reach across social media platforms, which can significantly enhance brand visibility. This is especially true for social media and video marketing strategies.
To maximize the effectiveness of video content, creators should focus on high-quality production, clear messaging, and optimizing for mobile viewing. Additionally, incorporating calls to action can guide viewers toward desired outcomes, such as subscribing or visiting a website. At Rapid Innovation, we specialize in developing AI-driven video solutions that can analyze viewer behavior and preferences, allowing you to tailor your content for maximum impact and return on investment, including video marketing for small business and video email marketing.
4.3. Audio Content
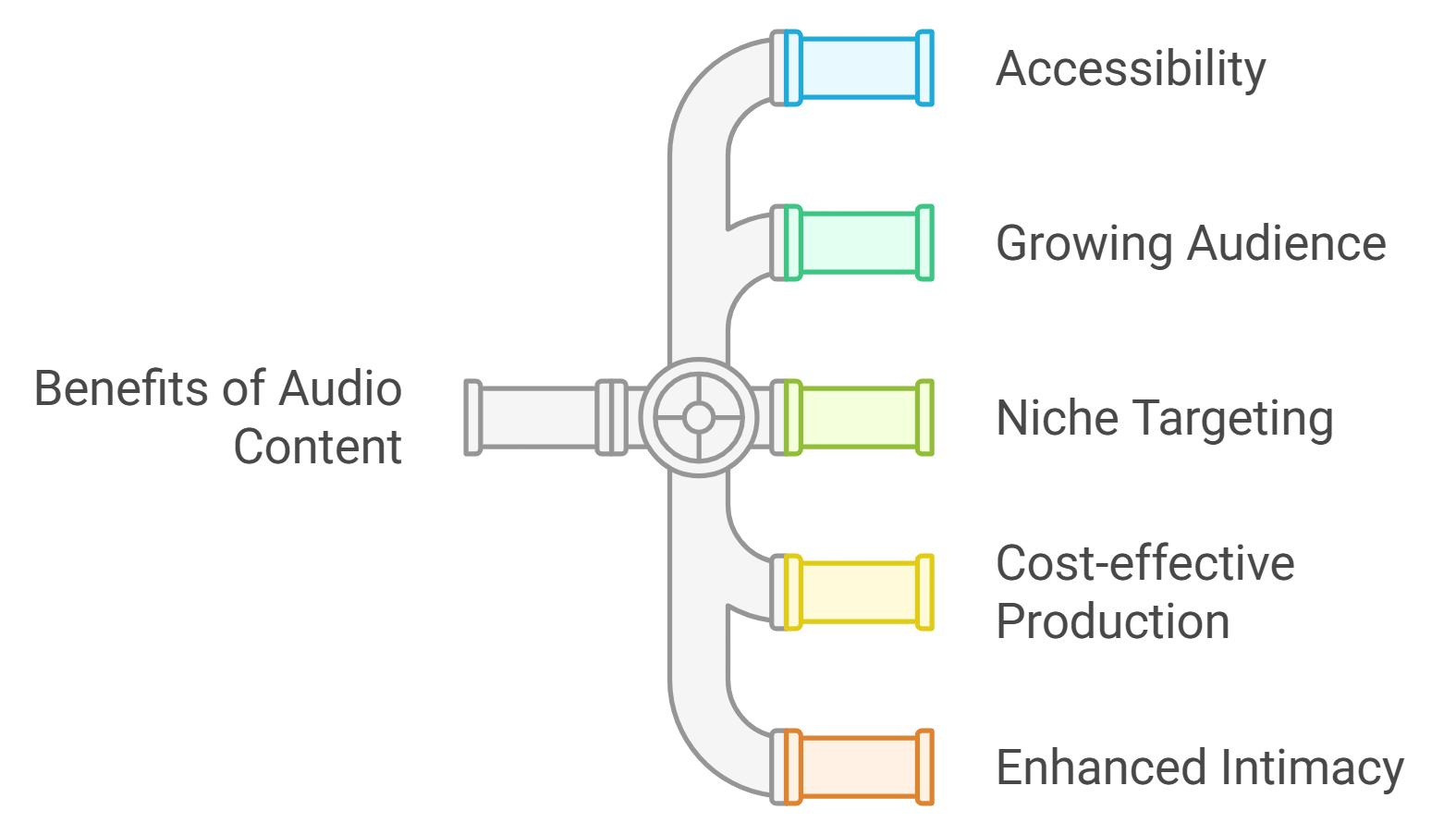
‍
Audio content has gained significant traction in recent years, particularly with the rise of podcasts and audiobooks. This format offers unique advantages for both creators and consumers.
- Accessibility: Audio content can be consumed on the go, allowing users to multitask while listening, which is particularly appealing in today’s fast-paced environment.
- Growing audience: The podcast industry has seen exponential growth, with over 100 million Americans listening to podcasts monthly. This presents a valuable opportunity for brands to connect with a dedicated audience.
- Niche targeting: Audio content allows creators to target specific audiences with tailored content, fostering a loyal listener base that can drive engagement and conversions.
- Cost-effective production: Producing audio content can be less expensive than video, making it accessible for creators with limited budgets while still delivering high-quality experiences.
- Enhanced intimacy: The personal nature of audio can create a deeper connection between the host and the audience, fostering trust and engagement that can translate into brand loyalty.
To create impactful audio content, it’s essential to focus on quality production, engaging scripts, and consistent publishing schedules. Additionally, promoting audio content through social media and other channels can help expand reach and attract new listeners.
4.3.1. Voiceovers
Voiceovers play a crucial role in enhancing audio and video content, providing a professional touch that can elevate the overall quality. They serve various purposes, from narrating stories to providing instructions in tutorials.
- Clarity and professionalism: A well-executed voiceover can clarify complex information and lend credibility to the content, ensuring that your message is communicated effectively.
- Emotional connection: The tone and delivery of a voiceover can evoke emotions, making the content more relatable and engaging for the audience.
- Brand identity: Consistent voiceover styles can help establish a brand's identity, making it recognizable to audiences and reinforcing brand values.
- Versatility: Voiceovers can be used across multiple platforms, including videos, podcasts, advertisements, and e-learning modules, providing a cohesive experience for users.
- Cost-effective: Hiring a professional voiceover artist can be a cost-effective way to enhance content without the need for extensive video production.
When creating voiceovers, it’s important to choose the right voice that aligns with the brand’s message and target audience. Additionally, investing in quality recording equipment and editing software can significantly improve the final product. Proper pacing, intonation, and clarity are essential for delivering an effective voiceover that resonates with listeners. At Rapid Innovation, we leverage AI technologies to optimize voiceover production, ensuring that your content not only sounds professional but also aligns with your strategic business goals.
4.3.2. Podcast Generation
Podcast generation has become a significant aspect of content creation, allowing individuals and organizations to share their ideas, stories, and expertise in an audio format. The rise of podcasts can be attributed to their accessibility and the growing demand for on-the-go content consumption. Podcasts can be produced on various topics, catering to diverse audiences, and they can be easily distributed through platforms like Apple Podcasts, Spotify, and Google Podcasts. The format allows for interviews, discussions, and storytelling, making it versatile. Engaging content can help build a loyal listener base, enhancing brand visibility, and podcasts can be monetized through sponsorships, advertisements, and listener donations.
The process of podcast generation typically involves several key steps:
- Planning: Define the podcast's theme, target audience, and episode structure.
- Recording: Use quality microphones and recording software to ensure clear audio.
- Editing: Edit the audio to remove mistakes, add music, and enhance sound quality.
- Publishing: Upload the final product to a podcast hosting platform.
- Promotion: Share episodes on social media and other channels to attract listeners.
As the podcasting landscape continues to evolve, creators are exploring innovative formats and technologies, such as interactive podcasts and augmented reality experiences, to engage their audiences further. At Rapid Innovation, we leverage AI-driven tools to streamline the podcast generation process, enabling clients to produce high-quality content efficiently. By utilizing AI for editing and sound enhancement, we help clients reduce production time and costs, ultimately leading to a greater return on investment (ROI). For more insights on the latest advancements in AI, check out top AI startups.
4.3.3. Music Composition
Music composition is a creative process that involves the arrangement of sounds to create a cohesive piece of music. This art form has evolved significantly with advancements in technology, allowing composers to explore new genres and styles. Composers can work in various fields, including film, television, video games, and advertising. Digital audio workstations (DAWs) have revolutionized music production, making it accessible to aspiring musicians. Collaboration with other artists and producers can enhance the creative process, and music composition can evoke emotions and set the tone for visual media, making it a crucial element in storytelling.
The music composition process generally includes the following stages:
- Inspiration: Draw ideas from personal experiences, emotions, or existing works.
- Melody Creation: Develop a catchy melody that serves as the foundation of the piece.
- Harmonic Structure: Add chords and harmonies to support the melody.
- Arrangement: Organize the different musical elements, including instrumentation and dynamics.
- Finalization: Record and produce the piece, ensuring high-quality sound.
With the rise of artificial intelligence in music composition, tools are emerging that can assist composers in generating melodies, harmonies, and even entire tracks, further expanding the possibilities in this field. Rapid Innovation harnesses these AI capabilities to help clients create unique musical compositions tailored to their specific needs, enhancing their projects and maximizing their investment.
4.4. Multimedia Content Integration
Multimedia content integration refers to the combination of various forms of media—such as text, audio, video, and graphics—into a cohesive presentation. This approach enhances user engagement and provides a richer experience for the audience. Integrated multimedia content can be used in websites, presentations, and social media campaigns. It caters to different learning styles, making information more accessible. The use of multimedia can improve retention rates and comprehension, and interactive elements, such as quizzes and polls, can further engage users.
Key components of multimedia content integration include:
- Content Planning: Identify the message and the best media formats to convey it.
- Design: Create visually appealing layouts that incorporate various media types.
- Development: Use software tools to combine text, images, audio, and video seamlessly.
- Testing: Ensure that all elements work together smoothly and are compatible across devices.
- Distribution: Share the integrated content through appropriate channels to reach the target audience.
As technology advances, the integration of augmented reality (AR) and virtual reality (VR) into multimedia content is becoming more prevalent, offering immersive experiences that captivate audiences and enhance storytelling. Rapid Innovation is at the forefront of this evolution, providing clients with cutting-edge solutions that integrate AI and multimedia to create compelling content that drives engagement and delivers measurable results.
5. Use Cases Across Industries
5.1. Marketing and Advertising
In the realm of marketing and advertising, the integration of advanced technologies and data analytics has transformed how brands connect with consumers. Here are some key use cases:
- Targeted Advertising: Marketers leverage data analytics to create highly targeted advertising campaigns. By analyzing consumer behavior and preferences, brands can deliver personalized ads that resonate with specific audiences, increasing engagement and conversion rates.
- Social Media Campaigns: Social media platforms provide a wealth of data that marketers can use to tailor their campaigns. By monitoring trends and user interactions, brands can adjust their strategies in real-time, ensuring their messages are relevant and timely.
- Content Marketing: Data-driven insights help marketers understand what type of content resonates with their audience. By analyzing engagement metrics, brands can create compelling content that drives traffic and builds brand loyalty, including data driven content marketing strategies.
- Influencer Marketing: Brands are increasingly collaborating with influencers to reach niche markets. By analyzing an influencer's audience demographics and engagement rates, companies can select the right partners to amplify their message.
- Email Marketing: Advanced segmentation techniques allow marketers to send personalized emails based on user behavior and preferences, which increases open rates and drives higher conversion rates.
- Predictive Analytics: Marketers use predictive analytics to forecast consumer behavior and trends, enabling them to make informed decisions about product launches, pricing strategies, and promotional campaigns.
- Data Driven Marketing: The overall approach to marketing has shifted towards data driven marketing strategies, where data analytics plays a crucial role in shaping campaigns and measuring their effectiveness.
5.2. Journalism and Media
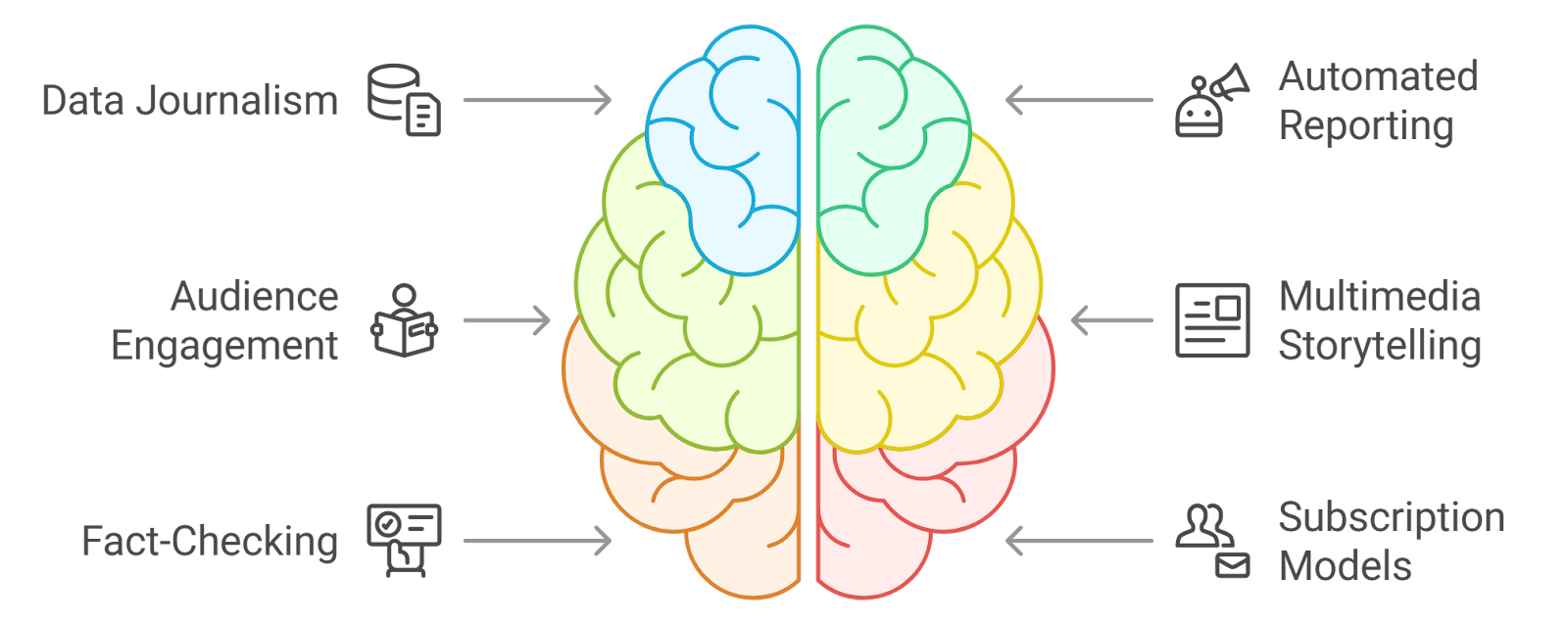
‍
The journalism and media industry has also seen significant changes due to technological advancements. Here are some notable use cases:
- Data Journalism: Journalists are increasingly using data to tell compelling stories. By analyzing large datasets, they can uncover trends and insights that traditional reporting might miss, enhancing the credibility and depth of news articles.
- Automated Reporting: News organizations are employing algorithms to generate reports on routine topics, such as financial earnings or sports scores. This allows journalists to focus on more complex stories while ensuring timely coverage of essential news.
- Audience Engagement: Media outlets are using analytics to understand their audience better. By tracking reader behavior, they can tailor content to meet the interests and preferences of their audience, leading to increased engagement.
- Multimedia Storytelling: The integration of video, audio, and interactive elements in news stories enhances the reader's experience. Journalists are using these tools to create immersive narratives that capture attention and convey information effectively.
- Fact-Checking: With the rise of misinformation, media organizations are employing technology to fact-check stories quickly. Automated tools can analyze claims and provide verification, helping to maintain journalistic integrity.
- Subscription Models: Many media outlets are shifting to subscription-based models. By analyzing user data, they can offer personalized content recommendations, increasing the likelihood of subscriptions and reader retention.
At Rapid Innovation, we harness these advanced technologies to empower our clients in marketing, advertising, journalism, and media. By implementing tailored AI solutions, we help businesses
5.3. Education and E-Learning
The landscape of education has transformed significantly with the advent of technology, particularly through online learning platforms. E-learning refers to the use of electronic technologies to access educational curriculum outside of a traditional classroom. This shift has made education more accessible and flexible for learners of all ages.
- Increased Accessibility: E-learning allows students from various geographical locations to access quality education, which is particularly beneficial for those in remote areas or with mobility issues. Rapid Innovation leverages AI to enhance accessibility features, ensuring that all learners can engage with content effectively.
- Flexible Learning: Students can learn at their own pace, choosing when and where to study. This flexibility accommodates different learning styles and schedules. Our AI-driven analytics can help institutions personalize learning paths, optimizing the educational experience for each student.
- Diverse Learning Resources: Online e learning platforms offer a variety of resources, including videos, quizzes, and interactive modules, catering to different learning preferences. Rapid Innovation can assist in developing intelligent content recommendation systems that adapt to individual learner needs.
- Cost-Effectiveness: E-learning courses often come at a lower cost compared to traditional education, making it more affordable for many learners. By implementing AI solutions, institutions can streamline operations and reduce costs, ultimately passing savings on to students.
- Global Reach: E-learning enables institutions to reach a global audience, allowing for diverse cultural exchanges and perspectives in education. Our consulting services can help organizations design and implement strategies to effectively engage international learners.
According to a report by Research and Markets, the global e-learning market is expected to reach $375 billion by 2026, highlighting the growing importance of this sector in education. For tailored solutions, consider our AI EdTech solutions to enhance your educational offerings.
5.4. Entertainment and Creative Industries
The entertainment and creative industries encompass a wide range of sectors, including film, music, gaming, and digital content creation. These industries have evolved dramatically with technological advancements, leading to new forms of entertainment and creative expression.
- Digital Transformation: The rise of streaming services has changed how audiences consume content. Platforms like Netflix and Spotify have revolutionized the way we access movies and music. Rapid Innovation can help content creators utilize AI for data-driven insights, enhancing viewer engagement and satisfaction.
- Interactive Experiences: Video games and virtual reality (VR) have created immersive experiences that engage users in ways traditional media cannot. This interactivity enhances user engagement and satisfaction. Our expertise in AI can assist developers in creating more responsive and adaptive gaming environments.
- Content Creation: Social media platforms have democratized content creation, allowing anyone with a smartphone to produce and share their work. This has led to the rise of influencers and user-generated content. Rapid Innovation can provide tools that analyze trends and optimize content strategies for creators.
- Global Collaboration: Technology enables collaboration across borders, allowing artists and creators to work together regardless of location. This fosters innovation and diversity in creative projects. Our consulting services can facilitate partnerships and collaborations in the creative sector.
- Economic Impact: The creative industries contribute significantly to the global economy. According to the World Economic Forum, the creative economy is projected to grow by 10% annually, underscoring its importance.
5.5. Technical and Professional Writing
Technical and professional writing is a specialized form of communication that conveys complex information clearly and concisely. This type of writing is essential in various fields, including technology, healthcare, and engineering.
- Clarity and Precision: Technical writing focuses on delivering information in a straightforward manner, ensuring that the audience understands the content without ambiguity. Rapid Innovation can enhance this process through AI tools that assist in content generation and editing.
- Audience Awareness: Writers must consider the knowledge level of their audience, tailoring the content to meet their needs. This may involve simplifying jargon or providing detailed explanations. Our AI solutions can analyze audience data to inform writing strategies.
- Documentation: Technical writers create user manuals, product specifications, and training materials that are crucial for effective communication in professional settings. Rapid Innovation can streamline documentation processes through
5.6. Customer Support and Communication
Effective customer support and communication are vital components of any successful business strategy. They not only enhance customer satisfaction but also foster loyalty and trust. Here are some key aspects to consider:
- Multi-Channel Support: Offering support through various channels such as email, phone, live chat, and social media ensures that customers can reach out in their preferred way. This flexibility can significantly improve the customer experience, allowing businesses to cater to diverse customer preferences. Utilizing help desk software can streamline this process.
- Response Time: Quick response times are crucial. Studies show that 60% of customers expect a response within an hour. Implementing automated responses for common queries can help manage expectations and improve efficiency, ultimately leading to higher customer retention rates. Tools like support ticketing software can assist in tracking and managing inquiries effectively.
- Personalization: Tailoring communication to individual customers can enhance their experience. Using customer data to address them by name and reference past interactions can make support feel more personal and attentive, fostering a deeper connection with the brand. Customer service platforms can facilitate this level of personalization.
- Feedback Mechanisms: Regularly soliciting feedback through surveys or follow-up emails can provide valuable insights into customer satisfaction and areas for improvement. This not only shows customers that their opinions matter but also helps businesses refine their services, leading to enhanced customer loyalty. Implementing customer care outsourcing services can also help gather and analyze feedback effectively.
- Training and Development: Investing in training for customer support representatives ensures they are knowledgeable and equipped to handle inquiries effectively. Continuous development can lead to better problem-solving skills and improved customer interactions, which are essential for maintaining a competitive edge. Utilizing customer service management software can aid in tracking training progress.
- Knowledge Base: Creating a comprehensive knowledge base or FAQ section can empower customers to find answers independently. This resource can reduce the volume of support requests and enhance the user experience, allowing support teams to focus on more complex issues. Simple help desk ticketing systems can help manage and organize this information efficiently. For more insights on enhancing customer support, check out AI-driven customer support.
5.7. Research and Academic Writing
Research and academic writing are essential skills in various fields, from education to business. They require a structured approach and adherence to specific guidelines. Here are some critical elements to consider:
- Understanding the Audience: Knowing the target audience is crucial for effective academic writing. Tailoring the content to meet the expectations and knowledge level of the audience can enhance engagement and comprehension.
- Thesis Development: A clear and concise thesis statement is the backbone of any academic paper. It should articulate the main argument or purpose of the research, guiding the direction of the writing.
- Research Methodology: Selecting the appropriate research methodology is vital. Whether qualitative, quantitative, or mixed methods, the chosen approach should align with the research question and objectives.
- Citing Sources: Proper citation of sources is essential to avoid plagiarism and lend credibility to the work. Familiarity with citation styles such as APA, MLA, or Chicago is necessary for academic integrity.
- Drafting and Revising: Writing is a process that involves drafting, revising, and editing. Allowing time for revisions can lead to clearer arguments and improved overall quality of the writing.
- Peer Review: Engaging in peer review can provide constructive feedback and enhance the quality of the research. Collaborating with colleagues can lead to new insights and strengthen the final output.
6. Solution Landscape
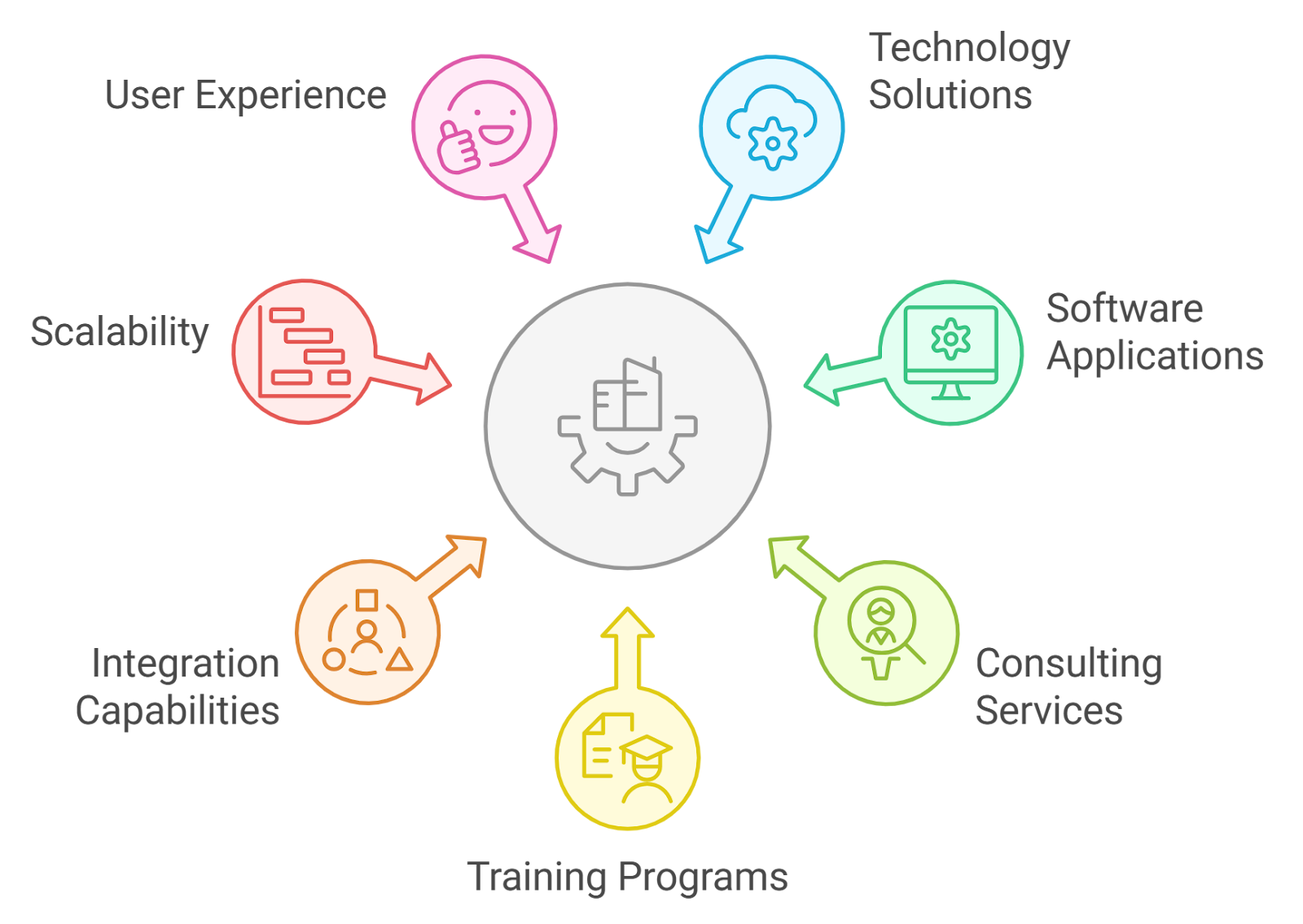
‍
The solution landscape refers to the array of tools, technologies, and methodologies available to address specific challenges within a given industry or sector. Understanding this landscape is crucial for organizations looking to innovate and improve their operations. Here are some key components:
- Technology Solutions: The rapid advancement of technology has led to a plethora of solutions, including cloud computing, artificial intelligence, and data analytics. These tools can streamline processes, enhance decision-making, and improve efficiency, ultimately driving greater ROI for businesses.
- Software Applications: Various software applications cater to different business needs, from customer relationship management (CRM) systems to project management tools. Selecting the right software, such as outsourced customer service solutions or customer care platforms, can significantly impact productivity and collaboration, enabling teams to work more effectively.
- Consulting Services: Many organizations turn to consulting firms for expertise in navigating complex challenges. These services can provide tailored strategies and insights based on industry best practices, helping clients achieve their business goals more efficiently.
- Training and Development Programs: Investing in employee training can enhance skills and knowledge, ensuring that teams are equipped to leverage new solutions effectively. Continuous learning is essential in a rapidly changing environment, fostering innovation and adaptability.
- Integration Capabilities: The ability to integrate different solutions is crucial for maximizing efficiency. Organizations should consider how new tools, such as outsourcing of customer service, will work with existing systems to avoid silos and ensure seamless operations, which can lead to improved performance.
- Scalability: Solutions should be scalable to accommodate growth. Organizations need to assess whether a solution can adapt to increasing demands without compromising performance, ensuring long-term sustainability.
- User Experience: The usability of a solution is paramount. Solutions that are intuitive and user-friendly, such as best help desk software or live chat software, can lead to higher adoption rates and better overall satisfaction among employees and customers alike, ultimately contributing to enhanced business outcomes.
6.1. Enterprise AI Content Platforms
Enterprise AI content platforms are sophisticated tools designed to streamline content creation, management, and distribution for large organizations. These platforms leverage artificial intelligence to enhance productivity and ensure consistency across various content types. They can generate articles, reports, and marketing materials automatically, saving time and resources. AI algorithms analyze user data to create tailored content that resonates with specific audiences, improving engagement rates. Many enterprise platforms offer collaborative tools that allow teams to work together seamlessly, regardless of location. Additionally, they provide in-depth analytics to track content performance, helping organizations refine their strategies based on real-time data. These platforms can easily scale to meet the growing content demands of large enterprises, accommodating various formats and channels.
At Rapid Innovation, we specialize in implementing these enterprise AI content platforms to help our clients achieve greater ROI. By automating content generation and management, organizations can significantly reduce operational costs while enhancing the quality and relevance of their content. Our expertise ensures that clients can leverage these platforms effectively, driving engagement and conversion rates.
Examples of popular enterprise AI content platforms include Adobe Experience Manager, HubSpot, and Contentful, which are known for their robust features and user-friendly interfaces. For more insights on the impact of AI in media and entertainment, check out our article on AI in Media and Entertainment.
6.2. Specialized Content Generation Tools
Specialized content generation tools focus on specific types of content or industries, providing tailored solutions that meet unique needs. These tools often incorporate advanced AI technologies to enhance the quality and efficiency of content production. Tools like Copy.ai and Jasper are designed for specific content types, such as marketing copy, blog posts, or social media content. Many specialized tools come equipped with SEO features that help users optimize their content for search engines, improving visibility and traffic. These tools often have intuitive interfaces that make it easy for users to create high-quality content without extensive training. They can often integrate with other marketing tools and platforms, streamlining workflows and enhancing productivity. Specialized tools can be more affordable than comprehensive enterprise solutions, making them accessible for small to medium-sized businesses.
At Rapid Innovation, we guide clients in selecting and implementing specialized content generation tools that align with their specific business objectives. By optimizing content production processes, we help clients achieve higher efficiency and better engagement metrics.
Some notable specialized content generation tools include Writesonic, Grammarly, and Surfer SEO, each offering unique features tailored to specific content needs.
6.3. Open-Source Solutions
Open-source solutions for content generation provide users with the flexibility to customize and modify the software according to their specific requirements. These solutions are often community-driven, allowing for continuous improvement and innovation. Open-source tools are typically free to use, making them an attractive option for startups and small businesses with limited budgets. Users can modify the source code to tailor the software to their unique content needs, ensuring it aligns with their business objectives. Open-source projects often have active communities that provide support, share best practices, and contribute to ongoing development. The open nature of these solutions allows users to inspect the code for security and reliability, fostering trust in the software. Many open-source solutions can be integrated with existing systems, enhancing their functionality and usability.
At Rapid Innovation, we assist clients in navigating the landscape of open-source content generation tools, ensuring they can leverage these solutions effectively while minimizing costs. Our expertise in customization and integration helps clients maximize the value derived from these tools.
Popular open-source content generation tools include WordPress, Ghost, and Hugo, which are widely used for creating and managing various types of content.
6.4. Cloud-Based Content Generation Services
Cloud-based content generation services have revolutionized the way businesses create and manage their content. These services leverage the power of cloud computing to provide scalable, efficient, and cost-effective solutions for cloudbased content generation.
- Accessibility: Users can access content generation tools from anywhere with an internet connection, facilitating remote work and collaboration.
- Scalability: Businesses can easily scale their content production up or down based on demand without the need for significant investment in infrastructure.
- Collaboration: Multiple users can work on content simultaneously, enhancing teamwork and speeding up the content creation process.
- Cost-Effectiveness: Many cloud-based services operate on a subscription model, allowing businesses to pay only for what they use, which can be more economical than traditional content creation methods.
- Automation: These services often include AI-driven tools that can automate various aspects of content generation, from writing to editing, saving time and resources.
At Rapid Innovation, we harness these cloud-based solutions to help our clients streamline their content creation processes, ultimately leading to greater ROI through enhanced efficiency and reduced operational costs. Our expertise in AI-driven solutions ensures that our clients can leverage data insights to refine their content strategies effectively. Additionally, we explore innovative applications of AI in business, such as leveraging ChatGPT to enhance content generation and engagement.
6.5. Integration Strategies
Integration strategies are essential for businesses looking to streamline their content generation processes. By effectively integrating various tools and platforms, organizations can enhance productivity and ensure a seamless workflow.
- API Utilization: Leveraging Application Programming Interfaces (APIs) allows different software systems to communicate, enabling data sharing and functionality across platforms.
- Centralized Dashboards: Implementing centralized dashboards can help teams monitor content performance and manage multiple content generation tools from one location.
- Workflow Automation: Automating repetitive tasks through integration can save time and reduce errors, allowing teams to focus on more strategic content initiatives.
- Cross-Platform Compatibility: Ensuring that content generation tools are compatible with existing systems (like CRM and CMS) can facilitate smoother operations and data flow.
- Training and Support: Providing adequate training for staff on integrated systems can maximize the benefits of these strategies, ensuring that everyone is on the same page.
At Rapid Innovation, we specialize in developing tailored integration strategies that enhance operational efficiency, improve content quality, and foster collaboration among team members, ultimately driving better business outcomes.
6.6. Customization and Tailoring Options
Customization and tailoring options are critical for businesses that want to create content that resonates with their target audience. By offering personalized content experiences, companies can improve engagement and drive better results.
- User Preferences: Allowing users to set preferences for content types, styles, and formats can lead to more relevant and appealing content.
- Template Creation: Customizable templates can help maintain brand consistency while allowing for flexibility in content creation.
- Dynamic Content: Implementing dynamic content that changes based on user behavior or demographics can enhance user experience and increase conversion rates.
- Feedback Mechanisms: Incorporating feedback tools can help businesses understand audience preferences and adjust content strategies accordingly.
- A/B Testing: Utilizing A/B testing for different content variations can provide insights into what resonates best with the audience, allowing for data-driven decisions.
By focusing on customization and tailoring options, Rapid Innovation empowers businesses to create more impactful content that meets the specific needs of their audience, ultimately leading to higher engagement and conversion rates. Our expertise in AI-driven solutions ensures that our clients can leverage data insights to refine their content strategies effectively.
7. Technical Implementation Strategies
Technical implementation strategies are crucial for the successful deployment of any project, especially in fields like software development, data science, and machine learning. These strategies ensure that the project is not only functional but also scalable, efficient, and maintainable.
7.1 Infrastructure Requirements
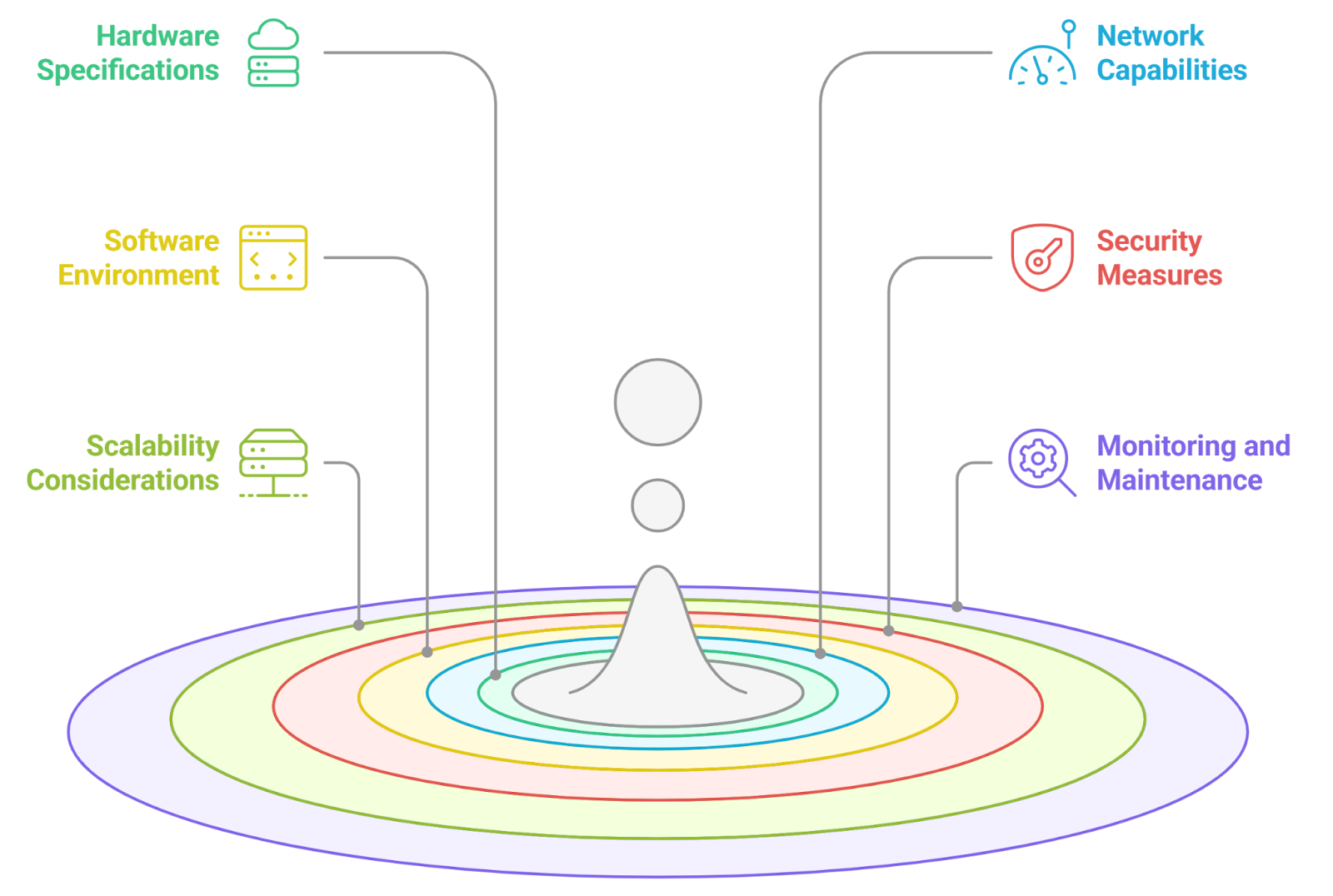
‍
Infrastructure requirements refer to the hardware and software resources necessary to support the implementation of a project. Proper infrastructure is essential for ensuring that applications run smoothly and can handle the expected load.
- Hardware Specifications: Determine the necessary server specifications, including CPU, RAM, and storage. Consider using cloud services like AWS, Google Cloud, or Azure for scalable resources, which Rapid Innovation can help you configure to meet your specific needs.
- Network Capabilities: Ensure a reliable and fast internet connection to support data transfer and application performance. Implement load balancers to distribute traffic evenly across servers, optimizing performance and user experience.
- Software Environment: Choose the right operating system (Linux, Windows) based on project requirements. Rapid Innovation can assist in selecting and installing necessary software tools and libraries, such as databases (MySQL, MongoDB) and programming languages (Python, Java), tailored to your project.
- Security Measures: Implement firewalls and intrusion detection systems to protect sensitive data. Use encryption protocols for data in transit and at rest, ensuring compliance with industry standards and safeguarding your business assets.
- Scalability Considerations: Design the infrastructure to accommodate future growth, including the ability to add more servers or increase storage. Utilize containerization technologies like Docker to ensure consistent environments across development and production, which Rapid Innovation can help you implement effectively.
- Monitoring and Maintenance: Set up monitoring tools (like Prometheus or Grafana) to track system performance and health. Plan for regular maintenance schedules to update software and address potential vulnerabilities, ensuring your systems remain robust and secure.
7.2 Model Selection and Evaluation
Model selection and evaluation are critical steps in the implementation of machine learning and data-driven projects. Choosing the right model can significantly impact the performance and accuracy of predictions.
- Understanding the Problem: Clearly define the problem you are trying to solve, whether it’s classification, regression, or clustering. Identify the key performance indicators (KPIs) that will measure success, allowing Rapid Innovation to align the model with your business objectives.
- Data Preparation: Clean and preprocess the data to ensure quality inputs for the model. Split the dataset into training, validation, and test sets to evaluate model performance accurately, a process that Rapid Innovation can streamline for you.
- Model Selection: Evaluate different algorithms based on the problem type, such as decision trees, neural networks, or support vector machines. Consider factors like interpretability, training time, and resource requirements when selecting a model, with guidance from Rapid Innovation's expertise.
- Hyperparameter Tuning: Optimize model performance by adjusting hyperparameters using techniques like grid search or random search. Use cross-validation to ensure that the model generalizes well to unseen data, enhancing its reliability.
- Model Evaluation: Assess model performance using metrics relevant to the problem, such as accuracy, precision, recall, and F1 score. Utilize confusion matrices and ROC curves to visualize model performance, providing insights that Rapid Innovation can help interpret.
- Iterative Improvement: Continuously monitor model performance and make adjustments as necessary. Incorporate feedback loops to refine the model based on new data or changing requirements, ensuring it remains effective over time.
- Deployment Considerations: Ensure that the selected model can be easily integrated into the existing infrastructure. Plan for ongoing maintenance and updates to the model as new data becomes available, a service that Rapid Innovation can provide to maximize your ROI.
By focusing on these technical implementation strategies, organizations can enhance their project outcomes, ensuring that they are well-equipped to handle the complexities of modern technology environments. Rapid Innovation is committed to guiding you through these processes, helping you achieve your business goals efficiently and effectively.
7.3. Custom Model Development
Custom model development is a crucial aspect of machine learning and artificial intelligence, allowing organizations to tailor solutions to their specific needs. This process involves creating models that are not only unique but also optimized for particular tasks or datasets, ultimately driving greater ROI for businesses.
- Understanding Requirements: Â
- Identify the specific problem to be solved.
- Gather requirements from stakeholders to ensure alignment with business goals.
- Data Collection and Preparation: Â
- Collect relevant data that reflects the problem domain.
- Clean and preprocess data to remove noise and inconsistencies.
- Split data into training, validation, and test sets for effective model evaluation.
- Model Selection: Â
- Choose the appropriate algorithms based on the problem type (e.g., classification, regression).
- Consider using frameworks like TensorFlow or PyTorch for flexibility in model design.
- Training the Model: Â
- Train the model using the training dataset while tuning hyperparameters for optimal performance.
- Utilize techniques like cross-validation to ensure the model generalizes well to unseen data.
- Evaluation and Iteration: Â
- Evaluate the model using the validation set and metrics such as accuracy, precision, and recall.
- Iterate on the model design based on performance feedback, making necessary adjustments.
- Deployment: Â
- Once satisfied with the model's performance, deploy it into a production environment.
- Monitor the model's performance post-deployment to ensure it continues to meet business objectives.
7.4. Performance Optimization
Performance optimization is essential for ensuring that machine learning models operate efficiently and effectively. This process involves refining models to improve their speed, accuracy, and resource utilization, which can significantly enhance the return on investment for clients.
- Algorithm Optimization: Â
- Select algorithms that are computationally efficient for the given task.
- Implement techniques like feature selection to reduce dimensionality and improve processing speed.
- Hyperparameter Tuning: Â
- Use methods such as grid search or random search to find the best hyperparameters.
- Consider automated tools like Optuna or Hyperopt for more efficient tuning.
- Model Compression: Â
- Apply techniques like pruning, quantization, or knowledge distillation to reduce model size without sacrificing performance. This is particularly important for deploying models on edge devices with limited resources.
- Parallel Processing: Â
- Leverage parallel processing capabilities to speed up training and inference.
- Utilize frameworks that support distributed computing, such as Apache Spark or Dask.
- Continuous Monitoring: Â
- Implement monitoring tools to track model performance in real-time.
- Use feedback loops to continuously improve the model based on new data and changing conditions.
7.5. Scalability Considerations
Scalability is a critical factor in the development and deployment of machine learning models, especially as data volumes and user demands grow. Ensuring that models can scale effectively is vital for long-term success and maximizing ROI.
- Infrastructure Planning: Â
- Choose cloud-based solutions like AWS, Google Cloud, or Azure that offer scalable resources.
- Consider containerization with Docker or orchestration with Kubernetes for flexible deployment.
- Load Balancing: Â
- Implement load balancing techniques to distribute incoming requests evenly across multiple instances of the model. This helps maintain performance during peak usage times.
- Data Management: Â
- Use scalable data storage solutions such as NoSQL databases or data lakes to handle large datasets.
- Ensure that data pipelines can efficiently process and feed data into the model.
- Model Versioning: Â
- Implement version control for models to manage updates and rollbacks effectively. This allows for experimentation with new models while maintaining stability in production.
- Future-Proofing: Â
- Design models with future scalability in mind, allowing for easy integration of new features or data sources.
- Stay updated with advancements in technology and methodologies to adapt to changing requirements.
By leveraging Rapid Innovation's expertise in best practices for transformer model development, performance optimization, and scalability considerations, organizations can achieve their business goals more efficiently and effectively, ultimately leading to greater returns on their investments.
8. Ethical and Legal Considerations
In the digital age, ethical and legal considerations are paramount for individuals and organizations alike. As content creation and distribution have become more accessible, understanding the implications of intellectual property and plagiarism is essential for maintaining integrity and compliance.
8.1 Intellectual Property Challenges
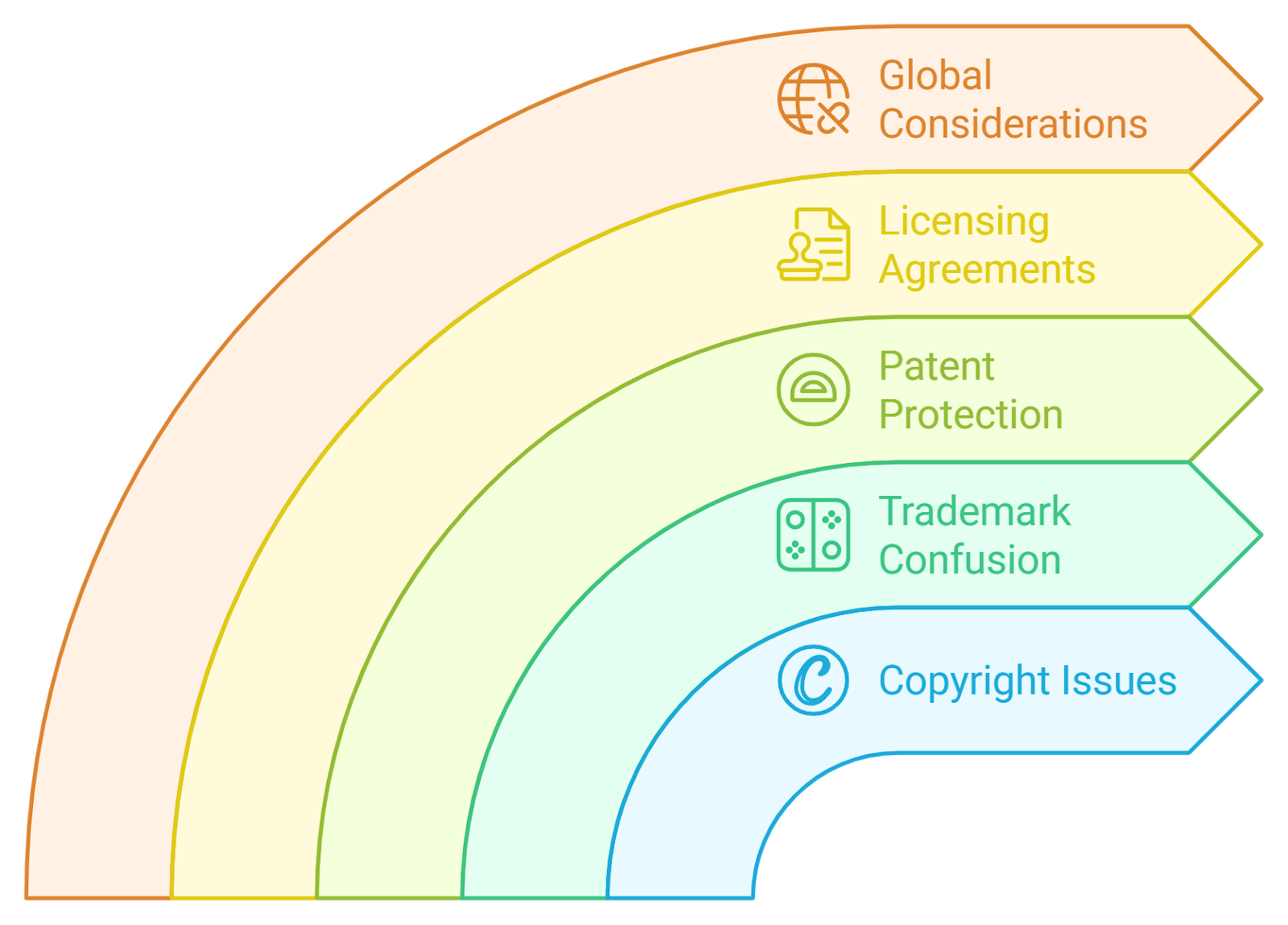
‍
Intellectual property (IP) refers to creations of the mind, including inventions, literary and artistic works, designs, symbols, names, and images used in commerce. The challenges surrounding IP are significant in today's interconnected world, including patent challenges, trademark challenges, and intellectual property challenges.
- Copyright Issues: Copyright protects original works of authorship, but the ease of copying and sharing digital content can lead to unintentional infringements. Creators must be vigilant about their rights and the rights of others, especially in the context of copyright challenges in the digital age.
- Trademark Confusion: Trademarks protect brand names and logos. Misuse or unauthorized use of trademarks can lead to legal disputes. Businesses must ensure that their branding does not infringe on existing trademarks, addressing challenges of intellectual property rights.
- Patent Protection: Innovations and inventions can be patented, but the process can be complex and costly. Understanding patent law is crucial for inventors to protect their creations effectively, particularly in light of challenges in protecting intellectual property.
- Licensing Agreements: Many creators use licensing agreements to allow others to use their work while retaining ownership. Understanding the terms of these agreements is vital to avoid legal issues, including no challenge clause patent license.
- Global Considerations: IP laws vary significantly across countries. What is permissible in one jurisdiction may not be in another, complicating international business and content sharing, and leading to intellectual property rights challenges.
8.2 Plagiarism and Content Originality
Plagiarism is the act of using someone else's work, ideas, or expressions without proper attribution, presenting them as one's own. This ethical breach can have serious consequences in academic, professional, and creative fields.
Plagiarism can take many forms, including direct copying, paraphrasing without credit, and self-plagiarism (reusing one's own previously published work without acknowledgment). The repercussions of plagiarism can range from academic penalties to legal action. Institutions often have strict policies against plagiarism, which can lead to expulsion or loss of credibility.
Original content is not only ethically sound but also essential for SEO. Search engines favor unique content, which can improve visibility and ranking. Various plagiarism detection tools are available to help writers ensure their work is original. These tools can scan documents for similarities with existing content, providing a safeguard against unintentional plagiarism.
Properly citing sources is crucial in maintaining academic and professional integrity. Familiarizing oneself with citation styles (APA, MLA, Chicago, etc.) can help ensure that all sources are credited appropriately. Fostering an environment that values original thought and creativity can reduce the temptation to plagiarize. Encouraging brainstorming and collaboration can lead to innovative ideas that are uniquely one's own, addressing challenges of protecting intellectual property.
At Rapid Innovation, we understand the importance of navigating these ethical and legal landscapes. Our AI-driven solutions can assist clients in ensuring compliance with IP laws and maintaining content originality, ultimately leading to greater ROI and sustainable business practices. By leveraging our expertise, organizations can focus on innovation while we help mitigate risks associated with intellectual property challenges, IP challenges, and IPR challenges.
8.3. Bias Mitigation
Bias mitigation is a critical aspect of developing fair and equitable artificial intelligence (AI) systems. Bias can manifest in various forms, including data bias, algorithmic bias, and human bias. Addressing these biases is essential to ensure that AI technologies do not perpetuate existing inequalities or create new forms of discrimination.
- Data Bias: This occurs when the data used to train AI models is not representative of the population it serves. For example, if an AI system is trained primarily on data from one demographic group, it may perform poorly for others.
- Algorithmic Bias: Algorithms can inadvertently favor certain outcomes based on how they are designed. This can lead to skewed results that reinforce stereotypes or unfairly disadvantage specific groups.
- Human Bias: Developers and data scientists may unintentionally introduce their biases into AI systems. Awareness and training are crucial to minimize this risk.
To mitigate bias, organizations can implement several strategies:
- Diverse Data Collection: Ensure that training datasets are diverse and representative of all relevant demographics.
- Regular Audits: Conduct regular audits of AI systems to identify and rectify biases.
- Inclusive Design: Involve diverse teams in the design and development process to bring multiple perspectives to the table.
- Algorithmic Fairness Techniques: Utilize techniques such as adversarial debiasing and fairness constraints to create more equitable algorithms. Tools like ai fairness 360 and ai bias mitigation can be instrumental in this process.
At Rapid Innovation, we specialize in helping organizations implement these strategies effectively. By leveraging our expertise in AI development, we assist clients in creating systems that not only perform well but also uphold fairness and equity, ultimately leading to greater trust and satisfaction among users. For a comprehensive understanding of ethical AI practices, refer to our ethical AI development guide.
8.4. Transparency and Disclosure
Transparency and disclosure are vital components of ethical AI development. They involve making the workings of AI systems understandable to users and stakeholders, which can help build trust and accountability.
- Explainability: AI systems should be designed to provide clear explanations for their decisions. This is particularly important in high-stakes areas such as healthcare, finance, and criminal justice, where decisions can significantly impact individuals' lives.
- Documentation: Comprehensive documentation of AI models, including their design, data sources, and decision-making processes, is essential. This allows stakeholders to understand how and why decisions are made.
- User Awareness: Organizations should inform users about the AI systems they interact with, including potential limitations and risks. This empowers users to make informed decisions about their engagement with AI technologies.
To enhance transparency, organizations can adopt the following practices:
- Open Source Models: Sharing code and models can promote transparency and allow for external scrutiny.
- Regular Reporting: Publish regular reports on AI system performance, including metrics related to fairness and bias.
- Stakeholder Engagement: Involve stakeholders in discussions about AI system design and implementation to gather diverse perspectives and foster trust.
At Rapid Innovation, we prioritize transparency in our AI solutions. By ensuring that our clients' AI systems are explainable and well-documented, we help them build trust with their users, which is essential for long-term success.
8.5. Regulatory Landscape
The regulatory landscape for AI is rapidly evolving as governments and organizations recognize the need for guidelines to govern AI development and deployment. This landscape varies significantly across regions, reflecting different cultural, ethical, and legal considerations.
- Existing Regulations: Some countries have already implemented regulations addressing AI, such as the European Union's General Data Protection Regulation (GDPR) and the proposed AI Act. These regulations focus on data protection, privacy, and accountability in AI systems.
- Emerging Frameworks: Many governments are developing frameworks to guide the ethical use of AI. These frameworks often emphasize principles such as fairness, accountability, and transparency.
- Industry Standards: Various industry groups are also working to establish standards for AI development. These standards aim to ensure that AI technologies are safe, reliable, and ethical.
Organizations must stay informed about the regulatory landscape to ensure compliance and mitigate legal risks. Key considerations include:
- Monitoring Legislation: Regularly review and adapt to new laws and regulations related to AI.
- Engaging with Policymakers: Collaborate with policymakers to contribute to the development of fair and effective AI regulations.
- Implementing Best Practices: Adopt industry best practices to align with regulatory expectations and promote ethical AI development.
At Rapid Innovation, we guide our clients through the complexities of the regulatory landscape, ensuring that their AI initiatives comply with current laws and standards. By doing so, we help them mitigate risks while fostering innovation and responsible AI development.
8.6. Responsible AI Content Creation
Responsible AI content creation refers to the ethical and thoughtful use of artificial intelligence in generating content. As AI technologies evolve, it is crucial to ensure that the content produced is not only high-quality but also aligns with ethical standards.
- Ethical considerations: AI-generated content should respect copyright laws, avoid plagiarism, and ensure that it does not propagate misinformation or harmful stereotypes. This is particularly important when using ai content creation tools and automated content generation methods.
- Transparency: It is essential to disclose when content is AI-generated. This builds trust with the audience and allows them to understand the source of the information, especially when utilizing ai tools for content creation.
- Inclusivity: AI systems should be trained on diverse datasets to avoid biases, ensuring that the content reflects a wide range of perspectives and experiences. This is vital for ai content creators and those employing ai driven content creation strategies.
- Accountability: Organizations using ai for content creation should have clear policies in place to address any issues that arise from the content produced, including mechanisms for feedback and correction. This is crucial for those relying on ai content creation software.
- Continuous improvement: Regularly updating AI models and datasets can help improve the quality and relevance of the content generated, ensuring it meets the evolving needs of users. This is particularly relevant for automated content creation and ai powered content creation.
9. Performance and Quality Management
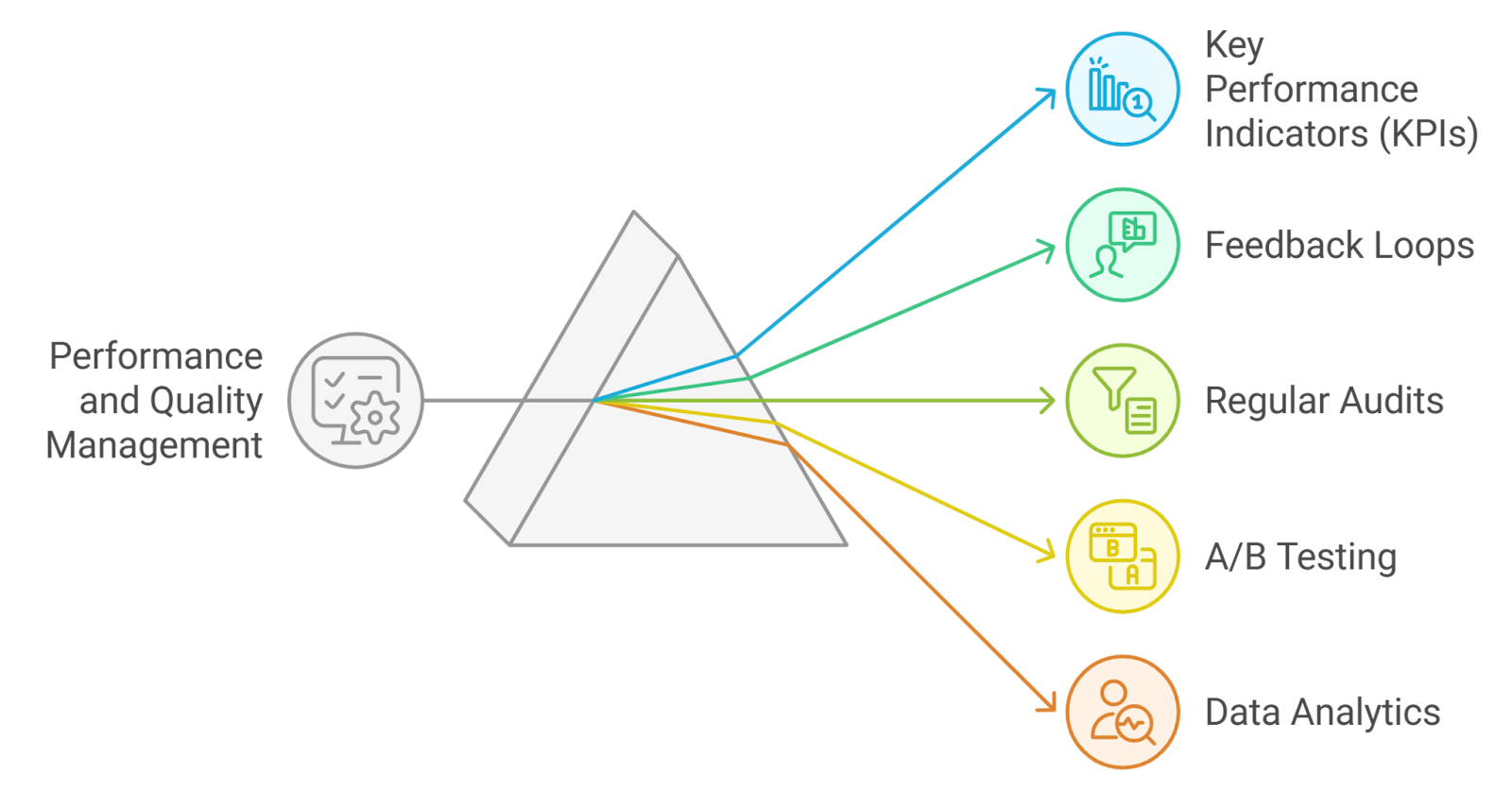
‍
Performance and quality management in content creation involves monitoring and evaluating the effectiveness and quality of the content produced. This process is vital for maintaining high standards and ensuring that the content meets the intended goals.
- Key performance indicators (KPIs): Establishing KPIs helps measure the success of content. Common KPIs include engagement rates, conversion rates, and audience reach.
- Feedback loops: Gathering feedback from users can provide insights into how well the content resonates with the audience. This can be done through surveys, comments, and social media interactions.
- Regular audits: Conducting content audits helps identify areas for improvement, including checking for outdated information, broken links, and overall content relevance. This is essential for those using content generation tools.
- A/B testing: Experimenting with different content formats, headlines, or calls to action can help determine what works best for the audience.
- Data analytics: Utilizing analytics tools can provide valuable data on user behavior, helping to refine content strategies and improve overall performance.
9.1. Content Validation Techniques
Content validation techniques are essential for ensuring the accuracy, reliability, and quality of the content produced. These techniques help organizations maintain credibility and trust with their audience.
- Fact-checking: Verifying the accuracy of information before publication is crucial. This can involve cross-referencing with reputable sources and using fact-checking tools.
- Peer review: Having content reviewed by experts in the field can enhance credibility. Peer reviews can provide insights and catch errors that may have been overlooked.
- User-generated content: Encouraging audience participation can help validate content. User comments and contributions can provide additional perspectives and insights.
- Plagiarism detection: Using plagiarism detection tools ensures that the content is original and does not infringe on others' intellectual property. This is particularly important for those engaged in ai automated content creation.
- Consistency checks: Ensuring that the content aligns with the organization's voice, style, and messaging is vital for maintaining brand integrity. Regular reviews can help achieve this consistency.
At Rapid Innovation, we understand the importance of responsible AI content creation and performance management. By implementing these practices, we help our clients achieve greater ROI through high-quality, ethical content that resonates with their target audience. Our expertise in ai content creator tools allows us to develop tailored solutions that not only meet business goals but also uphold the highest standards of integrity and effectiveness. We also offer free ai content creation tools to help organizations get started on their journey. For a deeper understanding of the methodologies involved, you can refer to our retrieval-augmented generation.
9.2. Human-in-the-Loop Strategies
Human-in-the-loop (HITL) strategies are essential in enhancing the performance of machine learning models and artificial intelligence systems. These strategies involve integrating human feedback into the decision-making processes of AI systems, ensuring that the technology aligns with human values and expectations.
- Enhances accuracy: By incorporating human judgment, models can be fine-tuned to improve accuracy, especially in complex scenarios where automated systems may struggle.
- Provides contextual understanding: Humans can offer insights that machines may overlook, particularly in nuanced situations requiring emotional intelligence or cultural awareness.
- Facilitates iterative learning: Continuous human feedback allows AI systems to learn and adapt over time, refining their algorithms based on real-world applications.
- Reduces bias: Human oversight can help identify and mitigate biases in AI systems, leading to fairer and more equitable outcomes.
- Increases trust: When users see that human input is valued in AI processes, it fosters trust in the technology, encouraging wider adoption.
Implementing HITL strategies can involve various methods, such as active learning, where human annotators label data to improve model training, or using human feedback loops to adjust model predictions. This approach is particularly beneficial in fields like healthcare, finance, and autonomous vehicles, where the stakes are high, and human oversight is crucial. At Rapid Innovation, we leverage HITL strategies to ensure that our AI solutions not only meet technical specifications but also resonate with end-users, ultimately driving greater ROI for our clients.
9.3. Quality Metrics and Evaluation
Quality metrics and evaluation are vital for assessing the performance and reliability of AI systems. Establishing clear metrics helps organizations understand how well their models are functioning and where improvements are needed.
- Accuracy: Measures the proportion of correct predictions made by the model. High accuracy indicates that the model is performing well.
- Precision and Recall: Precision assesses the accuracy of positive predictions, while recall measures the model's ability to identify all relevant instances. Balancing these metrics is crucial for applications like fraud detection.
- F1 Score: The harmonic mean of precision and recall, providing a single score that balances both metrics, especially useful in imbalanced datasets.
- AUC-ROC: The Area Under the Receiver Operating Characteristic curve evaluates the trade-off between true positive rates and false positive rates, helping to understand model performance across different thresholds.
- User satisfaction: In user-facing applications, measuring user satisfaction through surveys or feedback can provide qualitative insights into the model's effectiveness.
Regular evaluation against these metrics allows organizations to identify weaknesses in their models and make data-driven decisions for improvements. Continuous monitoring is essential to ensure that models remain effective as data and conditions change. Rapid Innovation employs a robust framework for quality metrics and evaluation, ensuring that our clients' AI systems are not only effective but also continuously optimized for performance and user satisfaction.
9.4. Continuous Improvement Frameworks
Continuous improvement frameworks are structured approaches that organizations use to enhance their processes, products, and services over time. In the context of AI and machine learning, these frameworks help ensure that models evolve and adapt to changing environments and user needs.
- Plan-Do-Check-Act (PDCA): This iterative process involves planning improvements, implementing changes, checking the results, and acting on what has been learned to refine the approach further.
- Agile methodologies: Agile frameworks promote flexibility and responsiveness, allowing teams to adapt quickly to feedback and changing requirements. Regular sprints and reviews facilitate continuous enhancement.
- Lean principles: Focusing on eliminating waste and optimizing processes, lean principles can be applied to AI development to streamline workflows and improve efficiency.
- Data-driven decision-making: Utilizing data analytics to inform decisions ensures that improvements are based on empirical evidence rather than assumptions.
- Stakeholder engagement: Involving stakeholders throughout the improvement process helps align AI systems with user needs and expectations, fostering a culture of collaboration and innovation.
By adopting continuous improvement frameworks, organizations can create a culture of ongoing learning and adaptation, ensuring that their AI systems remain relevant and effective in meeting user demands and industry standards. At Rapid Innovation, we guide our clients through these frameworks, ensuring that their AI initiatives are not only successful at launch but continue to deliver value over time.
9.5. Error Detection and Correction
Error detection and correction are critical components in data transmission and storage systems. These processes ensure the integrity and reliability of data, which is essential in various applications, from telecommunications to computer memory systems.
- Error Detection: This involves identifying errors in data. Common techniques include: Â
- Parity Bits: A simple method where an extra bit is added to a binary string to make the number of 1s either even or odd.
- Checksums: A value calculated from a data set that helps verify its integrity. If the checksum does not match upon retrieval, an error is detected.
- Cyclic Redundancy Check (CRC): A more complex method that uses polynomial division to detect changes to raw data.
- Error Correction: Once an error is detected, correction methods are employed to fix the data. Techniques include: Â
- Hamming Code: This method adds redundant bits to the data, allowing the detection and correction of single-bit errors.
- Reed-Solomon Codes: Widely used in CDs and QR codes, these codes can correct multiple errors in data blocks.
- Turbo Codes and LDPC Codes: Advanced error correction techniques that are used in modern communication systems, providing high performance in noisy environments.
- Importance: Error detection and correction are vital for: Â
- Ensuring data integrity in communication systems.
- Enhancing the reliability of data storage solutions.
- Supporting error-free data transmission in critical applications like banking and healthcare.
- Applications: These techniques are used in various fields, including: Â
- Telecommunications (e.g., mobile networks)
- Data storage (e.g., hard drives, SSDs)
- Networking (e.g., data packets in the internet)
At Rapid Innovation, we leverage these error detection and correction techniques to enhance the reliability of our AI-driven solutions. By ensuring data integrity, we help our clients achieve greater ROI through improved system performance and reduced downtime. Our expertise in computer vision software development further supports these efforts. For more insights on AI technologies, check out our article on key concepts and technologies in AI.
10. Future Trends and Innovations
The landscape of technology is constantly evolving, with future trends and innovations shaping how we interact with the digital world. Key areas of focus include advancements in artificial intelligence, machine learning, and data analytics.
- Increased Automation: Automation is set to revolutionize industries by: Â
- Reducing human error and increasing efficiency.
- Streamlining processes in manufacturing, logistics, and customer service.
- Enabling smart factories and autonomous vehicles.
- Enhanced Connectivity: The rise of 5G and beyond will lead to: Â
- Faster data transfer rates and lower latency.
- Improved Internet of Things (IoT) capabilities, connecting more devices seamlessly.
- Greater opportunities for remote work and telemedicine.
- Sustainability and Green Technology: Innovations will focus on: Â
- Developing energy-efficient technologies to combat climate change.
- Utilizing renewable energy sources in tech infrastructures.
- Promoting sustainable practices in manufacturing and product design.
10.1. Emerging AI Technologies
Artificial intelligence continues to evolve, with new technologies emerging that promise to transform various sectors. These innovations are characterized by their ability to learn, adapt, and perform tasks that typically require human intelligence.
- Natural Language Processing (NLP): Advances in NLP are enabling machines to understand and generate human language more effectively. Key developments include: Â
- Improved chatbots and virtual assistants that provide more accurate responses.
- Enhanced sentiment analysis tools for better customer insights.
- Language translation services that are more context-aware.
- Computer Vision: This technology allows machines to interpret and understand visual information. Innovations include: Â
- Facial recognition systems used in security and marketing.
- Autonomous vehicles that rely on computer vision for navigation.
- Medical imaging technologies that assist in diagnostics.
- Generative AI: This emerging field focuses on creating new content, such as images, music, and text. Notable applications include: Â
- AI-generated art and design, pushing the boundaries of creativity.
- Content creation tools that assist writers and marketers.
- Deepfake technology, which raises ethical considerations in media.
- Reinforcement Learning: A subset of machine learning where algorithms learn by interacting with their environment. This technology is being applied in: Â
- Robotics for improved task performance.
- Game development, creating more intelligent and adaptive opponents.
- Financial modeling to optimize trading strategies.
- Ethical AI: As AI technologies advance, there is a growing emphasis on ethical considerations, including: Â
- Ensuring fairness and transparency in AI algorithms.
- Addressing biases in data that can lead to discriminatory outcomes.
- Developing regulations and guidelines to govern AI use responsibly.
At Rapid Innovation, we are committed to staying at the forefront of these emerging technologies, helping our clients harness their potential to drive efficiency and achieve their business goals effectively.
10.2. Multimodal Content Generation
Multimodal content generation refers to the creation of content that combines various forms of media, such as text, images, audio, and video, to enhance user engagement and convey information more effectively. This approach caters to diverse audience preferences and learning styles, making it a powerful tool in digital marketing and content strategy.
- Engages multiple senses: By integrating different media types, multimodal content can capture attention more effectively than single-format content.
- Increases retention: Studies show that people retain information better when it is presented in various formats.
- Enhances storytelling: Combining visuals with narrative elements can create a more compelling story, making the content more relatable and memorable.
- Improves accessibility: Multimodal content can cater to individuals with different abilities, ensuring that everyone can access and understand the information.
- Boosts SEO: Search engines favor diverse content types, which can improve visibility and ranking in search results.
At Rapid Innovation, we leverage multimodal content generation to help our clients create engaging and effective marketing strategies. By utilizing AI-driven tools, we can analyze audience preferences and tailor multimodal content that resonates with users, ultimately leading to higher engagement rates and improved overall performance. For more insights on this topic, check out our post on app development with stable diffusion model.
10.3. Hyper-Personalization
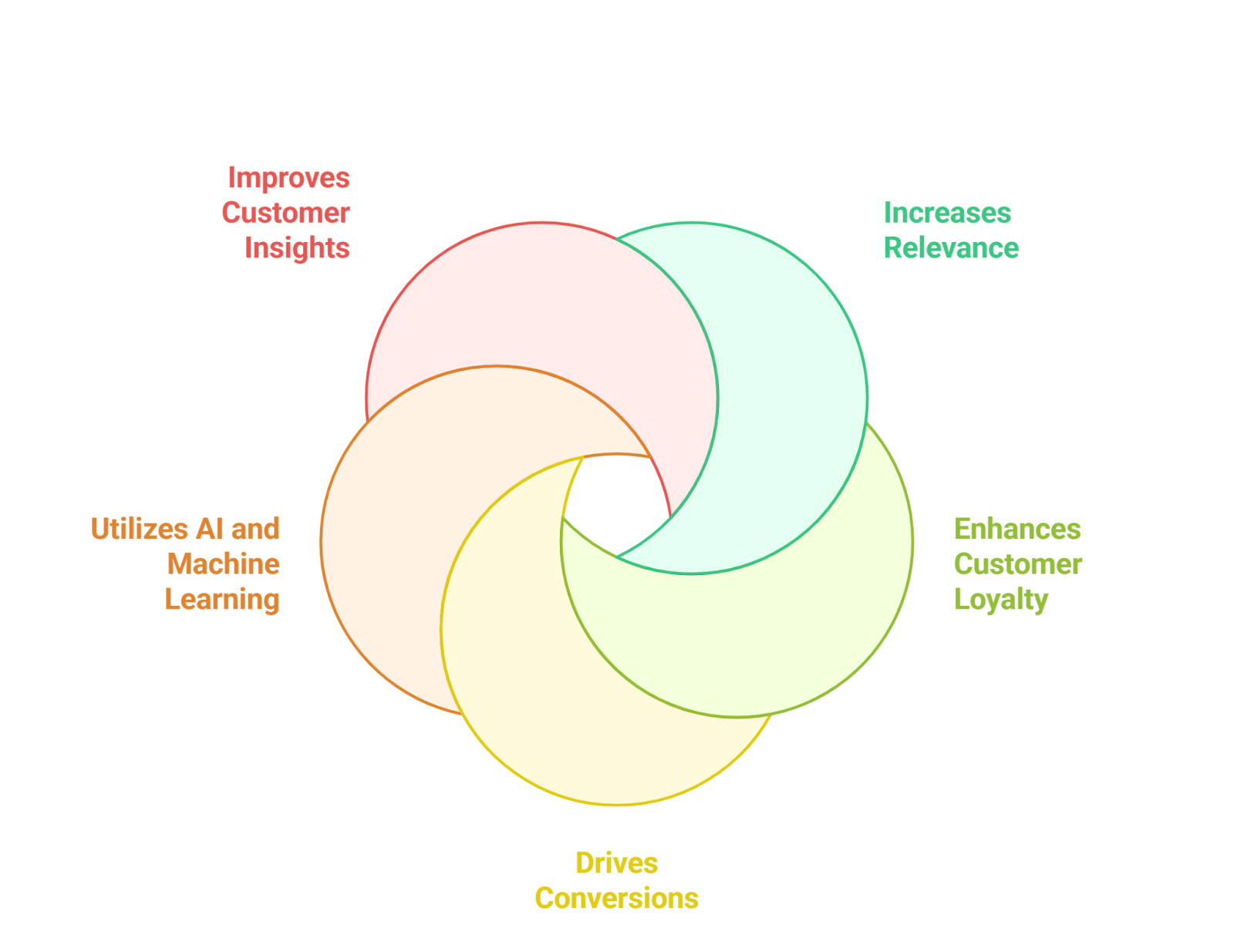
‍
Hyper-personalization takes personalization to the next level by using advanced data analytics and artificial intelligence to deliver highly tailored content to individual users. This approach goes beyond basic demographic information, leveraging behavioral data, preferences, and real-time interactions to create a unique experience for each user.
- Increases relevance: By analyzing user behavior, brands can deliver content that resonates with individual interests and needs.
- Enhances customer loyalty: Personalized experiences foster a stronger emotional connection, leading to increased customer retention and loyalty.
- Drives conversions: Hyper-personalized content can significantly improve conversion rates, as users are more likely to engage with content that speaks directly to them.
- Utilizes AI and machine learning: These technologies enable brands to analyze vast amounts of data quickly, allowing for real-time adjustments to content strategies.
- Improves customer insights: Hyper-personalization provides valuable data on user preferences, helping brands refine their marketing strategies and product offerings.
At Rapid Innovation, we implement hyper-personalization strategies that empower our clients to connect with their audience on a deeper level. By harnessing AI capabilities, we ensure that the content delivered is not only relevant but also timely, leading to a more engaged audience and increased revenue.
10.4. Predictive Content Creation
Predictive content creation involves using data analytics and machine learning algorithms to anticipate user needs and preferences, allowing brands to create content that is likely to resonate with their audience before it is even requested. This proactive approach can significantly enhance content marketing strategies.
- Anticipates trends: By analyzing historical data and user behavior, brands can identify emerging trends and create content that aligns with future interests.
- Saves time and resources: Predictive analytics can streamline the content creation process, allowing marketers to focus on high-impact strategies rather than reactive content.
- Increases engagement: Content that aligns with user expectations is more likely to be shared and engaged with, leading to higher visibility and reach.
- Enhances user experience: By delivering relevant content at the right time, brands can create a seamless experience that keeps users coming back for more.
- Supports data-driven decisions: Predictive content creation relies on data insights, enabling brands to make informed decisions about their content strategies.
By leveraging predictive content creation, Rapid Innovation helps businesses stay ahead of the curve, ensuring that their content remains relevant and engaging in an ever-evolving digital landscape. Our expertise in AI-driven analytics allows us to provide actionable insights that drive effective content strategies, ultimately leading to greater ROI for our clients.
10.5. Quantum Computing Implications
Quantum computing represents a significant leap forward in computational power, leveraging the principles of quantum mechanics to process information in ways that classical computers cannot. The implications of this technology are vast and multifaceted, affecting various sectors and industries.
- Enhanced Problem Solving: Quantum computers can solve complex problems much faster than traditional computers. This capability is particularly beneficial in fields like cryptography, materials science, and drug discovery. Rapid Innovation can assist clients in harnessing this power to tackle their most challenging problems, leading to faster and more effective solutions, including cloud based quantum computing and quantum computing cloud services.
- Cryptography Revolution: Quantum computing poses a threat to current encryption methods. Algorithms like Shor's algorithm can factor large numbers exponentially faster than classical algorithms, potentially compromising data security. Organizations must prepare for a shift in cryptographic standards to quantum-resistant algorithms. Rapid Innovation offers consulting services to help clients transition to secure quantum-resistant systems, ensuring their data remains protected, especially in the context of quantum cloud computing solutions.
- Optimization and Simulation: Industries such as logistics, finance, and manufacturing can benefit from quantum computing's ability to optimize complex systems and simulate molecular interactions, leading to more efficient operations and innovative products. Rapid Innovation can guide clients in implementing quantum solutions that enhance operational efficiency and drive product innovation, including cloud based quantum machine learning solutions.
- Economic Impact: The rise of quantum computing could lead to new markets and job opportunities, but it may also disrupt existing industries. Companies that adapt quickly to this technology will likely gain a competitive edge. Rapid Innovation helps clients navigate this landscape, identifying opportunities for growth and innovation through quantum technologies, such as quantum orchestration platforms and cloud based quantum services.
- Ethical Considerations: As quantum computing advances, ethical questions arise regarding data privacy, security, and the potential for misuse. Stakeholders must engage in discussions about responsible use and governance of quantum technologies. Rapid Innovation emphasizes ethical practices in AI and quantum computing, ensuring that clients adopt responsible strategies, particularly in the realm of quantum computing solutions.
- Research and Development: Investment in quantum research is crucial. Governments and private sectors are increasingly funding quantum initiatives to stay ahead in this emerging field. Rapid Innovation supports clients in securing funding and developing research initiatives that leverage quantum computing for competitive advantage, including partnerships with organizations like Honeywell and Cambridge Quantum Computing. Additionally, organizations must consider the implications of quantum-resistant blockchain technology as they prepare for the future of cybersecurity in 2024.
11. Practical Implementation Guide
Implementing quantum computing solutions requires a structured approach to ensure that organizations can effectively leverage this technology. A practical implementation guide can help organizations navigate the complexities of quantum computing.
- Define Objectives: Clearly outline the goals for adopting quantum computing. This could include improving computational efficiency, enhancing security, or driving innovation in product development. Rapid Innovation assists clients in setting clear, measurable objectives aligned with their business goals, including the adoption of free cloud based quantum computing solutions.
- Assess Current Infrastructure: Evaluate existing IT infrastructure to determine compatibility with quantum technologies. Identify gaps that need to be addressed to support quantum computing. Rapid Innovation provides comprehensive assessments to help clients understand their readiness for quantum integration, including cloud based quantum computer solutions.
- Develop a Roadmap: Create a strategic roadmap that outlines the steps for implementation. This should include timelines, resource allocation, and key milestones. Rapid Innovation collaborates with clients to develop tailored roadmaps that ensure successful implementation, incorporating quantum cloud solutions.
- Invest in Training: Equip your team with the necessary skills to work with quantum technologies. This may involve training programs, workshops, or partnerships with educational institutions. Rapid Innovation offers training solutions to empower teams with the knowledge needed to leverage quantum computing effectively, including cloud based quantum machine learning.
- Collaborate with Experts: Engage with quantum computing experts and organizations. Collaborations can provide valuable insights and accelerate the learning curve. Rapid Innovation connects clients with industry experts to foster collaboration and knowledge sharing, including those involved in open source quantum computer solutions.
- Pilot Projects: Start with small-scale pilot projects to test quantum solutions in real-world scenarios. This approach allows for experimentation and learning without significant risk. Rapid Innovation helps clients design and execute pilot projects that validate quantum solutions, including free quantum computing solutions.
- Monitor and Evaluate: Continuously monitor the performance of quantum solutions and evaluate their impact on organizational objectives. Use feedback to refine strategies and improve outcomes. Rapid Innovation provides ongoing support to ensure clients achieve their desired results.
11.1. Organizational Readiness Assessment
Before diving into quantum computing, organizations must conduct a readiness assessment to gauge their preparedness for this transformative technology. This assessment helps identify strengths, weaknesses, and areas for improvement.
- Current Technology Landscape: Analyze the existing technology stack to determine how well it aligns with quantum computing requirements. Consider hardware, software, and data management systems. Rapid Innovation assists clients in evaluating their technology landscape to identify alignment with quantum capabilities, including cloud based quantum computing services.
- Skill Set Evaluation: Assess the current skill levels of employees in relation to quantum computing. Identify gaps in knowledge and expertise that need to be addressed through training or hiring. Rapid Innovation offers skill assessments to help clients understand their workforce's readiness for quantum machine learning solutions.
- Cultural Readiness: Evaluate the organizational culture to determine if it supports innovation and change. A culture that embraces experimentation and learning is crucial for successful implementation. Rapid Innovation works with clients to foster a culture of innovation that supports quantum initiatives.
- Financial Resources: Review the budget and financial resources available for investing in quantum technologies. Determine if additional funding is needed for research, development, and training. Rapid Innovation provides financial analysis to help clients allocate resources effectively, including for free open source quantum computer solutions.
- Risk Management: Identify potential risks associated with adopting quantum computing. Develop strategies to mitigate these risks and ensure a smooth transition. Rapid Innovation helps clients create robust risk management frameworks tailored to quantum computing.
- Stakeholder Engagement: Involve key stakeholders in the assessment process. Their insights and support can facilitate a more comprehensive understanding of organizational readiness. Rapid Innovation facilitates stakeholder engagement to ensure alignment and support for quantum initiatives.
- Future Vision: Consider the long-term vision for quantum computing within the organization. Align the assessment with strategic goals to ensure that quantum initiatives contribute to overall business objectives. Rapid Innovation collaborates with clients to develop a future vision that integrates quantum computing into their strategic roadmap.
By conducting a thorough organizational readiness assessment, companies can position themselves for success in the quantum computing landscape with the guidance and expertise of Rapid Innovation.
11.2. Pilot Program Design
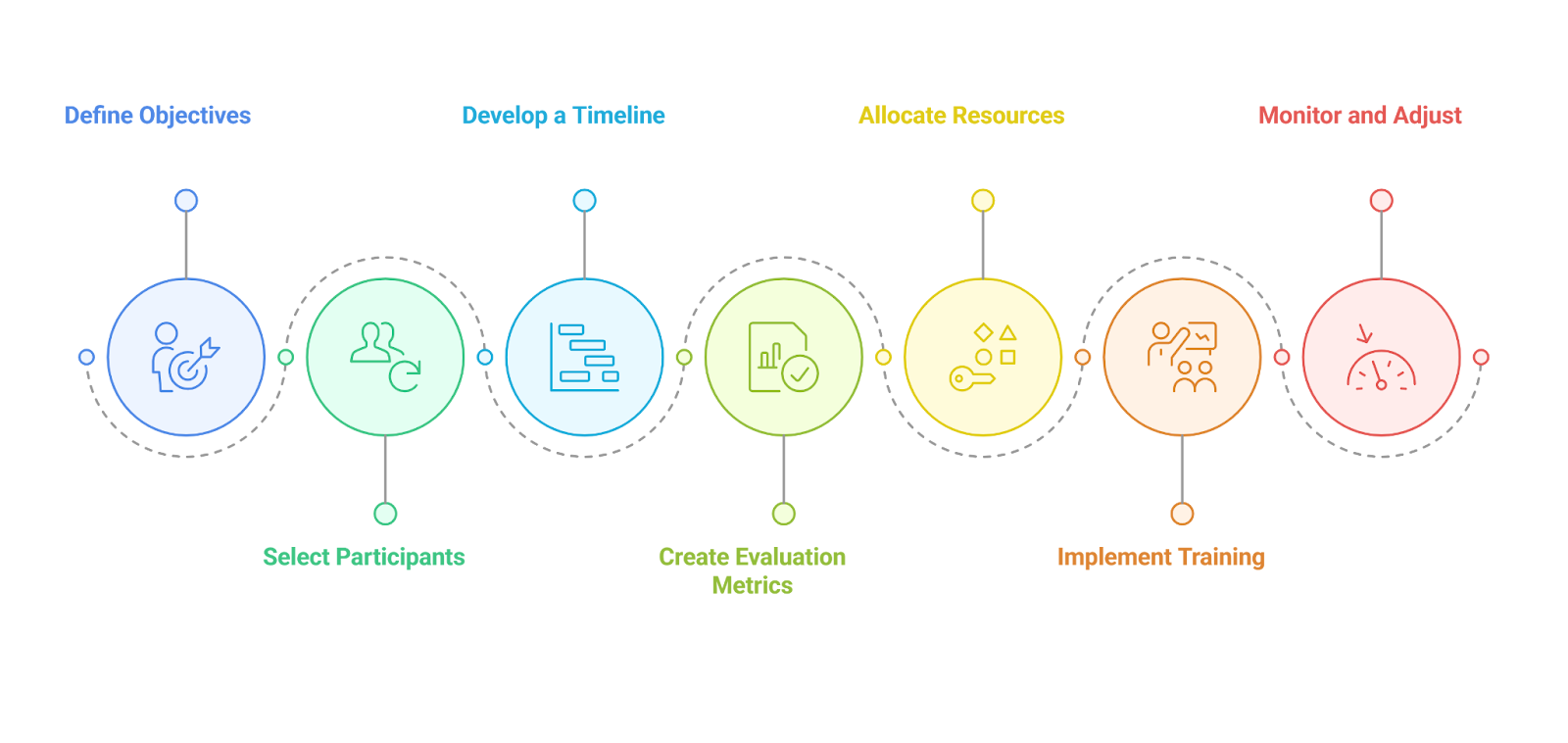
‍
A pilot program is a small-scale implementation of a project or initiative to test its feasibility, time, cost, and adverse events. Designing an effective pilot program involves several key steps:
- Define Objectives: Clearly outline what the pilot aims to achieve. This could include testing new processes, technologies, or methodologies that leverage AI capabilities to enhance operational efficiency.
- Select Participants: Choose a representative sample of users or stakeholders who will participate in the pilot program design. This helps in gathering diverse feedback, ensuring that the AI solutions are tailored to meet the needs of various user groups.
- Develop a Timeline: Establish a clear timeline for the pilot, including start and end dates, milestones, and evaluation points. This structured approach allows for timely adjustments based on real-time data and insights.
- Create Evaluation Metrics: Determine how success will be measured. This could include quantitative metrics (e.g., performance data) and qualitative feedback (e.g., user satisfaction), enabling a comprehensive assessment of the AI implementation's impact.
- Allocate Resources: Ensure that the necessary resources, including budget, personnel, and technology, are available for the pilot. Rapid Innovation can assist in identifying and deploying the right AI tools and technologies to maximize resource efficiency, including fine-tuning language models and understanding the potential of business AI engineering.
- Implement Training: Provide training for participants to ensure they understand the new processes or technologies being tested. This is crucial for fostering user adoption and maximizing the benefits of AI solutions.
- Monitor and Adjust: During the pilot program design, continuously monitor progress and be prepared to make adjustments based on feedback and observations. This iterative process is essential for refining AI applications and ensuring alignment with business goals.
A well-designed pilot program can provide valuable insights and help mitigate risks before a full-scale rollout, ultimately leading to greater ROI for your organization.
11.3. Skill Development and Training
Skill development and training are crucial for ensuring that employees are equipped to meet the demands of their roles and adapt to changes within the organization. Effective training programs should include:
- Needs Assessment: Identify the skills gaps within the organization through surveys, interviews, or performance reviews. This helps in pinpointing areas where AI training can enhance employee capabilities.
- Tailored Training Programs: Develop training programs that are specific to the needs of different teams or roles. This can include technical skills, soft skills, and leadership training, particularly in the context of AI technologies.
- Diverse Learning Methods: Utilize various training methods such as online courses, workshops, on-the-job training, and mentorship programs. Incorporating AI-driven learning platforms can further enhance the training experience.
- Continuous Learning: Encourage a culture of continuous learning by providing ongoing training opportunities and resources. This is vital in the rapidly evolving field of AI, where staying updated is key to maintaining a competitive edge.
- Evaluation and Feedback: Regularly assess the effectiveness of training programs through feedback and performance metrics to ensure they meet organizational goals.
- Certification and Recognition: Offer certifications or recognition for completed training programs to motivate employees and enhance their professional development.
Investing in skill development and training not only improves employee performance but also boosts morale and retention rates, particularly as organizations integrate AI into their operations.
11.4. Change Management
Change management is the structured approach to transitioning individuals, teams, and organizations from a current state to a desired future state. Effective change management involves several critical components:
- Clear Vision and Objectives: Establish a clear vision for the change initiative and communicate the objectives to all stakeholders. This is especially important when implementing AI solutions, as clarity can drive alignment and enthusiasm.
- Stakeholder Engagement: Involve key stakeholders early in the process to gain their support and address any concerns they may have. Engaging stakeholders in AI initiatives can foster a sense of ownership and collaboration.
- Communication Plan: Develop a comprehensive communication plan that outlines how information will be shared throughout the change process. This should include regular updates, feedback mechanisms, and channels for questions and concerns.
- Training and Support: Provide training and resources to help employees adapt to the changes. This can include workshops, FAQs, and one-on-one support, particularly focused on new AI tools and processes.
- Monitor Progress: Continuously monitor the implementation of the change initiative and gather feedback to identify areas for improvement. Utilizing AI analytics can enhance this monitoring process.
- Celebrate Successes: Recognize and celebrate milestones and successes throughout the change process to maintain momentum and morale.
Effective change management can lead to smoother transitions, reduced resistance, and improved overall outcomes for the organization, particularly as it embraces innovative AI solutions.
11.5. Continuous Learning Approach
The Continuous Learning Approach is a vital strategy in both personal and professional development, particularly in the realm of AI and technology. It emphasizes the importance of ongoing education and skill enhancement throughout an individual's life. This approach is particularly relevant in today's fast-paced world, where technological advancements and market dynamics are constantly evolving.
- Encourages Lifelong Learning
Promotes the idea that learning does not stop after formal education, fostering curiosity and a desire to acquire new knowledge and skills. It supports the notion that every experience can be a learning opportunity, especially in the rapidly changing landscape of AI, including continual ai. - Adapts to Changing Environments
Helps individuals stay relevant in their fields by adapting to new trends and technologies. This approach encourages flexibility and resilience in the face of change, preparing individuals for unexpected challenges and opportunities, such as the integration of AI solutions in various industries. - Enhances Career Development
Continuous learning can lead to better job performance and career advancement. Employees who engage in ongoing education are often more competitive in the job market, particularly in AI-related roles. Organizations that promote continuous learning tend to have higher employee satisfaction and retention rates, ultimately leading to greater ROI. - Utilizes Various Learning Methods
Incorporates formal education, online courses, workshops, and seminars. It encourages self-directed learning through reading, podcasts, and webinars, and supports collaborative learning through mentorship and peer-to-peer interactions, which are essential for mastering AI technologies, including continuous learning in ai. - Fosters a Growth Mindset
Encourages individuals to view challenges as opportunities for growth, promoting resilience and the ability to learn from failures. It instills a belief that abilities and intelligence can be developed through dedication and hard work, which is crucial in the ever-evolving field of AI. - Leverages Technology
Utilizes online platforms and resources for easy access to learning materials. It encourages the use of mobile apps and e-learning tools for on-the-go education and supports virtual classrooms and remote learning opportunities, enabling professionals to stay updated on the latest AI advancements. - Supports Organizational Learning
Organizations that embrace a continuous learning culture can innovate more effectively. This approach encourages knowledge sharing and collaboration among employees, helping organizations adapt to market changes and improve overall performance, particularly in implementing AI solutions that drive efficiency. - Measures Learning Outcomes
Establishes metrics to evaluate the effectiveness of learning initiatives. It encourages feedback and reflection to improve future learning experiences and supports the alignment of learning goals with organizational objectives, ensuring that AI training programs yield measurable results. - Builds a Learning Community
Fosters a sense of belonging and support among learners. It encourages networking and relationship-building through shared learning experiences and promotes a culture of knowledge sharing and collaboration, which is vital for fostering innovation in AI. - Increases Employee Engagement
Employees who engage in continuous learning are often more motivated and committed. Learning opportunities can enhance job satisfaction and reduce turnover, and organizations that invest in employee development tend to see improved morale and productivity, particularly in AI-driven environments. - Aligns with Personal Goals
Encourages individuals to set personal learning objectives that align with their career aspirations. It supports the pursuit of passions and interests outside of work and helps individuals create a balanced approach to personal and professional growth, especially in the context of AI and technology.
In conclusion, the Continuous Learning Approach is essential for adapting to the ever-changing landscape of work and life, particularly in the field of AI. By fostering a culture of lifelong learning, individuals and organizations can thrive in an increasingly complex world, ultimately achieving greater ROI through enhanced skills and innovative solutions. For those looking to enhance their capabilities in AI, partnering with an experienced AI software development can be a significant step forward.