Table Of Contents
Category
Artificial Intelligence
Healthcare & Medicine
CRM
Security
Marketing
1. Introduction to AI Transparency
AI transparency refers to the clarity and openness surrounding the operations, decisions, and data used by artificial intelligence systems. As AI technologies become increasingly integrated into various sectors, the demand for transparency in AI has grown significantly. This is crucial for building trust among users, stakeholders, and the general public. AI systems often operate as "black boxes," making it difficult to understand how decisions are made. Transparency can help demystify AI processes, allowing users to comprehend the rationale behind AI-driven outcomes, and it fosters accountability, ensuring that AI systems are used ethically and responsibly.
The importance of AI transparency is underscored by several factors:
- Ethical Considerations: As AI systems impact lives, understanding their decision-making processes is essential to ensure fairness and prevent bias, particularly in machine learning transparency.
- Regulatory Compliance: Governments and organizations are increasingly implementing regulations that require transparency in AI systems, such as the Microsoft transparency principle for responsible AI.
- User Trust: Transparency can enhance user confidence in AI technologies, leading to wider adoption and acceptance, especially in discussions around AI trust and transparency.
At Rapid Innovation, we recognize the critical role of AI model transparency in achieving business goals. By implementing transparent AI solutions, we help our clients not only comply with regulatory requirements but also build trust with their users, ultimately leading to greater ROI. Our expertise in developing AI systems that prioritize transparency allows businesses to leverage data-driven insights while ensuring ethical practices, particularly in the context of transparency in AI ethics. For more information on successful AI integration strategies.
In summary, AI transparency is a vital aspect of the ongoing development and deployment of AI technologies, influencing ethical practices, regulatory frameworks, and user trust, including the broader conversation about transparency in AI.
1.1. Defining AI Transparency
AI transparency refers to the clarity and openness with which artificial intelligence systems operate. It encompasses the ability to understand how AI models make decisions, the data they use, and the algorithms that drive their functionality. Transparency is crucial for building trust among users and stakeholders, as it allows them to comprehend the rationale behind AI-driven outcomes, particularly in areas like ai trust and transparency.
Key aspects of AI transparency include:
- Explainability: The degree to which an AI system can provide understandable explanations for its decisions. This is vital in sectors like healthcare and finance, where decisions can significantly impact lives and livelihoods, highlighting the importance of transparency in ai ethics.
- Data provenance: Understanding where the data comes from, how it is collected, and how it is processed. This helps in assessing the quality and bias of the data used in AI models, which is essential for machine learning transparency.
- Algorithmic accountability: Ensuring that the algorithms used in AI systems are fair, unbiased, and ethical. This involves scrutinizing the decision-making processes to prevent discrimination and promote fairness, a key aspect of ai model transparency.
At Rapid Innovation, we prioritize AI transparency in our development and consulting solutions. By implementing transparent AI systems, we help our clients build trust with their users, ultimately leading to greater adoption and improved ROI. Learn more about ethical AI development.
1.2. Historical Context and Evolution
The concept of AI transparency has evolved alongside the development of artificial intelligence itself. Initially, AI systems were often viewed as "black boxes," where the inner workings were not visible or understandable to users. Over the years, several key milestones have shaped the discourse around AI transparency:
- Early AI Development: In the 1950s and 1960s, AI research focused on rule-based systems, which were relatively transparent. However, as machine learning techniques gained prominence in the 1980s and 1990s, the complexity of models increased, leading to less transparency.
- Rise of Deep Learning: The advent of deep learning in the 2010s introduced highly complex neural networks that often operate without clear explanations for their decisions. This complexity raised concerns about accountability and trust, emphasizing the need for transparency in ai.
- Regulatory Developments: In recent years, there has been a growing push for regulations that mandate transparency in AI systems. Initiatives like the European Union's General Data Protection Regulation (GDPR) have emphasized the need for explainability in automated decision-making processes, reinforcing the principles of transparency in ai ethics.
At Rapid Innovation, we stay ahead of these regulatory developments, ensuring that our AI solutions not only comply with current standards but also anticipate future requirements, thereby safeguarding our clients' investments.
1.3. Importance in Modern AI Systems
AI transparency is increasingly recognized as a fundamental requirement for the responsible deployment of AI technologies. Its importance can be highlighted through several key points:
- Building Trust: Transparency fosters trust among users, stakeholders, and the general public. When people understand how AI systems work, they are more likely to accept and adopt these technologies, which is crucial for ai transparency.
- Enhancing Accountability: Transparent AI systems allow for better accountability. When decisions can be traced back to specific data and algorithms, it becomes easier to identify and rectify errors or biases, contributing to machine learning model transparency.
- Facilitating Compliance: As regulations around AI continue to evolve, transparency is essential for compliance. Organizations must be able to demonstrate that their AI systems adhere to ethical standards and legal requirements, aligning with the principles of the microsoft transparency principle for responsible ai.
- Improving Performance: Understanding the decision-making processes of AI systems can lead to improvements in model performance. By analyzing how decisions are made, developers can identify areas for enhancement and optimization, which is a key aspect of transparency machine learning.
- Encouraging Ethical AI Development: Transparency promotes ethical considerations in AI development. By making the workings of AI systems visible, developers are more likely to consider the societal implications of their technologies, reinforcing the importance of transparency in ai.
In conclusion, AI transparency is a critical aspect of modern artificial intelligence systems, influencing trust, accountability, compliance, performance, and ethical development. As AI continues to permeate various sectors, the demand for transparency will only grow, shaping the future of technology and its impact on society. At Rapid Innovation, we are committed to helping our clients navigate this landscape, ensuring that their AI initiatives are not only effective but also responsible and transparent, ultimately leading to greater ROI.
1.4. Key Stakeholders in AI Transparency
AI transparency is a critical aspect of developing and deploying artificial intelligence systems. Various stakeholders play significant roles in ensuring that AI technologies are transparent, accountable, and ethical. Understanding these stakeholders is essential for fostering trust and collaboration in the AI ecosystem.
- Developers and Engineers: These individuals are responsible for creating AI algorithms and systems. They must prioritize transparency in their designs, ensuring that the models are interpretable and that their decision-making processes can be understood by users and stakeholders.
- Organizations and Companies: Businesses that implement AI technologies must adopt transparent practices. This includes providing clear information about how AI systems work, the data they use, and the potential biases that may arise. Companies should also be accountable for the outcomes of their AI applications. At Rapid Innovation, we assist organizations in integrating transparent AI practices, enhancing their operational efficiency and trustworthiness, which ultimately leads to greater ROI.
- Regulators and Policymakers: Government bodies and regulatory agencies play a crucial role in establishing guidelines and standards for AI transparency. They can create frameworks that require organizations to disclose information about their AI systems, ensuring compliance with ethical and legal standards.
- Academics and Researchers: Scholars contribute to the understanding of AI transparency through research and analysis. They study the implications of AI technologies and advocate for best practices in transparency, helping to inform policy and industry standards.
- End Users: Individuals who interact with AI systems, whether as consumers or employees, are vital stakeholders. They should be informed about how AI impacts their lives and have access to information that allows them to understand and question AI decisions.
- Civil Society and Advocacy Groups: Non-profit organizations and advocacy groups work to promote ethical AI practices. They often push for greater transparency and accountability in AI systems, representing the interests of marginalized communities and ensuring that AI technologies do not perpetuate discrimination.
- Media and Journalists: The media plays a key role in raising awareness about AI transparency issues. Investigative journalism can uncover hidden biases and unethical practices in AI, prompting public discourse and encouraging organizations to adopt more transparent practices.
2. Fundamentals of Transparent AI Agents

Transparent AI agents are designed to operate in a way that their actions and decisions can be easily understood by humans. This transparency is essential for building trust and ensuring that AI systems are used responsibly. The fundamentals of transparent AI agents include several key principles and practices.
- Explainability: AI agents should provide clear explanations for their decisions. This means that users can understand the reasoning behind an AI's actions, which is crucial for trust and accountability.
- Data Transparency: It is important for AI systems to disclose the data they use for training and decision-making. Users should know where the data comes from, how it is processed, and any potential biases that may exist in the dataset.
- User Control: Transparent AI agents should empower users by giving them control over how the AI operates. This includes allowing users to set preferences, provide feedback, and even override AI decisions when necessary.
- Ethical Guidelines: AI agents should adhere to ethical principles that prioritize fairness, accountability, and respect for user privacy. This includes implementing safeguards to prevent discrimination and ensuring that AI systems are used for the benefit of society.
- Continuous Monitoring: Transparency is not a one-time effort; it requires ongoing monitoring and evaluation. AI systems should be regularly assessed for performance, bias, and compliance with ethical standards.
2.1. Core Components
The core components of transparent AI agents are essential for ensuring that these systems operate effectively and ethically. Each component contributes to the overall transparency and accountability of AI technologies.
- Model Interpretability: This refers to the ability to understand how an AI model makes decisions. Techniques such as feature importance analysis and visualization tools can help users grasp the inner workings of AI algorithms.
- Documentation: Comprehensive documentation is vital for transparency. This includes detailed descriptions of the AI system's architecture, data sources, and decision-making processes. Proper documentation helps stakeholders understand the system's capabilities and limitations.
- User Interfaces: The design of user interfaces plays a crucial role in transparency. Interfaces should be intuitive and provide users with relevant information about the AI's actions, including explanations and options for feedback.
- Feedback Mechanisms: Incorporating feedback loops allows users to provide input on AI decisions. This can help improve the system's performance and ensure that it aligns with user expectations and ethical standards.
- Audit Trails: Maintaining a record of AI decisions and actions is essential for accountability. Audit trails enable stakeholders to review the history of decisions made by the AI, facilitating transparency and trust.
- Compliance Frameworks: Establishing compliance frameworks ensures that AI systems adhere to legal and ethical standards. This includes regular audits and assessments to verify that the AI operates transparently and responsibly.
- Collaboration Tools: Tools that facilitate collaboration among ai transparency stakeholders can enhance transparency. These tools allow developers, users, and regulators to work together, share insights, and address concerns related to AI transparency.
By focusing on these core components, organizations can develop transparent AI agents that foster trust and accountability, ultimately leading to more responsible AI deployment. At Rapid Innovation, we leverage our expertise in AI to help clients implement these principles, ensuring that their AI systems are not only effective but also transparent and trustworthy, thereby maximizing their return on investment.
2.2. Architectural Frameworks
Architectural frameworks in technology refer to the structured approach to designing and implementing systems. They provide a blueprint that guides the development process, ensuring that all components work together effectively. In the context of software development and artificial intelligence, architectural frameworks play a crucial role in defining how different parts of a system interact.
- Types of Architectural Frameworks:
- Layered Architecture: This divides the system into layers, each with specific responsibilities, promoting separation of concerns.
- Microservices Architecture: This approach breaks down applications into smaller, independent services that can be developed and deployed separately, enhancing flexibility and scalability.
- Event-Driven Architecture: This framework focuses on the production, detection, consumption of, and reaction to events, allowing for real-time processing and responsiveness.
- Benefits of Architectural Frameworks:
- Improved Scalability: Frameworks allow systems to grow and adapt to increased loads without significant redesign, which is essential for businesses aiming for growth.
- Enhanced Maintainability: A well-defined architecture makes it easier to update and maintain systems over time, reducing operational costs and downtime.
- Better Collaboration: Clear frameworks facilitate teamwork by providing a common understanding of system components and interactions, leading to more efficient project execution.
- Examples of Architectural Frameworks:
- The Zachman Framework: A schema for organizing architectural artifacts that can help in aligning business goals with IT strategies.
- The TOGAF Framework: A method for developing enterprise architecture that supports the integration of AI and blockchain solutions.
- The 4+1 View Model: A framework that organizes the architecture of a system into five concurrent views, ensuring comprehensive coverage of all aspects of the system.
- The Federal Enterprise Architecture Framework: A framework that provides a common approach for the federal government to integrate and manage IT investments.
- The Department of Defense Architecture Framework (DoDAF): A framework that provides a structure for the development of architectures within the Department of Defense.
- The SABSA Framework: A framework for developing risk-driven enterprise security architectures.
- The Open Group Architecture Framework (TOGAF): A widely used framework for enterprise architecture that provides a comprehensive approach to design and implementation.
Architectural frameworks are essential for ensuring that complex systems are built efficiently and can evolve over time to meet changing needs. At Rapid Innovation, we leverage these frameworks, including TOGAF, DoDAF, and the Zachman Framework, to help clients streamline their development processes, ultimately leading to greater ROI. For developers interested in the integration of AI, you can explore more about implementing explainable AI for transparent agent decisions.
2.3. Transparency Mechanisms
Transparency mechanisms are essential in various fields, particularly in technology and governance, to ensure that processes and decisions are open and understandable. These mechanisms help build trust among users and stakeholders by providing insight into how systems operate.
- Importance of Transparency:
- Builds Trust: When users understand how decisions are made, they are more likely to trust the system, which is crucial for user adoption of AI and blockchain technologies.
- Enhances Accountability: Transparency allows stakeholders to hold organizations accountable for their actions, fostering a culture of responsibility.
- Facilitates Compliance: Many regulations require transparency in processes, especially in data handling and financial reporting, which is vital for businesses operating in regulated industries.
- Types of Transparency Mechanisms:
- Open Data Initiatives: Providing access to data sets for public scrutiny, which can enhance stakeholder engagement.
- Audit Trails: Keeping detailed records of actions taken within a system to track changes and decisions, ensuring accountability.
- User Interfaces: Designing systems with clear, understandable interfaces that explain how decisions are made, improving user experience.
- Challenges in Implementing Transparency:
- Complexity of Systems: As systems become more complex, it can be challenging to present information in an understandable way.
- Data Privacy Concerns: Balancing transparency with the need to protect sensitive information can be difficult, especially in AI applications.
- Resistance to Change: Organizations may be hesitant to adopt transparency measures due to fear of scrutiny.
Transparency mechanisms are vital for fostering an environment of trust and accountability, particularly in sectors like finance, healthcare, and technology. Rapid Innovation helps clients implement these mechanisms effectively, ensuring compliance and enhancing user trust.
2.4. Explainability vs. Interpretability
Explainability and interpretability are two critical concepts in the field of artificial intelligence and machine learning. They refer to how well users can understand the decisions made by AI systems.
- Explainability:
- Definition: Explainability refers to the degree to which an external observer can understand why an AI system made a specific decision.
- Importance: As AI systems are increasingly used in decision-making processes, explainability is crucial for ensuring that users can trust and validate the outcomes.
- Techniques: Common methods for enhancing explainability include:
- Feature Importance: Highlighting which features most influenced a decision.
- Decision Trees: Using simple models that are easy to follow and understand.
- Interpretability:
- Definition: Interpretability is the extent to which a human can comprehend the cause of a decision made by an AI system.
- Importance: Interpretability is essential for users to grasp the underlying logic of AI models, especially in high-stakes applications like healthcare and criminal justice.
- Techniques: Approaches to improve interpretability include:
- Simplified Models: Using models that are inherently easier to understand, such as linear regression.
- Visualization Tools: Providing graphical representations of model behavior and decision processes.
- Key Differences:
- Explainability focuses on the external understanding of a model's decisions, while interpretability emphasizes the internal logic and reasoning behind those decisions.
- Explainable models may not always be interpretable, especially in complex systems like deep learning, where the decision-making process can be opaque.
Understanding the distinction between explainability and interpretability is crucial for developing AI systems that are not only effective but also trustworthy and user-friendly. At Rapid Innovation, we prioritize these aspects to ensure that our AI solutions deliver value while maintaining user confidence.
2.5. Types of Transparent AI Agents
Transparent AI agents can be categorized into several types based on their design, functionality, and the level of transparency they offer. Understanding these types is crucial for developers and users alike, as it helps in selecting the right AI solution for specific needs.
- Rule-Based Agents: These agents operate on predefined rules and logic. Their decision-making process is straightforward, making it easy for users to understand how conclusions are reached. For example, a rule-based chatbot can follow a script to answer customer queries, providing businesses with a reliable tool for customer support.
- Explainable AI (XAI): XAI focuses on making AI decisions interpretable to humans. These agents provide insights into their reasoning processes, often using visualizations or natural language explanations. This type is particularly important in sectors like healthcare and finance, where understanding the rationale behind decisions is critical. Rapid Innovation can implement XAI solutions that enhance decision-making processes, leading to improved outcomes and greater ROI for clients.
- Interactive Agents: These agents engage with users in real-time, adapting their responses based on user input. They often incorporate feedback mechanisms that allow users to understand the agent's thought process. For instance, virtual assistants like Siri or Alexa can explain their actions based on user queries, which can be leveraged by businesses to create more engaging customer experiences.
- Self-Explaining Agents: These AI systems are designed to automatically generate explanations for their actions. They utilize machine learning techniques to analyze their decision-making processes and provide users with insights. This type is still in development but holds promise for enhancing user understanding, which can be a game-changer for organizations looking to improve user trust and satisfaction.
- Hybrid Agents: Combining elements from various types, hybrid agents leverage the strengths of rule-based systems and machine learning. They can provide clear explanations while also adapting to new data, making them versatile for different applications. Rapid Innovation can develop hybrid agents tailored to specific business needs, ensuring clients achieve optimal performance and ROI. For more information on how we can assist you, visit our AI technology consulting company.
3. Benefits of AI Transparency
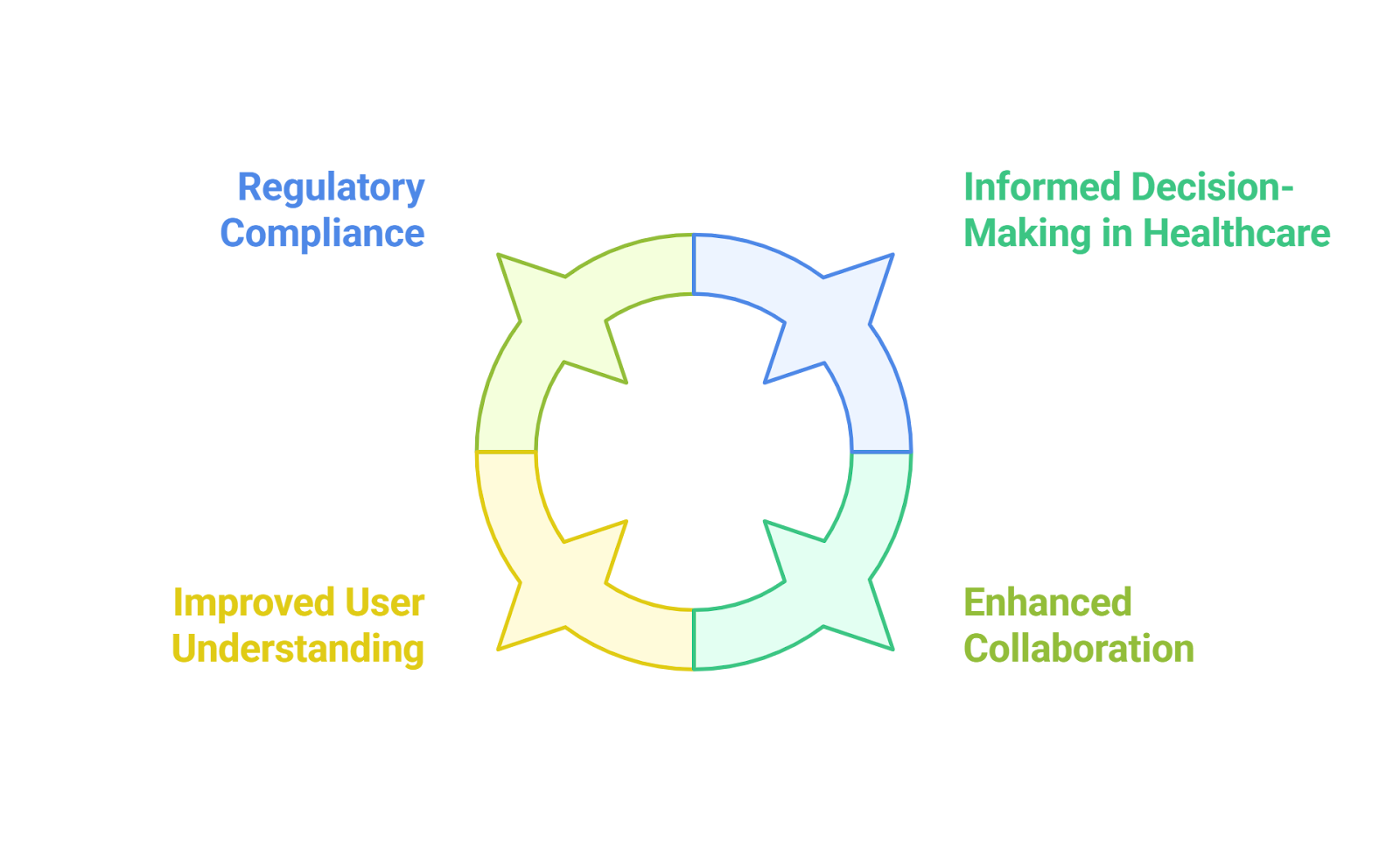
AI transparency offers numerous advantages that can significantly impact user experience, trust, and overall effectiveness of AI systems. Here are some key benefits:
- Improved User Understanding: Transparent AI systems allow users to grasp how decisions are made, which can enhance their interaction with the technology. This understanding can lead to better user engagement and satisfaction, ultimately driving business success.
- Informed Decision-Making: When users can see the reasoning behind AI decisions, they can make more informed choices. This is particularly important in critical areas like healthcare, where understanding the rationale can influence treatment options. Rapid Innovation's AI solutions empower clients to make data-driven decisions that enhance operational efficiency.
- Regulatory Compliance: Many industries are subject to regulations that require transparency in decision-making processes. Transparent AI can help organizations comply with these regulations, reducing the risk of legal issues and associated costs.
- Enhanced Collaboration: Transparency fosters collaboration between humans and AI. When users understand how AI systems operate, they can work more effectively alongside them, leveraging the strengths of both. This collaborative approach can lead to innovative solutions and improved business outcomes.
3.1. Enhanced Trust and Accountability
One of the most significant benefits of AI transparency is the enhancement of trust and accountability. This is crucial for the widespread adoption of AI technologies across various sectors.
- Building Trust: When users can see how AI systems arrive at their conclusions, they are more likely to trust the technology. Trust is essential for user acceptance, especially in sensitive areas like finance, healthcare, and law enforcement. Rapid Innovation's commitment to transparency helps clients build trust with their users, fostering long-term relationships.
- Accountability Mechanisms: Transparent AI systems allow for better accountability. If an AI makes a mistake, users can trace back the decision-making process to understand what went wrong. This accountability is vital for organizations to address errors and improve their systems, ultimately leading to better performance and ROI.
- Ethical Considerations: Transparency in AI can help address ethical concerns. By making the decision-making process visible, organizations can ensure that their AI systems are fair and unbiased. This is particularly important in preventing discrimination and ensuring equitable outcomes, which can enhance a company's reputation and customer loyalty.
- User Empowerment: Transparency empowers users by giving them the information they need to question and challenge AI decisions. This can lead to a more democratic approach to technology, where users feel they have a say in how AI impacts their lives.
- Reputation Management: Organizations that prioritize transparency in their AI systems can enhance their reputation. Being seen as a responsible and ethical user of AI can attract customers and partners who value these principles, ultimately contributing to greater business success.
In conclusion, the types of transparent AI agents, including extraamas, and the benefits of AI transparency, particularly in enhancing trust and accountability, are essential considerations for anyone involved in the development or use of AI technologies. By focusing on transparency, organizations can create more effective, ethical, and user-friendly AI systems, driving greater ROI and achieving their business goals efficiently and effectively. Rapid Innovation is dedicated to helping clients navigate this landscape, ensuring they leverage the full potential of transparent AI agents.
3.1.1. Stakeholder Confidence
Stakeholder confidence is crucial for the success of any organization. It refers to the trust and assurance that stakeholders—such as investors, employees, customers, and suppliers—have in the organization's ability to deliver on its promises and maintain ethical standards. Building and maintaining this confidence can lead to several benefits:
- Enhanced reputation: A strong reputation attracts new customers and retains existing ones.
- Increased investment: Investors are more likely to invest in companies they trust.
- Employee loyalty: Employees are more engaged and productive when they believe in their organization’s mission and values.
- Customer satisfaction: Trust leads to higher customer satisfaction and loyalty, which can drive sales and profitability.
To foster stakeholder confidence, organizations should focus on transparency, effective communication, and ethical practices. Regular updates on company performance, clear communication of goals, and adherence to ethical standards can significantly enhance stakeholder trust. At Rapid Innovation, we leverage AI-driven analytics to provide stakeholders with real-time insights into organizational performance, thereby reinforcing stakeholder confidence.
3.1.2. Audit Trails
Audit trails are essential for maintaining accountability and transparency within an organization. An audit trail is a chronological record that traces the steps taken in a process, allowing for the tracking of changes and decisions made over time. The importance of audit trails includes:
- Compliance: Many industries are subject to regulations that require maintaining detailed records. Audit trails help organizations comply with these regulations.
- Error detection: By reviewing audit trails, organizations can identify errors or discrepancies in processes, enabling timely corrections.
- Fraud prevention: A well-maintained audit trail can deter fraudulent activities by providing a clear record of actions taken.
- Performance evaluation: Audit trails allow organizations to assess the effectiveness of their processes and make informed decisions for improvement.
Implementing robust audit trails involves using technology to automate record-keeping and ensure that all actions are logged accurately. This not only enhances security but also simplifies the process of retrieving information when needed. Rapid Innovation employs blockchain technology to create immutable audit trails, ensuring that all transactions are transparent and verifiable, which significantly enhances organizational integrity.
3.1.3. Decision Validation
Decision validation is the process of ensuring that decisions made within an organization are based on accurate data and sound reasoning. This practice is vital for minimizing risks and maximizing the effectiveness of strategic initiatives. Key aspects of decision validation include:
- Data-driven decisions: Utilizing data analytics and business intelligence tools can help organizations make informed decisions based on real-time data.
- Scenario analysis: Evaluating different scenarios and their potential outcomes can provide insights into the best course of action.
- Stakeholder input: Involving relevant stakeholders in the decision-making process can lead to more comprehensive and well-rounded decisions.
- Continuous improvement: Regularly reviewing past decisions and their outcomes can help organizations refine their decision-making processes over time.
By prioritizing decision validation, organizations can enhance their strategic planning and operational efficiency, ultimately leading to better outcomes and increased competitiveness in the market. At Rapid Innovation, we integrate AI solutions that facilitate data-driven decision-making, ensuring that our clients can navigate complex business landscapes with confidence and achieve greater ROI.
3.2. Improved Decision Making
In today's fast-paced world, improved decision-making is crucial for businesses and individuals alike. Artificial Intelligence (AI) plays a significant role in enhancing decision-making processes by providing data-driven insights and predictive analytics. By leveraging AI technologies, organizations can make more informed choices, reduce risks, and optimize outcomes.
- AI systems analyze vast amounts of data quickly, identifying patterns and trends that humans might overlook.
- Enhanced decision-making leads to increased efficiency and productivity.
- AI can simulate various scenarios, allowing decision-makers to evaluate potential outcomes before taking action.
3.2.1. Understanding AI Reasoning
Understanding how AI systems reason is essential for harnessing their full potential in decision-making. AI reasoning involves the processes through which machines interpret data, draw conclusions, and make recommendations.
AI utilizes algorithms and models to process information and generate insights. Machine learning, a subset of AI, enables systems to learn from data and improve over time. Additionally, Explainable AI (XAI) is an emerging field focused on making AI reasoning transparent and understandable to users.
By grasping AI reasoning, decision-makers can better trust and utilize AI-generated insights, leading to more effective strategies. This understanding is particularly relevant in contexts such as AI decision making, AI and decision making, and artificial intelligence and decision making.
3.2.2. Validation of Outputs
Validation of outputs is a critical step in ensuring the reliability and accuracy of AI-driven decisions. It involves assessing the results produced by AI systems to confirm their validity before implementation.
- Regularly testing AI models against real-world data helps identify discrepancies and improve accuracy.
- Cross-validation techniques can be employed to ensure that AI outputs are consistent across different datasets.
- Stakeholder involvement in the validation process fosters trust and acceptance of AI-generated recommendations.
By prioritizing the validation of outputs, organizations can mitigate risks associated with erroneous AI decisions and enhance overall decision-making quality. This is especially important in areas like AI driven decision making and AI decision maker. At Rapid Innovation, we specialize in implementing robust AI solutions that not only streamline decision-making processes but also ensure that the insights generated are reliable and actionable, ultimately driving greater ROI for our clients. Our expertise extends to AI for decision making, AI for investment decisions, and AI decision making examples, ensuring that we provide comprehensive support in the realm of AI as a service and artificial intelligence driven decision making.
3.2.3. Error Detection
Error detection is a critical component in the development and deployment of artificial intelligence systems. It involves identifying inaccuracies or anomalies in data processing and decision-making processes. Effective error detection mechanisms ensure that AI systems operate reliably and produce trustworthy results.
- Types of Errors:
- Data Errors: Inaccuracies in the input data can lead to incorrect outputs. This includes missing values, outliers, or mislabeled data.
- Algorithmic Errors: Flaws in the algorithms can cause the AI to misinterpret data or make faulty predictions.
- System Errors: Hardware or software malfunctions can disrupt the functioning of AI systems.
- Techniques for Error Detection:
- Validation Checks: Implementing checks to verify the integrity and accuracy of data before processing.
- Anomaly Detection: Using statistical methods or machine learning models to identify unusual patterns that may indicate errors.
- Cross-Validation: Splitting data into subsets to train and test the model, ensuring that it performs well across different data samples.
- Importance of Error Detection:
- Enhances the reliability of AI systems.
- Reduces the risk of costly mistakes in critical applications such as healthcare, finance, and autonomous driving.
- Builds user trust in AI technologies by ensuring consistent performance.
At Rapid Innovation, we leverage advanced error detection techniques to enhance the performance of AI systems for our clients. By implementing robust validation checks and anomaly detection methods, we help organizations minimize risks and maximize their return on investment (ROI) in AI technologies.
3.3. Regulatory Compliance
Regulatory compliance refers to the adherence to laws, regulations, and guidelines that govern the use of artificial intelligence. As AI technologies evolve, so do the legal frameworks surrounding them, making compliance a vital aspect of AI development.
- Key Areas of Regulatory Compliance:
- Data Protection: Ensuring that AI systems comply with data privacy laws such as GDPR, which mandates the protection of personal data.
- Transparency: Regulations may require organizations to disclose how AI systems make decisions, especially in sensitive areas like hiring or lending.
- Accountability: Establishing clear lines of responsibility for AI outcomes, ensuring that organizations can be held accountable for their AI systems.
- Challenges in Compliance:
- Rapidly changing regulations can make it difficult for organizations to keep up.
- The complexity of AI systems can obscure understanding and compliance with regulations.
- Balancing innovation with compliance can be challenging, as overly stringent regulations may stifle technological advancement.
- Benefits of Regulatory Compliance:
- Protects organizations from legal penalties and reputational damage.
- Fosters consumer trust by demonstrating a commitment to ethical practices.
- Encourages the development of fair and responsible AI technologies.
Rapid Innovation assists clients in navigating the complex landscape of regulatory compliance. Our expertise ensures that AI systems are designed with compliance in mind, thereby reducing legal risks and enhancing trust among stakeholders.
3.4. Ethical AI Development
Ethical AI development focuses on creating AI systems that are fair, transparent, and beneficial to society. As AI becomes more integrated into daily life, the ethical implications of its use are increasingly scrutinized.
- Principles of Ethical AI:
- Fairness: AI systems should be designed to avoid bias and discrimination, ensuring equitable treatment for all users.
- Transparency: Developers should strive for clarity in how AI systems operate, making it easier for users to understand decision-making processes.
- Accountability: Organizations must take responsibility for the outcomes of their AI systems, ensuring that there are mechanisms in place to address any negative impacts.
- Strategies for Ethical AI Development:
- Diverse Data Sets: Using diverse and representative data sets to train AI models can help mitigate bias.
- Stakeholder Engagement: Involving a wide range of stakeholders, including ethicists, community representatives, and users, in the development process can provide valuable insights.
- Regular Audits: Conducting regular audits of AI systems to assess their performance and ethical implications can help identify and rectify issues.
- Importance of Ethical AI:
- Promotes social good by ensuring that AI technologies are used to enhance human welfare.
- Reduces the risk of negative societal impacts, such as job displacement or privacy violations.
- Builds public trust in AI technologies, encouraging wider adoption and acceptance.
At Rapid Innovation, we prioritize ethical AI development by integrating fairness, transparency, and accountability into our AI solutions. This commitment not only enhances the societal impact of our technologies but also drives greater ROI for our clients by fostering trust and acceptance in the marketplace.
The social impact of technology, particularly in areas like artificial intelligence, blockchain, and social media, is profound and multifaceted. Understanding this impact is crucial for fostering acceptance among users and stakeholders, as highlighted by the technology acceptance model (TAM) and its various iterations, including the unified theory of acceptance and use of technology.
- Community Engagement: Engaging communities in the development and implementation of technology can enhance acceptance. When people feel involved, they are more likely to embrace new technologies, as seen in the acceptance of new technology and the public acceptance of autonomous vehicles.
- Education and Awareness: Increasing awareness about the benefits and risks associated with new technologies can lead to informed acceptance. Educational programs can demystify complex technologies, making them more accessible to the general public, which is essential for the technology acceptance theory.
- Ethical Considerations: Addressing ethical concerns is vital for social acceptance. Technologies that prioritize user privacy, data security, and ethical use are more likely to gain public trust, aligning with the principles of the technological acceptance model.
- Cultural Sensitivity: Different cultures may respond differently to technology. Tailoring solutions to fit cultural contexts can improve acceptance rates, as demonstrated in the mobile technology acceptance model and senior technology acceptance model.
- Impact on Employment: The introduction of new technologies often raises concerns about job displacement. Addressing these concerns through reskilling and upskilling initiatives can mitigate fears and promote acceptance, which is a key aspect of the technology adoption model (TAM).
- Social Equity: Ensuring that technology benefits all segments of society, particularly marginalized groups, can enhance its social impact. Initiatives aimed at reducing the digital divide are essential for fostering inclusivity, as emphasized in recent advances in technology acceptance models and theories.
- Feedback Mechanisms: Establishing channels for user feedback can help developers understand public sentiment and make necessary adjustments to improve acceptance, which is crucial for the user acceptance of information technology toward a unified view.
4. Technical Implementation
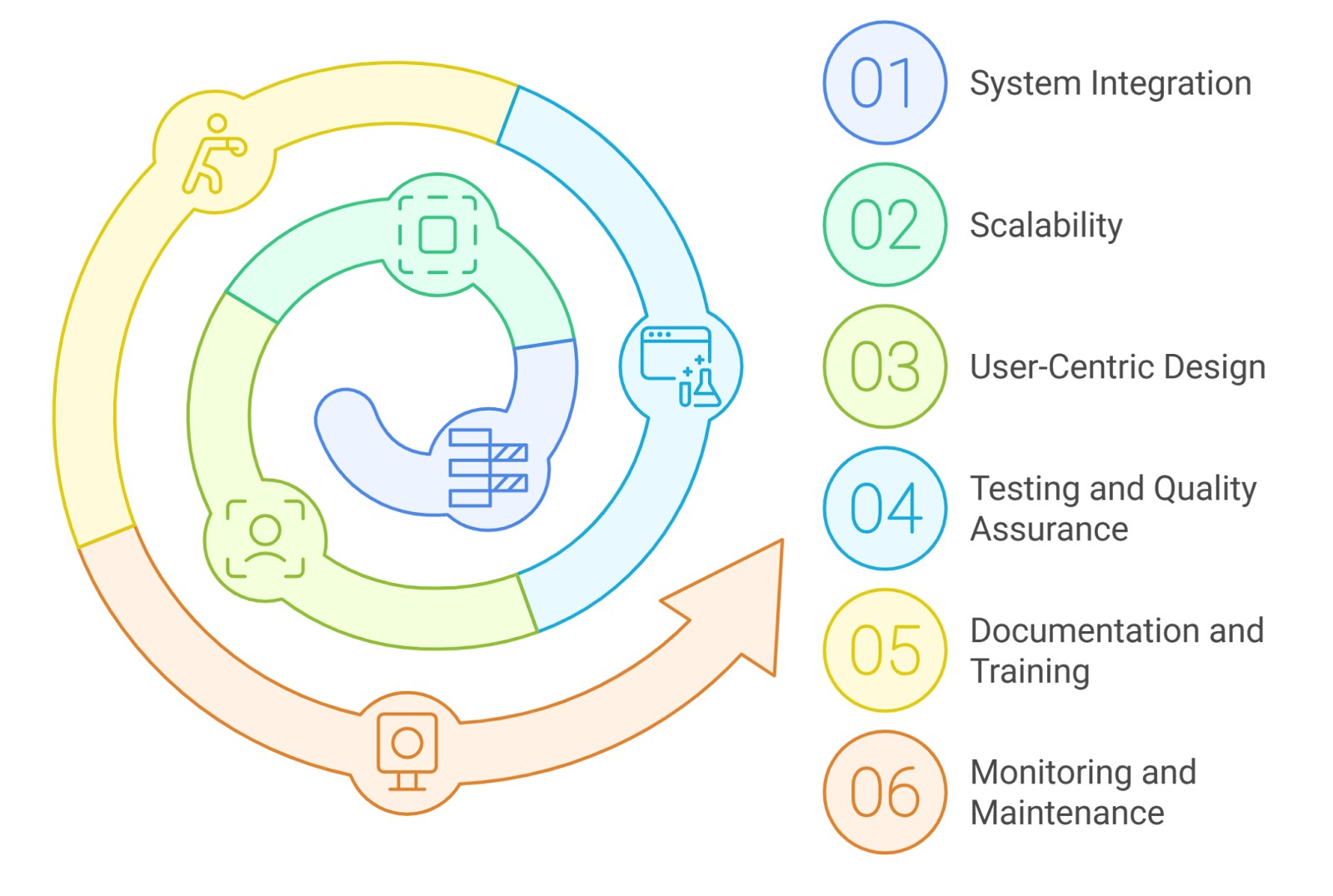
Technical implementation refers to the process of integrating new technologies into existing systems and workflows. This phase is critical for ensuring that the technology functions as intended and meets user needs.
- System Integration: Seamless integration with existing systems is essential. This may involve using APIs, middleware, or other tools to ensure compatibility.
- Scalability: The technology should be designed to scale efficiently, meaning it can handle increased loads without compromising performance.
- User-Centric Design: Focusing on user experience during implementation can lead to higher adoption rates. This includes intuitive interfaces and easy navigation, which are fundamental to the TAM model.
- Testing and Quality Assurance: Rigorous testing is necessary to identify and resolve issues before full deployment. This includes unit testing, integration testing, and user acceptance testing, which are critical for the technology acceptance model (TAM) research paper.
- Documentation and Training: Providing comprehensive documentation and training for users can facilitate smoother implementation, helping users understand how to use the technology effectively.
- Monitoring and Maintenance: Continuous monitoring is essential to ensure the technology operates as expected. Regular updates and maintenance can address any emerging issues.
4.1. Transparency by Design
Transparency by design is a principle that emphasizes openness and clarity in the development and deployment of technology. This approach fosters trust and accountability among users and stakeholders.
- Clear Communication: Providing clear information about how the technology works, including its algorithms and data usage, can enhance user trust.
- Data Privacy: Implementing robust data privacy measures and clearly communicating these practices can reassure users about their data security.
- Open Source Options: Utilizing open-source technologies can promote transparency, as users can inspect and modify the code. This can lead to greater community involvement and trust.
- Regular Audits: Conducting regular audits of technology systems can ensure compliance with ethical standards and regulations. Sharing audit results with stakeholders can further enhance transparency.
- User Control: Allowing users to control their data and how it is used can foster a sense of ownership and trust. Features like data portability and consent management are essential.
- Feedback Loops: Establishing mechanisms for user feedback can help developers understand user concerns and improve transparency. This can include surveys, forums, or direct communication channels.
- Ethical Guidelines: Adhering to ethical guidelines in technology development can promote transparency, including being upfront about potential biases and limitations of the technology.
At Rapid Innovation, we leverage our expertise in AI and blockchain to ensure that our solutions not only meet technical requirements but also align with social values, fostering acceptance through the technology acceptance model (TAM) and maximizing ROI for our clients. By prioritizing community engagement, ethical considerations, and transparency, we help organizations navigate the complexities of technology adoption while enhancing their social impact.
4.2. Explainable AI (XAI) Methods
Explainable AI (XAI) refers to a set of methods and techniques, including explainable ai methods and explainable ai algorithms, that aim to make the decision-making processes of AI systems transparent and understandable to humans. As AI systems become more complex, the need for interpretability grows, especially in critical applications such as healthcare, finance, and autonomous vehicles. XAI methods, such as neural network explainability and model explainability techniques, help stakeholders understand how AI models arrive at their predictions, which can enhance trust, accountability, and compliance with regulations. The benefits of XAI include:
- Enhances trust in AI systems.
- Facilitates compliance with regulations.
- Improves model performance through insights.
- Aids in debugging and refining models.
At Rapid Innovation, we leverage XAI techniques, including shapley explainability and explainability methods, to ensure that our AI solutions not only deliver high performance but also provide clarity and transparency, enabling our clients to achieve their business goals efficiently.
4.2.1. LIME (Local Interpretable Model-agnostic Explanations)
LIME is a popular XAI method that provides local explanations for individual predictions made by any machine learning model. It works by approximating the complex model with a simpler, interpretable model in the vicinity of the prediction being explained. This approach allows users to understand the factors influencing a specific decision without needing to comprehend the entire model. Key features of LIME include:
- Local focus: LIME explains individual predictions rather than the model as a whole.
- Model-agnostic: It can be applied to any machine learning model, regardless of its complexity.
- Perturbation-based: LIME generates perturbed samples of the input data to observe how changes affect the model's predictions.
- Interpretable models: It typically uses linear models or decision trees to provide clear explanations.
LIME has been widely adopted in various fields, including healthcare, where understanding the rationale behind a diagnosis is crucial. By providing insights into which features contributed to a specific prediction, LIME helps clinicians make informed decisions. At Rapid Innovation, we implement LIME in our healthcare AI solutions to enhance decision-making processes and improve patient outcomes.
4.2.2. SHAP (SHapley Additive exPlanations)
SHAP is another powerful XAI method that provides a unified measure of feature importance based on cooperative game theory. It assigns each feature an importance value for a particular prediction, ensuring that the contributions of all features sum up to the model's output. SHAP values are derived from Shapley values, which are used in cooperative games to fairly distribute payouts among players based on their contributions. The main characteristics of SHAP include:
- Global and local explanations: SHAP can provide insights at both the individual prediction level and the overall model level.
- Consistency: If a model changes so that a feature contributes more to a prediction, SHAP ensures that the importance value for that feature also increases.
- Additivity: The sum of SHAP values for all features equals the model's output, making it easy to understand how each feature contributes to the prediction.
- Model-agnostic: Like LIME, SHAP can be applied to any machine learning model.
SHAP has gained traction in various industries, including finance, where understanding the factors behind credit scoring is essential. By providing clear and consistent explanations, SHAP helps stakeholders make better decisions and fosters trust in AI systems. Rapid Innovation utilizes SHAP in our financial AI applications to enhance transparency and improve client trust, ultimately driving greater ROI for our clients. Additionally, we explore feature relevance quantification in explainable ai as a causal problem to further enhance our understanding of model behavior.
4.2.3. Counterfactual Explanations
Counterfactual explanations are a powerful tool in the realm of explainable artificial intelligence (XAI). They provide insights into how a model's predictions could change if certain input features were altered. This approach helps users understand the decision-making process of complex models, particularly in high-stakes domains like finance and healthcare.
- Definition: A counterfactual explanation answers the question, "What would need to change for the outcome to be different?" For instance, if a loan application is denied, a counterfactual explanation might indicate that if the applicant's income were $5,000 higher, the application would have been approved.
- Importance:
- Enhances transparency in AI systems.
- Helps users identify actionable changes to achieve desired outcomes.
- Supports fairness by revealing biases in model predictions.
- Applications:
- In healthcare, counterfactuals can help patients understand what lifestyle changes might improve their health outcomes.
- In finance, they can guide applicants on how to improve their creditworthiness.
- Challenges:
- Generating realistic counterfactuals can be computationally intensive.
- Ensuring that counterfactuals are interpretable and meaningful to users is crucial.
At Rapid Innovation, we leverage counterfactual explanations for machine learning to empower our clients with actionable insights, enabling them to make informed decisions that align with their business objectives. By integrating these counterfactual explanations into our AI solutions, we help clients in sectors like finance and healthcare achieve greater ROI through enhanced decision-making processes. Our work includes counterfactual explanations for machine learning a review, ensuring that we stay updated on the latest methodologies and best practices in the field.
4.3. Visualization Techniques
Visualization techniques play a critical role in making complex data and model outputs understandable. They help stakeholders grasp the intricacies of machine learning models and their predictions, facilitating better decision-making.
- Types of Visualization Techniques:
- Feature Importance: Displays which features most significantly impact model predictions, helping users focus on key variables.
- Partial Dependence Plots: Illustrate the relationship between a feature and the predicted outcome, holding other features constant.
- SHAP (SHapley Additive exPlanations) Values: Provide a unified measure of feature importance, showing how each feature contributes to the prediction.
- Benefits:
- Simplifies complex data, making it accessible to non-technical stakeholders.
- Enhances model interpretability, fostering trust in AI systems.
- Aids in identifying patterns and anomalies in data.
- Best Practices:
- Use clear labels and legends to ensure clarity.
- Choose appropriate color schemes to enhance readability.
- Provide interactive elements to allow users to explore data dynamically.
At Rapid Innovation, we implement advanced visualization techniques to ensure that our clients can easily interpret their data and model outputs. This not only aids in decision-making but also enhances stakeholder engagement, ultimately driving better business outcomes.
4.4. Documentation Standards
Documentation standards are essential for ensuring that AI systems are transparent, reproducible, and maintainable. Proper documentation helps stakeholders understand the model's development, deployment, and performance.
- Key Components of Documentation:
- Model Description: A clear overview of the model architecture, algorithms used, and the rationale behind their selection.
- Data Sources: Detailed information about the datasets used, including their origin, preprocessing steps, and any biases present.
- Performance Metrics: A comprehensive account of how the model performs, including accuracy, precision, recall, and any relevant benchmarks.
- Importance:
- Facilitates collaboration among team members by providing a shared understanding of the model.
- Supports compliance with regulations and ethical standards in AI development.
- Enhances reproducibility, allowing other researchers to replicate results and validate findings.
- Best Practices:
- Maintain version control for documentation to track changes over time.
- Use standardized templates to ensure consistency across different projects.
- Regularly update documentation to reflect changes in the model or data.
At Rapid Innovation, we prioritize robust documentation standards to ensure that our AI solutions are not only effective but also compliant with industry regulations. This commitment to transparency and reproducibility helps our clients achieve sustainable growth and a higher return on investment.
4.5. Testing and Validation
Testing and validation are critical components in the development of any product or system. They ensure that the final output meets the required specifications and functions as intended. This process involves several stages:
- Unit Testing: This is the first level of testing, where individual components or modules of the system are tested in isolation. The goal is to identify any bugs or issues at an early stage, which can save time and resources later in the development process.
- Integration Testing: After unit testing, the next step is to test how different modules work together. Integration testing helps to identify interface defects and ensures that combined parts of the application function correctly.
- System Testing: This phase involves testing the complete and integrated software to evaluate its compliance with the specified requirements. System testing checks the overall functionality, performance, and security of the application.
- User Acceptance Testing (UAT): This is the final phase of testing, where actual users test the system in a real-world environment. UAT ensures that the product meets user needs and is ready for deployment.
- Validation: Validation is the process of evaluating the final product to ensure it meets the intended use and requirements. This can involve various methods, including reviews, inspections, and testing against predefined criteria. Software validation testing is a key aspect of this process.
- Documentation: Proper documentation of the testing and validation process is essential. It provides a record of what was tested, the results, and any issues that were identified and resolved. This includes maintaining a requirements traceability verification matrix to track validation and verification efforts.
Effective testing and validation can significantly reduce the risk of failure and enhance the quality of the final product. According to a study, organizations that implement rigorous testing processes can reduce defects by up to 40%. This includes practices such as verification and validation in software testing and the use of the vv model in software testing.
5. Challenges and Limitations

Despite the importance of testing and validation, there are several challenges and limitations that organizations may face during these processes. Understanding these challenges can help teams prepare and mitigate risks effectively.
- Resource Constraints: Limited budgets and personnel can hinder the ability to conduct thorough testing. Organizations may have to prioritize certain tests over others, which can lead to potential oversights.
- Time Constraints: Tight deadlines often pressure teams to rush through testing phases. This can result in incomplete testing and increased chances of defects in the final product.
- Complexity of Systems: As systems become more complex, testing them becomes increasingly challenging. Interdependencies between components can make it difficult to isolate issues and ensure comprehensive coverage. Verification and validation in software engineering can help address some of these complexities.
- Changing Requirements: In agile environments, requirements can change frequently. This can complicate the testing process, as teams must continuously adapt their tests to align with new specifications.
- Lack of Standardization: Without standardized testing procedures, teams may employ inconsistent methods, leading to unreliable results. Establishing clear guidelines can help improve the quality of testing, including validation & verification in software testing.
5.1. Technical Challenges
Technical challenges in testing and validation can significantly impact the effectiveness of the process. These challenges often stem from the evolving nature of technology and the complexity of modern systems.
- Automation Limitations: While automated testing can enhance efficiency, it is not a one-size-fits-all solution. Some tests may require human judgment, and automated tests can miss context-specific issues.
- Integration Issues: As systems integrate with various third-party services and APIs, testing these integrations can become complicated. Ensuring that all components work seamlessly together requires extensive testing efforts, including verification testing in software testing.
- Data Management: Testing often requires large volumes of data, which can be difficult to manage. Ensuring data accuracy and relevance is crucial for effective testing, but it can be a significant challenge.
- Environment Consistency: Testing environments must closely mimic production environments to yield reliable results. Discrepancies between environments can lead to issues that only appear after deployment.
- Performance Testing: Evaluating how a system performs under load is essential, but it can be technically challenging. Simulating real-world usage scenarios requires careful planning and execution.
- Security Testing: With the increasing prevalence of cyber threats, security testing has become paramount. However, identifying vulnerabilities and ensuring robust security measures can be technically demanding.
Addressing these technical challenges requires a combination of skilled personnel, effective tools, and a commitment to continuous improvement in testing practices. At Rapid Innovation, we leverage our expertise in AI and Blockchain to implement advanced testing methodologies, including AI agents in software testing, that enhance product quality and ensure robust performance, ultimately driving greater ROI for our clients.
5.1.1. Complexity-Transparency Trade-off
In many systems, especially in software development and data management, there exists a trade-off between complexity and transparency. This trade-off can significantly impact how systems are designed and operated. Complexity refers to the intricacy of a system, including the number of components, interactions, and the overall architecture, while transparency involves the clarity and understandability of a system's processes and decisions.
When a system is highly complex, it often becomes less transparent, leading to several issues:
- User Confusion: Users may struggle to understand how the system works, leading to frustration and errors.
- Maintenance Challenges: Complex systems are harder to maintain and troubleshoot, as identifying the root cause of issues can be difficult.
- Decision-Making: Lack of transparency can hinder effective decision-making, as stakeholders may not fully grasp the implications of the system's operations.
To balance complexity and transparency, organizations can:
- Simplify processes where possible.
- Use clear documentation and user interfaces.
- Implement monitoring tools that provide insights into system operations.
At Rapid Innovation, we leverage our expertise in AI and Blockchain to help clients navigate this complexity-transparency trade-off. For instance, by utilizing AI-driven analytics, we can simplify data management processes, making them more transparent and user-friendly. In Blockchain projects, we ensure that the underlying protocols are well-documented and accessible, enhancing stakeholder understanding and trust. This is particularly relevant when addressing system design challenges, such as those encountered in embedded systems and system on chip design challenges. Additionally, we explore the use of AI agents for IT resource optimization to streamline operations and improve overall system transparency.
5.1.2. Performance Impact
Performance impact is a critical consideration in system design and implementation. It refers to how various factors, such as complexity and resource allocation, affect the efficiency and speed of a system. Performance can be measured in terms of response time, throughput, and resource utilization, and high performance is essential for user satisfaction and operational efficiency.
Several factors can influence performance:
- System Architecture: A well-designed architecture can enhance performance by optimizing resource usage and minimizing bottlenecks.
- Complexity: Increased complexity can lead to slower performance due to additional processing requirements and potential inefficiencies.
- Resource Allocation: Proper allocation of resources, such as CPU, memory, and bandwidth, is crucial for maintaining optimal performance.
To mitigate performance issues, organizations can:
- Conduct regular performance testing and monitoring.
- Optimize code and algorithms to reduce complexity.
- Scale resources according to demand to ensure consistent performance.
At Rapid Innovation, we focus on optimizing system architecture and resource allocation to enhance performance. For example, in AI projects, we implement advanced algorithms that not only improve processing speed but also reduce resource consumption, leading to a higher return on investment for our clients.
5.1.3. Implementation Difficulties
Implementation difficulties arise during the deployment of new systems or processes. These challenges can stem from various sources and can significantly affect the success of a project.
Common challenges include:
- Technical Challenges: Integrating new systems with existing infrastructure can be complex, often requiring specialized knowledge and skills.
- User Adoption: Resistance from users can hinder implementation, especially if they are accustomed to existing processes.
- Resource Constraints: Limited budgets and personnel can restrict the ability to implement new systems effectively.
Common implementation difficulties include:
- Inadequate Training: Users may not receive sufficient training, leading to improper use of the system.
- Poor Planning: Lack of a clear implementation strategy can result in missed deadlines and budget overruns.
- Data Migration Issues: Transferring data from old systems to new ones can be fraught with challenges, including data loss or corruption.
To address implementation difficulties, organizations can:
- Develop a comprehensive implementation plan that includes timelines and resource allocation.
- Provide thorough training and support for users to facilitate adoption.
- Engage stakeholders early in the process to gather feedback and ensure alignment with organizational goals.
At Rapid Innovation, we understand the intricacies of implementing AI and Blockchain solutions. We work closely with our clients to develop tailored implementation plans that address their unique challenges, ensuring a smooth transition and maximizing the potential for success. By providing comprehensive training and support, we empower users to embrace new technologies confidently, ultimately driving greater ROI. This is especially important when considering the design challenges of embedded systems and the complexities involved in system on chip design challenges.
5.2. Operational Challenges
Operational challenges are critical hurdles that organizations face in their day-to-day activities. These operational challenges can significantly impact efficiency, productivity, and overall performance. Understanding these challenges is essential for developing effective strategies to overcome them. Organizations often struggle with aligning their operational processes with strategic goals, and inefficient workflows can lead to wasted resources and time. Additionally, communication barriers can hinder collaboration among teams, while adapting to technological changes can be a daunting task for many businesses. Common problems in business operations can further complicate these issues.
5.2.1. Resource Requirements
Resource requirements refer to the necessary inputs needed to carry out operations effectively. This includes human resources, financial resources, and physical assets.
- Human Resources: Organizations need skilled personnel to manage operations. A shortage of qualified staff can lead to operational delays and decreased productivity. Rapid Innovation can assist in this area by providing AI-driven recruitment solutions that streamline the hiring process, ensuring that organizations attract and retain the right talent.
- Financial Resources: Adequate funding is crucial for maintaining operations. Insufficient financial resources can limit the ability to invest in technology, training, and infrastructure. Our consulting services can help organizations identify cost-effective solutions, including leveraging blockchain technology for transparent financial transactions and efficient resource allocation.
- Physical Assets: Equipment and facilities must be maintained and upgraded regularly. Aging infrastructure can lead to increased downtime and maintenance costs. Rapid Innovation offers predictive maintenance solutions powered by AI, which can help organizations anticipate equipment failures and optimize maintenance schedules, ultimately reducing costs and improving operational efficiency.
Meeting these resource requirements is essential for smooth operations. Organizations must conduct regular assessments to identify gaps in resources and develop strategies to address them, particularly in light of operational problems in business.
5.2.2. Integration Issues
Integration issues arise when different systems, processes, or teams within an organization fail to work together effectively. This can lead to inefficiencies and miscommunication, contributing to challenges in business operations.
- System Integration: Many organizations use multiple software systems for various functions. If these systems are not integrated, it can result in data silos, where information is trapped in one system and not accessible to others. Rapid Innovation specializes in developing custom APIs and integration solutions that ensure seamless data flow across platforms, enhancing overall operational efficiency.
- Process Integration: Different departments may have their own processes that do not align with one another. This can create bottlenecks and slow down overall operations. Our blockchain solutions can facilitate process integration by providing a single source of truth, ensuring that all departments have access to the same data and can collaborate effectively.
- Team Integration: Collaboration among teams is essential for operational success. If teams do not communicate effectively, it can lead to misunderstandings and duplicated efforts. Rapid Innovation can implement AI-driven collaboration tools that enhance communication and project management, fostering a culture of teamwork and efficiency.
Addressing integration issues requires a comprehensive approach that includes investing in technology, fostering a culture of collaboration, and ensuring that processes are aligned across the organization. By leveraging our expertise in AI and blockchain, Rapid Innovation can help organizations overcome these operational challenges and achieve greater ROI, ultimately addressing the operational challenges examples that many businesses face. Additionally, our expertise in the potential of business AI engineering best practices can further enhance operational efficiency and innovation.
5.2.3. Maintenance Overhead
Maintenance overhead refers to the ongoing costs and efforts required to keep a system, software, or infrastructure running efficiently. This aspect is crucial for organizations as it directly impacts operational budgets and resource allocation.
- Regular updates and patches are necessary to ensure security and functionality.
- Technical support is often required to address user issues and system failures.
- Documentation must be maintained to assist in troubleshooting and onboarding new staff.
- Hardware and software upgrades may be needed to keep up with technological advancements.
- Training for staff is essential to ensure they are proficient in using the system effectively.
The maintenance overhead can vary significantly based on the complexity of the system. For instance, a simple application may require minimal upkeep, while a large enterprise system could demand substantial resources. According to industry studies, maintenance costs can account for up to 70% of the total cost of ownership for software systems. This emphasizes the importance of planning for maintenance in the initial stages of project development. At Rapid Innovation, we leverage our expertise in AI and Blockchain to streamline maintenance processes, reducing overhead costs and enhancing system reliability. By implementing automated updates and utilizing smart contracts for system management, we help clients achieve greater efficiency and ROI.
In the context of physical systems, such as garage doors, regular maintenance is essential. Services like garage door maintenance near me, greasing garage door components, and garage door opener maintenance are crucial to ensure functionality. Additionally, understanding garage door maintenance cost and exploring garage door maintenance DIY options can help homeowners manage their expenses effectively. Preventive maintenance, including garage door springs maintenance and overhead door maintenance, can prolong the lifespan of the system. Companies like Amarr and LiftMaster offer specific guidelines for maintaining their products, ensuring that users can keep their garage doors in optimal condition.
Social and ethical challenges arise from the implementation and use of technology in society. These challenges can affect individuals, communities, and organizations, leading to significant implications for how technology is perceived and utilized.
- The digital divide can exacerbate inequalities, leaving marginalized groups without access to essential technologies.
- Job displacement due to automation raises concerns about the future of work and economic stability.
- Misinformation and the spread of fake news can undermine trust in institutions and create societal discord.
- Ethical considerations in AI and machine learning, such as bias in algorithms, can lead to unfair treatment of certain groups.
- Environmental impacts of technology, including e-waste and energy consumption, pose sustainability challenges.
Addressing these social and ethical challenges requires a collaborative approach involving policymakers, technologists, and community stakeholders. It is essential to create frameworks that promote responsible technology use while considering the broader societal implications.
5.3.1. Privacy Concerns
Privacy concerns are a significant aspect of the social and ethical challenges associated with technology. As digital platforms collect vast amounts of personal data, individuals often worry about how their information is used and protected.
- Data breaches can expose sensitive information, leading to identity theft and financial loss.
- Surveillance technologies raise questions about consent and the extent of monitoring in public and private spaces.
- The use of personal data for targeted advertising can feel invasive and manipulative to users.
- Regulations like GDPR aim to protect user privacy, but compliance can be complex for organizations.
- Users often lack transparency regarding how their data is collected, stored, and shared.
The growing awareness of privacy issues has led to increased demand for privacy-centric technologies and practices. Organizations must prioritize data protection and transparency to build trust with their users. At Rapid Innovation, we specialize in developing AI-driven solutions that enhance data privacy and security, ensuring compliance with regulations while fostering user trust.
5.3.2. Intellectual Property Protection
Intellectual property (IP) protection is crucial in safeguarding innovations, creations, and inventions across various industries. It ensures that creators and inventors can benefit from their work without the fear of unauthorized use or reproduction.
- Types of Intellectual Property:
- Patents: Protect inventions and processes for a specific period, typically 20 years, including design patent and patent design application.
- Trademarks: Safeguard brand names, logos, and slogans that distinguish goods or services, such as trademark registration law and trademark federal registration.
- Copyrights: Protect original works of authorship, such as literature, music, and art, including copyright a design and copyright for brand name.
- Trade Secrets: Guard confidential business information that provides a competitive edge, with trade secrets examples illustrating their importance.
- Importance of IP Protection:
- Encourages innovation by providing a legal framework for creators to monetize their inventions, including intellectual property protection and intellectual property ip protection.
- Helps businesses maintain a competitive advantage in the market, particularly through protected by intellectual property.
- Fosters economic growth by promoting research and development.
- Challenges in IP Protection:
- Globalization complicates enforcement, as IP laws vary by country, affecting trademark registration international and trademark registration global.
- Digital piracy and counterfeiting are rampant, especially in the online space, impacting copyright protected works.
- Small businesses often lack the resources to navigate complex IP laws, including unregistered trade marks.
- Strategies for Effective IP Protection:
- Conduct regular IP audits to identify and manage assets, including types of intellectual property.
- Register IP rights in key markets to ensure legal protection, such as business name patent and protected by patent.
- Educate employees about the importance of IP and how to protect it, including trade secret protection and protect trademark strategies.
At Rapid Innovation, we leverage our expertise in AI and Blockchain to enhance IP protection strategies for our clients. For instance, utilizing blockchain technology can create immutable records of IP ownership, making it easier to prove ownership and combat infringement. Additionally, AI-driven analytics can help identify potential IP risks and opportunities, enabling businesses to make informed decisions that maximize their ROI. Our services include comprehensive blockchain consulting to help you navigate these challenges effectively. Furthermore, we explore various AI applications in media and entertainment to enhance IP protection and drive innovation.
5.3.3. Misuse Prevention
Misuse prevention is essential for maintaining the integrity and security of intellectual property and sensitive information. Organizations must implement robust measures to prevent unauthorized access, use, or distribution of their assets.
- Key Aspects of Misuse Prevention:
- Access Control: Limit access to sensitive information based on roles and responsibilities.
- Data Encryption: Protect data in transit and at rest to prevent unauthorized access.
- Regular Audits: Conduct audits to identify vulnerabilities and ensure compliance with policies.
- Importance of Misuse Prevention:
- Protects the organization from financial losses due to theft or misuse of IP, including trade secrets are protected.
- Maintains customer trust by safeguarding personal and sensitive information.
- Ensures compliance with legal and regulatory requirements.
- Common Misuse Scenarios:
- Insider Threats: Employees may misuse access to sensitive information for personal gain.
- Cyber Attacks: Hackers may exploit vulnerabilities to steal or corrupt data.
- Inadvertent Sharing: Employees may unintentionally share sensitive information through unsecured channels.
- Strategies for Misuse Prevention:
- Implement comprehensive security policies and procedures.
- Provide regular training to employees on data protection and security best practices.
- Utilize technology solutions, such as firewalls and intrusion detection systems, to monitor and protect assets.
At Rapid Innovation, we understand the critical nature of misuse prevention. Our AI solutions can enhance threat detection and response capabilities, while our blockchain applications can ensure secure and transparent data sharing, significantly reducing the risk of misuse.
6. Industry Applications
The applications of intellectual property protection and misuse prevention span across various industries, each with unique challenges and requirements. Understanding these applications can help organizations leverage IP effectively while minimizing risks.
- Technology Sector:
- Software companies rely heavily on copyrights and patents to protect their innovations.
- Misuse prevention is critical due to the high risk of cyber threats and data breaches.
- Pharmaceutical Industry:
- Patents are essential for protecting drug formulations and research.
- Misuse prevention strategies are vital to safeguard sensitive clinical trial data and proprietary research.
- Entertainment Industry:
- Copyrights protect music, films, and other creative works from unauthorized reproduction.
- Misuse prevention measures help combat piracy and ensure fair compensation for creators.
- Manufacturing Sector:
- Trade secrets play a significant role in protecting proprietary processes and designs.
- Misuse prevention is necessary to prevent industrial espionage and safeguard competitive advantages.
- Retail Industry:
- Trademarks are crucial for brand recognition and consumer trust.
- Misuse prevention strategies help protect against counterfeit products and brand dilution.
- Education Sector:
- Copyrights protect educational materials and research outputs.
- Misuse prevention is essential to ensure academic integrity and protect student data.
By understanding the importance of intellectual property protection and misuse prevention, organizations can better navigate the complexities of their respective industries while fostering innovation and maintaining security. Rapid Innovation is committed to helping clients achieve these goals efficiently and effectively, ensuring a greater return on investment through our tailored solutions.
6.1. Healthcare
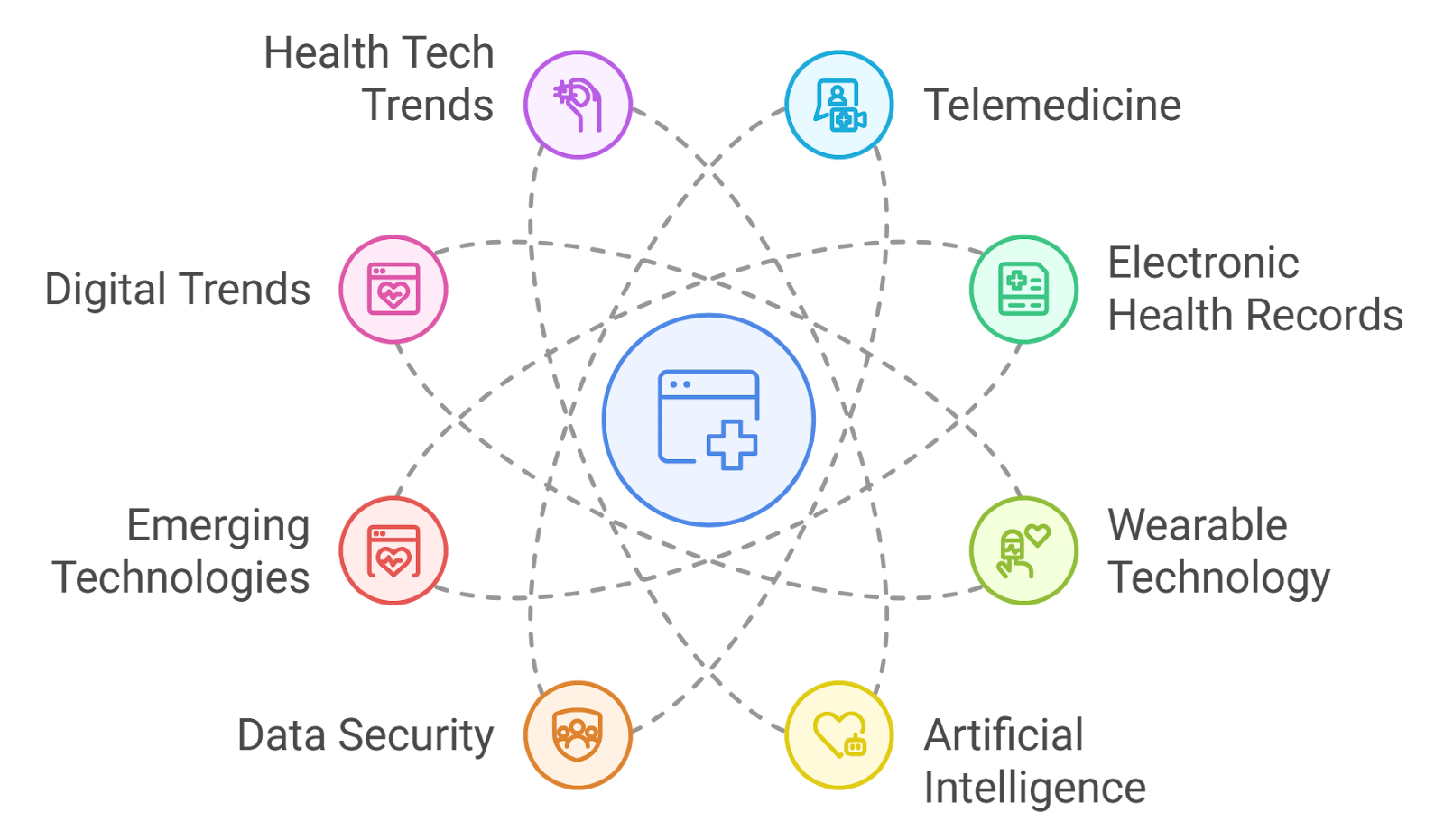
The healthcare industry is undergoing a significant transformation, driven by technological advancements and the need for improved patient care. Key trends and innovations are shaping the future of healthcare, including healthcare technology trends and healthcare innovation trends:
- Telemedicine: The rise of telehealth services allows patients to consult healthcare professionals remotely, improving access to care, especially in rural areas. Rapid Innovation can assist healthcare providers in implementing robust telemedicine platforms that enhance patient engagement and streamline consultations.
- Electronic Health Records (EHR): EHR systems streamline patient data management, enhancing communication among healthcare providers and improving patient outcomes. Our expertise in AI can optimize EHR systems for better data analytics, leading to improved decision-making and patient care.
- Wearable Technology: Devices like smartwatches and fitness trackers monitor health metrics, encouraging proactive health management and early detection of potential issues. Rapid Innovation can develop custom applications that integrate with these devices, providing healthcare professionals with real-time data to enhance patient monitoring.
- Artificial Intelligence (AI): AI is being utilized for diagnostics, treatment recommendations, and personalized medicine, leading to more accurate and efficient healthcare delivery. Our AI solutions can help healthcare organizations leverage predictive analytics to improve patient outcomes and operational efficiency, aligning with artificial intelligence trends in healthcare.
- Data Security: With the increase in digital health records, ensuring data privacy and security is paramount to protect sensitive patient information. Rapid Innovation specializes in implementing blockchain technology to enhance data security and ensure compliance with healthcare regulations, addressing trends in healthcare information technology.
- Emerging Technologies: The integration of emerging technologies in the healthcare industry is revolutionizing patient care and operational efficiency. Rapid Innovation is at the forefront of these healthcare tech trends, helping organizations adopt innovative solutions that drive better health outcomes.
- Digital Trends: The shift towards digital solutions in healthcare is evident, with a focus on enhancing patient experiences and streamlining processes. Our expertise in digital trends in healthcare enables us to support organizations in navigating this transformation effectively.
- Healthcare and Technology Trends: The convergence of healthcare and technology is creating new opportunities for improving patient care and operational efficiency. Rapid Innovation is committed to helping healthcare organizations stay ahead of these trends and leverage technology for better outcomes.
- Health Tech Trends: The rise of health tech is reshaping the healthcare landscape, with new tools and solutions emerging to address various challenges. Our team is dedicated to exploring and implementing the latest health tech trends to enhance healthcare delivery and patient engagement.
6.4. Public Sector
The public sector plays a crucial role in the economy and society by providing essential services and infrastructure. It encompasses government agencies, public institutions, and organizations that operate under government control. The public sector is vital for maintaining social order, promoting economic stability, and ensuring the welfare of citizens.
- Provides essential services such as healthcare, education, and public safety.
- Contributes to economic growth through job creation and infrastructure development, including public service careers and jobs in public service.
- Regulates industries to ensure fair practices and protect consumers.
- Implements policies aimed at social equity and environmental sustainability.
- Engages in public-private partnerships to enhance service delivery and innovation, such as public sector consulting.
The public sector is often funded through taxation, which allows it to operate and provide services to the community. However, it also faces challenges such as budget constraints, bureaucratic inefficiencies, and the need for modernization. Rapid Innovation can assist public sector entities in overcoming these challenges by leveraging AI and Blockchain technologies. For instance, AI can optimize resource allocation and improve decision-making processes, while Blockchain can enhance transparency and accountability in public transactions. The effectiveness of the public sector can significantly impact the quality of life for citizens and the overall health of the economy. Examples of public service and public service jobs near me highlight the diverse opportunities available in this sector. Additionally, leadership and public services play a critical role in shaping effective governance and community engagement. A career in public service can be rewarding, offering various paths, including jobs in the public sector and specific roles like those at Deloitte government and public services. For more insights on how AI can be integrated into government operations, visit AI Agents for Government: Key Components, Applications, and Use Cases.
6.5. Manufacturing
Manufacturing is a key driver of economic growth and innovation. It involves the transformation of raw materials into finished goods through various processes, including assembly, fabrication, and production. The manufacturing sector is essential for job creation, technological advancement, and international trade.
- Contributes significantly to GDP and employment rates in many countries.
- Drives innovation through research and development in production techniques.
- Supports supply chains and creates demand for raw materials and services.
- Enhances export capabilities, contributing to a favorable trade balance.
- Adapts to trends such as automation and sustainability to remain competitive.
The manufacturing industry is evolving with advancements in technology, such as automation and artificial intelligence. These innovations improve efficiency and reduce costs, but they also require a skilled workforce to operate and maintain new systems. Rapid Innovation can help manufacturers implement AI-driven solutions for predictive maintenance and quality control, ultimately leading to greater ROI. As the global market becomes more competitive, manufacturers must focus on quality, sustainability, and customer satisfaction to thrive.
6.6. Educational Systems
Educational systems are foundational to personal and societal development. They encompass the institutions, policies, and practices that facilitate learning and knowledge acquisition. A robust educational system is essential for fostering critical thinking, creativity, and social responsibility among individuals.
- Provides access to knowledge and skills necessary for personal and professional growth.
- Promotes social mobility by offering opportunities for disadvantaged groups.
- Contributes to economic development by creating a skilled workforce.
- Encourages civic engagement and informed citizenship.
- Adapts to technological advancements to enhance learning experiences.
Educational systems vary widely across countries, influenced by cultural, economic, and political factors. Effective education systems prioritize inclusivity, quality teaching, and continuous improvement. Rapid Innovation can support educational institutions by integrating AI and Blockchain technologies to personalize learning experiences and ensure secure credentialing. Challenges such as funding disparities, curriculum relevance, and access to technology must be addressed to ensure that all individuals can benefit from quality education.
7. Governance and Standards
Governance and standards play a crucial role in ensuring that organizations operate within legal frameworks and adhere to best practices. This section delves into the regulatory frameworks and industry standards that guide organizations in their operations.
7.1 Regulatory Frameworks

Regulatory frameworks are essential for maintaining order and accountability within industries. They provide guidelines that organizations must follow to ensure compliance with laws and regulations. Key aspects include:
- Legal Compliance: Organizations must adhere to local, national, and international laws, including labor laws, environmental regulations, and data protection laws. Rapid Innovation assists clients in navigating these complex legal landscapes, ensuring that their AI and Blockchain solutions are compliant and secure, including adherence to nist regulatory compliance.
- Risk Management: Regulatory frameworks help organizations identify and mitigate risks. By following these guidelines, companies can avoid legal penalties and reputational damage. Our expertise in AI-driven risk assessment tools enables clients to proactively manage potential risks associated with their operations.
- Transparency and Accountability: Regulations often require organizations to disclose information about their operations, financial performance, and governance practices. This transparency fosters trust among stakeholders. Rapid Innovation leverages Blockchain technology to enhance transparency and traceability in business processes, building stakeholder confidence.
- Sector-Specific Regulations: Different industries have unique regulatory requirements. For example, the financial sector is governed by strict regulations to protect consumers and maintain market integrity. Our team provides tailored consulting services to help clients in regulated industries implement compliant AI and Blockchain solutions, ensuring they meet various regulatory frameworks compliance.
- Global Standards: Many organizations operate internationally and must comply with regulations in multiple jurisdictions. Understanding these global standards is crucial for multinational companies. Rapid Innovation offers insights into global compliance requirements, ensuring that our clients' solutions are adaptable and compliant across borders.
Organizations must stay updated on changes in regulatory frameworks to ensure ongoing compliance. Failure to do so can result in significant fines and legal repercussions.
7.2 Industry Standards
Industry standards are established benchmarks that organizations use to ensure quality, safety, and efficiency in their operations. These standards are often developed by industry associations or standard-setting organizations. Key points include:
- Quality Assurance: Industry standards help organizations maintain high-quality products and services. Adhering to these standards can enhance customer satisfaction and loyalty. Rapid Innovation employs AI algorithms to monitor and improve product quality, ensuring that our clients meet industry benchmarks.
- Safety Protocols: Many industries have specific safety standards to protect employees and consumers. Compliance with these standards reduces the risk of accidents and injuries. Our Blockchain solutions can help track compliance with safety protocols, providing real-time data to enhance workplace safety.
- Operational Efficiency: Standards often provide best practices for processes and procedures, leading to improved efficiency and reduced costs. By integrating AI into operational workflows, Rapid Innovation helps clients streamline processes and achieve greater efficiency.
- Certification and Accreditation: Organizations can seek certification from recognized bodies to demonstrate compliance with industry standards. This can enhance credibility and marketability. We guide clients through the certification process, ensuring that their AI
7.3. Best Practices
Implementing best practices in any organization is crucial for ensuring efficiency, productivity, and compliance. Here are some key best practices to consider:
- Establish Clear Policies: Develop comprehensive policies that outline expectations and procedures. This helps in maintaining consistency and clarity across the organization.
- Regular Training: Conduct regular training sessions for employees to keep them updated on the latest practices, tools, and technologies relevant to their roles. This enhances skill sets and promotes a culture of continuous learning, particularly in areas like AI and Blockchain, where advancements occur rapidly. Incorporate compliance best practices and regulatory compliance best practices into training programs to ensure employees are well-informed.
- Utilize Technology: Leverage technology to streamline processes. Tools like project management software, communication platforms, and data analytics can significantly improve efficiency. For instance, integrating AI-driven analytics can provide insights that help in decision-making, while Blockchain can enhance transparency and security in transactions. Consider software compliance best practices to ensure that all tools used are compliant with relevant regulations.
- Encourage Open Communication: Foster an environment where employees feel comfortable sharing ideas and feedback. Open communication can lead to innovative solutions and improved morale, especially when exploring new technologies like AI and Blockchain.
- Monitor Performance: Regularly assess performance metrics to identify areas for improvement. This can include tracking key performance indicators (KPIs) and conducting employee evaluations, which can be enhanced through AI tools that provide real-time feedback. Compliance monitoring best practices should be integrated into performance assessments to ensure adherence to regulations.
- Document Processes: Maintain thorough documentation of processes and procedures. This not only aids in training new employees but also ensures continuity in operations, particularly when implementing new technologies. Good compliance practices should be documented to serve as a reference for all employees.
- Engage Stakeholders: Involve stakeholders in decision-making processes. This can lead to better outcomes and increased buy-in from those affected by changes, especially when introducing innovative solutions powered by AI and Blockchain.
7.4. Compliance Requirements
Compliance requirements are essential for organizations to adhere to legal and regulatory standards. Understanding these requirements is vital for risk management and operational integrity. Key compliance requirements include:
- Regulatory Frameworks: Familiarize yourself with relevant laws and regulations that apply to your industry. This may include data protection laws, financial regulations, and health and safety standards. Incorporate GRC best practices to ensure a comprehensive understanding of governance, risk, and compliance.
- Data Protection: Ensure compliance with data protection regulations such as GDPR or HIPAA. This involves implementing measures to protect sensitive information and ensuring proper data handling practices, which can be further secured through Blockchain technology.
- Financial Reporting: Adhere to financial reporting standards and regulations. Accurate financial reporting is crucial for transparency and accountability.
- Employee Rights: Understand and comply with labor laws that protect employee rights. This includes regulations on wages, working hours, and workplace safety.
- Environmental Regulations: If applicable, comply with environmental laws that govern waste management, emissions, and resource usage. This is increasingly important for sustainability efforts.
- Regular Audits: Conduct regular compliance audits to assess adherence to regulations. This helps identify gaps and areas for improvement. Implement OFAC screening best practices to ensure compliance with sanctions and trade regulations.
- Training and Awareness: Provide training to employees on compliance requirements. This ensures that everyone understands their responsibilities and the importance of compliance, including KYC and AML best practices.
7.5. Auditing Procedures
Auditing procedures are critical for evaluating the effectiveness of an organization’s operations and compliance with regulations. A well-structured auditing process includes:
- Define Objectives: Clearly outline the objectives of the audit. This could range from assessing financial accuracy to evaluating compliance with regulations.
- Develop an Audit Plan: Create a detailed audit plan that includes the scope, methodology, and timeline. This helps in organizing the audit process effectively.
- Gather Evidence: Collect relevant data and documentation to support the audit findings. This may include financial records, compliance reports, and operational procedures.
- Conduct Fieldwork: Perform fieldwork to assess processes and controls. This involves interviews, observations, and testing of transactions.
- Analyze Findings: Analyze the data collected to identify trends, discrepancies, and areas of concern. This step is crucial for drawing meaningful conclusions.
- Prepare Audit Report: Compile the findings into a comprehensive audit report. This report should include recommendations for improvement and a summary of key findings.
- Follow-Up: Implement a follow-up process to ensure that recommendations are acted upon. This may involve setting deadlines and assigning responsibilities for corrective actions.
- Continuous Improvement: Use audit findings to drive continuous improvement within the organization. Regular audits can help refine processes and enhance compliance efforts, particularly in the context of evolving technologies like AI and Blockchain. Best practices for compliance should be revisited regularly to ensure they remain effective and relevant.
8. Future Developments
The future of various industries is being shaped by rapid advancements in technology and innovative research directions. Understanding these developments is crucial for businesses, researchers, and consumers alike.
8.1 Emerging Technologies

Emerging technologies are at the forefront of transforming industries and enhancing everyday life. These technologies are characterized by their potential to disrupt existing markets and create new opportunities.
- Artificial Intelligence (AI) and Machine Learning (ML): AI and ML are revolutionizing sectors such as healthcare, finance, and transportation by enabling predictive analytics, automating processes, and enhancing decision-making. At Rapid Innovation, we leverage AI to help clients optimize operations, reduce costs, and improve customer experiences, ultimately driving greater ROI. New AI technology is continuously being developed to enhance these capabilities.
- Blockchain Technology: Originally developed for cryptocurrencies, blockchain is now being utilized in supply chain management, secure voting systems, and digital identity verification. Its decentralized nature ensures transparency and security. Rapid Innovation assists clients in implementing blockchain solutions that enhance trust and efficiency in their operations, leading to significant cost savings and improved accountability.
- Internet of Things (IoT): IoT connects everyday devices to the internet, allowing for real-time data collection and analysis. This technology is pivotal in smart homes, industrial automation, and healthcare monitoring. Our expertise in IoT enables clients to harness data for actionable insights, optimizing resource allocation and enhancing productivity.
- 5G Technology: The rollout of 5G networks promises faster internet speeds and lower latency, enhancing mobile connectivity and enabling advancements in autonomous vehicles, augmented reality, and smart cities. Rapid Innovation helps businesses integrate 5G capabilities to unlock new service offerings and improve operational efficiency.
- Quantum Computing: Although still in its infancy, quantum computing has the potential to solve complex problems much faster than traditional computers, which could revolutionize fields such as cryptography, drug discovery, and climate modeling. We are at the forefront of exploring quantum solutions that can provide our clients with a competitive edge in their respective markets.
- Biotechnology: Innovations in biotechnology, including CRISPR and gene editing, are paving the way for breakthroughs in medicine, agriculture, and environmental sustainability. Rapid Innovation collaborates with biotech firms to develop AI-driven solutions that accelerate research and development processes, enhancing their market readiness.
- Renewable Energy Technologies: Advances in solar, wind, and battery storage technologies are making renewable energy more efficient and accessible, which is crucial for combating climate change and promoting sustainable development. Our consulting services guide clients in adopting renewable technologies, resulting in reduced operational costs and improved sustainability metrics. New battery technology is particularly vital in this transition.
8.2 Research Directions
Research directions are essential for guiding the development of new technologies and addressing global challenges. The focus of research is shifting towards interdisciplinary approaches and collaborative efforts.
- Sustainability and Environmental Research: As climate change becomes a pressing issue, research is increasingly focused on sustainable practices, including developing eco-friendly materials, renewable energy sources, and waste reduction strategies. Rapid Innovation supports clients in integrating sustainable practices into their business models, enhancing their brand reputation and customer loyalty.
- Health and Medical Research: The COVID-19 pandemic has accelerated research in virology, vaccine development, and telemedicine. Future research will likely explore personalized medicine, mental health, and the integration of technology in healthcare. Our AI solutions are designed to assist healthcare providers in delivering personalized care, improving patient outcomes, and optimizing resource utilization.
- Data Privacy and Cybersecurity: With the rise of digital technologies, protecting personal data has become paramount. Research in cybersecurity aims to develop robust systems to safeguard against data breaches and cyberattacks. Rapid Innovation offers comprehensive cybersecurity solutions that help clients protect sensitive information, ensuring compliance and building trust with their customers.
- Human-Computer Interaction (HCI): As technology becomes more integrated into daily life, understanding how humans interact with machines is crucial. Research in HCI focuses on improving user experience, accessibility, and the ethical implications of technology. We prioritize user-centric design in our solutions, ensuring that technology enhances user engagement and satisfaction.
- Artificial Intelligence Ethics: As AI systems become more prevalent, ethical considerations are gaining importance. Research is directed towards ensuring fairness, accountability, and transparency in AI algorithms. Rapid Innovation is committed to developing ethical AI solutions that align with industry standards and promote responsible use of technology.
- Space Exploration and Technology: Research in space technology is expanding, focusing on Mars exploration, satellite technology, and the potential for space tourism. This field is driven by both governmental and private sector initiatives, including advancements in apple satellite technology. Our expertise in advanced technologies positions us to support clients in the aerospace sector, driving innovation and efficiency.
- Education Technology: The shift to online learning has prompted research into effective digital teaching methods, learning analytics, and the use of AI in education, aiming to enhance educational outcomes and accessibility. Rapid Innovation develops tailored educational solutions that leverage AI to improve learning experiences and outcomes.
- Interdisciplinary Collaboration: Future research is increasingly collaborative, combining insights from various fields such as engineering, social sciences, and humanities. This approach fosters innovation and addresses complex global challenges. At Rapid Innovation, we facilitate interdisciplinary partnerships that drive technological advancements and create value for our clients.
The landscape of emerging technologies and research directions is dynamic and ever-evolving. Staying informed about these developments, including new tech technologies and the latest technological advancements, is essential for leveraging opportunities and addressing challenges in the future. Rapid Innovation is dedicated to guiding clients through this landscape, ensuring they achieve their business goals efficiently and effectively.
8.3. Potential Innovations
The landscape of artificial intelligence (AI) is constantly evolving, and several potential innovations are on the horizon that could significantly impact various industries. These innovations are driven by advancements in technology, data analytics, and machine learning algorithms.
- Natural Language Processing (NLP) Enhancements: Future innovations in NLP could lead to more sophisticated chatbots and virtual assistants that understand context better and provide more accurate responses. This could revolutionize customer service and personal assistance, enabling businesses to enhance customer engagement and satisfaction. Recent AI innovations in this area are paving the way for more intuitive interactions.
- AI in Healthcare: Innovations in AI could lead to improved diagnostic tools, personalized medicine, and predictive analytics for patient care. For instance, AI algorithms could analyze medical images with greater accuracy, leading to earlier detection of diseases. Rapid Innovation can assist healthcare providers in implementing these AI solutions, ultimately improving patient outcomes and operational efficiency. The latest innovations in artificial intelligence are particularly promising for healthcare applications.
- Autonomous Systems: The development of more advanced autonomous systems, such as self-driving cars and drones, is a significant potential innovation. These systems could improve transportation efficiency and safety, reducing human error. Rapid Innovation can help organizations in the transportation sector leverage these technologies to optimize logistics and reduce costs. AI innovations 2022 have shown significant progress in this field.
- AI for Sustainability: Innovations in AI could also focus on environmental sustainability. AI can optimize energy consumption, improve waste management, and enhance resource allocation, contributing to a greener planet. By partnering with Rapid Innovation, businesses can implement AI-driven sustainability initiatives that not only reduce their carbon footprint but also lead to cost savings. AI data innovations are crucial in this area.
- Augmented Reality (AR) and Virtual Reality (VR): The integration of AI with AR and VR technologies could create immersive experiences in gaming, education, and training. This could lead to more engaging and effective learning environments. Rapid Innovation can support organizations in developing AR and VR applications that enhance training and customer experiences. The latest innovations in artificial intelligence are enhancing these technologies.
- AI-Driven Cybersecurity: As cyber threats become more sophisticated, AI innovations in cybersecurity could provide real-time threat detection and response, enhancing the security of sensitive data. Rapid Innovation can assist businesses in implementing AI-driven cybersecurity measures to protect their assets and maintain customer trust. Recent AI innovations are critical in developing robust cybersecurity frameworks.
8.4. Integration with Other AI Trends
The integration of AI with other emerging trends is crucial for maximizing its potential and creating synergies across various sectors. This convergence can lead to innovative solutions and improved efficiencies.
- AI and Internet of Things (IoT): The combination of AI and IoT can lead to smarter devices that learn from user behavior and optimize their functions. For example, smart home devices can adjust settings based on user preferences, enhancing comfort and energy efficiency. Rapid Innovation can help businesses harness this integration to create smarter products and services. AI and innovation in this space are driving significant advancements.
- AI and Blockchain: Integrating AI with blockchain technology can enhance data security and transparency. AI can analyze blockchain data for insights while ensuring that transactions are secure and tamper-proof. Rapid Innovation can guide organizations in leveraging this integration to improve operational efficiency and trust in their transactions. Innovations in artificial intelligence are making this integration more feasible.
- AI and Edge Computing: The integration of AI with edge computing allows for real-time data processing closer to the source. This can improve response times and reduce latency in applications such as autonomous vehicles and smart cities. Rapid Innovation can assist in developing edge AI solutions that enhance performance and user experience. AI innovations 2022 are particularly relevant in this context.
- AI and 5G Technology: The rollout of 5G networks will enable faster data transmission, which can enhance AI applications. This integration can support real-time analytics and improve the performance of AI-driven applications in various sectors. Rapid Innovation can help businesses capitalize on 5G to enhance their AI capabilities. The latest innovations in artificial intelligence are set to benefit from this technological leap.
- AI and Robotics: The combination of AI and robotics can lead to more intelligent machines capable of performing complex tasks. This integration can revolutionize manufacturing, logistics, and even healthcare, where robots can assist in surgeries or patient care. Rapid Innovation can support organizations in deploying AI-driven robotic solutions to streamline operations and improve service delivery. AI innovation examples in robotics are already demonstrating significant potential.
- AI and Augmented Analytics: The integration of AI with augmented analytics tools can empower users to derive insights from data without needing extensive technical expertise. This democratizes data analysis and enables more informed decision-making across organizations. Rapid Innovation can help businesses implement these tools to enhance their data-driven strategies. AI and innovation in analytics are transforming how organizations approach data.
9. Implementation Strategy
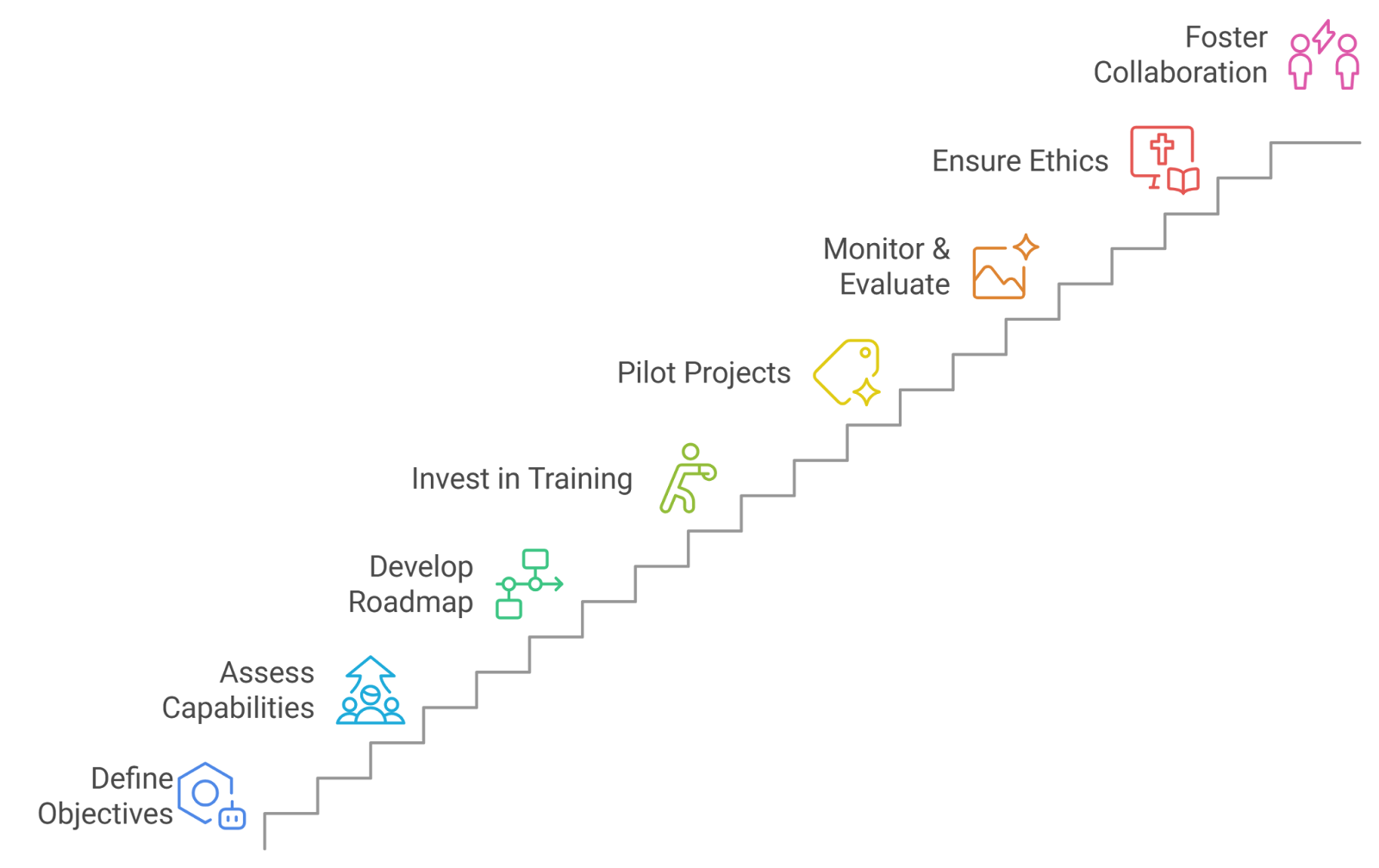
Implementing AI solutions requires a well-defined strategy to ensure successful integration and maximize benefits. A comprehensive implementation strategy involves several key steps.
- Define Objectives: Clearly outline the goals of the AI implementation. This could include improving efficiency, enhancing customer experience, or driving innovation.
- Assess Current Capabilities: Evaluate existing infrastructure, data quality, and workforce skills. Understanding current capabilities will help identify gaps that need to be addressed.
- Develop a Roadmap: Create a detailed roadmap that outlines the steps for implementation. This should include timelines, milestones, and resource allocation.
- Invest in Training: Equip employees with the necessary skills to work with AI technologies. Training programs should focus on both technical skills and understanding AI's implications for their roles.
- Pilot Projects: Start with pilot projects to test AI solutions in a controlled environment. This allows organizations to gather insights and make adjustments before a full-scale rollout.
- Monitor and Evaluate: Continuously monitor the performance of AI systems and evaluate their impact on business objectives. Use metrics to assess success and identify areas for improvement.
- Ensure Ethical Considerations: Address ethical concerns related to AI, such as bias and data privacy. Establish guidelines to ensure responsible AI use and maintain public trust.
- Foster Collaboration: Encourage collaboration between departments to ensure that AI initiatives align with overall business strategies. Cross-functional teams can provide diverse perspectives and drive innovation.
- Stay Updated: Keep abreast of the latest AI trends and technologies. Continuous learning and adaptation are essential for staying competitive in a rapidly changing landscape. AI and innovation are key to maintaining relevance in this dynamic environment.
9.1. Assessment and Planning
Assessment and planning are critical components of any successful project or initiative, particularly in the realms of AI and blockchain project planning. This phase involves evaluating the current situation, identifying needs, and developing a strategic plan to achieve desired outcomes efficiently and effectively.
- Conduct a thorough needs assessment to understand the specific requirements of the project, ensuring alignment with AI capabilities or blockchain functionalities.
- Gather data through surveys, interviews, and focus groups to inform decision-making, leveraging AI analytics for deeper insights.
- Analyze existing resources, capabilities, and constraints to identify gaps and opportunities, particularly in technology adoption and integration.
- Set clear, measurable objectives that align with the overall goals of the organization, focusing on maximizing ROI through innovative solutions.
- Develop a detailed project plan that outlines timelines, milestones, and responsibilities, ensuring that all stakeholders are on the same page.
- Utilize tools such as SWOT analysis (Strengths, Weaknesses, Opportunities, Threats) to guide planning efforts, particularly in assessing the competitive landscape in AI and blockchain project planning.
- Ensure that the plan is flexible enough to adapt to changing circumstances or new information, which is crucial in the fast-evolving tech landscape.
9.2. Stakeholder Engagement
Stakeholder engagement is essential for the success of any project, especially in AI and blockchain initiatives. It involves identifying, communicating with, and involving individuals or groups who have an interest in the project.
- Identify key stakeholders early in the process, including internal and external parties, to ensure comprehensive input and support.
- Develop a stakeholder map to visualize relationships and influence levels, which is vital for understanding the dynamics of technology adoption.
- Create a communication plan that outlines how and when stakeholders will be informed and consulted, ensuring transparency throughout the project lifecycle.
- Foster open lines of communication to encourage feedback and collaboration, which can lead to innovative solutions and improved project outcomes.
- Organize regular meetings or workshops to keep stakeholders engaged and informed, facilitating knowledge sharing and alignment on project goals.
- Address concerns and expectations proactively to build trust and support, particularly in projects involving complex technologies like AI and blockchain.
- Utilize surveys or feedback forms to gather input and measure stakeholder satisfaction, ensuring that their needs are met throughout the project.
9.3. Risk Management
Risk management is a systematic approach to identifying, assessing, and mitigating potential risks that could impact a project’s success, particularly in the context of AI and blockchain. Effective risk management helps organizations prepare for uncertainties and minimize negative outcomes.
- Begin by identifying potential risks through brainstorming sessions or risk assessment tools, focusing on technology-specific challenges.
- Classify risks based on their likelihood and potential impact on the project, particularly in terms of regulatory compliance and data security.
- Develop a risk management plan that outlines strategies for mitigating identified risks, ensuring that contingencies are in place for technology failures or market shifts.
- Assign responsibilities for monitoring and managing risks to specific team members, fostering accountability within the project team.
- Implement regular risk reviews to assess the effectiveness of mitigation strategies, adapting as necessary to the evolving landscape of AI and blockchain.
- Utilize risk management software or tools to track and manage risks efficiently, ensuring that all potential issues are monitored in real-time.
- Foster a culture of risk awareness within the organization to encourage proactive risk management, which is essential for navigating the complexities of AI and blockchain projects.
9.4. Change Management
Change management is a structured approach to transitioning individuals, teams, and organizations from a current state to a desired future state. It is essential for ensuring that changes are implemented smoothly and successfully, minimizing resistance and maximizing engagement.
- Understanding Change: Change can be driven by various factors, including technological advancements, market dynamics, and organizational restructuring. Recognizing the type of change is crucial for effective management, especially in the context of AI and Blockchain technologies, where rapid evolution is the norm.
- Key Components:
- Communication: Clear and consistent communication is vital. Stakeholders should be informed about the reasons for change, the benefits, and the expected outcomes. For instance, when implementing AI solutions, articulating the value of data-driven decision-making can enhance stakeholder buy-in. A change management communication plan can help structure these communications effectively.
- Training and Support: Providing adequate training and resources helps employees adapt to new processes or technologies. This can include workshops, online courses, and one-on-one coaching tailored to the specific tools and platforms being introduced, such as Blockchain systems or AI algorithms. Change management techniques can be employed to facilitate this process.
- Stakeholder Engagement: Involving stakeholders early in the process fosters buy-in and reduces resistance. This can be achieved through feedback sessions and collaborative planning, particularly important when integrating complex technologies like AI and Blockchain. Organizational change management strategies can guide this engagement.
- Models of Change Management: Various models exist to guide organizations through change, including:
- Kotter’s 8-Step Process: This model emphasizes creating urgency, forming a coalition, and anchoring new approaches in the culture, which is particularly relevant when introducing innovative technologies.
- ADKAR Model: Focuses on Awareness, Desire, Knowledge, Ability, and Reinforcement as key elements for successful change, ensuring that all aspects of the transition are addressed.
- Monitoring and Adjusting: Continuous monitoring of the change process allows for adjustments as needed. Feedback loops can help identify areas of resistance or misunderstanding, ensuring that the implementation of AI and Blockchain solutions remains aligned with organizational goals. Change management strategies for successful ERP implementation can also be considered in this context. For more information on how to implement AI solutions effectively, check out our AI knowledge management in 2024.
9.5. Success Metrics
Success metrics are critical for evaluating the effectiveness of initiatives and ensuring that organizational goals are met. They provide a framework for measuring progress and outcomes.
- Defining Success: Success can be defined in various ways, depending on the objectives of the project or initiative. Common definitions include increased revenue, improved customer satisfaction, and enhanced employee engagement, particularly relevant in the context of AI-driven insights and Blockchain transparency.
- Types of Metrics:
- Quantitative Metrics: These are numerical indicators that can be measured objectively. Examples include sales figures, market share, and production costs, which can be significantly impacted by the implementation of AI and Blockchain technologies.
- Qualitative Metrics: These metrics assess subjective factors, such as employee morale or customer feedback. Surveys and interviews can be useful tools for gathering qualitative data, especially when evaluating the impact of new technologies on user experience.
- Key Performance Indicators (KPIs): KPIs are specific metrics that align with strategic goals. They should be:
- SMART: Specific, Measurable, Achievable, Relevant, and Time-bound.
- Aligned with Objectives: Ensure that KPIs directly relate to the goals of the organization or project, such as tracking the efficiency gains from AI automation or the security improvements from Blockchain integration.
- Regular Review: Establishing a routine for reviewing success metrics is essential. This can involve:
- Monthly or quarterly performance reviews.
- Adjusting strategies based on metric outcomes.
- Celebrating successes and identifying areas for improvement, particularly in the context of ongoing AI and Blockchain projects.
10. Case Studies
Case studies provide real-world examples of how organizations have successfully implemented change management strategies and measured success metrics. They serve as valuable learning tools for other businesses.
- Example 1: Company A’s Digital Transformation:
- Challenge: Company A faced declining market share due to outdated technology.
- Change Management Approach: They adopted Kotter’s 8-Step Process, creating urgency and engaging employees through workshops focused on new AI tools and a change management strategy example.
- Success Metrics: Post-implementation, they measured success through increased online sales by 30% and improved customer satisfaction scores.
- Example 2: Company B’s Employee Engagement Initiative:
- Challenge: Company B struggled with low employee morale and high turnover rates.
- Change Management Approach: They implemented a feedback-driven culture, involving employees in decision-making processes, particularly around new Blockchain initiatives and organizational change strategies.
- Success Metrics: They tracked engagement through employee surveys, resulting in a 25% increase in employee satisfaction and a 15% reduction in turnover.
- Example 3: Company C’s Sustainability Program:
- Challenge: Company C aimed to reduce its carbon footprint but faced internal resistance.
- Change Management Approach: They provided training on sustainability practices and involved employees in green initiatives, leveraging AI for data analysis and employing an organizational change management strategy.
- Success Metrics: They measured success through a 40% reduction in waste and a 20% increase in employee participation in sustainability programs.
These case studies illustrate the importance of effective change management and the use of success metrics in achieving organizational goals. By learning from these examples, other organizations can develop their strategies for managing change and measuring success effectively, particularly in the rapidly evolving fields of AI and Blockchain.
10.1. Successful Implementations

Successful implementations of projects or strategies often serve as benchmarks for future endeavors. These implementations can be found across various sectors, including technology, healthcare, and education.
- Clear objectives: Successful projects begin with well-defined goals that align with the overall mission of the organization. At Rapid Innovation, we assist clients in establishing these objectives by leveraging AI-driven analytics to identify key performance indicators that matter most to their business.
- Stakeholder engagement: Involving all relevant stakeholders from the outset ensures that their needs and expectations are met. Our blockchain solutions facilitate transparent communication among stakeholders, ensuring that everyone is aligned and informed throughout the project lifecycle.
- Effective communication: Regular updates and transparent communication help maintain momentum and address any concerns promptly. We utilize AI-powered tools to automate updates and provide real-time insights, keeping all parties engaged and informed.
- Resource allocation: Adequate resources, including time, budget, and personnel, are crucial for the successful execution of any project. Our consulting services help clients optimize resource allocation by analyzing project requirements and aligning them with available resources.
- Continuous monitoring: Implementing a system for tracking progress allows for timely adjustments and improvements. Rapid Innovation employs AI algorithms to monitor project metrics continuously, enabling proactive decision-making and course corrections.
- Training and support: Providing necessary training and support to team members enhances their ability to contribute effectively. We offer tailored training programs that equip teams with the skills needed to leverage AI and blockchain technologies effectively.
- Flexibility: Being open to change and adapting to unforeseen challenges can significantly impact the success of a project. Our agile implementation strategy ensures that we can pivot quickly in response to changing project dynamics.
Examples of successful implementations include the rollout of electronic health records in hospitals, which improved patient care and streamlined operations, and the integration of cloud computing in businesses, leading to increased efficiency and cost savings. Rapid Innovation has played a pivotal role in similar projects, helping clients achieve greater ROI through innovative technology solutions, including our project estimation services.
10.2. Lessons Learned
Every project, whether successful or not, offers valuable lessons that can inform future initiatives. Analyzing these lessons helps organizations refine their strategies and avoid repeating past mistakes.
- Importance of planning: Comprehensive planning is essential to anticipate potential challenges and allocate resources effectively. Our team emphasizes the need for thorough planning in AI and blockchain projects to ensure alignment with business goals.
- Need for adaptability: Projects often encounter unexpected obstacles; being flexible allows teams to pivot and find alternative solutions. Rapid Innovation encourages a culture of adaptability, enabling teams to respond effectively to challenges.
- Value of feedback: Gathering feedback from stakeholders throughout the project can provide insights that enhance the final outcome. We implement feedback loops in our projects to ensure continuous improvement and stakeholder satisfaction.
- Risk management: Identifying and assessing risks early in the process can mitigate negative impacts and ensure smoother execution. Our risk assessment frameworks help clients navigate potential pitfalls in their AI and blockchain initiatives.
- Team dynamics: Understanding team strengths and weaknesses can improve collaboration and overall project performance. We conduct team assessments to ensure that the right skills are matched to project needs.
- Documentation: Keeping detailed records of processes and decisions can serve as a reference for future projects and help in knowledge transfer. Our documentation practices ensure that valuable insights are captured and shared across teams.
Organizations that embrace these lessons are better equipped to navigate complexities and achieve their objectives.
10.3. Failed Attempts Analysis
Analyzing failed attempts is crucial for understanding what went wrong and how to improve future efforts. Failure can provide insights that are just as valuable as success.
- Lack of clear goals: Many projects fail due to vague or unrealistic objectives that do not align with the organization's mission. Rapid Innovation helps clients define clear, measurable goals to guide their projects effectively.
- Insufficient stakeholder involvement: Ignoring the input of key stakeholders can lead to misalignment and resistance to change. Our approach emphasizes stakeholder engagement from the beginning, ensuring that all voices are heard.
- Poor communication: Inadequate communication can result in misunderstandings, confusion, and a lack of buy-in from team members. We utilize advanced communication tools to foster collaboration and clarity throughout the project.
- Resource constraints: Underestimating the resources needed, whether financial or human, can derail a project before it even begins. Our consulting services provide clients with accurate resource assessments to avoid such pitfalls.
- Inflexibility: A rigid approach that does not allow for adjustments in response to challenges can lead to failure. Rapid Innovation promotes an agile implementation strategy, enabling teams to adapt and thrive in dynamic environments.
- Ignoring data: Failing to analyze relevant data and metrics can prevent teams from making informed decisions. Our AI-driven analytics tools empower clients to leverage data effectively for better decision-making.
By conducting a thorough analysis of failed attempts, organizations can identify patterns and implement strategies to avoid similar pitfalls in the future. Rapid Innovation is committed to guiding clients through this process, ensuring that they learn and grow from every experience.
10.4. Best Practice Examples
Best practices are essential for organizations aiming to enhance efficiency, productivity, and overall performance. Here are some notable examples across various sectors:
- Lean Manufacturing: Companies like Toyota have successfully implemented lean manufacturing principles, focusing on waste reduction and continuous improvement. This approach has led to increased efficiency and reduced costs, aligning with business process management best practices.
- Agile Project Management: Organizations such as Spotify utilize agile methodologies to foster collaboration and adaptability. This practice allows teams to respond quickly to changes and deliver value incrementally, reflecting business analysis best practices.
- Sustainability Initiatives: Unilever has set ambitious sustainability goals, integrating environmental considerations into their business model. Their commitment to reducing plastic waste and promoting sustainable sourcing has garnered positive consumer response and brand loyalty, showcasing corporate responsibility best practices.
- Employee Engagement Programs: Google is renowned for its employee engagement strategies, including flexible work hours and a focus on work-life balance. These practices have resulted in high employee satisfaction and retention rates, which are considered good business practices.
- Data-Driven Decision Making: Amazon leverages big data analytics to optimize inventory management and enhance customer experience. This data-centric approach allows for informed decision-making and improved operational efficiency, embodying best practices of business analyst.
These best practice examples illustrate how organizations can adopt innovative strategies to achieve their goals while maintaining a competitive edge.
11. Economic Implications
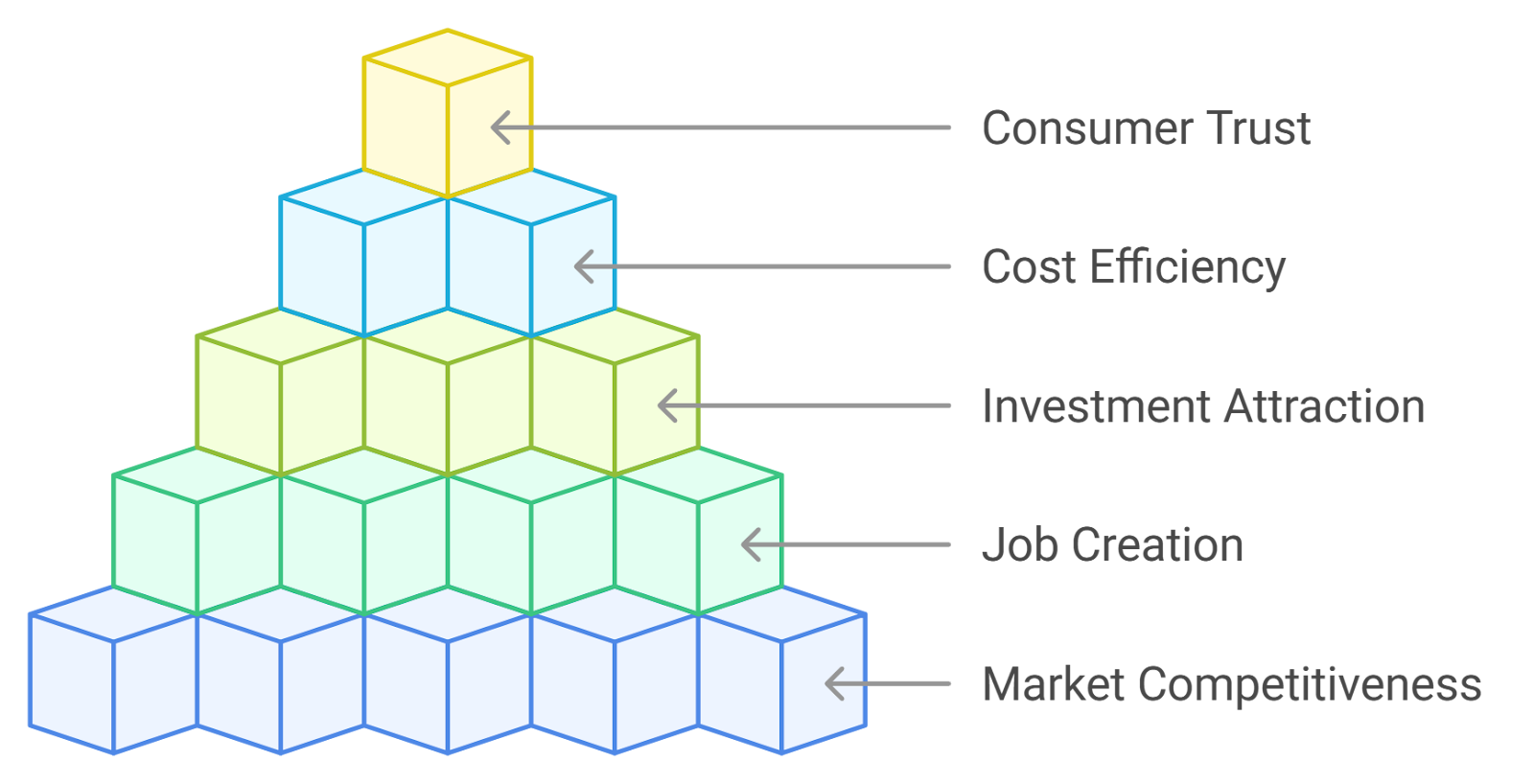
The economic implications of business practices can significantly influence an organization's bottom line and overall market dynamics. Understanding these implications is crucial for strategic planning and decision-making.
- Market Competitiveness: Effective business practices can enhance a company's competitive position, leading to increased market share and profitability. Companies that adopt innovative practices often outperform their competitors, as seen in best practices for small business.
- Job Creation: Organizations that implement best practices often experience growth, leading to job creation. This not only benefits the company but also contributes to the overall economy by reducing unemployment rates.
- Investment Attraction: Companies known for their efficient and sustainable practices are more likely to attract investors. Investors are increasingly looking for businesses that prioritize ethical practices and long-term sustainability, which aligns with best company practices.
- Cost Efficiency: Implementing best practices can lead to significant cost savings. For instance, lean manufacturing reduces waste, while data-driven decision-making optimizes resource allocation, reflecting best practices in a company.
- Consumer Trust and Loyalty: Businesses that prioritize ethical practices and sustainability often enjoy higher levels of consumer trust. This loyalty can translate into repeat business and positive word-of-mouth marketing, essential for best business practices.
11.1. Cost-Benefit Analysis
A cost-benefit analysis (CBA) is a systematic approach to evaluating the economic feasibility of a project or decision. It involves comparing the expected costs against the anticipated benefits to determine the overall value.
- Identifying Costs: The first step in a CBA is to identify all relevant costs, including direct costs (e.g., materials, labor) and indirect costs (e.g., overhead, opportunity costs).
- Estimating Benefits: Next, organizations must estimate the potential benefits, which can include increased revenue, cost savings, and intangible benefits such as improved customer satisfaction, reflecting business development best practices.
- Quantifying Data: Assigning monetary values to both costs and benefits is crucial. This may involve using historical data, market research, or expert opinions to ensure accuracy.
- Calculating Net Present Value (NPV): The NPV is calculated by discounting future cash flows to their present value. A positive NPV indicates that the benefits outweigh the costs, making the project worthwhile.
- Sensitivity Analysis: Conducting a sensitivity analysis helps assess how changes in key assumptions (e.g., cost estimates, benefit projections) impact the overall outcome. This step is vital for understanding the risks involved.
- Decision-Making: The final step involves making informed decisions based on the CBA results. If the benefits significantly exceed the costs, the project is likely a good investment.
Cost-benefit analysis is a powerful tool that aids organizations in making strategic decisions, ensuring that resources are allocated efficiently and effectively. At Rapid Innovation, we leverage our expertise in AI and Blockchain to enhance these best practices, enabling our clients to achieve greater ROI through tailored solutions that optimize their operations and drive sustainable growth, including business process mapping best practices and b2b customer onboarding best practices.
11.2. Investment Requirements
Investment requirements are crucial for any business venture, as they outline the financial resources needed to initiate and sustain operations. Understanding these requirements helps in planning and securing funding.
- Initial Capital: This includes costs for equipment, technology, and infrastructure. For instance, a tech startup may need to invest in software development and hardware, particularly when integrating AI solutions or blockchain technology to enhance operational efficiency. Investment requirements for technology can significantly influence the initial capital needed.
- Operational Costs: Ongoing expenses such as salaries, utilities, and rent must be accounted for. These costs can vary significantly based on location and industry, especially in sectors where AI and blockchain are being implemented to streamline processes and reduce overhead. Investment requirements for technology also encompass these operational costs.
- Marketing Budget: Allocating funds for marketing is essential to reach potential customers. This may include digital marketing, traditional advertising, and public relations efforts, particularly in promoting innovative AI-driven products or blockchain services. Investment requirements for technology should include a marketing budget to effectively communicate the value of these innovations.
- Contingency Funds: Setting aside a portion of the budget for unexpected expenses is wise. This can help mitigate risks associated with market fluctuations or operational challenges, especially in rapidly evolving fields like AI and blockchain. Investment requirements for technology should also consider the need for contingency funds.
- Funding Sources: Identifying potential funding sources, such as venture capital, loans, or grants, is vital. Each source has its own implications for ownership and repayment, and understanding these can help businesses leverage their investments in technology effectively. Investment requirements for technology will often dictate the types of funding sources that are most appropriate. For more insights on leveraging technology for investment strategies, you can refer to AI agents for investment strategy.
11.3. ROI Considerations
Return on Investment (ROI) is a key metric for evaluating the profitability of an investment. Understanding ROI considerations helps businesses make informed financial decisions.
- Calculation of ROI: ROI is calculated by dividing the net profit from an investment by the initial cost of the investment. A higher ROI indicates a more profitable investment, particularly when leveraging AI and blockchain to optimize processes and reduce costs.
- Time Frame: The time frame for achieving ROI can vary. Short-term investments may yield quicker returns, while long-term investments may take years to realize profits, especially in technology sectors where initial development costs can be high.
- Risk Assessment: Evaluating the risks associated with an investment is crucial. Higher potential returns often come with increased risks, so businesses must weigh these factors carefully, particularly in the volatile landscape of AI and blockchain technologies.
- Industry Benchmarks: Comparing ROI with industry benchmarks can provide insights into performance. This helps businesses understand if they are on track or need to adjust their strategies, especially when competing in tech-driven markets.
- Impact of Market Conditions: Economic factors can influence ROI. For example, a recession may reduce consumer spending, affecting sales and profitability, which is particularly relevant for businesses relying on emerging technologies.
11.4. Market Impact
Market impact refers to the effect that a business or investment has on the overall market. Understanding market impact is essential for strategic planning and positioning.
- Competitive Landscape: New entrants can disrupt existing markets, leading to increased competition. This can force established companies to innovate or reduce prices to maintain market share, particularly in sectors where AI and blockchain are reshaping traditional business models.
- Consumer Behavior: Changes in consumer preferences can significantly impact market dynamics. Businesses must stay attuned to trends to adapt their offerings accordingly, especially as consumers become more aware of the benefits of AI and blockchain technologies.
- Economic Factors: Broader economic conditions, such as inflation or unemployment rates, can influence market impact. A strong economy may boost consumer spending, while a downturn can have the opposite effect, affecting tech investments.
- Regulatory Environment: Government regulations can shape market impact. Compliance with laws and regulations is essential for sustainable operations, particularly in the highly regulated fields of AI and blockchain.
- Technological Advancements: Innovations can create new markets or transform existing ones. Companies that leverage technology effectively can gain a competitive edge and drive market growth, particularly through the strategic implementation of AI and blockchain solutions.