Table Of Contents
Category
Healthcare & Medicine
Supply Chain & Logistics
IoT
Artificial Intelligence
Blockchain
1. Introduction to AI-Driven Resource Allocation
AI-driven resource allocation refers to the use of artificial intelligence technologies to optimize the distribution of resources across various sectors. This approach leverages advanced algorithms and machine learning techniques to analyze data, predict outcomes, and make informed decisions. The goal is to enhance efficiency, reduce waste, and improve overall performance in resource management.
- AI can process vast amounts of data quickly, identifying patterns and trends that human analysts might miss, thereby enabling organizations to make data-driven decisions.
- Machine learning models can adapt to changing conditions, ensuring that resource allocation remains optimal over time and aligns with evolving business needs.
- AI-driven systems can operate in real-time, allowing for immediate adjustments based on current needs and demands, which is crucial for maintaining competitive advantage.
The significance of AI in resource allocation is evident across multiple industries, including:
- Healthcare: AI optimizes the allocation of medical staff, equipment, and supplies, improving patient care and operational efficiency. For instance, Rapid Innovation has helped healthcare providers implement AI solutions that streamline scheduling and resource distribution, resulting in enhanced patient outcomes and reduced operational costs.
- Supply Chain Management: AI enhances inventory management and logistics, ensuring that resources are available where and when they are needed. By partnering with Rapid Innovation, clients have experienced improved supply chain visibility and reduced lead times, leading to greater ROI.
- Energy Management: AI systems can predict energy demand and optimize the distribution of resources, leading to cost savings and reduced environmental impact. Rapid Innovation has assisted energy companies in deploying AI-driven analytics to forecast demand accurately, enabling them to allocate resources more efficiently.
As organizations increasingly adopt AI technologies, the potential for AI resource allocation strategies in resource allocation continues to grow. This shift not only improves operational efficiency but also contributes to sustainability efforts by minimizing resource waste. At Rapid Innovation, we are committed to helping our clients harness the power of AI to achieve their business goals effectively and efficiently, ultimately driving greater ROI.
Refer to the image for a visual representation of AI-driven resource allocation concepts:

1.1. Defining Resource Allocation
Resource allocation refers to the process of distributing available resources among various projects, departments, or tasks within an organization. This process is crucial for optimizing performance and achieving strategic goals. Resources can include financial assets, human capital, technology, and time. Effective resource allocation ensures that the right resources are assigned to the right tasks at the right time. It involves decision-making that balances competing demands and prioritizes initiatives based on their potential return on investment (ROI). Poor resource allocation can lead to wasted resources, missed opportunities, and decreased productivity. In essence, resource allocation is about making informed choices to maximize efficiency and effectiveness within an organization. At Rapid Innovation, we leverage our expertise in AI and Blockchain to help clients streamline their resource allocation processes, ensuring that investments yield the highest possible returns. This includes implementing resource allocation strategies that align with their overall strategy and resource allocation goals.
1.2. Evolution of Allocation Strategies
The strategies for resource allocation have evolved significantly over the years, influenced by changes in technology, market dynamics, and organizational structures. Traditional methods often relied on historical data and intuition, focusing on fixed budgets and linear planning. The rise of project management methodologies, such as Agile and Lean, introduced more flexible and iterative approaches to resource allocation. Modern allocation strategies now incorporate data analytics and performance metrics to inform decisions, allowing for real-time adjustments. The shift towards digital transformation has also led to the adoption of cloud-based tools that facilitate collaborative resource management across teams.
As organizations continue to adapt to a rapidly changing environment, the evolution of allocation strategies will likely focus on:
- Increased automation to reduce manual processes.
- Enhanced predictive analytics to forecast resource needs.
- Greater emphasis on sustainability and ethical considerations in resource distribution.
At Rapid Innovation, we utilize cutting-edge AI technologies to enhance predictive analytics, enabling our clients to make data-driven decisions that align with their strategic objectives, including capital allocation strategy and resource allocation in strategic management.
1.3. The Role of Artificial Intelligence
Artificial Intelligence (AI) is transforming resource allocation by providing advanced tools and techniques that enhance decision-making processes. AI algorithms can analyze vast amounts of data to identify patterns and trends, enabling organizations to allocate resources more effectively. Machine learning models can predict future resource needs based on historical data, helping organizations to proactively manage their assets. AI-driven tools can optimize scheduling and task assignments, ensuring that human resources are utilized efficiently. By automating routine allocation tasks, AI frees up managers to focus on strategic planning and innovation.
The integration of AI in resource allocation offers several benefits:
- Improved accuracy in forecasting and planning.
- Enhanced agility in responding to changing market conditions.
- Increased efficiency through automation, reducing the time spent on manual allocation processes.
As AI technology continues to advance, its role in resource allocation will likely expand, leading to more sophisticated and responsive allocation strategies, including resource allocation strategies for innovation portfolio management. Rapid Innovation is at the forefront of this transformation, helping clients harness the power of AI to achieve greater ROI and operational excellence, while recognizing resource allocation as a vital part of strategy.
Refer to the image for a visual representation of resource allocation concepts:
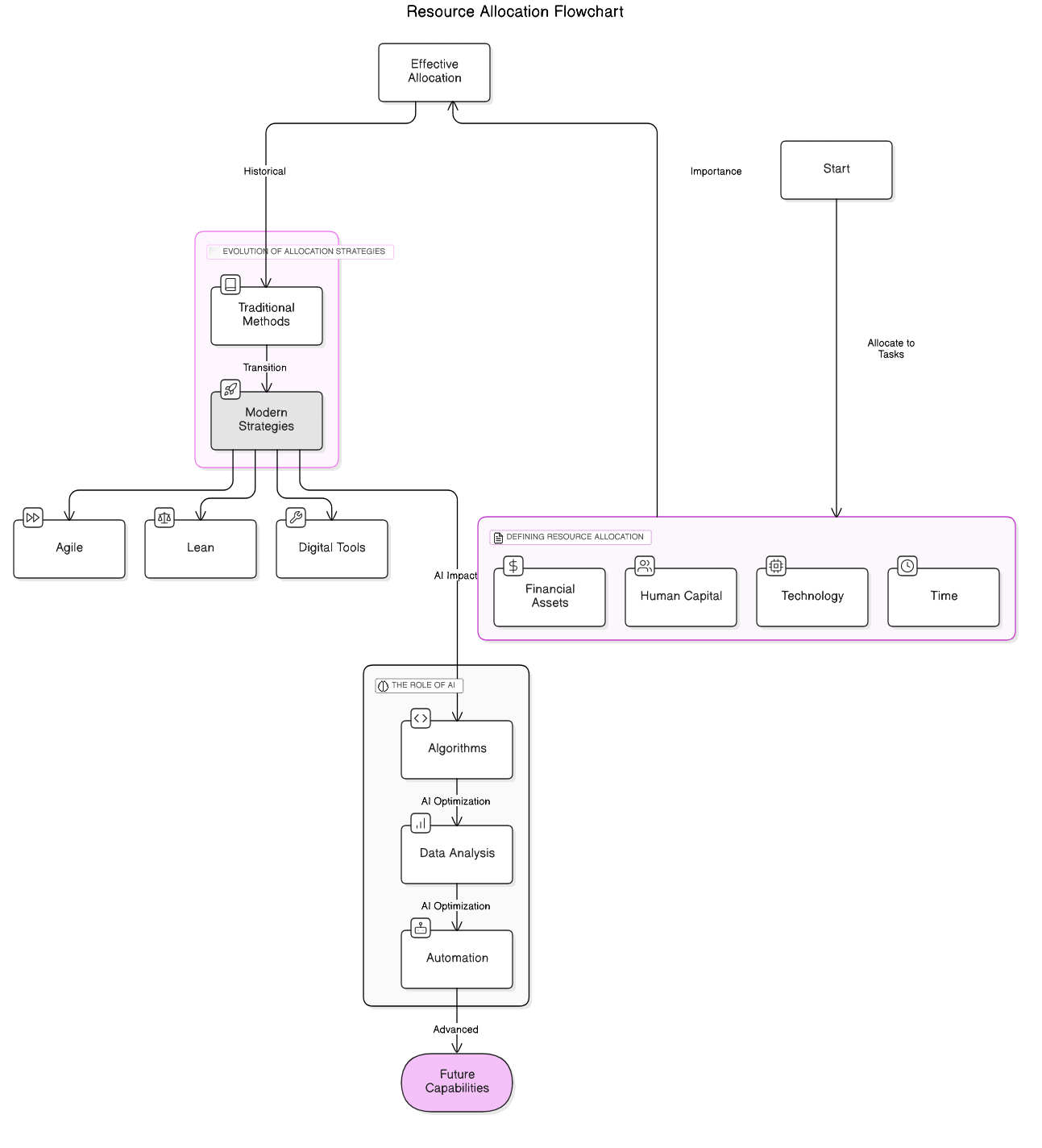
1.4. Paradigm Shift in Resource Management
The landscape of resource management is undergoing a significant transformation, driven by technological advancements and changing societal needs. This paradigm shift is characterized by a move from traditional, linear resource management approaches to more dynamic, integrated systems.
- Emphasis on Sustainability: Modern resource management prioritizes sustainability, focusing on long-term ecological balance rather than short-term gains. This shift encourages the use of renewable resources and minimizes waste, aligning with Rapid Innovation's commitment to sustainable development through AI and Blockchain solutions.
- Data-Driven Decision Making: The integration of big data analytics allows organizations to make informed decisions based on real-time data, leading to more efficient resource allocation and utilization. Rapid Innovation leverages AI to analyze data patterns, enabling clients to optimize their resource management strategies effectively, including the use of human resource computer programs and hr computer software.
- Collaborative Approaches: Stakeholders, including governments, businesses, and communities, are increasingly collaborating to manage resources. This collective effort fosters shared responsibility and enhances resource efficiency. Rapid Innovation facilitates these collaborations through blockchain technology, ensuring transparency and trust among all parties involved, which is essential in human capital management technology and human capital management tech.
- Technological Integration: The use of advanced technologies such as IoT (Internet of Things) and AI (Artificial Intelligence) enables better monitoring and management of resources. These technologies provide insights that help optimize resource use, a core focus of Rapid Innovation's development services, including technology in human resource and hr cloud based systems.
- Circular Economy: The shift towards a circular economy promotes the reuse and recycling of materials, reducing the demand for new resources. This approach minimizes environmental impact and fosters economic resilience, which Rapid Innovation supports through innovative solutions that enhance resource efficiency, including talent management technology and technology and talent management.
1.5. Key Challenges in Traditional Allocation Methods

‍
Traditional resource allocation methods face several challenges that hinder their effectiveness in today’s complex environment. These challenges can lead to inefficiencies and unsustainable practices.
- Inefficiency: Traditional methods often rely on outdated data and static models, resulting in inefficient resource distribution. This can lead to overuse in some areas and scarcity in others. Rapid Innovation addresses this issue by implementing AI-driven analytics to provide real-time insights, which can be enhanced by types of hr information systems.
- Lack of Flexibility: Conventional allocation methods are typically rigid, making it difficult to adapt to changing conditions or unexpected events. This inflexibility can exacerbate resource shortages during crises. Rapid Innovation's solutions are designed to be agile, allowing organizations to pivot quickly in response to new challenges, including technology and hrm.
- Inequitable Distribution: Traditional approaches may not consider the needs of all stakeholders, leading to unequal access to resources. This can create social tensions and exacerbate inequalities. By utilizing blockchain technology, Rapid Innovation ensures equitable resource distribution through transparent tracking and accountability, which is crucial in human resource management technology and hris open source.
- Environmental Impact: Many traditional methods do not adequately account for environmental sustainability, leading to resource depletion and ecological damage. This oversight can have long-term consequences for both the environment and society. Rapid Innovation emphasizes sustainable practices in all its projects, helping clients minimize their ecological footprint.
- Resistance to Change: Organizations often face resistance when attempting to implement new resource management strategies. This resistance can stem from a lack of understanding or fear of the unknown. Rapid Innovation provides consulting and training to ease this transition, ensuring that stakeholders are well-informed and engaged, particularly in the context of artificial intelligence in human resource management and artificial intelligence in human resources management.
2. Foundational Technologies
The evolution of resource management is heavily influenced by foundational technologies that enhance efficiency, transparency, and sustainability. These technologies are critical in addressing the challenges faced by traditional methods.
- Internet of Things (IoT): IoT devices enable real-time monitoring of resources, providing valuable data for decision-making. This technology allows for better tracking of resource usage and helps identify areas for improvement, a service Rapid Innovation excels in.
- Artificial Intelligence (AI): AI algorithms can analyze vast amounts of data to predict resource needs and optimize allocation. This predictive capability enhances efficiency and reduces waste, showcasing Rapid Innovation's expertise in AI development, including applications in hrm technology.
- Blockchain Technology: Blockchain offers a transparent and secure way to track resource transactions. This technology can improve accountability and trust among stakeholders, ensuring fair resource distribution, which is a key offering of Rapid Innovation's blockchain solutions.
- Geographic Information Systems (GIS): GIS technology allows for spatial analysis of resource distribution, helping organizations visualize and manage resources more effectively. This can lead to better planning and allocation strategies, supported by Rapid Innovation's technological integration.
- Cloud Computing: Cloud platforms facilitate data sharing and collaboration among stakeholders, enabling more integrated resource management. This technology supports scalability and flexibility in resource allocation, aligning with Rapid Innovation's commitment to providing scalable solutions, including open source human resource management system using php and mysql.
By leveraging these foundational technologies, organizations can overcome the limitations of traditional resource management methods and embrace a more sustainable and efficient approach, with Rapid Innovation guiding them every step of the way.
Refer to the image for a visual representation of the paradigm shift in resource management and its foundational technologies.

2.1. Machine Learning Fundamentals
Machine learning (ML) is a subset of artificial intelligence (AI) that focuses on the development of algorithms that allow computers to learn from and make predictions based on data. At Rapid Innovation, we leverage these fundamental concepts of machine learning to help our clients achieve their business goals efficiently and effectively.
- Types of Learning: Â
- Supervised Learning: Involves training a model on labeled data, where the outcome is known. Common algorithms include linear regression and decision trees. For instance, we have helped clients in the retail sector optimize inventory management by predicting demand using supervised learning techniques.
- Unsupervised Learning: Involves training a model on unlabeled data to find hidden patterns. Examples include clustering and dimensionality reduction techniques. Our work with financial institutions has utilized unsupervised learning to detect fraudulent transactions by identifying unusual patterns in transaction data.
- Semi-supervised Learning: Combines both labeled and unlabeled data for training, improving learning accuracy. This approach has been beneficial for clients with limited labeled data, allowing them to enhance model performance without extensive data labeling efforts.
- Reinforcement Learning: Involves training an agent to make decisions by rewarding desired actions and penalizing undesired ones. We have implemented reinforcement learning in optimizing supply chain logistics, where agents learn to make real-time decisions that minimize costs and maximize efficiency.
- Key Components: Â
- Data: The foundation of machine learning; quality and quantity of data significantly impact model performance. Rapid Innovation emphasizes data quality, ensuring our clients have the right data to drive insights.
- Features: Attributes or variables used to make predictions. Feature selection and engineering are crucial for model accuracy. We assist clients in identifying and engineering the most relevant features to improve their predictive models.
- Models: Mathematical representations of the data that can make predictions. Different algorithms are suited for different types of problems. Our expertise allows us to select the most appropriate models tailored to our clients' specific needs.
- Evaluation Metrics: Â
- Accuracy: The ratio of correctly predicted instances to the total instances. We guide clients in understanding and interpreting accuracy in the context of their business objectives.
- Precision and Recall: Metrics that help evaluate the performance of classification models, especially in imbalanced datasets. We help clients balance these metrics to align with their strategic goals.
- F1 Score: The harmonic mean of precision and recall, providing a balance between the two. Our team ensures that clients understand the implications of these metrics on their decision-making processes.
Understanding these fundamentals is essential for anyone looking to delve into the world of machine learning, as they form the basis for more advanced concepts and applications. At Rapid Innovation, we are committed to empowering our clients with the knowledge and tools necessary to harness the power of machine learning for greater ROI. This includes a comprehensive understanding of machine learning fundamentals and algorithms, as well as important concepts in machine learning that are crucial for data science and interview preparation.
2.2. Artificial Neural Networks
Artificial Neural Networks (ANNs) are computational models inspired by the human brain's structure and function. They consist of interconnected nodes (neurons) that process data in layers. Key aspects of ANNs include:
- Structure: Â
- Input Layer: The first layer that receives the input data.
- Hidden Layers: Intermediate layers where the actual processing occurs through weighted connections.
- Output Layer: The final layer that produces the output or prediction.
- Activation Functions: Functions that determine the output of a neuron based on its input. Common activation functions include: Â
- Sigmoid: Outputs values between 0 and 1, useful for binary classification.
- ReLU (Rectified Linear Unit): Outputs the input directly if positive; otherwise, it outputs zero, helping to mitigate the vanishing gradient problem.
- Softmax: Used in multi-class classification to convert raw scores into probabilities.
- Training Process: Â
- Forward Propagation: The process of passing input data through the network to obtain an output.
- Backpropagation: A method for updating the weights of the network based on the error of the output, using gradient descent to minimize loss.
- Applications: Â
- Image and speech recognition
- Natural language processing
- Game playing and autonomous systems
At Rapid Innovation, we have successfully implemented ANNs in various projects, enabling complex pattern recognition and decision-making processes. This has allowed our clients to enhance their operational efficiency and drive innovation in their respective fields, utilizing deep learning concepts and hands-on machine learning techniques.
2.3. Reinforcement Learning
Reinforcement Learning (RL) is a type of machine learning where an agent learns to make decisions by interacting with an environment. The agent receives feedback in the form of rewards or penalties, guiding its learning process. Key elements of reinforcement learning include:
- Agent and Environment: Â
- The agent is the learner or decision-maker, while the environment is everything the agent interacts with.
- Actions and States: Â
- Actions are the choices made by the agent, while states represent the current situation of the environment. The agent's goal is to learn a policy that maximizes cumulative rewards over time.
- Reward Signal: Â
- A feedback mechanism that indicates the success of an action taken by the agent. Positive rewards encourage the agent to repeat actions, while negative rewards discourage them.
- Exploration vs. Exploitation: Â
- A fundamental trade-off in RL where the agent must decide whether to explore new actions to discover their rewards or exploit known actions that yield high rewards.
- Algorithms: Â
- Q-Learning: A model-free algorithm that learns the value of actions in states to derive an optimal policy.
- Deep Q-Networks (DQN): Combines Q-learning with deep neural networks to handle high-dimensional state spaces.
- Policy Gradient Methods: Directly optimize the policy by adjusting the parameters based on the received rewards.
Reinforcement learning has been successfully applied in various domains, including robotics, game playing (e.g., AlphaGo), and autonomous driving, showcasing its potential to solve complex decision-making problems. At Rapid Innovation, we harness the power of RL to develop solutions that drive efficiency and innovation for our clients, ultimately leading to greater ROI. This includes a focus on reinforcement learning concepts and their applications in real-world scenarios.
Refer to the image for a visual representation of the concepts discussed in the Machine Learning Fundamentals section.
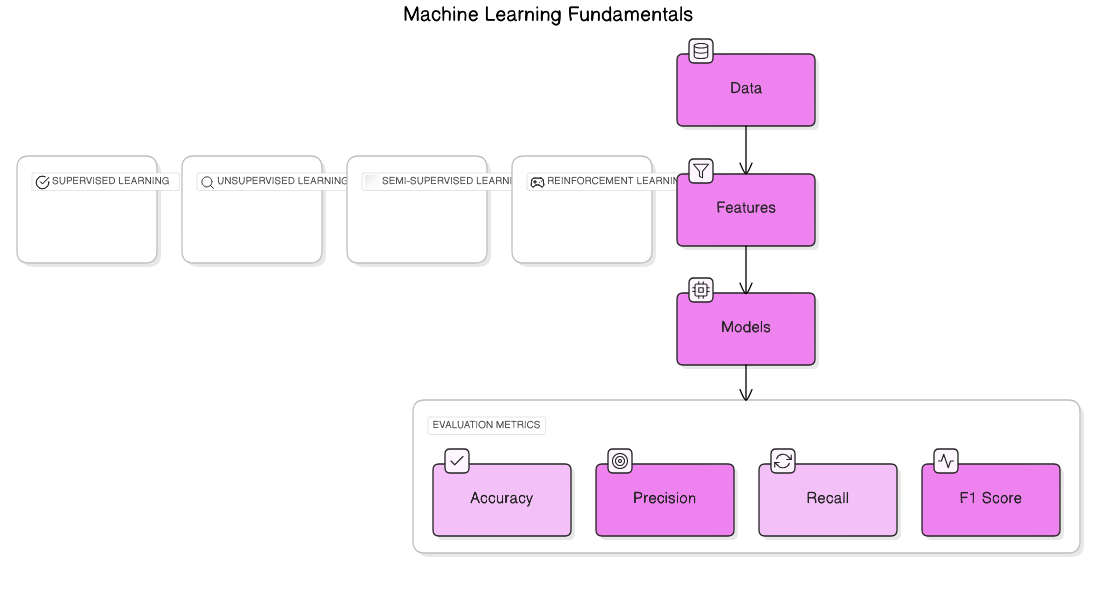
2.4. Optimization Algorithms
Optimization algorithms are essential tools in various fields, including operations research, machine learning, and artificial intelligence. These algorithms aim to find the best solution from a set of feasible solutions by maximizing or minimizing a particular objective function.
- Types of Optimization Algorithms: Â
- Linear Programming: Used for problems where the objective function and constraints are linear.
- Integer Programming: Focuses on problems where some or all variables are restricted to integer values.
- Non-linear Programming: Deals with problems where the objective function or constraints are non-linear.
- Dynamic Programming: Breaks down problems into simpler subproblems and solves them recursively.
- Genetic Algorithms: Inspired by natural selection, these algorithms use techniques such as mutation and crossover to evolve solutions.
- Bayesian Optimization: A probabilistic model-based optimization technique that is particularly useful for optimizing expensive-to-evaluate functions.
- Gradient Descent Algorithm: An iterative optimization algorithm used for finding the minimum of a function, commonly used in machine learning.
- Adaptive Optimization: Techniques that adjust the optimization process based on the performance of previous iterations.
- Particle Swarm Optimization: A population-based optimization technique inspired by the social behavior of birds and fish.
- Ant Colony Optimization (ACO): A metaheuristic inspired by the foraging behavior of ants, used for solving combinatorial optimization problems, including the traveling salesperson problem algorithm.
- Applications: Â
- Supply Chain Management: Optimizing logistics and inventory levels to reduce costs and improve service delivery.
- Finance: Portfolio optimization to maximize returns while minimizing risk, enabling better investment strategies.
- Engineering: Design optimization for structures and systems, ensuring efficiency and safety.
- Challenges: Â
- Complexity: Some optimization problems are NP-hard, making them computationally intensive and requiring advanced techniques.
- Local Optima: Algorithms may converge to local optima rather than the global optimum, which can limit effectiveness.
At Rapid Innovation, we leverage these optimization algorithms, including genetic optimization, bayesian optimisation, and dynamic programming problem techniques, to help our clients streamline operations, enhance decision-making, and ultimately achieve greater ROI. By applying tailored optimization strategies, we enable businesses to navigate complex challenges and unlock new efficiencies. For more insights on how these technologies are transforming industries, check out our article on AI-driven digital twins.
2.5. Predictive Analytics Technologies
Predictive analytics technologies leverage statistical algorithms and machine learning techniques to analyze historical data and predict future outcomes. These technologies are increasingly vital in decision-making processes across various industries.
- Key Components: Â
- Data Mining: Extracting patterns from large datasets to identify trends and relationships that inform strategic decisions.
- Statistical Modeling: Using mathematical models to represent data relationships and predict future events with accuracy.
- Machine Learning: Algorithms that learn from data to improve predictions over time, adapting to new information.
- Tools and Technologies: Â
- R and Python: Popular programming languages for data analysis and predictive modeling, enabling robust analytics solutions.
- Big Data Technologies: Tools like Hadoop and Spark facilitate the processing of large datasets, allowing for real-time insights.
- Cloud Computing: Platforms such as AWS and Azure provide scalable resources for predictive analytics, ensuring flexibility and efficiency.
- Applications: Â
- Healthcare: Predicting patient outcomes and optimizing treatment plans to improve care quality.
- Retail: Forecasting sales and managing inventory levels to enhance customer satisfaction and reduce waste.
- Finance: Credit scoring and fraud detection to mitigate risks and protect assets.
- Benefits: Â
- Improved Decision-Making: Data-driven insights lead to better strategic choices, enhancing overall business performance.
- Cost Reduction: Identifying inefficiencies can lead to significant savings, maximizing profitability.
- Enhanced Customer Experience: Personalization based on predictive insights can improve customer satisfaction and loyalty.
At Rapid Innovation, we harness predictive analytics technologies to empower our clients with actionable insights, driving informed decisions that lead to increased ROI and competitive advantage.
2.6. Multi-Agent Systems
Multi-agent systems (MAS) consist of multiple interacting intelligent agents that can work together to solve complex problems. These systems are designed to simulate and manage distributed problem-solving environments.
- Characteristics of Multi-Agent Systems: Â
- Autonomy: Each agent operates independently and makes its own decisions, contributing to overall system efficiency.
- Cooperation: Agents can collaborate to achieve common goals, enhancing problem-solving capabilities.
- Adaptability: Agents can adjust their behavior based on changes in the environment, ensuring resilience.
- Types of Agents: Â
- Reactive Agents: Respond to environmental stimuli without internal state, providing quick responses to dynamic situations.
- Deliberative Agents: Maintain an internal model of the world and plan actions based on that model, enabling strategic decision-making.
- Hybrid Agents: Combine reactive and deliberative approaches for more robust behavior, optimizing performance across various scenarios.
- Applications: Â
- Robotics: Coordinating multiple robots for tasks like search and rescue, improving operational efficiency.
- Traffic Management: Optimizing traffic flow through intelligent transportation systems, reducing congestion and improving safety.
- E-commerce: Facilitating automated negotiation and trading between agents, enhancing transaction efficiency.
- Challenges: Â
- Communication: Ensuring effective communication between agents can be complex, requiring sophisticated protocols.
- Scalability: As the number of agents increases, managing interactions becomes more challenging, necessitating robust frameworks.
- Conflict Resolution: Agents may have competing goals, requiring mechanisms to resolve conflicts and maintain system harmony.
In summary, optimization algorithms, predictive analytics technologies, and multi-agent systems are integral components of modern computational intelligence. Each plays a crucial role in enhancing decision-making, improving efficiency, and solving complex problems across various domains. At Rapid Innovation, we are committed to leveraging these technologies to help our clients achieve their business goals efficiently and effectively, ultimately driving greater ROI.
3. AI Agent Capabilities in Resource Allocation
AI agents are revolutionizing resource allocation across various industries by leveraging advanced algorithms and data analytics. Their capabilities in predictive demand forecasting and dynamic resource optimization are particularly noteworthy, enabling organizations to enhance efficiency and reduce costs.
3.1 Predictive Demand Forecasting
Predictive demand forecasting involves using AI algorithms to analyze historical data and predict future demand for products or services. This capability is crucial for businesses aiming to optimize inventory levels, manage supply chains, and improve customer satisfaction. AI agents utilize machine learning models to identify patterns in historical sales data, incorporating external factors such as seasonality, market trends, and economic indicators to refine predictions. By analyzing large datasets, AI can provide more accurate forecasts than traditional methods, reducing the risk of overstocking or stockouts.
Benefits of predictive demand forecasting include:
- Improved inventory management, leading to reduced holding costs.
- Enhanced customer satisfaction through better product availability.
- Increased operational efficiency by aligning resources with anticipated demand.
According to a study, companies that implement AI-driven demand forecasting can achieve up to a 20% reduction in inventory costs (source: McKinsey). At Rapid Innovation, we harness these AI resource allocation capabilities to help our clients streamline their operations, ensuring they meet customer demands while minimizing excess inventory. For more insights, check out our article on AI in Demand Forecasting.
3.2 Dynamic Resource Optimization
Dynamic resource optimization refers to the ability of AI agents to continuously adjust resource allocation based on real-time data and changing conditions. This capability is essential for organizations that need to respond quickly to fluctuations in demand or operational challenges. AI agents analyze real-time data from various sources, including sales, production, and logistics. They can automatically reallocate resources, such as labor and materials, to meet changing demands. By using optimization algorithms, AI can identify the most efficient allocation of resources, minimizing waste and maximizing productivity.
Key advantages of dynamic resource optimization include:
- Increased agility in responding to market changes, allowing businesses to capitalize on new opportunities.
- Enhanced cost savings through more efficient use of resources.
- Improved service levels by ensuring that the right resources are available at the right time.
Research indicates that organizations employing dynamic resource optimization can see a significant increase in operational efficiency, with some reporting up to a 30% improvement in resource utilization (source: Deloitte). Rapid Innovation leverages these AI-driven strategies to empower our clients, enabling them to adapt swiftly to market dynamics and optimize their resource management for greater ROI.
3.3. Real-time Decision Making

‍
Real-time decision making refers to the ability to analyze data and make informed choices instantly, often in response to changing conditions. This capability is crucial in various sectors, including finance, healthcare, and supply chain management.
- Enhances responsiveness: Organizations can react swiftly to market changes, customer demands, or operational challenges.
- Utilizes advanced technologies: Tools like artificial intelligence (AI) and machine learning (ML) enable the processing of vast amounts of data in real-time, allowing Rapid Innovation to implement solutions that enhance operational efficiency.
- Improves accuracy: Real-time analytics help reduce errors in decision-making by providing up-to-date information, ensuring that clients can trust the insights derived from their data.
- Supports proactive strategies: Businesses can anticipate issues and opportunities, allowing for preemptive actions rather than reactive measures, which is essential for maintaining a competitive edge.
- Increases competitive advantage: Companies that leverage real-time data can outperform competitors who rely on outdated information, a key area where Rapid Innovation can assist clients in achieving greater ROI.
For instance, in the stock market, algorithms analyze trends and execute trades within milliseconds, capitalizing on fleeting opportunities. In healthcare, real-time monitoring of patient vitals can lead to immediate interventions, improving patient outcomes. For more information on how AI can enhance healthcare management, visit AI Healthcare Management and learn from real-world AI implementations.
3.4. Adaptive Allocation Strategies
Adaptive allocation strategies involve dynamically adjusting resources based on real-time data and changing conditions. This approach is essential for optimizing performance and maximizing efficiency in various industries.
- Flexibility: Organizations can shift resources quickly in response to demand fluctuations or unexpected challenges, a capability that Rapid Innovation can help implement through tailored AI solutions.
- Data-driven decisions: By analyzing performance metrics, companies can allocate resources where they are most needed, ensuring that investments yield the highest returns.
- Cost efficiency: Adaptive strategies help minimize waste by ensuring that resources are used effectively, which is crucial for maximizing profitability.
- Enhanced customer satisfaction: By aligning resources with customer needs, businesses can improve service delivery and responsiveness, leading to increased customer loyalty.
- Continuous improvement: Regularly assessing and adjusting allocation strategies fosters a culture of innovation and adaptability, which Rapid Innovation promotes through its consulting services.
For example, in logistics, companies can reroute delivery trucks based on traffic conditions or customer requests, ensuring timely deliveries. In manufacturing, adaptive allocation can optimize labor and materials based on production schedules and demand forecasts.
3.5. Complex Constraint Management
Complex constraint management involves navigating and addressing multiple, often interrelated limitations that can impact decision-making and resource allocation. This is particularly relevant in industries with intricate operational frameworks.
- Identifying constraints: Organizations must recognize various constraints, such as regulatory requirements, resource limitations, and market conditions, which Rapid Innovation can help clients analyze effectively.
- Prioritizing issues: Not all constraints are equal; understanding which ones have the most significant impact is crucial for effective management, allowing for focused resource allocation.
- Integrating solutions: A holistic approach is necessary to address constraints without creating new problems in other areas, a principle that guides Rapid Innovation's development strategies.
- Utilizing technology: Advanced software tools can help model complex scenarios and simulate the effects of different decisions, enabling organizations to make informed choices.
- Enhancing collaboration: Engaging stakeholders across departments can lead to more comprehensive solutions to constraint-related challenges, fostering a collaborative environment that Rapid Innovation encourages.
For instance, in project management, teams must balance time, budget, and scope constraints to deliver successful outcomes. In supply chain management, companies face constraints related to inventory levels, supplier reliability, and transportation logistics, requiring careful coordination and planning. Rapid Innovation's expertise in AI and blockchain can streamline these processes, ultimately leading to improved efficiency and ROI for clients.
3.6. Optimization Under Uncertainty
Optimization under uncertainty is a critical aspect of decision-making processes in various fields, including finance, logistics, and artificial intelligence. This concept involves making the best possible decisions when faced with unpredictable variables and incomplete information.
- Uncertainty can arise from various sources, such as: Â
- Incomplete data
- Variability in system behavior
- External factors that are difficult to predict
- Techniques used in optimization under uncertainty include: Â
- Stochastic programming: This method incorporates randomness into the optimization model, allowing for the evaluation of different scenarios.
- Robust optimization: This approach focuses on finding solutions that perform well across a range of uncertain conditions, rather than optimizing for a single expected outcome.
- Monte Carlo simulations: These simulations use random sampling to estimate the impact of uncertainty on the optimization process.
- Applications of optimization under uncertainty can be found in: Â
- Supply chain management: where demand and supply fluctuations can significantly impact costs and efficiency.
- Financial portfolio management: where market conditions are unpredictable, and risk assessment is crucial.
- AI resource allocation: where agents must allocate resources effectively despite uncertain environments.
Understanding and implementing optimization under uncertainty, including techniques such as optimisation under uncertainty and linear programming under uncertainty, is essential for developing resilient systems that can adapt to changing conditions and make informed decisions. At Rapid Innovation, we leverage these techniques to help our clients navigate uncertainty, ultimately leading to greater ROI through improved decision-making and resource allocation. For more information on our services, visit our AI agent development company.
4. Technical Architecture of AI Resource Allocation Agents
The technical architecture of AI resource allocation agents is designed to facilitate efficient and effective distribution of resources in various applications. These agents leverage advanced algorithms and data analytics to optimize resource usage while considering constraints and objectives.
- Key components of the architecture include: Â
- Data input and processing: Agents gather and process data from various sources to inform their decision-making.
- Decision-making algorithms: These algorithms analyze the processed data to determine the best allocation strategies.
- Communication interfaces: Agents must communicate with other systems and stakeholders to coordinate resource allocation effectively.
- The architecture can be categorized into several layers: Â
- Sensor layer: Collects real-time data from the environment.
- Processing layer: Analyzes the data and generates insights.
- Action layer: Executes the resource allocation decisions based on the analysis.
- Challenges in designing AI resource allocation agents include: Â
- Ensuring scalability to handle large datasets and complex environments.
- Maintaining robustness against uncertainties and unexpected changes.
- Integrating with existing systems and workflows for seamless operation.
By focusing on these architectural elements, AI resource allocation agents can enhance efficiency and effectiveness in resource management across various sectors, ultimately driving better business outcomes for our clients.
4.1. Agent Design Principles
The design principles of AI resource allocation agents are fundamental to their functionality and effectiveness. These principles guide the development process, ensuring that agents can operate efficiently in dynamic environments.
- Core design principles include: Â
- Modularity: Agents should be designed in a modular fashion, allowing for easy updates and maintenance. This approach enables developers to enhance specific components without overhauling the entire system.
- Flexibility: Agents must be adaptable to changing conditions and requirements. This flexibility allows them to respond to new challenges and opportunities as they arise.
- Scalability: The design should accommodate growth in data volume and complexity. Scalable agents can handle increased workloads without compromising performance.
- Additional considerations in agent design: Â
- User-centric design: Agents should prioritize user needs and experiences, ensuring that their interfaces are intuitive and accessible.
- Transparency: Providing insights into the decision-making process can build trust and facilitate collaboration between agents and human stakeholders.
- Performance metrics: Establishing clear metrics for evaluating agent performance is essential for continuous improvement and optimization.
- The application of these design principles can lead to: Â
- Enhanced decision-making capabilities
- Improved resource utilization
- Greater overall efficiency in operations
By adhering to these design principles, developers can create AI resource allocation agents that are robust, efficient, and capable of navigating the complexities of modern resource management. At Rapid Innovation, we apply these principles to ensure our solutions not only meet but exceed client expectations, driving significant ROI through optimized resource allocation.
4.2. Data Integration Frameworks
Data integration frameworks are essential for combining data from different sources into a unified view. They facilitate the seamless flow of information across various systems, enabling organizations to make informed decisions based on comprehensive data analysis. At Rapid Innovation, we specialize in implementing these frameworks, including python data integration framework, to help our clients achieve greater ROI by ensuring that their data is accurate, accessible, and actionable.
- Key components of data integration frameworks include: Â
- Data Sources: These can be databases, cloud storage, APIs, or even real-time data streams.
- Data Transformation: This involves cleaning, normalizing, and enriching data to ensure consistency and usability.
- Data Loading: The process of moving transformed data into a target system, such as a data warehouse or data lake.
- Data Governance: Ensuring data quality, security, and compliance with regulations.
- Popular data integration frameworks include: Â
- Apache NiFi: A robust tool for automating data flow between systems.
- Talend: Offers a suite of tools for data integration, data quality, and data governance.
- Informatica: Known for its enterprise data integration solutions.
- SSIS ETL framework: A widely used framework for data integration and transformation.
- Benefits of using data integration frameworks: Â
- Improved data accuracy and consistency.
- Enhanced decision-making capabilities through comprehensive data analysis.
- Increased operational efficiency by automating data workflows.
4.3. Machine Learning Model Architectures
Machine learning model architectures refer to the structured frameworks that define how machine learning algorithms are designed and implemented. These architectures play a crucial role in determining the performance and effectiveness of machine learning models. Rapid Innovation leverages advanced machine learning architectures to develop solutions that drive business growth and efficiency.
- Common types of machine learning model architectures include: Â
- Supervised Learning: Models are trained on labeled data, learning to predict outcomes based on input features.
- Unsupervised Learning: Models identify patterns and relationships in unlabeled data, often used for clustering and association tasks.
- Reinforcement Learning: Models learn through trial and error, receiving feedback from their actions to improve performance over time.
- Popular machine learning architectures: Â
- Convolutional Neural Networks (CNNs): Primarily used for image processing tasks, CNNs excel at recognizing patterns in visual data.
- Recurrent Neural Networks (RNNs): Designed for sequential data, RNNs are effective in tasks like natural language processing and time series analysis.
- Transformer Models: These have revolutionized NLP tasks, enabling models to understand context and relationships in text data.
- Considerations for selecting a machine learning architecture: Â
- The nature of the data (structured vs. unstructured).
- The specific problem being addressed (classification, regression, etc.).
- Computational resources available for training and deployment.
4.4. Decision Support Systems
Decision support systems (DSS) are computer-based tools that help organizations make informed decisions by analyzing data and presenting actionable insights. These systems are crucial in various industries, including healthcare, finance, and logistics. Rapid Innovation's expertise in developing tailored DSS solutions empowers clients to enhance their decision-making processes and achieve strategic objectives.
- Key components of decision support systems include: Â
- Data Management: Collecting and storing relevant data from various sources.
- Model Management: Utilizing analytical models to process data and generate insights.
- User Interface: Providing an intuitive interface for users to interact with the system and visualize data.
- Types of decision support systems: Â
- Data-driven DSS: Focus on analyzing large volumes of data to support decision-making.
- Model-driven DSS: Utilize mathematical and statistical models to simulate scenarios and predict outcomes.
- Knowledge-driven DSS: Leverage expert knowledge and rules to guide decision-making processes.
- Benefits of decision support systems: Â
- Enhanced decision-making speed and accuracy.
- Improved collaboration among team members through shared insights.
- Ability to analyze complex data sets and identify trends that may not be immediately apparent.
In conclusion, data integration frameworks, including ssis etl framework and talend etl framework, machine learning model architectures, and decision support systems are integral components of modern data-driven decision-making processes. By leveraging these technologies, organizations can enhance their operational efficiency, improve data accuracy, and make more informed decisions. At Rapid Innovation, we are committed to helping our clients harness the power of AI and blockchain technologies to achieve their business goals efficiently and effectively.
4.5. Computational Complexity Management
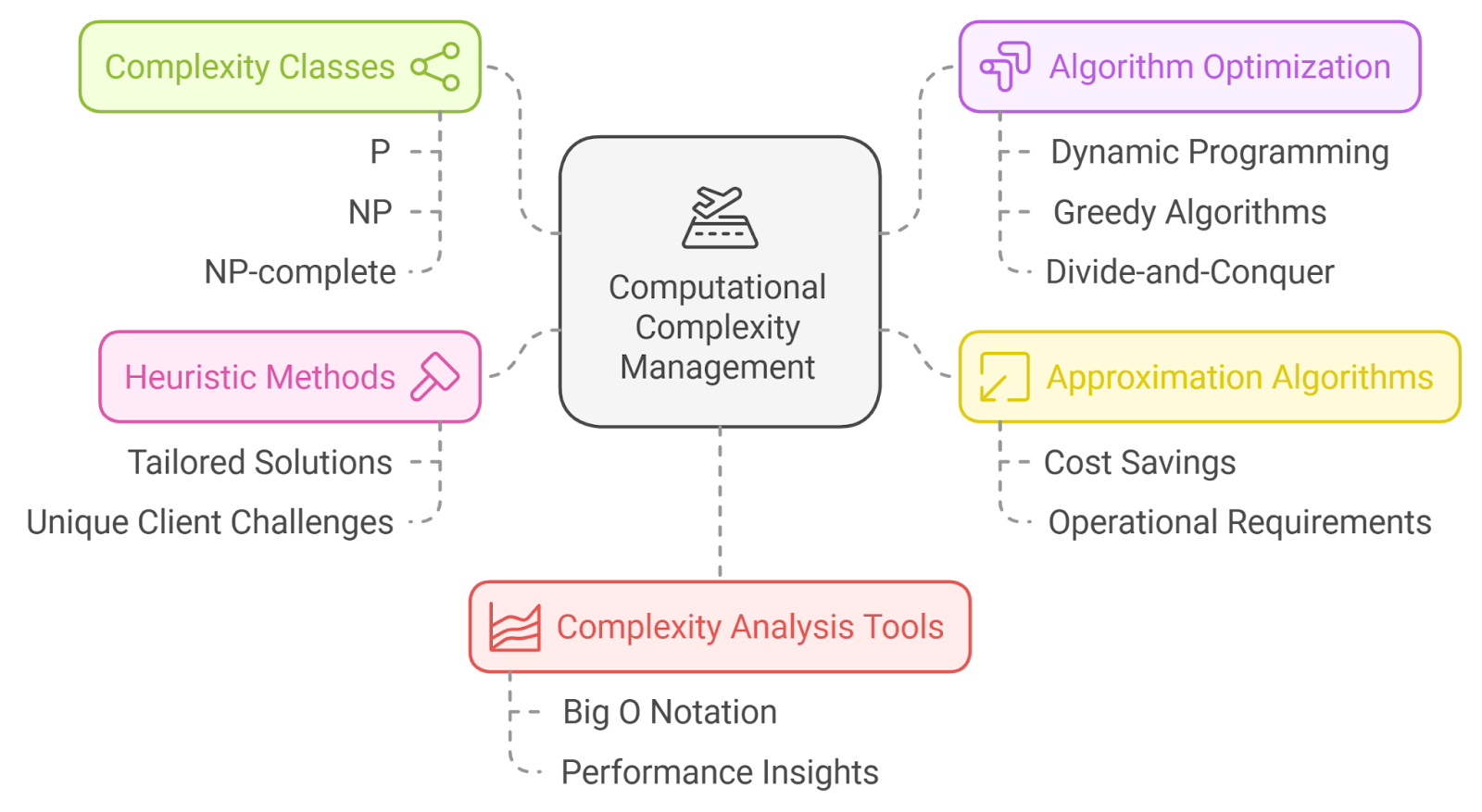
‍
Computational complexity management is crucial in optimizing algorithms and systems to ensure efficient performance. It involves analyzing the resources required for computation, including time and space, and finding ways to minimize these requirements.
- Understanding Complexity Classes: Problems are categorized into complexity classes such as P, NP, and NP-complete, which help in determining the feasibility of solving them within reasonable time limits. Rapid Innovation leverages this understanding to guide clients in selecting appropriate algorithms for their specific needs, ensuring timely project delivery.
- Algorithm Optimization: Techniques like dynamic programming, greedy algorithms, and divide-and-conquer can significantly reduce computational complexity. For example, using memoization in recursive algorithms can prevent redundant calculations, thus saving time. Our team at Rapid Innovation employs these techniques to enhance the performance of AI models, leading to faster insights and improved decision-making for our clients.
- Approximation Algorithms: For NP-hard problems, exact solutions may be impractical. Approximation algorithms provide near-optimal solutions in a fraction of the time. By implementing these algorithms, Rapid Innovation helps clients achieve substantial cost savings while still meeting their operational requirements.
- Heuristic Methods: Heuristics are rules of thumb that guide problem-solving. They can lead to faster solutions, especially in complex scenarios where traditional methods fail. Our expertise in heuristic approaches allows us to deliver tailored solutions that address unique client challenges efficiently.
- Complexity Analysis Tools: Tools and frameworks like Big O notation help in analyzing and communicating the efficiency of algorithms, making it easier to identify bottlenecks. Rapid Innovation utilizes these tools to provide clients with clear insights into their system performance, enabling informed decision-making. For more information on how we can assist with your AI project estimation needs, visit our AI project estimation services and learn about AI agents for service scheduling.
4.6. Distributed Computing Approaches
Distributed computing involves multiple interconnected computers working together to solve a problem. This approach enhances performance, scalability, and reliability.
- Scalability: Distributed systems can easily scale by adding more nodes, allowing for increased processing power and storage capacity. Rapid Innovation designs scalable solutions that grow with our clients' needs, ensuring they can handle increased workloads without compromising performance.
- Fault Tolerance: By distributing tasks across multiple machines, the system can continue functioning even if one or more nodes fail. Techniques like replication and checkpointing are commonly used. Our solutions incorporate these techniques to enhance system reliability, providing clients with peace of mind.
- Load Balancing: Efficiently distributing workloads across nodes ensures that no single machine is overwhelmed, leading to improved performance and resource utilization. Rapid Innovation implements advanced load balancing strategies to optimize resource allocation, resulting in better overall system efficiency.
- Communication Protocols: Distributed systems rely on communication protocols like TCP/IP and message-passing interfaces (MPI) to facilitate data exchange between nodes. Our expertise in these protocols ensures seamless integration and communication within distributed systems, enhancing operational efficiency.
- Cloud Computing: Cloud platforms provide distributed computing resources on-demand, allowing businesses to leverage powerful computing capabilities without investing in physical infrastructure. Rapid Innovation assists clients in adopting cloud solutions that align with their business goals, maximizing ROI.
5. Resource Allocation Domains
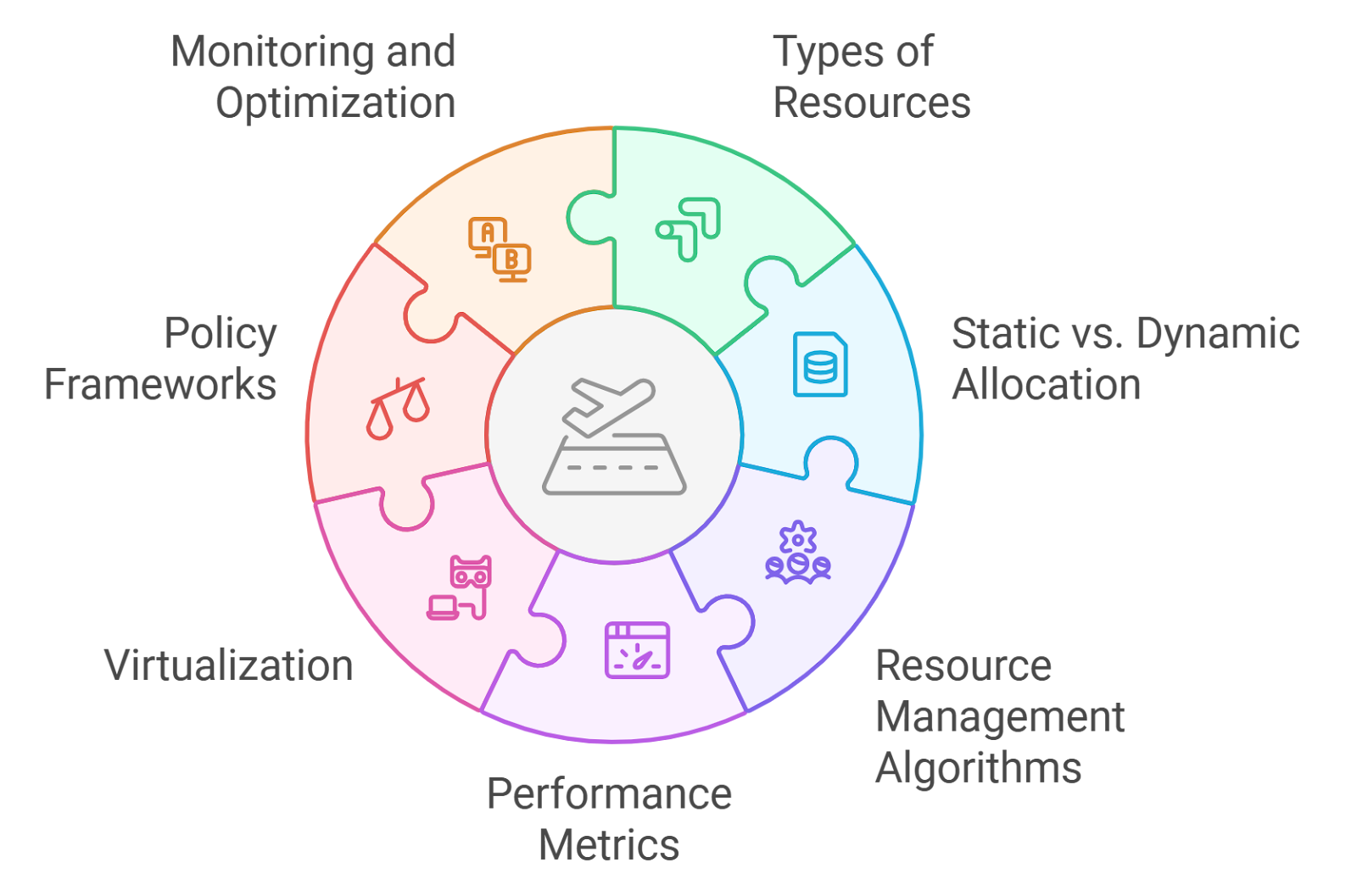
‍
Resource allocation is a critical aspect of computing that involves distributing available resources among various tasks or processes. Effective resource allocation ensures optimal performance and efficiency.
- Types of Resources: Resources can include CPU time, memory, bandwidth, and storage. Understanding the nature of these resources is essential for effective allocation. Rapid Innovation helps clients assess their resource needs to optimize allocation strategies.
- Static vs. Dynamic Allocation: Static allocation assigns resources at compile time, while dynamic allocation adjusts resources at runtime based on current needs. Dynamic allocation is often more efficient in fluctuating environments. Our team implements dynamic allocation strategies to enhance system responsiveness and efficiency.
- Resource Management Algorithms: Algorithms like First-Come-First-Served (FCFS), Round Robin, and Priority Scheduling help in managing resource allocation effectively. Rapid Innovation employs these algorithms to ensure that client systems operate smoothly and efficiently.
- Performance Metrics: Key performance indicators (KPIs) such as throughput, latency, and resource utilization are used to evaluate the effectiveness of resource allocation strategies. We provide clients with detailed performance metrics to help them understand their system's efficiency and make informed adjustments.
- Virtualization: Virtualization technologies allow multiple virtual machines to run on a single physical machine, optimizing resource usage and providing flexibility in resource allocation. Rapid Innovation leverages virtualization to help clients maximize their infrastructure investments.
- Policy Frameworks: Establishing clear policies for resource allocation helps in maintaining fairness and efficiency, especially in multi-tenant environments like cloud computing. Our consulting services include developing tailored policy frameworks that align with client objectives.
- Monitoring and Optimization: Continuous monitoring of resource usage enables proactive adjustments to allocation strategies, ensuring that resources are used efficiently and effectively. Rapid Innovation implements robust monitoring solutions that empower clients to optimize their resource allocation continuously.
5.1. Enterprise Resource Planning
Enterprise Resource Planning (ERP) systems are integrated software solutions that help organizations manage their core business processes. These systems streamline operations by consolidating data across various departments, enabling better decision-making and improved efficiency.
- Centralized Data Management: ERP systems provide a single source of truth for all business data, reducing data silos and ensuring that all departments have access to the same information. Rapid Innovation can implement AI-driven data integration tools that enhance data accuracy and accessibility, leading to more informed business decisions.
- Enhanced Reporting and Analytics: With real-time data access, businesses can generate reports and analyze performance metrics quickly, leading to informed strategic decisions. Our expertise in AI can help develop advanced analytics capabilities, allowing clients to uncover insights and trends that drive greater ROI.
- Improved Collaboration: ERP systems facilitate communication between departments, allowing for better coordination and collaboration on projects and tasks. By integrating AI-powered collaboration tools, Rapid Innovation can enhance team productivity and streamline workflows.
- Scalability: As businesses grow, ERP systems can be scaled to accommodate increased data and user demands, making them a long-term solution for organizations. Our blockchain solutions can ensure secure and efficient scaling, providing clients with a robust infrastructure that supports growth.
- Compliance and Risk Management: ERP systems often include features that help businesses comply with industry regulations and manage risks effectively. Rapid Innovation can leverage blockchain technology to enhance transparency and traceability, ensuring compliance and reducing risk exposure.
5.2. Manufacturing and Supply Chain
Manufacturing and supply chain management are critical components of any production-oriented business. Effective management in these areas ensures that products are produced efficiently and delivered to customers on time. Key strategies include:
- Lean Manufacturing: This approach focuses on minimizing waste while maximizing productivity. Techniques such as Just-In-Time (JIT) inventory help reduce excess stock and improve cash flow. Rapid Innovation can implement AI solutions that optimize production schedules and inventory levels, leading to significant cost savings.
- Supply Chain Visibility: Advanced technologies like IoT and blockchain provide real-time tracking of goods, enhancing transparency and accountability throughout the supply chain. Our blockchain expertise can create immutable records of transactions, ensuring that all stakeholders have access to accurate and timely information.
- Demand Forecasting: Accurate forecasting tools help manufacturers predict customer demand, allowing for better inventory management and production planning. By utilizing AI algorithms, Rapid Innovation can enhance forecasting accuracy, enabling clients to respond proactively to market changes.
- Supplier Relationship Management: Building strong relationships with suppliers can lead to better pricing, quality, and reliability, which are essential for maintaining a competitive edge. Our consulting services can help clients develop strategic partnerships that drive value and efficiency.
- Automation and Robotics: The integration of automation in manufacturing processes can significantly increase efficiency, reduce labor costs, and improve product quality. Rapid Innovation can assist in implementing AI-driven automation solutions that streamline operations and enhance productivity.
5.3. Cloud Computing and IT Infrastructure
Cloud computing has revolutionized the way businesses manage their IT infrastructure. By leveraging cloud services, organizations can enhance flexibility, scalability, and cost-effectiveness. The benefits include:
- Cost Savings: Cloud computing reduces the need for extensive on-premises hardware and maintenance, leading to significant cost savings for businesses. Rapid Innovation can help clients transition to cloud-based solutions that optimize resource allocation and reduce operational costs.
- Scalability: Cloud services allow organizations to easily scale their IT resources up or down based on demand, ensuring they only pay for what they use. Our expertise in cloud architecture ensures that clients can adapt their IT infrastructure to meet evolving business needs.
- Enhanced Collaboration: Cloud-based tools enable teams to collaborate in real-time, regardless of their physical location, improving productivity and communication. Rapid Innovation can implement AI-enhanced collaboration platforms that foster innovation and teamwork.
- Data Security: Many cloud providers offer robust security measures, including encryption and regular backups, to protect sensitive business data. Our blockchain solutions can further enhance data security by providing decentralized and tamper-proof storage options.
- Disaster Recovery: Cloud computing facilitates efficient disaster recovery solutions, ensuring that businesses can quickly recover from data loss or system failures. Rapid Innovation can design comprehensive disaster recovery plans that leverage cloud capabilities for minimal downtime and maximum resilience.
Incorporating enterprise resource planning systems, enterprise resource management, and ERP enterprise resource planning into these strategies ensures that organizations can effectively manage their resources and operations. The integration of ERP in IT and the use of enterprise resource programs further enhance the overall efficiency and effectiveness of business processes.
5.4. Healthcare Resource Management
Healthcare resource management is a critical aspect of ensuring that medical facilities operate efficiently and effectively. It involves the strategic allocation and utilization of resources such as personnel, equipment, and facilities to provide optimal patient care.
- Efficient staffing is essential to meet patient needs while controlling costs. Rapid Innovation employs AI-driven workforce management solutions that analyze patient data and predict staffing requirements, ensuring that healthcare facilities are adequately staffed without overspending. This is particularly important in the context of healthcare human resource management and human resource management in the healthcare industry.
- Inventory management of medical supplies helps prevent shortages and reduces waste. Our blockchain-based inventory tracking systems provide real-time visibility into supply levels, enabling healthcare providers to manage their resources more effectively and reduce costs associated with overstocking or stockouts. This is a key component of resource management in healthcare.
- Data analytics can optimize scheduling and resource allocation, improving patient flow. By utilizing advanced analytics, we help healthcare organizations identify bottlenecks and streamline operations, leading to improved patient experiences and increased throughput. This is essential for healthcare workforce management solutions.
- Telemedicine has emerged as a valuable tool, allowing healthcare providers to extend their reach and manage resources better. Rapid Innovation develops secure telehealth platforms that facilitate remote consultations, reducing the burden on physical facilities and improving access to care. This aligns with healthcare talent management strategies.
- Training and development of healthcare staff ensure that they are equipped with the latest skills and knowledge. Our AI-powered training solutions provide personalized learning experiences, helping healthcare professionals stay updated with the latest practices and technologies, which is crucial for human resources in healthcare managing for success.
Effective healthcare resource management not only enhances patient outcomes but also contributes to the sustainability of healthcare systems. By leveraging technology and data, healthcare organizations can make informed decisions that lead to better resource utilization, including the management of healthcare resources and management solutions.
5.5. Energy and Utility Sectors
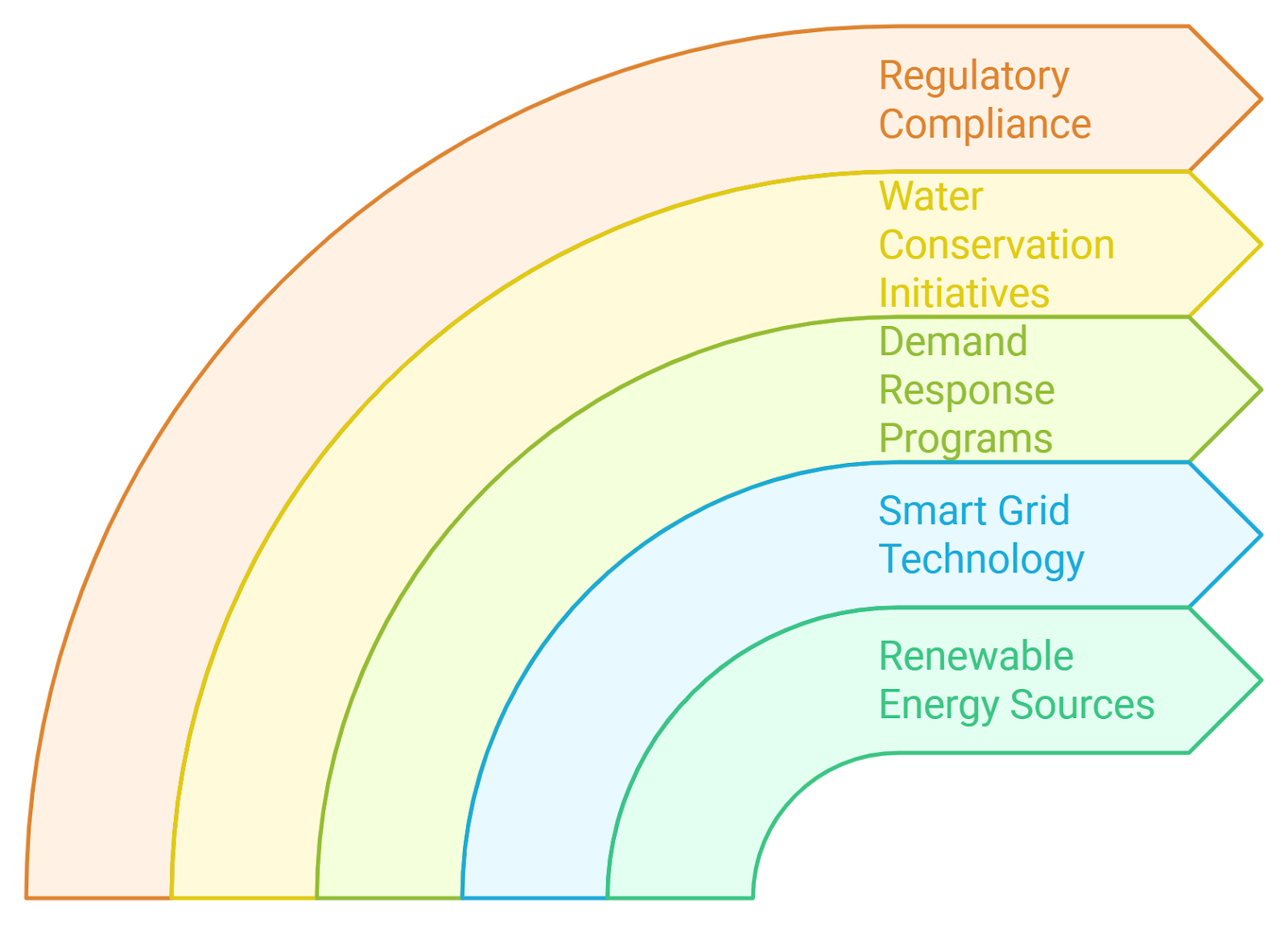
‍
The energy and utility sectors are vital for the functioning of modern society, providing essential services such as electricity, water, and gas. Effective management in these sectors is crucial for sustainability and efficiency.
- Renewable energy sources, such as solar and wind, are becoming increasingly important in reducing carbon footprints. Rapid Innovation assists organizations in integrating AI solutions that optimize the use of renewable energy, enhancing efficiency and reducing reliance on fossil fuels.
- Smart grid technology enhances the efficiency of energy distribution and consumption. Our blockchain solutions enable secure and transparent energy transactions, facilitating peer-to-peer energy trading and improving grid resilience.
- Demand response programs help manage energy loads during peak times, reducing the need for additional power plants. We implement AI algorithms that predict energy demand patterns, allowing utilities to adjust supply dynamically and minimize costs.
- Water conservation initiatives are essential for managing resources in areas facing drought or water scarcity. Our data analytics tools help utilities monitor water usage and identify areas for improvement, promoting sustainable practices.
- Regulatory compliance ensures that energy and utility companies adhere to environmental standards and safety protocols. Rapid Innovation provides compliance management solutions that leverage blockchain for secure record-keeping and reporting.
The integration of innovative technologies and sustainable practices in the energy and utility sectors is essential for meeting the growing demand for resources while minimizing environmental impact.
5.6. Financial Resource Allocation
Financial resource allocation is a fundamental aspect of organizational management, determining how funds are distributed across various departments and projects. Effective allocation ensures that resources are used efficiently to achieve strategic goals.
- Budgeting processes should be transparent and involve stakeholder input to align financial resources with organizational priorities. Rapid Innovation offers AI-driven budgeting tools that enhance transparency and facilitate collaborative decision-making.
- Performance metrics can help assess the effectiveness of resource allocation, guiding future decisions. Our analytics solutions provide real-time insights into financial performance, enabling organizations to make data-driven adjustments.
- Diversification of investments can mitigate risks and enhance financial stability. We assist clients in developing AI models that analyze market trends and optimize investment portfolios for better returns.
- Cost-benefit analysis is crucial for evaluating potential projects and initiatives before committing resources. Our data analytics capabilities enable organizations to conduct thorough analyses, ensuring that investments align with strategic objectives.
- Regular financial audits ensure accountability and help identify areas for improvement. Rapid Innovation provides blockchain-based audit solutions that enhance transparency and streamline the auditing process.
By implementing sound financial resource allocation strategies, organizations can enhance their operational efficiency and achieve long-term sustainability.
5.7. Human Resource Management
Human Resource Management (HRM) is a critical function within organizations that focuses on the recruitment, management, and development of employees. Effective HRM practices, including strategic human resource management, can significantly enhance organizational performance and employee satisfaction.
- Recruitment and Selection: Â
- Attracting the right talent is essential for any organization.
- Implementing structured interviews and assessments can improve the selection process, leveraging AI-driven tools to analyze candidate data and predict job fit.
- Training and Development: Â
- Continuous learning opportunities help employees grow their skills.
- Organizations that invest in training see higher employee retention rates, especially when utilizing AI to personalize learning paths based on individual performance metrics.
- Performance Management: Â
- Regular performance reviews provide feedback and set clear expectations.
- Utilizing performance metrics, enhanced by AI analytics, can help identify high performers and areas for improvement, leading to more informed decision-making.
- Employee Engagement: Â
- Engaged employees are more productive and committed to their work.
- Strategies such as employee recognition programs, supported by data analytics, can boost morale and foster a positive workplace culture.
- Compliance and Legal Issues: Â
- HR must ensure that the organization adheres to labor laws and regulations.
- Regular training on compliance, facilitated by AI systems that track changes in legislation, can mitigate legal risks.
- Diversity and Inclusion: Â
- Promoting a diverse workforce fosters innovation and creativity.
- Inclusive practices, supported by data-driven insights, can enhance team collaboration and performance, ensuring a balanced representation across all levels.
- Compensation and Benefits: Â
- Competitive salaries and benefits packages attract top talent.
- Regularly reviewing compensation structures using AI tools ensures fairness and equity, aligning with industry standards.
- Succession Planning: Â
- Preparing for future leadership needs is vital for organizational stability.
- Identifying and developing internal talent through predictive analytics can reduce turnover and recruitment costs, ensuring a robust leadership pipeline.
- Human Capital Management: Â
- Effective human capital management strategies align workforce capabilities with organizational goals, enhancing overall productivity.
- Employee Management Software: Â
- Utilizing employee management software can streamline HR processes, improve data management, and enhance communication within the organization.
- Human Resource Management Information System: Â
- Implementing a human resource management information system (HRMIS) can facilitate better decision-making and improve HR service delivery.
- Human Resources Degree: Â
- Pursuing a human resources degree can equip professionals with the necessary skills and knowledge to excel in HR roles.
- HR Software: Â
- Adopting HR software solutions can automate routine tasks, allowing HR professionals to focus on strategic initiatives.
- HR Management: Â
- Effective HR management practices are essential for fostering a positive work environment and driving organizational success.
- Human Resource Software: Â
- Investing in human resource software can enhance recruitment, onboarding, and employee engagement efforts.
- Software HR: Â
- Software HR solutions can provide valuable insights into workforce analytics, helping organizations make data-driven decisions.
- HRM Management: Â
- HRM management involves overseeing all aspects of human resources, ensuring alignment with business objectives.
- Human Resources and Management: Â
- Integrating human resources and management practices can lead to improved organizational performance and employee satisfaction.
- HRM Human Resource Management: Â
- HRM human resource management encompasses a strategic approach to managing an organization’s most valuable asset—its people.
6. Advanced Optimization Techniques
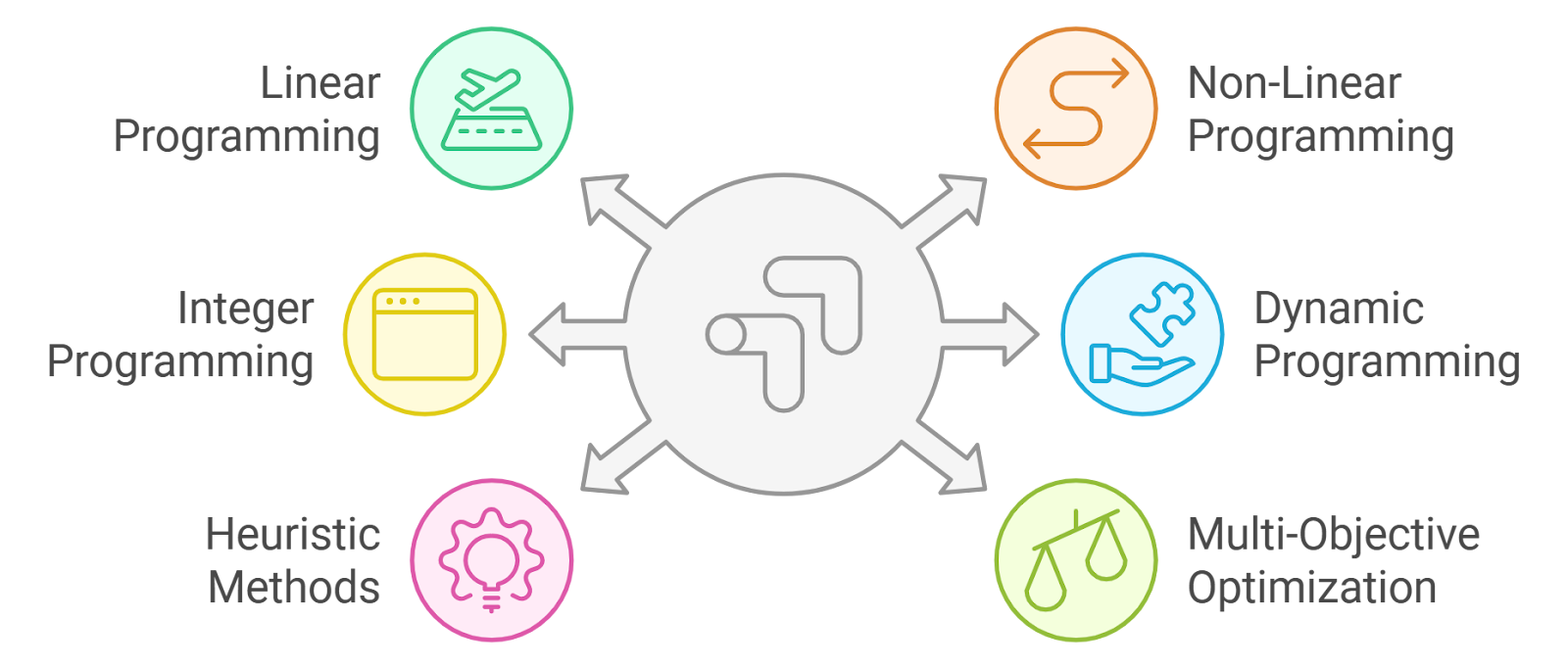
‍
Advanced optimization techniques are essential for solving complex problems in various fields, including engineering, finance, and logistics. These techniques help organizations make informed decisions that maximize efficiency and minimize costs.
- Linear Programming: Â
- A mathematical method for determining the best outcome in a given model.
- Commonly used in resource allocation and production scheduling.
- Non-Linear Programming: Â
- Deals with optimization problems where the objective function or constraints are non-linear.
- Useful in scenarios where relationships between variables are complex.
- Integer Programming: Â
- A type of linear programming where some or all variables are constrained to be integers.
- Often applied in scheduling and allocation problems.
- Dynamic Programming: Â
- A method for solving complex problems by breaking them down into simpler subproblems.
- Particularly effective in decision-making processes over time.
- Heuristic Methods: Â
- Techniques that find satisfactory solutions for complex problems when traditional methods are impractical.
- Examples include genetic algorithms and simulated annealing.
- Multi-Objective Optimization: Â
- Involves optimizing two or more conflicting objectives simultaneously.
- Useful in scenarios where trade-offs are necessary, such as cost vs. quality.
6.1. Multi-Objective Optimization
Multi-objective optimization (MOO) is a branch of optimization that deals with problems involving multiple objectives that need to be optimized simultaneously. This approach is crucial in real-world applications where trade-offs between conflicting objectives are common.
- Definition and Importance: Â
- MOO aims to find solutions that provide the best trade-offs among competing objectives.
- It is widely used in fields such as engineering design, finance, and logistics.
- Pareto Efficiency: Â
- A solution is considered Pareto efficient if no objective can be improved without worsening another.
- The Pareto front represents the set of optimal solutions in MOO.
- Techniques for MOO: Â
- Weighted Sum Method: Assigns weights to each objective and combines them into a single objective function.
- ε-constraint Method: Optimizes one objective while constraining others within specified limits.
- Multi-Objective Evolutionary Algorithms (MOEAs): Use evolutionary strategies to explore multiple solutions simultaneously.
- Applications: Â
- Engineering Design: Balancing cost, performance, and reliability in product development.
- Portfolio Optimization: Maximizing returns while minimizing risk in investment portfolios.
- Supply Chain Management: Optimizing logistics, inventory levels, and delivery times.
- Challenges: Â
- Identifying appropriate objectives and their relationships can be complex.
- The computational cost of solving MOO problems can be high, especially for large datasets.
- Future Trends: Â
- Increasing use of artificial intelligence and machine learning to enhance MOO techniques.
- Development of more efficient algorithms to handle larger and more complex problems.
6.2. Constrained Resource Allocation
Constrained resource allocation refers to the process of distributing limited resources among competing activities or projects while adhering to specific constraints. This concept is crucial in various fields, including project management, finance, and operations research. Resource constraints can include budget limits, time restrictions, manpower availability, and material shortages. The goal is to maximize the overall benefit or efficiency while ensuring that all constraints are respected. Techniques used in constrained resource allocation include linear programming, integer programming, and dynamic programming.
In practice, organizations often face challenges such as balancing multiple projects with overlapping resource needs, prioritizing projects based on strategic importance or return on investment, and adjusting allocations in response to changing conditions or unexpected events. The resource allocation optimization process is essential in addressing these challenges. Rapid Innovation leverages advanced AI algorithms to analyze resource allocation scenarios, enabling clients to make data-driven decisions that optimize their resource distribution effectively.
Effective constrained resource allocation can lead to improved project outcomes and efficiency, enhanced decision-making capabilities, and better alignment of resources with organizational goals. The resource allocation optimization problem is a common scenario that organizations encounter, and understanding the resource allocation problem in project management is vital for success.
6.3. Stochastic Optimization Models
Stochastic optimization models are mathematical frameworks that incorporate uncertainty into the decision-making process. Unlike deterministic models, which assume that all parameters are known and fixed, stochastic models account for variability and randomness in inputs. These models are particularly useful in scenarios where outcomes are uncertain, such as financial forecasting, supply chain management, and risk assessment. Stochastic optimization involves creating a set of possible scenarios and evaluating the performance of different strategies under these scenarios.
Key components of stochastic optimization include:
- Random variables: These represent uncertain parameters that can affect outcomes.
- Objective functions: These are the goals of the optimization, such as minimizing costs or maximizing profits.
- Constraints: These are the limitations that must be adhered to, similar to those in deterministic models.
Benefits of using stochastic optimization models include:
- More robust decision-making in the face of uncertainty.
- Improved risk management by evaluating potential outcomes.
- Enhanced ability to adapt to changing conditions.
Rapid Innovation employs stochastic optimization techniques to help clients navigate uncertainty, ensuring that their strategies are resilient and adaptable to market fluctuations.
6.4. Genetic and Evolutionary Algorithms
Genetic and evolutionary algorithms are optimization techniques inspired by the principles of natural selection and genetics. These algorithms are particularly effective for solving complex problems where traditional optimization methods may struggle. Genetic algorithms (GAs) use a population of potential solutions that evolve over time through processes analogous to natural selection, such as selection, crossover, and mutation. Evolutionary algorithms (EAs) encompass a broader range of techniques, including genetic algorithms, but also include other strategies inspired by biological evolution.
Key features of genetic and evolutionary algorithms include:
- Population-based search: Solutions are evaluated as a group, allowing for exploration of multiple areas of the solution space simultaneously.
- Fitness function: This measures how well a solution meets the optimization criteria, guiding the selection process.
- Iterative improvement: Solutions evolve over generations, gradually improving towards optimal or near-optimal solutions.
Advantages of using genetic and evolutionary algorithms include:
- Ability to handle complex, multi-dimensional optimization problems.
- Flexibility to adapt to various types of objective functions and constraints.
- Robustness against local optima, as the population-based approach allows for exploration beyond immediate neighbors.
These algorithms have applications in various fields, including engineering design, scheduling, and machine learning, making them a valuable tool for tackling complex optimization challenges. Rapid Innovation harnesses these advanced algorithms to deliver innovative solutions that drive efficiency and maximize ROI for our clients. The resource allocation optimization python tools can also be utilized to implement these algorithms effectively.
6.5. Quantum-Inspired Optimization
Quantum-inspired optimization refers to algorithms and techniques that draw inspiration from quantum computing principles to solve complex optimization problems. These methods leverage the unique properties of quantum mechanics, such as superposition and entanglement, to enhance traditional optimization techniques.
- Key Features: Â
- Utilizes quantum principles to improve search efficiency.
- Can handle large solution spaces more effectively than classical methods.
- Often combines classical algorithms with quantum-inspired heuristics.
- Applications: Â
- Supply chain optimization: Enhances logistics and inventory management, allowing businesses to reduce costs and improve delivery times.
- Financial modeling: Improves portfolio optimization and risk assessment, enabling firms to make more informed investment decisions.
- Machine learning: Aids in feature selection and hyperparameter tuning, leading to more accurate predictive models.
- Popular Algorithms: Â
- Quantum-inspired evolutionary algorithms: Mimic natural selection processes with quantum principles to find optimal solutions efficiently.
- Quantum annealing: Focuses on finding the global minimum of a function by exploring multiple states simultaneously, which can significantly reduce computation time.
- Benefits: Â
- Faster convergence to optimal solutions, allowing businesses to implement strategies more quickly.
- Ability to escape local minima more effectively, ensuring that the best possible solutions are identified.
- Improved performance in high-dimensional spaces, making it suitable for complex real-world problems.
6.6. Swarm Intelligence Approaches
Swarm intelligence is a collective behavior exhibited by decentralized systems, often inspired by the social behavior of animals such as birds, fish, and insects. These approaches are used to solve optimization problems by simulating the interactions of individuals within a group.
- Key Features: Â
- Emphasizes collaboration and communication among agents, leading to more robust solutions.
- Adapts to dynamic environments and changing conditions, making it suitable for real-time applications.
- Utilizes simple rules to achieve complex behaviors, which can simplify the implementation process.
- Common Algorithms: Â
- Particle Swarm Optimization (PSO): Models the social behavior of birds to find optimal solutions, often used in engineering and logistics.
- Ant Colony Optimization (ACO): Simulates the foraging behavior of ants to solve routing and scheduling problems, enhancing operational efficiency.
- Bee Algorithm: Inspired by the foraging patterns of honeybees to optimize resource allocation, applicable in various industries.
- Applications: Â
- Robotics: Enhances pathfinding and coordination among multiple robots, improving automation processes.
- Telecommunications: Optimizes network routing and resource allocation, leading to better service delivery.
- Data mining: Improves clustering and classification tasks, enabling businesses to extract valuable insights from large datasets.
- Benefits: Â
- Robustness to changes in the environment, ensuring consistent performance.
- Scalability to large problem sizes, making it suitable for enterprise-level applications.
- Flexibility in adapting to various optimization scenarios, allowing for tailored solutions.
7. Implementation Strategies

‍
Implementing optimization techniques, whether quantum-inspired optimization or swarm intelligence-based, requires a structured approach to ensure effectiveness and efficiency. Here are some strategies to consider:
- Define Clear Objectives: Â
- Establish specific goals for the optimization process.
- Identify key performance indicators (KPIs) to measure success.
- Select Appropriate Algorithms: Â
- Choose algorithms based on the problem characteristics and constraints.
- Consider hybrid approaches that combine multiple techniques for better results.
- Data Preparation: Â
- Ensure data quality and relevance for the optimization task.
- Preprocess data to remove noise and outliers.
- Parameter Tuning: Â
- Optimize algorithm parameters to enhance performance.
- Use techniques like grid search or random search for effective tuning.
- Testing and Validation: Â
- Implement a robust testing framework to evaluate algorithm performance.
- Validate results against known benchmarks or real-world scenarios.
- Iterative Improvement: Â
- Continuously monitor and refine the optimization process.
- Incorporate feedback loops to adapt to changing conditions and improve outcomes.
- Collaboration and Knowledge Sharing: Â
- Foster collaboration among team members to leverage diverse expertise.
- Share insights and best practices to enhance the overall implementation strategy.
By following these implementation strategies, organizations can effectively harness the power of quantum-inspired optimization and swarm intelligence approaches to solve complex problems and drive innovation. At Rapid Innovation, we specialize in integrating these advanced techniques into your business processes, ensuring that you achieve greater ROI and stay ahead in a competitive landscape. For more information on our services, including transformer model development, please visit our website.
7.1. Data Preparation and Preprocessing
Data preparation and preprocessing are critical steps in the machine learning pipeline. They ensure that the data is clean, relevant, and formatted correctly for model training. This phase can significantly impact the performance of the model.
- Data Cleaning: Â
- Remove duplicates and irrelevant data points.
- Handle missing values through imputation or removal.
- Correct inconsistencies in data formats (e.g., date formats, categorical values).
- Data Transformation: Â
- Normalize or standardize numerical features to bring them to a common scale.
- Encode categorical variables using techniques like one-hot encoding or label encoding.
- Apply feature engineering to create new features that can enhance model performance.
- Data Splitting: Â
- Divide the dataset into training, validation, and test sets to evaluate model performance accurately.
- Use techniques like stratified sampling to maintain the distribution of classes in each subset.
- Exploratory Data Analysis (EDA): Â
- Visualize data distributions and relationships using graphs and charts.
- Identify patterns, trends, and outliers that may influence model training.
Effective data preparation and preprocessing can lead to improved model accuracy and reliability. According to research, up to 80% of the time spent on a machine learning project is dedicated to data preparation, including data preparation for machine learning in Python and data preparation techniques in machine learning. At Rapid Innovation, we leverage our expertise in data preprocessing to ensure that our clients' models are built on a solid foundation, ultimately leading to greater ROI through enhanced model performance. For more detailed guidance, check out our step-by-step tech guide.
7.2. Model Training Methodologies
Model training methodologies refer to the various approaches and techniques used to train machine learning models. Selecting the right methodology is crucial for achieving optimal performance.
- Supervised Learning: Â
- Involves training a model on labeled data, where the input-output pairs are known. Common algorithms include linear regression, decision trees, and support vector machines.
- Unsupervised Learning: Â
- Used when the data is unlabeled, focusing on finding hidden patterns or intrinsic structures. Techniques include clustering (e.g., K-means) and dimensionality reduction (e.g., PCA).
- Semi-Supervised Learning: Â
- Combines both labeled and unlabeled data for training, often leading to better performance with less labeled data. This approach is useful in scenarios where labeling data is expensive or time-consuming.
- Reinforcement Learning: Â
- Involves training agents to make decisions by rewarding them for correct actions and penalizing them for incorrect ones. This methodology is commonly used in robotics, gaming, and autonomous systems.
- Transfer Learning: Â
- Utilizes a pre-trained model on a related task and fine-tunes it for a specific application. This approach can save time and resources, especially in deep learning.
Choosing the right model training methodology can significantly affect the model's ability to generalize to new data. Studies show that models trained with appropriate methodologies can achieve accuracy improvements of up to 30%. At Rapid Innovation, we guide our clients in selecting the most suitable training methodologies, ensuring that they maximize their investment in AI technologies.
7.3. Deployment Frameworks
Deployment frameworks are essential for operationalizing machine learning models, allowing them to be integrated into production environments. A well-structured deployment framework ensures that models can be accessed, monitored, and updated efficiently.
- Containerization: Â
- Tools like Docker allow models to be packaged with their dependencies, ensuring consistency across different environments. This facilitates easy scaling and management of resources.
- Model Serving: Â
- Frameworks such as TensorFlow Serving and TorchServe provide APIs for serving models in real-time. These frameworks support versioning, allowing for seamless updates and rollbacks.
- Cloud Platforms: Â
- Services like AWS SageMaker, Google AI Platform, and Azure Machine Learning offer comprehensive solutions for deploying models. They provide built-in tools for monitoring, scaling, and managing machine learning workflows.
- Batch vs. Real-Time Inference: Â
- Determine whether the model will be used for batch processing (processing large volumes of data at once) or real-time inference (providing immediate predictions). Choose deployment strategies accordingly to optimize performance.
- Monitoring and Maintenance: Â
- Implement monitoring tools to track model performance and detect drift over time. Regularly update models with new data to maintain accuracy and relevance.
Effective deployment frameworks can enhance the accessibility and usability of machine learning models, leading to better business outcomes. According to industry reports, organizations that implement robust deployment strategies can see a 20% increase in operational efficiency. Rapid Innovation specializes in creating tailored deployment frameworks that align with our clients' specific needs, ensuring that they achieve optimal results from their AI investments, including data preparation for deep learning and preparing dataset for machine learning.
7.4. Continuous Learning Mechanisms
Continuous learning mechanisms are essential for organizations to adapt and thrive in a rapidly changing environment. These mechanisms ensure that employees and systems are always updated with the latest knowledge and skills.
- Emphasizes the importance of lifelong learning in the workplace.
- Incorporates various learning methods, such as:
- Online courses and webinars
- Workshops and seminars
- Peer-to-peer learning and mentorship programs
- Utilizes technology to facilitate learning:
- Learning Management Systems (LMS) for tracking progress
- Mobile learning applications for on-the-go access
- Encourages a culture of feedback where employees can learn from their experiences and mistakes.
- Supports knowledge sharing through collaborative platforms and communities of practice.
- Regularly assesses learning needs to ensure relevance and effectiveness.
- Integrates learning into performance reviews to align personal development with organizational goals.
At Rapid Innovation, we leverage AI-driven learning platforms to personalize the learning experience for employees, ensuring that they acquire the skills necessary to meet evolving business demands. By implementing these continuous learning mechanisms, we help organizations enhance employee engagement and productivity, ultimately leading to greater ROI. Additionally, we recognize the significance of integrating AI ethics into our learning frameworks to foster responsible innovation.
7.5. Performance Monitoring
Performance monitoring is a critical component of organizational success, allowing businesses to track progress, identify areas for improvement, and ensure accountability.
- Establishes clear performance metrics and KPIs (Key Performance Indicators) to measure success.
- Utilizes various tools and techniques for effective monitoring:
- Dashboards for real-time data visualization
- Regular performance reviews and check-ins
- Employee self-assessments to encourage ownership of performance
- Encourages a data-driven approach to decision-making, using analytics to inform strategies.
- Provides timely feedback to employees, fostering a culture of continuous improvement.
- Aligns individual performance with organizational objectives to ensure everyone is working towards common goals.
- Identifies trends and patterns in performance data to anticipate challenges and opportunities.
- Engages employees in the monitoring process, promoting transparency and collaboration.
Rapid Innovation employs advanced analytics and AI tools to enhance performance monitoring, enabling organizations to make informed decisions based on real-time data. This proactive approach not only improves operational efficiency but also maximizes ROI by aligning resources with strategic objectives.
7.6. Ethical Considerations and Bias Mitigation
Ethical considerations and bias mitigation are crucial in today’s diverse workplace. Organizations must prioritize fairness and integrity in their practices to build trust and foster an inclusive environment.
- Recognizes the importance of ethical behavior in decision-making processes.
- Implements policies and training programs to raise awareness about ethical standards and practices.
- Conducts regular audits to identify and address potential biases in hiring, promotions, and evaluations.
- Utilizes diverse teams to minimize groupthink and enhance creativity.
- Encourages open dialogue about ethical dilemmas and bias, creating a safe space for discussion.
- Leverages technology to reduce bias in recruitment and performance assessments, such as using AI tools that are designed to be fair.
- Establishes clear reporting mechanisms for unethical behavior, ensuring accountability and transparency.
- Promotes diversity and inclusion initiatives to create a more equitable workplace.
At Rapid Innovation, we understand the importance of ethical practices in AI and Blockchain development. By implementing robust ethical frameworks and bias mitigation strategies, we help organizations build trust with their stakeholders, ultimately leading to enhanced brand reputation and increased ROI.
8. Performance Evaluation and Metrics
Performance evaluation and metrics are essential components in assessing the effectiveness of processes, systems, or individuals within an organization. By establishing clear metrics, organizations can identify areas for improvement, track progress, and ensure that goals are met efficiently. At Rapid Innovation, we leverage AI and Blockchain technologies to enhance these evaluations, providing clients with data-driven insights that lead to informed decision-making.
8.1 Efficiency Measurement
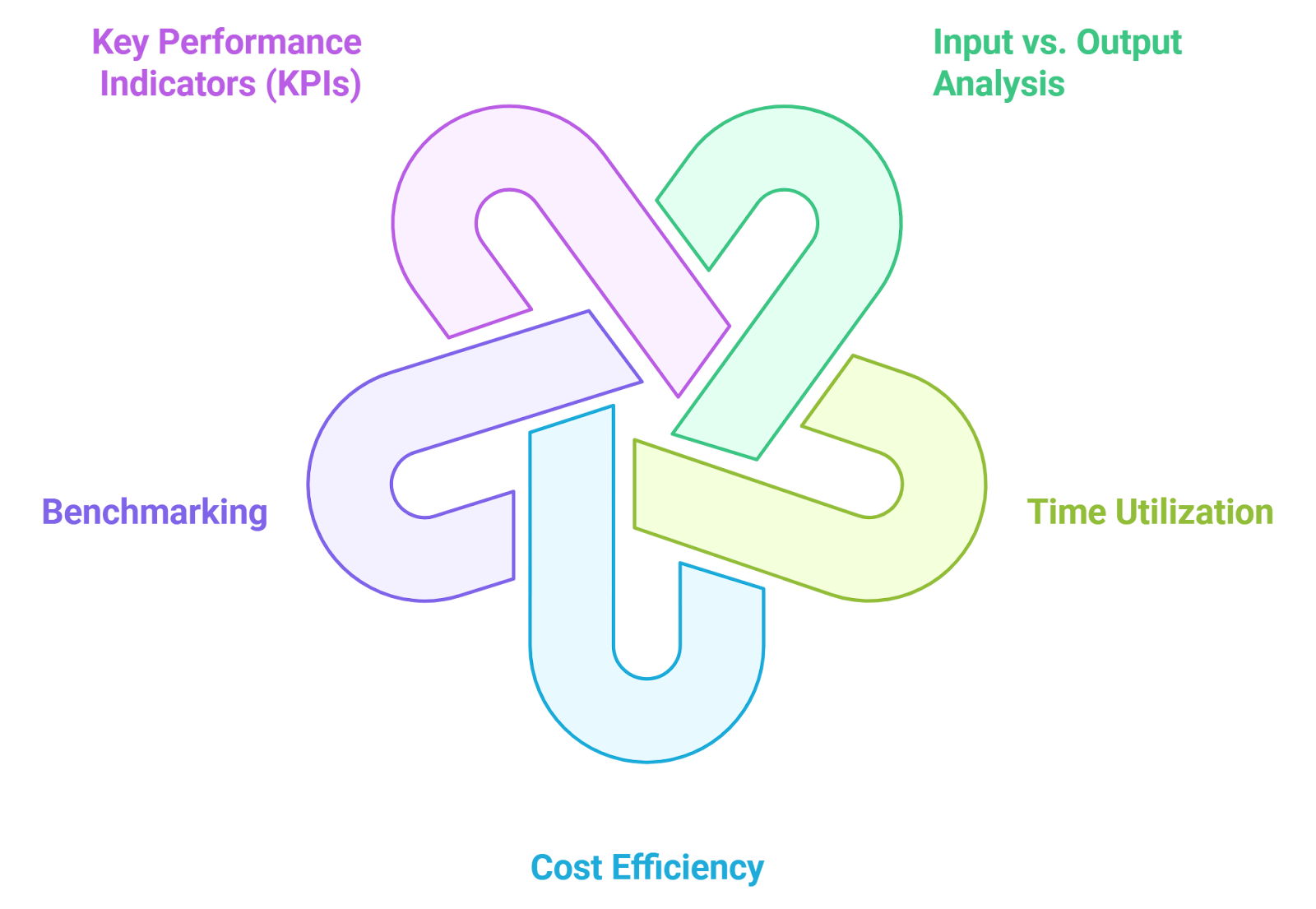
‍
Efficiency measurement focuses on how well resources are utilized to achieve desired outcomes. It helps organizations determine whether they are maximizing their output while minimizing input. Key aspects of efficiency measurement include:
- Input vs. Output Analysis: This involves comparing the resources used (input) to the results achieved (output). A high output-to-input ratio indicates greater efficiency. Rapid Innovation employs AI algorithms to analyze large datasets, enabling clients to identify inefficiencies and optimize resource allocation.
- Time Utilization: Measuring how time is spent on various tasks can reveal inefficiencies. Tools like time tracking software can help in identifying time wastage. Our AI-driven solutions can automate time tracking and provide real-time insights into task performance.
- Cost Efficiency: Analyzing costs associated with processes can highlight areas where expenses can be reduced without sacrificing quality. This includes evaluating labor costs, material costs, and overheads. By integrating Blockchain for transparent cost tracking, we help clients achieve greater financial clarity and control.
- Benchmarking: Comparing performance metrics against industry standards or competitors can provide insights into where improvements are needed. This can help organizations set realistic goals and identify best practices. Our consulting services include competitive analysis powered by AI, allowing clients to benchmark effectively.
- Key Performance Indicators (KPIs): Establishing specific KPIs related to efficiency, such as production rates, cycle times, and resource utilization rates, allows organizations to monitor performance closely. Rapid Innovation assists clients in defining and tracking KPIs through customized dashboards that utilize AI for predictive analytics. This includes sales performance evaluation and kpi evaluation to ensure comprehensive assessments.
By focusing on these aspects, organizations can enhance their operational efficiency, leading to better resource management and increased profitability.
8.2 Optimization Quality Indicators
Optimization quality indicators are metrics that assess the effectiveness of optimization efforts within an organization. These indicators help determine how well processes have been improved and whether the desired outcomes have been achieved. Important optimization quality indicators include:
- Quality of Output: This measures the standard of the final product or service. High-quality output indicates successful optimization, while defects or errors suggest areas needing further improvement. Our AI solutions can analyze product quality in real-time, ensuring that standards are consistently met.
- Customer Satisfaction: Feedback from customers can serve as a critical indicator of optimization success. High satisfaction rates often correlate with effective process improvements. We utilize AI-driven sentiment analysis to gauge customer feedback and enhance service delivery.
- Process Cycle Time: This metric tracks the time taken to complete a process from start to finish. A reduction in cycle time often indicates successful optimization efforts. Rapid Innovation employs AI to streamline processes, significantly reducing cycle times for our clients.
- Return on Investment (ROI): Evaluating the financial return from optimization initiatives helps organizations understand the value generated from their investments. A positive ROI signifies effective optimization. Our Blockchain solutions provide transparent tracking of investments and returns, ensuring clients can measure their ROI accurately.
- Resource Utilization Rates: Monitoring how effectively resources are used post-optimization can indicate the success of the changes implemented. Higher utilization rates often reflect better optimization. We help clients implement AI tools that provide insights into resource usage, enabling continuous improvement.
By focusing on these optimization quality indicators, organizations can ensure that their efforts lead to tangible improvements, ultimately enhancing overall performance and competitiveness. Rapid Innovation is committed to helping clients achieve these goals through our expertise in AI and Blockchain technologies, including performance evaluation metrics and performance appraisal metrics to guide their strategies.
8.3. Computational Overhead Analysis
Computational overhead refers to the additional computational resources required to execute a particular algorithm or process beyond the basic operations. Understanding computational overhead is crucial for optimizing performance in various applications, especially in fields like data science, machine learning, and software development.
- Definition: Computational overhead includes factors such as memory usage, processing time, and energy consumption.
- Importance: High overhead can lead to slower performance and increased costs, making it essential to analyze and minimize it.
- Measurement: Overhead can be measured using profiling tools that track resource usage during execution.
- Factors Influencing Overhead: Â
- Algorithm complexity: More complex algorithms typically incur higher overhead.
- Data size: Larger datasets can increase memory and processing requirements.
- Hardware limitations: The capabilities of the hardware can significantly impact overhead.
- Strategies to Reduce Overhead: Â
- Optimize algorithms: Use more efficient algorithms to reduce processing time, which can lead to significant cost savings and improved performance.
- Parallel processing: Distributing tasks across multiple processors can lower execution time, enhancing throughput and responsiveness.
- Resource management: Efficiently managing memory and storage can minimize overhead, allowing for better utilization of existing resources. For expert assistance in project estimation, consider our project estimation services and learn about successful AI integration strategies.
8.4. Comparative Benchmarking
Comparative benchmarking is the process of evaluating the performance of different systems, algorithms, or processes against each other. This analysis helps identify strengths and weaknesses, guiding improvements and decision-making.
- Purpose: The primary goal of benchmarking is to assess performance metrics such as speed, accuracy, and resource utilization.
- Types of Benchmarking: Â
- Performance benchmarking: Focuses on speed and efficiency.
- Functional benchmarking: Evaluates the functionality and features of systems.
- Cost benchmarking: Compares the cost-effectiveness of different solutions.
- Key Metrics to Consider: Â
- Execution time: How long it takes to complete a task.
- Throughput: The amount of work completed in a given time frame.
- Latency: The delay before a transfer of data begins following an instruction.
- Tools for Benchmarking: Â
- Benchmarking frameworks: Tools like Apache JMeter or SPEC can help automate the benchmarking process.
- Custom scripts: Writing tailored scripts can provide specific insights into performance.
- Benefits of Comparative Benchmarking: Â
- Identifies best practices: Helps organizations learn from top performers, enabling them to adopt strategies that yield higher returns on investment.
- Informs decision-making: Provides data-driven insights for selecting technologies or strategies, ensuring that investments align with business goals.
- Drives continuous improvement: Encourages ongoing evaluation and enhancement of processes, fostering a culture of innovation.
8.5. Long-term Impact Assessment
Long-term impact assessment involves evaluating the sustained effects of a project, technology, or policy over an extended period. This analysis is essential for understanding the broader implications and ensuring that initiatives align with strategic goals.
- Definition: Long-term impact assessment examines the enduring consequences of actions taken today.
- Importance: Understanding long-term impacts helps organizations make informed decisions that consider future sustainability.
- Key Areas of Focus: Â
- Economic impact: Evaluates how initiatives affect financial performance and economic growth.
- Social impact: Assesses changes in community well-being, equity, and quality of life.
- Environmental impact: Analyzes effects on natural resources, ecosystems, and climate.
- Methodologies for Assessment: Â
- Longitudinal studies: Collect data over time to observe trends and changes.
- Cost-benefit analysis: Weighs the costs of an initiative against its long-term benefits, ensuring that investments yield sustainable returns.
- Stakeholder feedback: Engaging with stakeholders can provide insights into perceived impacts, enhancing transparency and accountability.
- Challenges in Long-term Assessment: Â
- Data availability: Long-term data can be difficult to obtain and analyze.
- Uncertainty: Predicting future impacts involves inherent uncertainties.
- Complexity: The interconnectedness of various factors can complicate assessments.
- Benefits of Long-term Impact Assessment: Â
- Informed decision-making: Provides a comprehensive view of potential outcomes, allowing organizations to align their strategies with long-term objectives.
- Enhanced accountability: Encourages organizations to consider the long-term effects of their actions, fostering trust among stakeholders.
- Strategic planning: Supports the development of sustainable strategies that benefit future generations, ensuring that organizations remain competitive in a rapidly evolving landscape.
9. Challenges and Limitations
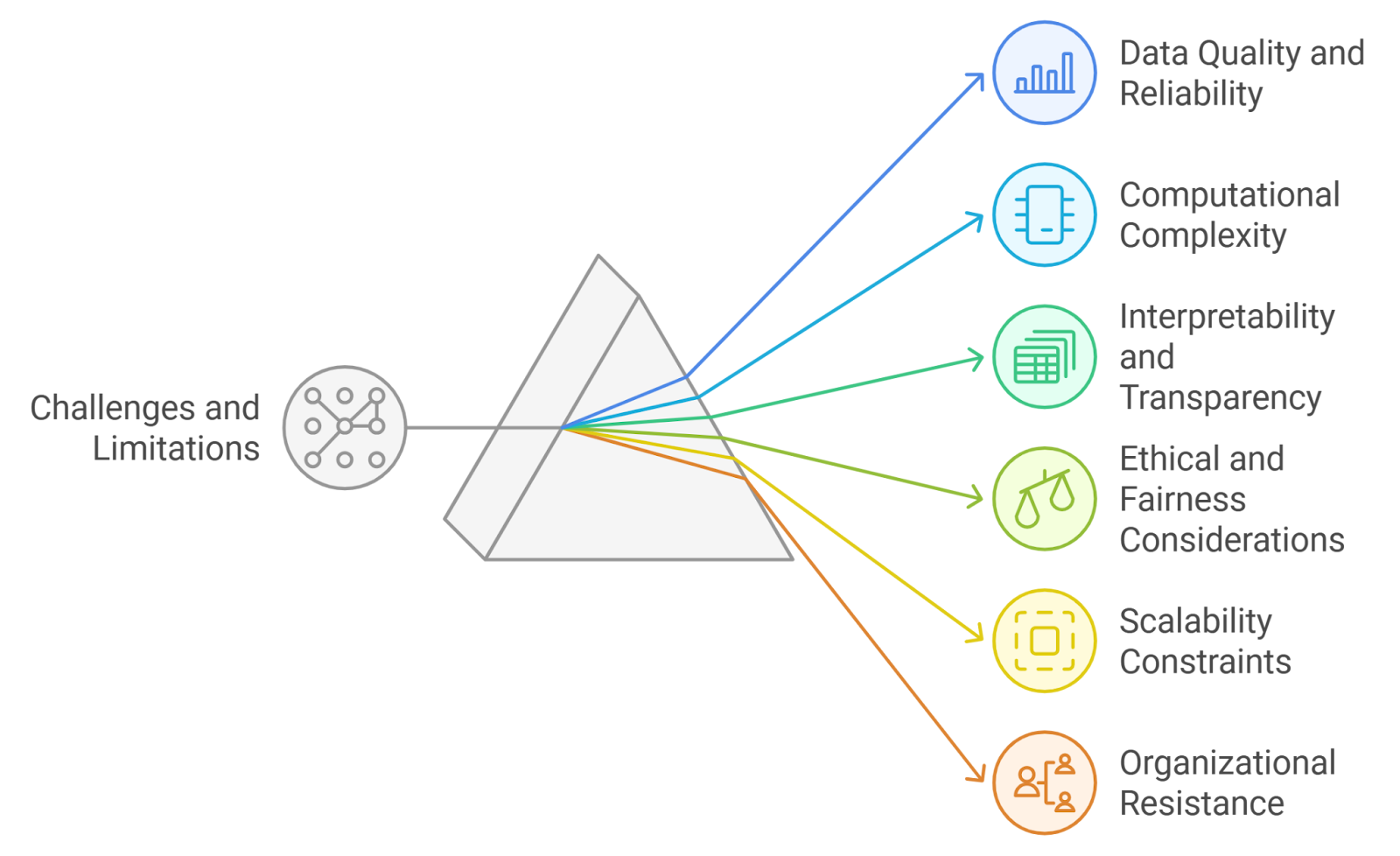
‍
In any field, challenges and limitations can hinder progress and effectiveness. In the context of data analysis, machine learning, and artificial intelligence, understanding these challenges is crucial for developing robust solutions.
9.1 Data Quality and Reliability
Data quality and reliability are fundamental to the success of any analytical model or machine learning algorithm. Poor data quality can lead to inaccurate results, which can have significant consequences, highlighting the data quality challenges faced in various domains.
Inaccurate data may contain errors due to human input, sensor malfunctions, or outdated information, skewing results and leading to incorrect conclusions. Incomplete data can create gaps in analysis, making it difficult to draw reliable insights; techniques like imputation can help, but they may introduce bias. Additionally, inconsistent data collected from different sources may not follow the same format or standards, leading to inconsistencies that complicate analysis, which are common challenges of data quality.
Bias in data is another critical issue; if the data used for training models is biased, the resulting models will also be biased, perpetuating stereotypes and leading to unfair outcomes in applications like hiring or lending. Furthermore, the sheer volume of data can overwhelm systems, making it challenging to ensure quality and reliability. Large datasets require robust validation processes to maintain integrity, especially when considering data quality challenges in data warehouses.
At Rapid Innovation, we emphasize the importance of high data quality by implementing rigorous data governance practices, including regular audits, validation checks, and employing advanced data cleaning techniques. This ensures that our clients can rely on accurate insights to drive their business decisions, ultimately leading to greater ROI. We also recognize the list and describe the most common data quality problems to better address these issues.
9.2 Computational Complexity
Computational complexity refers to the amount of computational resources required to process data and run algorithms. As models become more sophisticated, the complexity can increase significantly.
Advanced algorithms, especially in deep learning, are resource-intensive and require substantial computational power, which can lead to increased costs for hardware and energy consumption. Additionally, complex models can take a long time to train, especially with large datasets, delaying project timelines and hindering real-time applications.
As data grows, scalability issues arise; algorithms that work well on small datasets may struggle with larger volumes, necessitating optimization. Choosing the right algorithm is crucial, as some may be more computationally efficient than others but may sacrifice accuracy or interpretability. Moreover, complex models can easily overfit the training data, capturing noise rather than the underlying patterns, which can lead to poor performance on unseen data.
At Rapid Innovation, we address computational complexity by optimizing algorithms, utilizing parallel processing, and leveraging cloud computing resources to scale operations effectively. This approach not only enhances performance but also ensures that our clients can achieve their business goals efficiently, maximizing their return on investment. For those interested in advanced solutions, our Stable Diffusion Development services can provide tailored support to navigate these challenges.
9.3. Interpretability and Transparency
Interpretability and transparency are crucial aspects of artificial intelligence (AI) and machine learning (ML) systems. As these technologies become more integrated into decision-making processes, understanding how they arrive at conclusions is essential for trust and accountability.
- Interpretability refers to the degree to which a human can understand the cause of a decision made by an AI system.
- Transparency involves making the workings of AI systems clear and accessible to users and stakeholders.
- High interpretability can lead to better user trust, as stakeholders can see how decisions are made.
- Techniques such as model-agnostic methods, feature importance scores, and visualizations can enhance interpretability.
- Regulatory bodies are increasingly emphasizing the need for transparency in AI systems to ensure compliance with ethical standards.
The lack of interpretability can lead to significant issues, including misunderstandings of AI decisions, which can result in poor decision-making, difficulty in identifying biases within the model leading to unfair outcomes, and challenges in debugging and improving AI systems, as developers may not understand why a model fails. At Rapid Innovation, we prioritize ethical AI development in our AI solutions, ensuring that our clients can trust the systems we develop and deploy.
9.4. Ethical and Fairness Considerations
Ethical and fairness considerations are paramount in the development and deployment of AI systems. As AI technologies influence various sectors, ensuring that they operate fairly and ethically is essential to prevent harm and discrimination.
- AI systems can inadvertently perpetuate or amplify existing biases present in training data.
- Fairness in AI involves ensuring that decisions made by algorithms do not discriminate against individuals based on race, gender, age, or other protected characteristics.
- Ethical considerations include the potential for AI to invade privacy, manipulate behavior, or make life-altering decisions without human oversight.
Key strategies to address ethical and fairness concerns include implementing bias detection and mitigation techniques during the model training process, engaging diverse teams in the development of AI systems to bring multiple perspectives and reduce blind spots, and establishing clear guidelines and frameworks for ethical AI use, including accountability measures for AI-driven decisions. At Rapid Innovation, we are committed to embedding ethical practices into our AI solutions, helping our clients build trust with their users and stakeholders.
Organizations must prioritize ethical considerations to build trust with users and stakeholders, ensuring that AI technologies contribute positively to society.
9.5. Scalability Constraints
Scalability constraints refer to the challenges faced by AI systems when attempting to handle increasing amounts of data or user demands. As organizations grow, their AI solutions must be able to scale effectively to meet new requirements.
- Scalability can be affected by various factors, including computational resources, data storage, and algorithm efficiency.
- Many AI models require significant computational power, which can become a bottleneck as data volumes increase.
- Data management becomes increasingly complex as organizations scale, necessitating robust data pipelines and storage solutions.
To address scalability constraints, organizations can consider leveraging cloud computing resources to provide flexible and scalable infrastructure, utilizing distributed computing frameworks that allow for parallel processing of large datasets, and optimizing algorithms for efficiency, ensuring they can handle larger datasets without a proportional increase in resource consumption. Rapid Innovation offers tailored solutions that enhance scalability, ensuring that our clients' AI systems remain effective and responsive as they grow, ultimately enhancing their operational capabilities.
9.6. Organizational Resistance
Organizational resistance refers to the pushback or reluctance that employees and management may exhibit when faced with changes within an organization. This resistance can stem from various factors, including fear of the unknown, loss of control, or perceived threats to job security. Understanding and addressing these factors is crucial for successful change management, particularly in the context of managing change management and organizational change management.
- Fear of Change: Employees may fear how changes will affect their roles, responsibilities, and job security. This fear can lead to anxiety and decreased morale, especially in situations involving organizational change and change management.
- Lack of Trust: If employees do not trust leadership or the change process, they are more likely to resist. Building trust through transparent communication is essential in managing change organization-wide.
- Cultural Factors: An organization's culture can significantly influence resistance. A culture that values stability may resist changes more than one that encourages innovation, which is often seen in organizational change and culture.
- Inadequate Communication: Poor communication about the reasons for change and its benefits can lead to misunderstandings and resistance. Clear, consistent messaging is vital in organizational change and change management.
- Training and Support: Employees may resist changes if they feel unprepared. Providing adequate training and support can help ease the transition, particularly in managing change strategies.
- Involvement in the Process: Engaging employees in the change process can reduce resistance. When employees feel they have a voice, they are more likely to embrace change, which is a key aspect of organizational change management.
Addressing organizational resistance requires a strategic approach that includes:
- Identifying the sources of resistance through surveys or focus groups.
- Developing a change management plan that includes communication strategies.
- Providing training and resources to help employees adapt, especially in the context of managing organizational change.
- Encouraging feedback and making adjustments based on employee input.
10. Advanced Scenarios and Use Cases
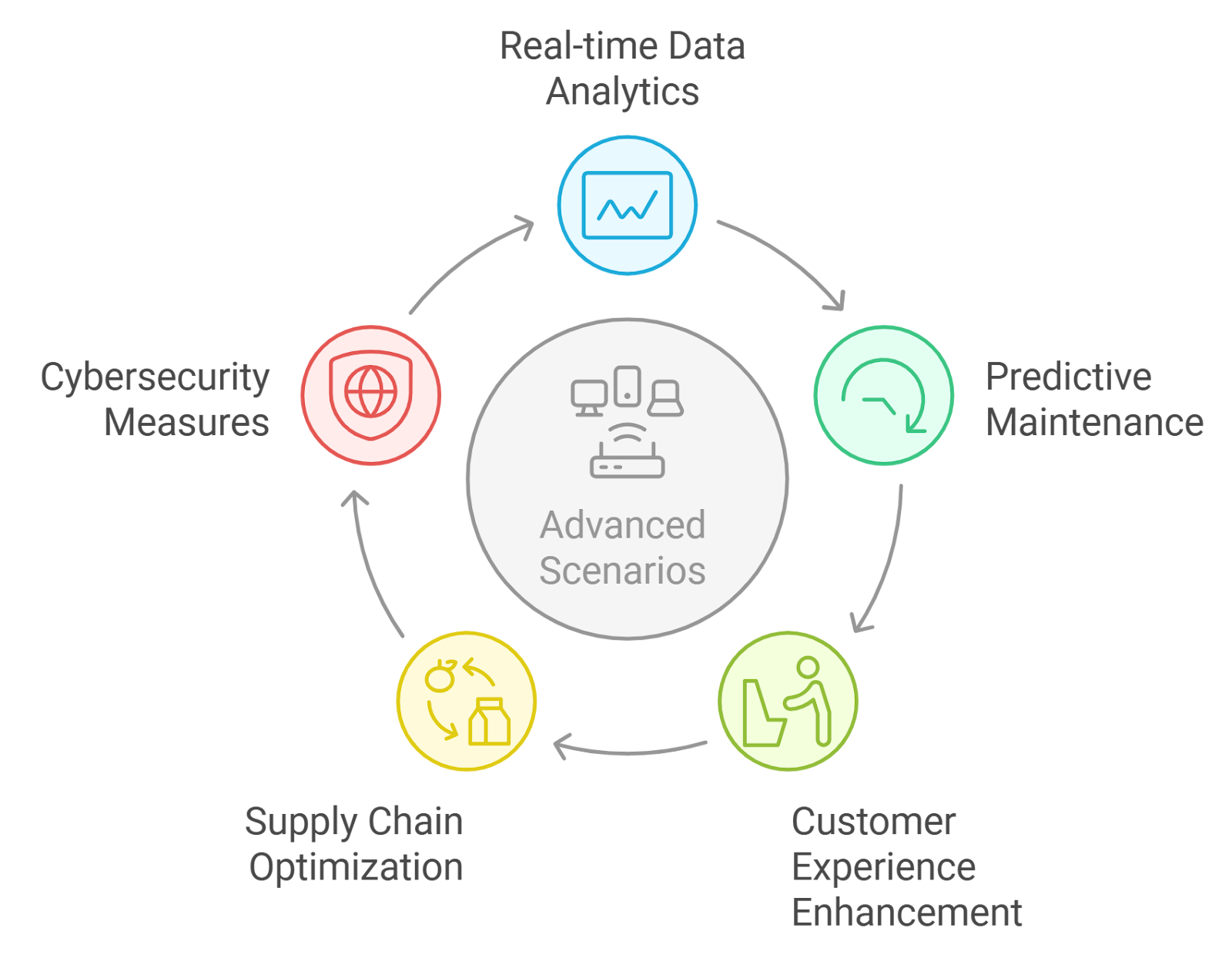
‍
Advanced scenarios and use cases refer to complex situations where innovative solutions are applied to solve specific problems or enhance processes. These scenarios often involve the integration of advanced technologies and methodologies to achieve desired outcomes.
- Real-time Data Analytics: Organizations can leverage real-time data analytics to make informed decisions quickly. This is particularly useful in industries like finance and healthcare, where timely information is critical.
- Predictive Maintenance: In manufacturing, predictive maintenance uses data analytics to predict equipment failures before they occur, reducing downtime and maintenance costs.
- Customer Experience Enhancement: Companies can use advanced scenarios to personalize customer experiences through data-driven insights, improving satisfaction and loyalty.
- Supply Chain Optimization: Advanced use cases in supply chain management can involve AI and machine learning to forecast demand and optimize inventory levels, leading to cost savings and efficiency.
- Cybersecurity Measures: Organizations can implement advanced cybersecurity measures, such as AI-driven threat detection, to protect sensitive data and systems from breaches.
These advanced scenarios require a thorough understanding of the technologies involved and the specific challenges faced by the organization. Successful implementation often involves collaboration across departments and a commitment to continuous improvement.
10.1. Autonomous Resource Management
Autonomous resource management refers to the use of advanced technologies, such as artificial intelligence (AI) and machine learning, to automate the management of resources within an organization. This approach aims to optimize resource allocation, reduce costs, and improve efficiency.
- AI-Driven Decision Making: Autonomous systems can analyze vast amounts of data to make real-time decisions about resource allocation, ensuring that resources are used effectively.
- Predictive Analytics: By utilizing predictive analytics, organizations can forecast resource needs based on historical data and trends, allowing for proactive management.
- Self-Optimizing Systems: Autonomous resource management systems can continuously learn and adapt to changing conditions, optimizing resource use without human intervention.
- Cost Reduction: Automating resource management can lead to significant cost savings by minimizing waste and improving operational efficiency.
- Enhanced Agility: Organizations can respond more quickly to changes in demand or market conditions, as autonomous systems can adjust resource allocation in real-time.
- Improved Accuracy: Reducing human error in resource management leads to more accurate forecasting and allocation, enhancing overall performance.
Implementing autonomous resource management requires:
- Investment in technology and infrastructure to support AI and machine learning capabilities.
- Training for employees to work alongside autonomous systems effectively.
- Continuous monitoring and evaluation to ensure systems are functioning as intended and delivering the desired outcomes.
By embracing autonomous resource management, organizations can position themselves for success in an increasingly competitive landscape. Rapid Innovation is here to guide you through these transformations, ensuring that your organization not only adapts but thrives in the face of change. Our expertise in AI and Blockchain technologies can help you streamline processes, enhance decision-making, and ultimately achieve greater ROI.
10.2. Cross-Domain Allocation
Cross-domain allocation refers to the strategic distribution of resources, tasks, or responsibilities across different domains or sectors. This approach is increasingly relevant in today's interconnected world, where collaboration between various fields can lead to enhanced efficiency and innovation.
- Facilitates collaboration between different industries, such as healthcare, finance, and technology. Â
- Encourages the sharing of best practices and knowledge, leading to improved outcomes. Â
- Enables organizations to leverage diverse skill sets and expertise, enhancing problem-solving capabilities. Â
- Supports the development of integrated solutions that address complex challenges, such as climate change or public health crises. Â
- Promotes flexibility and adaptability, allowing organizations to respond quickly to changing market demands. Â
At Rapid Innovation, we harness cross-domain allocation to create tailored solutions that drive efficiency and innovation for our clients. For example, by integrating AI and blockchain technologies, we can facilitate secure data sharing between healthcare providers and financial institutions, leading to improved patient outcomes and streamlined operations. This collaborative approach not only enhances resource utilization but also fosters a culture of innovation across sectors. Additionally, our expertise in custom AI model development allows us to create solutions that are specifically designed to meet the unique needs of different industries.
10.3. Real-time Adaptive Systems
Real-time adaptive systems are designed to respond dynamically to changing conditions and inputs. These systems utilize advanced algorithms and data analytics to make immediate adjustments, ensuring optimal performance and efficiency.
- Enhance decision-making by providing timely insights and recommendations based on real-time data. Â
- Improve operational efficiency by automatically adjusting processes in response to fluctuations in demand or resource availability. Â
- Enable organizations to maintain a competitive edge by quickly adapting to market changes or customer preferences. Â
- Support personalized experiences for users, as systems can tailor services or products based on individual behavior and needs. Â
- Foster innovation by allowing organizations to experiment and iterate rapidly, leading to the development of new solutions and services. Â
Industries such as transportation, healthcare, and finance are increasingly adopting real-time adaptive systems to improve service delivery and operational efficiency. For instance, Rapid Innovation has developed smart traffic management systems that utilize real-time data analytics to optimize traffic flow, reducing congestion and improving travel times. By implementing these systems, our clients can achieve significant cost savings and enhance user satisfaction.
10.4. Predictive Maintenance Integration
Predictive maintenance integration involves the use of data analytics and machine learning to anticipate equipment failures before they occur. This proactive approach helps organizations minimize downtime and reduce maintenance costs.
- Utilizes historical data and real-time monitoring to identify patterns and predict potential failures. Â
- Reduces unplanned downtime by scheduling maintenance activities during non-peak hours. Â
- Enhances asset lifespan by addressing issues before they escalate into major problems. Â
- Lowers maintenance costs by optimizing resource allocation and reducing emergency repairs. Â
- Improves safety by ensuring that equipment is functioning correctly and reducing the risk of accidents. Â
Industries such as manufacturing, aviation, and energy are increasingly implementing predictive maintenance strategies. For instance, Rapid Innovation has partnered with airlines to deploy predictive analytics that monitor engine performance, allowing them to schedule maintenance before a failure occurs. This not only ensures safety and operational efficiency but also significantly reduces maintenance costs, leading to a greater return on investment for our clients.
10.5. Self-Optimizing Ecosystems
Self-optimizing ecosystems refer to systems that can autonomously adjust and improve their performance based on real-time data and feedback. These ecosystems are increasingly relevant in various fields, including technology, agriculture, and environmental management.
- Autonomous Adjustments: Self-optimizing ecosystems utilize algorithms and machine learning to analyze data and make decisions without human intervention, leading to enhanced efficiency and productivity. At Rapid Innovation, we leverage these technologies to help clients streamline operations, resulting in significant cost savings and improved ROI.
- Real-Time Data Utilization: By leveraging IoT (Internet of Things) devices, these ecosystems can gather vast amounts of data, which is crucial for understanding system performance and identifying areas for improvement. Our expertise in integrating IoT solutions allows businesses to harness this data effectively, driving informed decision-making.
- Sustainability: Self-optimizing ecosystems contribute to sustainability efforts. For instance, in agriculture, precision farming techniques can optimize water usage and reduce chemical inputs, leading to more sustainable practices. Rapid Innovation assists clients in implementing such solutions, enhancing their sustainability profiles while reducing operational costs.
- Examples in Nature: Natural ecosystems often exhibit self-optimizing characteristics. For example, predator-prey dynamics help maintain balance within an ecosystem, showcasing how systems can self-regulate. Understanding these principles can inspire innovative solutions in various industries.
- Applications in Industry: Industries are adopting self-optimizing ecosystems to enhance operational efficiency. For example, smart manufacturing systems can adjust production processes based on real-time demand and resource availability. Rapid Innovation's development of tailored smart manufacturing solutions enables clients to respond swiftly to market changes, maximizing productivity and profitability.
- Challenges: While promising, self-optimizing ecosystems face challenges such as data privacy concerns, the need for robust cybersecurity measures, and the complexity of integrating various technologies. Rapid Innovation addresses these challenges by implementing comprehensive security protocols and ensuring seamless integration of advanced technologies.
11. Future Trends and Outlook
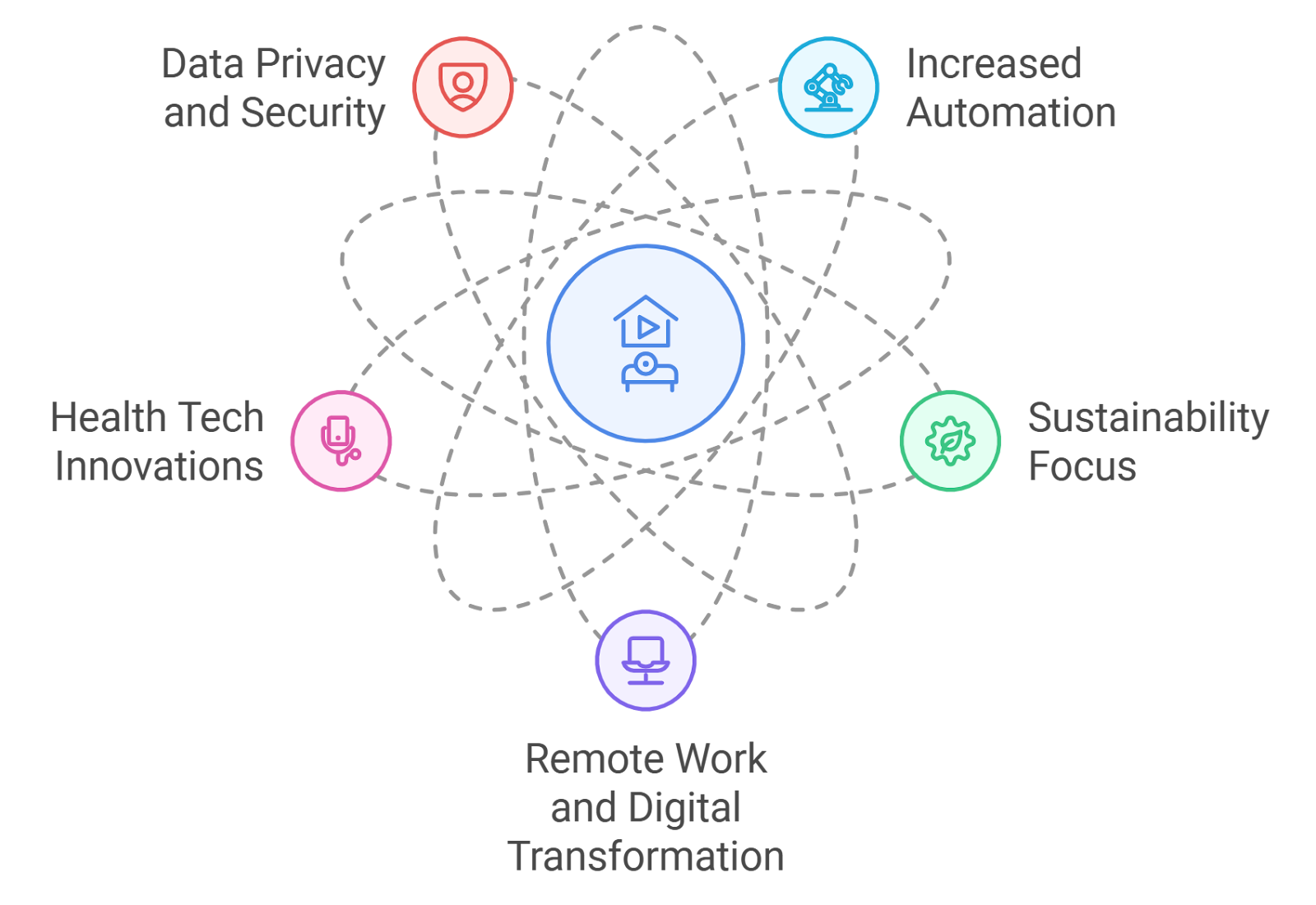
‍
The future of technology and various industries is shaped by emerging trends that promise to revolutionize how we live and work. Understanding these trends is essential for businesses and individuals looking to stay ahead.
- Increased Automation: Automation is expected to continue its rise, with more tasks being performed by machines. This trend will lead to increased efficiency but may also raise concerns about job displacement. Rapid Innovation helps clients navigate this transition by developing automation solutions that enhance workforce capabilities.
- Sustainability Focus: There is a growing emphasis on sustainable practices across industries. Companies are increasingly adopting green technologies and practices to reduce their environmental impact. Our consulting services guide clients in implementing sustainable strategies that align with their business goals.
- Remote Work and Digital Transformation: The shift towards remote work is likely to persist, driving further digital transformation. Businesses will need to invest in technology that supports remote collaboration and productivity. Rapid Innovation provides solutions that facilitate seamless remote operations, ensuring business continuity.
- Health Tech Innovations: The healthcare sector is witnessing rapid advancements in technology, including telemedicine and wearable health devices. These innovations are improving patient care and accessibility. Our expertise in health tech development enables clients to leverage these advancements for better patient outcomes.
- Data Privacy and Security: As technology evolves, so do concerns about data privacy and security. Companies will need to prioritize robust security measures to protect sensitive information. Rapid Innovation emphasizes cybersecurity in all our solutions, ensuring that client data remains secure.
11.1. Emerging AI Technologies
Emerging AI technologies are reshaping industries and enhancing capabilities across various sectors. These advancements are not only improving efficiency but also enabling new possibilities.
- Natural Language Processing (NLP): NLP technologies are becoming more sophisticated, allowing machines to understand and generate human language. This has applications in customer service, content creation, and more. Rapid Innovation develops NLP solutions that enhance customer engagement and streamline communication.
- Computer Vision: AI-driven computer vision technologies are being used in various fields, from healthcare diagnostics to autonomous vehicles. These systems can analyze visual data and make decisions based on it. Our expertise in computer vision allows clients to implement innovative solutions that improve operational efficiency.
- Generative AI: Generative AI is gaining traction, enabling machines to create content, designs, and even music. This technology is transforming creative industries and enhancing productivity. Rapid Innovation helps clients harness generative AI to drive creativity and innovation in their projects.
- AI in Cybersecurity: As cyber threats become more sophisticated, AI is being employed to detect and respond to security breaches in real-time. This proactive approach helps organizations safeguard their data. Our AI-driven cybersecurity solutions provide clients with robust protection against evolving threats.
- Edge AI: Edge AI refers to processing data closer to the source rather than relying on centralized cloud computing. This technology reduces latency and enhances real-time decision-making capabilities. Rapid Innovation integrates edge AI solutions to optimize performance and responsiveness for our clients.
- AI Ethics and Governance: As AI technologies advance, there is a growing focus on ethical considerations and governance. Organizations are developing frameworks to ensure responsible AI use and mitigate biases. Rapid Innovation advocates for ethical AI practices, helping clients establish governance frameworks that align with industry standards.
- Integration with IoT: The convergence of AI and IoT is creating smart environments where devices can communicate and make decisions autonomously. This integration is pivotal for smart cities and industrial automation. Our solutions facilitate this integration, enabling clients to create intelligent ecosystems that enhance operational efficiency.
- Healthcare Applications: AI technologies are revolutionizing healthcare through predictive analytics, personalized medicine, and improved diagnostics. These advancements are enhancing patient outcomes and operational efficiency. Rapid Innovation partners with healthcare organizations to implement AI solutions that drive innovation and improve care delivery.
- Education and Training: AI is being utilized in educational settings to provide personalized learning experiences. Adaptive learning platforms can tailor content to individual student needs, improving engagement and retention. Our educational technology solutions empower institutions to leverage AI for enhanced learning outcomes.
11.2. Edge Computing Integration
Edge computing is a distributed computing paradigm that brings computation and data storage closer to the location where it is needed. This integration is crucial for enhancing the performance of applications, especially those requiring real-time data processing.
- Reduces latency: By processing data near the source, edge computing minimizes the time it takes for data to travel to a centralized cloud server, enabling faster response times for critical applications.
- Bandwidth efficiency: It decreases the amount of data that needs to be sent to the cloud, thus optimizing bandwidth usage and reducing costs associated with data transmission.
- Enhanced security: Sensitive data can be processed locally, reducing the risk of exposure during transmission and ensuring compliance with data protection regulations.
- Scalability: Edge computing allows for the addition of more devices without overwhelming central servers, making it easier to scale operations and accommodate growing data demands.
- IoT applications: It is particularly beneficial for Internet of Things (IoT) devices, which generate massive amounts of data that need immediate processing, thus enabling real-time analytics and decision-making.
The integration of edge computing is transforming industries such as healthcare, manufacturing, and smart cities by enabling faster decision-making and improved operational efficiency. At Rapid Innovation, we leverage edge computing integration to help our clients optimize their operations, reduce costs, and enhance their service delivery, ultimately leading to greater ROI. Our expertise in AI copilot development further enhances our ability to deliver innovative solutions tailored to our clients' needs.
11.3. Quantum Computing Potential
Quantum computing represents a significant leap in computational power, utilizing the principles of quantum mechanics to process information in ways that classical computers cannot.
- Exponential speed: Quantum computers can solve complex problems much faster than traditional computers, potentially reducing computation time from years to seconds, which can significantly accelerate project timelines.
- Enhanced problem-solving: They excel in optimization problems, cryptography, and simulations of molecular structures, which can revolutionize fields like drug discovery and materials science, providing our clients with innovative solutions.
- Quantum algorithms: Algorithms such as Shor's and Grover's demonstrate the potential for quantum computing to outperform classical algorithms in specific tasks, enabling breakthroughs in various sectors.
- Industry impact: Sectors like finance, logistics, and artificial intelligence stand to benefit immensely from quantum computing capabilities, leading to more efficient operations and innovative solutions.
- Research and development: Ongoing investments in quantum technology are expected to yield breakthroughs that could redefine computing paradigms, positioning our clients at the forefront of technological advancements.
The potential of quantum computing is vast, and as research progresses, it may unlock solutions to problems that are currently unsolvable. Rapid Innovation is committed to guiding our clients through the complexities of quantum computing, ensuring they harness its full potential for enhanced business outcomes.
11.4. Explainable AI in Resource Allocation
Explainable AI (XAI) refers to methods and techniques in artificial intelligence that make the decision-making process of AI systems transparent and understandable to humans. This is particularly important in resource allocation, where decisions can significantly impact operations and outcomes.
- Transparency: XAI provides insights into how AI models make decisions, allowing stakeholders to understand the rationale behind resource allocation, which is essential for informed decision-making.
- Trust: By making AI decisions explainable, organizations can build trust among users and stakeholders, which is crucial for widespread adoption and effective implementation of AI solutions.
- Improved decision-making: With clear explanations, decision-makers can assess the effectiveness of resource allocation strategies and make informed adjustments, leading to better resource utilization.
- Compliance: In regulated industries, explainability helps ensure that AI systems comply with legal and ethical standards, reducing the risk of bias and discrimination.
- Enhanced performance: XAI can identify inefficiencies in resource allocation, leading to optimized use of resources and improved overall performance.
Incorporating explainable AI into resource allocation processes not only enhances transparency but also fosters a culture of accountability and continuous improvement. At Rapid Innovation, we prioritize the integration of XAI in our AI solutions, ensuring our clients can leverage AI effectively while maintaining ethical standards and operational excellence.
11.5. Interdisciplinary Convergence
Interdisciplinary convergence refers to the collaboration and integration of knowledge, methods, and insights from different academic disciplines to address complex problems. This approach is increasingly vital in today’s interconnected world, where challenges such as climate change, public health, and technological advancement require multifaceted solutions.
- Promotes innovation by combining diverse perspectives. Â
- Encourages creative problem-solving through collaborative efforts. Â
- Enhances research quality by integrating methodologies from various fields. Â
- Fosters a culture of inclusivity and diversity in thought processes. Â
- Supports the development of comprehensive solutions that are more effective and sustainable. Â
At Rapid Innovation, we leverage interdisciplinary convergence to enhance our AI and Blockchain solutions. For instance, our teams often include AI specialists, blockchain developers, and industry experts who collaborate to create innovative applications that address specific client needs. This collaborative approach not only leads to more robust solutions but also prepares organizations to adapt to rapid changes in their environments. Additionally, our expertise in generative AI consulting allows us to further enhance these interdisciplinary efforts.
12. Strategic Implementation Roadmap
A strategic implementation roadmap is a detailed plan that outlines the steps necessary to achieve specific goals within an organization. It serves as a guide for aligning resources, timelines, and responsibilities to ensure successful execution of strategies.
- Clearly defines objectives and desired outcomes. Â
- Identifies key stakeholders and their roles in the implementation process. Â
- Establishes timelines for each phase of the project. Â
- Allocates resources effectively to maximize efficiency. Â
- Incorporates metrics for measuring progress and success. Â
Creating a strategic implementation roadmap involves several critical steps, including conducting a thorough analysis of the current state, identifying gaps, and determining the necessary actions to bridge those gaps. This roadmap not only helps in tracking progress but also facilitates communication among team members and stakeholders, ensuring everyone is aligned with the organization’s vision.
12.1. Organizational Readiness Assessment
An organizational readiness assessment is a systematic evaluation of an organization’s capacity to implement change or new initiatives. This assessment helps identify strengths, weaknesses, and areas that require improvement before embarking on a strategic plan.
- Evaluates the current organizational culture and climate. Â
- Assesses the skills and competencies of the workforce. Â
- Identifies existing resources and infrastructure. Â
- Gauges stakeholder support and engagement levels. Â
- Determines potential barriers to change and strategies to overcome them. Â
Conducting an organizational readiness assessment involves gathering data through surveys, interviews, and focus groups. This information is crucial for understanding how prepared the organization is for change and what steps need to be taken to enhance readiness. By addressing these factors, organizations can increase the likelihood of successful implementation and minimize resistance to change. At Rapid Innovation, we utilize these assessments to tailor our AI and Blockchain solutions, ensuring they align with the unique needs and capabilities of our clients.
12.2. Pilot Program Design

‍
A pilot program is a small-scale implementation of a project or initiative to test its feasibility, time, cost, risk, and performance before a full-scale rollout. Designing an effective pilot program involves several key steps:
- Define Objectives: Clearly outline what you aim to achieve with the pilot program design, which could include testing new technologies, processes, or methodologies. For instance, Rapid Innovation can assist in defining objectives that align with your business goals, ensuring that the pilot addresses specific challenges and opportunities.
- Select Participants: Choose a representative group of users or stakeholders who will participate in the pilot program design. This group should reflect the diversity of the larger population. Rapid Innovation can help identify and recruit participants who can provide valuable insights during the pilot phase.
- Develop a Timeline: Establish a clear timeline for the pilot program design, including start and end dates, milestones, and evaluation points. Our expertise in project management ensures that timelines are realistic and achievable, facilitating smooth execution.
- Create Evaluation Metrics: Determine how success will be measured in the pilot program design, which could include quantitative metrics (e.g., performance data) and qualitative feedback (e.g., user satisfaction). Rapid Innovation can assist in developing tailored metrics that accurately reflect the pilot's impact on your business.
- Plan for Feedback: Design a system for collecting feedback throughout the pilot program design, which can include surveys, interviews, or focus groups. Our team can implement effective feedback mechanisms that ensure continuous improvement and adaptation.
- Document Everything: Keep detailed records of the pilot program design process, including challenges faced and solutions implemented. This documentation will be invaluable for future projects. Rapid Innovation emphasizes the importance of thorough documentation to capture lessons learned and best practices.
A well-structured pilot program can provide critical insights and help mitigate risks before a broader implementation, ultimately leading to greater ROI. Additionally, for projects involving language models, consider our services for fine-tuning language models to enhance performance and relevance.
12.3. Incremental Adoption Strategies
Incremental adoption strategies involve gradually implementing changes rather than making sweeping alterations all at once. This approach can help organizations manage change more effectively and reduce resistance. Key components include:
- Phased Implementation: Roll out changes in stages, allowing for adjustments based on feedback and performance at each phase. Rapid Innovation can guide you through this phased approach, ensuring that each stage is optimized for success.
- Training and Support: Provide ongoing training and support to users as they adapt to new systems or processes, which can help ease the transition and improve user confidence. Our training programs are designed to empower your team with the necessary skills to leverage new technologies effectively.
- Pilot Testing: Use pilot programs to test new initiatives on a smaller scale before full implementation, allowing for real-world testing and adjustments. Rapid Innovation specializes in pilot testing, ensuring that your initiatives are validated before a full rollout.
- Stakeholder Engagement: Involve stakeholders early in the process to gather input and build buy-in, which can help reduce resistance and foster a sense of ownership. Our approach emphasizes collaboration and communication to ensure stakeholder alignment.
- Monitor Progress: Continuously track the progress of the adoption strategy, using metrics to assess effectiveness and make necessary adjustments. Rapid Innovation provides tools and frameworks to monitor progress effectively, ensuring that your adoption strategy remains on track.
By adopting changes incrementally, organizations can minimize disruption and enhance the likelihood of successful implementation, leading to improved ROI.
12.4. Change Management
Change management is the structured approach to transitioning individuals, teams, and organizations from a current state to a desired future state. Effective change management is crucial for ensuring that changes are implemented smoothly and successfully. Key elements include:
- Communication: Clearly communicate the reasons for the change, the benefits it will bring, and how it will impact stakeholders. Transparency is key to gaining support. Rapid Innovation can help craft communication strategies that resonate with your audience.
- Leadership Support: Secure commitment from leadership to champion the change. Leaders should model the desired behaviors and provide resources for the transition. Our consultants work with leadership teams to ensure they are equipped to drive change effectively.
- Training and Development: Offer training programs to equip employees with the skills needed to adapt to new processes or technologies, which can help alleviate anxiety and build competence. Rapid Innovation provides tailored training solutions that align with your organizational needs.
- Resistance Management: Identify potential sources of resistance and develop strategies to address them. This may involve one-on-one discussions, additional training, or adjustments to the change plan. Our expertise in change management allows us to anticipate and mitigate resistance effectively.
- Feedback Mechanisms: Establish channels for ongoing feedback to understand how the change is being received and to make necessary adjustments. This can include surveys, suggestion boxes, or regular check-ins. Rapid Innovation emphasizes the importance of feedback in refining change initiatives.
- Celebrate Successes: Recognize and celebrate milestones and successes throughout the change process, which can help maintain momentum and encourage continued engagement. Our approach includes strategies for celebrating achievements, reinforcing positive behaviors, and sustaining engagement.
Implementing effective change management practices can significantly enhance the success of any initiative, ensuring that changes are embraced rather than resisted, ultimately leading to greater ROI for your organization.
12.5. Continuous Improvement Framework
The Continuous Improvement Framework (CIF) is a structured approach that organizations use to enhance their processes, products, and services over time. This framework is essential for fostering a culture of ongoing development and efficiency, particularly in the realms of AI and Blockchain, where rapid technological advancements necessitate constant adaptation.
- Focus on incremental changes rather than radical shifts, allowing organizations to adapt gradually to new technologies and methodologies, as outlined in the continuous improvement framework.
- Emphasizes the importance of employee involvement and feedback, ensuring that teams are engaged in the innovation process and can contribute insights from their experiences, which is a key aspect of the process improvement framework.
- Utilizes data-driven decision-making to identify areas for improvement, leveraging AI analytics to uncover trends and optimize operations, a principle found in the framework for process improvement.
- Encourages regular training and development to keep skills updated, particularly in emerging technologies like AI and Blockchain, which require continuous learning, as highlighted in continuous improvement itil.
- Implements tools such as Plan-Do-Check-Act (PDCA) cycles to facilitate improvements, ensuring that changes are systematically evaluated and refined, a method supported by the cqi framework.
- Promotes a customer-centric approach, ensuring that changes align with customer needs and expectations, which is crucial for maintaining competitive advantage, as seen in continuous quality improvement framework practices.
- Involves setting measurable goals and key performance indicators (KPIs) to track progress, enabling organizations to assess the impact of their improvements effectively, a step emphasized in continuous improvement framework steps.
- Supports the use of technology and automation to streamline processes, particularly through AI-driven solutions that enhance efficiency and reduce operational costs, aligning with itil process improvement strategies.
Organizations that adopt a Continuous Improvement Framework often see enhanced productivity, reduced waste, and improved quality. This framework is not just a one-time initiative but a long-term commitment to excellence, enabling businesses to achieve greater ROI through sustained innovation, as illustrated in continuous improvement framework example cases.
13. Economic and Strategic Implications
Understanding the economic and strategic implications of business decisions is crucial for organizations aiming to thrive in competitive markets. These implications can significantly affect an organization's bottom line and overall strategic direction.
- Economic implications include the impact on revenue, costs, and profitability, particularly in the context of investments in AI and Blockchain technologies.
- Strategic implications involve how decisions align with long-term goals and market positioning, ensuring that technological advancements support overarching business objectives.
- Organizations must consider market trends, consumer behavior, and competitive dynamics, particularly in rapidly evolving sectors influenced by AI and Blockchain.
- Effective decision-making requires a balance between short-term gains and long-term sustainability, particularly when investing in transformative technologies.
- Risk management is essential to navigate uncertainties in the economic landscape, especially when adopting new technologies that may disrupt traditional business models.
- Stakeholder analysis helps in understanding the broader impact of strategic decisions, ensuring that all parties are considered in the decision-making process.
By analyzing both economic and strategic implications, organizations can make informed decisions that drive growth and ensure resilience in changing environments.
13.1. Cost-Benefit Analysis
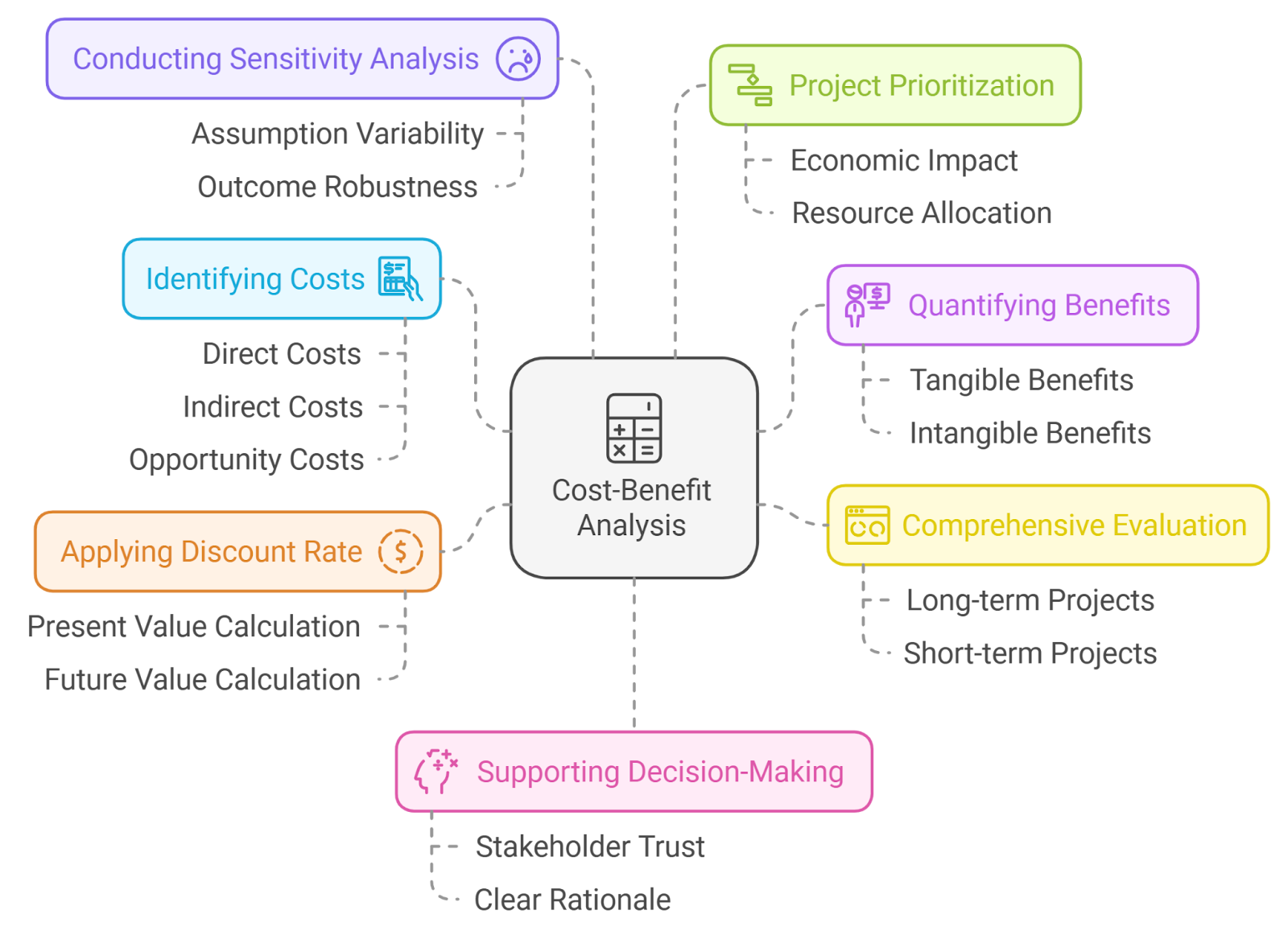
‍
Cost-Benefit Analysis (CBA) is a systematic approach used to evaluate the financial implications of a project or decision. It helps organizations weigh the expected benefits against the associated costs, providing a clear picture of the potential return on investment (ROI).
- CBA involves identifying all costs, including direct, indirect, and opportunity costs, particularly in the context of implementing AI and Blockchain solutions.
- Benefits should be quantified in monetary terms whenever possible, allowing organizations to assess the financial viability of their technology investments.
- The analysis typically includes both tangible and intangible factors, ensuring a comprehensive evaluation of potential projects.
- A discount rate may be applied to account for the time value of money, particularly relevant in long-term technology investments.
- Sensitivity analysis can help assess how changes in assumptions affect outcomes, providing insights into the robustness of the investment.
- CBA aids in prioritizing projects based on their potential economic impact, ensuring that resources are allocated to initiatives with the highest ROI.
- It supports transparent decision-making by providing a clear rationale for choices made, fostering trust among stakeholders.
By conducting a thorough Cost-Benefit Analysis, organizations can ensure that resources are allocated efficiently and that projects align with strategic objectives. This analysis is vital for making informed decisions that contribute to long-term success, particularly in the fast-paced domains of AI and Blockchain, as seen in itil continuous improvement model applications.
13.2. Competitive Advantage Strategies
Competitive advantage strategies are essential for businesses aiming to outperform their rivals in the marketplace. These strategies help organizations differentiate themselves and create value for customers. Key approaches include:
- Cost Leadership: This strategy focuses on becoming the lowest-cost producer in the industry, also known as a cost leadership strategy. Companies achieve this by optimizing operations, reducing overhead, and leveraging economies of scale. Rapid Innovation employs AI-driven analytics to streamline processes and reduce costs, enabling clients to achieve cost leadership in their respective markets.
- Differentiation: Businesses can stand out by offering unique products or services that provide added value. This could be through superior quality, innovative features, or exceptional customer service. Rapid Innovation assists clients in leveraging blockchain technology to enhance product authenticity and traceability, thereby differentiating their offerings in competitive landscapes.
- Focus Strategy: This involves targeting a specific market segment or niche. Companies can either pursue cost focus or differentiation focus. Rapid Innovation helps clients identify and penetrate niche markets by utilizing AI to analyze consumer behavior and preferences, ensuring tailored solutions that resonate with specific audiences.
- Innovation: Staying ahead of the competition often requires continuous innovation. Companies that invest in research and development can create new products or improve existing ones, thus maintaining a competitive edge. Rapid Innovation partners with clients to integrate cutting-edge AI and blockchain solutions, fostering an environment of continuous innovation that keeps them ahead of industry trends.
- Customer Relationship Management: Building strong relationships with customers can lead to loyalty and repeat business. Companies that prioritize customer service and engagement often see better retention rates. Rapid Innovation implements AI-driven customer relationship management systems that provide personalized experiences, enhancing customer satisfaction and loyalty. Additionally, Rapid Innovation offers specialized services such as AI banking solutions to further enhance customer engagement and operational efficiency.