Table Of Contents
Category
Artificial Intelligence
AIML
IoT
Blockchain
1. Introduction to AI-Driven Policy Design
Artificial Intelligence (AI) is revolutionizing various sectors, and governance is no exception. AI-driven policy design refers to the integration of AI technologies in the formulation, implementation, and evaluation of public policies. This innovative approach leverages data analytics, machine learning, and predictive modeling to enhance decision-making processes in government.
AI can analyze vast amounts of data quickly, providing insights that human analysts may overlook.
It enables real-time monitoring of policy impacts, allowing for timely adjustments.
AI tools can simulate various scenarios, helping policymakers understand potential outcomes before implementation.
The traditional policy-making process often involves lengthy deliberations and can be influenced by biases. AI-driven policy design aims to create a more objective, data-informed approach. By utilizing algorithms and data analytics, governments can make decisions that are more aligned with the needs and preferences of their constituents.
Enhanced data collection methods, such as surveys and social media analysis, provide a clearer picture of public sentiment.
AI can identify trends and patterns in data that inform policy adjustments.
The use of AI can lead to more transparent governance, as data-driven decisions can be more easily communicated to the public.
At Rapid Innovation, we specialize in harnessing AI technologies to support governments and organizations in their policy design efforts. Our expertise in AI allows us to develop tailored solutions that enhance data analysis, improve decision-making, and ultimately drive better outcomes for stakeholders. You can learn more about our approach to applying AI agents for public information.
As AI technologies continue to evolve, their application in policy design is expected to grow. This shift not only promises to improve the efficiency of governance but also aims to foster greater public trust in government institutions. By embracing AI, policymakers can create more responsive and effective policies that address the complex challenges of modern society.
Refer to the image for a visual representation of AI-driven policy design concepts.

1.1. Defining AI Agents in Policy Context
AI agents are systems that utilize artificial intelligence to perform tasks that typically require human intelligence. In the context of policy-making, these agents can analyze vast amounts of data, identify patterns, and provide insights that inform decision-making processes.
- AI agents can assist in drafting policies by analyzing historical data and predicting outcomes, enabling policymakers to make informed decisions that align with public needs.
- They can simulate various scenarios to evaluate the potential impact of different policy options, allowing for a more comprehensive understanding of possible consequences.
- AI agents can enhance public engagement by providing platforms for citizen feedback and sentiment analysis, fostering a more inclusive policy-making process.
The integration of AI agents in policymaking processes raises important questions about accountability, transparency, and ethical considerations. Policymakers must ensure that these systems are designed to operate fairly and without bias, as the decisions they influence can have significant societal implications. At Rapid Innovation, we specialize in developing AI solutions that prioritize ethical standards and transparency, ensuring that our clients can confidently leverage AI agents in their policy frameworks.
1.2. Technological Evolution of Policy-Making
The evolution of technology has dramatically transformed the landscape of policy-making. From the advent of the internet to the rise of big data and AI in policymaking, these advancements have reshaped how policies are formulated, implemented, and evaluated.
- The internet has facilitated greater access to information, allowing policymakers to gather data from diverse sources, enhancing the quality of their decisions.
- Big data analytics enables the processing of large datasets, providing insights that were previously unattainable, which can lead to more effective policy outcomes.
- Social media platforms have become vital tools for gauging public opinion and engaging citizens in the policy-making process, creating a more responsive governance model.
As technology continues to evolve, it is essential for policymakers to adapt their strategies to leverage these tools effectively. This includes investing in training and resources to ensure that government officials are equipped to utilize new technologies in their work. Rapid Innovation offers consulting services that help organizations navigate this technological landscape, ensuring they can harness the power of AI and big data for improved policy-making.
1.3. Paradigm Shift in Governance
The integration of AI and advanced technologies into governance represents a paradigm shift that alters traditional approaches to public administration. This shift is characterized by a move towards more data-driven, participatory, and responsive governance models.
- Data-driven governance relies on empirical evidence to inform policy decisions, reducing reliance on intuition or anecdotal evidence, which can lead to more effective governance.
- Participatory governance encourages citizen involvement in the decision-making process, fostering a sense of ownership and accountability among the public.
- Responsive governance emphasizes the need for agility in policy-making, allowing governments to adapt quickly to changing circumstances and emerging challenges.
This paradigm shift necessitates a reevaluation of existing governance structures and practices. Policymakers must embrace innovation while ensuring that ethical considerations and public trust remain at the forefront of their efforts. At Rapid Innovation, we are committed to guiding our clients through this transformation, providing the tools and expertise necessary to implement AI and blockchain solutions that enhance governance and drive greater ROI.
Refer to the image for a visual representation of AI agents in the policy context.

1.4. Interdisciplinary Approach
An interdisciplinary approach integrates knowledge and methodologies from various disciplines to address complex problems. This approach is particularly vital in fields such as healthcare, environmental science, and technology development.
- Promotes collaboration among experts from different fields, leading to innovative solutions.
- Encourages the sharing of diverse perspectives, enhancing creativity and problem-solving capabilities.
- Facilitates comprehensive understanding of multifaceted issues, such as climate change or public health crises.
- Examples include the collaboration between biologists, chemists, and engineers in developing sustainable materials or medical devices.
- Educational institutions increasingly emphasize interdisciplinary studies, preparing students for real-world challenges.
At Rapid Innovation, we leverage an interdisciplinary approach by combining our expertise in AI and Blockchain with insights from various sectors. For instance, our collaboration with healthcare professionals and data scientists has led to the development of AI-driven solutions that enhance patient care while ensuring data integrity through Blockchain technology.
The effectiveness of an interdisciplinary approach is evident in projects like the Human Genome Project, which required expertise from genetics, computer science, and ethics. This collaborative effort not only advanced scientific knowledge but also raised important ethical questions about genetic data usage.
Interdisciplinary learning is essential in fostering a holistic understanding of complex issues. An example of interdisciplinary studies can be seen in the integration of social sciences with natural sciences to address societal challenges. Interdisciplinary instruction and teaching methods are increasingly being adopted in educational settings to prepare students for collaborative work environments. The interdisciplinary approach in education encourages students to think critically and creatively, drawing from multiple disciplines to solve problems.
For more insights on AI, you can refer to this beginner's guide to understanding AI.
1.5. Ethical Considerations and Challenges
Ethical considerations are crucial in any field, especially when dealing with sensitive data, human subjects, or environmental impacts. Addressing these challenges requires a robust ethical framework.
- In research, informed consent is essential to ensure participants understand the risks and benefits.
- Data privacy is a significant concern, particularly with the rise of big data and AI technologies.
- Environmental ethics must be considered in projects that impact ecosystems and biodiversity.
- The potential for bias in algorithms and decision-making processes raises questions about fairness and accountability.
- Ongoing dialogue among stakeholders, including ethicists, scientists, and the public, is necessary to navigate these challenges.
For instance, the use of AI in healthcare must balance innovation with patient privacy and the potential for algorithmic bias. At Rapid Innovation, we prioritize ethical frameworks that guide our AI and Blockchain solutions, ensuring responsible decision-making that considers the broader societal implications of our work.
2. Foundational Technologies
Foundational technologies are the building blocks that enable advancements across various sectors. These technologies often serve as the backbone for innovation and development.
- Information Technology: The rise of the internet and cloud computing has transformed how data is stored, accessed, and shared.
- Artificial Intelligence: AI technologies, including machine learning and natural language processing, are revolutionizing industries by automating tasks and providing insights from data.
- Biotechnology: Advances in genetic engineering and synthetic biology are paving the way for breakthroughs in medicine, agriculture, and environmental sustainability.
- Renewable Energy: Technologies such as solar panels and wind turbines are essential for transitioning to sustainable energy sources and combating climate change.
- Robotics: The integration of robotics in manufacturing, healthcare, and logistics enhances efficiency and precision.
These foundational technologies not only drive economic growth but also address pressing global challenges. For example, AI is being used to improve healthcare outcomes by analyzing patient data for better diagnosis and treatment plans. Similarly, at Rapid Innovation, we harness Blockchain technology to ensure secure and transparent data management, which is crucial in sectors like finance and supply chain. Together, these technologies are vital in reducing carbon emissions and promoting sustainable practices.
Refer to the image for a visual representation of the interdisciplinary approach and its significance in addressing complex problems.

2.1. Machine Learning Fundamentals
Machine learning (ML) is a subset of artificial intelligence (AI) that focuses on the development of algorithms that allow computers to learn from and make predictions based on data. Understanding the fundamentals of machine learning is crucial for anyone looking to delve into this field, especially for businesses aiming to enhance their operational efficiency and decision-making processes.
- Types of Machine Learning: Â
- Supervised Learning: Involves training a model on a labeled dataset, where the outcome is known. Common algorithms include linear regression, decision trees, and support vector machines. Rapid Innovation can help clients implement supervised learning to optimize marketing strategies by predicting customer behavior based on historical data. This is a key aspect of machine learning fundamentals.
- Unsupervised Learning: Deals with unlabeled data, aiming to find hidden patterns or intrinsic structures. Techniques include clustering and dimensionality reduction. For instance, we assist clients in segmenting their customer base to tailor services and improve customer satisfaction, which is a fundamental concept in ai ml basics.
- Reinforcement Learning: Involves training an agent to make decisions by rewarding desired actions and penalizing undesired ones. This is often used in robotics and game playing. Rapid Innovation can leverage reinforcement learning to enhance automated systems, leading to increased efficiency and reduced operational costs. This is part of the fundamentals of reinforcement learning coursera.
- Key Concepts: Â
- Features and Labels: Features are the input variables used for prediction, while labels are the output variables.
- Training and Testing: The dataset is typically split into training and testing sets to evaluate the model's performance.
- Overfitting and Underfitting: Overfitting occurs when a model learns the training data too well, while underfitting happens when it fails to capture the underlying trend. Our expertise ensures that clients achieve a balanced model that maximizes predictive accuracy, which is crucial in machine learning fundamentals and algorithms.
- Applications: Â
- Image recognition
- Fraud detection
- Predictive analytics
Understanding these fundamentals is essential for leveraging machine learning in various applications, from business intelligence to healthcare, ultimately driving greater ROI for our clients. Courses like machine learning fundamentals course and datacamp machine learning fundamentals with python can provide additional insights.
2.2. Natural Language Processing
Natural Language Processing (NLP) is a branch of artificial intelligence that focuses on the interaction between computers and humans through natural language. It enables machines to understand, interpret, and respond to human language in a valuable way, which is crucial for enhancing customer engagement and operational efficiency.
- Core Components: Â
- Tokenization: The process of breaking down text into individual words or phrases.
- Part-of-Speech Tagging: Identifying the grammatical parts of speech in a sentence, such as nouns, verbs, and adjectives.
- Named Entity Recognition (NER): The identification of entities in text, such as names of people, organizations, and locations.
- Techniques: Â
- Sentiment Analysis: Determining the sentiment expressed in a piece of text, often used in social media monitoring. Rapid Innovation can implement sentiment analysis to help clients gauge public perception and adjust their strategies accordingly.
- Machine Translation: Automatically translating text from one language to another, as seen in tools like Google Translate. This can facilitate global business operations for our clients.
- Text Summarization: Creating a concise summary of a longer text while retaining its main ideas.
- Applications: Â
- Chatbots and virtual assistants
- Search engines
- Content recommendation systems
NLP is transforming how we interact with technology, making it more intuitive and user-friendly, which in turn enhances customer experiences and drives business growth.
2.3. Complex Systems Modeling
Complex systems modeling involves the study of systems with many interconnected components that interact in non-linear ways. These systems can be found in various fields, including biology, economics, and social sciences, and understanding them is vital for strategic decision-making.
- Characteristics of Complex Systems: Â
- Emergence: The whole system exhibits properties and behaviors that are not present in individual components.
- Non-linearity: Small changes in one part of the system can lead to significant effects elsewhere.
- Adaptation: Components of the system can change and evolve based on interactions with other components.
- Modeling Approaches: Â
- Agent-Based Modeling: Simulates the actions and interactions of autonomous agents to assess their effects on the system as a whole. Rapid Innovation can utilize this approach to model market dynamics and consumer behavior, providing clients with actionable insights.
- System Dynamics: Focuses on the feedback loops and time delays that affect system behavior over time.
- Network Theory: Analyzes the relationships and structures within a network of interconnected entities.
- Applications: Â
- Climate modeling
- Economic forecasting
- Epidemiology
Complex systems modeling provides valuable insights into how intricate systems function and can help in making informed decisions in various domains, ultimately leading to improved strategic outcomes for our clients. Understanding the fundamentals of machine learning and deep learning fundamentals is essential in this context.
Refer to the image for a visual representation of the Machine Learning Fundamentals discussed above:

2.4. Predictive Analytics
Predictive analytics is a branch of advanced analytics that uses historical data, statistical algorithms, and machine learning techniques to identify the likelihood of future outcomes. This approach is widely used across various industries to enhance decision-making processes. It utilizes historical data to forecast future trends, employs statistical algorithms and machine learning models, and is commonly applied in sectors like finance, healthcare, and marketing. Additionally, it helps organizations anticipate customer behavior and market trends while enhancing risk management by predicting potential issues before they arise.
At Rapid Innovation, we leverage predictive analytics to help our clients achieve greater ROI by optimizing their operations. For instance, in retail, we assist businesses in predicting inventory needs, which reduces overstock and stockouts, ultimately leading to increased sales and customer satisfaction. In healthcare, our predictive analytics models forecast patient admissions, allowing for better resource allocation and improved patient care. According to a report by McKinsey, companies that leverage predictive analytics can improve their decision-making speed by up to five times, a benefit we strive to deliver to our clients. We also utilize predictive modeling and prescriptive analytics to enhance our strategies. Our expertise extends to power bi predictive analytics, where we implement predictive analytics in power bi to provide actionable insights. Furthermore, we explore azure predictive analytics to optimize our solutions in the healthcare industry, focusing on predictive analytics in healthcare and predictive healthcare to improve patient outcomes. Our work with fp&a predictive analytics and predictive analytics softwares, including alteryx predictive analytics, allows us to create robust predictive analytics models tailored to our clients' needs.
2.5. Agent-Based Simulation Technologies
Agent-based simulation technologies are computational models that simulate the actions and interactions of autonomous agents to assess their effects on the system as a whole. This technology is particularly useful in complex systems where individual behaviors can lead to emergent phenomena. It models individual agents with specific behaviors and rules, simulates interactions among agents to observe system dynamics, and is useful in fields like economics, ecology, and social sciences. Furthermore, it allows for experimentation with different scenarios and policies and provides insights into how individual actions can impact larger systems.
At Rapid Innovation, we utilize agent-based simulations to help clients understand complex phenomena such as traffic flow, market dynamics, and disease spread. For example, in urban planning, our simulations can predict how changes in infrastructure will affect traffic patterns, enabling clients to make informed decisions. The flexibility of agent-based models allows us to test various hypotheses and visualize potential outcomes without real-world consequences, ultimately leading to more effective strategies and improved ROI.
2.6. Knowledge Representation Techniques
Knowledge representation techniques are methods used to encode information about the world into a format that a computer system can utilize to solve complex tasks. These techniques are crucial in artificial intelligence and cognitive science, enabling machines to understand and reason about the information. They involve structuring information in a way that machines can process, with common techniques including semantic networks, ontologies, and frames. These techniques facilitate natural language processing and machine learning applications, enhance the ability of AI systems to make inferences and decisions, and support knowledge sharing and reuse across different systems.
Effective knowledge representation allows for better communication between humans and machines. For instance, ontologies provide a shared vocabulary for a specific domain, improving interoperability among systems. In healthcare, knowledge representation can help in clinical decision support systems by providing relevant patient information and treatment guidelines. At Rapid Innovation, we emphasize the use of these techniques to develop intelligent systems that can learn and adapt over time, ensuring our clients can leverage their data effectively for enhanced decision-making and operational efficiency.
3. AI Agent Capabilities in Policy Development
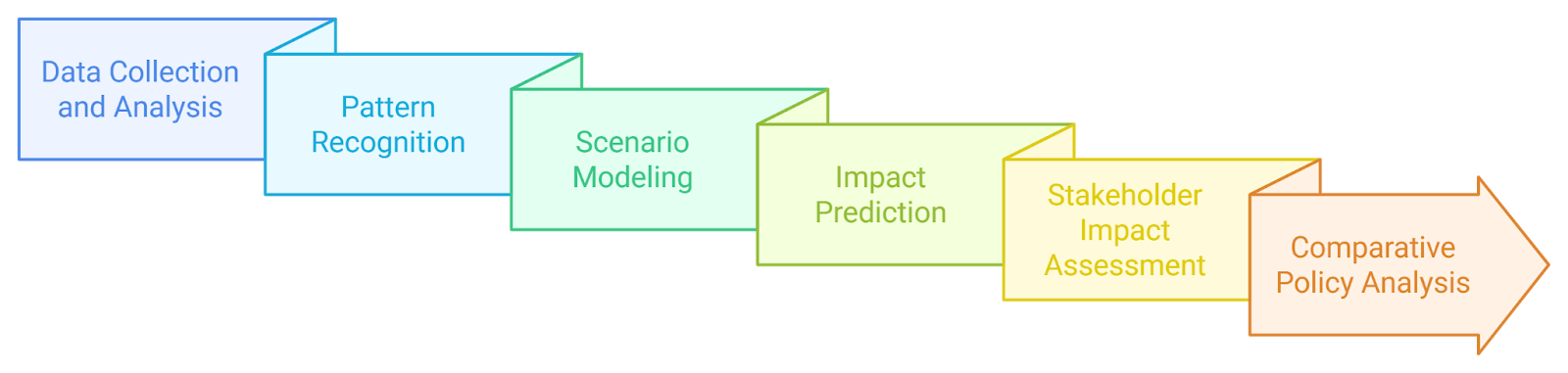
‍
AI agents are increasingly being utilized in policy development, offering innovative solutions to complex challenges. Their capabilities enhance the efficiency and effectiveness of the policy-making process, allowing for data-driven decisions that can lead to better outcomes.
3.1 Data Collection and Analysis
AI agents excel in data collection and analysis, which are critical components of effective policy development. They can process vast amounts of data from various sources, providing policymakers with comprehensive insights.
- Automated data gathering: AI agents can scrape data from websites, databases, and social media platforms, ensuring that policymakers have access to the most current information.
- Real-time analysis: These agents can analyze data in real-time, allowing for timely responses to emerging issues and trends.
- Enhanced accuracy: AI algorithms can reduce human error in data collection and analysis, leading to more reliable results.
- Predictive analytics: By utilizing historical data, AI can forecast future trends, helping policymakers anticipate challenges and opportunities.
- Cost-effectiveness: Automating data collection and analysis reduces the need for extensive human resources, saving time and money.
The ability to harness large datasets enables policymakers to make informed decisions based on empirical evidence rather than assumptions. This data-driven approach can lead to more effective policies that address the needs of the population, particularly in areas like ai in policy development.
3.2 Pattern Recognition
Pattern recognition is another significant capability of AI agents in policy development. By identifying trends and correlations within data, AI can provide valuable insights that inform policy decisions.
- Identifying trends: AI can analyze historical data to identify patterns over time, helping policymakers understand how certain factors influence outcomes.
- Correlation analysis: AI agents can uncover relationships between different variables, allowing policymakers to see how changes in one area may impact another.
- Anomaly detection: AI can spot outliers or unusual patterns in data, which may indicate emerging issues that require immediate attention.
- Scenario modeling: By recognizing patterns, AI can simulate various scenarios, helping policymakers evaluate the potential impact of different policy options.
- Improved decision-making: With the ability to recognize complex patterns, AI can support policymakers in making more nuanced decisions that consider multiple factors.
The insights gained from pattern recognition can lead to more targeted and effective policies. By understanding the underlying dynamics of social, economic, and environmental issues, policymakers can craft solutions that are more likely to succeed, especially when integrating ai in policy development.
In conclusion, the capabilities of AI agents in data collection, analysis, and pattern recognition significantly enhance the policy development process. By leveraging these technologies, policymakers can create more informed, effective, and responsive policies that address the needs of society.
At Rapid Innovation, we specialize in integrating AI solutions into your policy development processes, ensuring that you achieve greater ROI through enhanced decision-making and operational efficiency. Our expertise in AI and blockchain technology positions us to help you navigate the complexities of modern governance, ultimately leading to more impactful outcomes for your organization and the communities you serve.
3.3. Scenario Modeling
Scenario modeling is a strategic planning tool that helps organizations visualize potential future events and their impacts. It involves creating detailed representations of various possible scenarios based on different variables and assumptions. This technique is particularly useful in risk management, financial forecasting, and project planning, including moody's economic scenario generator and moody's scenario generator.
- Helps in understanding complex systems by simulating different conditions.
- Allows organizations to prepare for uncertainties by exploring "what-if" scenarios, which is a key aspect of scenario planning model and scenario strategic planning model.
- Facilitates better decision-making by providing insights into potential outcomes, especially through financial model scenarios and financial scenario modeling.
- Can be applied across various sectors, including finance, healthcare, and environmental studies, as seen in business model scenarios and business scenario modelling.
In practice, scenario modeling often involves the following steps:
- Identifying key variables that influence outcomes.
- Developing a range of plausible scenarios based on these variables, which can include carta scenario modeling and cap table scenario modeling.
- Analyzing the implications of each scenario on the organization’s objectives.
- Using the insights gained to inform strategic planning and resource allocation.
Organizations can leverage scenario modeling software and data analytics to enhance their scenario modeling efforts, making it easier to visualize and analyze complex data sets. At Rapid Innovation, we utilize advanced AI algorithms to create dynamic scenario models that adapt to real-time data, enabling our clients to make informed decisions that align with their business goals. For more information on how we can assist with your AI project estimation needs, visit our AI project estimation company.
3.4. Impact Prediction
Impact prediction refers to the process of forecasting the potential effects of a project, policy, or event on various stakeholders and the environment. This predictive analysis is crucial for effective planning and decision-making, as it helps organizations anticipate challenges and opportunities.
- Involves quantitative and qualitative assessments to gauge potential impacts.
- Can cover a wide range of areas, including economic, social, and environmental effects.
- Utilizes historical data, statistical models, and expert opinions to enhance accuracy.
Key components of impact prediction include:
- Defining the scope of the analysis to focus on relevant factors.
- Collecting and analyzing data to identify trends and patterns.
- Engaging stakeholders to gather insights and validate predictions.
- Communicating findings clearly to inform decision-makers.
Effective impact prediction can lead to more sustainable practices and better resource management, ultimately benefiting both organizations and the communities they serve. Rapid Innovation employs cutting-edge AI techniques to enhance the accuracy of impact predictions, ensuring our clients can navigate their projects with confidence.
3.5. Stakeholder Impact Assessment
Stakeholder impact assessment is a systematic approach to evaluating how a project or initiative affects various stakeholders, including employees, customers, suppliers, and the community. This assessment is essential for understanding the broader implications of business decisions and ensuring that all voices are considered.
- Helps identify key stakeholders and their interests.
- Assesses both positive and negative impacts on different groups.
- Supports transparency and accountability in decision-making processes.
The process typically involves:
- Mapping stakeholders to understand their influence and interests.
- Conducting surveys, interviews, or focus groups to gather feedback.
- Analyzing the data to identify potential impacts and areas of concern.
- Developing strategies to mitigate negative impacts and enhance positive outcomes.
By prioritizing stakeholder engagement, organizations can foster trust and collaboration, leading to more successful and sustainable outcomes. At Rapid Innovation, we integrate blockchain technology to ensure transparency and traceability in stakeholder assessments, enhancing trust and collaboration among all parties involved.
3.6. Comparative Policy Analysis
Comparative policy analysis is a method used to evaluate and compare different policies across various jurisdictions or contexts. This approach helps policymakers understand the effectiveness, efficiency, and equity of different policy options. It involves examining the similarities and differences in policies, their implementation, and outcomes. This analysis can be applied to various sectors, including healthcare, education, and environmental policy. By comparing policies, stakeholders can identify best practices and lessons learned from other regions or countries. It also aids in understanding the socio-economic and cultural factors that influence policy effectiveness. Comparative policy analysis can inform decision-making by providing evidence-based recommendations.
The process typically includes:
- Defining the policy issue and objectives.
- Selecting comparable cases or jurisdictions.
- Collecting and analyzing data on policy implementation and outcomes.
- Drawing conclusions and making recommendations based on the findings.
This method is particularly useful in a globalized world where policies can have cross-border implications. For instance, climate change policies in one country can affect environmental conditions in another. By understanding these interconnections, policymakers can develop more comprehensive and effective strategies.
For a deeper understanding, one can refer to the handbook of research methods and applications in comparative policy analysis, which provides valuable insights into the methodologies and approaches used in this field. Additionally, examining comparative policy analysis examples can illustrate how different policies have been evaluated and compared in practice. Various comparative policy analysis methods can be employed to ensure a thorough examination of the policies in question, allowing for a robust analysis of their impacts and effectiveness.
4. Technological Architecture

‍
Technological architecture refers to the structured framework that defines the components, relationships, and principles guiding the design and implementation of technology systems. It encompasses hardware, software, networks, and data management systems. A well-defined technological architecture ensures that systems are scalable, secure, and efficient. It facilitates interoperability between different systems and technologies. The architecture must align with organizational goals and user needs. Key components include:
- Infrastructure: The physical and virtual resources that support technology operations.
- Applications: Software solutions that perform specific functions for users.
- Data Management: Systems for storing, processing, and analyzing data.
- Security: Measures to protect systems and data from unauthorized access and breaches.
Technological architecture is crucial for organizations to adapt to rapid technological changes and evolving user demands. It provides a roadmap for integrating new technologies and optimizing existing systems.
4.1. AI Agent Design Principles
AI agent design principles are guidelines that inform the development of artificial intelligence systems, ensuring they are effective, ethical, and user-friendly. These principles are essential for creating AI agents that can operate autonomously while aligning with human values and societal norms. Key design principles include:
- Transparency: AI systems should be understandable and explainable to users, allowing them to comprehend how decisions are made.
- Accountability: Developers and organizations must take responsibility for the actions and outcomes of AI agents.
- Fairness: AI agents should be designed to avoid bias and discrimination, ensuring equitable treatment for all users.
- Robustness: AI systems must be resilient to errors and capable of handling unexpected situations without failure.
- User-Centric Design: AI agents should prioritize user needs and preferences, enhancing the overall user experience.
Incorporating these principles into the design process can lead to more trustworthy and effective AI systems. It also fosters public confidence in AI technologies, which is crucial for their widespread adoption. Continuous evaluation and improvement of AI agents are necessary to adapt to changing societal expectations and technological advancements. Collaboration among stakeholders, including developers, users, and policymakers, is vital for establishing best practices in AI design.
By adhering to these design principles, organizations can create AI agents that not only perform tasks efficiently but also contribute positively to society. At Rapid Innovation, we leverage these principles to develop AI solutions that align with your business goals, ensuring a greater return on investment through enhanced operational efficiency and user satisfaction.
4.2. Data Integration Frameworks
Data integration frameworks are essential for combining data from different sources into a unified view. They facilitate the seamless flow of information across various systems, enabling organizations to make informed decisions based on comprehensive data analysis. At Rapid Innovation, we leverage these frameworks, including python data integration framework, to help clients streamline their data processes, ensuring they can access and utilize their data effectively for maximum ROI.
- Key components of data integration frameworks include: Â
- Data Sources: These can be databases, APIs, or flat files that provide raw data.
- Data Transformation: This process involves cleaning, normalizing, and converting data into a usable format.
- Data Storage: Integrated data is often stored in data warehouses or lakes for easy access and analysis.
- Data Access: Tools and interfaces that allow users to query and retrieve integrated data.
- Benefits of data integration frameworks: Â
- Improved data quality and consistency.
- Enhanced decision-making capabilities through comprehensive insights.
- Increased operational efficiency by reducing data silos.
- Popular data integration frameworks include: Â
- Apache NiFi
- Talend ETL framework
- Informatica
- SSIS ETL framework
These frameworks support various integration patterns, such as ETL (Extract, Transform, Load) and ELT (Extract, Load, Transform), catering to different organizational needs. By implementing these frameworks, Rapid Innovation helps clients achieve greater efficiency and effectiveness in their data management processes, ultimately leading to improved business outcomes. If you're looking to enhance your data integration capabilities, consider hiring Action Transformer Developers from Rapid Innovation.
4.3. Multi-Agent Systems
Multi-agent systems (MAS) consist of multiple interacting intelligent agents that work together to solve complex problems. These systems are designed to mimic social behaviors and can operate autonomously or collaboratively. Rapid Innovation utilizes MAS to develop solutions that enhance operational efficiency and adaptability for our clients.
- Characteristics of multi-agent systems: Â
- Autonomy: Each agent operates independently, making its own decisions based on its environment.
- Social Ability: Agents can communicate and collaborate with one another to achieve common goals.
- Reactivity: Agents can respond to changes in their environment in real-time.
- Proactiveness: Agents can take initiative to fulfill their objectives without external prompting.
- Applications of multi-agent systems: Â
- Robotics: Coordinating multiple robots for tasks like search and rescue or warehouse management.
- Traffic Management: Optimizing traffic flow by using agents to control traffic signals and monitor congestion.
- E-commerce: Facilitating automated negotiations and transactions between buyers and sellers.
- Benefits of multi-agent systems: Â
- Scalability: Easily add more agents to handle increased complexity.
- Flexibility: Adapt to changing environments and requirements.
- Robustness: Failure of one agent does not compromise the entire system.
By integrating multi-agent systems into our clients' operations, Rapid Innovation enhances their ability to respond to dynamic market conditions, ultimately driving better ROI.
4.4. Decision Support Infrastructures
Decision support infrastructures (DSIs) are systems designed to assist in making informed decisions by providing relevant data, analytical tools, and models. They play a crucial role in various sectors, including business, healthcare, and government. Rapid Innovation specializes in developing DSIs that empower organizations to make data-driven decisions efficiently.
- Components of decision support infrastructures: Â
- Data Management: Collecting, storing, and organizing data from various sources.
- Analytical Tools: Software and algorithms that analyze data to generate insights.
- User Interface: Dashboards and visualization tools that present data in an understandable format.
- Collaboration Tools: Features that allow teams to work together on decision-making processes.
- Types of decision support systems: Â
- Data-driven DSS: Focuses on the analysis of large datasets to support decisions.
- Model-driven DSS: Utilizes mathematical and statistical models to simulate scenarios and outcomes.
- Knowledge-driven DSS: Leverages expert knowledge and rules to guide decision-making.
- Benefits of decision support infrastructures: Â
- Enhanced decision quality through data-driven insights.
- Increased efficiency by streamlining the decision-making process.
- Improved collaboration among stakeholders, leading to more comprehensive solutions.
In conclusion, data integration frameworks, multi-agent systems, and decision support infrastructures are vital components of modern information systems. They enable organizations to harness the power of data, automate processes, and make informed decisions, ultimately driving success in a competitive landscape. At Rapid Innovation, we are committed to helping our clients leverage these technologies to achieve their business goals efficiently and effectively, ensuring a greater return on investment.
4.5. Computational Policy Modeling
Computational policy modeling refers to the use of computational techniques to simulate, analyze, and evaluate public policies. This approach allows policymakers to understand the potential impacts of their decisions before implementation.
- Utilizes mathematical models and algorithms to represent complex systems. Â
- Helps in predicting outcomes based on various policy scenarios. Â
- Facilitates stakeholder engagement by visualizing potential impacts. Â
- Supports evidence-based decision-making through data analysis. Â
- Can incorporate various data sources, including social, economic, and environmental factors. Â
At Rapid Innovation, we leverage advanced computational policy modeling techniques, including policy neural network approaches, to assist our clients in sectors such as environmental policy, healthcare, and urban planning. By simulating different scenarios, we enable policymakers to identify the most effective strategies and anticipate unintended consequences. This method also allows for real-time adjustments based on new data, making it a dynamic tool for policy development that ultimately leads to greater ROI.
4.6. Semantic Web Technologies
Semantic web technologies enhance the web's ability to understand and interpret data. This technology enables machines to process and analyze information in a way that is meaningful, facilitating better data sharing and integration across different platforms.
- Involves the use of ontologies to define data relationships. Â
- Enhances data interoperability, allowing diverse systems to communicate. Â
- Supports advanced search capabilities, improving information retrieval. Â
- Enables the creation of linked data, connecting related information across the web. Â
- Facilitates knowledge representation, making complex data more accessible. Â
At Rapid Innovation, we recognize the importance of semantic web technologies in developing intelligent systems that assist in policy analysis and decision-making. By structuring data in a way that machines can understand, we help policymakers access relevant information quickly and efficiently. This leads to more informed decisions and better policy outcomes, ultimately enhancing the effectiveness of our clients' initiatives.
5. Policy Design Methodologies

‍
Policy design methodologies encompass various approaches and frameworks used to create effective public policies. These methodologies guide the process from problem identification to policy evaluation, ensuring that policies are well-structured and evidence-based.
- Involves stakeholder analysis to understand different perspectives. Â
- Utilizes frameworks like the Rational Model, Incrementalism, and Mixed-Scanning. Â
- Emphasizes the importance of data collection and analysis in the design process. Â
- Encourages iterative design, allowing for adjustments based on feedback and evaluation. Â
- Incorporates tools such as logic models and policy briefs to clarify objectives and strategies. Â
Effective policy design methodologies are essential for addressing complex societal issues. By employing a systematic approach, Rapid Innovation ensures that our clients' policies are not only feasible but also aligned with the needs of the community. This leads to more sustainable and impactful policy outcomes, ultimately driving greater ROI for our clients.
5.1. Traditional vs. AI-Augmented Approaches
Traditional policy-making approaches often rely on historical data, expert opinions, and qualitative assessments. These methods can be time-consuming and may not always capture the complexities of modern societal issues. Decision-making is often based on intuition and experience, stakeholder engagement is typically limited to a few key players, and the process can be slow, leading to delayed responses to emerging issues.
In contrast, AI-augmented approaches leverage advanced technologies to enhance decision-making processes. AI policymaking can analyze vast amounts of data quickly, identifying patterns and trends that may not be visible through traditional methods. Machine learning algorithms can provide insights that help policymakers understand the potential impacts of their decisions. Additionally, enhanced stakeholder engagement through AI tools can facilitate broader participation and feedback.
At Rapid Innovation, we specialize in integrating AI solutions that streamline policy-making processes, enabling our clients to achieve greater efficiency and responsiveness. By harnessing AI capabilities, we empower organizations to make data-driven decisions that lead to improved outcomes and higher ROI.
5.2. Data-Driven Policy Formulation
Data-driven policy formulation emphasizes the use of empirical evidence to guide decision-making. This approach relies on quantitative and qualitative data to inform policies, ensuring they are grounded in reality rather than assumptions. Policymakers can utilize big data analytics to gather insights from various sources, including social media, surveys, and economic indicators. Evidence-based policies are more likely to achieve desired outcomes, as they are tailored to address specific issues identified through data analysis. Furthermore, continuous monitoring and evaluation of policies can be facilitated through data collection, allowing for adjustments based on real-time feedback.
At Rapid Innovation, we provide advanced data analytics solutions that help our clients formulate policies based on solid evidence. Our expertise in big data allows organizations to respond effectively to societal needs and challenges, ultimately leading to better governance and improved public services.
5.3. Predictive Policy Modeling
Predictive policy modeling involves using statistical techniques and algorithms to forecast the potential outcomes of various policy options. This approach allows policymakers to simulate different scenarios and assess the likely impacts of their decisions before implementation. Predictive models can help identify potential risks and benefits associated with specific policies. By analyzing historical data, these models can provide insights into how similar policies have performed in the past. Policymakers can also use simulations to explore the effects of different variables, such as economic conditions or demographic changes, on policy outcomes.
The use of predictive policy modeling enhances the ability to make informed decisions. It allows for proactive rather than reactive governance, enabling policymakers to anticipate challenges and adapt strategies accordingly. This forward-thinking approach can lead to more effective and sustainable policies that better serve the public interest. Rapid Innovation's predictive modeling solutions equip our clients with the tools necessary to navigate complex policy landscapes, ensuring they can achieve optimal results and maximize their ROI.
5.4. Simulation-Based Policy Testing
Simulation-based policy testing is a crucial method for evaluating the effectiveness of various policy options before they are implemented in real-world scenarios. This approach allows policymakers to create virtual environments where they can model the potential impacts of different policies.
- Enables the exploration of complex systems and interactions. Â
- Provides a risk-free environment to test hypotheses and outcomes. Â
- Facilitates the identification of unintended consequences. Â
- Supports data-driven decision-making by using historical data and predictive analytics. Â
- Can incorporate various scenarios, including extreme cases, to assess resilience. Â
At Rapid Innovation, we leverage advanced AI algorithms to enhance policy testing simulation. By utilizing our AI-driven simulation tools, policymakers can visualize the potential effects of their decisions, leading to more informed and effective policy-making. This method is particularly useful in sectors like environmental policy, healthcare, and urban planning, where the implications of policies can be far-reaching and complex. Our clients have seen significant ROI by making data-backed decisions that minimize risks and optimize outcomes, including our AI insurance solutions.
5.5. Adaptive Policy Frameworks
Adaptive policy frameworks are designed to be flexible and responsive to changing conditions and new information. These frameworks allow for continuous learning and adjustment, ensuring that policies remain relevant and effective over time.
- Emphasizes iterative processes and feedback loops. Â
- Encourages stakeholder engagement and collaboration. Â
- Utilizes real-time data to inform policy adjustments. Â
- Supports resilience by allowing policies to evolve in response to unforeseen challenges. Â
- Promotes innovation by encouraging experimentation and pilot programs. Â
At Rapid Innovation, we assist organizations in developing adaptive policy frameworks that are particularly beneficial in dynamic environments, such as climate change mitigation and public health responses, where conditions can shift rapidly. By fostering a culture of adaptability, our clients can better navigate uncertainties and enhance their policy outcomes, ultimately leading to greater efficiency and effectiveness in achieving their business goals.
5.6. Real-Time Policy Adjustment Mechanisms
Real-time policy adjustment mechanisms are essential for ensuring that policies can be modified quickly in response to emerging data or changing circumstances. These mechanisms leverage technology and data analytics to facilitate immediate policy changes.
- Utilizes data analytics and monitoring tools to track policy performance. Â
- Enables rapid response to crises or unexpected events. Â
- Supports proactive rather than reactive policy-making. Â
- Enhances accountability by providing transparent metrics for evaluation. Â
- Encourages stakeholder feedback to inform adjustments. Â
Implementing real-time policy adjustment mechanisms can significantly improve the effectiveness of governance. For instance, in public health, real-time data on disease outbreaks can lead to swift policy changes that protect communities. At Rapid Innovation, we integrate these mechanisms into our clients' operations, ensuring that their policies remain effective and aligned with current realities. By harnessing the power of AI and data analytics, organizations can achieve a higher ROI through timely and informed decision-making.
6. Domain-Specific Applications
Domain-specific applications refer to the tailored use of technology, data, and methodologies to address unique challenges and opportunities within specific fields. This approach enhances the effectiveness of solutions by focusing on the nuances and requirements of each domain. Two critical areas where domain-specific applications are particularly impactful are public health policy and economic policy design.
6.1. Public Health Policy
Public health policy is essential for promoting health, preventing disease, and ensuring access to healthcare services. The integration of domain-specific applications in public health can lead to more effective strategies and interventions.
- Data-Driven Decision Making: Utilizing data analytics allows policymakers to identify health trends, assess risks, and allocate resources efficiently. For instance, real-time data on disease outbreaks can inform timely responses, enabling organizations to deploy resources where they are needed most.
- Predictive Modeling: Advanced modeling techniques can forecast the spread of diseases, helping public health officials prepare and respond effectively. This is particularly relevant in managing infectious diseases, where early intervention can save lives and reduce healthcare costs.
- Health Equity: Domain-specific applications can help identify disparities in health outcomes among different populations. By analyzing social determinants of health, policies can be designed to address these inequities and improve access to care for marginalized communities, ultimately leading to better health outcomes and reduced long-term costs.
- Telehealth Expansion: The rise of telehealth services has transformed public health delivery, especially during the COVID-19 pandemic. Policies that support telehealth can enhance access to care, particularly in rural or underserved areas, thereby improving patient engagement and satisfaction.
- Community Engagement: Engaging communities in the policymaking process ensures that health policies are culturally relevant and address the specific needs of the population. This can lead to higher compliance and better health outcomes, ultimately resulting in a healthier workforce and reduced economic burden.
- Public Health Policy Examples: Various public health policy examples illustrate the effectiveness of tailored interventions, such as healthy public policies that promote wellness and prevent disease.
- Public Health and Policy: The intersection of public health and policy is crucial for developing comprehensive strategies that address health challenges.
- Health Policy and Management: Integrating health policy and management practices can enhance the implementation of public health initiatives.
6.2. Economic Policy Design

‍
Economic policy design involves creating strategies that influence a country's economic performance. Domain-specific applications in this area can lead to more informed and effective economic policies.
- Evidence-Based Policy: Utilizing empirical data and research helps policymakers understand the potential impacts of various economic strategies. This approach can lead to more effective interventions that stimulate growth and reduce unemployment, ultimately enhancing the overall economic landscape.
- Behavioral Economics: Understanding how individuals make economic decisions can inform policies that encourage saving, investment, and responsible spending. This can be particularly useful in designing tax incentives or social programs that drive economic activity and improve financial literacy.
- Economic Modeling: Advanced economic models can simulate the effects of policy changes on various economic indicators, such as GDP, inflation, and employment rates. This allows for better forecasting and planning, enabling stakeholders to make informed decisions that maximize economic benefits.
- Targeted Interventions: Domain-specific applications can help identify sectors that require targeted support, such as small businesses or emerging industries. Tailored policies can foster innovation and economic resilience, leading to a more robust economy.
- Global Considerations: In an interconnected world, economic policies must consider global trends and challenges. Domain-specific applications can help analyze international trade dynamics, currency fluctuations, and geopolitical risks, ensuring that policies are adaptable and forward-thinking.
By leveraging domain-specific applications in public health policy and economic policy design, Rapid Innovation empowers stakeholders to create more effective, equitable, and sustainable solutions that address the unique challenges of each field, ultimately driving greater ROI and enhancing overall societal well-being. This includes advanced studies such as health policy PhD programs and MPA health policy initiatives that prepare future leaders in these critical areas, including AI healthcare management.
6.3. Environmental Regulation
Environmental regulation refers to the set of laws and policies designed to protect the environment from harmful activities. These regulations are crucial for maintaining ecological balance and ensuring sustainable development. They protect air and water quality by setting limits on pollutants, regulate waste management to minimize landfill use and promote recycling, enforce conservation efforts to protect endangered species and habitats, encourage the use of renewable energy sources to reduce carbon emissions, and promote sustainable land use practices to prevent deforestation and soil degradation.
Governments and organizations worldwide implement these regulations to combat climate change and promote environmental sustainability. For instance, the Clean Air Act in the United States has significantly reduced air pollution levels since its enactment. Similarly, the European Union's directives on waste management have led to increased recycling rates across member states. The Environmental Protection Agency (EPA) plays a critical role in enforcing these regulations, including the air quality standards and the national ambient air quality standards (NAAQS).
At Rapid Innovation, we leverage AI and blockchain technologies to help organizations comply with environmental regulations more efficiently. For example, our AI-driven analytics can monitor emissions in real-time, providing businesses with actionable insights to reduce their carbon footprint. Additionally, blockchain can enhance transparency in supply chains, ensuring that companies adhere to sustainable practices and regulatory requirements, such as the EPA regulations and the environmental protection act regulations.
6.4. Urban Planning and Infrastructure
Urban planning and infrastructure development are essential for creating functional, sustainable, and livable cities. Effective urban planning involves the strategic organization of land use, transportation, and public services to meet the needs of growing populations. It facilitates efficient transportation systems to reduce traffic congestion, promotes mixed-use developments to enhance community interaction, ensures adequate public services, such as water supply, sanitation, and waste management, incorporates green spaces to improve air quality and provide recreational areas, and addresses housing needs through affordable housing initiatives.
Urban infrastructure, including roads, bridges, and public transit systems, plays a vital role in economic development. Well-planned urban areas can attract businesses and improve the quality of life for residents. For example, cities like Singapore and Copenhagen are often cited for their innovative urban planning strategies that prioritize sustainability and livability.
Rapid Innovation can assist urban planners by utilizing AI to analyze data trends and predict future urban needs, ensuring that infrastructure development is both efficient and sustainable. Our blockchain solutions can also facilitate secure and transparent transactions in urban development projects, enhancing trust among stakeholders.
6.5. Social Welfare Policy
Social welfare policy encompasses a range of government programs and initiatives aimed at improving the well-being of individuals and communities. These policies are designed to provide support to vulnerable populations and promote social equity. They offer financial assistance to low-income families through programs like food stamps and unemployment benefits, provide access to healthcare services, ensuring that all individuals receive necessary medical care, support education and job training programs to enhance employability and reduce poverty, promote housing assistance to help individuals secure safe and affordable living conditions, and encourage community development initiatives to strengthen local economies and social networks.
Effective social welfare policies can lead to improved health outcomes, reduced poverty rates, and enhanced quality of life. Countries with robust social welfare systems, such as the Nordic nations, often report higher levels of social cohesion and overall happiness among their citizens.
At Rapid Innovation, we harness AI to analyze social welfare data, enabling governments and organizations to identify gaps in services and optimize resource allocation. Our blockchain technology can also ensure the integrity and transparency of welfare programs, fostering trust and accountability in the distribution of aid.
6.6. Education Policy Development
Education policy development is crucial for shaping the future of educational systems, including early childhood education policy. It involves creating frameworks that guide the delivery of education, ensuring that it meets the needs of students, educators, and society as a whole. Effective education policies, such as those focused on early childhood education and care policy, can lead to improved learning outcomes, increased access to education, and enhanced equity.
- Importance of Stakeholder Engagement
Involving teachers, parents, students, and community members in the policy-making process ensures that diverse perspectives are considered. Engaging stakeholders can lead to more effective and relevant policies, particularly in the context of early childhood education policies. - Data-Driven Decision Making
Utilizing data analytics helps identify areas needing improvement, such as student performance and resource allocation. Evidence-based policies can lead to better educational outcomes. At Rapid Innovation, we leverage advanced AI analytics to provide actionable insights that empower educational institutions to make informed decisions. - Focus on Equity and Inclusion
Policies should address disparities in education access and quality, particularly for marginalized groups. Promoting inclusive education practices ensures that all students have the opportunity to succeed, which is especially important in early childhood development policies. - Continuous Evaluation and Adaptation
Regularly assessing the effectiveness of education policies allows for timely adjustments. Feedback mechanisms can help policymakers understand the impact of their decisions, particularly in the context of education policy and international development. - Integration of Technology
Incorporating technology into education policy can enhance learning experiences and prepare students for a digital future. Policies should support the training of educators in using technology effectively. Rapid Innovation specializes in developing tailored technology solutions that facilitate this integration, ensuring that educational institutions are equipped for the future, including in the realm of curriculum development policy.
6.7. Cybersecurity and Digital Governance
Cybersecurity and digital governance are increasingly important in our interconnected world. As technology advances, the need for robust security measures and effective governance frameworks becomes paramount to protect sensitive information and maintain public trust.
- Importance of Cybersecurity
Cyber threats are on the rise, with businesses and governments facing constant attacks. Implementing strong cybersecurity measures is essential to safeguard data and maintain operational integrity. Rapid Innovation offers comprehensive cybersecurity solutions that help organizations mitigate risks and protect their assets. - Establishing Governance Frameworks
Clear governance structures help organizations manage cybersecurity risks effectively. Policies should define roles and responsibilities for cybersecurity management. - Risk Assessment and Management
Regular risk assessments help identify vulnerabilities and inform security strategies. Organizations should adopt a proactive approach to risk management. Our team at Rapid Innovation utilizes advanced AI tools to conduct thorough risk assessments, ensuring that organizations are prepared for potential threats. - Compliance with Regulations
Adhering to cybersecurity regulations and standards is crucial for legal compliance and risk mitigation. Organizations must stay updated on evolving laws and regulations. - Training and Awareness
Employee training programs are vital for fostering a culture of cybersecurity awareness. Regular training helps staff recognize potential threats and respond appropriately. Rapid Innovation provides tailored training solutions that empower employees to be the first line of defense against cyber threats.
7. Advanced AI Agent Capabilities
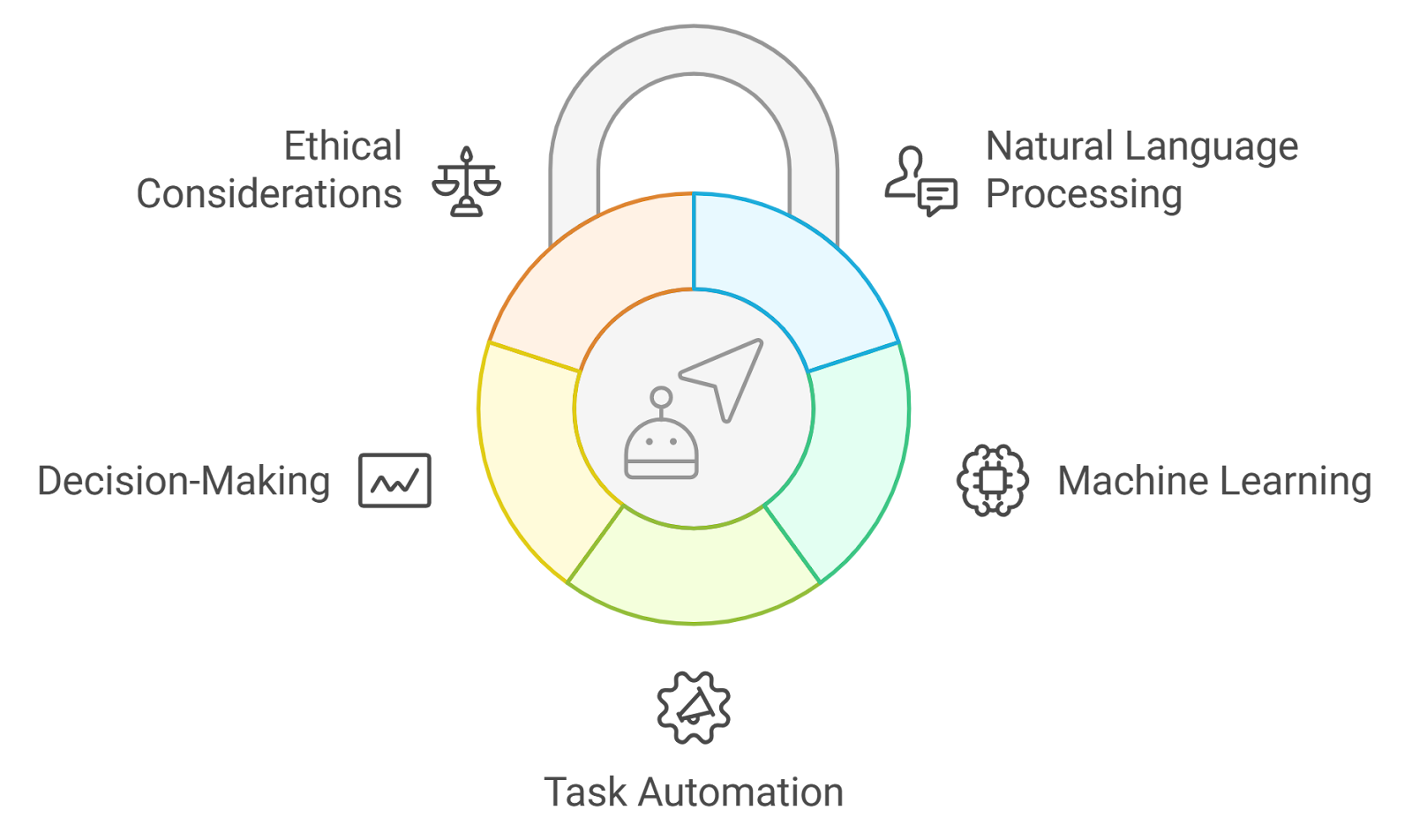
‍
Advanced AI agent capabilities are transforming various sectors by enhancing efficiency, decision-making, and user experiences. These intelligent systems can perform complex tasks, analyze vast amounts of data, and interact with users in natural language.
- Natural Language Processing (NLP)
NLP enables AI agents to understand and generate human language, facilitating seamless communication. Applications include chatbots, virtual assistants, and customer service automation. Rapid Innovation develops sophisticated NLP solutions that enhance customer engagement and streamline communication processes. - Machine Learning and Predictive Analytics
AI agents can learn from data patterns, improving their performance over time. Predictive analytics helps organizations anticipate trends and make informed decisions. Our expertise in machine learning allows us to create predictive models that drive strategic initiatives and improve ROI for our clients. - Automation of Routine Tasks
AI agents can automate repetitive tasks, freeing up human resources for more strategic activities. This leads to increased productivity and reduced operational costs. Rapid Innovation's automation solutions help organizations optimize workflows and focus on core business objectives. - Enhanced Decision-Making
AI agents can analyze data and provide insights that support better decision-making. Organizations can leverage AI to optimize processes and improve outcomes. Our AI-driven insights empower clients to make data-informed decisions that enhance operational efficiency. - Ethical Considerations
As AI capabilities expand, ethical considerations become increasingly important. Organizations must address issues such as bias, transparency, and accountability in AI systems. Rapid Innovation is committed to developing ethical AI solutions that prioritize fairness and transparency, ensuring that our clients can trust the technology they implement.
7.1. Autonomous Decision Making
Autonomous decision-making refers to the ability of systems, particularly artificial intelligence (AI) and machine learning (ML) models, to make decisions without human intervention. This capability is increasingly important in various sectors, including finance, healthcare, and transportation.
- AI systems can analyze vast amounts of data quickly, identifying patterns and trends that may not be apparent to human analysts. For instance, Rapid Innovation has helped financial institutions implement AI-driven trading algorithms that optimize investment strategies, resulting in significant ROI.
- These systems can operate in real-time, allowing for immediate responses to changing conditions, which is crucial in environments like stock trading or emergency response. Our solutions have enabled healthcare providers to utilize AI for real-time patient monitoring, improving response times and patient outcomes.
- Autonomous decision-making can enhance efficiency by reducing the time taken to make decisions, leading to faster execution of tasks. By automating routine processes, Rapid Innovation has assisted clients in various industries to streamline operations and reduce operational costs. This is particularly relevant in the context of autonomous driving decision making, where timely decisions are critical for safety and efficiency.
- Ethical considerations are paramount, as these systems must be designed to make decisions that align with societal values and norms. We prioritize ethical AI development, ensuring that our solutions are compliant with regulations and ethical standards, especially in areas like decision making in autonomous vehicles.
- Transparency in how decisions are made is essential to build trust among users and stakeholders. Rapid Innovation emphasizes the importance of explainable AI, providing clients with tools to understand and communicate the decision-making processes of their AI systems, including decisions made by autonomous driving systems. For advanced solutions in this area, consider our AI as a Service services.
7.2. Multi-Stakeholder Consensus Modeling
Multi-stakeholder consensus modeling involves creating frameworks that allow various parties with different interests to come together and reach an agreement. This approach is vital in complex environments where decisions impact multiple stakeholders, such as urban planning, environmental policy, and corporate governance.
- Consensus modeling helps in identifying common goals among stakeholders, facilitating collaboration and reducing conflicts. Rapid Innovation has successfully implemented consensus modeling in urban development projects, ensuring that community interests are represented.
- It employs techniques such as stakeholder mapping and participatory decision-making to ensure that all voices are heard. Our team utilizes advanced modeling tools to engage stakeholders effectively, fostering a collaborative environment.
- The process often includes simulations and scenario analysis to visualize potential outcomes and impacts of different decisions. By leveraging our expertise in AI, we provide clients with predictive analytics that inform decision-making processes.
- Effective communication is crucial in this modeling, as it helps to clarify misunderstandings and align expectations. Rapid Innovation offers communication strategies that enhance stakeholder engagement and understanding.
- Tools like Delphi methods and consensus-building workshops can be utilized to foster dialogue and agreement among diverse groups. We facilitate workshops that bring together stakeholders to collaboratively develop solutions that meet their needs.
7.3. Complex Scenario Generation
Complex scenario generation is the process of creating detailed and plausible future scenarios to explore potential outcomes and impacts of various decisions. This technique is particularly useful in strategic planning, risk management, and policy development.
- It allows organizations to anticipate challenges and opportunities by considering a wide range of variables and uncertainties. Rapid Innovation employs scenario generation to help clients in risk management, enabling them to prepare for potential disruptions.
- Scenarios can be developed using qualitative and quantitative methods, including simulations, expert opinions, and historical data analysis. Our data-driven approach ensures that the scenarios we create are grounded in reality and relevant to our clients' contexts.
- This approach encourages creative thinking and innovation, as stakeholders are prompted to consider "what if" situations that may not be immediately obvious. We guide organizations in exploring innovative solutions that can emerge from different scenarios, including planning and decision making for autonomous vehicles.
- Complex scenario generation can help in identifying critical success factors and potential pitfalls in decision-making processes. By analyzing various outcomes, Rapid Innovation equips clients with the insights needed to make informed decisions.
- By visualizing different futures, organizations can better prepare for change and adapt their strategies accordingly. Our advanced visualization tools allow clients to see the implications of their decisions, enhancing strategic planning efforts.
7.4. Ethical Decision Frameworks
Ethical decision frameworks are structured approaches that guide individuals and organizations in making choices that align with ethical principles. These frameworks, such as the ethical decision making framework and a framework for ethical decision making, are essential in various fields, including business, healthcare, and technology, as they help navigate complex moral dilemmas.
- Principles of Ethical Decision-Making: Â
- Utilitarianism: Focuses on the greatest good for the greatest number.
- Deontological ethics: Emphasizes duties and rules.
- Virtue ethics: Centers on the character and virtues of the decision-maker.
- Steps in Ethical Decision Frameworks: Â
- Identify the ethical issue: Recognize the dilemma at hand.
- Gather information: Collect relevant facts and perspectives.
- Evaluate alternatives: Consider the consequences of different actions.
- Make a decision: Choose the option that aligns with ethical principles.
- Reflect on the outcome: Assess the impact of the decision and learn from it.
- Application in Organizations: Â
- Establishing a code of ethics: Provides guidelines for employees.
- Training programs: Educate staff on ethical decision-making, including the ethical decision making framework social work.
- Ethical audits: Regularly review practices to ensure compliance with ethical standards.
At Rapid Innovation, we understand the importance of ethical decision-making in AI and Blockchain development. By implementing robust ethical decision making frameworks, we help our clients navigate the complexities of technology deployment while ensuring compliance and fostering trust among stakeholders.
7.5. Bias Detection and Mitigation
Bias detection and mitigation are critical in ensuring fairness and equity in decision-making processes, particularly in areas like hiring, lending, and law enforcement. Bias can lead to unjust outcomes and perpetuate systemic inequalities.
- Types of Bias: Â
- Implicit bias: Unconscious attitudes or stereotypes that affect understanding and actions.
- Confirmation bias: The tendency to search for, interpret, and remember information that confirms pre-existing beliefs.
- Selection bias: Occurs when individuals are selected for a study or analysis in a way that is not random.
- Techniques for Bias Detection: Â
- Data analysis: Use statistical methods to identify patterns of bias in datasets.
- Audits: Conduct regular reviews of processes to uncover potential biases.
- Surveys and feedback: Gather input from diverse groups to identify perceived biases.
- Mitigation Strategies: Â
- Training programs: Implement bias awareness training for employees.
- Diverse teams: Promote diversity in teams to bring different perspectives.
- Algorithmic fairness: Develop algorithms that are tested for bias and adjusted accordingly.
At Rapid Innovation, we leverage advanced AI techniques to detect and mitigate bias in our clients' systems. By ensuring algorithmic fairness, we help organizations achieve greater ROI while promoting inclusivity and equity in their operations.
7.6. Cross-Domain Policy Optimization
Cross-domain policy optimization refers to the process of creating and implementing policies that are effective across different domains or sectors. This approach is particularly relevant in areas like environmental policy, public health, and technology regulation.
- Importance of Cross-Domain Policies: Â
- Holistic approach: Addresses interconnected issues that span multiple domains.
- Resource efficiency: Optimizes the use of resources by aligning policies across sectors.
- Enhanced collaboration: Fosters partnerships between different stakeholders.
- Key Considerations: Â
- Stakeholder engagement: Involve various stakeholders in the policy-making process to ensure diverse perspectives.
- Evidence-based decision-making: Use data and research to inform policy choices.
- Flexibility: Allow for adjustments in policies as new information and technologies emerge.
- Examples of Cross-Domain Policy Optimization: Â
- Climate change initiatives: Integrating environmental, economic, and social policies to address climate issues effectively.
- Health and technology: Developing regulations that consider both public health and technological advancements, such as telemedicine.
- Education and workforce development: Aligning educational policies with labor market needs to ensure a skilled workforce.
Rapid Innovation excels in cross-domain policy optimization by utilizing AI and Blockchain technologies to create adaptable and efficient policies. Our expertise enables clients to navigate complex regulatory landscapes, ensuring compliance while maximizing their operational effectiveness and return on investment.
8. Implementation Strategies
Effective implementation strategies are crucial for the success of any project, especially in the context of technology and data management. These strategies ensure that organizations are prepared, data is properly handled, and the overall process runs smoothly. This includes various aspects such as strategy formulation and implementation, as well as the development of a strategy implementation plan.
8.1 Organizational Readiness Assessment
An organizational readiness assessment is a systematic evaluation of an organization's capacity to implement a new system or process. This assessment helps identify strengths, weaknesses, and areas that require improvement before the actual implementation begins.
- Identify Stakeholders: Recognize key stakeholders who will be involved in or affected by the implementation, including management, IT staff, and end-users.
- Evaluate Current Processes: Analyze existing workflows and processes to understand how they align with the new system, helping to identify gaps and areas for improvement.
- Assess Skills and Training Needs: Determine if the current workforce has the necessary skills to operate the new system. If not, plan for training sessions to bridge the skill gap.
- Cultural Readiness: Evaluate the organizational culture to see if it supports change, as a culture resistant to change can hinder the implementation process.
- Resource Availability: Assess the availability of resources, including budget, technology, and personnel, to support the implementation.
- Change Management Strategy: Develop a change management plan that outlines how to communicate changes to the organization and manage resistance.
Conducting a thorough organizational readiness assessment can significantly increase the likelihood of successful implementation. It allows organizations to proactively address potential challenges and align their resources effectively, which is a key aspect of implementation strategies.
8.2 Data Preparation and Normalization
Data preparation and normalization are critical steps in ensuring that the data used in a new system is accurate, consistent, and ready for analysis. Proper data handling can lead to better decision-making and improved outcomes.
- Data Collection: Gather data from various sources, ensuring that it is relevant and comprehensive. This may include internal databases, external sources, and user-generated data.
- Data Cleaning: Identify and rectify errors in the data, such as duplicates, missing values, and inconsistencies. This step is essential for maintaining data integrity.
- Data Transformation: Convert data into a suitable format for analysis, which may involve changing data types, aggregating data, or creating new calculated fields.
- Normalization: Standardize data to ensure consistency across different datasets, helping to reduce redundancy and improve data quality.
- Data Integration: Combine data from different sources into a unified view, which is crucial for comprehensive analysis and reporting.
- Documentation: Maintain clear documentation of data sources, cleaning processes, and transformation steps to ensure transparency and facilitate future audits.
By focusing on data preparation and normalization, organizations can ensure that they are working with high-quality data, which is essential for making informed decisions and achieving desired outcomes. This is particularly important when considering implementation strategy examples and change implementation strategies. At Rapid Innovation, we leverage our expertise in AI and Blockchain to enhance these processes, ensuring that our clients not only meet their implementation goals but also achieve greater ROI through efficient data management and the critical role of data quality in AI implementations.
8.3. AI Agent Training Methodologies

‍
AI agent training methodologies are crucial for developing effective artificial intelligence systems. These methodologies encompass various approaches and techniques that enable AI agents to learn from data, adapt to new situations, and improve their performance over time.
- Supervised Learning: This method involves training AI agents on labeled datasets, where the input data is paired with the correct output. The agent learns to map inputs to outputs, making it effective for tasks like image recognition and natural language processing. Rapid Innovation utilizes supervised learning to enhance client applications, ensuring accurate predictions and improved user experiences.
- Unsupervised Learning: In this approach, AI agents are trained on unlabeled data. The goal is to identify patterns or groupings within the data without explicit instructions, which is useful for clustering and anomaly detection. By employing unsupervised learning, Rapid Innovation helps clients uncover hidden insights in their data, driving strategic decision-making.
- Reinforcement Learning: This methodology involves training agents through trial and error. Agents learn to make decisions by receiving rewards or penalties based on their actions, making it particularly effective in dynamic environments, such as game playing or robotic control. Rapid Innovation leverages reinforcement learning to optimize processes, leading to increased efficiency and cost savings for clients.
- Transfer Learning: This technique allows AI agents to leverage knowledge gained from one task to improve performance on a different but related task, reducing the amount of data and time needed for training. Rapid Innovation applies transfer learning to accelerate project timelines, enabling clients to achieve faster returns on investment.
- Few-Shot Learning: This is an emerging methodology where AI agents learn to generalize from a very small number of training examples, which is particularly useful in scenarios where data is scarce. Rapid Innovation employs few-shot learning to help clients develop robust AI solutions even in data-limited environments, maximizing their potential for success.
8.4. Deployment Models
Deployment models refer to the various ways AI systems can be implemented and utilized in real-world applications. Choosing the right deployment model is essential for optimizing performance, scalability, and accessibility.
- On-Premises Deployment: In this model, AI systems are installed and run on local servers within an organization. This offers greater control over data security and compliance but may require significant infrastructure investment. Rapid Innovation assists clients in setting up on-premises solutions tailored to their specific security and compliance needs.
- Cloud-Based Deployment: AI systems can be deployed on cloud platforms, allowing for scalability and flexibility. This model enables organizations to access powerful computing resources without the need for extensive hardware. Rapid Innovation helps clients leverage cloud-based solutions to enhance scalability and reduce operational costs.
- Edge Computing: This deployment model involves processing data closer to the source, such as IoT devices. It reduces latency and bandwidth usage, making it ideal for real-time applications like autonomous vehicles and smart sensors. Rapid Innovation's expertise in edge computing allows clients to implement real-time solutions that enhance operational efficiency.
- Hybrid Deployment: A combination of on-premises and cloud-based solutions, hybrid deployment allows organizations to balance control and scalability. Sensitive data can be processed on-premises, while less critical tasks can be handled in the cloud. Rapid Innovation guides clients in designing hybrid solutions that optimize resource utilization and data management.
- Containerization: This approach involves packaging AI applications into containers, which can be easily deployed across different environments. It enhances portability and consistency, making it easier to manage and scale AI solutions. Rapid Innovation utilizes containerization to streamline deployment processes, ensuring clients can quickly adapt to changing business needs.
8.5. Continuous Learning Frameworks
Continuous learning frameworks are essential for ensuring that AI agents remain effective and relevant over time. These frameworks enable AI systems to adapt to new information and changing environments without requiring complete retraining.
- Online Learning: This method allows AI agents to learn incrementally from new data as it becomes available, which is particularly useful in dynamic environments where data is constantly changing. Rapid Innovation implements online learning to ensure that client systems remain up-to-date and responsive to market changes.
- Lifelong Learning: This approach focuses on enabling AI agents to retain knowledge from previous tasks while learning new ones, aiming to prevent catastrophic forgetting, where the agent loses previously acquired knowledge. Rapid Innovation's lifelong learning strategies help clients maintain the effectiveness of their AI systems over time.
- Active Learning: In this framework, AI agents actively query for new data points that would be most beneficial for improving their performance, which is particularly useful in scenarios where labeling data is expensive or time-consuming. Rapid Innovation employs active learning to optimize data collection processes, enhancing the overall efficiency of client projects.
- Federated Learning: This decentralized approach allows multiple AI agents to learn collaboratively from distributed data sources while keeping the data localized. It enhances privacy and security while still benefiting from collective learning. Rapid Innovation's expertise in federated learning enables clients to harness the power of collaborative AI while ensuring data privacy.
- Model Adaptation: Continuous learning frameworks often include mechanisms for adapting existing models to new tasks or environments. This can involve fine-tuning pre-trained models or incorporating new data into existing frameworks. Rapid Innovation assists clients in model adaptation, ensuring their AI systems remain relevant and effective in evolving business landscapes.
By implementing these AI training methodologies, deployment models, and continuous learning frameworks, organizations can develop robust AI systems that are capable of evolving and improving over time. Rapid Innovation is committed to helping clients achieve their business goals efficiently and effectively through tailored AI solutions that drive greater ROI.
8.6. Performance Monitoring and Evaluation
Performance monitoring and evaluation are critical components in ensuring that systems, particularly those driven by artificial intelligence (AI), function effectively and meet their intended goals. This process involves systematically assessing the performance of AI systems to ensure they are delivering accurate, reliable, and fair outcomes.
- Establishing Key Performance Indicators (KPIs): Â
- Define specific metrics that align with the objectives of the AI system.
- KPIs may include accuracy, speed, user satisfaction, and compliance with ethical standards.
- Continuous Data Collection: Â
- Implement mechanisms for ongoing data collection to monitor system performance.
- Use real-time analytics to identify trends and anomalies in the AI's decision-making processes.
- Regular Audits and Reviews: Â
- Conduct periodic audits to evaluate the AI system's performance against established KPIs.
- Review findings with stakeholders to ensure transparency and accountability.
- Feedback Loops: Â
- Create channels for user feedback to identify areas for improvement.
- Incorporate user insights into the evaluation process to enhance system performance.
- Adaptation and Improvement: Â
- Use evaluation results to make informed adjustments to the AI system.
- Implement iterative improvements based on performance data and user feedback.
- Compliance with Regulations: Â
- Ensure that performance monitoring aligns with industry regulations and standards.
- Stay updated on legal requirements related to AI performance and accountability.
9. Ethical and Governance Considerations
Ethical and governance considerations are paramount in the development and deployment of AI systems. These frameworks guide the responsible use of AI technologies, ensuring they are aligned with societal values and norms.
- Establishing Ethical Guidelines: Â
- Develop a set of ethical principles to guide AI development and implementation.
- Principles may include fairness, accountability, transparency, and respect for user privacy.
- Governance Frameworks: Â
- Create governance structures that oversee AI projects and ensure compliance with ethical standards.
- Involve diverse stakeholders in governance discussions to reflect a wide range of perspectives.
- Risk Assessment: Â
- Conduct thorough risk assessments to identify potential ethical dilemmas and governance challenges.
- Evaluate the impact of AI systems on various stakeholders, including marginalized communities.
- Training and Awareness: Â
- Provide training for developers and users on ethical AI practices.
- Foster a culture of ethical awareness within organizations that develop or utilize AI technologies.
- Public Engagement: Â
- Engage with the public to understand societal concerns regarding AI.
- Incorporate public input into the governance and ethical frameworks of AI systems.
9.1. Transparency in AI Decision-Making
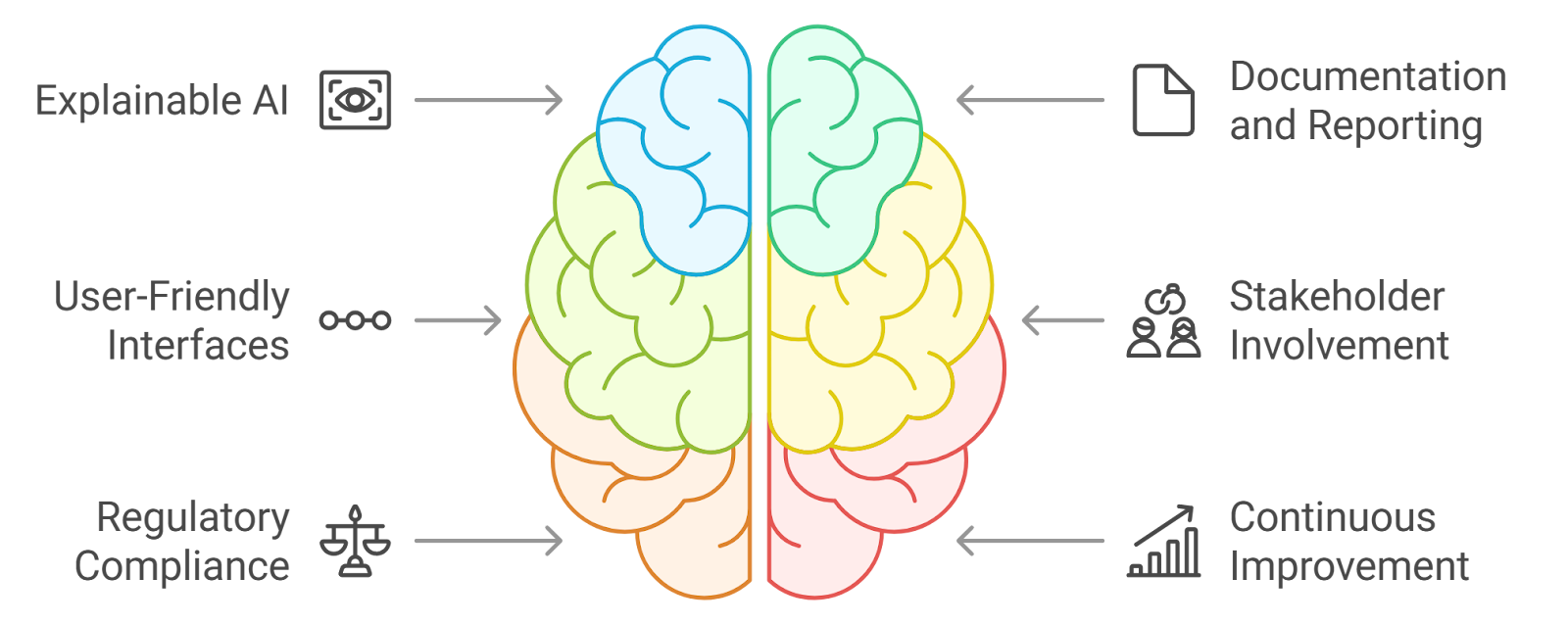
‍
Transparency in AI decision-making is essential for building trust and ensuring accountability. It involves making the processes and criteria used by AI systems clear and understandable to users and stakeholders.
- Explainable AI (XAI): Â
- Develop AI models that provide clear explanations for their decisions.
- Use techniques that allow users to understand how inputs lead to specific outputs.
- Documentation and Reporting: Â
- Maintain comprehensive documentation of AI algorithms, data sources, and decision-making processes.
- Regularly report on the performance and impact of AI systems to stakeholders.
- User-Friendly Interfaces: Â
- Design interfaces that present AI decision-making processes in an accessible manner.
- Use visualizations and simplified language to help users grasp complex AI functionalities.
- Stakeholder Involvement: Â
- Involve stakeholders in discussions about AI transparency.
- Gather input on what information is necessary for users to feel informed and secure.
- Regulatory Compliance: Â
- Adhere to regulations that mandate transparency in AI systems.
- Stay informed about evolving legal standards related to AI transparency and accountability.
- Continuous Improvement: Â
- Regularly assess and enhance transparency measures based on user feedback and technological advancements.
- Strive for ongoing improvements in how AI systems communicate their decision-making processes.
At Rapid Innovation, we leverage our expertise in AI performance monitoring and evaluation and Blockchain to help clients implement robust performance monitoring and evaluation frameworks. By establishing clear KPIs and utilizing real-time analytics, we ensure that your AI systems not only meet but exceed performance expectations, ultimately driving greater ROI for your business. Our commitment to ethical guidelines and transparency further enhances stakeholder trust, positioning your organization as a leader in responsible AI deployment.
9.2. Accountability Mechanisms
Accountability mechanisms are essential in ensuring that organizations and individuals are held responsible for their actions, particularly in the context of technology and data usage. These mechanisms help to establish trust and transparency in systems that rely on algorithms and data processing.
- Clear guidelines and policies should be established to define roles and responsibilities. Rapid Innovation can assist in developing these frameworks, ensuring that all stakeholders understand their obligations and the ethical implications of their actions, particularly regarding accountability mechanisms in technology.
- Regular audits and assessments can help identify areas of non-compliance or ethical concerns. Our team can implement robust auditing processes that leverage AI to analyze data usage and algorithm performance, providing actionable insights for improvement.
- Stakeholder engagement is crucial; involving the community can provide insights and foster a sense of shared responsibility. Rapid Innovation emphasizes collaborative approaches, ensuring that all voices are heard in the development and deployment of technology.
- Whistleblower protections can encourage individuals to report unethical practices without fear of retaliation. We can help organizations establish secure channels for reporting, fostering a culture of accountability.
- Transparency reports can be published to inform the public about data usage, algorithm performance, and any incidents of misuse. Our expertise in blockchain technology can enhance the integrity of these reports, ensuring that they are tamper-proof and verifiable.
Implementing these mechanisms can lead to improved governance and ethical standards in technology, ensuring that organizations are accountable for their actions. For a comprehensive understanding of the tools available, refer to the ultimate guide to AI platforms.
9.3. Privacy Protection
Privacy protection is a fundamental aspect of data management, especially in an era where personal information is frequently collected and analyzed. Ensuring privacy involves safeguarding individuals' data from unauthorized access and misuse.
- Data minimization principles should be applied, collecting only the information necessary for specific purposes. Rapid Innovation can guide organizations in implementing these principles effectively, optimizing data collection processes.
- Strong encryption methods can protect data both in transit and at rest, reducing the risk of breaches. Our team specializes in advanced encryption techniques, ensuring that sensitive information remains secure.
- User consent is vital; individuals should have control over their data and be informed about how it will be used. We can help design user-friendly consent management systems that empower individuals while ensuring compliance with regulations.
- Regular privacy impact assessments can help organizations identify potential risks and implement necessary safeguards. Rapid Innovation offers comprehensive assessment services, utilizing AI to predict and mitigate privacy risks.
- Compliance with regulations such as GDPR and CCPA is essential to ensure that privacy rights are upheld. Our consulting services can help organizations navigate complex regulatory landscapes, ensuring adherence to all relevant laws.
By prioritizing privacy protection, organizations can build trust with users and mitigate the risks associated with data breaches and misuse.
9.4. Algorithmic Bias Mitigation
Algorithmic bias refers to the systematic and unfair discrimination that can occur when algorithms produce results that are prejudiced due to flawed data or design. Mitigating this bias is crucial for ensuring fairness and equity in automated decision-making processes.
- Diverse data sets should be used to train algorithms, ensuring representation across different demographics. Rapid Innovation can assist in curating diverse datasets that reflect the populations they serve, enhancing the fairness of AI systems.
- Regular audits of algorithms can help identify and rectify biases that may emerge over time. Our team employs AI-driven auditing tools to continuously monitor algorithm performance and detect biases proactively.
- Incorporating fairness metrics into the evaluation of algorithms can provide insights into their performance across various groups. We can help organizations define and implement these metrics, ensuring that fairness is a core component of their AI strategy.
- Engaging interdisciplinary teams, including ethicists and social scientists, can help identify potential biases and develop more equitable solutions. Rapid Innovation promotes collaboration across disciplines, ensuring that diverse perspectives inform technology development.
- Continuous monitoring and feedback loops can help organizations adapt and improve their algorithms in response to real-world outcomes. Our solutions include real-time monitoring systems that provide ongoing feedback, enabling organizations to make data-driven adjustments.
Addressing algorithmic bias is essential for creating systems that are fair, transparent, and beneficial for all users. Rapid Innovation is committed to helping organizations achieve these goals through our expertise in AI Copilot development and blockchain technologies.
9.5. Human-AI Collaboration Protocols
Human-AI collaboration protocols are essential frameworks that guide the interaction between humans and artificial intelligence systems. These protocols ensure that both parties work together effectively, enhancing productivity and decision-making.
- Clear Communication: Establishing clear communication channels between humans and AI is crucial. This includes defining how AI will present information and how humans can provide feedback.
- Role Definition: Clearly defining the roles of humans and AI helps in understanding responsibilities. For instance, AI can handle data analysis while humans focus on strategic decision-making.
- Trust Building: Trust is vital for effective collaboration. AI systems should be transparent in their operations, allowing users to understand how decisions are made.
- Continuous Learning: Both humans and AI should engage in continuous learning. AI can adapt based on human feedback, while humans can learn to interpret AI outputs better.
- Ethical Considerations: Protocols must address ethical concerns, ensuring that AI systems operate within moral boundaries and respect user privacy. This includes the implementation of best practices for AI data privacy to ensure ethical interactions.
9.6. Regulatory Compliance
Regulatory compliance refers to the adherence to laws, regulations, and guidelines governing the use of AI technologies. As AI continues to evolve, regulatory frameworks are becoming increasingly important to ensure safety, fairness, and accountability.
- Data Protection: Compliance with data protection laws, such as GDPR, is essential. Organizations must ensure that AI systems handle personal data responsibly and transparently.
- Algorithmic Accountability: Regulations may require organizations to explain how their AI algorithms work, especially in high-stakes areas like finance and healthcare. This promotes accountability and reduces bias.
- Industry-Specific Regulations: Different industries may have specific regulations governing AI use. For example, the healthcare sector has strict guidelines to protect patient data and ensure ethical AI applications.
- Regular Audits: Organizations should conduct regular audits of their AI systems to ensure compliance with existing regulations. This helps identify potential risks and areas for improvement.
- Stakeholder Engagement: Engaging with stakeholders, including regulators, consumers, and advocacy groups, is crucial for understanding compliance requirements and addressing concerns.
10. Challenges and Limitations

‍
Despite the advancements in AI technology, several challenges and limitations hinder its widespread adoption and effectiveness.
- Data Quality: AI systems rely heavily on data. Poor-quality data can lead to inaccurate predictions and decisions. Ensuring high-quality, relevant data is a significant challenge.
- Bias and Fairness: AI algorithms can inadvertently perpetuate biases present in training data. Addressing bias is essential to ensure fairness and equity in AI applications.
- Interpretability: Many AI models, especially deep learning systems, operate as "black boxes," making it difficult for users to understand how decisions are made. This lack of interpretability can hinder trust and adoption.
- Integration with Existing Systems: Integrating AI into existing workflows and systems can be complex. Organizations may face technical challenges and resistance from employees accustomed to traditional methods.
- Ethical Concerns: The ethical implications of AI, such as job displacement and privacy issues, pose significant challenges. Organizations must navigate these concerns while implementing AI solutions.
- Resource Constraints: Developing and maintaining AI systems can be resource-intensive. Smaller organizations may struggle to allocate the necessary funds and expertise to leverage AI effectively.
At Rapid Innovation, we understand these challenges and are committed to providing tailored AI and Blockchain solutions that help organizations overcome these hurdles. By leveraging our expertise, clients can achieve greater ROI through efficient implementation, compliance with regulations, and enhanced collaboration between human and AI systems. Our approach ensures that businesses not only adopt cutting-edge technology but also do so in a manner that aligns with their strategic goals and ethical standards.
10.1. Data Quality and Reliability
Data quality and reliability are critical components in any data-driven decision-making process. High-quality data ensures that the insights derived from it are accurate and actionable, ultimately leading to greater ROI for businesses. Poor data quality can lead to misleading conclusions and ineffective strategies. Key aspects of data quality include:
- Accuracy: Data must be correct and free from errors. Inaccurate data can skew results and lead to poor decision-making, which can be costly for organizations.
- Completeness: Data should be comprehensive, covering all necessary aspects of the subject matter. Missing data can create gaps in analysis, hindering effective strategy formulation.
- Consistency: Data should be uniform across different datasets. Inconsistencies can arise from different data entry methods or sources, complicating the analysis process.
- Timeliness: Data must be up-to-date to be relevant. Outdated data can lead to decisions based on obsolete information, negatively impacting business outcomes.
- Reliability: Data sources should be trustworthy. Using data from unreliable sources can compromise the integrity of the analysis, leading to misguided strategies. The trustworthiness of data and data quality refers to the assurance that the data is both accurate and reliable.
At Rapid Innovation, we employ advanced data governance frameworks and regular audits to ensure high standards of data quality and reliability. By leveraging AI-driven data cleaning techniques, we help organizations maintain the integrity of their data, ultimately enhancing decision-making processes and driving better business results. Our focus on data accuracy and reliability ensures that our clients can confidently rely on the insights generated from their data. For organizations looking to enhance their data capabilities, our custom AI model development services can provide tailored solutions to meet specific needs.
10.2. Computational Complexity
Computational complexity refers to the amount of computational resources required to solve a problem or execute an algorithm. It is a crucial consideration in the fields of computer science and data analysis, as it directly impacts performance and scalability. Important factors include:
- Time Complexity: This measures how the time to complete a task grows with the size of the input data. Algorithms with high time complexity can become impractical as data volumes increase.
- Space Complexity: This refers to the amount of memory required by an algorithm as the input size grows. High space complexity can lead to resource exhaustion.
- Scalability: Algorithms should be designed to handle increasing amounts of data efficiently. Poorly designed algorithms may struggle with larger datasets, leading to slow performance.
- Algorithm Selection: Choosing the right algorithm is essential. Some algorithms are more efficient for specific types of data or problems, impacting overall computational complexity.
- Parallel Processing: Utilizing multiple processors can help reduce computational time. This approach is particularly useful for large datasets and complex calculations.
Understanding computational complexity helps organizations optimize their data processing capabilities, ensuring that they can handle large volumes of data without sacrificing performance. At Rapid Innovation, we specialize in developing scalable AI solutions that are tailored to meet the unique needs of our clients, enabling them to achieve greater efficiency and ROI.
10.3. Interpretability Issues
Interpretability refers to the degree to which a human can understand the cause of a decision made by a model. In the context of machine learning and data analysis, interpretability is vital for trust and transparency. Key considerations include:
- Model Complexity: Complex models, such as deep learning networks, often act as "black boxes," making it difficult to understand how they arrive at specific decisions. Simpler models tend to be more interpretable.
- Feature Importance: Understanding which features influence model predictions is crucial. Techniques like SHAP (SHapley Additive exPlanations) can help elucidate feature contributions.
- Regulatory Compliance: In many industries, regulations require that decisions made by algorithms be explainable. This is particularly important in sectors like finance and healthcare.
- User Trust: Stakeholders are more likely to trust models that provide clear explanations for their predictions. Lack of interpretability can lead to skepticism and resistance to adopting data-driven solutions.
- Bias Detection: Interpretability aids in identifying biases in models. Understanding how a model makes decisions can help ensure fairness and equity in outcomes.
At Rapid Innovation, we prioritize interpretability in our AI solutions, ensuring that our clients can trust the insights generated by their models. By addressing interpretability issues, we help organizations foster trust in data-driven systems, enabling stakeholders to make informed decisions based on model outputs. This commitment to transparency not only enhances user trust but also aligns with regulatory compliance, ultimately driving better business outcomes. Our focus on reliability in data quality ensures that the insights provided are both trustworthy and actionable.
10.4. Resistance to Technological Change
Resistance to technological change is a common phenomenon in various sectors, often stemming from fear, uncertainty, and a lack of understanding. Employees may feel threatened by new technologies that could potentially replace their jobs or alter their roles significantly. This resistance can manifest in several ways:
- Fear of job loss: Many workers worry that automation and advanced technologies will render their skills obsolete.
- Lack of understanding: Employees may not fully grasp how new technologies work or how they can enhance their productivity.
- Comfort with the status quo: People often prefer familiar processes and may resist changes that disrupt their routine.
- Concerns about training: Employees may feel overwhelmed by the prospect of learning new systems, especially if they believe they lack the necessary skills.
Organizations can mitigate resistance to technological change by fostering a culture of openness and communication. Providing clear information about the benefits of technological change can help alleviate fears. Additionally, involving employees in the decision-making process can create a sense of ownership and reduce resistance. Training programs that focus on upskilling can also empower employees to embrace new technologies rather than fear them. At Rapid Innovation, we specialize in guiding organizations through this transition by implementing tailored AI and Blockchain solutions that not only enhance productivity but also ensure that employees feel supported and informed throughout the process.
10.5. Ethical Dilemmas
Ethical dilemmas arise in the context of technological advancements, particularly when considering the implications of artificial intelligence, data privacy, and automation. These dilemmas can pose significant challenges for organizations and society as a whole:
- Data privacy concerns: With the rise of big data, organizations must navigate the ethical implications of collecting, storing, and using personal information. Misuse of data can lead to breaches of trust and legal repercussions.
- Job displacement: Automation and AI can lead to significant job losses, raising ethical questions about the responsibility of companies to their employees and the broader community.
- Bias in algorithms: AI systems can perpetuate existing biases if not designed and monitored carefully. This can lead to unfair treatment of certain groups, raising ethical concerns about equity and justice.
Organizations must develop ethical frameworks to guide their technological initiatives. This includes establishing clear policies on data usage, ensuring transparency in AI algorithms, and considering the social impact of automation. Engaging stakeholders in discussions about ethical practices can also help organizations navigate these complex dilemmas. Rapid Innovation is committed to helping clients establish these frameworks, ensuring that their AI and Blockchain implementations are not only effective but also ethically sound.
10.6. Skill Gap and Training Needs
The rapid pace of technological advancement has created a significant skill gap in the workforce. Many employees find themselves lacking the necessary skills to adapt to new technologies, which can hinder organizational growth and innovation. Addressing this skill gap is crucial for both employees and employers:
- Identifying skill gaps: Organizations need to assess the current skill levels of their workforce and identify areas where training is needed. This can involve surveys, performance reviews, and industry benchmarking.
- Providing training programs: Companies should invest in training initiatives that focus on upskilling employees. This can include workshops, online courses, and mentorship programs tailored to specific technologies.
- Encouraging continuous learning: Fostering a culture of continuous learning can help employees stay updated with the latest technological trends. This can be achieved through regular training sessions, access to online resources, and support for professional development.
By addressing the skill gap and providing adequate training, organizations can enhance employee confidence and productivity. This not only benefits the workforce but also positions the organization for success in an increasingly technology-driven landscape. At Rapid Innovation, we offer comprehensive training solutions that empower employees to harness the full potential of AI and Blockchain technologies, ultimately driving greater ROI for our clients.
11. Economic and Strategic Implications

‍
The economic and strategic implications of any initiative or policy are crucial for understanding its overall impact on society and the market. These implications can be analyzed through various lenses, including economic implications analysis, cost-benefit analysis, and productivity enhancement.
11.1 Cost-Benefit Analysis
Cost-benefit analysis (CBA) is a systematic approach to evaluating the economic pros and cons of a project or decision. It helps stakeholders determine whether the benefits outweigh the costs, guiding them in making informed choices.
- Definition: CBA quantifies the total expected costs against the total expected benefits of a project.
- Components: Â
- Direct Costs: Expenses directly associated with the project, such as materials, labor, and overhead.
- Indirect Costs: Costs that are not directly tied to the project but may arise, such as environmental impacts or social costs.
- Benefits: Positive outcomes that can be quantified, including increased revenue, improved efficiency, and enhanced quality of life.
- Importance: Â
- Informed Decision-Making: CBA provides a clear framework for decision-makers to evaluate the feasibility of a project.
- Resource Allocation: Helps in prioritizing projects that yield the highest net benefits.
- Risk Assessment: Identifies potential risks and uncertainties associated with the project, allowing for better planning.
- Challenges: Â
- Quantification Difficulties: Some benefits, like social or environmental impacts, can be hard to quantify.
- Subjectivity: Different stakeholders may have varying perspectives on what constitutes a benefit or cost.
CBA is widely used in various sectors, including public policy, healthcare, and infrastructure development. For instance, a study by the National Institute of Health found that every dollar spent on public health initiatives can yield a return of up to $10 in economic benefits.
11.2 Productivity Enhancement
Productivity enhancement refers to the strategies and practices aimed at increasing the efficiency and output of a workforce or organization. It is a critical factor in economic growth and competitiveness.
- Definition: Productivity is measured as the ratio of outputs to inputs in the production process.
- Key Factors: Â
- Technology Adoption: Implementing new technologies, such as AI and blockchain solutions, can streamline processes and reduce time spent on tasks. For example, Rapid Innovation has helped clients integrate AI-driven analytics to optimize supply chain management, resulting in significant cost savings and improved delivery times.
- Employee Training: Investing in workforce development enhances skills and knowledge, leading to better performance. Rapid Innovation offers tailored training programs that equip teams with the necessary skills to leverage AI and blockchain technologies effectively.
- Process Optimization: Analyzing and refining workflows can eliminate bottlenecks and improve efficiency. Our consulting services include process mapping and optimization strategies that have led clients to achieve higher operational efficiency.
- Benefits: Â
- Increased Output: Higher productivity leads to more goods and services being produced with the same resources.
- Cost Reduction: Efficient processes can lower operational costs, improving profit margins. For instance, clients who have adopted our blockchain solutions have reported reduced transaction costs and enhanced transparency.
- Competitive Advantage: Organizations that enhance productivity can outperform competitors, capturing a larger market share.
- Challenges: Â
- Resistance to Change: Employees may be hesitant to adopt new technologies or processes.
- Initial Investment: Upfront costs for training and technology can be significant, though they often pay off in the long run.
According to the Bureau of Labor Statistics, productivity in the non-farm business sector increased by 2.3% in 2021, highlighting the importance of continuous improvement in productivity for economic growth.
In conclusion, understanding the economic and strategic implications through economic implications analysis, cost-benefit analysis, and productivity enhancement is essential for making informed decisions that drive growth and efficiency.
11.3. Innovation Potential
Innovation potential refers to the capacity of an organization, region, or country to develop new ideas, products, and processes that can lead to economic growth and improved quality of life. It is a critical factor in maintaining a competitive edge in today's fast-paced global market. At Rapid Innovation, we leverage our expertise in AI and Blockchain to enhance this potential for our clients, ensuring they stay ahead of the curve.
- Research and Development (R&D) Investment: A strong commitment to R&D is essential for fostering innovation. Countries that allocate significant resources to R&D often see higher rates of technological advancement and economic growth. Rapid Innovation assists clients in optimizing their R&D investments by integrating AI-driven analytics to identify high-impact projects, thereby maximizing ROI.
- Education and Talent Development: A skilled workforce is vital for innovation. Educational institutions must focus on STEM (Science, Technology, Engineering, and Mathematics) fields to prepare students for future challenges. We partner with organizations to develop tailored training programs that enhance AI and Blockchain skills, ensuring that teams are equipped to drive innovation.
- Collaboration and Networking: Innovation thrives in environments where collaboration occurs. Partnerships between businesses, universities, and government can lead to groundbreaking discoveries and advancements. Rapid Innovation facilitates strategic partnerships, enabling clients to access cutting-edge technologies and insights that foster collaborative innovation.
- Intellectual Property Protection: Strong IP laws encourage innovation by ensuring that inventors can protect their creations and benefit from their work. We guide clients in navigating the complexities of IP protection in the AI and Blockchain domains, helping them safeguard their innovations and enhance their market position.
- Access to Funding: Startups and innovative companies often require funding to develop their ideas. Access to venture capital and government grants can significantly enhance innovation potential. Rapid Innovation provides consulting services to help clients identify and secure funding opportunities, ensuring they have the resources needed to bring their innovative ideas to fruition.
- Market Potential of Idea Innovation: Understanding the market potential of idea innovation is crucial for organizations looking to capitalize on their innovative capabilities. By analyzing market trends and consumer needs, Rapid Innovation helps clients identify opportunities that align with their innovation potential.
- Innovation Potential Meaning: The meaning of innovation potential extends beyond mere idea generation; it encompasses the ability to implement and scale innovations effectively. Rapid Innovation works with clients to translate their innovative ideas into actionable strategies that drive growth.
- Innovation Potential Example: A notable example of innovation potential can be seen in companies that have successfully integrated AI and Blockchain technologies to create new business models. Rapid Innovation showcases such examples to inspire clients and demonstrate the tangible benefits of harnessing innovation.
- Increased Potential for Innovation: Organizations that embrace a culture of continuous improvement and adaptability often experience increased potential for innovation. Rapid Innovation supports clients in fostering such a culture, enabling them to respond swiftly to market changes and emerging technologies. Additionally, our expertise in generative AI development can help clients explore new avenues for innovation and investment.
11.4. Global Competitiveness
Global competitiveness refers to the ability of a country or organization to compete effectively in the international market. It encompasses various factors that contribute to economic performance and market presence. Rapid Innovation empowers clients to enhance their global competitiveness through advanced AI and Blockchain solutions.
- Economic Stability: A stable economy attracts foreign investment and fosters business growth. Countries with low inflation and sound fiscal policies tend to be more competitive. Our solutions help clients streamline operations and reduce costs, contributing to overall economic stability.
- Infrastructure Development: Efficient transportation, communication, and energy systems are crucial for enhancing competitiveness. Well-developed infrastructure reduces costs and improves productivity. Rapid Innovation leverages Blockchain technology to optimize supply chain management, ensuring efficient resource allocation and operational excellence.
- Regulatory Environment: A business-friendly regulatory framework encourages entrepreneurship and innovation. Simplified regulations can help businesses thrive and attract foreign investment. We assist clients in navigating regulatory landscapes, particularly in the AI and Blockchain sectors, to ensure compliance while fostering innovation.
- Market Size and Access: Larger markets provide more opportunities for businesses to grow. Countries with access to regional and global markets can leverage their competitive advantages. Rapid Innovation helps clients expand their market reach through AI-driven market analysis and Blockchain-enabled cross-border transactions.
- Workforce Quality: A highly skilled and adaptable workforce is essential for maintaining competitiveness. Continuous training and education programs can help workers meet evolving market demands. We offer customized training solutions in AI and Blockchain to ensure that clients' teams remain at the forefront of industry advancements.
11.5. Long-Term Policy Strategy
A long-term policy strategy is essential for sustainable growth and development. It involves creating a comprehensive plan that addresses economic, social, and environmental challenges over an extended period. Rapid Innovation supports clients in formulating and implementing effective long-term strategies that leverage AI and Blockchain technologies.
- Vision and Goals: Establishing a clear vision and specific goals helps guide policy decisions. This ensures that all stakeholders are aligned and working towards common objectives. We collaborate with clients to define their strategic vision, ensuring alignment with technological advancements.
- Stakeholder Engagement: Involving various stakeholders, including businesses, communities, and government agencies, is crucial for developing effective policies. Collaborative approaches lead to more comprehensive solutions. Rapid Innovation facilitates stakeholder engagement through workshops and collaborative platforms, fostering a culture of innovation.
- Evidence-Based Decision Making: Policies should be based on data and research to ensure they are effective and relevant. Regular assessments can help identify areas for improvement. Our AI solutions provide clients with actionable insights derived from data analytics, enabling informed decision-making.
- Flexibility and Adaptability: Long-term strategies must be adaptable to changing circumstances. Policymakers should be prepared to revise plans in response to new challenges and opportunities. Rapid Innovation emphasizes the importance of agility in strategy formulation, ensuring clients can pivot as needed.
- Sustainability Focus: Incorporating sustainability into policy strategies ensures that economic growth does not come at the expense of environmental health. This approach promotes long-term viability and resilience. We advocate for sustainable practices in AI and Blockchain implementations, helping clients achieve their business goals while contributing to a healthier planet.
12. Future Trends and Outlook
The future of technology is rapidly evolving, with significant advancements on the horizon. Two key areas that are expected to shape the future landscape are emerging AI technologies and the integration of quantum computing.
12.1 Emerging AI Technologies
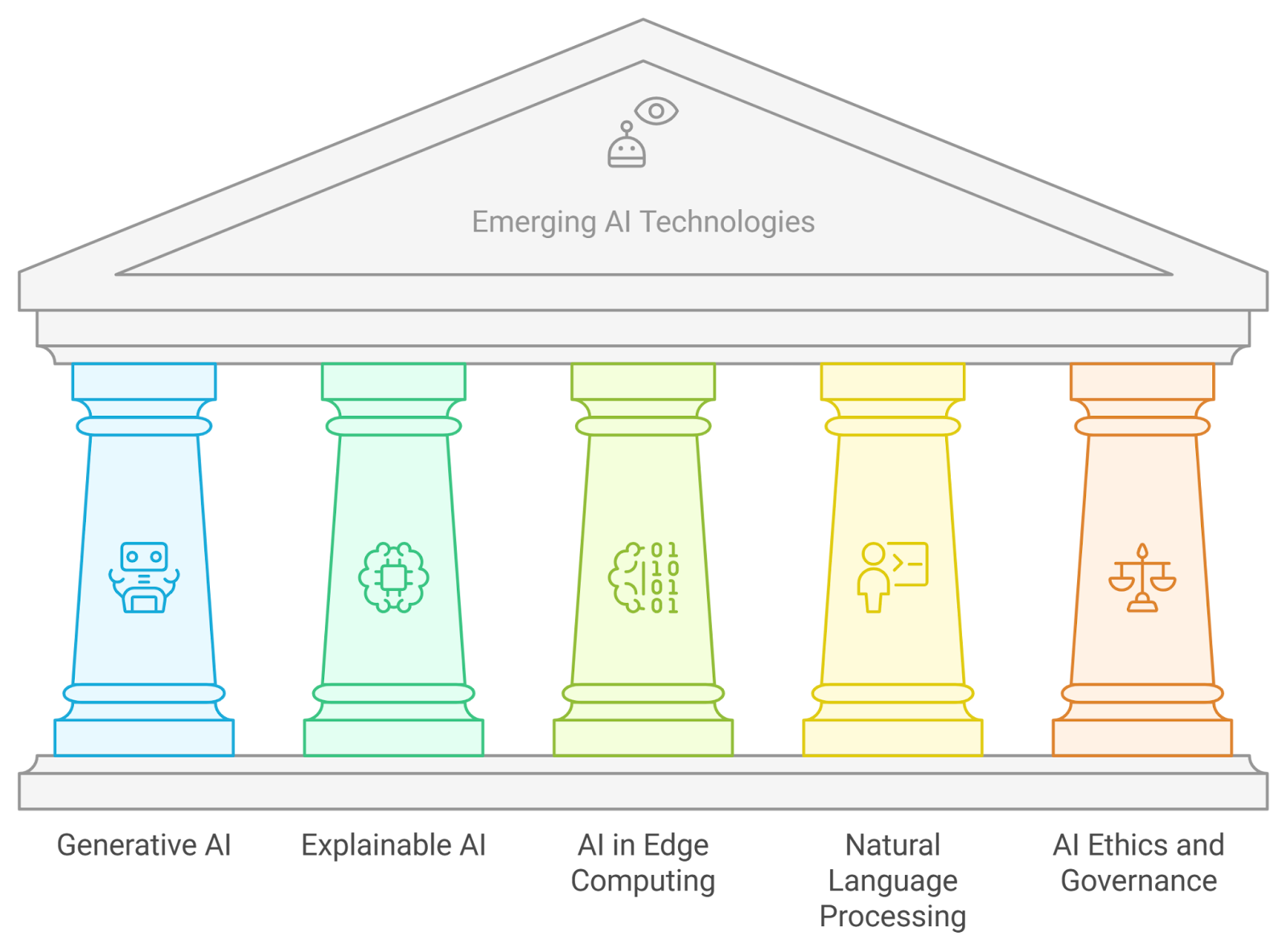
‍
Emerging AI technologies are set to revolutionize various industries, enhancing efficiency and creating new opportunities. Some notable trends include:
- Generative AI: This technology enables machines to create content, from text to images and music. Tools like OpenAI's ChatGPT and DALL-E exemplify generative AI, producing human-like text and unique images, respectively. Rapid Innovation can help businesses leverage generative AI to enhance marketing strategies and content creation, ultimately driving greater engagement and ROI. The latest AI technology is also focusing on generative capabilities, making it a hot topic in the field.
- Explainable AI (XAI): As AI systems become more complex, the need for transparency grows. XAI focuses on making AI decisions understandable to humans, which is crucial for sectors like healthcare and finance where accountability is essential. Rapid Innovation can assist organizations in implementing XAI frameworks, ensuring compliance and fostering trust among stakeholders. This is part of the broader conversation around AI ethics and governance, which is increasingly relevant in discussions about new artificial intelligence technology.
- AI in Edge Computing: With the rise of IoT devices, AI is increasingly being integrated at the edge of networks. This allows for real-time data processing and decision-making, reducing latency and bandwidth usage. Rapid Innovation can guide clients in deploying edge AI solutions, optimizing operations, and enhancing customer experiences. The latest in AI technology is also seeing a significant push towards edge computing solutions.
- Natural Language Processing (NLP): Advances in NLP are enabling machines to understand and respond to human language more effectively. This has applications in customer service, content creation, and even mental health support. Rapid Innovation can help businesses implement NLP solutions to improve customer interactions and streamline internal processes. The emergence of new AI technology in NLP is transforming how businesses communicate with their customers.
- AI Ethics and Governance: As AI technologies proliferate, ethical considerations are becoming paramount. Organizations are focusing on developing frameworks to ensure responsible AI use, addressing issues like bias, privacy, and accountability. Rapid Innovation can support clients in establishing robust AI governance policies, mitigating risks, and enhancing their reputational standing. This is particularly important as we see the emergence of artificial intelligence as an emerging technology.
These emerging AI technologies are not just trends; they represent a shift in how businesses operate and interact with customers. The potential for innovation is vast, and companies that embrace these technologies will likely gain a competitive edge. The latest technology on artificial intelligence is paving the way for new applications and solutions.
12.2 Quantum Computing Integration
Quantum computing is another frontier that promises to transform industries by solving complex problems that are currently beyond the reach of classical computers. Key aspects of quantum computing integration include:
- Enhanced Computational Power: Quantum computers leverage the principles of quantum mechanics to perform calculations at unprecedented speeds. This capability can revolutionize fields such as cryptography, materials science, and drug discovery. Rapid Innovation can assist organizations in exploring quantum computing applications to unlock new business models and enhance security measures.
- Optimization Problems: Many industries face complex optimization challenges, from logistics to finance. Quantum computing can provide solutions that are more efficient than traditional methods, leading to significant cost savings and improved performance. Rapid Innovation can help clients identify and implement quantum solutions tailored to their specific optimization needs.
- Collaboration with AI: The integration of quantum computing with AI can lead to breakthroughs in machine learning algorithms. Quantum-enhanced AI could analyze vast datasets more quickly and accurately, leading to better predictions and insights. Rapid Innovation can facilitate this integration, enabling clients to harness the full potential of both technologies for superior decision-making. This collaboration is part of the broader trend of emerging technologies in AI.
- Industry Applications: Various sectors are exploring quantum computing applications, including: Â
- Pharmaceuticals: Accelerating drug discovery by simulating molecular interactions.
- Finance: Improving risk analysis and portfolio optimization.
- Supply Chain: Enhancing logistics and inventory management through better forecasting.
- Challenges and Adoption: Despite its potential, quantum computing faces challenges such as high costs, technical complexity, and a shortage of skilled professionals. However, ongoing research and investment are paving the way for broader adoption. Rapid Innovation can guide organizations through these challenges, ensuring a smooth transition to quantum technologies.
The integration of quantum computing into existing systems will require collaboration between tech companies, researchers, and policymakers. As this technology matures, it will unlock new possibilities and drive innovation across various sectors.
In conclusion, both emerging AI technologies and quantum computing integration are poised to redefine the technological landscape. Organizations that stay ahead of these trends, including the latest AI technology and other emerging technologies in AI, will be better positioned to leverage their benefits and navigate the complexities of the future. Rapid Innovation stands ready to partner with clients in this journey, providing the expertise and solutions necessary to achieve greater ROI and operational excellence.
12.3. Blockchain and Distributed Governance
Blockchain technology is revolutionizing the way governance is approached by introducing transparency, security, and decentralization. Distributed governance refers to the management of resources and decision-making processes across multiple stakeholders without a central authority.
- Enhanced Transparency: Blockchain provides a public ledger that records all transactions, making it easy to track and verify actions taken by various parties. This transparency can reduce corruption and increase trust among stakeholders, ultimately leading to a more efficient governance structure.
- Decentralization: By distributing control among multiple nodes, blockchain eliminates the need for a central authority. This can empower local communities and allow for more democratic decision-making processes, aligning with Rapid Innovation's commitment to fostering inclusive governance solutions, such as blockchain governance and decentralized governance blockchain.
- Smart Contracts: These self-executing contracts, with the terms of the agreement directly written into code, can automate governance processes. They ensure that rules are followed without the need for intermediaries, streamlining operations and reducing costs for organizations, which is a key aspect of blockchain corporate governance.
- Increased Participation: Blockchain can facilitate greater participation from citizens in governance processes. Through decentralized applications (dApps), individuals can engage in decision-making, voting, and resource allocation, enhancing civic engagement and accountability. This is particularly relevant in the context of onchain governance and crypto governance.
- Case Studies: Various governments and organizations are experimenting with blockchain for governance. For instance, Estonia has implemented blockchain for secure digital identities and e-governance services, showcasing the potential for improved efficiency and security in public administration. Other examples include blockchain in governance and blockchain in public sector initiatives.
12.4. Global Policy Coordination
Global policy coordination is essential in an increasingly interconnected world. It involves collaboration among nations to address transnational issues such as climate change, trade, and public health.
- Shared Goals: Countries must align their policies to achieve common objectives. This can include setting targets for carbon emissions or establishing trade agreements that benefit all parties involved, which can be facilitated through innovative technologies.
- Multilateral Institutions: Organizations like the United Nations and World Trade Organization play a crucial role in facilitating dialogue and cooperation among nations. They provide platforms for negotiation and conflict resolution, essential for effective global governance.
- Data Sharing: Effective global policy coordination relies on the sharing of data and best practices. Countries can learn from each other’s experiences and adapt successful strategies to their own contexts, a process that can be enhanced through AI-driven analytics.
- Challenges: Despite the importance of global coordination, challenges such as nationalism, differing priorities, and economic disparities can hinder progress. Countries may prioritize their own interests over collective goals, necessitating innovative solutions to bridge these gaps.
- Examples: The Paris Agreement on climate change is a prime example of global policy coordination, where nations commit to reducing greenhouse gas emissions to combat climate change, demonstrating the need for collaborative frameworks.
12.5. Predictive Governance Models
Predictive governance models leverage data analytics and artificial intelligence to anticipate future trends and inform decision-making. These models can enhance the efficiency and effectiveness of governance.
- Data-Driven Insights: By analyzing large datasets, predictive models can identify patterns and trends that inform policy decisions. This can lead to more proactive governance rather than reactive measures, aligning with Rapid Innovation's focus on data-driven solutions.
- Risk Management: Predictive governance can help identify potential risks and vulnerabilities in systems, allowing governments to implement preventive measures. This is particularly useful in areas like public health and disaster management, where timely interventions can save lives.
- Resource Allocation: Governments can use predictive models to optimize resource allocation. By forecasting demand for services, they can ensure that resources are directed where they are most needed, maximizing efficiency and impact.
- Citizen Engagement: Predictive governance can enhance citizen engagement by providing insights into public needs and preferences. This can lead to more tailored services and improved satisfaction, fostering a stronger relationship between governments and their constituents.
- Real-World Applications: Cities like Los Angeles and New York are using predictive analytics to improve public safety and traffic management, demonstrating the potential of these models in urban governance and the value of integrating AI solutions for better outcomes.
At Rapid Innovation, we harness the power of AI and blockchain to help clients navigate these complex governance landscapes, ensuring they achieve their business goals efficiently and effectively while maximizing their return on investment, including exploring innovative concepts like blockchain governance models and crypto governance models.
13. Implementation Roadmap
An implementation roadmap is crucial for guiding a project from conception to completion. It outlines the steps necessary to achieve the desired outcomes, ensuring that all stakeholders are aligned and that resources are allocated efficiently. The roadmap typically includes phases such as initial assessment, strategy formulation, pilot program development, and full-scale implementation. Various types of implementation roadmaps, such as safe implementation roadmap, scaled agile implementation roadmap, and power bi implementation roadmap, can be utilized depending on the project's needs.
13.1 Initial Assessment and Strategy
The initial assessment and strategy phase is foundational for any successful implementation. This phase involves evaluating the current state of the organization, identifying gaps, and determining the resources required for the project.
- Conduct a SWOT Analysis: Assess the strengths, weaknesses, opportunities, and threats related to the project. This analysis helps in understanding the internal and external factors that could impact implementation.
- Stakeholder Engagement: Identify and engage key stakeholders early in the process. Their insights and buy-in are essential for the project's success. This includes team members, management, and external partners.
- Define Objectives: Clearly outline the objectives of the project. These should be SMART (Specific, Measurable, Achievable, Relevant, Time-bound) to ensure clarity and focus.
- Resource Assessment: Evaluate the resources available, including budget, personnel, and technology. Understanding what is available helps in planning effectively.
- Risk Assessment: Identify potential risks and develop mitigation strategies. This proactive approach can save time and resources later in the project.
- Develop a Strategic Plan: Based on the assessment, create a strategic plan that outlines the steps needed to achieve the project goals. This plan should include timelines, responsibilities, and key performance indicators (KPIs) to measure success. For instance, a scaled agile framework implementation roadmap can be beneficial in structuring this plan.
13.2 Pilot Program Development
Once the initial assessment and strategy are in place, the next step is to develop a pilot program. A pilot program allows organizations to test their strategies on a smaller scale before full implementation.
- Define Pilot Objectives: Clearly outline what the pilot program aims to achieve. This could include testing new processes, technologies, or methodologies.
- Select a Target Group: Choose a specific group or department to participate in the pilot. This group should be representative of the larger organization to ensure that the results are applicable.
- Develop a Detailed Plan: Create a comprehensive plan for the pilot program, including a timeline, resources required, and roles and responsibilities. This could be part of a safe agile implementation roadmap.
- Training and Support: Provide necessary training and support to the participants. This ensures that everyone involved understands their roles and the objectives of the pilot.
- Data Collection and Analysis: Establish methods for collecting data during the pilot. This data will be crucial for evaluating the program's success and making necessary adjustments.
- Feedback Mechanism: Implement a feedback mechanism to gather insights from participants. This can include surveys, interviews, or focus groups to understand their experiences and challenges.
- Evaluate Results: After the pilot program concludes, analyze the results against the defined objectives. This evaluation will inform decisions about whether to proceed with full-scale implementation.
- Iterate and Improve: Use the insights gained from the pilot to refine the strategy and address any issues before rolling out the program organization-wide. This iterative process is essential in agile implementation roadmaps, such as the scaled agile framework roadmap.
By following these steps in the implementation roadmap, organizations can ensure a structured approach to project execution, minimizing risks and maximizing the chances of success. At Rapid Innovation, we leverage our expertise in AI and Blockchain to enhance each phase of this roadmap, ensuring that our clients achieve greater ROI through efficient and effective project execution.
13.3. Scalability Considerations
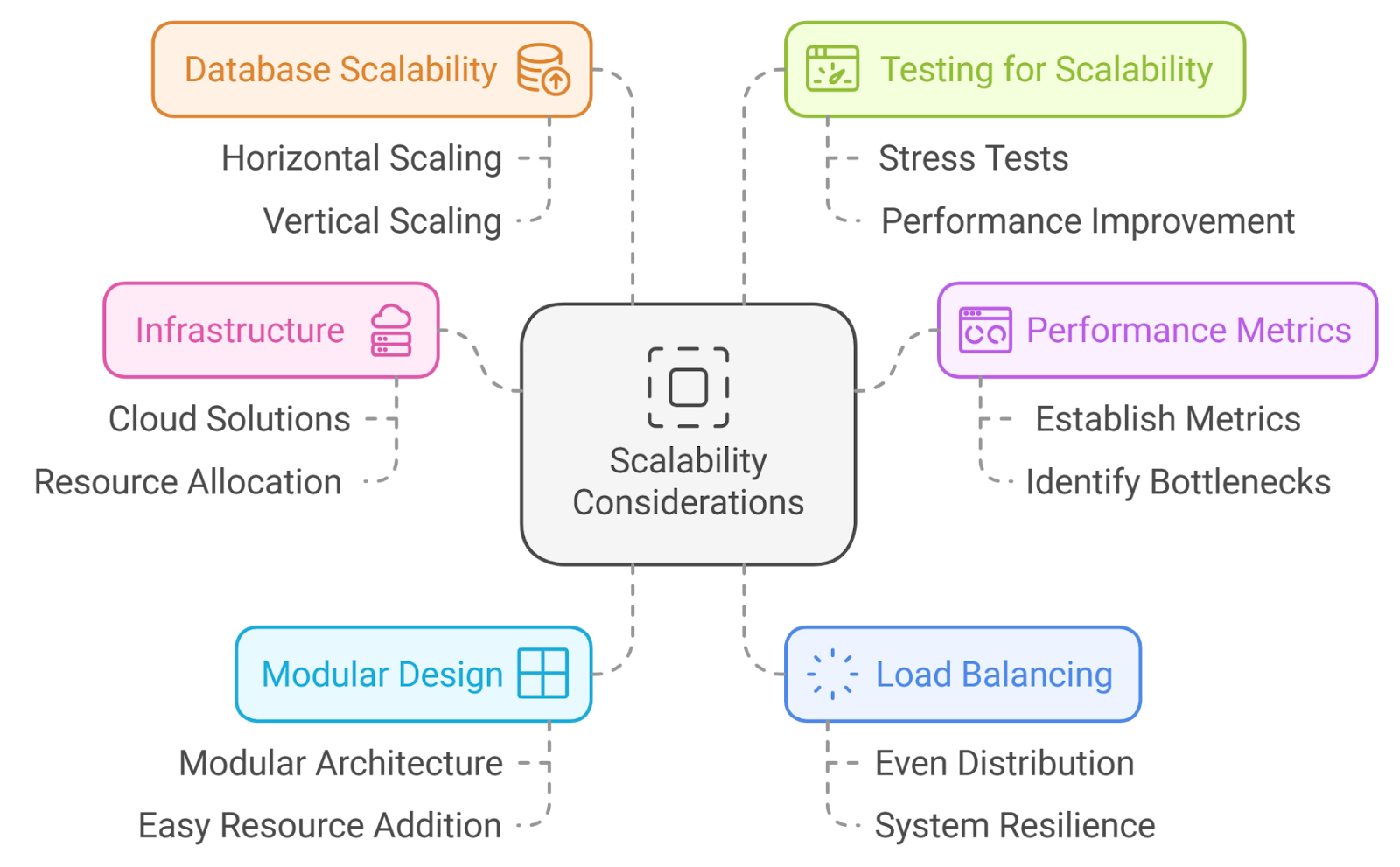
‍
Scalability is a critical aspect of any system or process, particularly in technology and business environments. It refers to the ability of a system to handle a growing amount of work or its potential to accommodate growth. Here are some key considerations for scalability:
- Infrastructure: Ensure that the underlying infrastructure can support increased loads. This may involve cloud solutions that allow for dynamic resource allocation, enabling businesses to scale their operations efficiently.
- Performance Metrics: Establish clear performance metrics to monitor system behavior under varying loads. This helps in identifying bottlenecks early, allowing for timely interventions that can enhance overall system performance.
- Modular Design: Implement a modular architecture that allows for easy addition of resources or components without significant rework. This flexibility is essential for adapting to changing business needs and technological advancements.
- Load Balancing: Utilize load balancing techniques to distribute workloads evenly across servers, enhancing performance and reliability. This ensures that no single server becomes a point of failure, thereby improving system resilience.
- Database Scalability: Consider database solutions that can scale horizontally (adding more servers) or vertically (upgrading existing servers) based on demand. This adaptability is crucial for managing data growth effectively.
- Testing for Scalability: Regularly conduct stress tests to evaluate how the system performs under peak loads and identify areas for improvement. This proactive approach helps in ensuring that the system can handle future growth without compromising performance. For those looking to enhance their language models, consider our real-world AI implementations.
13.4. Change Management
Change management is the structured approach to transitioning individuals, teams, and organizations from a current state to a desired future state. Effective change management is essential for minimizing resistance and ensuring successful implementation. Key elements include:
- Communication: Clearly communicate the reasons for change and the benefits it brings. Transparency helps in gaining buy-in from stakeholders, which is vital for successful transitions.
- Stakeholder Engagement: Involve key stakeholders early in the process to gather input and address concerns. This fosters a sense of ownership and commitment to the change initiative.
- Training and Support: Provide adequate training and resources to help employees adapt to new systems or processes. Ongoing support is crucial for long-term success and helps in mitigating resistance to change.
- Change Impact Assessment: Analyze how changes will affect various aspects of the organization, including processes, people, and technology. This comprehensive understanding aids in planning effective change strategies.
- Feedback Mechanisms: Establish channels for feedback to monitor the effectiveness of the change and make necessary adjustments. This iterative process ensures that the change remains aligned with organizational goals.
- Documentation: Maintain thorough documentation of the change process, including plans, training materials, and outcomes, to facilitate future changes. This creates a valuable resource for continuous improvement.
13.5. Continuous Improvement Framework
A continuous improvement framework is essential for organizations aiming to enhance their processes, products, and services consistently. This framework encourages a culture of ongoing enhancement and innovation. Key components include:
- Plan-Do-Check-Act (PDCA): Implement the PDCA cycle to systematically test changes and assess their impact. This iterative process promotes learning and adaptation, ensuring that improvements are sustainable.
- Data-Driven Decision Making: Utilize data analytics to identify areas for improvement and measure the effectiveness of changes. This ensures that decisions are based on evidence rather than assumptions, leading to more effective outcomes.
- Employee Involvement: Encourage employees at all levels to contribute ideas for improvement. This not only fosters engagement but also taps into diverse perspectives, driving innovation from within the organization.
- Benchmarking: Compare performance metrics against industry standards or competitors to identify gaps and opportunities for improvement. This practice helps organizations stay competitive and responsive to market changes.
- Regular Reviews: Schedule regular reviews of processes and outcomes to ensure that improvements are sustained and new opportunities are identified. This ongoing evaluation is key to maintaining a culture of excellence.
- Recognition and Rewards: Acknowledge and reward contributions to continuous improvement efforts. This reinforces a culture of innovation and motivates employees to participate actively, driving the organization toward its strategic goals.