Table Of Contents
Category
Artificial Intelligence
1. Introduction
In the realm of technology and business, Artificial Intelligence (AI) agents, or AI Agentic Systems, are becoming indispensable tools that streamline operations and enhance decision-making across various sectors. These agents are defined as autonomous systems powered by AI that can automate processes, handle data intelligently, and interact with users and other systems in ways that mimic human actions but with much greater efficiency and far less error to boot. Their integration into corporate environments is transforming mundane and complex tasks alike, allowing businesses to optimize their workflows and focus human talent on strategic thinking and creativity. And their number of uses are expected to only grow further and further with time; research done by Markets and Markets reports that the market value of the AI agent industry is expected to grow dramatically over the next 5 years, from $5 billion this year to over $50 billion next year. Additionally, Capgemini has reported that 10% of large enterprises are already making use of AI agent development, and more than 50% plan to utilize it in the next year, with a total of 82% using it within the next three years.
In this blog, we will be diving deep into AI Agents, as well as what they are, what they represent, what the different AI agents types are, their benefits, their limitations, and lastly, some of the most prominent real-world AI agents examples and applications, providing a clear view of how they contribute to business innovation and effectiveness. By harnessing the power and potential of this technology, AI agent development companies are not only boosting productivity but are also enhancing their adaptability in a rapidly changing market landscape. Let's find out why!
2. Understanding AI Agents
2.1 What Are AI Agents?
AI agents are advanced autonomous systems that function as "digital virtual assistants, but on steroids". They harness artificial intelligence (AI) to perform tasks independently and autonomously. They are intelligent AI entities that can perceive their environment, analyze data, and respond to queries with the help of their independent thinking and decision-making capabilities to achieve specific outcomes.
Utilizing AI, machine learning, and AI agent frameworks such as Microsoft Autogen or CrewAI, AI agents, or AI agentic systems, are able to process information, inputs, and business goals, continually learning and adapting to provide more accurate and informed results.
Unlike traditional AI automation tools that follow fixed instructions, AI agents offer dynamic capabilities, such as the following:
- Real-Time Data Collection: They gather and analyze data continuously to improve their performance.
- Adaptive Behavior: They adjust their actions based on new information and changing conditions.
- Learning and Adaptation: They evolve by learning from their environment and interactions.
- Responsive Action: They can take immediate actions based on real-time inputs.
- Natural Language Processing: They understand and respond in human language, making interactions more intuitive.
These features position AI agents as sophisticated tools that enhance productivity and precision, making them valuable assets in various business operations and intellectual tasks.
2.2 Architecture of AI Agents
AI agents may vary in function and setting, but they all share foundational components that enable them to perform effectively.
2.2.1. Architecture
The architecture serves as the foundation from which an agent operates. It can be a physical framework, a software system, or a blend of both. For example, a robotic AI agent architecture may include components like sensors, actuators, motors, and robotic limbs, while a software-based AI agent may rely on elements such as APIs, text prompts, and databases to execute tasks autonomously.
2.2.2. Best Practices in Designing Scalable AI Agent Architecture
When designing AI agent architecture for scalability, there are several best practices to consider. First, modularize components so that each function (e.g., data processing, decision-making) is separate and can be updated or scaled independently. Using cloud-based infrastructure and APIs allows for flexible scaling and integration with other services. Additionally, optimizing data storage and retrieval, as well as employing distributed processing techniques, can help the agent handle larger datasets and perform real-time tasks efficiently. Lastly, ensure security and redundancy in the architecture to maintain performance and protect against potential failures.
2.2.3 Agent Function
This component defines how the agent processes collected data to take actions that fulfill its purpose. When developing the agent function, factors like data types, AI capabilities, feedback mechanisms, knowledge bases, and other necessary technologies are considered.
2.2.4. Agent Program
The agent program is the practical application of the agent function. It includes creating, training, and deploying the AI agent within its architecture. The program integrates the agent's business logic, technical needs, and performance standards to ensure operational success.
2.3 Advanced Techniques in AI Agent Programming
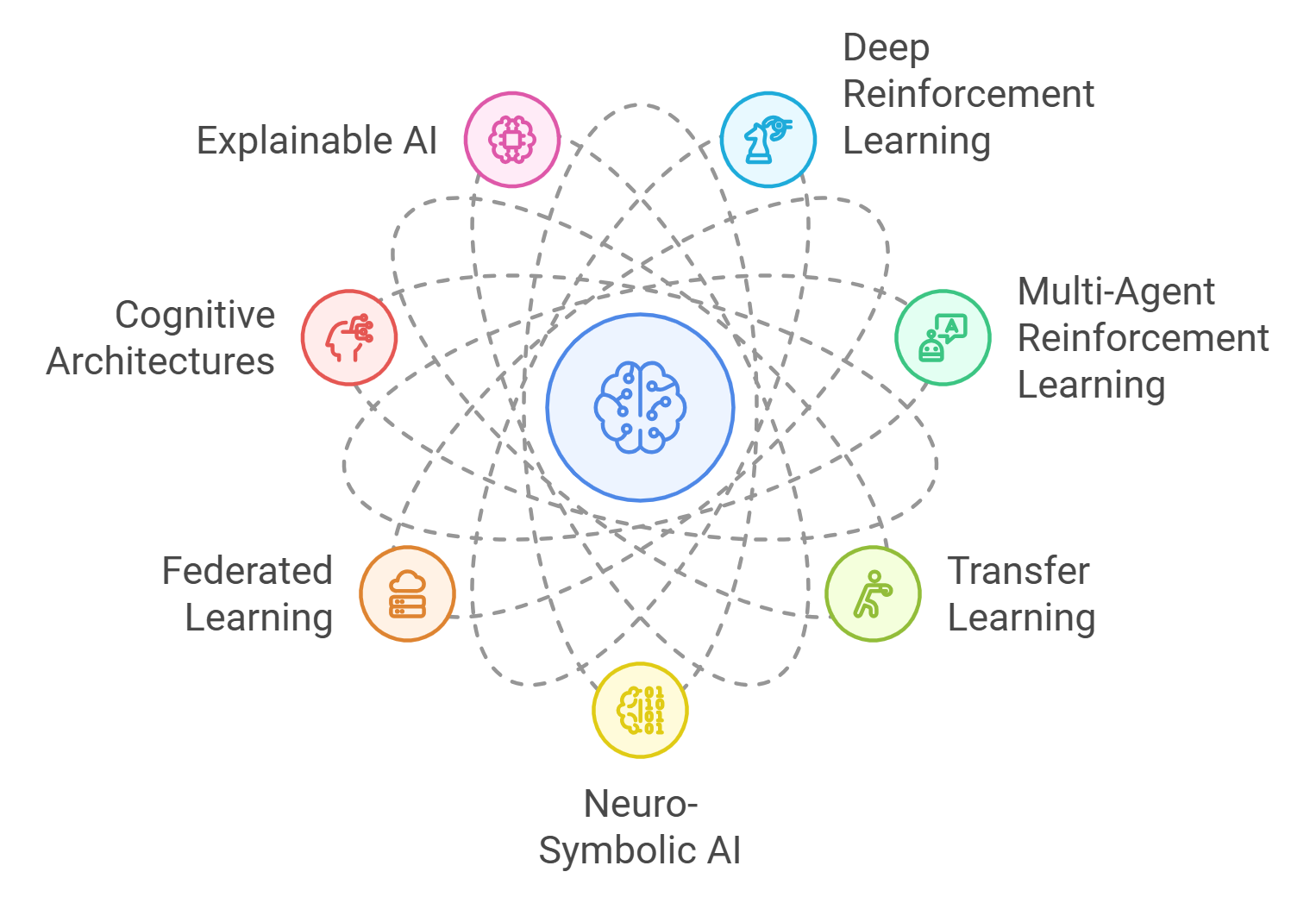
Advanced techniques in AI agent programming and AI agent development leverage cutting-edge methodologies to enhance the capabilities and performance of AI agents. These techniques include:
- Deep Reinforcement Learning (DRL): Combines deep learning with reinforcement learning to allow agents to learn optimal policies through trial and error. This technique is particularly useful in complex environments where the agent must make a series of decisions to achieve a specific goal, such as in robotics or game playing.
- Multi-Agent Reinforcement Learning (MARL): Focuses on scenarios involving multiple agents that must cooperate or compete to achieve their objectives. MARL techniques help agents learn how to interact and strategize within a group, which is critical for applications like AI-powered autonomous vehicle fleets or smart grid management.
- Transfer Learning: Enables AI agents to apply knowledge gained in one context to new, yet related tasks, reducing the need for extensive retraining. This technique is effective in accelerating the learning process, especially in environments where data is limited or expensive to obtain.
- Neuro-Symbolic AI: Combines neural networks with symbolic reasoning to create agents that can handle both unstructured data (like images and text) and structured logic (like rules and hierarchies). This approach enhances an agent's ability to understand complex, abstract concepts and improve decision-making in uncertain environments.
- Federated Learning: Allows multiple agents to collaboratively learn a shared model while keeping data decentralized. This is particularly important in domains like healthcare, where privacy and data security are crucial. Federated learning enables AI agents to improve collectively without compromising individual data privacy.
- Cognitive Architectures: These are frameworks that simulate human cognitive processes, allowing AI agents to reason, learn, and plan more like humans. Cognitive architectures enhance the flexibility and adaptability of AI agents, making them more effective in dynamic and unpredictable environments.
- Explainable AI (XAI): Techniques in XAI focus on making AI agent decisions transparent and understandable to humans. This is increasingly important in regulated industries like finance and healthcare, where accountability and trust are critical. XAI techniques provide insights into an agent's decision-making process, enhancing user trust and facilitating regulatory compliance.
By integrating these advanced techniques in their AI agent development services, Rapid Innovation's developer team creates sophisticated and intelligent AI agents capable of handling complex tasks, adapting to dynamic environments, and providing more reliable and effective solutions across various industries.
3.Types of AI Agents
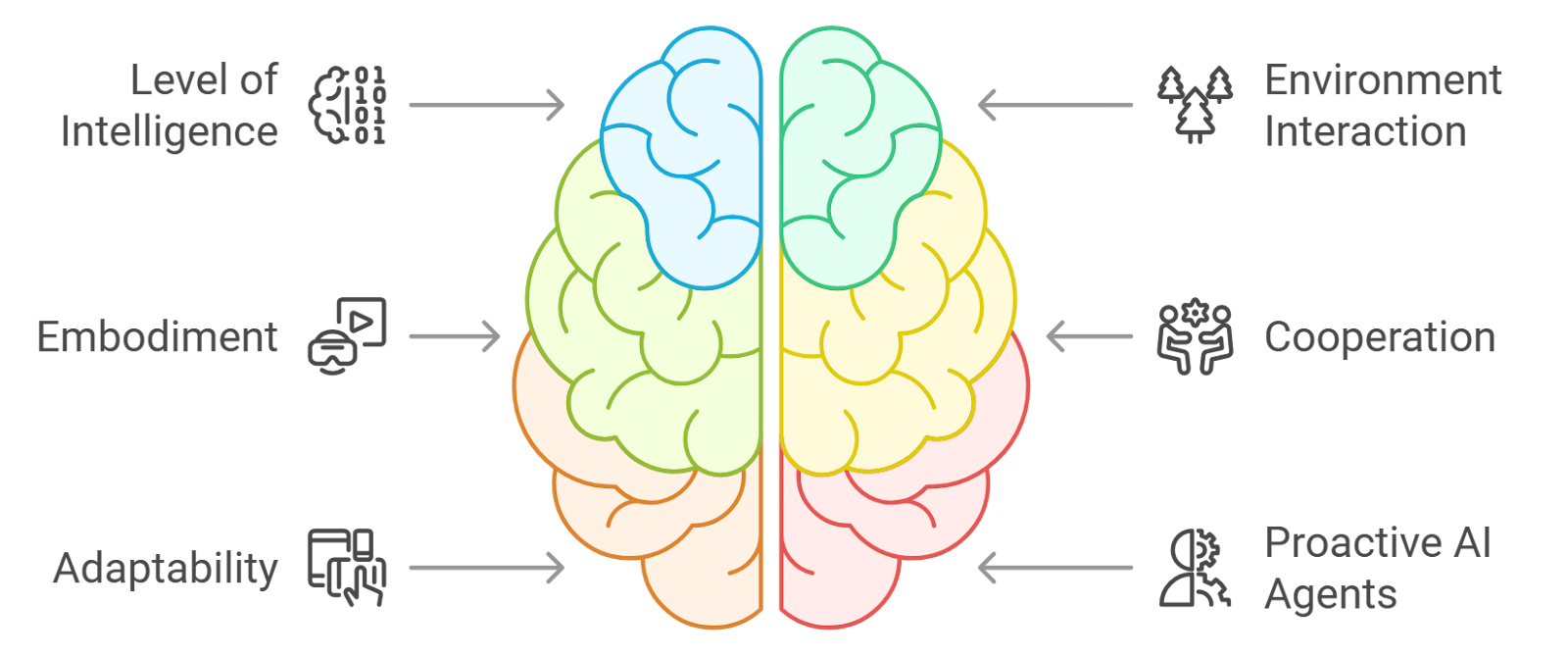
AI agents types can be divided into different categories based on a wide range of parameters:
3.1. Based on Level of Intelligence
3.1.1 Simple Reflex Agents
- Description: These agents act based on the current perception of their environment, ignoring the history of perceptions.
- Properties:
- Use condition-action rules
- Do not maintain internal state
- Suitable for fully observable environments
- Examples:
- AI-powered thermostat adjusting temperature based on current reading
- Basic AI chatbots that respond to keywords without context
3.1.2 Model-Based Reflex Agents
- Description: These agents maintain an internal state to track aspects of the world not visible in the current perception.
- Properties:
- Use condition-action rules
- Maintain internal models of the world
- Can handle partially observable environments
- Examples:
- AI-powered autonomous vacuum cleaners that map out a room
- AI-driven traffic light control systems that consider traffic flow patterns
3.1.3 Goal-Based Agents
- Description: These agents work towards specific goals, considering future actions and their outcomes.
- Properties:
- Use planning and search algorithms
- Can handle complex, multi-step problems
- Evaluate different action sequences
- Examples:
- Chess-playing AI that plans several moves ahead
- AI-powered logistics optimization systems for route planning
3.1.4 Utility-Based Agents
- Description: These agents make decisions based on a utility function that measures the desirability of different states.
- Properties:
- Balance multiple, potentially conflicting goals
- Can handle uncertainty through probabilistic reasoning
- Optimize for the best outcome rather than just achieving a goal
- Examples:
- AI trading systems that balance risk and reward
- Recommendation engines that personalize content based on user preferences with the help of AI
3.1.5 Learning Agents
- Description: These agents improve their performance over time through experience.
- Properties:
- Adapt to new situations
- Improve decision-making through feedback
- Can discover new knowledge and strategies
- Examples:
- Deep learning models for image recognition that improve with more data
- Reinforcement learning agents for game playing (e.g., AlphaGo)
3.2. Based on Environment Interaction
3.2.1 Passive Agents
- Description: These agents observe their environment but do not affect it.
- Properties:
- Gather information without taking actions
- Often used for monitoring and analysis
- Examples:
- Weather prediction models
- AI-powered market sentiment analysis tools
3.2.2 Active Agents
- Description: These agents interact with and modify their environment.
- Properties:
- Take actions that affect the environment
- Often involved in decision-making and control systems
- Examples:
- Industrial robots in manufacturing
- AI-powered virtual assistants (e.g., Siri, Alexa)
3.3. Based on Embodiment
3.3.1 Software Agents
- Description: These agents exist purely in digital environments.
- Properties:
- Can operate at high speeds
- Not limited by physical constraints
- Often used for data processing and analysis
- Examples:
- AI web crawlers for search engines
- Algorithmic AI trading bots in financial markets
3.3.2 Robotic Agents
- Description: These agents have a physical presence and interact with the real world.
- Properties:
- Combine AI with mechanical engineering
- Must deal with physical limitations and uncertainties
- Often require real-time processing and decision-making
- Examples:
- AI-driven (pun intended) autonomous vehicles
- Humanoid AI robots for research or customer service
3.4. Based on Cooperation
3.4.1 Single Agents
- Description: These agents operate independently to achieve their goals.
- Properties:
- Self-contained decision-making processes
- Do not require coordination with other agents
- Examples:
- Personal AI assistants on smartphones
- AI automated customer service chatbots
3.4.2 Multi-Agent Systems
- Description: These involve multiple agents working together or competing to achieve goals.
- Properties:
- Require coordination and communication protocols
- Can solve complex, distributed problems
- Often model real-world scenarios with multiple actors
- Examples:
- Swarm robotics for search and rescue operations
- Multi-agent AI simulations for economic modeling
3.5. Based on Adaptability
3.5.1 Static Agents
- Description: These agents have fixed capabilities and decision-making processes.
- Properties:
- Consistent and predictable behavior
- Well-suited for stable, well-defined environments
- Examples:
- Rule-based expert systems in AI-based medical diagnosis
- Fixed virtual AI opponents in video games
3.5.2 Adaptive Agents
- Description: These agents can modify their behavior and capabilities over time.
- Properties:
- Learn from experience and feedback
- Can handle changing environments and requirements
- Examples:
- Online AI recommendation systems that learn user preferences
- Adaptive control systems in industrial processes
3.6 Proactive AI Agents
Proactive AI agents are designed to anticipate needs and take actions autonomously without waiting for external commands. Unlike reactive agents, which respond only when prompted, proactive agents assess patterns, forecast outcomes, and initiate actions to meet objectives or improve processes. This proactive behavior makes them valuable in dynamic environments, such as personalized recommendations, predictive maintenance, and autonomous customer support.
4. Benefits of AI Agents
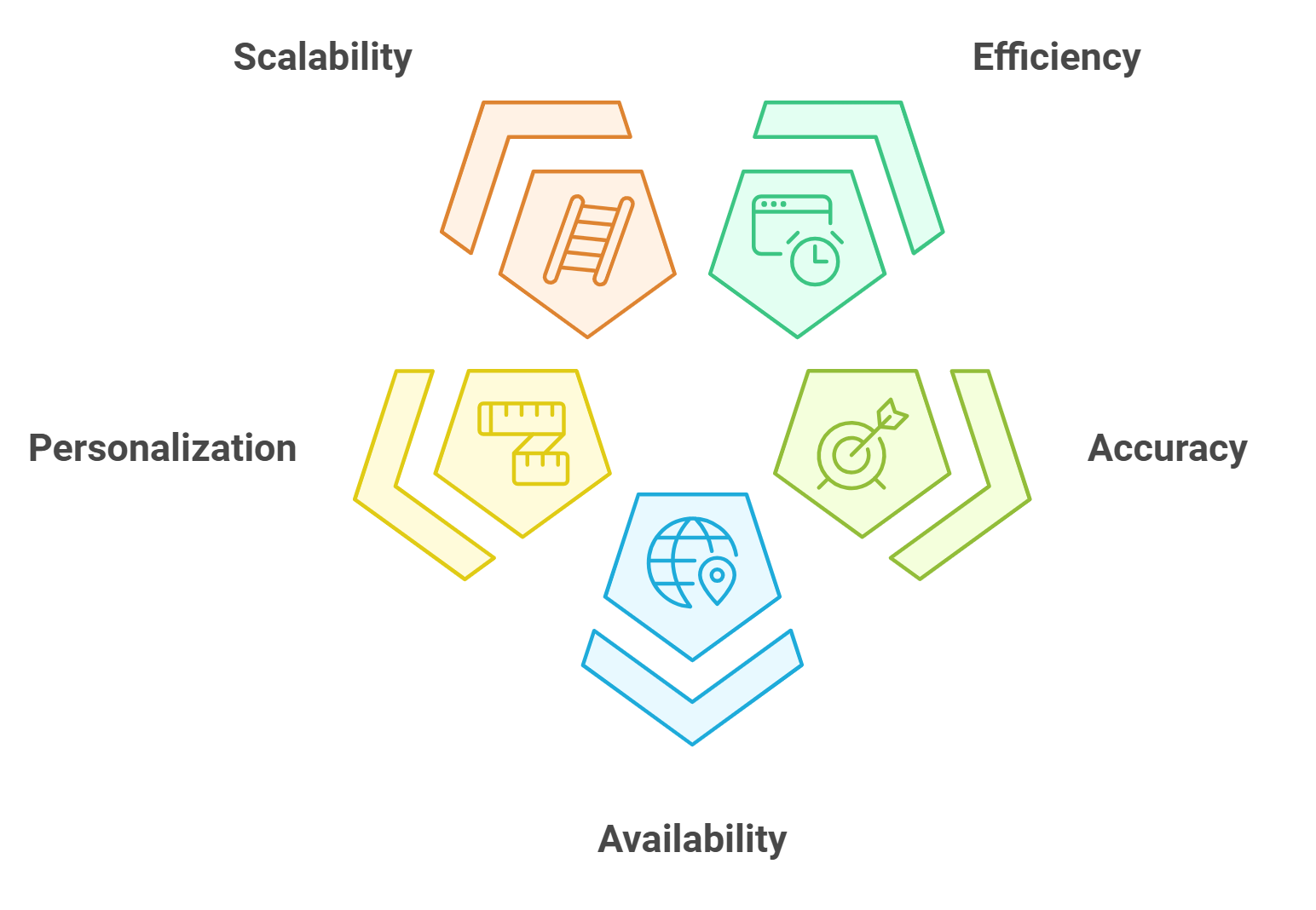
Incorporating AI agents into business processes offers several advantages:
- Efficiency: They automate and accelerate tasks, reducing the workload on human employees and enabling them to focus on more strategic activities. This automation not only speeds up processes but also ensures consistency across operations, leading to more predictable outcomes and streamlined workflows. As per a report from Forbes, more than 80% of employees in organizations utilizing AI agent development believe that it helps enhance their overall work productivity.
- Accuracy: AI agents minimize errors in data processing and decision-making, enhancing the quality of work. By relying on AI algorithms that consistently follow rules without the variability of human error, companies can ensure higher standards of performance and reliability in their services and products.
- Availability: They operate around the clock, providing constant productivity that surpasses human capabilities. This continuous operation is crucial in industries like healthcare and online retail, where customer needs must be addressed promptly, irrespective of the time of day.
- Personalization: AI agents analyze customer data to deliver tailored experiences, improving customer satisfaction and engagement. They can adapt interactions based on individual user behavior and preferences, making each interaction more relevant and effective, thereby increasing the likelihood of customer retention and loyalty.
- Scalability: Businesses can scale operations quickly without proportional increases in human resources or costs. AI agents are easily deployed and can manage increased loads without the need for extensive human intervention, making scaling both efficient and cost-effective.
The integration of AI agents thus not only boosts operational efficiency but also plays a pivotal role in enhancing customer experiences and managing growth effectively, which are key determinants of success in the modern business landscape.
5. Real-World Applications of AI Agents
AI agents are versatile tools that find applications in numerous sectors of the real, everyday world:
- Customer Service: Chatbots and virtual assistants powered by AI agent frameworks respond to customer inquiries instantly, offering information and resolving issues without human input. They are particularly effective in handling high volumes of inquiries simultaneously, which can drastically reduce wait times and improve customer satisfaction. Furthermore, they are able to reduce customer service costs by up to 30% and can learn from their individual interactions to better understand customer needs, enabling more effective communication and problem-solving over time. Reportedly, 54% of companies are using AI agents for customer service in the form of conversational AI.
- Healthcare: AI agents in healthcare help assist the process of rapid disease diagnostics by improving the accuracy rate of medical processes such as CT image processing for plaque detection up to 97%. They are also used to suggest treatment plans, manage patient data, and contribute to more informed healthcare decisions. AI agents are also capable of processing and analyzing vast amounts of medical data much faster than their human counterparts, which is why they can automate more than 80% of clinical documentation tasks, thereby improving the overall efficiency of healthcare providers all over the world. Additionally, AI agents support continuous monitoring and care management, enhancing personalized care delivery.
- Finance: Statistics mention that 43% of financial professionals have reported a much better operational efficiency after integrating AI agents into their systems. That number doesn't really come as a surprise, given that AI agents in finance are capable of autonomously performing high-volume trading, managing portfolios, detecting fraud, and offering personalized financial advice. Their ability to analyze market conditions and execute trades at speeds and volumes unattainable by human traders helps enhance the overall market efficiency.
- Retail: AI agents in retail help personalize shopping experiences, manage inventory, and optimize logistics. They use consumer data to tailor product recommendations and promotions, leading to increased customer engagement and sales. Nearly 72% of retailers stated that using AI agents led to an overall decrease in their operating costs due to improved efficiency in delivering personalized customer experiences. AI agents also streamline supply chain management by predicting demand trends, helping retailers manage stock levels more effectively.
- Manufacturing: These agents schedule production, predict maintenance, and control quality processes, driving efficiency in manufacturing lines. By predicting when machines will require maintenance with a detection accuracy rate of 90%, AI agents in manufacturing help avoid unexpected breakdowns and prolong equipment life. They also optimize production schedules based on various factors, such as demand forecasts and resource availability, ensuring maximal operational efficiency.
- Marketing: AI agents enhance marketing strategies through data-driven insights and automation. They analyze consumer behavior, segment audiences, and personalize marketing campaigns to target specific customer segments more effectively. AI agents for marketing also optimize ad placements and budgets by predicting the performance of various marketing channels, enabling more efficient use of resources. Additionally, they help in managing and automating AI content creation, A/B testing, and customer engagement, ultimately driving higher conversion rates and improving overall marketing ROI.
- Legal: AI agents streamline various processes in the legal industry, from document review and contract analysis to faster legal research (with a speed difference of nearly 30% in comparison to humans) and case management. They can automatically review and categorize legal documents, identify relevant case law, and generate summaries, significantly reducing the time and effort required for these tasks. Legal AI agents also assist in predictive analytics, helping legal professionals forecast case outcomes and assess risks. Additionally, they facilitate more efficient legal research by quickly sifting through vast amounts of legal data and providing relevant insights, enhancing decision-making and reducing operational costs.
- Insurance: AI agents revolutionize insurance risk evaluation by analyzing vast amounts of data to assess and predict risk more accurately. They process historical claims, demographic information, and behavioral data to identify patterns and potential risks. This enables insurers to better assess policyholder risk, set appropriate premiums, and prevent fraud. AI agents also enhance underwriting processes by automating data collection and analysis, leading to faster and more precise risk assessments.
- Robotic Hardware: AI agents with robotic hardware are agents integrated into physical robots, allowing them to interact with and respond to the real world through sensors, actuators, and various mechanical components. These agents combine AI algorithms with robotics technology to perform complex tasks autonomously, such as navigation, object manipulation, and environmental adaptation. This type of AI agent is commonly used in manufacturing, healthcare, and logistics to improve efficiency and precision.
- Real Estate: AI agents are transforming the real estate industry by automating tasks, improving customer experiences, and providing data-driven insights. For instance, AI agents can assist with virtual property tours, analyze market trends to optimize property listings, provide personalized property recommendations, and streamline customer interactions through chatbots. These agents help real estate professionals enhance operational efficiency, increase client engagement, and make more informed decisions.
6. Examples of AI Agents in Action
6.1 AI Agents in LangGraph
LangGraph is a framework that allows the development of AI agents capable of natural language understanding, reasoning, and decision-making. Through LangGraph, developers can build and integrate complex agents that handle sophisticated conversational tasks, process multi-turn dialogues, and respond to various queries across domains. This framework streamlines the creation of language-based AI agents by providing tools for natural language processing (NLP), machine learning integration, and logic-driven workflows, making it ideal for applications in customer service, personal assistants, and more.
6.2 Real-world implementations of AI agents vividly demonstrate their transformative impact across different industries:
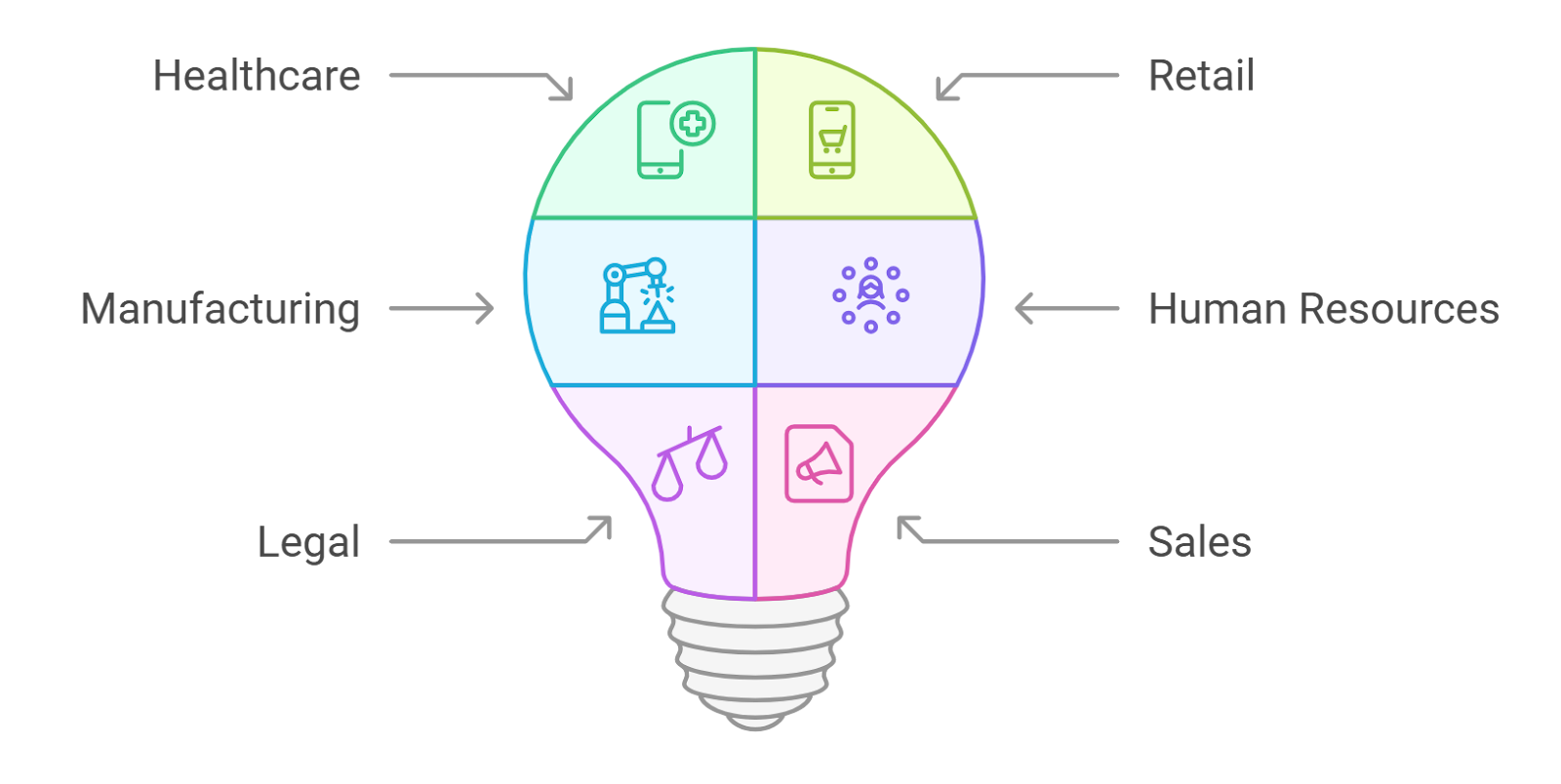
- Healthcare: IBM Watson Health utilizes AI to analyze extensive medical data and provide insights that assist healthcare professionals in diagnosing and treating patients more effectively. This includes analyzing unstructured data such as doctors' notes, medical images, and research articles to offer comprehensive patient care plans, which can lead to better health outcomes and more personalized treatment approaches.
- Retail: AI chatbots on e-commerce platforms manage customer service interactions, offer personalized shopping advice, and enhance the customer journey. These chatbots engage customers by answering questions, providing product recommendations based on browsing behavior and previous purchases, and facilitating transactions, which not only boosts customer satisfaction but also increases sales conversion rates.
- Manufacturing: AI systems in factories are designed to predict when machines require maintenance before breakdowns occur, thereby reducing downtime and maintenance costs.
- Human Resources: A notable real-world example of AI agents being used in a high-end business organization is of McKinsey & Company. McKinsey, which is one of the biggest consulting firms in the world, has lately been driving their R&D attention towards the development of an AI agent for human resources (HR) that can can speed up the client onboarding process significantly, leading to the lead time being reduced by 90% and overall administrative work being reduced by 30%.
- Legal: AI agents for the legal industry can be used for efficient legal research and documentation, and Thomson Reuters, a leading global provider of business information services, has recognized this use case early on to their advantage. They have worked tirelessly on the creation of an internal AI agent that can accelerate the long time taken for their legal due diligence processes. And the results have been considerably effective, with reports stating that this legal AI agent can reduce the time for legal processes in the company by 50%.
- Sales: AI agents in sales can work closely with human sales reps for greater efficiency in sales processes such as lead generation, AI powered sales forecasting, product recommendations, AI-created pitch decks, and customer service in the form of cold calling, follow ups, etc. Salesforce, the global leader in sales-based CRM platforms for companies, has released its own line of AI agents known as Agentforce, which comprises of intelligent AI agents for sales processes such as Sales Development Representative (SDR) Agent, which is used on the client-side to answer all questions and queries the customer might have about the product, or Sales Coach Agent, for providing new sales trainees and reps with real-time training in the form of realistic role-plays and negotiations with AI agents.
This predictive maintenance is achieved by continuously monitoring equipment conditions through sensors and using AI to analyze data to predict potential failures. As a result, manufacturing processes become more reliable, and factories can avoid the steep costs associated with unplanned downtime.
7. Further illustrating the diversity of AI agent applications:

7.1. Transportation and Logistics:
- Real-time route optimization
- AI agents analyze traffic patterns, weather conditions, and unexpected delays
- Dynamic rerouting to avoid congestion and reduce fuel consumption
- Predictive maintenance
- AI monitors vehicle health and predicts potential breakdowns
- Schedules maintenance proactively to minimize downtime
- Autonomous vehicle coordination
- AI agents manage fleets of self-driving vehicles
- Optimize passenger pickup and drop-off in ride-sharing services
7.2. Finance:
- High-frequency trading
- AI executes trades at millisecond speeds based on market conditions
- Analyzes vast amounts of financial data to identify profitable opportunities
- Fraud detection
- AI agents monitor transactions in real-time to identify suspicious patterns
- Adapt to new fraud techniques through machine learning
- Personalized financial advice
- AI-powered robo-advisors provide tailored investment strategies
- Continuously adjust portfolios based on market trends and individual goals
7.3. Energy:
- Smart grid management
- AI balances energy supply and demand in real-time
- Integrates renewable energy sources efficiently into the grid
- Energy consumption forecasting
- Predicts energy needs based on historical data and external factors
- Enables proactive energy production and distribution planning
- Optimization of renewable energy systems
- AI agents manage solar panel positioning for maximum sunlight exposure
- Optimize wind turbine performance based on weather forecasts
7.4. Healthcare:
- Diagnostic assistance
- AI analyzes medical images to detect anomalies and assist radiologists
- Provides second opinions and flags potential misdiagnoses
- Personalized treatment plans
- AI agents consider genetic information, lifestyle factors, and medical history
- Recommend tailored treatment options for individual patients
- Drug discovery
- AI accelerates the process of identifying potential new medications
- Simulates drug interactions to predict efficacy and side effects
7.5. Agriculture:
- Precision farming
- AI-powered drones and sensors monitor crop health and soil conditions
- Provide targeted recommendations for irrigation and fertilization
- Livestock management
- AI agents track animal health and behavior patterns
- Optimize feeding schedules and detect early signs of illness
- Crop yield prediction
- Analyze historical data, weather patterns, and soil quality
- Forecast harvest yields to inform planting and market decisions
7.6. Customer Service:
- Intelligent chatbots
- AI-powered assistants handle customer inquiries 24/7
- Learn from interactions to improve response accuracy over time
- Sentiment analysis
- AI agents analyze customer feedback across multiple channels
- Identify trends and potential issues before they escalate
- Personalized recommendations
- AI systems suggest products or services based on individual preferences
- Continuously refine recommendations through machine learning
7.7. Manufacturing:
- Quality control
- AI-powered computer vision systems detect defects in real-time
- Reduce waste and improve product consistency
- Supply chain optimization
- AI agents predict demand fluctuations and manage inventory levels
- Coordinate with suppliers to ensure timely delivery of materials
- Robotic process automation
- AI controls robotic systems for complex assembly tasks
- Adapts to changes in production requirements quickly
7.8. Education:
- Adaptive learning platforms
- AI tailors educational content to individual student needs
- Identifies knowledge gaps and provides targeted exercises
- Automated grading and feedback
- AI agents assess written assignments and provide constructive feedback
- Free up teacher time for more personalized instruction
- Early intervention systems
- AI analyzes student performance data to identify at-risk individuals
- Suggests intervention strategies to improve academic outcomes
These examples showcase the breadth of AI agent capabilities, highlighting their role in enhancing operational efficiency, improving customer experiences, and contributing to cost-effective business solutions across various industries.
8. Challenges of AI Agents
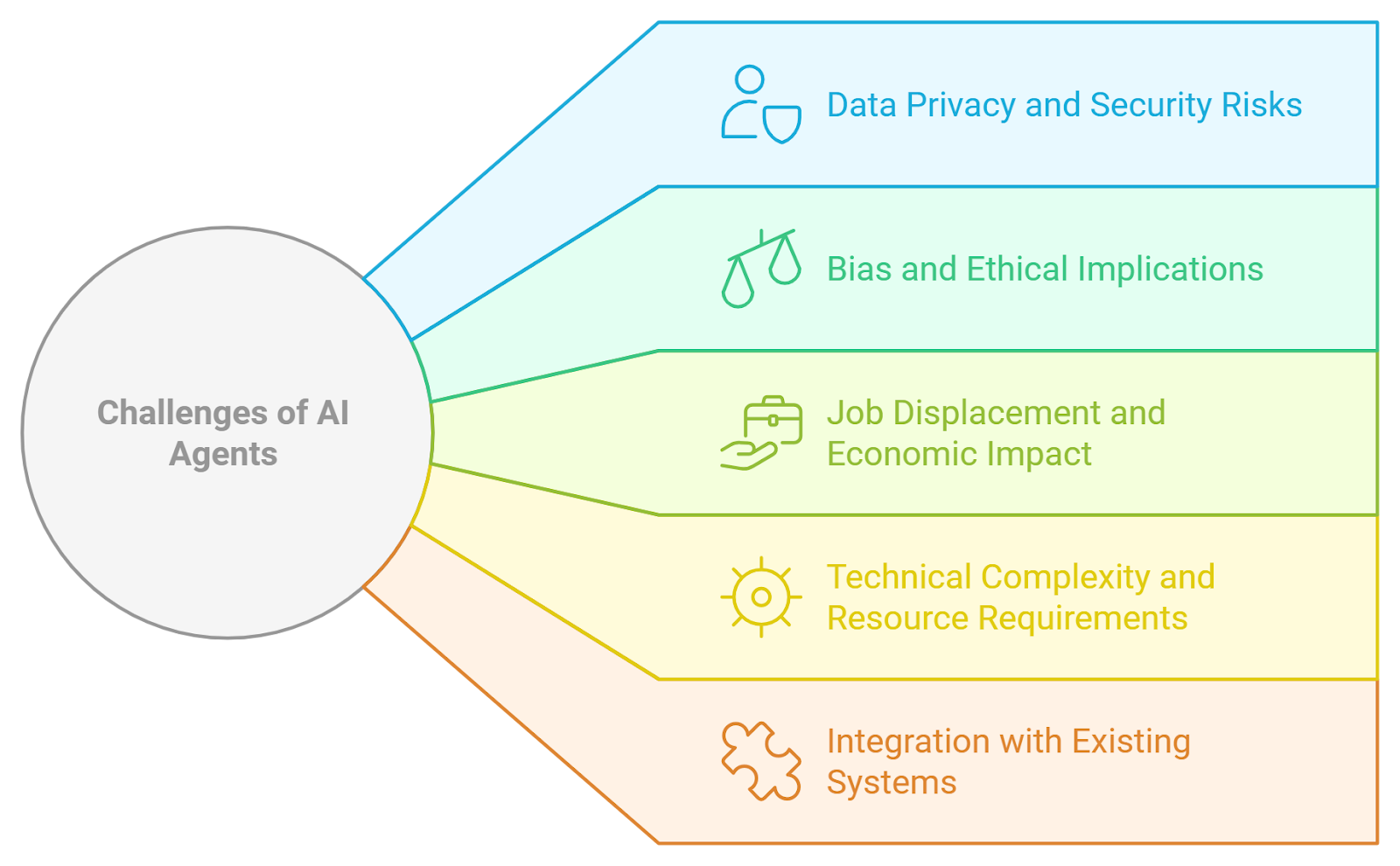
AI agents hold incredible potential across industries, promising automation, personalized customer interactions, and efficient data-driven insights. However, developing and implementing AI agents come with significant challenges that companies must carefully navigate. From data privacy issues to ethical concerns, the adoption of AI agents faces various obstacles that, if unaddressed, can hinder widespread usage. Below, we explore the core challenges associated with AI agents and why businesses remain cautious about implementing them on a broad scale.
8.1. Data Privacy and Security Risks
One of the primary challenges in AI agent development is safeguarding user data. AI agents require vast datasets to function effectively, especially for tasks like natural language processing or predictive analytics. However, collecting and processing such information can expose companies to data breaches and unauthorized data access. Privacy regulations such as GDPR and CCPA demand strict data handling and user consent practices, yet maintaining compliance while allowing AI agents to learn and improve is a complex task. The data privacy concerns associated with AI agents make many companies hesitant to deploy them, as even minor mismanagement can result in significant reputational and financial damage.
8.2. Bias and Ethical Implications
AI agents are only as good as the data they're trained on, which raises concerns about bias and ethics. AI models trained on biased datasets can inadvertently reinforce prejudices, leading to skewed or unethical decision-making. For instance, AI agents in recruitment may inadvertently discriminate based on race or gender if the training data reflects historical biases. Addressing these issues requires extensive data curation and monitoring, which can be resource-intensive. As a result, organizations must approach AI agent development with strict ethical oversight, further complicating the deployment process.
8.3. Job Displacement and Economic Impact
While AI agents can enhance productivity and automate routine tasks, they also pose a risk of job displacement, particularly in sectors like customer service, finance, and logistics. As AI agents become more capable of handling sophisticated tasks, the need for human intervention in certain roles decreases, raising fears of widespread job loss. Companies are often reluctant to adopt AI agents due to the economic and social implications of displacing employees, which can lead to morale issues, regulatory scrutiny, and public backlash. This potential for job displacement presents a major hurdle in AI agent deployment.
8.4. Technical Complexity and Resource Requirements
AI agent development involves substantial technical complexity, requiring expertise in machine learning, data engineering, and natural language processing. This high skill requirement can make it difficult for organizations to develop effective AI agents in-house. Additionally, training AI agents demands considerable computational resources and infrastructure. The cost of implementing and maintaining these resources can be prohibitive, especially for small to medium-sized businesses. Without the right technical foundation, companies risk creating AI agents that are unreliable or fail to perform as intended, discouraging their continued investment in AI solutions.
8.5. Integration with Existing Systems
For companies that rely on legacy systems, integrating AI agents can be particularly challenging. AI agents often require modern infrastructure to function optimally, which may not be compatible with outdated IT systems. The cost and complexity of upgrading or adapting these systems to support AI agents can be prohibitive. Furthermore, successful integration may require a hybrid approach, where human and AI agents work collaboratively. Balancing this relationship effectively is difficult and may create friction, particularly in industries that are slow to adopt new technologies.
9. Future of AI Agents

AI agents are on the path to becoming central to our digital and physical worlds, supporting both professional and personal tasks with unprecedented efficiency. In the next decade, we can expect AI agent development to advance rapidly, with AI agents becoming smarter, more adaptable, and seamlessly integrated into everyday life. Industries such as healthcare, finance, education, and environmental sciences stand to benefit enormously from AI agents, which will provide critical support in solving complex problems and driving productivity.
As we move into an AI-driven future, developing responsible and ethical AI agents will be key to ensuring that these technologies benefit society as a whole. Below, we delve into what the future holds for AI agents, their evolving forms, and the key industries poised to benefit from this technology.
9.1. AI Agents as Digital Work Companions
In the coming decade, AI agents are expected to become essential digital work companions, assisting with tasks that currently consume large portions of our day. Routine activities such as managing emails, organizing schedules, and conducting online research will increasingly be handled by AI agents. This shift will free up employees to focus on creative, strategic, and human-centered tasks, enhancing productivity across industries. Intelligent AI agents could act as personal project managers, reminding users of deadlines, prioritizing tasks, and even providing data insights for better decision-making. As these capabilities evolve, AI agents are set to become integral to the workplace of the future.
9.2. Transforming Customer Experiences
The future of AI agents in customer service is already taking shape, with the goal of providing hyper-personalized, seamless customer experiences. In the next few years, we can expect AI agents to operate with advanced natural language processing (NLP) and emotion recognition, allowing them to understand and respond to human emotions with empathy. For instance, an AI customer service agent might detect a customer’s frustration and adjust its tone and solutions accordingly, creating more satisfying interactions. As these AI-driven customer support tools become more refined, they will be widely adopted by retail, finance, and telecommunications industries, reshaping how brands connect with their audiences.
9.3. Automating Knowledge-Intensive Tasks in Healthcare
AI agents have transformative potential in the healthcare sector, where they can assist with diagnosing patients, managing patient records, and even offering initial treatment recommendations. Advanced AI agents will be capable of analyzing vast amounts of medical data, helping healthcare providers make accurate diagnoses based on a patient’s medical history and symptoms. Within the next decade, AI agents might serve as virtual healthcare assistants, supporting doctors in clinical decision-making or assisting patients in managing chronic conditions from home. By automating data-heavy and time-consuming processes, AI agents will help healthcare professionals deliver better and more efficient patient care.
9.4. Empowering Financial Decision-Making
AI agents will play an increasingly important role in the finance industry by assisting with complex data analysis, risk assessment, and customer service. Financial AI agents will not only offer real-time insights for investors and consumers but will also help automate compliance and fraud detection processes. Within the next few years, we might see AI agents operating as virtual financial advisors, providing personalized advice to clients by analyzing spending patterns, investment portfolios, and financial goals. Such AI-driven decision-making will allow financial institutions to offer more tailored services, meeting the demands of an increasingly digital-first customer base.
9.5. Elevating E-Learning and Personal Education
The future of education is also being shaped by AI agents that can act as personalized tutors, guiding students based on individual learning preferences and areas of improvement. As e-learning grows in popularity, AI agents will serve as digital mentors, assessing student progress in real-time and providing targeted feedback. In the next 5-10 years, AI-powered educational agents could transform how we acquire skills, allowing students of all ages to learn more effectively and in a personalized manner. This will be particularly impactful in online education platforms, which can integrate these AI tutors to offer a more engaging and customized learning experience.
9.6. Assisting in Environmental Monitoring and Climate Solutions
With growing environmental concerns, AI agents are anticipated to play an active role in monitoring ecosystems, predicting weather patterns, and aiding in resource conservation. These AI agents will process large datasets collected from satellites, IoT sensors, and research labs to track climate changes and make informed predictions. For industries involved in agriculture, urban planning, and environmental conservation, AI agents could provide insights for sustainable decision-making. By monitoring environmental data, they can help predict and mitigate climate-related disasters, supporting global efforts toward a greener future.
9.7. Developing AI-Driven Autonomous Systems
AI agents will significantly impact the development of autonomous vehicles and robotics. In transportation, future AI agents could work alongside human drivers or operate fully autonomously, creating safer and more efficient travel experiences. For instance, AI agents could monitor road conditions, make real-time driving decisions, and even communicate with other vehicles for coordinated driving. Similarly, in manufacturing, AI agents are expected to operate complex robotics systems, optimizing assembly lines and improving quality control. As autonomous systems advance, these AI agents will enhance efficiency and safety across various industries.
10. Rapid Innovation's AI Agent Development: Shaping the Future for Entrepreneurs and Innovators
Rapid Innovation and its expert AI agent development services offer entrepreneurs and innovators the tools to develop new solutions and bring them to market quickly. By automating routine tasks and analyzing vast amounts of data swiftly, our AI agents, created by an expert AI agent development team, will allow businesses to experiment with new ideas at a faster pace, reduce time to market, and respond to customer needs and market changes with agility.
This rapid cycle of innovation with the latest automated AI agentic systems is crucial for staying competitive in today's fast-paced business environment. Additionally, AI agents empower businesses to adapt existing products to better meet evolving technological trends and consumer expectations. They also provide a foundation for scalable growth, enabling companies to expand their operations without proportionally increasing their overhead costs. Furthermore, the use of AI agents in iterative design and development processes enhances product development, allowing for continuous improvements based on user feedback and behavior analysis.
11. Conclusion
AI agents are more than just technological advancements; they are transformational tools that redefine how businesses operate and interact with their environments. As we continue to advance in the digital age, the integration of AI agents into various business processes is becoming increasingly important. These agents not only streamline operations but also open up new avenues for growth, customer engagement, and strategic decision-making.
The strategic deployment of AI agents can significantly enhance operational efficiencies and decision accuracy, which are crucial in high-stakes industries like finance and healthcare. Furthermore, they facilitate a deeper understanding of consumer behaviors and market trends, enabling businesses to tailor their strategies more effectively and responsively. Their ability to quickly process and analyze vast amounts of data means decisions are informed and timely, giving businesses that employ these tools a clear advantage over competitors.
For companies aiming to thrive in an ever-evolving marketplace, leveraging the power of AI agents is not just an option but a necessity. Working with technology experts to implement these AI solutions can help businesses achieve sustainable growth and maintain a competitive edge. Implementing AI agents helps ensure that businesses are not only keeping pace with technological trends but are also setting the pace, pushing the boundaries of what is possible within their industries.