Table Of Contents
Category
Artificial Intelligence
Marketing
AIML
IoT
Blockchain
Retail & Ecommerce
Supply Chain & Logistics
1. Introduction to AI-Driven Personalization
AI-driven personalization is revolutionizing the way businesses interact with their customers. By leveraging artificial intelligence, companies can create tailored experiences that resonate with individual users, enhancing engagement and satisfaction. This approach goes beyond traditional marketing strategies, utilizing data analytics and machine learning to understand user preferences and behaviors.
At Rapid Innovation, we specialize in implementing aidriven personalization strategies that help our clients achieve their business goals efficiently and effectively. Our expertise in AI allows us to develop customized solutions that analyze vast amounts of data to identify patterns and trends, enabling personalization across various digital platforms, including websites, mobile apps, and social media. The goal is to create a seamless and relevant experience for each user, increasing the likelihood of conversion and loyalty.
The rise of AI-driven personalization is largely due to advancements in technology and the increasing availability of data. Businesses can now collect and analyze user data in real-time, allowing for immediate adjustments to marketing strategies and user experiences. This dynamic approach not only improves user satisfaction but also drives business growth. Enhanced user experience leads to higher retention rates, while personalized recommendations can significantly boost sales. Companies that implement aidriven personalization often see improved customer insights, resulting in greater ROI.
As consumers become more accustomed to personalized experiences, businesses must adapt to meet these expectations. AI-driven personalization is not just a trend; it is becoming a necessity for companies looking to thrive in a competitive digital landscape. At Rapid Innovation, we are committed to helping our clients navigate this landscape by providing tailored AI solutions that drive results and foster long-term success. For more insights, check out our article on enhancing online shopping with personalized product suggestions.
Refer to the image for a visual representation of AI-driven personalization concepts.

1.1. Defining User Experience Personalization
User experience personalization refers to the process of tailoring digital interactions to meet the specific needs, preferences, and behaviors of individual users. This approach enhances user satisfaction and engagement by creating a more relevant and meaningful experience. Personalization can be achieved through various methods, including:
- User data analysis: Collecting and analyzing data such as browsing history, purchase behavior, and demographic information.
- Dynamic content: Adjusting website or app content in real-time based on user interactions.
- Recommendations: Offering personalized product or content suggestions based on user preferences.
The goal of user experience personalization is to create a seamless and intuitive interaction that resonates with users, ultimately leading to increased loyalty and conversion rates. By understanding user behavior and preferences, businesses can deliver targeted experiences that drive engagement and satisfaction. At Rapid Innovation, we leverage advanced AI and blockchain technologies to enhance personalization, ensuring that our clients can achieve their business goals efficiently and effectively. This includes focusing on personalization ux and personalized user experience to create a more engaging environment. For more insights on how AI can enhance user experience, check out our article on understanding proactive AI agents.
1.2. Evolution of Personalization Technologies
The evolution of personalization technologies has transformed how businesses interact with their customers. Over the years, advancements in technology have enabled more sophisticated and effective personalization strategies. Key milestones in the evolution of personalization technologies include:
- Early days of personalization: Simple methods like email marketing and basic segmentation based on demographics.
- Introduction of cookies: The use of cookies allowed websites to track user behavior and preferences, paving the way for more tailored experiences.
- Machine learning and big data: The rise of machine learning algorithms and big data analytics has enabled businesses to process vast amounts of user data, leading to more accurate and effective personalization.
Today, personalization technologies leverage artificial intelligence (AI) and machine learning to create highly customized experiences. These technologies analyze user behavior in real-time, allowing businesses to adapt their offerings dynamically. As a result, personalization has become a critical component of digital marketing strategies, enhancing user engagement and driving conversions. Rapid Innovation harnesses these technologies to provide our clients with innovative solutions that maximize their return on investment (ROI). The focus on ux personalisation is essential in this evolving landscape.
1.3. The Role of AI in User Experience
Artificial intelligence plays a pivotal role in enhancing user experience personalization. By leveraging AI technologies, businesses can analyze user data more effectively and deliver tailored experiences that meet individual needs. Key contributions of AI to user experience include:
- Predictive analytics: AI algorithms can predict user behavior based on historical data, allowing businesses to anticipate user needs and preferences.
- Natural language processing (NLP): NLP enables chatbots and virtual assistants to understand and respond to user inquiries in a conversational manner, improving customer support and engagement.
- Personalization at scale: AI can process large volumes of data quickly, enabling businesses to deliver personalized experiences to millions of users simultaneously.
The integration of AI in user experience personalization not only enhances the relevance of interactions but also improves efficiency. Businesses can automate processes, reduce response times, and create a more engaging user journey. As AI technology continues to evolve, its impact on user experience will likely grow, leading to even more innovative personalization strategies. At Rapid Innovation, we are committed to helping our clients leverage AI to achieve greater efficiency and effectiveness in their operations, ultimately driving higher ROI. This commitment includes a focus on personalized user interface design to enhance overall user experience.
Refer to the image for a visual representation of user experience personalization and its components.

1.4. Paradigm Shift in Digital Interactions
The digital landscape has undergone a significant transformation in recent years, leading to a paradigm shift in how individuals and organizations interact. This shift is characterized by several key trends:
- Increased Connectivity: The proliferation of smartphones and high-speed internet has made it easier for people to connect anytime, anywhere. This constant connectivity has changed the way we communicate, work, and socialize.
- Social Media Influence: Platforms like Facebook, Twitter, and Instagram have redefined social interactions. They allow users to share experiences, opinions, and information instantly, creating a global conversation that transcends geographical boundaries.
- Emergence of Virtual and Augmented Reality: Technologies such as virtual reality (VR) and augmented reality (AR) are changing the way we experience digital content. These immersive technologies provide new avenues for interaction, from gaming to virtual meetings.
- Shift to Remote Work: The COVID-19 pandemic accelerated the adoption of remote work, leading to a reevaluation of traditional workplace dynamics. Tools like Zoom and Slack have become essential for collaboration, fostering a new culture of digital communication.
- Personalization and AI: Artificial intelligence (AI) is enabling more personalized digital interactions. Algorithms analyze user behavior to tailor content, recommendations, and advertisements, enhancing user engagement. At Rapid Innovation, we leverage AI to help businesses optimize their customer engagement strategies, resulting in improved user experiences and higher conversion rates.
This paradigm shift has profound implications for businesses and individuals alike, necessitating a reevaluation of strategies for engagement and communication.
1.5. Ethical Considerations and User Privacy
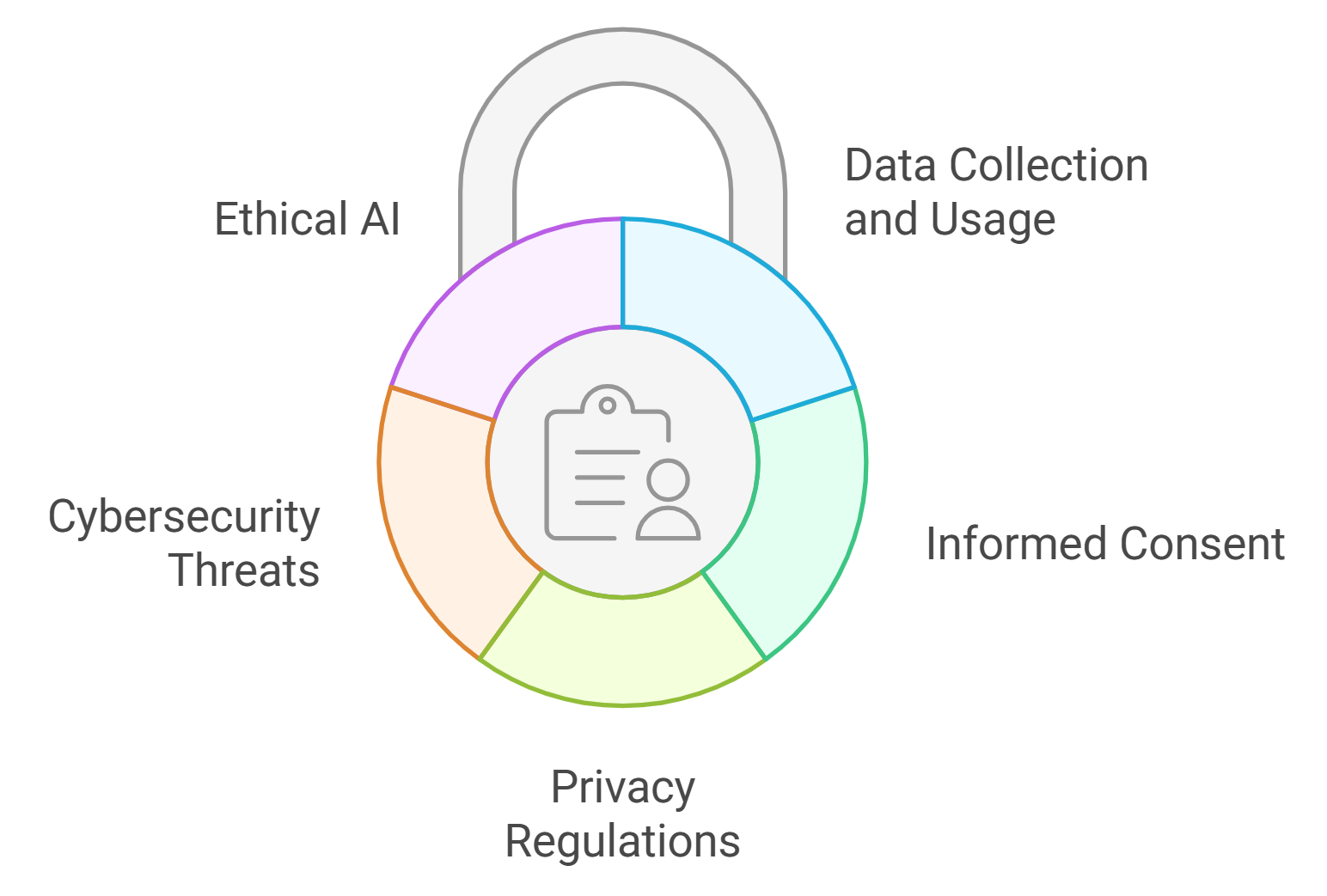
As digital interactions evolve, ethical considerations and user privacy have become paramount. The following points highlight the critical aspects of this issue:
- Data Collection and Usage: Companies collect vast amounts of user data to enhance services and target advertisements. However, this raises concerns about how data is used and whether users are adequately informed about data collection practices.
- Informed Consent: Users often agree to terms and conditions without fully understanding them. Ethical practices require clear communication about what data is collected and how it will be used, ensuring users can make informed decisions.
- Privacy Regulations: Legislation such as the General Data Protection Regulation (GDPR) in Europe and the California Consumer Privacy Act (CCPA) in the U.S. aims to protect user privacy. These regulations impose strict guidelines on data handling, emphasizing the need for transparency and accountability.
- Cybersecurity Threats: With increased data collection comes the risk of data breaches. Organizations must prioritize cybersecurity measures to protect user information from unauthorized access and potential misuse.
- Ethical AI: As AI becomes more integrated into digital interactions, ethical considerations surrounding bias, transparency, and accountability are crucial. Developers must ensure that AI systems are designed to be fair and do not perpetuate existing inequalities. At Rapid Innovation, we emphasize ethical AI practices, ensuring that our solutions not only meet business needs but also uphold user trust and integrity.
Addressing these ethical considerations is essential for building trust between users and organizations in the digital realm.
2. Foundational Technologies
The foundation of modern digital interactions is built on several key technologies that enable connectivity, communication, and data management. Understanding these foundational technologies is crucial for navigating the digital landscape:
- Cloud Computing: Cloud technology allows for the storage and processing of data over the internet, enabling businesses to scale operations and access resources on demand. This flexibility supports remote work and collaboration.
- Blockchain: This decentralized ledger technology enhances security and transparency in transactions. Blockchain is increasingly used in various sectors, including finance, supply chain, and healthcare, to ensure data integrity. Rapid Innovation specializes in blockchain solutions that help businesses streamline operations and enhance trust through transparent transactions.
- Artificial Intelligence and Machine Learning: AI and machine learning algorithms analyze vast amounts of data to identify patterns and make predictions. These technologies are integral to personalization, automation, and decision-making processes. Our expertise in AI allows us to develop tailored solutions that drive efficiency and improve ROI for our clients.
- Internet of Things (IoT): IoT connects everyday devices to the internet, allowing for data exchange and remote control. This technology is transforming industries by enabling smart homes, connected vehicles, and efficient supply chains.
- 5G Technology: The rollout of 5G networks promises faster internet speeds and lower latency, facilitating real-time communication and enhancing the capabilities of IoT devices. This technology is expected to drive innovations in various fields, including healthcare and transportation.
- Cybersecurity Solutions: As digital interactions increase, so do the threats to data security. Advanced cybersecurity technologies, including encryption, firewalls, and intrusion detection systems, are essential for protecting sensitive information.
These foundational technologies not only support current digital interactions but also pave the way for future innovations, shaping how we connect and communicate in an increasingly digital world. At Rapid Innovation, we harness these technologies to deliver cutting-edge solutions that empower our clients to achieve their business goals efficiently and effectively.
Refer to the image for a visual representation of the paradigm shift in digital interactions.

2.1. Machine Learning Fundamentals
Machine learning (ML) is a subset of artificial intelligence (AI) that focuses on the development of algorithms that allow computers to learn from and make predictions based on data. Understanding the fundamentals of machine learning is essential for anyone looking to leverage its capabilities in various applications, including designing machine learning systems, and Rapid Innovation is here to guide you through this transformative journey.
- Types of Machine Learning:
- Supervised Learning: Involves training a model on a labeled dataset, where the outcome is known. Common algorithms include linear regression, decision trees, and support vector machines. Rapid Innovation can help businesses implement supervised learning to enhance customer segmentation and improve marketing strategies, leading to increased ROI.
- Unsupervised Learning: Deals with unlabeled data, aiming to find hidden patterns or intrinsic structures. Techniques include clustering and dimensionality reduction. Our expertise in unsupervised learning can assist clients in identifying market trends and optimizing inventory management.
- Reinforcement Learning: Involves training models to make sequences of decisions by rewarding desired behaviors and punishing undesired ones. This is often used in robotics and game playing. Rapid Innovation can develop reinforcement learning solutions that optimize operational processes, resulting in significant cost savings.
- Key Concepts:
- Features and Labels: Features are the input variables used for prediction, while labels are the output variables.
- Training and Testing: The dataset is typically split into training and testing sets to evaluate the model's performance.
- Overfitting and Underfitting: Overfitting occurs when a model learns the training data too well, while underfitting happens when it fails to capture the underlying trend. Our team ensures that models are well-tuned to avoid these pitfalls, maximizing their effectiveness.
- Applications:
- Fraud detection in finance
- Recommendation systems in e-commerce
- Predictive maintenance in manufacturing
- Machine learning applications in various sectors, including machine learning for drug discovery and machine learning in business intelligence.
Understanding these fundamentals is crucial for developing effective machine learning models that can solve real-world problems, and Rapid Innovation is committed to helping clients harness this technology for greater business success. For more insights on related technologies, check out Natural Language Processing (NLP).
2.2. Natural Language Processing
Natural Language Processing (NLP) is a field of AI that focuses on the interaction between computers and humans through natural language. It enables machines to understand, interpret, and respond to human language in a valuable way, and Rapid Innovation specializes in implementing NLP solutions that drive efficiency and engagement.
- Core Components:
- Tokenization: The process of breaking down text into smaller units, such as words or phrases.
- Part-of-Speech Tagging: Identifying the grammatical parts of speech in a sentence, which helps in understanding the structure.
- Named Entity Recognition (NER): The identification of entities such as names, dates, and locations within text.
- Techniques:
- Sentiment Analysis: Determining the sentiment behind a piece of text, whether positive, negative, or neutral. Our NLP solutions can help businesses gauge customer sentiment, leading to improved service and product offerings.
- Machine Translation: Automatically translating text from one language to another, as seen in tools like Google Translate. Rapid Innovation can develop customized translation tools that enhance global communication.
- Chatbots and Virtual Assistants: Utilizing NLP to create conversational agents that can assist users in various tasks. We design intelligent chatbots that improve customer service and reduce operational costs.
- Challenges:
- Ambiguity in language
- Contextual understanding
- Sarcasm and idiomatic expressions
NLP is transforming how we interact with technology, making it more intuitive and user-friendly, and Rapid Innovation is at the forefront of this transformation, helping clients achieve their business goals.
2.3. Computer Vision in User Interaction
Computer vision is a field of AI that enables machines to interpret and make decisions based on visual data from the world. It plays a significant role in enhancing user interaction across various platforms, and Rapid Innovation leverages this technology to create innovative solutions for our clients.
- Key Technologies:
- Image Recognition: The ability of a system to identify objects, people, or scenes in images. This is widely used in social media tagging and security systems. Our image recognition solutions can enhance user experiences and improve security measures for businesses.
- Facial Recognition: A specific type of image recognition that identifies individuals based on their facial features. It is commonly used in security and authentication. Rapid Innovation can implement facial recognition systems that streamline access control and enhance security protocols.
- Gesture Recognition: Interpreting human gestures via mathematical algorithms, allowing for touchless interaction with devices. We develop gesture recognition applications that create immersive user experiences in various industries.
- Applications:
- Augmented reality (AR) experiences in gaming and retail
- Autonomous vehicles that rely on visual data for navigation
- Medical imaging analysis for diagnosing diseases
- Deep learning applications in computer vision and machine learning image classification.
- Challenges:
- Variability in lighting and angles
- Occlusion of objects
- Real-time processing requirements
Computer vision is revolutionizing user interaction by making it more immersive and engaging, paving the way for innovative applications in various industries. Rapid Innovation is dedicated to helping clients harness the power of computer vision to achieve their business objectives effectively and efficiently.
Refer to the image for a visual representation of the Machine Learning Fundamentals discussed above:

2.4. Predictive Analytics
Predictive analytics is a branch of advanced analytics that uses historical data, statistical algorithms, and machine learning techniques to identify the likelihood of future outcomes. This approach is widely used across various industries to enhance decision-making processes.
- Key components of predictive analytics include:
- Data collection: Gathering relevant data from various sources, including databases, sensors, and social media.
- Data preprocessing: Cleaning and transforming data to ensure accuracy and consistency.
- Model building: Utilizing statistical models and machine learning algorithms to analyze data patterns.
- Validation: Testing the model against a separate dataset to ensure its predictive power.
- Deployment: Implementing the model in real-world applications to generate predictions.
- Applications of predictive analytics are vast and include:
- Customer behavior prediction: Businesses can forecast customer preferences and buying habits, allowing for targeted marketing strategies.
- Risk management: Financial institutions use predictive analytics to assess credit risk and detect fraudulent activities.
- Healthcare: Predictive models can help in patient diagnosis and treatment planning by analyzing historical health data. For example, the application of predictive analytics in healthcare has led to improved patient outcomes.
At Rapid Innovation, we leverage predictive analytics to help our clients achieve greater ROI by enabling data-driven decision-making. For instance, a retail client utilized our predictive analytics solutions to optimize inventory management, resulting in a 20% reduction in stockouts and a 15% increase in sales. Additionally, we have developed hr predictive analytics tools that assist organizations in workforce planning and talent management.
Predictive analytics has proven to be a game-changer in various sectors, leading to improved efficiency and profitability. Organizations that leverage predictive analytics can achieve a significant competitive advantage. The applications of predictive modeling, such as predictive analytics applications examples, demonstrate the versatility of this approach across different industries, including business applications of predictive analytics and crm predictive modeling. For more information on related techniques, you can refer to deep learning.
2.5. Deep Learning Architectures
Deep learning architectures are a subset of machine learning that utilize neural networks with many layers (hence "deep") to model complex patterns in large datasets. These architectures have revolutionized fields such as computer vision, natural language processing, and speech recognition.
- Common types of deep learning architectures include:
- Convolutional Neural Networks (CNNs): Primarily used for image processing tasks, CNNs excel at recognizing patterns and features in visual data.
- Recurrent Neural Networks (RNNs): Designed for sequential data, RNNs are effective in tasks like language modeling and time series prediction.
- Generative Adversarial Networks (GANs): These consist of two neural networks that compete against each other, enabling the generation of realistic synthetic data.
- Benefits of deep learning architectures:
- High accuracy: Deep learning models often outperform traditional machine learning models in tasks involving large datasets.
- Automation: These architectures can automatically extract features from raw data, reducing the need for manual feature engineering.
- Scalability: Deep learning models can handle vast amounts of data, making them suitable for big data applications.
At Rapid Innovation, we have successfully implemented deep learning solutions for clients in various industries. For example, a healthcare provider utilized our deep learning models for medical image analysis, achieving a diagnostic accuracy improvement of over 30%. The impact of deep learning is evident in various industries, from autonomous vehicles to healthcare diagnostics.
2.6. Reinforcement Learning Techniques
Reinforcement learning (RL) is a type of machine learning where an agent learns to make decisions by taking actions in an environment to maximize cumulative rewards. This approach mimics the way humans and animals learn from their experiences.
- Key concepts in reinforcement learning include:
- Agent: The learner or decision-maker that interacts with the environment.
- Environment: The context in which the agent operates and makes decisions.
- Actions: The choices available to the agent that affect the state of the environment.
- Rewards: Feedback received by the agent based on the actions taken, guiding future decisions.
- Popular reinforcement learning techniques:
- Q-learning: A model-free algorithm that learns the value of actions in a given state, allowing the agent to make optimal decisions.
- Deep Q-Networks (DQN): Combines Q-learning with deep learning to handle high-dimensional state spaces, such as images.
- Policy Gradient Methods: These directly optimize the policy (the strategy used by the agent) to maximize expected rewards.
- Applications of reinforcement learning are diverse and include:
- Robotics: RL is used to train robots to perform complex tasks, such as navigation and manipulation.
- Game playing: RL has achieved remarkable success in games like Go and chess, where it can outperform human champions.
- Autonomous systems: RL techniques are employed in self-driving cars to make real-time decisions based on environmental feedback.
At Rapid Innovation, we harness reinforcement learning to develop intelligent systems that adapt and optimize their performance over time. For instance, we helped a logistics company implement RL algorithms to enhance route optimization, resulting in a 25% reduction in delivery times. Reinforcement learning continues to evolve, with ongoing research exploring its potential in various domains.
3. AI Agent Capabilities in UX Personalization
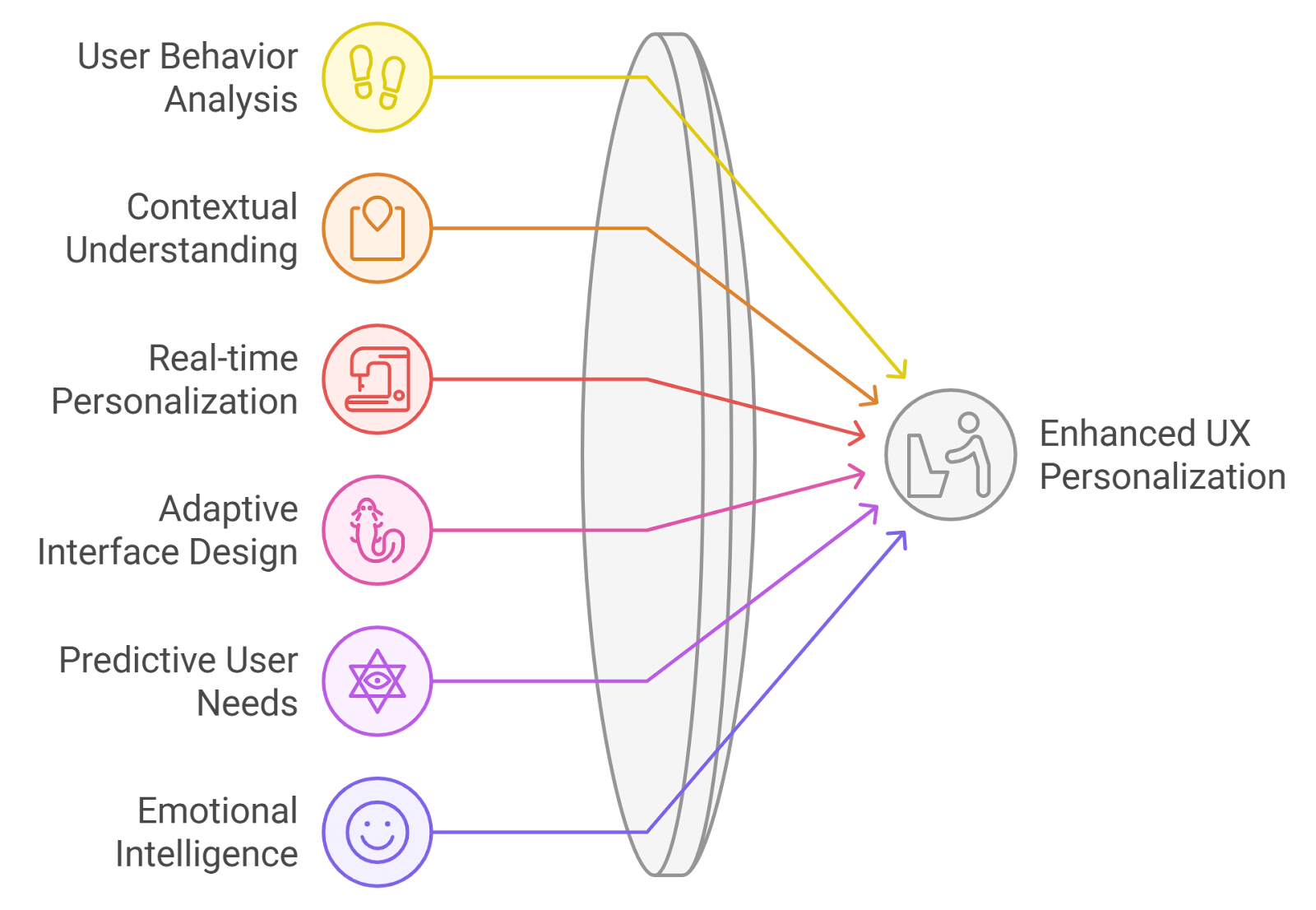
AI agents are revolutionizing user experience (UX) personalization by leveraging advanced technologies to create tailored interactions. By analyzing user behavior and understanding context, these agents can significantly enhance the overall user experience, ultimately driving greater ROI for businesses.
3.1 User Behavior Analysis
User behavior analysis is a critical component of UX personalization. AI agents utilize various techniques to gather and interpret data about how users interact with digital platforms. This analysis helps in creating a more personalized experience that aligns with business objectives.
- Data Collection: AI agents collect data from multiple sources, including click patterns, time spent on pages, and interaction with features. This comprehensive data collection enables businesses to understand user preferences better.
- Predictive Analytics: By analyzing historical data, AI can predict future user actions, allowing businesses to anticipate user needs and offer relevant content or products. This proactive approach can lead to increased customer satisfaction and loyalty.
- Segmentation: AI can segment users based on behavior, enabling targeted marketing strategies and customized user journeys. This targeted approach can enhance marketing efficiency and improve conversion rates.
- Feedback Loops: Continuous analysis of user behavior allows AI to adapt and refine its recommendations, ensuring improved accuracy over time and enhanced user satisfaction. This adaptability can lead to higher engagement and retention rates.
The effectiveness of user behavior analysis is evident in various industries. For instance, e-commerce platforms often use AI to analyze shopping habits, leading to personalized product recommendations that can significantly increase conversion rates and overall revenue.
3.2 Contextual Understanding
Contextual understanding is another vital capability of AI agents in UX personalization. It involves interpreting the environment and circumstances surrounding user interactions to deliver relevant experiences that resonate with users.
- Real-Time Context: AI agents can assess real-time data, such as location, device type, and time of day. This capability allows businesses to provide timely and relevant information to users.
- User Intent: By understanding the context, AI can infer user intent, which helps in delivering timely information and offering solutions that meet immediate needs. This understanding can enhance user satisfaction and drive engagement.
- Adaptive Interfaces: Contextual understanding allows for dynamic adjustments in user interfaces, such as changing layouts based on user preferences and modifying content presentation based on user behavior. This adaptability can lead to a more intuitive user experience.
- Emotional Recognition: Advanced AI systems can analyze user emotions through sentiment analysis of text inputs and facial recognition technology. This capability enables tailored responses that resonate with users and enhances engagement through empathetic interactions.
The integration of contextual understanding in UX personalization leads to more meaningful interactions. For example, a travel app that recognizes a user’s location can provide localized recommendations, enhancing the overall user experience and increasing the likelihood of bookings.
By combining user behavior analysis and contextual understanding, AI agents can create a seamless and personalized UX personalization that meets the diverse needs of users, ultimately helping businesses achieve their goals efficiently and effectively. Rapid Innovation is committed to leveraging these AI capabilities to drive greater ROI for our clients, ensuring they stay ahead in a competitive landscape.
3.3. Real-time Personalization
Real-time personalization refers to the ability of a system or application to tailor content and experiences to individual users based on their behavior, preferences, and interactions. This approach enhances user engagement and satisfaction by delivering relevant information at the right moment.
- Dynamic content delivery: Websites and applications can adjust their content in real-time based on user actions, such as browsing history or previous purchases. Rapid Innovation leverages AI algorithms to implement dynamic content delivery, ensuring that users receive the most relevant information, thereby increasing engagement and conversion rates.
- Behavioral tracking: Utilizing cookies and tracking technologies, businesses can gather data on user behavior to inform personalization strategies. Our expertise in AI allows us to develop sophisticated tracking systems that analyze user interactions, enabling businesses to refine their personalization efforts effectively.
- Enhanced user experience: Personalized experiences can lead to higher conversion rates, as users are more likely to engage with content that resonates with their interests. By integrating AI-driven personalization solutions, Rapid Innovation helps clients create tailored experiences that significantly boost user satisfaction and retention.
- Examples of real-time personalization include product recommendations on e-commerce sites, personalized email marketing campaigns, and tailored news feeds on social media platforms. Our team has successfully implemented these strategies for various clients, resulting in measurable improvements in ROI. Real time personalization is a key aspect of modern marketing strategies, such as those seen in marketo real time personalization, which showcases how businesses can effectively engage users.
3.4. Adaptive Interface Design
Adaptive interface design focuses on creating user interfaces that adjust to the needs and preferences of individual users. This design philosophy aims to improve usability and accessibility by considering various factors such as user behavior, device type, and environmental context.
- Responsive layouts: Interfaces that adapt to different screen sizes and orientations ensure a seamless experience across devices, from desktops to smartphones. Rapid Innovation employs advanced design techniques to create responsive layouts that enhance user engagement across all platforms.
- User preferences: Systems can learn from user interactions to modify layouts, colors, and functionalities, making the interface more intuitive and user-friendly. Our AI solutions enable businesses to gather insights on user preferences, allowing for continuous improvement of the interface design.
- Context-aware features: Adaptive interfaces can change based on the user's environment, such as switching to a dark mode in low-light conditions or simplifying navigation for users with disabilities. Rapid Innovation's expertise in AI and user experience design ensures that our clients can offer context-aware features that enhance usability.
- Benefits of adaptive design include increased user satisfaction, reduced cognitive load, and improved task completion rates. By implementing adaptive interface design, we help clients achieve their business goals more efficiently.
3.5. Predictive User Needs
Predictive user needs involve anticipating what users may require or desire based on their past behavior and contextual information. This proactive approach can significantly enhance user experience by providing solutions before users even realize they need them.
- Data analysis: By analyzing user data, businesses can identify patterns and trends that inform predictions about future behavior. Rapid Innovation utilizes advanced AI analytics to help clients make data-driven decisions that enhance user engagement.
- Contextual awareness: Understanding the context in which users interact with a product or service allows for more accurate predictions, such as suggesting relevant content based on location or time of day. Our solutions incorporate contextual awareness to deliver timely and relevant recommendations.
- Automation: Predictive systems can automate tasks, such as filling out forms or suggesting next steps, streamlining the user journey and reducing friction. Rapid Innovation's AI-driven automation solutions help clients improve operational efficiency and user satisfaction.
- Examples include virtual assistants that suggest actions based on previous queries, e-commerce platforms that recommend products based on browsing history, and content platforms that curate articles based on user interests. Our expertise in AI enables us to implement these predictive solutions effectively, driving greater ROI for our clients. Real time personalization examples illustrate the effectiveness of these strategies in enhancing user engagement and satisfaction.
3.6. Emotional Intelligence in Interactions
Emotional intelligence (EI) plays a crucial role in enhancing interactions between users and personalization agents. It refers to the ability to recognize, understand, and manage emotions in oneself and others. In the context of technology, particularly in AI and personalization agents, emotional intelligence in personalization agents can significantly improve user experience and engagement.
- Understanding User Emotions: Personalization agents equipped with emotional intelligence can analyze user inputs, tone, and context to gauge emotional states. This understanding allows agents to respond more appropriately, fostering a more human-like interaction.
- Tailored Responses: By recognizing emotions, agents can tailor their responses to suit the user's emotional state. For instance, if a user expresses frustration, the agent can respond with empathy and provide solutions that address the user's concerns.
- Building Trust: Emotional intelligence helps in building trust between users and agents. When users feel understood and valued, they are more likely to engage with the technology. This trust can lead to increased user satisfaction and loyalty.
- Enhancing User Engagement: Personalization agents that exhibit emotional intelligence can create a more engaging experience. By responding to users in a way that acknowledges their feelings, agents can keep users interested and invested in the interaction.
- Continuous Learning: Emotional intelligence in agents can evolve over time. By learning from past interactions, agents can improve their understanding of user emotions, leading to more effective and personalized interactions in the future.
4. Technical Architecture of Personalization Agents

The technical architecture of personalization agents is fundamental to their functionality and effectiveness. This architecture encompasses various components that work together to deliver personalized experiences to users.
- Data Collection: Personalization agents gather data from multiple sources, including user interactions, preferences, and behaviors. This data is essential for understanding user needs and tailoring experiences accordingly.
- Data Processing: Once data is collected, it undergoes processing to extract meaningful insights. This involves using algorithms and machine learning techniques to analyze user data and identify patterns.
- User Profiling: Personalization agents create user profiles based on the processed data. These profiles help agents understand individual preferences, interests, and behaviors, enabling them to deliver customized content and recommendations.
- Recommendation Engine: A core component of personalization agents is the recommendation engine. This engine uses algorithms to suggest products, services, or content based on user profiles and historical data. It plays a vital role in enhancing user experience by providing relevant suggestions.
- Feedback Loop: Personalization agents incorporate a feedback loop to continuously improve their recommendations. By analyzing user responses to suggestions, agents can refine their algorithms and enhance the accuracy of future recommendations.
4.1. Agent Design Principles
Designing effective personalization agents requires adherence to specific principles that ensure they meet user needs and expectations. These principles guide the development process and contribute to the overall success of the agents.
- User-Centric Design: The design of personalization agents should prioritize user needs and preferences. Understanding the target audience and their behaviors is crucial for creating agents that resonate with users.
- Transparency: Users should be aware of how their data is being used and how personalization works. Transparency builds trust and encourages users to engage with the agent more openly.
- Adaptability: Personalization agents should be adaptable to changing user preferences and behaviors. This flexibility allows agents to remain relevant and effective over time.
- Consistency: Consistent interactions help users develop familiarity with the agent. Maintaining a consistent tone, style, and approach in responses enhances user experience and satisfaction.
- Ethical Considerations: Designers must consider ethical implications when developing personalization agents. This includes ensuring user privacy, data security, and avoiding biases in recommendations.
- Performance Optimization: Personalization agents should be designed for optimal performance. This includes minimizing response times and ensuring that the agent can handle multiple user interactions simultaneously.
- Continuous Improvement: The design process should incorporate mechanisms for continuous improvement. Regular updates and refinements based on user feedback and technological advancements are essential for keeping the agent effective and relevant.
At Rapid Innovation, we leverage our expertise in AI and blockchain to develop personalization agents that embody these principles, ensuring that our clients achieve greater ROI through enhanced user engagement and satisfaction. By integrating emotional intelligence in personalization agents into our solutions, we help businesses foster deeper connections with their customers, ultimately driving loyalty and revenue growth.
4.2. Data Collection and Integration
Data collection and integration are critical components in the development of any data-driven project. The quality and relevance of the data collected directly influence the performance of machine learning models, which is where Rapid Innovation excels in providing tailored solutions to enhance your business outcomes.
- Types of Data Sources: Data can be collected from various sources, including:
- Structured data from databases.
- Unstructured data from social media, emails, and documents.
- Semi-structured data from XML and JSON files.
- Data Collection Methods: Common methods for data collection include:
- Surveys and questionnaires.
- Web scraping.
- APIs for accessing third-party data, including salesforce data collection.
- Data Integration Techniques: Integrating data from multiple sources can be challenging. Techniques include:
- ETL (Extract, Transform, Load) processes to consolidate data.
- Data warehousing for centralized storage.
- Data lakes for storing large volumes of raw data.
- Challenges in Data Collection and Integration:
- Data quality issues, such as missing or inconsistent data. For more insights on this topic, check out the critical role of data quality in AI implementations.
- Ensuring data privacy and compliance with regulations like GDPR.
- Handling data from disparate sources with different formats, including collection data types in salesforce.
- Tools and Technologies: Various tools can facilitate data collection and integration, such as:
- Apache NiFi for data flow automation.
- Talend for data integration.
- Microsoft Power BI for data visualization and reporting.
At Rapid Innovation, we leverage these tools and techniques to ensure that your data is not only collected efficiently but also integrated seamlessly, leading to improved machine learning model performance and ultimately greater ROI. Our expertise in data collection and integration, including salesforce data collection, ensures that your business can harness the full potential of its data assets. For more information on how we can assist you with adaptive AI development, visit our Adaptive AI Development page.
4.3. Machine Learning Model Architectures
Machine learning model architectures define how algorithms process data to make predictions or decisions. The choice of architecture can significantly impact the model's effectiveness, and Rapid Innovation specializes in selecting the right architecture tailored to your specific business needs.
- Types of Machine Learning Models:
- Supervised Learning: Models learn from labeled data (e.g., regression, classification).
- Unsupervised Learning: Models identify patterns in unlabeled data (e.g., clustering, dimensionality reduction).
- Reinforcement Learning: Models learn through trial and error to maximize rewards.
- Popular Architectures:
- Neural Networks: Composed of layers of interconnected nodes, suitable for complex tasks like image and speech recognition.
- Decision Trees: Simple yet effective for classification tasks, providing clear decision paths.
- Ensemble Methods: Combine multiple models to improve accuracy (e.g., Random Forest, Gradient Boosting).
- Deep Learning Architectures:
- Convolutional Neural Networks (CNNs): Primarily used for image processing tasks.
- Recurrent Neural Networks (RNNs): Effective for sequential data, such as time series or natural language processing.
- Transformers: State-of-the-art architecture for NLP tasks, known for their attention mechanisms.
- Model Evaluation:
- Metrics such as accuracy, precision, recall, and F1 score are essential for assessing model performance.
- Cross-validation techniques help ensure that models generalize well to unseen data.
By employing the right machine learning model architectures, Rapid Innovation helps clients achieve more accurate predictions and insights, leading to enhanced decision-making and increased ROI.
4.4. Real-time Processing Frameworks
Real-time processing frameworks enable the analysis of data as it is generated, allowing for immediate insights and actions. This capability is crucial for applications requiring timely decision-making, and Rapid Innovation is at the forefront of implementing these solutions for our clients.
- Importance of Real-time Processing:
- Businesses can respond quickly to changing conditions.
- Enhances user experience by providing instant feedback.
- Supports applications like fraud detection, recommendation systems, and IoT analytics.
- Popular Real-time Processing Frameworks:
- Apache Kafka: A distributed streaming platform that handles real-time data feeds.
- Apache Flink: A stream processing framework that provides high throughput and low latency.
- Apache Spark Streaming: Extends Apache Spark for real-time data processing, allowing batch and stream processing in a unified manner.
- Key Features of Real-time Frameworks:
- Scalability: Ability to handle large volumes of data.
- Fault Tolerance: Ensures system reliability and data integrity.
- Low Latency: Processes data with minimal delay, crucial for real-time applications.
- Use Cases:
- Financial services for real-time fraud detection.
- E-commerce for personalized recommendations based on user behavior.
- Smart cities for monitoring traffic and environmental conditions.
- Integration with Machine Learning:
- Real-time frameworks can be integrated with machine learning models to enable predictive analytics.
- Stream processing allows for continuous model training and updating based on incoming data.
By focusing on data collection and integration, machine learning model architectures, and real-time processing frameworks, organizations can build robust systems that leverage data effectively for decision-making and operational efficiency. Rapid Innovation is committed to guiding you through this journey, ensuring that your investments yield the highest returns.
4.5. Cross-Platform Compatibility
Cross-platform compatibility refers to the ability of software or applications to function seamlessly across different operating systems and devices. This is increasingly important in today's digital landscape, where users expect to access services from various platforms, including desktops, tablets, and smartphones.
- Enhances user experience by allowing seamless transitions between devices.
- Increases the potential user base, as applications can reach users on different platforms.
- Reduces development costs by allowing a single codebase to be used across multiple platforms.
- Facilitates easier updates and maintenance, as changes can be implemented universally.
- Promotes collaboration and integration with other applications and services, enhancing functionality.
To achieve cross-platform compatibility, developers often use frameworks such as React Native
, Flutter
, or Xamarin
. These tools allow for the creation of applications that can run on multiple operating systems without significant modifications. Additionally, adhering to web standards and utilizing responsive design principles can further enhance compatibility across devices.
At Rapid Innovation, we leverage our expertise in AI and Blockchain to develop cross-platform solutions that not only meet client needs but also maximize return on investment (ROI). For instance, by utilizing a single codebase, we can reduce development time and costs, allowing clients to allocate resources more effectively and achieve faster time-to-market. You can learn more about our approach to cross-platform solutions in our article on NFT marketplace development and overcoming challenges.
4.6. Security and Privacy Mechanisms
In an era where data breaches and privacy concerns are rampant, implementing robust security and privacy mechanisms is crucial for any application or system. These mechanisms protect sensitive information and ensure user trust.
- Encryption: Data should be encrypted both in transit and at rest to prevent unauthorized access. This includes using protocols like
HTTPS
for web applications. - Authentication: Strong authentication methods, such as multi-factor authentication (MFA), help verify user identities and reduce the risk of unauthorized access.
- Regular updates: Keeping software up-to-date with the latest security patches is essential to protect against vulnerabilities.
- Data minimization: Collect only the necessary data from users to limit exposure in case of a breach.
- User education: Informing users about best practices for maintaining their privacy and security can empower them to protect their information.
Implementing these security measures not only safeguards user data but also enhances the overall reputation of the application or service. Compliance with regulations such as GDPR or CCPA is also vital to ensure that user privacy is respected and maintained.
At Rapid Innovation, we integrate advanced security protocols into our AI and Blockchain solutions, ensuring that our clients' applications are not only functional but also secure. This commitment to security not only protects sensitive data but also builds trust with end-users, ultimately leading to greater customer loyalty and increased ROI.
5. Implementation Strategies

Effective implementation strategies are essential for the successful deployment of any project or system. These strategies outline the steps and methodologies that will be used to bring a project from conception to reality.
- Define clear objectives: Establish specific, measurable goals that the project aims to achieve. This helps in maintaining focus and direction throughout the implementation process.
- Develop a detailed plan: Create a comprehensive project plan that outlines timelines, resources, and responsibilities. This should include milestones to track progress.
- Engage stakeholders: Involve all relevant stakeholders early in the process to gather input and ensure that their needs are addressed. This can include team members, clients, and end-users.
- Utilize agile methodologies: Implementing agile practices allows for flexibility and adaptability throughout the project. Regular iterations and feedback loops can lead to continuous improvement.
- Monitor and evaluate: Establish metrics to assess the project's performance and impact. Regularly review these metrics to identify areas for improvement and make necessary adjustments.
By following these implementation strategies, organizations can enhance their chances of success and ensure that their projects meet the intended goals and objectives. At Rapid Innovation, we apply these strategies to our AI and Blockchain projects, ensuring that our clients achieve their business goals efficiently and effectively, leading to a higher return on investment.
5.1. Data Preparation and Normalization
Data preparation and normalization are critical steps in the data processing pipeline, especially in machine learning and data analysis. These processes ensure that the data is clean, consistent, and ready for analysis or model training, ultimately leading to improved decision-making and greater ROI for businesses.
- Data Cleaning: This involves removing inaccuracies, duplicates, and irrelevant information from the dataset. Techniques include:
- Handling missing values through imputation or removal, ensuring that the dataset is complete and reliable.
- Correcting inconsistencies in data formats (e.g., date formats) to maintain uniformity across the dataset.
- Data Transformation: This step modifies the data into a suitable format for analysis. Common techniques include:
- Encoding categorical variables into numerical formats using methods like one-hot encoding or label encoding, which is essential for machine learning algorithms.
- Scaling numerical features to a standard range, often using Min-Max scaling or Z-score normalization, to enhance model performance.
- Normalization: This process adjusts the values in the dataset to a common scale without distorting differences in the ranges of values. Key methods include:
- Min-Max Normalization: Rescales the data to a fixed range, typically [0, 1], which is beneficial for algorithms sensitive to the scale of input data.
- Z-score Normalization: Centers the data around the mean with a standard deviation of 1, making it useful for algorithms that assume a Gaussian distribution.
- Feature Selection: Identifying and selecting the most relevant features for the model can significantly improve performance. Techniques include:
- Filter methods (e.g., correlation coefficients) to assess the relevance of features.
- Wrapper methods (e.g., recursive feature elimination) to evaluate feature subsets based on model performance.
- Embedded methods (e.g., Lasso regression) that incorporate feature selection as part of the model training process.
5.2. User Profiling Techniques
User profiling techniques are essential for understanding user behavior and preferences, which can enhance personalization in applications such as recommendation systems and targeted marketing, ultimately driving higher engagement and conversion rates.
- Demographic Profiling: This involves collecting and analyzing data based on user characteristics such as age, gender, location, and income. It helps in segmenting users into distinct groups for targeted strategies.
- Behavioral Profiling: This technique focuses on user interactions and behaviors, such as:
- Tracking user activity on websites or applications to gather insights into user preferences.
- Analyzing purchase history and browsing patterns to tailor marketing efforts.
- Utilizing clickstream data to understand navigation paths and improve user experience.
- Psychographic Profiling: This approach delves into users' interests, values, and lifestyles. It can be gathered through surveys, social media analysis, and user-generated content. Key aspects include:
- Understanding user motivations and preferences to create more relevant content.
- Segmenting users based on personality traits and lifestyle choices for targeted marketing.
- Contextual Profiling: This technique considers the context in which users interact with a system, including:
- Time of day, location, and device used, which can influence user behavior and preferences.
- Environmental factors that may affect user interactions.
- Machine Learning for Profiling: Advanced algorithms can analyze large datasets to identify patterns and create dynamic user profiles. Techniques include:
- Clustering algorithms (e.g., K-means) to group similar users for targeted marketing.
- Classification algorithms to predict user preferences based on historical data, enhancing personalization efforts.
5.3. Agent Training Methodologies
Agent training methodologies are crucial for developing intelligent agents capable of performing tasks autonomously. These methodologies encompass various approaches to ensure agents learn effectively from their environment, leading to improved operational efficiency and cost savings.
- Supervised Learning: This method involves training agents on labeled datasets, where the correct output is provided for each input. Key aspects include:
- Using algorithms like decision trees, support vector machines, and neural networks to build predictive models.
- Evaluating performance through metrics such as accuracy, precision, and recall to ensure model reliability.
- Unsupervised Learning: In this approach, agents learn from unlabeled data, identifying patterns and structures without explicit guidance. Techniques include:
- Clustering methods to group similar data points, which can reveal hidden insights.
- Dimensionality reduction techniques like PCA (Principal Component Analysis) to simplify data representation and enhance interpretability.
- Reinforcement Learning: This methodology focuses on training agents through trial and error, where they learn to make decisions by receiving rewards or penalties based on their actions. Key components include:
- Defining a reward function to guide the agent's learning process, optimizing for desired outcomes.
- Utilizing algorithms like Q-learning and Deep Q-Networks (DQN) for complex environments, enabling agents to adapt to dynamic situations.
- Transfer Learning: This technique allows agents to leverage knowledge gained from one task to improve performance on a different but related task. It is particularly useful in scenarios with limited data. Key benefits include:
- Reducing training time and resource requirements, making it cost-effective.
- Enhancing model performance by utilizing pre-trained models, accelerating deployment.
- Multi-Agent Systems: In this approach, multiple agents interact and learn from each other, which can lead to more robust and adaptable systems. Key considerations include:
- Designing communication protocols for agents to share information, fostering collaboration.
- Implementing cooperative or competitive learning strategies to achieve common goals, enhancing overall system performance.
By leveraging these methodologies, Rapid Innovation can help clients optimize their AI and blockchain solutions, driving greater ROI and achieving business objectives efficiently and effectively.
5.4. Deployment Models
Deployment models refer to the various ways in which personalization technologies, such as personalized artificial intelligence and ai driven personalization, can be implemented and utilized across different platforms. These models are crucial for businesses aiming to enhance user experience and engagement through tailored content and services. The choice of deployment model can significantly impact the effectiveness of personalization strategies.
- Cloud-based solutions offer scalability and flexibility, allowing businesses to adapt quickly to changing demands and user preferences.
- On-premises solutions provide greater control over data and security, ensuring compliance with regulations and safeguarding sensitive information.
- Hybrid models combine both cloud and on-premises elements for a balanced approach, enabling organizations to leverage the benefits of both environments.
5.4.1. Web-Based Personalization
Web-based personalization involves customizing the online experience for users based on their behavior, preferences, and demographics. This approach is essential for businesses looking to improve user engagement and conversion rates on their websites. Key aspects of web-based personalization include dynamic content delivery, user segmentation, and A/B testing.
- Dynamic content delivery: Websites can change content in real-time based on user interactions, enhancing relevance and engagement.
- User segmentation: Visitors can be grouped based on behavior, allowing for targeted marketing strategies that resonate with specific audiences.
- A/B testing: Different versions of web pages can be tested to determine which performs better in terms of user engagement, optimizing the user experience.
Web-based personalization can be achieved through various techniques, including cookies, user accounts, and machine learning algorithms.
- Cookies: Tracking user behavior to deliver personalized content, ensuring that users receive relevant information.
- User accounts: Allowing users to create profiles that store their preferences, facilitating a more tailored experience.
- Machine learning algorithms: Analyzing data to predict user needs and preferences, enabling proactive engagement strategies.
The effectiveness of web-based personalization is supported by statistics. For instance, personalized web experiences can lead to a 20% increase in sales. Additionally, 74% of online consumers feel frustrated when content is not personalized. Companies that use personalization technologies often see significant improvements in user engagement.
5.4.2. Mobile Application Approaches
Mobile application approaches to personalization focus on delivering tailored experiences through mobile devices. With the increasing use of smartphones, businesses must adapt their personalization strategies to cater to mobile users effectively. Important features of mobile application personalization include location-based services, push notifications, and in-app recommendations.
- Location-based services: Apps can provide personalized content based on the user's geographical location, enhancing relevance and user engagement.
- Push notifications: Targeted messages can be sent to users based on their behavior and preferences, driving user interaction and retention.
- In-app recommendations: Personalized suggestions can enhance user engagement within the app, leading to increased usage and satisfaction.
Key techniques for mobile application personalization include user behavior tracking, user profiles, and integration with social media.
- User behavior tracking: Analyzing how users interact with the app to tailor experiences, ensuring that content remains relevant.
- User profiles: Allowing users to set preferences that influence the content they receive, creating a more personalized experience.
- Integration with social media: Leveraging social data to enhance personalization efforts, tapping into users' social interactions and preferences.
Mobile personalization is crucial for user retention and engagement. Research indicates that personalized push notifications can increase app engagement by up to 88%. Furthermore, 80% of consumers are more likely to make a purchase when brands offer personalized experiences, such as personalized video technology.
By implementing effective web-based and mobile application personalization strategies, businesses can significantly enhance user satisfaction and drive conversions. Rapid Innovation specializes in leveraging personalization technologies, including personalized ai and dynamic yield technology, to optimize these personalization strategies, ensuring that our clients achieve greater ROI through tailored solutions that meet their unique business needs, including virtual reality app development.
5.4.3. Enterprise Solutions
Enterprise solutions refer to comprehensive software systems designed to meet the needs of large organizations, such as enterprise resource planning systems (ERP) and enterprise resource management software. These solutions integrate various business processes and functions, enabling companies to operate more efficiently and effectively.
- Streamlined Operations: Enterprise solutions help in automating and streamlining business processes, reducing manual intervention and errors. For instance, Rapid Innovation can implement AI-driven automation tools that minimize human error and enhance productivity, particularly in ERP systems.
- Enhanced Collaboration: These systems facilitate better communication and collaboration among different departments, ensuring that everyone is on the same page. By leveraging blockchain technology, Rapid Innovation can create secure and transparent communication channels that foster teamwork, especially in enterprise messaging services.
- Data Management: They provide robust data management capabilities, allowing organizations to store, analyze, and retrieve data easily. Rapid Innovation utilizes advanced AI algorithms to enhance data analytics, enabling organizations to derive actionable insights from their data, which is crucial for enterprise resource planning software.
- Scalability: Enterprise solutions are designed to grow with the organization, accommodating increasing data volumes and user numbers without compromising performance. Rapid Innovation ensures that the solutions we provide, including ERP systems, are scalable, allowing businesses to expand seamlessly.
- Customization: Many enterprise solutions offer customization options to tailor the software to specific business needs, ensuring a better fit for the organization. Rapid Innovation works closely with clients to develop bespoke solutions that align with their unique requirements, such as enterprise application platforms.
- Cost Efficiency: By consolidating various functions into a single system, organizations can reduce operational costs and improve resource allocation. Our clients have experienced significant ROI through the implementation of integrated systems, including enterprise resource management systems, that optimize resource use.
5.5. Continuous Learning and Adaptation
Continuous learning and adaptation are crucial for organizations to remain competitive in a rapidly changing business environment. This approach emphasizes the importance of ongoing education and flexibility in processes and strategies.
- Employee Development: Organizations should invest in training programs to enhance employee skills and knowledge, fostering a culture of continuous improvement. Rapid Innovation offers tailored training sessions on AI and blockchain technologies to empower teams, including training on ERP software.
- Feedback Mechanisms: Implementing regular feedback loops allows organizations to assess performance and make necessary adjustments to strategies and processes. Our solutions include tools that facilitate real-time feedback, enhancing responsiveness.
- Agile Methodologies: Adopting agile practices enables teams to respond quickly to changes, ensuring that projects remain aligned with business goals. Rapid Innovation employs agile methodologies in our project management to ensure timely delivery and adaptability.
- Innovation Encouragement: Creating an environment that encourages experimentation and innovation can lead to new ideas and solutions that drive growth. We foster a culture of innovation by integrating cutting-edge technologies into our solutions, including enterprise cloud suites.
- Data-Driven Decisions: Utilizing data analytics helps organizations make informed decisions, adapting strategies based on real-time insights. Rapid Innovation leverages AI to provide predictive analytics, enabling proactive decision-making.
- Knowledge Sharing: Promoting knowledge sharing among employees can enhance collective intelligence and foster a culture of learning. Our platforms facilitate knowledge exchange, ensuring that best practices are shared across teams.
6. Use Cases and Scenarios

Use cases and scenarios are essential tools for understanding how enterprise solutions and continuous learning can be applied in real-world situations. They provide practical examples that illustrate the benefits and applications of these concepts.
- Customer Relationship Management (CRM): A company implements a CRM system to manage customer interactions, leading to improved customer satisfaction and retention rates. Rapid Innovation can develop a customized CRM solution that integrates AI for enhanced customer insights.
- Supply Chain Optimization: An organization uses enterprise solutions to streamline its supply chain processes, resulting in reduced costs and faster delivery times. Our blockchain solutions ensure transparency and traceability in supply chain management.
- Employee Training Programs: A business adopts continuous learning initiatives, offering online courses and workshops, which leads to higher employee engagement and productivity. Rapid Innovation provides e-learning platforms that incorporate AI to personalize learning experiences.
- Agile Project Management: A software development team utilizes agile methodologies to adapt to changing client requirements, improving project outcomes and client satisfaction. Our agile frameworks ensure that projects are delivered on time and within budget.
- Data Analytics for Marketing: A marketing team leverages data analytics to understand customer behavior, allowing for targeted campaigns that increase conversion rates. Rapid Innovation employs AI-driven analytics to optimize marketing strategies.
- Knowledge Management Systems: An organization implements a knowledge management system to facilitate information sharing, enhancing collaboration and innovation across teams. Our solutions utilize blockchain for secure and efficient knowledge sharing, including enterprise resource software and enterprise messaging services.
6.1. E-commerce Personalization
E-commerce personalization refers to the practice of tailoring the shopping experience to individual users based on their preferences, behaviors, and past interactions. This approach enhances customer satisfaction and increases conversion rates, ultimately driving greater ROI for businesses.
- Personalized product recommendations: Utilizing advanced algorithms, we analyze user data to suggest products that align with their interests, significantly increasing the likelihood of purchase. This includes ecommerce personalization strategies that focus on personalized product recommendations for ecommerce.
- Dynamic pricing: Our solutions enable businesses to adjust prices based on user behavior, location, and demand, creating a sense of urgency that can lead to higher sales.
- Customized marketing messages: We help clients tailor emails and advertisements to reflect users' previous purchases or browsing history, enhancing engagement and conversion rates. This is particularly effective in ecommerce personalization examples.
- User experience optimization: Our expertise allows websites to dynamically change layouts and content based on user profiles, making navigation easier and more intuitive, which can lead to increased sales. This is crucial for user experience ecommerce.
- Enhanced customer loyalty: By fostering personalized experiences, we help businesses create deeper connections with customers, encouraging repeat purchases and long-term loyalty. This is especially relevant in b2b ecommerce personalization and ecommerce personalization platform strategies.
According to a study, 80% of consumers are more likely to make a purchase when brands offer personalized experiences. This highlights the importance of ecommerce personalization in driving sales and customer retention, and how Rapid Innovation can facilitate this transformation for our clients.
6.2. Content Recommendation Systems
Content recommendation systems are algorithms designed to suggest relevant content to users based on their preferences and behavior. These systems are widely used in various platforms, including streaming services, news websites, and e-commerce sites, and can significantly enhance user engagement.
- User behavior analysis: By tracking user interactions, our systems can identify patterns and preferences, allowing for more accurate recommendations.
- Collaborative filtering: This technique recommends content based on the preferences of similar users, enhancing the relevance of suggestions and improving user satisfaction.
- Content-based filtering: We utilize characteristics of the content itself, such as genre, keywords, or themes, to make tailored recommendations that resonate with users.
- Real-time updates: As users interact with the platform, our systems can adjust recommendations in real-time to reflect their evolving interests, ensuring ongoing engagement.
- Increased engagement: Personalized content keeps users on the platform longer, leading to higher engagement rates and improved user satisfaction. Research indicates that personalized recommendations can lead to a 10-30% increase in user engagement, making content recommendation systems a vital tool for businesses aiming to enhance user experience.
6.3. Educational Platform Customization
Educational platform customization involves tailoring learning experiences to meet the unique needs of individual learners. This approach enhances engagement and improves learning outcomes, ultimately benefiting educational institutions and learners alike.
- Adaptive learning technologies: Our systems adjust the difficulty and type of content based on the learner's progress and understanding, ensuring a personalized learning journey.
- Personalized learning paths: We enable students to follow customized curricula that align with their interests and career goals, fostering motivation and engagement.
- Interactive assessments: Quizzes and tests can be tailored to focus on areas where the learner needs improvement, providing targeted feedback that enhances learning.
- Resource recommendations: Our platforms can suggest additional materials, such as videos or articles, based on the learner's performance and interests, enriching the educational experience.
- Community engagement: Customization can extend to social features, allowing learners to connect with peers who share similar goals or challenges, fostering a collaborative learning environment.
Studies show that personalized learning can lead to a 30% improvement in student performance, underscoring the effectiveness of educational platform customization in fostering better learning experiences. Rapid Innovation is committed to leveraging AI and blockchain technologies to create tailored solutions that drive success for our clients in the education sector.
6.4. Healthcare User Experiences
Healthcare user experiences are critical in ensuring patient satisfaction and improving health outcomes. A positive user experience in healthcare can lead to better patient engagement, adherence to treatment plans, and overall health improvement.
- User-friendly interfaces: Healthcare platforms should have intuitive designs that make it easy for patients to navigate. This includes clear menus, easy access to information, and straightforward appointment scheduling. Rapid Innovation specializes in developing user-centric interfaces that enhance usability, ensuring that patients can easily access the care they need. This is particularly important in healthcare user experience and healthcare user experience design.
- Telehealth services: The rise of telehealth has transformed how patients interact with healthcare providers. Virtual consultations offer convenience and accessibility, especially for those in remote areas. Our expertise in AI-driven telehealth solutions enables healthcare providers to deliver seamless virtual care, improving patient satisfaction and expanding their reach. This is a key aspect of ux design in healthcare.
- Patient education: Providing educational resources through user-friendly platforms can empower patients. This includes access to articles, videos, and interactive tools that help them understand their conditions and treatment options. Rapid Innovation can assist in creating engaging educational content that enhances patient knowledge and promotes better health outcomes, contributing to overall healthcare ux.
- Feedback mechanisms: Implementing systems for patients to provide feedback on their experiences can help healthcare providers identify areas for improvement. This can include surveys, suggestion boxes, or direct communication channels. Our solutions leverage AI to analyze feedback data, providing actionable insights that drive continuous improvement in patient care. This aligns with bringing user experience to healthcare improvement.
- Mobile health applications: The use of mobile apps for health tracking, medication reminders, and appointment scheduling enhances user experience. These tools allow patients to take an active role in managing their health. Rapid Innovation develops robust mobile health applications that integrate seamlessly with existing healthcare systems, ensuring a comprehensive approach to patient engagement. This is a vital part of health tech ux and healthcare ui ux design.
6.5. Customer Support Personalization
Personalized customer support is essential for building strong relationships with clients and enhancing their overall experience. Tailoring support to individual needs can lead to increased customer satisfaction and loyalty.
- Data-driven insights: Utilizing customer data to understand preferences and behaviors allows businesses to tailor their support. This can include personalized recommendations and proactive outreach based on past interactions. Rapid Innovation employs advanced analytics to help businesses harness their data for improved customer engagement.
- Omnichannel support: Offering support across multiple channels—such as phone, email, chat, and social media—ensures customers can reach out in their preferred way. Consistency across these channels is key to a seamless experience. Our solutions facilitate omnichannel support, ensuring that customer interactions are cohesive and efficient.
- AI and chatbots: Implementing AI-driven chatbots can provide instant responses to common queries while also allowing for personalized interactions. These tools can learn from customer interactions to improve future responses. Rapid Innovation specializes in developing intelligent chatbots that enhance customer support while reducing operational costs.
- Human touch: While technology plays a significant role, the human element remains crucial. Personalized support from knowledgeable representatives can enhance customer trust and satisfaction. Our training programs equip support teams with the skills needed to deliver exceptional service, blending technology with a personal touch.
- Follow-up communications: After resolving an issue, following up with customers to ensure their satisfaction can demonstrate commitment to their experience. This can include surveys or direct outreach to gather feedback. Rapid Innovation can help automate follow-up processes, ensuring that customers feel valued and heard.
6.6. Entertainment and Media Experiences
The entertainment and media industry is rapidly evolving, with user experiences becoming increasingly important. Engaging and personalized experiences can significantly impact audience retention and satisfaction.
- Streaming services: The rise of streaming platforms has changed how audiences consume media. User-friendly interfaces, personalized recommendations, and curated content enhance the viewing experience. Rapid Innovation can develop tailored streaming solutions that leverage AI to provide personalized content suggestions, increasing viewer engagement.
- Interactive content: Engaging users through interactive content—such as quizzes, polls, and live events—can create a more immersive experience. This encourages audience participation and fosters a sense of community. Our expertise in interactive media solutions can help brands create compelling content that resonates with their audience.
- Multi-device accessibility: Ensuring that content is accessible across various devices—smartphones, tablets, and smart TVs—allows users to enjoy media anytime, anywhere. This flexibility is crucial for modern audiences. Rapid Innovation designs cross-platform solutions that ensure a seamless viewing experience across all devices.
- Social media integration: Leveraging social media to promote content and engage with audiences can enhance user experiences. This includes sharing behind-the-scenes content, hosting live Q&A sessions, and encouraging user-generated content. Our strategies help brands effectively utilize social media to build stronger connections with their audience.
- Personalization algorithms: Utilizing algorithms to analyze user preferences and viewing habits can lead to tailored content recommendations. This not only improves user satisfaction but also increases the likelihood of content discovery. Rapid Innovation's AI-driven algorithms can enhance content delivery, ensuring that users receive recommendations that align with their interests.
7. Advanced AI Personalization Techniques
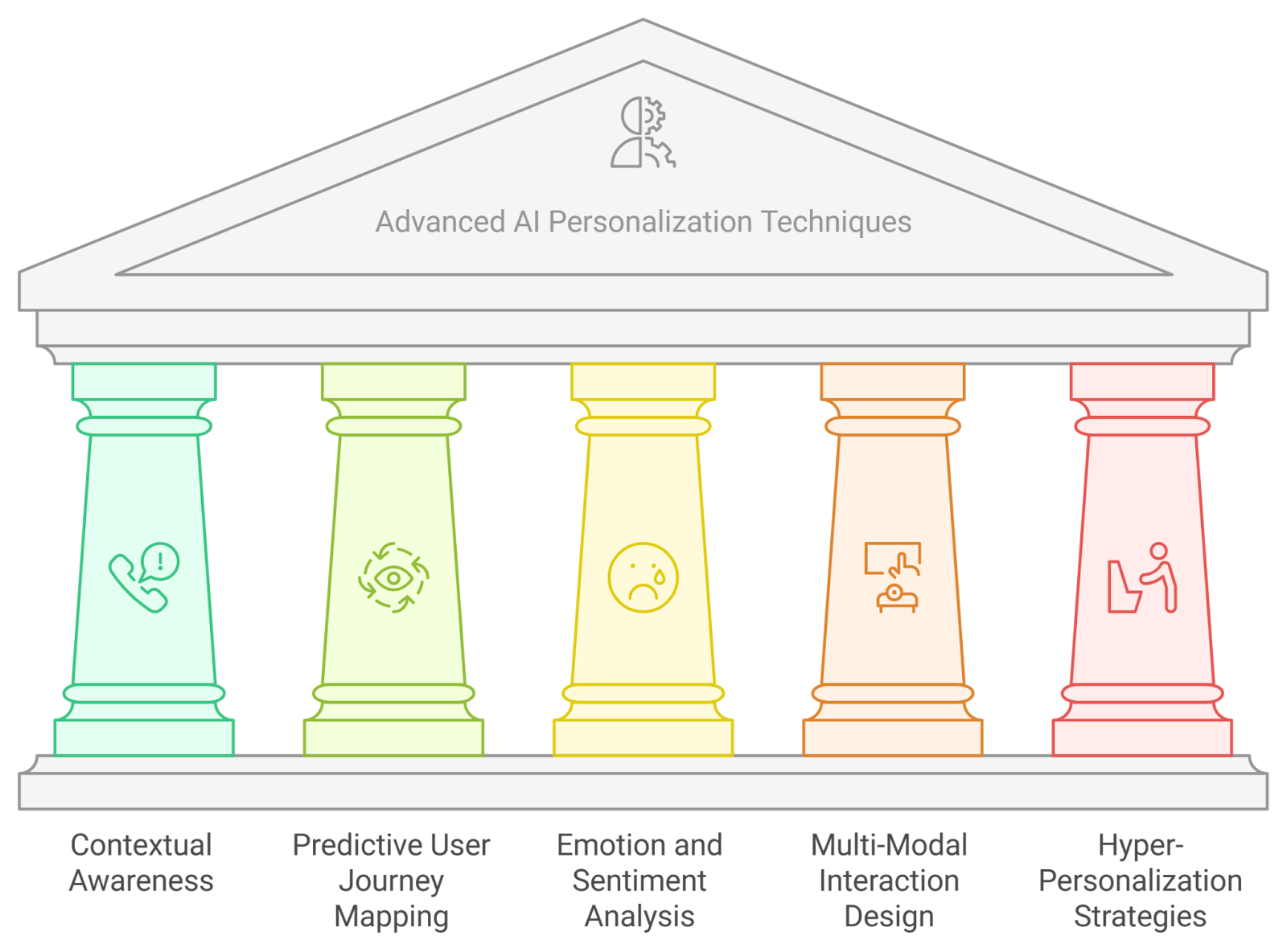
In the realm of digital marketing and user experience, advanced AI personalization techniques are revolutionizing how businesses interact with their customers. These techniques leverage data and machine learning to create tailored experiences that resonate with individual users. Two key components of this approach are contextual awareness and predictive user journey mapping.
7.1 Contextual Awareness
Contextual awareness refers to the ability of AI systems to understand the environment and circumstances surrounding a user’s interaction. This involves analyzing various factors that influence user behavior, such as location, time, device, and user behavior.
- Location: Knowing where a user is can help tailor content and offers relevant to that geographical area.
- Time: Understanding the time of day or season can influence the type of content presented, such as holiday promotions or time-sensitive offers.
- Device: Recognizing the device being used (mobile, tablet, desktop) allows for optimized content delivery and user experience.
- User Behavior: Tracking past interactions, preferences, and browsing history enables AI to provide personalized recommendations.
By integrating these elements, businesses can create a more engaging and relevant experience for users. For instance, a retail app might send a push notification about a sale when a user is near a store, or a travel website could suggest destinations based on the user’s previous searches and current location.
Contextual awareness not only enhances user satisfaction but also increases conversion rates. According to research, personalized experiences can lead to a 20% increase in sales.
7.2 Predictive User Journey Mapping
Predictive user journey mapping is a technique that utilizes AI to forecast the potential paths users may take during their interactions with a brand. This involves analyzing historical data and user behavior patterns to predict future actions. Key aspects include:
- Data Analysis: AI algorithms analyze vast amounts of data to identify trends and patterns in user behavior.
- Segmentation: Users are segmented into different groups based on their behaviors, preferences, and demographics, allowing for targeted marketing strategies.
- Journey Visualization: Creating visual representations of potential user journeys helps businesses understand how users interact with their brand across various touchpoints.
By employing predictive user journey mapping, businesses can proactively address user needs and optimize their marketing strategies. For example, if data indicates that users often abandon their shopping carts at a specific point, businesses can implement strategies to reduce friction at that stage, such as simplifying the checkout process or offering incentives.
This technique not only improves user experience but also enhances customer retention. Studies show that predictive analytics can improve customer retention rates by up to 15%.
In conclusion, advanced AI personalization techniques like contextual awareness and predictive user journey mapping are essential for businesses looking to enhance user engagement and drive conversions. By leveraging these strategies, companies can create more meaningful interactions with their customers, ultimately leading to increased loyalty and sales. At Rapid Innovation, we specialize in implementing these advanced AI personalization techniques, ensuring that our clients achieve greater ROI through enhanced customer experiences and optimized marketing strategies. For more information on best practices regarding AI and data privacy, visit this link.
7.3. Emotion and Sentiment Analysis
Emotion and sentiment analysis is a crucial aspect of understanding user interactions and experiences. This process involves the use of natural language processing (NLP) and machine learning techniques to identify and extract subjective information from text, speech, or other forms of communication.
- Emotion detection helps in recognizing feelings such as happiness, sadness, anger, or fear.
- Sentiment analysis categorizes opinions as positive, negative, or neutral, providing insights into user attitudes.
- Businesses leverage these insights to enhance customer service, improve products, and tailor marketing strategies.
Applications of emotion and sentiment analysis include:
- Social media monitoring to gauge public opinion on brands or products, often utilizing tools like twitter emotion analysis.
- Customer feedback analysis to identify areas for improvement through emotion analysis from text.
- Chatbots and virtual assistants that adapt responses based on user emotions, employing emotional sentiment analysis techniques.
The effectiveness of emotion and sentiment analysis can significantly impact user engagement and satisfaction. By understanding emotional cues, companies can create more empathetic and responsive interactions, leading to better customer relationships. At Rapid Innovation, we utilize advanced AI algorithms to implement emotion and sentiment analysis, including facial recognition sentiment analysis and ibm watson emotion analysis, enabling our clients to gain actionable insights that drive customer satisfaction and loyalty, ultimately enhancing their ROI. For more information, check out our post on voice and sentiment analysis in insurance claims.
7.4. Multi-Modal Interaction Design
Multi-modal interaction design refers to the integration of various input and output modalities in user interfaces. This approach enhances user experience by allowing users to interact with systems through multiple channels, such as voice, touch, gesture, and visual displays.
Key components of multi-modal interaction design include:
- Flexibility: Users can choose their preferred mode of interaction, making technology more accessible.
- Context-awareness: Systems can adapt to the user's environment and situation, providing a more personalized experience.
- Enhanced usability: Combining modalities can reduce cognitive load and improve task efficiency.
Examples of multi-modal interaction include:
- Voice-activated assistants that respond to spoken commands while displaying visual information on screens.
- Smart home devices that allow users to control settings through voice, mobile apps, or physical switches.
- Augmented reality applications that blend digital information with the physical world, enabling intuitive interactions.
By employing multi-modal interaction design, developers can create more engaging and user-friendly experiences that cater to diverse user needs and preferences. Rapid Innovation specializes in designing multi-modal interfaces that enhance user engagement and streamline interactions, leading to improved operational efficiency for our clients.
7.5. Hyper-Personalization Strategies
Hyper-personalization strategies involve tailoring products, services, and experiences to individual users based on their preferences, behaviors, and interactions. This approach goes beyond traditional personalization by leveraging advanced data analytics and artificial intelligence to deliver highly relevant content and recommendations.
Key elements of hyper-personalization include:
- Data collection: Gathering extensive data from various sources, including user behavior, preferences, and demographic information.
- Real-time analytics: Analyzing data in real-time to understand user needs and adapt offerings accordingly.
- Dynamic content delivery: Providing personalized content, product recommendations, and marketing messages that resonate with individual users.
Benefits of hyper-personalization strategies include:
- Increased customer engagement and loyalty through tailored experiences.
- Higher conversion rates as users are more likely to respond to relevant offers.
- Improved customer satisfaction by addressing specific needs and preferences.
Examples of hyper-personalization in action:
- E-commerce platforms that recommend products based on past purchases and browsing history, often informed by emotion analysis from text python.
- Streaming services that curate content suggestions based on viewing habits and preferences.
- Email marketing campaigns that deliver personalized messages based on user interactions and feedback.
By implementing hyper-personalization strategies, businesses can create deeper connections with their customers, ultimately driving growth and success in a competitive market. At Rapid Innovation, we harness the power of AI to develop hyper-personalized solutions that not only meet user expectations but also significantly enhance our clients' return on investment.
8. Challenges and Limitations
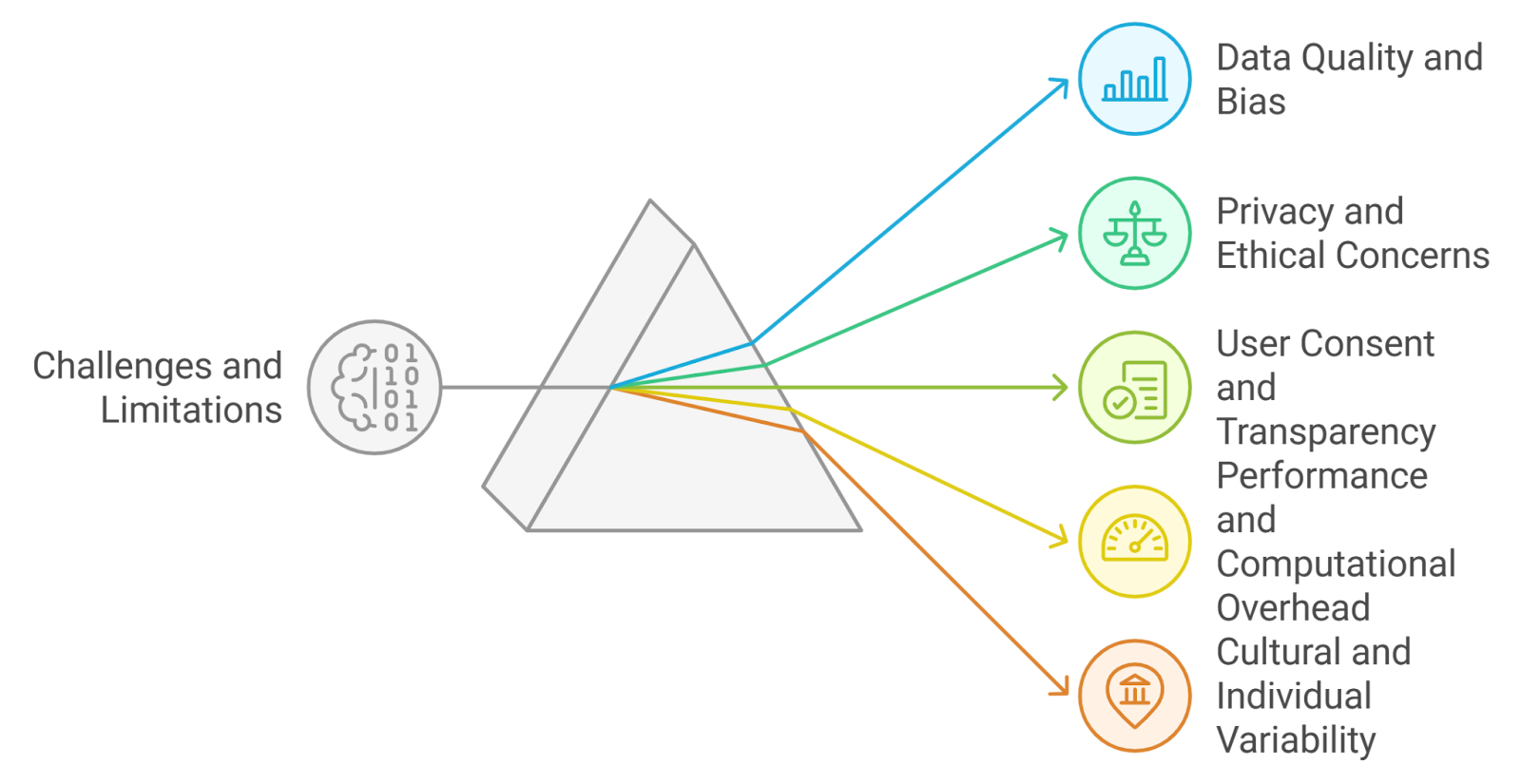
In the rapidly evolving landscape of technology and data analytics, several challenges and limitations can hinder progress and effectiveness. Understanding these challenges is crucial for organizations and individuals looking to leverage data-driven insights.
8.1 Data Quality and Bias
Data quality is a fundamental aspect of any data-driven initiative. Poor data quality can lead to inaccurate conclusions and misguided decisions. Key factors affecting data quality include:
- Inaccurate Data: Data can be incorrect due to human error, outdated information, or faulty data collection methods, which can skew results and lead to poor decision-making.
- Inconsistent Data: Variations in data formats, definitions, and collection methods can create inconsistencies that complicate analysis. For example, different departments may use different metrics for the same data point.
- Incomplete Data: Missing data can result in gaps that affect the overall analysis. Incomplete datasets can lead to biased outcomes, as the analysis may not represent the entire population.
Data quality challenges, such as data quality problems in data mining, are another significant concern, manifesting in various ways, including:
- Sampling Bias: If the data collected is not representative of the entire population, the results may be skewed. For instance, surveys conducted only among a specific demographic may not reflect the views of the broader population.
- Confirmation Bias: Analysts may unintentionally favor data that supports their preconceived notions, leading to selective reporting and analysis.
- Algorithmic Bias: Machine learning algorithms can perpetuate existing biases present in the training data, resulting in unfair or discriminatory outcomes. For example, facial recognition technology has been shown to have higher error rates for individuals with darker skin tones.
Addressing data quality and bias requires a multi-faceted approach, including:
- Implementing robust data governance frameworks.
- Regularly auditing data sources for accuracy and completeness.
- Training staff on recognizing and mitigating bias in data collection and analysis.
At Rapid Innovation, we assist organizations in establishing these frameworks and conducting thorough audits, ensuring that the data driving their AI and blockchain solutions is both accurate and unbiased, ultimately leading to greater ROI. It is essential to list and describe the most common data quality problems to effectively tackle these issues.
8.2 Privacy and Ethical Concerns
As data collection and analysis become more pervasive, privacy and ethical concerns have emerged as critical challenges. Organizations must navigate these issues carefully to maintain trust and comply with regulations. Key considerations include:
- Data Privacy: With the rise of big data, personal information is often collected without explicit consent, raising concerns about how data is used, stored, and shared. Regulations like the General Data Protection Regulation (GDPR) in Europe impose strict guidelines on data handling, requiring organizations to prioritize user privacy.
- Informed Consent: Obtaining informed consent from individuals before collecting their data is essential. However, many users may not fully understand the implications of consent agreements, leading to ethical dilemmas.
- Data Security: Protecting sensitive data from breaches is paramount. High-profile data breaches have highlighted vulnerabilities in data security practices, leading to significant financial and reputational damage for organizations.
- Ethical Use of Data: Organizations must consider the ethical implications of their data usage, ensuring that data-driven decisions do not harm individuals or communities, particularly marginalized groups. For example, predictive policing algorithms can disproportionately target specific demographics, raising ethical concerns about fairness and justice.
To address privacy and ethical concerns, organizations can take several steps:
- Develop clear data privacy policies that comply with relevant regulations.
- Implement strong data security measures, including encryption and access controls.
- Foster a culture of ethical data use by training employees on best practices and ethical considerations.
At Rapid Innovation, we guide our clients in developing comprehensive data privacy policies and implementing robust security measures, ensuring that their AI and blockchain initiatives are not only effective but also ethically sound. By acknowledging and addressing these challenges and limitations, organizations can enhance their data-driven initiatives while maintaining ethical standards and protecting individual privacy. Additionally, we explore the potential of generative AI in automated financial reporting to streamline processes and improve accuracy.
8.3. User Consent and Transparency
User consent and transparency are critical components in the realm of data privacy and ethical technology use. As organizations increasingly rely on data-driven insights, ensuring that users are informed and have control over their data is paramount. User consent is must whenever we collect personal data, and users should be fully aware of what data is being collected, how it will be used, and who it will be shared with. This means providing clear, concise information in user-friendly language. Organizations must decide whether to implement an opt-in or opt-out model for data collection. Opt-in requires users to actively agree to data collection, while opt-out allows data collection by default unless users choose to decline. Clear and accessible privacy policies are essential; these documents should outline data practices and be easily understandable to users, avoiding legal jargon. Users should have the ability to manage their data preferences, including the option to delete their data or withdraw consent at any time. Regular transparency reports can help build trust, detailing data requests from third parties and how user data is handled.
- Informed Consent: Users should be fully aware of what data is being collected, how it will be used, and who it will be shared with. This means providing clear, concise information in user-friendly language.
- Opt-in vs. Opt-out: Organizations must decide whether to implement an opt-in or opt-out model for data collection. Opt-in requires users to actively agree to data collection, while opt-out allows data collection by default unless users choose to decline.
- Privacy Policies: Clear and accessible privacy policies are essential. These documents should outline data practices and be easily understandable to users, avoiding legal jargon.
- User Control: Users should have the ability to manage their data preferences, including the option to delete their data or withdraw consent at any time.
- Transparency Reports: Regular transparency reports can help build trust. These reports should detail data requests from third parties and how user data is handled.
8.4. Performance and Computational Overhead
Performance and computational overhead are significant considerations in the design and implementation of technology solutions. As systems become more complex, understanding their impact on performance is crucial. High computational overhead can lead to increased resource consumption, affecting system performance, including CPU usage, memory consumption, and energy efficiency. Systems with excessive computational demands may experience latency, which can degrade user experience; thus, reducing latency is essential for applications requiring real-time processing. Solutions must be designed to scale efficiently, ensuring that as user demand increases, systems maintain performance without a proportional increase in computational resources. Employing optimization techniques, such as algorithmic improvements and efficient data structures, can help reduce overhead and enhance performance. Regular benchmarking against industry standards can help organizations assess their performance and identify areas for improvement.
- Resource Utilization: High computational overhead can lead to increased resource consumption, affecting system performance. This includes CPU usage, memory consumption, and energy efficiency.
- Latency Issues: Systems with excessive computational demands may experience latency, which can degrade user experience. Reducing latency is essential for applications requiring real-time processing.
- Scalability: Solutions must be designed to scale efficiently. As user demand increases, systems should maintain performance without a proportional increase in computational resources.
- Optimization Techniques: Employing optimization techniques, such as algorithmic improvements and efficient data structures, can help reduce overhead and enhance performance.
- Benchmarking: Regular benchmarking against industry standards can help organizations assess their performance and identify areas for improvement.
8.5. Cultural and Individual Variability
Cultural and individual variability plays a significant role in how technology is perceived and utilized across different demographics. Understanding these differences is essential for creating inclusive and effective technology solutions. Different cultures have varying attitudes towards technology, privacy, and data sharing; recognizing these differences can inform design choices and marketing strategies. Individual preferences can vary widely, influenced by factors such as age, education, and socio-economic status. Tailoring user experiences to accommodate these differences can enhance engagement. Ensuring that technology is accessible to individuals with disabilities or those from diverse backgrounds is crucial, which includes considering language, visual design, and usability. Implementing feedback mechanisms can help organizations understand user experiences across different cultural contexts, leading to more effective adaptations and improvements. As technology becomes increasingly global, understanding cultural nuances is vital for international success; companies must adapt their products and services to meet the needs of diverse user bases.
- Cultural Context: Different cultures have varying attitudes towards technology, privacy, and data sharing. Recognizing these differences can inform design choices and marketing strategies.
- User Preferences: Individual preferences can vary widely, influenced by factors such as age, education, and socio-economic status. Tailoring user experiences to accommodate these differences can enhance engagement.
- Accessibility: Ensuring that technology is accessible to individuals with disabilities or those from diverse backgrounds is crucial. This includes considering language, visual design, and usability.
- Feedback Mechanisms: Implementing feedback mechanisms can help organizations understand user experiences across different cultural contexts. This can lead to more effective adaptations and improvements.
- Globalization: As technology becomes increasingly global, understanding cultural nuances is vital for international success. Companies must adapt their products and services to meet the needs of diverse user bases.
At Rapid Innovation, we leverage our expertise in AI and Blockchain to help organizations navigate these complexities. By implementing robust data governance frameworks, we ensure that user consent and transparency are prioritized, enhancing trust and engagement. Our solutions are designed to optimize performance while minimizing computational overhead, allowing businesses to scale efficiently. Furthermore, we recognize the importance of cultural and individual variability, tailoring our technology solutions to meet the diverse needs of users across different demographics. This holistic approach not only drives greater ROI but also fosters a more inclusive and effective technological landscape.
9. Economic and Strategic Implications
The economic and strategic implications of personalization technologies, such as personalized artificial intelligence and ai driven personalization, are profound, influencing how businesses operate and compete in the marketplace. Understanding these implications can help organizations make informed decisions about investing in personalization strategies.
9.1 ROI of Personalization Technologies
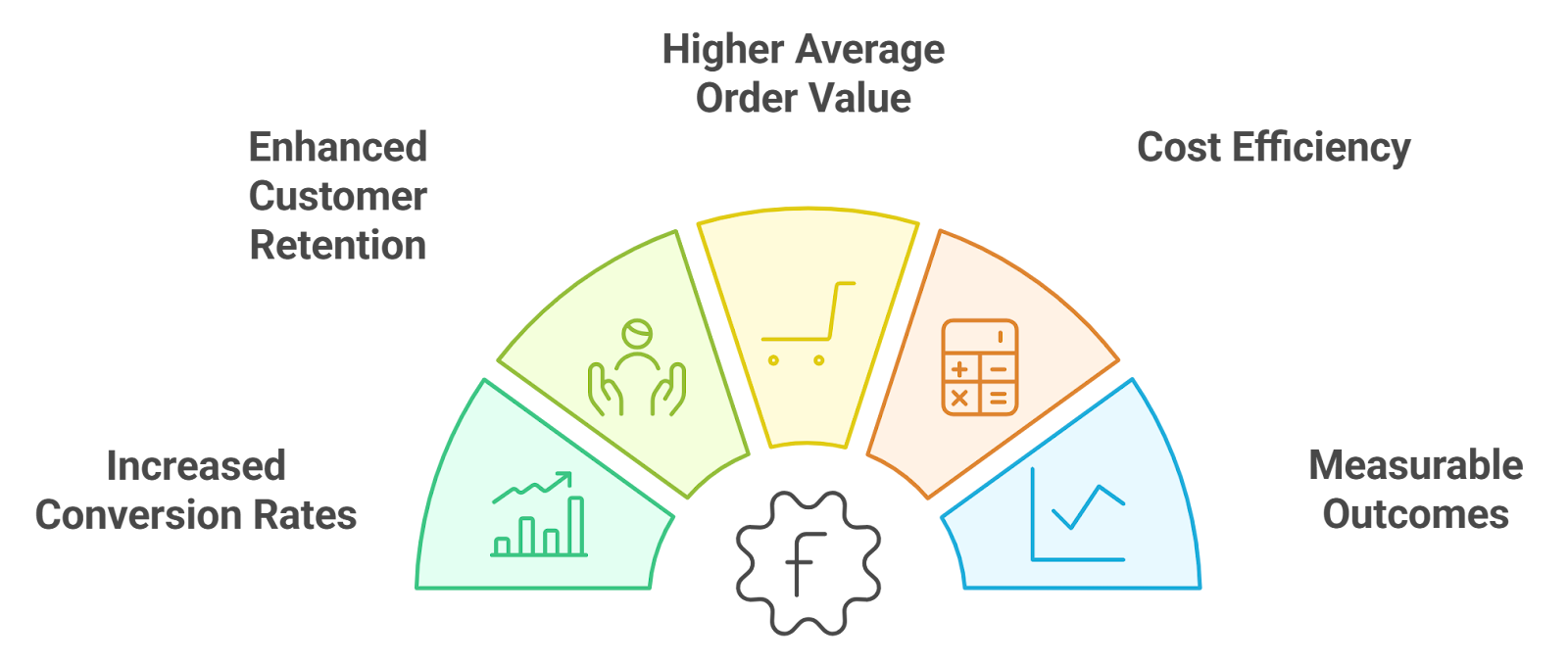
The return on investment (ROI) of personalization technologies is a critical factor for businesses considering their implementation. Personalization can significantly enhance customer engagement, leading to increased sales and customer loyalty.
- Increased Conversion Rates: Personalized experiences can lead to higher conversion rates. Studies show that personalized emails can generate up to 6 times higher transaction rates compared to non-personalized emails.
- Enhanced Customer Retention: Personalization fosters a deeper connection with customers, which can improve retention rates. Retaining existing customers is often more cost-effective than acquiring new ones, making personalization a valuable strategy.
- Higher Average Order Value: Personalized recommendations can encourage customers to spend more. For instance, e-commerce platforms that utilize personalization technologies can see an increase in average order value by up to 30%.
- Cost Efficiency: While there is an initial investment in personalization technologies, the long-term savings from improved customer retention and increased sales can outweigh these costs. Businesses can leverage data analytics to optimize their marketing strategies, reducing wasted expenditure.
- Measurable Outcomes: The effectiveness of personalization can be tracked through various metrics, such as customer lifetime value (CLV) and return on marketing investment (ROMI). This data allows businesses to adjust their strategies in real-time for maximum impact.
9.2 Competitive Advantage Analysis
In today's competitive landscape, personalization technologies can provide a significant edge over competitors. Companies that effectively implement personalization strategies, including personalization technologies and dynamic yield technology, can differentiate themselves and capture a larger market share.
- Differentiation: Personalization allows businesses to stand out in a crowded market. By offering tailored experiences, companies can create unique value propositions that resonate with their target audience.
- Customer Insights: Utilizing personalization technologies enables businesses to gather valuable customer data. This data can inform product development, marketing strategies, and customer service improvements, leading to a more customer-centric approach.
- Brand Loyalty: Personalized experiences can foster brand loyalty. When customers feel understood and valued, they are more likely to return to a brand, reducing churn rates and increasing lifetime value.
- Agility in Marketing: Companies that leverage personalization can respond quickly to changing customer preferences and market trends. This agility allows them to stay ahead of competitors who may be slower to adapt.
- Enhanced Customer Experience: Personalization improves the overall customer experience, leading to positive word-of-mouth and referrals. Satisfied customers are more likely to share their experiences, further enhancing a brand's reputation.
In conclusion, the economic and strategic implications of personalization technologies are significant. By understanding the ROI and competitive advantages associated with these technologies, such as companies that use personalization technologies and omnichannel personalization technology, businesses can make informed decisions that drive growth and success in an increasingly personalized marketplace. At Rapid Innovation, we specialize in harnessing personalized ai and personalization technology examples to implement effective personalization strategies, ensuring that our clients achieve greater ROI and maintain a competitive edge in their respective industries.
9.3. User Retention and Engagement
User retention and engagement are critical components of a successful business strategy, particularly in the digital landscape. Retaining users is often more cost-effective than acquiring new ones, making it essential to focus on customer retention strategies that enhance user loyalty and interaction.
- Understanding User Behavior: Analyzing user behavior through data analytics can provide insights into what keeps users engaged. Tools like Google Analytics can help track user activity and preferences. At Rapid Innovation, we leverage AI-driven analytics to provide deeper insights into user behavior, enabling businesses to tailor their customer retention management strategies effectively.
- Personalization: Tailoring content and experiences to individual users can significantly boost engagement. Personalized emails, recommendations, and targeted ads can make users feel valued and understood. Our AI solutions can automate and optimize personalization efforts, ensuring that users receive relevant content that resonates with their interests, thereby increasing customer retention.
- Feedback Mechanisms: Implementing feedback loops, such as surveys and reviews, allows users to express their opinions. This not only helps in improving services but also makes users feel involved in the development process. Rapid Innovation can develop blockchain-based feedback systems that ensure transparency and trust in user responses, contributing to effective customer retention programs.
- Loyalty Programs: Rewarding users for their continued engagement can enhance retention. Loyalty programs that offer discounts, exclusive content, or early access to new features can incentivize users to stay. We can help design and implement blockchain-enabled loyalty programs that provide secure and verifiable rewards, enhancing user trust and participation, which are key customer retention tactics.
- Regular Updates and Communication: Keeping users informed about new features, updates, or changes through newsletters or notifications can maintain interest and engagement. Regular communication fosters a sense of community. Our AI tools can automate communication strategies, ensuring timely and relevant updates reach users effectively, which is essential for a successful customer retention plan.
- Community Building: Creating forums or social media groups where users can interact with each other can enhance engagement. A strong community can lead to increased loyalty and user retention. Rapid Innovation can facilitate the development of decentralized platforms that empower users to connect and share experiences securely, showcasing customer retention examples.
9.4. Cost-Benefit Considerations
When evaluating business strategies, cost-benefit analysis is essential to ensure that investments yield positive returns. Understanding the financial implications of user retention and engagement strategies can guide decision-making.
- Cost of Acquisition vs. Retention: Acquiring new customers can be five times more expensive than retaining existing ones. Focusing on customer retention strategies can lead to significant cost savings. Our solutions can help businesses identify and implement cost-effective retention strategies that maximize ROI.
- Lifetime Value (LTV): Calculating the lifetime value of a customer helps in understanding how much can be spent on retention efforts. A higher LTV justifies more investment in user engagement strategies. Rapid Innovation can provide advanced analytics tools to accurately calculate LTV and inform retention investments, which is crucial for improving customer retention.
- Resource Allocation: Assessing the costs associated with various engagement strategies allows businesses to allocate resources effectively. Prioritizing high-impact, low-cost initiatives can maximize returns. Our consulting services can guide businesses in optimizing resource allocation for maximum impact, focusing on customer retention methods.
- Measuring ROI: Establishing metrics to measure the return on investment for retention strategies is crucial. This can include tracking user engagement rates, churn rates, and overall revenue growth. We can implement AI-driven dashboards that provide real-time insights into ROI metrics, enabling data-driven decision-making related to customer retention measures.
- Risk Assessment: Evaluating potential risks associated with different strategies can help in making informed decisions. Understanding market trends and user preferences can mitigate risks. Our expertise in blockchain technology can enhance risk assessment by providing secure and transparent data management, which is vital for customer retention tools.
9.5. Future Investment Strategies
As businesses evolve, so do the strategies for investment in user retention and engagement. Future investment strategies should be adaptable and forward-thinking to meet changing user needs.
- Technology Integration: Investing in advanced technologies, such as artificial intelligence and machine learning, can enhance user experiences. These technologies can provide personalized recommendations and automate customer service. Rapid Innovation specializes in integrating AI solutions that drive user engagement and satisfaction, aligning with customer retention marketing strategies.
- Data-Driven Decisions: Leveraging big data analytics can inform future strategies. Understanding user trends and preferences through data can lead to more effective engagement tactics. Our data analytics services can help businesses harness the power of big data to refine their engagement strategies, including customer retention marketing tactics.
- Omni-Channel Engagement: Future investments should focus on creating a seamless experience across multiple channels. Users expect consistent interactions whether they are on mobile, desktop, or social media. We can develop integrated solutions that ensure a cohesive user experience across all platforms, enhancing customer retention strategies in marketing.
- Sustainability Initiatives: As consumers become more environmentally conscious, investing in sustainable practices can enhance brand loyalty. Companies that prioritize sustainability may attract and retain users who value ethical practices. Rapid Innovation can assist in implementing blockchain solutions that promote transparency in sustainability efforts, contributing to customer retention ideas.
- Continuous Learning and Adaptation: Investing in training and development for teams can ensure that they are equipped to adapt to changing market conditions. A culture of continuous learning can foster innovation in user engagement strategies. Our consulting services can provide tailored training programs that empower teams to leverage AI and blockchain technologies effectively, enhancing customer retention best practices.
- Partnerships and Collaborations: Exploring partnerships with other brands or influencers can expand reach and enhance user engagement. Collaborations can introduce new audiences and create unique experiences for users. Rapid Innovation can facilitate strategic partnerships that leverage our technological expertise to enhance user engagement initiatives, supporting customer acquisition and retention strategies.
10. Emerging Trends and Future Outlook
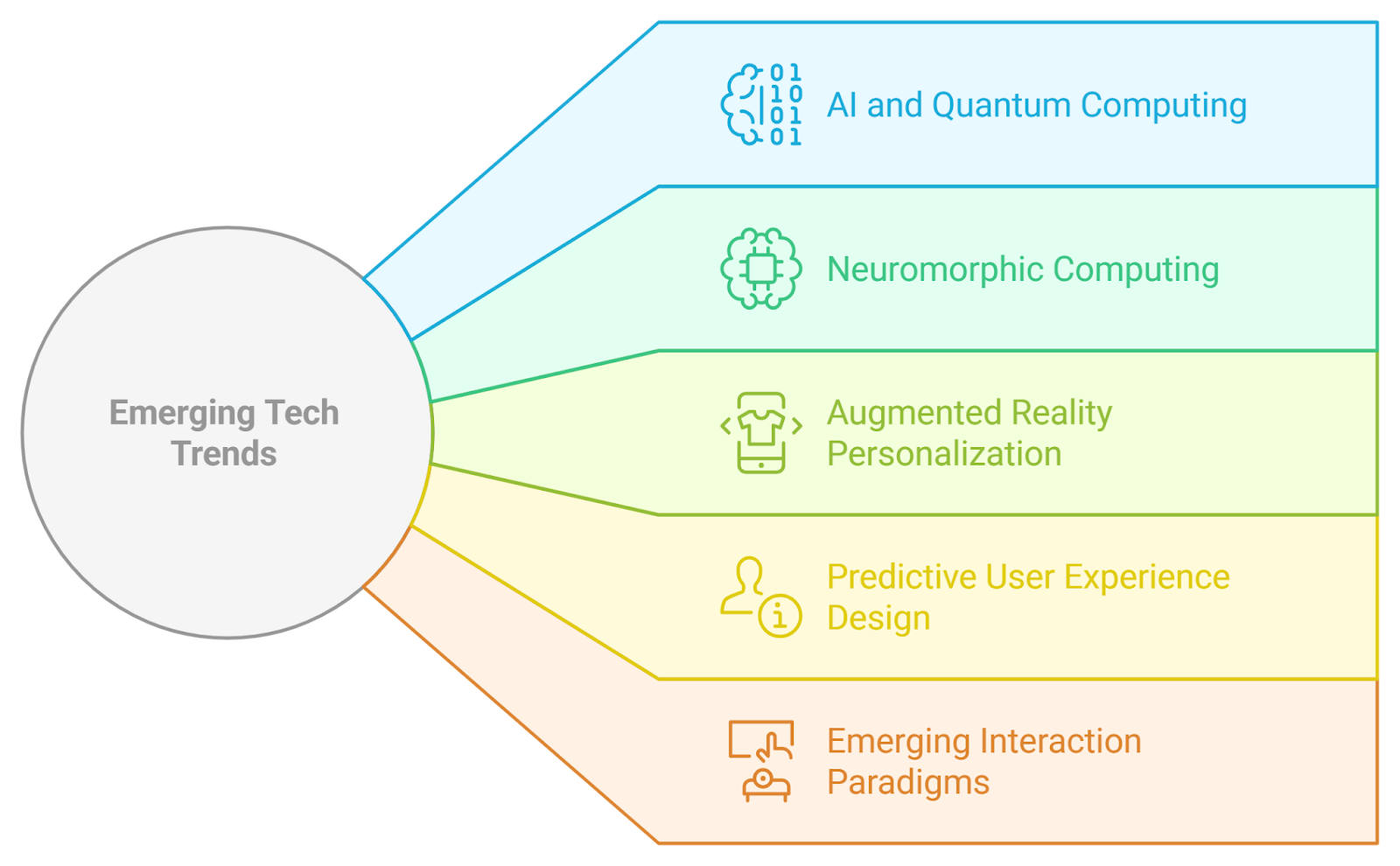
The landscape of technology is constantly evolving, with new trends shaping the future of computing. Two significant areas of development are AI and quantum computing, as well as neuromorphic computing. These technologies promise to revolutionize various sectors, enhancing efficiency and capabilities.
10.1 AI and Quantum Computing
Artificial Intelligence (AI) and quantum computing are two of the most transformative technologies today. Their convergence is expected to unlock unprecedented computational power and capabilities. AI leverages algorithms and data to perform tasks that typically require human intelligence, such as learning, reasoning, and problem-solving. Quantum computing utilizes the principles of quantum mechanics to process information in ways that classical computers cannot, including superposition and entanglement, allowing quantum computers to solve complex problems at incredible speeds.
The integration of AI with quantum computing can lead to enhanced machine learning models that can analyze vast datasets more efficiently, improved optimization algorithms for logistics, finance, and supply chain management, and breakthroughs in drug discovery and materials science by simulating molecular interactions at quantum levels. At Rapid Innovation, we harness these technologies to help our clients achieve greater ROI by developing tailored solutions that leverage the power of AI and quantum computing, ensuring they stay ahead in their respective industries.
As organizations invest in these technologies, the potential for innovation is immense. According to a report by McKinsey, the economic impact of quantum computing could reach $1 trillion by 2035, highlighting its significance in the future of technology.
The future outlook for AI and quantum computing includes:
- Increased collaboration between tech companies and research institutions to develop quantum algorithms tailored for AI applications, such as quantum artificial intelligence and quantum computing for AI.
- Growth in quantum cloud computing services, making quantum resources accessible to a broader audience, including quantum AI cloud services.
- Advancements in quantum error correction, which will enhance the reliability of quantum systems, paving the way for quantum computing and artificial intelligence applications.
10.2 Neuromorphic Computing
Neuromorphic computing is an emerging field that mimics the architecture and functioning of the human brain. This technology aims to create systems that can process information in a way similar to biological neural networks. Neuromorphic chips are designed to handle tasks like perception, learning, and decision-making with high efficiency and low power consumption. These chips utilize spiking neural networks (SNNs), which communicate through discrete spikes, resembling how neurons in the brain transmit information.
The advantages of neuromorphic computing include energy efficiency, as neuromorphic systems consume significantly less power compared to traditional computing architectures, making them ideal for mobile and IoT devices. They also offer real-time processing capabilities, enabling applications in robotics, autonomous vehicles, and smart devices. Additionally, neuromorphic systems can learn and adapt to new information, improving their performance over time without extensive retraining.
At Rapid Innovation, we are committed to exploring the potential of neuromorphic computing to create innovative solutions that drive efficiency and effectiveness for our clients.
The future outlook for neuromorphic computing is promising, with several key developments expected:
- Increased investment from tech giants and startups in neuromorphic hardware and software solutions, including research into quantum AI companies.
- Expansion of applications in fields such as healthcare, where neuromorphic systems can analyze medical images or assist in diagnostics, and quantum computing in AI.
- Research into hybrid systems that combine neuromorphic computing with traditional architectures to leverage the strengths of both.
As these technologies continue to evolve, they will play a crucial role in shaping the future of computing, driving innovation across various industries. Rapid Innovation is poised to guide organizations through this transformation, ensuring they capitalize on these emerging trends to achieve their business goals efficiently and effectively. For more information on how we can assist you, visit our AI software development company in the USA.
10.3. Augmented Reality Personalization
Augmented Reality (AR) personalization is transforming how users interact with digital content by overlaying virtual elements onto the real world. This technology allows businesses to create tailored experiences that resonate with individual preferences and behaviors. AR personalization enhances user engagement by providing immersive experiences, enabling users to visualize products in their environment before making a purchase, and facilitating personalized marketing campaigns that adapt to user interactions. It also utilizes data analytics to understand user preferences and behaviors. Examples include virtual try-ons for clothing and makeup, as well as interactive furniture placement apps.
At Rapid Innovation, we leverage augmented reality personalization to help our clients enhance customer satisfaction and drive sales by creating more informed buying decisions. By implementing AR features similar to those used by companies like IKEA and Sephora, we enable businesses to boost conversion rates and create memorable user experiences. Additionally, we explore how virtual environments improve AR speech recognition systems to enhance user interactions.
10.4. Predictive User Experience Design
Predictive User Experience Design leverages data analytics and machine learning to anticipate user needs and preferences. By analyzing user behavior, designers can create interfaces that adapt in real-time, providing a more intuitive experience. This approach utilizes historical data to predict future user actions, enhances usability by reducing the number of steps needed to complete tasks, increases user satisfaction through personalized content recommendations, and improves accessibility by adapting interfaces for users with different needs. Examples include Netflix's content suggestions and Amazon's product recommendations.
At Rapid Innovation, we harness the power of predictive analytics to streamline user interactions and foster loyalty. By creating a sense of understanding and anticipation, we help our clients deliver sophisticated user experiences that are crucial in today’s competitive landscape.
10.5. Emerging Interaction Paradigms
Emerging interaction paradigms are reshaping how users engage with technology. These new methods of interaction go beyond traditional interfaces, offering innovative ways to connect with digital content. Voice User Interfaces (VUIs) allow users to interact through speech, making technology more accessible. Gesture-based controls enable users to navigate devices with physical movements, enhancing the user experience. Brain-Computer Interfaces (BCIs) are in development, aiming to allow direct communication between the brain and devices. Haptic feedback technology provides tactile responses, making interactions feel more realistic. Examples include smart assistants like Amazon Alexa and gesture-controlled devices like the Microsoft Kinect.
At Rapid Innovation, we are at the forefront of integrating these emerging interaction paradigms into our solutions. By improving accessibility and creating more engaging user experiences, we help our clients stay ahead of the curve in a rapidly evolving technological landscape. As these interaction methods become more prevalent, we ensure that our clients are well-equipped to provide seamless interactions between users and devices.
11. Implementation Roadmap
An implementation roadmap is crucial for ensuring that a project is executed efficiently and effectively. It outlines the steps necessary to achieve the desired outcomes while minimizing risks and maximizing resources. This roadmap typically includes phases such as initial assessment, strategy formulation, pilot program design, and full-scale implementation. Various types of implementation roadmaps, such as safe implementation roadmap, scaled agile implementation roadmap, and power bi implementation roadmap, can be utilized depending on the project's needs.
11.1 Initial Assessment and Strategy
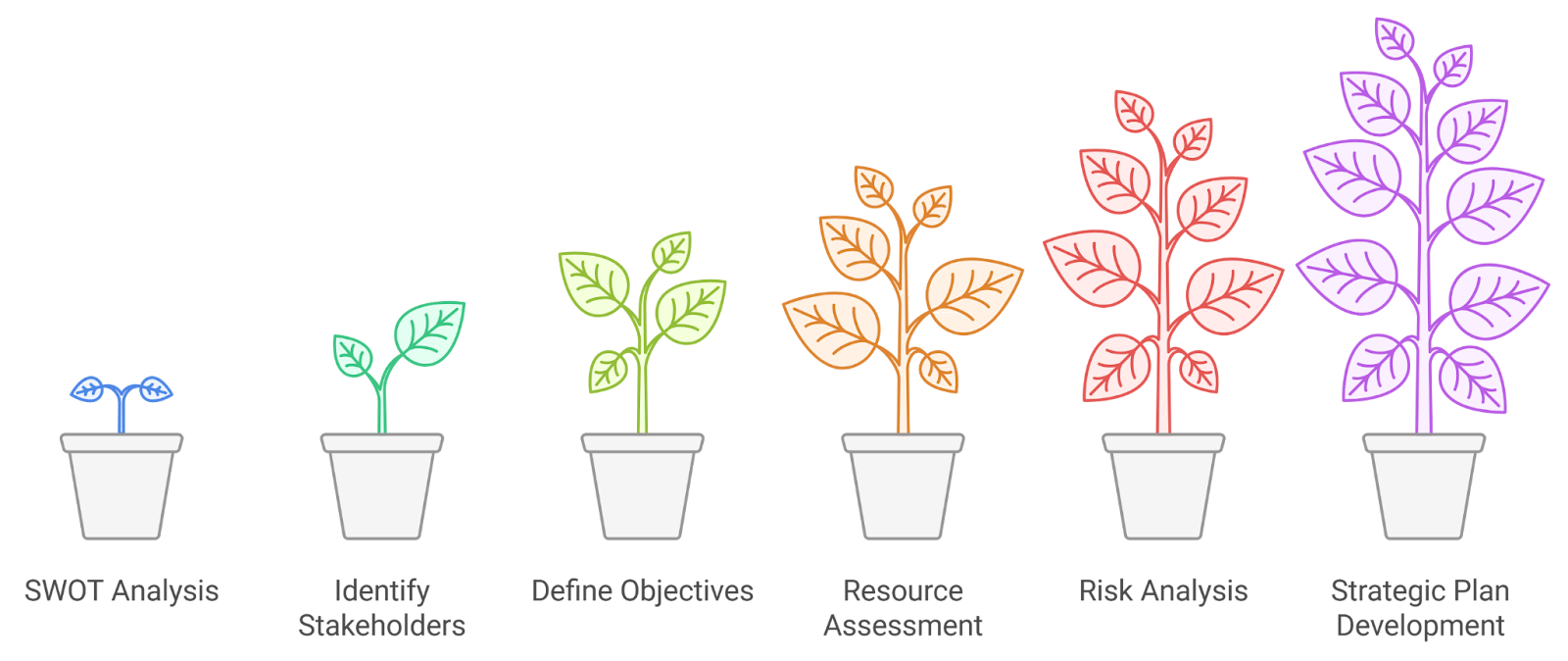
The initial assessment and strategy phase is foundational for any successful implementation. This step involves evaluating the current state of the organization, identifying gaps, and determining the resources required for the project.
- Conduct a SWOT Analysis: Assess the strengths, weaknesses, opportunities, and threats related to the project. This analysis helps in understanding the internal and external factors that could impact the implementation.
- Identify Stakeholders: Recognize all stakeholders involved, including team members, management, and external partners. Engaging stakeholders early ensures their needs and concerns are addressed.
- Define Objectives: Clearly outline the goals of the project. These objectives should be SMART (Specific, Measurable, Achievable, Relevant, Time-bound) to facilitate tracking progress.
- Resource Assessment: Evaluate the resources available, including budget, personnel, and technology. Understanding what is available helps in planning effectively.
- Risk Analysis: Identify potential risks that could hinder the project. Develop mitigation strategies to address these risks proactively.
- Develop a Strategic Plan: Based on the assessment, create a strategic plan that outlines the approach to be taken. This plan should include timelines, milestones, and key performance indicators (KPIs) to measure success. For instance, a scaled agile framework implementation roadmap can be beneficial in structuring this plan.
This phase sets the stage for a successful implementation by ensuring that all aspects are considered and planned for.
11.2 Pilot Program Design
Once the initial assessment and strategy are in place, the next step is to design a pilot program. A pilot program allows organizations to test the proposed solutions on a smaller scale before full implementation.
- Define the Scope: Clearly outline what the pilot program will cover, including specifying the objectives, target audience, and duration of the pilot.
- Select a Test Group: Choose a representative sample of users or stakeholders to participate in the pilot. This group should reflect the diversity of the larger population to ensure the results are applicable.
- Develop Implementation Guidelines: Create detailed guidelines for how the pilot will be executed, including training materials, communication plans, and support resources. This is particularly important for complex implementations like ERP implementation roadmap or Salesforce implementation roadmap.
- Set Evaluation Criteria: Establish metrics to evaluate the success of the pilot program. These metrics should align with the objectives defined in the initial assessment phase.
- Collect Feedback: Implement a system for gathering feedback from participants during and after the pilot. This feedback is invaluable for making necessary adjustments before full-scale implementation.
- Analyze Results: After the pilot concludes, analyze the data collected against the evaluation criteria. This analysis will help determine whether the project is ready for broader implementation or if adjustments are needed.
- Iterate and Improve: Use the insights gained from the pilot to refine the approach. This iterative process ensures that the final implementation is well-informed and more likely to succeed.
Designing a pilot program is a critical step in the implementation roadmap, as it allows organizations to validate their strategies and make informed decisions moving forward. At Rapid Innovation, we leverage our expertise in AI and Blockchain to ensure that each phase of the implementation roadmap, including safe agile implementation roadmap and DevOps implementation roadmap, is tailored to meet the unique needs of our clients, ultimately driving greater ROI and operational efficiency. For accurate project planning and resource allocation, our business roadmap for custom blockchain development.
11.3. Scalability Considerations
Scalability is a critical aspect of any system or process, particularly in technology and business environments. It refers to the ability of a system to handle a growing amount of work or its potential to accommodate growth. Here are some key considerations for scalability:
- Infrastructure: Ensure that the underlying infrastructure can support increased loads. This may involve cloud solutions that allow for dynamic resource allocation, which is essential for AI applications that require significant computational power. Cloud computing provides elastic scalability, which is vital for managing fluctuating workloads.
- Performance Metrics: Establish clear performance metrics to monitor system behavior under varying loads. This helps identify bottlenecks early, allowing for timely adjustments that can enhance the efficiency of blockchain transactions. High scalability in cloud computing is often measured through these metrics.
- Modular Design: Implement a modular architecture that allows for easy addition of resources or components without significant rework. This is particularly beneficial in AI development, where new algorithms or models can be integrated seamlessly. Understanding the meaning of scalability in cloud computing can guide this design.
- Load Balancing: Use load balancing techniques to distribute workloads evenly across servers, enhancing performance and reliability. This is crucial for maintaining the integrity of blockchain networks, where distributed processing is key. Kubernetes scalability can be leveraged to manage containerized applications effectively.
- Database Scalability: Consider database solutions that can scale horizontally (adding more servers) or vertically (upgrading existing servers) to manage increased data loads. This is vital for both AI and blockchain applications, which often deal with large datasets. Horizontal and vertical scalability in cloud computing are essential strategies for database management.
- Testing for Scalability: Regularly conduct stress tests to evaluate how the system performs under peak loads and identify areas for improvement. This proactive approach ensures that both AI models and blockchain systems can handle future growth. Types of scalability in cloud computing should be considered during these tests to ensure comprehensive evaluation. For more insights on AI development, you can refer to this AI development guide for businesses.
11.4. Change Management
Change management is essential for ensuring that changes to processes, systems, or technologies are implemented smoothly and effectively. It involves a structured approach to transitioning individuals, teams, and organizations from a current state to a desired future state. Key aspects include:
- Communication: Clearly communicate the reasons for the change and its benefits to all stakeholders. This helps in gaining buy-in and reducing resistance, particularly when introducing new AI tools or blockchain solutions.
- Training and Support: Provide adequate training and resources to help employees adapt to new systems or processes. Ongoing support is crucial for successful implementation, especially in complex areas like AI and blockchain.
- Stakeholder Involvement: Involve key stakeholders in the change process to gather insights and foster a sense of ownership. This is particularly important in blockchain projects, where stakeholder engagement can influence the success of the implementation.
- Change Impact Assessment: Conduct assessments to understand how changes will affect various aspects of the organization, including workflows and employee roles. This is vital for ensuring that AI integrations align with existing business processes.
- Feedback Mechanisms: Establish channels for feedback during and after the change process to identify issues and make necessary adjustments. Continuous feedback is essential for refining AI models and optimizing blockchain operations.
- Documentation: Maintain thorough documentation of the change process, including plans, training materials, and feedback received, to facilitate future changes.
11.5. Continuous Improvement Framework
A continuous improvement framework is a systematic approach to enhancing processes, products, or services over time. It focuses on incremental improvements rather than large-scale changes. Key components include:
- Plan-Do-Check-Act (PDCA): Utilize the PDCA cycle to plan improvements, implement changes, check results, and act on what has been learned. This iterative process is particularly effective in AI development, where models can be continuously refined.
- Data-Driven Decision Making: Use data analytics to identify areas for improvement and measure the impact of changes. This ensures that decisions are based on evidence rather than assumptions, which is crucial for both AI and blockchain initiatives.
- Employee Involvement: Encourage employees at all levels to contribute ideas for improvement. Their insights can lead to valuable enhancements, particularly in the context of AI applications that benefit from diverse perspectives.
- Regular Reviews: Schedule regular reviews of processes and performance metrics to identify opportunities for improvement. This is essential for maintaining the effectiveness of both AI systems and blockchain networks.
- Benchmarking: Compare performance against industry standards or competitors to identify gaps and set improvement goals. This practice can help organizations stay competitive in the rapidly evolving fields of AI and blockchain.
- Culture of Continuous Improvement: Foster a culture that embraces change and encourages experimentation. This mindset is crucial for sustaining long-term improvements and innovation in AI and blockchain solutions. Understanding scalability and elasticity in cloud computing can further enhance this culture.
12. Ethical Considerations and Governance
In the rapidly evolving landscape of technology, particularly in artificial intelligence (AI) and data analytics, ethical considerations of artificial intelligence and governance are paramount. Organizations must navigate complex issues surrounding user privacy, bias, and accountability to ensure responsible use of technology.
12.1 User Privacy Frameworks

User privacy frameworks are essential for protecting individuals' personal information in an increasingly digital world. These frameworks provide guidelines and standards for how organizations collect, store, and use data.
- Data Protection Regulations: Compliance with regulations such as the General Data Protection Regulation (GDPR) and the California Consumer Privacy Act (CCPA) is crucial. These laws mandate transparency in data collection and give users control over their personal information.
- Consent Management: Organizations should implement clear consent mechanisms that inform users about data usage. This includes explicit opt-in options for data collection, easy-to-understand privacy policies, and options for users to withdraw consent at any time.
- Data Minimization: Organizations should collect only the data necessary for specific purposes. This practice reduces the risk of data breaches and enhances user trust.
- Anonymization Techniques: Employ methods to anonymize data, ensuring that personal identifiers are removed. This allows for data analysis without compromising individual privacy.
- Regular Audits and Assessments: Conduct periodic audits to assess compliance with privacy policies and regulations. This helps identify potential vulnerabilities and areas for improvement.
- User Education: Inform users about their rights and the importance of privacy. Providing resources on how to protect their data can empower users and foster trust.
12.2 Bias Detection and Mitigation
Bias in AI and data analytics can lead to unfair treatment and discrimination. Addressing bias is critical for ethical governance and ensuring equitable outcomes.
- Understanding Bias: Bias can manifest in various forms, including data bias, which arises from unrepresentative training data, and algorithmic bias, which results from flawed algorithms that perpetuate existing stereotypes.
- Diverse Data Sets: Use diverse and representative data sets to train AI models. This helps minimize the risk of bias and ensures that the technology serves all demographic groups fairly.
- Bias Detection Tools: Implement tools and methodologies to detect bias in algorithms. Techniques include statistical analysis to identify disparities in outcomes and auditing algorithms for fairness and transparency.
- Continuous Monitoring: Regularly monitor AI systems for biased outcomes. This involves setting up feedback loops to gather user experiences and adjusting algorithms based on real-world performance and feedback.
- Inclusive Development Teams: Foster diversity within development teams. A diverse team is more likely to recognize and address potential biases in technology.
- Ethical Guidelines and Training: Establish ethical guidelines for AI development and provide training for developers on bias awareness. This ensures that ethical considerations in artificial intelligence are integrated into the design process.
- Stakeholder Engagement: Involve stakeholders, including affected communities, in discussions about bias and fairness. This collaborative approach can lead to more equitable solutions.
By prioritizing user privacy frameworks and bias detection and mitigation strategies, organizations can navigate the ethical considerations in technology responsibly. This not only enhances user trust but also contributes to a more equitable digital environment. At Rapid Innovation, we are committed to helping our clients implement these ethical practices, ensuring that their AI and blockchain solutions are not only effective but also responsible and trustworthy. By integrating these frameworks into your business strategy, we can help you achieve greater ROI while maintaining ethical integrity in your technological advancements, including ethical considerations in cloud computing systems and medical ethics considerations on artificial intelligence.
12.3. Transparency in AI Decisions
Transparency in AI decisions is crucial for building trust and accountability in artificial intelligence systems. As AI technologies become more integrated into various sectors, understanding how these systems make decisions is essential for users and stakeholders. Clear explanations of AI processes help users comprehend how outcomes are derived. Transparency can mitigate biases by allowing stakeholders to scrutinize algorithms and data sources. Additionally, providing insight into decision-making processes can enhance user confidence in AI applications.
At Rapid Innovation, we emphasize the importance of AI transparency and compliance in our AI solutions. By implementing explainable AI (XAI) techniques, we ensure that our clients can clearly understand how models arrive at specific conclusions. We also focus on offering user-friendly interfaces that present decision rationales in understandable terms, making it easier for stakeholders to engage with AI systems. Furthermore, we regularly publish reports on AI performance, including metrics on accuracy, fairness, and bias, to maintain transparency and accountability.
By prioritizing transparency, companies can foster a culture of accountability and ethical responsibility in AI development, ultimately leading to greater trust and improved ROI.
12.4. Regulatory Compliance
Regulatory compliance in AI refers to adhering to laws and guidelines that govern the use of artificial intelligence technologies. As AI continues to evolve, regulatory frameworks are being established to ensure ethical and responsible use. Compliance helps protect user data and privacy, addressing concerns about surveillance and misuse. Organizations must stay informed about local and international regulations, such as the General Data Protection Regulation (GDPR) in Europe. Non-compliance can lead to significant legal repercussions, including fines and reputational damage.
At Rapid Innovation, we assist our clients in navigating the complex landscape of regulatory compliance. Our expertise in data protection ensures that personal data is collected, processed, and stored in accordance with legal standards. We also focus on algorithmic accountability, developing systems that can be audited and assessed for fairness and bias. By maintaining transparency with regulators about AI systems and their impacts, we help organizations enhance their credibility and trustworthiness in the market.
By prioritizing regulatory compliance, organizations can not only avoid legal pitfalls but also enhance their credibility and trustworthiness in the market, leading to a more favorable business environment.
12.5. Ethical Design Principles
Ethical design principles in AI focus on creating systems that prioritize human values and societal well-being. As AI technologies become more pervasive, it is essential to embed ethical considerations into the design and development process. Ethical AI design promotes fairness, accountability, and transparency, ensuring that systems do not perpetuate biases. Incorporating diverse perspectives during the design phase can lead to more inclusive and equitable AI solutions. Continuous evaluation of AI systems is necessary to identify and mitigate potential ethical issues.
At Rapid Innovation, we are committed to ethical design principles in our AI solutions. We prioritize user-centric design, ensuring that the needs and rights of users are at the forefront of our development process. Our focus on fairness drives us to eliminate biases in algorithms, ensuring equitable treatment for all users. Additionally, we establish clear lines of accountability for AI outcomes and decisions, fostering a culture of responsibility.
By adhering to ethical design principles, organizations can create AI systems that not only meet technical requirements but also align with societal values and expectations, ultimately leading to greater user satisfaction and business success.