Table Of Contents
Category
legal
Artificial Intelligence
ARVR
IoT
Blockchain
Retail & Ecommerce
1. Introduction to AI in Educational Prediction
Artificial Intelligence (AI) is transforming various sectors, and education is no exception. The integration of AI in educational prediction is revolutionizing how institutions assess student performance and potential outcomes. By leveraging data analytics and machine learning algorithms, educational institutions can make informed decisions that enhance student success.
AI in educational prediction analyzes vast amounts of data, including student demographics, academic history, and engagement metrics. Predictive analytics helps identify at-risk students early, allowing for timely interventions. Institutions can tailor educational experiences to meet individual student needs, improving overall learning outcomes.
At Rapid Innovation, we specialize in implementing AI-driven solutions that empower educational institutions to harness the full potential of their data. Our expertise in developing customized AI tools enables clients to not only predict student performance but also to create personalized learning pathways that drive engagement and retention.
The use of AI in educational prediction is not just about improving grades; it’s about fostering a supportive learning environment that encourages student growth and achievement. As AI technology continues to evolve, its applications in education will likely expand, offering even more innovative solutions for enhancing student success. Rapid Innovation is committed to guiding institutions through this transformative journey, ensuring they achieve greater ROI through effective AI integration.
Refer to the image for a visual representation of how AI is integrated into educational prediction.
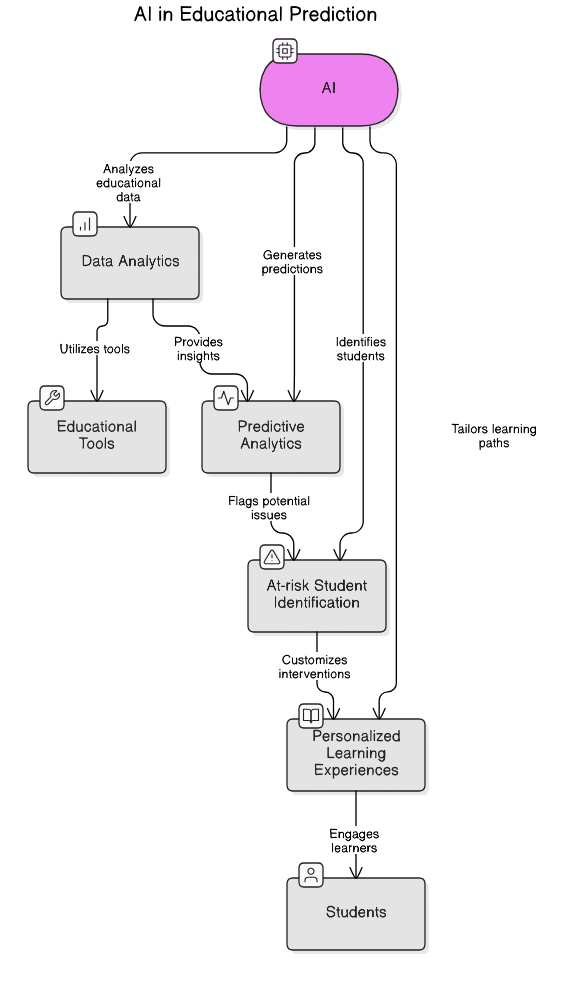
1.1. Defining Student Success Prediction
Student success prediction refers to the process of using data and analytics to forecast a student's likelihood of achieving academic success. This involves identifying key indicators that can signal whether a student will excel or struggle in their educational journey. Key indicators may include:
- Attendance records
- Grades and test scores
- Engagement in class activities
- Socioeconomic background
- Behavioral patterns
The goal of student success prediction is to provide educators and institutions with actionable insights that can help them intervene early and effectively. By leveraging predictive analytics, schools can tailor their support systems to meet the unique needs of each student, ultimately enhancing their chances of success. At Rapid Innovation, we utilize advanced AI algorithms to analyze these indicators, enabling educational institutions to make data-driven decisions that significantly improve student outcomes and return on investment (ROI).
1.2. Evolution of Educational Analytics
Educational analytics has undergone significant transformation over the years, evolving from basic data collection to sophisticated data-driven decision-making processes. The early stages focused on:
- Simple record-keeping
- Manual analysis of student performance
- Limited use of data for decision-making
The introduction of technology brought about:
- Learning Management Systems (LMS) that track student interactions
- Data mining techniques to analyze large datasets
- The rise of dashboards and visualization tools for real-time insights
Current trends in educational analytics include:
- Predictive analytics to forecast student outcomes
- Learning analytics that assess the effectiveness of teaching methods
- Big data applications that integrate various data sources for comprehensive analysis
As educational institutions recognize the value of data, they are increasingly adopting analytics to enhance teaching and learning experiences. Rapid Innovation supports this evolution by providing tailored solutions that integrate AI and blockchain technologies, ensuring data integrity and security while maximizing the effectiveness of educational analytics.
1.3. The Role of AI in Educational Intervention
Artificial Intelligence (AI) plays a pivotal role in educational intervention by providing personalized learning experiences and timely support to students. AI technologies can:
- Analyze vast amounts of data to identify at-risk students
- Offer personalized recommendations for study materials and resources
- Automate administrative tasks, allowing educators to focus on teaching
Key applications of AI in education include:
- Intelligent tutoring systems that adapt to individual learning styles
- Chatbots that provide instant support and answer student queries
- Predictive models that help educators intervene before students fall behind
By harnessing the power of AI, educational institutions can create a more responsive and supportive learning environment, ultimately leading to improved student outcomes. Rapid Innovation's expertise in AI enables us to deliver innovative solutions that empower educators and enhance student success prediction, driving greater ROI for educational institutions.
Refer to the image for a visual representation of student success prediction and its key indicators:
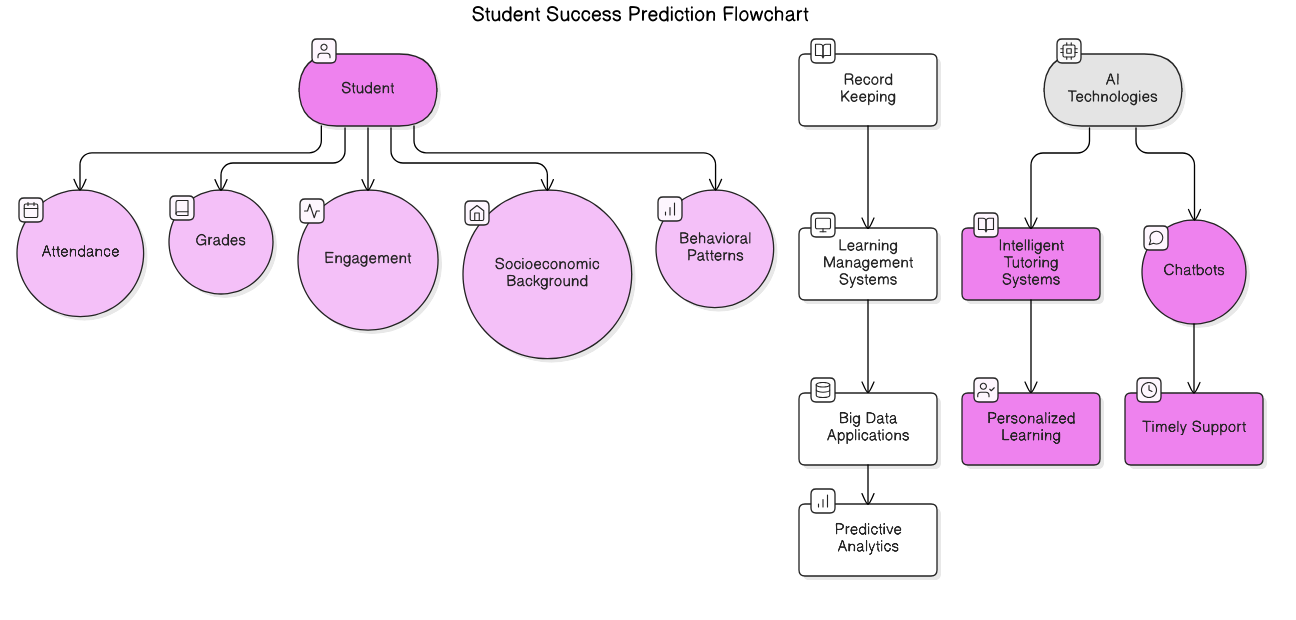
1.4. Ethical Considerations and Challenges
Ethical considerations in education, particularly with the integration of technology, are paramount. As educational institutions increasingly adopt digital tools, several ethical challenges arise that must be addressed to ensure a fair and equitable learning environment.
- Data Privacy: The collection and storage of student data raise significant privacy concerns. Educational platforms often gather personal information, which can be vulnerable to breaches. Institutions must implement robust data protection measures to safeguard student information, potentially leveraging blockchain technology for secure data management.
- Equity and Access: The digital divide remains a critical issue. Not all students have equal access to technology, which can exacerbate existing inequalities. Schools must strive to provide resources and support to ensure all students can benefit from technological advancements, including access to radiology tech school programs and radiology technician schools near me, and AI can help identify and address these gaps.
- Bias in Algorithms: Many educational technologies utilize algorithms that can inadvertently perpetuate biases. For instance, AI-driven tools may favor certain demographics over others, leading to unequal educational opportunities. Continuous monitoring and adjustment of these algorithms are necessary to mitigate bias, a process in which Rapid Innovation can assist through our expertise in AI ethics.
- Informed Consent: Students and parents should be fully informed about how their data will be used. Transparency in data usage policies is essential to build trust and ensure ethical practices in educational settings, an area where blockchain can provide immutable records of consent.
- Impact on Teacher Roles: The rise of technology in education can alter the traditional role of teachers. While technology can enhance learning, it may also lead to a devaluation of the teacher's role, raising questions about the importance of human interaction in education. Rapid Innovation can help institutions navigate this transition by integrating AI tools that support rather than replace educators, including technology for teachers and tech teacher training.
1.5. Paradigm Shift in Educational Support
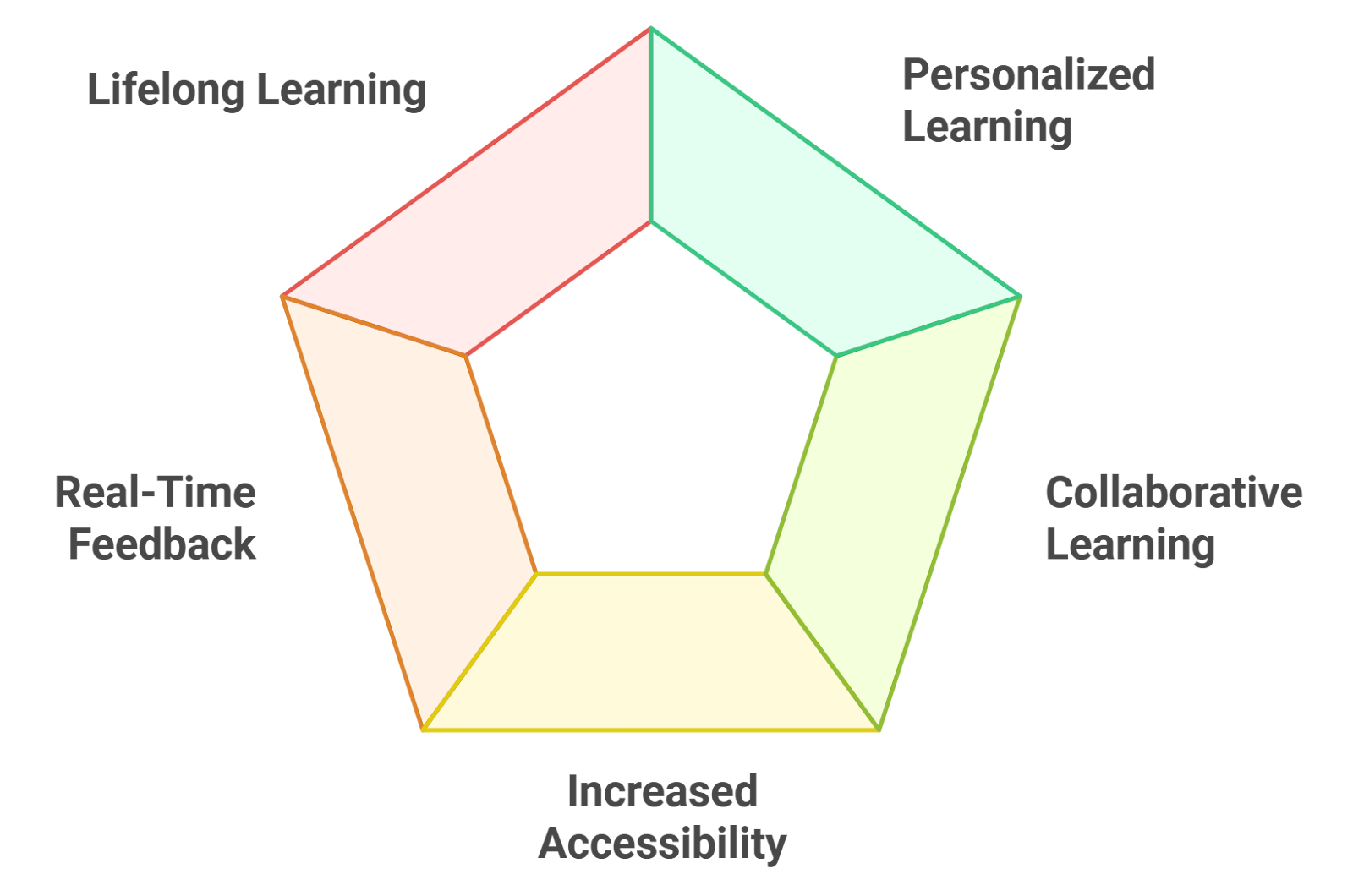
‍
The educational landscape is undergoing a significant paradigm shift, driven by advancements in technology and changing societal needs. This shift is redefining how educational support is delivered and experienced.
- Personalized Learning: Technology enables personalized learning experiences tailored to individual student needs. Adaptive learning platforms assess student performance and adjust content accordingly, fostering a more effective learning environment.
- Collaborative Learning: Online tools facilitate collaboration among students, allowing them to work together regardless of geographical barriers. This shift promotes teamwork and communication skills essential for the modern workforce.
- Increased Accessibility: Digital resources make education more accessible to diverse learners, including those with disabilities. Assistive technologies provide support that helps all students engage with the curriculum, including those in vocational technology programs.
- Real-Time Feedback: Technology allows for immediate feedback on student performance, enabling timely interventions. This shift enhances the learning process by addressing challenges as they arise.
- Lifelong Learning: The paradigm shift emphasizes the importance of lifelong learning. Online courses and resources empower individuals to continue their education beyond traditional schooling, adapting to the ever-changing job market, including options like electronic learning and edtech resources.
2. Foundational Technologies
Foundational technologies are the backbone of modern education, enabling innovative teaching and learning methods. Understanding these technologies is crucial for educators and institutions aiming to enhance educational outcomes.
- Learning Management Systems (LMS): LMS platforms like Moodle and Canvas provide a centralized hub for course materials, assignments, and communication. They streamline administrative tasks and enhance student engagement.
- Artificial Intelligence (AI): AI is transforming education by offering personalized learning experiences, automating administrative tasks, and providing insights into student performance. AI-driven tools can analyze data to identify learning gaps and suggest resources.
- Virtual and Augmented Reality (VR/AR): VR and AR technologies create immersive learning experiences that enhance understanding and retention. These tools allow students to explore complex concepts in a hands-on manner, making learning more engaging.
- Cloud Computing: Cloud technology enables easy access to educational resources and collaboration among students and teachers. It supports remote learning and ensures that materials are available anytime, anywhere.
- Mobile Learning: The proliferation of smartphones and tablets has made mobile learning a vital component of education. Educational apps and resources are now accessible on-the-go, catering to diverse learning styles and preferences.
- Gamification: Incorporating game elements into education can increase motivation and engagement. Gamification strategies, such as rewards and challenges, make learning more enjoyable and encourage active participation.
- Data Analytics: Data analytics tools help educators track student performance and identify trends. By analyzing data, teachers can make informed decisions to improve instructional strategies and support student success.
- Social Media: Social media platforms can be leveraged for educational purposes, fostering communication and collaboration among students and educators. They provide a space for sharing resources and ideas, enhancing the learning community.
By understanding and effectively implementing these foundational technologies, educational institutions can create a more dynamic and responsive learning environment that meets the needs of all students, including those seeking tech schools near me and radiography schools near me. Rapid Innovation stands ready to assist in this transformation, leveraging our expertise in AI and blockchain to enhance educational outcomes and ensure ethical practices.
Refer to the image based on the ethical considerations and challenges in education.

2.1. Machine Learning Fundamentals
Machine learning (ML) is a subset of artificial intelligence (AI) that focuses on the development of algorithms that allow computers to learn from and make predictions based on data. Understanding the fundamentals of machine learning is crucial for leveraging its capabilities in various fields, including education, healthcare, and finance. At Rapid Innovation, we specialize in implementing ML solutions that drive efficiency and enhance decision-making processes for our clients.
- Types of Machine Learning: Â
- Supervised Learning: Involves training a model on labeled data, where the outcome is known. Common algorithms include linear regression, decision trees, and support vector machines. Rapid Innovation employs supervised learning to help clients predict customer behavior, leading to improved marketing strategies and increased ROI.
- Unsupervised Learning: Deals with unlabeled data, aiming to find hidden patterns or intrinsic structures. Techniques include clustering and dimensionality reduction. Our team utilizes unsupervised learning to uncover insights from complex datasets, enabling businesses to identify new market opportunities.
- Reinforcement Learning: Focuses on training models to make sequences of decisions by rewarding desired behaviors and punishing undesired ones. We leverage reinforcement learning to optimize operational processes, resulting in significant cost savings for our clients.
- Key Concepts: Â
- Features and Labels: Features are the input variables used for prediction, while labels are the output variables. Understanding these concepts allows Rapid Innovation to tailor solutions that meet specific client needs.
- Training and Testing: The dataset is typically split into training and testing sets to evaluate the model's performance. Our rigorous testing methodologies ensure that the models we deploy are robust and reliable.
- Overfitting and Underfitting: Overfitting occurs when a model learns noise in the training data, while underfitting happens when it fails to capture the underlying trend. Rapid Innovation employs techniques to mitigate these issues, ensuring optimal model performance.
- Applications: Machine learning is widely used in various applications, such as image recognition, fraud detection, and recommendation systems. By integrating ML into their operations, our clients have seen enhanced customer experiences and increased revenue. This includes designing machine learning systems for specific tasks, applying machine learning in business intelligence, and developing machine learning applications in various sectors.
2.2. Predictive Analytics in Education
Predictive analytics in education involves using statistical techniques and machine learning algorithms to analyze educational data and predict future outcomes. This approach helps educators and institutions make informed decisions to improve student performance and optimize resources. Rapid Innovation offers tailored predictive analytics solutions that empower educational institutions to achieve their goals.
- Benefits of Predictive Analytics: Â
- Early Intervention: Identifying at-risk students early allows for timely support and resources, improving retention rates. Our solutions enable institutions to implement proactive measures that enhance student success.
- Personalized Learning: Tailoring educational experiences based on individual student data enhances engagement and learning outcomes. We help clients create personalized learning pathways that cater to diverse student needs.
- Resource Allocation: Institutions can optimize resource distribution by predicting enrollment trends and course demand. Our analytics tools provide actionable insights that drive efficient resource management.
- Key Techniques: Â
- Data Mining: Extracting patterns from large datasets to inform decision-making. Rapid Innovation employs advanced data mining techniques to uncover valuable insights for educational institutions.
- Regression Analysis: Used to predict student performance based on various factors, such as attendance and previous grades. Our regression models help educators identify key performance indicators and improve academic outcomes.
- Machine Learning Models: Algorithms like decision trees and neural networks can analyze complex relationships in educational data. We leverage these models to provide clients with comprehensive analytics solutions, including machine learning for systems that enhance educational outcomes.
- Challenges: Data privacy concerns and the need for high-quality data can hinder the effective implementation of predictive analytics in education. Rapid Innovation addresses these challenges by ensuring compliance with data protection regulations and implementing robust data management practices.
2.3. Natural Language Processing
Natural Language Processing (NLP) is a branch of artificial intelligence that focuses on the interaction between computers and human language. It enables machines to understand, interpret, and generate human language in a valuable way. At Rapid Innovation, we harness the power of NLP to enhance communication and streamline processes for our clients.
- Core Components of NLP: Â
- Tokenization: Breaking down text into smaller units, such as words or phrases, for analysis. Our NLP solutions utilize tokenization to process and analyze large volumes of text data efficiently.
- Part-of-Speech Tagging: Identifying the grammatical parts of speech in a sentence, which helps in understanding context. This capability allows us to develop more sophisticated language models for our clients.
- Named Entity Recognition: Detecting and classifying key entities in text, such as names, dates, and locations. Our NLP applications can extract critical information from unstructured data, providing valuable insights.
- Applications of NLP: Â
- Sentiment Analysis: Evaluating opinions in text data, useful for understanding customer feedback or social media sentiment. Rapid Innovation employs sentiment analysis to help clients gauge public perception and improve their offerings.
- Chatbots and Virtual Assistants: Enhancing user interaction through conversational agents that can understand and respond to queries. Our custom chatbot solutions improve customer service and engagement for businesses.
- Text Summarization: Automatically generating concise summaries of larger texts, aiding in information retrieval. We develop NLP tools that streamline information processing for our clients, including applications of machine learning in text analysis.
- Challenges in NLP: Â
- Ambiguity in language and the vast diversity of dialects and expressions can complicate NLP tasks. Our team at Rapid Innovation continuously refines our models to address these challenges and improve accuracy.
- Ensuring accuracy and relevance in language models requires continuous training and updates. We provide ongoing support and updates to ensure our clients' NLP solutions remain effective.
By understanding these fundamental concepts of machine learning, including manifold learning and deep learning applications, predictive analytics in education, and natural language processing, individuals and organizations can harness the power of data to drive innovation and improve outcomes across various sectors. Rapid Innovation is committed to helping clients achieve their business goals efficiently and effectively through our expertise in AI and blockchain technologies.
Refer to the image for a visual representation of the concepts discussed in 2.1 Machine Learning Fundamentals:
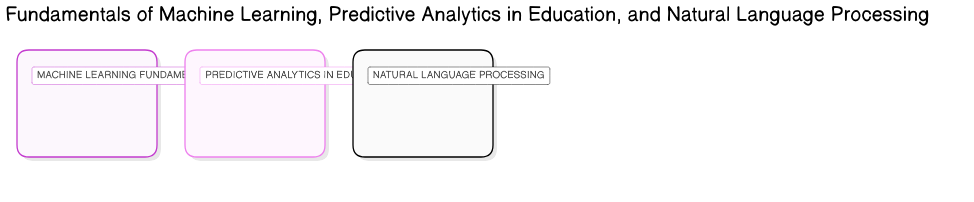
2.4. Deep Learning Architectures
Deep learning architectures are a subset of machine learning that utilize neural networks with many layers to analyze various forms of data. These architectures have gained immense popularity due to their ability to automatically learn features from raw data, making them particularly effective for complex tasks.
- Convolutional Neural Networks (CNNs): Primarily used for image processing and computer vision tasks. They excel in recognizing patterns and features in images through convolutional layers, enabling businesses to enhance their visual data analysis and improve customer engagement through personalized content. Architectures like vgg16 architecture and vgg19 are popular examples of CNNs that have been widely adopted in various applications.
- Recurrent Neural Networks (RNNs): Designed for sequential data, such as time series or natural language. They maintain a memory of previous inputs, making them suitable for tasks like language translation and speech recognition. Rapid Innovation leverages RNNs to help clients automate customer support systems, leading to increased efficiency and reduced operational costs. The architecture of recurrent neural network is crucial for handling such tasks effectively.
- Generative Adversarial Networks (GANs): Comprise two neural networks, a generator and a discriminator, that work against each other. They are used for generating realistic data, such as images or audio, and have applications in art and design. By utilizing GANs, Rapid Innovation assists clients in creating innovative marketing materials and enhancing product design processes.
- Transformer Models: Revolutionized natural language processing (NLP) by allowing for parallel processing of data. They utilize self-attention mechanisms to weigh the importance of different words in a sentence, leading to improved understanding and generation of human language. Rapid Innovation employs transformer models to develop advanced chatbots and virtual assistants, significantly improving user experience and engagement.
- Residual Networks: A type of CNN that introduces skip connections to allow gradients to flow through the network more effectively during training. The resnet18 architecture is a well-known example that has shown remarkable performance in image classification tasks.
- Variational Autoencoders: These architectures are used for generating new data points similar to the training data. They are particularly useful in scenarios where data augmentation is needed.
- Inception V3 Architecture: This architecture is known for its efficiency and effectiveness in image classification tasks, utilizing multiple filter sizes to capture different features.
- Deep Belief Networks: A type of generative model that consists of multiple layers of stochastic, latent variables. They are used for unsupervised learning tasks.
- Deep Neural Network Architecture: A general term that encompasses various architectures with multiple layers, allowing for complex feature extraction and representation learning.
Deep learning architectures have transformed various industries, including healthcare, finance, and entertainment, by enabling advanced analytics and automation, ultimately driving greater ROI for our clients.
2.5. Data Mining Techniques
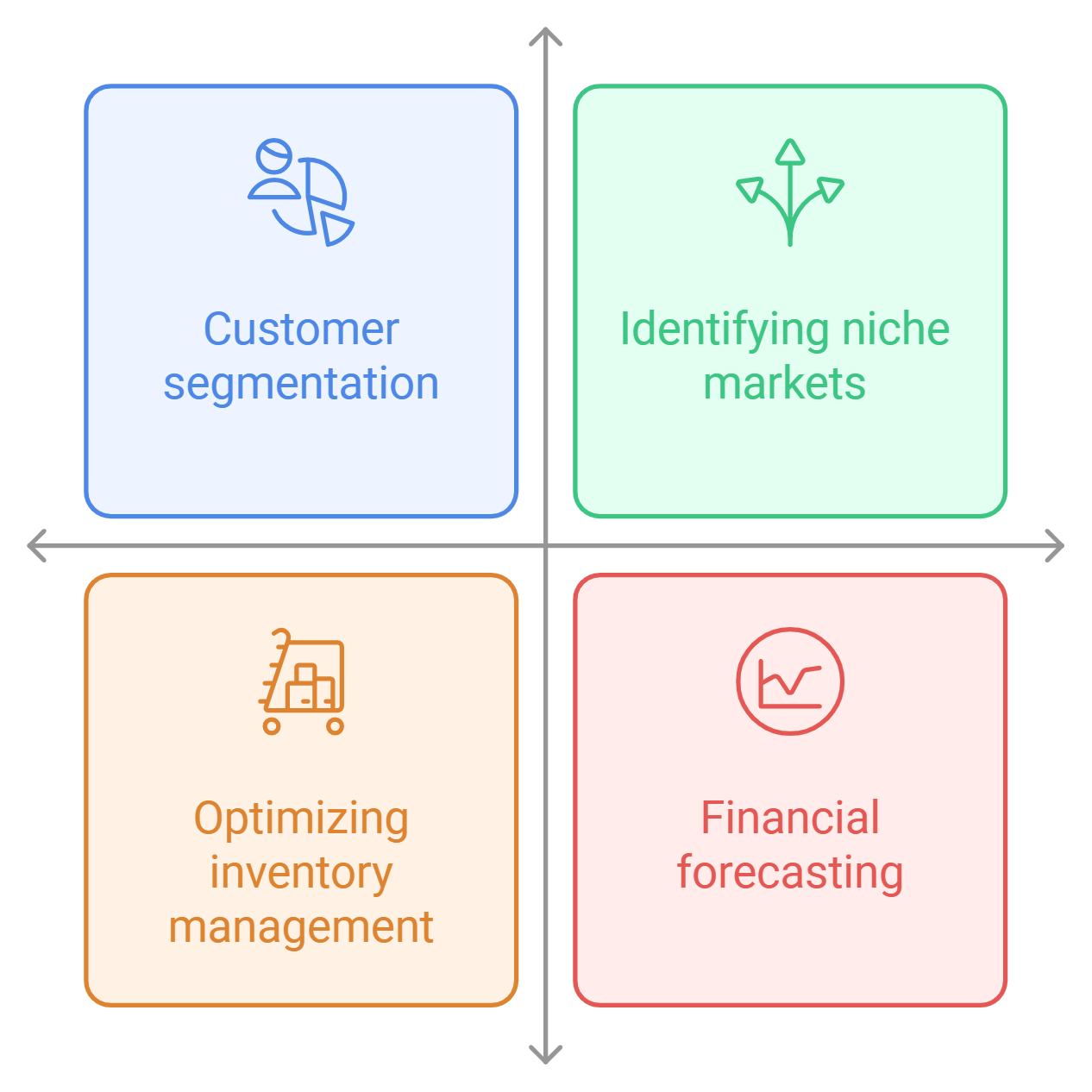
‍
Data mining techniques involve extracting useful information from large datasets. These techniques help organizations uncover patterns, trends, and relationships within their data, leading to informed decision-making.
- Classification: Involves categorizing data into predefined classes. Common algorithms include decision trees, support vector machines, and neural networks. Rapid Innovation utilizes classification techniques to help clients segment their customer base effectively, allowing for targeted marketing strategies that enhance conversion rates.
- Clustering: Groups similar data points together based on their characteristics. Techniques like k-means and hierarchical clustering are widely used for market segmentation and customer profiling. By implementing clustering methods, Rapid Innovation enables clients to identify niche markets and tailor their offerings accordingly.
- Association Rule Learning: Identifies relationships between variables in large datasets. It is often used in market basket analysis to determine which products are frequently purchased together. Rapid Innovation applies this technique to optimize inventory management and cross-selling strategies for retail clients.
- Regression Analysis: Predicts a continuous outcome based on one or more predictor variables. Linear regression and logistic regression are common methods used in forecasting and risk assessment. Our team employs regression analysis to help clients make data-driven decisions, improving their financial forecasting and resource allocation.
Data mining techniques are essential for businesses looking to leverage their data for competitive advantage, enabling them to identify opportunities and mitigate risks.
2.6. Statistical Modeling Approaches
Statistical modeling approaches are fundamental in analyzing data and making predictions based on statistical theory. These methods help researchers and analysts understand relationships between variables and draw conclusions from data.
- Linear Models: These models assume a linear relationship between the independent and dependent variables. They are widely used for their simplicity and interpretability, making them suitable for various applications. Rapid Innovation uses linear models to provide clients with clear insights into their data, facilitating strategic planning.
- Generalized Linear Models (GLMs): Extend linear models to accommodate non-normal response variables. They include logistic regression for binary outcomes and Poisson regression for count data. By employing GLMs, we assist clients in understanding complex relationships within their data, leading to more accurate predictions.
- Time Series Analysis: Focuses on data collected over time to identify trends, seasonal patterns, and cyclic behaviors. Techniques like ARIMA (AutoRegressive Integrated Moving Average) are commonly used for forecasting. Rapid Innovation applies time series analysis to help clients optimize their operations and improve demand forecasting.
- Bayesian Statistics: Incorporates prior knowledge or beliefs into the analysis, allowing for more flexible modeling. Bayesian methods are particularly useful in situations with limited data or when incorporating expert opinion. Our expertise in Bayesian statistics enables clients to make informed decisions even in uncertain environments.
Statistical modeling approaches are crucial for hypothesis testing, data interpretation, and predictive analytics, providing a robust framework for understanding complex datasets and driving business success.
3. Data Sources and Integration
In the realm of education, data sources and integration play a crucial role in understanding student performance data integration and enhancing learning outcomes. By leveraging various data types, educators can create a comprehensive view of student progress and engagement. This section delves into two key areas: academic performance metrics and behavioral and engagement data.
3.1. Academic Performance Metrics
Academic performance metrics are essential for evaluating student success and identifying areas for improvement. These metrics provide quantitative data that can inform instructional strategies and curriculum development. Key academic performance metrics include:
- Grades and Test Scores: Traditional measures of academic success, such as grades and standardized test scores, offer insights into student understanding and mastery of subjects. These scores can be analyzed over time to track progress and identify trends.
- Course Completion Rates: Monitoring the percentage of students who complete courses can highlight potential issues in curriculum design or student support. Low completion rates may indicate a need for additional resources or interventions.
- GPA Trends: Tracking Grade Point Averages (GPA) over time can reveal patterns in student performance. A declining GPA may signal academic struggles, while an increasing GPA can indicate improvement and effective teaching strategies.
- Standardized Assessments: These assessments provide a benchmark for comparing student performance across different demographics and schools. They can help identify achievement gaps and inform targeted interventions.
- Formative Assessments: Regular assessments, such as quizzes and assignments, allow educators to gauge student understanding in real-time. This data can be used to adjust teaching methods and provide timely feedback to students.
Integrating these academic performance metrics into a centralized data system enables educators to analyze trends and make data-driven decisions. By utilizing tools like Learning Management Systems (LMS) and Student Information Systems (SIS), schools can streamline data collection and reporting processes. Rapid Innovation can assist educational institutions in implementing AI-driven analytics solutions that enhance the integration of these metrics, ultimately leading to improved student outcomes and greater ROI.
3.2. Behavioral and Engagement Data
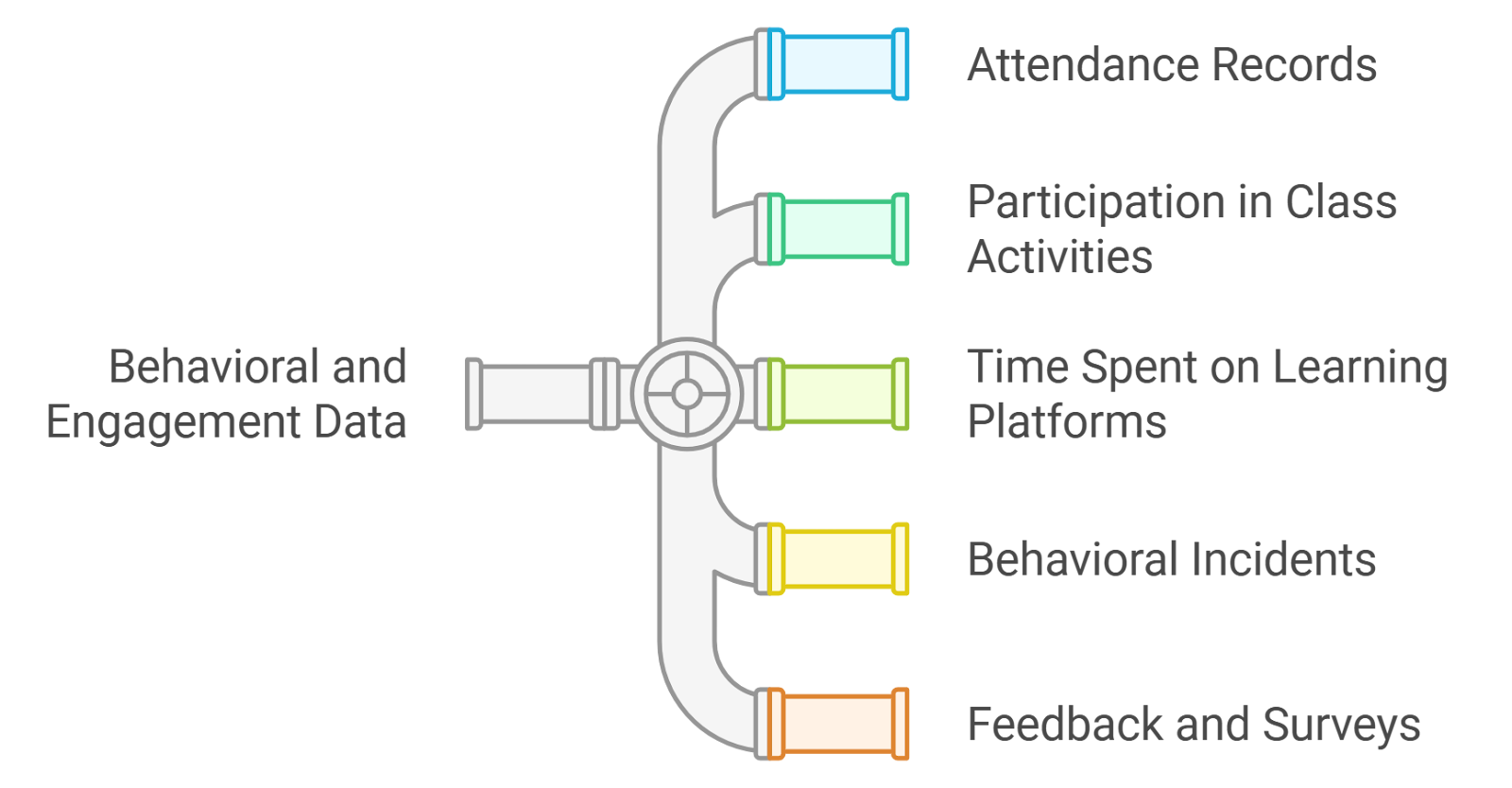
‍
Behavioral and engagement data provide a deeper understanding of how students interact with their learning environment. This type of data can reveal insights into student motivation, participation, and overall engagement. Key components of behavioral and engagement data include:
- Attendance Records: Tracking student attendance is vital for understanding engagement levels. High absenteeism can correlate with lower academic performance and may indicate underlying issues that need to be addressed.
- Participation in Class Activities: Monitoring student participation in discussions, group work, and extracurricular activities can provide insights into their engagement levels. Active participation often correlates with better academic outcomes.
- Time Spent on Learning Platforms: Analyzing the amount of time students spend on educational platforms can help educators assess engagement. Increased time spent on learning activities typically indicates higher levels of interest and commitment.
- Behavioral Incidents: Recording incidents of behavioral issues can help identify patterns that may affect learning. Understanding the context of these behaviors can inform interventions and support strategies.
- Feedback and Surveys: Collecting feedback from students through surveys can provide valuable insights into their perceptions of the learning environment. This data can help educators understand student needs and preferences.
Integrating behavioral and engagement data with academic performance metrics creates a holistic view of student success. By employing data analytics tools, educators can identify correlations between engagement and academic outcomes, allowing for targeted interventions that support student growth. Rapid Innovation's expertise in AI and data integration can empower educational institutions to harness these insights effectively, driving enhanced engagement and improved academic performance.
In conclusion, the integration of academic performance metrics and behavioral engagement data is essential for fostering a data-driven educational environment. By leveraging these data sources, educators can make informed decisions that enhance student learning and overall school performance. Rapid Innovation is committed to providing the necessary tools and consulting services to help educational institutions achieve these goals efficiently and effectively.
3.3. Psychological and Demographic Indicators
Psychological and demographic indicators play a crucial role in understanding the behavior and performance of learners. These indicators can provide insights into how different groups of students engage with educational content and their overall learning experience.
- Psychological Indicators: Â
- Motivation: A learner's motivation can significantly impact their engagement and success. High motivation often leads to better performance and persistence in learning tasks.
- Self-efficacy: This refers to a learner's belief in their ability to succeed. Higher self-efficacy can lead to increased effort and resilience in the face of challenges.
- Learning styles: Understanding whether a student is a visual, auditory, or kinesthetic learner can help tailor educational approaches to meet their needs.
- Demographic Indicators: Â
- Age: Different age groups may have varying levels of familiarity with technology and learning methods, affecting their learning outcomes.
- Gender: Research has shown that gender can influence learning preferences and performance in certain subjects.
- Socioeconomic status: Students from different socioeconomic backgrounds may have access to varying resources, impacting their educational experiences.
By analyzing these psychological and demographic indicators, including learner engagement analysis, educators can create more personalized and effective learning experiences that cater to the diverse needs of their students. At Rapid Innovation, we leverage AI-driven analytics to assess these indicators, enabling educational institutions to enhance learner engagement and optimize course delivery, ultimately leading to improved outcomes and greater ROI. For more insights on this topic, visit AI-Powered Multimodal Learning: Revolutionizing Cross-Disciplinary Innovation.
3.4. Learning Management System (LMS) Data
Learning Management Systems (LMS) are essential tools in modern education, providing a platform for delivering, tracking, and managing training and education. The data generated from LMS can offer valuable insights into learner behavior and course effectiveness.
- User Engagement: Â
- Tracking login frequency and time spent on the platform can help identify engaged learners versus those who may be struggling.
- Monitoring participation in discussions and activities can provide insights into student engagement levels.
- Performance Metrics: Â
- Assessment scores: Analyzing quiz and exam results can help identify areas where students excel or struggle.
- Completion rates: Understanding how many learners complete courses can indicate the effectiveness of the content and delivery methods.
- Feedback and Surveys: Â
- Collecting feedback through surveys can help educators understand learner satisfaction and areas for improvement.
- Analyzing qualitative data from open-ended responses can provide deeper insights into the learner experience.
Utilizing LMS data effectively can lead to improved course design, enhanced learner engagement, and better educational outcomes. Rapid Innovation employs advanced AI algorithms to analyze LMS data, providing actionable insights that help institutions refine their educational strategies and maximize their return on investment.
3.5. External Environmental Factors
External environmental factors can significantly influence the learning process and outcomes. These factors encompass a wide range of elements that exist outside the educational institution but can impact students' learning experiences.
- Economic Factors: Â
- Economic stability can affect funding for educational institutions, impacting resources available for students.
- Job market trends can influence students' choices in courses and career paths, affecting enrollment numbers.
- Technological Advancements: Â
- The rapid evolution of technology can create both opportunities and challenges for learners. Access to the latest tools can enhance learning, while a lack of access can hinder it.
- Online learning platforms and resources can provide additional support but may also lead to information overload.
- Cultural and Social Influences: Â
- Cultural background can shape a learner's attitudes towards education and learning styles.
- Social support systems, including family and peer influences, can impact motivation and engagement levels.
Understanding these external environmental factors is essential for educators and institutions to create supportive learning environments that address the diverse needs of their students. Rapid Innovation's expertise in AI and Blockchain can help educational institutions navigate these external factors, ensuring they remain agile and responsive to the changing landscape, thereby enhancing their overall effectiveness and ROI.
3.6. Data Collection and Privacy Considerations
Data collection is a critical aspect of any AI system, particularly when it comes to ensuring user privacy and compliance with regulations. As AI agents gather data to improve their functionality, it is essential to consider the implications of this data collection on user privacy, especially in the context of AI and privacy concerns.
- User Consent: Obtaining explicit consent from users before collecting their data is paramount. This not only builds trust but also aligns with legal requirements such as the General Data Protection Regulation (GDPR) in Europe. Rapid Innovation emphasizes the importance of user consent in our AI solutions, ensuring that clients can confidently engage with their users while adhering to legal standards, particularly in relation to AI privacy and security.
- Data Minimization: Collect only the data that is necessary for the AI agent to function effectively. This principle of data minimization helps reduce the risk of privacy breaches and ensures compliance with privacy laws. Our development practices at Rapid Innovation focus on implementing data minimization strategies, which not only protect user privacy but also enhance system efficiency, addressing concerns related to AI data privacy.
- Anonymization Techniques: Implementing anonymization techniques can help protect user identities. By removing personally identifiable information (PII) from datasets, organizations can mitigate risks associated with data breaches. Rapid Innovation integrates advanced anonymization methods into our AI systems, allowing clients to leverage data insights without compromising user privacy, which is crucial in discussions about artificial intelligence and data protection.
- Transparency: Clearly communicate to users what data is being collected, how it will be used, and who it will be shared with. Transparency fosters trust and allows users to make informed decisions about their data. We guide our clients in establishing transparent data practices, which can lead to improved user engagement and loyalty, particularly in the context of privacy in AI.
- Data Security Measures: Employ robust security measures to protect collected data from unauthorized access. This includes encryption, secure storage solutions, and regular security audits. At Rapid Innovation, we prioritize data security in our AI and blockchain solutions, ensuring that our clients' data is safeguarded against potential threats, addressing concerns such as chatgpt data privacy.
- User Rights: Ensure that users are aware of their rights regarding their data, including the right to access, rectify, or delete their information. This empowers users and enhances their control over personal data. We assist our clients in developing user-centric policies that respect and uphold user rights, fostering a positive relationship with their audience, which is essential in the context of data privacy and AI.
- Compliance with Regulations: Stay updated on local and international data protection laws to ensure compliance. Non-compliance can lead to significant legal repercussions and damage to reputation. Rapid Innovation provides consulting services to help clients navigate the complex landscape of data protection regulations, ensuring they remain compliant and avoid potential pitfalls, especially regarding artificial intelligence and privacy.
4. AI Agent Architectural Design
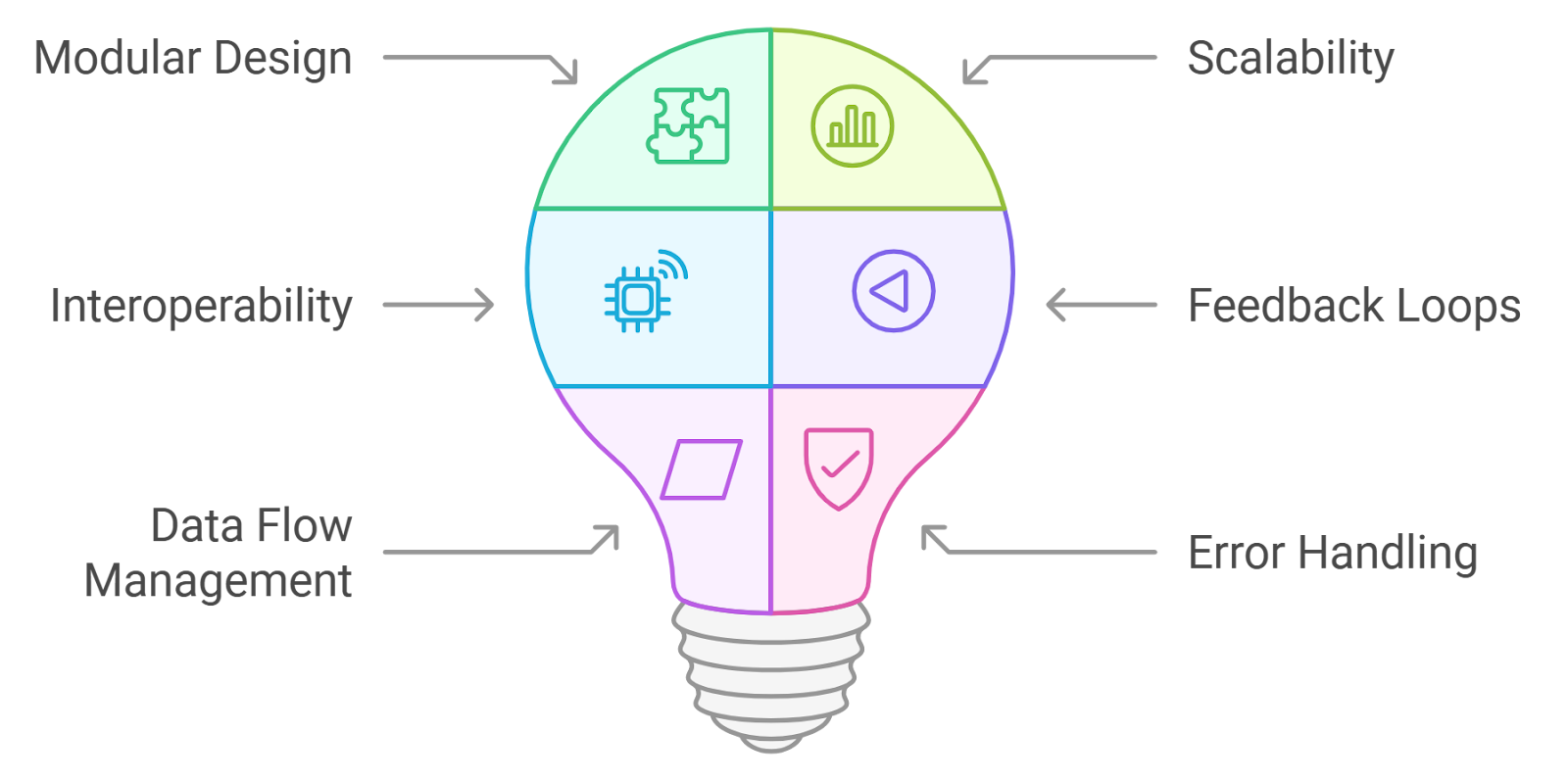
‍
The architectural design of AI agents is crucial for their performance, scalability, and adaptability. A well-structured architecture allows AI agents to process data efficiently and respond to user needs effectively.
- Modular Design: A modular architecture enables different components of the AI agent to function independently. This allows for easier updates and maintenance, as well as the ability to integrate new functionalities without overhauling the entire system. Rapid Innovation employs modular design principles in our AI solutions, facilitating seamless upgrades and enhancements.
- Scalability: The architecture should be designed to scale easily as the user base grows. This involves considering cloud-based solutions that can handle increased data loads and user interactions. Our team at Rapid Innovation ensures that scalability is a core feature of our AI systems, allowing clients to grow without limitations.
- Interoperability: AI agents should be able to communicate and interact with other systems and platforms. This requires the use of standard protocols and APIs to ensure seamless integration. Rapid Innovation focuses on creating interoperable AI solutions that can easily integrate with existing systems, maximizing the value of our clients' investments.
- Feedback Loops: Incorporating feedback loops into the architecture allows the AI agent to learn from user interactions continuously. This enhances the agent's ability to adapt and improve over time. We design our AI agents with feedback mechanisms that enable ongoing learning, ensuring that they remain relevant and effective.
- Data Flow Management: Efficient data flow management is essential for processing large volumes of data. This includes optimizing data pipelines and ensuring that data is processed in real-time when necessary. Rapid Innovation implements advanced data flow management techniques to enhance the performance of our AI solutions.
- Error Handling: Robust error handling mechanisms should be integrated into the architecture to manage unexpected issues gracefully. This ensures that the AI agent remains functional even in the face of errors. Our development practices include comprehensive error handling strategies, ensuring reliability and user satisfaction.
4.1. Agent Conceptual Framework
The conceptual framework for AI agents serves as a blueprint for understanding their functionality and interactions within various environments. This framework outlines the key components and processes that define how AI agents operate.
- Perception: AI agents must be able to perceive their environment through data collection methods such as sensors, APIs, or user inputs. This perception is the foundation for decision-making. Rapid Innovation designs AI agents with sophisticated perception capabilities, enabling them to gather and analyze data effectively.
- Reasoning: Once data is collected, the agent must analyze and interpret it. This involves applying algorithms and models to derive insights and make informed decisions. Our expertise in AI algorithms ensures that our agents can reason accurately and provide valuable insights to clients.
- Action: After reasoning, the AI agent must take action based on its conclusions. This could involve providing recommendations, executing tasks, or interacting with users. Rapid Innovation focuses on creating AI agents that can take meaningful actions, driving efficiency and productivity for our clients.
- Learning: Continuous learning is a vital aspect of AI agents. They should be designed to improve their performance over time by learning from past experiences and user interactions. Our AI solutions incorporate advanced learning techniques, ensuring that agents evolve and adapt to changing environments.
- Environment Interaction: The framework should account for how the AI agent interacts with its environment, including other agents, users, and external systems. This interaction is crucial for the agent's effectiveness. Rapid Innovation emphasizes the importance of environment interaction in our designs, ensuring that agents can operate seamlessly within their ecosystems.
- Ethical Considerations: Incorporating ethical considerations into the conceptual framework ensures that AI agents operate within acceptable moral boundaries. This includes fairness, accountability, and transparency in decision-making processes. We guide our clients in implementing ethical AI practices, fostering trust and responsibility in their operations.
- User-Centric Design: The framework should prioritize user experience, ensuring that AI agents are intuitive and easy to interact with. This enhances user satisfaction and engagement. Rapid Innovation champions user-centric design principles, creating AI agents that resonate with users and drive engagement.
By focusing on these elements, organizations can create effective AI agents that meet user needs while adhering to privacy and ethical standards. Rapid Innovation is committed to helping clients achieve their business goals efficiently and effectively through our expertise in AI and blockchain technologies, while addressing concerns related to AI invading privacy and data protection in artificial intelligence.
4.2. Predictive Modeling Techniques
Predictive modeling techniques are essential in various fields, including finance, healthcare, marketing, and more. These techniques utilize statistical algorithms and machine learning to identify patterns in historical data and predict future outcomes.
- Common predictive modeling techniques include: Â
- Regression Analysis: This technique estimates the relationships among variables and helps in predicting a dependent variable based on one or more independent variables.
- Decision Trees: These are flowchart-like structures that assist in making decisions based on certain conditions. They are easy to interpret and visualize.
- Neural Networks: Inspired by the human brain, neural networks consist of interconnected nodes that process data in layers, making them particularly effective for complex datasets.
- Time Series Analysis: This technique analyzes data points collected or recorded at specific time intervals and is widely used for forecasting trends over time, including time series forecasting machine learning and time series forecasting using machine learning.
- Applications of predictive modeling: Â
- Customer Segmentation: Businesses can identify different customer groups and tailor marketing strategies accordingly, leading to more effective campaigns and higher conversion rates.
- Risk Assessment: Financial institutions use predictive models to assess the risk of loan defaults, enabling them to make informed lending decisions and minimize losses.
- Disease Prediction: In healthcare, predictive modeling can forecast disease outbreaks or patient outcomes, allowing for proactive measures and better resource allocation.
Predictive modeling relies heavily on data quality and quantity. The more accurate and comprehensive the data, the better the predictions. At Rapid Innovation, we leverage these techniques, including applied predictive modeling and predictive analytics modeling techniques, to help our clients optimize their operations and achieve greater ROI through data-driven decision-making. For more insights on predictive modeling, check out our definitive guide on object detection in 2024.
4.3. Real-time Monitoring Systems
Real-time monitoring systems are crucial for tracking and analyzing data as it happens. These systems provide immediate insights, enabling organizations to make timely decisions and respond to events as they unfold.
- Key features of real-time monitoring systems include: Â
- Data Collection: Continuous data gathering from various sources, such as sensors, databases, and user inputs.
- Data Processing: Immediate processing of incoming data to extract meaningful information.
- Alerts and Notifications: Automated alerts that notify users of significant changes or anomalies in the data.
- Benefits of real-time monitoring systems: Â
- Enhanced Decision-Making: Organizations can make informed decisions based on the latest data, improving responsiveness and agility.
- Improved Efficiency: Real-time insights help in optimizing operations and resource allocation, leading to cost savings.
- Proactive Issue Resolution: Early detection of issues allows for quick intervention, minimizing potential damage or loss.
Real-time monitoring is widely used in various sectors, including: - Manufacturing: Monitoring equipment performance to prevent downtime and enhance productivity. - Healthcare: Tracking patient vitals for immediate medical response, improving patient care. - Finance: Monitoring transactions for fraud detection, safeguarding assets and maintaining trust.
The integration of IoT (Internet of Things) devices has significantly enhanced the capabilities of real-time monitoring systems, allowing for more comprehensive data collection and analysis. Rapid Innovation specializes in developing tailored real-time monitoring solutions that empower our clients to stay ahead of the curve.
4.4. Adaptive Learning Algorithms
Adaptive learning algorithms are designed to improve their performance over time by learning from new data and experiences. These algorithms adjust their parameters automatically based on the input they receive, making them highly effective in dynamic environments.
- Characteristics of adaptive learning algorithms: Â
- Self-Improvement: They continuously refine their models based on new data, leading to better accuracy and performance.
- Flexibility: These algorithms can adapt to changes in data patterns, making them suitable for various applications across industries.
- Personalization: They can tailor experiences or recommendations based on individual user behavior, enhancing user engagement.
- Common types of adaptive learning algorithms: Â
- Reinforcement Learning: This type of algorithm learns by receiving feedback from its actions, optimizing its strategy over time for better outcomes.
- Online Learning: This approach allows models to update continuously as new data arrives, rather than retraining from scratch, ensuring relevance.
- Ensemble Methods: These combine multiple models to improve prediction accuracy and robustness, leading to more reliable results, including ensemble modeling machine learning.
- Applications of adaptive learning algorithms: Â
- E-commerce: Personalizing product recommendations based on user behavior, increasing sales and customer satisfaction.
- Autonomous Vehicles: Adapting driving strategies based on real-time traffic conditions, enhancing safety and efficiency.
- Fraud Detection: Continuously learning from new transaction data to identify fraudulent activities, protecting businesses and consumers alike, such as credit card fraud detection using predictive modeling.
Adaptive learning algorithms are becoming increasingly important in the age of big data, where the ability to learn and adapt quickly can provide a competitive edge. At Rapid Innovation, we harness the power of these algorithms to deliver innovative solutions that drive business success and maximize ROI for our clients.
4.5. Multivariate Prediction Models
Multivariate prediction models are statistical techniques used to predict outcomes based on multiple variables. These models are essential in various fields, including finance, healthcare, marketing, and social sciences, where multiple factors influence the outcome.
- Definition: Multivariate prediction models analyze the relationship between two or more independent variables and one dependent variable. They help in understanding how different factors interact and contribute to the predicted outcome.
- Types of Models: Â
- Multiple Linear Regression: This model predicts the dependent variable by fitting a linear equation to observed data. It assumes a linear relationship between the independent variables and the dependent variable.
- Logistic Regression: Used for binary outcomes, logistic regression estimates the probability of a certain event occurring based on independent variables.
- Multivariate Adaptive Regression Splines (MARS): This non-parametric regression technique models complex relationships by fitting piecewise linear regressions.
- Applications: Â
- Healthcare: Predicting patient outcomes based on various health indicators, enabling healthcare providers to tailor treatments and improve patient care.
- Finance: Assessing credit risk by analyzing multiple financial indicators, allowing financial institutions to make informed lending decisions and reduce default rates.
- Marketing: Understanding customer behavior by evaluating multiple demographic and psychographic factors, which helps businesses optimize their marketing strategies and increase conversion rates.
- Benefits: Â
- Provides a comprehensive view of how multiple factors influence outcomes, allowing organizations to make data-driven decisions.
- Enhances predictive accuracy by considering interactions between variables, leading to more reliable forecasts.
- Helps in identifying key drivers of change in complex systems, enabling businesses to focus on critical areas for improvement.
4.6. Explainable AI Mechanisms
Explainable AI (XAI) mechanisms are essential for understanding and interpreting the decisions made by artificial intelligence systems. As AI becomes more integrated into decision-making processes, the need for transparency and accountability grows.
- Importance of Explainability: Â
- Trust: Users are more likely to trust AI systems that provide clear explanations for their decisions, fostering greater adoption of AI technologies.
- Compliance: Regulatory frameworks increasingly require transparency in AI decision-making, especially in sensitive areas like finance and healthcare, ensuring that organizations meet legal standards.
- Debugging: Explainable models help developers identify and rectify errors in AI systems, improving overall system reliability.
- Techniques for Explainability: Â
- Feature Importance: Identifying which features most significantly impact the model's predictions, allowing stakeholders to understand the rationale behind decisions.
- LIME (Local Interpretable Model-agnostic Explanations): A technique that explains individual predictions by approximating the model locally with an interpretable one, enhancing user comprehension.
- SHAP (SHapley Additive exPlanations): A method that assigns each feature an importance value for a particular prediction, based on cooperative game theory, providing a robust framework for understanding model outputs.
- Applications: Â
- Healthcare: Explaining AI-driven diagnoses to medical professionals and patients, ensuring that treatment decisions are well-informed and justifiable.
- Finance: Providing insights into credit scoring models to ensure fairness and transparency, which is crucial for maintaining customer trust.
- Legal: Justifying AI decisions in legal contexts to ensure accountability, thereby supporting ethical AI practices.
- Challenges: Â
- Balancing model complexity with interpretability, as more complex models may yield better performance but are harder to explain.
- Ensuring explanations are understandable to non-experts, which is vital for broader acceptance of AI technologies.
- Addressing biases in AI systems that may affect explanations, ensuring fairness and equity in AI-driven decisions.
5. Predictive Modeling Approaches

‍
Predictive modeling approaches are techniques used to forecast future outcomes based on historical data. These approaches leverage statistical algorithms and machine learning to identify patterns and make predictions.
- Types of Predictive Modeling Approaches: Â
- Statistical Methods: Traditional techniques like regression analysis, time series analysis, and Bayesian models that provide foundational insights.
- Machine Learning: Algorithms such as decision trees, random forests, support vector machines, and neural networks that learn from data to make predictions, enhancing predictive capabilities. This includes predictive analytics modeling and predictive modeling techniques.
- Hybrid Models: Combining statistical methods with machine learning techniques to enhance predictive accuracy, offering a more robust solution.
- Key Steps in Predictive Modeling: Â
- Data Collection: Gathering relevant historical data that will inform the model, ensuring a solid foundation for predictions.
- Data Preprocessing: Cleaning and transforming data to ensure quality and consistency, which is critical for model performance.
- Model Selection: Choosing the appropriate modeling technique based on the problem and data characteristics, aligning with business objectives. This may involve applied predictive modeling or predictive modeling methods.
- Model Training: Using historical data to train the model, allowing it to learn patterns and improve accuracy.
- Model Evaluation: Assessing the model's performance using metrics like accuracy, precision, recall, and F1 score, ensuring reliability.
- Deployment: Implementing the model in a real-world setting for ongoing predictions, facilitating continuous improvement.
- Applications: Â
- Retail: Forecasting sales and inventory needs based on historical purchasing patterns, enabling better stock management and customer satisfaction.
- Finance: Predicting stock prices and market trends using historical financial data, assisting investors in making informed decisions. This includes credit card fraud detection using predictive modeling.
- Manufacturing: Anticipating equipment failures to schedule maintenance proactively, reducing downtime and operational costs.
- Benefits: Â
- Enables data-driven decision-making, empowering organizations to leverage insights for strategic planning.
- Improves operational efficiency by anticipating future needs, leading to cost savings and resource optimization.
- Enhances customer satisfaction through personalized experiences, fostering loyalty and repeat business.
- Challenges: Â
- Data quality and availability can significantly impact model performance, necessitating robust data management practices.
- Overfitting can occur when models are too complex, leading to poor generalization, which must be carefully managed.
- Keeping models updated with new data is crucial for maintaining accuracy, ensuring that predictions remain relevant and actionable.
At Rapid Innovation, we leverage these advanced predictive modeling techniques, including predictive analytics and modeling, and explainable AI mechanisms to help our clients achieve their business goals efficiently and effectively, ultimately driving greater ROI.
5.1. Early Warning Systems
Early Warning Systems (EWS) are critical tools designed to predict and mitigate the impact of potential disasters, whether natural or man-made. These systems utilize a combination of technology, data analysis, and communication strategies to provide timely alerts to communities at risk.
- EWS typically involve: Â
- Monitoring environmental conditions through sensors and satellite imagery.
- Analyzing data to identify patterns that may indicate an impending disaster.
- Disseminating alerts through various channels, including mobile apps, social media, and traditional media.
- The effectiveness of EWS relies on: Â
- Accurate data collection and analysis, which can be enhanced through AI algorithms that process vast amounts of data in real-time.
- Community engagement and education to ensure that people understand alerts and know how to respond.
- Collaboration among government agencies, NGOs, and local communities, facilitated by blockchain technology for secure and transparent communication.
- Examples of successful EWS include: Â
- The Tsunami Warning System in the Pacific, which has saved countless lives by providing timely alerts.
- Flood warning systems in various countries that utilize real-time data to inform residents of rising water levels.
5.2. Risk Assessment Methodologies
Risk assessment methodologies are systematic approaches used to identify, evaluate, and prioritize risks associated with various hazards. These methodologies help organizations and governments make informed decisions about resource allocation and risk management strategies.
- Key components of risk assessment methodologies include: Â
- Hazard identification: Recognizing potential threats, such as natural disasters, technological failures, or human actions.
- Vulnerability analysis: Assessing the susceptibility of people, property, and infrastructure to the identified hazards.
- Impact evaluation: Estimating the potential consequences of hazards on communities and economies.
- Common methodologies used in risk assessment include: Â
- Qualitative assessments, which rely on expert judgment and stakeholder input.
- Quantitative assessments, which use statistical models and historical data to predict risks, often enhanced by AI-driven predictive analytics.
- Hybrid approaches that combine both qualitative and quantitative methods for a comprehensive analysis.
- Effective risk assessment methodologies lead to: Â
- Improved preparedness and response strategies.
- Enhanced understanding of community vulnerabilities.
- Better allocation of resources to mitigate risks.
5.3. Performance Trajectory Mapping
Performance trajectory mapping is a strategic tool used to visualize and analyze the progress of projects, programs, or policies over time. This method helps organizations track their performance against established goals and objectives.
- Key elements of performance trajectory mapping include: Â
- Defining clear performance indicators that align with organizational goals.
- Collecting data regularly to assess progress and identify trends, utilizing AI for real-time data processing.
- Creating visual representations, such as graphs or charts, to illustrate performance trajectories.
- Benefits of performance trajectory mapping include: Â
- Enhanced decision-making through data-driven insights.
- Identification of areas needing improvement or adjustment.
- Increased accountability among team members and stakeholders.
- Applications of performance trajectory mapping can be found in: Â
- Public health initiatives, where tracking vaccination rates or disease outbreaks is crucial.
- Environmental programs, monitoring changes in biodiversity or pollution levels.
- Economic development projects, assessing job creation or investment impacts.
By implementing early warning systems, risk assessment methodologies, and performance trajectory mapping, organizations can significantly enhance their ability to manage risks and improve overall resilience. Rapid Innovation leverages AI and blockchain technologies to optimize these processes, ensuring clients achieve greater ROI through efficient and effective solutions.
5.4. Personalized Intervention Strategies
Personalized intervention strategies are tailored approaches designed to meet the unique needs of individuals based on their specific characteristics, preferences, and circumstances. These strategies are increasingly being utilized in various fields, including healthcare, education, and mental health.
- Individual Assessment: Â
- Conduct thorough assessments to understand the individual's background, strengths, and challenges.
- Use data-driven insights to inform the development of personalized intervention strategies.
- Customization of Interventions: Â
- Design interventions that are flexible and adaptable to the individual's evolving needs.
- Incorporate various modalities, such as cognitive-behavioral therapy, medication, or lifestyle changes, depending on the individual’s situation.
- Continuous Monitoring and Feedback: Â
- Implement systems for ongoing monitoring of the individual's progress.
- Use feedback loops to adjust interventions as necessary, ensuring they remain effective.
- Engagement and Motivation: Â
- Foster a sense of ownership in the intervention process by involving individuals in decision-making.
- Utilize motivational interviewing techniques to enhance engagement and adherence to the intervention.
- Technology Integration: Â
- Leverage technology, such as mobile apps and telehealth, to deliver personalized interventions efficiently.
- Use data analytics to track outcomes and refine strategies over time.
At Rapid Innovation, we harness the power of AI to develop personalized intervention strategies that not only enhance individual outcomes but also drive greater ROI for organizations. By utilizing advanced data analytics and machine learning, we can create tailored solutions that adapt to the unique needs of each client, ensuring that resources are allocated effectively and efficiently. For more information on how AI predicts customer trends and behavior, visit our Adaptive AI Development page.
5.5. Machine Learning Classification Models
Machine learning classification models are algorithms that categorize data into predefined classes based on input features. These models are widely used in various applications, including image recognition, spam detection, and medical diagnosis.
- Types of Classification Models: Â
- Decision Trees: Simple models that split data into branches based on feature values.
- Support Vector Machines (SVM): Effective for high-dimensional spaces, SVMs find the optimal hyperplane that separates classes.
- Neural Networks: Complex models that mimic the human brain, capable of learning intricate patterns in data.
- Data Preparation: Â
- Clean and preprocess data to ensure quality inputs for the model.
- Split data into training and testing sets to evaluate model performance accurately.
- Feature Selection: Â
- Identify the most relevant features that contribute to classification accuracy.
- Use techniques like recursive feature elimination or LASSO regression to enhance model efficiency.
- Model Training and Evaluation: Â
- Train models using labeled datasets to learn the relationship between features and classes.
- Evaluate model performance using metrics such as accuracy, precision, recall, and F1 score.
- Real-World Applications: Â
- Healthcare: Classifying patients based on disease risk factors.
- Finance: Detecting fraudulent transactions.
- Marketing: Segmenting customers for targeted advertising.
Rapid Innovation employs machine learning classification models to help clients streamline operations and improve decision-making processes. By accurately categorizing data, organizations can target their efforts more effectively, leading to increased efficiency and higher returns on investment.
5.6. Probabilistic Outcome Prediction
Probabilistic outcome prediction involves estimating the likelihood of various outcomes based on available data. This approach is particularly useful in fields such as finance, healthcare, and sports analytics, where uncertainty is inherent.
- Understanding Probabilities: Â
- Use statistical models to calculate the probability of different outcomes occurring.
- Incorporate historical data and trends to inform predictions.
- Bayesian Methods: Â
- Employ Bayesian inference to update predictions as new data becomes available.
- This approach allows for a more dynamic understanding of probabilities over time.
- Risk Assessment: Â
- Identify potential risks associated with different outcomes.
- Use probabilistic models to quantify risks and inform decision-making.
- Decision Support Systems: Â
- Integrate probabilistic predictions into decision support systems to aid in strategic planning.
- Provide stakeholders with actionable insights based on predicted probabilities.
- Applications in Various Fields: Â
- Healthcare: Predicting patient outcomes based on treatment plans.
- Sports: Estimating the likelihood of winning based on team performance metrics.
- Finance: Forecasting stock prices and market trends.
By leveraging personalized intervention strategies, machine learning classification models, and probabilistic outcome prediction, organizations can enhance their decision-making processes and improve outcomes across various domains. At Rapid Innovation, we are committed to helping our clients achieve their business goals efficiently and effectively, ultimately leading to greater ROI.
6. Implementation Strategies
Implementation strategies are crucial for the successful deployment of any machine learning or data-driven project. They encompass various stages, including data preparation and model training, and evaluation. This section will delve into two key components: data preparation and preprocessing, and model training methodologies.
6.1 Data Preparation and Preprocessing

‍
Data preparation and preprocessing are foundational steps in any data science project. They ensure that the data is clean, relevant, and structured appropriately for analysis.
- Data Collection: Gather data from various sources, such as databases, APIs, or web scraping. Ensure that the data is relevant to the problem you are trying to solve.
- Data Cleaning: Remove any inconsistencies or errors in the dataset. This includes handling missing values by either imputing them or removing affected records, correcting data types (e.g., converting strings to dates), and eliminating duplicates to ensure data integrity.
- Data Transformation: Transform the data into a suitable format for analysis. This may involve normalization or standardization to bring different scales to a common scale, encoding categorical variables using techniques like one-hot encoding or label encoding, and feature extraction to create new variables that may enhance model performance.
- Data Splitting: Divide the dataset into training, validation, and test sets. This is essential for evaluating the model's performance and preventing overfitting. Common splits include 70% training, 15% validation, and 15% test, or 80% training and 20% test for simpler models.
- Exploratory Data Analysis (EDA): Conduct EDA to understand the data better. This includes visualizing distributions and relationships using graphs and charts, and identifying trends, patterns, and outliers that may influence model performance.
Effective data preparation and preprocessing can significantly impact the accuracy and reliability of the model. According to a study, up to 80% of a data scientist's time is spent on data preparation tasks.
6.2 Model Training Methodologies
Model training methodologies refer to the techniques and processes used to train machine learning models. The choice of methodology can greatly influence the model's performance and its ability to generalize to new data.
- Supervised Learning: Involves training a model on labeled data, where the input features are paired with the correct output. Common algorithms include linear regression for continuous outcomes, decision trees and random forests for classification tasks, and support vector machines (SVM) for complex decision boundaries.
- Unsupervised Learning: Used when the data is unlabeled. The model tries to identify patterns or groupings within the data. Techniques include clustering algorithms like K-means and hierarchical clustering, and dimensionality reduction methods such as Principal Component Analysis (PCA).
- Semi-Supervised Learning: Combines both labeled and unlabeled data for training. This approach is beneficial when acquiring labeled data is expensive or time-consuming, leveraging the strengths of both supervised and unsupervised learning.
- Reinforcement Learning: A type of machine learning where an agent learns to make decisions by taking actions in an environment to maximize cumulative reward. This methodology is widely used in robotics and game playing.
- Cross-Validation: A technique used to assess how the results of a statistical analysis will generalize to an independent dataset. Common methods include K-fold cross-validation, where the dataset is divided into K subsets, and the model is trained K times, each time using a different subset as the test set, and stratified K-fold, which ensures that each fold has the same proportion of classes as the entire dataset.
- Hyperparameter Tuning: Involves optimizing the parameters that govern the training process. Techniques include grid search, which exhaustively searches through a specified subset of hyperparameters, and random search, which samples a wide range of hyperparameters randomly.
- Model Evaluation: After training, it is essential to evaluate the model's performance using metrics such as accuracy, precision, recall, and F1 score for classification tasks, and mean squared error (MSE) and R-squared for regression tasks.
Choosing the right model training methodology is critical for achieving optimal results. Research indicates that the choice of algorithm can affect model performance by as much as 20%.
In conclusion, effective implementation strategies that encompass thorough data preparation and model training are essential for the success of any data-driven project. At Rapid Innovation, we leverage our expertise in AI and Blockchain to ensure that our clients not only achieve their business goals but also maximize their return on investment through efficient and effective project execution. For more detailed guidance, you can refer to this step-by-step tech guide on building your own GPT model.
6.3. Validation and Verification Processes
Validation and verification processes are critical components in ensuring the quality and reliability of systems, products, or services. These processes help organizations confirm that their outputs meet specified requirements and standards.
- Definition: Â
- Validation refers to the process of evaluating whether a product, service, or system meets the needs of the user and fulfills its intended purpose.
- Verification, on the other hand, involves checking whether the product or service complies with regulations, standards, or specifications.
- Importance: Â
- Ensures compliance with industry standards and regulations.
- Reduces the risk of errors and defects in products or services.
- Enhances customer satisfaction by delivering reliable and high-quality outputs.
- Methods: Â
- Testing: Conducting various tests to assess functionality and performance, including validation and verification in software testing.
- Inspections: Reviewing processes and outputs to ensure they meet quality standards.
- Audits: Systematic evaluations of processes and systems to ensure compliance.
- Challenges: Â
- Resource allocation for thorough validation and verification can be demanding.
- Keeping up with evolving standards and regulations requires continuous updates to processes.
- Balancing speed and quality can lead to conflicts in project timelines.
At Rapid Innovation, we leverage advanced AI algorithms to automate and enhance validation and verification processes, ensuring that our clients achieve higher accuracy and efficiency. For instance, our AI-driven testing frameworks can simulate user interactions, allowing for comprehensive testing without the extensive resource allocation typically required. We also address the diff between verification and validation to clarify their distinct roles in the process. For more insights on AI development, check out our AI Development Guide for Businesses.
6.4. Continuous Learning Frameworks
Continuous learning frameworks are essential for organizations aiming to foster a culture of ongoing development and improvement. These frameworks facilitate the acquisition of new skills and knowledge, ensuring that employees remain competitive and adaptable.
- Definition: Â
- A continuous learning framework is a structured approach that encourages individuals and organizations to engage in lifelong learning and skill enhancement.
- Key Components: Â
- Learning Opportunities: Providing access to training programs, workshops, and online courses.
- Feedback Mechanisms: Implementing systems for regular feedback to identify areas for improvement.
- Knowledge Sharing: Encouraging collaboration and sharing of insights among team members.
- Benefits: Â
- Increases employee engagement and job satisfaction.
- Enhances organizational agility by equipping employees with relevant skills.
- Promotes innovation by fostering a culture of experimentation and learning.
- Implementation Strategies: Â
- Establish clear learning objectives aligned with organizational goals.
- Utilize technology to facilitate access to learning resources.
- Encourage a growth mindset among employees to embrace challenges and learn from failures.
Rapid Innovation supports organizations in developing continuous learning frameworks by integrating AI-driven learning management systems that personalize training experiences based on individual employee needs, ultimately leading to improved performance and innovation.
6.5. Institutional Integration Challenges
Institutional integration challenges refer to the difficulties organizations face when attempting to unify various systems, processes, or departments. These challenges can hinder collaboration and efficiency within an organization.
- Definition: Â
- Institutional integration involves aligning different organizational components to work cohesively towards common goals.
- Common Challenges: Â
- Resistance to Change: Employees may be hesitant to adopt new processes or technologies.
- Siloed Departments: Lack of communication and collaboration between departments can lead to inefficiencies.
- Incompatible Systems: Different technologies or processes may not easily integrate, causing disruptions.
- Impact on Organizations: Â
- Reduced efficiency and productivity due to fragmented processes.
- Increased operational costs stemming from duplicated efforts.
- Lower employee morale as a result of unclear roles and responsibilities.
- Strategies for Overcoming Challenges: Â
- Foster a culture of collaboration by encouraging cross-departmental projects.
- Invest in training and change management to ease transitions.
- Utilize integration tools and technologies to streamline processes and improve communication.
At Rapid Innovation, we specialize in blockchain solutions that facilitate seamless institutional integration. By implementing decentralized systems, we help organizations break down silos and enhance collaboration, ultimately driving efficiency and reducing operational costs. Our expertise in both AI and blockchain positions us uniquely to address these integration challenges effectively, including the complexities of design verification and validation.
6.6. Performance Metrics and Evaluation
Performance metrics and evaluation are crucial in assessing the effectiveness of any system, especially in educational settings. These metrics help in understanding how well a model or program is performing and where improvements can be made, particularly in predicting student academic performance.
- Types of Performance Metrics: Â
- Accuracy: Measures the proportion of true results among the total number of cases examined.
- Precision: Indicates the number of true positive results divided by the number of all positive results predicted by the model.
- Recall: Reflects the ability of a model to find all relevant cases (true positives) in a dataset.
- F1 Score: The harmonic mean of precision and recall, providing a balance between the two.
- AUC-ROC: Area Under the Curve - Receiver Operating Characteristics, which evaluates the trade-off between true positive rates and false positive rates.
- Evaluation Techniques: Â
- Cross-Validation: A technique to assess how the results of a statistical analysis will generalize to an independent dataset.
- Confusion Matrix: A table used to describe the performance of a classification model, showing true positives, false positives, true negatives, and false negatives.
- Benchmarking: Comparing the performance of a model against a standard or baseline to gauge its effectiveness.
- Importance of Evaluation: Â
- Identifies strengths and weaknesses in the model.
- Guides decision-making for future improvements.
- Ensures accountability and transparency in educational assessments.
- Real-World Applications: Â
- Schools and universities use performance metrics to evaluate student progress and program effectiveness, especially in predicting students' academic performance using artificial neural networks.
- Educational technology companies rely on these metrics to refine their algorithms and improve user experience.
7. Key Prediction Dimensions
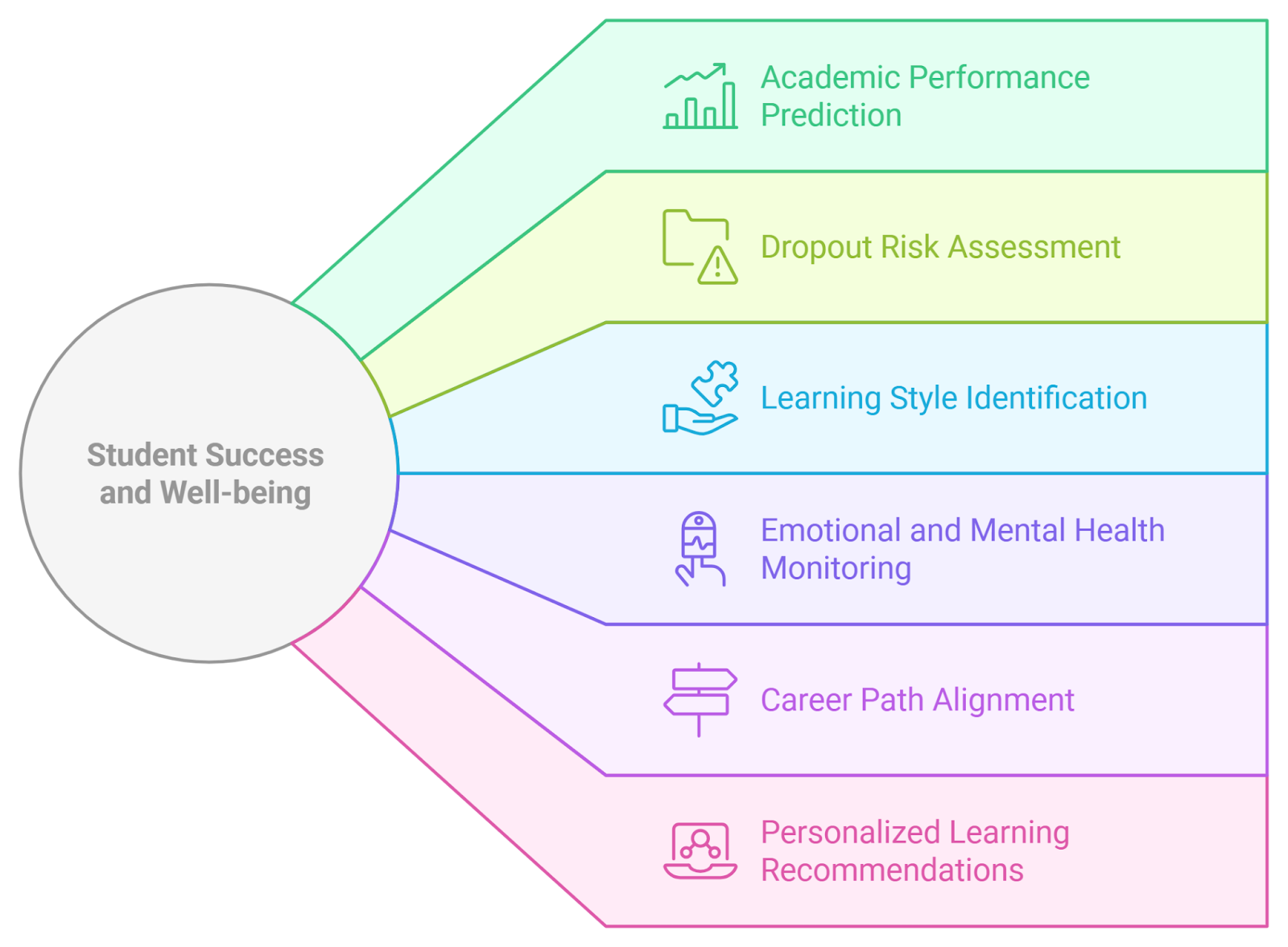
‍
Key prediction dimensions refer to the various factors and variables that influence the outcomes of predictive models, particularly in educational contexts. Understanding these dimensions is essential for developing accurate and reliable predictions.
- Factors Influencing Predictions: Â
- Demographic Information: Age, gender, socioeconomic status, and ethnicity can significantly impact academic performance.
- Behavioral Data: Attendance records, participation in class, and engagement in extracurricular activities are critical indicators of student success.
- Academic History: Previous grades, standardized test scores, and course selections provide insights into future performance.
- Data Sources: Â
- Institutional databases: Schools and universities often maintain extensive records that can be analyzed for predictive modeling.
- Surveys and assessments: Collecting data directly from students can provide valuable insights into their learning habits and challenges.
- Modeling Techniques: Â
- Machine Learning: Algorithms such as regression analysis, decision trees, and neural networks can be employed to analyze the key dimensions and predict outcomes, including academic performance prediction.
- Statistical Analysis: Traditional statistical methods can also be used to identify trends and correlations among various factors.
7.1. Academic Performance Prediction
Academic performance prediction involves forecasting students' future academic outcomes based on various data points and metrics. This process is essential for educators and institutions aiming to enhance student success and tailor interventions, particularly in predicting student performance by using data mining methods for classification.
- Importance of Academic Performance Prediction: Â
- Early Intervention: Identifying at-risk students allows for timely support and resources to improve their chances of success.
- Resource Allocation: Helps schools allocate resources effectively, ensuring that students who need the most help receive it.
- Personalized Learning: Enables the development of customized learning plans that cater to individual student needs.
- Key Predictive Factors: Â
- Attendance: Regular attendance is often correlated with better academic performance.
- Engagement: Active participation in class and extracurricular activities can enhance learning outcomes.
- Socioeconomic Status: Students from lower socioeconomic backgrounds may face additional challenges that impact their academic performance.
- Technological Tools: Â
- Learning Management Systems (LMS): These platforms can track student progress and engagement, providing valuable data for predictions.
- Predictive Analytics Software: Tools that utilize algorithms to analyze data and forecast academic outcomes.
- Challenges in Prediction: Â
- Data Quality: Inaccurate or incomplete data can lead to misleading predictions.
- Ethical Considerations: Ensuring that predictive models do not reinforce biases or discrimination is crucial.
- Dynamic Nature of Education: Changes in curriculum, teaching methods, and student demographics can affect the accuracy of predictions.
- Future Trends: Â
- Increased Use of AI: Artificial intelligence is expected to play a significant role in refining prediction models and enhancing their accuracy.
- Integration of Big Data: Leveraging large datasets from various sources can provide deeper insights into factors affecting academic performance.
- Focus on Holistic Approaches: Moving beyond traditional metrics to include emotional and social factors in academic performance predictions.
At Rapid Innovation, we leverage our expertise in AI and Blockchain to enhance the effectiveness of educational systems. By implementing advanced predictive analytics and performance evaluation tools, we help educational institutions achieve greater ROI through improved student outcomes and resource optimization. Our solutions not only streamline data analysis but also ensure that interventions are timely and tailored to individual needs, ultimately driving success in educational environments.
7.2. Dropout Risk Assessment
Dropout risk assessment is a critical process in educational settings aimed at identifying students who may be at risk of leaving school before completing their education. This assessment helps educators and administrators implement timely interventions to support at-risk students.
- Identifying risk factors: Â
- Academic performance: Poor grades or low attendance can indicate a higher risk of dropout.
- Socioeconomic status: Students from low-income families may face additional challenges that affect their education.
- Behavioral issues: Disciplinary problems or lack of engagement in school activities can signal potential dropout risks.
- Data collection methods: Â
- Surveys and questionnaires: Gathering information from students about their experiences and challenges can provide insights into their likelihood of dropping out.
- Academic records: Analyzing grades, attendance, and participation can help identify students who may need additional support.
- Intervention strategies: Â
- Personalized support: Tailoring academic and emotional support to meet individual student needs can help keep them engaged.
- Mentorship programs: Pairing at-risk students with mentors can provide guidance and encouragement.
- Family involvement: Engaging families in the educational process can create a supportive environment for students.
7.3. Learning Style Identification
Understanding a student's learning style is essential for effective teaching and learning. Learning style identification helps educators tailor their instructional methods to meet the diverse needs of students.
- Types of learning styles: Â
- Visual learners: Prefer to see information presented in charts, graphs, and images.
- Auditory learners: Benefit from listening to lectures, discussions, and audio materials.
- Kinesthetic learners: Learn best through hands-on activities and movement.
- Assessment tools: Â
- Learning style inventories: Various questionnaires can help identify a student's preferred learning style.
- Observational assessments: Teachers can observe students during different activities to determine which methods resonate most with them.
- Benefits of identifying learning styles: Â
- Enhanced engagement: Tailoring instruction to match learning styles can increase student interest and participation.
- Improved retention: Students are more likely to remember information presented in their preferred style.
- Personalized learning experiences: Understanding learning styles allows educators to create more effective and individualized lesson plans.
7.4. Emotional and Mental Health Monitoring
Emotional and mental health monitoring is vital in educational settings to ensure students' overall well-being. This monitoring helps identify students who may be struggling with mental health issues, allowing for timely intervention and support.
- Importance of mental health in education: Â
- Academic performance: Mental health issues can negatively impact a student's ability to focus, learn, and perform academically.
- Social interactions: Students with mental health challenges may struggle with relationships, leading to isolation and further issues.
- Monitoring methods: Â
- Regular check-ins: Teachers and counselors can conduct periodic assessments to gauge students' emotional well-being.
- Surveys and screenings: Anonymous surveys can help identify students who may be experiencing mental health challenges.
- Support strategies: Â
- Counseling services: Providing access to mental health professionals can help students cope with their challenges.
- Peer support programs: Encouraging students to support one another can foster a sense of community and belonging.
- Mental health education: Teaching students about mental health can reduce stigma and promote open discussions about emotional well-being.
At Rapid Innovation, we leverage advanced AI and Blockchain technologies to enhance student dropout risk assessment, learning style identification, and emotional health monitoring in educational institutions. By utilizing AI algorithms, we can analyze vast amounts of data to identify at-risk students more accurately and implement targeted interventions. Our Blockchain solutions ensure secure and transparent data management, fostering trust among stakeholders while maintaining student privacy. Through our innovative approaches, we help educational institutions achieve greater ROI by improving student retention and overall academic performance.
7.5. Career Path Alignment
Career path alignment is crucial for individuals seeking to navigate their professional journeys effectively. It involves matching personal skills, interests, and values with potential career opportunities. This alignment can lead to increased job satisfaction and better performance in the workplace.
- Understanding personal strengths and weaknesses is essential.
- Researching various career options helps in making informed decisions.
- Networking with professionals in desired fields can provide insights and guidance.
- Utilizing career assessment tools can clarify suitable paths.
- Setting short-term and long-term career goals can keep individuals focused.
Organizations can also play a role in career path alignment by offering resources such as mentorship programs, career counseling, and workshops. These initiatives can help employees identify their career aspirations and align them with the company’s objectives, fostering a culture of growth and development. At Rapid Innovation, we leverage AI-driven analytics to provide personalized career insights, helping individuals align their skills with market demands, ultimately enhancing their career trajectories and contributing to greater organizational success. Additionally, the rise of prompt engineers and AI managers in 2024 highlights emerging career paths that individuals may consider in their alignment efforts.
7.6. Personalized Learning Recommendations
Personalized learning recommendations are tailored educational strategies designed to meet the unique needs of each learner. This approach recognizes that individuals have different learning styles, paces, and preferences, making it essential to customize learning experiences.
- Adaptive learning technologies can provide personalized content based on performance.
- Regular assessments can help identify areas where learners need additional support.
- Incorporating various learning modalities (visual, auditory, kinesthetic) can enhance engagement.
- Feedback mechanisms allow learners to understand their progress and adjust their learning paths.
- Collaboration with educators can ensure that learning recommendations align with curriculum goals.
By implementing personalized learning recommendations, educational institutions and organizations can improve learner outcomes, increase retention rates, and foster a more inclusive learning environment. Rapid Innovation utilizes AI algorithms to analyze learner data, enabling the creation of customized learning paths that enhance engagement and effectiveness.
8. Intervention and Support Mechanisms
Intervention and support mechanisms are essential components in addressing challenges faced by individuals in educational and professional settings. These mechanisms aim to provide timely assistance and resources to help individuals overcome obstacles and achieve their goals.
- Early identification of at-risk individuals can lead to timely interventions.
- Support services such as tutoring, counseling, and mentoring can provide necessary guidance.
- Creating a supportive community fosters collaboration and shared learning experiences.
- Regular check-ins and progress monitoring can help track improvements and adjust strategies.
- Training staff to recognize signs of struggle can enhance the effectiveness of support systems.
Implementing robust intervention and support mechanisms can lead to improved outcomes for individuals, ensuring they have the resources and guidance needed to succeed in their educational and career pursuits. Rapid Innovation's blockchain solutions can enhance the transparency and security of these support systems, ensuring that individuals receive the assistance they need in a timely and efficient manner.
8.1. Personalized Learning Pathways
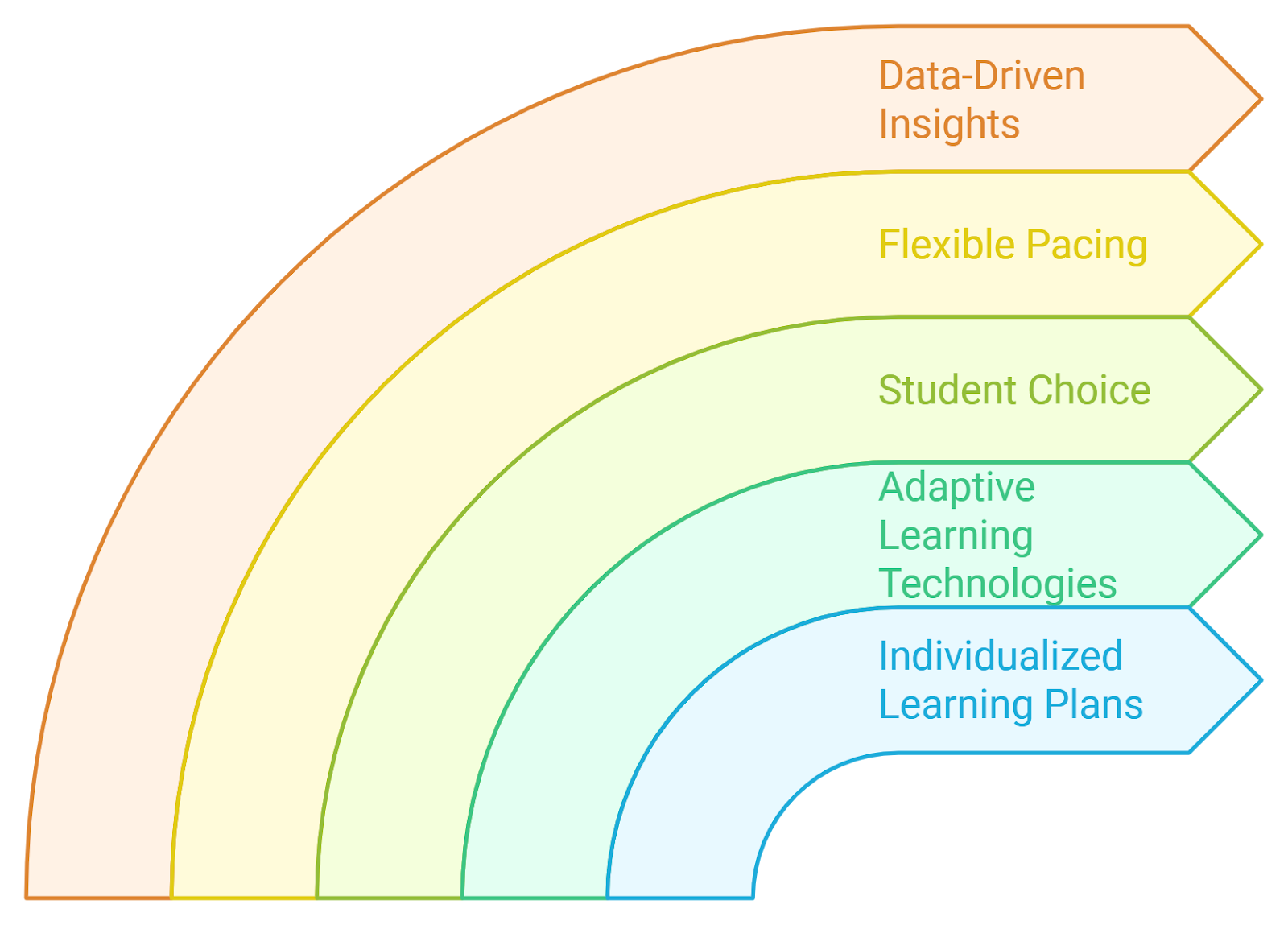
‍
Personalized learning pathways are tailored educational experiences designed to meet the unique needs, interests, and abilities of each student. This approach recognizes that every learner is different and requires a customized strategy to achieve their full potential.
- Individualized Learning Plans: These plans outline specific goals and strategies for each student, taking into account their strengths and weaknesses.
- Adaptive Learning Technologies: Tools like AI-driven platforms can adjust the difficulty of tasks based on real-time performance, ensuring that students are always challenged but not overwhelmed. Rapid Innovation leverages AI algorithms to create dynamic learning environments that adapt to individual student needs, enhancing engagement and retention.
- Student Choice: Allowing students to select topics or projects that interest them can increase engagement and motivation.
- Flexible Pacing: Students can progress through material at their own speed, which is particularly beneficial for those who may need more time to grasp certain concepts.
- Data-Driven Insights: Educators can use analytics to track student progress and adjust personal learning pathways accordingly, ensuring that interventions are timely and effective. Rapid Innovation's data analytics solutions empower educators with actionable insights, leading to improved educational outcomes and greater ROI.
8.2. Early Intervention Strategies
Early intervention strategies are proactive measures taken to identify and support students who may be at risk of falling behind academically or socially. These strategies are crucial for fostering a supportive learning environment and ensuring that all students have the opportunity to succeed.
- Screening and Assessment: Regular assessments can help identify students who may need additional support early on, allowing for timely interventions.
- Targeted Support Programs: Implementing programs that focus on specific skills, such as reading or math, can help struggling students catch up with their peers.
- Family Engagement: Involving families in the educational process can provide additional support for students, reinforcing learning at home.
- Professional Development for Educators: Training teachers to recognize early signs of struggle and equipping them with effective intervention strategies can enhance the overall support system.
- Multi-Tiered Systems of Support (MTSS): This framework provides varying levels of support based on student needs, ensuring that interventions are appropriate and effective. Rapid Innovation can assist educational institutions in implementing MTSS through tailored software solutions that streamline support processes.
8.3. Mentorship and Guidance Systems
Mentorship and guidance systems play a vital role in supporting students throughout their educational journey. These systems connect students with mentors who can provide advice, encouragement, and resources to help them navigate academic and personal challenges.
- Peer Mentoring: Pairing students with older peers can foster a sense of community and provide relatable guidance.
- Faculty Mentorship: Establishing relationships between students and faculty can enhance academic support and provide insights into career paths.
- Career Counseling: Offering guidance on career options and personal learning pathways can help students make informed decisions about their futures.
- Networking Opportunities: Creating events where students can meet professionals in their fields of interest can inspire and motivate them.
- Continuous Support: Mentorship should not be a one-time event; ongoing relationships can provide sustained encouragement and accountability for students. Rapid Innovation can facilitate mentorship programs through AI-driven platforms that match students with suitable mentors based on their interests and career aspirations, ultimately driving better educational outcomes and ROI.
Additionally, personalized learning pathways for aboriginal students can be developed to ensure that their unique cultural and educational needs are met effectively.
8.4. Resource Allocation Optimization
Resource allocation optimization is crucial for maximizing the efficiency and effectiveness of educational institutions. It involves strategically distributing resources—such as time, personnel, and materials—to achieve the best possible outcomes for students and educators.
- Efficient use of financial resources can lead to improved educational programs and facilities. By leveraging AI-driven analytics, institutions can forecast budgetary needs and allocate funds more effectively, ensuring that investments yield higher returns.
- Human resources should be allocated based on student needs, ensuring that teachers and support staff are effectively utilized. Blockchain technology can facilitate transparent tracking of staff performance and student outcomes, enabling data-informed staffing decisions.
- Technology resources, including software and hardware, should be integrated into the curriculum to enhance learning experiences. Rapid Innovation can assist in implementing AI tools that personalize learning, thereby optimizing resource use.
- Data-driven decision-making can help identify areas where resources are underutilized or overextended. Our AI solutions can analyze vast datasets to provide actionable insights, allowing institutions to reallocate resources dynamically.
- Regular assessments of resource allocation can lead to continuous improvement and adaptation to changing educational demands. By employing predictive analytics, schools can anticipate future resource needs and adjust accordingly.
By focusing on resource allocation optimization, including resource allocation optimization problem and resource allocation optimization python, schools can create a more sustainable and effective learning environment. The allocation optimization problem is a key aspect of this process, as it helps institutions identify the best strategies for distributing their resources.
8.5. Psychological Support Frameworks
Psychological support frameworks are essential for fostering a healthy learning environment. These frameworks provide students with the emotional and mental support they need to thrive academically and socially.
- Implementing counseling services can help students cope with stress, anxiety, and other mental health issues. AI-driven chatbots can provide immediate support and resources, ensuring students have access to help when they need it.
- Peer support programs encourage students to connect with one another, fostering a sense of community and belonging. Blockchain can be utilized to create secure platforms for peer interactions, ensuring privacy and trust.
- Training teachers in mental health awareness equips them to recognize and address students' psychological needs. Our consulting services can provide tailored training programs that incorporate the latest research and best practices.
- Creating a safe and inclusive school culture promotes positive mental health and reduces stigma around seeking help. AI tools can analyze school climate data to identify areas for improvement and measure the effectiveness of initiatives.
- Regular workshops and seminars on mental health can educate students and staff about the importance of psychological well-being. Rapid Innovation can facilitate these programs through interactive platforms that engage participants effectively.
By establishing robust psychological support frameworks, educational institutions can enhance student resilience and overall academic performance.
8.6. Adaptive Curriculum Design
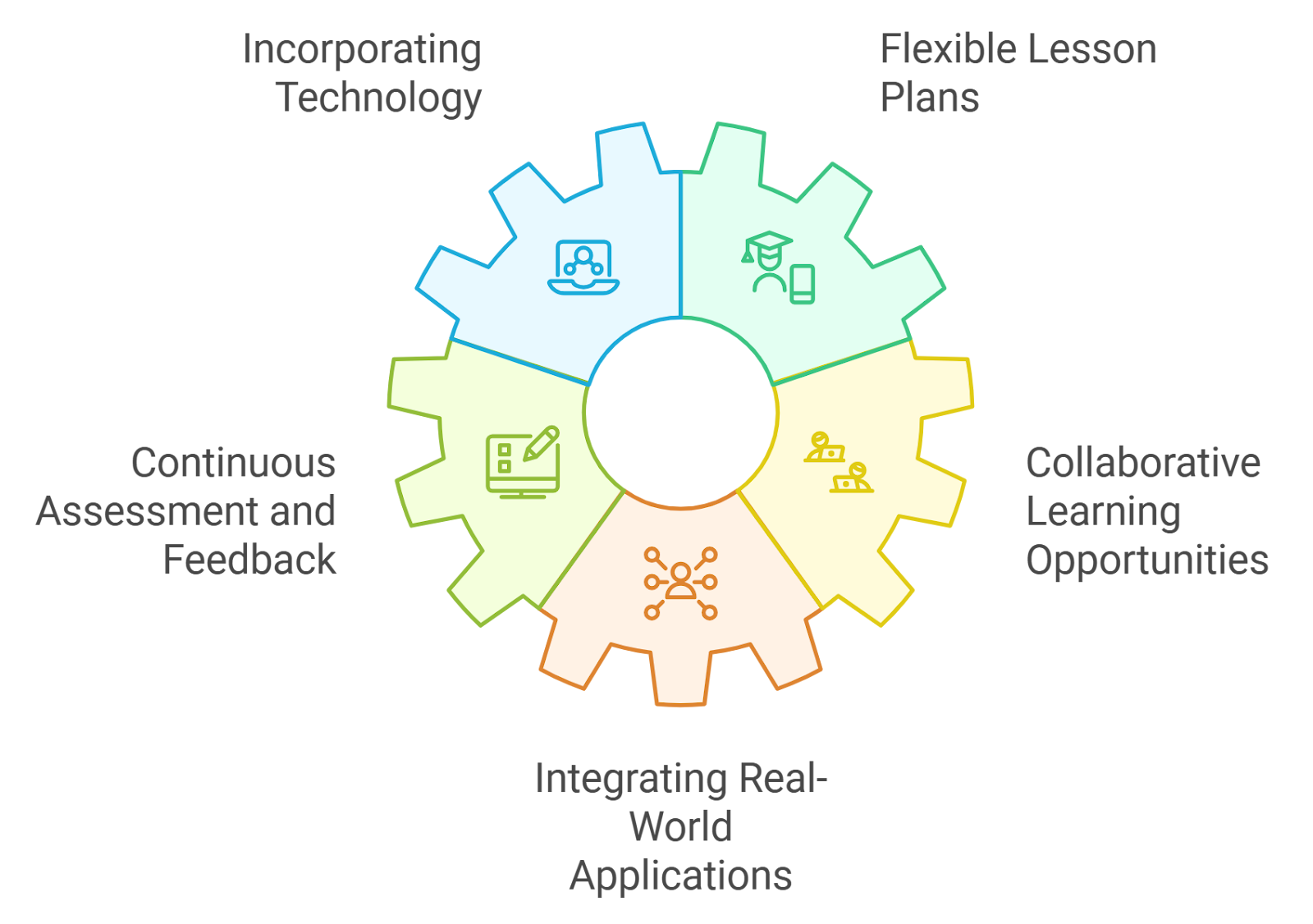
‍
Adaptive curriculum design is an innovative approach that tailors educational content to meet the diverse needs of students. This method recognizes that learners have varying abilities, interests, and learning styles.
- Incorporating technology allows for personalized learning experiences, enabling students to progress at their own pace. Our AI solutions can analyze individual learning patterns and recommend customized learning paths.
- Flexible lesson plans can accommodate different learning modalities, such as visual, auditory, and kinesthetic learning. Blockchain can ensure that educational content is securely shared and updated, providing educators with the latest resources.
- Continuous assessment and feedback help educators adjust the curriculum based on student performance and engagement. AI-driven assessment tools can provide real-time feedback, allowing for timely adjustments to teaching strategies.
- Collaborative learning opportunities encourage peer interaction and support, enhancing the learning experience. Our platforms can facilitate collaborative projects, leveraging technology to connect students across different locations.
- Integrating real-world applications into the curriculum makes learning more relevant and engaging for students. Rapid Innovation can assist in developing partnerships with industry leaders to provide students with practical experiences.
By embracing adaptive curriculum design, schools can create a more inclusive and effective educational experience that caters to the unique needs of every student, including addressing the resource allocation problem in project management and providing examples of resource allocation problems.
9. Technical Challenges and Limitations
In the rapidly evolving landscape of technology, particularly in fields like artificial intelligence (AI) and machine learning (ML), several technical challenges and limitations persist. These challenges can significantly impact the effectiveness and reliability of technological solutions.
9.1 Data Quality and Reliability
Data quality and reliability are critical components in the success of any data-driven project. Poor data quality can lead to inaccurate results, which can have far-reaching consequences. The trustworthiness of data and data quality refers to the degree to which data is accurate and reliable.
Ensuring high data quality involves implementing robust data governance frameworks and utilizing advanced data management technologies. Organizations must prioritize data quality to enhance the reliability of their insights and decisions. Key issues include:
- Inaccurate Data: Data may be outdated, incomplete, or incorrect, leading to flawed analyses and decisions.
- Data Sources: The reliability of data sources is paramount. Data collected from unverified or biased sources can skew results.
- Data Consistency: Inconsistent data formats and structures can complicate data integration and analysis, making it difficult to derive meaningful insights.
- Data Volume: The sheer volume of data can overwhelm systems, leading to performance issues and potential data loss.
- Data Management: Effective data management practices are essential to ensure data quality. This includes regular audits, validation processes, and the use of data cleaning tools.
At Rapid Innovation, we assist clients in overcoming these challenges by implementing tailored data governance strategies and advanced data management solutions. Our expertise ensures that your data is not only reliable but also actionable, leading to improved decision-making and greater ROI. The focus on data accuracy and reliability is crucial for achieving successful outcomes.
9.2 Bias and Fairness Considerations
Bias in AI and ML systems is a significant concern that can lead to unfair outcomes and perpetuate existing inequalities. Addressing bias and ensuring fairness is essential for building trust in technology.
To combat bias and promote fairness, organizations should adopt inclusive practices in data collection, involve diverse teams in the development process, and continuously monitor and evaluate their systems for bias. Key considerations include:
- Algorithmic Bias: Algorithms can inadvertently learn biases present in training data, leading to discriminatory outcomes. For example, facial recognition systems have been shown to have higher error rates for individuals with darker skin tones.
- Data Representation: If the training data does not adequately represent diverse populations, the resulting models may not perform well across different demographic groups.
- Human Bias: Human biases can be introduced during the data collection process, model development, and evaluation stages. This can result in systems that reflect societal prejudices.
- Fairness Metrics: Establishing fairness metrics is crucial for evaluating the performance of AI systems. These metrics help identify and mitigate bias, ensuring that technology serves all users equitably.
- Regulatory Compliance: Organizations must be aware of and comply with regulations regarding fairness and bias in AI. This includes adhering to guidelines set forth by governing bodies and industry standards.
At Rapid Innovation, we prioritize fairness and transparency in AI development. Our team works closely with clients to implement best practices that mitigate bias, ensuring that your AI solutions are equitable and compliant with industry standards. This commitment not only enhances trust but also drives better business outcomes, ultimately leading to a higher return on investment. The emphasis on reliability in data quality is essential for fostering trust in AI systems.
9.3. Interpretability of Predictions
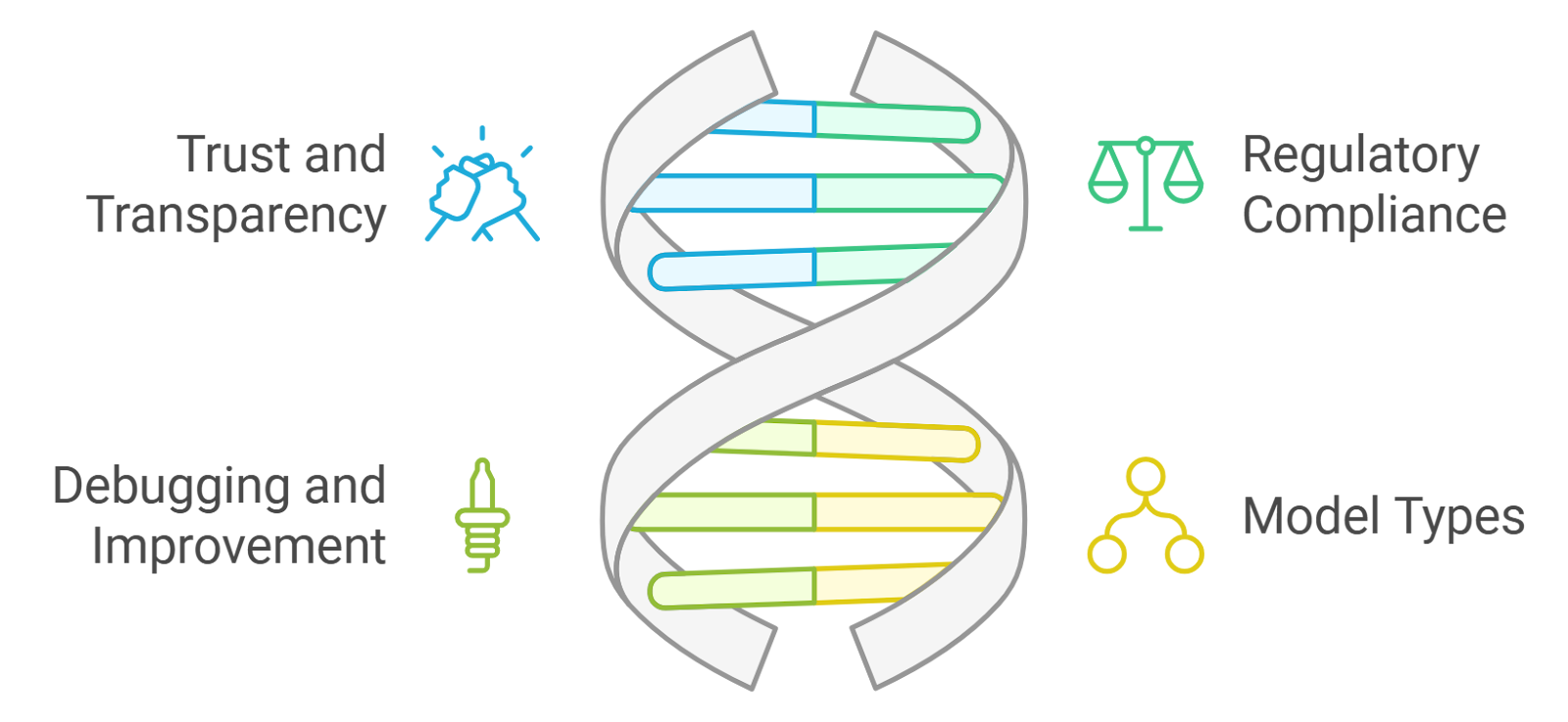
‍
Interpretability of predictions is a crucial aspect of machine learning and artificial intelligence. It refers to the degree to which a human can understand the cause of a decision made by a model. As AI systems become more complex, ensuring that their predictions are interpretable is essential for several reasons:
- Trust and Transparency: Users are more likely to trust AI systems when they can understand how decisions are made. This is particularly important in high-stakes fields like healthcare, finance, and criminal justice, where Rapid Innovation can assist clients in developing interpretable models that foster user confidence. The concept of interpretable AI plays a significant role in building this trust.
- Regulatory Compliance: Many industries are subject to regulations that require transparency in decision-making processes. For instance, the General Data Protection Regulation (GDPR) in Europe emphasizes the right to explanation for automated decisions. Rapid Innovation helps clients navigate these regulations by implementing AI solutions that prioritize interpretability and model explainability and interpretability.
- Debugging and Improvement: Understanding how a model makes predictions allows developers to identify biases or errors in the model. This understanding can lead to improvements in the model's performance and fairness. Rapid Innovation's expertise in AI enables clients to refine their models effectively, enhancing overall business outcomes. Techniques like model agnostic explainable AI are utilized to ensure that models are both effective and interpretable.
- Model Types: Some models, like linear regression and decision trees, are inherently more interpretable than others, such as deep neural networks. Researchers are actively working on methods to enhance the interpretability of complex models, including techniques like LIME (Local Interpretable Model-agnostic Explanations) and SHAP (SHapley Additive exPlanations). Rapid Innovation leverages these techniques to ensure that clients' AI systems are both powerful and understandable, aligning with the principles of AI interpretability and explainability.
9.4. Privacy and Ethical Concerns
Privacy and ethical concerns are paramount in the development and deployment of AI systems. As these technologies process vast amounts of personal data, the potential for misuse or unintended consequences increases. Key issues include:
- Data Privacy: AI systems often require large datasets, which may contain sensitive personal information. Ensuring that this data is collected, stored, and processed in compliance with privacy laws is critical. Techniques like differential privacy can help protect individual data points while still allowing for meaningful analysis. Rapid Innovation emphasizes data privacy in its AI solutions, ensuring compliance and safeguarding client interests.
- Bias and Fairness: AI models can inadvertently perpetuate or amplify biases present in the training data. This can lead to unfair treatment of certain groups, particularly in areas like hiring, lending, and law enforcement. Addressing bias requires careful data curation and ongoing monitoring of model outputs. Rapid Innovation assists clients in implementing strategies to mitigate bias, promoting fairness in AI applications, which is a key aspect of reliable and interpretable artificial intelligence.
- Accountability: When AI systems make decisions that impact individuals' lives, determining accountability can be challenging. Questions arise about who is responsible for errors or harmful outcomes—developers, organizations, or the AI itself. Rapid Innovation helps clients establish clear accountability frameworks, ensuring responsible AI deployment.
- Informed Consent: Users should be informed about how their data is being used and have the option to consent to its use. This is especially important in applications like facial recognition and surveillance, where individuals may not be aware that their data is being collected. Rapid Innovation advocates for transparency and informed consent in all AI projects, aligning with ethical standards.
9.5. Computational Complexity
Computational complexity refers to the resources required to run algorithms, including time and memory. In the context of machine learning, it is a significant consideration that can impact the feasibility and efficiency of deploying models. Key aspects include:
- Algorithm Efficiency: Some algorithms are computationally intensive, requiring significant processing power and time to train and make predictions. For example, deep learning models often require powerful GPUs and extensive training time, which can be a barrier for smaller organizations. Rapid Innovation provides clients with optimized solutions that balance performance and resource requirements.
- Scalability: As datasets grow in size, the computational demands of machine learning models can increase dramatically. Ensuring that models can scale effectively without a proportional increase in resource consumption is essential for practical applications. Rapid Innovation's expertise in scalable AI solutions enables clients to handle growing data needs efficiently.
- Trade-offs: There is often a trade-off between model complexity and interpretability. More complex models may provide better accuracy but at the cost of increased computational requirements and reduced interpretability. Striking the right balance is crucial for effective deployment. Rapid Innovation guides clients in making informed decisions about model selection based on their specific business goals, considering the implications of interpretability and explainability.
- Optimization Techniques: Researchers are developing various optimization techniques to reduce computational complexity, such as pruning, quantization, and knowledge distillation. These methods can help make models more efficient while maintaining performance. Rapid Innovation employs these techniques to enhance the efficiency of clients' AI systems, ensuring that they remain interpretable and explainable.
- Real-time Processing: In applications requiring real-time predictions, such as autonomous vehicles or fraud detection, computational complexity becomes even more critical. Ensuring that models can deliver timely results without excessive resource consumption is a key challenge. Rapid Innovation specializes in developing real-time AI solutions that meet the demands of dynamic environments, helping clients achieve greater ROI while maintaining the principles of explainable and interpretable models in computer vision and machine learning.
9.6. Generalizability of Models
Generalizability refers to the ability of a model to perform well on unseen data, beyond the specific dataset it was trained on. This is a crucial aspect of machine learning and artificial intelligence, as it determines how effectively a model can be applied in real-world scenarios.
- Importance of Generalizability: Â
- Ensures that the model is not just memorizing the training data.
- Enhances the model's applicability across different datasets and environments.
- Reduces the risk of overfitting, where a model performs well on training data but poorly on new data.
- Factors Affecting Generalizability: Â
- Data Quality: High-quality, diverse datasets improve generalizability.
- Model Complexity: Simpler models often generalize better than overly complex ones.
- Training Techniques: Techniques like cross-validation help assess a model's generalizability.
- Methods to Improve Generalizability: Â
- Regularization: Techniques like L1 and L2 regularization can help prevent overfitting.
- Data Augmentation: Increasing the diversity of the training data can enhance generalizability.
- Transfer Learning: Utilizing pre-trained models on similar tasks can improve performance on new datasets.
- Evaluation Metrics: Â
- Metrics such as accuracy, precision, recall, and F1 score can help assess a model's generalizability.
- Cross-validation techniques provide insights into how well a model will perform on unseen data.
At Rapid Innovation, we leverage our expertise in AI to ensure that the models we develop for our clients are not only accurate but also generalizable. By focusing on high-quality data and employing advanced training techniques, we help businesses achieve greater ROI through effective AI solutions, including our fine-tuning of language models.
10. Advanced AI Capabilities
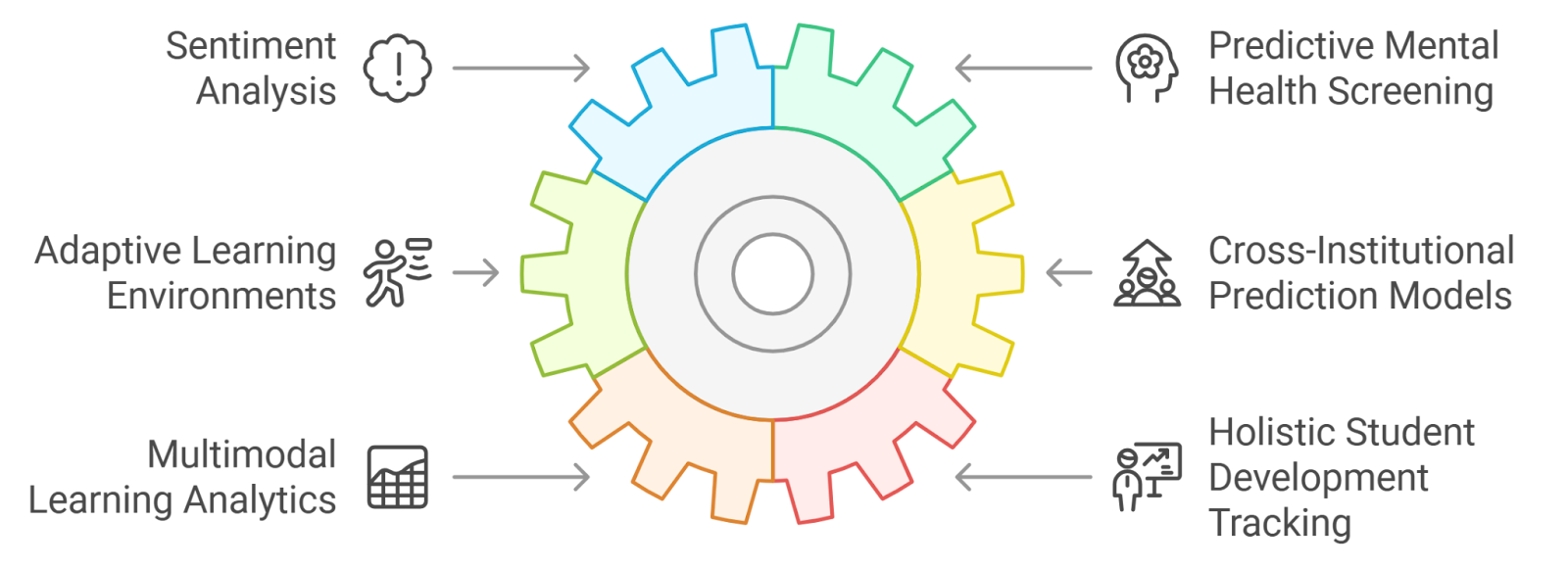
‍
Advanced AI capabilities refer to the sophisticated functions that modern AI systems can perform, often surpassing traditional algorithms. These capabilities enable AI to tackle complex problems and provide insights that were previously unattainable.
- Key Advanced AI Capabilities: Â
- Natural Language Processing (NLP): Understanding and generating human language.
- Computer Vision: Analyzing and interpreting visual data from the world.
- Reinforcement Learning: Learning optimal actions through trial and error in dynamic environments.
- Applications of Advanced AI: Â
- Healthcare: AI can analyze medical images and predict patient outcomes.
- Finance: Algorithms can detect fraudulent transactions and optimize trading strategies.
- Autonomous Vehicles: AI systems enable self-driving cars to navigate and make decisions.
- Challenges in Advanced AI: Â
- Data Privacy: Ensuring user data is protected while training AI models.
- Bias: Addressing biases in training data to prevent skewed outcomes.
- Interpretability: Making AI decisions understandable to users and stakeholders.
At Rapid Innovation, we harness these advanced AI capabilities to deliver tailored solutions that meet the unique needs of our clients, driving efficiency and effectiveness in their operations.
10.1. Sentiment Analysis
Sentiment analysis is a subfield of natural language processing that focuses on determining the emotional tone behind a body of text. It is widely used in various applications, from marketing to social media monitoring.
- Importance of Sentiment Analysis: Â
- Helps businesses understand customer opinions and feedback.
- Enables brands to gauge public sentiment about products or services.
- Assists in crisis management by identifying negative sentiments early.
- Techniques Used in Sentiment Analysis: Â
- Lexicon-Based Approaches: Utilizing predefined lists of words associated with positive or negative sentiments.
- Machine Learning Models: Training algorithms on labeled datasets to classify sentiments.
- Deep Learning: Using neural networks to capture complex patterns in text data.
- Applications of Sentiment Analysis: Â
- Social Media Monitoring: Analyzing tweets or posts to understand public opinion.
- Customer Feedback: Evaluating reviews and ratings to improve products.
- Market Research: Identifying trends and consumer preferences through sentiment trends.
- Challenges in Sentiment Analysis: Â
- Sarcasm and Irony: Difficulty in detecting nuanced expressions of sentiment.
- Contextual Understanding: Words can have different meanings based on context.
- Multilingual Sentiment: Analyzing sentiments across different languages and cultures.
- Future of Sentiment Analysis: Â
- Integration with other AI capabilities for more comprehensive insights.
- Enhanced algorithms for better accuracy and context understanding.
- Increased use in real-time applications for immediate feedback and response.
At Rapid Innovation, we utilize sentiment analysis to help our clients gain valuable insights into customer perceptions, enabling them to make informed decisions that enhance their market strategies and improve overall ROI.
10.2. Predictive Mental Health Screening
Predictive mental health screening utilizes data analytics and machine learning to identify individuals at risk of mental health issues before they escalate. This proactive approach can significantly enhance early intervention strategies.
- Uses historical data to predict future mental health outcomes. Â
- Incorporates various data sources, including surveys, social media activity, and academic performance. Â
- Aims to identify at-risk individuals in educational settings, workplaces, and healthcare systems. Â
- Can lead to timely support and resources, reducing the severity of mental health crises. Â
- Enhances the ability of mental health professionals to allocate resources effectively. Â
At Rapid Innovation, we leverage our expertise in AI to develop predictive models that can improve the accuracy of identifying individuals who may benefit from mental health services. For instance, studies show that predictive analytics can increase the identification rate of at-risk students by up to 30%. By implementing these solutions, organizations can achieve greater ROI through reduced healthcare costs and improved employee well-being. Learn more about our approach to AI for mental health care.
10.3. Adaptive Learning Environments
Adaptive learning environments are educational settings that adjust to the individual needs of students, enhancing their learning experience. These environments leverage technology to provide personalized learning pathways.
- Utilizes algorithms to assess student performance in real-time. Â
- Offers customized resources and activities based on individual learning styles and paces. Â
- Encourages student engagement by providing relevant content tailored to their interests. Â
- Facilitates immediate feedback, allowing students to understand their progress and areas for improvement. Â
- Supports diverse learning needs, including those of students with disabilities. Â
The implementation of adaptive learning technologies has shown promising results. For example, studies have found that adaptive learning can improve student retention rates by as much as 20%. Rapid Innovation can assist educational institutions in integrating these technologies, leading to enhanced learning outcomes and increased student satisfaction.
10.4. Cross-Institutional Prediction Models
Cross-institutional prediction models involve collaboration between multiple educational institutions to share data and insights for better predictive analytics. This approach enhances the understanding of student behaviors and outcomes across different contexts.
- Combines data from various institutions to create a more comprehensive dataset. Â
- Enables the identification of trends and patterns that may not be visible within a single institution. Â
- Facilitates benchmarking against peer institutions, allowing for improved strategies and interventions. Â
- Promotes collaboration among institutions to address common challenges in student retention and success. Â
- Enhances the ability to predict outcomes for diverse student populations. Â
By pooling resources and data, institutions can develop more robust predictive models. Research indicates that cross-institutional collaboration can lead to a 15% increase in the accuracy of predictions related to student success. Rapid Innovation can guide institutions in establishing these collaborative frameworks, ultimately driving better educational outcomes and maximizing institutional effectiveness.
10.5. Multimodal Learning Analytics
Multimodal learning analytics refers to the integration of various data sources and learning modalities to gain insights into student learning behaviors and outcomes. This approach leverages data from multiple channels, such as:
- Online learning platforms
- Classroom interactions
- Social media engagement
- Mobile applications
By analyzing these diverse data streams, educators can obtain a comprehensive view of student performance and engagement. This holistic perspective allows for:
- Enhanced understanding of individual learning styles
- Identification of at-risk students through predictive analytics
- Tailored interventions based on specific learning needs
The use of multimodal learning analytics can lead to improved educational outcomes. For instance, studies have shown that institutions employing these analytics can increase student retention rates by up to 15% (source: Educause). Furthermore, the insights gained can inform curriculum design and instructional strategies, ensuring they are aligned with student needs.
At Rapid Innovation, we harness the power of AI to develop sophisticated multimodal learning analytics solutions that empower educational institutions to make data-driven decisions. By implementing our AI-driven analytics tools, clients can achieve greater ROI through improved student engagement and retention, ultimately leading to enhanced institutional performance. Additionally, our expertise extends to various applications of AI in education, which can be explored further in our article on AI in education: use cases, benefits, solutions, and implementation.
10.6. Holistic Student Development Tracking
Holistic student development tracking encompasses a comprehensive approach to monitoring and supporting students' academic, social, emotional, and physical growth. This method recognizes that education extends beyond academics and includes various aspects of a student's life. Key components include:
- Academic performance metrics
- Social and emotional learning assessments
- Extracurricular involvement tracking
- Health and wellness indicators
By utilizing a holistic tracking system, educators can:
- Foster a supportive learning environment
- Identify strengths and weaknesses in student development
- Implement targeted support programs that address specific needs
Research indicates that students who receive holistic support are more likely to succeed academically and socially (source: ASCD). This approach not only enhances individual student outcomes but also contributes to a positive school culture, promoting collaboration and community engagement.
Rapid Innovation's expertise in blockchain technology can further enhance holistic student development tracking by ensuring secure and transparent data management. Our blockchain solutions can provide educational institutions with a reliable framework for tracking student progress and achievements, thereby increasing trust among stakeholders and improving overall educational outcomes.
11. Economic and Strategic Implications
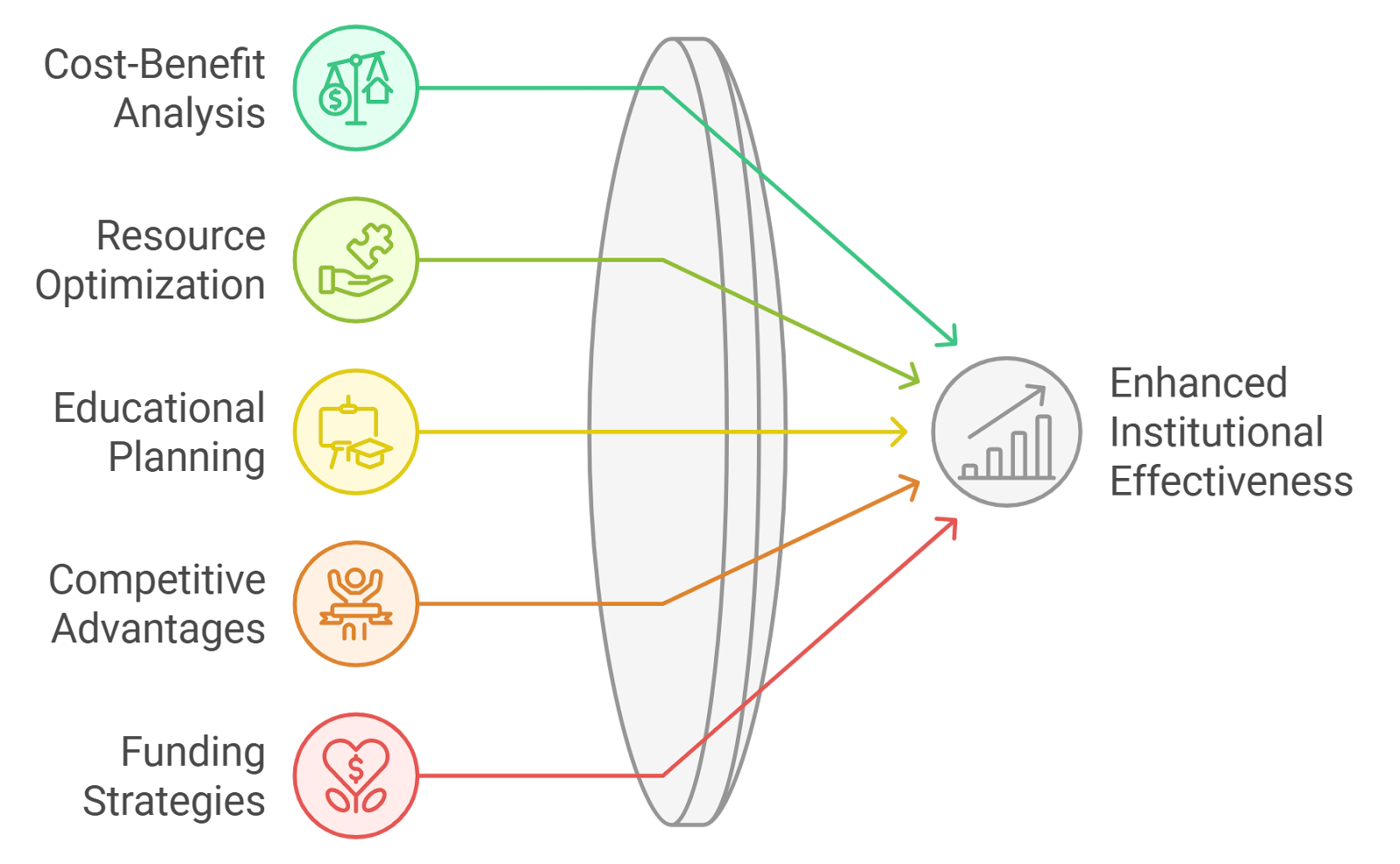
‍
The economic and strategic implications of adopting advanced educational technologies and analytics are significant. Institutions that invest in these areas can expect:
- Increased operational efficiency: By utilizing data analytics, schools can streamline administrative processes, reducing costs and improving resource allocation.
- Enhanced student outcomes: Improved multimodal learning analytics and holistic tracking can lead to higher graduation rates and better job placement statistics, which can attract more students and funding.
- Competitive advantage: Institutions that leverage innovative technologies can differentiate themselves in a crowded educational market, appealing to prospective students and parents.
Moreover, the strategic implications extend to policy-making and funding. As educational institutions demonstrate the effectiveness of these approaches, they may secure additional funding from government and private sources. This can lead to:
- Greater investment in technology and infrastructure
- Development of new programs that cater to emerging job markets
- Strengthened partnerships with industry stakeholders
In conclusion, the integration of multimodal learning analytics and holistic student development tracking not only enhances educational practices but also has profound economic and strategic implications for educational institutions. By embracing these innovations, schools can better prepare students for the future while ensuring their own sustainability and growth. Rapid Innovation stands ready to assist educational institutions in navigating this transformative landscape, leveraging our AI and blockchain expertise to drive meaningful change and achieve greater ROI.
11.1. Cost-Benefit Analysis
Cost-benefit analysis (CBA) is a systematic approach to evaluating the economic pros and cons of different educational programs or initiatives, such as medical student performance evaluation and educational program evaluation. It helps institutions make informed decisions by quantifying the expected benefits and costs associated with a project.
- Identifying Costs: Â
- Direct costs (tuition, materials, facilities)
- Indirect costs (administrative expenses, opportunity costs)
- Estimating Benefits: Â
- Increased student enrollment
- Improved graduation rates
- Enhanced job placement rates
- Quantifying Outcomes: Â
- Use metrics such as return on investment (ROI) to measure financial returns.
- Consider non-monetary benefits like student satisfaction and community impact.
- Decision-Making: Â
- Compare the total costs against the total benefits to determine feasibility.
- Prioritize projects that yield the highest net benefits, including those related to wes course by course evaluation and curriculum evaluator assessments.
CBA is essential for educational institutions to allocate resources effectively and ensure that investments lead to meaningful improvements in educational outcomes. At Rapid Innovation, we leverage advanced AI algorithms to enhance the accuracy of CBA, enabling institutions to make data-driven decisions that maximize ROI.
11.2. Institutional Resource Optimization
Institutional resource optimization involves strategically managing and utilizing resources to enhance educational effectiveness and efficiency. This process ensures that institutions can deliver quality education while minimizing waste, including resources related to cpse evaluations and wes evaluation course by course.
- Resource Assessment: Â
- Conduct audits to evaluate current resource allocation (staff, facilities, technology).
- Identify underutilized resources that can be repurposed or eliminated.
- Strategic Planning: Â
- Develop a resource management plan that aligns with institutional goals.
- Set clear priorities for resource allocation based on data-driven insights.
- Technology Integration: Â
- Leverage technology to streamline operations (e.g., online learning platforms, administrative software).
- Invest in tools that enhance teaching and learning experiences, such as ece course by course evaluation tools.
- Continuous Improvement: Â
- Implement feedback mechanisms to assess the effectiveness of resource allocation.
- Regularly review and adjust strategies based on performance metrics.
Optimizing institutional resources not only improves operational efficiency but also enhances the overall educational experience for students and faculty. Rapid Innovation employs blockchain technology to ensure transparency and accountability in resource allocation, further driving efficiency and trust within educational institutions.
11.3. Long-Term Educational Planning
Long-term educational planning is a strategic approach that focuses on setting goals and developing actionable plans to achieve them over an extended period. This process is crucial for ensuring that educational institutions remain relevant and responsive to changing societal needs, including the need for effective educational evaluation and research.
- Vision and Mission Development: Â
- Establish a clear vision and mission that reflects the institution's values and goals.
- Engage stakeholders (faculty, students, community) in the planning process.
- Goal Setting: Â
- Define specific, measurable, achievable, relevant, and time-bound (SMART) goals.
- Focus on areas such as curriculum development, faculty recruitment, and infrastructure improvements.
- Environmental Scanning: Â
- Analyze external factors (economic trends, technological advancements, demographic shifts) that may impact education.
- Use data to anticipate future challenges and opportunities.
- Implementation and Evaluation: Â
- Create a timeline for achieving goals and assign responsibilities to relevant parties.
- Regularly assess progress and make adjustments as needed to stay on track.
Long-term educational planning ensures that institutions can adapt to changes and continue to provide high-quality education that meets the needs of students and the community. By integrating AI-driven insights and blockchain solutions, Rapid Innovation empowers educational institutions to navigate future challenges effectively and sustainably, including those related to cpse evaluation near me and wes course evaluation.
11.4. Competitive Advantages
In today's dynamic market landscape, businesses must identify and leverage their competitive advantages to thrive. Competitive advantages are unique attributes or capabilities that allow a company to outperform its rivals. Here are some key aspects to consider:
- Cost Leadership: Companies that can produce goods or services at a lower cost than competitors can offer lower prices, attracting price-sensitive customers. This strategy often involves economies of scale, efficient production processes, and cost-effective supply chain management. Rapid Innovation utilizes AI-driven analytics to optimize operational efficiencies, enabling clients to achieve cost leadership in their respective markets.
- Differentiation: Offering unique products or services that stand out in the market can create a strong competitive edge. This could be through superior quality, innovative features, or exceptional customer service. Brands like Apple exemplify differentiation through their innovative technology and design. Rapid Innovation helps clients leverage AI and blockchain to create distinctive offerings that resonate with consumers, enhancing their market position.
- Brand Loyalty: Building a strong brand can lead to customer loyalty, which is a significant competitive advantage. Loyal customers are less likely to switch to competitors, even when prices fluctuate. Companies like Coca-Cola have cultivated brand loyalty through consistent marketing and product quality. By integrating AI-driven customer insights, Rapid Innovation assists clients in developing strategies that foster brand loyalty and engagement.
- Technological Innovation: Staying ahead in technology can provide a significant advantage. Companies that invest in research and development can create cutting-edge products that meet evolving consumer needs. For instance, Tesla's advancements in electric vehicle technology have positioned it as a leader in the automotive industry. Rapid Innovation empowers clients to harness the latest advancements in AI and blockchain, ensuring they remain at the forefront of technological innovation.
- Market Positioning: Understanding and effectively targeting specific market segments can enhance competitive advantage. Companies that tailor their offerings to meet the unique needs of niche markets can dominate those segments. Rapid Innovation's expertise in data analytics allows clients to identify and capitalize on niche opportunities, optimizing their market positioning.
- Strategic Partnerships: Collaborating with other businesses can enhance capabilities and market reach. Strategic alliances can provide access to new technologies, distribution channels, and customer bases. Rapid Innovation facilitates strategic partnerships by connecting clients with key players in the AI and blockchain ecosystems, enhancing their competitive landscape.
- Sustainable Competitive Advantage: Companies that develop sustainable competitive advantages can maintain their market position over the long term. This involves creating unique value propositions that are difficult for competitors to replicate. Rapid Innovation assists clients in identifying and nurturing these sustainable competitive edges.
- Competitive Advantage Defined: Understanding what constitutes a competitive advantage is crucial for businesses. It encompasses various factors, including cost leadership, differentiation, and brand loyalty, which collectively contribute to a company's ability to outperform its rivals.
- Examples of Competitive Advantage: Real-world examples of competitive advantages can provide valuable insights for businesses. Companies like Amazon leverage their vast distribution network and customer data to maintain a competitive edge in the e-commerce space.
- Sample of Competitive Advantage: A sample of competitive advantage could include a company that utilizes advanced technology to streamline operations, resulting in lower costs and faster delivery times compared to competitors. For instance, businesses looking to enhance their trading strategies can benefit from specialized services like crypto arbitrage bot development.
11.5. Funding and Investment Strategies
Securing funding and making informed investment decisions are crucial for business growth and sustainability. Here are some effective strategies:
- Bootstrapping: Many startups begin by self-funding their operations. This approach allows entrepreneurs to maintain control over their business without incurring debt or giving away equity. However, it may limit growth potential in the early stages.
- Angel Investors: Attracting angel investors can provide essential capital for startups. These individuals often invest their personal funds in exchange for equity. They can also offer valuable mentorship and industry connections.
- Venture Capital: For businesses with high growth potential, venture capital can be a significant source of funding. Venture capitalists invest in exchange for equity and often provide strategic guidance to help scale the business.
- Crowdfunding: Platforms like Kickstarter and Indiegogo allow businesses to raise funds from a large number of people. This method not only provides capital but also validates the product idea through consumer interest.
- Grants and Competitions: Many governments and organizations offer grants and business competitions that provide funding without requiring repayment. These can be excellent opportunities for startups to secure capital.
- Debt Financing: Traditional loans from banks or financial institutions can provide necessary funds for expansion. However, businesses must ensure they can manage repayment obligations to avoid financial strain.
- Public Offerings: For established companies, going public through an Initial Public Offering (IPO) can raise substantial capital. This strategy, however, requires compliance with regulatory standards and can expose the company to market volatility.
12. Future Trends and Outlook
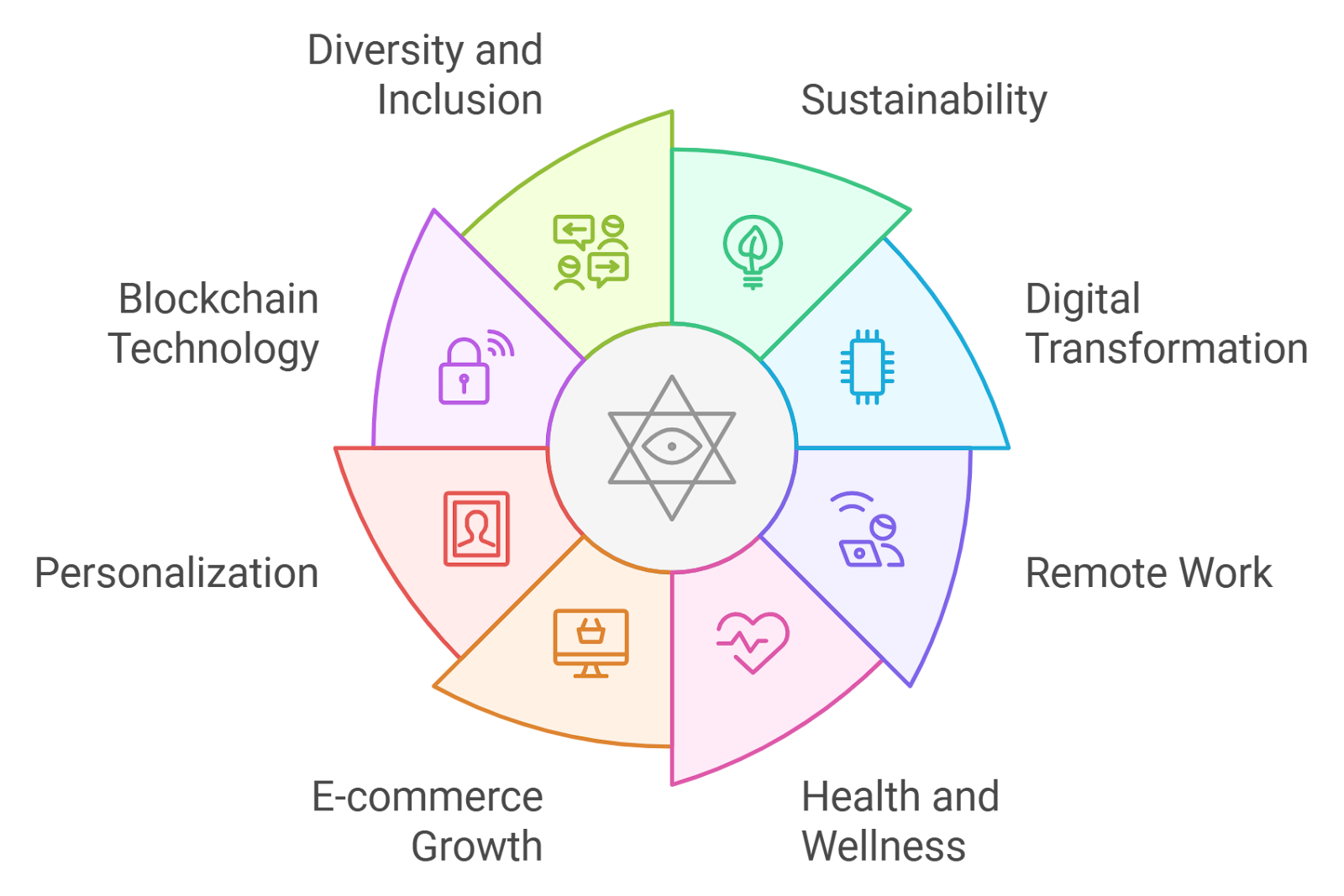
‍
As industries evolve, understanding future trends is essential for strategic planning. Here are some key trends shaping the future:
- Sustainability: There is a growing emphasis on sustainable practices across industries. Companies that adopt eco-friendly practices and products are likely to attract environmentally conscious consumers. This trend is not just a moral imperative but also a competitive advantage.
- Digital Transformation: The shift towards digital solutions continues to accelerate. Businesses are increasingly adopting technologies like artificial intelligence, machine learning, and automation to enhance efficiency and customer experience.
- Remote Work: The COVID-19 pandemic has permanently altered workplace dynamics. Remote work is becoming a standard practice, leading to changes in company culture, employee engagement, and talent acquisition strategies.
- Health and Wellness: Consumers are prioritizing health and wellness more than ever. Businesses that offer products and services promoting well-being are likely to see increased demand. This trend spans various sectors, including food, fitness, and mental health.
- E-commerce Growth: The rise of online shopping is reshaping retail. Companies must invest in robust e-commerce platforms and digital marketing strategies to capture the growing online consumer base.
- Personalization: Consumers expect personalized experiences tailored to their preferences. Businesses leveraging data analytics to provide customized offerings can enhance customer satisfaction and loyalty.
- Blockchain Technology: The adoption of blockchain is increasing across various sectors, including finance, supply chain, and healthcare. This technology offers enhanced security, transparency, and efficiency in transactions.
- Diversity and Inclusion: There is a heightened focus on diversity and inclusion within organizations. Companies that prioritize diverse hiring practices and inclusive cultures are likely to attract top talent and foster innovation.
By staying informed about these trends, businesses can adapt their strategies to remain competitive and meet the evolving needs of their customers. Rapid Innovation is committed to guiding clients through these changes, leveraging AI and blockchain technologies to drive growth and enhance operational efficiency.
12.1. Emerging Technologies
Emerging technologies are innovations that are currently developing or will be developed over the next few years, significantly impacting various sectors. These technologies often lead to new products, services, and ways of doing business. The impact of these technologies is profound, as they drive innovation and efficiency across various sectors, including healthcare, finance, and education.
- Artificial Intelligence (AI): AI is transforming industries by automating processes, enhancing decision-making, and improving customer experiences. At Rapid Innovation, we leverage new AI technology to help businesses streamline operations, reduce costs, and enhance customer engagement, ultimately leading to greater ROI.
- Blockchain: This decentralized ledger technology is revolutionizing finance, supply chain management, and data security by providing transparency and reducing fraud. Rapid Innovation assists clients in implementing blockchain solutions that enhance trust and accountability in transactions, resulting in improved operational efficiency and reduced risk.
- Internet of Things (IoT): IoT connects everyday devices to the internet, enabling data collection and analysis for smarter homes, cities, and industries. Our expertise in IoT allows us to develop solutions that optimize resource management and improve decision-making processes, driving significant cost savings for our clients.
- Augmented Reality (AR) and Virtual Reality (VR): These technologies are changing how we interact with digital content, offering immersive experiences in gaming, education, and training. Rapid Innovation creates AR and VR applications that enhance user engagement and training effectiveness, providing businesses with innovative ways to connect with their audiences.
- 5G Technology: The rollout of 5G networks is set to enhance connectivity, enabling faster data transfer and supporting the growth of IoT and smart devices. We help clients harness the power of 5G to improve their operational capabilities and customer experiences, paving the way for new business opportunities. For businesses looking to integrate these advanced technologies, our expertise in AI software development can provide tailored solutions that drive innovation and efficiency.
12.2. AI and Personalized Education
Artificial Intelligence is reshaping the educational landscape by enabling personalized learning experiences tailored to individual student needs. AI-driven platforms can assess a student's strengths and weaknesses, adjusting the curriculum accordingly to optimize learning outcomes. Intelligent tutoring systems provide real-time feedback and support, helping students grasp complex concepts at their own pace. Additionally, AI analyzes vast amounts of educational data to identify trends and insights, allowing educators to make informed decisions about teaching strategies. AI tools can assist students with disabilities by providing customized resources and support, ensuring an inclusive learning environment. Furthermore, AI can enhance student engagement through gamification and interactive content, making learning more enjoyable and effective. The integration of AI in education not only improves learning outcomes but also prepares students for a future where technology plays a crucial role in various professions. At Rapid Innovation, we specialize in developing AI solutions that empower educational institutions to deliver personalized learning experiences, ultimately leading to improved student performance and satisfaction.
12.3. Quantum Computing Applications
Quantum computing represents a significant leap in computational power, utilizing the principles of quantum mechanics to process information in ways that classical computers cannot. Quantum computers can tackle problems involving large datasets and complex variables, such as drug discovery and climate modeling, much faster than traditional computers. This technology has the potential to revolutionize cybersecurity by creating unbreakable encryption methods, ensuring data security in an increasingly digital world. Industries such as logistics and finance can benefit from quantum algorithms that optimize routes, schedules, and resource allocation, leading to significant cost savings. Moreover, quantum computing can enhance machine learning algorithms, enabling faster data processing and more accurate predictions. Researchers can use quantum simulations to discover new materials with unique properties, paving the way for advancements in technology and manufacturing. As quantum computing continues to evolve, its applications will likely transform various fields, driving innovation and efficiency in ways previously thought impossible. Rapid Innovation is at the forefront of this technological revolution, helping clients explore and implement quantum computing solutions that can significantly enhance their operational capabilities and competitive edge.
In addition to these advancements, the latest technologies, such as new battery technology and emerging tech, are also playing a crucial role in shaping the future. The focus on new advanced technology and the latest technological advancements, including new artificial intelligence technology and emerging IT technologies, is driving innovation across various sectors. As we look towards 2023, the emphasis on new technologies 2023 and the latest technology trends will continue to influence how businesses operate and compete in the market.
12.4. Global Educational Transformation
The landscape of education is undergoing a significant transformation on a global scale. This shift is driven by various factors, including technological advancements, changing societal needs, and the demand for more personalized learning experiences. Key aspects of this transformation include:
- Increased access to education through online platforms and resources.
- The rise of blended learning models that combine traditional and digital methods.
- Emphasis on lifelong learning to adapt to rapidly changing job markets.
- Focus on developing critical thinking, creativity, and problem-solving skills.
- Integration of global perspectives in curricula to prepare students for a diverse world.
Countries are re-evaluating their educational systems to ensure they meet the needs of the 21st century. For instance, nations are investing in teacher training and curriculum development to incorporate technology effectively. The use of data analytics in education is also becoming prevalent, allowing for tailored learning experiences that cater to individual student needs. At Rapid Innovation, we leverage AI and blockchain technologies to enhance these educational transformations, providing solutions that increase efficiency and effectiveness, ultimately leading to greater ROI for educational institutions. This includes initiatives like digital transformation for education and educational transformation that focus on integrating technology into learning environments.
12.5. Interdisciplinary Integration
Interdisciplinary integration in education refers to the blending of different academic disciplines to create a more holistic learning experience. This approach encourages students to make connections between subjects, fostering a deeper understanding of complex issues. The benefits of interdisciplinary integration include:
- Promotes critical thinking and problem-solving skills.
- Encourages collaboration among students from diverse backgrounds.
- Prepares students for real-world challenges that require multifaceted solutions.
- Enhances creativity by allowing students to explore topics from various angles.
- Supports the development of a more adaptable workforce.
Educational institutions are increasingly adopting interdisciplinary curricula, where subjects like science, technology, engineering, arts, and mathematics (STEAM) are integrated. This method not only engages students but also reflects the interconnected nature of knowledge in the real world. For example, projects that combine art and technology can lead to innovative solutions in design and engineering. Rapid Innovation can assist educational institutions in developing interdisciplinary programs that utilize AI and blockchain to create engaging, real-world applications that enhance learning outcomes. This aligns with the principles of creative schools as advocated by Ken Robinson, emphasizing the need for teaching for transformation.
12.6. Predictive Education Ecosystem
The predictive education ecosystem leverages data analytics and artificial intelligence to anticipate educational trends and student needs. This forward-thinking approach aims to enhance learning outcomes and streamline educational processes. Key features of this ecosystem include:
- Utilizes data to identify at-risk students and provide timely interventions.
- Predicts future skill requirements based on labor market trends.
- Enhances personalized learning experiences through adaptive learning technologies.
- Facilitates resource allocation by predicting enrollment trends and course demand.
- Supports continuous improvement in teaching methods and curricula.
By analyzing vast amounts of data, educational institutions can make informed decisions that benefit both students and educators. For instance, predictive analytics can help identify which teaching strategies are most effective, allowing for a more tailored approach to education. This ecosystem not only improves student engagement but also prepares them for future challenges in an ever-evolving job market. Rapid Innovation's expertise in AI can empower educational institutions to implement predictive analytics effectively, ensuring they remain at the forefront of educational excellence and innovation. This is particularly relevant in the context of digital transformation in higher education and the broader digital education transformation initiatives.
13. Implementation Roadmap
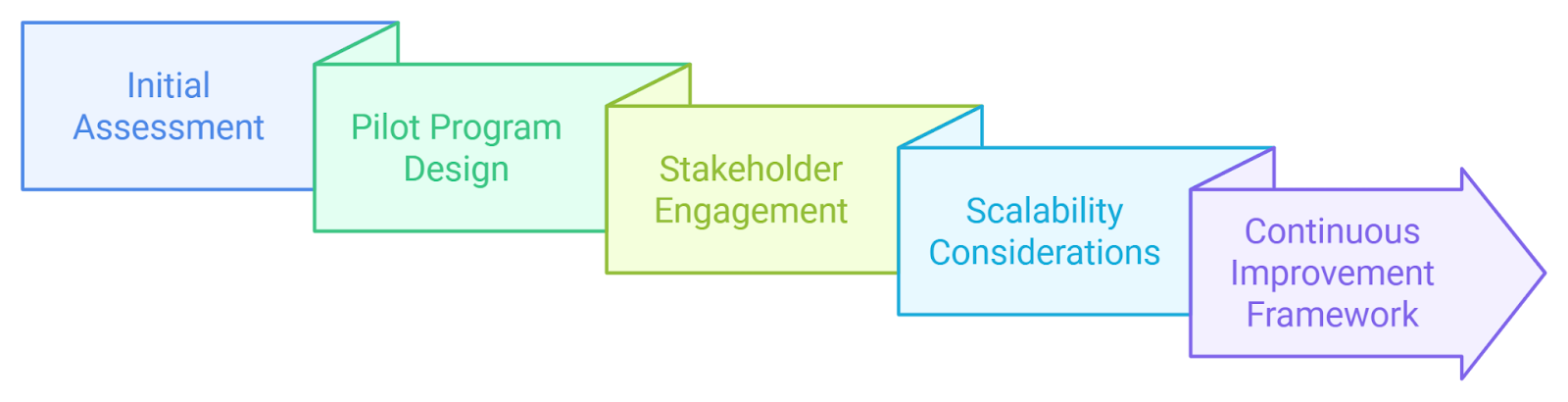
‍
An implementation roadmap is a strategic plan that outlines the steps necessary to execute a project successfully. It serves as a guide for stakeholders, ensuring that everyone is aligned and aware of their roles and responsibilities. The roadmap typically includes timelines, milestones, and key performance indicators (KPIs) to measure progress. This can include specific frameworks such as the safe implementation roadmap or the scaled agile framework implementation roadmap.
13.1 Initial Assessment
The initial assessment is a critical first step in the implementation roadmap. It involves evaluating the current state of the organization, identifying gaps, and determining the resources required for successful implementation. This process includes several key activities:
- Identify Objectives: Clearly define the goals of the project to understand what you are trying to achieve.
- Stakeholder Engagement: Involve key stakeholders early in the process to gather insights and foster buy-in.
- Resource Inventory: Assess existing resources, including personnel, technology, and budget.
- Risk Analysis: Identify potential risks and challenges that could hinder implementation.
- Data Collection: Gather relevant data to inform decision-making and establish a baseline for measuring success.
Conducting a thorough initial assessment helps to ensure that the project is grounded in reality and that the implementation plan is feasible. It sets the stage for a more effective pilot program design, whether it follows a safe implementation roadmap or a scaled agile implementation roadmap.
13.2 Pilot Program Design
Once the initial assessment is complete, the next step is to design a pilot program. This program serves as a test run for the larger implementation, allowing organizations to evaluate the effectiveness of their strategies on a smaller scale. The design process includes the following steps:
- Define Scope: Clearly outline what the pilot program will cover, including specific objectives and deliverables.
- Select Participants: Choose a representative group of users or stakeholders to participate in the pilot. This group should reflect the diversity of the larger population.
- Develop Timeline: Create a detailed timeline for the pilot program, including key milestones and deadlines.
- Establish Metrics: Determine how success will be measured, which could include user satisfaction, performance metrics, or financial outcomes.
- Feedback Mechanism: Implement a system for collecting feedback from participants throughout the pilot to help identify areas for improvement.
- Iterate and Adjust: Be prepared to make adjustments based on feedback and performance data. The pilot program should be flexible to accommodate changes as needed.
Designing a pilot program allows organizations to test their strategies in a controlled environment, minimizing risk and maximizing learning opportunities. It provides valuable insights that can inform the full-scale implementation, ensuring a smoother transition and greater chances of success. This could involve various implementation roadmaps, such as a power bi implementation roadmap, a salesforce implementation roadmap, or a devops implementation roadmap. At Rapid Innovation, we leverage our expertise in AI and Blockchain to enhance the effectiveness of these pilot programs, ensuring that our clients achieve greater ROI through data-driven insights and innovative solutions. For more information on how we can assist with your AI project estimation, visit our AI project estimation company.
13.3. Stakeholder Engagement
Stakeholder engagement is a critical component of any successful project or initiative. It involves identifying, understanding, and actively involving all parties who have an interest in the project. Effective stakeholder engagement can lead to better decision-making, increased support, and improved project outcomes.
- Identify stakeholders: Recognize all individuals, groups, or organizations that may be affected by or can influence the project. This includes internal stakeholders like employees and management, as well as external ones such as customers, suppliers, and community members.
- Understand stakeholder needs: Conduct surveys, interviews, or focus groups to gather insights about stakeholders' expectations, concerns, and interests. This information is vital for tailoring communication and engagement strategies.
- Develop a communication plan: Create a structured approach to keep stakeholders informed and involved throughout the project lifecycle. This plan should outline the frequency, methods, and types of communication to be used, which can be detailed in a stakeholder communication plan.
- Foster relationships: Build trust and rapport with stakeholders through regular interactions. This can include meetings, workshops, or informal gatherings to discuss project progress and gather feedback. Utilizing stakeholder engagement strategies can enhance these interactions.
- Monitor and adapt: Continuously assess stakeholder engagement efforts and be willing to adjust strategies based on feedback and changing circumstances. This ensures that stakeholders remain engaged and supportive. Implementing stakeholder engagement techniques can be beneficial in this process.
Engaging stakeholders effectively can lead to enhanced collaboration, reduced resistance to change, and ultimately, project success. At Rapid Innovation, we leverage AI-driven analytics to better understand stakeholder sentiments and preferences, ensuring that our engagement strategies are data-informed and impactful. We also provide examples of stakeholder engagement plans and stakeholder management strategies to guide organizations in their efforts. For more insights on effective strategies, check out our successful AI integration strategies.
13.4. Scalability Considerations
Scalability is the ability of a system, process, or organization to grow and adapt to increased demands without compromising performance. When planning for scalability, several key considerations should be taken into account.
- Assess current capacity: Evaluate the existing resources, infrastructure, and processes to determine their ability to handle increased workloads. This includes analyzing technology, personnel, and operational workflows.
- Plan for growth: Develop a strategic plan that outlines how the organization will scale in response to future demands. This may involve investing in new technologies, hiring additional staff, or streamlining processes.
- Implement flexible systems: Choose systems and technologies that can easily adapt to changing needs. Cloud-based solutions, for example, offer the flexibility to scale resources up or down as required.
- Monitor performance metrics: Establish key performance indicators (KPIs) to track the effectiveness of scaling efforts. Regularly review these metrics to identify areas for improvement and ensure that growth does not negatively impact service quality.
- Prepare for challenges: Anticipate potential obstacles that may arise during the scaling process, such as resource constraints or resistance to change. Develop contingency plans to address these challenges proactively.
By considering scalability from the outset, organizations can position themselves for sustainable growth and long-term success. Rapid Innovation specializes in developing scalable AI and blockchain solutions that can grow alongside your business, ensuring that you are always prepared for future demands.
13.5. Continuous Improvement Framework
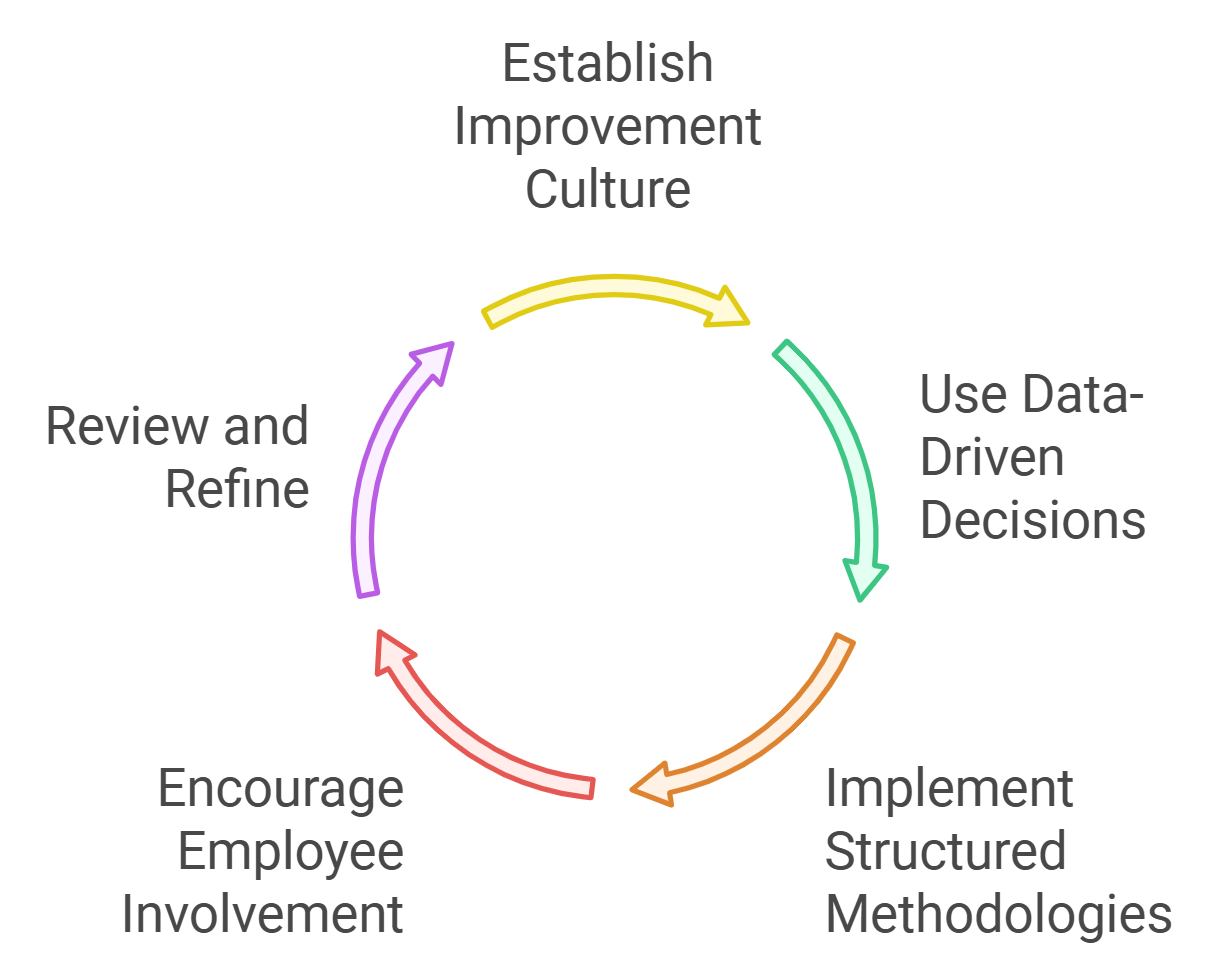
‍
A continuous improvement framework is a systematic approach to enhancing processes, products, or services over time. This framework encourages organizations to regularly evaluate their performance and implement changes that lead to better outcomes.
- Establish a culture of improvement: Foster an organizational culture that values innovation and encourages employees to identify areas for enhancement. This can be achieved through training, recognition programs, and open communication.
- Use data-driven decision-making: Collect and analyze data to identify trends, inefficiencies, and opportunities for improvement. Tools such as performance dashboards and analytics software can aid in this process.
- Implement structured methodologies: Adopt established methodologies like Lean, Six Sigma, or the Plan-Do-Check-Act (PDCA) cycle to guide improvement efforts. These frameworks provide a clear structure for identifying problems, testing solutions, and measuring results.
- Encourage employee involvement: Engage employees at all levels in the continuous improvement process. Solicit their feedback and ideas, as they often have valuable insights into operational challenges and potential solutions.
- Review and refine: Regularly assess the effectiveness of improvement initiatives and make necessary adjustments. This iterative process ensures that the organization remains responsive to changing needs and continues to evolve.
By implementing a continuous improvement framework, organizations can enhance efficiency, reduce waste, and ultimately deliver greater value to customers and stakeholders. At Rapid Innovation, we integrate AI and blockchain technologies into this framework, enabling real-time data analysis and process optimization that drive continuous improvement and maximize ROI. Additionally, we provide stakeholder engagement best practices and examples of stakeholder engagement plans to support organizations in their continuous improvement efforts.