Table Of Contents
Category
Artificial Intelligence
Healthcare & Medicine
1. Introduction to AI in Medication Safety
Artificial Intelligence (AI) is transforming various sectors, and healthcare is no exception. In the realm of AI in medication safety, AI agents are emerging as powerful tools that enhance patient care and pharmaceutical safety. These intelligent systems leverage vast amounts of data to identify potential medication errors, monitor patient responses, and ensure adherence to treatment protocols. AI algorithms can analyze patient data in real-time, allowing for immediate intervention when issues arise. Additionally, machine learning models can predict adverse drug reactions based on historical data, improving patient outcomes. Natural language processing (NLP) enables AI to interpret clinical notes and identify discrepancies in medication administration.
At Rapid Innovation, we understand that the integration of AI in medication safety monitoring is not just about technology; it represents a paradigm shift in how healthcare providers approach patient care. By utilizing our AI solutions, healthcare professionals can make more informed decisions, ultimately leading to safer medication practices and improved operational efficiency.
AI's role in medication safety is multifaceted, encompassing various applications such as:
- Error Detection: Our AI systems can flag potential medication errors before they reach the patient, significantly reducing the risk of harm and enhancing patient safety.
- Adherence Monitoring: We provide AI-driven tools that track patient adherence to prescribed medications, offering timely reminders and alerts to both patients and healthcare providers, thereby improving treatment outcomes.
- Data Analysis: Our advanced AI capabilities can sift through large datasets to identify trends and patterns that may indicate safety concerns, enabling proactive measures to be taken.
As the healthcare landscape continues to evolve, the importance of AI in medication safety will only grow. At Rapid Innovation, we are committed to paving the way for improved patient care and enhanced pharmaceutical safety, ensuring that our clients achieve greater ROI through effective and efficient AI solutions.
Refer to the image for a visual representation of AI's role in medication safety:

1.1. The Critical Need for Advanced Medication Monitoring
Medication monitoring is essential for ensuring patient safety and treatment efficacy. With the increasing complexity of medication regimens, the need for advanced medication monitoring systems has become critical.
- Rising chronic diseases: Conditions such as diabetes, hypertension, and heart disease require patients to manage multiple medications, increasing the risk of errors.
- Adverse drug reactions: According to studies, nearly 1.3 million people are injured each year due to medication errors in the U.S. alone. Advanced medication monitoring can help mitigate these risks.
- Patient adherence: Non-adherence to medication regimens can lead to worsening health outcomes. Advanced medication monitoring systems can track adherence and provide reminders to patients.
- Data integration: Advanced medication monitoring systems can integrate data from various sources, including electronic health records (EHRs) and wearable devices, providing a comprehensive view of a patient's health status.
- Personalized care: With advanced medication monitoring, healthcare providers can tailor medication plans based on real-time data, improving treatment outcomes.
1.2. Challenges in Traditional Medication Management
Traditional medication management methods face several challenges that can compromise patient safety and treatment effectiveness.
- Manual processes: Many healthcare providers still rely on paper-based systems, which are prone to errors and inefficiencies.
- Lack of real-time data: Traditional methods often do not provide real-time insights into a patient's medication adherence or health status, making it difficult to make timely adjustments.
- Communication gaps: Poor communication between healthcare providers, patients, and pharmacists can lead to misunderstandings about medication regimens.
- Fragmented care: Patients often see multiple specialists, leading to disjointed medication management and increased risk of drug interactions.
- Limited patient engagement: Traditional methods may not actively involve patients in their medication management, reducing adherence and overall satisfaction.
1.3. AI as a Transformative Technology in Healthcare
Artificial Intelligence (AI) is revolutionizing healthcare, particularly in medication management, by providing innovative solutions to existing challenges.
- Predictive analytics: AI can analyze vast amounts of data to predict potential medication-related issues, allowing for proactive interventions. For instance, Rapid Innovation employs AI algorithms to identify patients at risk of non-adherence, enabling healthcare providers to intervene before issues arise.
- Personalized medicine: AI algorithms can tailor medication plans based on individual patient data, improving efficacy and reducing side effects. Rapid Innovation's solutions can help healthcare providers customize treatment plans that align with each patient's unique health profile.
- Enhanced decision support: AI tools can assist healthcare providers in making informed decisions by providing evidence-based recommendations and alerts for potential drug interactions. Rapid Innovation integrates these tools into existing healthcare systems, enhancing the decision-making process.
- Automation of routine tasks: AI can automate administrative tasks, such as prescription refills and appointment scheduling, freeing up healthcare professionals to focus on patient care. Rapid Innovation's automation solutions streamline these processes, leading to increased efficiency and reduced operational costs.
- Improved patient engagement: AI-driven applications can empower patients by providing personalized reminders and educational resources, enhancing adherence and understanding of their treatment plans. Rapid Innovation develops user-friendly applications that foster better communication between patients and healthcare providers, ultimately improving health outcomes.
By leveraging AI technologies, Rapid Innovation helps healthcare organizations achieve greater ROI through enhanced patient safety, improved treatment efficacy, and streamlined operations.
Refer to the image for a visual representation of the critical need for advanced medication monitoring and the role of AI in healthcare.

1.4. Ethical and Regulatory Considerations
The integration of AI in healthcare, particularly in medication safety, raises several ethical and regulatory considerations that must be addressed to ensure patient safety and trust.
- Patient Privacy: AI systems often require access to vast amounts of patient data. Ensuring that this data is handled in compliance with regulations like HIPAA in the U.S. is crucial. Protecting patient confidentiality while utilizing data for AI training is a significant concern that Rapid Innovation prioritizes in its development processes, especially in the context of AI in healthcare.
- Bias and Fairness: AI algorithms can inadvertently perpetuate biases present in the training data, leading to unequal treatment outcomes among different demographic groups. Rapid Innovation emphasizes the importance of regularly auditing AI systems for bias and ensuring diverse data representation to foster equitable healthcare solutions, particularly in areas like medical AI and artificial intelligence in healthcare.
- Accountability: Determining who is responsible when an AI system makes a mistake is complex. Clear guidelines must be established regarding liability, especially when AI recommendations lead to adverse patient outcomes. Rapid Innovation advocates for transparent accountability frameworks to enhance trust in AI applications, including those related to artificial intelligence and medical diagnosis.
- Informed Consent: Patients should be informed about how AI is used in their care, including understanding the role of AI in medication safety and the potential risks involved. Rapid Innovation supports the development of clear communication strategies to ensure patients are adequately informed about AI in medicine and its implications.
- Regulatory Frameworks: Regulatory bodies like the FDA are developing frameworks to evaluate AI technologies in healthcare. These frameworks must balance innovation with safety, ensuring that AI tools are rigorously tested before deployment. Rapid Innovation stays abreast of these developments to ensure compliance and promote safe AI integration, particularly in the context of healthcare AI companies.
1.5. Scope of AI-Powered Medication Safety
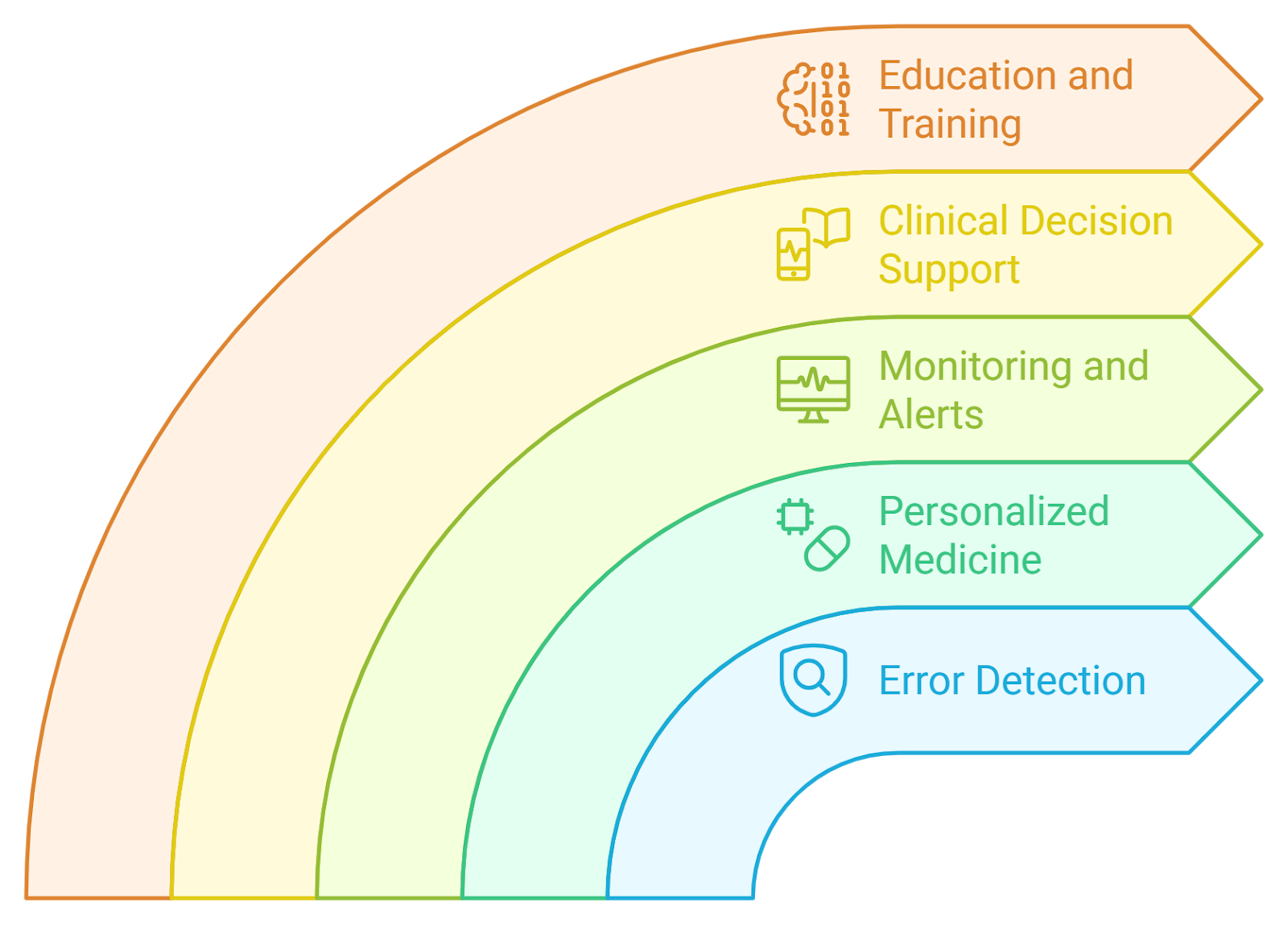
AI-powered medication safety encompasses a wide range of applications aimed at reducing medication errors and improving patient outcomes.
- Error Detection: AI systems can analyze prescription data to identify potential errors, such as incorrect dosages or drug interactions. This proactive approach can significantly reduce the incidence of medication-related adverse events, showcasing how Rapid Innovation's solutions can enhance patient safety through AI for medical applications.
- Personalized Medicine: AI can help tailor medication regimens to individual patients based on their genetic makeup, lifestyle, and other factors. This personalized approach enhances the effectiveness of treatments and minimizes side effects, demonstrating the value of Rapid Innovation's AI capabilities in delivering customized healthcare solutions, including artificial intelligence in medical field applications.
- Monitoring and Alerts: AI can continuously monitor patient data and alert healthcare providers to potential issues, such as adverse reactions or non-compliance with medication regimens. This real-time monitoring can lead to timely interventions, illustrating how Rapid Innovation's technology can improve patient outcomes in the context of AI medical diagnosis.
- Clinical Decision Support: AI tools can assist healthcare providers in making informed decisions about medication management. By analyzing vast amounts of clinical data, AI can provide evidence-based recommendations that enhance patient care, reflecting Rapid Innovation's commitment to empowering healthcare professionals through artificial intelligence in healthcare.
- Education and Training: AI can be used to develop training programs for healthcare professionals, ensuring they are well-versed in the latest medication safety protocols and technologies. Rapid Innovation's expertise in AI can facilitate the creation of effective educational resources, particularly in the realm of AI in healthcare companies.
2. Foundational Technologies
The foundational technologies that support AI in medication safety are critical for the successful implementation and effectiveness of these systems.
- Machine Learning: This subset of AI enables systems to learn from data and improve over time. Machine learning algorithms can analyze historical medication data to identify patterns and predict potential errors, showcasing Rapid Innovation's proficiency in developing advanced AI solutions for medical artificial intelligence.
- Natural Language Processing (NLP): NLP allows AI systems to understand and interpret human language. This technology is essential for analyzing clinical notes, prescriptions, and patient communications to extract relevant information for medication safety, highlighting Rapid Innovation's capabilities in harnessing NLP for healthcare applications, including AI for health.
- Big Data Analytics: The ability to process and analyze large datasets is fundamental to AI applications in healthcare. Big data analytics can uncover insights from diverse sources, including electronic health records (EHRs), lab results, and patient feedback, demonstrating how Rapid Innovation can drive data-driven decision-making in the context of AI companies in healthcare.
- Cloud Computing: Cloud technology provides the infrastructure needed to store and process vast amounts of data securely. It enables healthcare organizations to access AI tools and share information seamlessly across platforms, reflecting Rapid Innovation's commitment to scalable and secure solutions in the medical field.
- Interoperability Standards: For AI systems to function effectively, they must be able to communicate with various healthcare systems. Adopting interoperability standards ensures that data can be shared and utilized across different platforms, enhancing the overall effectiveness of AI in medication safety, a key focus area for Rapid Innovation and its initiatives in healthcare AI companies.
Refer to the image for a visual representation of the ethical and regulatory considerations in AI-powered medication safety.
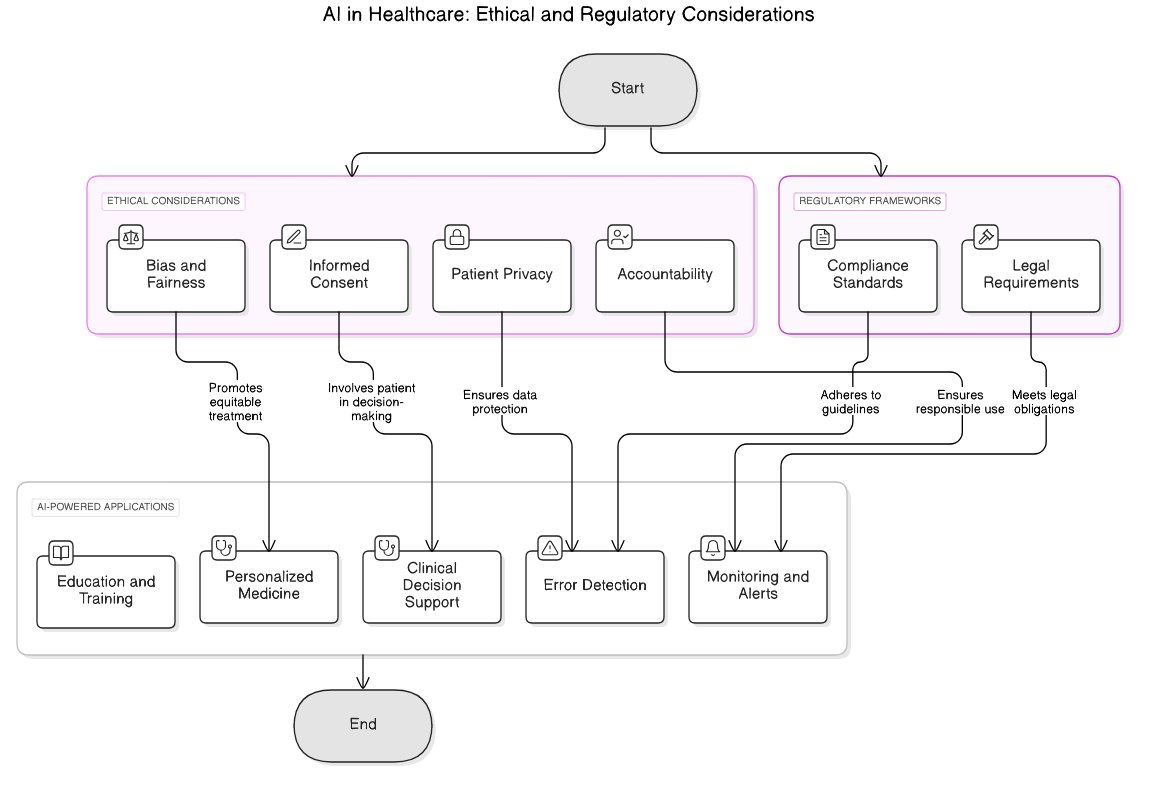
2.1. Machine Learning Architectures
Machine learning architectures are the frameworks that define how algorithms process data and learn from it. These architectures can vary significantly based on the type of data being analyzed and the specific tasks being performed. At Rapid Innovation, we leverage these architectures, including lstm network and deep learning architecture, to create tailored solutions that drive efficiency and maximize ROI for our clients.
- Supervised Learning: This architecture involves training a model on a labeled dataset, where the input data is paired with the correct output. Common algorithms include linear regression, decision trees, and support vector machines. By implementing supervised learning, we help clients predict outcomes and make data-driven decisions, enhancing their operational efficiency.
- Unsupervised Learning: In this architecture, models are trained on data without labeled responses. The goal is to identify patterns or groupings within the data. Techniques include clustering algorithms like K-means and hierarchical clustering. Rapid Innovation utilizes unsupervised learning to uncover hidden insights in client data, enabling them to identify new market opportunities and optimize their strategies.
- Reinforcement Learning: This architecture is based on the idea of agents that learn to make decisions by taking actions in an environment to maximize cumulative rewards. It is widely used in robotics and game playing. Our expertise in reinforcement learning allows us to develop intelligent systems that adapt and improve over time, providing clients with innovative solutions that enhance their competitive edge.
- Deep Learning: A subset of machine learning that uses neural networks with many layers (deep networks) to analyze various forms of data. It excels in tasks like image and speech recognition. Rapid Innovation employs deep learning to create advanced applications that can process complex data, leading to improved customer experiences and operational efficiencies. We also explore architectures of neural networks in machine learning to optimize performance.
- Hybrid Models: These combine different architectures to leverage the strengths of each. For example, combining supervised and unsupervised learning can enhance model performance. By utilizing hybrid models, we ensure that our clients benefit from the most effective machine learning strategies tailored to their unique needs, including architecture of machine learning model and architecture machine learning.
Understanding these architectures is crucial for developing effective machine learning solutions tailored to specific applications, and at Rapid Innovation, we are committed to guiding our clients through this process to achieve their business goals.
2.2. Natural Language Processing
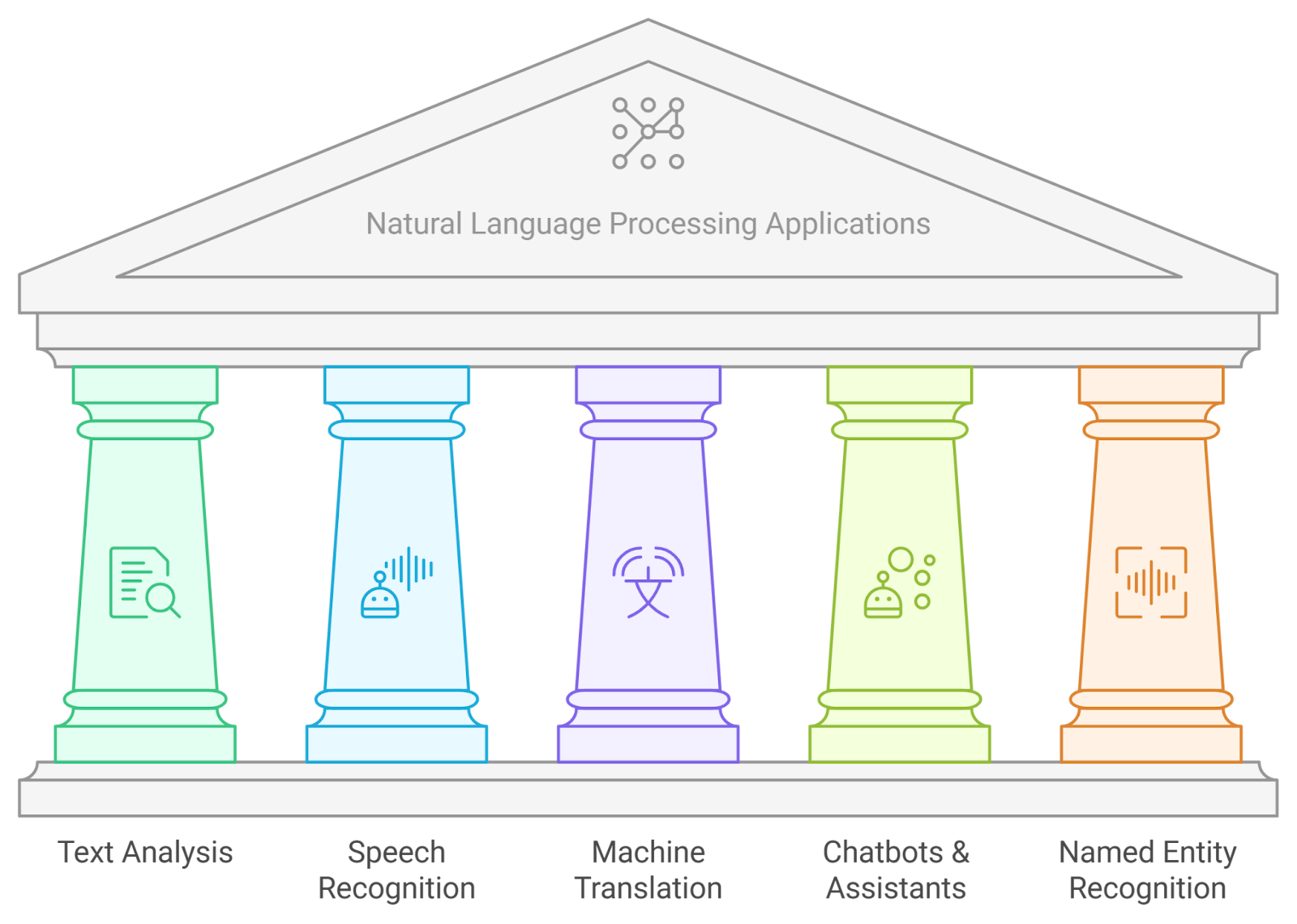
Natural Language Processing (NLP) is a field of artificial intelligence that focuses on the interaction between computers and humans through natural language. It enables machines to understand, interpret, and generate human language in a valuable way. Rapid Innovation harnesses NLP to enhance communication and engagement for our clients.
- Text Analysis: NLP techniques are used to analyze text data, extracting meaningful information and insights. This includes sentiment analysis, topic modeling, and keyword extraction. By implementing text analysis, we help clients gain valuable insights into customer sentiment and market trends, driving informed decision-making.
- Speech Recognition: This technology converts spoken language into text. It is widely used in virtual assistants like Siri and Google Assistant, allowing for hands-free operation and accessibility. Our solutions in speech recognition enable clients to enhance user experiences and accessibility, leading to increased customer satisfaction.
- Machine Translation: NLP powers translation services, enabling real-time translation of text and speech across different languages. Google Translate is a prominent example of this application. Rapid Innovation's machine translation solutions help clients expand their reach in global markets by breaking down language barriers.
- Chatbots and Virtual Assistants: These systems use NLP to understand user queries and provide relevant responses, enhancing customer service and user engagement. By developing intelligent chatbots, we empower our clients to improve customer interactions and streamline support processes.
- Named Entity Recognition (NER): This process identifies and classifies key entities in text, such as names, organizations, and locations, which is essential for information extraction. Our NER solutions enable clients to extract critical information from large datasets, enhancing their data analysis capabilities.
NLP is transforming how we interact with technology, making it more intuitive and user-friendly, and at Rapid Innovation, we are dedicated to leveraging these advancements to help our clients achieve their business objectives.
2.3. Computer Vision Technologies
Computer vision is a field of artificial intelligence that enables machines to interpret and make decisions based on visual data from the world. It encompasses a variety of techniques and applications that allow computers to "see" and understand images and videos. Rapid Innovation utilizes computer vision technologies to create innovative solutions that drive efficiency and effectiveness for our clients.
- Image Recognition: This technology identifies objects, people, and scenes within images. It is widely used in applications like facial recognition and automated tagging on social media platforms. Our image recognition solutions help clients enhance security and automate processes, leading to significant cost savings.
- Object Detection: Beyond recognizing objects, this technology locates them within an image. It is crucial for applications such as autonomous vehicles, where identifying pedestrians and obstacles is essential for safety. Rapid Innovation's expertise in object detection allows us to develop systems that improve safety and operational efficiency for our clients.
- Image Segmentation: This process divides an image into segments to simplify its analysis. It is used in medical imaging to identify tumors or other anomalies in scans. By implementing image segmentation, we assist clients in the healthcare sector to improve diagnostic accuracy and patient outcomes.
- Video Analysis: Computer vision technologies can analyze video streams in real-time, enabling applications like surveillance, traffic monitoring, and sports analytics. Our video analysis solutions provide clients with actionable insights, enhancing their operational capabilities and decision-making processes.
- Augmented Reality (AR): AR combines computer vision with real-world environments, allowing users to interact with digital elements overlaid on their physical surroundings. This technology is used in gaming, education, and retail. Rapid Innovation's AR solutions enable clients to create immersive experiences that engage customers and drive sales.
Computer vision technologies are revolutionizing industries by enabling machines to process and understand visual information, leading to smarter and more efficient systems. At Rapid Innovation, we are committed to helping our clients harness these technologies to achieve their business goals effectively and efficiently.
Refer to the image based on the Machine Learning Architectures for a visual representation of the concepts discussed.
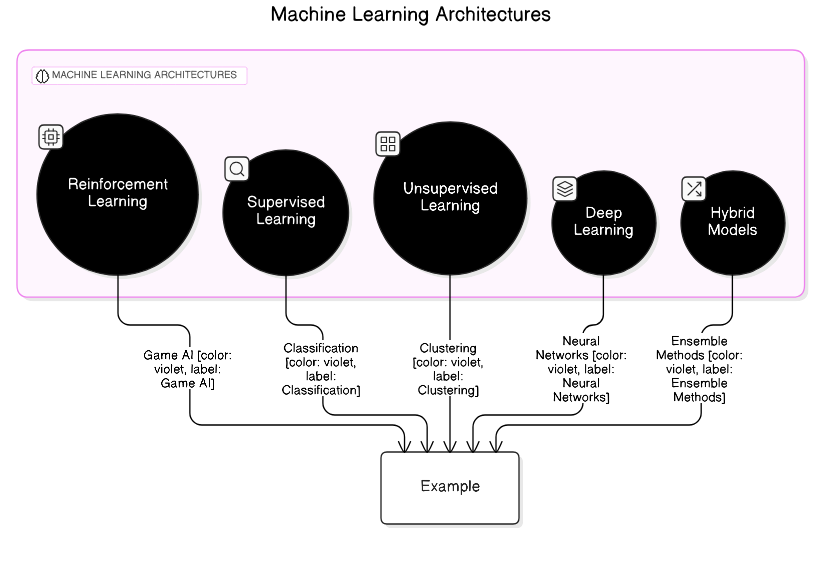
2.4. Big Data Analytics
Big Data Analytics refers to the process of examining large and varied data sets to uncover hidden patterns, correlations, and insights. This field has gained immense importance due to the exponential growth of data generated from various sources, including social media, IoT devices, and transactional systems.
- Key components of Big Data Analytics include:
- Volume: The sheer amount of data generated daily, often measured in terabytes or petabytes.
- Velocity: The speed at which data is generated and processed, requiring real-time analytics capabilities.
- Variety: The different types of data, including structured, semi-structured, and unstructured data.
- Benefits of Big Data Analytics:
- Enhanced decision-making through data-driven insights.
- Improved operational efficiency by identifying bottlenecks and optimizing processes.
- Better customer experiences by analyzing consumer behavior and preferences.
- Tools and technologies used in Big Data Analytics:
- Apache Hadoop: A framework that allows for distributed storage and processing of large data sets.
- Apache Spark: A fast and general-purpose cluster computing system for big data processing.
- NoSQL databases: Such as MongoDB and Cassandra, which are designed to handle unstructured data.
At Rapid Innovation, we leverage Big Data Analytics, including big data and data analytics, to help our clients achieve greater ROI by transforming raw data into actionable insights. For instance, we have assisted a retail client in analyzing customer purchasing patterns, leading to targeted marketing strategies that increased sales by 20%. By utilizing advanced analytics tools, we enable organizations to make informed decisions that drive efficiency and enhance customer satisfaction. For more information on how AI can enhance marketing applications.
Big Data Analytics is transforming industries by enabling organizations to leverage big data and data analysis for strategic advantages.
2.5. Predictive Modeling Techniques
Predictive modeling techniques are statistical methods used to forecast future outcomes based on historical data. These techniques are widely used in various fields, including finance, healthcare, marketing, and risk management.
- Common predictive modeling techniques include:
- Regression Analysis: A statistical method for estimating the relationships among variables. It helps in predicting a dependent variable based on one or more independent variables.
- Decision Trees: A flowchart-like structure that helps in making decisions based on different conditions. It is intuitive and easy to interpret.
- Neural Networks: A set of algorithms modeled after the human brain, used for complex pattern recognition and prediction tasks.
- Applications of predictive modeling:
- Customer segmentation and targeting in marketing campaigns.
- Fraud detection in financial transactions.
- Predicting patient outcomes in healthcare settings.
- Challenges in predictive modeling:
- Data quality and availability can significantly impact model accuracy.
- Overfitting occurs when a model is too complex and captures noise instead of the underlying trend.
- Interpretability of models, especially with complex algorithms like neural networks, can be a concern for stakeholders.
At Rapid Innovation, we employ predictive modeling techniques to help clients anticipate market trends and customer needs. For example, we developed a predictive model for a healthcare provider that improved patient outcome predictions, leading to a 15% reduction in readmission rates. Our expertise in predictive analytics empowers organizations to make proactive decisions that enhance operational efficiency and customer satisfaction.
2.6. Real-time Data Integration Frameworks
Real-time data integration frameworks are systems designed to combine data from various sources and provide immediate access to that data for analysis and decision-making. These frameworks are crucial for organizations that require timely insights to respond to changing conditions.
- Key features of real-time data integration frameworks:
- Data Streaming: The ability to process data in real-time as it is generated, allowing for immediate analysis.
- Event-Driven Architecture: Systems that react to events or changes in data, enabling quick responses to new information.
- Data Quality Management: Ensuring that the integrated data is accurate, consistent, and reliable.
- Benefits of real-time data integration:
- Enhanced agility in decision-making processes.
- Improved customer engagement through timely responses to inquiries or issues.
- Better operational efficiency by monitoring processes in real-time.
- Popular tools and technologies for real-time data integration:
- Apache Kafka: A distributed event streaming platform capable of handling trillions of events a day.
- Apache NiFi: A tool for automating the flow of data between systems, providing real-time data integration capabilities.
- Microsoft Azure Stream Analytics: A real-time analytics service that enables the processing of streaming data.
At Rapid Innovation, we implement real-time data integration frameworks to ensure our clients can respond swiftly to market changes. For instance, we helped a financial services firm integrate real-time data streams, which improved their fraud detection capabilities and reduced response times by 30%. Our solutions enable businesses to maintain a competitive edge in a fast-paced environment by providing timely insights for informed decision-making.
3. AI Agent Capabilities in Medication Safety
Artificial Intelligence (AI) is revolutionizing the healthcare sector, particularly in medication safety. At Rapid Innovation, we leverage AI agents designed to enhance patient safety by identifying potential risks associated with medication use. Their capabilities in detecting adverse drug reactions (ADRs) and predicting drug interactions are crucial for improving patient outcomes and achieving greater ROI for healthcare providers.
3.1 Adverse Drug Reaction (ADR) Detection
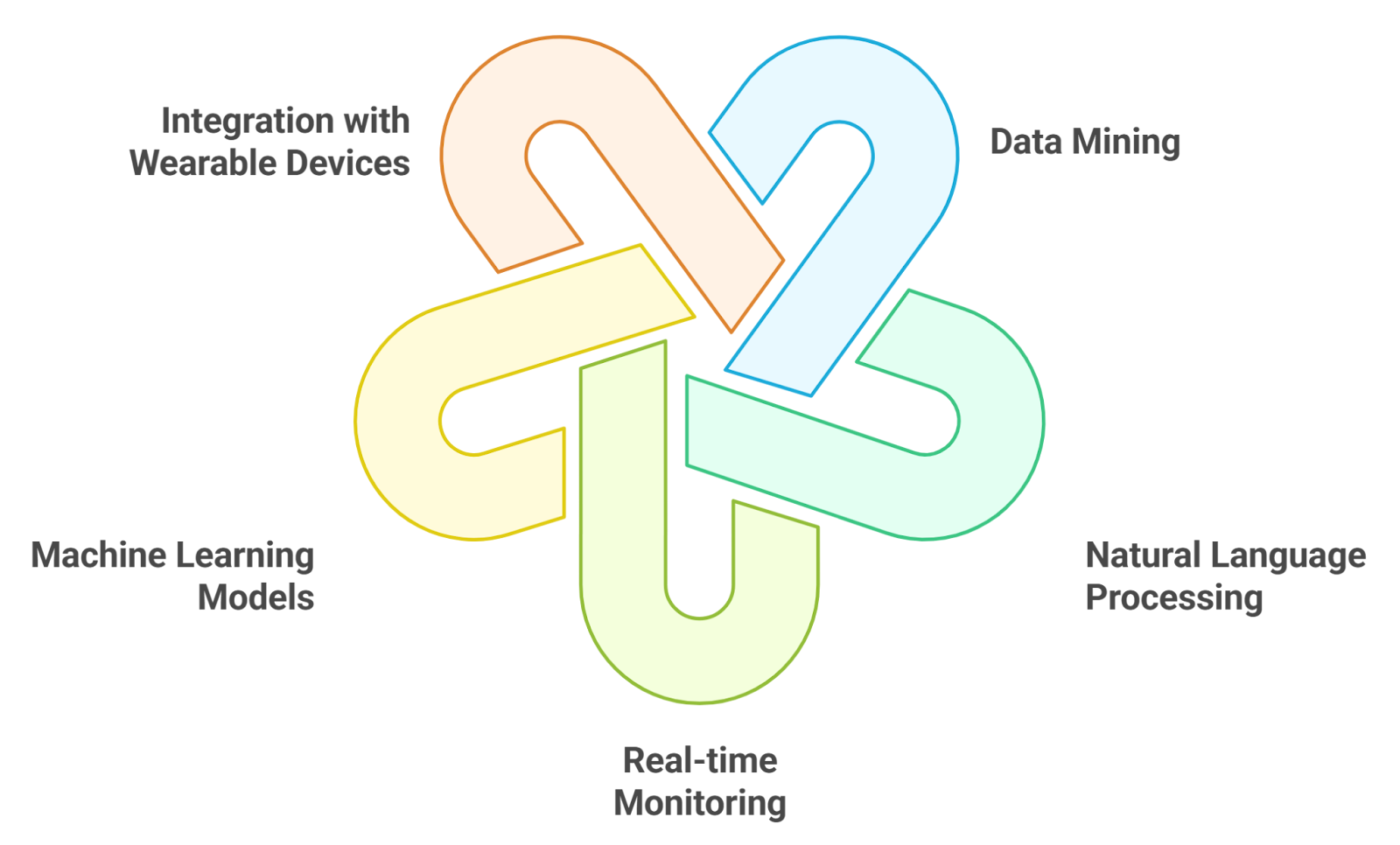
Adverse Drug Reactions (ADRs) are harmful or unintended responses to medications. Detecting ADRs is vital for ensuring patient safety and effective treatment. AI agents can significantly enhance ADR detection through various methods:
- Data Mining: Our AI algorithms analyze vast amounts of data from electronic health records (EHRs), clinical trials, and pharmacovigilance databases to identify patterns associated with ADRs. This helps in recognizing potential ADRs earlier than traditional methods, ultimately reducing healthcare costs.
- Natural Language Processing (NLP): We utilize NLP to sift through unstructured data, such as clinical notes and patient reports. By extracting relevant information, our AI can identify ADRs that may not be documented in structured formats, leading to more comprehensive patient safety measures.
- Real-time Monitoring: Our AI systems monitor patient data in real-time, allowing for immediate detection of ADRs as they occur. This proactive approach can lead to quicker interventions, enhancing patient safety and reducing the burden on healthcare systems.
- Machine Learning Models: These models predict the likelihood of ADRs based on patient-specific factors, such as age, gender, genetic makeup, and existing health conditions. This personalized approach enhances the accuracy of ADR detection, leading to better treatment outcomes.
- Integration with Wearable Devices: Our AI can analyze data from wearable health devices to monitor patients' physiological responses to medications. This continuous monitoring helps in identifying ADRs that may arise post-medication, ensuring timely interventions.
The implementation of AI in ADR detection has shown promising results. For instance, studies indicate that AI can improve the detection rate of ADRs by up to 30% compared to traditional methods.
3.2 Drug Interaction Prediction
Drug interactions occur when the effects of one drug are altered by the presence of another drug, food, or substance. Predicting these interactions is essential for preventing adverse effects and ensuring effective treatment. AI agents play a pivotal role in drug interaction prediction through the following capabilities:
- Predictive Analytics: Our AI algorithms analyze historical data to predict potential drug interactions. By examining previous cases and outcomes, AI can identify combinations of drugs that may lead to adverse effects, thereby enhancing patient safety.
- Knowledge Graphs: We utilize knowledge graphs to map relationships between different drugs, their mechanisms of action, and known interactions. This visual representation helps healthcare providers understand complex interactions more easily, facilitating informed decision-making.
- Simulation Models: Our AI can create simulation models to predict how different drugs interact within the body. These models consider various factors, including dosage, timing, and patient-specific characteristics, to provide accurate predictions.
- Integration with Clinical Decision Support Systems (CDSS): Our AI enhances CDSS by providing real-time alerts about potential drug interactions during the prescribing process. This integration helps healthcare providers make informed decisions and reduces the risk of harmful interactions.
- Continuous Learning: Our AI systems continuously learn from new data, improving their predictive capabilities over time. As more data becomes available, AI can refine its algorithms to enhance the accuracy of drug interaction predictions.
The effectiveness of AI in drug interaction prediction is supported by research showing that AI can reduce the incidence of drug interactions by up to 50% in clinical settings.
In conclusion, AI agents are transforming medication safety by enhancing the detection of adverse drug reactions and predicting drug interactions. These capabilities not only improve patient safety but also contribute to more effective and personalized healthcare. At Rapid Innovation, we are committed to harnessing the power of AI technology to expand its role in medication safety, leading to better health outcomes for patients and greater ROI for healthcare organizations. AI in medication safety is a critical focus area for us, ensuring that we stay at the forefront of innovation in this essential aspect of healthcare. For more insights on the role of AI in healthcare, check out our article on the role of generative AI in accelerating drug discovery and personalized medicine.
3.3. Personalized Medication Risk Assessment
Personalized medication risk assessment is a crucial component of modern healthcare, focusing on tailoring medication plans to individual patient needs. This approach considers various factors that can influence a patient's response to medication, thereby enhancing safety and efficacy.
- Individualized factors include:
- Genetic makeup: Pharmacogenomics can identify how a patient metabolizes drugs, allowing for tailored dosages.
- Medical history: Previous reactions to medications can inform future prescriptions.
- Current health conditions: Chronic illnesses may affect drug interactions and effectiveness.
- Benefits of personalized medication risk assessment:
- Reduces adverse drug reactions: By understanding a patient's unique profile, healthcare providers can minimize the risk of harmful side effects.
- Optimizes therapeutic outcomes: Tailored medication plans can lead to better management of conditions and improved patient satisfaction.
- Enhances patient engagement: Patients are more likely to adhere to treatment plans that consider their individual circumstances.
- Tools and technologies:
- Electronic health records (EHRs): These can integrate patient data for comprehensive assessments.
- Decision support systems: These can provide real-time recommendations based on patient-specific information.
At Rapid Innovation, we leverage AI algorithms to analyze patient data and predict medication responses, ensuring that healthcare providers can make informed decisions that align with personalized treatment plans. Our blockchain solutions can enhance the security and integrity of patient data, ensuring that sensitive information is protected while enabling seamless access for healthcare professionals. Additionally, our Metaverse Healthcare Solutions can further enhance personalized medication risk assessment by providing immersive environments for patient education and engagement.
3.4. Prescription Error Prevention
Prescription errors can have serious consequences for patient safety and healthcare costs. Implementing effective strategies for prescription error prevention is essential in reducing these risks.
- Common types of prescription errors:
- Incorrect dosage: Prescribing too much or too little can lead to ineffective treatment or toxicity.
- Wrong medication: Confusing similar drug names can result in administering the incorrect medication.
- Incomplete information: Failing to include necessary instructions can lead to misuse.
- Strategies for preventing prescription errors:
- Utilize technology: E-prescribing systems can reduce handwriting errors and improve clarity.
- Implement double-check systems: Having a second healthcare professional review prescriptions can catch potential mistakes.
- Educate healthcare providers: Regular training on best practices for prescribing can enhance awareness of common pitfalls.
- Importance of communication:
- Encourage open dialogue: Between patients and providers to clarify any uncertainties regarding prescriptions.
- Provide clear instructions: And educational materials to ensure patients understand their medication regimens.
Rapid Innovation can assist healthcare organizations in implementing advanced e-prescribing systems powered by AI, which can analyze historical data to flag potential errors before they occur. Our blockchain technology can also ensure that prescription records are immutable and transparent, reducing the likelihood of fraud and enhancing trust in the system.
3.5. Patient Adherence Monitoring
Patient adherence monitoring is vital for ensuring that individuals follow their prescribed treatment plans. Non-adherence can lead to poor health outcomes and increased healthcare costs.
- Factors influencing patient adherence:
- Complexity of medication regimens: Multiple medications or complicated dosing schedules can overwhelm patients.
- Side effects: Adverse reactions may discourage patients from continuing their treatment.
- Lack of understanding: Patients may not fully grasp the importance of their medications or how to take them correctly.
- Strategies to improve adherence:
- Simplify medication regimens: Whenever possible, consolidate medications to reduce the number of doses.
- Use technology: Mobile apps and reminders can help patients keep track of their medications.
- Foster a supportive environment: Encourage family involvement and support systems to motivate patients.
- Monitoring tools:
- Medication adherence tools: Such as pillboxes or smart caps, can help track usage.
- Regular follow-ups and check-ins: Can provide opportunities to address any concerns or barriers to adherence.
By focusing on personalized medication risk assessment, prescription error prevention, and patient adherence monitoring, healthcare providers can significantly enhance patient safety and treatment outcomes. Rapid Innovation's expertise in AI and blockchain can empower healthcare organizations to implement these strategies effectively, ultimately leading to improved patient care and greater ROI.
3.6. Real-time Clinical Decision Support
Real-time clinical decision support (CDS) systems are designed to assist healthcare professionals in making informed decisions at the point of care. These systems leverage advanced algorithms and data analytics to provide timely, evidence-based recommendations. They enhance patient safety by reducing the likelihood of errors, improve clinical outcomes through timely interventions, and integrate seamlessly with electronic health records (EHR) to provide contextual information. Additionally, they utilize machine learning to analyze vast amounts of patient data and identify patterns, while offering alerts and reminders for preventive care, medication interactions, and diagnostic suggestions.
Real-time CDS systems can significantly reduce the cognitive load on healthcare providers, allowing them to focus more on patient care rather than administrative tasks. By providing actionable insights, these systems can lead to better patient management and improved healthcare efficiency. According to a study, implementing real-time clinical decision support can reduce medication errors by up to 55%.
At Rapid Innovation, we specialize in developing and implementing advanced real-time clinical decision support systems tailored to the unique needs of healthcare organizations. Our expertise in AI and data analytics enables us to create solutions that not only enhance clinical decision-making but also drive greater ROI by improving patient outcomes and operational efficiency. For more information on the benefits and implementation strategies of clinical decision support software.
4. Technical Architecture of AI Safety Agents
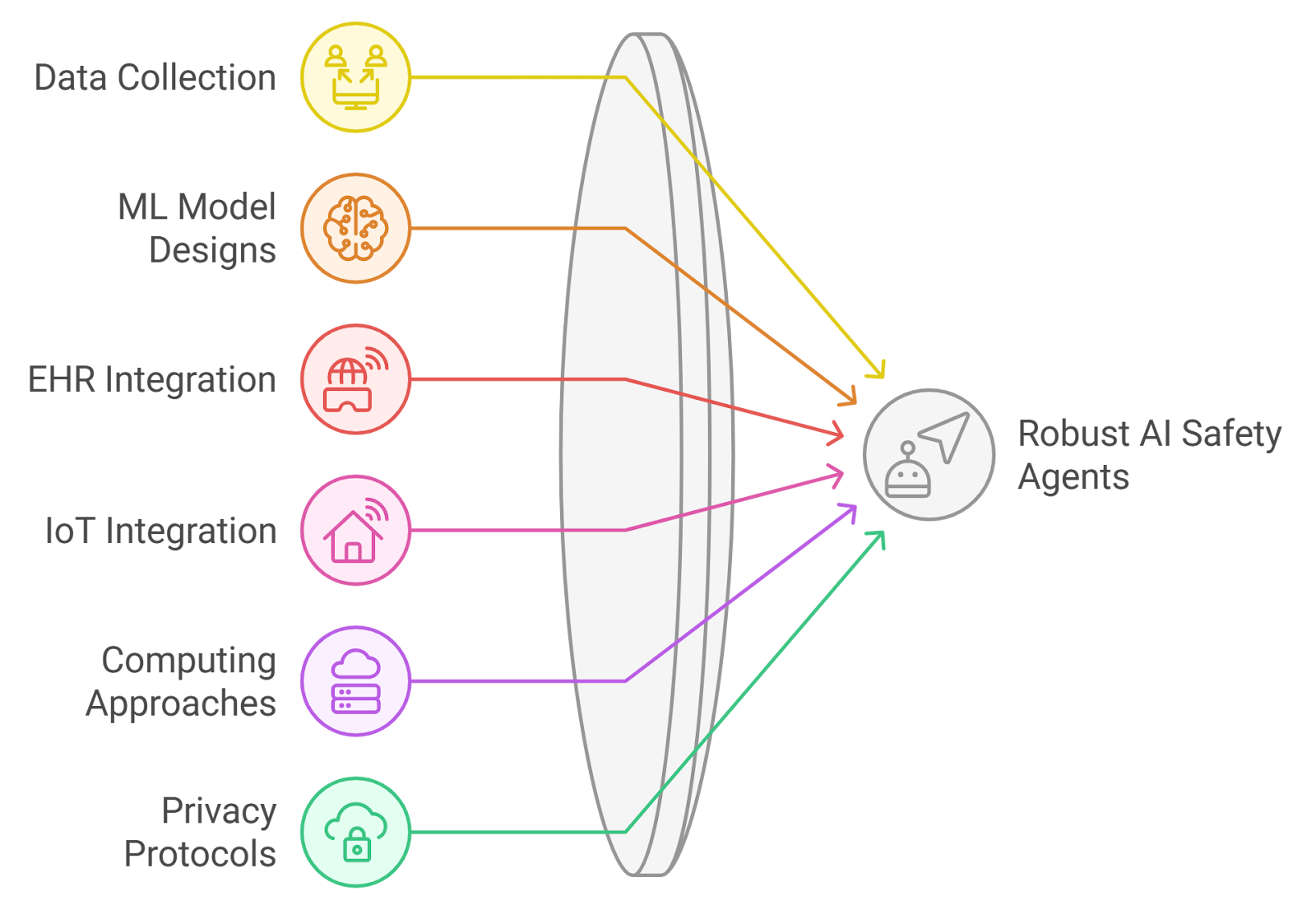
The technical architecture of AI safety agents is crucial for ensuring their effectiveness and reliability in various applications, particularly in healthcare. This architecture typically consists of several layers that work together to process data, make decisions, and provide feedback.
- Data Layer: This layer is responsible for collecting and storing data from various sources, including EHRs, medical devices, and patient monitoring systems.
- Processing Layer: Here, data is analyzed using machine learning algorithms and artificial intelligence techniques to derive insights and recommendations.
- Decision Layer: This layer interprets the processed data and generates actionable recommendations for healthcare providers.
- User Interface Layer: The final layer presents the information in a user-friendly format, allowing healthcare professionals to easily access and understand the insights provided by the AI safety agents.
The architecture must prioritize security and privacy, ensuring that sensitive patient data is protected. Additionally, it should be designed for scalability to accommodate the growing volume of healthcare data. A well-structured architecture can enhance the performance of AI safety agents, leading to better decision-making and improved patient outcomes.
4.1. Data Collection Mechanisms
Data collection mechanisms are essential for the functionality of AI safety agents, as they provide the necessary information for analysis and decision-making. These mechanisms can vary widely, depending on the sources and types of data being collected.
- Electronic Health Records (EHR): EHRs are a primary source of patient data, containing comprehensive medical histories, treatment plans, and medication records.
- Wearable Devices: Devices such as smartwatches and fitness trackers collect real-time health data, including heart rate, activity levels, and sleep patterns.
- Patient Surveys and Feedback: Collecting patient-reported outcomes and experiences can provide valuable insights into treatment effectiveness and patient satisfaction.
- Clinical Trials and Research Studies: Data from clinical trials can be used to inform AI models, ensuring they are based on the latest scientific evidence.
- Public Health Data: Aggregated data from public health sources can help identify trends and inform decision-making at a population level.
Effective data collection mechanisms must ensure data accuracy, timeliness, and relevance. They should also comply with regulations such as HIPAA to protect patient privacy. By utilizing diverse data sources, AI safety agents can provide more comprehensive insights, ultimately leading to better healthcare outcomes. At Rapid Innovation, we are committed to developing robust data collection strategies that empower healthcare organizations to leverage their data effectively for improved decision-making and enhanced patient care.
4.2. Machine Learning Model Designs
Machine learning (ML) model designs are crucial in developing effective healthcare applications, including machine learning healthcare and machine learning applications in healthcare. These models analyze vast amounts of data to identify patterns, predict outcomes, and support clinical decision-making. At Rapid Innovation, we leverage our expertise in ML model designs to help clients achieve their business goals efficiently and effectively. Key aspects of ML model designs include:
- Data Preprocessing: Cleaning and preparing data is essential for accurate model performance. This includes handling missing values, normalizing data, and encoding categorical variables. Our team ensures that the data is primed for optimal model training, leading to improved accuracy and reliability.
- Feature Selection: Identifying the most relevant features can significantly enhance model accuracy. Techniques such as recursive feature elimination and LASSO regression help in selecting the best predictors. By focusing on the right features, we help clients maximize their return on investment (ROI) through more precise predictions.
- Model Selection: Various algorithms can be employed, including:
- Decision Trees
- Support Vector Machines (SVM)
- Neural Networks
- Ensemble Methods (e.g., Random Forests)
- Our experts guide clients in selecting the most suitable algorithms based on their specific needs, ensuring that the chosen model aligns with their operational objectives, such as machine learning for healthcare applications.
- Training and Validation: Splitting data into training and validation sets ensures that the model generalizes well to unseen data. Cross-validation techniques help in assessing model performance. This rigorous approach minimizes the risk of overfitting, leading to more robust solutions.
- Hyperparameter Tuning: Optimizing hyperparameters can lead to better model performance. Techniques like grid search and random search are commonly used. Our systematic tuning process enhances model efficiency, ultimately driving better business outcomes.
- Interpretability: Understanding how models make predictions is vital, especially in healthcare. Tools like SHAP (SHapley Additive exPlanations) and LIME (Local Interpretable Model-agnostic Explanations) can help in interpreting complex models. We prioritize model transparency, enabling clients to trust and act on the insights generated, particularly in applications of machine learning in healthcare.
4.3. Integration with Electronic Health Records (EHR)
Integrating machine learning models with Electronic Health Records (EHR) is a transformative step in healthcare. EHRs contain comprehensive patient data, making them a rich source for training ML models, including deep learning in healthcare. At Rapid Innovation, we specialize in seamless integration, ensuring that our clients can harness the full potential of their data. Key considerations for integration include:
- Data Interoperability: Ensuring that different EHR systems can communicate effectively is crucial. Standards like HL7 and FHIR facilitate data exchange between systems. Our solutions ensure that clients can access and utilize data from multiple sources, enhancing their analytical capabilities.
- Real-time Data Access: ML models can provide real-time insights when integrated with EHRs. This allows healthcare providers to make informed decisions based on the latest patient data. Our integration solutions empower clients to respond swiftly to patient needs, improving care delivery.
- Clinical Decision Support: ML models can enhance clinical decision support systems (CDSS) by providing predictive analytics. For example, they can predict patient deterioration or recommend personalized treatment plans. By implementing these systems, we help clients improve patient outcomes and operational efficiency, showcasing current uses of AI in healthcare.
- Data Privacy and Security: Protecting patient data is paramount. Compliance with regulations like HIPAA ensures that sensitive information is handled securely during integration. Our commitment to data security helps clients maintain trust and compliance in their operations.
- User-Friendly Interfaces: The integration should provide intuitive interfaces for healthcare professionals, ensuring that insights from ML models are easily accessible and actionable. We design user-centric solutions that enhance usability and facilitate better decision-making.
4.4. Sensor and IoT Integration
The integration of sensors and Internet of Things (IoT) devices in healthcare is revolutionizing patient monitoring and data collection. These technologies enable continuous health tracking and real-time data analysis, including applications of machine learning in healthcare. Rapid Innovation is at the forefront of this transformation, helping clients leverage IoT for enhanced patient care. Key aspects of sensor and IoT integration include:
- Wearable Devices: Devices like smartwatches and fitness trackers collect vital signs such as heart rate, blood pressure, and activity levels. This data can be used for predictive analytics and personalized care. Our expertise in IoT integration allows clients to harness this data for improved patient engagement and outcomes.
- Remote Patient Monitoring: IoT devices allow healthcare providers to monitor patients remotely, reducing the need for hospital visits. This is particularly beneficial for chronic disease management. We enable clients to implement effective remote monitoring solutions, enhancing patient convenience and care quality.
- Data Collection and Analysis: Sensors generate vast amounts of data that can be analyzed using machine learning models, helping in identifying trends and making proactive healthcare decisions. Our analytical capabilities empower clients to make data-driven decisions that enhance operational efficiency, including machine learning in healthcare research papers.
- Interoperability with EHRs: Integrating IoT data with EHR systems enhances the richness of patient records, providing a comprehensive view of patient health over time. Our solutions ensure that clients can leverage all available data for holistic patient management.
- Patient Engagement: IoT devices can empower patients to take an active role in their health management. Mobile applications can provide feedback and reminders based on sensor data. We help clients develop engaging applications that foster patient involvement and adherence to treatment plans, such as machine learning healthcare applications.
- Challenges and Considerations: While integrating sensors and IoT devices offers numerous benefits, challenges such as data security, device compatibility, and user adoption must be addressed. Our team is dedicated to navigating these challenges, ensuring that clients can fully realize the potential of IoT in healthcare, including ethical machine learning in health care.
4.5. Cloud and Edge Computing Approaches
Cloud computing and edge computing are two pivotal technologies that are transforming how data is processed, stored, and managed. Each approach has its unique advantages and use cases, making them suitable for different scenarios.
- Cloud Computing: Centralized data storage and processing, offering scalability that allows businesses to adjust resources based on demand. It provides access to a wide range of services, including machine learning, analytics, and storage solutions, making it ideal for applications requiring significant computational power and large data sets. Rapid Innovation leverages cloud computing to help clients optimize their operations, reduce costs, and enhance their data analytics capabilities. Examples include Amazon Web Services (AWS), Microsoft Azure, and Google Cloud Platform. The integration of cloud and edge computing is becoming increasingly important, as seen in solutions like AWS Wavelengths, which extend AWS services to the edge.
- Edge Computing: Processes data closer to the source, reducing latency and bandwidth usage. It enhances real-time data processing, making it suitable for IoT devices and applications. By reducing the amount of data sent to the cloud, it can lower costs and improve response times. Rapid Innovation assists clients in implementing edge computing solutions that are critical for applications such as autonomous vehicles and smart cities, ensuring immediate data analysis and operational efficiency. Examples include Microsoft Azure IoT Edge and AWS Greengrass. The concept of edge clouding is also gaining traction, as it combines the benefits of edge computing with cloud capabilities.
- Hybrid Approaches: Combines both cloud and edge computing to leverage the strengths of each. This approach allows for flexibility in data management and processing, enabling businesses to choose where to process data based on specific needs, optimizing performance and cost. Rapid Innovation guides clients in developing hybrid solutions that align with their unique business objectives, ensuring they maximize their return on investment. The edge compute platform is an essential component of these hybrid solutions, allowing for seamless integration between edge and cloud environments. For more insights on the future of data processing with edge AI and micro data centers, check out this article on revolutionizing data processing.
4.6. Privacy and Security Protocols
As digital transformation accelerates, privacy and security protocols have become essential in protecting sensitive data. Organizations must implement robust measures to safeguard information from unauthorized access and breaches.
- Data Encryption: Encrypts data both at rest and in transit to prevent unauthorized access, ensuring that even if data is intercepted, it remains unreadable without the proper decryption keys.
- Access Control: Implements strict access controls to ensure that only authorized personnel can access sensitive information. It utilizes role-based access control (RBAC) and multi-factor authentication (MFA) to enhance security.
- Regular Audits and Compliance: Conducts regular security audits to identify vulnerabilities and ensure compliance with regulations such as GDPR and HIPAA. This establishes a framework for continuous monitoring and improvement of security practices.
- Incident Response Plans: Develops and maintains an incident response plan to address potential security breaches swiftly, ensuring that all employees are trained on the protocols to follow in case of a data breach.
- Data Anonymization: Uses techniques to anonymize data, making it difficult to trace back to individual users. This helps in complying with privacy regulations while still allowing for data analysis.
5. Implementation Strategies

Implementing cloud and edge computing solutions requires a well-defined strategy to ensure success. Organizations must consider various factors to effectively integrate these technologies into their operations.
- Assessment of Needs: Evaluate the specific needs of the organization to determine whether cloud, edge, or a hybrid approach is most suitable, considering factors such as data volume, processing speed, and application requirements.
- Choosing the Right Providers: Research and select cloud and edge service providers that align with the organization’s goals and compliance requirements. Assess factors such as reliability, scalability, and support services.
- Pilot Programs: Implement pilot programs to test the effectiveness of cloud and edge solutions before full-scale deployment. Gather feedback and make necessary adjustments based on performance and user experience.
- Training and Development: Invest in training programs for employees to ensure they are equipped to work with new technologies. Foster a culture of continuous learning to keep pace with evolving technologies.
- Monitoring and Optimization: Establish monitoring systems to track performance and identify areas for improvement. Regularly review and optimize cloud and edge computing strategies to adapt to changing business needs.
- Collaboration and Integration: Encourage collaboration between IT and business units to ensure alignment of technology with business objectives. Integrate cloud and edge solutions with existing systems to create a seamless workflow.
- Budgeting and Cost Management: Develop a clear budget for cloud and edge computing initiatives, considering both initial and ongoing costs. Monitor expenses to ensure that the organization remains within budget while maximizing the return on investment.
At Rapid Innovation, we are committed to helping our clients navigate these technologies effectively, ensuring they achieve their business goals efficiently and effectively while maximizing their ROI. The convergence of edge computing and cloud computing is reshaping the landscape, and we are here to guide you through this transformation. For more information on how we can assist you, check out our generative AI consulting services.
5.1. Healthcare System Assessment
Assessing a healthcare system is crucial for identifying strengths, weaknesses, and areas for improvement. This assessment involves a comprehensive evaluation of various components, including infrastructure, processes, and outcomes.
- Infrastructure Evaluation: Analyze the physical and technological resources available, such as hospitals, clinics, and telehealth services. Rapid Innovation can assist in integrating advanced technologies, ensuring that healthcare facilities are equipped to meet modern demands.
- Process Analysis: Examine workflows, patient care protocols, and administrative procedures to identify inefficiencies or bottlenecks. Our expertise in healthcare system assessment can streamline these processes, enhancing operational efficiency and reducing costs.
- Outcome Measurement: Evaluate patient outcomes, including recovery rates, readmission rates, and patient satisfaction scores, to gauge the effectiveness of the healthcare system. By leveraging data analytics, we can provide insights that lead to improved patient care and satisfaction.
- Stakeholder Feedback: Gather insights from healthcare professionals, patients, and administrators to understand their experiences and perceptions of the system. Rapid Innovation can facilitate this feedback loop through AI-driven surveys and analysis tools.
- Regulatory Compliance: Ensure that the healthcare system adheres to local and national regulations, including patient privacy laws and quality standards. Our blockchain solutions can enhance transparency and security, ensuring compliance with regulatory requirements.
A thorough healthcare system assessment can lead to actionable insights that drive improvements in patient care and operational efficiency. For more information on how we can assist in this area, check out our AI Healthcare Management services.
5.2. Data Preparation and Normalization
Data preparation and normalization are essential steps in the data analysis process, particularly in healthcare, where data can be complex and varied. Properly prepared data ensures that analyses yield accurate and meaningful results.
- Data Collection: Gather data from multiple sources, including electronic health records (EHRs), patient surveys, and clinical trials. Rapid Innovation can implement systems that seamlessly integrate these diverse data sources.
- Data Cleaning: Identify and rectify errors, inconsistencies, and missing values in the dataset to enhance data quality. Our AI algorithms can automate this process, significantly reducing the time and effort required.
- Normalization: Standardize data formats and scales to ensure consistency across different datasets. This may involve converting categorical data into numerical formats and scaling numerical data to a common range. Our solutions ensure that data is ready for advanced analytics.
- Feature Selection: Identify the most relevant variables that contribute to the analysis, which can improve model performance and reduce complexity. Rapid Innovation employs machine learning techniques to optimize feature selection.
- Data Transformation: Apply techniques such as aggregation or disaggregation to create a dataset that is suitable for analysis. Our expertise in data engineering ensures that the transformed data is actionable and insightful.
Effective data preparation and normalization are critical for leveraging data analytics and machine learning in healthcare, ultimately leading to better decision-making and patient outcomes.
5.3. AI Agent Training Methodologies
Training AI agents in healthcare involves using various methodologies to ensure that these systems can effectively analyze data and make informed decisions. The choice of training methodology can significantly impact the performance of AI applications.
- Supervised Learning: This method involves training AI agents on labeled datasets, where the input data is paired with the correct output. It is commonly used for tasks such as predicting patient outcomes and classifying medical images. Rapid Innovation can develop tailored models that enhance diagnostic accuracy.
- Unsupervised Learning: In this approach, AI agents learn from unlabeled data, identifying patterns and relationships without explicit guidance. Applications include clustering similar patient profiles and anomaly detection in patient data. Our solutions can uncover hidden insights that drive better patient management.
- Reinforcement Learning: This methodology involves training AI agents through trial and error, where they learn to make decisions based on feedback from their actions. It is particularly useful for optimizing treatment plans and enhancing operational efficiencies in healthcare settings. Rapid Innovation can implement reinforcement learning strategies that adapt to real-time data.
- Transfer Learning: This technique allows AI agents to leverage knowledge gained from one task to improve performance on a related task. It can be beneficial in scenarios where labeled data is scarce. Our expertise ensures that models are efficiently repurposed for various healthcare applications.
- Continuous Learning: AI agents can be designed to adapt and improve over time by continuously learning from new data and experiences, ensuring they remain effective as healthcare practices evolve. Rapid Innovation focuses on creating systems that evolve with the changing landscape of healthcare.
Implementing robust AI training methodologies is essential for developing intelligent systems that can support healthcare professionals and improve patient care. By partnering with Rapid Innovation, clients can achieve greater ROI through enhanced operational efficiencies and improved patient outcomes.
5.4. Deployment Models
Deployment models refer to the various ways in which healthcare solutions and systems can be implemented and utilized within healthcare settings. These models are crucial for ensuring that healthcare providers can effectively manage their operations, improve patient care, and streamline processes. The choice of deployment model can significantly impact the efficiency, scalability, and accessibility of healthcare services.
- On-Premises Deployment: This model involves installing software and hardware within the healthcare facility. It offers complete control over data and security but requires significant upfront investment.
- Cloud-Based Deployment: In this model, healthcare solutions are hosted on the cloud, allowing for remote access and scalability. It reduces the need for physical infrastructure and can lower operational costs.
- Hybrid Deployment: This combines both on-premises and cloud-based solutions, allowing healthcare organizations to leverage the benefits of both models. It provides flexibility and can be tailored to specific needs.
5.4.1. Hospital-Based Solutions
Hospital-based solutions are specialized systems designed to enhance the operational efficiency of hospitals. These solutions encompass a wide range of functionalities, including patient management, electronic health records (EHR), and billing systems.
- Electronic Health Records (EHR): EHR systems centralize patient information, making it easily accessible to healthcare providers. This improves coordination of care and reduces the risk of errors.
- Patient Management Systems: These systems streamline patient scheduling, admissions, and discharge processes, helping to optimize resource allocation and improve patient flow.
- Billing and Revenue Cycle Management: Hospital-based solutions often include integrated billing systems that automate the revenue cycle, ensuring timely payments and reducing administrative burdens.
- Interoperability: Modern hospital-based solutions emphasize interoperability, allowing different systems to communicate effectively. This is crucial for coordinated care and data sharing among various healthcare providers.
5.4.2. Pharmacy Management Systems
Pharmacy management systems are essential tools for managing pharmacy operations within healthcare settings. These systems help in tracking medication inventory, processing prescriptions, and ensuring compliance with regulations.
- Inventory Management: Pharmacy management systems provide real-time tracking of medication stock levels, helping to prevent shortages and overstock situations, ensuring that patients receive their medications on time.
- Prescription Processing: These systems streamline the prescription workflow, from order entry to dispensing, reducing the chances of errors and enhancing patient safety by providing alerts for potential drug interactions.
- Compliance and Reporting: Pharmacy management systems assist in maintaining compliance with regulatory requirements. They generate reports that help pharmacies adhere to laws and regulations, ensuring safe medication practices.
- Integration with EHR: Many pharmacy management systems integrate seamlessly with EHR systems, allowing for better communication between pharmacists and healthcare providers. This integration enhances medication management and improves patient outcomes.
- Patient Engagement: Some pharmacy management systems include features that promote patient engagement, such as medication reminders and refill notifications. This encourages adherence to prescribed therapies and improves overall health outcomes.
At Rapid Innovation, we leverage our expertise in AI and Blockchain to enhance these healthcare deployment models and solutions. For instance, by integrating AI algorithms into hospital-based solutions, we can provide predictive analytics that help healthcare providers anticipate patient needs and optimize resource allocation. Additionally, utilizing Blockchain technology can enhance the security and interoperability of EHR systems, ensuring that patient data is not only secure but also easily accessible across different healthcare providers. This strategic approach not only improves operational efficiency but also drives greater ROI for our clients.
5.4.3. Telehealth Platforms
Telehealth platforms have revolutionized the way healthcare is delivered, making it more accessible and efficient. These digital solutions allow patients to consult healthcare providers remotely, breaking down geographical barriers and reducing the need for in-person visits.
- Increased Accessibility: Telehealth platforms enable patients in rural or underserved areas to access medical care without the need for long travel.
- Cost-Effectiveness: Virtual consultations often reduce costs associated with traditional healthcare, such as transportation and time off work.
- Convenience: Patients can schedule appointments at their convenience, leading to higher satisfaction rates.
- Diverse Services: Telehealth platforms offer a range of services, including primary care, mental health support, and specialist consultations.
- Enhanced Patient Engagement: Many telehealth platforms include features that allow patients to track their health metrics, leading to better self-management and adherence to treatment plans.
The rise of telehealth has been significant, with a report indicating that telehealth visits increased by 154% in March 2020 compared to the previous year. This trend highlights the growing acceptance and reliance on telehealth solutions in modern healthcare.
5.5. Continuous Learning and Adaptation
Continuous learning and adaptation are crucial for healthcare providers and organizations to stay relevant and effective in a rapidly changing environment. This approach involves regularly updating knowledge, skills, and practices based on new evidence and feedback.
- Embracing Innovation: Healthcare professionals must stay informed about the latest technologies and treatment methodologies to provide the best care.
- Feedback Loops: Implementing systems for collecting patient and staff feedback can help identify areas for improvement and innovation.
- Training Programs: Regular training sessions and workshops can enhance the skills of healthcare workers, ensuring they are equipped to handle new challenges.
- Data-Driven Decisions: Utilizing data analytics can help organizations understand trends and make informed decisions about patient care and resource allocation.
- Collaborative Learning: Encouraging teamwork and knowledge sharing among healthcare professionals fosters a culture of continuous improvement.
Organizations that prioritize continuous learning are better positioned to adapt to changes in regulations, patient needs, and technological advancements, ultimately leading to improved patient outcomes.
6. Specific Use Cases
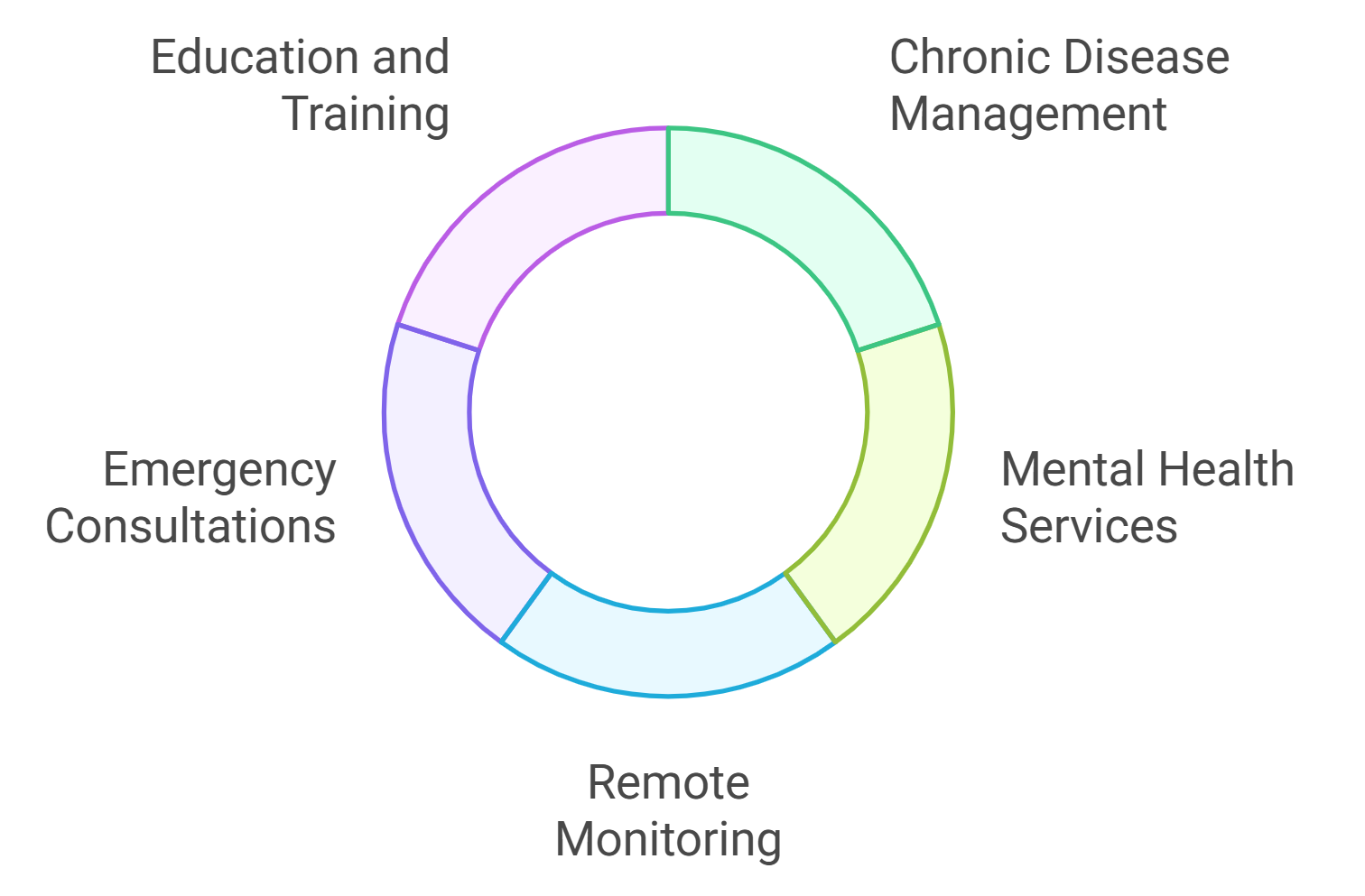
Specific use cases of telehealth and continuous learning illustrate their practical applications in real-world scenarios. These examples highlight the effectiveness of these approaches in addressing various healthcare challenges.
- Chronic Disease Management: Telehealth platforms enable patients with chronic conditions, such as diabetes or hypertension, to monitor their health remotely. Regular virtual check-ins with healthcare providers can lead to better disease management and reduced hospitalizations.
- Mental Health Services: Teletherapy has become a vital resource for individuals seeking mental health support. Telehealth platforms allow patients to connect with therapists from the comfort of their homes, increasing access to care and reducing stigma.
- Remote Monitoring: Wearable devices and mobile health applications facilitate continuous monitoring of patients' vital signs. This data can be shared with healthcare providers, allowing for timely interventions and personalized care plans.
- Emergency Consultations: In urgent situations, telehealth can provide immediate access to medical advice, helping patients determine whether they need to seek in-person care.
- Education and Training: Continuous learning initiatives, such as online courses and webinars, help healthcare professionals stay updated on best practices and emerging trends, ultimately enhancing patient care.
These specific use cases demonstrate the transformative potential of telehealth and continuous learning in improving healthcare delivery and patient outcomes.
At Rapid Innovation, we leverage AI and Blockchain technologies to enhance telehealth platforms, ensuring secure data management, improved patient engagement, and streamlined operations. By integrating advanced analytics and machine learning, we help healthcare organizations optimize their telehealth services, including AI EdTech solutions, telemedicine software providers and HIPAA compliant telemedicine software, leading to greater ROI and improved patient outcomes. Our expertise in these domains positions us as a valuable partner in your journey towards innovative healthcare solutions, including white label telemedicine and telehealth platforms for providers.
6.1. Hospital Medication Management
Hospital medication management is a critical component of patient care that ensures the safe and effective use of medications within a healthcare facility. This process involves several key elements:
- Medication Reconciliation: This is the process of comparing a patient's medication orders to all of the medications that the patient has been taking. It helps to identify discrepancies and prevent medication errors.
- Formulary Management: Hospitals maintain a formulary, which is a list of approved medications. This helps streamline medication use and ensures that only safe and effective drugs are available.
- Clinical Pharmacists: These healthcare professionals play a vital role in medication management by reviewing medication orders, providing drug information, and collaborating with physicians to optimize therapy.
- Patient Education: Educating patients about their medications, including how to take them and potential side effects, is essential for adherence and safety.
- Monitoring and Reporting: Continuous monitoring of medication effects and side effects is crucial. Hospitals often have systems in place to report adverse drug reactions and medication errors.
At Rapid Innovation, we leverage AI-driven analytics to enhance medication reconciliation processes, ensuring that discrepancies are identified swiftly and accurately. Our blockchain solutions can also facilitate secure and transparent formulary management, allowing hospitals to maintain an up-to-date list of approved medications while ensuring compliance and safety. Effective hospital medication management can significantly reduce the risk of medication errors, improve patient outcomes, and enhance overall healthcare quality.
6.2. Chronic Disease Medication Monitoring
Chronic disease medication monitoring is essential for patients with long-term health conditions such as diabetes, hypertension, and asthma. This process ensures that medications are taken correctly and effectively manage the disease. Key aspects include:
- Regular Follow-ups: Patients with chronic diseases require regular check-ups to assess their medication effectiveness and make necessary adjustments.
- Adherence Monitoring: Tools such as pill organizers, medication reminders, and digital apps can help patients adhere to their medication regimens.
- Side Effect Management: Monitoring for side effects is crucial, as chronic medications can lead to adverse reactions. Patients should be educated on what to watch for and when to seek help.
- Lab Tests and Assessments: Regular lab tests may be necessary to monitor the effects of medications, especially for conditions like diabetes and hypertension.
- Interdisciplinary Collaboration: Healthcare providers, including doctors, nurses, and pharmacists, must work together to ensure comprehensive care and medication management.
Rapid Innovation can assist healthcare providers by implementing AI solutions that analyze patient data to predict medication adherence and optimize treatment plans. Our blockchain technology can also ensure secure sharing of patient information among interdisciplinary teams, enhancing collaboration and improving patient outcomes. By focusing on chronic disease medication monitoring, healthcare providers can improve patient quality of life and reduce hospitalizations related to medication issues.
6.3. Pediatric Medication Safety
Pediatric medication safety is a vital concern in healthcare, as children are particularly vulnerable to medication errors. Ensuring the safe administration of medications to children involves several strategies:
- Weight-Based Dosing: Medications for children are often dosed based on their weight. Accurate weight measurement is essential to prevent overdosing or underdosing.
- Clear Labeling: Medication labels should be clear and easy to understand, using age-appropriate language and units of measurement.
- Parental Education: Parents and caregivers should be educated about the medications their children are taking, including dosages, administration routes, and potential side effects.
- Use of Technology: Electronic prescribing and medication administration systems can help reduce errors by providing alerts for potential drug interactions and allergies.
- Monitoring for Adverse Effects: Close monitoring for side effects is crucial, as children may react differently to medications than adults.
- Involvement of Pediatric Specialists: Pediatricians and pharmacists specializing in children's health can provide valuable insights into safe medication practices.
At Rapid Innovation, we can enhance pediatric medication safety through AI-powered tools that assist in weight-based dosing calculations and provide real-time alerts for potential medication errors. Our blockchain solutions can also ensure that medication records are securely maintained and easily accessible to healthcare providers, minimizing risks and ensuring that children receive the best possible care. By prioritizing pediatric medication safety, healthcare providers can minimize risks and ensure that children receive the best possible care.
6.4. Geriatric Medication Management
Geriatric medication management is a critical aspect of healthcare for older adults, who often have multiple chronic conditions and take several medications simultaneously. This complexity increases the risk of adverse drug reactions, medication errors, and non-adherence. Effective management strategies are essential to ensure the safety and efficacy of medications in this population.
- Polypharmacy is common among older adults, with many taking five or more medications.
- Regular medication reviews can help identify unnecessary medications and potential drug interactions.
- Healthcare providers should consider the patient's overall health, including kidney and liver function, when prescribing medications.
- Education on the importance of adherence and understanding medication regimens is vital for older patients and their caregivers.
- Utilizing tools like medication reconciliation can help track changes and ensure accuracy in prescriptions.
At Rapid Innovation, we leverage AI-driven analytics to enhance geriatric medication management. By utilizing machine learning algorithms, we can analyze patient data to identify patterns and predict potential adverse drug interactions, ultimately improving patient safety and outcomes.
6.5. Mental Health Medication Tracking
Mental health medication tracking is essential for individuals managing mental health disorders. Proper tracking can enhance treatment outcomes, minimize side effects, and improve overall quality of life. Mental health medications often require careful monitoring due to their complex nature and potential side effects.
- Regular follow-ups with healthcare providers can help assess the effectiveness of medications and make necessary adjustments.
- Patients should maintain a medication diary to track dosages, side effects, and any changes in mood or behavior.
- Digital tools and apps can assist in medication reminders and tracking, making it easier for patients to adhere to their treatment plans.
- Collaboration between mental health professionals and primary care providers is crucial for comprehensive care.
- Educating patients about their medications, including potential side effects and interactions, empowers them to take an active role in their treatment.
Rapid Innovation can develop customized digital solutions that integrate AI to facilitate mental health medication tracking. Our platforms can provide real-time insights and reminders, ensuring patients remain compliant with their treatment plans, thereby enhancing overall mental health outcomes.
6.6. Oncology Medication Precision
Oncology medication precision refers to the tailored approach in cancer treatment, focusing on the specific characteristics of an individual's cancer. This personalized medicine strategy aims to improve treatment efficacy and minimize side effects by selecting the most appropriate therapies based on genetic, biomarker, and other relevant factors.
- Precision oncology utilizes genetic testing to identify mutations that drive cancer growth, allowing for targeted therapies.
- This approach can lead to better outcomes, as treatments are designed to attack specific cancer cells while sparing healthy cells.
- Ongoing research is crucial for developing new targeted therapies and understanding resistance mechanisms.
- Multidisciplinary teams, including oncologists, pharmacists, and genetic counselors, play a vital role in implementing precision medicine.
- Patient education about the benefits and risks of precision oncology is essential for informed decision-making.
At Rapid Innovation, we harness blockchain technology to ensure the secure sharing of genomic data among healthcare providers. This facilitates a more collaborative approach to precision oncology, allowing for better-informed treatment decisions and ultimately improving patient outcomes. By integrating AI and blockchain, we help healthcare organizations achieve greater ROI through enhanced efficiency and effectiveness in medication management.
7. Advanced AI Agent Capabilities
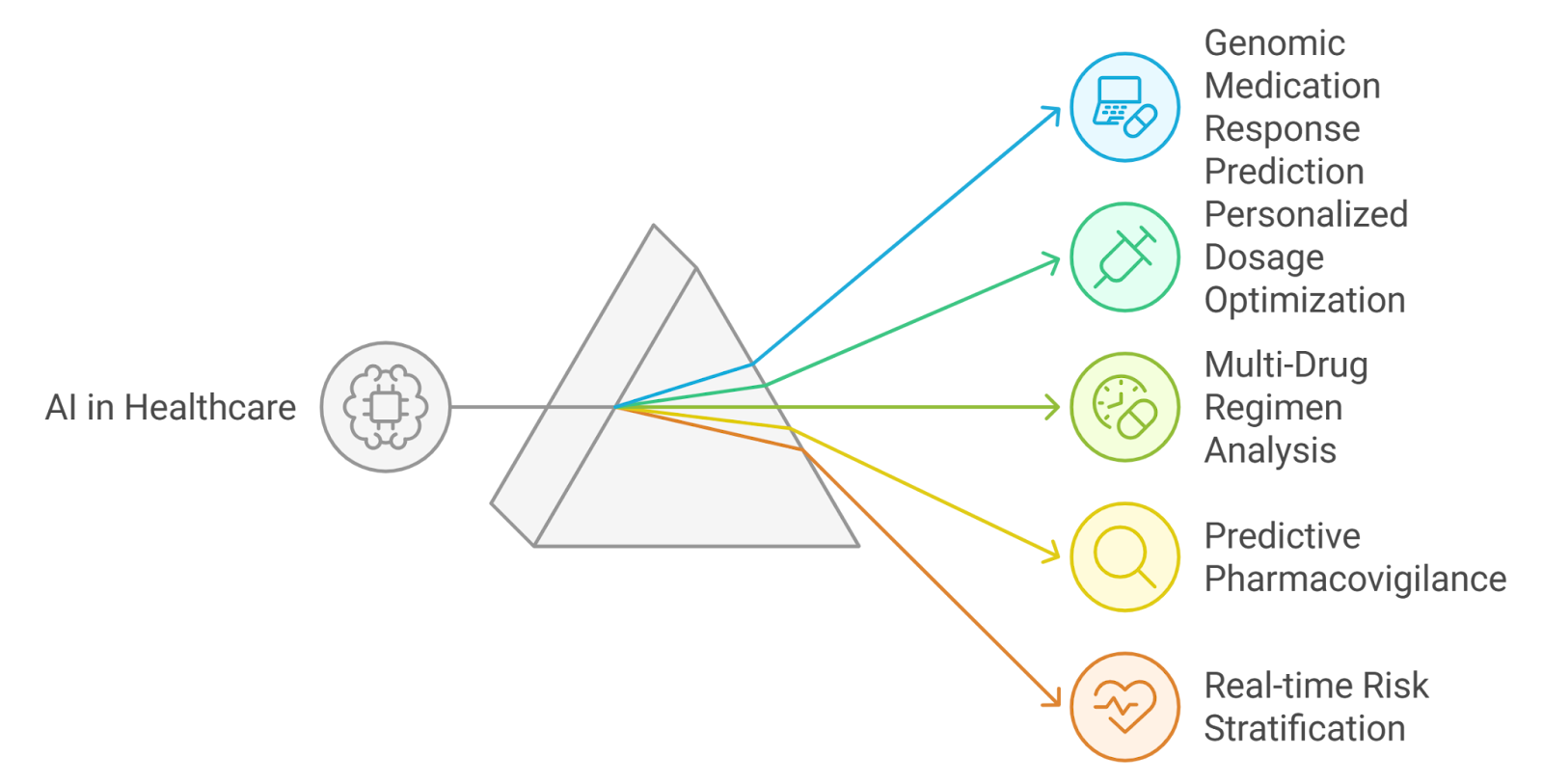
The integration of advanced AI agent capabilities in healthcare is revolutionizing the way we approach treatment and medication. These technologies, including artificial intelligence in healthcare, are enhancing precision medicine, leading to better patient outcomes and more efficient healthcare systems.
7.1 Genomic Medication Response Prediction
Genomic medication response prediction involves using AI in healthcare to analyze genetic information to forecast how patients will respond to specific medications. This capability is crucial for tailoring treatments to individual patients, minimizing adverse effects, and maximizing therapeutic efficacy. AI algorithms can process vast amounts of genomic data, identifying patterns that predict drug responses. By analyzing single nucleotide polymorphisms (SNPs) and other genetic markers, AI can help determine the likelihood of a patient experiencing side effects or achieving desired outcomes with a particular drug. This predictive capability can significantly reduce trial-and-error prescribing, leading to faster and more effective treatment plans.
At Rapid Innovation, we harness these advanced AI capabilities to assist healthcare providers in developing customized solutions that enhance patient care. By implementing our AI-driven genomic analysis tools, clients can expect to see improved drug efficacy and reduced adverse reactions, ultimately leading to a greater return on investment (ROI) through enhanced patient satisfaction and loyalty.
7.2 Personalized Dosage Optimization
Personalized dosage optimization is another advanced AI capability that focuses on determining the most effective dosage of medication for individual patients. This process takes into account various factors, including genetics, age, weight, and overall health. AI systems can analyze patient data to recommend dosages that maximize therapeutic effects while minimizing side effects. Machine learning models can continuously learn from new patient data, refining dosage recommendations over time. This approach can lead to more accurate dosing, particularly for medications with narrow therapeutic windows, where the difference between an effective dose and a toxic dose is small.
At Rapid Innovation, we empower healthcare organizations to implement personalized dosage optimization strategies that can significantly improve patient outcomes. For instance, our AI solutions have been shown to reduce the risk of complications in anticoagulant therapy by up to 40%. By facilitating real-time monitoring of patient responses, our clients can make timely adjustments to dosages, ensuring optimal treatment for chronic conditions and enhancing overall healthcare efficiency.
In conclusion, the advanced AI agent capabilities in genomic medication response prediction and personalized dosage optimization are transforming healthcare. By leveraging these technologies, including medical AI and artificial intelligence in medical diagnosis, healthcare providers can offer more tailored and effective treatment options, ultimately improving patient care and outcomes. Rapid Innovation is committed to helping clients achieve these advancements, ensuring they realize greater ROI through innovative AI solutions in the medical field, exploring the future of AI-powered healthcare solutions.
7.3. Multi-Drug Regimen Analysis
Multi-drug regimen analysis is a critical aspect of pharmacology and clinical practice, particularly in the treatment of complex diseases such as HIV, tuberculosis, and cancer. This analysis involves evaluating the effectiveness, safety, and interactions of multiple medications prescribed simultaneously.
- Understanding drug interactions is essential to avoid adverse effects.
- Analyzing the pharmacokinetics and pharmacodynamics of each drug helps in optimizing therapy.
- Patient adherence to multi-drug regimens can be challenging, necessitating strategies to improve compliance.
- Utilizing software tools and databases can aid healthcare professionals in assessing potential drug-drug interactions.
- Clinical trials often focus on multidrug regimen analysis to establish efficacy and safety profiles.
At Rapid Innovation, we leverage advanced AI algorithms to analyze vast datasets, enabling healthcare providers to make informed decisions about multi-drug therapies. By implementing our solutions, clients can enhance patient outcomes and reduce the risk of treatment failures, ultimately leading to greater ROI.
Research indicates that approximately 50% of patients do not adhere to their prescribed multi-drug regimens, which can lead to treatment failure and increased healthcare costs.
7.4. Predictive Pharmacovigilance
Predictive pharmacovigilance is an emerging field that leverages data analytics and machine learning to anticipate adverse drug reactions (ADRs) before they occur. This proactive approach enhances patient safety and improves drug development processes.
- Data mining from electronic health records (EHRs) can identify patterns of ADRs.
- Machine learning algorithms can predict potential side effects based on patient demographics and genetic information.
- Real-time monitoring of drug safety signals allows for timely interventions.
- Collaboration between regulatory agencies and pharmaceutical companies is crucial for effective predictive pharmacovigilance.
- The integration of social media data can provide insights into patient experiences and ADR reporting.
At Rapid Innovation, our expertise in AI and data analytics empowers clients to implement predictive pharmacovigilance strategies that can significantly reduce the incidence of serious ADRs. This not only enhances patient safety but also leads to substantial cost savings in drug development and healthcare management.
Studies show that predictive models can significantly reduce the incidence of serious ADRs, potentially saving billions in healthcare costs.
7.5. Real-time Risk Stratification
Real-time risk stratification is a vital process in clinical decision-making, allowing healthcare providers to assess the risk levels of patients based on various factors. This approach is particularly useful in managing chronic diseases and during drug therapy.
- Utilizing algorithms that analyze patient data can help identify high-risk individuals.
- Factors such as age, comorbidities, and medication history are considered in risk assessments.
- Real-time data collection from wearable devices can enhance monitoring and risk evaluation.
- Risk stratification aids in personalizing treatment plans, improving outcomes.
- Continuous updates to risk models ensure they remain relevant and accurate.
Rapid Innovation's AI-driven solutions facilitate real-time risk stratification, enabling healthcare providers to tailor treatment plans effectively. By identifying high-risk patients early, our clients can improve patient outcomes and reduce hospital readmissions, leading to a more efficient healthcare system.
Research indicates that effective risk stratification can lead to a 30% reduction in hospital readmissions for high-risk patients.
8. Challenges and Limitations
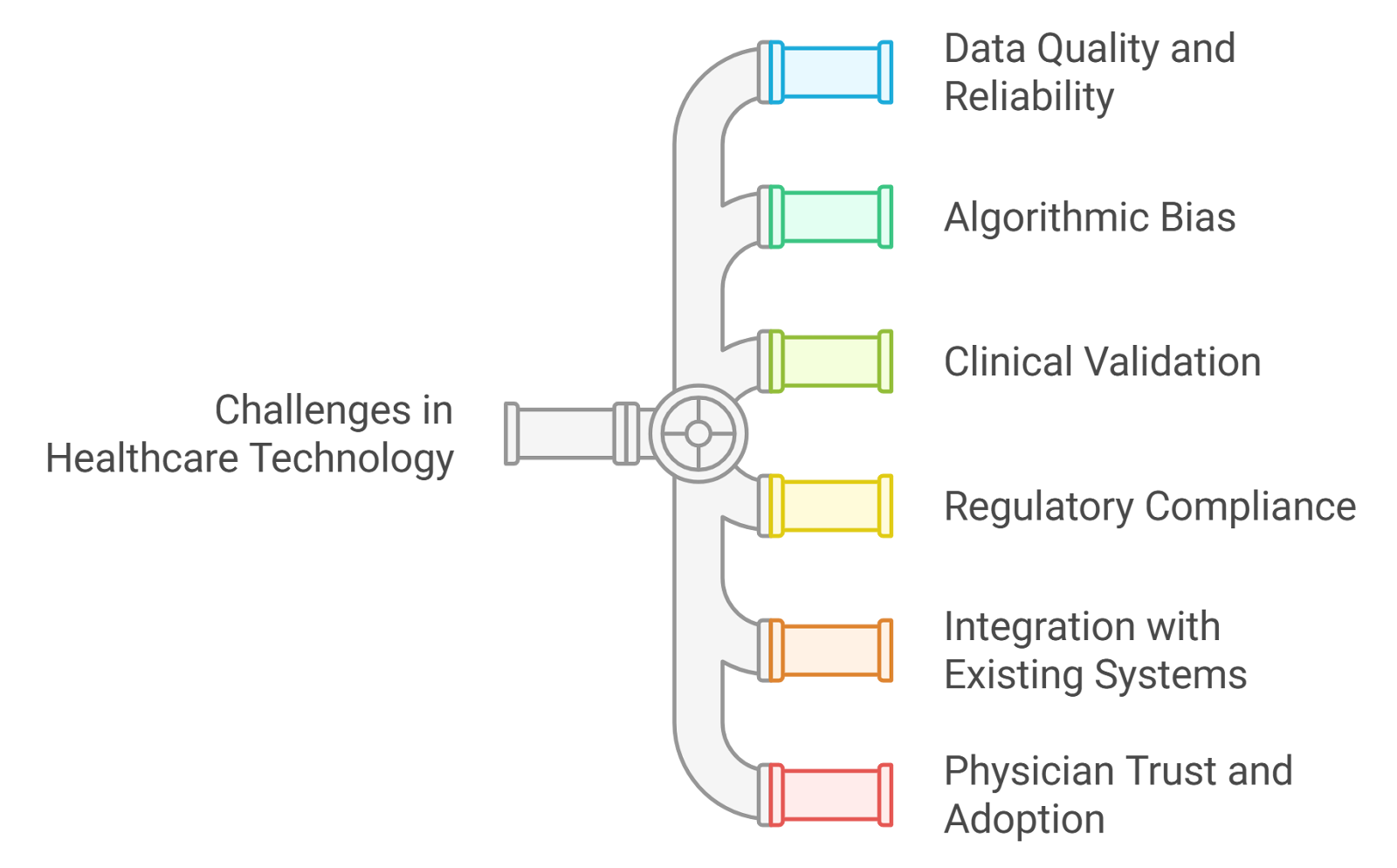
The integration of technology in various sectors has brought about significant advancements, but it also presents several challenges and limitations that need to be addressed. Two critical areas of concern are data accuracy and reliability, as well as algorithmic bias.
8.1 Data Quality and Reliability
Data quality and reliability are paramount for the success of any data-driven initiative. Poor data quality can lead to inaccurate insights, misguided decisions, and ultimately, failure of projects. Issues related to data quality include:
- Inaccurate Data: Data can be flawed due to human error, outdated information, or incorrect data entry, which can skew results and lead to erroneous conclusions.
- Inconsistent Data Sources: When data is collected from multiple sources, inconsistencies can arise. Different formats, units, and definitions can complicate data integration and analysis.
- Data Completeness: Missing data can significantly impact the reliability of analyses. Incomplete datasets can lead to biased results and limit the ability to draw meaningful conclusions.
- Timeliness: Data must be current to be relevant. Stale data can misrepresent the current state of affairs, leading to decisions based on outdated information.
- Data Security: Ensuring the integrity and security of data is crucial. Breaches can compromise data quality and lead to a loss of trust among stakeholders.
Addressing these issues requires robust data governance frameworks, regular audits, and the implementation of best practices in data management. Organizations must invest in training and tools to enhance data quality reliability. At Rapid Innovation, we assist clients in establishing comprehensive data management strategies that ensure high-quality data, ultimately leading to improved decision-making and greater ROI. The trustworthiness of data and data quality refers to the confidence stakeholders have in the data being used, which is essential for effective decision-making.
8.2 Algorithmic Bias
Algorithmic bias refers to the systematic and unfair discrimination that can occur in automated decision-making processes. This bias can arise from various sources and can have significant implications for individuals and society. Key factors contributing to algorithmic bias include:
- Training Data Bias: Algorithms learn from historical data. If this data reflects existing prejudices or inequalities, the algorithm may perpetuate or even exacerbate these biases. For example, if a hiring algorithm is trained on data from a company with a history of gender bias, it may favor male candidates over equally qualified female candidates.
- Feature Selection: The choice of features used in an algorithm can introduce bias. If certain demographic factors are included or excluded without careful consideration, it can lead to skewed outcomes.
- Lack of Diversity in Development Teams: Homogeneous teams may overlook potential biases in algorithms. Diverse teams are more likely to identify and mitigate biases during the development process.
- Transparency and Accountability: Many algorithms operate as "black boxes," making it difficult to understand how decisions are made. This lack of transparency can hinder accountability and make it challenging to identify and correct biases.
- Impact on Marginalized Groups: Algorithmic bias can disproportionately affect marginalized communities, leading to unfair treatment in areas such as hiring, lending, and law enforcement.
To combat algorithmic bias, organizations should prioritize fairness and inclusivity in their data practices. This includes conducting regular bias audits, using diverse datasets, and fostering diverse teams to ensure a broader perspective in algorithm development. Additionally, promoting transparency in algorithms can help build trust and accountability. At Rapid Innovation, we guide our clients in implementing ethical AI practices, ensuring that their algorithms are fair and unbiased, which not only enhances their reputation but also drives better business outcomes. Reliability in data quality is essential to mitigate these biases and ensure equitable outcomes.
8.3. Clinical Validation
Clinical validation is a critical process in the healthcare sector, ensuring that medical devices, software, and diagnostic tools perform accurately and effectively in real-world settings. This process involves rigorous testing and evaluation to confirm that a product meets its intended use and provides reliable results. It ensures the safety and efficacy of medical products, involves clinical trials and studies to gather data on performance, requires collaboration with healthcare professionals for real-world insights, must adhere to established protocols and guidelines set by regulatory bodies, and helps in building trust among healthcare providers and patients.
At Rapid Innovation, we leverage advanced AI algorithms to enhance the clinical validation healthcare process. By utilizing machine learning models, we can analyze vast datasets to identify patterns and predict outcomes, thereby streamlining the validation process. This not only accelerates time-to-market but also ensures that healthcare providers can rely on data-driven insights for better patient care.
Clinical validation is not just about meeting regulatory requirements; it also plays a vital role in improving patient outcomes. By confirming that a product works as intended, healthcare providers can make informed decisions based on reliable data. This process often includes pre-market clinical studies to assess performance, post-market surveillance to monitor long-term effectiveness, and continuous feedback loops to refine and improve products. Additionally, our expertise in AI copilot development can further enhance the clinical validation process by providing intelligent support and insights.
8.4. Regulatory Compliance
Regulatory compliance is essential for any medical product or service to ensure it meets the legal and safety standards set by governing bodies. Compliance varies by region but generally includes adherence to guidelines established by organizations such as the FDA in the United States or the EMA in Europe. It involves understanding and following local and international regulations, requires documentation of processes, testing, and results, includes regular audits and inspections by regulatory authorities, ensures that products are safe for public use and meet quality standards, and helps avoid legal issues and potential recalls.
Achieving regulatory compliance can be a complex process, often requiring comprehensive risk assessments to identify potential hazards, detailed reporting and documentation to demonstrate compliance, and ongoing training for staff to stay updated on regulatory changes. Non-compliance can lead to severe consequences, including fines, product recalls, and damage to reputation. Therefore, organizations must prioritize regulatory compliance as part of their operational strategy.
8.5. Integration with Existing Systems
Integration with existing systems is crucial for the successful implementation of new healthcare technologies. This process involves ensuring that new tools and software can seamlessly work with current systems, such as electronic health records (EHRs), laboratory information systems (LIS), and other healthcare IT solutions. It enhances workflow efficiency by reducing data silos, facilitates better communication between different departments, improves data accuracy and reduces the risk of errors, supports interoperability, allowing different systems to exchange information, and enables healthcare providers to access comprehensive patient data quickly.
At Rapid Innovation, we specialize in developing blockchain solutions that ensure secure and transparent data exchange between systems. By implementing blockchain technology, we can enhance data integrity and traceability, which is essential for maintaining compliance and improving patient care. Successful integration requires careful planning and execution, including conducting a thorough assessment of existing systems to identify compatibility, engaging stakeholders from various departments to gather input and address concerns, providing adequate training for staff to adapt to new technologies, and implementing robust data security measures to protect sensitive information. By focusing on integration, healthcare organizations can maximize the benefits of new technologies, ultimately leading to improved patient care and operational efficiency.
8.6. Physician Trust and Adoption
Physician trust is a critical factor in the successful adoption of new technologies and practices in healthcare. When physicians trust a system, they are more likely to embrace it, leading to improved patient outcomes and operational efficiency. Trust is built through transparency, reliability, and consistent performance of the technology. Physicians are more inclined to adopt technologies that have been validated through clinical studies and peer-reviewed research. Training and support play a significant role in fostering trust; when physicians feel confident in their ability to use a new system, they are more likely to adopt it. Peer influence is also significant; recommendations from colleagues can enhance trust and encourage adoption. Continuous feedback mechanisms can help address concerns and improve the technology, further solidifying trust among physicians.
The relationship between physician trust and technology adoption is crucial for the integration of electronic health records (EHRs), telemedicine, and other digital health solutions. A study indicated that 70% of physicians reported that trust in technology significantly influenced their willingness to adopt new systems. At Rapid Innovation, we leverage AI-driven solutions to enhance the transparency and reliability of healthcare technologies, ensuring that physicians can trust the systems they use.
9. Economic and Strategic Implications
The economic and strategic implications of healthcare technologies are profound. As healthcare systems increasingly adopt digital solutions, understanding these implications becomes essential for stakeholders.
- Cost savings can be realized through improved operational efficiencies and reduced administrative burdens.
- Enhanced patient care can lead to better health outcomes, which can reduce long-term costs associated with chronic disease management.
- Strategic partnerships with technology providers can lead to innovative solutions that address specific healthcare challenges.
- Investment in technology can improve patient engagement, leading to higher satisfaction and retention rates.
- Data analytics can provide insights that drive better decision-making and resource allocation.
The economic impact of adopting new technologies can be significant, with some estimates suggesting that healthcare organizations can save up to 30% in operational costs through effective technology integration. Rapid Innovation specializes in developing tailored AI and blockchain solutions that not only streamline operations but also enhance the overall efficiency of healthcare delivery.
9.1. Cost Reduction Strategies
Cost reduction strategies in healthcare are essential for maintaining financial viability while improving patient care. Implementing effective strategies can lead to substantial savings and better resource management.
- Streamlining administrative processes through automation can reduce labor costs and minimize errors.
- Utilizing telehealth services can lower overhead costs associated with in-person visits and expand access to care.
- Implementing preventive care programs can reduce the incidence of costly emergency interventions.
- Bulk purchasing agreements for medical supplies can lead to significant savings.
- Leveraging data analytics to identify inefficiencies can help organizations optimize their operations and reduce waste.
By focusing on these cost reduction strategies, healthcare organizations can not only improve their financial health but also enhance the quality of care provided to patients. Rapid Innovation is committed to helping healthcare providers implement these strategies through our advanced AI and blockchain solutions, ensuring a greater return on investment and improved patient outcomes.
9.2. Healthcare Resource Optimization
Healthcare resource optimization refers to the strategic management of healthcare resources to improve efficiency, reduce waste, and enhance patient outcomes. This involves analyzing and reallocating resources such as staff, equipment, and facilities to ensure they are used effectively.
- Efficient scheduling of healthcare staff can lead to better patient care and reduced overtime costs. Rapid Innovation leverages AI algorithms to optimize staff schedules, ensuring that healthcare providers are available when needed most, thus enhancing patient care and reducing operational costs.
- Implementing telemedicine can optimize the use of healthcare facilities and reduce patient wait times. Our blockchain solutions can secure patient data during telemedicine consultations, ensuring compliance and trust in virtual healthcare services.
- Utilizing data analytics helps identify patterns in patient care, allowing for better resource allocation. Rapid Innovation employs advanced AI analytics to provide actionable insights, enabling healthcare organizations to allocate resources more effectively based on real-time data.
- Streamlining supply chain management can minimize excess inventory and reduce costs associated with storage and waste. Our blockchain technology can enhance transparency and traceability in the supply chain, leading to more efficient inventory management.
- Integrating technology, such as electronic health records (EHR), can improve communication among healthcare providers and enhance patient management. Rapid Innovation specializes in developing EHR systems that are not only user-friendly but also secure, ensuring that patient information is accessible yet protected.
By focusing on these strategies, healthcare organizations can maximize their resources, leading to improved service delivery and patient satisfaction.
9.3. Potential Cost Savings
Cost savings in healthcare can be achieved through various strategies aimed at reducing unnecessary expenditures while maintaining or improving the quality of care. Identifying areas for cost reduction is crucial for the sustainability of healthcare systems.
- Preventive care initiatives can reduce the incidence of chronic diseases, leading to lower treatment costs over time. Our AI-driven predictive analytics can identify at-risk patients, allowing for timely interventions that prevent costly health issues.
- Bulk purchasing of medical supplies can lead to significant discounts and lower overall expenses. Rapid Innovation can assist healthcare organizations in negotiating better terms through data-driven insights into purchasing patterns.
- Reducing hospital readmissions through better discharge planning and follow-up care can save healthcare systems substantial amounts. Our AI solutions can automate follow-up reminders and care plans, ensuring patients receive the necessary post-discharge support.
- Implementing value-based care models encourages providers to focus on patient outcomes rather than the volume of services, potentially lowering costs. Rapid Innovation can help design and implement these models using data analytics to track and improve patient outcomes.
- Utilizing generic medications instead of brand-name drugs can lead to significant savings for both patients and healthcare providers. Our consulting services can guide organizations in formulating strategies to promote the use of generics effectively.
By focusing on these potential cost savings, healthcare organizations can allocate funds more effectively, ultimately benefiting both patients and the system as a whole.
9.4. Return on Investment Analysis
Return on Investment (ROI) analysis in healthcare is a critical tool for evaluating the financial performance of healthcare initiatives and investments. It helps organizations determine the value generated from their expenditures and make informed decisions about future investments.
- Calculating ROI involves comparing the net benefits of an investment to its costs, providing a clear picture of financial performance. Rapid Innovation employs sophisticated AI models to accurately forecast ROI for various healthcare initiatives.
- Investments in technology, such as EHR systems, can lead to improved efficiency and reduced administrative costs, resulting in a positive ROI. Our custom EHR solutions are designed to streamline workflows, thereby enhancing productivity and financial returns.
- Training programs for staff can enhance skills and productivity, leading to better patient care and increased revenue. We offer tailored training solutions that leverage AI to ensure staff are equipped with the latest skills and knowledge.
- Analyzing patient outcomes and satisfaction can provide insights into the effectiveness of programs, helping to justify expenditures. Our data analytics services can track and report on these metrics, providing a clear justification for investments made.
- Regularly conducting ROI analyses allows healthcare organizations to adapt and refine their strategies based on financial performance. Rapid Innovation provides ongoing support in ROI analysis, ensuring that healthcare organizations can continuously improve their financial health.
By conducting thorough ROI analyses, healthcare organizations can ensure that their investments align with their financial goals and contribute to overall organizational success.
9.5. Insurance and Liability Considerations
Insurance and liability considerations are critical components in the healthcare landscape, particularly as the industry evolves with new technologies and practices. Understanding these aspects can help healthcare providers mitigate risks and ensure compliance.
- Types of Insurance: Healthcare providers typically require various types of insurance, including:
- Professional liability insurance (malpractice insurance)
- Healthcare liability
- General liability insurance
- Cyber liability insurance, especially with the rise of electronic health records (EHRs)
- Risk Management: Effective risk management strategies can help reduce liability exposure. This includes:
- Regular training for staff on compliance and best practices
- Implementing robust patient safety protocols
- Conducting regular audits and assessments of practices
- Legal Considerations: Healthcare providers must navigate complex legal frameworks, including:
- State and federal regulations
- Patient consent laws
- Data protection laws, such as HIPAA in the United States
- Emerging Technologies: The integration of new technologies, such as telemedicine and AI, raises unique liability concerns. Providers must consider:
- The accuracy and reliability of AI-driven diagnostics
- The potential for misdiagnosis or treatment errors
- The implications of remote consultations on patient care
- Insurance Coverage Gaps: As healthcare evolves, providers may face gaps in coverage. It is essential to:
- Review insurance policies regularly
- Consult with insurance experts to ensure adequate coverage for new services, including professional liability for healthcare workers and allied health professional liability
- Litigation Trends: Understanding current litigation trends can help providers prepare for potential legal challenges. Notable trends include:
- Increased lawsuits related to telehealth services
- Growing scrutiny of AI applications in clinical settings
- Medical liability for health care organizations and providers
10. Emerging Trends and Future Outlook
The healthcare industry is continuously evolving, driven by technological advancements, changing patient expectations, and regulatory shifts. Emerging trends are shaping the future of healthcare delivery and patient care.
- Telehealth Expansion: The COVID-19 pandemic accelerated the adoption of telehealth services. Key points include:
- Increased patient acceptance of virtual consultations
- Expansion of telehealth services across various specialties
- Ongoing regulatory changes to support telehealth reimbursement
- Patient-Centric Care: There is a growing emphasis on patient-centered care, which focuses on:
- Personalized treatment plans
- Enhanced patient engagement and communication
- Integration of patient feedback into care processes
- Value-Based Care: The shift from fee-for-service to value-based care models is gaining traction. This approach emphasizes:
- Quality of care over quantity
- Improved patient outcomes
- Cost-effectiveness in treatment options
- Data Analytics and Big Data: The use of data analytics is transforming healthcare decision-making. Key benefits include:
- Enhanced patient outcomes through predictive analytics
- Improved operational efficiency
- Better resource allocation based on data insights
- Sustainability in Healthcare: There is an increasing focus on sustainability practices within healthcare organizations. This includes:
- Reducing waste and energy consumption
- Implementing eco-friendly practices in facilities
- Promoting sustainable supply chain management
10.1. AI and Precision Medicine
Artificial Intelligence (AI) is revolutionizing precision medicine, enabling more tailored and effective treatment options for patients. This intersection of technology and healthcare is paving the way for significant advancements.
- Personalized Treatment Plans: AI algorithms analyze vast amounts of data to create individualized treatment plans based on:
- Genetic information
- Patient history
- Lifestyle factors
- Predictive Analytics: AI enhances predictive analytics, allowing healthcare providers to:
- Identify at-risk patients before symptoms arise
- Tailor preventive measures based on individual risk profiles
- Improve early diagnosis and treatment outcomes
- Drug Development: AI is streamlining the drug discovery process by:
- Analyzing biological data to identify potential drug candidates
- Reducing the time and cost associated with clinical trials
- Enhancing the precision of drug targeting
- Clinical Decision Support: AI-powered clinical decision support systems assist healthcare professionals by:
- Providing evidence-based recommendations
- Reducing diagnostic errors
- Enhancing the overall quality of care
- Ethical Considerations: The integration of AI in precision medicine raises ethical questions, including:
- Data privacy concerns
- The potential for bias in AI algorithms
- The need for transparency in AI decision-making processes
- Future Outlook: The future of AI in precision medicine looks promising, with potential developments such as:
- Greater integration of AI tools in everyday clinical practice
- Continued advancements in genomics and personalized therapies
- Enhanced collaboration between technology companies and healthcare providers
By understanding these insurance, liability, and emerging trends, healthcare providers can better navigate the complexities of the industry and leverage new technologies to improve patient care. At Rapid Innovation, we specialize in harnessing AI and blockchain technologies to help healthcare providers enhance their operational efficiency, mitigate risks, and ultimately achieve greater ROI through innovative solutions tailored to their specific needs, including addressing united healthcare third party liability and healthcare insurance liability. For more information on AI agents for treatment recommendation.
10.2. Quantum Computing in Pharmacology
Quantum computing is revolutionizing the field of pharmacology by enabling researchers to simulate molecular interactions at unprecedented speeds and accuracy. This technology leverages the principles of quantum mechanics to process vast amounts of data, which is particularly beneficial in drug discovery and development.
- Accelerated Drug Discovery: Quantum computers can analyze complex biological systems and predict how different compounds will interact with specific targets. This capability can significantly reduce the time required for drug discovery, which traditionally takes years. At Rapid Innovation, we harness quantum computing pharmacology to streamline the drug discovery process for our clients, enabling them to bring new therapies to market faster and more efficiently.
- Enhanced Molecular Simulations: Quantum computing allows for more accurate simulations of molecular behavior, leading to better predictions of drug efficacy and safety. This can help in identifying potential side effects early in the development process. Our expertise in quantum algorithms ensures that clients can leverage these simulations to make informed decisions throughout the drug development lifecycle.
- Optimized Drug Design: By utilizing quantum algorithms, researchers can design drugs that are more effective and have fewer side effects. This precision in drug design can lead to personalized medicine approaches tailored to individual patient needs. Rapid Innovation collaborates with pharmaceutical companies to implement these advanced design techniques, ultimately enhancing patient outcomes and maximizing ROI.
The potential of quantum computing in pharmacology is still being explored, but its implications for the future of drug development are promising. For more information on how we can assist with blockchain solutions in this area, visit our Blockchain as a Service page.
10.3. Blockchain for Medication Tracking
Blockchain technology is emerging as a powerful tool for enhancing the transparency and security of medication tracking. By providing a decentralized and immutable ledger, blockchain can help ensure the integrity of pharmaceutical supply chains.
- Improved Traceability: Blockchain allows for real-time tracking of medications from manufacturers to end-users. This traceability helps in identifying counterfeit drugs and ensuring that patients receive authentic medications. Rapid Innovation implements blockchain solutions that empower clients to maintain high standards of safety and compliance.
- Enhanced Data Security: The decentralized nature of blockchain means that data is less vulnerable to tampering. This is crucial for maintaining the integrity of sensitive health information related to medications. Our blockchain expertise ensures that clients can safeguard their data while enhancing trust among stakeholders.
- Streamlined Supply Chain Management: By using smart contracts, blockchain can automate various processes in the supply chain, reducing delays and improving efficiency. This can lead to cost savings and better resource allocation. Rapid Innovation's blockchain solutions help clients optimize their supply chain operations, resulting in improved profitability and operational efficiency.
The integration of blockchain in medication tracking not only enhances safety but also builds trust among stakeholders in the healthcare system.
10.4. Global Health Monitoring Networks
Global health monitoring networks are essential for tracking health trends, disease outbreaks, and the overall health status of populations. These networks utilize advanced technologies and data analytics to provide timely and accurate information.
- Real-Time Data Collection: Health monitoring networks can gather data from various sources, including hospitals, clinics, and public health agencies. This real-time data collection enables quicker responses to emerging health threats. Rapid Innovation supports the development of these networks, ensuring that clients can respond effectively to public health challenges.
- Enhanced Disease Surveillance: By analyzing data from multiple regions, these networks can identify patterns and trends in disease outbreaks. This information is vital for public health officials to implement effective interventions. Our data analytics capabilities empower clients to leverage this information for proactive health management.
- Improved Resource Allocation: With accurate health data, governments and organizations can allocate resources more effectively. This ensures that areas with the greatest need receive the necessary support, improving overall health outcomes. Rapid Innovation's solutions facilitate better decision-making, ultimately leading to healthier populations worldwide.
Global health monitoring networks play a crucial role in enhancing public health preparedness and response, ultimately leading to healthier populations worldwide.
10.5. Predictive Global Pandemic Response
Predictive global pandemic response refers to the proactive measures and strategies that nations and organizations can implement to anticipate and mitigate the effects of potential pandemics. This approach relies on data analytics, modeling, and forecasting to identify risks and prepare for outbreaks before they escalate.
- Utilization of advanced data analytics to monitor health trends and identify potential outbreaks.
- Development of predictive models that simulate the spread of infectious diseases.
- Collaboration between governments, health organizations, and tech companies to share data and resources.
- Investment in research to understand pathogens and their transmission dynamics.
- Establishment of early warning systems that can alert authorities to emerging health threats.
- Training healthcare professionals in rapid response protocols to ensure swift action during outbreaks.
- Public health campaigns to educate communities about preventive measures and the importance of vaccination.
By adopting a predictive global pandemic response, countries can reduce the impact of infectious diseases, save lives, and minimize economic disruption. At Rapid Innovation, we leverage our expertise in AI and data analytics to help organizations implement these pandemic preparedness strategies effectively, ensuring they are well-prepared for any health crisis. Additionally, we explore the role of AI for mental health care in supporting overall public health during such crises.
11. Implementation Roadmap
An implementation roadmap outlines the steps necessary to execute a strategic plan effectively. In the context of pandemic preparedness, this roadmap serves as a guide for governments and organizations to enhance their response capabilities.
- Define clear objectives and goals for pandemic preparedness.
- Identify key stakeholders, including public health agencies, healthcare providers, and community organizations.
- Develop a timeline for implementation, including short-term and long-term milestones.
- Allocate resources, including funding, personnel, and technology, to support the plan.
- Establish communication channels to ensure information flows smoothly among stakeholders.
- Create training programs for healthcare workers and emergency responders.
- Regularly review and update the roadmap based on new data and lessons learned from past pandemics.
An effective implementation roadmap is crucial for ensuring that all parties are aligned and prepared to respond to future health crises. Rapid Innovation can assist in developing this roadmap by integrating AI-driven insights and blockchain technology for secure data sharing and collaboration.
11.1. Initial Assessment and Readiness
The initial assessment and readiness phase is critical in determining a country's or organization's preparedness for a pandemic. This phase involves evaluating existing resources, capabilities, and vulnerabilities to identify areas for improvement.
- Conduct a comprehensive review of current public health infrastructure and resources.
- Assess the availability of medical supplies, including personal protective equipment (PPE) and vaccines.
- Evaluate the capacity of healthcare systems to handle surges in patient volume during an outbreak.
- Identify gaps in training and knowledge among healthcare professionals regarding infectious disease management.
- Engage with community stakeholders to understand local health needs and concerns.
- Analyze past pandemic responses to identify strengths and weaknesses in current strategies.
- Develop a readiness plan that includes protocols for communication, resource allocation, and patient care.
By performing an initial assessment and ensuring readiness, organizations can build a robust foundation for effective pandemic response and resilience. Rapid Innovation's expertise in AI and blockchain can enhance this process, providing data-driven insights and secure frameworks for collaboration among stakeholders.
11.2. Pilot Program Design
Designing a pilot program is a crucial step in testing new initiatives before full-scale implementation. A well-structured pilot program allows organizations to evaluate the feasibility, effectiveness, and potential challenges of a project.
- Define clear objectives: Establish what you aim to achieve with the pilot program. This could include improving efficiency, enhancing customer satisfaction, or testing new technologies. For instance, Rapid Innovation can help clients define objectives that align with their strategic goals, ensuring that the pilot program is focused on delivering measurable outcomes.
- Select a representative sample: Choose a group that reflects the larger population. This ensures that the results are applicable when the program is rolled out on a larger scale. By leveraging AI analytics, Rapid Innovation can assist in identifying the most representative sample for accurate results.
- Develop a detailed plan: Outline the timeline, resources needed, and specific activities involved in the pilot. This plan should also include metrics for success. Rapid Innovation can provide expertise in project management tools that facilitate effective planning and tracking.
- Engage stakeholders: Involve key stakeholders early in the process to gain their support and insights. Their feedback can help refine the program. Rapid Innovation emphasizes the importance of stakeholder engagement, utilizing blockchain technology to ensure transparency and trust in the process.
- Monitor and evaluate: Implement a system for tracking progress and collecting data throughout the pilot. Regular evaluations will help identify areas for improvement. Rapid Innovation can integrate AI-driven analytics to provide real-time insights, enabling data-driven decision-making.
- Prepare for adjustments: Be flexible and ready to make changes based on feedback and results. This adaptability can enhance the overall success of the program. Rapid Innovation's agile methodologies ensure that clients can pivot quickly in response to pilot findings.
11.3. Scalability Considerations
Scalability is a vital aspect of any program or initiative, as it determines the ability to grow and adapt to increased demands. When designing a program, consider the following factors to ensure scalability:
- Infrastructure: Assess whether the current infrastructure can support growth. This includes technology, human resources, and operational processes. Rapid Innovation can evaluate existing systems and recommend scalable solutions, particularly in AI and blockchain environments.
- Resource allocation: Plan for the necessary resources, including budget, personnel, and technology, to accommodate expansion. Our team can assist in creating a resource allocation strategy that maximizes ROI.
- Standardization: Develop standardized processes and procedures that can be easily replicated across different teams or locations. This helps maintain quality and efficiency as the program scales. Rapid Innovation can implement best practices in AI and blockchain to ensure consistency.
- Training and support: Ensure that staff are adequately trained and supported as the program expands. This may involve creating training materials or establishing mentorship programs. Rapid Innovation offers tailored training solutions to equip teams with the necessary skills for new technologies.
- Feedback loops: Implement mechanisms for continuous feedback to identify potential scalability issues early. This allows for proactive adjustments before they become significant problems. Our expertise in AI can facilitate the creation of feedback systems that enhance responsiveness.
- Market demand: Analyze market trends and customer needs to ensure that the program can adapt to changing demands. This foresight can help in planning for future growth. Rapid Innovation leverages data analytics to provide insights into market dynamics, ensuring that clients remain competitive.
11.4. Change Management
Change management is essential for successfully implementing new initiatives within an organization. It involves preparing, supporting, and helping individuals and teams to adapt to change. Effective change management can minimize resistance and enhance the likelihood of success.
- Communicate clearly: Provide transparent information about the changes being made, the reasons behind them, and the expected outcomes. Clear communication helps alleviate uncertainty and builds trust. Rapid Innovation emphasizes the importance of communication strategies that leverage AI tools for effective dissemination.
- Involve employees: Engage employees in the change process by seeking their input and feedback. This involvement can foster a sense of ownership and reduce resistance. Our approach includes utilizing blockchain for secure and transparent feedback mechanisms.
- Provide training: Offer training and resources to help employees develop the skills needed to adapt to the changes. This support can ease the transition and boost confidence. Rapid Innovation provides comprehensive training programs tailored to the specific needs of the organization.
- Monitor progress: Regularly assess how the change is being received and its impact on the organization. This can help identify areas that need additional support or adjustment. Our AI-driven monitoring tools can provide insights into employee engagement and program effectiveness.
- Celebrate successes: Acknowledge and celebrate milestones and achievements throughout the change process. Recognizing progress can motivate employees and reinforce positive attitudes toward change. Rapid Innovation encourages a culture of recognition to foster a positive environment during transitions.
- Foster a culture of adaptability: Encourage a mindset that embraces change and innovation. This cultural shift can make future transitions smoother and more successful. Rapid Innovation supports organizations in building a culture that values continuous improvement and innovation, essential for thriving in today's dynamic business landscape.
11.5. Continuous Improvement Framework
A Continuous Improvement Framework (CIF) is essential for organizations aiming to enhance their processes, products, and services consistently. This framework is built on the principle that there is always room for improvement, and it encourages a culture of ongoing development.
- Focus on incremental changes rather than large-scale transformations.
- Utilize methodologies such as Plan-Do-Check-Act (PDCA) to guide improvement efforts.
- Engage employees at all levels to foster a sense of ownership and accountability.
- Collect and analyze data to identify areas needing improvement.
- Implement feedback loops to ensure that changes are effective and sustainable.
- Encourage innovation by allowing teams to experiment with new ideas.
- Regularly review and update processes to adapt to changing environments and needs.
At Rapid Innovation, we leverage AI and Blockchain technologies to enhance the CIF, enabling organizations to achieve greater efficiency and effectiveness. For instance, AI-driven analytics can provide real-time insights into operational performance, allowing businesses to identify improvement areas swiftly. Blockchain can ensure data integrity and transparency, fostering trust in the feedback loops established within the CIF. The CIF can be applied across various sectors, including healthcare, manufacturing, and education, to drive efficiency and enhance quality. By embedding continuous improvement into the organizational culture, companies can achieve long-term success and customer satisfaction.
To implement a continuous improvement framework example, organizations can follow the steps of continuous improvement framework, which includes defining objectives, measuring performance, analyzing data, and implementing changes. The itil continuous improvement model can also be integrated into this framework to align IT services with business needs. Additionally, organizations can utilize the itil service improvement plan and itil service improvement plan template to structure their improvement initiatives effectively.
Furthermore, the continuous quality improvement framework and cqi framework provide methodologies for enhancing service quality. The driver framework for continuous improvement can help identify key factors that influence improvement efforts. Organizations may also consider the commercial continuous improvement assessment framework to evaluate their current practices and identify areas for growth. By adopting the baldrige continuous improvement and baldrige process improvement principles, organizations can ensure a comprehensive approach to enhancing their operations. For more insights on leveraging AI for continuous improvement, check out the AI Development Guide for Businesses.
12. Ethical Considerations
Ethical considerations are crucial in any organization, particularly in sectors like healthcare, where the stakes are high. Ethical practices ensure that organizations operate with integrity, respect, and accountability.
- Establish clear ethical guidelines and standards for all employees.
- Promote transparency in decision-making processes.
- Encourage open dialogue about ethical dilemmas and challenges.
- Provide training on ethical practices and decision-making.
- Foster a culture of respect and inclusivity within the organization.
By prioritizing ethical considerations, organizations can build trust with stakeholders, enhance their reputation, and ensure compliance with legal and regulatory requirements.
12.1. Patient Privacy
Patient privacy is a fundamental ethical consideration in healthcare. Protecting patient information is not only a legal requirement but also a moral obligation that healthcare providers must uphold.
- Adhere to regulations such as the Health Insurance Portability and Accountability Act (HIPAA) in the United States.
- Implement robust data security measures to protect patient information from unauthorized access.
- Train staff on the importance of patient confidentiality and the proper handling of sensitive information.
- Use secure communication channels for sharing patient data.
- Regularly audit and assess privacy practices to identify vulnerabilities and areas for improvement.
At Rapid Innovation, we understand the importance of patient privacy and utilize advanced AI and Blockchain solutions to enhance data security and compliance. Maintaining patient privacy fosters trust between healthcare providers and patients, encouraging individuals to seek care without fear of their information being mishandled. By prioritizing patient privacy, healthcare organizations can enhance their credibility and ensure compliance with ethical standards.
12.2. Informed Consent
Informed consent is a fundamental principle in both medical and technological fields, ensuring that individuals understand what they are agreeing to when participating in research or using a service. In the context of data collection and algorithmic processes, informed consent becomes increasingly complex due to the intricacies of data usage and the potential implications for privacy, particularly in areas such as telemedicine informed consent requirements and informed consent in technology.
- Individuals must be provided with clear, concise information about how their data will be used.
- Consent should be obtained freely, without coercion, and individuals should have the right to withdraw their consent at any time.
- Transparency is key; users should know what data is being collected, the purpose of the collection, and how it will be processed.
- Organizations should implement user-friendly consent forms that avoid technical jargon, making it easier for individuals to understand their rights.
- Regular updates should be provided to users if there are changes in data usage policies, ensuring ongoing informed consent.
The ethical implications of informed consent are significant, as failure to obtain proper consent can lead to breaches of trust and legal repercussions. Organizations must prioritize informed consent to foster a culture of respect and accountability. At Rapid Innovation, we emphasize the importance of informed consent in our AI and Blockchain solutions, ensuring that our clients can build trust with their users while maximizing the value of their data.
12.3. Algorithmic Transparency
Algorithmic transparency refers to the clarity and openness surrounding the algorithms that drive decision-making processes in various technologies. As algorithms increasingly influence critical areas such as finance, healthcare, and criminal justice, understanding their workings becomes essential.
- Transparency allows stakeholders to understand how decisions are made, which is crucial for accountability.
- It helps identify potential biases in algorithms, ensuring that they do not perpetuate discrimination or inequality.
- Organizations should disclose the data sources used to train algorithms, as well as the methodologies employed in their development.
- Providing access to algorithmic processes can empower users to question and challenge decisions that affect them.
- Regular audits and assessments of algorithms can enhance transparency and build public trust.
By promoting algorithmic transparency, organizations can mitigate risks associated with opaque decision-making processes and foster a more equitable technological landscape. Rapid Innovation integrates algorithmic transparency into our AI solutions, enabling clients to demonstrate accountability and build user confidence, ultimately leading to greater ROI.
12.4. Bias Mitigation Strategies
Bias in algorithms can lead to unfair outcomes, reinforcing existing inequalities and discrimination. Therefore, implementing bias mitigation strategies is crucial for developing fair and equitable systems.
- Diverse data collection is essential; using datasets that represent various demographics can help reduce bias.
- Regularly auditing algorithms for bias can identify problematic areas and allow for timely corrections.
- Employing fairness-aware algorithms can help ensure that decision-making processes are equitable across different groups.
- Engaging with stakeholders, including affected communities, can provide valuable insights into potential biases and their impacts.
- Continuous training and education for developers on bias and its implications can foster a culture of awareness and responsibility.
By actively addressing bias through these strategies, organizations can create more inclusive technologies that serve all individuals fairly and justly. At Rapid Innovation, we assist our clients in implementing these bias mitigation strategies within their AI and Blockchain projects, ensuring that their systems are not only effective but also equitable, thereby enhancing their overall business performance.
12.5. Ethical AI Development Principles
Ethical AI development principles are essential guidelines that ensure artificial intelligence technologies are created and implemented responsibly. These principles aim to promote fairness, accountability, transparency, and respect for human rights. Here are the key components of ethical AI development:
- Fairness: AI systems should be designed to avoid bias and discrimination. This involves ensuring diverse datasets that represent various demographics, regularly auditing algorithms to identify and mitigate biases, and implementing fairness metrics to evaluate AI outcomes. At Rapid Innovation, we prioritize fairness in our ethical AI development solutions, ensuring that our clients can trust the integrity of their AI systems.
- Accountability: Developers and organizations must take responsibility for the AI systems they create. This includes establishing clear lines of accountability for AI decisions, creating mechanisms for redress when AI systems cause harm, and documenting decision-making processes to ensure traceability. Rapid Innovation helps clients implement accountability frameworks that enhance trust and reliability in their ethical AI development applications.
- Transparency: AI systems should operate in a transparent manner, allowing users to understand how decisions are made. This can be achieved by providing clear explanations of AI algorithms and their functions, making data sources and methodologies publicly available, and engaging stakeholders in discussions about AI system design and deployment. Our consulting services at Rapid Innovation emphasize transparency, enabling clients to communicate effectively about their ethical AI development technologies.
- Privacy: Protecting user data is crucial in AI development. Key practices include implementing data minimization strategies to collect only necessary information, using encryption and anonymization techniques to safeguard personal data, and ensuring compliance with data protection regulations, such as GDPR. Rapid Innovation assists clients in developing ethical AI solutions that prioritize user privacy, thereby enhancing customer trust and compliance.
- Safety and Security: AI systems must be designed to operate safely and securely. This involves conducting thorough testing to identify vulnerabilities, implementing robust security measures to protect against cyber threats, and establishing protocols for responding to AI failures or malfunctions. Our team at Rapid Innovation ensures that safety and security are integral to the ethical AI development process, reducing risks for our clients.
- Human-Centric Design: AI should enhance human capabilities and well-being. This principle emphasizes designing AI systems that prioritize user experience and accessibility, involving users in the design process to ensure their needs are met, and fostering collaboration between humans and AI to achieve optimal outcomes. Rapid Innovation focuses on human-centric design in our ethical AI development, ensuring that our AI solutions are intuitive and beneficial for end-users.
- Sustainability: AI development should consider environmental impacts. This includes reducing the carbon footprint of AI training and deployment, promoting energy-efficient algorithms and hardware, and supporting initiatives that leverage AI for environmental conservation. At Rapid Innovation, we advocate for sustainable ethical AI practices, helping clients align their technologies with environmental goals.
- Collaboration and Inclusivity: Engaging diverse stakeholders in AI development is vital. This can be achieved by encouraging interdisciplinary collaboration among technologists, ethicists, and social scientists, involving marginalized communities in discussions about AI impacts, and sharing best practices and lessons learned across organizations and sectors. Rapid Innovation fosters collaboration and inclusivity in our ethical AI development, ensuring that our AI solutions reflect a wide range of perspectives.
- Continuous Learning and Improvement: The field of AI is rapidly evolving, necessitating ongoing education and adaptation. This involves staying informed about emerging ethical challenges and technological advancements, regularly updating AI systems based on user feedback and new research, and fostering a culture of ethical awareness within organizations. Rapid Innovation promotes continuous learning in ethical AI development, ensuring that our clients remain at the forefront of AI innovation.
- Regulatory Compliance: Adhering to laws and regulations governing AI is essential. This includes understanding and implementing relevant legal frameworks, engaging with policymakers to shape future regulations, and ensuring that AI systems align with international human rights standards. Our expertise at Rapid Innovation helps clients navigate the complex landscape of AI regulations, ensuring compliance and ethical alignment in their AI development.
- Public Engagement: Building trust in AI technologies requires open communication with the public. This can be achieved by providing clear information about AI capabilities and limitations, encouraging public discourse on ethical AI issues, and actively seeking public input on AI policies and practices. Rapid Innovation supports clients in fostering public engagement, enhancing the societal acceptance of their ethical AI development initiatives.
- Ethical Leadership: Organizations must cultivate a culture of ethical AI development. This involves appointing ethics officers or committees to oversee AI initiatives, providing training on ethical considerations for all employees involved in AI, and promoting ethical decision-making at all levels of the organization. Rapid Innovation assists clients in establishing ethical leadership frameworks, ensuring that ethical considerations are embedded in their AI strategies.
By adhering to these ethical AI development principles, organizations can create technologies that not only advance innovation but also respect human rights and promote societal well-being. The commitment to ethical AI is not just a regulatory requirement; it is a moral imperative that shapes the future of technology and its impact on humanity. At Rapid Innovation, we are dedicated to guiding our clients through this ethical landscape, ensuring that their AI initiatives yield greater ROI while upholding the highest ethical standards. For more information on our services, visit our Ethical AI Development Guide.