Table Of Contents
Category
Artificial Intelligence
CRM
Marketing
AIML
IoT
Blockchain
1. Introduction to AI-Powered Technical Troubleshooting
AI-powered technical troubleshooting refers to the use of artificial intelligence technologies to identify, diagnose, and resolve technical issues in various systems and devices. This innovative approach leverages machine learning, natural language processing, and data analytics to enhance the efficiency and effectiveness of troubleshooting processes.
AI technical troubleshooting can analyze vast amounts of data quickly, allowing for faster problem resolution.
They can learn from past incidents, improving their ability to predict and solve future issues.
AI tools can operate 24/7, providing continuous support without the need for human intervention.
The integration of AI in technical troubleshooting is transforming how organizations manage their IT infrastructure, customer support, and product maintenance. By automating routine tasks and providing intelligent insights, AI agents can significantly reduce downtime and enhance user satisfaction.
Organizations can benefit from reduced operational costs due to fewer human resources needed for troubleshooting.
AI can help in identifying patterns in technical issues, leading to proactive maintenance and fewer recurring problems.
The use of AI in troubleshooting can improve the overall user experience by providing quicker resolutions to technical problems.
At Rapid Innovation, we harness the power of AI to deliver tailored troubleshooting solutions that align with your business objectives. Our expertise in AI development allows us to create customized AI agents that not only streamline your troubleshooting processes but also enhance your overall operational efficiency.
As businesses increasingly rely on technology, the demand for efficient troubleshooting solutions continues to grow. AI-powered agents are becoming essential tools for IT departments, customer service teams, and product support organizations, enabling them to address issues more effectively and efficiently. By partnering with Rapid Innovation, you can leverage our AI capabilities to achieve greater ROI and drive your business forward.
Refer to the image for a visual representation of AI-powered technical troubleshooting concepts:

1.1. Evolution of Technical Support Systems
Technical support systems have undergone significant transformations since their inception. Initially, support was primarily provided through face-to-face interactions or over the phone. As technology advanced, so did the methods of providing support.
- Early Days: Â
- Support was limited to in-person assistance or telephone calls.
- Technicians relied on manuals and personal expertise to resolve issues.
- Introduction of Help Desks: Â
- The 1980s saw the emergence of help desks, which centralized support requests.
- Ticketing systems were introduced to track and manage customer issues.
- Online Support: Â
- The rise of the internet in the 1990s allowed for email support and online forums.
- Companies began to create knowledge bases, enabling users to find solutions independently.
- Self-Service Portals: Â
- In the 2000s, self-service portals became popular, allowing users to troubleshoot issues without direct support.
- FAQs and instructional videos became common resources.
- Integration of Chatbots: Â
- The last decade has seen the integration of chatbots, providing instant responses to common queries. This includes the rise of artificial intelligence customer service solutions that enhance user interactions.
- These systems can handle multiple requests simultaneously, improving efficiency.
The evolution of technical support systems reflects the growing need for faster, more efficient solutions in an increasingly digital world. At Rapid Innovation, we leverage these advancements to provide tailored artificial intelligence in customer support and Blockchain solutions that enhance operational efficiency and customer satisfaction.
1.2. Role of AI in Modern Troubleshooting
Artificial Intelligence (AI) has revolutionized the way technical support is delivered. By automating processes and enhancing decision-making, AI plays a crucial role in modern troubleshooting.
- Predictive Analytics: Â
- AI can analyze historical data to predict potential issues before they arise, minimizing downtime and enhancing user satisfaction. Rapid Innovation employs predictive analytics to help clients proactively address challenges, leading to improved ROI.
- Natural Language Processing (NLP): Â
- AI-driven chatbots utilize NLP to understand and respond to user queries effectively, allowing for more human-like interactions and improving the user experience. Our solutions integrate NLP capabilities to enhance customer engagement and streamline support processes.
- Automated Ticketing: Â
- AI systems can automatically categorize and prioritize support tickets based on urgency and complexity, streamlining the support process and ensuring that critical issues are addressed promptly. Rapid Innovation's automated ticketing solutions help clients reduce response times and improve service quality.
- Knowledge Management: Â
- AI can analyze past support interactions to identify common problems and solutions. This information can be used to update knowledge bases, making it easier for users to find answers. We assist clients in developing robust knowledge management systems that enhance self-service capabilities.
- Continuous Learning: Â
- AI systems improve over time by learning from new data and user interactions, ensuring that support remains relevant and effective. Our continuous learning frameworks enable clients to adapt to changing customer needs and market dynamics.
The integration of AI in troubleshooting not only enhances efficiency but also allows support teams to focus on more complex issues, ultimately leading to better customer experiences and higher ROI.
1.3. Current State of AI Support Technologies
The current landscape of AI support technologies is characterized by rapid advancements and widespread adoption across various industries. These technologies are reshaping how organizations approach customer service and technical support.
- Chatbots and Virtual Assistants: Â
- Many companies now employ AI chatbots to handle routine inquiries and provide 24/7 support, resolving a significant percentage of issues without human intervention. This includes artificial intelligence customer service companies that specialize in these solutions. Rapid Innovation develops customized chatbot solutions that align with our clients' specific needs.
- Machine Learning Algorithms: Â
- Machine learning is being used to analyze user behavior and improve support interactions. These algorithms can identify patterns and suggest solutions based on previous cases. Our machine learning models help clients optimize their support strategies and enhance user satisfaction.
- Integration with CRM Systems: Â
- AI support technologies are increasingly integrated with Customer Relationship Management (CRM) systems, allowing for a more holistic view of customer interactions and enhancing service quality. Rapid Innovation facilitates seamless integration of AI technologies with existing CRM systems to improve customer insights.
- Omnichannel Support: Â
- AI technologies enable seamless support across multiple channels, including social media, email, and live chat. This omnichannel approach ensures that customers receive consistent support regardless of the platform. We help clients implement omnichannel strategies that enhance customer engagement and loyalty.
- Data Security and Privacy: Â
- As AI technologies evolve, so do concerns about data security and privacy. Organizations are implementing robust measures to protect customer information while leveraging AI capabilities. Rapid Innovation prioritizes data security in all our solutions, ensuring compliance with industry standards.
The current state of AI support technologies indicates a shift towards more intelligent, responsive, and user-friendly support systems, paving the way for future innovations in the field. At Rapid Innovation, we are committed to helping our clients navigate this landscape, achieving their business goals efficiently and effectively through our AI and Blockchain expertise, including the latest in ai support technologies.
Refer to the image for a visual representation of the evolution of technical support systems.

1.4. Transition from Traditional to AI-Enhanced Support
The transition from traditional support systems to AI-enhanced customer support represents a significant shift in how businesses interact with customers. Traditional support often relies on human agents, which can lead to longer response times and inconsistent service quality. In contrast, AI-enhanced support leverages advanced technologies to improve efficiency and customer satisfaction.
- Increased efficiency: AI systems can handle multiple inquiries simultaneously, significantly reducing wait times for customers.
- 24/7 availability: Unlike traditional support, AI can provide assistance around the clock, ensuring that customers receive help whenever they need it.
- Consistency in responses: AI can deliver uniform answers to common questions, minimizing the risk of human error and ensuring that customers receive accurate information.
- Data-driven insights: AI systems can analyze customer interactions to identify trends and areas for improvement, allowing businesses to refine their support strategies effectively.
- Cost-effectiveness: By automating routine inquiries, companies can reduce the need for large support teams, leading to substantial cost savings.
The integration of AI into support systems is not just about replacing human agents; it’s about enhancing their capabilities. Human agents can focus on more complex issues while AI handles routine tasks, creating a more efficient workflow that ultimately drives greater ROI for businesses. For more insights on this transformation, check out AI Chatbots: The Revolution in Customer Support.
2. Fundamental Technologies and Components
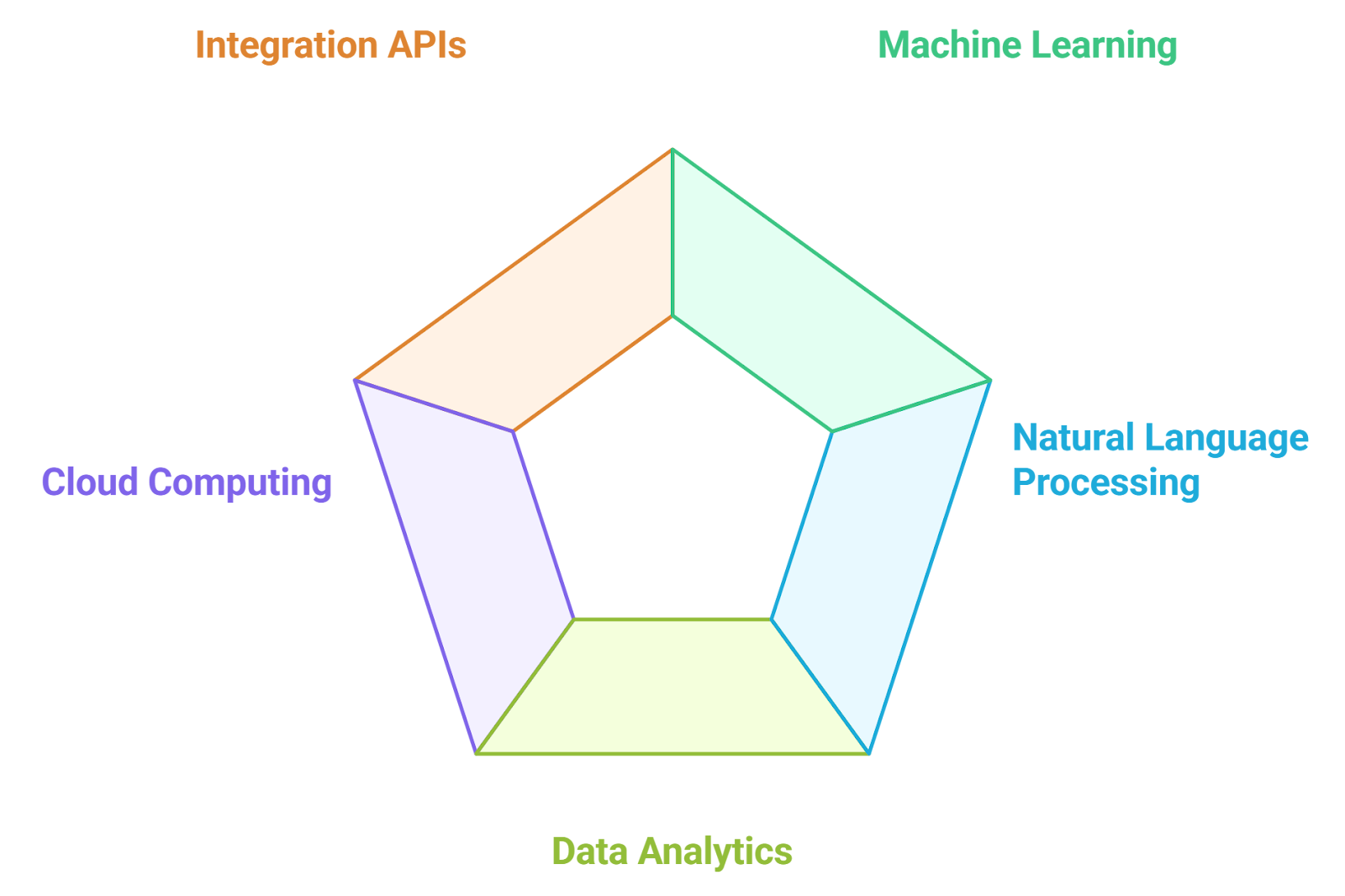
‍
Understanding the fundamental technologies and components that drive AI-enhanced support is crucial for businesses looking to implement these systems effectively. These technologies form the backbone of AI applications, enabling them to function seamlessly and deliver value.
- Machine Learning: This technology allows systems to learn from data and improve over time without explicit programming. It is essential for predictive analytics and personalizing customer interactions.
- Natural Language Processing (NLP): NLP enables machines to understand and interpret human language, making it possible for AI systems to engage in meaningful conversations with customers.
- Data Analytics: This component involves analyzing large volumes of data to extract insights that can inform business decisions and improve customer experiences.
- Cloud Computing: Cloud technology provides the infrastructure needed to store and process vast amounts of data, making AI applications scalable and accessible.
- Integration APIs: Application Programming Interfaces (APIs) facilitate the integration of AI systems with existing software, allowing for seamless communication between different platforms.
These technologies work together to create a robust AI-enhanced support system that can adapt to the needs of both businesses and customers.
2.1. Natural Language Processing (NLP) Systems
Natural Language Processing (NLP) is a critical component of AI-enhanced customer support systems. It enables machines to understand, interpret, and respond to human language in a way that is both meaningful and contextually relevant.
NLP systems can analyze text data from customer inquiries, extracting key information and intent to provide accurate responses. By assessing the emotional tone of customer messages, NLP can help businesses gauge customer satisfaction and adjust their support strategies accordingly. Additionally, NLP powers chatbots that can engage in real-time conversations with customers, answering questions and resolving issues without human intervention. It also facilitates communication across language barriers, allowing businesses to support a global customer base. Advanced NLP systems use machine learning to improve their understanding of language over time, adapting to new phrases, slang, and industry-specific terminology.
The effectiveness of NLP systems in AI-enhanced customer support is evident in their ability to provide quick, accurate, and context-aware responses, significantly improving the customer experience. As businesses continue to adopt these technologies, the role of NLP will only grow, making it an essential component of modern customer support strategies. Rapid Innovation is well-positioned to assist organizations in implementing these advanced technologies, ensuring they achieve their business goals efficiently and effectively.
Refer to the image for a visual representation of the transition from traditional support systems to AI-enhanced support.

2.2. Machine Learning Models in Troubleshooting
Machine learning models play a crucial role in troubleshooting by automating the identification and resolution of issues across various domains. These models analyze vast amounts of data to detect anomalies, predict failures, and suggest solutions, ultimately driving greater ROI for businesses.
- Predictive Maintenance: Machine learning algorithms can analyze historical data to predict when equipment is likely to fail, allowing for proactive maintenance. This not only reduces downtime but also minimizes repair costs, leading to significant savings.
- Anomaly Detection: By learning from normal operational patterns, machine learning models can identify deviations that may indicate a problem, enabling quicker responses. This rapid identification helps organizations maintain operational efficiency and customer satisfaction.
- Root Cause Analysis: Machine learning can assist in determining the underlying causes of issues by correlating various data points, leading to more effective solutions. By addressing root causes rather than symptoms, businesses can prevent recurring problems and enhance overall productivity.
- Continuous Learning: These models improve over time as they are exposed to more data, enhancing their accuracy and reliability in troubleshooting scenarios. This adaptability ensures that organizations remain agile and responsive to changing conditions.
2.3. Knowledge Base Integration
Integrating a knowledge base into troubleshooting processes enhances efficiency and effectiveness. A well-structured knowledge base serves as a repository of information that can be accessed quickly to resolve issues, ultimately contributing to improved ROI.
- Centralized Information: A knowledge base consolidates troubleshooting guides, FAQs, and best practices, making it easier for users to find solutions. This centralization reduces the time spent searching for information, allowing teams to focus on core business activities.
- Self-Service Capabilities: Users can independently search the knowledge base for answers, reducing the need for direct support and speeding up resolution times. This self-service approach empowers users and decreases operational costs associated with support services.
- Contextual Relevance: Advanced knowledge bases can provide context-aware suggestions based on the specific issues users are facing, improving the relevance of the information retrieved. This targeted assistance enhances user experience and satisfaction.
- Continuous Updates: Regularly updating the knowledge base with new findings and solutions ensures that it remains a valuable resource for troubleshooting. This ongoing improvement fosters a culture of knowledge sharing and continuous learning within the organization.
2.4. Pattern Recognition Systems
Pattern recognition systems are essential in identifying trends and recurring issues in data, which can significantly enhance troubleshooting efforts. These systems utilize algorithms to detect patterns that may not be immediately obvious, providing organizations with actionable insights.
- Data Analysis: Pattern recognition systems analyze large datasets to uncover hidden relationships and trends, aiding in the identification of common problems. This analysis allows businesses to address issues proactively, reducing the likelihood of future disruptions.
- Classification: By categorizing issues based on recognized patterns, these systems can streamline the troubleshooting process, allowing for quicker resolutions. This efficiency translates into reduced operational costs and improved service delivery.
- Predictive Insights: Recognizing patterns can lead to predictive insights, helping organizations anticipate potential issues before they escalate. This foresight enables businesses to allocate resources more effectively and mitigate risks.
- Enhanced Decision-Making: With the ability to recognize and analyze patterns, organizations can make informed decisions based on data-driven insights, improving overall operational efficiency. This strategic approach not only enhances performance but also drives greater ROI for the organization.
Machine learning troubleshooting techniques are integral to these processes, ensuring that organizations can effectively leverage data to improve their operations and resolve issues efficiently. For a more in-depth understanding of how these techniques work, you can refer to pattern recognition in machine learning.
Refer to the image for a visual representation of the role of machine learning models in troubleshooting.

2.5. Automated Diagnostic Tools
Automated diagnostic tools are essential in various industries, particularly in healthcare, IT, and manufacturing. These tools leverage advanced algorithms and machine learning to identify issues quickly and accurately, reducing the time and effort required for manual diagnostics. Examples of such tools include ansible monitoring and diagnostics, automated diagnostic system, and automated diagnostic tools.
- Efficiency: Automated diagnostic tools streamline the diagnostic process, allowing for faster identification of problems. This is particularly crucial in healthcare, where timely diagnosis can significantly impact patient outcomes.
- Accuracy: By utilizing data-driven approaches, these tools minimize human error. For instance, in IT, automated diagnostic tools can analyze system logs and performance metrics to pinpoint issues that may not be immediately apparent to human technicians. The b&r system diagnostic manager is one such tool that enhances accuracy in diagnostics.
- Cost-Effectiveness: Reducing the need for extensive manual diagnostics can lead to significant cost savings. Organizations can allocate resources more effectively, focusing on critical areas that require human intervention. Vehicle automated diagnostic systems are examples of tools that help in this regard.
- Scalability: Automated tools can handle large volumes of data, making them suitable for organizations of all sizes. They can adapt to increasing workloads without a corresponding increase in labor costs.
- Integration: Many automated diagnostic tools can be integrated with existing systems, enhancing their functionality without requiring a complete overhaul of current processes. Automated diagnostics and analytics for buildings exemplify this integration capability. For more information on how we can assist with these solutions, visit our Enterprise AI Development page. For insights on the role of predictive analytics in healthcare, check out our article on advanced AI in healthcare.
2.6. Predictive Analysis Frameworks
Predictive analysis frameworks are designed to forecast future events based on historical data. These frameworks utilize statistical algorithms and machine learning techniques to identify patterns and trends, enabling organizations to make informed decisions.
- Data-Driven Insights: Predictive analysis frameworks rely on vast amounts of data to generate insights. By analyzing historical data, organizations can identify trends that inform future strategies.
- Risk Management: These frameworks help organizations anticipate potential risks and challenges. For example, in finance, predictive analytics can identify potential defaults on loans, allowing institutions to take proactive measures.
- Enhanced Decision-Making: By providing actionable insights, predictive analysis frameworks empower decision-makers to act strategically. This can lead to improved operational efficiency and better resource allocation.
- Customer Behavior Prediction: In marketing, predictive analytics can forecast customer behavior, enabling businesses to tailor their offerings and improve customer satisfaction.
- Continuous Improvement: Predictive analysis frameworks can evolve over time, learning from new data to enhance their accuracy and effectiveness. This adaptability is crucial in fast-paced industries.
3. Core Use Cases
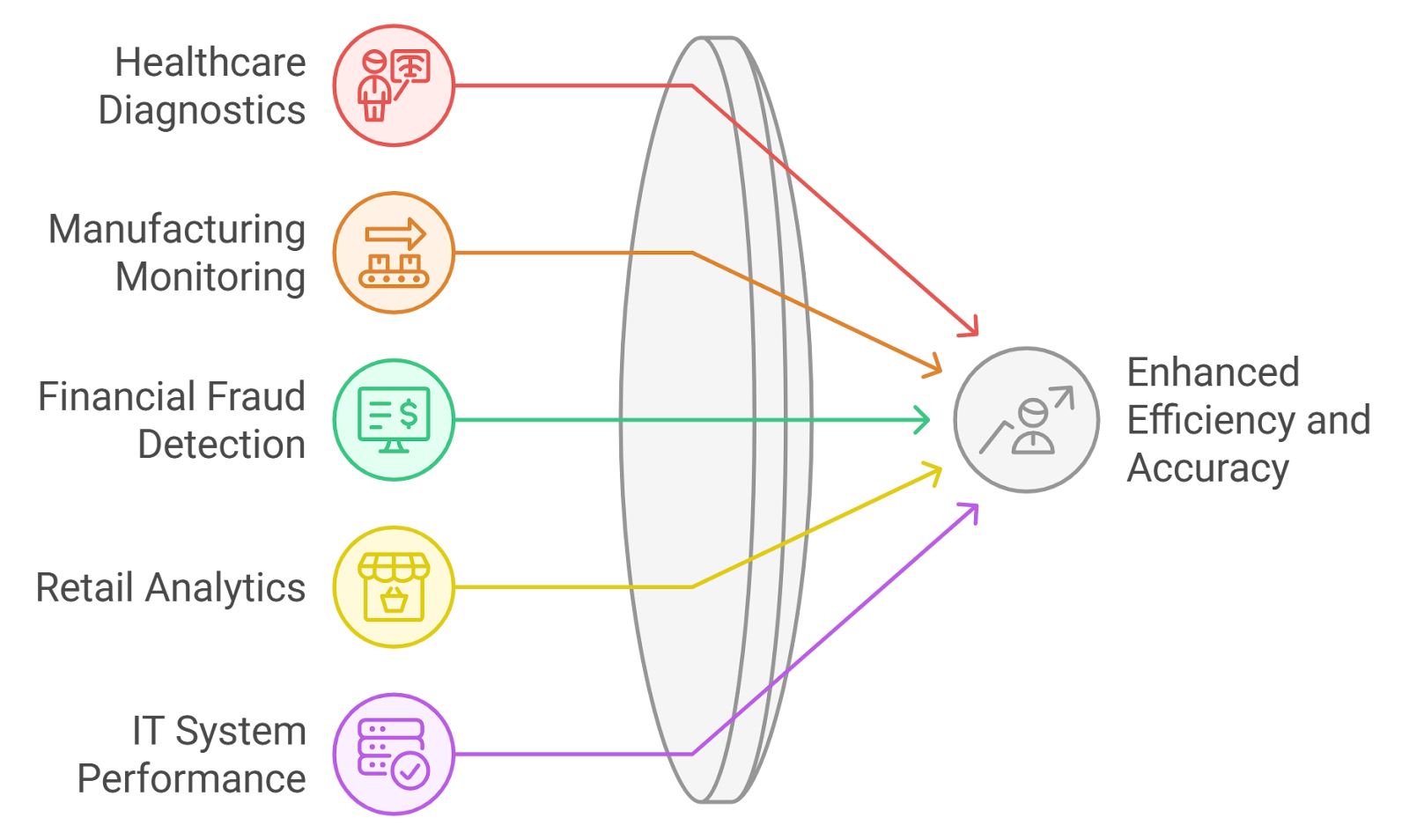
‍
Core use cases for automated diagnostic tools and predictive analysis frameworks span various sectors, showcasing their versatility and effectiveness.
- Healthcare: Automated diagnostic tools can analyze medical images, lab results, and patient histories to assist healthcare professionals in diagnosing conditions more accurately and quickly. Predictive analytics can forecast patient outcomes, helping in resource allocation and treatment planning.
- Manufacturing: In manufacturing, automated diagnostic tools monitor equipment performance, predicting failures before they occur. Predictive analytics can optimize supply chain management by forecasting demand and identifying potential disruptions.
- Finance: Automated diagnostic tools in finance can analyze transaction data to detect fraudulent activities. Predictive analysis frameworks can assess credit risk, helping lenders make informed decisions about loan approvals.
- Retail: Retailers use predictive analytics to understand customer preferences and optimize inventory management. Automated diagnostic tools can monitor sales data in real-time, identifying trends and anomalies that require immediate attention.
- IT Operations: In IT, automated diagnostic tools can monitor system performance and identify potential issues before they escalate. Predictive analytics can forecast system failures, allowing for proactive maintenance and minimizing downtime.
These use cases illustrate the transformative potential of automated diagnostic tools and predictive analysis frameworks across various industries, driving efficiency, accuracy, and informed decision-making. At Rapid Innovation, we harness the power of AI and blockchain technologies to implement these solutions, ensuring our clients achieve greater ROI and operational excellence.
3.1. Network Troubleshooting
Network troubleshooting is a critical process that involves diagnosing and resolving issues within a network. Effective troubleshooting ensures that network services are reliable and efficient, minimizing downtime and enhancing user experience. The process typically includes identifying symptoms, isolating the problem, and implementing solutions.
- Importance of network troubleshooting:
- Ensures continuous network availability
- Enhances performance and user satisfaction
- Reduces operational costs by preventing prolonged outages
3.1.1. Connectivity Issues
Connectivity issues are among the most common problems faced in network environments, such as wifi not working or xfinity wifi not working. These issues can stem from various sources, including hardware failures, configuration errors, or external factors. Identifying and resolving connectivity problems, like connected to wifi network but no internet, is essential for maintaining a functional network.
- Common causes of connectivity issues: Â
- Faulty hardware: (e.g., routers, switches, cables)
- Incorrect network configurations: (e.g., IP address conflicts)
- Network congestion or bandwidth limitations
- Firewall settings blocking access
- External factors: like ISP outages, which can lead to internet access problems.
- Steps to troubleshoot connectivity issues: Â
- Check physical connections: Ensure all cables are securely connected and devices are powered on.
- Use ping tests: Ping the target device to check for response and latency.
- Verify IP configurations: Use commands like
ipconfig
(Windows) orifconfig
(Linux) to check IP settings. - Examine network devices: Access router and switch logs for error messages or alerts.
- Test with different devices: Isolate the issue by connecting other devices to the network.
- Tools for diagnosing connectivity issues: Â
- Network analyzers: (e.g., Wireshark)
- Command-line tools: (e.g., traceroute, nslookup)
- Network monitoring software: (e.g., SolarWinds)
3.1.2. Performance Optimization
Performance optimization focuses on enhancing the speed and efficiency of a network. Slow network performance can lead to frustration among users and hinder productivity. Identifying bottlenecks and implementing optimization strategies is crucial for maintaining a high-performing network, especially when dealing with issues like my internet is not working or spectrum internet is not working.
- Factors affecting network performance: Â
- Bandwidth limitations: Insufficient bandwidth can lead to slow data transfer rates.
- Latency: High latency can cause delays in data transmission.
- Packet loss: Loss of data packets can result in retransmissions and slow performance.
- Network congestion: Too many devices on the network can lead to traffic jams.
- Strategies for optimizing network performance: Â
- Upgrade bandwidth: Consider increasing the internet connection speed or upgrading hardware.
- Implement Quality of Service (QoS): Prioritize critical applications to ensure they receive adequate bandwidth.
- Optimize network configurations: Adjust settings on routers and switches for better performance.
- Use caching: Implement caching solutions to reduce load times for frequently accessed data.
- Monitor network traffic: Regularly analyze traffic patterns to identify and address congestion points.
- Tools for performance optimization: Â
- Bandwidth monitoring tools: (e.g., PRTG Network Monitor)
- Network performance management software: (e.g., NetFlow Analyzer)
- Load testing tools: (e.g., Apache JMeter)
By addressing connectivity issues, such as wifi troubleshooting and spectrum internet troubleshooting, and focusing on performance optimization, organizations can ensure a robust and efficient network that meets the demands of users and applications. At Rapid Innovation, we leverage our expertise in AI and Blockchain technologies to enhance network troubleshooting processes, enabling clients to achieve greater ROI through improved operational efficiency and reduced downtime. Our tailored solutions can help organizations implement advanced monitoring systems and predictive analytics, ensuring proactive management of network performance and connectivity challenges, including network diagnosis and troubleshooting spectrum internet. For more information, visit our AI agents for network monitoring.
3.1.3. Security Incident Response
Security incident response is a critical aspect of cybersecurity management. It involves a structured approach to handle and mitigate the impact of security breaches or attacks. An effective incident response plan, such as a cybersecurity incident response plan or a sample incident response plan, can significantly reduce the damage caused by security incidents.
- Key components of a security incident response plan include:
- Preparation: Establishing policies, procedures, and tools to respond to incidents, including the development of an incident response in cyber security framework.
- Detection and Analysis: Identifying potential security incidents through monitoring and analysis of security alerts, which is a key function of a CSIRT (Computer Security Incident Response Team).
- Containment: Implementing measures to limit the impact of the incident on systems and data.
- Eradication: Removing the root cause of the incident to prevent recurrence, as outlined in the NIST incident response guidelines.
- Recovery: Restoring affected systems and services to normal operation, following the protocols in the NIST SP 800 61.
- Post-Incident Review: Analyzing the incident to improve future response efforts, which is essential for refining the security incident response plan.
Organizations should regularly test their incident response plans through simulations and drills, including those based on the NIST security incident response framework. This ensures that all team members are familiar with their roles and responsibilities during an actual incident. Additionally, maintaining clear communication channels is essential for effective incident management. At Rapid Innovation, we leverage AI-driven analytics to enhance detection and analysis, enabling organizations to respond swiftly and effectively to incidents, thereby minimizing potential losses and maximizing ROI.
3.2. Hardware Diagnostics
Hardware diagnostics refer to the process of testing and evaluating the physical components of a computer or network system. This is crucial for identifying issues that may affect performance, reliability, and security. Regular hardware diagnostics can help prevent unexpected failures and extend the lifespan of equipment.
- Common hardware diagnostic tools and techniques include:
- POST (Power-On Self-Test): A built-in diagnostic test that runs when a computer is powered on.
- Diagnostic Software: Programs that can run tests on various hardware components, such as CPU, RAM, and hard drives.
- Visual Inspection: Checking for physical damage, loose connections, or signs of wear and tear.
Performing hardware diagnostics can lead to early detection of potential failures, improved system performance, and reduced downtime and maintenance costs.
3.2.1. Component Failure Detection
Component failure detection is a vital part of hardware diagnostics. It involves identifying when a specific hardware component is malfunctioning or has failed. Early detection can prevent further damage and ensure system reliability.
- Techniques for component failure detection include:
- SMART (Self-Monitoring, Analysis, and Reporting Technology): A monitoring system for hard drives that predicts failures based on various metrics.
- Temperature Monitoring: Keeping track of component temperatures to prevent overheating.
- Error Checking: Utilizing error-checking codes (ECC) in memory to detect and correct data corruption.
The benefits of effective component failure detection include minimizing the risk of data loss, enhancing system stability and performance, and reducing repair costs by addressing issues before they escalate. Rapid Innovation employs advanced diagnostic tools powered by AI to streamline this process, ensuring that organizations can maintain optimal hardware performance and achieve greater ROI. Incorporating regular diagnostics and failure detection into maintenance routines can significantly improve the overall health of hardware systems.
3.2.2. Performance Analysis
Performance analysis is a critical aspect of evaluating the efficiency and effectiveness of systems, processes, or equipment. It involves collecting and analyzing data to understand how well a system is functioning and identifying areas for improvement.
- Key metrics to consider include: Â
- Response time: How quickly a system reacts to inputs.
- Throughput: The amount of work completed in a given time frame.
- Resource utilization: How effectively resources (CPU, memory, etc.) are being used.
- Techniques for performance analysis: Â
- Benchmarking: Comparing performance metrics against industry standards or competitors.
- Profiling: Identifying bottlenecks in processes or systems to optimize performance.
- Load testing: Simulating high-demand scenarios to assess system behavior under stress, such as site load test.
- Tools for performance analysis: Â
- Application Performance Monitoring (APM) tools: These provide real-time insights into application performance, including tools like power bi performance analyzer.
- Log analysis tools: They help in understanding system behavior by analyzing logs generated by applications.
- Network monitoring tools: These track network performance and identify issues affecting data transmission, similar to windows performance analyser.
Regular performance analysis can lead to enhanced user experience by reducing latency and improving response times, increased operational efficiency through optimized resource allocation, and better decision-making based on data-driven insights. At Rapid Innovation, we leverage advanced AI algorithms to automate performance analysis, including AI agents in software testing, python performance analysis and performance analysis visual studio, ensuring that our clients can swiftly identify and rectify inefficiencies, ultimately driving greater ROI.
3.2.3. Predictive Maintenance
Predictive maintenance is an advanced maintenance strategy that uses data analysis to predict when equipment failure might occur. This approach allows organizations to perform maintenance at the right time, reducing downtime and maintenance costs.
- Key components of predictive maintenance: Â
- Data collection: Gathering data from sensors, equipment logs, and historical maintenance records.
- Data analysis: Using statistical methods and machine learning algorithms to identify patterns and predict failures.
- Condition monitoring: Continuously monitoring equipment health to detect anomalies.
- Benefits of predictive maintenance: Â
- Reduced downtime: By predicting failures, organizations can schedule maintenance before issues arise.
- Cost savings: Preventing unexpected breakdowns can significantly lower repair costs and extend equipment lifespan.
- Improved safety: Regular monitoring and timely maintenance reduce the risk of accidents caused by equipment failure.
- Technologies enabling predictive maintenance: Â
- Internet of Things (IoT): Sensors collect real-time data from equipment, providing insights into performance.
- Artificial Intelligence (AI): Machine learning algorithms analyze data to predict potential failures.
- Cloud computing: Facilitates data storage and processing, allowing for scalable predictive maintenance solutions.
Implementing predictive maintenance can lead to increased operational efficiency by minimizing unplanned downtime, enhanced asset management through better tracking of equipment health, and improved return on investment (ROI) by optimizing maintenance schedules. Rapid Innovation employs cutting-edge AI and IoT technologies to develop tailored predictive maintenance solutions that empower our clients to maximize their operational capabilities.
3.3. Software Issues
Software issues can significantly impact the performance, security, and usability of applications. Identifying and addressing these issues is crucial for maintaining system integrity and user satisfaction.
- Common software issues include: Â
- Bugs and glitches: Errors in the code that cause unexpected behavior or crashes.
- Compatibility problems: Software that does not function correctly with certain operating systems or hardware.
- Security vulnerabilities: Flaws that can be exploited by malicious actors to gain unauthorized access or cause damage.
- Strategies for addressing software issues: Â
- Regular updates: Keeping software up-to-date with the latest patches and versions to fix known issues.
- Testing: Implementing rigorous testing protocols, including unit testing, integration testing, and user acceptance testing, which may involve tools for sql query analyze.
- User feedback: Actively seeking and analyzing user feedback to identify and resolve issues quickly.
- Tools for managing software issues: Â
- Issue tracking systems: These help teams log, prioritize, and resolve software bugs and feature requests.
- Automated testing tools: They streamline the testing process, ensuring that software is reliable before deployment.
- Security assessment tools: These identify vulnerabilities and help organizations strengthen their software security posture.
Addressing software issues can lead to improved user experience by providing a stable and reliable application, enhanced security by proactively identifying and mitigating vulnerabilities, and increased productivity by minimizing disruptions caused by software failures. At Rapid Innovation, we utilize blockchain technology to enhance software security and integrity, ensuring that our clients' applications are resilient against potential threats while maximizing their operational efficiency.
3.3.1. Application Errors
Application errors are common issues that can disrupt the functionality of software programs. These errors can arise from various sources, leading to unexpected behavior or crashes. Understanding the types of application errors can help in troubleshooting and resolving them effectively.
- Types of Application Errors: Â
- Syntax Errors: Mistakes in the code that prevent the application from running.
- Runtime Errors: Issues that occur while the application is running, often due to invalid operations.
- Logic Errors: Flaws in the algorithm that lead to incorrect results, even if the application runs without crashing.
- Common Causes: Â
- Coding Mistakes: Errors made during the development process, such as typos or incorrect logic.
- Data Input Issues: Invalid or unexpected data inputs can lead to application errors.
- Resource Limitations: Insufficient memory or processing power can cause applications to fail.
- Impact of Application Errors: Â
- User Frustration: Frequent errors can lead to a poor user experience.
- Data Loss: Crashes may result in unsaved work being lost.
- Increased Support Costs: More errors can lead to higher demand for technical support.
At Rapid Innovation, we leverage AI-driven testing tools to identify and rectify these application errors early in the development cycle, ensuring a smoother user experience and reducing support costs. Our expertise in AI allows us to automate error detection, leading to faster resolution times and improved application reliability. For more complex issues, event viewer troubleshooting can be employed to gain insights into the underlying problems, while application errors troubleshooting can help in systematically addressing and resolving these errors.
3.3.2. Configuration Problems
Configuration problems occur when software settings are not correctly set up, leading to performance issues or failures. These problems can arise during installation, updates, or changes in the system environment.
- Types of Configuration Problems: Â
- Incorrect Settings: Misconfigured parameters that do not align with the software requirements.
- Missing Dependencies: Required components or libraries that are not installed or configured properly.
- Network Configuration Issues: Problems with network settings that prevent applications from communicating effectively.
- Common Causes: Â
- User Error: Mistakes made during the initial setup or subsequent changes.
- Incompatibility with Other Software: Conflicts with other applications or services that affect performance.
- Changes in Environment: Updates to the operating system or network that require reconfiguration.
- Impact of Configuration Problems: Â
- Decreased Performance: Applications may run slower or not at all.
- Increased Downtime: Configuration issues can lead to extended periods of unavailability.
- Security Vulnerabilities: Misconfigurations can expose systems to security risks.
Rapid Innovation provides comprehensive consulting services to ensure that your software configurations are optimized for performance and security. Our blockchain expertise allows us to implement decentralized configurations that enhance security and reduce the risk of misconfigurations.
3.3.3. Update and Compatibility Issues
Update and compatibility issues arise when software updates are not compatible with existing systems or applications. These problems can lead to functionality loss or system instability.
- Types of Update Issues: Â
- Failed Updates: Updates that do not install correctly, leaving the software in an unstable state.
- Incompatibility with Existing Software: New updates may not work well with older versions of applications or operating systems.
- Feature Changes: Updates that alter or remove features can disrupt user workflows.
- Common Causes: Â
- Version Mismatches: Running different versions of software that are not designed to work together.
- Insufficient Testing: Updates that are released without thorough testing can lead to unforeseen issues.
- Hardware Limitations: New updates may require hardware capabilities that older systems do not possess.
- Impact of Update and Compatibility Issues: Â
- User Disruption: Changes in functionality can frustrate users and hinder productivity.
- Increased Support Requests: Users may seek help more frequently when facing issues after updates.
- Potential Data Loss: Incompatibility can lead to data corruption or loss if not handled properly.
At Rapid Innovation, we utilize our AI capabilities to conduct rigorous testing and compatibility checks before deploying updates. This proactive approach minimizes disruptions and ensures that your systems remain stable and efficient, ultimately leading to greater ROI for your business.
3.4. Cloud Infrastructure
Cloud infrastructure refers to the collection of hardware and software components that are required to support cloud computing, including iaas (infrastructure as a service) solutions. This includes servers, storage systems, networking equipment, and virtualization software. The cloud infrastructure is essential for delivering services over the internet, enabling businesses to scale their operations, improve efficiency, and reduce costs. It provides on-demand access to computing resources, supports various deployment models (public, private, and hybrid clouds), facilitates data storage, processing, and management, enhances collaboration and remote work capabilities, and offers flexibility and scalability to meet changing business needs.
3.4.1. Service Availability
Service availability is a critical aspect of cloud infrastructure, ensuring that services are accessible to users whenever needed. High availability is achieved through redundancy, load balancing, and failover mechanisms.
- Redundancy: Multiple instances of services are deployed to prevent downtime. Â
- Load Balancing: Distributes incoming traffic across multiple servers to optimize resource use. Â
- Failover Mechanisms: Automatically switches to a backup system in case of a failure. Â
- Service Level Agreements (SLAs): Define the expected uptime and performance metrics. Â
- Monitoring Tools: Track service performance and alert administrators to issues. Â
According to a report, 99.9% uptime is often expected from cloud service providers, which translates to only about 8.76 hours of downtime per year. This level of availability is crucial for businesses that rely on cloud services for their operations. At Rapid Innovation, we leverage our expertise in cloud infrastructure, including iaas infrastructure as service and cloud computing service providers, to ensure that our clients experience minimal downtime, thereby enhancing their operational efficiency and customer satisfaction.
3.4.2. Resource Optimization
Resource optimization in cloud infrastructure involves efficiently managing and allocating resources to maximize performance while minimizing costs. This is essential for businesses to ensure they are getting the most out of their cloud investments.
- Auto-scaling: Automatically adjusts resources based on demand, ensuring optimal performance during peak times and cost savings during low usage. Â
- Cost Management Tools: Help track and analyze cloud spending, identifying areas for potential savings. Â
- Virtualization: Allows multiple virtual machines to run on a single physical server, improving resource utilization. Â
- Performance Monitoring: Continuously assesses resource usage and application performance to identify bottlenecks. Â
- Right-sizing: Involves adjusting the size of cloud resources to match actual usage, preventing over-provisioning. Â
Effective resource optimization can lead to significant cost savings. For instance, businesses can reduce their cloud spending by up to 30% through proper resource management and optimization strategies. At Rapid Innovation, we implement tailored resource optimization strategies that not only enhance performance but also drive greater ROI for our clients, ensuring they achieve their business goals efficiently and effectively. This includes leveraging private cloud solutions and cloud based infrastructure to optimize resource allocation and management. Additionally, our expertise in generative AI consulting can further enhance your cloud infrastructure capabilities.
3.4.3. Cost Management
Cost management is a critical aspect of any project or business operation, ensuring that resources are used efficiently and effectively. It involves planning, estimating, budgeting, and controlling costs to keep the project within its financial constraints. Effective cost management can lead to increased profitability and sustainability.
- Planning: This is the first step in cost management, where the financial resources required for the project are identified. It involves defining the project scope and objectives, identifying all potential costs associated with the project, and developing a cost management plan that outlines how costs will be managed throughout the project lifecycle. At Rapid Innovation, we leverage AI-driven analytics to enhance the planning phase, allowing for more accurate forecasting and resource allocation. This aligns with various cost management strategies that organizations can adopt.
- Estimating: Accurate cost estimation is vital for successful project execution. This involves analyzing historical data and using estimation techniques such as analogous estimating, parametric estimating, and bottom-up estimating. It also includes considering direct costs (labor, materials) and indirect costs (overhead, administrative expenses), as well as utilizing software tools for more precise calculations. Our expertise in AI can automate and refine these estimation processes, leading to more reliable budgets and reduced financial risk. This is particularly important in strategic cost management and control.
- Budgeting: Once costs are estimated, the next step is to create a budget. This includes allocating funds to different project components based on the estimates, establishing a baseline for measuring project performance, and ensuring that the budget aligns with the overall financial goals of the organization. Rapid Innovation employs blockchain technology to enhance transparency in budgeting, ensuring that all stakeholders have real-time access to financial data and can track expenditures effectively. This is essential for implementing effective cost control strategies.
- Controlling Costs: This involves monitoring project expenditures and performance against the budget. Key activities include regularly reviewing financial reports to track spending, implementing corrective actions if costs exceed the budget, and using techniques like Earned Value Management (EVM) to assess project performance. Our solutions integrate AI for predictive analytics, enabling proactive cost control measures that can significantly improve ROI. This is where strategic cost management examples can be applied to enhance overall project performance.
Effective cost management not only helps in keeping projects on track but also enhances decision-making and resource allocation. By focusing on cost control, organizations can improve their financial health and achieve their strategic objectives, including the need for strategic cost management. For more insights on leveraging AI in cost management, check out our AI Development and Integration Guide for Individuals.
4. Implementation Strategies
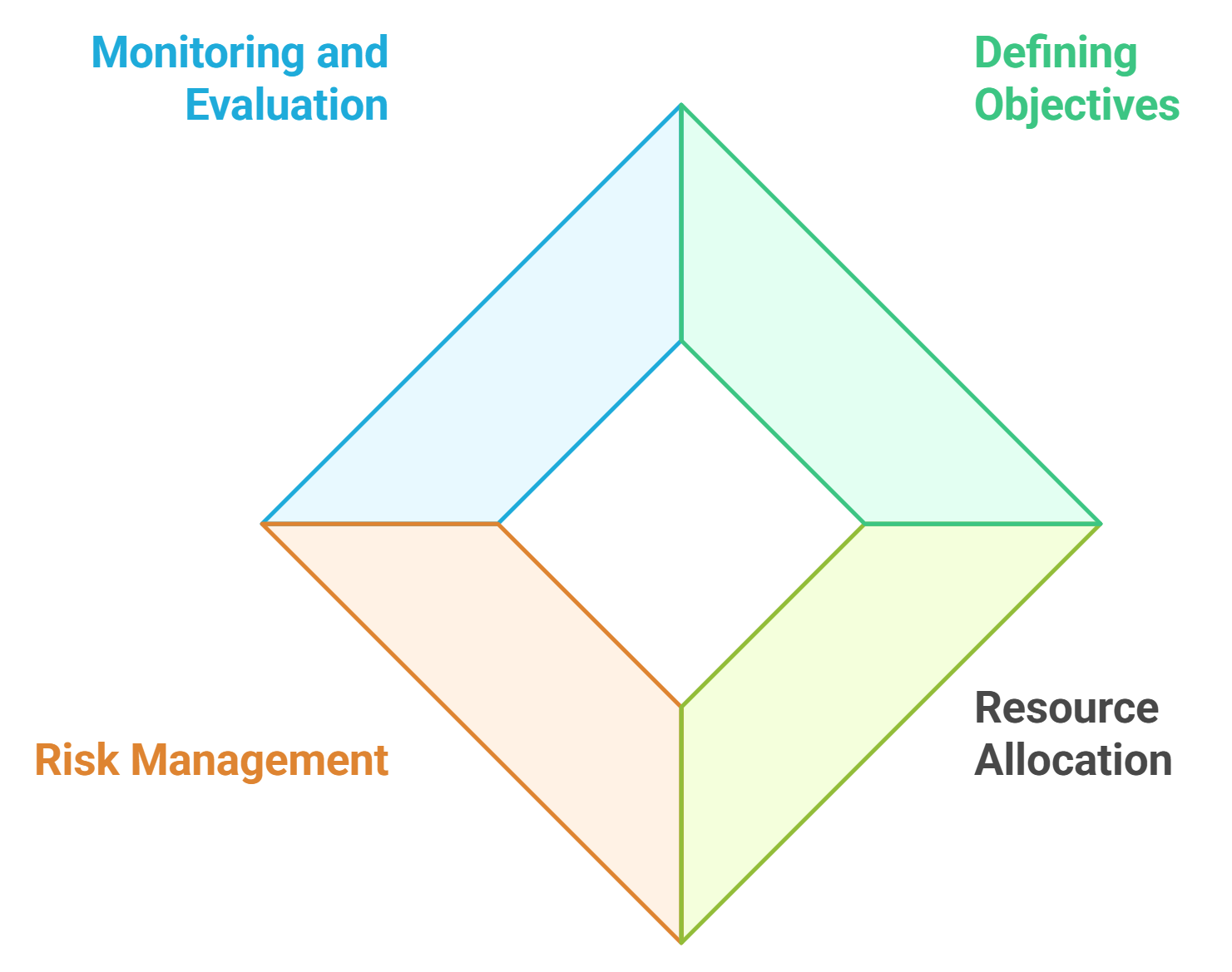
‍
Implementation strategies are essential for translating plans into action. They provide a roadmap for executing projects and achieving desired outcomes. A well-defined implementation strategy ensures that resources are utilized efficiently and that stakeholders are aligned with the project goals.
- Defining Objectives: Clear objectives guide the implementation process. This involves setting specific, measurable, achievable, relevant, and time-bound (SMART) goals and communicating these objectives to all stakeholders to ensure alignment.
- Resource Allocation: Effective resource allocation is crucial for successful implementation. This includes identifying the necessary resources (human, financial, technological), assigning roles and responsibilities to team members, and ensuring that resources are available when needed to avoid delays.
- Risk Management: Identifying and mitigating risks is a key component of implementation strategies. This involves conducting a risk assessment to identify potential challenges, developing a risk management plan that outlines strategies for addressing risks, and monitoring risks throughout the implementation process and adjusting plans as necessary. This is particularly relevant when considering the best strategy to mitigate cost risk.
- Monitoring and Evaluation: Continuous monitoring and evaluation help ensure that the project stays on track. This includes establishing key performance indicators (KPIs) to measure progress, conducting regular reviews to assess performance against objectives, and making data-driven decisions to improve project outcomes.
4.1. Assessment and Planning
Assessment and planning are foundational steps in the implementation process. They involve evaluating the current situation and developing a comprehensive plan to guide project execution.
- Situation Analysis: Understanding the current environment is crucial for effective planning. This involves conducting a SWOT analysis (Strengths, Weaknesses, Opportunities, Threats) to identify internal and external factors that may impact the project and gathering data on market trends, competitor activities, and stakeholder needs.
- Stakeholder Engagement: Engaging stakeholders early in the planning process is essential. This includes identifying key stakeholders and understanding their interests and concerns, involving stakeholders in the planning process to gain their insights and buy-in, and communicating the project vision and objectives to foster collaboration.
- Developing a Detailed Plan: A comprehensive plan serves as a blueprint for implementation. This includes outlining the project scope, objectives, timelines, and deliverables, creating a detailed work breakdown structure (WBS) to organize tasks and responsibilities, and establishing a communication plan to keep stakeholders informed throughout the project.
- Resource Planning: Identifying and planning for the resources needed is critical. This involves estimating the budget and securing funding, identifying the skills and expertise required for the project team, and planning for training and development to ensure team members are equipped to succeed. This is where cost reduction strategies in supply chain management can play a significant role.
By conducting thorough assessments and developing detailed plans, organizations can set the stage for successful project implementation. This proactive approach minimizes risks and enhances the likelihood of achieving project goals, including the implementation of effective pricing strategies in supply chain management.
4.1.1. Infrastructure Requirements
Infrastructure requirements are critical for the successful deployment and operation of any system or application. These requirements encompass both hardware and software components that ensure optimal performance, security, and scalability.
- Hardware Specifications: Â
- Servers: High-performance servers are essential for processing large volumes of data, particularly in AI applications where real-time data analysis is crucial for decision-making.
- Storage: Adequate storage solutions, such as SSDs or cloud storage, are necessary to handle data efficiently, especially for blockchain applications that require immutable data storage.
- Network: A robust network infrastructure, including routers and switches, is vital for seamless connectivity, ensuring that data flows smoothly between AI models and blockchain nodes.
- Software Requirements: Â
- Operating Systems: Compatibility with various operating systems (Windows, Linux, etc.) is crucial to support diverse development environments.
- Middleware: Software that connects different applications and services is necessary for smooth operations, particularly in integrating AI algorithms with blockchain platforms.
- Security Software: Firewalls, antivirus, and intrusion detection systems are essential to protect data and infrastructure, safeguarding sensitive information in both AI and blockchain applications.
- Scalability: The infrastructure should be designed to scale easily as demand increases, allowing for the addition of resources without significant downtime, which is particularly important for businesses leveraging AI and blockchain technologies.
- Backup and Recovery: Implementing a reliable backup and recovery system is essential to prevent data loss and ensure business continuity, especially in environments where data integrity is paramount, such as in blockchain systems.
4.1.2. Data Requirements
Data requirements define the types and formats of data needed for a system to function effectively. Understanding these requirements is crucial for data management, analysis, and compliance.
- Data Types: Â
- Structured Data: Organized data that fits into predefined models, such as databases, is essential for AI algorithms that rely on structured inputs for training and inference.
- Unstructured Data: Data that does not have a specific format, such as emails, videos, and social media content, can be harnessed by AI for insights and predictions.
- Semi-structured Data: Data that does not conform to a rigid structure but still contains tags or markers, like XML or JSON files, is often used in both AI and blockchain applications for data interchange.
- Data Volume: Estimating the volume of data that will be processed is essential for planning storage and processing capabilities. For instance, organizations may deal with terabytes or petabytes of data, necessitating robust infrastructure to support AI analytics and blockchain transactions.
- Data Quality: Ensuring high data quality is vital for accurate analysis and decision-making. This includes data accuracy, completeness, consistency, and timeliness, which are critical for both AI model performance and blockchain integrity. Ensuring high data quality is vital for accurate analysis and decision-making.
- Data Governance: Establishing data governance policies helps manage data integrity, security, and compliance with regulations such as GDPR or HIPAA, ensuring that both AI and blockchain applications adhere to legal standards.
- Data Integration: The ability to integrate data from various sources is crucial for comprehensive analysis and reporting. This may involve using ETL (Extract, Transform, Load) processes or data lakes, facilitating the seamless flow of data between AI systems and blockchain networks.
4.1.3. Integration Points
Integration points refer to the specific locations or methods through which different systems, applications, or components connect and communicate with each other. Identifying these points is essential for ensuring seamless data flow and functionality.
- APIs (Application Programming Interfaces): APIs are critical for enabling communication between different software applications. They allow for data exchange and functionality sharing, particularly between AI services and blockchain platforms.
- Data Connectors: Utilizing data connectors can facilitate the integration of various data sources, such as databases, cloud services, and third-party applications, enhancing the interoperability of AI and blockchain solutions.
- Middleware Solutions: Middleware acts as a bridge between different applications, allowing them to communicate and share data effectively, which is essential for integrating AI-driven insights with blockchain transactions.
- Webhooks: Webhooks enable real-time data transfer between applications by sending automated messages when specific events occur, ensuring timely updates in both AI and blockchain systems.
- Batch Processing: For systems that require processing large volumes of data at once, batch processing integration points can be established to handle data in scheduled intervals, optimizing performance for both AI analytics and blockchain operations.
- User Interfaces: Integration points may also include user interfaces that allow users to interact with multiple systems seamlessly, enhancing user experience and accessibility in AI and blockchain applications.
- Monitoring and Logging: Implementing monitoring and logging at integration points helps track data flow and identify issues quickly, ensuring system reliability and performance in both AI and blockchain environments.
4.2. Agent Training Methods
Agent training methods are crucial in developing intelligent systems capable of performing tasks autonomously. These methods can be broadly categorized into various approaches, with agent training methods such as supervised learning and reinforcement learning being two of the most prominent. Each method has its unique characteristics, advantages, and applications.
4.2.1. Supervised Learning
Supervised learning is a machine learning paradigm where an agent learns from labeled data. In this approach, the model is trained on a dataset that contains input-output pairs, allowing it to learn the relationship between the inputs and the corresponding outputs.
- The training process involves: Â
- Feeding the model a set of input data along with the correct output labels.
- The model makes predictions based on the input data.
- The predictions are compared to the actual labels, and the model's parameters are adjusted to minimize the error.
- Key characteristics of supervised learning include: Â
- Requires a large amount of labeled data, which can be time-consuming and expensive to obtain.
- The performance of the model is heavily dependent on the quality of the training data.
- Common algorithms used in supervised learning include linear regression, decision trees, and support vector machines.
- Applications of supervised learning are vast and include: Â
- Image recognition, where models are trained to identify objects within images.
- Natural language processing, enabling machines to understand and generate human language.
- Fraud detection, where models learn to identify fraudulent transactions based on historical data.
Supervised learning is particularly effective when the relationship between input and output is well-defined, making it a popular choice in many fields. However, its reliance on labeled data can be a significant limitation. At Rapid Innovation, we leverage supervised learning to help clients enhance their data-driven decision-making processes, ultimately leading to greater ROI through improved accuracy and efficiency in their operations.
4.2.2. Reinforcement Learning
Reinforcement learning (RL) is a different approach where an agent learns to make decisions by interacting with an environment. Instead of relying on labeled data, the agent learns through trial and error, receiving feedback in the form of rewards or penalties based on its actions.
- The key components of reinforcement learning include: Â
- Agent: The learner or decision-maker.
- Environment: The context in which the agent operates.
- Actions: The choices available to the agent.
- Rewards: Feedback received after taking an action, guiding the agent's learning process.
- The training process involves: Â
- The agent explores the environment by taking actions.
- It receives rewards or penalties based on the outcomes of those actions.
- The agent updates its strategy to maximize cumulative rewards over time.
- Key characteristics of reinforcement learning include: Â
- Does not require labeled data, making it suitable for complex problems where the optimal solution is not known in advance.
- The learning process is often more dynamic, as the agent continuously adapts to changes in the environment.
- Common algorithms used in reinforcement learning include Q-learning, deep Q-networks (DQN), and policy gradients.
- Applications of reinforcement learning are diverse and include: Â
- Game playing, where agents learn to play games like chess or Go at superhuman levels.
- Robotics, enabling robots to learn complex tasks through interaction with their environment.
- Autonomous vehicles, where RL helps in decision-making processes for navigation and obstacle avoidance.
Reinforcement learning is particularly powerful in scenarios where the environment is complex and dynamic, allowing agents to learn optimal strategies through experience. However, it can require significant computational resources and time to converge to an effective policy. At Rapid Innovation, we harness the potential of reinforcement learning to develop adaptive systems that can optimize processes in real-time, driving efficiency and maximizing ROI for our clients. For more information on our services, check out our AI EdTech solutions and our complete guide to machine learning.
4.2.3. Transfer Learning
Transfer learning is a machine learning technique that allows a model trained on one task to be adapted for a different but related task. This approach is particularly useful in scenarios where labeled data is scarce or expensive to obtain. By leveraging knowledge gained from a pre-trained model, transfer learning can significantly reduce the time and resources needed for training.
- Pre-trained models: These are models that have been trained on large datasets, such as ImageNet for image classification tasks. They can be fine-tuned for specific applications, such as medical image analysis or facial recognition. Applications of transfer learning include computer vision transfer learning, where models are adapted for tasks like transfer learning for medical image classification and transfer learning for object detection.
- Reduced training time: Since the model has already learned useful features from the original dataset, only a small amount of additional training is needed to adapt it to the new task. For example, using keras transfer learning image classification can streamline the process of training models for specific image tasks.
- Improved performance: Transfer learning often leads to better performance on the target task, especially when the target dataset is small. This is because the model can utilize the generalized features learned from the larger dataset. Techniques like deep learning image style transfer and neural style transfer tutorial demonstrate how transfer learning can enhance performance in creative applications.
- Applications: Transfer learning is widely used in various fields, including natural language processing (NLP), computer vision, and speech recognition. For instance, models like BERT and GPT-3 in NLP have shown remarkable results by transferring knowledge from large corpora of text. In computer vision, transfer learning algorithms and applications are crucial for tasks such as transfer learning for image classification using keras and transfer learning in medical imaging.
4.3. Deployment Models
Deployment models refer to the various ways in which machine learning models can be implemented and made available for use. The choice of deployment model can significantly impact the performance, scalability, and accessibility of the machine learning solution.
- Cloud-based deployment: This model involves hosting the machine learning model on cloud platforms, allowing for easy scalability and access from anywhere. It is ideal for applications requiring high computational power and storage.
- Edge deployment: In this model, machine learning models are deployed on local devices, such as smartphones or IoT devices. This approach reduces latency and bandwidth usage, making it suitable for real-time applications.
- Hybrid deployment: A combination of cloud and edge deployment, this model allows for flexibility and optimization. Critical tasks can be processed on the edge, while less time-sensitive tasks can be handled in the cloud.
4.3.1. On-Premise Solutions
On-premise solutions refer to the deployment of machine learning models within an organization's own infrastructure. This model offers several advantages and challenges that organizations must consider when deciding on their deployment strategy.
- Data security: On-premise solutions provide greater control over sensitive data, as it remains within the organization's infrastructure. This is particularly important for industries like healthcare and finance, where data privacy is paramount.
- Customization: Organizations can tailor the deployment environment to meet specific needs, including hardware configurations and software integrations. This flexibility can lead to optimized performance for particular applications.
- Cost considerations: While on-premise solutions may require significant upfront investment in hardware and software, they can be more cost-effective in the long run for organizations with high usage levels.
- Maintenance and support: Organizations are responsible for maintaining their on-premise infrastructure, which can require dedicated IT resources. This can be a challenge for smaller organizations with limited technical expertise.
- Scalability: Scaling on-premise solutions can be more complex compared to cloud-based options, as it often involves purchasing additional hardware and managing capacity planning.
In conclusion, transfer learning and deployment models, including on-premise solutions, play crucial roles in the successful implementation of machine learning applications. Understanding these concepts helps organizations make informed decisions that align with their specific needs and resources. At Rapid Innovation, we leverage these advanced techniques to help our clients achieve greater ROI by optimizing their machine learning strategies and ensuring that their deployment models align with their business objectives.
4.3.2. Cloud-Based Systems
Cloud-based systems have revolutionized the way businesses operate by providing scalable, flexible, and cost-effective solutions. These systems allow organizations to store and manage data on remote servers, accessible via the internet.
- Scalability: Cloud services can easily scale up or down based on demand, allowing businesses to adjust resources without significant upfront investments. Rapid Innovation assists clients in implementing cloud solutions that align with their growth trajectories, ensuring they only pay for what they use. This includes options like cloud based phone systems and cloud based erp solutions.
- Cost Efficiency: Organizations can reduce costs associated with hardware, maintenance, and energy consumption by utilizing cloud services. Pay-as-you-go models further enhance financial flexibility. Our consulting services help clients identify the most cost-effective cloud solutions tailored to their specific needs, such as cloud based server cost analysis and cloud based accounting software.
- Accessibility: Employees can access data and applications from anywhere, promoting remote work and collaboration. This is particularly beneficial in today’s globalized work environment. Rapid Innovation enables seamless integration of cloud systems, enhancing collaboration across teams and locations, including cloud based contact center solutions.
- Automatic Updates: Cloud providers regularly update their systems, ensuring that businesses always have access to the latest features and security patches without manual intervention. We guide clients in selecting cloud providers that prioritize security and compliance, safeguarding their data, especially with cloud based security solutions.
- Disaster Recovery: Many cloud services offer built-in backup and recovery options, minimizing data loss risks and ensuring business continuity. Rapid Innovation helps clients develop robust disaster recovery plans, leveraging cloud capabilities to protect critical business data, including cloud based identity management solutions.
Cloud-based systems are particularly advantageous for small to medium-sized enterprises (SMEs) that may lack the resources for extensive IT infrastructure. By leveraging cloud technology, these businesses can compete more effectively with larger organizations, utilizing solutions like cloud based servers for small business and cloud based inventory management software.
4.3.3. Hybrid Approaches
Hybrid approaches combine the benefits of both cloud-based and on-premises systems, allowing organizations to tailor their IT infrastructure to meet specific needs. This flexibility is increasingly appealing to businesses looking to optimize performance and security.
- Flexibility: Organizations can choose which applications and data to host in the cloud and which to keep on-premises, allowing for a customized approach that meets unique operational requirements. Rapid Innovation collaborates with clients to design hybrid solutions that align with their operational goals, including cloud based erp and cloud based dbms.
- Enhanced Security: Sensitive data can be stored on-premises while less critical applications can be moved to the cloud, providing a balance between accessibility and security. Our expertise in blockchain technology further enhances data security, ensuring that sensitive information is protected through decentralized solutions, particularly in cloud based legal case management software.
- Cost Management: Hybrid systems can help manage costs by allowing businesses to utilize cloud resources for peak loads while maintaining core operations on-premises. We assist clients in optimizing their resource allocation, ensuring maximum ROI, especially with cloud based erps and cloud based business management software.
- Seamless Integration: Hybrid approaches facilitate the integration of existing systems with new cloud services, enabling businesses to leverage their current investments while adopting new technologies. Rapid Innovation specializes in creating integration strategies that enhance operational efficiency, including cloud based software and cloud based pos systems.
- Improved Performance: By strategically placing workloads where they perform best, organizations can enhance overall system performance and user experience. Our team analyzes client workloads to recommend optimal configurations that drive performance improvements, utilizing cloud based enterprise resource planning and cloud based storage solutions.
Hybrid approaches are particularly beneficial for industries with strict regulatory requirements, as they allow for greater control over sensitive data while still taking advantage of cloud efficiencies.
5. Benefits and Advantages
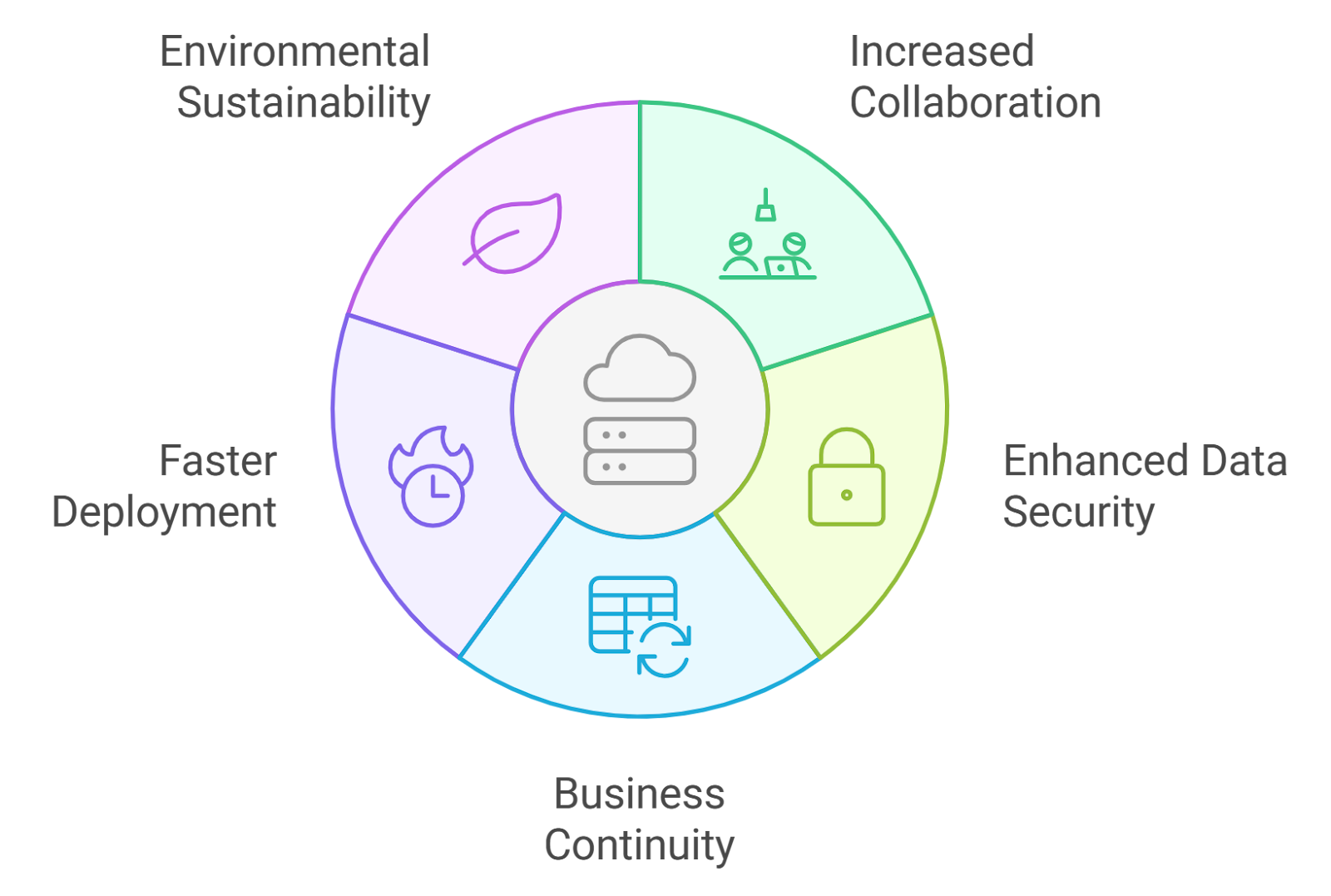
‍
The adoption of cloud-based and hybrid systems offers numerous benefits and advantages that can significantly impact an organization’s efficiency and competitiveness.
- Increased Collaboration: Cloud systems enable real-time collaboration among teams, regardless of location. This fosters innovation and improves productivity. Rapid Innovation implements collaborative tools that enhance team dynamics and project outcomes, including cloud based crm and cloud based customer relationship management.
- Enhanced Data Security: Many cloud providers invest heavily in security measures, offering advanced encryption and compliance with industry standards, which can enhance data protection. Our blockchain solutions provide an additional layer of security, ensuring data integrity and trust, particularly in cloud based security solutions.
- Business Continuity: With cloud-based solutions, businesses can ensure continuity in the face of disasters, as data is stored off-site and can be quickly restored. We help clients develop comprehensive business continuity plans that leverage cloud capabilities, including cloud based payroll software and cloud based bookkeeping software.
- Faster Deployment: Cloud services can be deployed quickly, allowing businesses to launch new applications and services without lengthy setup times. Rapid Innovation accelerates deployment processes, enabling clients to bring products to market faster, utilizing cloud based inventory software and cloud based enterprise solutions.
- Environmental Sustainability: By utilizing shared resources in the cloud, organizations can reduce their carbon footprint, contributing to more sustainable business practices. Our solutions promote eco-friendly practices, aligning with corporate social responsibility goals.
Overall, the shift towards cloud-based and hybrid systems represents a significant evolution in how businesses manage their IT resources, leading to improved operational efficiency and strategic advantages in a competitive marketplace. Rapid Innovation is committed to guiding clients through this transformation, ensuring they achieve their business goals efficiently and effectively.
5.1. Operational Efficiency
Operational efficiency refers to the ability of an organization to deliver products or services in the most cost-effective manner while maintaining high quality. It is crucial for businesses to streamline their processes, reduce waste, and optimize resource utilization. Achieving operational efficiency can lead to increased profitability, improved customer satisfaction, and a competitive edge in the market. Key components of operational efficiency include:
- Process optimization
- Resource management
- Technology integration
- Employee training and engagement
- Performance measurement and analysis
At Rapid Innovation, we leverage our expertise in AI and Blockchain technologies to help organizations enhance these areas, ultimately leading to better overall performance and greater ROI.
5.1.1. 24/7 Availability
In today's fast-paced business environment, 24/7 availability has become a critical factor for operational efficiency. Customers expect immediate access to services and support, regardless of the time of day. Providing round-the-clock availability can significantly enhance customer satisfaction and loyalty. Key aspects of 24/7 availability include:
- Customer support: Offering continuous customer service through various channels, such as phone, chat, and email, ensures that customers can get assistance whenever they need it. Our AI-driven chatbots can provide instant responses, reducing wait times and improving customer experience.
- Online services: Businesses can leverage e-commerce platforms and self-service portals to allow customers to access products and services at any time. Our Blockchain solutions can enhance security and transparency in transactions, fostering trust with customers.
- Global reach: For companies operating in multiple time zones, 24/7 availability enables them to cater to a diverse customer base without delays.
Implementing 24/7 availability may require investment in technology and staffing, but the long-term benefits often outweigh the costs. Companies that prioritize this aspect of operational efficiency can gain a significant advantage over competitors.
5.1.2. Rapid Response Times
Rapid response times are essential for maintaining operational efficiency and ensuring customer satisfaction. In an era where consumers expect quick solutions, businesses must be agile and responsive to meet these demands. Key factors contributing to rapid response times include:
- Streamlined processes: By optimizing workflows and eliminating bottlenecks, organizations can respond to customer inquiries and issues more quickly. Our consulting services can identify inefficiencies and recommend AI solutions to automate repetitive tasks, thereby improving operational efficiency in manufacturing.
- Technology utilization: Implementing advanced technologies, such as chatbots and automated ticketing systems, can help businesses address customer needs promptly. Our AI solutions can analyze customer interactions to provide insights for faster resolutions, measuring operational efficiency effectively.
- Employee training: Ensuring that staff are well-trained and knowledgeable enables them to handle customer requests efficiently and effectively. We offer tailored training programs that incorporate AI tools to enhance employee performance and improve operational effectiveness.
Organizations that prioritize rapid response times can enhance their reputation, foster customer loyalty, and ultimately drive sales. By focusing on these elements, businesses can achieve a higher level of operational efficiency and remain competitive in their respective markets. At Rapid Innovation, we are committed to helping our clients harness the power of AI and Blockchain to achieve these goals effectively and efficiently, ultimately increasing operational productivity and enhancing operational efficiency management.
5.1.3. Consistent Service Quality
Consistent service quality is crucial for any business aiming to build a loyal customer base and enhance its reputation. It involves delivering the same high level of service across all customer interactions, ensuring that customers have a reliable experience every time they engage with the brand.
- Establishing Standard Operating Procedures (SOPs) helps in maintaining uniformity in service delivery. Rapid Innovation can assist in developing tailored SOPs that integrate AI-driven insights to enhance service consistency, which is a key aspect of service quality improvement.
- Regular training and development programs for employees ensure they are equipped with the necessary skills and knowledge. Our AI solutions can provide personalized training modules that adapt to individual learning paces, ensuring employees are always at their best, contributing to better quality service.
- Implementing customer feedback mechanisms allows businesses to identify areas for improvement and address them promptly. Leveraging blockchain technology, we can create transparent feedback systems that ensure authenticity and trust in customer responses, which is essential for quality service improvement.
- Utilizing technology, such as CRM systems, can streamline processes and enhance communication, leading to better service quality. Rapid Innovation specializes in integrating AI with CRM systems to provide predictive analytics, helping businesses anticipate customer needs and improve their quality score in BPO.
- Monitoring key performance indicators (KPIs) related to service quality can help in assessing performance and making necessary adjustments. Our AI tools can automate KPI tracking, providing real-time insights that drive continuous improvement, aligning with strategies for delivering service quality. For more information on how AI can enhance service quality, check out our AI Development Guide for Businesses.
5.2. Cost Optimization
Cost optimization is the process of reducing expenses while maintaining the quality of products or services. It is essential for improving profitability and ensuring long-term sustainability. Businesses can achieve cost optimization through various strategies.
- Conducting a thorough analysis of current expenses helps identify areas where costs can be reduced. Rapid Innovation employs AI algorithms to analyze spending patterns and uncover hidden savings opportunities.
- Implementing lean management principles can eliminate waste and improve efficiency. Our blockchain solutions can enhance supply chain transparency, reducing inefficiencies and associated costs.
- Negotiating with suppliers for better rates or exploring alternative suppliers can lead to significant savings. We can leverage AI to analyze supplier performance and market trends, empowering businesses to negotiate more effectively.
- Automating repetitive tasks can reduce labor costs and increase productivity. Rapid Innovation offers AI-driven automation solutions that streamline operations, allowing teams to focus on higher-value tasks.
- Regularly reviewing and adjusting pricing strategies can help maintain competitiveness without sacrificing margins. Our data analytics capabilities can provide insights into market dynamics, enabling businesses to optimize their pricing strategies effectively.
5.2.1. Resource Allocation
Effective resource allocation is vital for maximizing efficiency and achieving business objectives. It involves distributing resources—such as time, money, and personnel—strategically to ensure optimal performance.
- Assessing the needs of different departments or projects helps prioritize resource distribution. Rapid Innovation can implement AI tools that analyze project requirements and resource availability, ensuring optimal allocation.
- Utilizing project management tools can aid in tracking resource usage and identifying bottlenecks. Our solutions integrate AI to provide real-time visibility into resource utilization, enabling proactive management.
- Regularly reviewing resource allocation strategies ensures alignment with changing business goals and market conditions. We can assist in developing adaptive resource allocation frameworks powered by AI insights.
- Encouraging cross-department collaboration can lead to more effective use of resources and innovative solutions. Our blockchain technology fosters secure and transparent collaboration across departments, enhancing resource sharing.
- Investing in employee training and development can enhance skill sets, leading to better resource utilization. Rapid Innovation offers AI-driven training solutions that ensure employees are equipped with the latest skills to maximize resource efficiency, which is essential for quality improvement services. 5.2.2. Labor Cost Reduction
Labor cost reduction is a critical strategy for businesses aiming to improve their bottom line. By optimizing labor expenses, companies can allocate resources more effectively and enhance overall productivity.
- Automation: Implementing automation tools can significantly reduce the need for manual labor. This includes software for data entry, customer service chatbots, and robotic process automation (RPA) in manufacturing. At Rapid Innovation, we leverage AI-driven automation solutions that not only streamline operations but also enhance accuracy, leading to substantial cost savings and improved ROI for our clients.
- Streamlined Processes: Analyzing and refining workflows can eliminate unnecessary steps, reducing the time employees spend on tasks. Lean methodologies can help identify waste and improve efficiency. Our consulting services focus on integrating AI analytics to identify bottlenecks and optimize processes, ensuring that our clients achieve maximum productivity.
- Outsourcing: Outsourcing non-core functions can lead to substantial labor cost savings. By hiring third-party service providers, businesses can focus on their primary operations while reducing overhead costs. Rapid Innovation assists clients in identifying the right outsourcing partners and implementing blockchain solutions to ensure transparency and security in these transactions.
- Flexible Work Arrangements: Offering remote work options can lower costs associated with office space and utilities. Additionally, it can lead to higher employee satisfaction and retention. Our AI tools facilitate seamless collaboration and communication among remote teams, ensuring that productivity remains high regardless of location.
- Training and Development: Investing in employee training can enhance skills and productivity, leading to better performance and reduced turnover costs. Rapid Innovation provides tailored training programs that incorporate AI technologies, enabling employees to adapt to new tools and processes effectively. Additionally, learning from real-world AI implementations can provide valuable insights for optimizing labor cost reduction strategies.
5.2.3. Prevention of Major Issues
Preventing major issues is essential for maintaining operational efficiency and safeguarding a company's reputation. Proactive measures can mitigate risks and ensure smooth business operations.
- Risk Assessment: Regularly conducting risk assessments helps identify potential vulnerabilities within the organization. This allows businesses to implement strategies to address these risks before they escalate. Our AI-driven risk assessment tools provide real-time insights, enabling clients to stay ahead of potential threats.
- Maintenance Programs: Establishing routine maintenance schedules for equipment and technology can prevent breakdowns and costly repairs. Predictive maintenance can further enhance reliability by addressing issues before they become critical. Rapid Innovation employs AI algorithms to predict maintenance needs, reducing downtime and associated costs.
- Employee Training: Providing ongoing training for employees on safety protocols and operational procedures can reduce the likelihood of accidents and errors. A well-informed workforce is better equipped to handle challenges. Our training solutions utilize AI simulations to create realistic scenarios, enhancing learning outcomes.
- Quality Control: Implementing strict quality control measures can prevent defects and ensure that products meet industry standards. This reduces the risk of recalls and damage to brand reputation. We integrate blockchain technology to enhance traceability and accountability in quality control processes, ensuring that our clients maintain high standards.
- Communication Channels: Establishing clear communication channels within the organization can facilitate quick responses to emerging issues. Encouraging feedback from employees can also help identify potential problems early. Our AI-powered communication platforms streamline information flow, ensuring that teams can respond swiftly to challenges.
5.3. Enhanced User Experience
Enhancing user experience (UX) is vital for businesses looking to retain customers and foster loyalty. A positive UX can lead to increased engagement and higher conversion rates.
- User-Centric Design: Focusing on user needs during the design process ensures that products and services are intuitive and easy to use. This includes conducting user research and usability testing to gather feedback. Rapid Innovation employs AI tools to analyze user behavior, allowing us to create designs that resonate with target audiences.
- Mobile Optimization: With the rise of mobile device usage, ensuring that websites and applications are mobile-friendly is crucial. A responsive design improves accessibility and enhances the overall user experience. Our development team specializes in creating mobile-optimized solutions that enhance user engagement.
- Personalization: Tailoring content and recommendations based on user behavior can create a more engaging experience. Utilizing data analytics allows businesses to understand customer preferences and deliver relevant offerings. Rapid Innovation harnesses AI to provide personalized experiences that drive customer loyalty and satisfaction.
- Fast Load Times: Website speed is a critical factor in user satisfaction. Optimizing images, reducing server response times, and minimizing redirects can significantly improve load times. Our technical expertise ensures that our clients' platforms are optimized for speed, enhancing overall user experience.
- Customer Support: Providing multiple channels for customer support, such as live chat, email, and phone, enhances user experience. Quick and effective support can resolve issues promptly, leading to higher customer satisfaction. We implement AI chatbots that provide 24/7 support, ensuring that customers receive timely assistance.
5.3.1. Personalized Support
Personalized support is essential in enhancing customer satisfaction and loyalty. It involves tailoring assistance to meet the unique needs of each customer, significantly improving the overall customer experience. At Rapid Innovation, we leverage AI-driven analytics to understand customer preferences and behaviors, enabling us to provide targeted solutions that align with their business goals.
- Understanding customer preferences: Collecting data on customer interactions helps in identifying their preferences and pain points. Our AI algorithms analyze this data to deliver insights that inform personalized strategies.
- Customized communication: Using customer data to personalize emails, chat responses, and support interactions can make customers feel valued. Our AI tools automate this process, ensuring timely and relevant communication.
- Proactive outreach: Anticipating customer needs and reaching out with solutions before they encounter issues can enhance trust and satisfaction. Our predictive analytics capabilities allow us to foresee potential challenges and address them proactively.
- Dedicated account managers: Assigning specific representatives to high-value clients ensures they receive focused attention and support. This personalized approach fosters stronger relationships and drives higher ROI.
- Feedback loops: Regularly soliciting feedback allows businesses to adjust their support strategies to better meet customer needs. Our blockchain solutions ensure that feedback is securely stored and easily accessible for continuous improvement.
5.3.2. Multi-language Capabilities
In today's global marketplace, offering multi-language capabilities is crucial for reaching a diverse customer base. This feature allows businesses to communicate effectively with customers from different linguistic backgrounds, enhancing their overall experience.
- Expanding market reach: Providing support in multiple languages can attract customers from various regions, increasing market penetration. Our AI translation tools facilitate seamless communication across languages.
- Enhancing customer experience: Customers are more likely to engage with brands that communicate in their native language, leading to higher satisfaction rates. Our solutions ensure that language barriers are minimized, fostering better engagement.
- Reducing misunderstandings: Clear communication in the customer's preferred language minimizes the risk of misinterpretation and frustration. Our AI-driven language processing tools enhance clarity and understanding.
- Cultural sensitivity: Understanding cultural nuances in language can improve interactions and foster better relationships with customers. Our team is trained to recognize and respect these nuances, ensuring effective communication.
- Utilizing technology: Implementing translation tools and hiring bilingual staff can streamline the process of offering multi-language support. Rapid Innovation integrates advanced technology to provide efficient and effective multi-language solutions.
5.3.3. Self-Service Options
Self-service options empower customers to find solutions independently, enhancing their experience and reducing the burden on support teams. This approach is increasingly popular as customers seek quick and efficient resolutions.
- Knowledge bases: Creating comprehensive online resources, such as FAQs and how-to guides, allows customers to find answers without needing direct support. Our AI systems continuously update these resources based on user interactions.
- Chatbots: Implementing AI-driven chatbots can provide instant responses to common queries, improving response times and customer satisfaction. Our chatbots are designed to learn from interactions, becoming more effective over time.
- Community forums: Establishing user communities where customers can share experiences and solutions fosters a sense of belonging and support. Our blockchain technology ensures that these interactions are secure and trustworthy.
- User-friendly interfaces: Designing intuitive self-service portals ensures customers can easily navigate and find the information they need. Our UX/UI experts focus on creating seamless experiences that drive engagement.
- Analytics and feedback: Monitoring self-service usage and gathering feedback helps businesses refine their offerings and improve the overall experience. Our AI analytics tools provide actionable insights to enhance self-service capabilities.
At Rapid Innovation, we are committed to helping our clients achieve greater ROI through tailored solutions that leverage the power of AI and blockchain technology. This includes providing support for services like wal mart money card customer service, stitch fix customer service, verizon live agent, and dave banking customer service, ensuring that personalized customer care is always a priority.
5.4. Knowledge Management
Knowledge management (KM) is a systematic approach to capturing, distributing, and effectively using knowledge within an organization. It involves the processes of creating, sharing, using, and managing the knowledge and information of an organization. Effective knowledge management can lead to improved efficiency, innovation, and competitive advantage. Key benefits of knowledge management include:
- Enhances decision-making capabilities
- Fosters a culture of continuous learning
- Increases collaboration among teams
- Reduces redundancy and improves productivity
- Supports better customer service and satisfaction
At Rapid Innovation, we leverage advanced AI and blockchain technologies to enhance knowledge management systems, ensuring that organizations can maximize their intellectual assets and achieve greater ROI. Our knowledge management software and solutions are designed to streamline processes and improve overall effectiveness. For more insights on the future of knowledge management, check out our article on AI knowledge management in 2024.
5.4.1. Automated Documentation
Automated documentation refers to the use of technology to create, manage, and store documents without the need for manual input. This process streamlines the documentation workflow, making it faster and more efficient. The advantages of automated documentation include:
- Reduces human error by minimizing manual data entry
- Saves time by automating repetitive tasks
- Ensures consistency in documentation formats
- Facilitates easy access to information for employees
- Supports compliance with regulatory requirements
Rapid Innovation employs AI-driven tools that automate documentation processes, allowing organizations to focus on strategic initiatives rather than administrative tasks. Our personal knowledge management system integrates seamlessly with existing systems, ensuring real-time updates and data accuracy.
5.4.2. Pattern Recognition
Pattern recognition is the ability to identify trends, correlations, and anomalies in data. In the context of knowledge management, it plays a crucial role in analyzing information to derive insights that can inform decision-making and strategy development. The benefits of pattern recognition include:
- Helps in identifying customer behavior trends
- Aids in predicting market changes and demands
- Enhances risk management by spotting potential issues early
- Supports innovation by uncovering new opportunities
- Facilitates personalized experiences for customers
At Rapid Innovation, we utilize machine learning and artificial intelligence to implement advanced pattern recognition techniques. By analyzing large datasets, we uncover hidden insights that empower organizations to make informed, data-driven decisions, ultimately enhancing their overall performance and strategic direction. Our expertise in knowledge management systems and cloud-based knowledge management systems ensures that clients can adapt to market changes swiftly and effectively, driving greater ROI. Additionally, we provide examples of knowledge management systems and best knowledge management software to help organizations choose the right solutions for their needs. For more information on how we can assist you, visit our AI consulting company.
5.4.3. Knowledge Base Evolution
Knowledge base evolution refers to the continuous process of updating, refining, and expanding a knowledge base to ensure it remains relevant and useful. This evolution is crucial for organizations that rely on knowledge management systems, such as cloud based knowledge management systems and salesforce knowledge management, to support decision-making, enhance customer service, and foster innovation.
- Dynamic Content Updates: Knowledge bases must adapt to new information, technologies, and user needs. Regular updates help maintain accuracy and relevance, ensuring that organizations can leverage the latest insights for strategic advantage. This is particularly important for knowledge base management systems like sharepoint knowledge management and jira service management knowledge base.
- User Contributions: Encouraging users to contribute knowledge can enhance the richness of the knowledge base. This can include feedback, suggestions, or new content submissions, fostering a collaborative environment that drives innovation. Platforms like proprofs knowledge base and asana knowledge base can facilitate user contributions effectively.
- Integration with Other Systems: Evolving a knowledge base often involves integrating it with other systems, such as customer relationship management (CRM) or enterprise resource planning (ERP) systems, to provide a holistic view of information. This integration can streamline processes and improve data accessibility, especially when using tools like manageengine knowledge base and clickup knowledge base.
- Machine Learning and AI: Leveraging artificial intelligence and machine learning can automate the process of knowledge base updates, making it easier to identify gaps and suggest new content. Rapid Innovation utilizes these technologies to enhance the efficiency of knowledge management, ultimately leading to greater ROI for our clients.
- Version Control: Implementing version control mechanisms ensures that changes are tracked, allowing users to access previous versions of content if needed. This feature is essential for maintaining the integrity of information over time, particularly in knowledge base management systems.
- User-Centric Design: Evolving a knowledge base should focus on user experience. Regularly gathering user feedback can guide improvements in navigation, search functionality, and content organization, ensuring that users can easily find the information they need. Tools like knowledge base tools free and open source knowledge management can aid in creating a user-friendly experience.
6. Challenges and Limitations
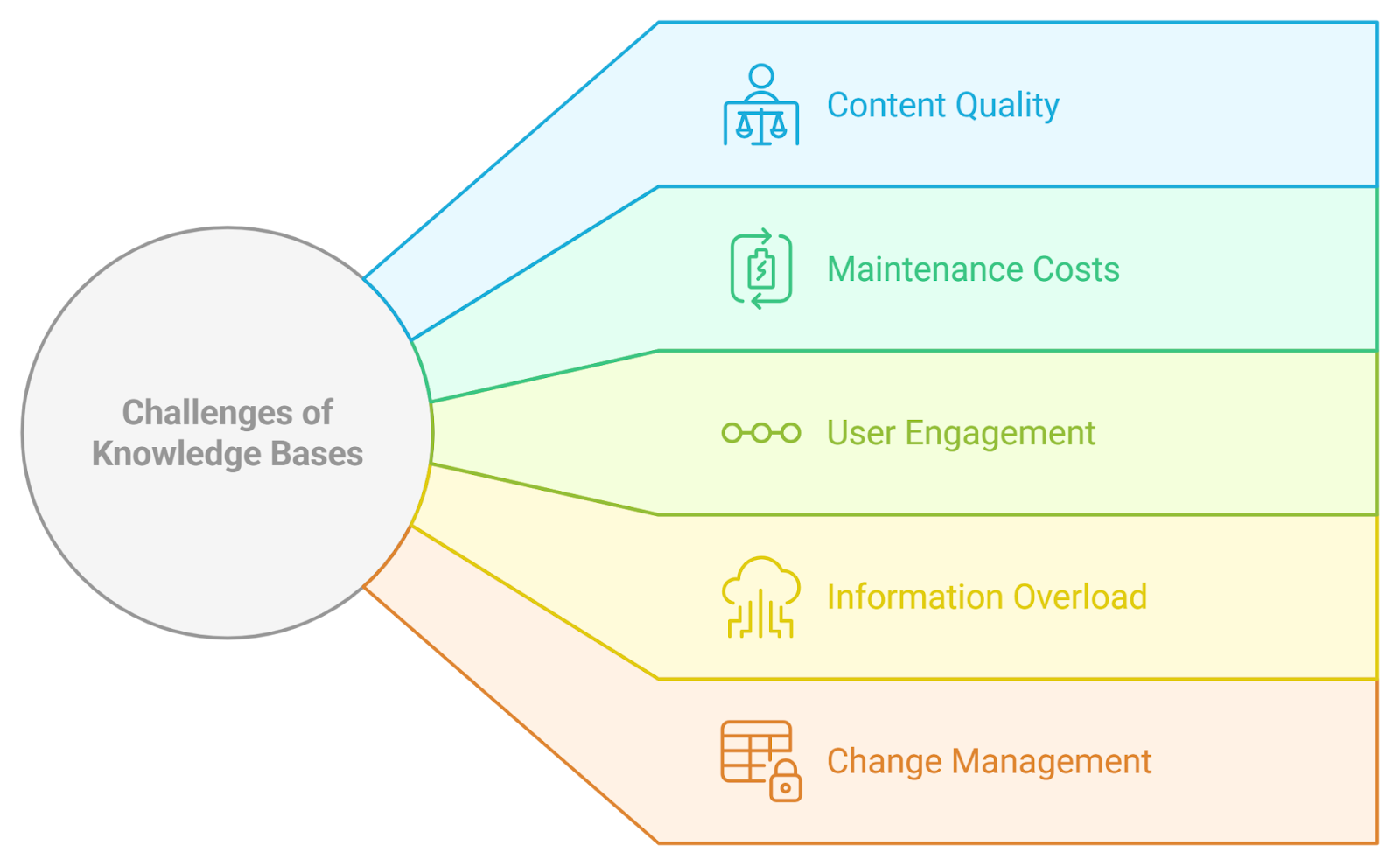
‍
Despite the benefits of knowledge bases, several challenges and limitations can hinder their effectiveness. Understanding these challenges is essential for organizations aiming to optimize their knowledge management strategies.
- Content Quality: Ensuring high-quality, accurate, and relevant content is a significant challenge. Poorly curated information can lead to misinformation and decreased user trust, which can adversely affect decision-making.
- Maintenance Costs: Regularly updating and maintaining a knowledge base can be resource-intensive. Organizations must allocate sufficient time and budget for ongoing management to ensure the knowledge base remains effective.
- User Engagement: Encouraging users to actively engage with the knowledge base can be difficult. If users do not find the content valuable or easy to navigate, they may not utilize the system effectively, leading to underutilization of resources.
- Information Overload: A knowledge base that grows too large can overwhelm users. It is essential to implement effective categorization and search functionalities to help users find relevant information quickly, thereby enhancing user satisfaction.
- Change Management: As knowledge bases evolve, organizations may face resistance from employees who are accustomed to existing processes. Effective change management strategies are necessary to facilitate smooth transitions and ensure user buy-in.
6.1. Technical Challenges
Technical challenges are a significant aspect of knowledge base management. These challenges can impact the performance, usability, and overall effectiveness of the knowledge base.
- Scalability: As the volume of information grows, ensuring that the knowledge base can scale effectively is crucial. Organizations must choose platforms that can handle increased data without compromising performance, which is a key consideration for Rapid Innovation's solutions.
- Integration Issues: Integrating a knowledge base with other systems can present technical difficulties. Compatibility issues may arise, leading to data silos and inefficiencies that can hinder operational effectiveness.
- Search Functionality: Implementing an effective search function is vital for user satisfaction. Poor search capabilities can result in users struggling to find relevant information, leading to frustration and decreased usage.
- Data Security: Protecting sensitive information within a knowledge base is paramount. Organizations must implement robust security measures to prevent unauthorized access and data breaches, ensuring compliance with industry standards.
- User Interface Design: A poorly designed user interface can hinder user engagement. Ensuring that the knowledge base is intuitive and user-friendly is essential for maximizing its effectiveness and encouraging user adoption.
- Performance Optimization: As the knowledge base grows, maintaining optimal performance becomes challenging. Regular performance assessments and optimizations are necessary to ensure quick load times and responsiveness, which are critical for user satisfaction.
By addressing these challenges, organizations can enhance the effectiveness of their knowledge bases, ultimately leading to improved decision-making and operational efficiency. Rapid Innovation is committed to providing the expertise and solutions necessary to navigate these complexities, helping clients achieve greater ROI through effective knowledge management strategies.
6.1.1. Data Quality Issues
Data quality issues can significantly impact the effectiveness of data-driven decision-making processes. Poor data quality can lead to incorrect insights, which can ultimately affect business outcomes. Key aspects of data quality include:
- Accuracy: Data must be correct and reliable. Inaccurate data can stem from human error, outdated information, or incorrect data entry.
- Completeness: Data should be comprehensive and contain all necessary information. Missing data can lead to incomplete analyses and misguided strategies.
- Consistency: Data should be uniform across different systems and databases. Inconsistencies can arise from multiple data sources or formats, leading to confusion and errors.
- Timeliness: Data must be up-to-date to be relevant. Stale data can mislead decision-makers and result in missed opportunities.
- Relevance: Data should be pertinent to the specific business context. Irrelevant data can clutter analyses and distract from key insights.
Addressing data quality issues requires implementing robust data governance practices, regular audits, and validation processes to ensure that data remains accurate, complete, and relevant. Common data quality problems, such as data quality challenges and data quality concerns, must be identified and resolved. At Rapid Innovation, we leverage AI-driven data quality tools to automate these processes, ensuring that our clients can trust their data for strategic decision-making, ultimately leading to greater ROI. Additionally, we provide insights into poor data quality examples and the costs associated with poor data quality, helping organizations understand the implications of neglecting data quality issues.
6.1.2. Integration Complexities
Integration complexities arise when combining data from various sources, systems, or platforms. These challenges can hinder the seamless flow of information and impact overall data usability. Key factors contributing to integration complexities include:
- Diverse Data Sources: Organizations often use multiple systems (e.g., CRM, ERP, databases) that may not be compatible, making integration difficult.
- Data Silos: Isolated data repositories can prevent a holistic view of information, leading to inefficiencies and missed insights.
- Inconsistent Data Formats: Different systems may use varying formats for data, complicating the integration process and requiring additional transformation efforts.
- Real-time vs. Batch Processing: Organizations may need to decide between real-time data integration or batch processing, each with its own set of challenges and requirements.
- Scalability: As organizations grow, their data integration needs may evolve, necessitating scalable solutions that can adapt to increasing data volumes and complexity.
To overcome integration complexities, organizations should invest in data integration tools and platforms that facilitate seamless data flow, standardize formats, and promote interoperability among systems. Rapid Innovation specializes in developing custom integration solutions that harness the power of blockchain technology to ensure data integrity and security, enabling our clients to achieve a unified view of their data landscape.
6.1.3. Performance Bottlenecks
Performance bottlenecks can severely limit the efficiency and effectiveness of data processing and analytics. Identifying and addressing these bottlenecks is crucial for optimizing data workflows. Common causes of performance bottlenecks include:
- Insufficient Hardware Resources: Limited processing power, memory, or storage can slow down data processing and analytics tasks.
- Inefficient Queries: Poorly designed queries can lead to slow response times and increased load on databases, affecting overall performance.
- Data Volume: Large volumes of data can overwhelm systems, leading to delays in processing and analysis.
- Network Latency: Slow network connections can hinder data transfer speeds, impacting real-time analytics and reporting.
- Concurrency Issues: Multiple users or processes accessing the same data simultaneously can create contention, leading to slower performance.
To mitigate performance bottlenecks, organizations should regularly monitor system performance, optimize queries, and invest in scalable infrastructure that can handle growing data demands. Rapid Innovation employs advanced AI algorithms to analyze system performance and identify bottlenecks proactively, ensuring that our clients can maintain optimal performance and maximize their return on investment. Data quality observability is also a critical aspect that organizations should consider to monitor and improve their data quality continuously.
6.2. Operational Challenges
Operational challenges are critical hurdles that organizations face in their day-to-day activities. These challenges can significantly impact efficiency, productivity, and overall performance. Addressing operational challenges, such as operational problems and challenges in business operations, is essential for maintaining a competitive edge in the market. Key operational challenges include:
- Inefficient processes that lead to wasted resources.
- Resistance to change among staff.
- Inadequate training and skill gaps.
- Poor communication and collaboration.
- Technology integration issues.
Understanding and addressing these operational challenges is vital for organizations aiming to optimize their operations and achieve their strategic goals. At Rapid Innovation, we leverage our expertise in AI and Blockchain to help organizations streamline their processes, enhance communication, and integrate technology effectively, ultimately driving greater ROI.
6.2.1. Change Management
Change management refers to the structured approach to transitioning individuals, teams, and organizations from a current state to a desired future state. Effective change management is crucial for minimizing resistance and ensuring that changes are implemented smoothly. Key aspects of change management include:
- Communication: Clear and transparent communication about the reasons for change helps in reducing uncertainty and building trust among employees.
- Stakeholder Engagement: Involving key stakeholders in the change process fosters a sense of ownership and commitment to the new direction.
- Leadership Support: Strong leadership is essential for guiding teams through change and addressing any concerns that may arise.
- Feedback Mechanisms: Establishing channels for feedback allows employees to voice their concerns and suggestions, which can be invaluable for refining the change process.
- Monitoring and Evaluation: Regularly assessing the impact of changes helps organizations make necessary adjustments and ensures that objectives are being met.
Organizations that fail to manage change effectively may experience disruptions, decreased morale, and increased turnover. According to studies, approximately 70% of change initiatives fail due to resistance from employees and inadequate change management strategies. Rapid Innovation assists clients in implementing AI-driven change management solutions that facilitate smoother transitions and enhance employee engagement.
6.2.2. Staff Training Requirements
Staff training is a fundamental component of operational success. As organizations evolve, the need for continuous learning and skill development becomes increasingly important. Key considerations for staff training requirements include:
- Identifying Skill Gaps: Conducting assessments to identify areas where employees lack the necessary skills or knowledge is crucial for effective training programs.
- Tailored Training Programs: Developing customized training programs that address specific needs ensures that employees receive relevant and applicable knowledge.
- Onboarding and Orientation: Comprehensive onboarding processes help new employees acclimate to the company culture and understand their roles and responsibilities.
- Ongoing Development: Providing opportunities for continuous learning, such as workshops, seminars, and online courses, keeps employees engaged and up-to-date with industry trends.
- Measuring Training Effectiveness: Evaluating the impact of training programs through performance metrics and feedback helps organizations refine their training strategies.
Investing in staff training not only enhances employee performance but also contributes to higher job satisfaction and retention rates. Research indicates that organizations with strong training programs can see a 24% higher profit margin compared to those that do not prioritize employee development. Rapid Innovation offers tailored training solutions that incorporate AI and Blockchain technologies, ensuring that your workforce is equipped with the skills necessary to thrive in a rapidly changing environment. Addressing common problems in business operations is crucial for long-term success. For more insights on optimizing staffing and schedules, check out our article on AI for Workforce Management in Retail.
6.2.3. Process Adaptation
Process adaptation refers to the ability of an organization to modify its processes in response to changing conditions, requirements, or technologies. This flexibility is crucial for maintaining efficiency and competitiveness in a rapidly evolving market. Organizations must regularly assess their processes to identify areas for improvement. Adaptation can involve changes in workflows, technology integration, or even shifts in organizational culture.
Key factors influencing process adaptation include: - Technological advancements - Market demands - Regulatory changes
Successful process adaptation often requires: - Employee training and development - Stakeholder engagement - Continuous feedback loops
Companies that embrace process adaptation can achieve: - Increased operational efficiency - Enhanced customer satisfaction - Greater innovation potential
At Rapid Innovation, we leverage AI and Blockchain technologies to facilitate seamless process adaptation for our clients. For instance, by implementing AI-driven analytics, organizations can gain insights into their operational workflows, allowing them to identify bottlenecks and optimize processes in real-time. Additionally, Blockchain can enhance transparency and traceability in supply chains, enabling organizations to adapt quickly to market demands and regulatory changes.
6.3. Security Concerns
Security concerns encompass a wide range of issues that organizations face in protecting their data, systems, and networks from threats. As businesses increasingly rely on digital technologies, understanding and addressing these concerns is paramount.
Common security threats include: - Cyberattacks (e.g., phishing, ransomware) - Data breaches - Insider threats
Organizations must implement robust security measures to mitigate risks, such as: - Firewalls and antivirus software - Regular security audits - Employee training on security best practices
Compliance with regulations (e.g., GDPR, HIPAA) is essential for maintaining security and avoiding legal repercussions. The cost of security breaches can be significant, impacting both finances and reputation.
6.3.1. Data Privacy
Data privacy is a critical aspect of security that focuses on the proper handling of personal and sensitive information. With increasing data collection and usage, organizations must prioritize data privacy to build trust and comply with legal requirements.
Key principles of data privacy include: - Transparency: Informing users about data collection and usage. - Consent: Obtaining explicit permission from individuals before processing their data. - Data minimization: Collecting only the data necessary for specific purposes.
Organizations should implement data privacy policies that address: - Data storage and retention - Access controls - Data sharing practices
Regular audits and assessments can help ensure compliance with data privacy regulations. The consequences of failing to protect data privacy can include: - Legal penalties - Loss of customer trust - Damage to brand reputation
By focusing on process adaptation, security concerns, and data privacy, organizations can create a resilient framework that supports growth and innovation while safeguarding their assets and customer information. Rapid Innovation is committed to guiding clients through these challenges, ensuring they not only meet compliance requirements but also enhance their overall operational effectiveness and customer trust.
6.3.2. Access Control
Access control is a critical component of information security, ensuring that only authorized individuals can access specific resources and data. Effective access control mechanisms help protect sensitive information from unauthorized access, breaches, and potential data loss.
- Types of Access Control: Â
- Discretionary Access Control (DAC): Users have the ability to control access to their own resources.
- Mandatory Access Control (MAC): Access is regulated by a central authority based on multiple levels of security.
- Role-Based Access Control (RBAC): Access is granted based on the user’s role within the organization, streamlining permissions management. Implementing role-based access control best practices can further enhance security.
- Key Elements of Access Control: Â
- Authentication: Verifying the identity of users through passwords, biometrics, or tokens.
- Authorization: Determining what resources a user can access and what actions they can perform.
- Accountability: Keeping logs of user activities to track access and changes made to resources.
- Best Practices for Access Control: Â
- Implement the principle of least privilege, granting users only the access necessary for their roles. This aligns with access control best practices.
- Regularly review and update access permissions to reflect changes in roles or employment status, following user access review best practices.
- Use multi-factor authentication (MFA) to enhance security and reduce the risk of unauthorized access. Consider incorporating authentication and access control best practices.
6.3.3. Compliance Requirements
Compliance requirements refer to the legal, regulatory, and industry standards that organizations must adhere to in order to protect sensitive data and maintain operational integrity. Non-compliance can lead to severe penalties, including fines and reputational damage.
- Common Compliance Standards: Â
- General Data Protection Regulation (GDPR): Governs data protection and privacy in the European Union.
- Health Insurance Portability and Accountability Act (HIPAA): Sets standards for protecting sensitive patient information in the healthcare sector.
- Payment Card Industry Data Security Standard (PCI DSS): Ensures secure handling of credit card information.
- Importance of Compliance: Â
- Protects sensitive data from breaches and misuse.
- Builds trust with customers and stakeholders by demonstrating a commitment to data security.
- Reduces the risk of legal penalties and financial losses associated with non-compliance.
- Strategies for Ensuring Compliance: Â
- Conduct regular audits to assess compliance with relevant regulations and standards, including access control practices.
- Provide training for employees on compliance requirements and best practices.
- Implement robust data protection measures, including encryption and access controls, to safeguard sensitive information.
7. Best Practices and Success Strategies
Implementing best practices and success strategies is essential for organizations aiming to enhance their security posture and ensure compliance with regulations. These strategies help mitigate risks and foster a culture of security awareness.
- Establish a Security Framework: Â
- Develop a comprehensive security policy that outlines roles, responsibilities, and procedures.
- Utilize established frameworks such as NIST, ISO 27001, or COBIT to guide security practices, including azure rbac best practices.
- Foster a Culture of Security Awareness: Â
- Conduct regular training sessions to educate employees about security threats and best practices, including physical access control best practices.
- Encourage reporting of suspicious activities and provide clear channels for communication.
- Regularly Update Security Measures: Â
- Stay informed about the latest security threats and vulnerabilities.
- Implement timely updates and patches to software and systems to protect against exploits, following web application access control best practices.
- Conduct Risk Assessments: Â
- Regularly evaluate potential risks to identify vulnerabilities and prioritize mitigation efforts.
- Use risk assessment findings to inform security policies and resource allocation.
- Monitor and Respond to Incidents: Â
- Establish a robust incident response plan to address security breaches effectively.
- Utilize security information and event management (SIEM) tools to monitor for unusual activities and respond promptly.
- Collaborate with Third-Party Vendors: Â
- Ensure that third-party vendors comply with your organization’s security standards, including aws access control best practices.
- Conduct due diligence and regular assessments of vendor security practices.
By implementing these best practices and success strategies, organizations can significantly enhance their security posture, ensure compliance with regulations, and protect sensitive data from potential threats. At Rapid Innovation, we leverage our expertise in AI and Blockchain to provide tailored solutions that not only meet compliance requirements but also enhance access control mechanisms, including azure identity management and access control security best practices, ultimately driving greater ROI for our clients.
7.1. Implementation Guidelines

‍
Implementing a new strategy or system requires careful planning and execution, particularly in the realms of AI and blockchain implementation, where precision and adaptability are paramount. Here are some essential guidelines to ensure successful implementation:
- Define clear objectives: Establish specific, measurable, achievable, relevant, and time-bound (SMART) goals to guide the implementation process. For instance, if integrating AI for customer service, aim for a specific reduction in response time.
- Engage stakeholders: Involve all relevant parties, including employees, management, and customers, to gain insights and foster buy-in. This is crucial in blockchain projects, where transparency and trust are essential.
- Develop a detailed plan: Create a step-by-step roadmap that outlines tasks, timelines, and responsibilities. This plan should also include risk management strategies, especially for blockchain implementations that may face regulatory scrutiny.
- Allocate resources: Ensure that adequate resources, including budget, personnel, and technology, are available to support the implementation. For AI projects, this may include investing in data infrastructure and machine learning tools.
- Provide training: Equip team members with the necessary skills and knowledge through training sessions and workshops. This is particularly important for AI, where understanding algorithms and data handling is critical.
- Communicate effectively: Maintain open lines of communication throughout the process to keep everyone informed and address any concerns. In blockchain, clear communication can help demystify the technology for stakeholders.
- Monitor progress: Regularly assess the implementation against the established objectives to identify any deviations and make necessary adjustments. Utilize AI-driven analytics to gain real-time insights into performance. For expert assistance, consider hiring generative AI engineers to enhance your implementation efforts.
7.2. Performance Monitoring
Performance monitoring is crucial for evaluating the effectiveness of the implemented strategy. It involves tracking key performance indicators (KPIs) and making data-driven decisions. Here are some key aspects of performance monitoring:
- Identify KPIs: Select relevant metrics that align with your objectives. Common KPIs include sales growth, customer satisfaction, and operational efficiency. For AI initiatives, consider metrics like accuracy and model performance.
- Use technology: Leverage software tools and analytics platforms to collect and analyze data efficiently. AI can automate data collection and provide predictive insights, enhancing decision-making.
- Set benchmarks: Establish performance standards based on historical data or industry averages to gauge success. In blockchain, benchmarks may include transaction speed and cost-effectiveness.
- Conduct regular reviews: Schedule periodic assessments to evaluate performance against KPIs and benchmarks. This ensures that AI models remain relevant and effective over time.
- Gather feedback: Solicit input from stakeholders to gain insights into areas of improvement and success. This feedback loop is vital for refining AI algorithms and blockchain applications.
- Adjust strategies: Be prepared to modify your approach based on performance data and feedback to enhance outcomes. Continuous iteration is key in both AI and blockchain projects.
7.3. Continuous Improvement
Continuous improvement is an ongoing effort to enhance products, services, or processes. It fosters a culture of innovation and adaptability. Here are some strategies for promoting continuous improvement:
- Encourage a growth mindset: Cultivate an environment where employees feel empowered to share ideas and take risks. This is essential in AI, where experimentation can lead to breakthroughs.
- Implement feedback loops: Regularly collect feedback from customers and employees to identify areas for improvement. In blockchain, user feedback can drive enhancements in usability and security.
- Utilize data analysis: Analyze performance data to uncover trends and insights that can inform improvement initiatives. AI tools can assist in identifying patterns that may not be immediately apparent.
- Foster collaboration: Encourage teamwork and cross-departmental collaboration to leverage diverse perspectives and expertise. This is particularly beneficial in blockchain projects, where interdisciplinary knowledge is crucial.
- Set incremental goals: Break down larger objectives into smaller, manageable targets to facilitate progress and maintain motivation. This approach can help in AI projects where complex models are developed iteratively.
- Celebrate successes: Recognize and reward achievements to reinforce a culture of continuous improvement and motivate employees. Highlighting successful AI implementations can inspire further innovation.
- Stay informed: Keep up with industry trends and best practices to ensure your organization remains competitive and innovative. Rapid Innovation is committed to helping clients navigate these evolving landscapes effectively.
7.4. Quality Assurance Methods
Quality assurance (QA) methods are essential for ensuring that products and services meet specified requirements and standards. These methods help organizations maintain high levels of quality, reduce defects, and enhance customer satisfaction.
- Process Audits: Regular audits of processes help identify areas for improvement by reviewing workflows, procedures, and compliance with standards. At Rapid Innovation, we leverage blockchain technology to create immutable records of these audits, ensuring transparency and accountability.
- Statistical Process Control (SPC): This method uses statistical techniques to monitor and control processes. By analyzing data, organizations can detect variations and take corrective actions before defects occur. Our AI-driven analytics tools can enhance this process, providing real-time insights that lead to quicker decision-making.
- Quality Control Circles (QCC): Small groups of employees meet regularly to discuss and solve quality-related issues, encouraging teamwork and empowering employees to take ownership of quality. Rapid Innovation fosters a collaborative environment where teams can utilize AI tools to streamline discussions and track progress.
- Root Cause Analysis (RCA): This technique identifies the underlying causes of defects or problems. By addressing root causes, organizations can prevent recurrence and improve overall quality. Our AI algorithms can assist in identifying patterns and correlations that may not be immediately apparent.
- Continuous Improvement (CI): Implementing methodologies like Six Sigma or Lean helps organizations focus on ongoing improvements. CI fosters a culture of quality and encourages employees to seek efficiencies. Rapid Innovation integrates AI solutions that continuously learn and adapt, driving ongoing enhancements in processes.
- Customer Feedback: Gathering and analyzing customer feedback is crucial for understanding quality from the user's perspective. Surveys, reviews, and direct communication can provide valuable insights. Our blockchain solutions ensure that customer feedback is securely stored and easily accessible for analysis.
- Training and Development: Regular training ensures that employees are equipped with the necessary skills and knowledge to maintain quality standards, including both initial training and ongoing professional development. Rapid Innovation offers AI-powered training modules that adapt to individual learning paces and styles.
- QA Methodologies: Various QA methodologies, such as agile methodology in QA and software quality assurance methodologies, are employed to enhance the effectiveness of quality assurance processes.
- QA Testing Methodologies: Implementing QA testing methodologies and quality assurance testing methodologies ensures that products are rigorously tested before release.
- Quality Assurance Techniques: Utilizing quality assurance techniques and software QA methods can significantly improve the overall quality of products and services.
- SQA Methodologies: Software quality assurance (SQA) methodologies and processes are critical for maintaining high standards in software development. For organizations looking to enhance their project estimation capabilities, partnering with an AI project estimation company can provide valuable insights and efficiencies.
7.5. Change Management Strategies
Change management strategies are vital for successfully implementing changes within an organization. These strategies help minimize resistance and ensure that transitions are smooth and effective.
- Clear Communication: Communicating the reasons for change and its benefits is essential. Transparency helps build trust and reduces uncertainty among employees.
- Stakeholder Engagement: Involving key stakeholders in the change process fosters buy-in and support, including managers, employees, and even customers who may be affected by the change.
- Training and Support: Providing training and resources helps employees adapt to new processes or technologies. Ongoing support can alleviate concerns and enhance confidence in the change.
- Change Champions: Identifying and empowering change champions within the organization can facilitate smoother transitions. These individuals can advocate for change and help address concerns among their peers.
- Feedback Mechanisms: Establishing channels for feedback allows employees to voice their concerns and suggestions, helping to identify issues early and adjust strategies as needed.
- Monitoring and Evaluation: Regularly assessing the impact of changes helps organizations understand what is working and what needs adjustment. This can involve performance metrics, surveys, and other evaluation tools.
- Celebrating Successes: Recognizing and celebrating milestones during the change process can boost morale and reinforce the benefits of the change, encouraging continued support and engagement.
8. Future Trends and Evolution

‍
The landscape of quality assurance and change management is continuously evolving. Understanding future trends can help organizations stay ahead and adapt to new challenges.
- Increased Automation: Automation tools are becoming more prevalent in quality assurance processes, including automated testing, data analysis, and reporting, which can enhance efficiency and accuracy.
- Data-Driven Decision Making: Organizations are increasingly relying on data analytics to inform their quality assurance and change management strategies, allowing for more informed decisions based on real-time data.
- Agile Methodologies: The adoption of agile methodologies is on the rise, particularly in software development. Agile emphasizes flexibility, collaboration, and rapid iteration, which can enhance both quality and change management.
- Focus on Customer Experience: There is a growing emphasis on customer experience in quality assurance, with organizations prioritizing customer feedback and satisfaction as key metrics for quality.
- Remote Work Considerations: The shift to remote work has changed how organizations approach quality assurance and change management, requiring strategies that account for virtual collaboration and communication.
- Sustainability and Quality: As sustainability becomes a priority, organizations are integrating environmental considerations into their quality assurance processes, including assessing the sustainability of materials and processes.
- Integration of AI and Machine Learning: Artificial intelligence and machine learning are being utilized to enhance quality assurance. These technologies can predict defects, optimize processes, and improve decision-making.
By staying informed about these trends, organizations can better prepare for the future and ensure that their quality assurance and change management practices remain effective and relevant. At Rapid Innovation, we are committed to helping our clients navigate these changes through our expertise in AI and blockchain, ultimately driving greater ROI and operational excellence.
8.1. Emerging Technologies
Emerging technologies, such as new technology and new advanced technology, are reshaping industries and driving innovation across various sectors. These technologies are characterized by their potential to create significant economic and social impacts. As we delve into the specifics, two key areas stand out: advanced AI models and quantum computing integration.
8.1.1. Advanced AI Models
Advanced AI models are at the forefront of technological innovation, enabling machines to perform tasks that traditionally required human intelligence. These models leverage vast amounts of data and sophisticated algorithms to learn, adapt, and make decisions.
Key components of advanced AI models include:
- Deep Learning: A subset of machine learning that uses neural networks with many layers, excelling in image and speech recognition.
- Natural Language Processing (NLP): This technology allows machines to understand and generate human language, enhancing applications like chatbots and virtual assistants.
- Reinforcement Learning: A type of machine learning where an agent learns to make decisions by receiving rewards or penalties, which is particularly useful in robotics and game playing.
The impact of advanced AI models is profound, leading to:
- Increased Efficiency: Businesses can automate routine tasks, resulting in significant time and cost savings. Rapid Innovation helps clients implement AI-driven automation solutions that streamline operations and reduce overhead costs.
- Enhanced Decision-Making: AI models analyze data faster and more accurately than humans, providing insights that drive strategic decisions. Our expertise in AI allows us to develop tailored analytics solutions that empower businesses to make data-driven choices.
- Personalization: AI enables tailored experiences in sectors like e-commerce and entertainment, improving customer satisfaction. Rapid Innovation collaborates with clients to create personalized customer engagement strategies that enhance user experience and loyalty.
According to a report by McKinsey, AI could potentially add $13 trillion to the global economy by 2030. This highlights the transformative potential of advanced AI models in various industries, including new AI technology and latest technological advancements. For businesses looking to harness this potential, Rapid Innovation offers specialized services in generative AI development.
8.1.2. Quantum Computing Integration
Quantum computing represents a paradigm shift in computational power, utilizing the principles of quantum mechanics to process information in ways that classical computers cannot. This technology is still in its infancy but holds immense promise for the future.
Key features of quantum computing include:
- Quantum Bits (Qubits): Unlike traditional bits, which can be either 0 or 1, qubits can exist in multiple states simultaneously, allowing for complex calculations at unprecedented speeds.
- Superposition and Entanglement: These quantum phenomena enable quantum computers to perform multiple calculations at once and solve problems that are currently intractable for classical computers.
The integration of quantum computing into various fields is expected to revolutionize industries, particularly in:
- Drug Discovery: Quantum computing can simulate molecular interactions, significantly speeding up the process of discovering new drugs. Rapid Innovation is positioned to assist pharmaceutical companies in leveraging quantum computing for accelerated research and development.
- Cryptography: Quantum computers could break traditional encryption methods, prompting the development of quantum-resistant algorithms. Our team is actively exploring solutions to enhance security protocols in anticipation of quantum advancements.
- Optimization Problems: Industries such as logistics and finance can benefit from quantum computing's ability to solve complex optimization problems more efficiently. Rapid Innovation offers consulting services to help businesses identify and implement quantum solutions for operational challenges.
As of 2023, companies like IBM and Google are leading the charge in quantum computing research, with IBM's Quantum System One being one of the first commercially available quantum computers. The potential of quantum computing is vast, with estimates suggesting it could solve problems in seconds that would take classical computers thousands of years.
In conclusion, both advanced AI models and quantum computing integration are pivotal emerging technologies that are set to redefine the landscape of innovation and efficiency across various sectors. Their continued development and application will likely lead to groundbreaking advancements in how we live and work. Rapid Innovation is committed to guiding clients through this technological evolution, ensuring they achieve their business goals efficiently and effectively, including the latest technology trends and new technologies in 2023.
8.1.3. Edge Computing Applications
Edge computing is revolutionizing how data is processed, analyzed, and utilized across various industries. By bringing computation and data storage closer to the source of data generation, edge computing reduces latency and bandwidth use, enhancing the performance of applications.
- IoT Devices: Edge computing is crucial for Internet of Things (IoT) devices, enabling real-time data processing. This is particularly important in smart homes, industrial automation, and healthcare, where immediate responses are necessary. Rapid Innovation can assist clients in developing IoT solutions that leverage mobile edge computing to enhance operational efficiency and customer engagement.
- Autonomous Vehicles: In the automotive industry, edge computing allows for real-time data processing from sensors and cameras, which is essential for the safe operation of autonomous vehicles. This reduces the need for constant communication with centralized data centers. Our expertise in AI and blockchain can help clients create secure and efficient systems for autonomous vehicle data management, including edge computing in autonomous vehicles.
- Smart Cities: Edge computing supports smart city initiatives by processing data from various sensors and devices locally. This can improve traffic management, waste management, and public safety by enabling quicker decision-making. Rapid Innovation can guide municipalities in implementing edge solutions that enhance urban living and resource management, including examples of edge computing.
- Healthcare: In healthcare, edge computing can facilitate remote patient monitoring and telemedicine. By processing data locally, healthcare providers can respond to patient needs more swiftly, improving outcomes. Our consulting services can help healthcare organizations integrate edge computing with AI to optimize patient care and operational workflows, including edge computing applications in healthcare.
- Retail: Retailers use edge computing to analyze customer behavior in real-time, allowing for personalized marketing and inventory management. This enhances the shopping experience and optimizes supply chains. Rapid Innovation can support retail clients in deploying edge computing solutions that drive sales and improve customer satisfaction, leveraging applications of predictive analytics and AI-driven edge computing.
8.2. Industry Developments
The landscape of edge computing is rapidly evolving, with significant developments across various sectors. These advancements are driven by the increasing demand for faster data processing and the proliferation of connected devices.
- 5G Integration: The rollout of 5G networks is a game-changer for edge computing. With higher speeds and lower latency, 5G enhances the capabilities of edge devices, enabling more complex applications and services. Rapid Innovation can help businesses harness 5G technology to enhance their edge computing strategies, including mobile edge computing 5G.
- AI and Machine Learning: The integration of artificial intelligence (AI) and machine learning at the edge allows for smarter data processing. This enables devices to learn from data patterns and make decisions without needing to communicate with a central server. Our expertise in AI can empower clients to develop intelligent edge applications that improve decision-making and operational efficiency, including industrial edge computing.
- Security Enhancements: As edge computing grows, so does the focus on security. New protocols and technologies are being developed to protect data at the edge, ensuring that sensitive information remains secure. Rapid Innovation can assist organizations in implementing robust security measures for their edge computing environments, addressing edge computing security.
- Standardization Efforts: Industry groups are working towards standardizing edge computing frameworks. This will facilitate interoperability between devices and applications, making it easier for businesses to adopt edge solutions. Our consulting services can guide clients through the complexities of standardization in edge computing, including fog and edge computing.
- Partnerships and Collaborations: Companies are increasingly forming partnerships to leverage edge computing technologies. Collaborations between tech giants and startups are driving innovation and expanding the range of edge applications. Rapid Innovation can help clients identify and establish strategic partnerships to enhance their edge computing capabilities, including partnerships with edge computing companies.
8.3. Predictive Capabilities
Predictive capabilities are becoming a cornerstone of modern business strategies, enabled by advancements in data analytics and machine learning. These capabilities allow organizations to anticipate future trends and behaviors, leading to more informed decision-making.
- Data-Driven Insights: Predictive analytics utilizes historical data to forecast future outcomes. Businesses can analyze patterns and trends to make proactive decisions, improving operational efficiency. Rapid Innovation can assist clients in implementing predictive analytics solutions that drive strategic decision-making, including applications of predictive analytics in edge computing.
- Customer Behavior Prediction: Companies can use predictive capabilities to understand customer preferences and behaviors. This allows for targeted marketing strategies and personalized customer experiences, ultimately driving sales. Our AI expertise can help businesses develop models that accurately predict customer behavior, leveraging edge computing for real-time insights.
- Maintenance and Operations: In manufacturing and industrial sectors, predictive maintenance can reduce downtime by forecasting equipment failures before they occur. This leads to cost savings and improved productivity. Rapid Innovation can support clients in deploying predictive maintenance solutions that enhance operational reliability, including industrial IoT edge computing.
- Supply Chain Optimization: Predictive analytics can enhance supply chain management by forecasting demand and optimizing inventory levels. This ensures that businesses can meet customer needs without overstocking. Our consulting services can help organizations implement predictive analytics for effective supply chain management, including edge computing infrastructure.
- Risk Management: Organizations can leverage predictive capabilities to identify potential risks and vulnerabilities. This proactive approach allows for the development of strategies to mitigate risks before they impact the business. Rapid Innovation can assist clients in building risk management frameworks that utilize predictive analytics for better resilience, including edge computing in risk management.
In conclusion, edge computing applications, industry developments, and predictive capabilities are interlinked, driving innovation and efficiency across various sectors. As technology continues to evolve, these elements will play a crucial role in shaping the future of business and technology. Rapid Innovation is committed to helping clients navigate this landscape, ensuring they achieve their business goals efficiently and effectively.
8.4. Integration with Emerging Platforms
The integration of emerging technology integration into existing systems is crucial for businesses looking to stay competitive in a rapidly evolving digital landscape. This integration can enhance functionality, improve user experience, and streamline operations.
- Cloud Computing: Many organizations are leveraging cloud platforms like AWS, Google Cloud, and Microsoft Azure to enhance scalability and flexibility. These platforms allow businesses to store and process data more efficiently, enabling real-time analytics and collaboration.
- Artificial Intelligence (AI): Integrating AI tools can automate processes, improve decision-making, and personalize customer experiences. For instance, chatbots powered by AI can handle customer inquiries 24/7, reducing the workload on human agents and allowing businesses to allocate resources more effectively.
- Internet of Things (IoT): IoT devices can collect and transmit data, providing valuable insights into customer behavior and operational efficiency. Businesses can integrate IoT platforms to monitor equipment, track inventory, and enhance supply chain management, ultimately leading to cost savings and improved service delivery.
- Blockchain Technology: This technology offers secure and transparent transactions, making it ideal for industries like finance and supply chain. Integrating blockchain can enhance trust and reduce fraud, ensuring that all parties involved in a transaction have access to the same information.
- Social Media Platforms: Businesses can integrate social media tools to enhance marketing efforts and customer engagement. This includes using APIs to connect social media accounts with customer relationship management (CRM) systems, allowing for more targeted marketing strategies and improved customer interactions.
- Mobile Applications: With the rise of mobile usage, integrating mobile platforms into business operations can improve accessibility and user engagement. Mobile apps can facilitate transactions, provide customer support, and offer personalized content, enhancing the overall customer experience.
- Data Analytics Tools: Integrating advanced analytics platforms can help businesses make data-driven decisions. These tools can analyze customer data, market trends, and operational performance to identify opportunities for growth, enabling organizations to stay ahead of the competition.
- Collaboration Tools: Platforms like Slack, Microsoft Teams, and Zoom have become essential for remote work. Integrating these tools can enhance communication and collaboration among teams, regardless of their physical location, fostering a more productive work environment.
9. Case Studies and Real-World Applications
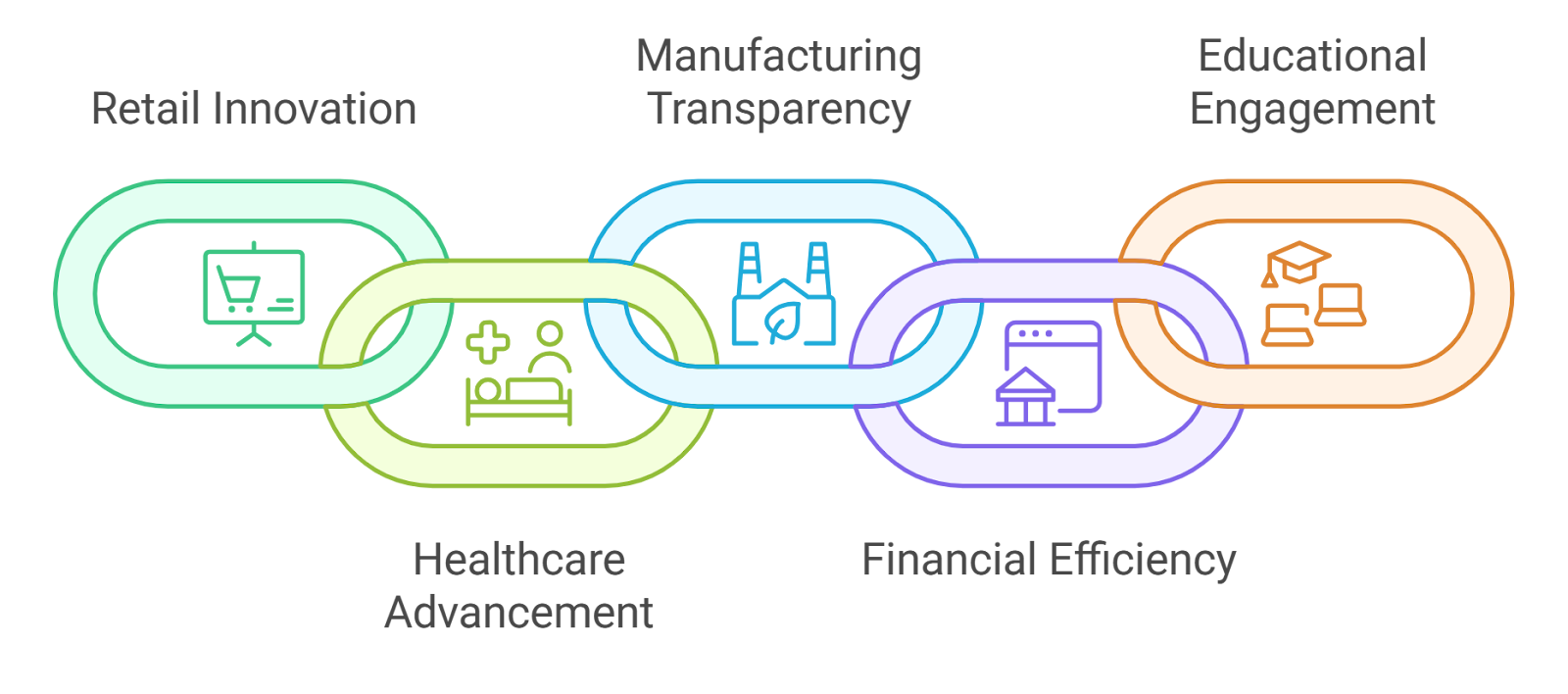
‍
Real-world applications of technology integration provide valuable insights into how businesses can leverage emerging technology integration to drive success. Case studies illustrate the practical benefits and challenges of implementing new technologies.
- Retail Industry: A leading retail chain integrated AI-driven analytics to optimize inventory management. By analyzing customer purchasing patterns, the company reduced stockouts by 30% and improved overall sales.
- Healthcare Sector: A hospital implemented IoT devices to monitor patient vitals in real-time. This integration led to a 20% reduction in emergency response times and improved patient outcomes.
- Manufacturing: A manufacturing firm adopted blockchain technology to enhance supply chain transparency. This integration reduced fraud and improved trust among suppliers, resulting in a 15% decrease in operational costs.
- Financial Services: A bank integrated AI chatbots into its customer service operations. This led to a 40% reduction in response times and increased customer satisfaction ratings.
- Education: An educational institution utilized cloud-based platforms to facilitate remote learning. This integration allowed for seamless access to resources and improved student engagement during the pandemic.
9.1. Enterprise Implementation Examples
Enterprise-level implementation of emerging technology integration can significantly transform operations and drive growth. Here are some notable examples:
- Global E-commerce Company: This company integrated machine learning algorithms to personalize product recommendations. As a result, they experienced a 25% increase in conversion rates and a 15% boost in average order value.
- Automotive Manufacturer: By adopting IoT sensors in their production line, the manufacturer achieved real-time monitoring of equipment performance. This proactive approach reduced downtime by 20% and improved overall efficiency.
- Telecommunications Provider: A major telecom company implemented AI-driven predictive maintenance for its network infrastructure. This integration reduced service outages by 30% and improved customer satisfaction.
- Pharmaceutical Company: This enterprise utilized blockchain technology to track drug shipments. The integration enhanced traceability and compliance, reducing the risk of counterfeit products entering the market.
- Hospitality Industry: A hotel chain integrated mobile applications for guest services, allowing customers to check in, order room service, and request housekeeping through their smartphones. This led to a 50% increase in guest satisfaction scores.
- Energy Sector: An energy company adopted data analytics to optimize energy consumption across its facilities. This integration resulted in a 10% reduction in energy costs and improved sustainability efforts.
- Insurance Provider: By integrating AI for claims processing, an insurance company reduced processing times by 60%. This efficiency not only improved customer experience but also lowered operational costs.
These examples highlight the transformative potential of integrating emerging technology integration into enterprise operations, showcasing how technology can drive efficiency, enhance customer experiences, and ultimately lead to increased profitability. Rapid Innovation is committed to helping businesses navigate this integration process, ensuring they achieve their goals effectively and efficiently.
9.2. Success Metrics and ROI Analysis
Success metrics and roi analysis are critical components in evaluating the effectiveness of any business strategy or marketing campaign. Understanding these metrics helps organizations gauge their performance and make informed decisions.
- Key Performance Indicators (KPIs): Establishing KPIs is essential for measuring success. Common KPIs include revenue growth, customer acquisition cost (CAC), customer lifetime value (CLV), conversion rates, and return on investment (ROI). At Rapid Innovation, we assist clients in defining and tracking KPIs that align with their specific business objectives, ensuring that they can measure success accurately.
- Calculating ROI: ROI is calculated by comparing the net profit of an investment to its cost. The formula is:
language="language-plaintext"ROI = (Net Profit / Cost of Investment) x 100
A positive ROI indicates that the investment has generated more revenue than it cost. Our team leverages advanced AI algorithms to provide precise roi calculations, enabling clients to make data-driven investment decisions.
- Data Analysis Tools: Utilizing data analysis tools can enhance the accuracy of roi calculations. Tools like Google Analytics, Tableau, and Excel can help track performance metrics effectively. Rapid Innovation integrates AI-driven analytics solutions that provide deeper insights into data, allowing clients to optimize their strategies for better returns.
- Benchmarking: Comparing your metrics against industry standards can provide insights into your performance. This can help identify areas for improvement and set realistic goals. We guide clients in benchmarking their performance against industry leaders, helping them to identify gaps and opportunities for growth.
- Continuous Monitoring: Regularly reviewing success metrics allows businesses to adapt strategies in real-time. This agility can lead to better outcomes and increased profitability. Our blockchain solutions facilitate secure and transparent tracking of performance metrics, ensuring that clients can monitor their progress continuously and make timely adjustments.
9.3. Lessons Learned
Every project or campaign offers valuable lessons that can inform future strategies. Analyzing these lessons learned is crucial for continuous improvement.
- Importance of Flexibility: Being adaptable to changes in the market or consumer behavior is vital. Projects may not always go as planned, and flexibility can lead to better outcomes. Rapid Innovation emphasizes agile methodologies, allowing clients to pivot quickly in response to market dynamics.
- Stakeholder Engagement: Engaging stakeholders throughout the process can provide diverse perspectives and enhance decision-making. Regular communication helps align goals and expectations. We facilitate stakeholder workshops that harness collective insights, ensuring that all voices are heard in the decision-making process.
- Data-Driven Decisions: Relying on data rather than assumptions leads to more effective strategies. Analyzing past performance can guide future initiatives and reduce risks. Our AI solutions empower clients to harness big data, transforming it into actionable insights that drive strategic decisions.
- Resource Allocation: Understanding which resources yield the best results can optimize future investments. This includes both financial resources and human capital. Rapid Innovation helps clients analyze resource allocation through predictive modeling, ensuring that investments are directed toward the most impactful initiatives.
- Feedback Loops: Establishing feedback mechanisms allows teams to learn from successes and failures. This can involve surveys, performance reviews, and post-project evaluations. We implement continuous feedback systems that leverage blockchain technology for secure and transparent data collection, enhancing the learning process.
9.4. Industry-Specific Applications
Different industries can leverage unique applications of strategies and technologies to enhance their operations and customer engagement.
- Healthcare: In the healthcare sector, data analytics can improve patient outcomes. Predictive analytics helps in anticipating patient needs and optimizing resource allocation. Rapid Innovation develops AI-driven healthcare solutions that empower providers to deliver personalized care.
- Retail: Retailers can use customer data to personalize shopping experiences. Implementing loyalty programs and targeted marketing campaigns can increase customer retention. Our blockchain solutions ensure secure transactions and enhance customer trust, driving loyalty and repeat business.
- Finance: Financial institutions can utilize machine learning algorithms for fraud detection. This technology analyzes transaction patterns to identify anomalies in real-time. Rapid Innovation's AI solutions provide robust fraud detection mechanisms, safeguarding client assets and enhancing operational efficiency.
- Manufacturing: Industry 4.0 technologies, such as IoT and automation, can streamline production processes. These innovations lead to increased efficiency and reduced operational costs. We assist manufacturers in integrating AI and blockchain technologies to optimize supply chain management and production workflows.
- Education: E-learning platforms can analyze student performance data to tailor educational content. This personalized approach enhances learning outcomes and student engagement. Rapid Innovation develops AI-powered educational tools that adapt to individual learning styles, improving overall educational effectiveness.
10. Implementation Roadmap
An implementation roadmap is crucial for the successful execution of any project, including safe implementation roadmap and scaled agile implementation roadmap. It outlines the steps necessary to achieve the desired outcomes, ensuring that all stakeholders are aligned and aware of their responsibilities. This roadmap typically consists of several phases, including planning and pilot programs.
10.1 Planning Phase
The planning phase is the foundation of the implementation roadmap. It involves detailed preparation and strategizing to ensure that the project is set up for success. Key components of this phase include:
- Defining Objectives: Clearly outline the goals of the project. What do you want to achieve? Setting SMART (Specific, Measurable, Achievable, Relevant, Time-bound) objectives can help in this process.
- Stakeholder Engagement: Identify all stakeholders involved in the project, including team members, management, and external partners. Engaging stakeholders early helps in gathering insights and securing buy-in.
- Resource Allocation: Determine the resources required for the project, including budget, personnel, and technology. Ensure that resources are allocated efficiently to avoid bottlenecks later on.
- Risk Assessment: Conduct a thorough risk analysis to identify potential challenges that may arise during implementation. Develop mitigation strategies to address these risks proactively.
- Timeline Development: Create a detailed timeline that outlines key milestones and deadlines. This helps in tracking progress and ensuring that the project stays on schedule.
- Communication Plan: Establish a communication strategy to keep all stakeholders informed throughout the project. Regular updates and feedback loops are essential for maintaining transparency and engagement.
- Training and Development: Identify any training needs for team members to ensure they have the necessary skills and knowledge to execute the project effectively.
The planning phase sets the stage for a successful implementation. By addressing these components, organizations can minimize risks and enhance the likelihood of achieving their objectives, whether it be through a scaled agile framework implementation roadmap or an agile implementation roadmap.
10.2 Pilot Program
Once the planning phase is complete, the next step is to launch a pilot program. A pilot program serves as a test run for the larger project, allowing organizations to evaluate its feasibility and effectiveness before full-scale implementation. Key aspects of a pilot program include:
- Selection of Pilot Group: Choose a representative sample of users or stakeholders to participate in the pilot. This group should reflect the diversity of the larger population to ensure comprehensive feedback.
- Implementation of Pilot: Execute the pilot program according to the established plan. Monitor the process closely to identify any issues or areas for improvement.
- Data Collection: Gather quantitative and qualitative data during the pilot, including user feedback, performance metrics, and any challenges encountered. Data collection is crucial for assessing the pilot's success.
- Evaluation and Analysis: After the pilot program concludes, analyze the collected data to evaluate its effectiveness. Determine whether the objectives were met and identify any lessons learned.
- Adjustments and Refinements: Based on the evaluation, make necessary adjustments to the project plan. This may involve refining processes, enhancing training, or reallocating resources.
- Stakeholder Feedback: Engage with stakeholders to gather their insights and feedback on the pilot program. Their perspectives can provide valuable information for improving the overall project.
- Decision Making: Finally, based on the results of the pilot program, decide whether to proceed with full-scale implementation, make further adjustments, or reconsider the project altogether.
A pilot program is an essential step in the implementation roadmap. It allows organizations to test their strategies in a controlled environment, reducing the risk of failure during full-scale deployment. By carefully planning and executing a pilot, organizations can ensure a smoother transition to the final implementation phase, whether it involves a power bi implementation roadmap, salesforce implementation roadmap, or devops implementation roadmap.
At Rapid Innovation, we leverage our expertise in AI and Blockchain to guide clients through each phase of the implementation roadmap, including safe agile implementation roadmap and ERP implementation roadmap, ensuring that they achieve greater ROI and operational efficiency. By utilizing advanced analytics and decentralized technologies, we help organizations make informed decisions and optimize their processes, ultimately driving business success.
10.3. Full-Scale Deployment
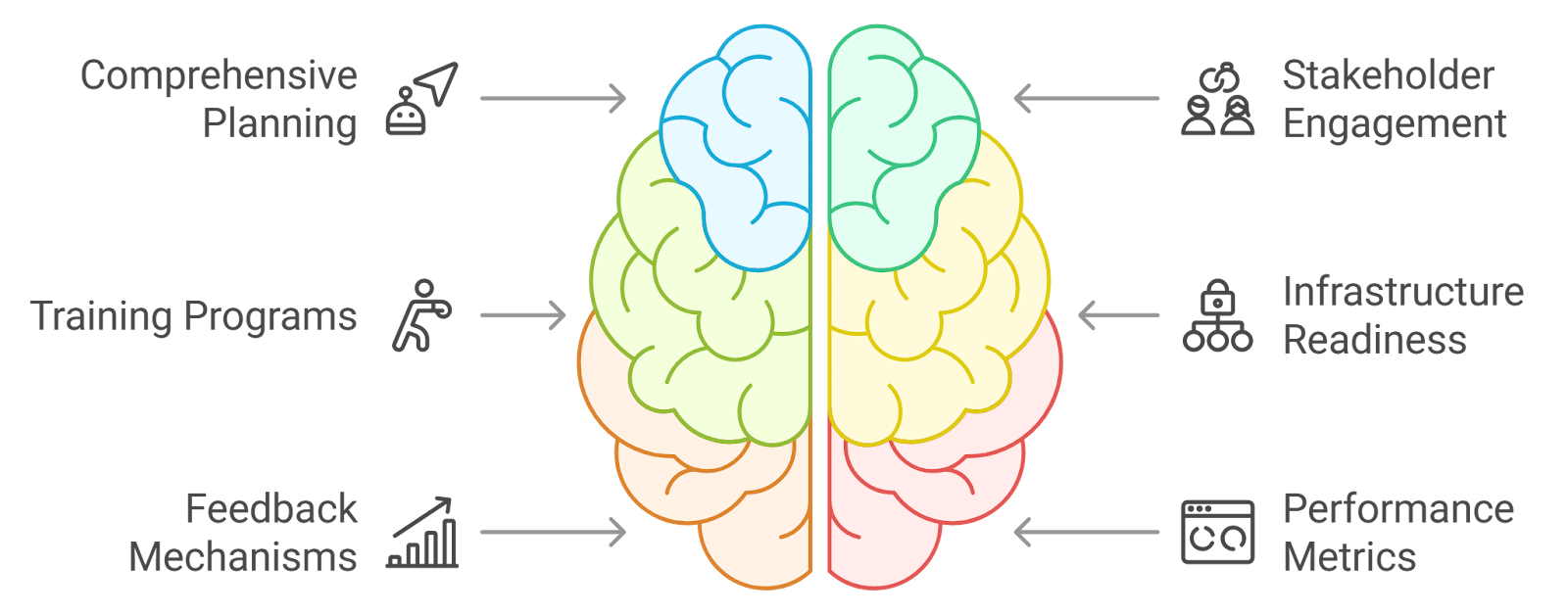
‍
Full-scale deployment is the phase where a project transitions from a pilot or limited implementation to a comprehensive rollout across the organization or target market. This stage is crucial for ensuring that the solution is effectively integrated into existing systems and processes.
- Comprehensive Planning: A detailed deployment plan is essential, including timelines, resource allocation, and risk management strategies. At Rapid Innovation, we leverage AI-driven analytics to optimize planning, ensuring that resources are allocated efficiently and potential risks are mitigated.
- Stakeholder Engagement: Involving all relevant stakeholders, including end-users, management, and IT teams, ensures that everyone is aligned and prepared for the changes. Our approach emphasizes collaboration, utilizing blockchain technology to create transparent communication channels that foster trust and engagement.
- Training Programs: Providing adequate training for users is vital, as it helps minimize resistance and enhances user adoption rates. We design tailored training programs that incorporate AI tools to facilitate learning and ensure users are well-equipped to utilize the new system effectively.
- Infrastructure Readiness: Ensuring that the necessary infrastructure is in place, including hardware and software, is critical for a smooth full-scale deployment. Rapid Innovation assesses existing infrastructure and recommends enhancements, ensuring compatibility with advanced AI and blockchain solutions.
- Feedback Mechanisms: Establishing channels for feedback during the deployment phase allows for real-time adjustments and improvements. We implement AI-driven feedback systems that analyze user input and provide actionable insights for continuous enhancement.
- Performance Metrics: Defining key performance indicators (KPIs) helps measure the success of the full-scale deployment and identify areas for improvement. Our expertise in data analytics enables us to establish relevant KPIs that align with your business objectives, ensuring a clear path to greater ROI. Additionally, our services include custom AI model development to further enhance your deployment strategy. For more insights on effective strategies, check out our article on successful AI integration strategies.
10.4. Monitoring and Optimization
Once a system or solution is deployed, continuous monitoring and optimization are necessary to ensure it operates efficiently and meets organizational goals. This phase focuses on assessing performance and making necessary adjustments.
- Data Collection: Regularly collecting data on system performance, user engagement, and other relevant metrics is essential for informed decision-making. Rapid Innovation employs AI algorithms to automate data collection, providing real-time insights into system performance.
- Performance Analysis: Analyzing the collected data helps identify trends, bottlenecks, and areas that require optimization. Our team utilizes advanced analytics tools to interpret data, enabling proactive adjustments that enhance system efficiency.
- User Feedback: Actively seeking user feedback can provide insights into usability issues and areas for enhancement. We integrate user feedback mechanisms that leverage AI to analyze sentiment and prioritize improvements.
- Iterative Improvements: Implementing changes based on analysis and feedback fosters a culture of continuous improvement. Our agile methodology ensures that updates are made swiftly, keeping your system aligned with evolving business needs.
- Automation Tools: Utilizing monitoring tools can streamline the process of tracking performance and identifying issues in real-time. Rapid Innovation offers AI-powered monitoring solutions that provide alerts and recommendations for immediate action.
- Reporting: Regular reporting on performance metrics keeps stakeholders informed and engaged in the optimization process. We create customized dashboards that visualize key metrics, ensuring transparency and facilitating informed decision-making.
10.5. Evolution and Updates
In a rapidly changing technological landscape, evolution and updates are necessary to keep systems relevant and effective. This phase involves adapting to new trends, technologies, and user needs.
- Regular Updates: Scheduling regular updates ensures that the system remains secure and incorporates the latest features and improvements. Our proactive approach to updates leverages AI to predict necessary enhancements based on user behavior and industry trends.
- User-Centric Design: Continuously evolving the system based on user feedback and changing needs enhances user satisfaction and engagement. We prioritize user experience in our design process, ensuring that updates resonate with end-users.
- Technology Integration: Keeping abreast of emerging technologies allows for the integration of new tools and features that can improve functionality. Rapid Innovation is committed to exploring and integrating cutting-edge technologies, including AI and blockchain, to enhance system capabilities.
- Scalability: Ensuring that the system can scale with the organization’s growth is crucial for long-term success. Our solutions are designed with scalability in mind, allowing for seamless expansion as your business evolves.
- Compliance: Staying updated with industry regulations and compliance requirements is essential to avoid legal issues and maintain trust. We provide guidance on compliance best practices, ensuring that your systems adhere to relevant regulations.
- Strategic Roadmap: Developing a strategic roadmap for future updates and enhancements helps align the evolution of the system with organizational goals. Our consulting services include crafting a tailored roadmap that aligns technology evolution with your business objectives, maximizing ROI.