Table Of Contents
Category
Artificial Intelligence
Blockchain
Security
CRM
FinTech
1. Introduction to AI-Powered Transaction Monitoring
AI-powered transaction monitoring is revolutionizing the way financial institutions detect and prevent fraudulent activities. By leveraging advanced algorithms and machine learning techniques, these systems analyze vast amounts of transaction data in real-time, enabling organizations to identify suspicious patterns and behaviors that may indicate fraud or money laundering.
- AI systems can process data at incredible speeds, far surpassing human capabilities.
- They utilize historical data to learn and adapt, improving their accuracy over time.
- These systems can operate 24/7, ensuring continuous surveillance without the need for breaks or downtime.
At Rapid Innovation, we specialize in implementing AI-driven solutions that enhance AI transaction monitoring capabilities for our clients. Our expertise allows us to tailor systems that not only improve detection rates but also align with specific business goals, ultimately driving greater ROI.
The integration of AI in transaction monitoring not only enhances the efficiency of detecting anomalies but also reduces the number of false positives. Traditional methods often lead to a high volume of alerts, overwhelming compliance teams. AI helps streamline this process by focusing on genuine threats, allowing human analysts to concentrate on more complex cases.
- Machine learning models can be trained to recognize specific patterns associated with fraudulent activities.
- Natural language processing (NLP) can analyze unstructured data, such as customer communications, to identify potential risks.
- AI can adapt to new threats by continuously learning from new data inputs, making it a dynamic solution for financial surveillance.
As financial institutions face increasing regulatory pressures and the need for robust risk management strategies, AI-powered transaction monitoring systems are becoming essential tools. They not only help in compliance with regulations but also protect the institution's reputation and financial stability.
- Regulatory bodies are increasingly mandating the use of advanced technologies for transaction monitoring.
- Institutions that adopt AI solutions can gain a competitive edge by improving their risk management capabilities.
- The cost-effectiveness of AI systems can lead to significant savings in operational expenses related to fraud detection.
At Rapid Innovation, we guide our clients through the implementation of these advanced technologies, ensuring they not only meet regulatory requirements but also enhance their operational efficiency. Our solutions are designed to provide a measurable impact on your bottom line, allowing you to focus on strategic growth.
In conclusion, AI-powered transaction monitoring represents a significant advancement in financial surveillance and risk management. By harnessing the power of artificial intelligence, financial institutions can enhance their ability to detect and prevent fraudulent activities, ensuring a safer and more secure financial environment. Rapid Innovation is here to support you in this transformative journey, helping you achieve your business goals efficiently and effectively. For more insights on the advantages and future of AI in finance.
Refer to the image for a visual representation of AI-powered transaction monitoring and its benefits:

1.1. Evolution of Transaction Monitoring Systems
Transaction monitoring systems have undergone significant evolution since their inception. Initially, these systems were rudimentary, focusing primarily on basic transaction tracking. Over the years, advancements in technology and the increasing complexity of financial transactions have driven the development of more sophisticated monitoring systems, including automated transaction monitoring systems and AML transaction monitoring solutions.
- Early systems relied on manual processes and simple algorithms to flag suspicious activities.
- The introduction of automated systems in the late 1990s marked a turning point, allowing for real-time monitoring of transactions.
- With the rise of digital banking and online transactions, the need for more robust systems, such as transaction monitoring systems for banks, became evident.
- Modern transaction monitoring systems utilize advanced analytics, machine learning, and big data to detect anomalies and potential fraud.
- These systems are now capable of analyzing vast amounts of data across multiple channels, providing a comprehensive view of customer behavior.
The evolution of transaction monitoring systems reflects the growing need for financial institutions to combat fraud and comply with regulatory requirements. As financial crimes become more sophisticated, so too must the systems designed to detect them. At Rapid Innovation, we leverage our expertise in AI and blockchain technology to develop tailored transaction monitoring solutions, including AML monitoring systems and Actimize transaction monitoring, that enhance security and efficiency, ultimately driving greater ROI for our clients. For more insights on how AI agents can enhance transaction security.
1.2. The Role of AI in Modern Financial Surveillance
Artificial Intelligence (AI) has become a cornerstone of modern financial surveillance, enhancing the capabilities of transaction monitoring systems. AI technologies enable financial institutions to process and analyze large datasets more efficiently, leading to improved detection of suspicious activities.
- AI algorithms can learn from historical data, identifying patterns that may indicate fraudulent behavior.
- Machine learning models continuously adapt to new data, improving their accuracy over time.
- Natural language processing (NLP) allows for the analysis of unstructured data, such as customer communications, to identify potential risks.
- AI-driven systems can reduce false positives, allowing compliance teams to focus on genuine threats rather than sifting through numerous alerts.
- Predictive analytics powered by AI can forecast potential risks, enabling proactive measures to mitigate them.
The integration of AI in financial surveillance not only enhances detection capabilities but also streamlines compliance processes, making it easier for institutions to adhere to regulatory standards. Rapid Innovation specializes in implementing AI-driven solutions, including automated transaction monitoring systems and AML transaction monitoring procedures, that empower financial institutions to achieve operational excellence and maximize their return on investment.
1.3. Regulatory Framework and Compliance Requirements
The regulatory framework surrounding transaction monitoring and financial surveillance is complex and continually evolving. Financial institutions must navigate a myriad of regulations designed to prevent money laundering, fraud, and other financial crimes.
- Key regulations include the Bank Secrecy Act (BSA), the USA PATRIOT Act, and the European Union's Anti-Money Laundering (AML) directives.
- Compliance requirements often mandate the implementation of robust transaction monitoring systems, such as Oracle AML transaction monitoring and Norkom transaction monitoring, to detect and report suspicious activities.
- Institutions are required to conduct regular risk assessments to identify vulnerabilities in their systems and processes.
- Failure to comply with regulatory requirements can result in severe penalties, including fines and reputational damage.
- Regulatory bodies are increasingly emphasizing the importance of technology in compliance, encouraging the adoption of advanced monitoring systems like Fircosoft transaction monitoring and Fortent transaction monitoring.
Understanding the regulatory landscape is crucial for financial institutions to ensure compliance and protect themselves from potential risks. As regulations continue to evolve, staying informed and adapting to new requirements will be essential for effective financial surveillance. Rapid Innovation offers consulting services to help clients navigate these complexities, ensuring they remain compliant while optimizing their transaction monitoring systems for better ROI.
Refer to the image for a visual representation of the evolution of transaction monitoring systems.
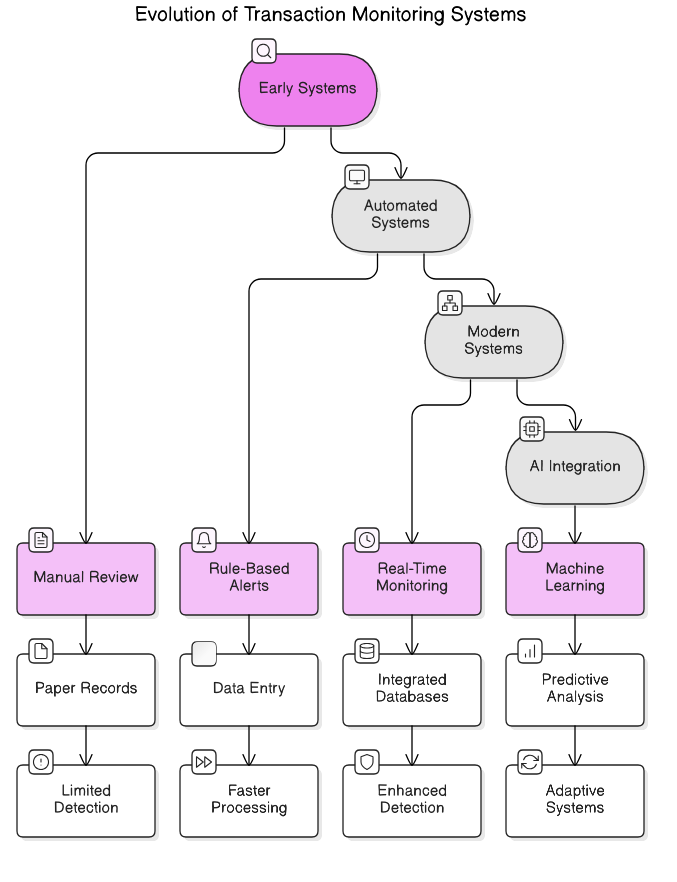
1.4. Key Challenges in Traditional Transaction Monitoring
Traditional transaction monitoring systems face several key challenges that can hinder their effectiveness in detecting fraudulent activities. These challenges include:
- High False Positive Rates: Traditional systems often generate a significant number of false positives, leading to unnecessary investigations. This can overwhelm compliance teams and divert resources from genuine threats, particularly when using transaction monitoring tools.
- Limited Adaptability: Many traditional systems rely on static rules and thresholds that do not adapt to evolving fraud patterns. As fraudsters develop new tactics, these systems may fail to identify emerging risks, which is a significant drawback of conventional aml transaction monitoring tools.
- Data Silos: Transaction data is often stored in separate systems, making it difficult to obtain a comprehensive view of customer behavior. This fragmentation can result in missed opportunities to detect suspicious activities, a common issue with traditional transaction monitoring solutions.
- Manual Processes: Traditional monitoring often involves manual reviews of flagged transactions, which can be time-consuming and prone to human error. This reliance on manual intervention can slow down response times to potential fraud, especially when using aml monitoring software.
- Regulatory Compliance: Keeping up with changing regulations can be challenging for traditional systems. Failure to comply can result in hefty fines and reputational damage, a risk that organizations face when relying on outdated transaction monitoring software vendors.
- Scalability Issues: As transaction volumes increase, traditional systems may struggle to scale effectively. This can lead to delays in monitoring and increased risk of undetected fraud, particularly for those using saas transaction monitoring solutions.
- Lack of Real-Time Analysis: Many traditional systems do not provide real-time monitoring capabilities, which is crucial for timely detection and response to fraudulent activities. This limitation is often seen in legacy aml monitoring tools.
2. Core AI Technologies in Transaction Monitoring
Artificial Intelligence (AI) technologies are transforming transaction monitoring by enhancing detection capabilities and improving efficiency. Key AI technologies include:
- Machine Learning: Machine learning algorithms analyze vast amounts of transaction data to identify patterns and anomalies. These algorithms can learn from historical data, improving their accuracy over time, which is a significant advantage over traditional aml transaction monitoring solutions.
- Natural Language Processing (NLP): NLP techniques can be used to analyze unstructured data, such as customer communications and transaction notes, to identify potential fraud indicators.
- Predictive Analytics: Predictive analytics leverage historical data to forecast future trends and behaviors, allowing organizations to proactively address potential risks.
- Neural Networks: These complex algorithms mimic the human brain's structure and function, enabling them to recognize intricate patterns in data that traditional methods may overlook.
- Anomaly Detection: AI-driven anomaly detection systems can identify unusual transaction patterns in real-time, flagging them for further investigation, which is essential for effective transaction monitoring services.
2.1. Machine Learning Algorithms
Machine learning algorithms are at the forefront of modern transaction monitoring systems. They offer several advantages over traditional methods:
- Adaptive Learning: Machine learning models can adapt to new data and evolving fraud patterns, reducing the reliance on static rules. This adaptability enhances the system's ability to detect emerging threats, a critical feature for organizations using nice actimize transaction monitoring.
- Improved Accuracy: By analyzing large datasets, machine learning algorithms can identify subtle patterns that may indicate fraudulent behavior. This leads to a reduction in false positives and more accurate risk assessments.
- Automated Decision-Making: Machine learning can automate the decision-making process for flagging transactions, allowing compliance teams to focus on high-risk cases that require human intervention.
- Real-Time Processing: Many machine learning algorithms can process transactions in real-time, enabling organizations to respond quickly to potential fraud.
- Feature Engineering: Machine learning allows for the creation of new features from existing data, enhancing the model's ability to detect fraud. This can include customer behavior patterns, transaction history, and more.
- Scalability: Machine learning algorithms can handle large volumes of data, making them suitable for organizations with high transaction rates. This scalability ensures that monitoring remains effective as transaction volumes grow, particularly for those utilizing norkom aml system solutions.
- Continuous Improvement: Machine learning models can be continuously updated with new data, allowing them to improve over time. This ongoing learning process helps maintain the system's effectiveness against evolving fraud tactics.
At Rapid Innovation, we leverage these advanced AI technologies to help our clients overcome the challenges of traditional transaction monitoring. By implementing machine learning and other AI-driven solutions, we enable organizations to achieve greater efficiency, reduce false positives, and enhance their overall fraud detection capabilities. This not only leads to improved compliance but also significantly increases return on investment (ROI) by allowing teams to focus on high-risk cases and streamline their operations, especially when using tools like sumsub transaction monitoring. For more information on how AI agents can assist in transaction anomaly detection.
Refer to the image for a visual representation of the key challenges in traditional transaction monitoring systems.
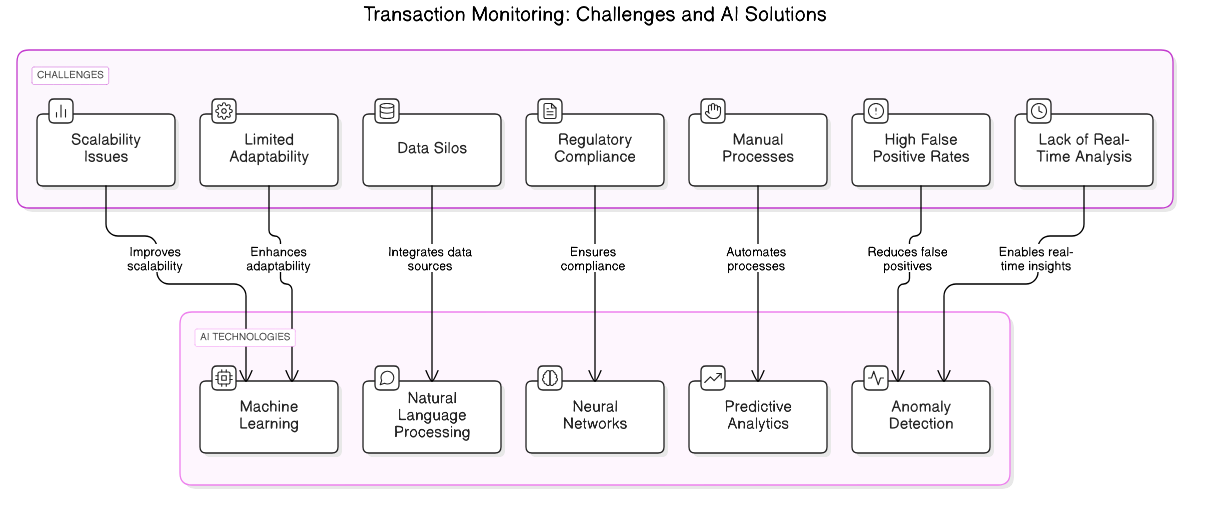
2.1.1. Supervised Learning Applications
Supervised learning is a type of machine learning where a model is trained on labeled data. This means that the input data is paired with the correct output, allowing the model to learn the relationship between the two. Supervised learning has a wide range of applications across various industries, and at Rapid Innovation, we leverage these capabilities to help our clients achieve their business goals efficiently and effectively.
- Image Recognition: Supervised learning is extensively used in image classification tasks. For instance, convolutional neural networks (CNNs) can identify objects in images, which is crucial for applications like facial recognition and autonomous vehicles. Rapid Innovation can develop tailored image recognition solutions that enhance security systems or improve user experiences in applications. This includes applications of supervised learning in computer vision, where models are trained to recognize and classify images accurately.
- Spam Detection: Email services utilize supervised learning algorithms to classify emails as spam or not. By training on a dataset of labeled emails, the model learns to identify patterns that distinguish spam from legitimate messages. Our expertise in this area can help businesses maintain clean communication channels, thereby increasing productivity.
- Medical Diagnosis: In healthcare, supervised learning models can assist in diagnosing diseases by analyzing patient data. For example, algorithms can predict the likelihood of conditions like diabetes or heart disease based on historical patient records. Rapid Innovation can collaborate with healthcare providers to implement predictive analytics that improve patient outcomes and reduce costs. Big self-supervised models can also advance medical image classification, enhancing diagnostic accuracy.
- Financial Forecasting: Supervised learning is also applied in finance for predicting stock prices or credit scoring. By analyzing historical data, models can forecast future trends and help in making informed investment decisions. Our solutions can empower financial institutions to optimize their investment strategies and enhance risk management.
- Natural Language Processing (NLP): Supervised learning techniques are used in NLP for tasks such as sentiment analysis and language translation. Models are trained on labeled text data to understand and generate human language effectively. Rapid Innovation can help businesses harness NLP to improve customer interactions and gain insights from customer feedback.
- Real World Applications: The practical application of supervised learning spans various sectors, including retail, where it can be used for customer segmentation and targeted marketing. Real-time examples of supervised learning can be seen in recommendation systems that suggest products based on user behavior.
2.1.2. Unsupervised Learning for Anomaly Detection
Unsupervised learning involves training a model on data without labeled outputs. This approach is particularly useful for anomaly detection, where the goal is to identify unusual patterns that do not conform to expected behavior. Rapid Innovation employs unsupervised learning techniques to enhance operational efficiency and security for our clients.
- Fraud Detection: In finance, unsupervised learning algorithms can detect fraudulent transactions by identifying anomalies in spending patterns. These models analyze transaction data to flag unusual activities that may indicate fraud. Our solutions can significantly reduce financial losses for businesses by providing real-time fraud detection capabilities.
- Network Security: Unsupervised learning is employed in cybersecurity to monitor network traffic. By establishing a baseline of normal behavior, the model can identify deviations that may signify a security breach or cyber attack. Rapid Innovation can implement robust security measures that protect sensitive data and maintain business integrity.
- Manufacturing Quality Control: In manufacturing, unsupervised learning can help detect defects in products. By analyzing sensor data from production lines, models can identify anomalies that suggest quality issues, allowing for timely interventions. Our expertise can lead to improved product quality and reduced waste for manufacturing clients.
- Healthcare Monitoring: In healthcare, unsupervised learning can be used to monitor patient vitals. By analyzing data from wearable devices, models can detect anomalies that may indicate health risks, prompting early medical intervention. Rapid Innovation can assist healthcare providers in implementing proactive monitoring systems that enhance patient care.
- Customer Behavior Analysis: Businesses can use unsupervised learning to analyze customer behavior. By identifying unusual purchasing patterns, companies can tailor marketing strategies or improve customer service. Our solutions can help businesses better understand their customers, leading to increased satisfaction and loyalty.
2.1.3. Reinforcement Learning in Dynamic Systems
Reinforcement learning (RL) is a type of machine learning where an agent learns to make decisions by interacting with an environment. The agent receives feedback in the form of rewards or penalties, allowing it to learn optimal strategies over time. This approach is particularly effective in dynamic systems where conditions change frequently, and Rapid Innovation is at the forefront of applying RL to drive innovation.
- Robotics: RL is widely used in robotics for training autonomous robots to perform tasks. Robots learn to navigate environments, manipulate objects, and complete complex tasks through trial and error, improving their performance over time. Our expertise in robotics can lead to the development of advanced automation solutions that enhance operational efficiency.
- Game Playing: Reinforcement learning has gained attention for its success in game playing. Algorithms like AlphaGo have demonstrated the ability to defeat human champions in complex games by learning optimal strategies through extensive simulations. Rapid Innovation can apply similar techniques to develop engaging and adaptive gaming experiences.
- Autonomous Vehicles: In the realm of self-driving cars, RL is employed to make real-time driving decisions. The vehicle learns to navigate traffic, avoid obstacles, and follow traffic rules by receiving feedback from its environment. Our solutions can contribute to the advancement of autonomous vehicle technology, improving safety and efficiency.
- Resource Management: RL can optimize resource allocation in dynamic systems, such as energy management in smart grids. By learning from past decisions, the system can adjust energy distribution based on demand fluctuations. Rapid Innovation can help organizations implement smart resource management systems that reduce costs and enhance sustainability.
- Personalized Recommendations: In e-commerce and streaming services, RL is used to provide personalized recommendations. The system learns user preferences over time, improving the relevance of suggestions based on user interactions. Our expertise can help businesses enhance customer engagement and drive sales through tailored recommendations.
At Rapid Innovation, we are committed to leveraging AI and blockchain technologies to help our clients achieve greater ROI and drive their business success.
Refer to the image for a visual representation of the applications of supervised learning in various industries.

2.2. Natural Language Processing
Natural Language Processing (NLP) is a subfield of artificial intelligence that focuses on the interaction between computers and humans through natural language. The goal of NLP is to enable machines to understand, interpret, and respond to human language in a valuable way.
- NLP encompasses various tasks, including:
- Text analysis
- Sentiment analysis
- Language translation
- Speech recognition
- Chatbots and virtual assistants
- Key components of NLP include:
- Tokenization: Breaking down text into smaller units, such as words or phrases.
- Part-of-speech tagging: Identifying the grammatical parts of speech in a sentence.
- Named entity recognition: Detecting and classifying key entities in text, such as names, dates, and locations.
NLP techniques often utilize machine learning algorithms to improve accuracy and efficiency. For instance, models like BERT and GPT-3 have revolutionized the field by enabling more nuanced understanding and generation of human language. Natural language processing techniques are essential for tasks such as natural language understanding and natural language generation.
- Applications of NLP are vast and include:
- Customer service automation through chatbots, which can significantly reduce operational costs and improve response times.
- Content recommendation systems that enhance user engagement and retention by providing personalized experiences.
- Social media monitoring for brand sentiment, allowing businesses to gauge public perception and adjust strategies accordingly.
- Language translation services that facilitate global communication and expand market reach.
At Rapid Innovation, we leverage NLP to help clients streamline operations, enhance customer interactions, and ultimately achieve greater ROI through improved efficiency and user satisfaction. Our expertise in natural language programming and natural language processing using Python allows us to develop tailored solutions for various industries. For more information on related techniques, you can read about pattern recognition in machine learning.
2.3. Pattern Recognition Systems
Pattern recognition systems are a branch of machine learning that focuses on the identification and classification of patterns in data. These systems are designed to recognize regularities and anomalies in various forms of data, including images, audio, and text.
- Key aspects of pattern recognition include:
- Feature extraction: Identifying the most relevant features from raw data to improve classification accuracy.
- Classification: Assigning labels to data based on learned patterns.
- Clustering: Grouping similar data points together without predefined labels.
- Common techniques used in pattern recognition are:
- Statistical methods: Utilizing probability and statistics to identify patterns.
- Neural networks: Employing deep learning architectures to recognize complex patterns in large datasets.
- Support vector machines: A supervised learning model that finds the optimal hyperplane for classification tasks.
- Applications of pattern recognition systems are widespread, including:
- Facial recognition technology in security systems, enhancing safety and access control.
- Optical character recognition (OCR) for digitizing printed text, improving data accessibility and management.
- Medical image analysis for disease detection, aiding healthcare professionals in making timely and accurate diagnoses.
- Voice recognition systems in virtual assistants, streamlining user interactions and enhancing user experience.
At Rapid Innovation, we implement advanced pattern recognition systems to help clients unlock insights from their data, leading to informed decision-making and increased operational efficiency.
2.4. Deep Learning Networks
Deep learning networks are a subset of machine learning that utilize neural networks with many layers (hence "deep") to analyze various forms of data. These networks are particularly effective in handling large datasets and complex problems.
- Characteristics of deep learning networks include:
- Multi-layer architecture: Comprising an input layer, multiple hidden layers, and an output layer.
- Non-linear activation functions: Enabling the network to learn complex patterns and relationships in data.
- Backpropagation: A method for training the network by adjusting weights based on the error of predictions.
- Types of deep learning networks include:
- Convolutional Neural Networks (CNNs): Primarily used for image processing and computer vision tasks.
- Recurrent Neural Networks (RNNs): Designed for sequential data, such as time series or natural language processing.
- Generative Adversarial Networks (GANs): Comprising two networks that compete against each other to generate new data samples.
Deep learning networks have transformed various industries through applications such as:
- Autonomous vehicles using computer vision for navigation, enhancing safety and efficiency in transportation.
- Real-time language translation in applications like Skype, breaking down language barriers and facilitating global communication.
- Personalized content recommendations on streaming platforms, driving user engagement and satisfaction.
- The success of deep learning is largely attributed to:
- The availability of large datasets
- Advances in computational power, particularly GPUs
- Improved algorithms and frameworks, such as TensorFlow and PyTorch
At Rapid Innovation, we harness the power of deep learning networks to deliver innovative solutions that drive business growth and maximize ROI. Deep learning networks continue to evolve, pushing the boundaries of what is possible in artificial intelligence and machine learning.
2.5. Real-time Analytics Engines
Real-time analytics engines are crucial for processing and analyzing data as it is generated. These engines enable organizations to make immediate decisions based on current data, which is essential in today’s fast-paced business environment.
- Speed and Efficiency: Real-time analytics engines can process large volumes of data in milliseconds, allowing businesses to respond quickly to changing conditions. This speed is vital for applications like fraud detection, where immediate action can prevent significant losses.
- Data Sources: These engines can ingest data from various sources, including social media, IoT devices, and transactional databases. This capability allows organizations to have a comprehensive view of their operations and customer interactions.
- Use Cases: Common use cases for real-time analytics include monitoring customer behavior on e-commerce platforms, analyzing social media sentiment during product launches, and tracking supply chain logistics in real-time.
- Technologies: Popular technologies used in real-time analytics engines include Apache Kafka, Apache Flink, and Amazon Kinesis. These tools facilitate the streaming of data and enable complex event processing.
- Benefits: The benefits of real-time analytics engines include enhanced decision-making capabilities, improved customer experiences through personalized interactions, and increased operational efficiency by identifying bottlenecks in real-time.
- Challenges: Despite their advantages, real-time analytics engines face challenges such as data quality issues that can lead to inaccurate insights, the need for robust infrastructure to handle high data throughput, and ensuring data security and compliance with regulations.
At Rapid Innovation, we leverage real-time analytics engines to help our clients achieve greater ROI by enabling them to make data-driven decisions swiftly. For instance, in the retail sector, we have implemented real-time analytics solutions that allow businesses to monitor customer behavior and adjust marketing strategies on-the-fly, resulting in increased sales and customer satisfaction. For more information on best practices in designing scalable AI agent architecture.
3. AI Agent Architectures
AI agent architectures refer to the frameworks and structures that enable the development of intelligent agents capable of performing tasks autonomously. These architectures are foundational for creating systems that can learn, adapt, and interact with their environment.
- Types of Architectures: There are several types of AI agent architectures, including reactive agents that respond to stimuli without internal state, deliberative agents that plan and reason about their actions, and hybrid agents that combine both reactive and deliberative approaches.
- Key Components: Essential components of AI agent architectures include:
- Perception: The ability to sense and interpret data from the environment.
- Reasoning: The capability to make decisions based on the perceived data.
- Action: The execution of decisions through various means, such as robotic movement or digital responses.
- Applications: AI agent architectures are used in various fields, such as autonomous vehicles that navigate and make driving decisions, virtual assistants that understand and respond to user queries, and smart home devices that learn user preferences over time.
- Scalability: A well-designed AI agent architecture should be scalable, allowing for the addition of new agents or functionalities without significant rework.
- Interoperability: Ensuring that different agents can communicate and work together effectively is crucial for complex systems.
3.1. Agent Design Principles
Agent design principles are guidelines that inform the development of effective and efficient AI agents. These principles help ensure that agents are capable of performing their intended tasks while being adaptable to changing environments.
- Autonomy: Agents should operate independently, making decisions without human intervention. This autonomy allows for faster responses and reduces the need for constant oversight.
- Adaptability: Agents must be able to learn from their experiences and adapt to new situations. This adaptability is essential for maintaining performance in dynamic environments.
- Social Ability: Agents should be designed to interact with humans and other agents effectively. This social ability enhances collaboration and improves user experience.
- Robustness: Agents need to be resilient to failures and capable of recovering from errors. A robust design ensures that agents can continue functioning even in adverse conditions.
- Efficiency: The design should prioritize resource efficiency, ensuring that agents use minimal computational power and memory while performing their tasks.
- Transparency: Agents should operate in a way that their decision-making processes are understandable to users. Transparency builds trust and allows for better human-agent collaboration.
- Ethical Considerations: Designers must consider the ethical implications of agent behavior, ensuring that agents act in ways that are aligned with societal values and norms.
- Testing and Evaluation: Continuous testing and evaluation of agents are crucial to ensure they meet performance standards and can handle real-world scenarios effectively.
By adhering to these design principles, developers can create AI agents that are not only functional but also reliable and user-friendly. At Rapid Innovation, we apply these principles to develop AI solutions that enhance operational efficiency and drive business growth for our clients, including the integration of real-time analytics engines to optimize decision-making processes.
3.2. Multi-Agent Systems
Multi-Agent Systems (MAS) consist of multiple interacting intelligent agents that can work together to solve complex problems. These systems are designed to mimic the collaborative behavior observed in nature, such as flocks of birds or swarms of bees. Agents in MAS can be autonomous, meaning they can operate independently while still communicating and coordinating with other agents. They are capable of learning from their environment and adapting their behavior based on experiences. MAS can be applied in various fields, including robotics, telecommunications, and distributed control systems.
Key characteristics of Multi-Agent Systems include:
- Decentralization: Unlike traditional systems that rely on a central controller, MAS distribute control among agents, enhancing robustness and scalability.
- Cooperation: Agents can work together to achieve common goals, sharing information and resources to improve overall system performance.
- Flexibility: MAS can easily adapt to changes in the environment or system requirements, making them suitable for dynamic applications.
Applications of Multi-Agent Systems are vast:
- Traffic Management: Agents can monitor and control traffic flow, optimizing routes and reducing congestion.
- Supply Chain Management: MAS can coordinate activities among suppliers, manufacturers, and retailers to enhance efficiency.
- Smart Grids: Agents can manage energy distribution and consumption, improving reliability and sustainability.
At Rapid Innovation, we leverage MAS to help clients streamline operations and enhance decision-making processes. For instance, in supply chain management, our multi-agent systems solutions can optimize logistics, leading to reduced costs and improved delivery times, ultimately resulting in a greater return on investment (ROI). Additionally, we explore multi-agent optimization techniques to further enhance system performance.
3.3. Autonomous Decision-Making Frameworks
Autonomous Decision-Making Frameworks (ADMF) are systems designed to enable machines or agents to make decisions without human intervention. These frameworks leverage artificial intelligence (AI) and machine learning algorithms to analyze data and determine the best course of action. ADMF can process vast amounts of data quickly, allowing for real-time decision-making. They are essential in environments where rapid responses are critical, such as autonomous vehicles or financial trading systems.
Key components of Autonomous Decision-Making Frameworks include:
- Data Acquisition: Collecting relevant data from various sources, including sensors, databases, and user inputs.
- Data Processing: Analyzing the collected data using algorithms to identify patterns and insights.
- Decision Algorithms: Implementing algorithms that evaluate options and select the most appropriate action based on predefined criteria.
Benefits of Autonomous Decision-Making Frameworks include:
- Increased Efficiency: Automating decision-making processes reduces the time and resources required for human intervention.
- Improved Accuracy: Algorithms can minimize human error, leading to more reliable outcomes.
- Scalability: ADMF can be scaled to handle larger datasets and more complex decision-making scenarios.
Applications of Autonomous Decision-Making Frameworks include:
- Healthcare: AI-driven systems can assist in diagnosing diseases and recommending treatment plans.
- Finance: Automated trading systems can analyze market trends and execute trades at optimal times.
- Manufacturing: ADMF can optimize production schedules and inventory management.
At Rapid Innovation, we implement ADMF to empower businesses to make data-driven decisions swiftly. For example, in the finance sector, our automated trading solutions can analyze market conditions in real-time, enabling clients to capitalize on opportunities and enhance their ROI.
3.4. Integration with Legacy Systems
Integrating new technologies with legacy systems is a critical challenge faced by many organizations. Legacy systems are older software or hardware that may still be in use but lack the capabilities of modern solutions. Successful integration allows organizations to leverage existing investments while enhancing functionality and performance, leading to improved efficiency, reduced operational costs, and better data management.
Key considerations for integrating with legacy systems include:
- Compatibility: Ensuring that new technologies can communicate and operate with older systems without significant modifications.
- Data Migration: Transferring data from legacy systems to new platforms while maintaining data integrity and security.
- User Training: Providing training for staff to adapt to new systems while still utilizing legacy components.
Benefits of integrating with legacy systems include:
- Cost-Effectiveness: Organizations can avoid the high costs associated with completely replacing legacy systems.
- Incremental Improvement: Gradual upgrades can enhance system capabilities without disrupting ongoing operations.
- Risk Mitigation: Maintaining legacy systems while integrating new technologies reduces the risk of operational failures.
Examples of integration scenarios include:
- Enterprise Resource Planning (ERP): Integrating modern ERP solutions with existing financial or inventory management systems.
- Customer Relationship Management (CRM): Connecting new CRM tools with legacy databases to enhance customer insights.
- IoT Solutions: Incorporating IoT devices into legacy manufacturing systems to improve monitoring and control.
By addressing these integration challenges, organizations can create a more cohesive and efficient technological ecosystem. At Rapid Innovation, we specialize in seamless integration strategies that not only preserve existing investments but also enhance overall system performance, leading to improved operational efficiency and ROI. Our expertise in multi-agent systems in artificial intelligence further supports these integration efforts, ensuring that organizations can fully leverage their technological capabilities.
3.5. Scalability and Performance Considerations
Scalability and performance are critical factors in the design and implementation of any system, especially in environments that experience fluctuating workloads. When considering scalability, it is essential to ensure that the system can handle increased loads without compromising performance, particularly in the context of performance and scalability of services in cloud computing.
- Horizontal vs. Vertical Scaling:
- Horizontal scaling involves adding more machines or nodes to distribute the load, which is particularly beneficial for cloud-based solutions that Rapid Innovation can implement for clients. This approach is essential for improving net application performance and scalability.
- Vertical scaling means upgrading existing hardware to improve performance, allowing businesses to enhance their current infrastructure efficiently.
- Load Balancing:
- Distributing incoming traffic across multiple servers can enhance performance. Rapid Innovation employs load balancers to manage traffic efficiently, ensuring no single server becomes a bottleneck, thus improving user experience and overall application scalability and performance.
- Caching Mechanisms:
- Implementing caching can significantly improve response times by storing frequently accessed data in memory, reducing the need for repeated database queries. This approach can lead to faster application performance and increased customer satisfaction, addressing the need for performance scalability.
- Database Optimization:
- Proper indexing and query optimization can enhance database performance. Additionally, using read replicas can help distribute read loads, improving overall system responsiveness. Rapid Innovation's expertise in database management ensures that clients' systems operate at peak efficiency, contributing to the performance and scalability of web applications.
- Monitoring and Analytics:
- Continuous monitoring of system performance can help identify bottlenecks. Analytics tools can provide insights into usage patterns, allowing for proactive scaling. Rapid Innovation integrates advanced monitoring solutions to help clients maintain optimal performance, which is crucial for software performance and scalability.
- Cloud Solutions:
- Utilizing cloud services can offer flexible scaling options. Cloud providers often have built-in tools for auto-scaling based on demand, which Rapid Innovation leverages to provide clients with scalable and cost-effective solutions, enhancing both performance and scalability.
By focusing on scalability and performance, organizations can create systems that not only meet current demands but also adapt to future challenges. Rapid Innovation is committed to helping clients achieve these goals efficiently and effectively, ensuring a greater return on investment.
4.1.1. Payment Fraud
Payment fraud is a significant concern in the digital landscape, affecting both consumers and businesses. It involves unauthorized transactions that can lead to financial losses and a breach of trust.
- Types of payment fraud include:
- Credit card fraud: Using stolen credit card information to make purchases.
- E-commerce fraud: Scammers create fake online stores to trick consumers into providing payment details.
- Phishing scams: Fraudsters send emails or messages that appear legitimate to steal payment information.
- The impact of payment fraud can be severe:
- Financial losses for businesses and consumers.
- Increased costs for merchants due to chargebacks and fraud prevention measures.
- Damage to brand reputation and customer trust.
- Prevention strategies include:
- Implementing secure payment gateways: Rapid Innovation can assist in developing robust payment systems that utilize advanced encryption and tokenization to protect sensitive data.
- Utilizing two-factor authentication for transactions: Our AI-driven solutions can enhance security protocols, ensuring that only authorized users can complete transactions.
- Educating consumers about recognizing fraudulent activities: We offer tailored training programs that empower users to identify and report suspicious behavior, including online payment fraud detection. For more insights on enhancing digital identity and security, check out this article on AI and blockchain fusion.
4.1.2. Identity Theft
Identity theft occurs when someone unlawfully obtains and uses another person's personal information, typically for financial gain. This crime can have long-lasting effects on victims, including financial loss and emotional distress.
- Common methods of identity theft:
- Data breaches: Hackers access sensitive information from companies or organizations.
- Social engineering: Scammers manipulate individuals into revealing personal information.
- Skimming: Devices are used to capture card information during transactions.
- The consequences of identity theft can be extensive:
- Financial loss due to unauthorized transactions.
- Damage to credit scores, making it difficult to secure loans or mortgages.
- Legal issues arising from fraudulent activities conducted in the victim's name.
- Preventive measures include:
- Regularly monitoring credit reports for unusual activity: Rapid Innovation can implement AI-based monitoring systems that alert users to any suspicious changes in their credit profiles.
- Using strong, unique passwords for online accounts: We provide solutions that enforce password policies and offer password management tools to enhance security.
- Enabling alerts for transactions on financial accounts: Our blockchain technology can facilitate real-time transaction monitoring, ensuring immediate alerts for any unauthorized activities, which is crucial for payment fraud protection.
4.1.3. Account Takeover
Account takeover is a form of fraud where a criminal gains unauthorized access to a victim's online account, often leading to financial loss and personal data exposure. This type of fraud is increasingly common as more people conduct transactions online.
- How account takeover occurs:
- Credential stuffing: Attackers use stolen usernames and passwords from one site to access accounts on other platforms.
- Phishing: Victims are tricked into providing login credentials through fake websites or emails.
- Malware: Malicious software is used to capture login information.
- The repercussions of account takeover can be significant:
- Unauthorized transactions can drain bank accounts or deplete credit lines.
- Personal information may be exposed, leading to further identity theft.
- Victims may face challenges in regaining control of their accounts.
- To protect against account takeover:
- Use multi-factor authentication to add an extra layer of security: Rapid Innovation can integrate advanced authentication methods into your systems, significantly reducing the risk of unauthorized access.
- Regularly update passwords and avoid reusing them across different sites: Our solutions can automate password updates and enforce best practices for password management.
- Be cautious of suspicious emails or messages requesting personal information: We provide AI-driven tools that analyze communication patterns to detect and flag potential phishing attempts, enhancing overall security, which is essential for effective fraud prevention payments.
4.2. Money Laundering Detection
Money laundering is the process of making illegally obtained money appear legitimate. Detecting money laundering is crucial for financial institutions and regulatory bodies to maintain the integrity of the financial system. Rapid Innovation leverages advanced AI and blockchain technologies to enhance the detection and prevention of money laundering, ensuring compliance and safeguarding financial integrity.
- Know Your Customer (KYC): Financial institutions must implement robust KYC procedures to verify the identity of their clients. Rapid Innovation can develop AI-driven KYC solutions that automate the collection of personal information, assess the nature of businesses, and continuously monitor transactions for suspicious activity, thereby improving efficiency and accuracy.
- Transaction Monitoring: Continuous monitoring of transactions is essential. Our automated systems utilize machine learning algorithms to flag unusual patterns, such as large cash deposits or rapid movement of funds between accounts, allowing for timely intervention and reducing the risk of financial crime. This includes the use of money laundering detection methods to identify suspicious transactions.
- Red Flags: Certain behaviors can indicate potential money laundering, including unexplained wealth or sudden changes in financial status, frequent large transactions that do not match the customer’s profile, and the use of shell companies or complex corporate structures. Rapid Innovation's AI models can analyze historical data to identify these red flags more effectively, including those related to aml fraud detection.
- Reporting Obligations: Financial institutions are required to report suspicious activities to relevant authorities, such as the Financial Crimes Enforcement Network (FinCEN) in the U.S. Our solutions streamline the process of filing Suspicious Activity Reports (SARs), ensuring compliance and reducing the administrative burden on financial institutions. This is particularly important in the context of bsa aml detection.
- Collaboration with Law Enforcement: Effective money laundering detection often involves collaboration between financial institutions and law enforcement agencies. Rapid Innovation facilitates secure information sharing through blockchain technology, enhancing the ability to identify and prosecute offenders. This collaboration is essential for effective aml fraud prevention.
4.3. Terrorist Financing
Terrorist financing refers to the process of providing financial support to individuals or groups engaged in terrorism. Detecting and preventing terrorist financing is critical for national security. Rapid Innovation employs AI and blockchain solutions to enhance the detection capabilities of financial institutions.
- Understanding the Sources: Terrorist financing can come from various sources, including charitable donations, criminal activities such as drug trafficking, and state sponsorship. Our AI systems can analyze transaction data to identify potential sources of funding.
- Risk Assessment: Financial institutions must conduct risk assessments to identify potential vulnerabilities in their systems. Rapid Innovation provides AI-driven risk assessment tools that evaluate customer profiles and transaction types associated with terrorist financing.
- Enhanced Due Diligence (EDD): For high-risk customers or transactions, enhanced due diligence is necessary. Our solutions enable deeper investigations into the source of funds and the purpose of transactions, ensuring compliance with regulatory requirements.
- Monitoring and Reporting: Similar to money laundering, financial institutions must monitor transactions for signs of terrorist financing. Rapid Innovation's AI systems can detect unusual patterns of donations or transfers, transactions involving high-risk jurisdictions, and the use of cash-intensive businesses.
- International Cooperation: Terrorist financing is a global issue that requires international cooperation. Rapid Innovation supports organizations like the Financial Action Task Force (FATF) by providing tools that promote effective measures to combat terrorist financing.
4.4. Market Manipulation
Market manipulation involves artificially inflating or deflating the price of a security to create a false or misleading appearance of market activity. Detecting market manipulation is essential for maintaining fair and efficient markets. Rapid Innovation's AI and blockchain solutions play a pivotal role in enhancing market integrity.
- Types of Market Manipulation: Common forms include pump and dump schemes, spoofing, and wash trading. Our AI algorithms are designed to detect these manipulative practices by analyzing trading patterns and identifying anomalies in real-time.
- Surveillance Systems: Financial markets employ sophisticated surveillance systems to detect unusual trading patterns. Rapid Innovation develops advanced surveillance solutions that analyze vast amounts of data to identify potential manipulation, ensuring market fairness.
- Regulatory Oversight: Regulatory bodies, such as the Securities and Exchange Commission (SEC) in the U.S., monitor trading activities and investigate suspicious behavior. Our solutions assist regulatory agencies by providing them with the tools needed to effectively monitor and enforce compliance.
- Whistleblower Programs: Encouraging whistleblowers to report suspicious activities can be an effective tool in detecting market manipulation. Rapid Innovation supports the development of secure reporting platforms that protect whistleblower anonymity while facilitating the reporting process.
- Education and Awareness: Educating investors about the signs of market manipulation can help them avoid falling victim to fraudulent schemes. Rapid Innovation can assist in creating awareness campaigns that empower investors to report suspicious activities to authorities, ultimately contributing to a more transparent market environment.
By integrating AI and blockchain technologies, Rapid Innovation helps clients achieve greater ROI through enhanced compliance, reduced risk, and improved operational efficiency in the fight against financial crime, including the detection of money laundering and the implementation of money laundering detection techniques.
4.5. Insider Trading
Insider trading refers to the buying or selling of publicly-traded securities based on material, non-public information about the company. This practice is illegal and undermines investor confidence in the fairness and integrity of the securities markets.
- Insider trading can occur when corporate executives, employees, or other insiders have access to confidential information that could influence an investor's decision.
- The Securities and Exchange Commission (SEC) actively monitors trading patterns to detect suspicious activities that may indicate insider trading, including sec insider information and sec insider trades.
- Penalties for insider trading can be severe, including hefty fines and imprisonment. For instance, individuals found guilty of insider trading can face fines up to three times the profit gained or loss avoided, as outlined in sec insider trading rules.
- The impact of insider trading extends beyond legal repercussions; it can lead to a loss of trust among investors and can negatively affect a company's stock price, which is why insider trading regulations are crucial.
- Companies often implement strict internal policies to prevent insider trading, including blackout periods and mandatory reporting of trades by insiders, in accordance with insider trading rules for employees.
4.6. Emerging Financial Crimes
Emerging financial crimes encompass a range of illicit activities that exploit advancements in technology and changes in the financial landscape. These crimes are becoming increasingly sophisticated and pose significant risks to individuals and organizations.
- Cybercrime: With the rise of digital banking and online transactions, cybercriminals are targeting financial institutions and consumers through phishing, ransomware, and identity theft.
- Money laundering: Criminals use complex schemes to disguise the origins of illegally obtained money, making it appear legitimate. This often involves multiple transactions across various jurisdictions.
- Fraud schemes: New types of fraud, such as investment scams and Ponzi schemes, are emerging as criminals leverage social media and online platforms to reach potential victims.
- Regulatory challenges: As financial crimes evolve, regulatory bodies are struggling to keep pace, leading to gaps in enforcement and oversight.
- Prevention measures: Organizations are increasingly investing in advanced technologies, such as artificial intelligence and machine learning, to detect and prevent financial crimes before they occur. Rapid Innovation specializes in implementing AI-driven solutions that enhance fraud detection capabilities, allowing organizations to identify suspicious activities in real-time and significantly reduce their risk exposure.
5. Implementation Framework
An effective implementation framework is crucial for organizations to combat insider trading and emerging financial crimes. This framework outlines the strategies, policies, and procedures necessary to mitigate risks and ensure compliance with regulations.
- Risk assessment: Organizations should conduct regular risk assessments to identify vulnerabilities related to insider trading and financial crimes. This includes evaluating internal controls and monitoring trading activities, particularly focusing on sec insider transactions and insider dealing.
- Training and awareness: Employees must be educated about the legal implications of insider trading and the signs of financial crimes. Regular training sessions can help foster a culture of compliance.
- Reporting mechanisms: Establishing clear reporting channels for employees to report suspicious activities is essential. Whistleblower protections can encourage individuals to come forward without fear of retaliation.
- Technology integration: Leveraging technology, such as data analytics and surveillance systems, can enhance monitoring capabilities and improve the detection of unusual trading patterns or fraudulent activities. Rapid Innovation offers tailored solutions that integrate blockchain technology to ensure transparency and traceability in financial transactions, further mitigating risks associated with financial crimes. Additionally, organizations can explore the intersection of artificial intelligence with margin trading exchanges to enhance their strategies.
- Collaboration with authorities: Organizations should work closely with regulatory bodies, such as the CFTC and FINRA, and law enforcement to share information and best practices in combating financial crimes. This collaboration can lead to more effective enforcement and deterrence strategies.
5.1. Data Collection and Preparation
Data collection and preparation are critical steps in any data-driven project. This phase involves gathering relevant data, cleaning it, and organizing it for analysis. Proper data collection and preparation ensure that the insights derived from the data are accurate and actionable, ultimately enabling businesses to achieve their goals efficiently and effectively. This includes preparation for data collection, data collection and preparation, and any process of preparing and collecting data.
- Importance of data collection:
- Provides the foundation for analysis.
- Influences the quality of insights.
- Affects decision-making processes.
- Key steps in data preparation:
- Data cleaning: Removing inaccuracies and inconsistencies to ensure the integrity of the data.
- Data transformation: Converting data into a suitable format that aligns with analytical requirements.
- Data integration: Combining data from different sources to create a comprehensive dataset for analysis, which is part of the process of preparing and collecting data.
5.1.1. Data Sources
Identifying the right data sources is essential for effective data collection. Data can be sourced from various channels, each offering unique insights and information that can drive business strategies.
- Types of data sources:
- Primary data: Collected directly from original sources through surveys, interviews, or experiments, providing firsthand insights.
- Secondary data: Obtained from existing sources such as reports, databases, or online repositories, which can supplement primary data.
- Open data: Publicly available datasets provided by governments or organizations, offering valuable information for analysis.
- Considerations for selecting data sources:
- Relevance: Ensure the data aligns with the research objectives to maximize its utility.
- Reliability: Choose sources that are credible and trustworthy to enhance the quality of insights.
- Timeliness: Use up-to-date data to reflect current trends and conditions, ensuring that decisions are based on the latest information.
5.1.2. Data Quality Management
Data quality management is crucial for ensuring that the data used in analysis is accurate, complete, and reliable. Poor data quality can lead to misleading conclusions and ineffective strategies, ultimately impacting ROI.
- Key components of data quality management:
- Data accuracy: Ensuring data is correct and free from errors, which is vital for trustworthy analysis.
- Data completeness: Verifying that all necessary data is present to support comprehensive insights.
- Data consistency: Maintaining uniformity across datasets to avoid discrepancies that could skew results.
- Strategies for improving data quality:
- Regular audits: Conduct periodic checks to identify and rectify data issues, ensuring ongoing data integrity.
- Data validation: Implement checks to ensure data meets predefined standards, enhancing reliability.
- Training: Educate team members on best practices for data entry and management, fostering a culture of data quality.
- Benefits of effective data quality management:
- Enhanced decision-making: Reliable data leads to better insights, empowering organizations to make informed choices.
- Increased efficiency: Reduces time spent on correcting data errors, allowing teams to focus on strategic initiatives.
- Improved trust: Builds confidence in data-driven initiatives, which is essential for fostering a data-centric culture within organizations.
At Rapid Innovation, we leverage our expertise in AI and Blockchain to streamline data gathering and preparation processes, ensuring that our clients can harness the full potential of their data. By implementing robust data quality management practices, we help organizations achieve greater ROI through informed decision-making and strategic insights.
5.1.3. Data Normalization
Data normalization is a crucial step in the data preprocessing phase of machine learning and data analysis. It involves adjusting the values in a dataset to a common scale without distorting differences in the ranges of values. This process is essential for ensuring that the model can learn effectively from the data.
- Ensures that each feature contributes equally to the distance calculations in algorithms like k-nearest neighbors (KNN) and support vector machines (SVM).
- Helps in speeding up the convergence of gradient descent algorithms, which are commonly used in training neural networks.
- Reduces the risk of numerical instability, which can occur when dealing with very large or very small numbers.
Common methods of data normalization include:
- Min-Max Scaling: Rescales the feature to a fixed range, usually [0, 1]. This is a common method in min max normalization.
- Z-score Normalization: Centers the data around the mean with a standard deviation of 1.
- Robust Scaling: Uses the median and the interquartile range, making it robust to outliers.
Implementing data normalization can significantly improve the performance of machine learning models, making it a vital step in the data preparation process. At Rapid Innovation, we leverage data normalization techniques, including data normalization methods and data normalization in machine learning, to enhance the accuracy and efficiency of our clients' AI models, ultimately leading to greater ROI.
5.2. Model Development
Model development is the phase where the actual machine learning model is created and trained using the prepared dataset. This process involves selecting the appropriate algorithms, tuning hyperparameters, and validating the model's performance.
- Selection of Algorithms: Choosing the right algorithm is critical. Options include decision trees, random forests, neural networks, and support vector machines, among others.
- Hyperparameter Tuning: Adjusting the parameters that govern the training process can lead to better model performance. Techniques like grid search and random search are commonly used.
- Model Validation: It is essential to evaluate the model's performance using techniques such as cross-validation, which helps in assessing how the model will generalize to unseen data.
The model development phase is iterative, often requiring multiple rounds of training and evaluation to achieve optimal results. The ultimate goal is to create a model that not only performs well on the training data but also generalizes effectively to new, unseen data. Rapid Innovation employs a systematic approach to model development, ensuring that our clients receive robust and scalable AI solutions that align with their business objectives.
5.2.1. Feature Engineering
Feature engineering is the process of using domain knowledge to create new input features from existing ones, enhancing the predictive power of machine learning algorithms. This step is vital as the quality of features directly impacts the model's performance.
- Identifying Relevant Features: Understanding which features are most relevant to the target variable can significantly improve model accuracy.
- Creating New Features: This can involve combining existing features, transforming them, or extracting new ones from raw data. For example, creating interaction terms or polynomial features can capture non-linear relationships.
- Feature Selection: Not all features contribute equally to the model's performance. Techniques like recursive feature elimination and feature importance scores can help in selecting the most impactful features.
Effective feature engineering can lead to improved model accuracy and performance, reduced overfitting by eliminating irrelevant features, and enhanced interpretability of the model, making it easier to understand the relationships between features and the target variable. At Rapid Innovation, we emphasize the importance of feature engineering in our AI projects, ensuring that our clients benefit from models that are not only accurate but also interpretable and actionable.
In summary, feature engineering is a critical component of the machine learning pipeline, requiring creativity and a deep understanding of the data and the problem domain. Data normalization techniques, such as data cleaning and normalization, also play a significant role in this process, ensuring that the data is prepared effectively for analysis.
5.2.2. Model Selection
Model selection is a critical step in the machine learning process, as it determines the algorithm that will be used to make predictions or classifications based on the data. The choice of model can significantly impact the performance and accuracy of the results.
- Understand the problem type: Classification, regression, or clustering? Different models are suited for different tasks, and Rapid Innovation can assist in identifying the most appropriate model for your specific business needs.
- Consider the data characteristics:
- Size of the dataset: Some models perform better with large datasets, while others are more effective with smaller ones. Our expertise ensures that you leverage the right model based on your data volume.
- Feature types: Categorical, numerical, or text data may require different models. We help clients analyze their data features to select the optimal approach.
- Evaluate model complexity:
- Simpler models (e.g., linear regression) are easier to interpret but may underfit complex data. Rapid Innovation can guide you in choosing a model that balances interpretability and performance.
- Complex models (e.g., deep learning) can capture intricate patterns but may overfit if not managed properly. Our team employs best practices to mitigate overfitting risks.
- Use cross-validation: This helps in assessing how the model generalizes to an independent dataset. Techniques like k-fold cross-validation can provide a more reliable estimate of model performance, ensuring that your investment yields maximum returns.
- Compare multiple models: Implement various algorithms (e.g., decision trees, support vector machines, neural networks) and compare their performance using metrics like accuracy, precision, recall, and F1 score. Rapid Innovation's analytical capabilities enable clients to make data-driven decisions for enhanced ROI.
5.2.3. Training Methodologies
Training methodologies refer to the strategies and techniques used to train machine learning models. The choice of methodology can influence the model's ability to learn from data effectively.
- Supervised learning: Involves training a model on labeled data, where the input-output pairs are known. Common algorithms include linear regression, logistic regression, and support vector machines. Our team ensures that the right supervised learning techniques are applied to meet your business objectives.
- Unsupervised learning: Used when the data is unlabeled, focusing on finding patterns or groupings within the data. Techniques include clustering (e.g., K-means) and dimensionality reduction (e.g., PCA). Rapid Innovation can help uncover valuable insights from your data through effective unsupervised learning strategies.
- Reinforcement learning: Involves training models through trial and error, where an agent learns to make decisions by receiving rewards or penalties. This approach is commonly used in robotics and game playing, and we can tailor reinforcement learning solutions to optimize your operational processes.
- Batch vs. online learning: Batch learning trains the model on the entire dataset at once, while online learning updates the model incrementally as new data arrives. Online learning is beneficial for real-time applications where data is continuously generated, and our expertise ensures that your systems remain agile and responsive.
- Hyperparameter tuning: Involves adjusting the parameters that govern the training process to optimize model performance. Techniques like grid search and random search can be employed to find the best hyperparameters, maximizing the effectiveness of your machine learning initiatives, including automl model selection.
5.3. Testing and Validation
Testing and validation are essential steps in the machine learning lifecycle, ensuring that the model performs well on unseen data and generalizes effectively.
- Split the dataset: Divide the data into training, validation, and test sets to evaluate model performance. A common split is 70% training, 15% validation, and 15% testing. Rapid Innovation employs rigorous testing protocols to ensure robust model performance.
- Use performance metrics: Evaluate the model using various metrics depending on the problem type:
- Classification: Accuracy, precision, recall, F1 score, ROC-AUC.
- Regression: Mean squared error (MSE), mean absolute error (MAE), R-squared. Our team helps clients interpret these metrics to gauge the success of their machine learning projects, including model evaluation model selection and algorithm selection in machine learning.
- Conduct cross-validation: Implement k-fold cross-validation to ensure that the model's performance is consistent across different subsets of the data. This helps in identifying overfitting or underfitting issues, allowing for timely adjustments.
- Analyze results: Examine confusion matrices for classification tasks to understand misclassifications. For regression tasks, visualize predicted vs. actual values to assess model accuracy. Rapid Innovation provides comprehensive analysis to enhance decision-making.
- Model robustness: Test the model against various scenarios, including edge cases and noisy data, to ensure reliability. Consider using techniques like adversarial testing to evaluate model performance under challenging conditions, ensuring that your solutions are resilient.
- Continuous monitoring: After deployment, continuously monitor the model's performance to detect any degradation over time. Implement retraining strategies to update the model with new data as it becomes available. Our commitment to ongoing support ensures that your AI solutions remain effective and aligned with your business goals, including choosing a machine learning model and selecting the best machine learning algorithm for your regression problem.
5.4. Deployment Strategies
Deployment strategies are critical for ensuring that software applications are released efficiently and effectively. The choice of deployment strategy can significantly impact the performance, reliability, and user experience of the application. Here are some common deployment strategies:
- Blue-Green Deployment: This strategy involves maintaining two identical environments, one active (blue) and one idle (green). New versions of the application are deployed to the green environment. Once testing is complete, traffic is switched from blue to green, minimizing downtime and risk. Rapid Innovation leverages this strategy to ensure seamless transitions for clients, enhancing user satisfaction and reducing operational disruptions.
- Canary Releases: In this approach, a new version of the application is rolled out to a small subset of users before a full-scale deployment. This allows for monitoring of the new version's performance and user feedback, reducing the risk of widespread issues. By implementing canary releases, Rapid Innovation helps clients gather valuable insights early, enabling data-driven decisions that lead to improved ROI.
- Rolling Deployment: This strategy involves gradually replacing instances of the previous version of the application with the new version. This method allows for continuous availability and minimizes downtime, as only a portion of the application is updated at any given time. Rapid Innovation utilizes rolling deployments to ensure that clients maintain service continuity while enhancing their applications.
- Recreate Deployment: In this method, the existing application is completely shut down before the new version is deployed. While this is straightforward, it can lead to significant downtime, making it less favorable for applications requiring high availability. Rapid Innovation advises clients on the potential risks of this approach, ensuring they choose strategies that align with their business needs.
- Shadow Deployment: This strategy involves deploying the new version alongside the existing version without exposing it to users. It allows for testing in a production-like environment, gathering performance data without impacting the user experience. Rapid Innovation employs shadow deployments to help clients validate new features and performance enhancements before full-scale rollout.
Choosing the right deployment strategy depends on various factors, including application architecture, user base, and business requirements. Each strategy has its pros and cons, and understanding these can help teams make informed decisions. Rapid Innovation's expertise in deployment strategies, including hoshin strategy deployment and lean deployment strategy, ensures that clients achieve optimal performance and reliability, ultimately driving greater ROI.
Additionally, organizations may consider types of deployment strategies such as application deployment strategies and API deployment strategy to align with their specific needs. The use of strategy deployment software can also facilitate the implementation of these methodologies. Rapid Innovation's knowledge of policy strategy and goal deployment, as well as strategic planning and deployment, further enhances their ability to support clients in achieving their objectives.
5.5. Performance Monitoring
Performance monitoring is essential for maintaining the health and efficiency of applications. It involves tracking various metrics to ensure that applications run smoothly and meet user expectations. Key aspects of performance monitoring include:
- Real-Time Monitoring: Continuous tracking of application performance metrics such as response time, error rates, and server load. This helps in identifying issues as they arise.
- User Experience Monitoring: Tools that measure how users interact with the application, including page load times and transaction times. Understanding user experience is crucial for optimizing performance.
- Infrastructure Monitoring: Monitoring the underlying infrastructure, including servers, databases, and network components. This ensures that all parts of the application stack are functioning optimally.
- Log Management: Collecting and analyzing logs from various components of the application. Logs can provide insights into errors, performance bottlenecks, and user behavior.
- Alerts and Notifications: Setting up alerts for specific performance thresholds. This allows teams to respond quickly to potential issues before they escalate.
- Performance Testing: Regularly conducting performance tests to identify bottlenecks and areas for improvement. This can include load testing, stress testing, and scalability testing.
Effective performance monitoring not only helps in maintaining application health but also enhances user satisfaction by ensuring a seamless experience. Rapid Innovation integrates advanced monitoring solutions to provide clients with actionable insights, enabling them to optimize their applications and achieve higher returns on investment.
6. Risk Assessment and Management
Risk assessment and management are vital components of any project, particularly in software development. Identifying potential risks early allows teams to mitigate them effectively. Here are key elements of risk assessment and management:
- Risk Identification: The first step involves identifying potential risks that could impact the project. This can include technical risks, operational risks, and external risks such as regulatory changes.
- Risk Analysis: Once risks are identified, they need to be analyzed to determine their potential impact and likelihood. This can involve qualitative and quantitative methods to prioritize risks based on their severity.
- Risk Mitigation Strategies: Developing strategies to minimize the impact of identified risks can include implementing additional testing, adopting new technologies, or adjusting project timelines.
- Risk Monitoring: Continuously monitoring identified risks throughout the project lifecycle ensures that any changes in risk status are promptly addressed.
- Communication: Keeping all stakeholders informed about potential risks and mitigation strategies fosters a culture of transparency and collaboration.
- Documentation: Maintaining thorough documentation of identified risks, analysis, and mitigation strategies serves as a reference for future projects and helps in learning from past experiences.
By implementing a robust risk assessment and management process, organizations can enhance project success rates and reduce the likelihood of unforeseen issues derailing their efforts. Rapid Innovation's expertise in risk management ensures that clients are well-prepared to navigate challenges, ultimately leading to successful project outcomes and improved ROI.
6.1. Risk Scoring Models
Risk scoring models are essential tools used in various industries, particularly in finance, healthcare, and cybersecurity, to assess the potential risk associated with specific entities or actions. These models utilize quantitative and qualitative data to generate a score that reflects the level of risk.
- Risk scoring models often incorporate:
- Historical data analysis to identify patterns and trends.
- Machine learning algorithms to improve accuracy over time.
- Industry-specific metrics that are relevant to the risk being assessed.
- Common applications include:
- Credit scoring in finance to evaluate loan applicants using different credit score models.
- Patient risk assessment in healthcare to prioritize treatment.
- Threat detection in cybersecurity to identify potential breaches.
- Benefits of risk scoring models:
- Enhanced decision-making through data-driven insights.
- Improved resource allocation by focusing on high-risk areas.
- Increased efficiency in risk management processes.
At Rapid Innovation, we leverage advanced AI techniques to develop customized risk scoring models tailored to your specific industry needs. By integrating machine learning algorithms, we ensure that your risk assessments become more accurate over time, ultimately leading to greater ROI through informed decision-making. Our expertise includes developing credit scoring models and credit scorecards, such as the credit scorecard example, to enhance financial assessments.
6.2. Alert Generation and Prioritization
Alert generation and prioritization are critical components of risk management systems, particularly in environments where timely responses to threats are essential. This process involves creating alerts based on predefined criteria and prioritizing them based on their potential impact.
- Key elements of alert generation include:
- Real-time monitoring of systems and data to detect anomalies.
- Use of automated tools to generate alerts based on specific triggers.
- Customizable alert settings to align with organizational needs.
- Prioritization of alerts is crucial for effective response:
- Alerts are categorized based on severity, urgency, and potential impact.
- High-priority alerts are escalated for immediate attention, while lower-priority alerts may be reviewed later.
- This approach helps organizations focus their resources on the most critical issues.
- Benefits of effective alert generation and prioritization:
- Reduced response times to potential threats.
- Minimized risk of overlooking significant issues.
- Enhanced overall security posture through proactive management.
Rapid Innovation employs cutting-edge AI technologies to enhance your alert generation and prioritization processes. By automating these systems, we help you reduce response times and improve your overall security posture, ensuring that your organization can focus on what matters most.
6.3. Case Management
Case management refers to the systematic approach to managing incidents or risks once they have been identified. This process ensures that all necessary steps are taken to address the issue effectively and efficiently.
- Components of case management include:
- Documentation of incidents, including details of the event and actions taken.
- Assignment of responsibilities to team members for follow-up and resolution.
- Tracking progress and outcomes to ensure accountability.
- Effective case management involves:
- Utilizing case management software to streamline processes and improve collaboration.
- Regularly reviewing cases to identify trends and areas for improvement.
- Engaging stakeholders to ensure comprehensive resolution strategies.
- Benefits of robust case management:
- Improved incident resolution times through organized processes.
- Enhanced communication among team members and stakeholders.
- Better insights into risk management practices, leading to continuous improvement.
At Rapid Innovation, we understand the importance of effective case management in mitigating risks. Our tailored solutions integrate AI-driven insights and blockchain technology to ensure transparency and accountability throughout the case management process, ultimately leading to improved outcomes and greater ROI for your organization. We also consider factors such as bankruptcy risk score and fico risk score classic 04 in our assessments to provide a comprehensive view of financial risks.
6.4. Investigation Workflows
Investigation workflows are essential for organizations to effectively manage and respond to incidents, particularly in sectors like finance, healthcare, and cybersecurity. These workflows provide a structured approach to identifying, analyzing, and resolving issues.
- Definition and Purpose: Investigation workflows outline the steps taken during an investigation, ensuring that all necessary actions are documented and executed systematically. This helps in maintaining accountability and transparency.
- Key Components:
- Incident Identification: Recognizing potential issues through alerts, reports, or monitoring systems.
- Data Collection: Gathering relevant information, including logs, emails, and other documentation.
- Analysis: Evaluating the collected data to determine the nature and scope of the incident.
- Resolution: Implementing corrective actions to address the identified issues.
- Documentation: Keeping detailed records of the investigation process for future reference and compliance.
- Best Practices:
- Standardization: Develop standardized procedures to ensure consistency across investigations.
- Training: Regularly train staff on investigation protocols and tools.
- Collaboration: Foster communication between departments to enhance information sharing and problem-solving.
- Technology Integration: Utilize software tools to automate parts of the investigation process, such as data collection and reporting, which can improve efficiency and accuracy. Rapid Innovation leverages AI-driven analytics to enhance data analysis and incident resolution, ensuring that organizations can respond swiftly and effectively to incidents, ultimately leading to greater ROI. Investigation workflows play a crucial role in this process, providing a clear framework for action.
6.5. Regulatory Reporting
Regulatory reporting is a critical aspect of compliance for organizations operating in regulated industries. It involves submitting required information to regulatory bodies to demonstrate adherence to laws and regulations.
- Importance of Regulatory Reporting:
- Transparency and Accountability: Ensures that organizations operate openly and responsibly.
- Avoiding Penalties: Helps organizations avoid penalties and legal issues.
- Building Trust: Builds trust with stakeholders, including customers and investors.
- Types of Reports:
- Financial Reports: Required by financial regulatory bodies to ensure accurate reporting of financial health.
- Compliance Reports: Document adherence to specific regulations, such as data protection laws.
- Incident Reports: Detail any breaches or incidents that may affect compliance status, often linked to investigation workflows.
- Challenges:
- Complexity: Navigating various regulations can be daunting, especially for multinational organizations.
- Data Accuracy: Ensuring that all reported data is accurate and up-to-date is crucial.
- Timeliness: Meeting reporting deadlines is essential to avoid penalties.
- Best Practices:
- Regular Audits: Conduct internal audits to ensure compliance and identify areas for improvement.
- Automated Reporting Tools: Implement software solutions to streamline the reporting process and reduce errors. Rapid Innovation offers blockchain-based solutions that enhance data integrity and traceability, ensuring that reports are accurate and compliant.
- Training and Awareness: Educate employees on regulatory requirements and the importance of accurate reporting.
7. Compliance and Regulatory Considerations
Compliance and regulatory considerations are vital for organizations to operate legally and ethically. Understanding these aspects helps mitigate risks and fosters a culture of accountability.
- Understanding Compliance: Compliance refers to adhering to laws, regulations, and internal policies. It encompasses various areas, including financial reporting, data protection, and workplace safety.
- Regulatory Frameworks:
- Industry-Specific Regulations: Different industries have unique regulations, such as HIPAA for healthcare or GDPR for data protection.
- International Standards: Organizations operating globally must be aware of international regulations that may impact their operations.
- Risk Management:
- Identifying Risks: Regularly assess potential compliance risks within the organization.
- Mitigation Strategies: Develop strategies to address identified risks, such as implementing new policies or training programs.
- Monitoring and Enforcement:
- Internal Controls: Establish robust internal controls to monitor compliance and detect violations.
- Reporting Mechanisms: Create channels for employees to report compliance concerns without fear of retaliation.
- Continuous Improvement:
- Feedback Loops: Regularly review compliance processes and incorporate feedback for improvement.
- Stay Informed: Keep abreast of changes in regulations and adjust policies accordingly.
- Engagement with Regulators: Maintain open lines of communication with regulatory bodies to ensure understanding and compliance with evolving regulations. Rapid Innovation can assist organizations in navigating these complexities through tailored consulting services, ensuring that compliance is not just a requirement but a strategic advantage. Investigation workflows can also support compliance efforts by providing a clear process for addressing incidents and ensuring proper documentation.
7.1. AML Compliance
Anti-Money Laundering (AML) compliance is a critical aspect of financial institutions and businesses that deal with monetary transactions. It involves a set of laws, regulations, and procedures designed to prevent, detect, and report money laundering activities.
- AML compliance programs must be tailored to the specific risks associated with the business.
- Key components of an effective AML program include:
- Risk assessment: Identifying and assessing the risks of money laundering specific to the organization.
- Customer due diligence (CDD): Verifying the identity of customers and understanding their financial activities.
- Transaction monitoring: Continuously monitoring transactions for suspicious activities.
- Reporting: Filing Suspicious Activity Reports (SARs) when suspicious transactions are detected.
- Training and awareness: Regular training for employees on AML regulations and procedures is essential.
- Technology integration: Utilizing AML compliance software and other software solutions to enhance monitoring and reporting capabilities.
At Rapid Innovation, we leverage advanced AI algorithms to enhance transaction monitoring and risk assessment processes, enabling organizations to detect suspicious activities in real-time. Our blockchain solutions can also provide immutable records of transactions, ensuring transparency and accountability, which are crucial for AML compliance. Organizations must stay updated with the latest AML regulations and guidelines from authorities such as the Financial Action Task Force (FATF) and local regulatory bodies. Non-compliance can lead to severe penalties, including fines and reputational damage. Additionally, our AI-driven solutions for KYC verification enhance the customer due diligence process, further supporting AML compliance efforts.
7.2. KYC Integration
Know Your Customer (KYC) integration is a fundamental part of AML compliance. It involves verifying the identity of clients and assessing their suitability for the services offered.
- KYC processes help in:
- Preventing fraud: By ensuring that the identity of customers is verified, businesses can reduce the risk of fraudulent activities.
- Enhancing customer trust: A robust KYC compliance software builds trust between the business and its customers.
- Regulatory compliance: KYC is a legal requirement in many jurisdictions, ensuring that businesses adhere to AML laws.
- Key elements of KYC integration include:
- Customer identification: Collecting and verifying personal information such as name, address, and date of birth.
- Risk assessment: Evaluating the risk level associated with each customer based on their profile and transaction history.
- Ongoing monitoring: Continuously reviewing customer transactions to identify any unusual patterns or behaviors.
At Rapid Innovation, we implement AI-driven KYC AML solutions that automate the customer identification process, significantly reducing onboarding time while enhancing accuracy. Our technology not only ensures compliance but also improves overall customer experience by making onboarding smoother and faster.
7.3. Regulatory Reporting Requirements
Regulatory reporting requirements are essential for maintaining transparency and accountability in financial transactions. These requirements vary by jurisdiction but generally involve the submission of specific reports to regulatory authorities.
- Key aspects of regulatory reporting include:
- Timeliness: Reports must be submitted within specified timeframes to ensure compliance.
- Accuracy: Information provided in reports must be accurate and complete to avoid penalties.
- Types of reports: Common reports include:
- Suspicious Activity Reports (SARs): Filed when suspicious transactions are detected.
- Currency Transaction Reports (CTRs): Required for transactions exceeding a certain threshold.
Organizations must have robust systems in place to track and report relevant data efficiently. Rapid Innovation offers AML screening software and blockchain-based solutions that ensure data integrity and facilitate seamless regulatory reporting. Regular audits and reviews of reporting processes can help identify areas for improvement and ensure compliance with evolving regulations. Staying informed about changes in regulatory requirements is crucial for businesses to avoid non-compliance and potential legal repercussions.
7.4. Model Governance
Model governance refers to the framework and processes that ensure the effective management, oversight, and accountability of models used within an organization. This is particularly crucial in industries such as finance, healthcare, and insurance, where models can significantly impact decision-making and regulatory compliance.
- Establishing clear ownership and accountability for each model.
- Implementing a structured model development lifecycle, including design, validation, and deployment.
- Regularly reviewing and updating models to reflect changes in data, regulations, or business objectives.
- Ensuring compliance with relevant regulations and standards, such as the Basel III framework for financial institutions.
- Utilizing a centralized repository for model documentation, making it easier to track changes and maintain version control.
- Engaging stakeholders from various departments, including risk management, compliance, and IT, to ensure a holistic approach to model governance, including the three lines of defense risk management framework.
- Conducting regular audits and assessments to evaluate model performance and effectiveness.
Effective model governance not only mitigates risks but also enhances the credibility of the models used, fostering trust among stakeholders. At Rapid Innovation, we assist organizations in establishing robust model governance frameworks, such as the data governance model and the carver governance model, that align with their strategic objectives, ensuring that they can leverage AI and blockchain technologies effectively while maintaining compliance and accountability.
7.5. Audit Trails and Documentation
Audit trails and documentation are essential components of model governance, providing transparency and accountability in the model development and deployment process. They serve as a record of all actions taken on a model, ensuring that organizations can track changes and understand the rationale behind decisions.
- Maintaining comprehensive documentation for each model, including model assumptions and methodologies, data sources and preprocessing steps, and validation results and performance metrics.
- Implementing audit trails that log changes made to the model, including who made the changes and when, as well as version history to track the evolution of the model over time.
- Documenting decisions made during the model development process, providing context for stakeholders.
- Ensuring that documentation is easily accessible to relevant parties, facilitating collaboration and knowledge sharing.
- Regularly reviewing and updating documentation to reflect any changes in the model or its environment.
- Utilizing automated tools to streamline the documentation process and ensure consistency.
Having robust audit trails and documentation not only supports compliance with regulatory requirements but also enhances the organization's ability to learn from past experiences and improve future model development efforts. Rapid Innovation provides tailored solutions to implement effective audit trails and documentation practices, ensuring that our clients can maintain transparency and accountability in their AI and blockchain initiatives.
8. Advanced Features and Capabilities
Advanced features and capabilities in model governance and management can significantly enhance an organization's ability to leverage data-driven insights effectively. These features often include sophisticated tools and methodologies that streamline processes and improve decision-making.
- Integration of machine learning and artificial intelligence to automate model development and validation processes.
- Real-time monitoring of model performance, allowing for immediate adjustments based on changing conditions.
- Advanced analytics capabilities, enabling organizations to derive deeper insights from their data.
- User-friendly dashboards and visualization tools that make it easier for stakeholders to understand model outputs and performance metrics.
- Enhanced collaboration features that allow cross-functional teams to work together seamlessly on model development and governance, including the business governance model and IT governance model.
- Support for various modeling techniques, including traditional statistical methods and modern machine learning approaches, as well as the model risk governance framework.
- Scalability to accommodate growing data volumes and complexity, ensuring that the organization can adapt to changing business needs.
By leveraging these advanced features and capabilities, organizations can improve their model governance practices, enhance operational efficiency, and drive better business outcomes. Rapid Innovation is committed to empowering our clients with cutting-edge AI and blockchain solutions that not only optimize their model governance but also maximize their return on investment.
8.1. Behavioral Analytics
Behavioral analytics involves the collection and analysis of data regarding user behavior to identify patterns and trends. This approach is crucial for businesses aiming to enhance customer experience and optimize marketing strategies.
- Helps in understanding customer preferences and habits.
- Enables personalized marketing efforts, leading to higher conversion rates.
- Assists in identifying potential security threats by monitoring unusual behavior patterns.
- Provides insights into product usage, allowing for better product development and improvement.
- Facilitates segmentation of users based on behavior, which can enhance targeted advertising.
At Rapid Innovation, we leverage advanced behavioral analytics tools, including user behavior analytics and customer behavior analytics, to empower organizations to make data-driven decisions that improve customer engagement and retention. By utilizing our expertise in user behavior and user and entity behavior analytics, clients can gain actionable insights that inform future strategies, ensuring they remain competitive in a rapidly changing market.
8.2. Network Analysis
Network analysis is the study of relationships and interactions within a network, often visualized through graphs and nodes. This technique is widely used in various fields, including social media, telecommunications, and cybersecurity.
- Identifies key influencers within a network, which can be crucial for marketing campaigns.
- Helps in understanding the flow of information and resources, leading to more efficient operations.
- Assists in detecting anomalies and potential security breaches by analyzing network traffic patterns.
- Enables organizations to optimize their network infrastructure for better performance.
- Provides insights into collaboration and communication patterns, enhancing team dynamics.
Incorporating network analysis into business strategies can lead to improved decision-making and resource allocation. At Rapid Innovation, we help organizations understand the connections within their networks, fostering better relationships and driving innovation.
8.3. Entity Resolution
Entity resolution is the process of identifying and merging records that refer to the same entity across different data sources. This is essential for maintaining data integrity and ensuring accurate analysis.
- Enhances data quality by eliminating duplicates and inconsistencies.
- Improves customer relationship management by providing a unified view of customer data.
- Facilitates better decision-making by ensuring that data used for analysis is accurate and reliable.
- Supports compliance with data regulations by maintaining clean and organized data sets.
- Enables organizations to gain deeper insights into customer behavior and preferences.
At Rapid Innovation, we recognize that effective entity resolution is critical for businesses that rely on data for strategic planning and operational efficiency. By ensuring that data is accurate and consistent, we empower organizations to enhance their overall performance and drive growth.
8.4. Cross-Channel Monitoring
Cross-channel monitoring refers to the practice of tracking and analyzing customer interactions across various platforms and channels. This approach is essential for businesses aiming to provide a seamless customer experience. It enables businesses to gather data from multiple sources, including social media, email, websites, and customer service interactions. Additionally, it helps identify trends and patterns in customer behavior, allowing for more informed decision-making. By facilitating a holistic view of customer journeys, businesses can understand how customers interact with their brand across different touchpoints. This practice also supports targeted marketing efforts by providing insights into which channels are most effective for specific customer segments and enhances customer service by allowing teams to respond to inquiries and issues across channels in a coordinated manner.
At Rapid Innovation, we leverage advanced AI algorithms to enhance cross-channel monitoring capabilities, enabling businesses to gain deeper insights into customer behavior and preferences. By implementing AI-driven analytics, we help clients optimize their marketing strategies, leading to improved customer satisfaction and loyalty, ultimately driving revenue growth. For more information on best practices in business AI engineering.
8.5. Real-time Alert Management
Real-time alert management is a critical component of effective operational monitoring. It involves the immediate notification of relevant stakeholders when specific events or thresholds are met, allowing for swift action. This system provides instant alerts for critical issues, such as system failures, security breaches, or significant changes in customer behavior. It enables teams to respond quickly to potential problems, minimizing downtime and reducing the impact on customer experience. Furthermore, it supports proactive management by allowing businesses to address issues before they escalate into larger problems. The alert management system can be customized to fit the needs of different departments, ensuring that the right people receive the right alerts. Additionally, it enhances collaboration among teams by providing a centralized platform for monitoring and responding to alerts.
With real-time alert management, organizations can maintain operational efficiency and improve their overall responsiveness to customer needs. Rapid Innovation employs cutting-edge blockchain technology to ensure the integrity and security of alert management systems, providing clients with a robust framework for operational excellence.
9. Integration and Interoperability
Integration and interoperability are essential for modern businesses that rely on multiple software systems and platforms. These concepts refer to the ability of different systems to work together seamlessly, sharing data and functionality. Integration facilitates data sharing between various applications, reducing the need for manual data entry and minimizing errors. It enhances operational efficiency by streamlining workflows and automating processes across different systems. Moreover, it supports better decision-making by providing a unified view of data from multiple sources, enabling comprehensive analysis. Integration also improves customer experience by ensuring that customer data is consistent and accessible across all touchpoints. Finally, it encourages innovation by allowing businesses to leverage new technologies and tools without being constrained by existing systems.
By focusing on integration and interoperability, organizations can create a more agile and responsive business environment, ultimately leading to improved performance and customer satisfaction. At Rapid Innovation, we specialize in developing tailored integration solutions that harness the power of AI and blockchain, ensuring that our clients can adapt swiftly to changing market demands while maximizing their return on investment.
9.1. API Integration
API integration is a crucial aspect of modern software development, allowing different applications to communicate and share data seamlessly. APIs, or Application Programming Interfaces, serve as intermediaries that enable various software systems to interact with each other.
- Enhances functionality: By integrating APIs, such as the Salesforce API, LinkedIn API, and Mailchimp API, businesses can extend the capabilities of their applications without having to build everything from scratch. This is particularly beneficial for organizations looking to innovate quickly and efficiently.
- Streamlines processes: API integration automates workflows, reducing manual input and minimizing errors. This leads to increased operational efficiency and allows teams to focus on strategic initiatives. For instance, integrating API to API integration can simplify data flow between systems.
- Facilitates real-time data access: APIs allow for real-time data exchange, ensuring that users have the most up-to-date information available. This is essential for making informed decisions in a fast-paced business environment, especially when using APIs like the Jira REST API or HubSpot API.
- Supports scalability: As businesses grow, API integration allows for easy addition of new features and services without significant overhauls. This adaptability is vital for maintaining a competitive edge, particularly with tools like the Workday API and Zendesk API.
- Promotes innovation: Developers can leverage existing APIs, such as the Klaviyo API and Salesforce REST API, to create new applications or enhance existing ones, fostering a culture of innovation. Rapid Innovation specializes in identifying and integrating the right APIs to drive business growth.
For example, integrating payment processing APIs can simplify transactions, while social media APIs can enhance user engagement by allowing users to share content easily. Rapid Innovation can assist in selecting and implementing the most effective APIs tailored to your business needs, including the integration of the ShipStation API and the GoHighLevel API. Additionally, consider exploring our AI as a Service offerings to further enhance your API integration capabilities, or learn more about how to integrate OpenAI API into your business app.
9.2. Data Exchange Standards
Data exchange standards are essential for ensuring that data can be shared and understood across different systems and platforms. These standards define the format and structure of data, making it easier for disparate systems to communicate effectively.
- Ensures compatibility: Adhering to data exchange standards guarantees that different systems can interpret and process data correctly. This is crucial for organizations that rely on multiple systems for their operations, including those using the Netsuite API and Monday.com APIs.
- Reduces integration costs: Standardized data formats minimize the need for custom coding, leading to lower integration costs and faster deployment. Rapid Innovation helps clients implement these standards to optimize their integration processes.
- Enhances data quality: By following established standards, organizations can improve the accuracy and consistency of their data. This is vital for maintaining trust in data-driven decision-making.
- Facilitates compliance: Many industries have regulatory requirements regarding data exchange. Using standardized formats can help organizations meet these compliance needs, reducing the risk of penalties.
- Promotes interoperability: Data exchange standards enable different systems to work together, fostering collaboration and data sharing across platforms. Rapid Innovation can guide organizations in adopting the right standards for their specific industry.
Common data exchange standards include XML, JSON, and CSV, which are widely used for data interchange in various applications.
9.3. Third-party System Integration
Third-party system integration involves connecting external software applications or services to an organization's existing systems. This integration can enhance functionality, improve efficiency, and provide access to additional resources.
- Expands capabilities: Integrating third-party systems allows organizations to leverage specialized tools and services that enhance their core offerings. Rapid Innovation can identify the best third-party solutions, such as the Freshdesk API and PagerDuty API, that align with your business objectives.
- Increases efficiency: By automating data transfer between systems, organizations can streamline operations and reduce manual tasks. This leads to improved productivity and cost savings.
- Provides access to new markets: Third-party integrations can open up new channels for business, such as e-commerce platforms or marketing tools. Rapid Innovation can help you navigate these opportunities effectively.
- Enhances user experience: Integrating third-party services can improve the overall user experience by providing additional features and functionalities. This is essential for retaining customers and driving engagement.
- Supports data analytics: Third-party integrations can facilitate better data collection and analysis, leading to more informed decision-making. Rapid Innovation can assist in implementing analytics solutions that provide actionable insights.
Examples of third-party system integrations include CRM systems, marketing automation tools, and cloud storage services, which can all work together to create a more cohesive business environment. Rapid Innovation is committed to helping clients achieve greater ROI through strategic integrations that align with their business goals, including the integration of the ADP API and the Courrier API integration.
9.4. Cloud Integration
Cloud integration refers to the process of connecting various cloud services and applications to streamline data sharing and enhance operational efficiency. As businesses increasingly adopt cloud solutions, effective cloud integration becomes essential for maximizing the benefits of these technologies.
- Seamless Data Flow: Cloud integration allows for real-time data exchange between different cloud applications, ensuring that all systems are updated and synchronized. Rapid Innovation leverages advanced integration techniques to ensure that your data flows effortlessly across platforms, reducing latency and improving decision-making. Solutions like iPaaS (integration platforms as a service) are often utilized to facilitate this seamless data flow.
- Improved Collaboration: By integrating cloud services, teams can collaborate more effectively, accessing shared data and tools from anywhere, at any time. Our solutions empower your workforce to work together seamlessly, enhancing productivity and innovation. Tools such as Salesforce Service Cloud and Jira Cloud Based can enhance collaboration across teams.
- Cost Efficiency: Cloud integration can reduce operational costs by minimizing the need for on-premises infrastructure and enabling businesses to pay only for the services they use. Rapid Innovation helps clients optimize their cloud expenditures, ensuring that they achieve maximum ROI from their cloud investments. Utilizing SaaS integration and platforms like Oracle Integration Cloud can further enhance cost efficiency.
- Scalability: As businesses grow, cloud integration provides the flexibility to scale services up or down based on demand without significant investment in hardware. Our expertise in cloud architecture ensures that your systems can adapt to changing business needs without disruption. Solutions like Azure Integration Service and Boomi Integrate offer scalable options for businesses.
- Enhanced Customer Experience: Integrated cloud solutions can lead to faster response times and improved service delivery, ultimately enhancing customer satisfaction. Rapid Innovation focuses on creating customer-centric solutions that drive engagement and loyalty. For instance, Salesforce for email marketing and customer service in Salesforce can significantly improve customer interactions.
To achieve successful cloud integration, organizations often utilize middleware solutions, APIs, and integration platforms as a service (iPaaS). These tools facilitate the connection between disparate systems, enabling businesses to create a cohesive digital ecosystem. Cloud-based integration platforms, such as cloud integration platforms and cloud-based integration, play a crucial role in this process.
9.5. Security Protocols
Security protocols are essential frameworks that govern the protection of data and systems in a digital environment. With the rise of cyber threats, implementing robust security protocols is critical for safeguarding sensitive information.
- Data Encryption: Encrypting data both in transit and at rest ensures that unauthorized users cannot access sensitive information. This is a fundamental aspect of data security that Rapid Innovation prioritizes in all our solutions.
- Authentication Mechanisms: Strong authentication protocols, such as multi-factor authentication (MFA), help verify user identities and prevent unauthorized access to systems. We implement cutting-edge authentication technologies to enhance your security posture.
- Regular Security Audits: Conducting regular security audits and vulnerability assessments helps identify potential weaknesses in the system, allowing organizations to address them proactively. Our team conducts thorough assessments to ensure your systems remain secure against evolving threats.
- Compliance Standards: Adhering to industry-specific compliance standards, such as GDPR or HIPAA, ensures that organizations meet legal requirements for data protection. Rapid Innovation guides clients through compliance processes, ensuring that they meet all necessary regulations.
- Incident Response Plans: Developing and maintaining an incident response plan prepares organizations to respond effectively to security breaches, minimizing damage and recovery time. We assist in crafting tailored incident response strategies that align with your business needs.
By implementing comprehensive security protocols, organizations can protect their data, maintain customer trust, and comply with regulatory requirements.
10. Performance Optimization
Performance optimization involves enhancing the efficiency and speed of applications and systems to ensure they operate at their best. This is crucial for delivering a seamless user experience and maintaining competitive advantage.
- Resource Management: Efficiently managing resources, such as CPU, memory, and storage, can significantly improve application performance. This includes load balancing and resource allocation strategies. Rapid Innovation employs advanced resource management techniques to ensure optimal performance.
- Code Optimization: Regularly reviewing and optimizing code can reduce execution time and improve application responsiveness. Techniques include refactoring, minimizing dependencies, and using efficient algorithms. Our development team focuses on writing clean, efficient code to enhance application performance.
- Caching Strategies: Implementing caching mechanisms can drastically reduce load times by storing frequently accessed data in memory, allowing for quicker retrieval. We design caching solutions tailored to your specific application needs.
- Monitoring and Analytics: Utilizing performance monitoring tools helps identify bottlenecks and areas for improvement. Analytics can provide insights into user behavior and system performance. Rapid Innovation integrates monitoring solutions that provide real-time insights into application performance.
- Regular Updates: Keeping software and systems updated ensures that they benefit from the latest performance enhancements and security patches. We provide ongoing support and maintenance to keep your systems running smoothly.
By focusing on performance optimization, organizations can enhance user satisfaction, reduce operational costs, and improve overall productivity. Rapid Innovation is committed to helping clients achieve their business goals through tailored solutions that drive efficiency and effectiveness.
10.1. Model Tuning
Model tuning is a critical step in the machine learning process that involves optimizing the parameters of a model to improve its performance. This process ensures that the model generalizes well to unseen data, reducing the risk of overfitting or underfitting.
- Hyperparameter Optimization: Adjusting hyperparameters such as learning rate, batch size, and number of layers can significantly impact model performance. Techniques like grid search, random search, and Bayesian hyperparameter optimization are commonly used. At Rapid Innovation, we leverage these techniques to fine-tune models, ensuring our clients achieve optimal performance and higher returns on investment.
- Cross-Validation: Implementing k-fold cross-validation helps in assessing how the results of a statistical analysis will generalize to an independent dataset. This method divides the dataset into k subsets and trains the model k times, each time using a different subset for validation. Our approach, including cross validation for hyperparameter tuning, ensures that clients can trust the robustness of their models before deployment.
- Regularization Techniques: Applying regularization methods like L1 (Lasso) and L2 (Ridge) can help prevent overfitting by penalizing large coefficients in the model. This encourages the model to focus on the most significant features, ultimately leading to more reliable predictions and better business outcomes.
- Feature Selection: Identifying and selecting the most relevant features can enhance model performance. Techniques such as Recursive Feature Elimination (RFE) and feature importance from tree-based models can be employed. Rapid Innovation assists clients in this process, ensuring that only the most impactful features are utilized, thereby maximizing efficiency. This includes feature selection and hyperparameter tuning to optimize results.
- Ensemble Methods: Combining multiple models can lead to better performance. Techniques like bagging, boosting, and stacking can help in leveraging the strengths of different models. Our expertise in ensemble methods allows us to deliver superior predictive capabilities, driving greater ROI for our clients. For more insights on improving model performance, you can refer to our article on debugging and troubleshooting common issues in computer vision models.
10.2. False Positive Reduction
False positives occur when a model incorrectly predicts a positive outcome, leading to potential misinterpretations and unnecessary actions. Reducing false positives is crucial for improving the reliability of predictive models, especially in sensitive applications like healthcare and fraud detection.
- Threshold Adjustment: Modifying the decision threshold can help balance sensitivity and specificity. Lowering the threshold may increase true positives but can also raise false positives, so careful calibration is necessary. Rapid Innovation employs this strategy to ensure our clients' models are finely tuned for their specific needs.
- Anomaly Detection: Implementing anomaly detection techniques can help identify outliers that may lead to false positives. Methods like Isolation Forest or One-Class SVM can be effective in distinguishing normal from abnormal instances. Our team utilizes these methods to enhance model accuracy, particularly in high-stakes environments.
- Confusion Matrix Analysis: Regularly analyzing the confusion matrix provides insights into the model's performance. It helps in understanding the trade-offs between true positives, false positives, true negatives, and false negatives. We guide our clients through this analysis to refine their models continuously.
- Advanced Algorithms: Utilizing advanced algorithms like Support Vector Machines (SVM) or Random Forests can improve classification accuracy and reduce false positives. These algorithms often have built-in mechanisms to handle class imbalance. Rapid Innovation's expertise in these algorithms ensures that our clients benefit from cutting-edge technology.
- Data Quality Improvement: Ensuring high-quality, clean data can significantly reduce false positives. This includes removing duplicates, handling missing values, and ensuring accurate labeling of data. Our commitment to data integrity helps clients achieve more reliable outcomes.
10.3. Processing Efficiency
Processing efficiency refers to the speed and resource utilization of a machine learning model during training and inference. Enhancing processing efficiency is essential for deploying models in real-time applications and ensuring scalability.
- Algorithm Selection: Choosing efficient algorithms can drastically reduce processing time. For instance, decision trees and linear models are generally faster than complex models like deep neural networks. Rapid Innovation helps clients select the most suitable algorithms for their specific use cases, optimizing performance and cost.
- Parallel Processing: Leveraging parallel processing techniques can significantly speed up computations. Utilizing frameworks like Apache Spark or Dask allows for distributed computing, making it easier to handle large datasets. Our expertise in these technologies enables clients to scale their operations effectively.
- Model Simplification: Simplifying models by reducing complexity can enhance processing efficiency. Techniques like pruning in decision trees or using fewer layers in neural networks can help maintain performance while improving speed. We work closely with clients to ensure their models are both effective and efficient.
- Batch Processing: Implementing batch processing during training and inference can optimize resource usage. Instead of processing one instance at a time, processing multiple instances simultaneously can lead to faster execution. Rapid Innovation incorporates this strategy to enhance the overall efficiency of our clients' systems.
- Hardware Utilization: Utilizing specialized hardware like GPUs or TPUs can accelerate model training and inference. These devices are designed to handle parallel computations, making them ideal for deep learning tasks. Our team ensures that clients leverage the best hardware solutions to maximize their model performance and return on investment.
- Nested Cross Validation: To further enhance model tuning, we also implement nested cross validation hyperparameter tuning, which provides a more robust evaluation of model performance by ensuring that the hyperparameter tuning process does not lead to overfitting.
- Hyperparameter Tuning Techniques: We employ various hyperparameter tuning techniques, including k fold cross validation hyperparameter tuning, to systematically explore the hyperparameter space and identify the best configurations for our models.
- Model Tuning Techniques: Our comprehensive approach to model tuning techniques ensures that we consider all aspects of model performance, from hyperparameter tuning and cross validation to feature selection and regularization, to deliver the best possible outcomes for our clients.
10.4. Scalability Solutions
Scalability is a critical aspect of any system, especially in the context of technology and business. It refers to the ability of a system to handle a growing amount of work or its potential to accommodate growth. Here are some key scalability solutions that Rapid Innovation can implement to help clients achieve their business goals efficiently:
- Cloud Computing: Utilizing cloud services allows businesses to scale resources up or down based on demand. This flexibility is essential for managing costs and ensuring performance during peak times. Rapid Innovation can assist in selecting the right cloud provider and architecture to optimize resource allocation.
- Microservices Architecture: By breaking down applications into smaller, independent services, organizations can scale individual components without affecting the entire system. This approach enhances deployment speed and reliability, enabling businesses to respond quickly to market changes.
- Load Balancing: Distributing workloads across multiple servers ensures that no single server becomes a bottleneck. This not only improves performance but also enhances redundancy and fault tolerance. Rapid Innovation can design and implement load balancing strategies tailored to specific business needs.
- Database Sharding: This technique involves splitting a database into smaller, more manageable pieces, or shards. Each shard can be hosted on different servers, allowing for improved performance and scalability. Our team can help clients implement sharding strategies that align with their data access patterns.
- Content Delivery Networks (CDNs): CDNs help in distributing content closer to users, reducing latency and improving load times. This is particularly beneficial for websites and applications with a global audience. Rapid Innovation can integrate CDN solutions to enhance user experience and engagement.
- Horizontal vs. Vertical Scaling: Horizontal scaling involves adding more machines to a pool, while vertical scaling means upgrading existing machines. Understanding the right approach for your needs is crucial for effective scalability. Our experts can analyze your current infrastructure and recommend the most suitable scaling strategy, including scalability solutions for blockchain and crypto scaling.
10.5. Resource Optimization
Resource optimization focuses on making the best use of available resources to improve efficiency and reduce costs. Here are some strategies for effective resource optimization that Rapid Innovation can implement:
- Performance Monitoring: Regularly monitoring system performance helps identify bottlenecks and areas for improvement. Tools like application performance management (APM) can provide insights into resource usage, allowing for informed decision-making.
- Automated Resource Allocation: Implementing automation tools can help allocate resources dynamically based on real-time demand. This ensures that resources are used efficiently without manual intervention, leading to cost savings and improved performance.
- Virtualization: Virtualization allows multiple virtual machines to run on a single physical server, maximizing hardware utilization. This leads to reduced costs and improved resource management, which Rapid Innovation can facilitate through tailored virtualization solutions.
- Energy Efficiency: Optimizing energy consumption not only reduces costs but also contributes to sustainability. Implementing energy-efficient hardware and practices can lead to significant savings, aligning with corporate social responsibility goals.
- Capacity Planning: Anticipating future resource needs based on historical data and trends helps in making informed decisions about resource allocation. This proactive approach minimizes downtime and enhances performance, ensuring that businesses are always prepared for growth.
- Regular Audits: Conducting regular audits of resource usage can help identify underutilized assets. This allows organizations to reallocate or decommission resources that are no longer needed, optimizing overall resource utilization.
11. Future Trends and Innovation
The landscape of technology and business is constantly evolving. Staying ahead of future trends and innovations is essential for maintaining a competitive edge. Here are some key trends to watch, which Rapid Innovation can help clients navigate:
- Artificial Intelligence (AI) and Machine Learning (ML): AI and ML are transforming industries by enabling data-driven decision-making and automating processes. Businesses that leverage these technologies can gain insights and improve efficiency. Rapid Innovation specializes in developing AI solutions tailored to specific business needs.
- 5G Technology: The rollout of 5G networks promises faster internet speeds and lower latency. This will enable new applications, such as IoT devices and augmented reality, to flourish. Our team can help clients harness the power of 5G to enhance their offerings.
- Blockchain: Beyond cryptocurrencies, blockchain technology is being explored for various applications, including supply chain management, secure transactions, and data integrity. Rapid Innovation can guide organizations in implementing blockchain solutions that enhance transparency and security, including scalability of blockchain and blockchain scalability solutions.
- Remote Work Solutions: The shift towards remote work has led to increased demand for collaboration tools and platforms. Innovations in this space will continue to evolve as organizations adapt to hybrid work models. We can assist in developing robust remote work solutions that foster collaboration and productivity.
- Sustainability Initiatives: As environmental concerns grow, businesses are focusing on sustainable practices. Innovations in renewable energy, waste reduction, and sustainable sourcing are becoming increasingly important. Rapid Innovation can help organizations integrate sustainable technologies into their operations.
- Edge Computing: With the rise of IoT devices, edge computing is gaining traction. This technology processes data closer to the source, reducing latency and bandwidth usage, which is crucial for real-time applications. Our expertise can help clients implement edge computing solutions that enhance operational efficiency.
- Cybersecurity Advances: As cyber threats become more sophisticated, innovations in cybersecurity are essential. This includes AI-driven security solutions, zero-trust architectures, and enhanced encryption methods. Rapid Innovation can develop comprehensive cybersecurity strategies to protect client assets.
- Augmented and Virtual Reality (AR/VR): These technologies are finding applications in various fields, from gaming to training and education. As hardware becomes more accessible, AR and VR will likely see broader adoption. We can assist in creating immersive experiences that engage users effectively.
- Personalization: Businesses are increasingly focusing on personalized experiences for customers. Innovations in data analytics and AI will enable more tailored marketing and product offerings. Rapid Innovation can help organizations leverage data to create personalized customer journeys.
- Quantum Computing: Although still in its infancy, quantum computing holds the potential to revolutionize industries by solving complex problems much faster than traditional computers. Our team is keeping a close eye on developments in this area to prepare clients for future advancements.
By keeping an eye on these trends and innovations, organizations can better prepare for the future and adapt to the ever-changing technological landscape, with Rapid Innovation as a trusted partner in their journey, including exploring scalability solutions in crypto and the scalability of cryptocurrency.
11.1. Quantum Computing Applications
Quantum computing represents a revolutionary leap in computational power, leveraging the principles of quantum mechanics to process information in ways that classical computers cannot. The applications of quantum computing are vast and varied, impacting numerous fields.
- Cryptography: Quantum computers can potentially break traditional encryption methods, leading to the development of quantum-resistant algorithms. This is crucial for securing sensitive data in finance and government sectors, where Rapid Innovation can assist clients in transitioning to these advanced security measures.
- Drug Discovery: By simulating molecular interactions at an atomic level, quantum computing can accelerate the drug discovery process, allowing researchers to identify potential compounds more efficiently. Rapid Innovation can partner with pharmaceutical companies to implement quantum solutions that enhance their research capabilities.
- Optimization Problems: Industries such as logistics and finance can benefit from quantum algorithms that solve complex optimization problems faster than classical methods, improving efficiency and reducing costs. Rapid Innovation can help businesses integrate these algorithms into their operations for better resource management.
- Artificial Intelligence: Quantum computing can enhance machine learning algorithms, enabling faster data processing and more sophisticated models that can learn from vast datasets. The intersection of quantum computing and AI presents exciting opportunities for innovation. Rapid Innovation can leverage this synergy to develop AI solutions that drive innovation and competitive advantage for clients.
- Climate Modeling: Quantum computers can simulate complex climate systems, providing insights that can help in understanding climate change and developing mitigation strategies. Rapid Innovation can collaborate with environmental organizations to utilize quantum computing for impactful climate research.
- Quantum Computing Applications: The potential of quantum computing is still being explored, but its applications promise to transform industries and solve problems that are currently intractable. From quantum computing machine learning to applications for quantum computing, the future is bright. For businesses looking to integrate blockchain technology into their operations, Rapid Innovation offers specialized services to develop blockchain applications that can complement quantum computing advancements.
11.2. Blockchain Integration
Blockchain technology has gained significant traction across various sectors due to its decentralized, secure, and transparent nature. Integrating blockchain into existing systems can enhance efficiency and trust.
- Supply Chain Management: Blockchain can provide real-time tracking of goods, ensuring transparency and reducing fraud. Each transaction is recorded on a public ledger, making it easy to trace the origin of products. Rapid Innovation can help businesses implement blockchain solutions that enhance supply chain integrity.
- Financial Services: Blockchain enables faster and cheaper cross-border transactions by eliminating intermediaries. Smart contracts can automate processes, reducing the need for manual intervention. Rapid Innovation can assist financial institutions in adopting blockchain to streamline their operations.
- Healthcare: Patient records can be securely stored on a blockchain, ensuring privacy and accessibility. This can streamline data sharing among healthcare providers while maintaining patient confidentiality. Rapid Innovation can work with healthcare organizations to develop blockchain-based solutions that enhance patient data management.
- Voting Systems: Blockchain can enhance the integrity of voting processes by providing a tamper-proof record of votes, increasing public trust in electoral outcomes. Rapid Innovation can support governmental bodies in implementing secure voting systems using blockchain technology.
- Digital Identity Verification: Blockchain can facilitate secure and verifiable digital identities, reducing identity theft and fraud in online transactions. Rapid Innovation can help businesses create robust identity verification systems that leverage blockchain for enhanced security.
The integration of blockchain technology is reshaping how businesses operate, fostering innovation and improving security across various sectors.
11.3. Advanced AI Capabilities
Artificial Intelligence (AI) continues to evolve, with advanced capabilities that are transforming industries and enhancing human decision-making. The integration of AI into various applications is driving efficiency and innovation.
- Natural Language Processing (NLP): AI systems can understand and generate human language, enabling applications like chatbots, virtual assistants, and sentiment analysis tools that improve customer engagement. Rapid Innovation can develop tailored NLP solutions that enhance client interactions.
- Computer Vision: AI can analyze and interpret visual data, leading to advancements in facial recognition, autonomous vehicles, and medical imaging, enhancing accuracy and efficiency in various fields. Rapid Innovation can assist companies in implementing computer vision technologies to optimize their operations.
- Predictive Analytics: AI algorithms can analyze historical data to predict future trends, helping businesses make informed decisions in areas like marketing, inventory management, and risk assessment. Rapid Innovation can provide predictive analytics solutions that empower clients to stay ahead of market trends.
- Robotics: Advanced AI capabilities are enabling robots to perform complex tasks in manufacturing, healthcare, and logistics, improving productivity and safety. Rapid Innovation can collaborate with organizations to integrate AI-driven robotics into their workflows.
- Personalization: AI can analyze user behavior to deliver personalized experiences in e-commerce, entertainment, and online services, enhancing customer satisfaction and loyalty. Rapid Innovation can help businesses harness AI for personalized marketing strategies that drive engagement.
The advanced capabilities of AI are not only improving operational efficiency but also creating new opportunities for innovation across multiple sectors. Rapid Innovation is committed to helping clients leverage these technologies to achieve their business goals effectively and efficiently.
11.4. Regulatory Technology Evolution
Regulatory Technology, or RegTech, has emerged as a vital component in the financial services industry, particularly in response to increasing regulatory demands. The evolution of RegTech is driven by the need for organizations to comply with complex regulations efficiently and cost-effectively.
- Increased automation: RegTech solutions leverage automation to streamline compliance processes, reducing the need for manual intervention. This leads to faster reporting and improved accuracy, ultimately enhancing operational efficiency and reducing costs.
- Data analytics: Advanced data analytics tools help organizations identify compliance risks and monitor regulatory changes in real-time. This proactive approach allows firms to adapt quickly to new regulations, minimizing potential penalties and enhancing their compliance posture.
- Cloud computing: The adoption of cloud technology enables RegTech solutions to offer scalable and flexible compliance tools. Organizations can access these tools without significant upfront investments in infrastructure, allowing for better resource allocation and improved ROI.
- Artificial Intelligence (AI): AI is increasingly being integrated into RegTech solutions to enhance decision-making processes. Machine learning algorithms can analyze vast amounts of data to detect anomalies and predict potential compliance issues, enabling organizations to address risks before they escalate. For more insights on AI and machine learning in regulatory compliance.
- Collaboration with regulators: RegTech firms are increasingly collaborating with regulatory bodies to develop solutions that meet compliance requirements. This partnership fosters innovation and ensures that technology aligns with regulatory expectations, ultimately leading to more effective compliance strategies.
The evolution of RegTech is reshaping how organizations approach compliance, making it more efficient and less burdensome. At Rapid Innovation, we leverage our expertise in AI and blockchain to help clients implement cutting-edge regtech software that drives compliance efficiency and enhances their overall business performance.
11.5. Emerging Risk Scenarios
As the financial landscape evolves, new risk scenarios are emerging that organizations must address. Understanding these risks is crucial for effective risk management and compliance strategies.
- Cybersecurity threats: With the rise of digital banking and online transactions, cybersecurity risks have become a significant concern. Organizations must invest in robust cybersecurity measures to protect sensitive data from breaches and attacks, ensuring the integrity of their operations.
- Regulatory changes: Rapid changes in regulations can create uncertainty and risk for organizations. Staying informed about regulatory developments and adapting compliance strategies accordingly is essential to mitigate potential disruptions.
- Market volatility: Economic fluctuations can lead to increased market risk. Organizations need to develop strategies to mitigate the impact of market volatility on their operations and financial stability, ensuring resilience in challenging environments.
- Operational risks: As organizations adopt new technologies, operational risks associated with system failures, data integrity, and process inefficiencies can arise. Implementing strong governance frameworks can help manage these risks effectively.
- Reputational risks: Negative publicity or failure to comply with regulations can damage an organization's reputation. Proactive communication and transparency are vital in managing reputational risks, safeguarding stakeholder trust.
By identifying and addressing these emerging risk scenarios, organizations can enhance their resilience and ensure long-term sustainability. Rapid Innovation is committed to helping clients navigate these challenges through tailored AI and blockchain solutions that bolster risk management and compliance frameworks, including compliance regtech and regulatory technology compliance.
12. Implementation Best Practices
Implementing effective compliance and risk management strategies requires careful planning and execution. Here are some best practices to consider:
- Define clear objectives: Establish specific compliance and risk management goals that align with the organization's overall strategy. This clarity will guide implementation efforts and ensure alignment with business objectives.
- Engage stakeholders: Involve key stakeholders from various departments in the implementation process. Their insights and expertise can help identify potential challenges and solutions, fostering a collaborative approach.
- Invest in training: Provide comprehensive training for employees on compliance requirements and risk management practices. A well-informed workforce is essential for successful implementation and adherence to compliance standards.
- Leverage technology: Utilize technology solutions, such as regtech and data analytics, to enhance compliance and risk management processes. Automation can significantly improve efficiency and accuracy, leading to better resource utilization.
- Monitor and evaluate: Continuously monitor compliance and risk management efforts to assess their effectiveness. Regular evaluations can help identify areas for improvement and ensure ongoing compliance with evolving regulations.
- Foster a culture of compliance: Encourage a culture that prioritizes compliance and risk management at all levels of the organization. Leadership should model this behavior to reinforce its importance and commitment to ethical practices.
By following these best practices, organizations can effectively implement compliance and risk management strategies that support their long-term success. At Rapid Innovation, we are dedicated to providing the expertise and technology solutions necessary to help our clients achieve their compliance and risk management goals efficiently and effectively, including the use of Complysci NRS and regtech regulatory compliance.
12.1. Project Planning
Project planning is a critical phase in the project management lifecycle. It involves defining the project scope, objectives, and deliverables, as well as outlining the steps necessary to achieve them. Effective project planning sets the foundation for successful project execution, particularly in the realms of AI and Blockchain, where precision and clarity are paramount.
- Define project goals and objectives clearly, ensuring they align with your business strategy and desired outcomes.
- Identify stakeholders and their roles, including technical teams, business leaders, and end-users, to foster collaboration and accountability.
- Develop a detailed project schedule, including milestones and deadlines, to track progress and ensure timely delivery using tools like project scheduling software and project schedule templates.
- Create a budget that outlines costs associated with resources, materials, and labor, allowing for informed financial decisions.
- Assess risks and develop a risk management plan to mitigate potential issues, particularly those unique to AI and Blockchain projects, such as data privacy and regulatory compliance.
- Utilize project management tools and software, such as free project management software and project planning software, for tracking progress and collaboration, enhancing transparency and communication.
- Ensure communication plans are in place to keep all stakeholders informed, facilitating alignment and engagement throughout the project lifecycle.
A well-structured project plan not only guides the team but also helps in aligning resources and expectations. According to the Project Management Institute, organizations that invest in project planning are more likely to meet their project goals and stay within budget, ultimately leading to greater ROI.
12.2. Resource Allocation
Resource allocation is the process of assigning available resources in an efficient manner to maximize productivity and achieve project objectives. This includes human resources, financial resources, and physical assets, all of which are crucial in AI and Blockchain initiatives.
- Identify all resources required for the project, including personnel with specialized skills in AI algorithms or Blockchain development, equipment, and materials.
- Assess the availability of resources and their skill sets, ensuring that the right expertise is applied to the right tasks.
- Prioritize tasks based on resource availability and project timelines, optimizing workflow and efficiency.
- Allocate resources based on project needs, ensuring that the right people are assigned to the right tasks to leverage their strengths.
- Monitor resource usage throughout the project to avoid overallocation or underutilization, which can lead to delays and increased costs.
- Adjust allocations as necessary to respond to project changes or unforeseen challenges, maintaining flexibility in a dynamic environment.
Effective resource allocation can significantly impact project success. According to a study by the Project Management Institute, organizations that excel in resource management are 2.5 times more likely to complete projects on time and within budget, enhancing overall ROI.
12.3. Change Management
Change management is the process of managing changes to a project’s scope, objectives, or deliverables. It is essential for maintaining project integrity and ensuring that all stakeholders are aligned with any modifications, especially in fast-evolving fields like AI and Blockchain.
- Establish a change management process that includes documentation and approval procedures to ensure all changes are tracked and justified.
- Communicate the reasons for changes to all stakeholders to ensure understanding and buy-in, fostering a culture of transparency.
- Assess the impact of changes on project timelines, costs, and resources, particularly in relation to the complex nature of AI and Blockchain technologies.
- Involve key stakeholders in the change approval process to foster collaboration and ensure that all perspectives are considered.
- Update project plans and documentation to reflect approved changes, maintaining an accurate record of project evolution, utilizing change management plan templates and project management plan templates.
- Monitor the implementation of changes to ensure they are executed effectively, minimizing disruptions and maintaining project momentum.
A structured change management approach helps minimize disruptions and keeps the project on track. Research indicates that effective change management can lead to a 70% success rate in project outcomes, highlighting its importance in project management and its potential to drive greater ROI for clients.
12.4. Training and Skill Development
Training and skill development are crucial components for enhancing employee performance and ensuring organizational growth. A well-structured training program, such as management training for managers or training manager training courses, can lead to increased productivity, improved job satisfaction, and reduced turnover rates.
- Importance of Training:
- Keeps employees updated with the latest industry trends and technologies, particularly in AI and Blockchain, which are rapidly evolving fields.
- Enhances employee confidence and competence in their roles, enabling them to leverage advanced tools and methodologies effectively.
- Fosters a culture of continuous learning within the organization, essential for staying competitive in the tech landscape.
- Types of Training Programs:
- Onboarding: Introduces new hires to company culture and processes, ensuring they understand the integration of AI and Blockchain in business operations.
- Technical Skills Training: Focuses on specific job-related skills, such as software proficiency in AI algorithms or Blockchain development frameworks.
- Soft Skills Development: Enhances interpersonal skills, communication, and teamwork, which are vital for collaborative projects in tech environments.
- Methods of Training:
- In-person Workshops: Interactive sessions that encourage hands-on learning, particularly beneficial for complex technologies like AI and Blockchain.
- E-Learning: Flexible online courses that employees can complete at their own pace, allowing for self-directed learning in specialized areas, including online training courses for employees and online staff training courses.
- Mentorship Programs: Pairing less experienced employees with seasoned professionals for guidance, fostering knowledge transfer in cutting-edge technologies.
- Measuring Training Effectiveness:
- Employee Feedback: Surveys and interviews to gauge satisfaction and learning outcomes, ensuring the training aligns with business goals.
- Performance Metrics: Analyzing productivity and quality of work pre- and post-training to assess the impact on organizational performance.
- Return on Investment (ROI): Evaluating the financial impact of training on the organization, particularly in terms of enhanced capabilities in AI and Blockchain projects.
Investing in training and skill development, such as employee training programs and supervisor training, not only benefits employees but also contributes to the overall success of the organization. For more insights on effective training strategies, you can refer to learning from real-world AI implementations.
12.5. Success Metrics
Success metrics are essential for evaluating the effectiveness of various initiatives within an organization. They provide a quantitative basis for decision-making and help in identifying areas for improvement.
- Key Performance Indicators (KPIs):
- Employee Engagement: Measures how committed and motivated employees are towards their work, particularly in innovative fields like AI and Blockchain.
- Productivity Rates: Assesses the output of employees in relation to their input, crucial for understanding the efficiency of tech-driven processes.
- Customer Satisfaction: Gauges how well the organization meets customer expectations, especially in delivering AI and Blockchain solutions.
- Financial Metrics:
- Revenue Growth: Tracks the increase in sales over a specific period, reflecting the success of AI and Blockchain initiatives.
- Profit Margins: Evaluates the profitability of the organization by comparing revenue to expenses, particularly in tech investments.
- Cost per Hire: Analyzes the total cost involved in recruiting new employees, ensuring efficient resource allocation.
- Qualitative Metrics:
- Employee Feedback: Collecting insights from employees about their experiences and satisfaction levels, which can inform future training and development, including employee development training and employee diversity training.
- Brand Reputation: Monitoring public perception and employee advocacy for the organization, especially in the tech sector.
- Innovation Rate: Evaluating the frequency and impact of new ideas and improvements implemented, particularly in AI and Blockchain projects.
- Importance of Regular Review:
- Continuous Monitoring: Regularly assessing success metrics ensures that the organization stays on track in achieving its strategic goals.
- Data-Driven Decisions: Utilizing metrics to inform strategic planning and resource allocation, particularly in tech-driven initiatives.
- Adaptability: Being able to pivot strategies based on metric outcomes to enhance performance and respond to market changes.
Establishing clear success metrics allows organizations to measure progress and make informed decisions that drive growth.
13. Case Studies
Case studies provide valuable insights into real-world applications of theories and practices within organizations. They illustrate how specific strategies have been implemented and the outcomes achieved.
- Importance of Case Studies:
- Learning Tool: Offers practical examples that can guide decision-making and strategy formulation, particularly in the context of AI and Blockchain.
- Benchmarking: Allows organizations to compare their performance against industry leaders, identifying best practices in tech implementation.
- Problem-Solving: Highlights challenges faced and solutions implemented, providing a roadmap for others in similar industries.
- Examples of Successful Case Studies:
- Company A: Implemented a comprehensive training program, including supervisor and management training, that resulted in a 30% increase in employee productivity within six months. This case demonstrates the impact of targeted skill development on overall performance.
- Company B: Adopted a new customer relationship management (CRM) system, leading to a 25% improvement in customer satisfaction scores. This case illustrates the importance of technology in enhancing customer interactions.
- Company C: Launched an employee engagement initiative that reduced turnover rates by 15% over a year. This case emphasizes the significance of fostering a positive workplace culture.
- Analyzing Case Studies:
- Identify Key Takeaways: Extract lessons learned and best practices that can be applied to other organizations, particularly in tech-driven environments.
- Evaluate Context: Understand the specific circumstances and environment in which the case study was conducted, ensuring relevance to current challenges.
- Adapt Strategies: Consider how the strategies used in the case study can be tailored to fit different organizational needs, especially in the context of AI and Blockchain.
Case studies serve as a powerful tool for organizations looking to learn from the experiences of others and implement effective strategies for success, including programs for employee development and training plans for employees.
13.1. Large Financial Institutions
Large financial institutions play a crucial role in the global economy, providing a wide range of services including banking, investment, and insurance. These institutions are characterized by their significant assets, extensive customer bases, and complex operational structures.
- Global Presence: They often have a global presence, operating in multiple countries and jurisdictions.
- Regulatory Requirements: Large financial institutions are subject to stringent regulatory requirements, which vary by region.
- Technology Investment: They invest heavily in technology to enhance their services and improve customer experience, often utilizing fintech solutions and financial technology platforms.
- Risk Management: Risk management is a top priority, as they deal with large volumes of transactions and sensitive customer data.
- Sustainability Focus: These institutions are increasingly focusing on sustainability and corporate social responsibility, aligning their operations with environmental, social, and governance (ESG) criteria.
The impact of large financial institutions on the economy is profound, as they facilitate capital flow, support businesses, and contribute to job creation. Their ability to innovate and adapt to market changes is essential for maintaining competitiveness in the financial sector. At Rapid Innovation, we understand the unique challenges faced by these institutions and offer tailored AI and blockchain solutions that enhance operational efficiency, improve risk management, and ensure compliance with regulatory requirements, ultimately driving greater ROI.
13.2. Fintech Implementation
Fintech, or financial technology, refers to the integration of technology into offerings by financial services companies to improve their use of financial services. The implementation of fintech solutions has transformed the financial landscape, making services more accessible and efficient.
- Technology Leverage: Fintech companies leverage technology to provide services such as mobile banking, peer-to-peer lending, and robo-advisors, including fintech payment solutions and fintech software solutions.
- User Experience: They often focus on user experience, offering intuitive interfaces and seamless transactions.
- Increased Competition: The rise of fintech has led to increased competition, pushing traditional banks to innovate and enhance their services, particularly through fintech banking solutions and fintech platforms.
- Regulatory Compliance: Regulatory compliance is a significant challenge for fintech companies, as they must navigate complex legal frameworks.
- Collaboration: Collaboration between fintech firms and traditional financial institutions is becoming more common, leading to hybrid models that combine the strengths of both.
The adoption of fintech solutions can lead to cost savings, improved customer satisfaction, and greater financial inclusion, particularly for underserved populations. Rapid Innovation specializes in developing cutting-edge fintech applications that streamline processes and enhance user engagement, ensuring our clients stay ahead in a competitive market.
13.3. Cross-border Transaction Monitoring
Cross-border transaction monitoring is essential for ensuring compliance with international regulations and preventing financial crimes such as money laundering and fraud. As globalization increases, the volume of cross-border transactions has surged, necessitating robust monitoring systems.
- Advanced Technologies: Financial institutions must implement advanced technologies to track and analyze cross-border transactions in real-time, utilizing fintech software services and fintech solutions for banks.
- Regulatory Compliance: Compliance with regulations such as the Anti-Money Laundering (AML) and the Foreign Account Tax Compliance Act (FATCA) is critical.
- Effective Monitoring: Effective monitoring systems can help identify suspicious activities and mitigate risks associated with cross-border transactions.
- Collaboration: Collaboration between financial institutions and regulatory bodies is vital for sharing information and best practices.
- AI and Machine Learning: The use of artificial intelligence and machine learning is becoming more prevalent in transaction monitoring, enhancing the ability to detect anomalies.
Cross-border transaction monitoring not only protects financial institutions from regulatory penalties but also contributes to the integrity of the global financial system. At Rapid Innovation, we leverage AI and machine learning technologies to develop sophisticated monitoring solutions that enhance compliance and reduce operational risks, ultimately leading to improved ROI for our clients.
13.4. Cryptocurrency Exchanges
Cryptocurrency exchanges are platforms that facilitate the buying, selling, and trading of cryptocurrencies. They play a crucial role in the cryptocurrency ecosystem by providing a marketplace for users to exchange digital assets. Here are some key aspects of cryptocurrency exchanges:
- Types of Exchanges:
- Centralized exchanges (CEX) are operated by companies that act as intermediaries between buyers and sellers. Examples include Binance and Coinbase, as well as the best cryptocurrency exchange options available.
- Decentralized exchanges (DEX) allow users to trade directly with one another without a central authority. Examples include Uniswap and SushiSwap.
- Trading Pairs:
- Exchanges offer various trading pairs, allowing users to trade one cryptocurrency for another. Common pairs include BTC/USD, ETH/BTC, and LTC/ETH. Users can also explore crypto to crypto exchange options for more diverse trading strategies.
- Liquidity:
- High liquidity on an exchange means that users can buy or sell assets quickly without significantly affecting the price. Major exchanges typically have higher liquidity due to a larger user base, making them ideal for crypto arbitrage trading.
- Security:
- Security is a major concern for cryptocurrency exchanges. Users should look for exchanges that implement strong security measures, such as two-factor authentication (2FA), cold storage for funds, and regular security audits. At Rapid Innovation, we emphasize the importance of security in our blockchain solutions, ensuring that our clients' platforms are fortified against potential threats.
- Fees:
- Exchanges charge fees for trading, which can vary widely. Users should compare fees across different platforms to find the most cost-effective option. Our consulting services can help clients analyze fee structures and optimize their trading strategies for better ROI, especially when considering trading platforms for crypto.
- Regulation:
- The regulatory environment for cryptocurrency exchanges varies by country. Some regions have strict regulations, while others are more lenient. Users should be aware of the legal implications of trading in their jurisdiction. Rapid Innovation provides guidance on compliance, helping clients navigate the complex regulatory landscape.
- User Experience:
- A user-friendly interface can enhance the trading experience. Many exchanges offer mobile apps and advanced trading tools for experienced traders. We assist clients in designing intuitive user interfaces that improve customer engagement and satisfaction, particularly for crypto trading for beginners.
- Customer Support:
- Reliable customer support is essential for resolving issues quickly. Users should check the support options available, such as live chat, email, or phone support. Our team can help implement robust customer support systems that enhance user trust and retention.
13.5. Payment Service Providers
Payment service providers (PSPs) are companies that facilitate online payments for businesses and consumers. In the context of cryptocurrency, PSPs enable merchants to accept digital currencies as a form of payment. Here are some important points regarding payment service providers:
- Functionality:
- PSPs act as intermediaries between merchants and customers, processing transactions securely and efficiently. They handle various payment methods, including credit cards, bank transfers, and cryptocurrencies.
- Integration:
- Many PSPs offer easy integration with e-commerce platforms, allowing merchants to accept cryptocurrency payments without extensive technical knowledge. Popular platforms include Shopify and WooCommerce. Rapid Innovation specializes in seamless integration solutions, ensuring that our clients can quickly adopt cryptocurrency payment options.
- Security:
- Security is paramount for PSPs, as they handle sensitive financial information. They typically use encryption and tokenization to protect transaction data. Our blockchain expertise allows us to implement cutting-edge security measures that safeguard transactions.
- Fees:
- PSPs charge fees for their services, which can include transaction fees, monthly fees, and setup fees. Merchants should evaluate the cost structure to find a provider that fits their budget. We provide analytical tools to help clients assess and minimize payment processing costs.
- Multi-Currency Support:
- Many PSPs support multiple cryptocurrencies, allowing merchants to accept a variety of digital assets. This flexibility can attract a broader customer base. Rapid Innovation can assist in developing multi-currency payment solutions that cater to diverse consumer preferences.
- Regulatory Compliance:
- PSPs must comply with financial regulations, including anti-money laundering (AML) and know your customer (KYC) requirements. This compliance helps build trust with users and regulators. Our consulting services ensure that clients meet all necessary compliance standards.
- Customer Support:
- Good customer support is essential for resolving payment issues. Merchants should choose PSPs that offer responsive support channels. We can help implement effective customer support strategies that enhance user experience.
- Market Trends:
- The adoption of cryptocurrencies as a payment method is growing. More businesses are recognizing the benefits of accepting digital currencies, such as lower transaction fees and faster processing times. Rapid Innovation keeps clients informed about market trends, enabling them to stay competitive.
- User Experience:
- A seamless payment experience can enhance customer satisfaction. PSPs should provide a smooth checkout process, minimizing friction for users. Our team focuses on optimizing user experience to drive higher conversion rates for our clients.
In conclusion, both cryptocurrency exchanges and payment service providers are integral to the cryptocurrency landscape. They facilitate trading and transactions, making it easier for users to engage with digital assets. Understanding their features, benefits, and potential drawbacks can help users make informed decisions in the evolving world of cryptocurrency. At Rapid Innovation, we are committed to helping our clients leverage these platforms effectively to achieve their business goals and maximize ROI.