Table Of Contents
Category
Machine Learning (ML)
Natural Language Processing (NLP)
Artificial Intelligence (AI)
Decentralized Finance (DeFi)
Blockchain-as-a-Service (BaaS)
Smart Contracts
1. Introduction to AI-Powered Side Effect Monitoring
Artificial Intelligence (AI) is revolutionizing the field of pharmacovigilance, which is the science of monitoring the safety of pharmaceutical products. AI-powered side effect monitoring systems are designed to enhance the detection, assessment, and prevention of adverse drug reactions (ADRs). These systems leverage machine learning algorithms, natural language processing, and big data analytics to analyze vast amounts of data from various sources, including clinical trials, electronic health records, and social media.
AI-powered side effect monitoring can process and analyze data at a speed and scale that is impossible for human analysts.
By identifying patterns and trends in side effects, AI can help predict potential safety issues before they become widespread.
These systems can continuously learn and adapt, improving their accuracy over time.
The integration of AI in pharmacovigilance not only increases efficiency but also enhances the overall safety of medications. Traditional methods of monitoring side effects often rely on voluntary reporting systems, which can lead to underreporting and delayed recognition of safety signals. AI-powered systems can automate the collection and analysis of data, providing real-time insights into drug safety.
AI can aggregate data from multiple sources, providing a comprehensive view of a drug's safety profile.
Machine learning algorithms can identify signals of adverse effects that may not be apparent through conventional methods.
Natural language processing can analyze unstructured data, such as patient reviews and social media posts, to capture real-world experiences with medications.
At Rapid Innovation, we understand the critical role that AI-powered side effect monitoring plays in enhancing pharmacovigilance. Our expertise in AI development allows us to create tailored solutions that help clients streamline their drug safety processes, ultimately leading to greater ROI. By implementing our advanced AI systems, clients can expect improved detection of ADRs, reduced time to market for new drugs, and enhanced compliance with regulatory requirements.
As the healthcare landscape continues to evolve, the need for advanced pharmacovigilance systems becomes increasingly critical. AI-powered side effect monitoring not only improves patient safety but also supports regulatory compliance and enhances the overall quality of healthcare. By harnessing the power of AI, stakeholders in the pharmaceutical industry can make more informed decisions regarding drug safety and efficacy. For more insights on the advancements in AI, you can read about AI's leap in driving safety and vigilance.
1.1. Evolution of Pharmacovigilance
Pharmacovigilance is the science and activities related to the detection, assessment, understanding, and prevention of adverse effects or any other drug-related problems. Its evolution can be traced back to the early 20th century, with significant milestones marking its development.
- The thalidomide tragedy in the 1960s highlighted the need for rigorous drug safety monitoring. This incident led to the establishment of more stringent regulatory frameworks.
- In 1961, the World Health Organization (WHO) launched the International Drug Monitoring Program, which aimed to collect and analyze data on adverse drug reactions (ADRs) globally.
- The establishment of the FDA's Adverse Event Reporting System (AERS) in the United States further formalized pharmacovigilance practices.
- Over the years, advancements in technology and data analysis have transformed pharmacovigilance from a reactive to a proactive approach, allowing for real-time monitoring of drug safety.
The evolution of pharmacovigilance has been driven by the need to ensure patient safety and improve drug efficacy. As the pharmaceutical landscape continues to grow, the importance of robust pharmacovigilance systems becomes increasingly evident, including the integration of pharmacovigilance AI solutions.
1.2. The Need for AI in Side Effect Detection
Artificial Intelligence (AI) is becoming an essential tool in pharmacovigilance, particularly in the detection of side effects. The complexity and volume of data generated in healthcare necessitate innovative solutions.
- AI can analyze vast amounts of data from various sources, including electronic health records, social media, and clinical trial reports, to identify potential ADRs.
- Machine learning algorithms can improve the accuracy of signal detection, reducing false positives and negatives in adverse event reporting.
- AI-driven tools can automate the data extraction process, significantly speeding up the analysis and reporting of side effects.
- Predictive analytics powered by AI can help identify at-risk populations and potential drug interactions before they lead to adverse events.
At Rapid Innovation, we leverage pharmacovigilance AI solutions to enhance pharmacovigilance processes, ensuring that our clients can achieve greater ROI through improved efficiency and accuracy in side effect detection. By integrating AI-driven solutions, we help organizations not only comply with regulatory requirements but also enhance patient safety.
1.3. Current Challenges in Traditional Monitoring
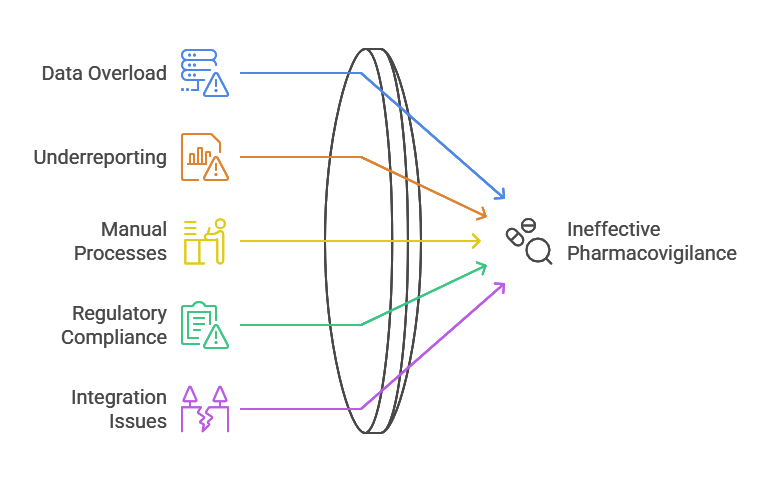
‍
Despite advancements in pharmacovigilance, traditional monitoring systems face several challenges that hinder their effectiveness.
- Data Overload: The sheer volume of data generated from various sources can overwhelm traditional systems, making it difficult to identify significant signals.
- Underreporting: Many adverse drug reactions go unreported due to a lack of awareness among healthcare professionals and patients, leading to incomplete safety profiles.
- Manual Processes: Traditional pharmacovigilance relies heavily on manual data entry and analysis, which is time-consuming and prone to human error.
- Regulatory Compliance: Keeping up with evolving regulations and guidelines can be challenging for organizations, leading to potential compliance issues.
- Integration Issues: Many pharmacovigilance systems operate in silos, making it difficult to share data and insights across different platforms and stakeholders.
At Rapid Innovation, we understand these challenges and offer tailored AI solutions that address them effectively. By automating processes and enhancing data integration, we empower our clients to navigate the complexities of pharmacovigilance, ultimately ensuring patient safety and improving operational efficiency. Addressing these challenges is crucial for enhancing the effectiveness of pharmacovigilance and ensuring patient safety in an increasingly complex healthcare environment.
1.4. Regulatory Framework and Requirements
The regulatory framework surrounding technology, particularly in sectors like finance, healthcare, and data privacy, is crucial for ensuring compliance and protecting consumers. Understanding these regulations is essential for businesses to operate legally and ethically.
- Data Protection Laws: Regulations such as the General Data Protection Regulation (GDPR) in Europe and the California Consumer Privacy Act (CCPA) in the United States set strict guidelines on how personal data should be collected, stored, and processed. Companies must ensure they have robust data protection measures in place to avoid hefty fines. Rapid Innovation assists clients in implementing compliant data management systems, ensuring that their operations align with these regulations.
- Industry-Specific Regulations: Different industries have unique regulatory requirements. For example, the Health Insurance Portability and Accountability Act (HIPAA) governs the handling of medical information in the healthcare sector, while the Financial Industry Regulatory Authority (FINRA) oversees trading practices in finance. Compliance with these regulations is critical for maintaining trust and avoiding legal repercussions. Rapid Innovation provides tailored consulting services to help clients navigate these complex regulatory landscapes effectively, including the use of regtech software to streamline compliance processes.
- Emerging Technologies: As technologies evolve, so do regulations. The rise of artificial intelligence (AI) and machine learning has prompted discussions around ethical AI use, bias in algorithms, and accountability. Regulatory bodies are increasingly focusing on creating frameworks that address these concerns, ensuring that technology is used responsibly. Rapid Innovation stays ahead of these trends, advising clients on best practices for ethical AI deployment, including compliance regtech solutions that facilitate adherence to regulatory requirements.
- International Compliance: For businesses operating globally, understanding international regulations is vital. Companies must navigate a complex landscape of laws that vary by country, which can impact data transfer, consumer rights, and operational practices. Rapid Innovation offers expertise in international compliance, helping clients develop strategies that align with diverse regulatory requirements, including information technology regulatory compliance.
- Regular Audits and Assessments: Organizations should conduct regular audits to ensure compliance with applicable regulations. This includes reviewing data handling practices, employee training on compliance issues, and updating policies as regulations change. Rapid Innovation supports clients in establishing robust audit frameworks, ensuring ongoing compliance and risk management, particularly through the use of regtech regulatory reporting tools.
2. Core Technologies and Components
Core technologies and components form the backbone of modern digital solutions. Understanding these technologies is essential for businesses looking to innovate and stay competitive in a rapidly evolving landscape.
- Cloud Computing: Cloud technology allows businesses to store and access data over the internet, providing scalability and flexibility. It enables organizations to reduce infrastructure costs and improve collaboration. Rapid Innovation helps clients leverage cloud solutions to enhance operational efficiency and scalability.
- Big Data Analytics: The ability to analyze large datasets helps businesses make informed decisions. Big data analytics tools can uncover trends, customer preferences, and operational efficiencies, driving strategic initiatives. Rapid Innovation empowers clients with advanced analytics capabilities, enabling them to derive actionable insights from their data, which is crucial for big data regulatory compliance.
- Internet of Things (IoT): IoT connects devices and systems, enabling real-time data exchange. This technology is transforming industries by improving efficiency, enhancing customer experiences, and enabling predictive maintenance. Rapid Innovation assists clients in implementing IoT solutions that optimize processes and drive innovation.
- Blockchain: This decentralized ledger technology enhances security and transparency in transactions. It is particularly valuable in sectors like finance and supply chain management, where trust and traceability are paramount. Rapid Innovation provides blockchain consulting services, helping clients harness this technology for secure and efficient operations, especially in the context of blockchain regulatory compliance.
- Artificial Intelligence (AI): AI technologies, including machine learning and natural language processing, are revolutionizing how businesses operate. They enable automation, enhance decision-making, and improve customer interactions. Rapid Innovation specializes in AI development, offering tailored solutions that drive efficiency and innovation, while also addressing the regulatory compliance challenges associated with new technologies.
2.1. Natural Language Processing
Natural Language Processing (NLP) is a critical component of AI that focuses on the interaction between computers and human language. It enables machines to understand, interpret, and respond to human language in a meaningful way.
- Text Analysis: NLP techniques can analyze text data to extract insights, identify sentiment, and summarize information. This is particularly useful in customer feedback analysis and social media monitoring. Rapid Innovation employs NLP to help clients gain valuable insights from their textual data.
- Chatbots and Virtual Assistants: NLP powers chatbots and virtual assistants, allowing them to understand user queries and provide relevant responses. This technology enhances customer service by providing instant support and information. Rapid Innovation develops customized chatbot solutions that improve customer engagement and satisfaction.
- Language Translation: NLP facilitates real-time language translation, breaking down communication barriers. Tools like Google Translate utilize NLP algorithms to provide accurate translations across multiple languages. Rapid Innovation integrates translation capabilities into client applications, enhancing global reach.
- Speech Recognition: NLP enables speech recognition systems to convert spoken language into text. This technology is widely used in applications like voice-activated assistants and transcription services. Rapid Innovation implements speech recognition solutions that streamline operations and improve user experiences.
- Sentiment Analysis: Businesses use NLP for sentiment analysis to gauge public opinion and customer satisfaction. By analyzing social media posts, reviews, and surveys, organizations can better understand consumer sentiment and adjust their strategies accordingly. Rapid Innovation provides sentiment analysis tools that help clients refine their marketing strategies.
- Content Generation: Advanced NLP models can generate human-like text, assisting in content creation for marketing, journalism, and more. This capability can save time and resources while maintaining quality. Rapid Innovation leverages NLP for content generation, enabling clients to produce high-quality materials efficiently.
- Challenges in NLP: Despite its advancements, NLP faces challenges such as understanding context, sarcasm, and cultural nuances. Continuous research and development are necessary to improve the accuracy and effectiveness of NLP applications. Rapid Innovation is committed to ongoing innovation in NLP, ensuring that our solutions remain at the forefront of technology, including addressing the challenges posed by information technology regulations and compliance, and offering natural language processing solutions.
2.2. Machine Learning Algorithms
Machine learning algorithms are the backbone of artificial intelligence, enabling systems to learn from data and make predictions or decisions without being explicitly programmed. These algorithms can be broadly categorized into two main types: supervised learning and unsupervised learning. Each type serves different purposes and is suited for various applications.
2.2.1. Supervised Learning Models
Supervised learning is a type of machine learning where the model is trained on a labeled dataset. This means that the input data is paired with the correct output, allowing the algorithm to learn the relationship between the two. The goal is to make predictions on new, unseen data based on the learned patterns.
- Key characteristics of supervised learning: Â
- Requires labeled data for training.
- The model learns to map inputs to outputs.
- Commonly used for classification and regression tasks.
- Common supervised learning algorithms include: Â
- Linear Regression: Used for predicting continuous values, such as house prices based on features like size and location.
- Logistic Regression: A classification algorithm that predicts binary outcomes, such as whether an email is spam or not.
- Decision Trees: A model that splits data into branches to make decisions based on feature values.
- Support Vector Machines (SVM): Effective for high-dimensional spaces, SVMs classify data by finding the optimal hyperplane. This includes variations like support vector classification and SVM in machine learning.
- Neural Networks: Inspired by the human brain, these models consist of layers of interconnected nodes and are particularly powerful for complex tasks like image and speech recognition, often referred to as neural net machine learning.
- Applications of supervised learning: Â
- Fraud detection in banking by identifying unusual transaction patterns.
- Medical diagnosis by predicting diseases based on patient data.
- Customer segmentation for targeted marketing strategies, which can be enhanced using machine learning algorithms.
At Rapid Innovation, we leverage supervised learning models to help our clients enhance their decision-making processes and improve operational efficiency. For instance, by implementing predictive analytics in customer segmentation, businesses can tailor their marketing strategies, leading to increased engagement and higher ROI.
2.2.2. Unsupervised Learning Applications
Unsupervised learning, in contrast to supervised learning, deals with unlabeled data. The algorithm attempts to learn the underlying structure of the data without any explicit guidance on what the output should be. This type of learning is particularly useful for discovering hidden patterns or groupings in data.
- Key characteristics of unsupervised learning: Â
- Does not require labeled data.
- The model identifies patterns and relationships in the data.
- Commonly used for clustering and association tasks.
- Common unsupervised learning algorithms include: Â
- K-Means Clustering: Groups data points into K clusters based on feature similarity, often used in market segmentation, similar to the nearest neighbors algorithm.
- Hierarchical Clustering: Builds a tree of clusters, allowing for a more detailed view of data relationships.
- Principal Component Analysis (PCA): Reduces the dimensionality of data while preserving variance, useful for data visualization.
- t-Distributed Stochastic Neighbor Embedding (t-SNE): A technique for visualizing high-dimensional data in lower dimensions, often used in exploratory data analysis.
- Applications of unsupervised learning: Â
- Customer segmentation to identify distinct groups within a customer base for personalized marketing.
- Anomaly detection in network security to identify unusual patterns that may indicate a breach.
- Market basket analysis to discover associations between products purchased together, aiding in inventory management and promotions.
At Rapid Innovation, we utilize unsupervised learning techniques to uncover valuable insights from complex datasets. For example, through customer segmentation analysis, we help businesses identify distinct customer groups, enabling them to create personalized marketing campaigns that drive sales and enhance customer loyalty.
Both supervised and unsupervised learning play crucial roles in the field of machine learning, each offering unique advantages and applications. Understanding these algorithms, including gradient descent and stochastic gradient descent, and their functionalities is essential for leveraging machine learning effectively in various domains. By partnering with Rapid Innovation, clients can harness the power of these algorithms to achieve their business goals efficiently and effectively, ultimately leading to greater ROI. Additionally, our expertise in adaptive AI development allows us to create tailored solutions that meet the specific needs of our clients.
2.2.3. Deep Learning Architectures
Deep learning architectures are the backbone of many modern artificial intelligence applications. These architectures consist of multiple layers of neural networks that can learn complex patterns from large datasets. Key types of deep learning architectures include:
- Convolutional Neural Networks (CNNs): Primarily used for image processing, CNNs excel at recognizing patterns and features in visual data. They utilize convolutional layers to automatically detect edges, shapes, and textures, making them ideal for tasks like image classification and object detection. At Rapid Innovation, we leverage CNNs, including architectures like VGG16 architecture and VGG19, to develop advanced image recognition systems that enhance product quality control and automate visual inspections, leading to significant cost savings and improved accuracy.
- Recurrent Neural Networks (RNNs): RNNs are designed for sequential data, such as time series or natural language. They maintain a memory of previous inputs, allowing them to capture temporal dependencies. Long Short-Term Memory (LSTM) networks, a type of RNN, are particularly effective for tasks like language translation and speech recognition. Our team at Rapid Innovation employs LSTMs to create predictive models for customer behavior analysis, enabling businesses to tailor their marketing strategies and increase ROI.
- Generative Adversarial Networks (GANs): GANs consist of two neural networks, a generator and a discriminator, that work against each other. The generator creates new data instances, while the discriminator evaluates them. This architecture is widely used for generating realistic images, videos, and even music. Rapid Innovation utilizes GANs to enhance creative processes in industries such as fashion and entertainment, allowing clients to generate innovative designs and content that resonate with their target audiences.
- Transformer Models: Transformers have revolutionized natural language processing (NLP) by enabling parallel processing of data. They use self-attention mechanisms to weigh the importance of different words in a sentence, making them highly effective for tasks like text generation and sentiment analysis. At Rapid Innovation, we implement transformer models to develop chatbots and virtual assistants that provide personalized customer support, improving user engagement and satisfaction.
Deep learning architectures are characterized by their ability to learn from vast amounts of data, making them suitable for applications in various fields, including healthcare, finance, and autonomous systems. The choice of architecture often depends on the specific problem being addressed and the nature of the data involved. Architectures such as residual networks, ResNet18 architecture, and Inception V3 architecture are examples of advanced models that can be utilized. By partnering with Rapid Innovation, organizations can harness the power of these architectures to drive innovation and achieve their business goals efficiently and effectively. For a more comprehensive understanding, you can refer to deep learning.
2.3. Real-time Data Processing Systems
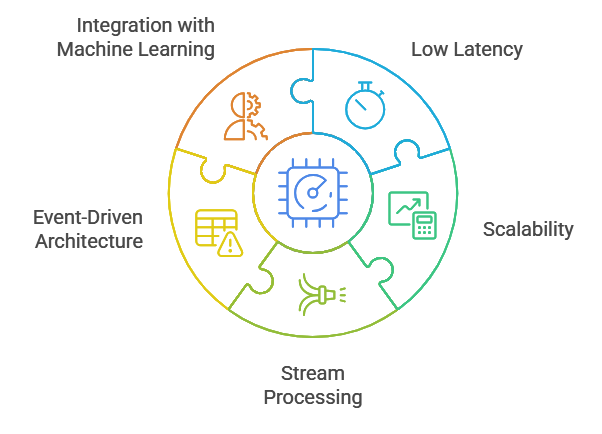
‍
Real-time data processing systems are essential for applications that require immediate insights and actions based on incoming data. These systems are designed to handle high-velocity data streams and provide timely responses. Key features of real-time data processing systems include:
- Low Latency: Real-time systems aim to minimize the delay between data ingestion and processing. This is crucial for applications like fraud detection, where immediate action can prevent losses.
- Scalability: As data volumes grow, real-time systems must scale efficiently. This often involves distributed architectures that can handle increased loads without sacrificing performance.
- Stream Processing: Unlike traditional batch processing, real-time systems process data continuously as it arrives. Technologies like Apache Kafka and Apache Flink are commonly used for stream processing, allowing for the handling of large data streams in real-time.
- Event-Driven Architecture: Real-time systems often employ an event-driven approach, where actions are triggered by specific events in the data stream. This allows for dynamic responses to changing conditions.
- Integration with Machine Learning: Many real-time systems incorporate machine learning models to analyze data on-the-fly. This enables predictive analytics and automated decision-making, enhancing the system's responsiveness.
Real-time data processing is critical in various industries, including finance for trading algorithms, healthcare for monitoring patient vitals, and e-commerce for personalized recommendations. The ability to process and analyze data in real-time can provide a competitive edge and improve operational efficiency.
2.4. Knowledge Graph Integration
Knowledge graph integration involves the process of combining and linking data from various sources into a unified graph structure. Knowledge graphs represent relationships between entities, enabling more meaningful data analysis and insights. Key aspects of knowledge graph integration include:
- Semantic Relationships: Knowledge graphs capture the semantics of data by defining relationships between entities. This allows for richer queries and insights, as users can explore connections between different data points.
- Data Interoperability: Integrating data from diverse sources often involves addressing differences in formats and structures. Knowledge graphs facilitate interoperability by providing a common framework for representing data.
- Enhanced Search Capabilities: Knowledge graphs improve search functionality by enabling more intuitive queries. Users can search for entities and their relationships, leading to more relevant results.
- Contextual Understanding: By integrating data into a knowledge graph, systems can gain a better understanding of context. This is particularly useful in applications like recommendation systems, where understanding user preferences and behaviors is crucial.
- Machine Learning and AI Integration: Knowledge graphs can enhance machine learning models by providing additional context and relationships. This can lead to improved accuracy in predictions and recommendations.
Knowledge graph integration is increasingly used in various domains, including search engines, social networks, and enterprise data management. By leveraging the power of knowledge graphs, organizations can unlock valuable insights and drive better decision-making. Rapid Innovation specializes in knowledge graph integration, helping clients to create a cohesive data ecosystem that enhances their analytical capabilities and supports strategic initiatives.
2.5. Signal Detection Mechanisms
Signal detection mechanisms are essential in identifying and monitoring potential safety issues related to medical products, including drugs and devices. These mechanisms help in recognizing adverse events and ensuring patient safety.
- Types of Signal Detection Mechanisms: Â
- Spontaneous Reporting Systems: Healthcare professionals and patients report adverse events voluntarily. This system relies on the willingness of individuals to report incidents, which can lead to underreporting.
- Automated Data Mining: Advanced algorithms analyze large datasets to identify patterns that may indicate safety concerns. This method can uncover signals that might not be apparent through traditional reporting.
- Active Surveillance: This involves proactive monitoring of specific populations to detect adverse events. It often includes cohort studies or registries that track patient outcomes over time.
- Challenges in Signal Detection: Â
- Data Quality: Inconsistent reporting and incomplete data can hinder the effectiveness of signal detection.
- False Positives: Automated systems may flag signals that are not clinically significant, leading to unnecessary investigations.
- Timeliness: Rapid detection is crucial, but delays in reporting can lead to prolonged exposure to unsafe products.
- Regulatory Framework: Various regulatory bodies, such as the FDA and EMA, have established guidelines for signal detection. These guidelines help standardize processes and improve the reliability of safety monitoring.
3. Data Sources and Integration
Data sources and integration play a critical role in enhancing the effectiveness of signal detection mechanisms. By leveraging diverse data sources, healthcare organizations can gain comprehensive insights into patient safety and product efficacy.
- Key Data Sources: Â
- Clinical Trials: Data from clinical trials provide initial safety and efficacy information about new drugs and devices.
- Post-Marketing Surveillance: Ongoing monitoring after a product is released helps identify long-term effects and rare adverse events.
- Patient Registries: These databases track specific patient populations and their outcomes, offering valuable insights into the real-world use of medical products.
- Integration Challenges: Â
- Data Silos: Different healthcare systems may store data in isolated silos, making it difficult to access comprehensive information.
- Interoperability: Ensuring that various data systems can communicate effectively is crucial for seamless integration.
- Data Privacy: Balancing the need for data sharing with patient privacy concerns is a significant challenge.
- Benefits of Data Integration: Â
- Enhanced Signal Detection: Combining data from multiple sources improves the ability to identify safety signals.
- Holistic Patient Insights: Integrated data provides a more complete picture of patient health, leading to better decision-making.
- Improved Regulatory Compliance: Streamlined data integration can help organizations meet regulatory requirements more efficiently.
3.1. Electronic Health Records (EHR)
Electronic Health Records (EHR) are digital versions of patients' paper charts and are a vital component of modern healthcare. They facilitate the collection, storage, and sharing of patient information, significantly impacting signal detection and patient safety.
- Key Features of EHR: Â
- Comprehensive Patient Data: EHRs contain a wealth of information, including medical history, medications, allergies, and lab results.
- Real-Time Access: Healthcare providers can access patient information in real-time, improving clinical decision-making.
- Interoperability: Many EHR systems are designed to share data with other healthcare systems, enhancing collaboration among providers.
- Role in Signal Detection: Â
- Adverse Event Reporting: EHRs can streamline the reporting of adverse events by integrating reporting tools directly into the clinical workflow.
- Data Mining Capabilities: EHRs can be analyzed to identify patterns and trends that may indicate safety concerns, allowing for timely intervention.
- Longitudinal Data Tracking: EHRs enable the tracking of patient outcomes over time, providing valuable insights into the long-term effects of treatments.
- Challenges with EHR: Â
- Data Quality and Completeness: Inaccurate or incomplete data can lead to misinterpretation and hinder signal detection efforts.
- User Training: Healthcare providers must be adequately trained to use EHR systems effectively to maximize their benefits.
- Cost of Implementation: Transitioning to EHR systems can be costly and time-consuming for healthcare organizations.
- Future of EHR in Signal Detection: Â
- Artificial Intelligence: The integration of AI can enhance data analysis capabilities, improving the identification of safety signals.
- Patient Engagement: EHRs can facilitate better communication with patients, encouraging them to report adverse events directly.
- Regulatory Support: As regulatory bodies increasingly recognize the value of EHR data, there may be more initiatives to standardize EHR systems for signal detection purposes.
At Rapid Innovation, we leverage our expertise in AI to enhance these signal detection mechanisms. By implementing advanced algorithms and data integration solutions, we help healthcare organizations improve their safety monitoring processes, ultimately leading to greater ROI and better patient outcomes. Our AI-driven tools can automate data mining and enhance the accuracy of signal detection, allowing clients to respond swiftly to potential safety issues.
3.2. Clinical Trial Data
Clinical trial data is a critical component in the evaluation of new medical treatments and interventions. This data is collected through rigorous research protocols designed to assess the safety and efficacy of drugs, devices, or therapies. Clinical trials are typically divided into phases (Phase I, II, III, and IV), each serving a specific purpose in the drug development process.
- Phase I trials focus on safety and dosage, involving a small number of healthy volunteers.
- Phase II trials assess the efficacy of the treatment in a larger group of patients who have the condition.
- Phase III trials compare the new treatment to standard treatments, often involving thousands of participants.
- Phase IV trials occur after a treatment is approved, monitoring long-term effects and effectiveness in the general population.
The data generated from these trials is essential for regulatory approval and can influence clinical practice guidelines. It provides insights into:
- The effectiveness of a treatment in specific populations.
- Potential side effects and adverse reactions.
- Dosage recommendations and treatment protocols.
Regulatory bodies like the FDA and EMA rely heavily on this data to make informed decisions about drug approvals. Access to clinical trial data can also empower patients and healthcare providers to make better-informed choices regarding treatment options.
At Rapid Innovation, we leverage advanced AI analytics to streamline the collection and analysis of clinical trial data management. By employing machine learning algorithms, we can identify patterns and insights that may not be immediately apparent, thus enhancing the decision-making process for our clients. This not only accelerates the time to market for new treatments but also significantly improves the return on investment (ROI) by optimizing resource allocation throughout the trial phases. Our expertise in clinical data management solutions and the use of EDC systems for clinical trials further enhances our capabilities in this area. For more information on how AI agents can be utilized in patient care.
3.3. Patient-Reported Outcomes
Patient-reported outcomes (PROs) are valuable metrics that capture the patient's perspective on their health status, treatment satisfaction, and quality of life. These outcomes are increasingly recognized as essential in clinical research and practice. PROs can include measures of symptoms, functional status, and overall well-being. They provide insights into how a treatment affects a patient's daily life, beyond clinical measures. PROs are often collected through validated questionnaires and surveys, ensuring reliability and validity.
Incorporating PROs into clinical trials can enhance the understanding of treatment impact, leading to:
- Improved patient-centered care by aligning treatment goals with patient preferences.
- Better communication between healthcare providers and patients regarding treatment options.
- Enhanced regulatory submissions, as agencies are increasingly considering PRO data in their evaluations.
The use of PROs can also facilitate shared decision-making, allowing patients to express their values and preferences in the treatment process.
Rapid Innovation employs AI-driven tools to analyze PRO data efficiently, enabling healthcare providers to tailor treatments based on real-world patient feedback. This approach not only enhances patient satisfaction but also contributes to better clinical outcomes, ultimately driving greater ROI for our clients.
3.4. Social Media and Online Forums

‍
Social media and online forums have transformed the way patients and healthcare professionals share information and experiences. These platforms serve as valuable resources for gathering insights about treatments, side effects, and overall patient experiences. Patients often turn to social media to connect with others facing similar health challenges, fostering a sense of community. Online forums provide a space for individuals to discuss their experiences with specific treatments, offering real-world insights that may not be captured in clinical trials. Social media platforms can also serve as a source of information about ongoing clinical trials, new treatments, and health-related news.
The impact of social media on healthcare includes:
- Increased awareness of health issues and treatment options.
- Opportunities for patients to share their stories, which can influence public perception and policy.
- The ability for healthcare providers to engage with patients, answer questions, and provide support.
However, it is essential to approach information from social media critically, as not all sources are reliable. Patients should verify information with healthcare professionals and consider the context of shared experiences.
At Rapid Innovation, we harness the power of AI to analyze social media data, extracting valuable insights that can inform clinical strategies and enhance patient engagement. By understanding patient sentiments and trends, we help our clients make data-driven decisions that lead to improved health outcomes and increased ROI. Our commitment to clinical research statistics and the integration of data safety monitoring board clinical trials further solidify our position as leaders in the field.
3.5. Medical Literature and Publications
Medical literature and publications play a crucial role in advancing healthcare knowledge and practice. They encompass a wide range of materials, including peer-reviewed medical literature, clinical guidelines, case reports, and systematic reviews. These publications serve as a foundation for evidence-based medicine, allowing healthcare professionals to make informed decisions based on the latest research findings.
- Peer-reviewed journals are essential for disseminating new research findings. They ensure that studies undergo rigorous evaluation by experts in the field before publication.
- Clinical guidelines provide recommendations based on a synthesis of the best available evidence. They help clinicians apply research findings to real-world practice.
- Systematic reviews and meta-analyses aggregate data from multiple studies, offering a comprehensive overview of a particular topic or treatment.
- Case reports highlight unique or rare clinical scenarios, contributing to the broader understanding of diseases and treatment outcomes.
Access to medical literature publications is facilitated by various databases and platforms, such as PubMed, Cochrane Library, and Google Scholar. These resources enable healthcare professionals to stay updated on the latest developments in their fields. Furthermore, open-access journals are becoming increasingly popular, allowing wider access to research findings without subscription barriers. Additionally, AI agents are being utilized for diagnostic support.
3.6. Adverse Event Reporting Systems
Adverse event reporting systems are critical components of patient safety and pharmacovigilance. These systems collect and analyze data on adverse events related to medical treatments, devices, and procedures. By monitoring these events, healthcare organizations can identify potential safety issues and take appropriate actions to mitigate risks.
- The primary goal of adverse event reporting is to enhance patient safety by identifying trends and patterns in adverse events.
- Reporting systems can be voluntary or mandatory, depending on regulatory requirements. For example, the FDA's MedWatch program allows healthcare professionals and consumers to report adverse events related to drugs and medical devices.
- Data collected through these systems can lead to important regulatory actions, such as label changes, product recalls, or the withdrawal of unsafe products from the market.
- The analysis of adverse event data can also inform clinical practice guidelines and improve the overall quality of care.
Healthcare professionals play a vital role in these reporting systems. By documenting and reporting adverse events, they contribute to a larger database that can help identify safety signals and improve patient outcomes. Additionally, education and training on the importance of reporting can enhance participation in these systems.
4. AI Agent Capabilities
AI agents are transforming the healthcare landscape by providing innovative solutions to various challenges. Their capabilities range from data analysis to patient interaction, significantly enhancing the efficiency and effectiveness of healthcare delivery.
- Data analysis: AI agents can process vast amounts of medical data quickly and accurately. They can identify patterns and trends that may not be apparent to human analysts, leading to improved diagnostic accuracy and treatment recommendations.
- Natural language processing (NLP): AI agents equipped with NLP can understand and interpret human language, enabling them to assist in clinical documentation, patient communication, and even literature reviews.
- Predictive analytics: AI can analyze historical patient data to predict outcomes, such as the likelihood of disease progression or the effectiveness of specific treatments. This capability allows for personalized medicine approaches tailored to individual patient needs.
- Virtual health assistants: AI agents can serve as virtual health assistants, providing patients with information about their conditions, medication reminders, and appointment scheduling. This enhances patient engagement and adherence to treatment plans.
- Decision support: AI can assist healthcare professionals in making clinical decisions by providing evidence-based recommendations and alerts for potential drug interactions or contraindications.
At Rapid Innovation, we leverage these AI capabilities to help healthcare organizations enhance their operational efficiency and improve patient outcomes. By integrating AI solutions into existing systems, we enable our clients to achieve greater ROI through improved data management, streamlined processes, and enhanced patient engagement. The integration of AI agents into healthcare systems is not without challenges. Issues such as data privacy, ethical considerations, and the need for human oversight must be addressed to ensure safe and effective use. However, the potential benefits of AI in improving patient care and operational efficiency are significant, making it a vital area of ongoing research and development.
4.1. Automated Signal Detection
Automated signal detection is a crucial component in various fields, particularly in finance, healthcare, and cybersecurity. This process involves the use of algorithms and machine learning techniques to identify significant patterns or anomalies in large datasets.
- Enhances efficiency by processing vast amounts of data quickly. Â
- Reduces human error by relying on data-driven insights. Â
- Utilizes advanced statistical methods to detect signals that may indicate potential issues or opportunities. Â
- Can be applied in pharmacovigilance to monitor adverse drug reactions, ensuring patient safety. Â
- In finance, it helps in identifying fraudulent transactions or market anomalies. Â
At Rapid Innovation, we leverage our expertise in AI to develop automated signal detection systems that not only enhance operational efficiency but also provide actionable insights. By integrating real-time data feeds, our solutions empower organizations to respond promptly to emerging threats or opportunities. The incorporation of advanced AI algorithms further enhances the accuracy and reliability of these systems, making them indispensable in today's data-driven environment.
4.2. Pattern Recognition
Pattern recognition is the ability of a system to identify and classify patterns within data. This technology is widely used in various applications, including image and speech recognition, medical diagnosis, and financial forecasting.
- Involves the use of algorithms to analyze data and identify recurring themes or trends. Â
- Can be supervised (trained with labeled data) or unsupervised (identifies patterns without prior labeling). Â
- Plays a significant role in machine learning, where systems learn from data to improve their accuracy over time. Â
- Essential in fields like healthcare for diagnosing diseases based on medical imaging. Â
- In finance, it aids in predicting stock market trends by analyzing historical data. Â
At Rapid Innovation, we harness the power of pattern recognition to drive innovation across various sectors. Our tailored solutions enable organizations to extract valuable insights from their data, enhancing decision-making processes and improving overall performance. As more data becomes accessible, our expertise ensures that clients can effectively leverage these systems to uncover trends and opportunities that drive growth.
4.3. Risk Assessment
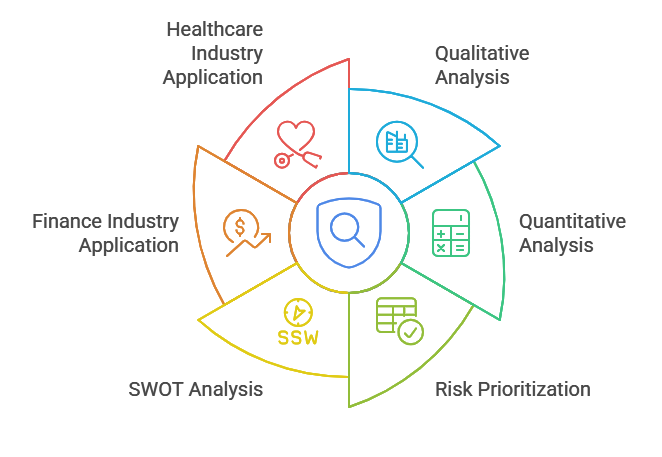
‍
Risk assessment is a systematic process of identifying, analyzing, and evaluating potential risks that could negatively impact an organization or project. This process is vital in decision-making and strategic planning.
- Involves qualitative and quantitative analysis to determine the likelihood and impact of risks. Â
- Helps organizations prioritize risks based on their potential consequences. Â
- Utilizes various tools and methodologies, such as SWOT analysis, to assess internal and external risks. Â
- Essential in industries like finance, where it helps in evaluating investment risks and regulatory compliance. Â
- In healthcare, it aids in identifying potential hazards to patient safety and implementing preventive measures. Â
At Rapid Innovation, we understand the importance of effective risk assessment in maintaining a competitive edge. Our AI-driven solutions enable organizations to develop comprehensive strategies to mitigate identified risks, ensuring better preparedness and resilience. By continuously monitoring and updating risk assessments, we help clients adapt to changing environments, ultimately leading to greater ROI and sustainable growth.
4.4. Causality Assessment
Causality assessment is a critical process in various fields, particularly in healthcare, epidemiology, and social sciences. It involves determining whether a specific factor or intervention directly causes an outcome. This assessment is essential for establishing effective interventions and understanding the dynamics of complex systems. Establishing cause-and-effect relationships is vital for effective decision-making.
Various methods are used for causality assessment, including: - Randomized controlled trials (RCTs) - Observational studies - Statistical modeling techniques
The Bradford Hill criteria are often employed to evaluate causality, which includes: - Strength of association - Consistency of findings - Specificity of the association - Temporality - Biological gradient - Plausibility - Coherence - Experiment - Analogy
Causality assessment helps in identifying risk factors and guiding public health policies. It also plays a significant role in drug safety evaluations, where understanding adverse event causality is crucial. The process of causality assessment in pharmacovigilance is particularly important for evaluating adverse events related to drug therapies. At Rapid Innovation, we leverage advanced AI algorithms to enhance the accuracy and efficiency of causality assessments, including causality assessment methods in pharmacovigilance, enabling our clients to make informed decisions that lead to better health outcomes and optimized resource allocation.
Causality assessment examples can illustrate how different factors are evaluated in clinical trials and signal detection. Understanding the causality assessment of adverse drug reactions (ADR) is essential for ensuring patient safety and effective treatment protocols. For more insights on how AI can enhance predictive analytics in insurance.
4.5. Real-time Monitoring
Real-time monitoring refers to the continuous observation and analysis of data as it is generated. This approach is increasingly utilized across various sectors, including healthcare, finance, and environmental science, to enhance decision-making and responsiveness. Real-time monitoring systems provide immediate feedback, allowing for timely interventions.
Key benefits include: - Enhanced situational awareness - Improved resource allocation - Faster response to emerging issues
Technologies used in real-time monitoring include: - Internet of Things (IoT) devices - Wearable health technology - Data analytics platforms
In healthcare, real-time monitoring can track patient vitals, enabling proactive care and reducing hospital readmissions. In environmental science, it helps in tracking pollution levels and natural disasters, facilitating quicker responses to mitigate risks. Rapid Innovation specializes in developing tailored real-time monitoring solutions that empower organizations to respond swiftly to critical situations, ultimately driving greater efficiency and ROI.
4.6. Predictive Analytics
Predictive analytics involves using statistical algorithms and machine learning techniques to analyze historical data and make predictions about future events. This approach is widely adopted in various industries, including marketing, finance, and healthcare, to enhance strategic planning and operational efficiency. Predictive analytics can identify trends and patterns that inform decision-making.
Key components include: - Data collection and preparation - Model building and validation - Deployment and monitoring of predictive models
Applications of predictive analytics include: - Customer behavior forecasting in retail - Risk assessment in finance - Patient outcome predictions in healthcare
The use of predictive analytics can lead to: - Increased efficiency and cost savings - Improved customer satisfaction - Enhanced risk management strategies
Organizations leveraging predictive analytics can gain a competitive edge by anticipating market changes and customer needs. At Rapid Innovation, we empower our clients to harness the power of predictive analytics, enabling them to optimize operations, enhance customer experiences, and achieve significant returns on investment.
5. Technical Architecture
5.1. System Design Principles
System design principles are foundational guidelines that help in creating robust, scalable, and maintainable systems. These principles ensure that the architecture can adapt to changing requirements and handle increased loads efficiently. Key principles include:
- Modularity: Breaking down a system into smaller, manageable components or modules, allowing for easier updates, testing, and maintenance. Rapid Innovation employs modular design to facilitate agile development, enabling clients to implement changes swiftly and reduce time-to-market.
- Scalability: Designing systems that can grow in capacity and performance as demand increases. This can be achieved through horizontal scaling (adding more machines) or vertical scaling (upgrading existing machines). Our solutions are tailored to ensure that clients can scale their operations seamlessly, optimizing resource allocation and enhancing ROI.
- Reliability: Ensuring that the system is dependable and can recover from failures. This often involves redundancy, failover mechanisms, and regular backups. Rapid Innovation emphasizes reliability in our architectures, ensuring that clients experience minimal downtime and maintain business continuity.
- Performance: Optimizing the system for speed and efficiency, which includes minimizing latency and maximizing throughput. By leveraging advanced algorithms and efficient coding practices, we help clients achieve superior performance, translating to better user experiences and increased customer satisfaction.
- Security: Incorporating security measures at every level of the architecture to protect data and prevent unauthorized access. This includes encryption, authentication, and regular security audits. Rapid Innovation prioritizes security, ensuring that our clients' data is safeguarded against emerging threats, thereby enhancing trust and compliance.
- Maintainability: Designing systems that are easy to update and modify, involving clear documentation, coding standards, and using design patterns that promote clarity. Our focus on maintainability allows clients to adapt their systems to evolving business needs without incurring excessive costs.
- Interoperability: Ensuring that different systems and components can work together seamlessly, often involving the use of standard protocols and APIs. Rapid Innovation's architectures are designed for interoperability, enabling clients to integrate with existing systems and third-party services effortlessly.
By adhering to these principles, developers can create systems that not only meet current needs but are also prepared for future challenges.
5.2. Data Pipeline Architecture
Data pipeline architecture refers to the structured flow of data from its source to its destination, often involving various processing stages. A well-designed data pipeline is crucial for effective data management and analytics. Key components of data pipeline architecture include:
- Data Sources: The origin of data, which can include databases, APIs, IoT devices, and more. Identifying reliable data sources is essential for accurate analytics. Rapid Innovation assists clients in selecting and integrating the most relevant data sources to enhance their analytical capabilities.
- Data Ingestion: The process of collecting and importing data into the pipeline, which can be done in real-time (streaming) or in batches. Tools like Apache Kafka and AWS Kinesis are commonly used for real-time ingestion. Our expertise in data ingestion ensures that clients can access timely data, enabling informed decision-making. This is a critical aspect of data ingestion architecture diagram and data ingestion pipeline architecture.
- Data Processing: Transforming raw data into a usable format, which can involve cleaning, filtering, aggregating, and enriching data. Technologies like Apache Spark and Apache Flink are popular for processing large datasets. Rapid Innovation employs cutting-edge processing techniques to help clients derive actionable insights from their data, aligning with data engineering pipeline architecture. For advanced processing needs, including those involving transformer models, we offer specialized services in transformer model development.
- Data Storage: Storing processed data in a way that is accessible and efficient. Options include data lakes (e.g., Amazon S3) for unstructured data and data warehouses (e.g., Google BigQuery) for structured data. We guide clients in selecting the appropriate storage solutions that align with their data strategy and business objectives, including big data pipeline architecture considerations.
- Data Analysis: Analyzing the stored data to derive insights, which can involve using business intelligence tools like Tableau or programming languages like Python and R for statistical analysis. Our consulting services empower clients to leverage advanced analytics, driving better business outcomes. This is particularly relevant in the context of data pipeline architecture examples.
- Data Visualization: Presenting data in a visual format to make insights easily understandable. Effective visualization helps stakeholders make informed decisions. Rapid Innovation specializes in creating intuitive dashboards and visualizations that enhance data comprehension and facilitate strategic planning.
- Data Monitoring and Management: Continuously monitoring the pipeline for performance and errors, including setting up alerts and dashboards to track data flow and quality. Our proactive monitoring solutions ensure that clients can maintain data integrity and optimize their operations, which is essential in both azure data pipeline architecture and aws data pipeline architecture.
A well-architected data pipeline ensures that organizations can efficiently process and analyze data, leading to better decision-making and strategic planning. Rapid Innovation is committed to helping clients build robust data pipelines that drive innovation and maximize ROI, whether through streaming data pipeline architecture or traditional ETL pipeline architecture.
5.3. Security and Privacy Framework
A robust security and privacy framework is essential for any organization to protect sensitive data and maintain user trust. This framework encompasses various policies, procedures, and technologies designed to safeguard information from unauthorized access and breaches.
- Data Encryption: Implementing encryption protocols ensures that data is unreadable to unauthorized users. This includes both data at rest and data in transit, providing a critical layer of security for sensitive information.
- Access Control: Establishing strict access controls helps limit who can view or manipulate sensitive information. Role-based access control (RBAC) is a common method used to enforce these restrictions, ensuring that only authorized personnel have access to critical data.
- Regular Audits: Conducting regular security audits and assessments can identify vulnerabilities within the system. This proactive approach allows organizations to address potential threats before they can be exploited, thereby enhancing overall security posture.
- Compliance with Regulations: Adhering to regulations such as GDPR, HIPAA, or CCPA is crucial for maintaining privacy standards. Organizations must ensure that their security measures align with these legal requirements, thereby avoiding potential penalties and fostering trust with users.
- Incident Response Plan: Developing a comprehensive incident response plan prepares organizations to react swiftly to security breaches. This plan should outline steps for containment, investigation, and communication, ensuring a coordinated response to incidents.
- User Education: Training employees on security best practices can significantly reduce the risk of human error, which is often a leading cause of data breaches. Empowering staff with knowledge helps create a culture of security awareness within the organization.
- Data Protection Frameworks: Implementing data protection frameworks is essential for establishing a structured approach to managing sensitive information. These frameworks provide guidelines and best practices to ensure that data is handled securely throughout its lifecycle.
- Security and Privacy Framework: A comprehensive security and privacy framework integrates various elements, including data protection frameworks, to create a cohesive strategy for safeguarding sensitive information and maintaining compliance with relevant regulations. For organizations looking to enhance their security measures, our MLOps consulting services can provide expert guidance and support.
5.4. Integration with Existing Systems

‍
Integrating new systems with existing infrastructure is a critical step in ensuring seamless operations and maximizing efficiency. A well-planned integration strategy can enhance productivity and reduce operational disruptions.
- Compatibility Assessment: Before integration, assess the compatibility of new systems with existing software and hardware. This includes checking for API availability and data format compatibility to ensure smooth integration.
- Data Migration: Carefully plan the data migration process to ensure that data is transferred accurately and securely. This may involve data cleansing and validation to maintain data integrity, minimizing the risk of errors during the transition.
- Interoperability: Ensure that the new system can communicate effectively with existing systems. This may require the use of middleware or integration platforms to facilitate data exchange, promoting a cohesive operational environment.
- Testing and Validation: Conduct thorough testing of the integrated systems to identify any issues before going live. This includes functional testing, performance testing, and user acceptance testing to ensure that the systems meet organizational needs.
- Training and Support: Provide training for staff on how to use the integrated systems effectively. Ongoing support is also essential to address any issues that may arise post-integration, ensuring that users can maximize the benefits of the new systems.
- Monitoring and Optimization: After integration, continuously monitor system performance and user feedback. This allows for ongoing optimization and adjustments to improve efficiency, ensuring that the systems evolve with organizational needs.
5.5. Scalability Considerations
Scalability is a vital aspect of system design, allowing organizations to grow and adapt to changing demands without compromising performance. A scalable system can accommodate increased workloads and user demands efficiently.
- Cloud Solutions: Utilizing cloud-based services can provide the flexibility needed for scalability. Cloud solutions often offer on-demand resources that can be adjusted based on current needs, allowing organizations to scale efficiently.
- Modular Architecture: Designing systems with a modular architecture allows for easy upgrades and expansions. This approach enables organizations to add new features or components without overhauling the entire system, facilitating growth.
- Load Balancing: Implementing load balancing techniques can distribute workloads evenly across servers, preventing any single server from becoming a bottleneck. This enhances performance and reliability, ensuring a smooth user experience.
- Performance Monitoring: Regularly monitor system performance metrics to identify potential scalability issues. This proactive approach allows organizations to address problems before they impact users, maintaining optimal performance levels.
- Cost Management: Consider the cost implications of scaling. Organizations should evaluate whether scaling up (adding resources) or scaling out (adding more instances) is more cost-effective for their specific needs, ensuring financial sustainability.
- Future-Proofing: Anticipate future growth and technological advancements when designing systems. This includes selecting technologies that are adaptable and can integrate with emerging solutions, ensuring long-term viability and competitiveness.
6. Implementation Strategies
Effective implementation strategies are crucial for the success of any project, particularly in technology-driven initiatives. These strategies ensure that the project meets its objectives while adhering to timelines and budgets. Below are two key components of implementation strategies: System Requirements Analysis and Data Preparation and Standardization.
6.1 System Requirements Analysis

‍
System Requirements Analysis is the foundational step in any implementation strategy. It involves identifying and documenting the needs and expectations of stakeholders to ensure that the system developed meets its intended purpose.
- Stakeholder Engagement: Involve all relevant stakeholders, including end-users, management, and IT staff, to gather comprehensive requirements. Conduct interviews, surveys, and workshops to understand their needs and expectations.
- Functional Requirements: Define what the system should do, including specific functionalities and features. Examples include user authentication, data processing capabilities, and reporting functions.
- Non-Functional Requirements: Identify performance metrics such as speed, reliability, and security. Consider scalability to accommodate future growth and changes in user demand.
- Documentation: Create a detailed requirements specification document that serves as a reference throughout the project lifecycle. Ensure that the document is clear, concise, and accessible to all stakeholders.
- Validation and Verification: Regularly review requirements with stakeholders to ensure alignment and make adjustments as necessary. Use prototypes or mock-ups to validate requirements before full-scale development.
- Tools and Techniques: Utilize tools like UML diagrams, flowcharts, and requirement management software to visualize and manage requirements effectively.
6.2 Data Preparation and Standardization
Data Preparation and Standardization is a critical phase in the implementation strategy, particularly for projects involving data analytics, machine learning, or database management. This phase ensures that the data used is accurate, consistent, and ready for analysis.
- Data Collection: Gather data from various sources, including databases, APIs, and user inputs. Ensure that data collection methods comply with relevant regulations and standards.
- Data Cleaning: Identify and rectify errors in the data, such as duplicates, missing values, and inconsistencies. Use automated tools and scripts to streamline the cleaning process.
- Data Transformation: Convert data into a suitable format for analysis, which may involve normalization, aggregation, or encoding. Ensure that the transformed data maintains its integrity and relevance.
- Standardization: Establish data standards to ensure consistency across datasets, including naming conventions, data types, and formats. Implement data governance policies to maintain quality and compliance.
- Data Integration: Combine data from different sources to create a unified dataset for analysis. Use ETL (Extract, Transform, Load) processes to facilitate integration.
- Documentation and Metadata: Maintain thorough documentation of data sources, transformations, and standards. Create metadata to provide context and facilitate understanding of the data.
- Testing and Validation: Conduct tests to ensure that the prepared data meets the required standards and is ready for analysis. Validate the data against known benchmarks or historical data to ensure accuracy.
By focusing on System Requirements Analysis and Data Preparation and Standardization, organizations can lay a solid foundation for successful implementation strategies. These steps not only enhance the quality of the final product but also improve stakeholder satisfaction and project outcomes. At Rapid Innovation, we leverage our expertise in AI development to ensure that these implementation strategies, including strategy formulation and implementation, are executed efficiently, ultimately leading to greater ROI for our clients. Additionally, we emphasize the importance of a strategy implementation plan and change implementation strategies to adapt to evolving project needs. Our approach includes strategic planning and implementation, ensuring that all aspects of business strategy implementation are considered. We also provide examples of implementation strategy examples and implementing strategies example to guide our clients through the process.
6.3. Model Training and Validation
Model training and validation are critical steps in the machine learning lifecycle. They ensure that the model not only learns from the training data but also generalizes well to unseen data.
- Training Phase: In this phase, the model learns patterns from the training dataset. The dataset is typically split into features (input variables) and labels (output variables). Various algorithms can be employed, such as linear regression, decision trees, or neural networks, depending on the problem type. This phase is essential for preparing the model for deployment, whether it be through machine learning model deployment or deploying machine learning models in production.
- Validation Phase: After training, the model is validated using a separate validation dataset. This helps in assessing the model's performance and tuning hyperparameters. Common metrics for validation include accuracy, precision, recall, and F1 score. Techniques like cross-validation are often used to ensure the model's robustness before moving on to deployment options.
- Cross-Validation: A technique used to ensure that the model is robust and not overfitting. K-fold cross-validation is a popular method where the dataset is divided into 'k' subsets. The model is trained on 'k-1' subsets and validated on the remaining subset, repeating this process 'k' times. This is crucial for ensuring the model will perform well when deployed.
- Overfitting and Underfitting: Overfitting occurs when the model learns the training data too well, capturing noise rather than the underlying pattern. Underfitting happens when the model is too simple to capture the data's complexity. Techniques like regularization, dropout (for neural networks), and pruning (for decision trees) can help mitigate these issues. Understanding these concepts is vital for successful model deployment.
- Final Evaluation: Once the model is trained and validated, it is evaluated on a test dataset to gauge its performance in real-world scenarios. This step is crucial for understanding how the model will perform when deployed, whether through ml deployment or deploying machine learning models to production. For fine-tuning language models, we offer specialized services that can enhance your model's performance.
6.4. Deployment Options
Once a model has been trained and validated, the next step is deployment. Deployment refers to the process of making the model available for use in a production environment. There are several deployment options available, each with its own advantages and challenges.
- On-Premises Deployment: The model is hosted on local servers within an organization, offering greater control over data security and compliance. However, it requires significant infrastructure investment and maintenance. This option is often chosen for sensitive applications, such as deploying deep learning models.
- Cloud-Based Solutions: Models are hosted on cloud platforms, allowing for scalability and flexibility. Organizations can leverage cloud services to reduce infrastructure costs and improve accessibility. Cloud providers often offer tools for monitoring and managing models post-deployment, such as AWS SageMaker deploy model and mlflow model serving.
- Hybrid Deployment: This option combines both on-premises and cloud solutions. Organizations can keep sensitive data on-premises while utilizing the cloud for less sensitive operations, providing a balance between control and scalability.
- Edge Deployment: This involves deploying models on edge devices, such as IoT devices or mobile phones. It reduces latency and bandwidth usage by processing data closer to the source, making it ideal for applications requiring real-time decision-making.
6.4.1. Cloud-based Solutions
Cloud-based solutions have become increasingly popular for deploying machine learning models due to their numerous benefits.
- Scalability: Cloud platforms can easily scale resources up or down based on demand, allowing organizations to handle varying workloads without significant upfront investment.
- Cost-Effectiveness: Pay-as-you-go pricing models enable organizations to only pay for the resources they use, reducing the need for large capital expenditures on hardware and infrastructure.
- Accessibility: Cloud-based models can be accessed from anywhere with an internet connection, facilitating collaboration among teams distributed across different locations.
- Integrated Tools: Many cloud providers offer integrated tools for model training, deployment, and monitoring. Services like AWS SageMaker, Google AI Platform, and Azure Machine Learning streamline the deployment process, including mlflow serving and mlflow model deployment.
- Automatic Scaling and Load Balancing: Cloud solutions can automatically adjust resources based on traffic and usage patterns, ensuring that the model remains responsive even during peak usage times.
- Security and Compliance: Major cloud providers invest heavily in security measures to protect data, and compliance with regulations such as GDPR and HIPAA is often built into their services.
- Continuous Integration and Continuous Deployment (CI/CD): Cloud platforms support CI/CD practices, allowing for frequent updates and improvements to models, ensuring that the deployed model remains relevant and effective over time.
In conclusion, both model training and validation, as well as deployment options, play a crucial role in the success of machine learning projects. Understanding these processes helps organizations make informed decisions that align with their goals and resources. At Rapid Innovation, we leverage our expertise in AI to guide clients through these critical phases, ensuring they achieve greater ROI and operational efficiency in their machine learning initiatives, including deploying machine learning models on AWS and other platforms.
6.4.2. On-premise Systems
On-premise systems refer to software and hardware solutions that are installed and run on the physical premises of an organization. This traditional model has been widely used for many years and offers several advantages and disadvantages.
- Control and Security: Organizations have complete control over their data and systems. Sensitive information remains within the company’s infrastructure, reducing the risk of data breaches associated with third-party cloud services.
- Customization: On-premise systems can be tailored to meet specific business needs. Companies can modify software and hardware configurations to align with their operational requirements.
- Performance: With on-premise systems, organizations can optimize performance based on their hardware capabilities. This can lead to faster processing times and reduced latency, especially for resource-intensive applications.
- Compliance: Certain industries have strict regulatory requirements regarding data storage and processing. On-premise systems can help organizations meet these compliance standards more easily.
- Cost: While initial setup costs can be high, ongoing operational costs may be lower compared to cloud solutions. However, organizations must consider maintenance, upgrades, and staffing costs.
Despite these advantages, on-premise systems also come with challenges:
- High Initial Investment: The upfront costs for hardware, software, and installation can be significant.
- Maintenance Burden: Organizations are responsible for maintaining and updating their systems, which can require dedicated IT resources.
- Scalability Issues: Scaling on-premise systems can be complex and costly, as it often requires additional hardware purchases and installations.
At Rapid Innovation, we understand the intricacies of on-premise systems and can assist organizations in optimizing their infrastructure. Our expertise in AI can help automate maintenance tasks, predict hardware failures, and enhance system performance, ultimately leading to a greater return on investment (ROI). For more insights on integrating AI with robotic hardware, you can read our article on the challenges and solutions.
6.4.3. Hybrid Approaches
Hybrid approaches combine both on-premise systems and cloud solutions, allowing organizations to leverage the benefits of both environments. This model is increasingly popular as businesses seek flexibility and scalability.
- Flexibility: Organizations can choose which applications and data to keep on-premise and which to move to the cloud, allowing for a tailored approach that meets specific needs.
- Cost Efficiency: By utilizing cloud resources for less critical applications, organizations can reduce costs associated with on-premise infrastructure while still maintaining control over sensitive data.
- Scalability: Hybrid systems allow organizations to scale their operations more easily. They can quickly add cloud resources to accommodate increased demand without the need for significant upfront investments in hardware.
- Disaster Recovery: Hybrid approaches can enhance disaster recovery strategies. Critical data can be stored on-premise, while backups and less critical applications can be hosted in the cloud, ensuring business continuity.
- Performance Optimization: Organizations can optimize performance by running resource-intensive applications on-premise while leveraging the cloud for less demanding tasks.
However, hybrid approaches also present challenges:
- Complexity: Managing a hybrid environment can be complex, requiring skilled IT personnel to ensure seamless integration between on-premise and cloud systems.
- Security Concerns: Organizations must implement robust security measures to protect data as it moves between on-premise and cloud environments.
- Compliance Issues: Maintaining compliance can be more challenging in a hybrid model, as organizations must ensure that both environments meet regulatory requirements.
At Rapid Innovation, we specialize in creating hybrid solutions that maximize efficiency and security. Our AI-driven analytics can provide insights into usage patterns, helping organizations make informed decisions about resource allocation and system optimization.
6.5. Quality Assurance Protocols
Quality assurance (QA) protocols are essential for ensuring that products and services meet specified requirements and standards. Implementing effective QA protocols can significantly enhance product quality and customer satisfaction.
- Standardization: Establishing standardized processes helps ensure consistency in product development and testing. This can include defining clear criteria for quality metrics and testing procedures.
- Continuous Testing: Integrating continuous testing into the development lifecycle allows for early detection of defects. This approach helps reduce the cost and time associated with fixing issues later in the process.
- Automated Testing: Utilizing automated testing tools can increase efficiency and accuracy. Automated tests can quickly execute repetitive tasks, allowing QA teams to focus on more complex testing scenarios.
- User Acceptance Testing (UAT): Involving end-users in the testing process ensures that the final product meets their needs and expectations. UAT can provide valuable feedback that helps refine the product before launch.
- Documentation: Maintaining thorough documentation of QA processes, test cases, and results is crucial. This documentation serves as a reference for future projects and helps ensure compliance with industry standards.
Challenges in implementing QA protocols include:
- Resource Allocation: Adequate resources must be allocated for QA activities, which can sometimes be overlooked in favor of development.
- Skill Gaps: Organizations may face challenges in finding skilled QA professionals who are familiar with the latest testing tools and methodologies.
- Integration with Development: Ensuring that QA protocols are integrated seamlessly with development processes can be difficult, especially in agile environments.
By focusing on these aspects, organizations can enhance their quality assurance efforts, leading to improved product quality and customer satisfaction. Rapid Innovation offers tailored QA solutions that leverage AI to streamline testing processes, ensuring that products not only meet but exceed quality standards, thereby driving greater ROI for our clients.
7. Clinical Applications
Clinical applications are essential in the healthcare sector, particularly in ensuring the safety and efficacy of medical products and treatments. This section delves into two critical aspects of clinical applications: post-market surveillance and clinical trial monitoring.
7.1 Post-Market Surveillance
Post-market surveillance refers to the processes and activities that monitor the safety and effectiveness of medical products after they have been approved for public use. This ongoing evaluation is crucial for identifying any long-term effects or rare adverse events that may not have been evident during clinical trials.
- Purpose: Â
- To ensure continued safety and efficacy of medical products.
- To detect any unforeseen side effects or complications.
- To gather real-world data that can inform future research and regulatory decisions.
- Methods: Â
- Adverse Event Reporting: Healthcare professionals and patients report any adverse events associated with a product.
- Registry Studies: Long-term studies that track patient outcomes and product performance over time.
- Surveys and Questionnaires: Collecting data from users to assess satisfaction and any issues encountered.
- Regulatory Oversight: Â
- Agencies like the FDA and EMA require manufacturers to conduct post-market surveillance as part of their regulatory obligations.
- Regular updates and reports must be submitted to these agencies to ensure compliance.
- Importance: Â
- Helps in identifying trends that may indicate safety concerns.
- Provides valuable information for healthcare providers and patients.
- Supports the development of risk management strategies.
Post-market surveillance is vital for maintaining public trust in medical products and ensuring that they continue to meet safety standards throughout their lifecycle. At Rapid Innovation, we leverage AI-driven analytics to enhance post-market surveillance processes, enabling clients to efficiently analyze vast amounts of real-world data and identify potential safety signals faster, ultimately leading to improved patient outcomes and greater ROI.
7.2 Clinical Trial Monitoring
Clinical trial monitoring is a systematic process that ensures the integrity, quality, and compliance of clinical trials. This monitoring is essential for protecting the rights and welfare of participants while ensuring that the data collected is reliable and valid.
- Objectives: Â
- To ensure compliance with regulatory requirements and Good Clinical Practice (GCP).
- To verify that the trial is conducted according to the approved protocol.
- To ensure accurate and complete data collection.
- Key Activities: Â
- Site Visits: Regular visits to trial sites to review records, interview staff, and ensure adherence to protocols.
- Data Verification: Cross-checking data collected during the trial against source documents to ensure accuracy.
- Safety Monitoring: Continuous assessment of participant safety and reporting of any adverse events.
- Roles and Responsibilities: Â
- Clinical Research Associates (CRAs): Responsible for monitoring trial sites and ensuring compliance through clinical trial monitoring.
- Principal Investigators (PIs): Oversee the trial at each site and ensure that it is conducted ethically and according to the protocol.
- Data Monitoring Committees (DMCs): Independent groups that review data and safety information to make recommendations about the continuation of the trial.
- Importance: Â
- Ensures the credibility of trial results, which is crucial for regulatory approval.
- Protects the rights and safety of participants, fostering ethical research practices.
- Facilitates timely identification of issues that may arise during the trial, allowing for prompt corrective actions.
Clinical trial monitoring, including risk based monitoring and remote monitoring in clinical trials, is a cornerstone of clinical research, ensuring that trials are conducted ethically and that the data generated is robust and reliable. Rapid Innovation employs advanced AI technologies to streamline clinical trial monitoring, enhancing data accuracy and compliance while reducing operational costs. By automating routine tasks and providing real-time insights, we help our clients achieve greater efficiency and ROI in their clinical research endeavors, including remote clinical monitoring and central monitoring clinical trials. For more information on how AI agents can enhance clinical trial management.
7.3. Drug-Drug Interaction Detection
Drug-drug interactions (DDIs) occur when two or more drugs react with each other, potentially altering their effectiveness or increasing the risk of adverse effects. Detecting these interactions is crucial for patient safety and effective treatment.
- Importance of DDI detection: Â
- Prevents adverse drug reactions (ADRs).
- Ensures optimal therapeutic outcomes.
- Reduces healthcare costs associated with complications.
- Methods for DDI detection: Â
- Clinical decision support systems (CDSS) that alert healthcare providers about potential interactions.
- Comprehensive medication reviews during patient consultations.
- Utilizing databases and software tools that compile known interactions.
- Common types of DDIs: Â
- Pharmacokinetic interactions: Affecting drug absorption, distribution, metabolism, or excretion.
- Pharmacodynamic interactions: Altering the drug's effects at the site of action.
- Factors influencing DDIs: Â
- Patient-specific factors such as age, weight, and genetic makeup.
- Polypharmacy, especially in elderly patients or those with chronic conditions.
- Resources for DDI information: Â
- The FDA and other regulatory bodies provide guidelines and databases.
- Online platforms like Medscape and Lexicomp offer detailed interaction checkers.
At Rapid Innovation, we leverage advanced AI algorithms to enhance drug interaction detection capabilities. By integrating machine learning models with existing clinical decision support systems, we can provide real-time alerts to healthcare providers, significantly improving patient safety and therapeutic outcomes. Our solutions not only streamline the detection process but also reduce the time and costs associated with managing adverse drug reactions, ultimately leading to greater ROI for healthcare organizations. For more insights on how AI can transform healthcare communication strategies.
7.4. Special Population Monitoring
Special populations, including pregnant women, children, the elderly, and individuals with comorbidities, require tailored monitoring due to their unique physiological and pharmacological characteristics.
- Importance of monitoring special populations: Â
- Increased risk of adverse effects due to altered drug metabolism.
- Variability in drug response based on age, weight, and health status.
- Key considerations for different populations: Â
- Pregnant women: Monitoring for teratogenic effects and drug transfer through breast milk.
- Pediatric patients: Adjusting dosages based on weight and developmental stage.
- Elderly patients: Considering polypharmacy and age-related changes in pharmacokinetics.
- Strategies for effective monitoring: Â
- Regular follow-ups to assess drug efficacy and side effects.
- Utilizing age-appropriate formulations and dosing guidelines.
- Engaging caregivers in the monitoring process for children and elderly patients.
- Tools and resources: Â
- Clinical guidelines from organizations like the American Academy of Pediatrics and the American Geriatrics Society.
- Pharmacovigilance databases to track adverse events in special populations.
Rapid Innovation's AI-driven solutions can assist healthcare providers in monitoring special populations more effectively. By employing predictive analytics, we can identify potential risks and tailor treatment plans accordingly, ensuring that patients receive the safest and most effective care. This proactive approach not only enhances patient outcomes but also optimizes resource allocation, leading to improved ROI for healthcare systems.
7.5. Rare Side Effect Identification
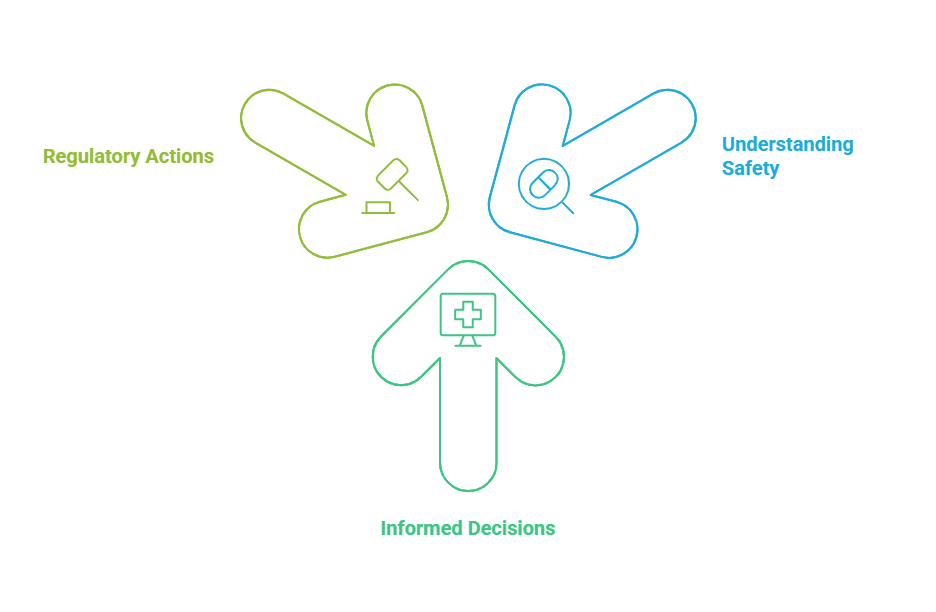
‍
Rare side effects, though infrequent, can have significant implications for patient safety and treatment adherence. Identifying these effects is essential for comprehensive pharmacovigilance.
- Importance of identifying rare side effects: Â
- Enhances understanding of a drug's safety profile.
- Informs healthcare providers and patients about potential risks.
- Supports regulatory actions and label updates.
- Methods for identifying rare side effects: Â
- Spontaneous reporting systems, such as the FDA's Adverse Event Reporting System (FAERS).
- Post-marketing surveillance studies to monitor long-term effects.
- Data mining techniques to analyze large databases for unexpected patterns.
- Challenges in rare side effect identification: Â
- Underreporting by healthcare professionals and patients.
- Difficulty in establishing causality due to the low incidence of events.
- Variability in patient populations and concurrent medications.
- Strategies to improve identification: Â
- Encouraging healthcare professionals to report adverse events.
- Educating patients about recognizing and reporting side effects.
- Utilizing advanced analytics and artificial intelligence to detect signals in large datasets.
- Resources for rare side effect information: Â
- The World Health Organization's VigiBase for global adverse event data.
- Research articles and case reports in medical journals that highlight rare side effects.
At Rapid Innovation, we harness the power of AI and data analytics to enhance the identification of rare side effects. By employing sophisticated data mining techniques, we can uncover hidden patterns in large datasets, enabling healthcare providers to make informed decisions and improve patient safety. This innovative approach not only supports regulatory compliance but also contributes to better patient outcomes, ultimately driving greater ROI for our clients in the healthcare sector.
8. Advanced Features
Advanced features in data analysis tools, such as database analytics and business analytics software, enhance the ability to extract insights from complex datasets. Two significant advanced features are multi-modal data analysis and temporal pattern detection.
8.1 Multi-modal Data Analysis
Multi-modal data analysis refers to the capability of analyzing data from various sources and formats simultaneously. This approach allows for a more comprehensive understanding of the data landscape.
- Integration of Diverse Data Types: Multi-modal analysis can handle structured data (like databases), unstructured data (like text and images), and semi-structured data (like JSON files). This integration provides a holistic view of the data.
- Enhanced Insights: By combining different data modalities, analysts can uncover relationships and patterns that may not be visible when examining a single data type. For example, correlating social media sentiment (text data) with sales figures (numerical data) can reveal how public perception affects purchasing behavior.
- Applications Across Industries: In healthcare, combining patient records (structured) with medical imaging (unstructured) can improve diagnostic accuracy. In marketing, analyzing customer feedback (text) alongside purchase history (numerical) can enhance targeted advertising strategies using marketing analytics platforms.
- Tools and Technologies: Various tools support multi-modal data analysis, including data analysis software and Python libraries like
TensorFlow
andPyTorch
, which facilitate the integration of different data types for machine learning applications. - Challenges: Despite its advantages, multi-modal data analysis can be complex due to data compatibility issues, increased computational requirements, and the need for advanced analytical skills.
At Rapid Innovation, we specialize in implementing multi-modal data analysis solutions that help our clients harness the full potential of their data. By integrating diverse data types, we enable organizations to gain deeper insights, ultimately leading to improved decision-making and greater ROI.
8.2 Temporal Pattern Detection
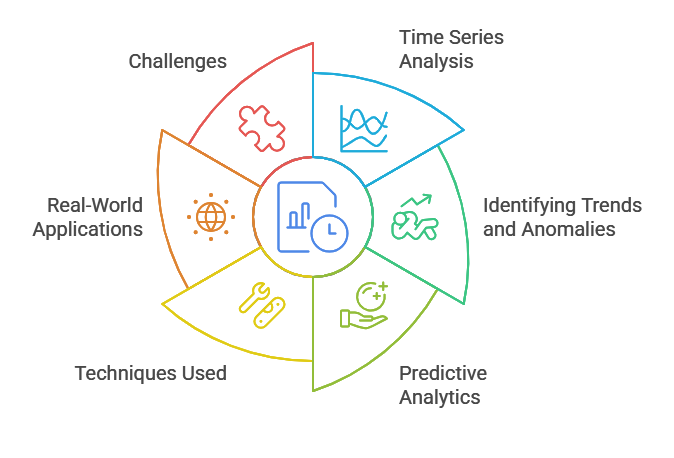
‍
Temporal pattern detection focuses on identifying trends and patterns over time within datasets. This feature is crucial for understanding how data evolves and for making predictions based on historical trends.
- Time Series Analysis: Temporal pattern detection often involves time series analysis, where data points are collected or recorded at specific time intervals. This method is widely used in various fields, including finance, weather forecasting, and resource management.
- Identifying Trends and Anomalies: Analysts can detect seasonal trends, cyclical patterns, and anomalies (outliers) that may indicate significant events or changes in behavior. For instance, a sudden spike in website traffic may correlate with a marketing campaign or a news event.
- Predictive Analytics: By understanding historical patterns, organizations can forecast future trends. This capability is essential for inventory management, financial forecasting, and demand planning, often supported by predictive data analytics.
- Techniques Used: Common techniques for temporal pattern detection include moving averages, exponential smoothing, and Autoregressive Integrated Moving Average (ARIMA) models.
- Real-World Applications: In finance, temporal pattern detection helps in stock price prediction and risk assessment. In healthcare, it can be used to track disease outbreaks over time, allowing for timely interventions.
- Challenges: Data quality and completeness are critical for accurate temporal analysis. Additionally, the complexity of models can lead to overfitting if not managed properly.
At Rapid Innovation, we leverage temporal pattern detection to empower our clients with predictive analytics capabilities. By identifying trends and anomalies, we help organizations make proactive decisions that enhance operational efficiency and drive profitability.
In conclusion, both multi-modal data analysis and temporal pattern detection are essential advanced features that empower organizations to derive deeper insights from their data. By leveraging these capabilities, businesses can make informed decisions, optimize operations, and enhance strategic planning. Rapid Innovation is committed to helping clients achieve these goals efficiently and effectively, ensuring a greater return on investment through tools in data analytics and ai for data analytics.
8.3. Population-specific Risk Stratification
Population-specific risk stratification is a critical component in healthcare that focuses on identifying and categorizing patients based on their risk levels for various health outcomes. This approach allows healthcare providers to tailor interventions and allocate resources more effectively.
- Identifying high-risk populations: By analyzing demographic data, medical history, and social determinants of health, healthcare organizations can pinpoint groups at higher risk for chronic diseases, hospitalizations, or adverse health events. Rapid Innovation leverages advanced AI algorithms to enhance this analysis, ensuring more accurate identification of at-risk populations. This is particularly relevant in the context of healthcare risk stratification.
- Targeted interventions: Once high-risk populations are identified, healthcare providers can implement targeted interventions, such as preventive care programs, chronic disease management, and lifestyle modification initiatives. Our AI-driven solutions enable the design of personalized intervention strategies that align with the specific needs of these populations, utilizing risk stratification tools in healthcare.
- Improved resource allocation: Risk stratification helps in optimizing the use of healthcare resources by directing them toward populations that need them the most, ultimately leading to better health outcomes and reduced healthcare costs. Rapid Innovation's data analytics tools facilitate efficient resource allocation, maximizing ROI for healthcare organizations, especially in the realm of population health risk stratification.
- Enhanced patient engagement: Understanding the specific risks faced by different populations allows for more personalized communication and education, fostering greater patient engagement in their own health management. Our AI solutions support the development of tailored communication strategies that resonate with diverse patient groups, contributing to effective population health stratification.
- Data-driven decision-making: Utilizing data analytics and predictive modeling, healthcare organizations can continuously refine their risk stratification processes, ensuring that interventions remain relevant and effective. Rapid Innovation provides the necessary tools to harness data effectively, driving informed decision-making, which is essential for successful stratification in healthcare. For more information on our AI-driven solutions in healthcare management, visit AI Healthcare Management.
8.4. Automated Reporting Systems
Automated reporting systems are essential tools in modern healthcare that streamline the collection, analysis, and dissemination of data. These systems enhance efficiency and accuracy in reporting, which is crucial for quality improvement and regulatory compliance.
- Real-time data access: Automated reporting systems provide healthcare professionals with immediate access to critical data, enabling timely decision-making and interventions. Rapid Innovation's AI solutions ensure that data is not only accessible but also actionable in real-time.
- Reduced administrative burden: By automating the reporting process, healthcare organizations can minimize the time and resources spent on manual data entry and report generation, allowing staff to focus on patient care. Our systems are designed to significantly reduce administrative overhead, enhancing operational efficiency.
- Enhanced accuracy: Automated systems reduce the likelihood of human error in data reporting, leading to more reliable information for clinical and operational decision-making. Rapid Innovation employs advanced AI techniques to ensure data integrity and accuracy in reporting.
- Compliance with regulations: These systems help healthcare organizations meet regulatory requirements by ensuring that reports are generated accurately and submitted on time. Our solutions are built with compliance in mind, helping clients navigate complex regulatory landscapes.
- Customizable reporting: Automated reporting systems can be tailored to meet the specific needs of different healthcare organizations, allowing for the generation of reports that are relevant to their unique goals and objectives. Rapid Innovation offers customizable reporting features that align with client-specific requirements.
8.5. Decision Support Integration
Decision support integration refers to the incorporation of clinical decision support systems (CDSS) into healthcare workflows. These systems provide healthcare professionals with evidence-based information and recommendations at the point of care, enhancing clinical decision-making.
- Improved clinical outcomes: By providing timely and relevant information, decision support systems can help clinicians make better-informed decisions, leading to improved patient outcomes. Rapid Innovation's AI-driven CDSS enhances the quality of care by delivering precise recommendations based on real-time data.
- Standardization of care: CDSS can promote adherence to clinical guidelines and best practices, ensuring that all patients receive consistent and high-quality care. Our solutions help standardize care protocols, reducing variability and improving overall patient safety.
- Enhanced efficiency: Integrating decision support into electronic health records (EHR) and other clinical systems streamlines workflows, allowing healthcare providers to access critical information without disrupting their patient interactions. Rapid Innovation's integration capabilities ensure seamless workflow enhancements.
- Reduced variability in care: Decision support systems can help minimize variations in clinical practice by guiding providers toward evidence-based treatment options, ultimately leading to more uniform care delivery. Our AI solutions are designed to promote best practices across diverse clinical settings.
- Continuous learning: Many decision support systems incorporate machine learning and artificial intelligence, allowing them to evolve and improve over time based on new evidence and clinical experiences. Rapid Innovation's commitment to continuous improvement ensures that our systems remain at the forefront of clinical decision support technology.
9. Validation and Performance Metrics
Validation and performance metrics are crucial in evaluating the effectiveness of a model, particularly in fields like machine learning, data science, and statistics. These metrics help in understanding how well a model performs and its reliability in making predictions, ultimately guiding businesses in achieving their goals efficiently and effectively.
9.1 Accuracy Assessment
Accuracy assessment is a fundamental metric used to evaluate the performance of a classification model. It measures the proportion of true results (both true positives and true negatives) among the total number of cases examined.
- Accuracy is calculated using the formula:
language="language-plaintext"``` -a1b2c3- Â Accuracy = (True Positives + True Negatives) / Total Cases
- A high accuracy percentage indicates that the model is performing well, but it is essential to consider the context of the data.
- Accuracy can be misleading, especially in imbalanced datasets where one class significantly outnumbers the other. For instance, if 95% of the data belongs to one class, a model that predicts only that class can still achieve 95% accuracy without being useful.
- It is important to complement accuracy with other metrics to get a comprehensive view of model performance. At Rapid Innovation, we emphasize the importance of a multi-faceted approach to model evaluation, ensuring that our clients can trust the insights derived from their AI solutions. This includes metrics such as precision, recall, and f1 score, which are essential components of model evaluation metrics.
9.2 Sensitivity and Specificity Analysis
Sensitivity and specificity are two critical metrics that provide deeper insights into the performance of a classification model, especially in binary classification tasks.
- Sensitivity, also known as recall or true positive rate, measures the proportion of actual positives that are correctly identified by the model. It is calculated as follows:
language="language-plaintext"``` -a1b2c3- Â Sensitivity = True Positives / (True Positives + False Negatives)
- A high sensitivity indicates that the model is effective at identifying positive cases, which is particularly important in medical diagnoses where missing a positive case can have serious consequences.
- Specificity, on the other hand, measures the proportion of actual negatives that are correctly identified. It is calculated as:
language="language-plaintext"``` -a1b2c3- Â Specificity = True Negatives / (True Negatives + False Positives)
- A high specificity indicates that the model is effective at identifying negative cases, which is crucial in scenarios where false positives can lead to unnecessary interventions or anxiety.
- Both sensitivity and specificity are essential for understanding the trade-offs in model performance. A model with high sensitivity may have lower specificity, and vice versa. The balance between these metrics can be adjusted based on the specific requirements of the application, such as prioritizing sensitivity in disease detection or specificity in fraud detection.
- Receiver Operating Characteristic (ROC) curves and Area Under the Curve (AUC) are often used to visualize and summarize the performance of a model across different thresholds, providing a comprehensive view of both sensitivity and specificity. At Rapid Innovation, we leverage these advanced metrics to fine-tune our models, ensuring that our clients achieve greater ROI through precise and reliable AI solutions. Additionally, confusion matrix precision and recall are also utilized to provide a clearer picture of model performance.
In conclusion, validation and performance metrics like accuracy, sensitivity, specificity, and confusion matrix precision and recall are vital for assessing the effectiveness of predictive models. They help in making informed decisions about model selection and deployment, ensuring that the chosen model meets the specific needs of the application. By partnering with Rapid Innovation, clients can harness the power of these metrics, including machine learning performance metrics and regression evaluation metrics, to drive their business objectives forward with confidence.
9.3. Time-to-Detection Metrics
Time-to-detection metrics are crucial in evaluating the efficiency of security systems, particularly in cybersecurity and physical security domains. These metrics measure the time taken from the occurrence of a security incident to its detection by the monitoring system.
- Importance of Time-to-Detection: Â
- Faster detection can significantly reduce the impact of a security breach.
- It allows for quicker response times, minimizing potential damage.
- Organizations can better allocate resources to areas needing improvement.
- Key Components: Â
- Detection Time: The total time taken from the moment an incident occurs until it is detected.
- Response Time: The time taken from detection to the initiation of a response.
- Overall Time-to-Detection: A combination of detection and response times, providing a comprehensive view of system efficiency.
- Best Practices for Improvement: Â
- Implement automated monitoring tools to enhance detection speed, such as those found in the oracle enterprise performance management system security configuration guide.
- Regularly review and update detection algorithms to adapt to new threats.
- Conduct drills and simulations to test and improve response times, similar to how snort is capable of real-time traffic analysis.
9.4. False Signal Rate Management
False signal rate management is essential for maintaining the integrity and reliability of security systems. A high false signal rate can lead to alarm fatigue, where security personnel become desensitized to alerts, potentially overlooking genuine threats.
- Understanding False Signals: Â
- False positives occur when a security system incorrectly identifies a benign event as a threat.
- False negatives happen when a genuine threat goes undetected.
- Impacts of High False Signal Rates: Â
- Increased operational costs due to unnecessary investigations.
- Decreased trust in the security system, leading to potential oversight of real threats.
- Strain on resources as personnel are diverted to handle false alarms.
- Strategies for Management: Â
- Fine-tune detection algorithms to reduce false positives.
- Implement machine learning techniques to improve threat identification.
- Regularly analyze and adjust thresholds for alerts based on historical data.
9.5. System Performance Monitoring
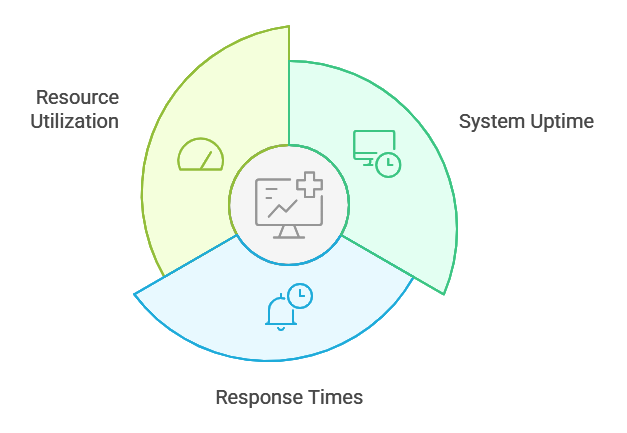
‍
System performance monitoring is vital for ensuring that security systems operate effectively and efficiently. Continuous monitoring helps identify potential issues before they escalate into significant problems.
- Key Aspects of Performance Monitoring: Â
- System Uptime: Tracking the operational status of security systems to ensure they are always functional.
- Response Times: Measuring how quickly the system reacts to detected threats.
- Resource Utilization: Assessing how effectively system resources are being used, which is critical for security system performance.
- Benefits of Effective Monitoring: Â
- Early detection of system failures or inefficiencies.
- Improved decision-making based on real-time data.
- Enhanced overall security posture through proactive management.
- Tools and Techniques: Â
- Utilize performance monitoring software to track key metrics.
- Set up alerts for unusual patterns or performance dips.
- Regularly review performance reports to identify trends and areas for improvement.
At Rapid Innovation, we leverage advanced AI technologies to enhance these metrics, ensuring that our clients achieve greater ROI through improved security system efficiency and effectiveness. By implementing tailored solutions, we help organizations minimize risks and optimize their security operations.
10. Regulatory Compliance and Ethics
Regulatory compliance and ethics are critical components for businesses operating in today's complex legal landscape. Companies must navigate a myriad of laws and regulations that govern their operations, particularly concerning regulatory compliance and ethics, data privacy and reporting standards. Adhering to these regulations not only helps avoid legal penalties but also builds trust with customers and stakeholders.
10.1 Data Privacy Requirements
Data privacy requirements are designed to protect individuals' personal information from misuse and unauthorized access. With the rise of digital data collection, businesses must be vigilant in ensuring compliance with various data protection laws.
- General Data Protection Regulation (GDPR): This regulation applies to organizations operating within the European Union (EU) and those outside the EU that handle the personal data of EU citizens. Key principles include: Â
- Consent: Organizations must obtain explicit consent from individuals before collecting their data.
- Right to Access: Individuals have the right to request access to their personal data.
- Data Minimization: Only necessary data should be collected and processed.
- California Consumer Privacy Act (CCPA): This law enhances privacy rights for California residents. It includes provisions such as: Â
- Right to Know: Consumers can request information about the personal data collected about them.
- Right to Delete: Consumers can request the deletion of their personal data.
- Opt-Out: Consumers can opt out of the sale of their personal data.
- Health Insurance Portability and Accountability Act (HIPAA): For healthcare organizations, HIPAA sets standards for protecting sensitive patient information. Key requirements include: Â
- Privacy Rule: Establishes national standards for the protection of health information.
- Security Rule: Sets standards for safeguarding electronic health information.
Organizations must implement robust data protection measures, including encryption, access controls, and regular audits, to comply with these regulations. Rapid Innovation can assist businesses in developing AI-driven solutions that automate compliance processes, ensuring that data protection measures are consistently applied and monitored. This not only mitigates risks but also enhances operational efficiency, ultimately leading to greater ROI.
10.2 Regulatory Reporting Standards
Regulatory reporting standards are essential for ensuring transparency and accountability in business operations. These standards require organizations to report specific information to regulatory bodies, which helps maintain market integrity and protect stakeholders.
- Financial Reporting Standards: Companies must adhere to Generally Accepted Accounting Principles (GAAP) or International Financial Reporting Standards (IFRS) depending on their jurisdiction. Key aspects include: Â
- Accurate Financial Statements: Organizations must prepare and present financial statements that reflect their true financial position.
- Timely Reporting: Companies are required to submit their financial reports within specified deadlines to ensure timely access to information.
- Environmental Reporting: Many jurisdictions require companies to disclose their environmental impact. This includes: Â
- Sustainability Reporting: Organizations may need to report on their sustainability practices and environmental performance.
- Compliance with Environmental Regulations: Companies must demonstrate adherence to laws governing emissions, waste management, and resource usage.
- Industry-Specific Regulations: Different industries have unique reporting requirements. For example: Â
- Financial Services: Banks and financial institutions must comply with regulations such as the Dodd-Frank Act, which mandates reporting on risk management and capital adequacy.
- Pharmaceuticals: Companies in the pharmaceutical sector must report on clinical trial results and adverse drug reactions to regulatory bodies like the FDA.
To ensure compliance with regulatory reporting standards, organizations should establish internal controls, conduct regular training for employees, and utilize technology for accurate data collection and reporting. Rapid Innovation can provide tailored AI solutions that streamline the reporting process, reducing the likelihood of errors and ensuring compliance with regulatory requirements. This proactive approach not only safeguards against potential penalties but also enhances the overall credibility of the organization, contributing to a stronger market position and improved ROI. Non-compliance can lead to legal repercussions, financial penalties, and loss of business credibility.
10.3. Ethical Considerations
Ethical considerations in AI research are paramount in any research or data management process, particularly in the realm of AI development. They ensure that the rights and dignity of individuals are respected and that the integrity of the research is maintained. Key aspects include:
- Informed Consent: Participants should be fully informed about the nature of the research, its purpose, and any potential risks involved. They must voluntarily agree to participate without any coercion, which is crucial when developing AI systems that rely on user data.
- Confidentiality: Researchers must protect the privacy of participants by ensuring that personal data is kept confidential. This includes using anonymization techniques and secure data storage methods, which are essential in AI projects to maintain user trust.
- Data Integrity: Maintaining the accuracy and reliability of data is crucial. Researchers should avoid fabrication, falsification, or plagiarism in their work, as these practices can severely undermine the effectiveness of AI models.
- Ethical Review: Many institutions require an ethical review process before research can commence. This process evaluates the potential risks and benefits of the research and ensures compliance with ethical standards, particularly in AI applications that may impact society.
- Vulnerable Populations: Special care must be taken when working with vulnerable groups, such as children or individuals with disabilities. Additional safeguards should be implemented to protect their rights, especially when AI systems are designed to interact with these populations. For more detailed guidance, refer to the ethical AI development guide.
10.4. Audit Trail Management
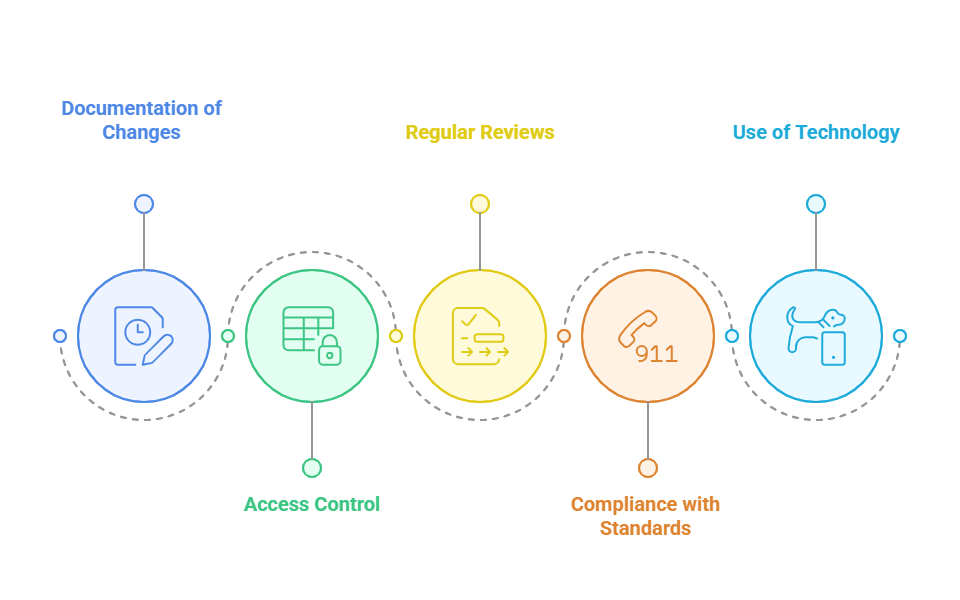
‍
Audit trail management is essential for ensuring transparency and accountability in research and data management, especially in AI development. An audit trail provides a chronological record of all activities related to data handling, which can be crucial for verifying the integrity of the research process. Key components include:
- Documentation of Changes: Every modification made to the data or research process should be documented. This includes who made the change, when it was made, and the reason for the change, which is vital for maintaining the reliability of AI algorithms.
- Access Control: Limiting access to sensitive data is vital. Only authorized personnel should be able to make changes or access certain information, and this should be logged to prevent unauthorized manipulation of AI training data.
- Regular Reviews: Conducting regular audits of the data management process helps identify any discrepancies or unauthorized changes. This can be done through internal audits or third-party evaluations, ensuring that AI systems are developed responsibly.
- Compliance with Standards: Adhering to industry standards and regulations, such as GDPR or HIPAA, is crucial for audit trail management. This ensures that the audit trail meets legal and ethical requirements, particularly in AI applications that handle personal data.
- Use of Technology: Implementing software solutions that automatically track changes and maintain logs can streamline the audit trail process. This reduces the risk of human error and enhances data security, which is critical in AI development.
10.5. Documentation Requirements
Proper documentation is a cornerstone of effective research and data management, particularly in AI projects. It ensures that all aspects of the research process are recorded and can be referenced in the future. Key documentation requirements include:
- Research Protocol: A detailed plan outlining the objectives, methodology, and procedures of the research should be created. This serves as a roadmap for the research process, guiding AI development efforts.
- Data Management Plan: This document outlines how data will be collected, stored, and shared. It should include details on data security measures and compliance with relevant regulations, ensuring that AI systems are built on a solid foundation.
- Participant Information Sheets: These documents provide potential participants with information about the study, including its purpose, procedures, and any risks involved. They should be clear and accessible, fostering transparency in AI research.
- Consent Forms: Written consent from participants is essential. These forms should clearly state that participants understand their rights and the nature of their involvement in the research, which is particularly important in AI applications that utilize personal data.
- Final Reports: At the conclusion of the research, a comprehensive report should be produced. This should include findings, methodologies, and any limitations of the study, providing valuable insights for future AI projects.
- Archiving Procedures: Documentation should include guidelines for the long-term storage of data and research materials. This ensures that they are accessible for future reference or audits, which is crucial for maintaining the integrity of AI systems.
By adhering to these ethical considerations in AI research, audit trail management practices, and documentation requirements, researchers can enhance the credibility and reliability of their work while protecting the rights of participants, ultimately leading to more effective and responsible AI solutions.
11. Challenges and Solutions
In any data-driven environment, challenges are inevitable. Understanding these challenges and implementing effective solutions is crucial for success. Two significant challenges in data analysis are data quality issues and the signal-to-noise ratio.
11.1 Data Quality Issues
Data quality issues can severely impact the reliability and accuracy of analysis. Poor data quality can lead to incorrect conclusions, wasted resources, and misguided strategies. Common data quality issues include inaccurate data, incomplete data, inconsistent data, and duplicate data.
- Inaccurate Data: Data may be incorrect due to human error, outdated information, or faulty data entry processes.
- Incomplete Data: Missing values can skew results and lead to incomplete analyses.
- Inconsistent Data: Variations in data formats or definitions can create confusion and hinder data integration.
- Duplicate Data: Redundant entries can inflate datasets and lead to misleading insights.
To address these data quality issues, organizations can implement several solutions:
- Data Validation: Establishing validation rules during data entry can help catch errors early.
- Regular Audits: Conducting periodic data audits can identify inaccuracies and inconsistencies.
- Data Cleansing: Implementing data cleansing processes can help remove duplicates and fill in missing values.
- Standardization: Creating standardized data formats and definitions can improve consistency across datasets.
Organizations can also leverage data quality tools from the Gartner Magic Quadrant to enhance their data quality management software. By focusing on these solutions, organizations can enhance the quality of their data, leading to more reliable analyses and better decision-making. At Rapid Innovation, we leverage advanced AI tools to automate data validation and cleansing processes, ensuring that our clients have access to high-quality data that drives effective decision-making and maximizes ROI. For more insights on the importance of data quality in AI implementations.
11.2 Signal-to-Noise Ratio
The signal-to-noise ratio (SNR) is a critical concept in data analysis, referring to the relationship between meaningful data (signal) and irrelevant or misleading data (noise). A low SNR can obscure valuable insights and lead to poor decision-making. Factors that contribute to a low signal-to-noise ratio include excessive data volume, data redundancy, and external influences.
- Excessive Data Volume: Large datasets can contain a significant amount of irrelevant information, making it difficult to extract meaningful insights.
- Data Redundancy: Repeated or similar data points can create noise, complicating analysis.
- External Influences: External factors, such as market fluctuations or seasonal trends, can introduce noise into the data.
To improve the signal-to-noise ratio, organizations can adopt the following strategies:
- Data Filtering: Implementing filtering techniques can help isolate relevant data from noise.
- Feature Selection: Identifying and selecting the most relevant features for analysis can enhance the SNR.
- Statistical Techniques: Utilizing statistical methods, such as regression analysis or machine learning algorithms, can help differentiate between signal and noise.
- Data Visualization: Employing data visualization tools can aid in identifying patterns and trends, making it easier to focus on the signal.
Organizations can also explore data quality solutions and platforms, such as Talend Data Quality and Ataccama Data Governance, to improve their data management processes. By enhancing the signal-to-noise ratio, organizations can improve the clarity of their analyses, leading to more informed decisions and better outcomes. Rapid Innovation employs cutting-edge machine learning algorithms and data visualization techniques to help our clients filter out noise and focus on actionable insights, ultimately driving greater ROI.
11.3. Integration Challenges
Integration challenges are common in various sectors, especially when merging new technologies or systems with existing ones. These challenges, including data integration challenges and challenges of data integration, can hinder operational efficiency and lead to increased costs if not addressed properly.
- Compatibility Issues: Different systems may not communicate effectively, leading to data silos. This can result in inconsistent information across platforms, which can be mitigated through Rapid Innovation's expertise in developing custom APIs that ensure seamless data flow and integration.
- Data Migration: Transferring data from one system to another can be complex. Ensuring data integrity and accuracy during migration is crucial to avoid operational disruptions. Rapid Innovation employs advanced data migration strategies and tools to facilitate smooth transitions, minimizing downtime and ensuring data fidelity. This is particularly important in addressing data integration issues and data integration problems.
- User Adoption: Employees may resist using new systems due to unfamiliarity or perceived complexity, which can slow down the integration process and reduce overall productivity. Our tailored training programs at Rapid Innovation focus on user engagement, helping employees feel confident and competent in using new technologies. This is especially relevant in overcoming challenges faced in Salesforce development and Salesforce integration challenges.
- Technical Limitations: Legacy systems may not support new integrations, requiring significant upgrades or replacements, which can be costly and time-consuming. Rapid Innovation assists clients in assessing their current infrastructure and provides strategic recommendations for upgrades or replacements that align with their business goals. This is crucial in addressing integration challenges and challenges of system integration.
- Security Concerns: Integrating new technologies can expose organizations to security vulnerabilities. Ensuring robust security measures during integration is essential to protect sensitive data. Rapid Innovation prioritizes security by implementing best practices and compliance standards throughout the integration process, particularly in the context of cloud integration challenges and SaaS integration challenges. For more information on integration services, visit our ChatGPT integration services.
11.4. Resource Requirements

‍
Resource requirements are critical for the successful implementation of any project or system. Understanding these needs helps organizations allocate the right amount of time, personnel, and budget.
- Human Resources: Skilled personnel are essential for managing and executing projects. This includes project managers, IT specialists, and support staff who can facilitate smooth operations. Rapid Innovation provides access to a pool of experienced professionals who can be integrated into your team as needed, including data integration specialists.
- Financial Resources: Budgeting for new systems or technologies is vital. Organizations must consider costs for software, hardware, training, and ongoing maintenance. Rapid Innovation offers cost-effective solutions that maximize ROI, ensuring that clients receive value for their investment.
- Time Allocation: Adequate time must be set aside for planning, implementation, and testing. Rushed projects often lead to errors and inefficiencies. Our project management methodologies at Rapid Innovation emphasize thorough planning and execution timelines to ensure successful outcomes.
- Training and Development: Investing in training programs ensures that employees are equipped to use new systems effectively, enhancing productivity and reducing resistance to change. Rapid Innovation designs customized training sessions that cater to the specific needs of your workforce, addressing challenges in application integration and challenges in data integration.
- Infrastructure: Organizations may need to upgrade their existing infrastructure to support new technologies, including hardware, software, and network capabilities. Rapid Innovation conducts comprehensive assessments to identify infrastructure gaps and provides tailored solutions to enhance operational capabilities, particularly in the context of system integration problems.
11.5. Change Management
Change management is a structured approach to transitioning individuals, teams, and organizations from a current state to a desired future state. Effective change management is crucial for minimizing resistance and ensuring successful implementation.
- Communication: Clear and consistent communication is vital. Stakeholders should be informed about the reasons for change, the benefits, and the expected outcomes. Rapid Innovation emphasizes transparent communication strategies to keep all parties informed and engaged throughout the process.
- Stakeholder Engagement: Involving key stakeholders early in the process can foster buy-in and support, including gathering feedback and addressing concerns. Our collaborative approach at Rapid Innovation ensures that stakeholder input is valued and integrated into the change process, particularly in addressing challenges faced in Mulesoft.
- Training and Support: Providing adequate training and resources helps employees adapt to changes. Ongoing support can alleviate anxiety and build confidence in using new systems. Rapid Innovation offers continuous support and resources to ensure a smooth transition for all users, especially in overcoming Salesforce data migration challenges.
- Monitoring and Evaluation: Regularly assessing the impact of changes allows organizations to make necessary adjustments, helping to identify areas for improvement and ensuring that objectives are met. Rapid Innovation implements monitoring frameworks that provide insights into the effectiveness of changes and guide future decisions.
- Cultural Considerations: Understanding the organizational culture is essential for successful change management. Tailoring approaches to fit the culture can enhance acceptance and reduce resistance. Rapid Innovation works closely with clients to align change initiatives with their unique organizational culture, fostering a supportive environment for transformation, particularly in the context of business integration challenges and challenges of integration.
12. Future Developments
12.1. Emerging Technologies
The landscape of healthcare is rapidly evolving, driven by emerging technologies that promise to enhance patient care, streamline operations, and improve outcomes. These technologies are reshaping how healthcare providers deliver services and how patients engage with their health.
- Artificial Intelligence (AI) and Machine Learning: At Rapid Innovation, we leverage AI to analyze vast amounts of healthcare data, enabling predictive analytics that can forecast patient outcomes and optimize treatment plans. Our machine learning algorithms identify patterns in patient data, leading to more personalized medicine and ultimately greater ROI for healthcare providers. The integration of artificial intelligence in healthcare is becoming increasingly vital, especially in the context of electronic health record (EHR) systems.
- Telemedicine: The rise of telehealth platforms has transformed patient-provider interactions. Rapid Innovation assists clients in developing robust telemedicine solutions, allowing patients to consult with healthcare professionals remotely. This not only improves access to care, especially in rural areas, but also enhances operational efficiency, particularly highlighted during the COVID-19 pandemic. The use of digital health records is essential in facilitating these remote consultations.
- Wearable Health Devices: We support the integration of wearable health devices, such as smartwatches and fitness trackers, into healthcare systems. These devices monitor health metrics in real-time, providing valuable data for both patients and healthcare providers, which can lead to improved patient engagement and outcomes. The data collected can be seamlessly integrated into electronic health record (EHR) software for better tracking and analysis.
- Blockchain Technology: Rapid Innovation harnesses blockchain technology to offer secure methods for storing and sharing patient data. This enhances data integrity and security, ensuring that patient information is accessible only to authorized individuals, thereby building trust and compliance in healthcare systems. The application of blockchain in health information technology is a promising area for future developments.
- Robotics: Our expertise extends to the development of robotic-assisted surgical solutions, which are becoming more common. These technologies allow for greater precision and reduced recovery times, ultimately leading to improved patient outcomes and operational efficiencies. The integration of robotics in healthcare is part of a broader trend towards advanced medical technologies.
12.2. Integration of Genomic Data
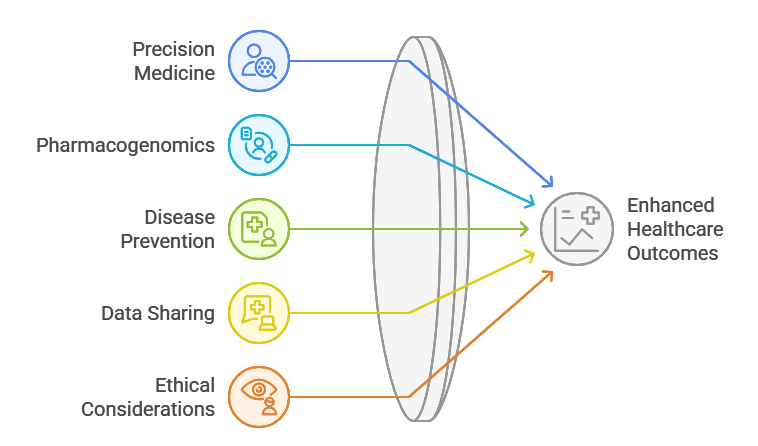
‍
The integration of genomic data into healthcare is a significant advancement that holds the potential to revolutionize personalized medicine. By understanding an individual's genetic makeup, healthcare providers can tailor treatments to achieve better outcomes.
- Precision Medicine: Rapid Innovation facilitates the use of genomic data for precision medicine, where treatments are customized based on a patient's genetic profile. This approach can lead to more effective therapies with fewer side effects, particularly in fields like oncology, enhancing the overall effectiveness of treatment protocols. The role of health information technology in managing genomic data is crucial for its successful implementation.
- Pharmacogenomics: Our solutions incorporate pharmacogenomic data, which studies how genes affect a person's response to drugs. By integrating this data, healthcare providers can prescribe medications that are more likely to be effective for individual patients, minimizing trial and error and improving patient satisfaction. This integration is often supported by advanced electronic health record (EHR) systems.
- Disease Prevention: We help clients utilize genomic data to identify individuals at risk for certain diseases, enabling proactive measures. For example, genetic testing can reveal predispositions to conditions like breast cancer or heart disease, allowing for early intervention and better resource allocation. The use of health information and technology is essential in tracking and managing these risks.
- Data Sharing and Collaboration: The integration of genomic data requires collaboration among various stakeholders, including researchers, healthcare providers, and patients. Rapid Innovation promotes initiatives that enhance data sharing, accelerating discoveries and improving treatment protocols. The collaboration is often facilitated through health information technology platforms.
- Ethical Considerations: As genomic data becomes more integrated into healthcare, we emphasize the importance of ethical considerations surrounding privacy and consent. Ensuring that patients understand how their data will be used is crucial for maintaining trust in the healthcare system, and we guide our clients in establishing transparent practices. The ethical management of health information technology is a key focus area as we move forward.
12.3. Advanced Predictive Capabilities
Advanced predictive capabilities refer to the use of sophisticated algorithms and data analytics to forecast future events or trends. These capabilities are increasingly being integrated into various sectors, including healthcare, finance, and marketing.
- Predictive analytics utilizes historical data to identify patterns and make informed predictions.
- Machine learning models enhance predictive accuracy by continuously learning from new data inputs.
- Businesses can anticipate customer behavior, optimize inventory, and improve decision-making processes.
- In healthcare, predictive models can forecast disease outbreaks, patient admissions, and treatment outcomes.
- Financial institutions use predictive analytics to assess credit risk and detect fraudulent activities.
At Rapid Innovation, we specialize in implementing advanced predictive capabilities tailored to your business needs. By leveraging our expertise in predictive analytics, predictive data analytics, and machine learning, we help organizations achieve greater ROI through proactive decision-making and enhanced operational efficiency. The integration of these tools, including power bi predictive analytics and predictive analytics in power bi, can lead to significant cost savings and improved efficiency, allowing you to stay ahead of the competition with data-driven insights. For custom solutions, consider our custom AI model development.
12.4. Global Surveillance Networks
Global surveillance networks are systems designed to monitor and collect data on a worldwide scale. These networks play a crucial role in various fields, including security, public health, and environmental monitoring.
- Surveillance networks utilize a combination of satellite imagery, drones, and ground-based sensors to gather real-time data.
- They are essential for tracking global events, such as natural disasters, disease outbreaks, and geopolitical tensions.
- In public health, global surveillance helps identify and respond to emerging infectious diseases, enhancing global health security.
- Environmental monitoring networks track climate change, pollution levels, and biodiversity, providing critical data for policy-making.
- Collaboration among countries and organizations enhances the effectiveness of these networks, allowing for a more comprehensive understanding of global issues.
At Rapid Innovation, we understand the importance of global surveillance networks in making informed decisions. Our solutions integrate cutting-edge technology and data-sharing capabilities, enabling organizations to respond swiftly to global challenges and enhance their operational effectiveness.
12.5. Personalized Monitoring Systems
Personalized monitoring systems are tailored solutions designed to track individual behaviors, health metrics, or preferences. These systems leverage technology to provide customized insights and recommendations.
- Wearable devices, such as fitness trackers and smartwatches, collect data on physical activity, heart rate, and sleep patterns.
- In healthcare, personalized monitoring systems enable remote patient monitoring, allowing healthcare providers to track patients' health in real-time.
- These systems can alert users to potential health issues, encouraging proactive management of chronic conditions.
- Personalized recommendations based on collected data can enhance user engagement and improve overall well-being.
- Businesses use personalized monitoring to understand customer preferences, leading to targeted marketing strategies and improved customer satisfaction.
Rapid Innovation is at the forefront of developing personalized monitoring systems that cater to the unique needs of individuals and organizations. By harnessing data analytics and technology, we help businesses create tailored experiences that foster better health outcomes and enhance user satisfaction across various sectors.
‍