Table Of Contents
Category
Artificial Intelligence
AIML
IoT
Blockchain
1. Introduction to AI-Driven Troubleshooting
In today's fast-paced technological landscape, the need for efficient troubleshooting methods has never been more critical. AI-driven troubleshooting leverages advanced algorithms and machine learning to enhance diagnostic processes across various industries. This approach not only improves the speed of problem resolution but also increases accuracy, leading to better overall performance. At Rapid Innovation, we harness the power of AI agents for troubleshooting to analyze vast amounts of data quickly, identify patterns and anomalies that may not be visible to human technicians, and reduce downtime and operational costs through their integration in troubleshooting.
1.1 Evolution of Diagnostic Techniques
The evolution of diagnostic techniques has been a journey from manual processes to sophisticated AI-driven systems. Understanding this progression helps in appreciating the current capabilities of AI agents in troubleshooting.
- Manual Troubleshooting: Initially, troubleshooting relied heavily on human expertise. Technicians would diagnose issues based on experience and intuition, often leading to longer resolution times.
- Rule-Based Systems: The introduction of rule-based systems marked a significant shift. These systems used predefined rules to identify problems, but they were limited in scope and adaptability.
- Data-Driven Approaches: With the advent of big data, diagnostic techniques began to incorporate data analytics. This allowed for more informed decision-making, but still required significant human intervention.
- Machine Learning and AI: The latest evolution involves machine learning and AI. These technologies enable systems to learn from past incidents, adapt to new situations, and provide real-time solutions. AI agents can now autonomously troubleshoot issues, significantly reducing the need for human oversight. Rapid Innovation specializes in implementing these advanced technologies to help clients achieve greater efficiency and ROI.
- Predictive Analytics: AI-driven troubleshooting also incorporates predictive analytics, which anticipates potential issues before they occur. This proactive approach minimizes disruptions and enhances system reliability, allowing businesses to maintain operational continuity.
- Natural Language Processing (NLP): NLP allows AI agents to understand and process human language, making it easier for users to interact with troubleshooting systems. This capability enhances user experience and streamlines the troubleshooting process, further contributing to improved operational efficiency.
The evolution of diagnostic techniques illustrates a clear trend towards automation and intelligence, paving the way for more efficient and effective troubleshooting solutions. As AI technology continues to advance, the potential for even more sophisticated diagnostic tools will only grow, further transforming the landscape of troubleshooting. At Rapid Innovation, we are committed to guiding our clients through this transformation, ensuring they leverage AI-driven troubleshooting solutions to meet their business goals effectively.
Refer to the image for a visual representation of the evolution of AI-driven troubleshooting techniques:

.
1.2. Limitations of Traditional Troubleshooting Methods
Traditional troubleshooting methods have been the backbone of problem resolution in various industries for decades. However, they come with several limitations that can hinder efficiency and effectiveness.
- Time-Consuming: Traditional methods often require extensive manual effort, leading to longer resolution times. Technicians may spend hours or even days diagnosing issues.
- Human Error: Reliance on human judgment can lead to mistakes. Misdiagnosis can result in unnecessary repairs or overlooked problems, complicating the resolution process.
- Inconsistent Results: Different technicians may approach the same problem in various ways, leading to inconsistent outcomes. This variability can affect service quality and customer satisfaction.
- Limited Data Utilization: Traditional methods often do not leverage available data effectively. Technicians may rely on experience rather than data-driven insights, missing opportunities for quicker resolutions.
- Scalability Issues: As organizations grow, traditional troubleshooting methods can struggle to keep pace. The manual nature of these methods makes it difficult to scale operations efficiently.
- Reactive Approach: Traditional troubleshooting is often reactive, addressing problems only after they occur. This can lead to increased downtime and lost productivity.
1.3. Role of Artificial Intelligence in Problem Resolution

‍
Artificial Intelligence (AI) is revolutionizing the way organizations approach problem resolution. By integrating AI into troubleshooting processes, companies can enhance efficiency and accuracy.
- Data Analysis: AI can analyze vast amounts of data quickly, identifying patterns and anomalies that may not be visible to human technicians. This capability allows for faster diagnosis and resolution.
- Predictive Maintenance: AI algorithms can predict potential issues before they occur by analyzing historical data and trends. This proactive approach minimizes downtime and reduces the need for reactive troubleshooting.
- Automated Solutions: AI can automate routine troubleshooting tasks, freeing up human technicians to focus on more complex issues. This leads to improved productivity and faster resolution times.
- Natural Language Processing (NLP): AI-powered chatbots and virtual assistants can interact with users in natural language, providing immediate assistance and troubleshooting guidance. This enhances user experience and satisfaction.
- Continuous Learning: AI systems can learn from each interaction, improving their problem-solving capabilities over time. This continuous learning process ensures that AI becomes more effective in resolving issues.
- Integration with IoT: AI can work seamlessly with Internet of Things (IoT) devices, enabling real-time monitoring and troubleshooting. This integration allows for immediate responses to emerging problems.
1.4. Transformative Potential of AI Agents
AI agents hold transformative potential for various industries, particularly in the realm of problem resolution. Their capabilities can significantly enhance operational efficiency and customer satisfaction.
- 24/7 Availability: AI agents can operate around the clock, providing support and troubleshooting assistance at any time. This ensures that issues can be addressed promptly, regardless of time constraints.
- Scalability: AI agents can handle multiple inquiries simultaneously, making them highly scalable. This capability allows organizations to manage increased workloads without compromising service quality.
- Cost Efficiency: By automating routine tasks and reducing the need for extensive human intervention, AI agents can lower operational costs. This cost efficiency can lead to significant savings for organizations.
- Enhanced Customer Experience: AI agents can provide instant responses and solutions, improving the overall customer experience. Quick resolutions lead to higher satisfaction rates and increased loyalty.
- Data-Driven Insights: AI agents can gather and analyze data from interactions, providing valuable insights into customer behavior and preferences. This information can inform future strategies and improvements.
- Collaboration with Human Agents: AI agents can work alongside human technicians, providing them with relevant information and suggestions. This collaboration enhances the problem-solving process and leads to better outcomes.
- Adaptability: AI agents can be trained to adapt to various industries and specific organizational needs. This flexibility allows them to be tailored to different environments, maximizing their effectiveness.
At Rapid Innovation, we leverage these AI troubleshooting solutions to help our clients streamline their troubleshooting processes, ultimately leading to greater ROI and enhanced operational efficiency. By integrating AI business automation solutions, organizations can overcome the limitations of traditional methods and achieve their business goals more effectively. Additionally, exploring AI agents for marketing applications can provide further insights into their capabilities and best practices.
Refer to the image for a visual representation of the limitations of traditional troubleshooting methods.
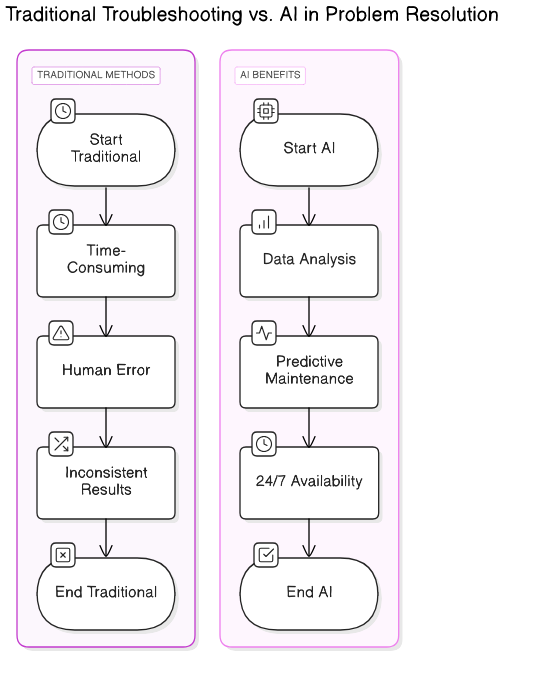
2. Foundational Concepts of AI Troubleshooting Agents
AI troubleshooting agents are designed to assist users in diagnosing and resolving issues within various systems, applications, or devices. Understanding the foundational concepts of these agents is crucial for effective implementation and utilization, especially in a rapidly evolving technological landscape.
2.1 Conceptual Framework
The conceptual framework of AI troubleshooting agents encompasses several key components that define their functionality and effectiveness:
- Problem Identification: The first step involves recognizing the issue at hand. AI troubleshooting agents utilize natural language processing (NLP) to interpret user queries and identify the problem accurately, ensuring that users receive timely assistance.
- Data Collection: Once the problem is identified, the agent gathers relevant data from various sources, including system logs, user inputs, and historical data. This data is essential for diagnosing the issue and is a critical aspect of the value Rapid Innovation brings to its clients.
- Analysis and Diagnosis: AI troubleshooting agents employ machine learning algorithms to analyze the collected data. They compare current data against known patterns and anomalies to diagnose the problem effectively, allowing businesses to minimize downtime and enhance operational efficiency.
- Solution Generation: After diagnosing the issue, the agent generates potential solutions. This may involve suggesting troubleshooting steps, providing relevant documentation, or even automating certain fixes. Rapid Innovation's expertise in AI ensures that these solutions are tailored to meet specific business needs.
- Feedback Loop: A critical aspect of the framework is the feedback mechanism. Users can provide feedback on the effectiveness of the solutions, which helps the AI improve its future performance through continuous learning. This iterative process is vital for maintaining high service quality.
- User Interaction: The design of user interfaces is vital for effective communication between the user and the AI troubleshooting agents. A well-structured interface enhances user experience and ensures that users can easily navigate through troubleshooting steps, a focus area for Rapid Innovation in developing user-centric solutions.
- Integration with Existing Systems: AI troubleshooting agents must be compatible with existing systems and tools. This integration allows for seamless operation and enhances the overall efficiency of the troubleshooting process, aligning with Rapid Innovation's commitment to providing comprehensive solutions.
2.2 Types of Troubleshooting Agents
AI troubleshooting agents can be categorized into several types based on their functionality and application areas:
- Rule-Based Agents: These agents operate on predefined rules and logic. They follow a set of instructions to diagnose and resolve issues. While they are straightforward, their effectiveness is limited to the scenarios they are programmed to handle.
- Machine Learning Agents: These agents leverage machine learning algorithms to learn from past experiences and improve their troubleshooting capabilities over time. They can adapt to new problems and provide more accurate solutions as they gather more data, showcasing the advanced capabilities Rapid Innovation can implement for clients.
- Expert Systems: Expert systems are designed to mimic human expertise in specific domains. They utilize a knowledge base of expert information to diagnose problems and suggest solutions. These systems are particularly useful in complex fields like healthcare and engineering, where Rapid Innovation can provide specialized support.
- Chatbot Agents: Chatbots are conversational agents that interact with users through text or voice. They can guide users through troubleshooting processes, answer questions, and provide solutions in a user-friendly manner. Their ability to engage in natural language makes them accessible to a wide range of users, enhancing customer service capabilities.
- Remote Monitoring Agents: These agents continuously monitor systems and applications for potential issues. They can proactively identify problems before they escalate, allowing for timely intervention and resolution, which is crucial for maintaining business continuity.
- Automated Repair Agents: These agents not only diagnose issues but also implement fixes automatically. They can perform tasks such as restarting services, applying patches, or reconfiguring settings without user intervention, significantly reducing the time and resources required for troubleshooting.
- Hybrid Agents: Combining features from various types of agents, hybrid agents offer a more comprehensive troubleshooting solution. They can utilize rule-based logic for straightforward issues while employing machine learning for more complex scenarios, providing a versatile approach to problem-solving.
Understanding these foundational concepts and types of AI troubleshooting agents is essential for organizations looking to enhance their support systems and improve user satisfaction. By leveraging the right type of agent, businesses can streamline their troubleshooting processes and reduce downtime effectively, ultimately achieving greater ROI with the expertise of Rapid Innovation.
Refer to the image for a visual representation of the foundational concepts of AI troubleshooting agents.

2.2.1. Reactive Diagnostic Agents
Reactive diagnostic agents are systems designed to respond to specific inputs or conditions without the ability to learn from past experiences. These agents operate based on predefined rules and algorithms, making them effective in straightforward diagnostic scenarios.
- Characteristics: Â
- Operate on a "if-then" basis, responding to specific triggers.
- Lack the ability to adapt or learn from previous interactions.
- Often used in environments where conditions are stable and predictable.
- Applications: Â
- Commonly found in automated troubleshooting systems.
- Used in medical diagnostics to identify conditions based on symptoms, such as piflufolastat f 18 diagnostic and diagnostic agents for kidney function.
- Employed in network security to detect known threats.
- Limitations: Â
- Cannot handle unexpected situations or novel problems.
- May lead to false positives or negatives if the predefined rules are not comprehensive.
- Limited scalability as they require constant updates to their rule sets.
At Rapid Innovation, we leverage reactive diagnostic agents to enhance operational efficiency for our clients. By implementing these systems, businesses can streamline their troubleshooting processes, ensuring quick responses to known issues, thereby reducing downtime and improving service delivery. For more information on how we can assist you, visit our AI agent development services.
2.2.2. Predictive Diagnostic Agents
Predictive diagnostic agents utilize data analysis and machine learning techniques to forecast potential issues before they occur. These agents analyze historical data and patterns to identify trends, enabling proactive measures to be taken.
- Characteristics: Â
- Use algorithms to analyze large datasets for predictive insights.
- Capable of identifying potential problems based on historical trends.
- Often incorporate statistical models and machine learning techniques.
- Applications: Â
- Widely used in predictive maintenance for machinery and equipment.
- Employed in healthcare to predict disease outbreaks or patient deterioration, including the use of iodinated diagnostic agents and etarfolatide.
- Utilized in finance for risk assessment and fraud detection.
- Benefits: Â
- Enhance operational efficiency by preventing issues before they arise.
- Reduce costs associated with downtime and repairs.
- Improve decision-making through data-driven insights.
- Limitations: Â
- Require substantial amounts of historical data for accurate predictions.
- May produce inaccurate forecasts if the underlying data is flawed.
- Depend on continuous model updates to remain effective.
At Rapid Innovation, we specialize in developing predictive diagnostic agents that empower our clients to anticipate challenges and optimize their operations. By utilizing these agents, businesses can significantly enhance their ROI through reduced maintenance costs and improved resource allocation.
2.2.3. Adaptive Learning Agents
Adaptive learning agents are advanced systems that can learn from their environment and experiences, adjusting their behavior over time. These agents utilize machine learning algorithms to improve their performance based on feedback and new data.
- Characteristics: Â
- Capable of self-improvement through continuous learning.
- Adapt their strategies based on changing conditions and feedback.
- Utilize reinforcement learning, supervised learning, or unsupervised learning techniques.
- Applications: Â
- Used in personalized learning systems in education to tailor content to individual students.
- Employed in autonomous vehicles to improve navigation and safety.
- Utilized in customer service chatbots that learn from interactions to enhance user experience.
- Benefits: Â
- Provide more accurate and relevant responses over time.
- Enhance user engagement through personalized experiences.
- Improve efficiency by adapting to new challenges and environments.
- Limitations: Â
- Require significant computational resources for training and operation.
- Risk of overfitting to specific datasets, leading to poor generalization.
- Ethical concerns regarding data privacy and decision-making transparency.
Rapid Innovation harnesses the power of adaptive learning agents to create tailored solutions that evolve with our clients' needs. By implementing these agents, businesses can achieve higher levels of customer satisfaction and operational efficiency, ultimately driving greater ROI.
Refer to the image for a visual representation of the concepts discussed in the sections on Reactive, Predictive, and Adaptive Learning Agents:
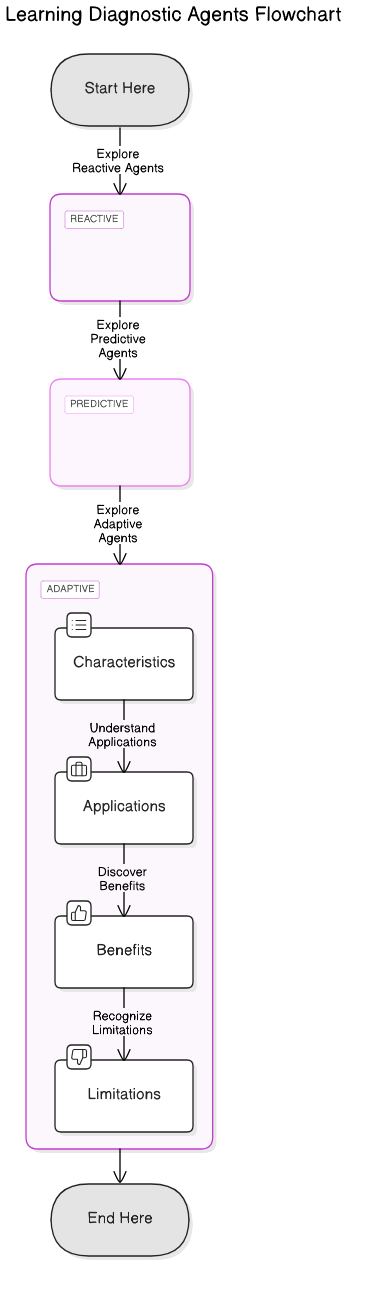
2.2.4. Autonomous Remediation Agents
Autonomous remediation agents are advanced software systems designed to identify, diagnose, and resolve issues within IT environments without human intervention. These autonomous remediation agents leverage artificial intelligence (AI) and machine learning (ML) to enhance their capabilities, making them essential in modern IT operations.
- Self-Management: These autonomous remediation agents can autonomously manage resources, monitor system performance, and execute remediation actions based on predefined policies or learned behaviors.
- Real-Time Monitoring: They continuously monitor systems for anomalies, ensuring that potential issues are detected and addressed before they escalate into significant problems.
- Decision-Making: Utilizing AI algorithms, these autonomous remediation agents can analyze vast amounts of data to make informed decisions about the best course of action for remediation.
- Integration with Existing Systems: Autonomous remediation agents can integrate seamlessly with existing IT infrastructure, enhancing their effectiveness without requiring extensive changes to current systems.
- Cost Efficiency: By automating routine troubleshooting tasks, organizations can reduce operational costs and free up IT staff to focus on more strategic initiatives.
- Scalability: These autonomous remediation agents can scale with the organization, adapting to increasing workloads and complexities in IT environments.
The implementation of autonomous remediation agents can lead to improved system reliability and reduced downtime, ultimately enhancing overall business performance. At Rapid Innovation, we specialize in deploying these agents to help our clients achieve greater ROI by streamlining their IT operations and minimizing disruptions. For more insights on the differences between multi-agent systems and single agents, check out our article on Multi-Agent Systems vs. Single Agents.
2.3. Core Technological Foundations
The core technological foundations of troubleshooting systems encompass the essential components and technologies that enable effective problem identification and resolution. These foundations are critical for developing robust troubleshooting solutions.
- Artificial Intelligence (AI): AI technologies, including machine learning and natural language processing, are fundamental in enabling systems to learn from past incidents and improve their troubleshooting capabilities over time.
- Data Analytics: Advanced data analytics tools help in processing and analyzing large volumes of data generated by IT systems, allowing for the identification of patterns and anomalies that may indicate underlying issues.
- Cloud Computing: The adoption of cloud technologies provides scalable resources and services, facilitating the deployment of troubleshooting systems that can operate across diverse environments.
- Automation Tools: Automation frameworks and tools are essential for executing remediation actions quickly and efficiently, reducing the time taken to resolve issues.
- Integration Frameworks: These frameworks allow troubleshooting systems to connect with various IT management tools, ensuring a cohesive approach to incident management and resolution.
By leveraging these core technological foundations, organizations can build effective troubleshooting systems that enhance operational efficiency and minimize downtime. Rapid Innovation harnesses these technologies to deliver tailored solutions that align with our clients' business objectives.
2.4. Cognitive Architecture of Troubleshooting Systems
Cognitive architecture refers to the underlying structure and design of troubleshooting systems that enable them to mimic human-like reasoning and problem-solving capabilities. This architecture is crucial for developing systems that can effectively diagnose and resolve complex issues.
- Knowledge Representation: Effective troubleshooting systems utilize knowledge representation techniques to store and retrieve information about known issues, solutions, and best practices.
- Reasoning Mechanisms: These systems employ reasoning mechanisms, such as rule-based systems or probabilistic reasoning, to analyze data and draw conclusions about potential problems and their solutions.
- Learning Capabilities: Cognitive architectures incorporate machine learning algorithms that allow systems to learn from past experiences, improving their ability to diagnose and resolve issues over time.
- User Interaction: A well-designed cognitive architecture facilitates intuitive user interactions, enabling IT staff to collaborate with the system effectively and leverage its capabilities.
- Feedback Loops: Incorporating feedback loops allows troubleshooting systems to refine their processes based on user input and outcomes, leading to continuous improvement in performance.
The cognitive architecture of troubleshooting systems is essential for creating intelligent solutions that can adapt to changing environments and increasingly complex IT challenges. At Rapid Innovation, we focus on developing cognitive architectures that empower organizations to enhance their troubleshooting capabilities, ultimately driving better business outcomes.
3. Technical Architecture of AI Troubleshooting Systems
The technical architecture of AI troubleshooting systems is crucial for their effectiveness in diagnosing and resolving issues. This architecture typically consists of several components that work together to collect, analyze, and act on data. Understanding these components helps in designing robust systems that can efficiently troubleshoot problems.
3.1. Data Collection and Ingestion Mechanisms
Data collection and ingestion are foundational elements of AI troubleshooting systems. These mechanisms ensure that relevant data is gathered from various sources, enabling the system to analyze and identify issues effectively.
- Types of Data Sources: Â
- System logs: Capture events related to the operating system and hardware.
- Application performance metrics: Provide insights into application behavior and performance.
- User feedback: Collects user experiences and issues directly from end-users.
- Network traffic data: Monitors data flow across the network to identify potential bottlenecks or failures.
- External APIs: Integrates data from third-party services and applications.
- Ingestion Techniques: Â
- Batch Processing: Collecting data at scheduled intervals. This method is suitable for large volumes of data that do not require real-time analysis.
- Stream Processing: Continuous data collection in real-time. This approach is essential for monitoring live systems and quickly identifying issues as they arise.
- Data Quality Assurance: Ensuring the accuracy and reliability of the collected data is vital. Techniques include: Â
- Data validation checks: Verifying the correctness of the data.
- Duplicate detection: Identifying and removing duplicate entries.
- Anomaly detection: Recognizing outliers or unusual patterns in the data.
- Integration with Existing Systems: AI troubleshooting systems must seamlessly integrate with existing IT infrastructure to gather data from various sources without disruption.
3.1.1. Log Analysis Techniques
Log analysis is a critical component of data collection in AI troubleshooting systems. Logs provide a wealth of information about system performance, user interactions, and error occurrences. Effective log analysis techniques can significantly enhance the troubleshooting process.
- Log Types: Â
- System Logs: Capture events related to the operating system and hardware.
- Application Logs: Record events specific to applications, including errors and performance metrics.
- Security Logs: Monitor access and security-related events.
- Analysis Techniques: Â
- Pattern Recognition: Identifying recurring patterns in logs can help pinpoint common issues. Machine learning algorithms can be employed to automate this process.
- Keyword Search: Searching for specific keywords or phrases in logs can quickly highlight relevant entries related to an issue.
- Time-Series Analysis: Analyzing logs over time can reveal trends and anomalies that may indicate underlying problems.
- Visualization Tools: Utilizing visualization tools can help in interpreting log data more effectively. Dashboards can display key metrics and trends, making it easier for teams to identify issues at a glance.
- Automated Alerting: Setting up automated alerts based on log analysis can ensure that teams are notified of critical issues in real-time, allowing for quicker response times.
- Correlation with Other Data Sources: Combining log data with other data sources, such as performance metrics and user feedback, can provide a more comprehensive view of system health and help in diagnosing complex issues.
By implementing robust data collection and log analysis techniques, AI troubleshooting systems can enhance their ability to identify and resolve issues efficiently, ultimately leading to improved system performance and user satisfaction. At Rapid Innovation, we leverage these advanced methodologies to help our clients achieve greater ROI by minimizing downtime and optimizing system performance through tailored AI troubleshooting systems.
3.1.2. Telemetry and Sensor Data Integration
Telemetry and sensor data integration is a crucial aspect of modern data analysis, particularly in industries such as healthcare, automotive, and manufacturing. This process involves collecting telemetry data from various sensors and telemetry systems to create a comprehensive view of operations or conditions.
- Real-time data collection: Sensors continuously gather telemetry data, providing real-time insights into system performance or environmental conditions. Rapid Innovation leverages this capability to help clients monitor their operations in real-time, enabling swift responses to any anomalies.
- Data fusion: Integrating telemetry data from multiple sources enhances accuracy and reliability. For instance, combining temperature, pressure, and humidity readings can provide a more complete picture of a system's status. Our expertise in AI allows us to implement advanced data fusion techniques that improve the quality of insights derived from telemetry data.
- Improved decision-making: With integrated telemetry data, organizations can make informed decisions quickly, leading to enhanced operational efficiency. Rapid Innovation assists clients in developing dashboards and analytics tools that facilitate data-driven decision-making.
- Predictive maintenance: By analyzing telemetry data, companies can predict equipment failures before they occur, reducing downtime and maintenance costs. Our machine learning models can be tailored to specific operational contexts, ensuring that clients can proactively manage their assets.
- Enhanced monitoring: Continuous telemetry data allows for better monitoring of critical systems, ensuring they operate within safe parameters. Rapid Innovation provides solutions that enable clients to set alerts and thresholds, enhancing their operational safety. Additionally, our expertise in anomaly detection can further enhance these monitoring capabilities.
3.1.3. Multi-Source Information Correlation
Multi-source information correlation refers to the process of analyzing and correlating data from various sources to derive meaningful insights. This technique is particularly valuable in complex environments where data is generated from multiple systems.
- Data integration: Combining data from different sources, such as databases, APIs, and IoT devices, creates a unified view of information. Rapid Innovation specializes in developing integration frameworks that allow clients to consolidate their data seamlessly.
- Pattern recognition: By correlating data from various sources, organizations can identify patterns and trends that may not be apparent when analyzing data in isolation. Our AI-driven analytics tools empower clients to uncover hidden insights that drive strategic initiatives.
- Enhanced analytics: Multi-source correlation improves the depth of analysis, allowing for more sophisticated insights and predictions. Rapid Innovation's expertise in data science enables us to provide clients with advanced analytical capabilities that enhance their competitive edge.
- Risk assessment: Correlating data from different sources can help identify potential risks and vulnerabilities, enabling proactive measures to mitigate them. We work with clients to develop risk assessment models that leverage multi-source data for comprehensive risk management.
- Improved customer insights: Businesses can gain a better understanding of customer behavior by correlating data from sales, social media, and customer feedback. Rapid Innovation helps clients harness this data to refine their marketing strategies and improve customer engagement.
3.2. Machine Learning Models for Diagnostics
Machine learning models for diagnostics leverage advanced algorithms to analyze data and identify patterns that can indicate issues or anomalies. This approach is increasingly used in various fields, including healthcare, finance, and manufacturing.
- Predictive analytics: Machine learning models can predict potential failures or health issues based on historical data, allowing for timely interventions. Rapid Innovation develops customized predictive models that align with our clients' specific operational needs.
- Anomaly detection: These models can identify unusual patterns in data that may signify a problem, enabling quicker responses to potential issues. Our solutions empower clients to implement real-time anomaly detection systems that enhance operational resilience.
- Automation of diagnostics: Machine learning can automate the diagnostic process, reducing the need for manual analysis and speeding up decision-making. Rapid Innovation's automation frameworks help clients streamline their diagnostic workflows, improving efficiency.
- Continuous learning: Machine learning models improve over time as they are exposed to more data, enhancing their accuracy and reliability. We ensure that our clients' models are designed for continuous learning, adapting to new data and evolving conditions.
- Cost reduction: By identifying issues early and automating diagnostics, organizations can reduce operational costs and improve resource allocation. Rapid Innovation's solutions are focused on delivering measurable ROI, helping clients achieve significant cost savings through optimized operations.
3.2.1. Supervised Learning Approaches
Supervised learning is a machine learning paradigm where a model is trained on labeled data. This means that the input data is paired with the correct output, allowing the model to learn the relationship between the two. Supervised learning is widely used in various applications, including classification and regression tasks.
- Key Characteristics: Â
- Requires a labeled dataset for training.
- The model learns to predict outcomes based on input features.
- Common algorithms include linear regression, decision trees, and support vector machines.
- Applications: Â
- Image recognition: Identifying objects in images.
- Spam detection: Classifying emails as spam or not spam.
- Medical diagnosis: Predicting diseases based on patient data.
- Applications of supervised learning in machine learning are vast, including tasks like supervised anomaly detection and applications of supervised machine learning.
- Advantages: Â
- High accuracy when sufficient labeled data is available.
- Clear performance metrics can be established.
- Easier to interpret results compared to some other methods.
- Challenges: Â
- Requires a large amount of labeled data, which can be expensive and time-consuming to obtain.
- Overfitting can occur if the model is too complex relative to the amount of training data.
At Rapid Innovation, we leverage supervised learning to help clients enhance their decision-making processes. For instance, in the healthcare sector, we have developed predictive models that assist in diagnosing diseases more accurately, ultimately leading to improved patient outcomes and reduced costs. We also explore applications of supervised and unsupervised learning to provide comprehensive solutions.
3.2.2. Unsupervised Anomaly Detection
Unsupervised anomaly detection is a technique used to identify unusual patterns or outliers in data without prior labeling. This approach is particularly useful in scenarios where anomalies are rare and difficult to label.
- Key Characteristics: Â
- Does not require labeled data.
- Focuses on identifying patterns and deviations from the norm.
- Common techniques include clustering, density estimation, and dimensionality reduction.
- Applications: Â
- Fraud detection: Identifying unusual transactions in financial data.
- Network security: Detecting intrusions or abnormal behavior in network traffic.
- Quality control: Monitoring manufacturing processes for defects.
- Anomaly detection supervised learning can also be applied in various domains to enhance detection capabilities.
- Advantages: Â
- Can uncover hidden patterns in data that may not be apparent with supervised methods.
- More flexible as it does not rely on labeled datasets.
- Useful in exploratory data analysis.
- Challenges: Â
- Determining what constitutes an anomaly can be subjective.
- May produce false positives, leading to unnecessary investigations.
- Requires careful tuning of parameters to achieve optimal results.
At Rapid Innovation, we implement unsupervised anomaly detection techniques to enhance our clients' security measures. For example, in the financial sector, our solutions have successfully identified fraudulent transactions, thereby protecting our clients from significant financial losses.
3.2.3. Reinforcement Learning Strategies
Reinforcement learning (RL) is a type of machine learning where an agent learns to make decisions by interacting with an environment. The agent receives feedback in the form of rewards or penalties based on its actions, allowing it to learn optimal strategies over time.
- Key Characteristics: Â
- Involves an agent, environment, actions, and rewards.
- The agent learns through trial and error.
- Common algorithms include Q-learning, deep Q-networks, and policy gradients.
- Applications: Â
- Robotics: Teaching robots to navigate and perform tasks.
- Game playing: Developing AI that can play games like chess or Go at a high level.
- Autonomous vehicles: Enabling cars to make driving decisions in real-time.
- Advantages: Â
- Can learn complex behaviors and strategies that are difficult to program manually.
- Adapts to changing environments and conditions.
- Capable of solving problems with delayed rewards.
- Challenges: Â
- Requires a significant amount of data and computational resources.
- Training can be time-consuming, especially in complex environments.
- Balancing exploration (trying new actions) and exploitation (using known actions) is crucial for success.
At Rapid Innovation, we harness reinforcement learning to develop intelligent systems that can adapt and optimize their performance over time. For instance, in the realm of autonomous vehicles, our RL-based solutions enable cars to make real-time driving decisions, enhancing safety and efficiency on the roads.
By integrating these advanced AI methodologies, including self supervised learning computer vision and applications of semi supervised learning, Rapid Innovation empowers clients to achieve their business goals efficiently and effectively, ultimately driving greater ROI.
3.3. Knowledge Representation
Knowledge representation is a crucial aspect of artificial intelligence (AI) that focuses on how information and knowledge can be structured and stored in a way that a computer system can utilize it effectively. This process involves creating a framework that allows machines to understand and manipulate knowledge, including knowledge representation in AI and knowledge representation in artificial intelligence.
- Types of Knowledge Representation: Â
- Semantic Networks: Graph structures that represent knowledge in terms of nodes (concepts) and edges (relationships).
- Frames: Data structures for representing stereotyped situations, containing attributes and values, such as frame representation in AI.
- Rules: Conditional statements that define relationships and can be used for reasoning.
- Importance of Knowledge Representation: Â
- Enables machines to simulate human-like understanding.
- Facilitates the sharing and reuse of knowledge across different systems.
- Supports complex problem-solving and decision-making processes, highlighting the importance of knowledge representation in AI.
- Challenges in Knowledge Representation: Â
- Ambiguity in natural language can lead to misinterpretation.
- The need for a balance between expressiveness and computational efficiency.
- Difficulty in representing dynamic and evolving knowledge, which is a key concern in different types of knowledge representation in AI.
Effective knowledge representation is foundational for various AI applications, including natural language processing, expert systems, and robotics. Approaches of knowledge representation in AI are essential for developing systems that can reason and learn. At Rapid Innovation, we leverage advanced knowledge representation techniques, including knowledge representation and reasoning in AI, to enhance our AI solutions, ensuring that our clients can achieve greater efficiency and ROI through improved decision-making and problem-solving capabilities. For those looking to enhance their capabilities, you can hire generative AI engineers to assist in this area.
3.4. Inference and Reasoning Engines
Inference and reasoning engines are integral components of AI systems that allow machines to derive new information from existing knowledge. These engines utilize logical rules and algorithms to process data and make decisions.
- Types of Inference: Â
- Deductive Inference: Drawing specific conclusions from general premises. For example, if all humans are mortal and Socrates is a human, then Socrates is mortal.
- Inductive Inference: Making generalizations based on specific observations. For instance, observing that the sun rises every day leads to the conclusion that it will rise tomorrow.
- Abductive Inference: Inferring the best explanation for a set of observations. This is often used in diagnostic reasoning.
- Reasoning Techniques: Â
- Forward Chaining: Starts with known facts and applies rules to infer new facts until a goal is reached.
- Backward Chaining: Begins with a goal and works backward to determine which facts must be true to support that goal.
- Applications of Inference Engines: Â
- Expert systems for medical diagnosis.
- Automated reasoning in legal applications.
- Decision support systems in business intelligence.
Inference and reasoning engines enhance the capability of AI systems to make informed decisions and solve complex problems. Rapid Innovation employs these engines to develop tailored solutions that drive efficiency and effectiveness for our clients, ultimately leading to a higher return on investment.
4. Diagnostic Capabilities and Methodologies
Diagnostic capabilities in AI refer to the ability of systems to identify problems, analyze symptoms, and suggest solutions. This is particularly important in fields such as healthcare, engineering, and IT.
- Key Components of Diagnostic Methodologies: Â
- Data Collection: Gathering relevant data from various sources, including sensors, user inputs, and historical records.
- Data Analysis: Utilizing algorithms and statistical methods to analyze the collected data for patterns and anomalies.
- Hypothesis Generation: Formulating potential explanations for the observed symptoms based on the analyzed data.
- Diagnostic Techniques: Â
- Rule-Based Systems: Use predefined rules to diagnose issues based on symptoms. Common in medical diagnosis.
- Machine Learning: Employs algorithms to learn from data and improve diagnostic accuracy over time.
- Bayesian Networks: Probabilistic graphical models that represent a set of variables and their conditional dependencies.
- Importance of Diagnostic Capabilities: Â
- Enhances the speed and accuracy of problem identification.
- Reduces the need for manual intervention, leading to cost savings.
- Improves decision-making by providing actionable insights.
AI-driven diagnostic capabilities are transforming industries by enabling faster and more accurate problem-solving, ultimately leading to better outcomes and increased efficiency. At Rapid Innovation, we harness these capabilities to provide our clients with innovative solutions that not only address their immediate challenges but also contribute to long-term success and profitability.
4.1. Root Cause Analysis
Root Cause Analysis (RCA) is a systematic approach used to identify the fundamental cause of a problem. It goes beyond merely addressing symptoms to uncover the underlying issues that lead to failures or inefficiencies. RCA is essential in various fields, including manufacturing, healthcare, and IT, as it helps organizations improve processes and prevent the recurrence of issues.
Common techniques for RCA include the "5 Whys," Fishbone Diagram (Ishikawa), and Failure Mode and Effects Analysis (FMEA). The process typically involves:
- Defining the problem clearly.
- Collecting data related to the issue.
- Identifying possible causes.
- Analyzing the causes to determine the root cause.
- Implementing corrective actions to address the root cause.
Effective RCA can lead to significant cost savings and improved operational efficiency by reducing downtime and enhancing product quality. At Rapid Innovation, we utilize advanced AI algorithms to automate data collection and analysis, ensuring a more accurate identification of root causes. This not only streamlines the RCA process but also enhances the overall effectiveness of the solutions we provide to our clients. Techniques such as the 5 whys approach and the five whys root cause analysis are particularly useful in this context, allowing teams to drill down to the core issues effectively.
4.2. Pattern Recognition
Pattern recognition is the ability to identify and understand recurring themes or trends within data or situations. This skill is crucial in various domains, including data analysis, machine learning, and even everyday decision-making. Recognizing patterns allows individuals and organizations to make informed predictions and decisions based on historical data.
Key aspects of pattern recognition include:
- Data collection: Gathering relevant information from various sources.
- Analysis: Using statistical methods or algorithms to identify trends.
- Interpretation: Understanding the implications of the identified patterns.
Applications of pattern recognition include:
- Fraud detection in finance.
- Predictive maintenance in manufacturing.
- Customer behavior analysis in marketing.
By leveraging pattern recognition, businesses can enhance their strategic planning and operational efficiency. Rapid Innovation employs cutting-edge machine learning techniques to help clients uncover hidden patterns in their data, leading to improved decision-making and increased ROI.
4.3. Contextual Problem Understanding
Contextual problem understanding involves grasping the broader environment and circumstances surrounding a specific issue. This approach emphasizes the importance of context in problem-solving and decision-making. Understanding the context helps in:
- Identifying relevant factors that may influence the problem.
- Recognizing stakeholders and their perspectives.
- Evaluating the impact of external variables, such as market trends or regulatory changes.
Key components of contextual problem understanding include:
- Gathering background information: Researching the history and environment related to the issue.
- Engaging stakeholders: Involving those affected by the problem to gain diverse insights.
- Analyzing the environment: Considering economic, social, and technological factors that may play a role.
This approach is particularly valuable in complex situations where multiple variables interact, leading to more effective and sustainable solutions. At Rapid Innovation, we integrate blockchain technology to ensure transparency and traceability in stakeholder engagement, enhancing the contextual understanding of problems and facilitating more informed decision-making. Techniques like the 5 why method for root cause analysis can also be employed to ensure that the context is fully understood before implementing solutions.
4.4. Predictive Failure Detection
Predictive failure detection is a proactive approach that leverages data analytics and machine learning to identify potential failures before they occur. This technique is crucial in various industries, including manufacturing, automotive, and IT, where unplanned downtime can lead to significant financial losses.
- Utilizes historical data to identify patterns and trends that precede failures. Â
- Employs algorithms to analyze real-time data from sensors and equipment. Â
- Enables organizations to schedule maintenance activities based on predictive failure detection, reducing unexpected breakdowns. Â
- Enhances operational efficiency by minimizing downtime and extending the lifespan of equipment. Â
- Integrates with IoT devices to gather data continuously, providing a comprehensive view of system health. Â
By implementing predictive failure detection, companies can shift from reactive maintenance to a more strategic, data-driven approach. This not only saves costs but also improves safety and reliability. At Rapid Innovation, we specialize in developing tailored predictive analytics solutions that empower organizations to optimize their maintenance strategies, ultimately leading to greater ROI. For more information on our services, check out our custom AI model development and learn about our AI-powered product recommendations in e-commerce.
4.5. Automated Diagnostic Workflows
Automated diagnostic workflows streamline the process of identifying and resolving issues within systems and equipment. This technology is particularly beneficial in complex environments where manual diagnostics can be time-consuming and prone to errors.
- Reduces the time required to diagnose problems by automating data collection and analysis. Â
- Utilizes predefined rules and algorithms to guide technicians through troubleshooting steps. Â
- Increases accuracy in diagnosing issues, leading to faster resolution times. Â
- Enhances collaboration among teams by providing a centralized platform for diagnostics. Â
- Supports continuous improvement by capturing data on past issues and resolutions for future reference. Â
Automated diagnostic workflows not only improve efficiency but also enhance the overall quality of service. By minimizing human error and expediting the diagnostic process, organizations can better meet customer expectations and maintain operational continuity. Rapid Innovation's expertise in automation technologies ensures that our clients can achieve seamless diagnostics, driving operational excellence and maximizing their return on investment.
4.6. Intelligent Recommendation Systems
Intelligent recommendation systems are designed to provide users with personalized suggestions based on their preferences, behaviors, and historical data. These systems are widely used in e-commerce, content delivery, and various service industries to enhance user experience and drive engagement.
- Leverages machine learning algorithms to analyze user data and predict preferences. Â
- Offers tailored recommendations that can increase conversion rates and customer satisfaction. Â
- Utilizes collaborative filtering and content-based filtering techniques to enhance accuracy. Â
- Continuously learns from user interactions to improve future recommendations. Â
- Can be integrated into various platforms, including websites, mobile apps, and customer service interfaces. Â
By implementing intelligent recommendation systems, businesses can create a more engaging and personalized experience for their users. This not only fosters customer loyalty but also drives sales and enhances brand reputation. At Rapid Innovation, we harness the power of AI to develop sophisticated recommendation systems that not only meet but exceed user expectations, ultimately leading to increased revenue and customer retention.
5. Domain-Specific Troubleshooting Approaches
Troubleshooting is a critical skill in various domains, especially in IT and networking. Each domain has its unique challenges and requires specific approaches to effectively identify and resolve issues. Here, we will explore two key areas: IT infrastructure troubleshooting and network and connectivity diagnostics.
5.1. IT Infrastructure Troubleshooting
IT infrastructure troubleshooting involves diagnosing and resolving issues related to hardware, software, and systems that support an organization's IT environment. This process is essential for maintaining operational efficiency and minimizing downtime.
To effectively troubleshoot IT infrastructure, follow these steps:
- Identify the Problem: Start by gathering information about the issue, including understanding the symptoms, error messages, and the context in which the problem occurs.
- Check Hardware Components: Inspect physical devices such as servers, storage systems, and networking equipment for signs of failure, such as unusual noises, warning lights, or overheating.
- Review System Logs: Analyze logs from operating systems, applications, and security systems, as they can provide valuable insights into what went wrong and when.
- Test Software Configurations: Ensure that software settings are correctly configured, as misconfigurations can lead to performance issues or system failures.
- Utilize Diagnostic Tools: Employ tools like performance monitors, network analyzers, and system checkers to gather data and pinpoint issues.
- Implement a Structured Approach: Use methodologies like ITIL (Information Technology Infrastructure Library) or Six Sigma to guide the troubleshooting process. These frameworks help standardize procedures and improve efficiency.
- Document Findings: Keep detailed records of the troubleshooting process, including steps taken, findings, and resolutions. This documentation can be invaluable for future reference and training.
5.2. Network and Connectivity Diagnostics

‍
Network and connectivity diagnostics focus on identifying and resolving issues related to network performance and connectivity. Given the complexity of modern networks, a systematic approach is crucial.
To effectively diagnose network and connectivity issues, consider the following steps:
- Check Physical Connections: Ensure that all cables, switches, and routers are properly connected, as loose or damaged cables can lead to connectivity issues.
- Ping and Traceroute: Use tools like
ping
andtraceroute
to test connectivity and measure response times. These tools help identify where the connection is failing. - Examine Network Configuration: Review the configuration of routers, switches, and firewalls, as incorrect settings can block traffic or cause slowdowns.
- Monitor Network Traffic: Utilize network monitoring tools to analyze traffic patterns, since high traffic loads can lead to congestion and performance degradation.
- Check for Interference: In wireless networks, interference from other devices can disrupt connectivity. Use spectrum analyzers to identify and mitigate interference sources.
- Update Firmware and Software: Ensure that all network devices are running the latest firmware and software, as updates often include bug fixes and performance improvements.
- Conduct Speed Tests: Regularly perform speed tests to assess network performance, which can help identify issues related to bandwidth and latency.
By employing these domain-specific troubleshooting approaches, IT professionals can effectively address issues in IT infrastructure troubleshooting and network connectivity, ensuring smooth operations and enhanced productivity. At Rapid Innovation, we leverage our expertise in AI and Blockchain to enhance these troubleshooting processes, providing clients with advanced diagnostic tools and methodologies that lead to greater operational efficiency and improved ROI.
5.3. Software Application Debugging
Software application debugging is a critical process in software development that involves identifying, isolating, and fixing bugs or defects in a software application. Debugging ensures that the software functions as intended and meets user requirements, ultimately contributing to the overall success of a project.
- Types of Bugs: Â
- Syntax errors: Mistakes in the code that prevent it from compiling.
- Logic errors: Flaws in the program's logic that lead to incorrect results.
- Runtime errors: Issues that occur while the program is running, often due to invalid operations.
- Debugging Techniques: Â
- Print debugging: Involves adding print statements to the code to track variable values and program flow.
- Interactive debugging: Utilizes debugging tools that allow developers to step through code execution, inspect variables, and evaluate expressions.
- Automated testing: Employs unit tests and integration tests to catch bugs early in the development cycle.
- Different debugging techniques can be applied depending on the context, such as debugging techniques in software testing and debugging strategies in software testing.
- Tools for Debugging: Â
- Integrated Development Environments (IDEs): Tools like Visual Studio and Eclipse offer built-in debugging tools.
- Standalone debuggers: Such as
GDB
(GNU Debugger) provide command-line debugging capabilities. - Profilers: Help identify performance bottlenecks and memory leaks.
- Embedded software debugging techniques are also crucial for developers working on specific applications.
Effective debugging not only improves software quality but also enhances user satisfaction and reduces maintenance costs. According to studies, fixing a bug during the design phase can be up to 100 times cheaper than fixing it after deployment. At Rapid Innovation, we leverage advanced debugging techniques, including debugging practices in software engineering and debugging techniques in software engineering, to ensure that our clients' software applications are robust and reliable, leading to greater ROI and user satisfaction. For custom solutions, consider partnering with a custom AI development company in the USA and learn more about AI agents in software testing.
5.4. Hardware System Diagnostics
Hardware system diagnostics is the process of evaluating and testing the physical components of a computer or electronic system to ensure they are functioning correctly. This process is essential for maintaining system reliability and performance.
- Common Diagnostic Tools: Â
- Built-in diagnostics: Many systems come with pre-installed diagnostic tools that can run tests on hardware components.
- Third-party software: Tools like
MemTest86
for memory testing andCrystalDiskInfo
for hard drive health monitoring are widely used. - Hardware diagnostic kits: These kits often include multimeters and oscilloscopes for in-depth analysis.
- Key Areas of Focus: Â
- CPU: Testing for overheating, performance issues, and proper functioning.
- Memory: Checking for errors and ensuring adequate performance.
- Storage: Assessing hard drive health and data integrity.
- Power supply: Ensuring stable voltage and current delivery to components.
- Importance of Diagnostics: Â
- Early detection of hardware failures can prevent data loss and system downtime.
- Regular diagnostics can extend the lifespan of hardware components.
- Helps in planning upgrades and replacements based on performance metrics.
Implementing a routine hardware diagnostic schedule can significantly enhance system reliability and performance, ultimately leading to a more efficient computing environment. Rapid Innovation assists clients in establishing effective diagnostic protocols, ensuring their hardware systems operate at peak performance and minimizing unexpected costs.
5.5. Cloud and Distributed Systems
Cloud and distributed systems refer to a network of interconnected computers that work together to provide resources, services, and applications over the internet. These systems are designed to enhance scalability, reliability, and accessibility.
- Key Characteristics: Â
- Scalability: Resources can be easily scaled up or down based on demand.
- Redundancy: Data is replicated across multiple locations to ensure availability.
- Flexibility: Users can access services from anywhere with an internet connection.
- Types of Cloud Services: Â
- Infrastructure as a Service (IaaS): Provides virtualized computing resources over the internet.
- Platform as a Service (PaaS): Offers a platform allowing developers to build, deploy, and manage applications.
- Software as a Service (SaaS): Delivers software applications over the internet on a subscription basis.
- Challenges in Cloud and Distributed Systems: Â
- Security: Protecting data from unauthorized access and breaches is a major concern.
- Latency: Network delays can affect performance, especially in real-time applications.
- Management: Coordinating resources and services across distributed environments can be complex.
Cloud and distributed systems have transformed how businesses operate, enabling them to leverage technology for improved efficiency and innovation. According to a report, the global cloud computing market is expected to reach $832.1 billion by 2025, highlighting the growing importance of these systems in the digital landscape. Rapid Innovation specializes in developing and implementing cloud solutions that address these challenges, ensuring our clients can maximize their operational efficiency and achieve their business goals effectively.
5.6. IoT and Embedded Systems
The Internet of Things (IoT) and embedded systems are revolutionizing how devices communicate and operate. These technologies are integral to creating smart environments, enhancing efficiency, and improving user experiences. IoT refers to a network of interconnected devices that can collect and exchange data, while embedded systems are specialized computing systems that perform dedicated functions within larger mechanical or electrical systems. Together, they enable real-time data processing and automation across various sectors, including healthcare, manufacturing, and smart homes.
Key features of IoT and embedded systems include:
- Interconnectivity: Devices can communicate with each other and share data seamlessly.
- Automation: Processes can be automated, reducing the need for human intervention.
- Data Analytics: Collected data can be analyzed to derive insights, improving decision-making.
- Remote Monitoring: Users can monitor and control devices from anywhere, enhancing convenience.
The integration of IoT and embedded systems leads to significant advancements in various applications:
- Smart Homes: Devices like smart thermostats and security cameras enhance home automation and security.
- Healthcare: Wearable devices monitor patient health in real-time, allowing for timely interventions.
- Industrial IoT: Sensors in manufacturing equipment can predict failures, reducing downtime and maintenance costs.
At Rapid Innovation, we leverage our expertise in IoT and embedded systems, including windows 10 embedded and embedded iot solutions, to help clients optimize their operations and achieve greater ROI. For instance, by implementing IoT solutions in manufacturing, we have enabled clients to reduce operational costs through predictive maintenance, ultimately leading to increased productivity and profitability.
As IoT and embedded systems continue to evolve, they will play a crucial role in shaping the future of technology and everyday life. The rise of embedded internet of things and embedded systems internet of things is paving the way for innovative applications, such as embedded devices in IoT and embedded iot devices that enhance connectivity and functionality. Additionally, the role of natural language processing in AI agents is becoming increasingly important in managing and interpreting the vast amounts of data generated by these systems.
6. Advanced AI Troubleshooting Techniques
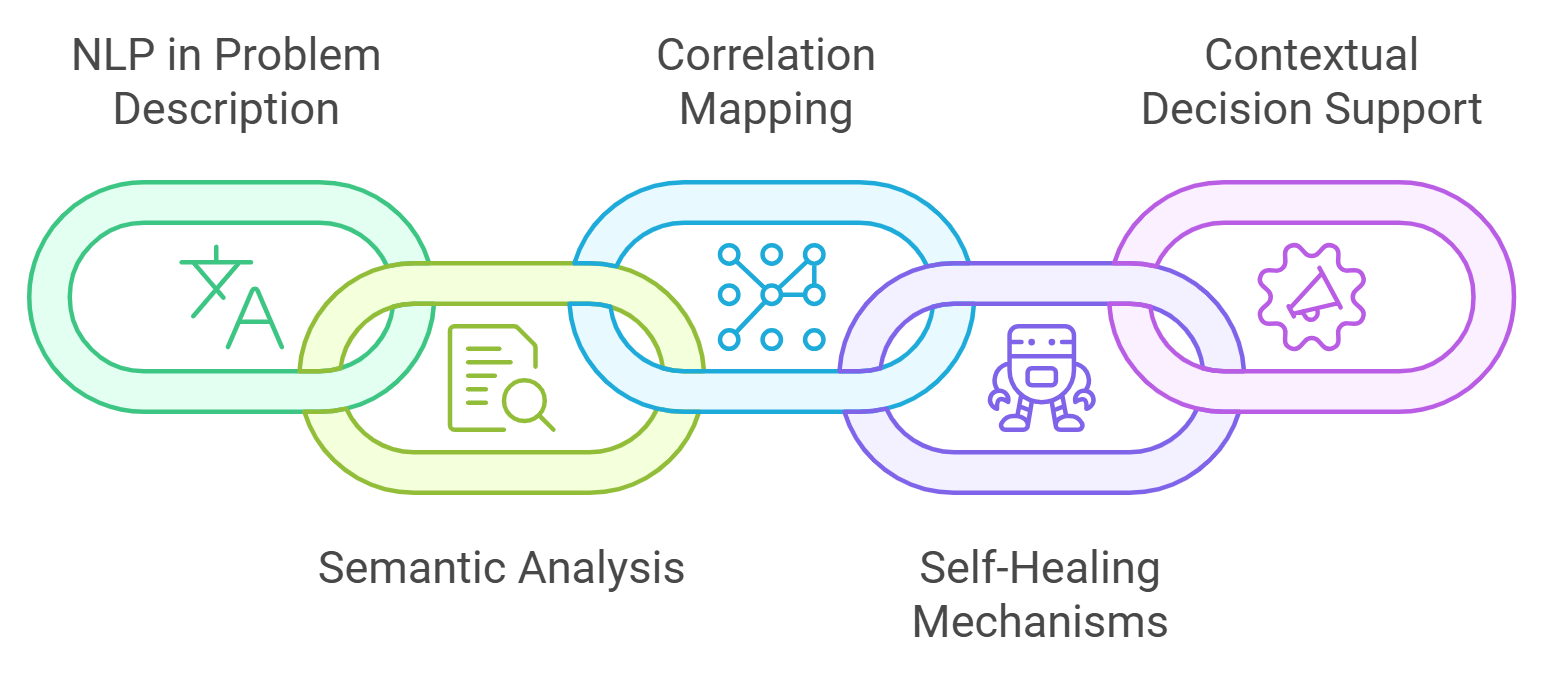
‍
Advanced AI troubleshooting techniques are transforming how problems are identified and resolved in various systems. These techniques leverage artificial intelligence to enhance efficiency and accuracy in troubleshooting processes. AI can analyze vast amounts of data quickly, identifying patterns and anomalies that may indicate issues. Machine learning algorithms can learn from past incidents, improving their ability to predict and resolve future problems. Additionally, natural language processing (NLP) plays a significant role in understanding user queries and providing relevant solutions.
Benefits of advanced AI troubleshooting techniques include:
- Speed: AI can process information and provide solutions faster than traditional methods.
- Accuracy: AI reduces human error by relying on data-driven insights.
- Scalability: AI systems can handle a large volume of troubleshooting requests simultaneously.
These techniques are being applied across various industries:
- IT Support: AI chatbots can assist users in troubleshooting software issues, providing instant solutions.
- Manufacturing: AI systems can monitor equipment performance and predict failures before they occur.
- Customer Service: AI can analyze customer interactions to identify common issues and suggest improvements.
At Rapid Innovation, we implement advanced AI troubleshooting techniques to enhance our clients' operational efficiency. For example, by deploying AI-driven chatbots in customer service, we have helped clients reduce response times and improve customer satisfaction, leading to higher retention rates and increased revenue.
As AI technology advances, its role in troubleshooting will continue to expand, leading to more efficient and effective problem resolution.
6.1. Natural Language Processing in Problem Description
Natural Language Processing (NLP) is a branch of artificial intelligence that focuses on the interaction between computers and human language. In the context of troubleshooting, NLP plays a vital role in understanding and interpreting problem descriptions provided by users. NLP enables machines to comprehend, interpret, and respond to human language in a meaningful way, allowing for the extraction of relevant information from user queries, which facilitates quicker problem identification.
Key applications of NLP in problem description include:
- Sentiment Analysis: Understanding the emotional tone of user messages can help prioritize issues based on urgency.
- Keyword Extraction: Identifying key terms in a problem description helps in categorizing and routing issues to the appropriate support channels.
- Contextual Understanding: NLP can analyze the context of a problem, allowing for more accurate troubleshooting suggestions.
Benefits of using NLP in troubleshooting include:
- Improved User Experience: Users can describe their issues in natural language, making the process more intuitive.
- Faster Resolution: By quickly understanding user queries, support systems can provide relevant solutions more efficiently.
- Reduced Workload: Automating the initial stages of troubleshooting allows human agents to focus on more complex issues.
NLP is increasingly being integrated into various troubleshooting platforms, enhancing their capabilities and improving overall efficiency. As NLP technology continues to advance, its impact on problem description and resolution will only grow, leading to more effective support systems. At Rapid Innovation, we harness NLP to streamline troubleshooting processes, ensuring our clients can resolve issues swiftly and maintain high levels of service quality.
6.2. Semantic Analysis of Error Logs
Semantic analysis of error logs involves the examination of log data to extract meaningful insights and patterns. This process is crucial for identifying the root causes of issues within software systems. By applying natural language processing (NLP) techniques, organizations can enhance their ability to interpret error messages and system alerts.
- Error logs often contain unstructured data, making it challenging to derive actionable insights.
- Semantic analysis helps in categorizing errors based on their context and severity.
- It enables the identification of recurring issues, allowing teams to prioritize fixes effectively.
- By understanding the semantics of error messages, developers can improve the clarity of logs, making them easier to analyze in the future.
- Tools like Elasticsearch and Kibana can be utilized for visualizing and analyzing log data semantically.
The benefits of semantic analysis include reduced troubleshooting time and improved system reliability. By leveraging machine learning algorithms, organizations can automate the classification of errors, leading to faster resolution times. At Rapid Innovation, we implement these advanced techniques to help our clients streamline their operations, ultimately enhancing their return on investment (ROI) through improved system performance and reduced downtime.
6.3. Intelligent Correlation and Causation Mapping
Intelligent correlation and causation mapping refer to the process of linking various events and errors to understand their relationships and underlying causes. This approach is essential for diagnosing complex issues in distributed systems where multiple components interact.
- Correlation analysis helps in identifying patterns between different logs and events.
- Causation mapping goes a step further by establishing a cause-and-effect relationship between incidents.
- By using advanced analytics and machine learning, organizations can uncover hidden dependencies that may not be immediately apparent.
- This process can significantly reduce the time spent on root cause analysis, allowing teams to focus on implementing solutions.
- Tools like Splunk and Grafana can assist in visualizing correlations and causations effectively.
The outcome of intelligent correlation and causation mapping is a more proactive approach to incident management. By understanding how different components interact, organizations can anticipate potential failures and mitigate risks before they escalate. Rapid Innovation leverages these methodologies to empower our clients with insights that lead to more informed decision-making and ultimately, a greater ROI.
6.4. Self-Healing Mechanisms
Self-healing mechanisms are automated processes that enable systems to detect and resolve issues without human intervention. This capability is increasingly important in modern IT environments, where downtime can lead to significant financial losses and customer dissatisfaction.
- Self-healing systems can automatically restart services, reallocate resources, or apply patches when issues are detected.
- These mechanisms rely on monitoring tools that continuously assess system health and performance.
- By utilizing AI and machine learning, self-healing systems can learn from past incidents to improve their response strategies.
- Implementing self-healing capabilities can lead to increased system uptime and reduced operational costs.
- Examples of self-healing technologies include Kubernetes for container orchestration and cloud services that offer auto-scaling features.
The primary advantage of self-healing mechanisms is their ability to maintain system stability and performance with minimal human oversight. This not only enhances operational efficiency but also allows IT teams to focus on strategic initiatives rather than routine maintenance tasks. At Rapid Innovation, we integrate self-healing technologies into our clients' systems, ensuring they achieve optimal performance and reliability, which translates to a significant increase in ROI.
6.5. Contextual Decision Support
Contextual Decision Support (CDS) refers to systems and tools that provide relevant information and insights to aid decision-making in specific contexts. This approach enhances the quality of decisions by integrating data, analytics, and contextual information. CDS provides real-time insights tailored to the specific situation, utilizes historical data and predictive analytics to forecast outcomes, enhances collaboration among stakeholders by sharing relevant information, supports various industries, including healthcare, finance, and manufacturing, and improves efficiency by reducing the time spent on data gathering and analysis.
At Rapid Innovation, we leverage advanced AI algorithms to develop CDS solutions that empower organizations to make informed decisions quickly. For instance, in healthcare, our CDS tools can analyze vast amounts of patient data and suggest personalized treatment options based on similar cases, ultimately improving patient outcomes and reducing costs. In finance, our systems can assess market conditions in real-time and provide actionable investment recommendations, helping clients maximize their returns. The key to effective CDS is our ability to filter and present information that is most relevant to the decision-maker's needs, ensuring that they can act decisively and strategically. To learn more about our services in healthcare management, visit our AI Healthcare Management page.
7. Remediation and Resolution Strategies
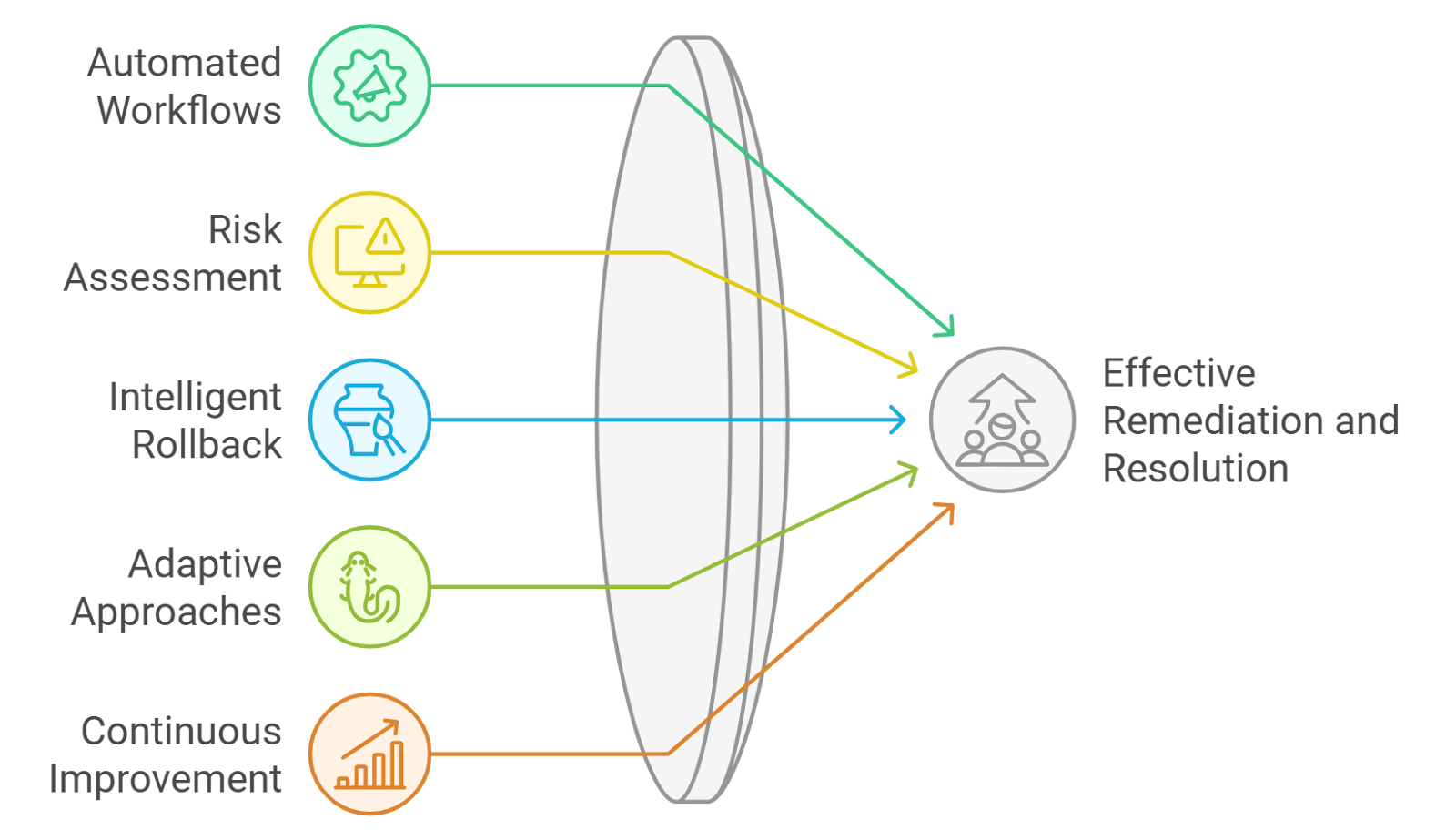
‍
Remediation and resolution strategies are essential for addressing issues and challenges that arise in various processes, particularly in IT and cybersecurity. These strategies focus on identifying problems, implementing solutions, and ensuring that similar issues do not recur. Effective strategies involve a systematic approach to problem-solving, aim to minimize downtime and operational disruptions, include both immediate fixes and long-term solutions, require collaboration across teams to ensure comprehensive coverage, and utilize data analysis to identify root causes of issues.
At Rapid Innovation, we understand that effective remediation strategies often involve a combination of manual and automated processes. By leveraging our expertise in AI and Blockchain technologies, organizations can streamline their response to incidents and improve overall resilience.
7.1. Automated Remediation Workflows
Automated remediation workflows are processes that use technology to automatically address and resolve issues without human intervention. These workflows are particularly valuable in environments where speed and accuracy are critical. They reduce the time required to respond to incidents, minimize human error by relying on predefined rules and algorithms, enhance consistency in how issues are handled, free up human resources for more complex tasks, and can be integrated with existing systems for seamless operation.
For example, in IT operations, our automated workflows can detect anomalies in system performance and trigger predefined actions, such as restarting a service or reallocating resources. In cybersecurity, these workflows can automatically isolate affected systems in response to detected threats, thereby containing potential damage.
By implementing automated remediation workflows, organizations can improve their operational efficiency and enhance their ability to respond to challenges swiftly and effectively. Rapid Innovation is committed to helping clients achieve greater ROI through these innovative solutions, ensuring they remain competitive in an ever-evolving landscape.
7.2. Risk Assessment and Mitigation
Risk assessment and mitigation are critical components of project management and operational strategy, particularly in the realms of AI and Blockchain development. They involve identifying potential risks, analyzing their impact, and implementing strategies to minimize their effects, ultimately leading to greater ROI for our clients.
- Identify Risks: Â
- Conduct thorough research to pinpoint potential risks in your project or operation, such as data breaches in Blockchain applications or algorithmic biases in AI systems. This includes vendor risk management cyber security and cybersecurity vendor risk management.
- Engage stakeholders to gather insights on possible vulnerabilities, ensuring a comprehensive understanding of the risk landscape.
- Analyze Risks: Â
- Evaluate the likelihood of each risk occurring and its potential impact on the project. For instance, assess the implications of regulatory changes on Blockchain compliance or the risks associated with AI model performance.
- Use qualitative and quantitative methods to assess risks, such as SWOT analysis or risk matrices, tailored to the specific challenges of AI and Blockchain projects. This may involve risk analysis and mitigation or risk analysis mitigation.
- Develop Mitigation Strategies: Â
- Create action plans to address identified risks, focusing on prevention and response. This may include implementing robust security protocols for Blockchain systems or conducting regular audits of AI models to ensure ethical compliance. Strategies may also encompass risk evaluation mitigation strategies and risk identification and mitigation.
- Prioritize risks based on their severity and likelihood, allocating resources accordingly to ensure that the most critical risks are managed effectively. This includes developing a risk assessment management plan and a risk assessment and management plan.
- Monitor and Review: Â
- Continuously monitor risks throughout the project lifecycle, utilizing AI-driven analytics to identify emerging threats in real-time. This includes assessing hazards where the step is performed after the risk mitigation step.
- Adjust mitigation strategies as necessary based on new information or changes in the project environment, ensuring that your approach remains agile and responsive.
Effective risk assessment and mitigation can lead to improved project outcomes, reduced costs, and enhanced stakeholder confidence. By leveraging our expertise in AI and Blockchain, Rapid Innovation helps clients navigate these complexities, ultimately driving greater ROI. For more information on how we can assist with your AI projects, visit our AI project estimation services and learn about the future of personalized risk evaluation in insurance with AI agents.
7.3. Intelligent Rollback Mechanisms
Intelligent rollback mechanisms are essential for maintaining system stability and ensuring data integrity during software updates or system changes, particularly in AI and Blockchain applications. These mechanisms allow for quick recovery from failures or errors, minimizing downtime and disruption.
- Automated Backups: Â
- Implement automated backup systems to create restore points before changes are made, ensuring that critical data is preserved.
- Ensure backups are stored securely and are easily accessible, particularly in decentralized Blockchain environments.
- Version Control: Â
- Use version control systems to track changes and maintain a history of updates. This allows for easy reversion to previous versions if issues arise, which is crucial for maintaining the integrity of AI models and Blockchain smart contracts.
- Real-Time Monitoring: Â
- Employ real-time monitoring tools to detect anomalies during updates. This enables immediate action to be taken if a rollback is necessary, ensuring that systems remain operational and reliable.
- User Notifications: Â
- Inform users of potential issues and the possibility of rollbacks during updates. Clear communication helps manage expectations and reduces frustration, fostering trust in the system.
Intelligent rollback mechanisms not only enhance system reliability but also improve user experience by ensuring that services remain available even in the face of unexpected challenges.
7.4. Adaptive Problem-Solving Approaches
Adaptive problem-solving approaches are essential in today’s fast-paced and ever-changing environments, especially in the fields of AI and Blockchain. These strategies allow teams to respond effectively to unforeseen challenges and evolving circumstances.
- Flexibility: Â
- Encourage a culture of flexibility within teams, allowing for quick adjustments to plans and strategies. Foster an environment where team members feel comfortable proposing changes based on new information, particularly in response to rapid technological advancements.
- Collaborative Decision-Making: Â
- Involve diverse team members in the problem-solving process to leverage different perspectives and expertise. Use brainstorming sessions and workshops to generate innovative solutions that can address complex challenges in AI and Blockchain projects.
- Continuous Learning: Â
- Promote a mindset of continuous learning and improvement. Encourage teams to analyze past challenges and successes to inform future strategies, ensuring that lessons learned are integrated into ongoing projects.
- Iterative Processes: Â
- Implement iterative processes, such as Agile methodologies, to allow for regular reassessment and adaptation. Break projects into smaller phases, enabling teams to learn and adapt as they progress, which is particularly beneficial in the dynamic fields of AI and Blockchain.
Adaptive problem-solving approaches enhance resilience and innovation, enabling organizations to thrive in dynamic environments. By embracing these strategies, Rapid Innovation empowers teams to effectively navigate challenges and seize opportunities for growth, ultimately driving success for our clients.
7.5. Continuous Learning and Improvement
Continuous learning and improvement are essential components of any successful organization, particularly in the rapidly evolving fields of AI and Blockchain. This approach fosters a culture where employees are encouraged to develop their skills and adapt to changing environments. Organizations that prioritize continuous learning in organizations often see increased employee engagement, higher retention rates, and improved overall performance. By fostering an environment that values growth and development, companies can adapt more effectively to market changes and technological advancements.
- Emphasizes the importance of ongoing education and training, particularly in emerging technologies like AI and Blockchain.
- Encourages employees to seek feedback and learn from experiences, enhancing their ability to innovate and solve complex problems.
- Promotes a growth mindset, where challenges are viewed as opportunities for development, crucial for navigating the complexities of AI and Blockchain projects.
- Utilizes data and analytics to assess performance and identify areas for improvement, ensuring that teams are aligned with business goals.
- Implements regular training sessions, workshops, and seminars to keep skills updated, particularly in the fast-paced tech landscape.
- Encourages collaboration and knowledge sharing among team members, fostering a community of innovation and support.
- Supports the use of technology and online resources for self-directed learning, enabling employees to stay ahead in their fields.
- Establishes clear metrics to measure progress and success in learning initiatives, ensuring accountability and continuous improvement.
Continuous learning for employees is vital in maintaining a competitive edge and ensuring that the workforce is equipped to handle new challenges. For more insights on effective strategies, check out successful AI integration strategies.
8. Implementation Considerations
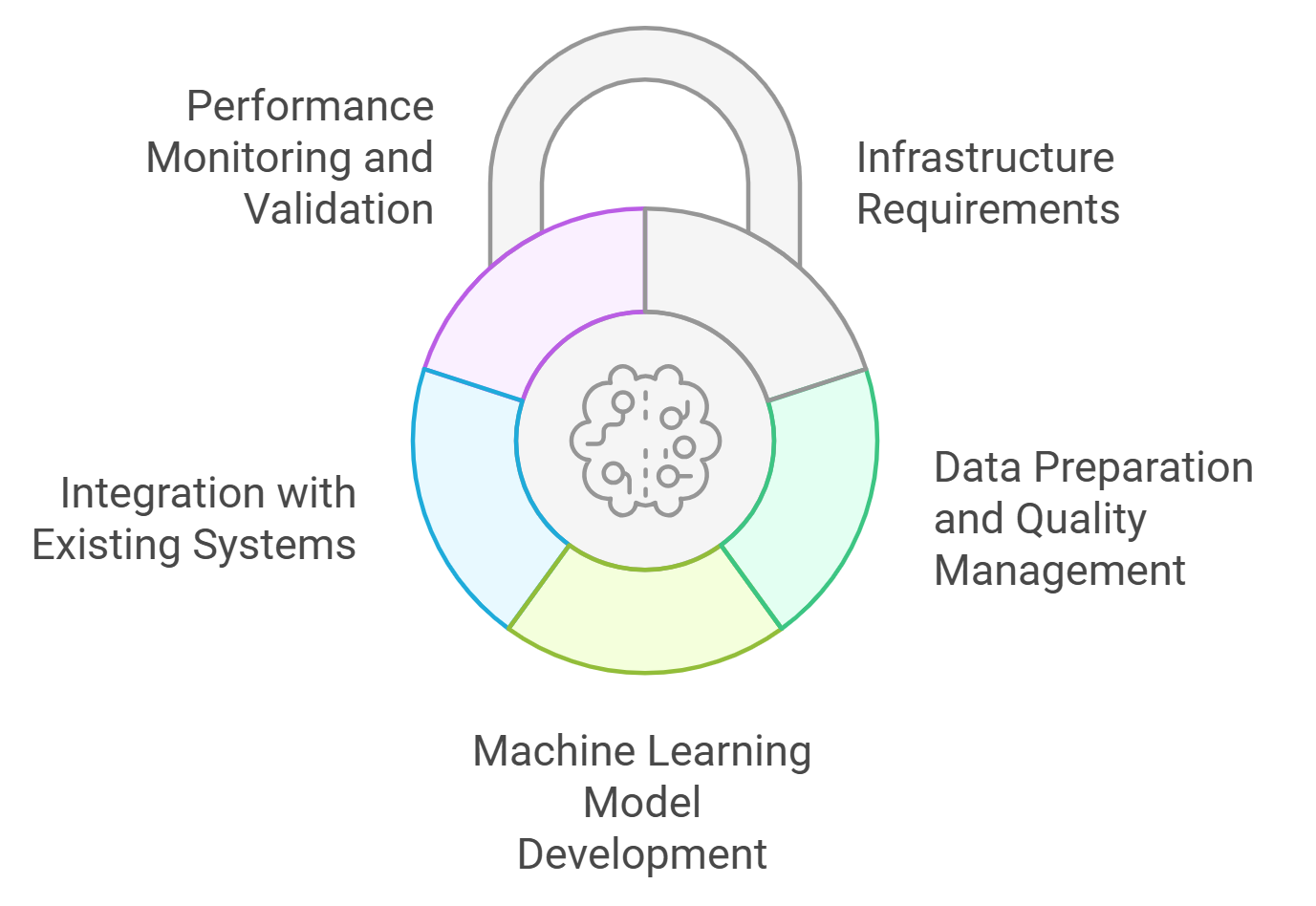
‍
When implementing new strategies or technologies, several considerations must be taken into account to ensure success. These considerations can significantly impact the effectiveness of the implementation process. By carefully considering these factors, organizations can enhance their chances of successful implementation and achieve their desired outcomes.
- Assess organizational readiness for change.
- Identify key stakeholders and involve them in the planning process.
- Develop a clear communication plan to keep everyone informed.
- Allocate sufficient resources, including time and budget, for the implementation.
- Establish a timeline with milestones to track progress.
- Prepare for potential resistance and develop strategies to address concerns.
- Monitor the implementation process and make adjustments as needed.
- Evaluate the outcomes to determine the effectiveness of the implementation.
8.1. Infrastructure Requirements
Infrastructure requirements are critical for the successful implementation of any new system or process. A well-planned infrastructure ensures that the necessary resources are in place to support operations effectively. A robust infrastructure not only supports current operations but also positions organizations for future success. By investing in the right infrastructure, companies can enhance efficiency, improve service delivery, and maintain a competitive edge in their industry.
- Assess current infrastructure capabilities and identify gaps.
- Determine hardware and software needs based on project requirements.
- Ensure network reliability and security to support new technologies.
- Plan for scalability to accommodate future growth and changes.
- Consider cloud solutions for flexibility and cost-effectiveness.
- Evaluate the need for training and support for staff to use new systems.
- Implement backup and disaster recovery solutions to protect data.
- Regularly review and update infrastructure to keep pace with technological advancements.
In a business environment that is constantly changing, continuous learning in business environment is crucial for organizations to thrive and remain relevant.
8.2. Data Preparation and Quality Management
Data preparation is a critical step in the machine learning pipeline. It involves cleaning, transforming, and organizing data to ensure it is suitable for analysis. Quality management in this context refers to the processes that ensure the data is accurate, consistent, and reliable.
- Data Cleaning: This involves identifying and correcting errors or inconsistencies in the dataset. Common tasks include: Â
- Removing duplicates
- Handling missing values
- Correcting data types
- Data Transformation: This step modifies the data into a format that is more suitable for analysis. Techniques include: Â
- Normalization or standardization of numerical values
- Encoding categorical variables
- Feature extraction and selection
- Data Quality Assessment: Regularly assessing data quality is essential. Key metrics include: Â
- Completeness: Are all required data points present?
- Accuracy: Is the data correct and reliable?
- Consistency: Is the data uniform across different datasets?
- Documentation: Keeping detailed records of data sources, transformations, and quality checks is vital for reproducibility and transparency.
Effective data preparation and quality management can significantly enhance the performance of machine learning models. At Rapid Innovation, we emphasize the importance of these processes to ensure that our clients' models achieve optimal accuracy and reliability, ultimately leading to greater ROI. According to a study, poor data quality can lead to a 30% decrease in model accuracy. For more information on how we can assist you with these processes, check out our MLOps consulting services and our best practices for transformer model development.
8.3. Machine Learning Model Development
Machine learning model development is the process of creating algorithms that can learn from and make predictions based on data. This phase involves several key steps:
- Model Selection: Choosing the right algorithm is crucial. Common types include: Â
- Supervised learning (e.g., regression, classification)
- Unsupervised learning (e.g., clustering, dimensionality reduction)
- Reinforcement learning
- Training the Model: This involves feeding the model with training data to learn patterns. Key considerations include: Â
- Splitting data into training, validation, and test sets
- Using techniques like cross-validation to ensure robustness
- Hyperparameter Tuning: Adjusting the model's hyperparameters can significantly impact performance. Techniques include: Â
- Grid search
- Random search
- Bayesian optimization
- Model Evaluation: Assessing the model's performance is essential. Common metrics include: Â
- Accuracy
- Precision and recall
- F1 score
- Iteration: Model development is an iterative process. Based on evaluation results, you may need to revisit earlier steps, such as data preparation or model selection.
The success of machine learning models heavily relies on the quality of the data and the appropriateness of the chosen algorithms. Research indicates that 70% of data science time is spent on data preparation and model tuning. At Rapid Innovation, we leverage our expertise to streamline this process, ensuring that our clients can focus on deriving actionable insights and maximizing their return on investment.
8.4. Integration with Existing Systems
Integrating machine learning models with existing systems is crucial for operationalizing insights and predictions. This process ensures that the models can be effectively utilized within the organization's infrastructure.
- API Development: Creating Application Programming Interfaces (APIs) allows different systems to communicate. Key benefits include: Â
- Real-time predictions
- Easy access to model outputs
- Scalability
- Data Pipeline Integration: Establishing a seamless data flow between the model and existing databases or data warehouses is essential. Considerations include: Â
- Automating data ingestion
- Ensuring data consistency and quality
- User Interface Design: Developing user-friendly interfaces for stakeholders to interact with the model outputs can enhance usability. This may involve: Â
- Dashboards for visualization
- Reporting tools for insights
- Monitoring and Maintenance: Continuous monitoring of model performance is necessary to ensure it remains effective over time. This includes: Â
- Setting up alerts for performance degradation
- Regularly updating the model with new data
- Security and Compliance: Ensuring that the integration adheres to data protection regulations is vital. Key aspects include: Â
- Data encryption
- Access controls
Successful integration of machine learning models can lead to improved decision-making and operational efficiency. According to a report, organizations that effectively integrate AI into their systems can see a 20-30% increase in productivity. At Rapid Innovation, we are committed to helping our clients achieve these results through tailored integration solutions that align with their business objectives.
8.5. Performance Monitoring and Validation
Performance monitoring and validation are critical components of any successful project or operational strategy, especially in the realms of AI and Blockchain. This process ensures that the objectives set forth are being met and that the methods employed are effective. Continuous tracking of performance metrics allows organizations to identify trends and make informed decisions. Regular validation of results ensures that the data collected is accurate and reliable. Monitoring can involve various tools and techniques, including dashboards, reports, and real-time analytics, which can be enhanced through AI-driven insights. It is essential to establish a baseline for performance to measure improvements or declines effectively. Stakeholder engagement is crucial; feedback from team members can provide insights into potential issues or areas for improvement. Performance monitoring should be aligned with organizational goals to ensure that all efforts contribute to the overall mission. Utilizing automated systems, particularly those leveraging Blockchain for data integrity, can enhance the efficiency of monitoring processes, reducing human error and increasing data accuracy. A performance monitoring and evaluation framework can help structure these efforts, while a performance monitoring and evaluation system can provide the necessary tools for effective implementation. For more information on the business impact of Blockchain, visit ROI of Enterprise Blockchain.
9. Performance Metrics and Evaluation
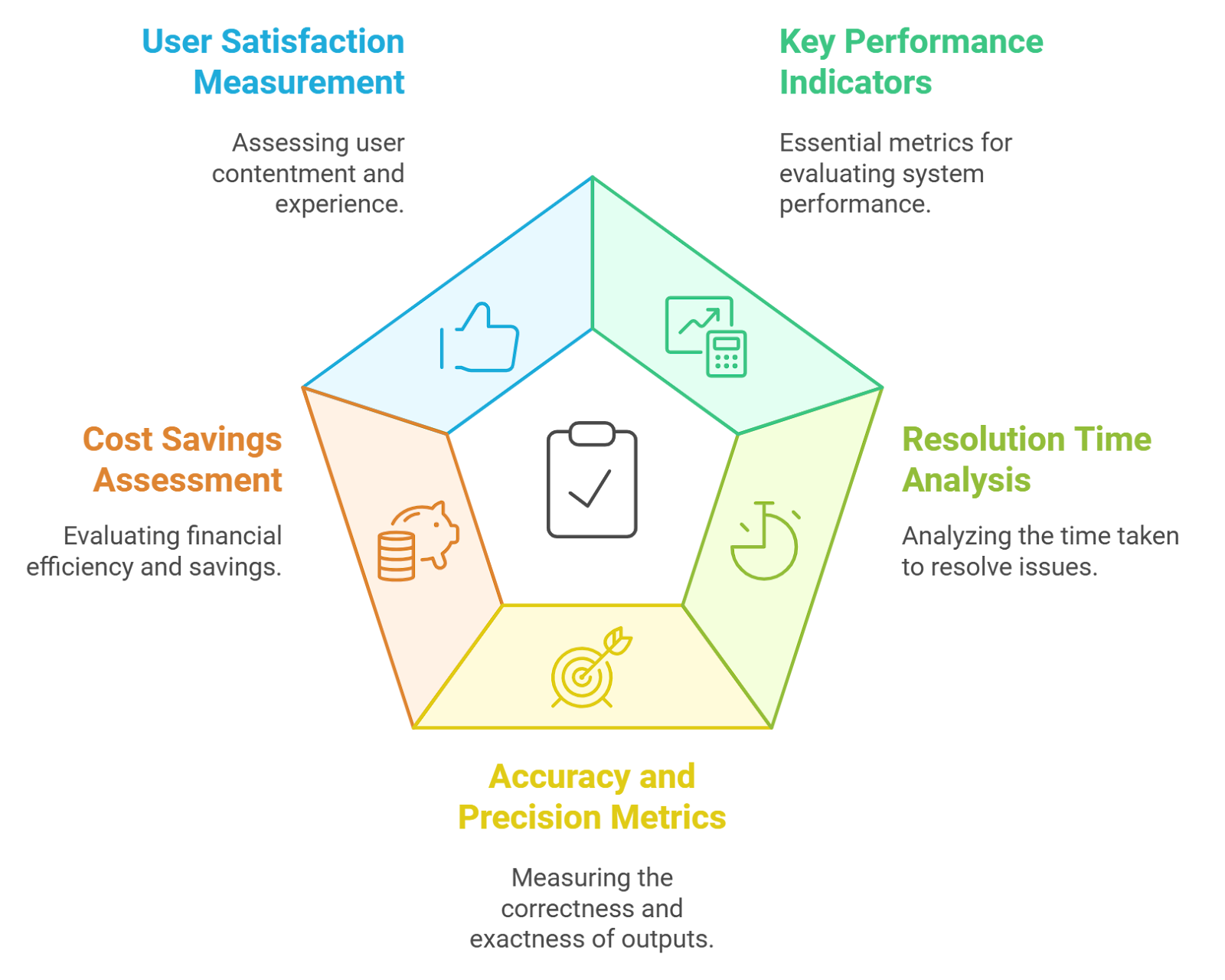
‍
Performance metrics are quantifiable measures used to gauge the success of an organization, project, or individual. Evaluating these metrics helps in understanding the effectiveness of strategies and identifying areas for improvement. Metrics can be categorized into various types, including financial, operational, and customer satisfaction metrics. Regular evaluation of performance metrics allows organizations to pivot strategies as needed. Data visualization tools can help in presenting metrics in an easily digestible format, making it simpler for stakeholders to understand performance. Benchmarking against industry standards can provide context for performance metrics, helping organizations to identify where they stand in comparison to competitors. A systematic approach to evaluation can lead to continuous improvement, fostering a culture of accountability and excellence. Monitoring and evaluating individual performance is also essential to ensure that team members are contributing effectively to organizational goals.
9.1. Key Performance Indicators (KPIs)
Key Performance Indicators (KPIs) are specific metrics that organizations use to measure their success in achieving key business objectives. KPIs are essential for tracking progress and making data-driven decisions. KPIs should be SMART: Specific, Measurable, Achievable, Relevant, and Time-bound. They can vary widely depending on the industry and organizational goals, including sales growth, customer retention rates, and operational efficiency. Regularly reviewing KPIs helps organizations stay aligned with their strategic objectives and make necessary adjustments. Effective communication of KPIs to all stakeholders ensures that everyone understands their role in achieving organizational goals. KPIs can be both leading (predictive) and lagging (historical), providing a comprehensive view of performance. Utilizing technology and software solutions, particularly those powered by AI, can streamline the tracking and reporting of KPIs, enhancing visibility and accountability.
Incorporating performance monitoring, evaluation, and KPIs into an organization’s strategy is vital for sustained success and growth. By focusing on these areas, organizations can ensure they are on the right path to achieving their goals. Rapid Innovation is committed to helping clients leverage these strategies effectively, ensuring that they achieve greater ROI through informed decision-making and optimized performance.
9.2. Resolution Time Analysis
Resolution time analysis is a critical metric in evaluating the efficiency of processes, particularly in customer service and technical support. It measures the time taken to resolve an issue from the moment it is reported until it is fully addressed. Understanding resolution time can help organizations improve their service delivery and customer satisfaction.
- Identifying bottlenecks: By analyzing resolution times, businesses can pinpoint stages in their processes that cause delays, leading to targeted improvements. Rapid Innovation employs AI-driven analytics to identify these bottlenecks, enabling clients to streamline their operations effectively.
- Enhancing customer experience: Shorter resolution times often correlate with higher customer satisfaction. Quick responses can foster loyalty and positive word-of-mouth. Our AI solutions can automate responses and prioritize issues, ensuring that customer queries are addressed promptly.
- Setting benchmarks: Organizations can establish standard resolution times based on historical data, allowing them to set realistic expectations for both staff and customers. Rapid Innovation assists clients in leveraging data analytics to create these benchmarks, enhancing operational transparency.
- Continuous improvement: Regularly reviewing resolution times can help teams identify trends and implement changes that lead to faster resolutions over time. Our consulting services guide organizations in adopting a culture of continuous improvement, utilizing AI tools to monitor and optimize performance. Additionally, organizations can learn from real-world AI implementations to further enhance their resolution strategies.
9.3. Accuracy and Precision Metrics
Accuracy and precision metrics are essential for assessing the quality of data and processes in various fields, including manufacturing, healthcare, and data analysis. These metrics help organizations ensure that their outputs meet the required standards and specifications.
- Accuracy refers to how close a measured value is to the true value. High accuracy means that the results are correct and reliable. Rapid Innovation employs advanced AI algorithms to enhance data accuracy, ensuring that clients make informed decisions.
- Precision, on the other hand, indicates the consistency of measurements. High precision means that repeated measurements yield similar results, even if they are not accurate. Our blockchain solutions can provide immutable records, enhancing the precision of data across various applications.
- Both metrics are crucial for decision-making. For instance, in healthcare, accurate and precise measurements can significantly impact patient outcomes. Rapid Innovation's expertise in AI and blockchain ensures that organizations can trust their data for critical decision-making.
- Organizations can use statistical methods to evaluate accuracy and precision, such as calculating the mean, standard deviation, and confidence intervals. Our consulting services help clients implement these statistical methods effectively, driving better outcomes.
9.4. Cost Savings Assessment
Cost savings assessment is a vital process for organizations looking to improve their financial health and operational efficiency. It involves analyzing expenses to identify areas where costs can be reduced without compromising quality or performance.
- Identifying waste: By assessing costs, organizations can uncover inefficiencies and wasteful practices that can be eliminated or improved. Rapid Innovation utilizes AI analytics to identify these areas, enabling clients to optimize their resource allocation.
- Evaluating alternatives: Cost savings assessments can lead to the exploration of alternative suppliers, materials, or processes that may offer better value. Our blockchain solutions facilitate transparent supplier evaluations, ensuring clients make cost-effective choices.
- Implementing technology: Investing in technology can streamline operations and reduce labor costs, leading to significant savings over time. Rapid Innovation's AI and blockchain solutions empower organizations to automate processes, resulting in substantial cost reductions.
- Measuring impact: Organizations should track the results of cost-saving initiatives to ensure they are achieving the desired financial outcomes. This can involve comparing pre- and post-implementation costs and analyzing return on investment (ROI). Our consulting services provide clients with the tools to measure and report on these impacts effectively, ensuring they realize the full benefits of their investments.
9.5. User Satisfaction Measurement
User satisfaction measurement is a critical aspect of understanding how well a product or service meets the needs and expectations of its users. It involves collecting and analyzing feedback to gauge user experiences and identify areas for improvement, often through user satisfaction surveys.
- Importance of User Satisfaction Â
- Enhances customer loyalty and retention.
- Drives positive word-of-mouth and referrals.
- Informs product development and service enhancements.
- Methods of Measurement Â
- Surveys: Utilize tools like Net Promoter Score (NPS) and Customer Satisfaction Score (CSAT) to gather quantitative data. Examples of user satisfaction survey examples can help in designing effective surveys.
- Interviews: Conduct one-on-one discussions to gain qualitative insights into user experiences.
- Usability Testing: Observe users interacting with the product to identify pain points and areas for improvement.
- Key Metrics Â
- NPS: Measures the likelihood of users recommending the product to others.
- CSAT: Assesses user satisfaction with a specific interaction or overall experience.
- Customer Effort Score (CES): Evaluates how easy it is for users to achieve their goals with the product.
- Analyzing Feedback Â
- Identify trends and patterns in user responses.
- Segment data by demographics or user behavior for deeper insights.
- Use sentiment analysis tools to gauge emotional responses.
- Continuous Improvement Â
- Implement changes based on user feedback.
- Regularly revisit measurement strategies to adapt to evolving user needs.
- Foster a culture of user-centricity within the organization.
10. Challenges and Limitations

‍
While measuring user satisfaction is essential, it comes with its own set of challenges and limitations that can impact the accuracy and effectiveness of the insights gathered.
- Data Collection Issues Â
- Low response rates can skew results.
- Bias in survey design may lead to misleading conclusions.
- Timing of feedback collection can affect user responses.
- Interpretation of Data Â
- Misinterpretation of qualitative feedback can lead to incorrect assumptions.
- Quantitative data may not capture the full user experience.
- Over-reliance on metrics can overshadow qualitative insights.
- Resource Constraints Â
- Limited budget for comprehensive user research.
- Time constraints may hinder thorough analysis.
- Lack of skilled personnel to interpret complex data.
10.1. Data Complexity and Variability
Data complexity and variability present significant challenges in user satisfaction measurement. Understanding these factors is crucial for accurate analysis and actionable insights.
- Nature of Data Â
- User feedback can be diverse and multifaceted, making it difficult to categorize.
- Variability in user expectations and experiences can lead to inconsistent data.
- Data Sources Â
- Multiple channels (surveys, social media, customer support) can produce conflicting information.
- Integrating data from various sources requires sophisticated tools and methodologies.
- Analytical Challenges Â
- Complex data sets may require advanced statistical techniques for meaningful analysis.
- Variability in user demographics can complicate the interpretation of results.
- Strategies to Address Complexity Â
- Use data visualization tools to simplify complex data sets.
- Employ machine learning algorithms to identify patterns and trends.
- Regularly update measurement frameworks to adapt to changing user behaviors.
- Importance of Context Â
- Consider external factors (market trends, economic conditions) that may influence user satisfaction.
- Contextualize data within the broader user journey for a comprehensive understanding.
By addressing these challenges and understanding the complexities of data, organizations can enhance their user satisfaction measurement efforts, leading to improved products and services that better meet user needs. At Rapid Innovation, we leverage AI and blockchain technologies to streamline data collection and analysis, ensuring that our clients can effectively measure user satisfaction and drive greater ROI through informed decision-making. This includes utilizing user satisfaction questionnaires and calculating user satisfaction scores to better understand the user satisfaction gap. Additionally, our expertise in chatbot development can further enhance user interactions and feedback collection. For more insights on how AI can transform customer support, check out our article on AI in Customer Support: Use Cases, Solutions, Development, and Implementation.
10.2. Interpretability of AI Models
Interpretability in AI refers to the degree to which a human can understand the cause of a decision made by an AI model. As AI systems become more complex, particularly with deep learning, the challenge of interpretability grows.
- Importance of Interpretability: Â
- Enhances trust in AI systems, which is crucial for client adoption and satisfaction.
- Facilitates compliance with regulations, such as GDPR, which mandates transparency and accountability in AI decision-making.
- Aids in debugging and improving models by understanding their decision-making processes, ultimately leading to better performance and ROI.
- Techniques for Improving Interpretability: Â
- Model-Agnostic Methods: Techniques like LIME (Local Interpretable Model-agnostic Explanations) and SHAP (SHapley Additive exPlanations) can be applied to any model to explain predictions, allowing clients to gain insights into model behavior. This aligns with the principles of model agnostic explainable AI.
- Simpler Models: Using simpler models like decision trees or linear regression can provide clearer insights into how decisions are made, making it easier for stakeholders to understand and trust the outcomes. This is a key aspect of AI interpretability.
- Visualization Tools: Tools that visualize model behavior can help stakeholders understand how inputs affect outputs, enhancing communication and decision-making processes. This is essential for explainability and interpretability in machine learning.
- Challenges: Â
- Balancing accuracy and interpretability; more complex models often yield better performance but are harder to interpret, which can hinder client confidence.
- The risk of oversimplification, which may lead to misinterpretation of model behavior and potential misalignment with business objectives.
10.3. Handling Unknown Scenarios
Handling unknown scenarios is crucial for the robustness of AI systems. These scenarios refer to situations that the model has not encountered during training, which can lead to unpredictable behavior.
- Importance of Robustness: Â
- Ensures that AI systems can operate effectively in real-world conditions where data may differ from training datasets, thereby minimizing risks for clients.
- Reduces the risk of catastrophic failures in critical applications like healthcare or autonomous driving, which can have significant financial and reputational implications.
- Strategies for Managing Unknown Scenarios: Â
- Data Augmentation: Expanding the training dataset with synthetic data can help models generalize better to unseen situations, improving their reliability and effectiveness.
- Anomaly Detection: Implementing systems that can identify when inputs deviate significantly from the training data can trigger alerts or fallback mechanisms, ensuring continuous operation.
- Continuous Learning: Allowing models to learn from new data over time can help them adapt to changing environments and unknown scenarios, enhancing their long-term value.
- Challenges: Â
- The unpredictability of unknown scenarios makes it difficult to prepare models adequately, which can impact client trust.
- Balancing the need for flexibility with the risk of overfitting to new data, which can lead to performance degradation.
10.4. Ethical and Operational Considerations
The deployment of AI systems raises significant ethical and operational considerations that must be addressed to ensure responsible use.
- Ethical Considerations: Â
- Bias and Fairness: AI models can perpetuate or even exacerbate existing biases present in training data. It is essential to implement fairness audits and bias mitigation strategies to ensure equitable outcomes for all stakeholders.
- Transparency: Stakeholders should understand how AI systems make decisions, which ties back to interpretability and is vital for maintaining client trust. This is closely related to AI interpretability and explainability.
- Accountability: Establishing clear lines of accountability for AI decisions is crucial, especially in high-stakes environments, to mitigate risks and enhance client confidence.
- Operational Considerations: Â
- Integration with Existing Systems: AI solutions must be compatible with current workflows and technologies to ensure smooth implementation, which is a key focus for Rapid Innovation.
- Scalability: As organizations grow, AI systems should be able to scale without significant re-engineering, ensuring that clients can achieve their long-term business goals.
- Maintenance and Monitoring: Continuous monitoring of AI systems is necessary to ensure they perform as expected and to identify any drift in model performance over time, safeguarding client investments.
- Challenges: Â
- Navigating the regulatory landscape, which can vary significantly across regions and industries, requires expertise that Rapid Innovation can provide.
- Balancing innovation with ethical considerations, as rapid advancements in AI can outpace the development of appropriate guidelines and regulations, necessitating a proactive approach to compliance and governance. This includes addressing issues related to model explainability and interpretability, as well as ensuring that explainable and interpretable models in computer vision and machine learning are developed responsibly.
10.5. Bias Mitigation in Diagnostic Processes
Bias in diagnostic processes can lead to significant disparities in healthcare outcomes. It is crucial to identify and mitigate these biases to ensure equitable treatment for all patients.
- Understanding Bias: Bias can stem from various sources, including data collection methods, algorithm design, and clinician decision-making. Recognizing these sources is the first step in addressing them.
- Data Diversity: Ensuring that datasets used for training diagnostic algorithms are diverse and representative of the population is essential. This includes considering factors such as race, gender, age, and socioeconomic status.
- Algorithm Transparency: Developing transparent algorithms allows for better scrutiny and understanding of how decisions are made. This can help identify potential biases in the diagnostic process.
- Regular Audits: Conducting regular audits of diagnostic tools and processes can help identify biases that may have developed over time. This includes analyzing outcomes across different demographic groups.
- Training and Education: Providing training for healthcare professionals on the impact of bias in diagnostics can foster awareness and encourage more equitable practices.
- Patient Involvement: Engaging patients in the diagnostic process can provide valuable insights and help identify biases that may not be apparent to healthcare providers.
- Regulatory Oversight: Implementing regulatory frameworks that require bias assessment in diagnostic tools can help ensure that these tools are fair and effective.
- Collaborative Approaches: Collaborating with interdisciplinary teams, including ethicists and sociologists, can provide a broader perspective on bias and its implications in healthcare.
11. Emerging Technologies and Future Trends
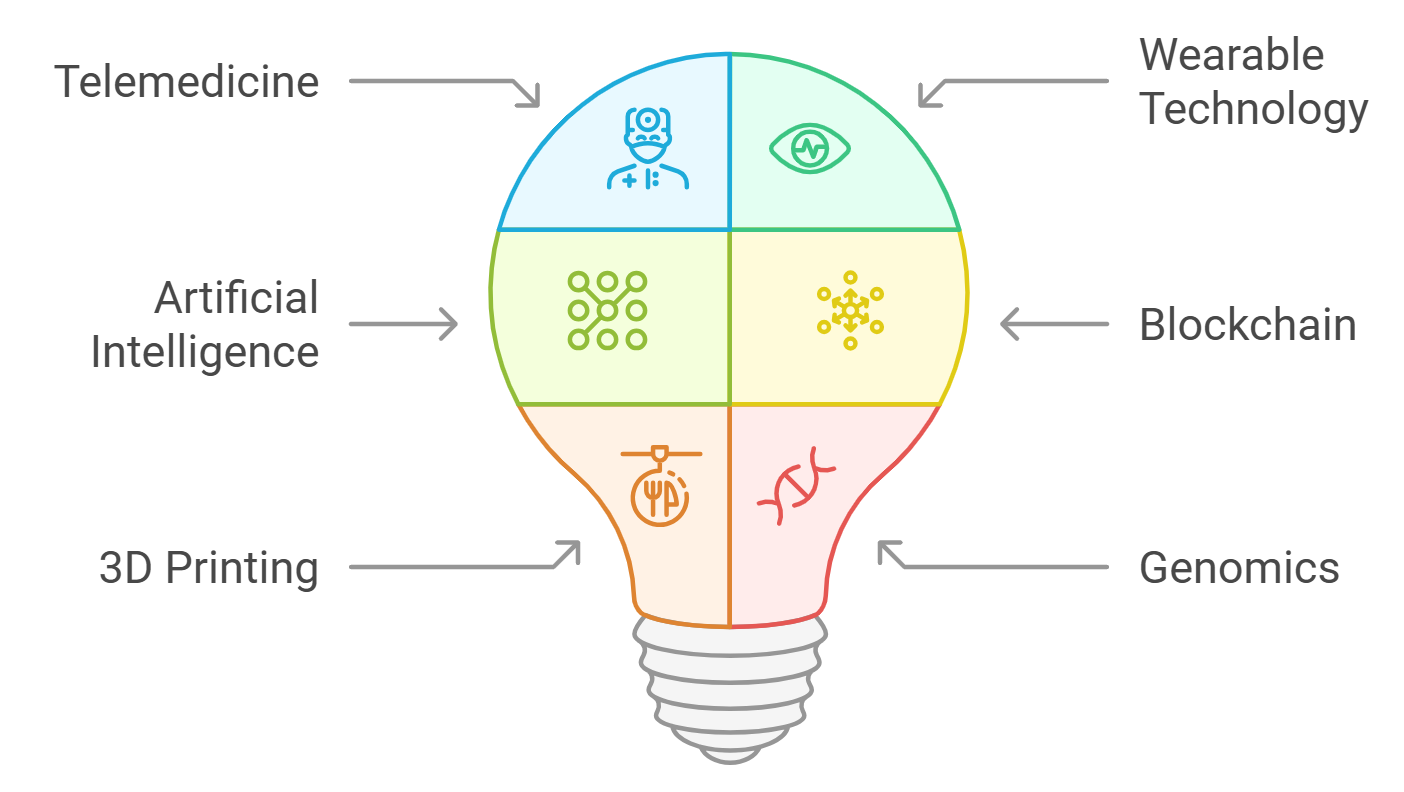
‍
The healthcare landscape is rapidly evolving, driven by emerging technologies that promise to enhance diagnostic processes and patient care. Understanding these trends is vital for healthcare professionals and organizations.
- Telemedicine: The rise of telemedicine has transformed how patients access care, making it more convenient and accessible. This trend is likely to continue, with advancements in virtual consultations and remote monitoring.
- Wearable Technology: Wearable devices are becoming increasingly popular for monitoring health metrics in real-time. This technology can provide valuable data for diagnostics and personalized treatment plans.
- Artificial Intelligence (AI): AI is revolutionizing diagnostics by enabling faster and more accurate analysis of medical data. Machine learning algorithms can identify patterns that may be missed by human clinicians. At Rapid Innovation, we leverage AI to develop tailored diagnostic solutions that enhance accuracy and efficiency, ultimately leading to improved patient outcomes and greater ROI for healthcare providers. Bias mitigation in healthcare is a key focus in our AI development process.
- Blockchain: Blockchain technology offers secure and transparent data sharing, which can enhance patient privacy and improve the integrity of medical records. Rapid Innovation utilizes blockchain to create robust systems that ensure data integrity and security, fostering trust among patients and providers.
- 3D Printing: 3D printing is being used to create customized medical devices and prosthetics, allowing for more personalized patient care.
- Genomics: Advances in genomics are paving the way for precision medicine, where treatments are tailored to an individual's genetic makeup.
11.1. Edge AI and Distributed Intelligence
Edge AI refers to the deployment of artificial intelligence algorithms on local devices rather than relying on centralized cloud computing. This trend is gaining traction due to its numerous advantages in healthcare.
- Real-Time Processing: Edge AI allows for real-time data processing, which is crucial in emergency situations where immediate decisions can save lives.
- Reduced Latency: By processing data closer to the source, edge AI minimizes latency, enhancing the responsiveness of diagnostic tools and applications.
- Data Privacy: Edge AI can improve data privacy by keeping sensitive patient information on local devices rather than transmitting it to the cloud, reducing the risk of data breaches.
- Resource Efficiency: Utilizing distributed intelligence can lead to more efficient use of resources, as devices can operate independently without relying heavily on cloud infrastructure.
- Scalability: Edge AI systems can be easily scaled to accommodate more devices and data sources, making them adaptable to various healthcare settings.
- Interoperability: Edge AI can facilitate better interoperability between devices and systems, allowing for seamless data exchange and improved patient care.
- Enhanced Patient Monitoring: With edge AI, continuous patient monitoring becomes more feasible, enabling timely interventions and better management of chronic conditions.
- Cost-Effectiveness: Implementing edge AI can reduce costs associated with data transmission and cloud storage, making advanced diagnostic tools more accessible to healthcare providers.
In conclusion, bias mitigation in diagnostic processes and the adoption of emerging technologies like edge AI are critical for advancing healthcare. By addressing biases and leveraging innovative solutions, the healthcare industry can improve patient outcomes and ensure equitable access to care. At Rapid Innovation, we are committed to helping our clients navigate these advancements, ensuring they achieve greater ROI while enhancing the quality of care they provide.
11.2. Quantum Computing in Diagnostics
Quantum computing is revolutionizing the field of diagnostics by leveraging the principles of quantum mechanics to process information at unprecedented speeds. This technology has the potential to enhance diagnostic accuracy and efficiency in various medical applications.
- Quantum computers can analyze vast datasets much faster than classical computers, enabling quicker diagnosis.
- They can solve complex problems, such as protein folding and molecular interactions, which are crucial for drug discovery and disease understanding.
- Quantum algorithms can improve imaging techniques, leading to better detection of diseases like cancer at earlier stages.
- The ability to perform multiple calculations simultaneously allows for more comprehensive analysis of patient data, improving personalized medicine approaches.
As research continues, the integration of quantum computing diagnostics into diagnostics could lead to breakthroughs in understanding complex diseases and tailoring treatments to individual patients. At Rapid Innovation, we harness the power of quantum computing to develop cutting-edge diagnostic solutions that drive efficiency and accuracy, ultimately enhancing our clients' return on investment (ROI). For more information on our services, visit our AI EdTech Solutions.
11.3. Advanced Machine Learning Techniques
Advanced machine learning techniques are transforming diagnostics by enabling systems to learn from data and improve over time. These techniques are particularly effective in identifying patterns and making predictions based on large datasets.
- Deep learning, a subset of machine learning, uses neural networks to analyze images, such as X-rays and MRIs, with high accuracy.
- Natural language processing (NLP) allows for the extraction of valuable insights from unstructured data, such as clinical notes and research articles.
- Predictive analytics can forecast disease outbreaks and patient outcomes, aiding in proactive healthcare measures.
- Reinforcement learning can optimize treatment plans by learning from patient responses and adjusting recommendations accordingly.
The application of these advanced machine learning techniques in diagnostics not only enhances accuracy but also streamlines workflows, ultimately improving patient care. Rapid Innovation specializes in implementing these techniques to help our clients achieve greater efficiency and effectiveness in their diagnostic processes, leading to improved ROI.
11.4. Cognitive Computing Approaches
Cognitive computing approaches aim to mimic human thought processes in a computerized model, enhancing the ability to analyze and interpret complex medical data. This technology is particularly useful in diagnostics, where understanding context and nuance is crucial.
- Cognitive systems can process and analyze unstructured data, such as medical literature and patient histories, to provide actionable insights.
- They can assist healthcare professionals in decision-making by offering evidence-based recommendations tailored to individual patient needs.
- These systems can learn from interactions, improving their performance over time and adapting to new information.
- Cognitive computing can enhance telemedicine by providing real-time support to healthcare providers during virtual consultations.
By integrating cognitive computing into diagnostics, healthcare can become more efficient, personalized, and responsive to patient needs. At Rapid Innovation, we leverage cognitive computing to empower healthcare providers with the tools they need to make informed decisions, ultimately driving better patient outcomes and maximizing ROI.
11.5. Autonomous IT Operations (AIOps)
Autonomous IT Operations, commonly referred to as AIOps, is a transformative approach that leverages artificial intelligence (AI) and machine learning (ML) to enhance IT operations. AIOps aims to automate and optimize IT processes, enabling organizations to respond to incidents and manage their infrastructure more efficiently.
- Real-time Data Analysis: AIOps platforms analyze vast amounts of data from various sources in real-time, allowing for quicker identification of issues and anomalies.
- Predictive Analytics: By utilizing historical data, AIOps can predict potential outages or performance degradation, enabling proactive measures to be taken before issues escalate.
- Automated Incident Response: AIOps can automate routine tasks and incident responses, reducing the workload on IT teams and allowing them to focus on more strategic initiatives.
- Enhanced Collaboration: AIOps fosters better collaboration between IT and business units by providing insights that align IT operations with business objectives.
- Cost Efficiency: By automating processes and improving operational efficiency, AIOps can lead to significant cost savings for organizations.
The adoption of AIOps is becoming increasingly critical as organizations face growing complexities in their IT environments. According to a report by Gartner, by 2025, 70% of organizations will be using AIOps to enhance their IT operations. This shift is driven by the need for faster incident resolution and improved service delivery.
At Rapid Innovation, we specialize in aiops implementation tailored to your organization's unique needs. By leveraging our expertise in AI and ML, we help clients streamline their IT operations, resulting in improved service delivery and a higher return on investment (ROI). For instance, one of our clients experienced a 40% reduction in incident response time after integrating our AIOps solution, leading to enhanced customer satisfaction and operational efficiency.
12. Strategic Implementation Roadmap

‍
A strategic implementation roadmap is a structured plan that outlines the steps necessary to achieve specific business objectives. This roadmap is essential for organizations looking to implement new technologies, processes, or initiatives effectively.
- Define Objectives: Clearly outline the goals and objectives of the implementation. This ensures that all stakeholders are aligned and understand the desired outcomes.
- Assess Current State: Evaluate the existing processes, technologies, and resources to identify gaps and areas for improvement.
- Develop a Timeline: Create a realistic timeline for the implementation, including key milestones and deadlines to keep the project on track.
- Allocate Resources: Determine the necessary resources, including budget, personnel, and technology, to support the implementation.
- Risk Management: Identify potential risks and develop mitigation strategies to address them proactively.
- Stakeholder Engagement: Involve key stakeholders throughout the process to ensure buy-in and support for the initiative.
A well-defined strategic implementation roadmap not only guides the execution of projects but also helps in measuring progress and success. It serves as a communication tool that keeps all parties informed and engaged.
12.1. Organizational Readiness Assessment
An organizational readiness assessment is a critical evaluation process that determines whether an organization is prepared to undertake a significant change or initiative. This assessment helps identify strengths, weaknesses, and areas that require attention before implementation.
- Cultural Readiness: Assess the organizational culture to determine if it supports change. A culture that embraces innovation and flexibility is more likely to succeed in implementing new initiatives.
- Skill Assessment: Evaluate the skills and competencies of the workforce to ensure they align with the requirements of the new initiative. Identify any skill gaps that may need to be addressed through training or hiring.
- Technology Infrastructure: Review the existing technology infrastructure to ensure it can support the new initiative. This includes hardware, software, and network capabilities.
- Change Management Processes: Examine the current change management processes to determine if they are robust enough to handle the upcoming changes. Effective change management is crucial for minimizing resistance and ensuring a smooth transition.
- Stakeholder Support: Gauge the level of support from key stakeholders, including leadership and employees. Their buy-in is essential for the success of any initiative.
Conducting an organizational readiness assessment helps organizations identify potential challenges and develop strategies to address them. This proactive approach increases the likelihood of successful implementation and minimizes disruptions. At Rapid Innovation, we guide our clients through this assessment, ensuring they are well-prepared to embrace new technologies and achieve their business goals effectively.
12.2. Pilot Project Development
Pilot project development is a crucial step in testing new ideas, products, or services before a full-scale launch. This phase allows organizations to assess feasibility, gather data, and make necessary adjustments.
- Define objectives: Clearly outline what the pilot aims to achieve, such as testing a new technology or service model. For instance, Rapid Innovation can assist clients in defining specific goals for AI-driven solutions, ensuring alignment with their strategic vision.
- Select a target group: Choose a representative sample of users or customers to participate in the pilot. This helps in obtaining relevant feedback. By leveraging our expertise in blockchain, we can ensure that the selected group reflects the diverse user base that will ultimately benefit from the technology.
- Develop a project plan: Create a detailed plan that includes timelines, resources, and responsibilities. This ensures that all team members are aligned. Rapid Innovation can provide project management tools and methodologies tailored to the unique needs of AI and blockchain projects.
- Implement the pilot project development: Execute the project according to the plan, ensuring that all participants are informed and engaged. Our team can facilitate the implementation process, ensuring that the technology is integrated smoothly into existing systems.
- Monitor and evaluate: Collect data throughout the pilot to assess performance against the defined objectives. Use surveys, interviews, and analytics to gather insights. Rapid Innovation employs advanced analytics tools to provide clients with actionable insights that drive decision-making.
- Adjust and iterate: Based on the feedback and data collected, make necessary changes to improve the project before a wider rollout. Our iterative approach ensures that clients can refine their solutions based on real-world performance, maximizing their return on investment. For accurate planning and budgeting, consider our project estimation services and our business roadmap for custom blockchain development.
Pilot projects can significantly reduce risks associated with new initiatives, allowing organizations to make informed decisions based on real-world data.
12.3. Scalability and Expansion Strategies
Scalability refers to the ability of a project or business to grow and manage increased demand without compromising performance. Developing effective expansion strategies is essential for long-term success.
- Assess current capabilities: Evaluate existing resources, processes, and technologies to determine how well they can support growth. Rapid Innovation can conduct a thorough assessment to identify strengths and weaknesses in your current setup.
- Identify market opportunities: Research potential markets for expansion, considering factors such as demand, competition, and regulatory environment. Our team can leverage AI-driven market analysis tools to uncover lucrative opportunities for blockchain solutions.
- Develop a scalable business model: Create a model that can easily adapt to increased production or service delivery without significant additional costs. Rapid Innovation specializes in designing scalable architectures for AI and blockchain applications, ensuring that clients can grow without constraints.
- Leverage technology: Utilize automation and digital tools to streamline operations and enhance efficiency, making it easier to scale. Our expertise in AI allows us to implement intelligent automation solutions that optimize workflows.
- Build strategic partnerships: Collaborate with other organizations to share resources, knowledge, and networks, facilitating smoother expansion. Rapid Innovation can help clients forge strategic alliances within the blockchain ecosystem to enhance their market presence.
- Monitor performance metrics: Establish key performance indicators (KPIs) to track growth and make data-driven decisions. We provide clients with customized dashboards that visualize performance metrics, enabling proactive management.
By focusing on scalability and expansion strategies, organizations can position themselves for sustainable growth and increased market share.
12.4. Continuous Improvement Framework
A continuous improvement framework is essential for organizations seeking to enhance their processes, products, and services over time. This approach fosters a culture of ongoing development and innovation.
- Establish a baseline: Assess current performance levels to identify areas for improvement. This can involve gathering data on customer satisfaction, operational efficiency, and product quality. Rapid Innovation utilizes AI analytics to establish a comprehensive baseline for performance evaluation.
- Set improvement goals: Define specific, measurable objectives that align with the organization’s overall strategy. These goals should be realistic and achievable. Our team can assist in formulating goals that leverage the unique capabilities of AI and blockchain technologies.
- Implement improvement initiatives: Develop and execute projects aimed at achieving the set goals. This may involve process reengineering, employee training, or technology upgrades. Rapid Innovation offers tailored training programs to ensure that teams are equipped to implement improvements effectively.
- Monitor progress: Regularly review performance against the established goals. Use data analytics and feedback mechanisms to track improvements and identify any issues. Our continuous monitoring solutions provide real-time insights into project performance.
- Foster a culture of feedback: Encourage employees and customers to provide input on processes and products. This can lead to valuable insights and innovative ideas. Rapid Innovation promotes a feedback-driven culture, ensuring that all stakeholders are engaged in the improvement process.
- Review and refine: Continuously assess the effectiveness of improvement initiatives and make adjustments as necessary. This iterative process ensures that the organization remains agile and responsive to change. Our commitment to continuous improvement helps clients stay ahead of the competition.
By adopting a continuous improvement framework, organizations can enhance their competitiveness and adapt to evolving market demands.
12.5. Change Management Considerations
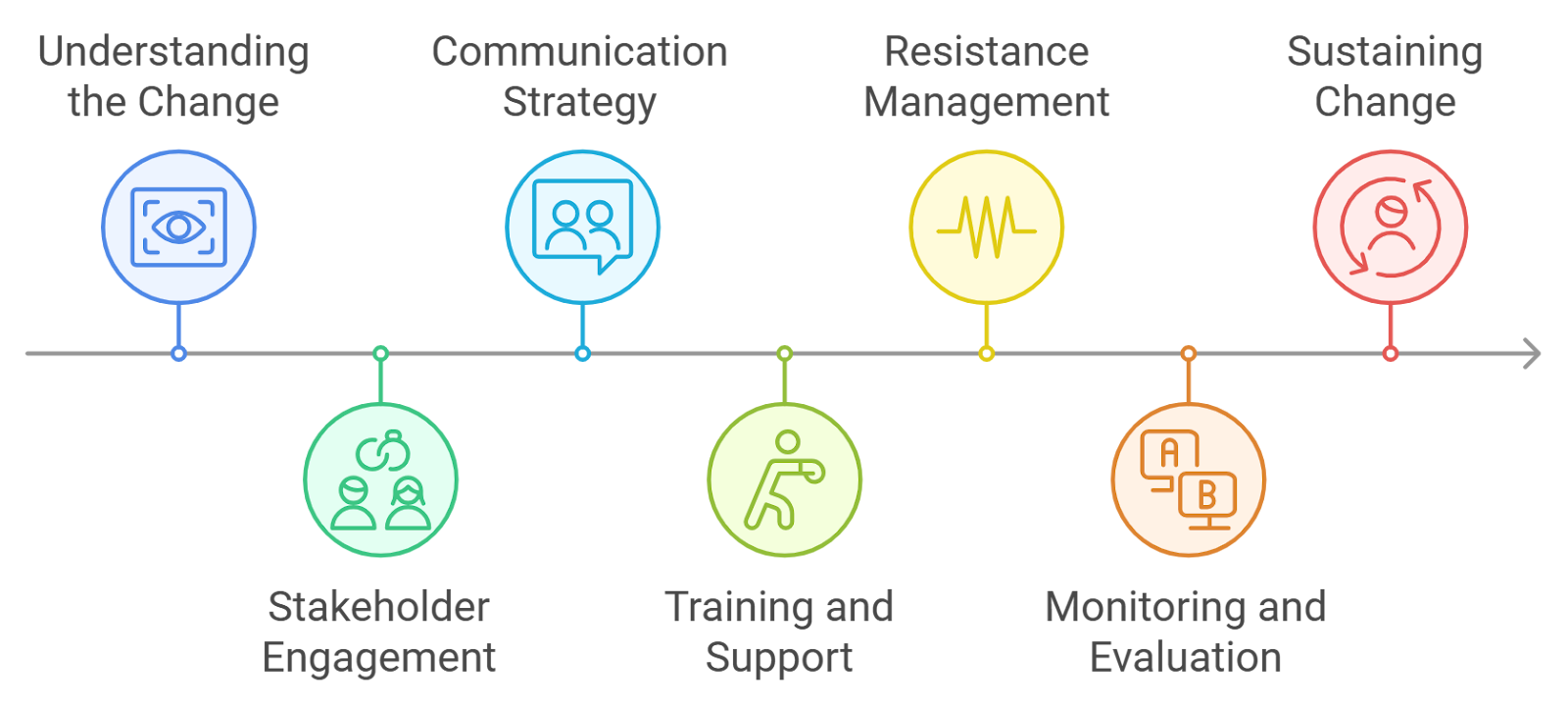
‍
Change management is a critical aspect of any organizational transformation. It involves preparing, supporting, and helping individuals and teams in making organizational change. Effective change management ensures that changes are smoothly and successfully implemented, and that lasting benefits are achieved.
- Understanding the Change: Clearly define the change and its objectives. Communicate the reasons behind the change to all stakeholders. This includes utilizing change management strategies to ensure clarity.
- Stakeholder Engagement: Identify all stakeholders affected by the change. Involve them in the change process to gain their support and insights. Organizational change management strategies can be beneficial in this phase.
- Communication Strategy: Develop a comprehensive communication plan, which is a key component of change management communication plan. Use multiple channels to disseminate information (emails, meetings, newsletters).
- Training and Support: Provide training sessions to equip employees with necessary skills. Offer ongoing support through coaching or mentoring, which is essential in coping with change in the workplace.
- Resistance Management: Anticipate resistance and address concerns proactively. Create a feedback loop to understand employee sentiments and adjust strategies accordingly. Change management techniques can help in managing resistance effectively.
- Monitoring and Evaluation: Establish metrics to measure the success of the change initiative. Regularly review progress and make necessary adjustments. This is part of the strategies for implementing change.
- Sustaining Change: Reinforce the change through recognition and rewards. Embed the change into the organizational culture to ensure longevity. This is crucial for successful organizational transformation strategies.
Effective change management can lead to improved employee morale, increased productivity, and a smoother transition during periods of transformation, especially when employing strategic change management and change management strategies for successful ERP implementation. For organizations looking to enhance their change management processes, consider partnering with experts to hire prompt engineers who can facilitate these transitions effectively.
Additionally, organizations can benefit from examining successful case studies in change management, such as those found in the top 5 successful Solana blockchain projects case studies.
13. Case Studies
Case studies provide real-world examples of how organizations have successfully implemented strategies, overcome challenges, and achieved their goals. They serve as valuable learning tools for other businesses looking to navigate similar situations.
- Learning from Experience: Case studies highlight practical applications of theories and concepts and showcase the impact of strategic decisions on organizational performance.
- Diverse Industries: Case studies span various sectors, including technology, healthcare, finance, and manufacturing. Each industry presents unique challenges and solutions, offering a broad perspective.
- Key Takeaways: Identify best practices and lessons learned from each case. Understand the importance of adaptability and innovation in achieving success.
13.1. Enterprise Success Scenarios
Enterprise success scenarios illustrate how large organizations have effectively navigated challenges and achieved significant milestones. These scenarios often highlight strategic initiatives, innovative solutions, and the role of leadership in driving change.
- Digital Transformation: Many enterprises have embraced digital technologies to enhance operations. Successful examples include companies that have integrated AI and automation to improve efficiency, leading to significant cost savings and enhanced productivity.
- Cultural Change: Organizations that prioritize a positive workplace culture often see higher employee engagement. Case studies show how companies have implemented initiatives to foster inclusivity and collaboration, resulting in lower turnover rates and higher job satisfaction.
- Sustainability Initiatives: Enterprises are increasingly focusing on sustainability as a core business strategy. Success stories include companies that have reduced their carbon footprint and embraced eco-friendly practices, which not only enhance their brand image but also attract environmentally conscious consumers.
- Customer-Centric Approaches: Businesses that prioritize customer experience often outperform their competitors. Case studies reveal how organizations have leveraged data analytics to better understand customer needs, leading to improved product offerings and increased customer loyalty.
- Agile Methodologies: Many enterprises have adopted agile practices to enhance project management. Success scenarios demonstrate how agility leads to faster decision-making and improved project outcomes, allowing organizations to respond swiftly to market changes.
By examining these enterprise success scenarios, organizations can glean insights into effective strategies and practices that can be tailored to their unique contexts. Rapid Innovation stands ready to assist clients in navigating these transformations through our expertise in AI and Blockchain, ensuring that they achieve their business goals efficiently and effectively.
13.2. Industry-Specific Implementations

‍
Industry-specific implementations refer to tailored solutions designed to meet the unique needs and challenges of various sectors, including industry specific erp solutions. These implementations can significantly enhance operational efficiency, customer satisfaction, and overall performance. Here are some key aspects:
- Healthcare: In the healthcare sector, electronic health records (EHR) systems have transformed patient care by allowing for real-time access to patient data, which improves diagnosis and treatment. Additionally, telemedicine has gained traction, enabling remote consultations and reducing the burden on healthcare facilities. Rapid Innovation can assist healthcare providers in integrating AI-driven analytics to enhance patient outcomes and streamline operations.
- Manufacturing: The adoption of Industry 4.0 technologies, such as IoT and AI, has revolutionized manufacturing processes. Smart factories utilize connected devices to monitor production in real-time, leading to reduced downtime and optimized supply chains. Predictive maintenance is another critical implementation that minimizes equipment failures and enhances productivity. Rapid Innovation can help manufacturers implement AI algorithms that predict equipment failures before they occur, thus maximizing ROI.
- Retail: Retailers are leveraging data analytics to personalize customer experiences. Implementations like customer relationship management (CRM) systems help businesses understand consumer behavior, leading to targeted marketing strategies. Furthermore, omnichannel retailing ensures a seamless shopping experience across various platforms. Rapid Innovation can develop AI-powered recommendation engines that enhance customer engagement and drive sales.
- Finance: The financial sector has seen significant advancements through fintech solutions. Mobile banking apps and blockchain technology have improved transaction security and efficiency. Additionally, regulatory compliance tools are essential for managing risks and adhering to industry standards. Rapid Innovation specializes in blockchain solutions that enhance transparency and security in financial transactions, ultimately leading to greater customer trust and satisfaction.
- Education: E-learning platforms have transformed traditional education methods by offering flexible learning options that enable students to access resources anytime, anywhere. Learning management systems (LMS) facilitate course management and student engagement. Rapid Innovation can create AI-driven personalized learning experiences that adapt to individual student needs, improving educational outcomes. Additionally, for organizations looking to implement advanced blockchain solutions, Rapid Innovation can provide expertise in zk-rollup development to enhance their educational platforms.
13.3. Lessons Learned and Best Practices
Implementing industry-specific solutions, including industry specific erp examples, comes with valuable lessons and best practices that can guide future initiatives. Understanding these can help organizations avoid common pitfalls and maximize their investments.
- Engage Stakeholders Early: Involve key stakeholders from the beginning to ensure that the implementation aligns with their needs and expectations. This collaboration fosters buy-in and reduces resistance to change.
- Focus on Training: Comprehensive training programs are essential for successful adoption. Employees should be well-equipped to use new technologies and processes, and ongoing support and resources can help reinforce learning.
- Iterate and Adapt: Flexibility is crucial in implementation. Organizations should be prepared to iterate on their solutions based on feedback and changing market conditions, with continuous improvement as a core principle.
- Measure Success: Establish clear metrics to evaluate the effectiveness of the implementation. Regularly assess performance against these metrics to identify areas for improvement and celebrate successes.
- Prioritize Security: With the increasing reliance on technology, cybersecurity must be a top priority. Implement robust security measures to protect sensitive data and maintain customer trust.
- Leverage Data Analytics: Utilize data analytics to gain insights into performance and customer behavior. This information can inform decision-making and drive strategic initiatives.
14. Conclusion
In conclusion, industry-specific implementations play a crucial role in enhancing operational efficiency and meeting the unique demands of various sectors. By understanding the lessons learned and best practices from these implementations, organizations can navigate challenges more effectively and achieve their goals. Emphasizing stakeholder engagement, training, flexibility, and security will pave the way for successful outcomes. As industries continue to evolve, staying informed about emerging technologies and trends will be essential for maintaining a competitive edge. Rapid Innovation is committed to providing the expertise and tailored solutions necessary to help organizations thrive in this dynamic landscape.
14.1. Key Insights
Understanding the current landscape is crucial for making informed decisions. Here are some key insights that can guide strategic planning:
- Market Trends: The market is increasingly leaning towards digital transformation. Companies that embrace technology, particularly AI and Blockchain solutions, are seeing significant growth and improved operational efficiencies. The focus on digitalization strategy is becoming essential for businesses aiming to stay competitive.
- Consumer Behavior: There is a noticeable shift in consumer preferences towards sustainability and ethical practices. Brands that align with these values tend to foster stronger customer loyalty, especially when they leverage transparent Blockchain technologies to showcase their commitment. Digital transformation consultancy can help organizations navigate these changes effectively.
- Competitive Analysis: Analyzing competitors reveals that those who innovate continuously, particularly through the integration of AI and Blockchain, are more likely to capture market share. Staying ahead of trends, such as digital transformation in business, is essential for maintaining a competitive edge.
14.2. Strategic Recommendations
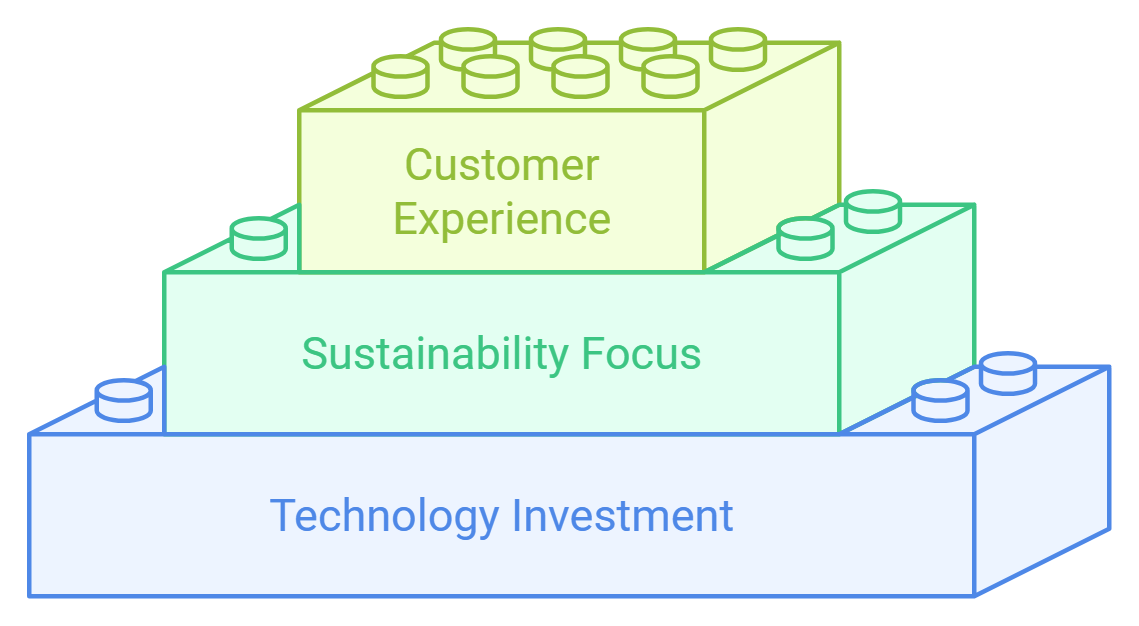
‍
To capitalize on the insights gathered, organizations should consider the following strategic recommendations:
- Invest in Technology: Embrace digital tools and platforms to enhance operational efficiency and customer engagement. This includes adopting AI for predictive analytics and Blockchain for secure transactions and data integrity. A well-defined digitalization strategy can guide these investments.
- Focus on Sustainability: Develop and promote sustainable practices within the organization. This can involve sourcing materials responsibly, reducing waste, and engaging in community initiatives, all of which can be tracked and verified through Blockchain technology.
- Enhance Customer Experience: Prioritize customer feedback and use it to improve products and services. Implementing personalized marketing strategies powered by AI can also increase customer satisfaction and retention, further supported by a robust digital transformation strategy.
14.3. Future Outlook
Looking ahead, several trends and developments are likely to shape the future landscape:
- Continued Digital Evolution: The integration of advanced technologies, particularly AI and Blockchain, will continue to transform industries. Companies that adapt quickly will thrive in this environment, achieving greater ROI through enhanced efficiencies and innovative solutions. The emphasis on digital transformation technologies will be critical.
- Rise of Remote Work: The shift towards remote and hybrid work models is expected to persist. Organizations should invest in tools that facilitate collaboration and communication among remote teams, leveraging AI-driven platforms for seamless interaction.
- Increased Regulatory Focus: As sustainability becomes a priority, regulatory frameworks will likely tighten. Businesses must stay informed and compliant to avoid potential penalties and maintain their reputation, utilizing Blockchain for transparency and accountability in their operations.