Table Of Contents
Category
Artificial Intelligence
AIML
IoT
Blockchain
Retail & Ecommerce
1. Introduction to AI Agents in Data Analysis
Artificial Intelligence (AI) agents in data analysis are revolutionizing the way we analyze multi-dimensional data. These intelligent systems can process vast amounts of information, uncover patterns, and provide insights that would be difficult or impossible for humans to achieve alone. The integration of AI agents into data analysis offers numerous benefits, but it also presents several challenges that organizations must navigate.
AI agents can handle complex datasets, including structured and unstructured data. They utilize machine learning algorithms to improve their performance over time and can automate repetitive tasks, freeing up human analysts for more strategic work. At Rapid Innovation, we leverage these capabilities to help our clients streamline their data analysis processes, ultimately leading to greater efficiency and ROI.
The rise of big data has made traditional data analysis methods less effective. AI agents in data analysis can analyze data from various sources, such as social media, IoT devices, and transactional databases, providing a more comprehensive view of the information landscape. This capability is essential for businesses looking to make data-driven decisions in real-time. By implementing AI solutions, Rapid Innovation enables clients to harness the full potential of their data, driving informed decision-making and strategic growth.
Benefits of AI agents in data analysis include: - Enhanced decision-making through predictive analytics, allowing businesses to anticipate market trends and customer needs. - Improved accuracy in identifying trends and anomalies, which can lead to more effective marketing strategies and operational efficiencies. - Ability to process data at unprecedented speeds, enabling organizations to respond swiftly to changing market conditions.
However, the implementation of AI agents in data analysis is not without its challenges. Organizations must consider factors such as data quality, algorithm bias, and the need for skilled personnel to manage these systems. Rapid Innovation provides consulting services to help clients navigate these challenges, ensuring that they can effectively integrate AI into their operations.
Challenges include: - Data quality issues can lead to inaccurate insights, which we address through robust data governance frameworks. - Algorithm bias can result in skewed analysis and decision-making; our team emphasizes ethical AI practices to mitigate this risk. - The demand for skilled data scientists and AI specialists is increasing, and we offer training and support to empower our clients' teams.
In summary, AI agents in data analysis are transforming multi-dimensional data analysis by providing powerful tools for insight generation. While the benefits are significant, organizations must also address the challenges to fully leverage the potential of AI in their data analysis efforts. At Rapid Innovation, we are committed to guiding our clients through this transformative journey, ensuring they achieve their business goals efficiently and effectively. For more information on AI agents for marketing applications, you can read about their use cases, capabilities, best practices, and benefits.
1.1. Understanding Multi-dimensional Data
Multi-dimensional data refers to data that can be viewed and analyzed from multiple perspectives or dimensions. This type of data is crucial in various fields, including business intelligence, scientific research, and social sciences.
- Multi-dimensional data is often represented in the form of cubes, where each axis represents a different dimension.
- Common dimensions include time, geography, and product categories, allowing for complex analysis and insights.
- This data structure enables users to perform operations like slicing, dicing, and drilling down into the data for deeper insights.
- Multi-dimensional databases, such as OLAP (Online Analytical Processing), are specifically designed to handle this type of data efficiently.
- Understanding multi-dimensional data is essential for effective decision-making, as it provides a comprehensive view of the factors influencing outcomes.
At Rapid Innovation, we leverage multidimensional data analysis to help our clients gain deeper insights into their operations, enabling them to make informed decisions that drive greater ROI. We also utilize multidimensional data analysis techniques and provide examples of multidimensional data analysis in cube space to enhance our services.
1.2. Evolution of Data Analysis Techniques
The evolution of data analysis techniques has transformed how organizations interpret and utilize data.
- Early data analysis methods were primarily manual and involved basic statistical techniques.
- The introduction of computers in the 1960s and 1970s revolutionized data processing, allowing for more complex analyses.
- The rise of databases in the 1980s led to the development of SQL (Structured Query Language), enabling users to query large datasets efficiently.
- In the 1990s, data mining emerged, allowing analysts to discover patterns and relationships within large datasets. Techniques such as multidimensional analysis in data mining became prominent during this time.
- The 2000s saw the advent of big data technologies, such as Hadoop and Spark, which enabled the processing of vast amounts of unstructured data.
- Today, advanced analytics techniques, including predictive analytics and machine learning, are widely used to derive actionable insights from data.
At Rapid Innovation, we stay at the forefront of these evolving techniques, ensuring our clients can harness the latest advancements to optimize their data strategies and achieve significant returns on their investments. We also explore the role of Azure Analysis Services multidimensional in enhancing data analysis capabilities.
1.3. Role of AI Agents in Modern Analytics
AI agents play a pivotal role in modern analytics by automating processes and enhancing decision-making capabilities.
- AI agents can analyze large datasets quickly, identifying trends and anomalies that may not be apparent to human analysts.
- They utilize machine learning algorithms to improve their performance over time, adapting to new data and changing conditions.
- AI-driven analytics tools can provide real-time insights, enabling organizations to respond swiftly to market changes.
- Natural language processing (NLP) allows AI agents to interpret and analyze unstructured data, such as customer feedback and social media posts.
- By automating routine tasks, AI agents free up human analysts to focus on more strategic initiatives, enhancing overall productivity.
- The integration of AI in analytics is expected to continue growing, with predictions indicating that AI will significantly impact decision-making processes across industries.
Rapid Innovation specializes in deploying AI agents that empower organizations to unlock the full potential of their data. By integrating AI-driven analytics into their operations, our clients can achieve faster, more accurate insights, leading to improved decision-making and increased ROI. We also emphasize the importance of tabular and multidimensional models in our AI-driven analytics solutions.
1.4. Current State of the Industry
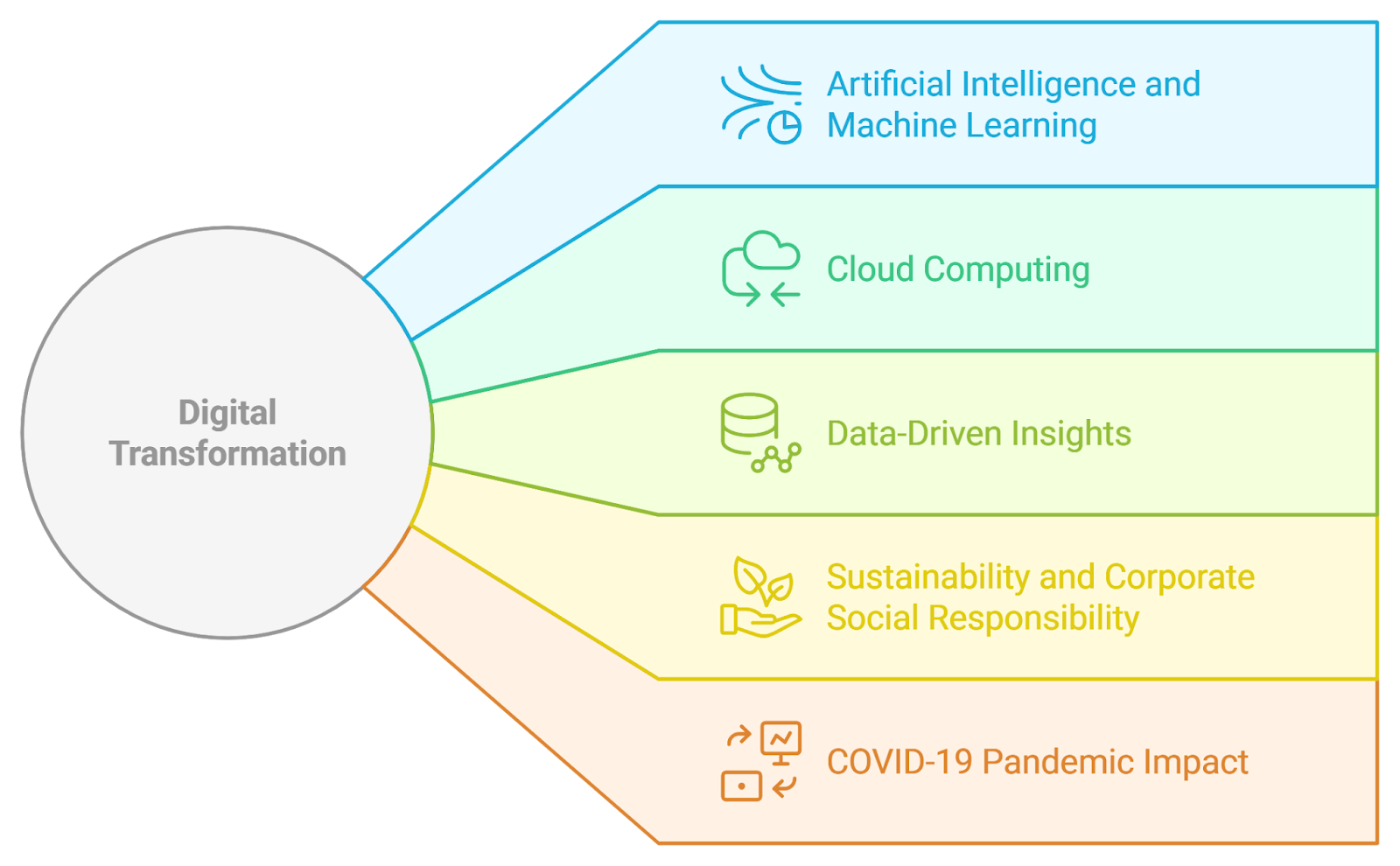
‍
The current state of the industry is characterized by rapid advancements in technology, shifting consumer preferences, and increasing competition. Various sectors are experiencing transformative changes due to digitalization and the integration of innovative solutions, leading to a growing demand for digital transformation consulting.
- The rise of artificial intelligence (AI) and machine learning (ML) is reshaping how businesses operate, enabling them to analyze vast amounts of data for better decision-making. At Rapid Innovation, we leverage AI to develop tailored solutions that enhance operational efficiency and drive significant ROI for our clients, which is a key focus of digital transformation management consulting.
- Companies are increasingly adopting cloud computing, which allows for greater flexibility, scalability, and cost-effectiveness in managing resources. Our expertise in cloud-based solutions ensures that clients can optimize their infrastructure while reducing overhead costs, a critical aspect of digital transformation consulting services.
- The demand for data-driven insights is growing, leading to a surge in analytics tools and platforms that help organizations harness their data effectively. Rapid Innovation specializes in creating advanced analytics solutions that empower businesses to make informed decisions, ultimately boosting their profitability, a service often provided by digital transformation consulting firms.
- Sustainability and corporate social responsibility are becoming essential components of business strategies, as consumers are more inclined to support environmentally friendly practices. We assist clients in integrating sustainable practices into their operations through innovative technology solutions, enhancing their brand reputation and customer loyalty, which is increasingly part of business transformation consulting services.
- The COVID-19 pandemic has accelerated digital transformation across industries, pushing businesses to adapt quickly to remote work and online services. Our consulting services guide organizations through this transition, ensuring they remain competitive in a rapidly evolving landscape, a necessity for digital transformation consulting companies.
According to a report by McKinsey, companies that have embraced digital transformation are 2.5 times more likely to experience revenue growth than their peers. This highlights the importance of staying ahead in a rapidly evolving landscape, underscoring the value of engaging with the best digital transformation consulting firms.
2. Fundamental Concepts
Understanding fundamental concepts is crucial for grasping the complexities of any industry. These concepts serve as the building blocks for more advanced theories and practices.
- Fundamental concepts often include key terminologies, principles, and frameworks that guide decision-making and strategy formulation.
- They provide a common language for professionals within the industry, facilitating communication and collaboration.
- A solid grasp of these concepts enables individuals and organizations to adapt to changes and challenges effectively.
2.1. Multi-dimensional Data Structures
Multi-dimensional data structures are essential for organizing and analyzing complex datasets. They allow for the representation of data in multiple dimensions, making it easier to extract meaningful insights.
- These structures can represent data in various forms, such as arrays, matrices, or cubes, enabling users to analyze relationships and patterns across different dimensions.
- Multi-dimensional data structures are particularly useful in fields like data warehousing, business intelligence, and analytics, where large volumes of data need to be processed efficiently.
- They support advanced analytical techniques, such as OLAP (Online Analytical Processing), which allows users to perform complex queries and generate reports quickly.
- By utilizing multi-dimensional data structures, organizations can enhance their decision-making processes, leading to improved operational efficiency and strategic planning.
In summary, the current state of the industry reflects a dynamic environment driven by technological advancements and changing consumer expectations. Understanding fundamental concepts, particularly multi-dimensional data structures, is vital for navigating this landscape effectively. Rapid Innovation is committed to helping clients harness these advancements to achieve their business goals efficiently and effectively through comprehensive digital transformation consulting.
2.1.1. Data Cubes
Data cubes are a multidimensional array of values, primarily used in data warehousing and online analytical processing (OLAP). They allow for the organization of data in a way that enables efficient querying and analysis.
- Data cubes can represent data across multiple dimensions, such as time, geography, and product categories.
- They facilitate complex calculations and aggregations, making it easier to derive insights from large datasets.
- Users can perform operations like slicing, dicing, and drilling down into the data to explore different perspectives.
- Data cubes are particularly useful in business intelligence applications, where decision-makers need to analyze trends and patterns quickly.
- They can be implemented using various database technologies, including SQL databases and specialized OLAP systems, such as SQL Server Analysis Services cube and SSAS cube.
At Rapid Innovation, we leverage data cubes, including bi cube and analysis service cube, to help clients optimize their data analysis processes, enabling them to make informed decisions that drive greater ROI. By implementing tailored data cube solutions, we empower organizations to uncover actionable insights from their data, enhancing their strategic initiatives. Our expertise in data cubes in data warehouse environments ensures that we provide the best solutions for our clients. For more information on key concepts and technologies in AI.
2.1.2. Tensors
Tensors are mathematical objects that generalize scalars, vectors, and matrices to higher dimensions. They are fundamental in various fields, including physics, engineering, and machine learning. A tensor can be thought of as a multi-dimensional array, where a scalar is a 0-dimensional tensor, a vector is a 1-dimensional tensor, and a matrix is a 2-dimensional tensor.
- Tensors are crucial in deep learning, where they are used to represent data inputs, weights, and outputs in neural networks.
- Operations on tensors, such as addition, multiplication, and contraction, are essential for training machine learning models.
- Libraries like TensorFlow and PyTorch provide extensive support for tensor operations, making it easier for developers to build and train complex models.
At Rapid Innovation, our expertise in tensor manipulation allows us to develop advanced machine learning models that can significantly enhance predictive analytics for our clients. By utilizing state-of-the-art tensor operations, we help businesses achieve higher accuracy in their forecasts, leading to improved operational efficiency and ROI.
2.1.3. High-dimensional Arrays
High-dimensional arrays are data structures that extend the concept of arrays to multiple dimensions. They are commonly used in scientific computing, data analysis, and machine learning.
- High-dimensional arrays can store data in three or more dimensions, allowing for the representation of complex datasets.
- They enable efficient storage and manipulation of large datasets, which is essential in fields like image processing and genomics.
- Operations on high-dimensional arrays include reshaping, slicing, and broadcasting, which facilitate data manipulation and analysis.
- Libraries such as NumPy and SciPy in Python provide robust support for high-dimensional arrays, making it easier for researchers and developers to work with complex data.
- High-dimensional arrays are particularly useful in applications that require the analysis of multi-faceted data, such as time series analysis and multi-channel image processing.
Rapid Innovation employs high-dimensional arrays to enhance data processing capabilities for our clients, particularly in sectors like healthcare and finance. By implementing solutions that utilize these advanced data structures, we enable organizations to analyze complex datasets more effectively, ultimately driving better business outcomes and maximizing ROI.
2.2. AI Agent Architecture
AI agent architecture refers to the structured framework that defines how an AI agent operates, interacts with its environment, and processes information. This architecture is crucial for developing intelligent systems capable of performing tasks autonomously. The design of an AI agent typically includes several layers that facilitate perception, decision-making, and action. The architecture can be categorized into various types, such as reactive agents, deliberative agents, and hybrid agents. Each type has its own strengths and weaknesses, depending on the complexity of the tasks and the environment in which the agent operates. A well-defined architecture allows for scalability and adaptability, enabling agents to learn from experiences and improve their performance over time. This includes various models such as the bdi architecture in artificial intelligence and logic based agent architecture in ai.
2.2.1. Core Components
The core components of an AI agent architecture are essential for its functionality. These components work together to enable the agent to perceive its environment, make decisions, and take actions.
- Sensors: These are the input devices that allow the agent to gather information from its surroundings. Sensors can include cameras, microphones, and other data-gathering tools.
- Actuators: Actuators are the output devices that enable the agent to perform actions in the environment. This can include motors, speakers, or any mechanism that allows the agent to interact with the world.
- Knowledge Base: This component stores information and rules that the agent uses to make decisions. It can be static or dynamic, depending on whether the agent learns from new experiences.
- Reasoning Engine: The reasoning engine processes the information from the sensors and the knowledge base to make decisions. It can employ various algorithms, such as rule-based systems or machine learning models.
- Communication Interface: This allows the agent to interact with other agents or systems. Effective communication is vital for collaboration and information sharing.
2.2.2. Learning Mechanisms
Learning mechanisms are critical for AI agents to adapt and improve their performance over time. These mechanisms enable agents to learn from their experiences and modify their behavior based on new information.
- Supervised Learning: In this approach, the agent learns from labeled data. It uses input-output pairs to understand the relationship between them, allowing it to make predictions on new, unseen data.
- Unsupervised Learning: Here, the agent analyzes data without labeled outputs. It identifies patterns and structures within the data, which can be useful for clustering and anomaly detection.
- Reinforcement Learning: This mechanism involves agents learning through trial and error. They receive feedback in the form of rewards or penalties based on their actions, allowing them to optimize their behavior over time.
- Transfer Learning: This technique allows an agent to apply knowledge gained from one task to a different but related task. It enhances learning efficiency and reduces the amount of data needed for training.
- Online Learning: In this method, the agent continuously learns from new data as it becomes available. This is particularly useful in dynamic environments where conditions change frequently.
By integrating these learning mechanisms into the AI agent architecture, developers can create systems that are not only intelligent but also capable of evolving and improving in response to their environments. At Rapid Innovation, we leverage this architecture to build tailored AI solutions that help our clients achieve greater ROI by automating processes, enhancing decision-making, and providing actionable insights that drive business growth. This includes exploring various types of agent architecture in ai and the architecture of intelligent agent in ai to ensure optimal performance. For more details, check out the key components of modern AI agent architecture.
2.2.3. Decision-making Systems
Decision-making systems are integral components of artificial intelligence (AI) that facilitate the process of making informed choices based on data analysis. These systems utilize algorithms and models to evaluate various options and predict outcomes, ultimately guiding users or organizations in their decision-making processes, including mis and decision making.
- Types of Decision-making Systems:
- Rule-based systems: These systems apply predefined rules to make decisions. They are straightforward and effective for well-defined problems.
- Expert systems: These mimic human expertise in specific domains, using knowledge bases and inference engines to provide recommendations.
- Machine learning systems: These systems learn from data patterns and improve their decision-making capabilities over time, adapting to new information.
- Key Features:
- Data-driven: Decision-making systems rely heavily on data inputs to generate insights and recommendations.
- Predictive analytics: Many systems incorporate predictive models to forecast future trends and behaviors, enhancing decision quality.
- Real-time processing: Advanced systems can analyze data in real-time, allowing for immediate decision-making in dynamic environments.
- Applications:
- Business intelligence: Organizations use decision-making systems to analyze market trends, customer behavior, and operational efficiency. Rapid Innovation leverages these systems to help clients optimize their strategies and improve ROI through data-driven insights, including mis for decision making.
- Healthcare: AI systems assist in diagnosing diseases and recommending treatment plans based on patient data, enabling healthcare providers to make timely and effective decisions.
- Finance: These systems evaluate investment opportunities and risk management strategies, optimizing financial decisions. Rapid Innovation supports financial institutions in implementing robust decision-making systems that enhance profitability and reduce risks, including mis decision making.
2.3. Data Processing Paradigms
Data processing paradigms refer to the methodologies and frameworks used to handle, analyze, and interpret data. With the exponential growth of data, various paradigms have emerged to address the challenges of data processing effectively.
- Traditional Paradigms:
- Batch processing: This method involves collecting data over a period and processing it in bulk. It is efficient for large datasets but may not provide real-time insights.
- Stream processing: This paradigm processes data in real-time as it is generated. It is essential for applications requiring immediate analysis, such as fraud detection.
- Modern Paradigms:
- Distributed processing: This approach utilizes multiple machines to process data concurrently, enhancing speed and efficiency. Technologies like Apache Hadoop and Apache Spark exemplify this paradigm.
- Cloud computing: Leveraging cloud infrastructure allows for scalable data processing, enabling organizations to handle vast amounts of data without significant upfront investment.
- Key Considerations:
- Scalability: The chosen paradigm should accommodate growing data volumes without compromising performance.
- Flexibility: A good data processing paradigm should adapt to various data types and sources, including structured and unstructured data.
- Cost-effectiveness: Organizations must evaluate the cost implications of different paradigms, balancing performance with budget constraints.
3. Benefits of AI Agents in Data Analysis
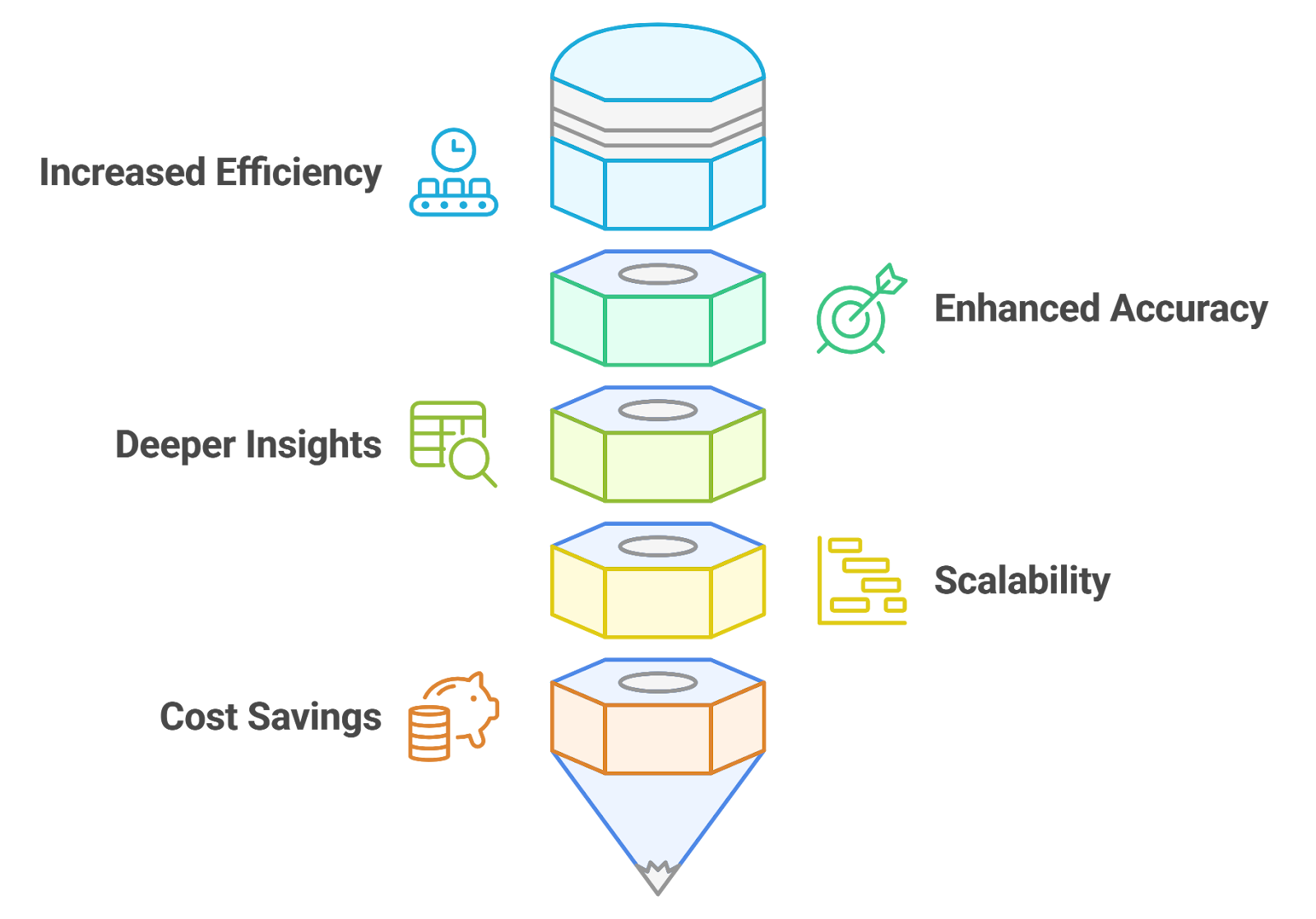
‍
AI agents play a crucial role in enhancing data analysis by automating processes, providing insights, and improving decision-making. Their integration into data analysis workflows offers numerous advantages.
- Increased Efficiency:
- Automation of repetitive tasks: AI agents can handle data cleaning, preprocessing, and analysis, freeing up human analysts for more strategic tasks.
- Faster data processing: AI algorithms can analyze large datasets in a fraction of the time it would take traditional methods.
- Enhanced Accuracy:
- Reduced human error: AI agents minimize the risk of mistakes associated with manual data handling and analysis.
- Improved predictive capabilities: Machine learning models can identify patterns and trends that may be overlooked by human analysts.
- Deeper Insights:
- Advanced analytics: AI agents can perform complex analyses, such as sentiment analysis and anomaly detection, providing richer insights.
- Real-time monitoring: AI systems can continuously analyze data streams, offering immediate feedback and insights for timely decision-making.
- Scalability:
- Handling large datasets: AI agents can efficiently process and analyze vast amounts of data, making them suitable for big data applications.
- Adaptability: These agents can be trained on new data, allowing them to evolve and improve their analytical capabilities over time.
- Cost Savings:
- Reduced labor costs: Automating data analysis tasks can lead to significant savings in labor expenses.
- Optimized resource allocation: AI agents help organizations allocate resources more effectively by providing data-driven insights.
Incorporating AI agents into data analysis not only streamlines processes but also enhances the overall quality of insights derived from data, making them invaluable in today’s data-driven landscape. Rapid Innovation is committed to helping clients harness the power of AI and decision-making systems, including decisionmaking systems, to achieve their business goals efficiently and effectively, ultimately driving greater ROI.
3.1. Enhanced Pattern Recognition
Enhanced pattern recognition is a critical advancement in data analysis and machine learning. This capability allows systems to identify and interpret complex patterns within large datasets, leading to more accurate predictions and insights.
- Utilizes advanced algorithms, such as deep learning and neural networks, to detect intricate patterns that traditional methods may overlook.
- Improves decision-making processes across various industries, including finance, healthcare, and marketing.
- Enables businesses to identify trends and anomalies in customer behavior, leading to more targeted marketing strategies. For instance, python stock pattern recognition can be employed to analyze stock market trends effectively.
- Facilitates predictive maintenance in manufacturing by recognizing patterns that indicate equipment failure before it occurs.
- Supports fraud detection by analyzing transaction patterns to flag unusual activities.
At Rapid Innovation, we leverage enhanced pattern recognition to help our clients optimize their operations and drive greater ROI. For instance, in the finance sector, our solutions can identify fraudulent transactions in real-time, significantly reducing losses and enhancing security.
The ability to recognize patterns enhances the overall effectiveness of data-driven strategies, making it a vital component of modern analytics. Additionally, pattern recognition in data analysis plays a crucial role in extracting meaningful insights from complex datasets.
3.2. Automated Feature Engineering
Automated feature engineering is a transformative process in machine learning that streamlines the preparation of data for analysis. This technique automates the extraction and selection of relevant features from raw data, significantly reducing the time and effort required for data preprocessing.
- Increases efficiency by automating repetitive tasks, allowing data scientists to focus on model development and interpretation.
- Enhances model performance by identifying the most relevant features that contribute to predictive accuracy.
- Reduces the risk of human error in feature selection, leading to more reliable models.
- Supports a wide range of data types, including structured, unstructured, and time-series data.
- Facilitates the use of advanced techniques, such as ensemble learning, by providing a richer set of features for model training.
By automating feature engineering, organizations can accelerate their data analysis processes and improve the quality of their machine learning models. At Rapid Innovation, we implement automated feature engineering to help clients achieve faster insights and better decision-making, ultimately leading to increased ROI.
3.3. Real-time Analysis Capabilities
Real-time analysis capabilities are essential for organizations that require immediate insights from their data. This functionality allows businesses to process and analyze data as it is generated, enabling timely decision-making and responsiveness to changing conditions.
- Supports industries such as finance, where real-time data analysis is crucial for trading and risk management.
- Enhances customer experience by allowing businesses to respond to customer inquiries and behaviors instantly.
- Facilitates operational efficiency by monitoring systems and processes in real-time, identifying issues before they escalate.
- Enables predictive analytics by analyzing current data trends to forecast future outcomes.
- Integrates with IoT devices, allowing for continuous data collection and analysis from various sources.
At Rapid Innovation, our real-time analysis capabilities empower organizations to stay competitive in fast-paced environments. For example, in retail, we help businesses analyze customer behavior in real-time, allowing for immediate adjustments to marketing strategies and inventory management, thus maximizing ROI.
Real-time analysis capabilities are a key feature in modern data analytics solutions, ensuring that our clients can make informed decisions swiftly and effectively.
3.4. Scalability Advantages
Scalability is a crucial factor in the growth and efficiency of any system, particularly in technology and business. The scalability advantages of modern systems, especially those leveraging cloud computing and artificial intelligence, are significant. Scalable systems can allocate resources dynamically based on demand, meaning that during peak times, additional resources can be deployed without significant delays or costs. Businesses can save on costs by only paying for the resources they use, allowing for better financial planning and reducing waste through a pay-as-you-go model. Furthermore, scalability and flexibility in cloud computing enable systems to easily expand to serve a global audience, which is particularly important for businesses looking to enter new markets without the need for extensive infrastructure investments. Organizations can quickly adapt to changing market conditions or customer needs, providing essential flexibility in today’s fast-paced business environment. As user demand increases, scalable systems maintain performance levels, ensuring that users have a consistent experience without degradation in service.
- Resource Allocation: Scalable systems can allocate resources dynamically based on demand. This means that during peak times, additional resources can be deployed without significant delays or costs.
- Cost Efficiency: Businesses can save on costs by only paying for the resources they use. This pay-as-you-go model allows for better financial planning and reduces waste.
- Global Reach: Scalable systems can easily expand to serve a global audience. This is particularly important for businesses looking to enter new markets without the need for extensive infrastructure investments.
- Flexibility: Organizations can quickly adapt to changing market conditions or customer needs. This flexibility is essential in today’s fast-paced business environment, highlighting the flexibility and scalability of cloud computing.
- Performance Maintenance: As user demand increases, scalable systems maintain performance levels, ensuring that users have a consistent experience without degradation in service.
3.5. Reduced Human Bias
Human bias can significantly impact decision-making processes, often leading to unfair or inaccurate outcomes. The integration of technology, particularly artificial intelligence and machine learning, helps mitigate these biases. AI systems analyze vast amounts of data objectively, reducing the influence of personal biases that can affect human judgment. Automated systems follow predefined algorithms and rules, ensuring that decisions are made consistently and fairly across all cases. By utilizing diverse datasets, AI can provide a more balanced perspective, minimizing the risk of bias that may arise from limited or homogeneous data. Additionally, machine learning algorithms can be trained to recognize and adjust for biases in data, leading to more equitable outcomes over time. Many AI systems offer insights into their decision-making processes, allowing for greater scrutiny and accountability, which can help identify and correct biases.
- Data-Driven Decisions: AI systems analyze vast amounts of data objectively, reducing the influence of personal biases that can affect human judgment.
- Standardized Processes: Automated systems follow predefined algorithms and rules, ensuring that decisions are made consistently and fairly across all cases.
- Diverse Data Sources: By utilizing diverse datasets, AI can provide a more balanced perspective, minimizing the risk of bias that may arise from limited or homogeneous data.
- Continuous Learning: Machine learning algorithms can be trained to recognize and adjust for biases in data, leading to more equitable outcomes over time.
- Transparency: Many AI systems offer insights into their decision-making processes, allowing for greater scrutiny and accountability, which can help identify and correct biases.
3.6. Improved Accuracy and Precision
Accuracy and precision are vital in various fields, from healthcare to finance. The advancements in technology have significantly enhanced these aspects, leading to better outcomes. Advanced algorithms can process and analyze data with a level of accuracy that surpasses human capabilities, resulting in more reliable insights and predictions. Automated systems reduce the likelihood of human error, which is often a significant factor in inaccuracies, particularly in critical areas such as medical diagnostics and financial transactions. Technologies can analyze data in real-time, allowing for immediate adjustments and corrections, which is crucial in environments where timely decisions are essential. Machine learning models can improve over time, leading to increasingly accurate predictions based on historical data and trends. In manufacturing and production, automated systems can monitor processes continuously, ensuring that products meet quality standards with high precision.
- Data Analysis: Advanced algorithms can process and analyze data with a level of accuracy that surpasses human capabilities. This leads to more reliable insights and predictions.
- Error Reduction: Automated systems reduce the likelihood of human error, which is often a significant factor in inaccuracies. This is particularly important in critical areas such as medical diagnostics and financial transactions.
- Real-Time Processing: Technologies can analyze data in real-time, allowing for immediate adjustments and corrections. This is crucial in environments where timely decisions are essential.
- Enhanced Predictive Models: Machine learning models can improve over time, leading to increasingly accurate predictions based on historical data and trends.
- Quality Control: In manufacturing and production, automated systems can monitor processes continuously, ensuring that products meet quality standards with high precision.
By leveraging these advantages, organizations can enhance their operational efficiency, make better decisions, and ultimately achieve greater success in their respective fields. At Rapid Innovation, we specialize in harnessing the power of AI and blockchain technologies to help our clients realize these benefits, driving greater ROI and ensuring sustainable growth. For more insights, you can read about learning from real-world AI implementations.
4. Technical Implementation
Technical implementation is a crucial phase in any project, particularly in software development and system design. It involves translating the conceptual framework into a functional system. This section will delve into agent design principles and architecture selection, which are fundamental to creating effective and efficient agents.
4.1 Agent Design Principles
Agent design principles are guidelines that help in creating intelligent agents capable of performing tasks autonomously. These principles ensure that agents are efficient, reliable, and adaptable to changing environments. Key design principles include:
- Autonomy: Agents should operate independently, making decisions without human intervention. This autonomy allows for real-time responses to dynamic conditions, which can significantly enhance operational efficiency and reduce response times for businesses.
- Reactivity: Agents must be able to perceive their environment and respond to changes promptly. This involves continuous monitoring and quick adaptation to new information, ensuring that businesses can stay ahead of market trends and customer needs.
- Proactivity: Beyond mere reaction, agents should anticipate future events and take initiative. This proactive behavior enhances their effectiveness in achieving goals, leading to improved customer satisfaction and retention.
- Social Ability: Agents should be capable of interacting with other agents and humans. This includes communication protocols and collaboration strategies to work towards common objectives, fostering a more integrated and efficient workflow.
- Learning: Incorporating machine learning techniques allows agents to improve their performance over time. Learning from past experiences enables agents to adapt to new situations and optimize their actions, ultimately driving greater ROI for clients.
- Scalability: The design should accommodate growth, allowing the system to handle an increasing number of agents or tasks without significant performance degradation. This scalability is essential for businesses looking to expand their operations without incurring excessive costs.
- Robustness: Agents must be resilient to failures and capable of recovering from errors. This involves implementing error-handling mechanisms and redundancy, ensuring that business operations remain uninterrupted.
These principles guide the development of agents that can function effectively in various applications, from customer service bots to autonomous vehicles, ultimately helping clients achieve their business goals efficiently.
4.1.1 Architecture Selection
Choosing the right architecture is vital for the successful implementation of agents. The architecture defines how agents are structured, how they interact with their environment, and how they process information. Key considerations in architecture selection include:
- Type of Agent: Different types of agents (reactive, deliberative, hybrid) require different architectural approaches. Reactive agents respond to stimuli, while deliberative agents plan their actions based on goals, allowing businesses to tailor solutions to their specific needs.
- Modularity: A modular architecture allows for easier updates and maintenance. Components can be developed, tested, and replaced independently, enhancing flexibility and reducing downtime for clients.
- Scalability: The architecture should support scalability, enabling the system to grow without significant redesign. This is particularly important for applications that may expand in user base or functionality, ensuring that clients can adapt to changing market demands.
- Performance: The architecture must ensure that agents can process information and respond quickly. Performance metrics should be established to evaluate the efficiency of the architecture, directly impacting the ROI for clients.
- Interoperability: The ability to integrate with other systems and technologies is crucial. The architecture should support standard protocols and interfaces for seamless communication, facilitating collaboration across different platforms.
- Resource Management: Efficient use of resources (CPU, memory, bandwidth) is essential for optimal performance. The architecture should include mechanisms for resource allocation and management, helping clients maximize their investments.
- Security: As agents often operate in sensitive environments, the architecture must incorporate security measures to protect against unauthorized access and data breaches, safeguarding client information and maintaining trust.
Selecting the appropriate architecture involves balancing these considerations to meet the specific needs of the application. Various architectural frameworks, such as layered architectures, agent-oriented architectures, and service-oriented architectures, can be employed based on the project requirements.
In conclusion, the technical implementation of agents hinges on sound design principles and careful architecture selection. By adhering to these guidelines, Rapid Innovation can help clients create robust, efficient, and adaptable agents capable of performing complex tasks in dynamic environments, ultimately driving greater ROI and achieving business goals effectively. For more information on how we can assist you, visit our AI agent development company.
4.1.2. Algorithm Choice
Choosing the right algorithm is crucial for the success of any data-driven project. The algorithm you select can significantly impact the performance, accuracy, and efficiency of your model. Here are some key considerations when making your algorithm choice:
- Nature of the Problem: Identify whether your problem is a classification, regression, clustering, or time-series forecasting task. Different algorithms are suited for different types of problems, and Rapid Innovation can assist in selecting the most appropriate algorithm tailored to your specific business needs. For example, if you are implementing a selection sort algorithm, understanding its suitability for your data structure is essential.
- Data Characteristics: Analyze the size, quality, and type of data you have. For instance, decision trees work well with categorical data, while linear regression is better suited for continuous data. Our team at Rapid Innovation can help you assess your data characteristics to ensure optimal algorithm selection. If you are working with sorting algorithms like selection sort in Java or Python selection sort, the data characteristics will influence your choice.
- Performance Metrics: Define what success looks like for your project. Depending on whether you prioritize accuracy, speed, or interpretability, your choice of algorithm may vary. We can guide you in establishing clear performance metrics that align with your business objectives. For instance, comparing the performance of selection sort versus quickselect can provide insights into efficiency.
- Scalability: Consider how well the algorithm can handle increasing amounts of data. Some algorithms, like k-nearest neighbors, may struggle with large datasets, while others, like gradient boosting machines, can scale more effectively. Rapid Innovation specializes in scalable solutions that grow with your business. When implementing algorithms like bubble sort and selection sort, scalability can be a significant factor.
- Complexity and Interpretability: Simpler models like linear regression are easier to interpret, while complex models like deep learning may offer better performance but at the cost of interpretability. Our experts can help you balance complexity and interpretability based on your project requirements. For example, understanding the differences between insertion sort and selection sort can aid in making informed decisions.
- Computational Resources: Assess the computational power available. Some algorithms require more resources and time to train, which can be a limiting factor. Rapid Innovation can optimize your resource allocation to ensure efficient algorithm training and deployment. When considering algorithms like C++ selection sort or Java selection sort program, resource allocation is crucial.
4.1.3. Integration Patterns
Integration patterns refer to the methods and strategies used to combine different systems, applications, or data sources. Effective integration is essential for ensuring that data flows seamlessly across platforms. Here are some common integration patterns:
- Point-to-Point Integration: This is the simplest form of integration where two systems are directly connected. While easy to implement, it can become complex as the number of systems increases. Rapid Innovation can streamline this process to ensure efficient connectivity.
- Hub-and-Spoke Integration: In this pattern, a central hub connects multiple systems. This approach simplifies management and reduces the number of direct connections, making it easier to maintain. Our team can design a hub-and-spoke model that enhances your operational efficiency.
- API-Based Integration: Using APIs (Application Programming Interfaces) allows different systems to communicate with each other. This pattern is flexible and scalable, enabling real-time data exchange. Rapid Innovation excels in developing robust APIs that facilitate seamless integration.
- Event-Driven Integration: This pattern relies on events to trigger data exchanges. It is useful for real-time applications where immediate responses are required. We can implement event-driven architectures that enhance responsiveness and agility in your operations.
- Batch Processing: In scenarios where real-time integration is not necessary, batch processing can be used to transfer data at scheduled intervals. This is often more efficient for large datasets. Rapid Innovation can optimize batch processing strategies to improve data handling efficiency.
- Microservices Architecture: This modern approach involves breaking down applications into smaller, independent services that can be developed, deployed, and scaled independently. It enhances flexibility and allows for easier integration. Our expertise in microservices can help you build a resilient and scalable architecture.
4.2. Data Preprocessing Strategies

‍
Data preprocessing is a critical step in the data analysis pipeline. It involves cleaning and transforming raw data into a format suitable for analysis. Here are some effective data preprocessing strategies:
- Data Cleaning: Remove or correct inaccuracies in the data. This includes handling missing values, correcting inconsistencies, and eliminating duplicates. Rapid Innovation can implement comprehensive data cleaning processes to ensure data integrity.
- Normalization and Standardization: Scale numerical data to ensure that it fits within a specific range or follows a standard distribution. Normalization rescales data to a range of [0, 1], while standardization transforms data to have a mean of 0 and a standard deviation of 1. Our team can apply these techniques to enhance model performance.
- Encoding Categorical Variables: Convert categorical data into numerical format using techniques like one-hot encoding or label encoding. This is essential for algorithms that require numerical input. We can assist in effectively encoding your data for optimal algorithm compatibility.
- Feature Selection: Identify and retain only the most relevant features for your model. This can improve model performance and reduce overfitting. Rapid Innovation employs advanced feature selection techniques to enhance your model's predictive power.
- Data Transformation: Apply transformations such as logarithmic or polynomial transformations to make the data more suitable for analysis. This can help in stabilizing variance and making relationships more linear. Our experts can determine the best transformations for your dataset.
- Outlier Detection: Identify and handle outliers that can skew your analysis. Techniques like Z-score or IQR (Interquartile Range) can be used to detect and manage outliers effectively. We can implement robust outlier detection methods to ensure accurate analysis.
- Data Splitting: Divide your dataset into training, validation, and test sets. This ensures that your model is evaluated on unseen data, providing a better estimate of its performance in real-world scenarios. Rapid Innovation can guide you in effectively splitting your data for optimal model evaluation.
4.3. Model Training Approaches
Model training is a critical phase in the machine learning lifecycle, where algorithms learn from data to make predictions or decisions. Various approaches can be employed to enhance the training process, each with its own advantages and challenges.
- Supervised Learning: This approach involves training a model on labeled data, where the input-output pairs are known. It is widely used for tasks like classification and regression. Rapid Innovation leverages supervised learning to help clients develop predictive models that drive informed decision-making, ultimately leading to increased ROI. Techniques such as training in machine learning and training machine learning models are commonly utilized in this context.
- Unsupervised Learning: In this method, the model is trained on data without labels, allowing it to identify patterns and relationships. Common applications include clustering and dimensionality reduction. By utilizing unsupervised learning, Rapid Innovation assists clients in uncovering hidden insights within their data, enabling them to optimize operations and enhance customer experiences.
- Semi-Supervised Learning: This hybrid approach combines labeled and unlabeled data, leveraging the strengths of both supervised and unsupervised learning. It is particularly useful when acquiring labeled data is expensive or time-consuming. Rapid Innovation employs semi-supervised learning to maximize the value of existing data, ensuring clients achieve their business objectives efficiently.
- Reinforcement Learning: This approach focuses on training models through trial and error, where an agent learns to make decisions by receiving rewards or penalties based on its actions. It is commonly used in robotics and game playing. Rapid Innovation's expertise in reinforcement learning allows clients to develop adaptive systems that improve over time, leading to enhanced operational efficiency and cost savings.
- Transfer Learning: This technique involves taking a pre-trained model and fine-tuning it on a new, but related task. It significantly reduces training time and resource requirements, making it ideal for scenarios with limited data. Rapid Innovation utilizes transfer learning to accelerate project timelines and reduce costs for clients, ensuring they achieve greater ROI. Approaches like transfer learning deep learning are particularly effective in this area.
- Online Learning: This method allows models to be trained incrementally as new data becomes available, making it suitable for dynamic environments. Rapid Innovation incorporates online machine learning techniques to ensure models remain relevant and accurate over time.
- Federated Learning: This approach enables training models across multiple decentralized devices while keeping data localized. It enhances privacy and security, making it an attractive option for sensitive applications. Rapid Innovation explores federated learning model strategies to address client needs in data-sensitive industries.
- XGBoost Training: This specific technique focuses on training models using the XGBoost algorithm, known for its efficiency and performance in structured data tasks. Rapid Innovation employs XGBoost training to deliver high-performing models for clients.
4.4. Deployment Frameworks
Once a model is trained, deploying it effectively is crucial for real-world applications. Deployment frameworks provide the necessary tools and infrastructure to integrate machine learning models into production environments.
- TensorFlow Serving: This is an open-source framework designed for serving machine learning models in production. It supports various model formats and provides features like versioning and monitoring.
- Flask and FastAPI: These lightweight web frameworks allow developers to create APIs for their models, making it easy to integrate machine learning capabilities into web applications.
- Kubernetes: This container orchestration platform is widely used for deploying machine learning models at scale. It automates the deployment, scaling, and management of containerized applications.
- MLflow: An open-source platform that manages the machine learning lifecycle, MLflow provides tools for tracking experiments, packaging code into reproducible runs, and sharing models.
- Amazon SageMaker: A fully managed service that provides tools for building, training, and deploying machine learning models quickly. It offers built-in algorithms and supports custom model deployment.
4.5. Performance Optimization
Optimizing the performance of machine learning models is essential to ensure they operate efficiently and effectively in production. Various strategies can be employed to enhance model performance.
- Hyperparameter Tuning: This involves adjusting the parameters that govern the training process to improve model accuracy. Techniques like grid search and random search are commonly used for this purpose.
- Feature Engineering: Selecting and transforming input features can significantly impact model performance. Techniques such as normalization, encoding categorical variables, and creating interaction terms can enhance model accuracy.
- Model Compression: Reducing the size of a model while maintaining its performance can lead to faster inference times and lower resource consumption. Techniques include pruning, quantization, and knowledge distillation.
- Ensemble Methods: Combining multiple models can lead to improved performance. Techniques like bagging, boosting, and stacking leverage the strengths of different models to achieve better results.
- Monitoring and Maintenance: Continuous monitoring of model performance in production is crucial. Implementing feedback loops and retraining models with new data can help maintain accuracy over time. Rapid Innovation emphasizes the importance of ongoing model performance monitoring to ensure that clients' systems remain effective and aligned with their evolving business goals.
5. Advanced Analysis Techniques
Advanced analysis techniques are essential for extracting meaningful insights from complex datasets. These methods help in simplifying data, enhancing interpretability, and improving the performance of machine learning models. Among these techniques, dimensionality reduction plays a crucial role in managing high-dimensional data, which is particularly relevant for organizations looking to leverage AI for data-driven decision-making.
5.1. Dimensionality Reduction
Dimensionality reduction refers to the process of reducing the number of random variables under consideration, obtaining a set of principal variables. This technique is particularly useful in scenarios where datasets have a large number of features, which can lead to overfitting and increased computational costs. At Rapid Innovation, we utilize dimensionality reduction techniques, including feature reduction techniques and dimensionality reduction with PCA, to help our clients streamline their data analysis processes, ultimately leading to greater ROI.
Benefits of dimensionality reduction include:
- Improved Visualization: Reducing dimensions allows for easier visualization of data, making it possible to plot high-dimensional data in two or three dimensions. This is particularly beneficial for stakeholders who need to understand complex data insights quickly.
- Reduced Overfitting: By eliminating irrelevant features, models can generalize better to unseen data. This is crucial for businesses aiming to deploy robust AI models that perform well in real-world scenarios.
- Enhanced Performance: Fewer dimensions can lead to faster training times and improved model performance. This efficiency translates into cost savings and quicker time-to-market for AI solutions.
- Noise Reduction: Dimensionality reduction can help in filtering out noise from the data, leading to more accurate predictions. This is vital for organizations that rely on precise data analytics for strategic decision-making.
5.1.1. Principal Component Analysis
Principal Component Analysis (PCA) is one of the most widely used techniques for dimensionality reduction. It transforms the original variables into a new set of variables, known as principal components, which are orthogonal and capture the maximum variance in the data. At Rapid Innovation, we implement PCA for dimensionality reduction to enhance our clients' data processing capabilities, ensuring they derive actionable insights from their datasets.
Key aspects of PCA include:
- Variance Maximization: PCA identifies the directions (principal components) in which the data varies the most. The first principal component captures the most variance, followed by the second, and so on. This allows businesses to focus on the most significant factors influencing their operations.
- Linear Transformation: PCA is a linear technique, meaning it assumes that the relationships between variables are linear. This can be a limitation in cases where the data has non-linear relationships, prompting us to explore alternative methods when necessary.
- Feature Reduction: By selecting a subset of principal components, PCA reduces the dimensionality of the dataset while retaining most of the information. This is particularly useful for clients with large datasets, as it simplifies analysis without sacrificing quality.
- Data Preprocessing: PCA often requires data to be standardized or normalized, especially when the features have different units or scales. Our team at Rapid Innovation ensures that data preprocessing is handled meticulously to maximize the effectiveness of PCA.
Applications of PCA include:
- Image Compression: PCA can reduce the size of image files while preserving essential features, making it a valuable tool for companies in the media and entertainment sectors.
- Genomics: In bioinformatics, PCA is used to analyze gene expression data and identify patterns, aiding research institutions in their scientific endeavors.
- Finance: PCA helps in risk management by identifying key factors that influence asset prices, providing financial institutions with critical insights for investment strategies.
PCA is a powerful tool, but it is essential to understand its limitations. For instance, it may not perform well with non-linear data distributions. In such cases, other techniques like t-Distributed Stochastic Neighbor Embedding (t-SNE) or Uniform Manifold Approximation and Projection (UMAP) for dimension reduction may be more appropriate, and our experts are well-versed in selecting the right approach for each unique dataset.
In conclusion, advanced analysis techniques like dimensionality reduction and PCA are vital for effective data analysis. They enable organizations to manage high-dimensional data efficiently, leading to better insights and decision-making. At Rapid Innovation, we are committed to helping our clients harness these techniques, including dimensionality reduction in machine learning and feature reduction in machine learning, to achieve their business goals efficiently and effectively, ultimately driving greater ROI.
5.1.2. t-SNE
t-Distributed Stochastic Neighbor Embedding (t-SNE) is a powerful technique for dimensionality reduction, particularly useful for visualizing high-dimensional data. It is widely used in machine learning and data science to help understand complex datasets, including applications of dimensionality reduction in machine learning.
- t-SNE works by converting similarities between data points into joint probabilities. It aims to minimize the divergence between these probabilities in high-dimensional space and their corresponding probabilities in a lower-dimensional space.
- The algorithm is particularly effective for visualizing clusters in data, making it a popular choice for exploratory data analysis, often used alongside dimensionality reduction techniques like PCA for better visualization.
- t-SNE is sensitive to the choice of parameters, particularly the perplexity, which can affect the resulting visualization. A typical range for perplexity is between 5 and 50.
- It is computationally intensive, especially for large datasets, which can lead to longer processing times.
- t-SNE is often used in conjunction with other techniques, such as PCA, to first reduce dimensionality before applying t-SNE for better visualization, highlighting the importance of feature reduction techniques.
5.1.3. UMAP
Uniform Manifold Approximation and Projection (UMAP) is another dimensionality reduction technique that has gained popularity for its speed and effectiveness in preserving the global structure of data.
- UMAP is based on manifold learning and topological data analysis, making it suitable for a wide range of applications, including clustering and visualization.
- It is generally faster than t-SNE, allowing it to handle larger datasets more efficiently, which is crucial for dimensionality reduction with PCA and other algorithms.
- UMAP preserves both local and global structures, which can lead to more meaningful visualizations compared to t-SNE.
- The algorithm requires tuning of parameters such as the number of neighbors and minimum distance, which can significantly influence the output.
- UMAP is increasingly being used in various fields, including genomics, image processing, and natural language processing, due to its versatility and effectiveness in dimensionality reduction algorithms.
5.2. Cluster Analysis

‍
Cluster analysis is a statistical technique used to group similar data points into clusters, allowing for better understanding and interpretation of data.
- The primary goal of cluster analysis is to identify natural groupings within a dataset, which can reveal patterns and relationships that may not be immediately apparent.
- There are several clustering algorithms available, including K-means, hierarchical clustering, and DBSCAN, each with its strengths and weaknesses.
- K-means is one of the most popular clustering methods, known for its simplicity and efficiency. It partitions data into K clusters by minimizing the variance within each cluster.
- Hierarchical clustering builds a tree-like structure of clusters, allowing for a more detailed view of data relationships. It can be agglomerative (bottom-up) or divisive (top-down).
- DBSCAN (Density-Based Spatial Clustering of Applications with Noise) is effective for identifying clusters of varying shapes and sizes, making it suitable for datasets with noise and outliers.
- Cluster analysis is widely used in various fields, including marketing, biology, and social sciences, to segment populations, identify trends, and make data-driven decisions.
At Rapid Innovation, we leverage advanced techniques like t-SNE and UMAP in our AI solutions to help clients visualize and interpret their data more effectively. By employing cluster analysis and dimensionality reduction in machine learning, we assist businesses in uncovering valuable insights from their datasets, ultimately driving better decision-making and enhancing ROI. Our expertise in these areas ensures that clients can harness the full potential of their data, leading to more efficient and effective business strategies.
5.3. Anomaly Detection
Anomaly detection is a critical process in data analysis that focuses on identifying unusual patterns or outliers in datasets. These anomalies can indicate significant events, errors, or fraud, making their detection essential in various fields.
- Definition: Anomaly detection refers to the identification of data points that deviate significantly from the majority of the data. These deviations can be due to noise, errors, or genuine anomalies.
- Applications:
- Fraud detection in banking and finance, where Rapid Innovation employs advanced machine learning algorithms to identify suspicious transactions in real-time, significantly reducing financial losses.
- Network security to identify potential breaches, utilizing AI-driven solutions that monitor network traffic and detect anomalies indicative of cyber threats, including network traffic anomaly detection.
- Quality control in manufacturing processes, where our AI models analyze production data to flag defects early, ensuring product quality and reducing waste.
- Techniques:
- Statistical methods, such as Z-scores and Grubbs' test, which Rapid Innovation integrates into its analytics platforms for baseline anomaly detection, including statistical anomaly detection.
- Machine learning approaches, including supervised and unsupervised learning, allowing for adaptive learning from historical data to improve detection accuracy, as seen in anomaly detection using machine learning.
- Clustering methods, like k-means and DBSCAN, to group data and identify outliers, enhancing the robustness of our anomaly detection systems, which can also be applied in outlier detection algorithms.
- Challenges:
- High dimensionality can complicate the detection process, but our expertise in dimensionality reduction techniques helps streamline analysis.
- The need for labeled data in supervised learning can limit effectiveness; however, Rapid Innovation employs semi-supervised learning techniques to mitigate this issue.
- Balancing false positives and false negatives is crucial for practical applications, and our solutions are designed to optimize this balance, ensuring reliable outcomes.
5.4. Time Series Analysis
Time series analysis involves examining data points collected or recorded at specific time intervals. This technique is vital for understanding trends, seasonal patterns, and cyclical behaviors in data over time.
- Definition: Time series analysis is the statistical technique used to analyze time-ordered data to extract meaningful insights and forecast future values.
- Applications:
- Economic forecasting, such as predicting GDP growth, where Rapid Innovation utilizes sophisticated models to provide accurate economic insights.
- Stock market analysis to identify trends and make investment decisions, leveraging AI algorithms that analyze historical stock data for predictive analytics.
- Weather forecasting to predict climate patterns, employing machine learning models that analyze historical weather data for improved accuracy.
- Components:
- Trend: The long-term movement in the data.
- Seasonality: Regular patterns that repeat over specific intervals.
- Noise: Random variations that do not follow a pattern.
- Techniques:
- Autoregressive Integrated Moving Average (ARIMA) models for forecasting, which Rapid Innovation customizes for client-specific datasets, including time series outlier detection python.
- Seasonal decomposition of time series (STL) to separate components, enhancing the interpretability of time series data.
- Exponential smoothing methods for trend analysis, providing clients with actionable insights for strategic planning.
- Challenges:
- Handling missing data points can skew results; our solutions include robust imputation techniques to address this issue.
- Non-stationarity in time series data may require transformation, and our expertise ensures that data is appropriately pre-processed for analysis.
- Overfitting models can lead to poor predictive performance, which we mitigate through rigorous model validation processes.
5.5. Pattern Mining
Pattern mining is the process of discovering interesting and useful patterns from large datasets. This technique is widely used in data mining to extract valuable insights that can inform decision-making.
- Definition: Pattern mining involves identifying regularities, correlations, or trends within data, often using algorithms to automate the process.
- Applications:
- Market basket analysis to understand consumer purchasing behavior, where Rapid Innovation helps retailers optimize inventory and marketing strategies based on consumer patterns.
- Web usage mining to analyze user interactions on websites, providing insights that enhance user experience and engagement.
- Bioinformatics for discovering patterns in genetic data, where our advanced analytics support research and development in healthcare.
- Techniques:
- Association rule mining, such as the Apriori algorithm, to find relationships between variables, which we implement in various client projects to uncover hidden insights.
- Sequential pattern mining to identify patterns over time, enabling businesses to anticipate customer needs and behaviors.
- Clustering techniques to group similar data points, facilitating targeted marketing and personalized customer experiences.
- Challenges:
- The sheer volume of data can make pattern discovery computationally intensive; our scalable solutions ensure efficient processing.
- Identifying meaningful patterns without overwhelming noise is crucial, and our expertise in data cleaning and preprocessing enhances the quality of insights.
- Ensuring the patterns discovered are actionable and relevant to business objectives is a core focus of Rapid Innovation, driving tangible results for our clients, including anomaly detection techniques and outlier detection in data mining.
6. Challenges and Limitations
The implementation of advanced technologies and methodologies often comes with a set of challenges and limitations. Understanding these obstacles is crucial for effective planning and execution.
6.1 Technical Challenges
Technical challenges encompass a wide range of issues that can arise during the development and deployment of technology-driven solutions. These challenges can hinder progress and affect the overall effectiveness of a project. Some of the key technical challenges include:
- Integration with existing systems can be difficult, leading to compatibility issues that may disrupt business operations, particularly in the context of challenges implementing electronic health records.
- Data quality and availability can impact the accuracy of results, making it essential to establish robust data governance practices, especially when addressing challenges in implementing ehr.
- Security concerns may arise, especially when handling sensitive information, necessitating the implementation of stringent security protocols, which is crucial in overcoming challenges of implementing an ehr system.
- Rapid technological changes can make it hard to keep systems updated, requiring ongoing investment in training and resources, particularly in the face of emr implementation challenges.
- User adoption can be slow if the technology is not user-friendly, highlighting the importance of intuitive design and user experience, a common issue in challenges presented by implementing technology in a healthcare setting.
6.1.1 Computational Complexity
Computational complexity refers to the amount of computational resources required to solve a problem or execute an algorithm. This complexity can pose significant challenges in various fields, particularly in data science, machine learning, and artificial intelligence. The challenges associated with computational complexity include:
- High computational demands can lead to increased costs for hardware and software, impacting overall ROI, which is a concern in challenges of implementing health information systems.
- Algorithms with high complexity may take an impractical amount of time to execute, especially with large datasets, which can delay project timelines, similar to the top 10 ehr implementation challenges and how to overcome them.
- Optimization of algorithms is often necessary to reduce computational load, which can be a complex task in itself and may require specialized expertise.
- Scalability issues may arise when trying to apply solutions to larger datasets or more complex problems, necessitating a forward-thinking approach to architecture.
- The need for specialized knowledge in algorithm design and optimization can limit the pool of available talent, making it crucial to partner with experienced firms like Rapid Innovation.
Understanding these technical challenges and computational complexities is essential for organizations looking to leverage technology effectively. Addressing these issues proactively can lead to more successful outcomes and a smoother implementation process, ultimately helping clients achieve their business goals efficiently and effectively, especially when navigating challenges in implementing telemedicine and AI challenges and limitations.
6.1.2. Memory Management
Memory management is a critical aspect of computer systems and software development. It involves the efficient allocation, use, and release of memory resources to ensure optimal performance and stability. Effective memory management can significantly impact the speed and efficiency of applications, particularly when considering memory management techniques.
- Dynamic Memory Allocation: This allows programs to request memory at runtime, which is essential for applications that require varying amounts of memory. Techniques like
malloc
andfree
in C ornew
anddelete
in C++ are commonly used. At Rapid Innovation, we leverage dynamic memory allocation to build scalable AI solutions that adapt to varying workloads, ensuring efficient resource utilization. - Garbage Collection: Automatic memory management techniques, such as garbage collection, help reclaim memory that is no longer in use. Languages like Java and Python utilize garbage collectors to prevent memory leaks and optimize memory usage. Our blockchain applications also benefit from garbage collection, as it helps maintain system performance by managing memory effectively.
- Memory Leaks: A memory leak occurs when a program allocates memory but fails to release it after use. This can lead to increased memory consumption and eventual system crashes. Regular monitoring and profiling tools can help identify and fix memory leaks. Rapid Innovation employs advanced monitoring tools to ensure our applications remain robust and efficient, minimizing the risk of memory leaks.
- Fragmentation: Memory fragmentation can occur when free memory is split into small, non-contiguous blocks. This can hinder the allocation of larger memory requests. Techniques like compaction can help mitigate fragmentation issues. Our development practices include strategies to minimize fragmentation, ensuring that our applications run smoothly even under heavy loads.
- Performance Impact: Poor memory management can lead to slow application performance, increased latency, and higher resource consumption. Optimizing memory usage is crucial for applications that handle large datasets or require real-time processing. At Rapid Innovation, we focus on optimizing memory management techniques in OS to enhance the performance of our AI solutions and blockchain solutions, ultimately leading to greater ROI for our clients. Additionally, understanding Rust's memory management and ownership model can provide valuable insights into efficient memory handling practices.
6.1.3. Algorithm Scalability
Algorithm scalability refers to the ability of an algorithm to maintain its performance as the size of the input data increases. A scalable algorithm can efficiently handle growing datasets without a significant increase in resource consumption or processing time.
- Time Complexity: The efficiency of an algorithm is often measured in terms of time complexity, which describes how the execution time increases with the size of the input. Common complexities include O(1), O(n), O(log n), and O(n²). Our team at Rapid Innovation designs algorithms with optimal time complexity to ensure that our solutions can handle large-scale data efficiently.
- Space Complexity: Similar to time complexity, space complexity measures the amount of memory an algorithm uses relative to the input size. An algorithm with low space complexity is preferable, especially in memory-constrained environments. We prioritize space-efficient algorithms in our AI models, ensuring that they perform well even in resource-limited scenarios.
- Big O Notation: Big O notation is a mathematical representation used to describe the upper limit of an algorithm's performance. It helps developers understand how an algorithm will scale with larger inputs. Our expertise in algorithm design allows us to communicate performance expectations clearly to our clients, ensuring transparency in our development process.
- Real-World Applications: Scalable algorithms are essential in various fields, including data processing, machine learning, and web services. For instance, sorting algorithms like QuickSort and MergeSort are known for their scalability and efficiency with large datasets. Rapid Innovation applies these principles to develop scalable AI solutions that can grow alongside our clients' businesses.
- Testing Scalability: To ensure an algorithm is scalable, developers often conduct stress tests and performance benchmarks. These tests help identify bottlenecks and areas for optimization. Our rigorous testing methodologies at Rapid Innovation ensure that our solutions are not only scalable but also resilient under varying loads.
6.2. Data Quality Issues
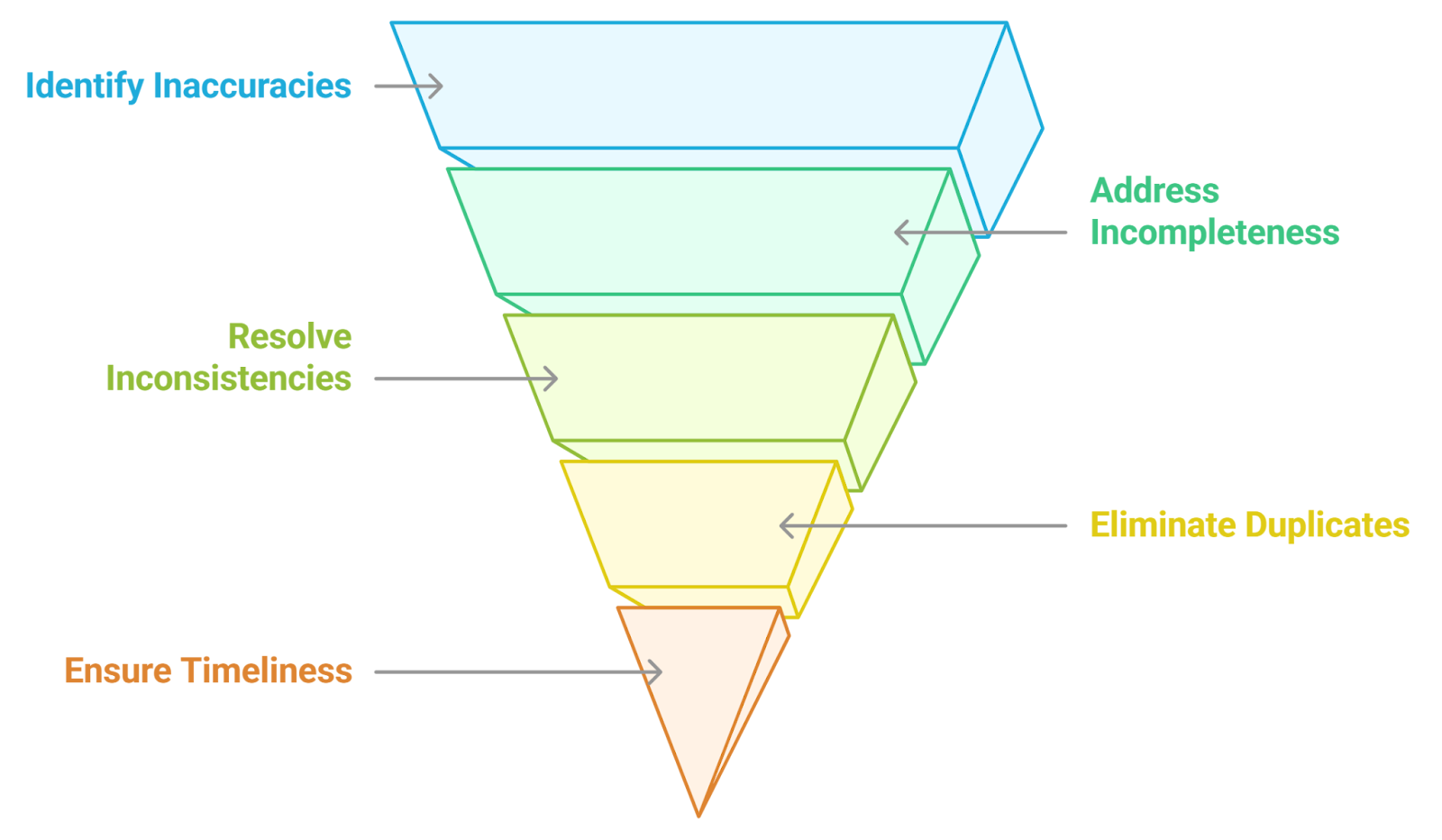
‍
Data quality issues can significantly impact the effectiveness of data-driven decision-making processes. High-quality data is essential for accurate analysis, reporting, and insights. Common data quality issues include:
- Inaccurate Data: Data inaccuracies can arise from human error, system glitches, or outdated information. Regular data validation and cleansing processes are necessary to maintain data accuracy. Rapid Innovation implements robust data validation frameworks to ensure that our AI models are trained on accurate datasets, leading to better outcomes.
- Incomplete Data: Missing values or incomplete records can lead to skewed analysis and unreliable results. Implementing data entry protocols and using imputation techniques can help address this issue. Our data engineering practices focus on completeness, ensuring that our clients have access to comprehensive datasets for analysis.
- Inconsistent Data: Data inconsistency occurs when the same data is represented differently across various sources. Standardizing data formats and implementing data governance policies can help ensure consistency. At Rapid Innovation, we establish data governance frameworks that promote consistency across all data sources, enhancing the reliability of insights derived from our solutions.
- Duplicate Data: Duplicate records can inflate datasets and lead to erroneous conclusions. Data deduplication techniques, such as fuzzy matching and record linkage, can help identify and remove duplicates. Our data management strategies include advanced deduplication techniques to maintain the integrity of our clients' datasets.
- Data Timeliness: Data must be up-to-date to be relevant. Stale data can mislead decision-makers. Establishing regular data update schedules and monitoring data freshness are crucial for maintaining timeliness. Rapid Innovation emphasizes the importance of data timeliness in our solutions, ensuring that our clients make decisions based on the most current information available.
- Impact on Decision-Making: Poor data quality can lead to misguided strategies, wasted resources, and lost opportunities. Organizations must prioritize data quality management to enhance their decision-making processes. By partnering with Rapid Innovation, clients can leverage our expertise in data quality management to drive informed decision-making and achieve their business goals effectively.
6.3. Interpretability Concerns
Interpretability in machine learning refers to the degree to which a human can understand the cause of a decision made by a model. This is a significant concern, especially in high-stakes fields like healthcare, finance, and criminal justice.
- Complex models, such as deep learning algorithms, often act as "black boxes," making it challenging to decipher how they arrive at specific conclusions. The interpretability of machine learning models is crucial, particularly when using techniques like deep learning interpretability.
- Lack of interpretability can lead to mistrust among users and stakeholders, as they may be hesitant to rely on decisions made by systems they do not understand. This is where model interpretability and AI model interpretability come into play.
- Regulatory requirements in certain industries demand transparency, making it essential for organizations to ensure that their models can be interpreted and explained. The interpretability of machine learning models is often a regulatory concern.
- Techniques like LIME (Local Interpretable Model-agnostic Explanations) and SHAP (SHapley Additive exPlanations) are being developed to enhance interpretability, but they may not always provide clear insights. LIME interpretability is one such approach that aims to make complex models more understandable.
- The trade-off between accuracy and interpretability is a critical consideration; simpler models may be more interpretable but less accurate, while complex models may excel in performance but lack clarity. This trade-off is often discussed in the context of machine learning interpretability and model explainability and interpretability.
At Rapid Innovation, we understand the importance of interpretability in machine learning. Our team employs advanced techniques to ensure that our models not only perform well but also provide clear insights that stakeholders can trust. By enhancing interpretability, we help our clients build confidence in their AI solutions, ultimately leading to better decision-making and greater ROI. We also focus on interpretable machine learning models, ensuring that our solutions are both effective and understandable.
6.4. Integration Difficulties
Integrating machine learning models into existing systems can pose significant challenges. Organizations often face hurdles that can impede the successful deployment of these technologies.
- Compatibility issues may arise when trying to merge new models with legacy systems, leading to increased costs and extended timelines.
- Data silos can hinder integration, as disparate data sources may not communicate effectively, resulting in incomplete or inaccurate datasets.
- The need for continuous updates and maintenance of machine learning models can complicate integration efforts, requiring ongoing collaboration between data scientists and IT teams.
- Organizations may also struggle with aligning machine learning initiatives with business objectives, leading to miscommunication and wasted resources.
- Training staff to work with new technologies is essential, as a lack of expertise can slow down the integration process and reduce the effectiveness of the models.
At Rapid Innovation, we specialize in seamless integration of machine learning models into existing infrastructures. Our approach ensures that compatibility issues are minimized, and we work closely with your teams to align AI initiatives with your business goals. This collaborative effort not only streamlines the integration process but also maximizes the return on your investment.
6.5. Resource Requirements
Implementing machine learning solutions demands significant resources, both in terms of financial investment and human capital. Organizations must be prepared to allocate adequate resources to ensure successful deployment and maintenance.
- Financial costs can include expenses related to software, hardware, and cloud services, which can quickly add up, especially for large-scale projects.
- Skilled personnel, such as data scientists, machine learning engineers, and domain experts, are crucial for developing and maintaining effective models. The demand for these professionals often exceeds supply, leading to increased hiring costs.
- Data preparation and cleaning require substantial time and effort, as high-quality data is essential for training accurate models. This process can be resource-intensive and may require specialized tools and technologies.
- Organizations must also consider the ongoing costs associated with model monitoring and retraining, as machine learning models can degrade over time if not properly maintained.
- Investing in training and development for existing staff can help bridge the skills gap, but this requires additional resources and commitment from leadership.
Rapid Innovation is committed to helping organizations navigate these resource requirements effectively. We provide tailored solutions that optimize resource allocation, ensuring that your investment in machine learning yields the highest possible returns. Our expertise in both AI and Blockchain technologies allows us to offer comprehensive support, enabling you to focus on your core business objectives while we handle the complexities of implementation and maintenance.
7. Applications and Use Cases
Data analytics has become an integral part of various industries, driving decision-making and innovation. Below are two significant applications of data analytics: Business Intelligence and Scientific Research.
7.1 Business Intelligence
Business Intelligence (BI) refers to the technologies and strategies used by organizations to analyze business data. The primary goal of BI is to support better business decision-making. BI is widely used across various sectors, including retail, finance, healthcare, and manufacturing. According to a report by Gartner, the global business intelligence market is expected to grow significantly, reflecting the increasing reliance on data-driven decision-making.
- Data Visualization: BI tools transform complex data sets into visual formats like charts and graphs, making it easier for stakeholders to understand trends and patterns. Rapid Innovation leverages advanced AI algorithms to enhance data visualization, ensuring that insights are not only accessible but also actionable.
- Performance Metrics: Organizations can track key performance indicators (KPIs) to measure success and identify areas for improvement. Our consulting services help clients define and implement effective KPIs tailored to their specific business objectives, driving greater ROI.
- Predictive Analytics: By analyzing historical data, businesses can forecast future trends, helping them to make proactive decisions. Rapid Innovation employs machine learning techniques to refine predictive models, enabling clients to anticipate market shifts and optimize their strategies accordingly.
- Customer Insights: BI tools analyze customer behavior and preferences, enabling businesses to tailor their products and services to meet customer needs. Our AI-driven analytics solutions provide deep insights into customer journeys, allowing organizations to enhance customer satisfaction and loyalty.
- Competitive Analysis: Companies can assess their market position relative to competitors, allowing them to strategize effectively. Rapid Innovation assists clients in conducting comprehensive competitive analyses, empowering them to make informed decisions that enhance their market positioning.
- Business Intelligence Software Examples: Various business intelligence software examples, such as embedded business intelligence software and applications for business intelligence, are utilized to streamline data analysis processes.
7.2 Scientific Research

‍
Data analytics plays a crucial role in scientific research, enabling researchers to derive meaningful insights from complex data sets. The impact of data analytics in scientific research is profound, as it enhances the accuracy and efficiency of studies, leading to groundbreaking discoveries. According to a study published in Nature, data-driven research methodologies are becoming increasingly prevalent, underscoring the importance of analytics in advancing scientific knowledge.
- Data Collection and Management: Researchers can gather vast amounts of data from experiments, surveys, and simulations, which can be managed and analyzed using advanced analytics tools. Rapid Innovation provides tailored data management solutions that streamline the research process, ensuring data integrity and accessibility.
- Statistical Analysis: Data analytics allows scientists to apply statistical methods to validate hypotheses and draw conclusions from their research. Our expertise in statistical modeling equips researchers with the tools necessary to derive robust conclusions from their data.
- Pattern Recognition: Advanced algorithms can identify patterns in large data sets, leading to new discoveries and insights in various fields, such as genomics and climate science. Rapid Innovation utilizes cutting-edge AI techniques to enhance pattern recognition capabilities, facilitating innovative research outcomes.
- Collaboration and Sharing: Data analytics platforms facilitate collaboration among researchers by allowing them to share data and findings easily, fostering innovation and accelerating research progress. We develop collaborative platforms that enhance communication and data sharing among research teams, driving collective advancements.
- Real-time Monitoring: In fields like environmental science, data analytics enables real-time monitoring of variables, helping researchers respond quickly to changes and trends. Our solutions incorporate real-time data analytics, empowering researchers to make timely decisions based on current data insights.
- Applications for Data Analytics: The use of data analytics applications, such as data analysis applications and data analytics apps, is crucial in scientific research to derive insights from complex datasets.
Through our expertise in AI and Blockchain, Rapid Innovation is committed to helping clients achieve their business goals efficiently and effectively, ultimately driving greater ROI across various applications and use cases.
7.3. Healthcare Analytics
Healthcare analytics involves the systematic use of data to improve patient outcomes, streamline operations, and reduce costs. This field leverages various data sources, including electronic health records (EHRs), clinical data, and patient feedback, to derive actionable insights. Predictive analytics can forecast patient admissions, helping hospitals manage resources effectively. Descriptive analytics provides insights into patient demographics, treatment outcomes, and operational efficiency. Prescriptive analytics recommends optimal treatment plans based on historical data and patient profiles.
The integration of healthcare analytics, including healthcare data analytics and medical analytics, can lead to enhanced patient care through personalized treatment plans, improved operational efficiency by identifying bottlenecks in care delivery, and cost reduction by minimizing unnecessary tests and procedures. Healthcare analytics is also pivotal in public health, enabling the tracking of disease outbreaks and the effectiveness of interventions. By utilizing advanced technologies like machine learning and artificial intelligence, healthcare providers can analyze vast amounts of data, including healthcare claims data analytics and big data in healthcare, to uncover trends and patterns that inform clinical decisions. At Rapid Innovation, we specialize in implementing AI-driven healthcare analytics solutions that empower healthcare organizations to achieve greater ROI through improved patient outcomes and operational efficiencies.
7.4. Financial Analysis
Financial analysis is crucial for businesses to assess their financial health and make informed decisions. It involves evaluating financial statements, cash flow, and market trends to understand a company's performance and potential risks. Ratio analysis helps in comparing financial metrics, such as profitability, liquidity, and solvency. Trend analysis identifies patterns over time, allowing businesses to forecast future performance. Variance analysis compares actual results to budgeted figures, highlighting areas needing attention.
Key benefits of financial analysis include improved budgeting and forecasting, leading to better resource allocation; enhanced investment decisions by evaluating potential returns and risks; and increased transparency for stakeholders, fostering trust and confidence. In today's digital age, financial analysis is increasingly supported by advanced analytics tools that automate data collection and reporting. This allows for real-time insights, enabling businesses to respond swiftly to market changes. Rapid Innovation offers tailored financial analysis solutions that leverage AI and blockchain technologies to enhance data integrity and provide actionable insights, ultimately driving better financial performance and ROI for our clients.
7.5. IoT Data Processing
IoT data processing refers to the collection, analysis, and management of data generated by Internet of Things (IoT) devices. With the proliferation of connected devices, the volume of data produced is immense, necessitating efficient processing methods. Data ingestion involves capturing data from various IoT sensors and devices in real-time. Data storage solutions, such as cloud computing, provide scalable options for managing large datasets. Data analytics tools help in extracting meaningful insights from raw data, enabling informed decision-making.
The significance of IoT data processing includes enhanced operational efficiency through real-time monitoring and predictive maintenance, improved customer experiences by personalizing services based on user behavior, and increased innovation by enabling new business models and services. As IoT technology continues to evolve, the need for robust data processing frameworks becomes critical. Organizations must invest in secure and scalable solutions to harness the full potential of IoT data, ensuring they can adapt to changing market demands and technological advancements. Rapid Innovation is at the forefront of IoT data processing, providing clients with innovative solutions that maximize the value of their IoT investments and drive significant ROI.
Social media analytics involves the collection, measurement, and analysis of data from social media platforms to understand user behavior, engagement, and overall performance. This process is crucial for businesses and marketers aiming to enhance their social media strategies.
- Key Metrics to Track:
- Engagement Rate: Measures interactions (likes, shares, comments) relative to total followers.
- Reach and Impressions: Indicates how many people see your content and how often.
- Follower Growth Rate: Tracks the increase in followers over time, reflecting brand popularity.
- Tools for Social Media Analytics:
- Google Analytics: Offers insights into traffic generated from social media.
- Hootsuite: Provides comprehensive analytics across multiple platforms, including social media reporting platforms.
- Sprout Social: Delivers detailed reports on engagement and audience demographics.
- Benefits of Social Media Analytics:
- Improved Decision-Making: Data-driven insights help refine marketing strategies.
- Enhanced Audience Understanding: Identifying audience preferences leads to better-targeted content.
- Performance Measurement: Regular analysis allows for tracking progress against goals, including social media metrics.
- Challenges in Social Media Analytics:
- Data Overload: The vast amount of data can be overwhelming and difficult to interpret.
- Platform Variability: Different social media platforms have unique metrics and reporting tools.
- Privacy Concerns: Navigating user privacy regulations can complicate data collection.
At Rapid Innovation, we leverage advanced AI algorithms to automate the analysis of social media data, enabling businesses to derive actionable insights efficiently. For instance, our AI-driven tools can identify trends in user engagement, allowing clients to adjust their strategies in real-time, ultimately leading to a greater return on investment (ROI).
8. Best Practices and Guidelines
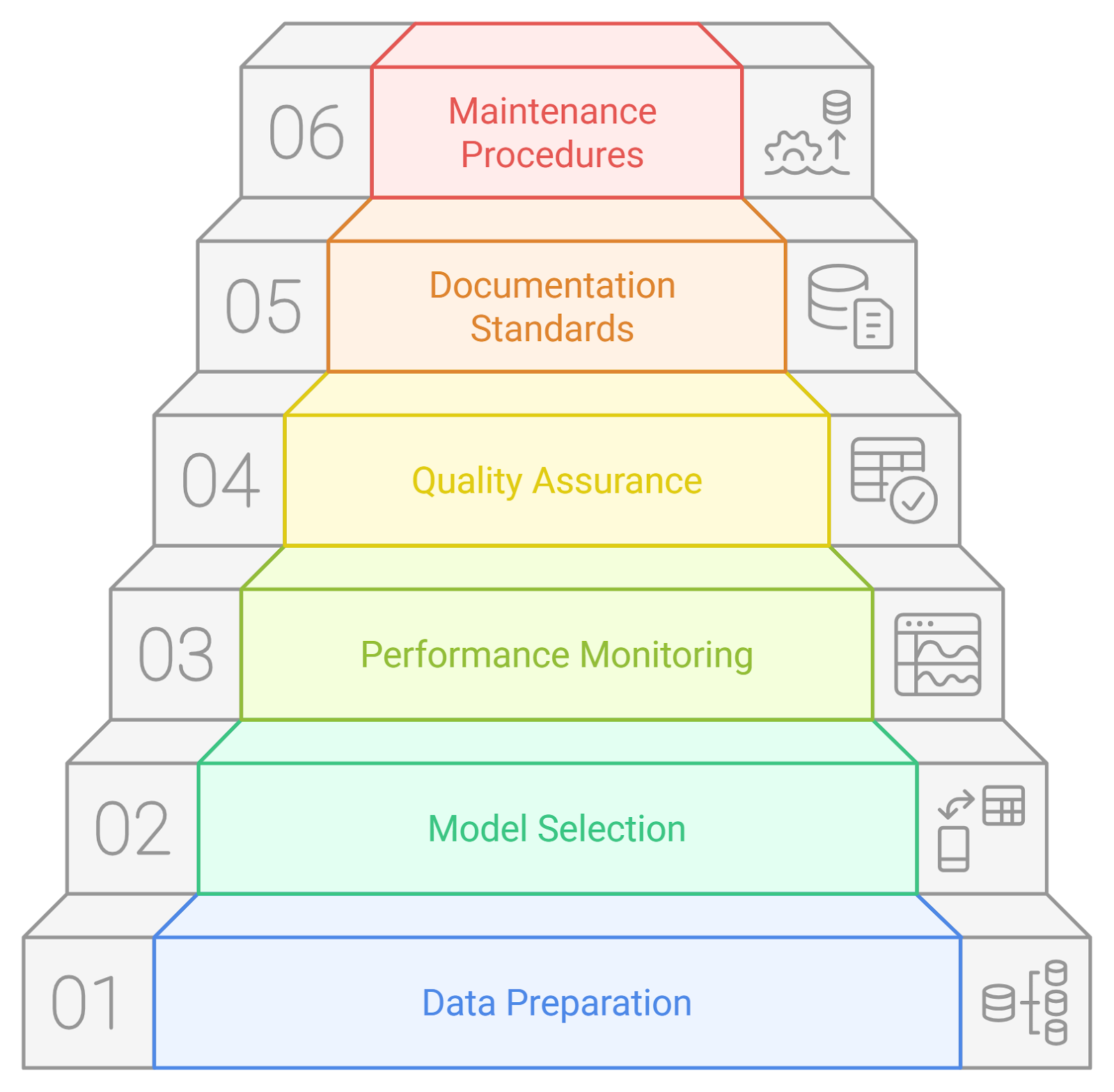
‍
Implementing best practices in social media analytics ensures that businesses can effectively leverage data to drive engagement and growth. Following established guidelines can enhance the accuracy and relevance of insights gained.
- Establish Clear Objectives:
- Define what you want to achieve with your social media efforts (e.g., brand awareness, lead generation).
- Align analytics goals with overall business objectives for a cohesive strategy.
- Regularly Monitor Performance:
- Set up a routine for checking analytics to stay updated on trends and changes.
- Use dashboards for real-time insights to make timely adjustments, including social media analytics dashboards.
- Focus on Quality Over Quantity:
- Prioritize meaningful engagement metrics rather than just follower counts.
- Analyze the quality of interactions to gauge true audience interest.
- A/B Testing:
- Experiment with different content types, posting times, and formats to see what resonates best.
- Use the results to refine future content strategies.
- Stay Updated on Trends:
- Keep an eye on emerging social media trends and platform updates.
- Adapt strategies to incorporate new features and audience behaviors, including social media marketing analytics.
8.1. Data Preparation
Data preparation is a critical step in the analytics process, ensuring that the data collected is clean, organized, and ready for analysis. Proper data preparation enhances the reliability of insights derived from social media analytics.
- Data Collection:
- Gather data from various social media platforms, ensuring a comprehensive view.
- Use APIs or analytics tools to automate data collection for efficiency, including social media data analytics.
- Data Cleaning:
- Remove duplicates and irrelevant data to maintain accuracy.
- Standardize formats (e.g., date formats, naming conventions) for consistency.
- Data Integration:
- Combine data from different sources (e.g., social media, website analytics) for a holistic view.
- Use data visualization tools to create integrated reports that highlight key insights.
- Data Transformation:
- Convert raw data into a usable format, such as aggregating metrics or creating calculated fields.
- Ensure that the data is structured in a way that facilitates analysis.
- Documentation:
- Maintain clear documentation of data sources, processes, and transformations.
- This helps in understanding the data lineage and ensures transparency in reporting.
- Data Security:
- Implement measures to protect sensitive data, especially when dealing with user information.
- Stay compliant with regulations like GDPR to avoid legal issues.
By following these practices in social media analytics and data preparation, businesses can effectively harness the power of data to drive their social media strategies and achieve their marketing goals. Rapid Innovation is committed to providing the tools and expertise necessary to navigate these complexities, ensuring our clients achieve optimal results and ROI, including social media insights and social media competitor analysis.
8.2. Model Selection
Model selection is a critical step in the machine learning process, as it determines which algorithm will be used to make predictions based on the data. The right model can significantly impact the performance and accuracy of the outcomes, ultimately leading to greater ROI for your business.
- Understand the problem type:
Identify whether the task is classification, regression, clustering, or time-series forecasting to choose the most suitable approach. - Consider the data characteristics:
- Size of the dataset.
- Number of features.
- Presence of missing values or outliers.
- Evaluate different algorithms:
- Linear models (e.g., Linear Regression, Logistic Regression) for straightforward relationships.
- Tree-based models (e.g., Decision Trees, Random Forests) for handling complex interactions.
- Neural networks for capturing intricate patterns in large datasets.
- Use cross-validation:
This technique helps in assessing how the results of a statistical analysis will generalize to an independent dataset, ensuring robustness. - Compare performance metrics:
- For classification tasks, consider accuracy, precision, recall, F1 score, and AUC-ROC.
- For regression tasks, evaluate Mean Absolute Error (MAE), Mean Squared Error (MSE), and R-squared.
- Consider interpretability:
Some models are easier to interpret than others, which can be crucial for decision-making and stakeholder buy-in. - Leverage automated tools:
Tools like AutoML can assist in selecting the best model based on the data provided, streamlining the process and enhancing efficiency. This includes techniques such as adaptive deep learning model selection on embedded systems and scikit learn model selection.
At Rapid Innovation, we guide our clients through this model selection process, ensuring that the chosen algorithms align with their specific business objectives, thereby maximizing their return on investment. We also emphasize the importance of model evaluation and selection in machine learning, as well as model selection and validation in machine learning to ensure optimal outcomes.
8.3. Performance Monitoring
Performance monitoring is essential to ensure that the machine learning model continues to perform well over time. It involves tracking the model's performance metrics and making adjustments as necessary to maintain effectiveness.
- Establish baseline performance:
Determine initial performance metrics to compare against future results. - Set up monitoring tools:
Use dashboards and visualization tools to track key performance indicators (KPIs) that matter to your business. - Regularly evaluate model performance:
Conduct periodic assessments using validation datasets to ensure ongoing accuracy. - Watch for data drift:
Monitor changes in the input data distribution that may affect model performance, allowing for timely adjustments. - Implement alert systems:
Set up alerts for significant drops in performance metrics to proactively address issues. - Conduct A/B testing:
Compare the performance of the current model against a new model or version to identify improvements. - Document performance trends:
Keep records of performance over time to identify patterns or issues, facilitating informed decision-making.
At Rapid Innovation, we emphasize the importance of performance monitoring to our clients, ensuring that their machine learning solutions remain effective and aligned with their evolving business needs.
8.4. Quality Assurance
Quality assurance (QA) in machine learning ensures that the models developed are reliable, accurate, and meet the required standards. It involves systematic processes to validate and verify the model's performance, ultimately contributing to better business outcomes.
- Define quality criteria:
Establish clear metrics and benchmarks for model performance that align with business goals. - Conduct thorough testing:
Use various testing methods, including unit tests, integration tests, and system tests, to ensure robustness. - Validate data quality:
Ensure that the data used for training and testing is clean, relevant, and representative, which is crucial for model accuracy. - Implement peer reviews:
Encourage team members to review each other's work to catch potential issues early, fostering a collaborative environment. - Use version control:
Maintain version control for datasets and models to track changes and facilitate rollback if necessary. - Perform regular audits:
Schedule audits to assess compliance with quality standards and identify areas for improvement. - Foster a culture of continuous improvement:
Encourage feedback and iterative improvements to enhance model quality over time.
At Rapid Innovation, we prioritize quality assurance in our machine learning projects, ensuring that our clients receive reliable solutions that drive efficiency and effectiveness in achieving their business goals. This includes a focus on choosing a machine learning model and model selection in deep learning to ensure the best outcomes.
8.5. Documentation Standards
Documentation standards are essential for ensuring consistency, clarity, and accessibility in all forms of documentation within an organization. These standards help maintain quality and facilitate effective communication among team members and stakeholders.
- Clear formatting guidelines should be established, including font type, size, and spacing.
- Use of standardized templates for reports, manuals, and other documents can streamline the documentation process, such as the standard invoice template.
- All documents should include version control to track changes and updates over time.
- Consistent terminology and language should be used to avoid confusion and misinterpretation.
- Documentation should be easily accessible, with a centralized repository for all files, including important resources like the iso 9001 audit checklist and auditing guidelines.
- Regular reviews and updates of documentation are necessary to keep information current and relevant, including the audit data standards order to cash and iso audit checklist.
- Training sessions on documentation standards can help ensure that all team members are aligned and understand the importance of these practices, particularly in relation to the iso 13485 audit checklist and gaap disclosure checklist. Additionally, leveraging AI agents for maintenance tracking can enhance the documentation process and improve overall efficiency.
8.6. Maintenance Procedures
Maintenance procedures are critical for ensuring the longevity and efficiency of systems, equipment, and processes within an organization. Proper maintenance can prevent costly downtime and extend the lifespan of assets.
Establishing a routine maintenance schedule is essential to regularly check and service equipment. All maintenance activities should be documented, including dates, tasks performed, and personnel involved. Implementing a system for tracking maintenance requests and issues ensures timely resolution. Additionally, using predictive maintenance techniques, which leverage data analytics, can help anticipate potential failures before they occur.
Training staff on proper maintenance procedures and the importance of adhering to them is crucial. Regular reviews and updates of maintenance procedures should be conducted to incorporate new technologies and best practices, including the use of the iso internal audit checklist and as9100 audit checklist. Finally, encouraging a culture of proactive maintenance empowers employees to report issues and suggest improvements.
9. Future Trends and Innovations
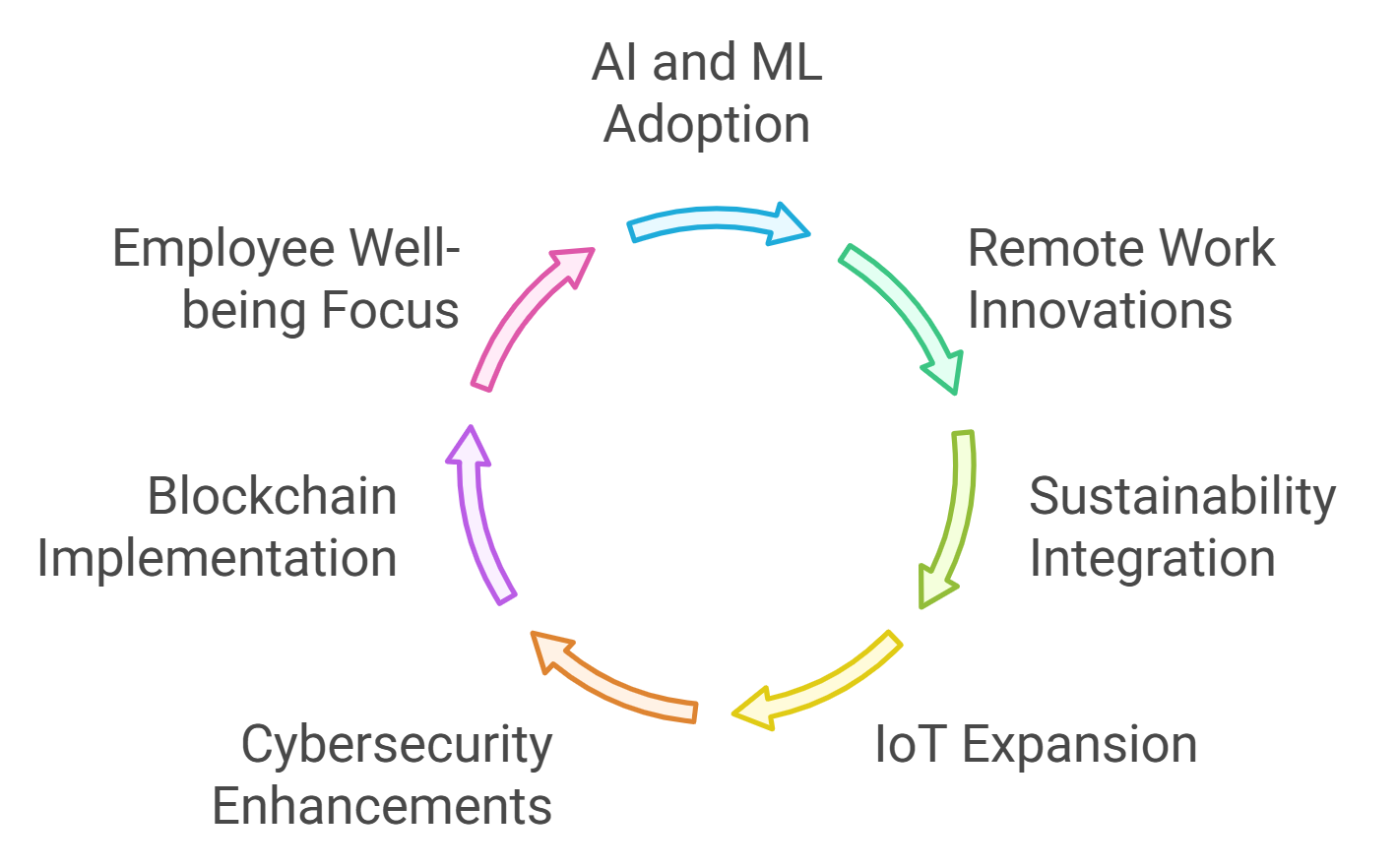
‍
The landscape of technology and business practices is constantly evolving, and staying ahead of future trends and innovations is crucial for organizations aiming for long-term success.
- Increased adoption of artificial intelligence (AI) and machine learning (ML) is transforming how businesses operate, enabling more efficient processes and data-driven decision-making. Rapid Innovation leverages AI to optimize workflows, enhance customer experiences, and drive significant ROI for clients.
- The rise of remote work has led to innovations in collaboration tools and virtual communication platforms, enhancing productivity and flexibility. Our solutions help organizations implement effective remote work strategies, ensuring seamless communication and collaboration.
- Sustainability and eco-friendly practices are becoming a priority, with organizations seeking innovative solutions to reduce their carbon footprint. Rapid Innovation assists clients in integrating sustainable technologies, enhancing their market reputation and operational efficiency.
- The Internet of Things (IoT) is expanding, connecting devices and systems to improve efficiency and data collection. We provide IoT solutions that enable real-time data analysis, leading to informed decision-making and increased operational efficiency.
- Cybersecurity innovations are critical as organizations face increasing threats; investing in advanced security measures is essential. Rapid Innovation offers robust cybersecurity solutions to protect sensitive data and maintain client trust.
- Blockchain technology is gaining traction for its potential to enhance transparency and security in transactions across various industries. Our blockchain solutions empower clients to streamline operations, reduce fraud, and improve trust in their business processes.
- Organizations are focusing on employee well-being and mental health, leading to innovations in workplace culture and benefits. We help clients develop programs that prioritize employee well-being, resulting in higher productivity and retention rates.
By keeping abreast of these trends and innovations, organizations can adapt and thrive in an ever-changing environment, and Rapid Innovation is here to guide you through this transformation.
9.1. Emerging Technologies
Emerging technologies are reshaping industries and driving innovation across various sectors. These technologies are characterized by their potential to create significant economic and social impacts. As businesses and governments invest in these advancements, they are transforming how we live, work, and interact. Key emerging technologies include artificial intelligence, blockchain, quantum computing, edge computing, and new technology trends. They offer new solutions to existing problems, enhance efficiency and productivity, and often lead to the creation of new markets and job opportunities.
9.1.1. Quantum Computing Integration
Quantum computing is a revolutionary technology that leverages the principles of quantum mechanics to process information. Unlike classical computers, which use bits as the smallest unit of data, quantum computers use qubits. This allows them to perform complex calculations at unprecedented speeds.
- Speed and Efficiency: Quantum computers can solve problems that are currently intractable for classical computers, such as optimization problems and simulations of molecular interactions. Rapid Innovation can assist clients in harnessing quantum computing to streamline operations and enhance decision-making processes, ultimately leading to greater ROI.
- Applications: Industries such as pharmaceuticals, finance, and logistics are exploring quantum computing for drug discovery, risk analysis, and supply chain optimization. By partnering with Rapid Innovation, organizations can leverage our expertise to implement quantum solutions tailored to their specific needs, driving innovation and competitive advantage.
- Integration Challenges: Despite its potential, integrating quantum computing into existing systems poses challenges, including the need for specialized algorithms and hardware. Rapid Innovation offers consulting services to navigate these complexities, ensuring a smooth transition and maximizing the benefits of quantum technologies.
The global quantum computing market is expected to grow significantly, with estimates suggesting it could reach $65 billion by 2030. As organizations begin to adopt quantum technologies, they will need to invest in training and infrastructure to fully leverage its capabilities, and Rapid Innovation is here to guide them through this transformative journey.
9.1.2. Edge Computing
Edge computing refers to the practice of processing data closer to the source of data generation rather than relying on a centralized data center. This technology is becoming increasingly important as the Internet of Things (IoT) expands and the volume of data generated continues to rise.
- Reduced Latency: By processing data at the edge, organizations can achieve faster response times, which is critical for applications like autonomous vehicles and real-time analytics. Rapid Innovation can help clients implement edge computing solutions that enhance operational efficiency and improve customer experiences.
- Bandwidth Efficiency: Edge computing reduces the amount of data that needs to be transmitted to the cloud, saving bandwidth and lowering costs. Our team at Rapid Innovation can assist businesses in optimizing their data management strategies, leading to significant cost savings and improved performance.
- Enhanced Security: Keeping data processing local can improve security by minimizing the risk of data breaches during transmission. Rapid Innovation provides robust security solutions to ensure that edge computing implementations are secure and compliant with industry standards.
The edge computing market is projected to grow to $43.4 billion by 2027, driven by the increasing demand for real-time data processing and analytics. As businesses adopt edge computing, they will need to consider the implications for their IT infrastructure and data management strategies, and Rapid Innovation is well-equipped to support them in this transition.
In addition to these advancements, new AI technology and emerging IT technologies are also playing a crucial role in shaping the future landscape. The latest technological advancements, including new battery technology and emerging tech, are paving the way for innovative solutions that address contemporary challenges. As we move into 2023, the focus on new technologies and the latest technology trends will continue to drive progress and transformation across industries. For instance, our expertise in ChatGPT applications development can help businesses leverage AI to enhance their operations and customer interactions.
9.1.3. Federated Learning
Federated Learning is a decentralized approach to machine learning that allows multiple devices to collaboratively learn a shared model while keeping their data localized. This method addresses privacy concerns and reduces the need for data transfer, making it particularly valuable in sensitive applications.
- Key Features:
- Data remains on the device, enhancing privacy and security.
- Only model updates are shared, minimizing bandwidth usage.
- Enables learning from diverse data sources without compromising individual data privacy.
- Applications:
- Healthcare: Federated Learning can be used to train models on patient data across hospitals without sharing sensitive information, allowing healthcare providers to improve patient outcomes while maintaining compliance with regulations. This includes federated learning in medical imaging and applications of federated learning in various healthcare scenarios.
- Finance: Banks can collaborate on fraud detection models while keeping customer data secure, enabling them to enhance their security measures without compromising client confidentiality. This is particularly relevant in the context of federated learning for malware detection in IoT devices.
- Mobile Devices: Personal assistants can improve their performance by learning from user interactions without sending personal data to the cloud, thus providing a more personalized experience while safeguarding user privacy. This can also extend to federated learning for mobile keyboard prediction.
- Challenges:
- Communication efficiency: Frequent updates can lead to high communication costs, which can impact the overall efficiency of the learning process. This is a critical consideration in scaffold federated learning.
- Model convergence: Ensuring that the global model converges effectively with diverse local data distributions can be complex, requiring sophisticated algorithms and strategies, especially in federated machine learning concept and applications.
- Security risks: While data is not shared, the model updates can still be vulnerable to attacks, necessitating robust security measures to protect the integrity of the learning process. This is particularly important in federated learning anomaly detection.
9.2. Research Directions
Research in machine learning and artificial intelligence is rapidly evolving, with several promising directions that aim to enhance the capabilities and applications of these technologies.
- Explainable AI (XAI):
- Developing models that provide clear explanations for their predictions.
- Enhancing trust and transparency in AI systems, especially in critical sectors like healthcare and finance.
- Robustness and Security:
- Creating models that are resilient to adversarial attacks.
- Ensuring that AI systems can operate reliably in unpredictable environments.
- Transfer Learning:
- Investigating methods to transfer knowledge from one domain to another.
- Reducing the need for large labeled datasets in new applications.
- Ethical AI:
- Addressing biases in AI algorithms to ensure fairness.
- Establishing guidelines for responsible AI usage and deployment.
- Human-AI Collaboration:
- Exploring ways to enhance collaboration between humans and AI systems.
- Focusing on augmenting human decision-making rather than replacing it.
9.3. Industry Developments
The landscape of machine learning and AI is continuously evolving, with significant developments across various industries. These advancements are shaping how businesses operate and interact with technology.
- Healthcare Innovations:
- AI is being used for predictive analytics, improving patient outcomes by anticipating health issues.
- Machine learning algorithms are aiding in drug discovery, significantly reducing the time and cost involved.
- Financial Services:
- AI-driven algorithms are enhancing risk assessment and fraud detection.
- Robo-advisors are becoming more prevalent, providing personalized investment advice based on user data.
- Retail and E-commerce:
- Machine learning is optimizing supply chain management and inventory forecasting.
- Personalized marketing strategies are being developed using customer behavior analysis.
- Autonomous Vehicles:
- AI technologies are crucial for the development of self-driving cars, focusing on safety and efficiency.
- Machine learning models are being trained to interpret sensor data and make real-time driving decisions.
- Smart Manufacturing:
- AI is streamlining production processes through predictive maintenance and quality control.
- Machine learning is being integrated into IoT devices to enhance operational efficiency, including federated learning edge computing and federated learning in vehicular networks.
These developments highlight the transformative potential of machine learning and AI across various sectors, driving innovation and improving efficiency. At Rapid Innovation, we leverage these advancements to help our clients achieve their business goals efficiently and effectively, ensuring a greater return on investment through tailored AI and blockchain solutions.
9.4. Potential Breakthroughs
In the realm of innovation and technology, potential breakthroughs can significantly alter the landscape of industries and improve quality of life. These breakthroughs often stem from advancements in research, technology, and methodologies.
- Emerging Technologies: Innovations such as artificial intelligence breakthroughs, quantum computing, and blockchain technology are paving the way for unprecedented advancements. For instance, AI is revolutionizing sectors like healthcare, finance, and transportation by enhancing decision-making processes and automating tasks. Rapid Innovation leverages AI to develop predictive analytics tools that help businesses optimize operations and increase efficiency, ultimately leading to greater ROI.
- Sustainable Solutions: Breakthroughs in renewable energy technologies, such as solar and wind power, are crucial for combating climate change. Innovations in energy storage, like advanced battery technologies, such as the tesla battery breakthrough, are also essential for maximizing the efficiency of renewable energy sources. Rapid Innovation can assist organizations in integrating blockchain solutions to enhance transparency and traceability in renewable energy transactions, fostering trust and accountability.
- Healthcare Innovations: The development of personalized medicine and gene editing technologies, such as CRISPR, holds the potential to treat genetic disorders and improve patient outcomes. These advancements can lead to more effective treatments tailored to individual genetic profiles. Rapid Innovation employs AI-driven data analysis to help healthcare providers identify patient-specific treatment plans, thereby improving outcomes and reducing costs. The latest technology breakthroughs in healthcare are paving the way for these innovations.
- Space Exploration: Breakthroughs in space technology, including reusable rockets and advanced propulsion systems, are making space travel more accessible and cost-effective. This could lead to new opportunities for exploration and even colonization of other planets. Rapid Innovation is at the forefront of developing AI algorithms that optimize mission planning and resource allocation for space missions, enhancing the feasibility of ambitious projects. The next big technology breakthrough in space exploration could redefine our understanding of the universe.
- Data Analytics: The ability to analyze vast amounts of data in real-time is transforming industries. Big data analytics can provide insights that drive better business decisions, enhance customer experiences, and optimize operations. Rapid Innovation specializes in creating AI-powered analytics platforms that enable businesses to harness their data effectively, leading to improved decision-making and increased profitability. The latest discoveries in technology are enhancing data analytics capabilities across various sectors. For more insights on the potential of business AI, you can read about best practices in AI engineering.
10. Implementation Strategy
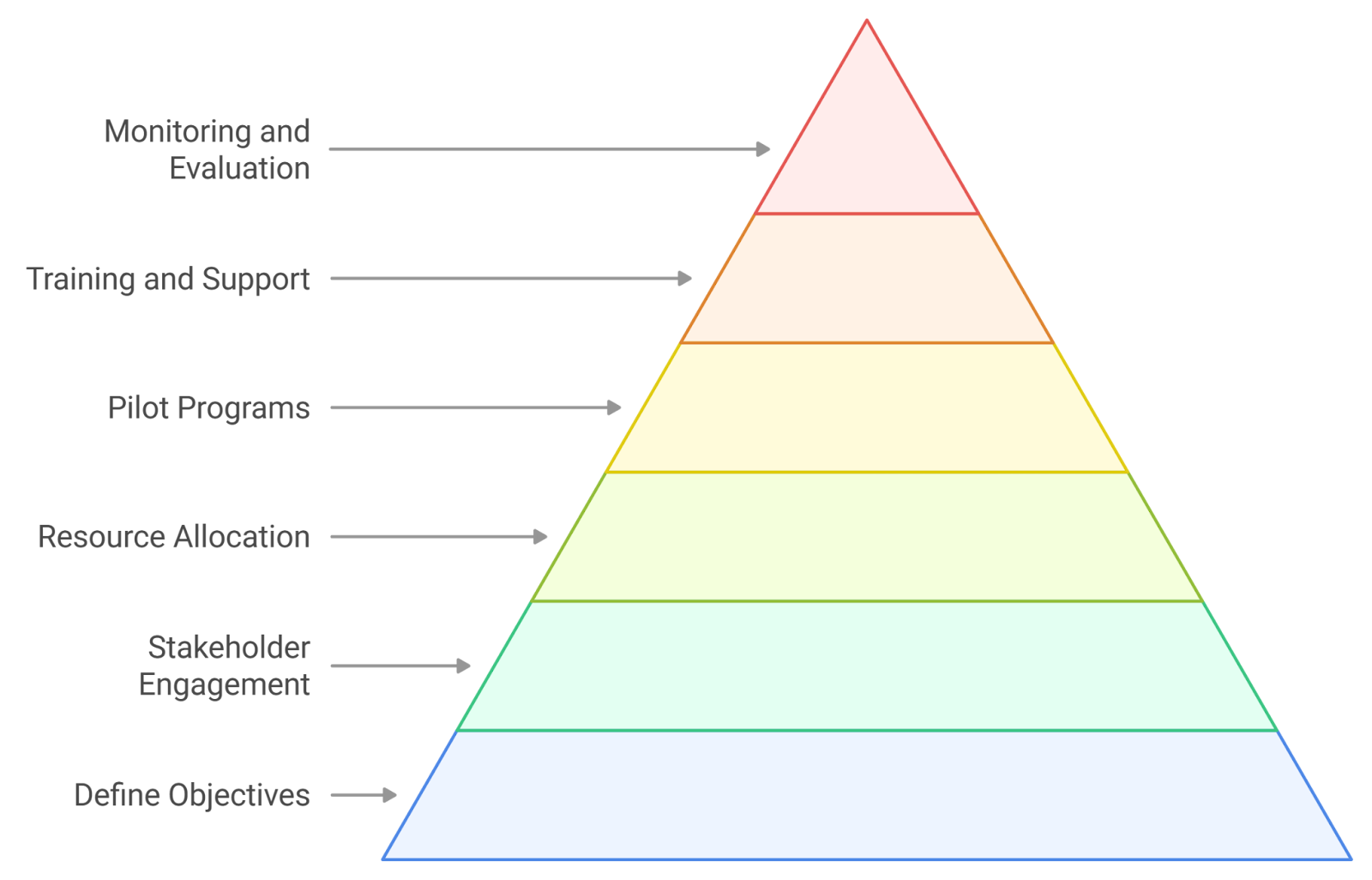
‍
An effective implementation strategy is crucial for translating potential breakthroughs into tangible results. This strategy outlines the steps necessary to integrate new technologies or processes into existing systems.
- Define Objectives: Clearly outline the goals of the implementation, including identifying the specific problems the breakthrough aims to solve and the desired outcomes.
- Stakeholder Engagement: Involve all relevant stakeholders early in the process, including team members, management, and external partners. Their input can provide valuable insights and foster a sense of ownership.
- Resource Allocation: Assess the resources required for successful implementation, including financial investments, human resources, and technological infrastructure.
- Pilot Programs: Before a full-scale rollout, consider implementing pilot programs. These smaller-scale tests can help identify potential challenges and allow for adjustments before wider implementation.
- Training and Support: Provide adequate training for all users involved in the new system or technology. Ongoing support is essential to address any issues that arise during the transition.
- Monitoring and Evaluation: Establish metrics to evaluate the success of the implementation. Regularly monitor progress and make necessary adjustments based on feedback and performance data.
10.1. Readiness Assessment
A readiness assessment is a critical step in the implementation strategy, ensuring that an organization is prepared to adopt new technologies or processes. This assessment evaluates various factors that influence the success of the implementation.
- Organizational Culture: Assess the existing culture within the organization. A culture that embraces change and innovation is more likely to support new initiatives.
- Technical Infrastructure: Evaluate the current technological capabilities. Determine if the existing systems can support the new technology or if upgrades are necessary.
- Skill Levels: Analyze the skill levels of employees and identify any gaps in knowledge or expertise that may hinder the implementation process.
- Change Management: Consider the organization’s experience with change management. A history of successful change initiatives can indicate a higher likelihood of success in adopting new technologies.
- Financial Readiness: Review the financial resources available for the implementation. Ensure that there is a budget allocated for both initial costs and ongoing maintenance.
- Regulatory Compliance: Assess any regulatory requirements that may impact the implementation. Understanding these regulations is crucial to avoid potential legal issues.
- Stakeholder Support: Gauge the level of support from key stakeholders. Their backing can be instrumental in overcoming resistance and ensuring a smooth transition. The next breakthrough technology could hinge on the readiness of stakeholders to embrace change.
10.2. Resource Planning
Resource planning is a critical component of project management that involves identifying, allocating, and managing resources effectively to ensure project success. It encompasses human resources, financial resources, and physical assets.
- Identifying Resources: Determine what resources are needed for the project, including personnel, equipment, and materials. At Rapid Innovation, we leverage AI-driven analytics to assess resource requirements accurately, ensuring that our clients have the right tools and talent at their disposal. This includes understanding the needs for enterprise resource planning and enterprise resource management.
- Allocation: Assign resources to specific tasks based on their availability and skill sets. This ensures that the right people are working on the right tasks. Our blockchain solutions facilitate transparent tracking of resource allocation, enhancing accountability and efficiency. We also consider the integration of ERP systems to streamline this process.
- Monitoring: Continuously track resource usage to avoid overallocation or underutilization. This helps in making timely adjustments. By employing AI algorithms, we can predict resource needs and optimize usage, leading to significant cost savings. Utilizing enterprise resource planning software can enhance our monitoring capabilities.
- Budgeting: Develop a budget that reflects the costs associated with the resources, including salaries, equipment costs, and any other expenses. Our consulting services include financial modeling that incorporates AI insights to forecast expenses accurately, particularly in the context of enterprise resource management software.
- Tools: Utilize software tools for resource planning, such as Microsoft Project or Trello, to visualize resource allocation and availability. We also offer custom-built solutions that integrate AI and blockchain technology for enhanced resource management, including ERP enterprise resource planning systems.
Effective resource planning leads to improved efficiency, reduced costs, and enhanced project outcomes. It is essential to regularly review and adjust plans as project dynamics change, especially when considering manufacturing resource planning and material requirement planning.
10.3. Risk Management
Risk management is the process of identifying, assessing, and mitigating risks that could potentially impact a project. It is essential for ensuring that projects are completed on time and within budget.
- Risk Identification: Recognize potential risks that could affect the project, including financial risks, operational risks, and external risks such as market changes. Our AI tools can analyze historical data to identify patterns and predict potential risks, including those related to enterprise resource systems.
- Risk Assessment: Evaluate the likelihood and impact of each identified risk to prioritize which risks need immediate attention. We utilize advanced analytics to provide a comprehensive risk assessment framework tailored to our clients' needs.
- Mitigation Strategies: Develop strategies to minimize the impact of risks, which can include contingency plans, insurance, or alternative approaches. Our blockchain solutions ensure that risk management processes are transparent and verifiable.
- Monitoring: Continuously monitor risks throughout the project lifecycle to allow for timely responses to any emerging risks. Our AI systems provide real-time monitoring and alerts, enabling proactive risk management.
- Communication: Keep stakeholders informed about risks and mitigation strategies. Transparency helps in building trust and ensuring everyone is on the same page. We emphasize clear communication channels supported by our technology solutions.
Effective risk management can lead to increased project success rates and reduced negative impacts on project objectives.
10.4. Change Management
Change management is the structured approach to managing changes in a project or organization. It ensures that changes are implemented smoothly and effectively, minimizing disruption.
- Change Identification: Recognize the need for change, whether due to external factors, stakeholder requests, or internal improvements. Our AI tools can analyze market trends to identify when changes are necessary, particularly in the context of ERP in IT.
- Impact Analysis: Assess how the proposed change will affect the project, including timelines, costs, and resources. We provide data-driven insights to help clients understand the implications of changes, especially when considering the integration of oracle cloud enterprise resource planning or SAP enterprise resource planning.
- Stakeholder Engagement: Involve stakeholders in the change process, as their input can provide valuable insights and foster buy-in. Our blockchain solutions facilitate stakeholder engagement through transparent communication.
- Implementation Plan: Develop a clear plan for implementing the change, including timelines, responsibilities, and communication strategies. We assist clients in creating robust implementation plans that align with their strategic goals.
- Training and Support: Provide necessary training and support to help team members adapt to the change, which can include workshops, manuals, or one-on-one coaching. Our AI-driven training modules ensure that team members are well-equipped to handle transitions.
Effective change management helps organizations adapt to new circumstances while maintaining productivity and morale. It is crucial for ensuring that changes align with overall project goals and objectives.
10.5. Success Metrics
Success metrics are essential for evaluating the effectiveness of any project or initiative. They provide a quantitative basis for assessing performance and determining whether objectives have been met. In the context of business, success metrics can vary widely depending on the goals of the organization.
- Key Performance Indicators (KPIs): These are specific metrics that align with business objectives. Common KPIs include:
- Revenue growth
- Customer acquisition cost
- Customer lifetime value
- Business operations KPIs
- KPIs for agile project management
- Engagement Metrics: These metrics help gauge how well your audience interacts with your content or services. Examples include:
- Website traffic
- Social media engagement rates
- Email open and click-through rates
- Conversion Rates: This metric measures the percentage of users who take a desired action, such as making a purchase or signing up for a newsletter. High conversion rates indicate effective marketing strategies.
- Customer Satisfaction: Surveys and feedback mechanisms can provide insights into customer satisfaction levels. Metrics like Net Promoter Score (NPS) can help assess customer loyalty.
- Operational Efficiency: Metrics such as time to market, production costs, and resource utilization can help evaluate how efficiently a business operates. This includes primary business metrics to track success of your product.
At Rapid Innovation, we leverage advanced AI analytics to track and interpret these success metrics, enabling our clients to make informed decisions that drive growth and enhance operational efficiency. By integrating AI-driven insights, we help businesses optimize their strategies and achieve their objectives more effectively. This includes measuring success of a business through various business success metrics and metrics to measure business success.
10.6. ROI Evaluation
Return on Investment (ROI) evaluation is a critical process for determining the profitability of an investment relative to its cost. It helps businesses understand the financial benefits of their initiatives and make data-driven decisions.
- Calculating ROI: The basic formula for ROI is:
```
ROI = (Net Profit / Cost of Investment) x 100
- Time Frame: Evaluating ROI over different time frames can provide insights into short-term versus long-term benefits.
- Qualitative Benefits: While ROI is often expressed in financial terms, qualitative benefits should also be considered. These can include:
- Brand reputation enhancement
- Improved customer relationships
- Employee satisfaction
- Benchmarking: Comparing ROI against industry standards or competitors can provide context and help identify areas for improvement.
- Continuous Monitoring: Regularly assessing ROI allows businesses to adapt strategies and optimize resource allocation.
At Rapid Innovation, we utilize blockchain technology to ensure transparency and traceability in ROI evaluations. By providing immutable records of transactions and investments, we help our clients gain a clearer understanding of their financial performance. Effective ROI evaluation not only highlights successful investments but also uncovers areas that may require adjustment or reevaluation.
11. Ethical Considerations
Ethical considerations are paramount in any business operation. They guide decision-making processes and help maintain a positive reputation. Addressing ethical issues can also enhance customer trust and loyalty.
- Transparency: Businesses should be open about their practices, including pricing, sourcing, and data usage. Transparency fosters trust and accountability.
- Fair Treatment: Ensuring fair treatment of employees, customers, and suppliers is crucial. This includes:
- Equal pay for equal work
- Non-discriminatory practices
- Respect for diversity and inclusion
- Environmental Responsibility: Companies should consider their environmental impact and strive for sustainable practices. This can involve:
- Reducing waste
- Using renewable resources
- Supporting eco-friendly initiatives
- Data Privacy: With increasing concerns about data security, businesses must prioritize protecting customer information. This includes:
- Implementing robust cybersecurity measures
- Being transparent about data collection and usage
- Corporate Social Responsibility (CSR): Engaging in CSR initiatives can enhance a company's reputation and contribute positively to society. This can include:
- Supporting local communities
- Investing in education and health programs
- Promoting ethical sourcing and production practices
By integrating ethical considerations into their operations, businesses can build a strong foundation for long-term success and foster a positive impact on society. At Rapid Innovation, we are committed to upholding these ethical standards in all our AI and blockchain solutions, ensuring that our clients not only achieve their business goals but do so responsibly and sustainably.
11.1. Privacy Concerns
Privacy concerns are increasingly significant in today's digital landscape, especially with the rise of data-driven technologies such as AI and privacy. Individuals are more aware of how their personal information is collected, stored, and used. Personal data can include sensitive information such as names, addresses, financial details, and health records. The potential for misuse of this data raises alarms about identity theft and unauthorized access, leading to various AI privacy concerns. Regulations like the General Data Protection Regulation (GDPR) and the California Consumer Privacy Act (CCPA) have been established to protect consumer privacy rights. Companies must be transparent about their data collection practices and obtain explicit consent from users. Additionally, the use of cookies and tracking technologies can lead to invasive advertising practices, further heightening privacy concerns, particularly in the context of big data and privacy issues.
- Personal data can include sensitive information such as names, addresses, financial details, and health records.
- The potential for misuse of this data raises alarms about identity theft and unauthorized access.
- Regulations like the General Data Protection Regulation (GDPR) and the California Consumer Privacy Act (CCPA) have been established to protect consumer privacy rights.
- Companies must be transparent about their data collection practices and obtain explicit consent from users.
- The use of cookies and tracking technologies can lead to invasive advertising practices, further heightening privacy concerns.
At Rapid Innovation, we understand the importance of privacy in the digital age. Our AI and Blockchain solutions are designed to help businesses implement robust privacy measures, ensuring compliance with regulations while maintaining user trust. By leveraging blockchain technology, we can create transparent data management systems that empower users with control over their personal information, ultimately enhancing customer loyalty and driving greater ROI. We also address specific issues such as data privacy concerns and AI privacy issues to ensure comprehensive protection. Our expertise in enterprise AI development ensures that your business can navigate the complexities of privacy in the digital landscape effectively.
11.4. Transparency Requirements
Transparency requirements are essential for fostering trust and accountability in various sectors, particularly in finance, healthcare, and corporate governance. These requirements mandate organizations to disclose relevant information to stakeholders, ensuring that operations are conducted openly and ethically.
- Clear communication of financial performance, risks, and governance structures is crucial.
- Organizations must provide accessible information regarding their policies, practices, and decision-making processes, including compliance with cms price transparency and cms hospital price transparency.
- Transparency helps in building stakeholder confidence, which can lead to increased investment and customer loyalty. For example, adherence to state price transparency reporting can enhance trust among consumers.
- Regulatory bodies often impose transparency requirements, such as the transparency requirements outlined in the CAA transparency in coverage, to protect consumers and ensure fair practices.
- Companies are encouraged to adopt best practices in reporting, such as sustainability reports and corporate social responsibility disclosures, including compliance with cms chargemaster requirements and cms hospital charges.
The implementation of transparency requirements, such as those specified in cms price transparency requirements and cms transparency reporting, can lead to improved organizational performance and reputation. For instance, companies that prioritize transparency often experience better employee engagement and lower turnover rates. At Rapid Innovation, we leverage AI-driven analytics to help organizations streamline their reporting processes, ensuring that transparency is not just a requirement but a competitive advantage.
11.5. Compliance Standards
Compliance standards are established guidelines that organizations must follow to ensure they operate within legal and ethical boundaries. These standards vary by industry and are designed to protect consumers, employees, and the environment.
- Compliance standards often include regulations related to data protection, financial reporting, and workplace safety, as well as transparency reporting requirements in healthcare.
- Organizations must regularly assess their compliance with these standards to avoid legal penalties and reputational damage.
- Training and awareness programs are essential for employees to understand compliance requirements and their implications, including health plan price transparency requirements and health plan transparency requirements.
- Non-compliance can result in significant financial losses, including fines and legal fees, as well as damage to brand reputation.
- Many industries have specific compliance frameworks, such as ISO standards for quality management and GDPR for data protection, alongside regulations like 26 CFR 54.9815 2715A3.
Adhering to compliance standards not only mitigates risks but also enhances operational efficiency and fosters a culture of integrity within the organization. Rapid Innovation offers tailored blockchain solutions that ensure data integrity and compliance, enabling organizations to maintain trust while navigating complex regulatory landscapes.
12. Case Studies
Case studies provide valuable insights into how organizations implement transparency requirements and compliance standards in real-world scenarios. They illustrate best practices, challenges faced, and the outcomes of various strategies.
- A notable case is the implementation of the Sarbanes-Oxley Act in the United States, which was enacted to enhance corporate governance and accountability. Companies had to adopt stricter financial reporting and auditing practices, leading to increased transparency in financial disclosures.
- Another example is the General Data Protection Regulation (GDPR) in the European Union, which set a new standard for data protection and privacy. Organizations had to revamp their data handling practices, ensuring compliance while maintaining transparency with consumers about how their data is used.
- In the healthcare sector, the Affordable Care Act introduced transparency requirements for health insurance providers, mandating clear communication about coverage options and costs, including hospital price transparency 2021 and price transparency healthcare cms. This led to improved consumer understanding and choice in healthcare services.
These case studies highlight the importance of transparency and compliance in building trust and ensuring ethical practices across various industries. They serve as a guide for organizations looking to enhance their own practices in these areas. At Rapid Innovation, we are committed to helping our clients navigate these complexities through innovative AI and blockchain solutions, ultimately driving greater ROI and sustainable growth.
12.1. Enterprise Implementation Examples
Enterprise implementation refers to the process of integrating new systems, technologies, or methodologies within a business to enhance efficiency and productivity. Here are some notable examples:
- Customer Relationship Management (CRM) Systems: Companies like Salesforce have transformed how businesses manage customer interactions. By implementing CRM systems, enterprises can track customer data, streamline communication, and improve sales processes. Rapid Innovation can assist in customizing CRM solutions that leverage AI to provide predictive insights, enhancing customer engagement and driving sales growth.
- Enterprise Resource Planning (ERP) Systems: Organizations such as SAP and Oracle provide ERP solutions that integrate various business functions, including finance, HR, and supply chain management. This integration allows for real-time data access and improved decision-making. Rapid Innovation specializes in integrating blockchain technology into ERP systems, ensuring data integrity and transparency across all business functions. Additionally, effective erp change management is crucial for a smooth transition during these implementations.
- Cloud Computing Solutions: Companies like Amazon Web Services (AWS) and Microsoft Azure have enabled enterprises to migrate their operations to the cloud. This shift reduces infrastructure costs, enhances scalability, and improves collaboration among teams. Rapid Innovation can help businesses transition to cloud-based solutions while implementing AI-driven analytics for better resource management and operational efficiency.
- Artificial Intelligence (AI) Integration: Businesses are increasingly adopting AI technologies for predictive analytics, customer service chatbots, and process automation. For instance, IBM's Watson has been implemented in various sectors to enhance data analysis and decision-making. Rapid Innovation offers tailored AI solutions that can automate routine tasks, improve customer interactions, and provide actionable insights, ultimately leading to greater ROI. Furthermore, incorporating erp organizational change management strategies can facilitate the adoption of AI technologies within organizations. For more insights on the impact of predictive analysis in retail, check out this article on the power of predictive analysis in retail.
12.2. Research Applications
Research applications leverage advanced technologies and methodologies to gather, analyze, and interpret data across various fields. Here are some key areas where research applications are making an impact:
- Healthcare Research: Technologies like machine learning and big data analytics are used to analyze patient data, leading to improved treatment plans and drug discovery. For example, researchers utilize AI algorithms to identify potential new drugs by analyzing vast datasets. Rapid Innovation can develop AI models that enhance predictive analytics in healthcare, improving patient outcomes and reducing costs.
- Environmental Studies: Remote sensing technologies and geographic information systems (GIS) are employed to monitor environmental changes, assess natural resources, and study climate change impacts. These tools help researchers collect and analyze data on deforestation, pollution, and biodiversity. Rapid Innovation can integrate blockchain solutions to ensure the authenticity and traceability of environmental data.
- Social Sciences: Data analytics and survey tools are used to conduct sociological research, enabling researchers to gather insights on public opinion, behavior patterns, and demographic trends. This data can inform policy decisions and social programs. Rapid Innovation can provide AI-driven analytics tools that enhance the accuracy and depth of social research.
- Market Research: Businesses utilize research applications to analyze consumer behavior, market trends, and competitive landscapes. Tools like Google Analytics and social media analytics provide valuable insights that guide marketing strategies. Rapid Innovation can develop customized AI solutions that offer deeper insights into consumer behavior, enabling more effective marketing strategies.
12.3. Industry-specific Solutions
Industry-specific solutions are tailored technologies and methodologies designed to address the unique challenges faced by different sectors. Here are some examples:
- Manufacturing: Industry 4.0 technologies, such as IoT and automation, are revolutionizing manufacturing processes. Companies like Siemens and GE are implementing smart factories that utilize connected devices to optimize production efficiency and reduce downtime. Rapid Innovation can help integrate AI and blockchain into manufacturing processes, enhancing supply chain transparency and operational efficiency.
- Finance: Fintech solutions, including blockchain and robo-advisors, are transforming the financial services industry. Companies like Square and Robinhood are providing innovative payment solutions and investment platforms that cater to a tech-savvy audience. Rapid Innovation specializes in developing secure blockchain applications that enhance transaction transparency and reduce fraud in financial services.
- Retail: E-commerce platforms and inventory management systems are essential for modern retail businesses. Companies like Shopify and Magento offer solutions that help retailers manage online sales, track inventory, and enhance customer experiences. Rapid Innovation can implement AI-driven analytics to optimize inventory management and personalize customer experiences, driving sales and customer loyalty.
- Education: Learning management systems (LMS) and online course platforms are reshaping education. Institutions are adopting solutions like Moodle and Coursera to provide flexible learning options and access to a broader range of resources. Rapid Innovation can develop AI-enhanced LMS solutions that adapt to individual learning styles, improving educational outcomes.
- Transportation and Logistics: Companies are utilizing route optimization software and fleet management systems to enhance logistics operations. Solutions from providers like Fleet Complete and Trimble help businesses reduce costs and improve delivery times. Rapid Innovation can integrate AI and blockchain technologies to optimize logistics operations, ensuring real-time tracking and improved supply chain efficiency. Additionally, enterprise implementation solutions can further streamline these logistics processes.
12.4. Success Stories
Success stories are powerful narratives that showcase how individuals, organizations, or communities have achieved their goals despite challenges. These stories often serve as inspiration and motivation for others facing similar obstacles. Here are some notable success stories across various sectors:
- Business Innovation: Companies like Apple and Amazon have transformed their industries through innovative products and services. Apple’s introduction of the iPhone revolutionized mobile technology, while Amazon’s e-commerce model changed how consumers shop. Their success is attributed to a strong focus on customer experience and continuous innovation. At Rapid Innovation, we leverage AI to help businesses analyze customer data, enabling them to create tailored solutions that enhance user experience and drive sales. This is similar to the inspiring business stories of entrepreneurs who have successfully navigated the startup landscape.
- Social Impact: Organizations such as TOMS Shoes have made a significant impact by integrating social responsibility into their business model. For every pair of shoes sold, TOMS donates a pair to a child in need. This model not only drives sales but also creates a positive social impact, demonstrating that businesses can thrive while contributing to society. Rapid Innovation supports similar initiatives by utilizing blockchain technology to ensure transparency in charitable donations, fostering trust and accountability. The success stories of women entrepreneurs in this space highlight the potential for social enterprises to make a difference.
- Education: Programs like Teach for America have successfully placed thousands of teachers in underserved communities, improving educational outcomes. By recruiting passionate individuals to teach in low-income areas, they have made strides in closing the educational gap and inspiring future generations. Rapid Innovation employs AI-driven analytics to assess educational needs and optimize resource allocation, helping organizations maximize their impact in education. The story of a young entrepreneur who started an educational initiative can serve as a powerful example of this impact.
- Health and Wellness: The success of the anti-smoking campaign in various countries has led to a significant decrease in smoking rates. For instance, Australia’s plain packaging laws and graphic health warnings have contributed to a 15% drop in smoking prevalence since their implementation. These initiatives highlight the effectiveness of public health campaigns in changing behaviors. Rapid Innovation can assist health organizations by implementing AI solutions that analyze patient data, leading to more effective health interventions and improved outcomes. The motivational business stories from health startups illustrate the potential for innovation in this sector.
- Environmental Sustainability: Companies like Patagonia have set a benchmark for environmental responsibility in the apparel industry. By using sustainable materials and promoting recycling, Patagonia has built a loyal customer base that values eco-friendly practices. Their commitment to sustainability has not only enhanced their brand image but also encouraged other companies to adopt similar practices. Rapid Innovation utilizes blockchain to create transparent supply chains, allowing businesses to track their environmental impact and promote sustainability. The success stories of startups focused on sustainability further emphasize the importance of eco-conscious business practices.
12.5. Lessons Learned

‍
Lessons learned from both successes and failures are crucial for growth and improvement. Analyzing these experiences can provide valuable insights that can be applied in future endeavors. Here are some key lessons learned from various sectors:
- Adaptability is Key: The ability to pivot and adapt to changing circumstances is essential for success. Businesses that can quickly respond to market trends or consumer needs are more likely to thrive. For example, during the COVID-19 pandemic, many restaurants shifted to takeout and delivery models to survive. Rapid Innovation helps clients implement AI solutions that enable real-time data analysis, allowing for swift adaptations to market changes. This adaptability is a common theme in many entrepreneur stories.
- Collaboration Drives Innovation: Working together with diverse teams can lead to innovative solutions. Companies that foster a culture of collaboration often see increased creativity and problem-solving capabilities. This is evident in tech firms where cross-functional teams work together to develop groundbreaking products. At Rapid Innovation, we promote collaborative environments by integrating AI tools that enhance communication and project management. The success stories of business collaborations often highlight the power of teamwork.
- Data-Driven Decisions: Utilizing data analytics can significantly enhance decision-making processes. Organizations that leverage data to understand customer behavior and market trends can make informed choices that lead to better outcomes. For instance, Netflix uses viewer data to inform its content creation strategy, resulting in popular original series. Rapid Innovation empowers businesses to harness AI-driven analytics for strategic decision-making, ultimately leading to greater ROI. The success stories of online businesses often showcase the importance of data in driving growth.
- Embrace Failure as a Learning Opportunity: Failure is often a stepping stone to success. Many successful entrepreneurs have faced setbacks before achieving their goals. Learning from these failures can provide insights that lead to better strategies in the future. For example, Thomas Edison famously stated that he did not fail but rather found 10,000 ways that won’t work. Rapid Innovation encourages a culture of experimentation, where AI can be used to analyze past failures and inform future strategies. The stories of startup failures often provide valuable lessons for aspiring entrepreneurs.
- Focus on Customer Experience: Prioritizing customer satisfaction can lead to long-term loyalty and success. Companies that actively seek feedback and make improvements based on customer input tend to outperform their competitors. Brands like Zappos have built their reputation on exceptional customer service, resulting in a loyal customer base. Rapid Innovation utilizes AI to gather and analyze customer feedback, enabling businesses to enhance their service offerings and improve customer satisfaction. The success stories of small businesses often highlight the importance of customer-centric approaches.
- Sustainability Matters: As consumers become more environmentally conscious, businesses must consider their impact on the planet. Companies that adopt sustainable practices not only contribute to environmental preservation but also attract customers who value corporate responsibility. This trend is evident in the rise of eco-friendly products and brands. Rapid Innovation assists organizations in implementing blockchain solutions that promote sustainability and transparency in their operations. The success stories of entrepreneurs focused on sustainability illustrate the growing demand for responsible business practices.
- Continuous Learning and Development: Investing in employee training and development is crucial for organizational success. Companies that prioritize learning create a more skilled workforce, leading to increased productivity and innovation. Organizations like Google offer extensive training programs to foster employee growth and adaptability. Rapid Innovation provides AI-driven training solutions that personalize learning experiences, ensuring employees are equipped with the skills needed to thrive in a rapidly changing environment. The success stories of young entrepreneurs often emphasize the importance of continuous learning.
By reflecting on these success stories and lessons learned, individuals and organizations can better navigate challenges and seize opportunities for growth and improvement. Rapid Innovation stands ready to partner with you in leveraging AI and blockchain technologies to achieve your business goals efficiently and effectively. For more insights on integrating AI ethics into transformative innovation.