Table Of Contents
Category
Artificial Intelligence
Manufacturing
IoT
1. Introduction to AI-Driven Maintenance
Artificial Intelligence (AI) is revolutionizing various industries, and maintenance is no exception. AI-driven maintenance strategies leverage advanced algorithms and data analytics to enhance operational efficiency, reduce downtime, and optimize resource allocation. This shift from traditional maintenance practices to AI-driven approaches is transforming how organizations manage their assets. AI technologies, such as machine learning and predictive analytics, are being integrated into maintenance processes, enabling real-time monitoring and analysis of equipment performance. As a result, organizations can anticipate failures before they occur, leading to proactive maintenance strategies.
1.1 Evolution of Maintenance Strategies
The evolution of maintenance strategies has been marked by significant advancements in technology and methodologies. Understanding this evolution is crucial for grasping the impact of AI on maintenance practices.
- Reactive Maintenance: This is the most basic form of maintenance, where repairs are made only after equipment fails. While it is cost-effective in the short term, it often leads to unexpected downtime and higher repair costs.
- Preventive Maintenance: This strategy involves scheduled maintenance activities based on time intervals or usage metrics. It aims to prevent equipment failures by performing regular inspections and servicing. However, it can lead to unnecessary maintenance if not properly aligned with actual equipment needs.
- Predictive Maintenance: With the advent of data analytics, predictive maintenance emerged as a more sophisticated approach. It uses data from sensors and historical performance to predict when equipment is likely to fail. This allows organizations to perform maintenance only when necessary, reducing costs and downtime.
- Prescriptive Maintenance: The latest evolution in maintenance strategies is prescriptive maintenance, which goes a step further than predictive maintenance. It not only predicts failures but also recommends specific actions to optimize maintenance schedules and resource allocation. AI plays a crucial role in this strategy by analyzing vast amounts of data to provide actionable insights.
- AI-Driven Maintenance Strategies: The integration of AI into maintenance strategies represents the pinnacle of this evolution. AI agents can analyze real-time data, learn from historical trends, and adapt maintenance strategies accordingly. This leads to enhanced decision-making capabilities, improved asset lifespan, and reduced operational costs.
The transition from reactive to AI-driven maintenance reflects a broader trend towards digital transformation in industries. Organizations that adopt AI-driven maintenance strategies can gain a competitive edge by maximizing asset performance and minimizing unplanned downtime. At Rapid Innovation, we specialize in implementing these advanced AI solutions, ensuring that our clients achieve greater ROI through optimized maintenance processes and reduced operational disruptions. For more information on AI agents and their role in equipment monitoring.
Refer to the image for a visual representation of the evolution of maintenance strategies in AI-driven maintenance.
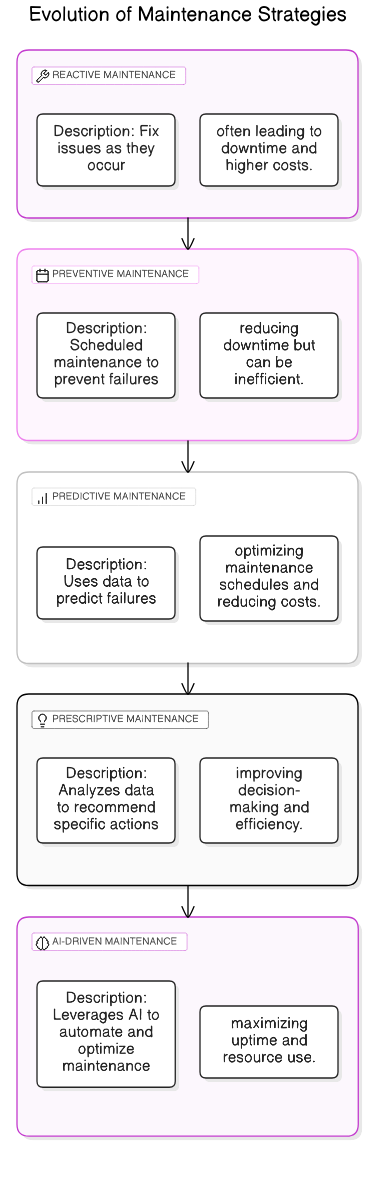
1.2. Role of Artificial Intelligence in Maintenance
Artificial Intelligence (AI) is revolutionizing the maintenance landscape across various industries. By integrating AI technologies, organizations can enhance their maintenance strategies, leading to improved efficiency and reduced costs. At Rapid Innovation, we specialize in implementing ai maintenance solutions that empower businesses to achieve their operational goals effectively.
- Predictive Maintenance: Our AI algorithms analyze historical data to predict equipment failures before they occur. This proactive approach minimizes downtime and extends the lifespan of machinery, ultimately leading to a greater return on investment (ROI) for our clients.
- Condition Monitoring: We deploy AI systems that continuously monitor equipment conditions using sensors and IoT devices. This real-time data collection allows for immediate responses to any anomalies, ensuring that operations run smoothly and efficiently.
- Automated Scheduling: Our AI solutions optimize maintenance schedules based on usage patterns and equipment health, ensuring that maintenance is performed at the most effective times. This not only enhances productivity but also reduces unnecessary costs.
- Data Analysis: Rapid Innovation utilizes advanced AI tools that can process vast amounts of data quickly, identifying trends and patterns that human analysts might miss. This capability leads to more informed decision-making, allowing organizations to allocate resources more effectively.
- Resource Allocation: Our AI systems help optimize the allocation of maintenance resources, ensuring that the right personnel and tools are available when needed. This strategic approach enhances operational efficiency and reduces labor costs.
- Cost Reduction: By minimizing unplanned downtime and optimizing maintenance schedules, our ai maintenance solutions can significantly reduce operational costs, providing clients with a measurable increase in ROI.
1.3. Key Challenges in Traditional Maintenance Approaches
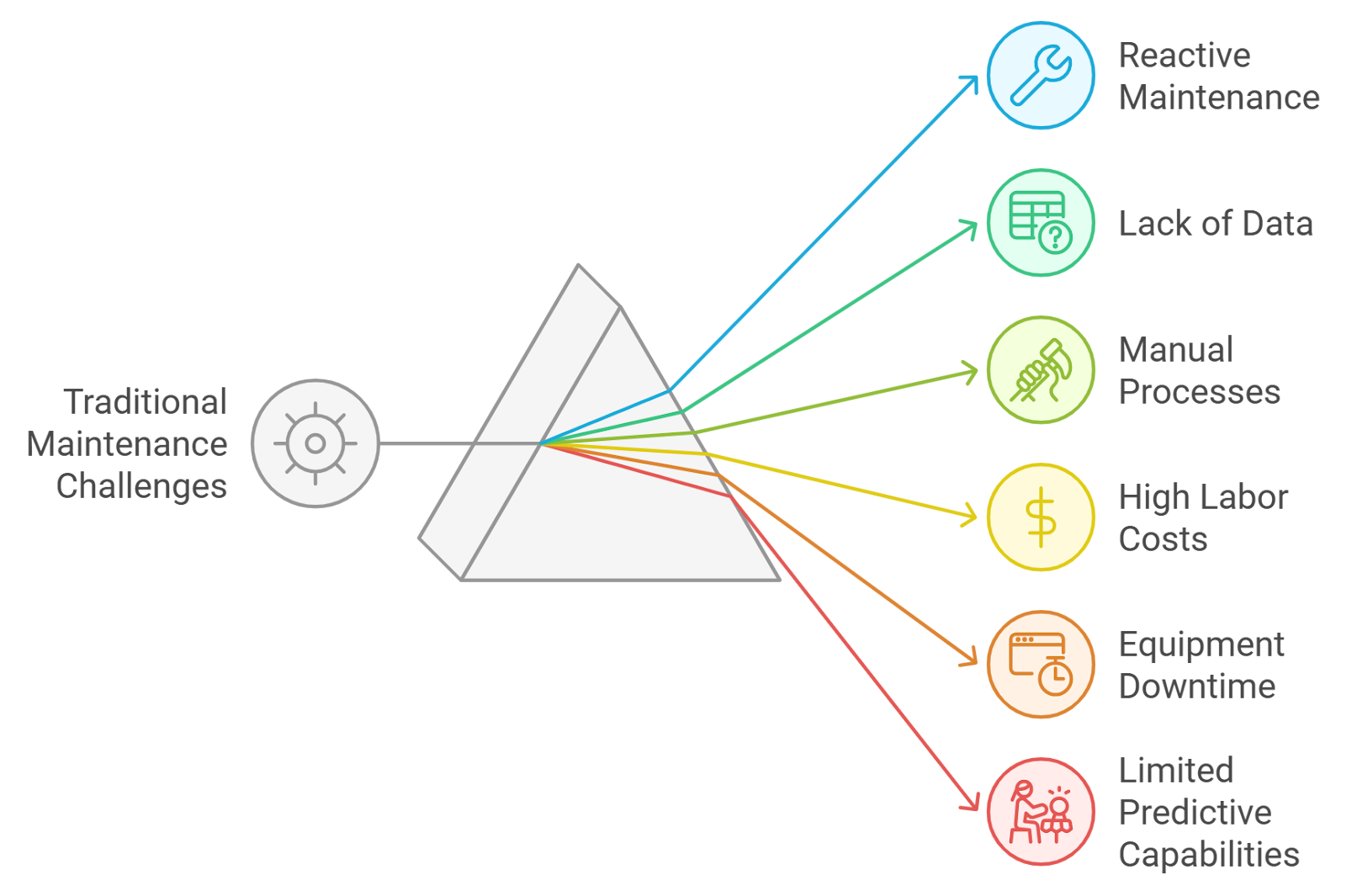
Traditional maintenance approaches often face several challenges that can hinder operational efficiency and increase costs. Understanding these challenges is crucial for organizations looking to improve their maintenance strategies.
- Reactive Maintenance: Many organizations still rely on reactive maintenance, addressing issues only after they occur. This approach can lead to unexpected downtime and higher repair costs.
- Lack of Data: Traditional methods often lack the data-driven insights necessary for effective decision-making. Without accurate data, maintenance strategies can be inefficient and ineffective.
- Manual Processes: Many maintenance tasks are still performed manually, leading to human error and inconsistencies in maintenance practices.
- High Labor Costs: Traditional maintenance approaches can be labor-intensive, resulting in higher costs associated with staffing and training.
- Equipment Downtime: Unplanned equipment failures can lead to significant downtime, affecting productivity and revenue.
- Limited Predictive Capabilities: Traditional maintenance lacks the predictive capabilities that modern technologies offer, making it difficult to anticipate and prevent failures.
2. Fundamentals of AI Maintenance Agents
AI maintenance agents are specialized systems designed to enhance maintenance processes through automation and intelligent decision-making. Understanding the fundamentals of these agents is essential for organizations looking to implement AI in their maintenance strategies.
- Machine Learning: AI maintenance agents utilize machine learning algorithms to analyze historical data and learn from it. This enables them to make predictions about equipment performance and maintenance needs.
- Natural Language Processing: Some AI agents can understand and process human language, allowing for easier communication between maintenance teams and the AI system.
- Integration with IoT: AI maintenance agents often work in conjunction with IoT devices, collecting real-time data from equipment and providing insights for maintenance decisions.
- Autonomous Decision-Making: These agents can make autonomous decisions based on predefined criteria, reducing the need for human intervention in routine maintenance tasks.
- User-Friendly Interfaces: Many AI maintenance agents come with intuitive interfaces that allow maintenance personnel to easily interact with the system and access critical information.
- Continuous Learning: AI maintenance agents continuously learn from new data, improving their predictive capabilities and decision-making processes over time.
By leveraging AI maintenance agents, organizations can transform their maintenance practices, leading to increased efficiency, reduced costs, and enhanced equipment reliability. At Rapid Innovation, we are committed to helping our clients harness the power of AI to achieve their business objectives and maximize their ROI.
Refer to the image for a visual representation of the role of Artificial Intelligence in maintenance strategies.

2.1. Definition and Conceptual Framework
Artificial Intelligence (AI) refers to the simulation of human intelligence processes by machines, particularly computer systems. These processes include learning, reasoning, and self-correction. The conceptual framework of AI encompasses various components that work together to create intelligent behavior in machines.
- Learning: This involves acquiring information and rules for using it. Machine learning, a subset of AI, enables systems to learn from data and improve over time without being explicitly programmed.
- Reasoning: AI systems can solve problems through logical deduction. This involves using algorithms to process information and make decisions based on available data.
- Self-correction: AI systems can adjust their actions based on feedback from their environment, enhancing their performance over time.
The framework also includes different types of AI, such as narrow AI, which is designed for specific tasks, and general AI, which aims to perform any intellectual task that a human can do. The integration of these components allows AI systems to operate autonomously, making them valuable in various fields, including maintenance and AI agents in maintenance.
At Rapid Innovation, we leverage this conceptual framework to develop tailored AI solutions that align with our clients' business goals, ensuring they achieve greater ROI through enhanced operational efficiency and decision-making capabilities.
2.2. Types of AI Agents in Maintenance
AI agents in maintenance can be categorized based on their functionality and the level of autonomy they possess. These agents are designed to enhance operational efficiency, reduce downtime, and improve decision-making processes in maintenance activities. The main types of AI agents include:
- Reactive Agents: These agents respond to specific stimuli or events in their environment. They do not possess memory or the ability to learn from past experiences. Instead, they operate based on predefined rules and conditions.
- Deliberative Agents: These agents have a more complex structure, allowing them to plan and make decisions based on their goals and the current state of their environment. They can learn from past experiences and adapt their strategies accordingly.
- Hybrid Agents: Combining features of both reactive and deliberative agents, hybrid agents can respond to immediate situations while also planning for future actions based on learned experiences.
Each type of AI agent plays a crucial role in maintenance, contributing to predictive maintenance, fault detection, and overall operational efficiency. At Rapid Innovation, we implement these AI agents to help our clients streamline their maintenance processes, ultimately leading to significant cost savings and improved asset management.
2.2.1. Reactive Agents
Reactive agents are the simplest form of AI agents, characterized by their immediate response to environmental stimuli. They operate on a set of predefined rules and do not retain information from past interactions. This makes them suitable for tasks that require quick responses without the need for complex decision-making processes.
- Characteristics:
- No Memory: Reactive agents do not store past experiences, which limits their ability to learn or adapt over time.
- Rule-Based: They operate based on a fixed set of rules, responding to specific inputs with predetermined outputs.
- Fast Response: Their simplicity allows for rapid responses to changes in the environment, making them effective in time-sensitive situations.
- Applications in Maintenance:
- Fault Detection: Reactive agents can monitor equipment and trigger alarms when predefined thresholds are exceeded, indicating potential failures.
- Real-Time Monitoring: They can continuously assess the condition of machinery and provide immediate feedback to operators.
- Basic Automation: Reactive agents can automate routine maintenance tasks, such as turning equipment on or off based on operational conditions.
While reactive agents are limited in their capabilities, they are valuable in environments where quick, reliable responses are essential. Their straightforward design allows for easy implementation in various maintenance scenarios, contributing to improved operational efficiency and reduced downtime. Rapid Innovation harnesses the power of reactive agents in maintenance to deliver solutions that enhance our clients' operational resilience and responsiveness.
Refer to the image for a visual representation of the AI conceptual framework and types of AI agents in maintenance:

.
2.2.2. Predictive Agents
Predictive agents technology refers to advanced systems designed to analyze data and forecast future events or behaviors. They utilize machine learning algorithms and statistical models to identify patterns and trends, enabling them to make informed predictions. These agents are increasingly used across various industries, including finance, healthcare, and marketing.
- Key features of predictive agents include:
- Data analysis: They process large datasets to extract meaningful insights.
- Pattern recognition: They identify trends and correlations that may not be immediately apparent.
- Forecasting: They provide predictions based on historical data, helping organizations make strategic decisions.
At Rapid Innovation, we leverage predictive agents technology to enhance decision-making for our clients, providing actionable insights that drive greater ROI. For instance, in retail, our predictive models can forecast customer purchasing behavior, allowing businesses to optimize inventory and marketing strategies effectively. In healthcare, we utilize predictive agents technology to forecast patient outcomes, thereby improving treatment plans and resource allocation. As an AI agent development company, we are committed to delivering innovative solutions that meet the unique needs of our clients. Additionally, our expertise extends to multi-agent systems, which enhance the capabilities of predictive agents.
2.2.3. Proactive Agents
Proactive agents go a step further than predictive agents by not only forecasting future events but also taking preemptive actions based on those predictions. These agents are designed to anticipate needs and respond accordingly, often in real-time. They are particularly valuable in dynamic environments where timely responses are crucial.
- Characteristics of proactive agents include:
- Anticipation: They predict potential issues or opportunities before they arise.
- Automation: They can execute tasks automatically based on predefined criteria.
- Adaptability: They adjust their actions based on changing circumstances or new information.
Proactive agents are widely used in customer service, where they can anticipate customer inquiries and provide solutions before issues escalate. In cybersecurity, they can detect potential threats and take preventive measures to safeguard systems. This capability not only enhances efficiency but also improves user experience and satisfaction, ultimately leading to increased ROI for our clients.
2.3. Core Technologies and Architectures
The development of intelligent agents relies on several core technologies and architectures that enable their functionality. These technologies form the backbone of agent systems, allowing them to operate effectively in various applications.
- Essential technologies include:
- Machine Learning: This technology allows agents to learn from data and improve their performance over time.
- Natural Language Processing (NLP): NLP enables agents to understand and process human language, facilitating communication between users and systems.
- Data Mining: This involves extracting useful information from large datasets, which is crucial for predictive and proactive agents technology.
- Common architectures for intelligent agents include:
- Agent-based Systems: These systems consist of multiple agents that interact with each other and their environment to achieve specific goals.
- Multi-Agent Systems: This architecture involves multiple agents working collaboratively or competitively to solve complex problems.
- Service-Oriented Architectures (SOA): SOA allows agents to communicate and share resources across different platforms, enhancing interoperability.
At Rapid Innovation, we integrate these technologies and architectures to develop robust and efficient intelligent agents tailored to our clients' needs. As the field continues to evolve, our commitment to leveraging advancements in these areas will further enhance the capabilities of predictive agents technology and proactive agents, leading to more sophisticated applications across various sectors and driving greater business success.
Refer to the image for a visual representation of predictive and proactive agents and their core technologies.
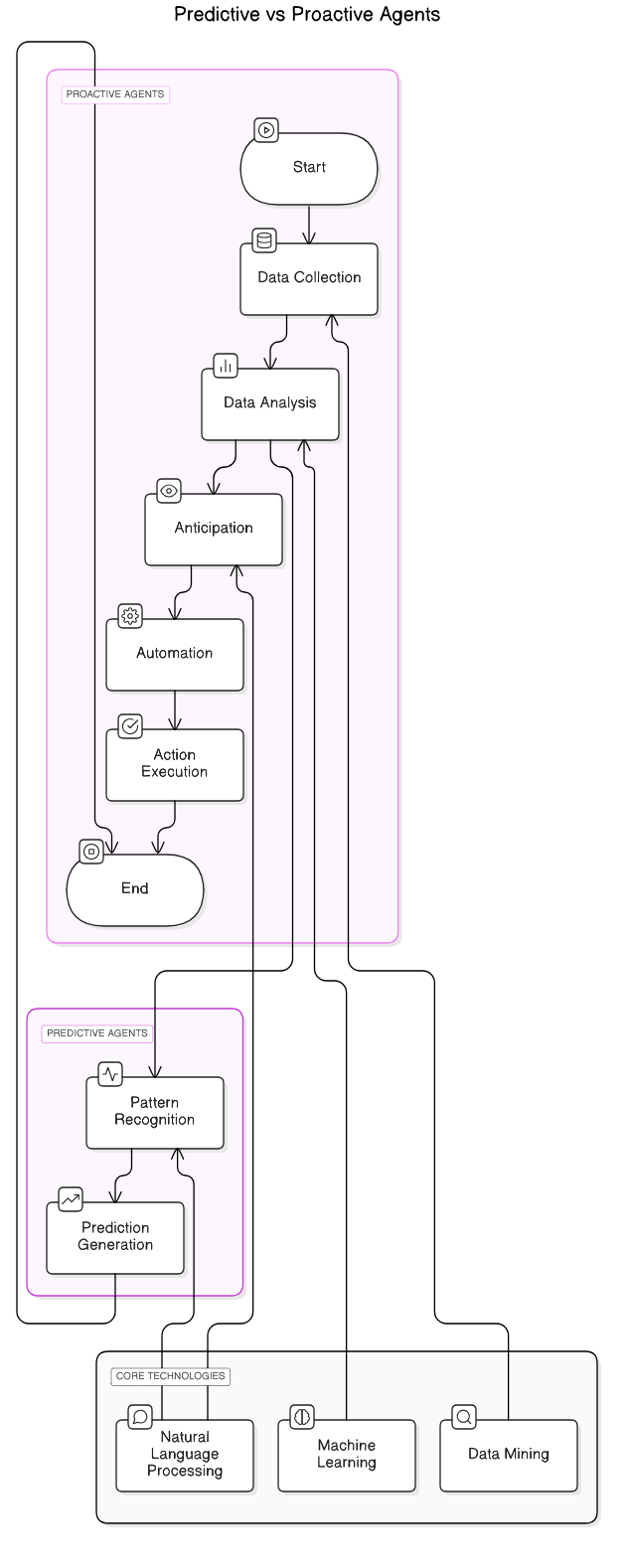
3. Technical Architecture of Maintenance AI Agents
The technical architecture of Maintenance AI Agents is crucial for their effectiveness in predictive maintenance and operational efficiency. This architecture typically consists of several key components that work together to gather data, analyze it, and provide actionable insights.
3.1. Sensor and Data Integration
Sensor and data integration is the backbone of Maintenance AI Agents. These agents rely on a variety of sensors to collect real-time data from machinery and equipment. The integration of these sensors is essential for creating a comprehensive view of the operational environment.
- Types of Sensors:
- Vibration sensors monitor the mechanical health of equipment.
- Temperature sensors track overheating issues.
- Pressure sensors detect anomalies in fluid systems.
- Acoustic sensors identify unusual sounds that may indicate mechanical failure.
- Data Sources:
- IoT devices provide continuous streams of data.
- Historical maintenance records offer context for current performance.
- Environmental data, such as humidity and temperature, can influence equipment performance.
- Data Integration Techniques:
- Edge computing processes data close to the source, reducing latency.
- Cloud computing allows for scalable storage and advanced analytics.
- APIs facilitate communication between different systems and platforms.
- Challenges in Data Integration:
- Data silos can hinder the flow of information across departments.
- Ensuring data quality and consistency is vital for accurate analysis.
- Real-time data processing requires robust infrastructure.
Effective sensor and data integration enables Maintenance AI Agents to monitor equipment health continuously, predict failures, and optimize maintenance schedules. This proactive approach can lead to significant cost savings and increased operational efficiency.
3.2. Machine Learning Models
Machine learning models are at the heart of Maintenance AI Agents, enabling them to analyze vast amounts of data and derive insights that inform maintenance strategies. These models can identify patterns, predict failures, and recommend actions based on historical and real-time data.
- Types of Machine Learning Models:
- Supervised learning models use labeled data to predict outcomes, such as failure probabilities.
- Unsupervised learning models identify hidden patterns in data without predefined labels.
- Reinforcement learning models optimize decision-making processes through trial and error.
- Key Algorithms:
- Decision trees provide interpretable models for classification tasks.
- Neural networks excel in recognizing complex patterns in large datasets.
- Support vector machines are effective for classification problems with high-dimensional data.
- Model Training and Validation:
- Training involves feeding the model historical data to learn patterns.
- Cross-validation techniques ensure the model's robustness and prevent overfitting.
- Continuous learning allows models to adapt to new data and changing conditions.
- Applications of Machine Learning in Maintenance:
- Predictive maintenance identifies potential failures before they occur, reducing downtime.
- Anomaly detection alerts operators to unusual patterns that may indicate issues.
- Optimization algorithms improve maintenance schedules based on equipment usage and condition.
The implementation of machine learning models in Maintenance AI Agents enhances their ability to make data-driven decisions, ultimately leading to improved reliability and efficiency in operations. By leveraging advanced analytics, organizations can transition from reactive to proactive maintenance strategies, significantly reducing costs and increasing productivity. At Rapid Innovation, we specialize in developing and integrating maintenance AI agents, ensuring that our clients achieve greater ROI through enhanced operational efficiency and reduced maintenance costs.
3.2.1. Supervised Learning Techniques
Supervised learning is a type of machine learning where the model is trained on labeled data. This means that the input data is paired with the correct output, allowing the algorithm to learn the relationship between the two. Supervised learning is widely used in various applications, including classification and regression tasks.
- Classification: This involves predicting a discrete label for a given input. Common algorithms include:
- Logistic Regression
- Decision Trees
- Support Vector Machines (SVM)
- Neural Networks
- machine learning classification algorithms
- supervised machine learning algorithms
- Regression: This focuses on predicting continuous values. Popular algorithms include:
- Linear Regression
- Polynomial Regression
- Ridge and Lasso Regression
- Key Characteristics:
- Requires a large amount of labeled data for training.
- Performance can be evaluated using metrics like accuracy, precision, recall, and F1 score.
- Commonly used in applications such as spam detection, sentiment analysis, and image recognition.
- machine learning and classification
At Rapid Innovation, we leverage supervised learning techniques to help clients enhance their decision-making processes. For instance, by implementing classification algorithms, we can assist businesses in automating customer support through effective spam detection systems, leading to improved customer satisfaction and reduced operational costs. This includes using supervised machine learning to optimize various business functions. Additionally, our expertise in adaptive AI development allows us to create tailored solutions that evolve with changing data and business needs.
3.2.2. Unsupervised Learning Approaches
Unsupervised learning is a type of machine learning that deals with unlabeled data. The model tries to learn the underlying structure of the data without any explicit guidance on what the output should be. This approach is particularly useful for exploratory data analysis and pattern recognition.
- Clustering: This technique groups similar data points together. Common algorithms include:
- K-Means Clustering
- Hierarchical Clustering
- DBSCAN (Density-Based Spatial Clustering of Applications with Noise)
- clustering machine learning
- example of unsupervised machine learning
- Dimensionality Reduction: This involves reducing the number of features in a dataset while preserving its essential characteristics. Popular methods include:
- Principal Component Analysis (PCA)
- t-Distributed Stochastic Neighbor Embedding (t-SNE)
- Autoencoders
- Key Characteristics:
- Does not require labeled data, making it suitable for large datasets where labeling is impractical.
- Useful for discovering hidden patterns and insights in data.
- Commonly applied in market segmentation, anomaly detection, and recommendation systems.
- non supervised machine learning
- methods of machine learning
At Rapid Innovation, we utilize unsupervised learning approaches to uncover valuable insights from complex datasets. For example, through clustering techniques, we can help businesses identify distinct customer segments, enabling targeted marketing strategies that drive higher engagement and conversion rates. This includes methods in machine learning to enhance our analytical capabilities.
3.2.3. Reinforcement Learning
Reinforcement learning (RL) is a type of machine learning where an agent learns to make decisions by interacting with an environment. The agent receives feedback in the form of rewards or penalties based on its actions, allowing it to learn optimal strategies over time.
- Key Components:
- Agent: The learner or decision-maker.
- Environment: The context in which the agent operates.
- Actions: The choices available to the agent.
- Rewards: Feedback received after taking an action, guiding the learning process.
- Learning Process:
- The agent explores the environment and takes actions.
- It receives rewards or penalties based on the outcomes of its actions.
- The agent updates its strategy to maximize cumulative rewards over time.
- Key Characteristics:
- Suitable for problems where the optimal solution is not known in advance.
- Commonly used in applications such as game playing (e.g., AlphaGo), robotics, and autonomous vehicles.
- Involves concepts like exploration vs. exploitation, where the agent must balance trying new actions and leveraging known successful actions.
Rapid Innovation employs reinforcement learning to develop adaptive systems that can optimize operations in real-time. For instance, in supply chain management, our RL solutions can dynamically adjust inventory levels based on demand fluctuations, ultimately leading to cost savings and improved service levels.
By integrating these advanced machine learning techniques, including ensemble learning and feature engineering for machine learning, Rapid Innovation empowers clients to achieve their business goals efficiently and effectively, driving greater ROI through data-driven decision-making.
3.3. Data Processing and Feature Engineering
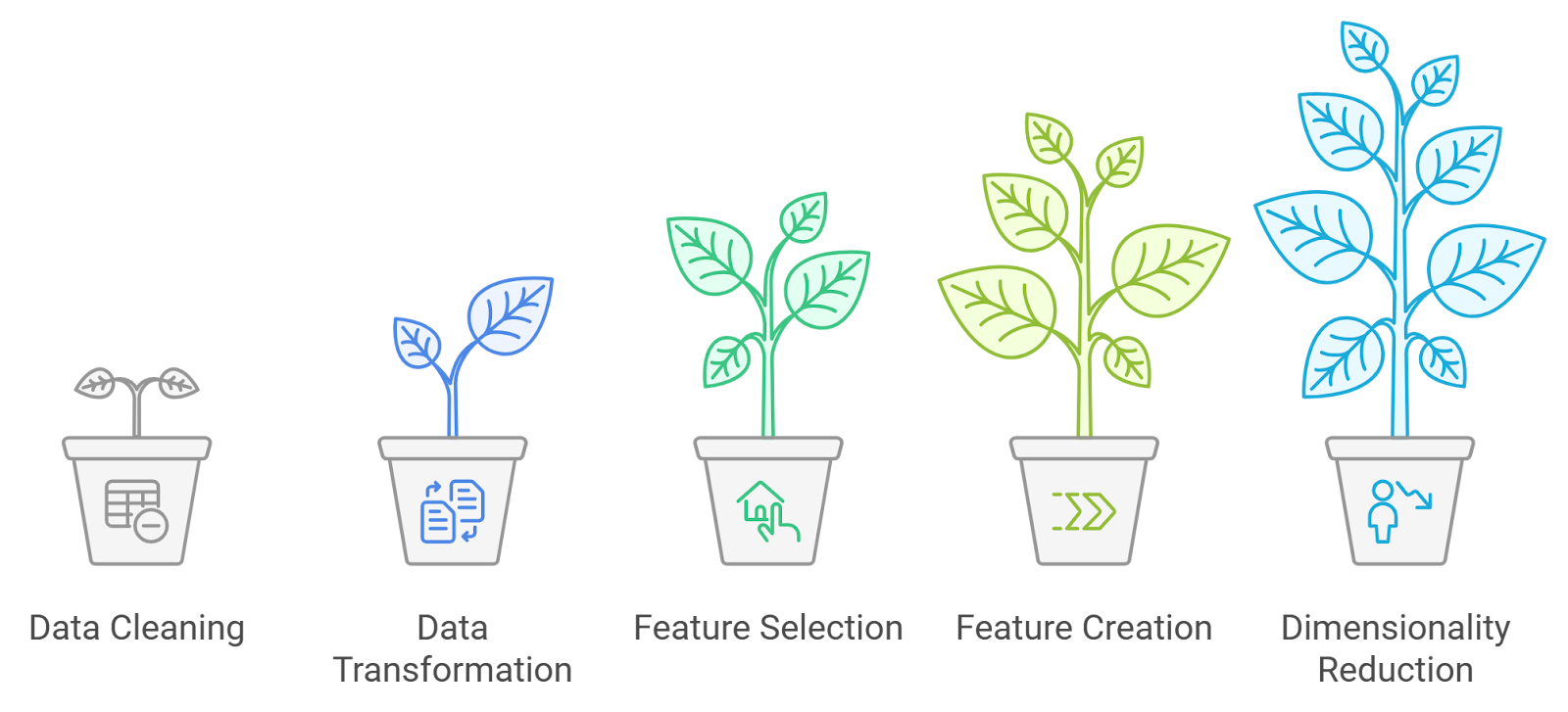
Data processing and feature engineering are critical steps in the machine learning pipeline. They ensure that the data used for training models is clean, relevant, and structured in a way that enhances model performance. Techniques such as data cleaning and feature engineering in data science are essential for achieving this goal.
- Data Cleaning: Remove duplicates, handle missing values, and correct inconsistencies. Techniques like imputation or deletion can be employed based on the context of the data. Data cleaning and feature engineering are often intertwined, as clean data is a prerequisite for effective feature engineering.
- Data Transformation: Normalize or standardize data to bring all features to a similar scale. Convert categorical variables into numerical formats using techniques like one-hot encoding or label encoding. This step is crucial in data preprocessing and feature engineering to ensure that the model can learn effectively.
- Feature Selection: Identify and select the most relevant features that contribute to the predictive power of the model. Techniques such as Recursive Feature Elimination (RFE) or using algorithms like Random Forest can help in this process. Feature engineering in data analytics often involves selecting features that enhance model performance.
- Feature Creation: Generate new features from existing data to capture additional information. Examples include creating interaction terms, polynomial features, or aggregating data over time. This is a key aspect of feature engineering for data science, as it allows for the extraction of meaningful insights from raw data.
- Dimensionality Reduction: Use techniques like Principal Component Analysis (PCA) to reduce the number of features while retaining essential information. This helps in improving model performance and reducing overfitting. Dimensionality reduction is often a part of the broader data feature engineering process.
Effective data processing and feature engineering can significantly enhance the accuracy and efficiency of machine learning models, making them more robust in real-world applications. At Rapid Innovation, we leverage these techniques, including exploratory data analysis and feature engineering, to ensure that our clients' AI models deliver optimal performance, ultimately leading to greater ROI. For expert guidance in this area, consider our MLOps consulting services and learn about the critical role of data quality in AI implementations.
3.4. Real-time Decision Making Frameworks
Real-time decision-making frameworks are essential for applications that require immediate responses based on incoming data. These frameworks leverage advanced analytics and machine learning to provide timely insights.
- Stream Processing: Utilize technologies like Apache Kafka or Apache Flink to process data streams in real-time. This allows for the continuous ingestion and analysis of data as it arrives.
- Event-Driven Architecture: Implement an event-driven model where actions are triggered by specific events or conditions. This architecture supports scalability and responsiveness in decision-making processes.
- Machine Learning Integration: Integrate machine learning models that can make predictions on-the-fly based on real-time data. This enables businesses to adapt quickly to changing conditions and make informed decisions.
- Feedback Loops: Establish feedback mechanisms to continuously improve decision-making processes. Collect data on the outcomes of decisions to refine models and strategies over time.
- Visualization Tools: Use dashboards and visualization tools to present real-time data insights. This aids stakeholders in understanding trends and making informed decisions quickly.
Real-time decision-making frameworks are crucial for industries such as finance, healthcare, and e-commerce, where timely insights can lead to competitive advantages. Rapid Innovation specializes in developing these frameworks, ensuring that our clients can respond swiftly to market changes and enhance their operational efficiency.
4. Predictive Maintenance Capabilities

Predictive maintenance capabilities leverage data analytics and machine learning to anticipate equipment failures before they occur. This proactive approach can save costs and improve operational efficiency.
- Data Collection: Gather data from various sources, including sensors, historical maintenance records, and operational logs. This data serves as the foundation for predictive analytics.
- Condition Monitoring: Continuously monitor the condition of equipment using IoT devices and sensors. This real-time data helps in identifying anomalies and potential failures.
- Predictive Analytics: Use statistical models and machine learning algorithms to analyze historical data and predict future failures. Techniques like regression analysis, time series forecasting, and classification can be employed.
- Maintenance Scheduling: Develop optimized maintenance schedules based on predictive insights. This minimizes downtime and extends the lifespan of equipment.
- Cost Reduction: By anticipating failures, organizations can reduce unplanned downtime and maintenance costs. Predictive maintenance can lead to significant savings, with some studies suggesting reductions of up to 30% in maintenance costs.
- Enhanced Safety: Predictive maintenance contributes to a safer working environment by preventing equipment failures that could lead to accidents. Regular monitoring and timely interventions ensure that machinery operates within safe parameters.
Predictive maintenance capabilities are transforming industries by enabling organizations to shift from reactive to proactive maintenance strategies, ultimately leading to improved reliability and efficiency. At Rapid Innovation, we empower our clients to implement these capabilities, driving down costs and enhancing operational performance.
4.1. Failure Prediction Algorithms
Failure prediction algorithms are essential tools in predictive maintenance, enabling organizations to anticipate equipment failures before they occur. These algorithms analyze historical data and real-time sensor readings to identify patterns that may indicate impending failures.
- Machine Learning Techniques:
- Supervised learning methods, such as decision trees and support vector machines, are commonly used to classify equipment states and predict failures.
- Unsupervised learning techniques, like clustering, help identify anomalies in operational data that could signal potential issues.
- Data Sources:
- Sensor data from machinery, operational logs, and maintenance records are crucial inputs for these algorithms.
- The integration of IoT devices enhances data collection, providing real-time insights into equipment health, particularly in the context of IoT predictive maintenance.
- Benefits:
- Reduces unplanned downtime by allowing for timely interventions.
- Optimizes maintenance schedules, leading to cost savings and improved resource allocation.
At Rapid Innovation, we leverage these failure prediction algorithms to help our clients minimize operational disruptions and maximize their return on investment (ROI). By implementing tailored predictive maintenance solutions, including custom AI model development, we enable organizations to transition from reactive to proactive maintenance strategies, ultimately enhancing their operational efficiency.
4.2. Remaining Useful Life (RUL) Estimation
Remaining Useful Life (RUL) estimation is a critical aspect of predictive maintenance, providing insights into how much longer a piece of equipment can operate effectively before it requires maintenance or replacement.
- Techniques for RUL Estimation:
- Statistical methods, such as survival analysis, help estimate the lifespan of equipment based on historical failure data.
- Machine learning approaches, including regression models and deep learning, can predict RUL by analyzing complex patterns in data.
- Importance of RUL:
- Enables proactive maintenance planning, reducing the risk of unexpected failures.
- Helps in inventory management by predicting when spare parts will be needed.
- Applications:
- Widely used in industries such as aerospace, manufacturing, and energy, where equipment reliability is critical.
- RUL estimation can also enhance safety by ensuring that equipment is maintained before it reaches a critical failure point.
At Rapid Innovation, our expertise in RUL estimation allows us to provide clients with actionable insights that facilitate better maintenance planning and resource allocation. By accurately predicting when equipment will require attention, we help organizations avoid costly downtime and improve their overall productivity, leveraging solutions from companies like AWS predictive maintenance and SAP predictive maintenance.
4.3. Performance Degradation Modeling
Performance degradation modeling focuses on understanding how equipment performance declines over time due to wear and tear, environmental factors, and operational stresses. This modeling is vital for effective maintenance strategies and operational efficiency.
- Key Components of Degradation Models:
- Physical models simulate the wear mechanisms affecting equipment, providing insights into how different factors contribute to performance loss.
- Data-driven models leverage historical performance data to identify trends and predict future degradation.
- Benefits of Degradation Modeling:
- Helps organizations schedule maintenance activities based on actual performance rather than arbitrary timelines.
- Informs design improvements by identifying failure modes and their causes, leading to more robust equipment.
- Implementation:
- Requires continuous monitoring of equipment performance through sensors and data analytics.
- Collaboration between engineering teams and data scientists is essential to develop effective models that accurately reflect real-world conditions.
By utilizing performance degradation modeling, Rapid Innovation empowers clients to make informed decisions regarding maintenance and equipment upgrades. Our data-driven approach not only enhances operational efficiency but also contributes to significant cost savings, ultimately driving greater ROI for our clients through industrial predictive maintenance and predictive maintenance platforms.
4.4. Anomaly Detection Techniques
Anomaly detection is a critical aspect of data analysis, particularly in fields such as cybersecurity, finance, and healthcare. It involves identifying patterns in data that do not conform to expected behavior. Here are some common techniques used for anomaly detection:
- Statistical Methods: These techniques rely on statistical tests to identify outliers. Common methods include Z-score analysis and the Grubbs' test. They are effective for normally distributed data but may struggle with non-linear patterns. Statistical anomaly detection is often used in various applications, including outlier detection in time series data.
- Machine Learning Approaches:
- Supervised Learning: Involves training a model on labeled data to classify normal and anomalous instances. Algorithms like Support Vector Machines (SVM) and Decision Trees are often used. Anomaly detection using machine learning has gained popularity due to its effectiveness in various domains.
- Unsupervised Learning: Techniques such as clustering (e.g., K-means) and dimensionality reduction (e.g., PCA) help identify anomalies without labeled data. Scikit-learn outlier detection methods are commonly employed in this context.
- Deep Learning Techniques: Neural networks, particularly autoencoders, can learn complex patterns in data and identify anomalies by reconstructing input data. Recurrent Neural Networks (RNNs) are useful for time-series data, detecting anomalies based on temporal patterns. Neural network anomaly detection has shown promising results in various applications.
- Hybrid Methods: Combining multiple techniques can enhance detection accuracy. For instance, using statistical methods to filter data before applying machine learning algorithms can improve results. This approach is often seen in anomaly detection techniques that leverage both statistical and machine learning methods.
- Domain-Specific Techniques: Certain industries may require tailored approaches. For example, in finance, techniques like the Isolation Forest algorithm are popular for detecting fraudulent transactions. Network traffic anomaly detection is another area where specialized techniques are applied. For a deeper understanding of these methods, you can refer to this introduction to AI anomaly detection.
5. Implementation Strategies
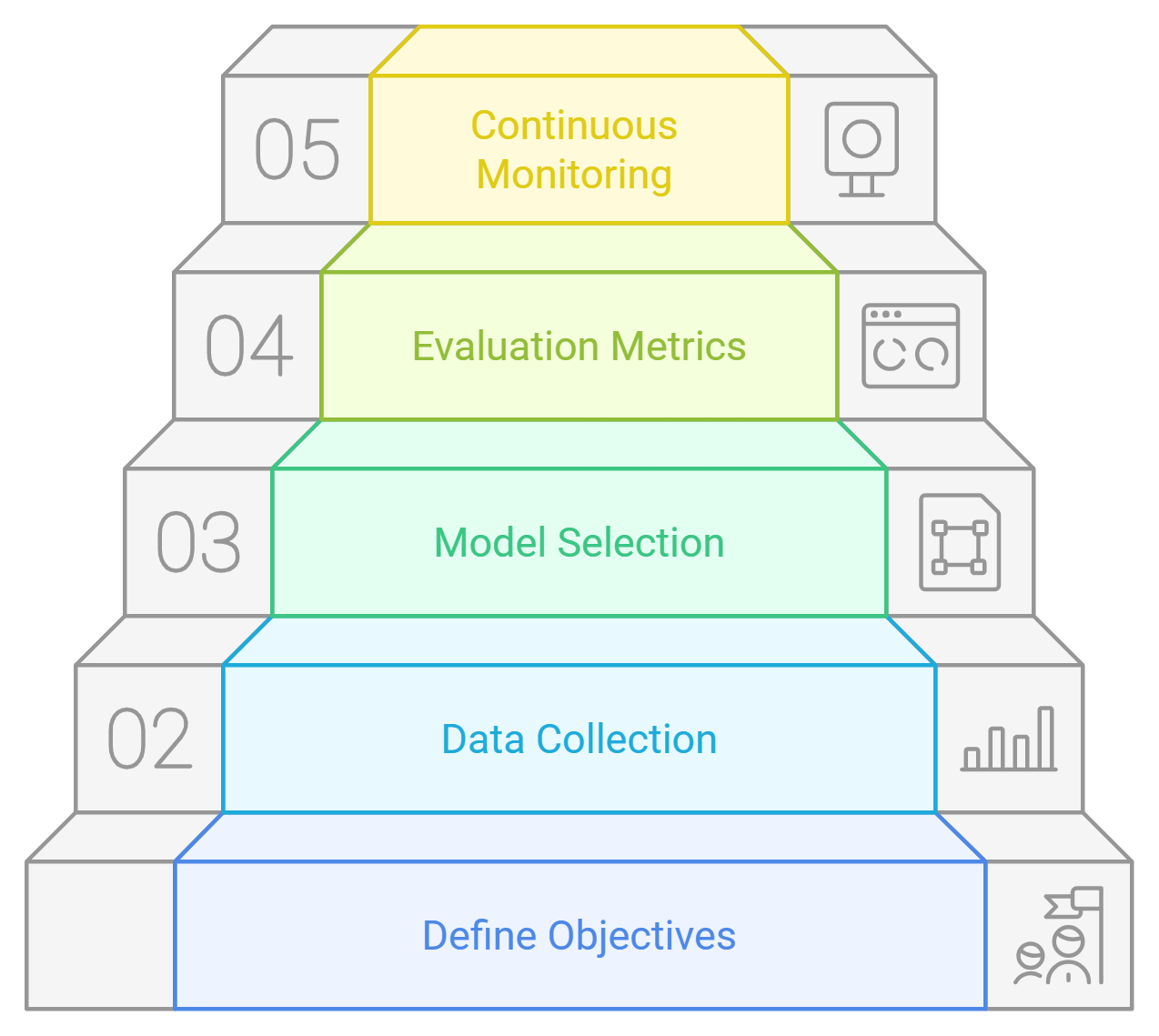
Implementing anomaly detection systems requires careful planning and execution. Here are key strategies to consider:
- Define Objectives: Clearly outline the goals of the anomaly detection system. This could include reducing false positives, improving detection speed, or enhancing overall accuracy.
- Data Collection and Preparation: Gather relevant data from various sources. Ensure data quality by cleaning and preprocessing it to remove noise and irrelevant information. Normalize and standardize data to ensure consistency across different datasets. Anomaly detection in data mining often involves extensive data preparation.
- Model Selection: Choose the appropriate anomaly detection technique based on the nature of the data and the specific use case. Consider factors such as the volume of data, the complexity of patterns, and the computational resources available. Outlier detection algorithms can vary significantly based on these factors.
- Evaluation Metrics: Establish metrics to evaluate the performance of the anomaly detection system. Common metrics include precision, recall, F1-score, and area under the ROC curve (AUC-ROC). Regularly assess the model's performance and make adjustments as necessary.
- Continuous Monitoring and Maintenance: Anomaly detection is not a one-time task. Continuously monitor the system to adapt to new patterns and changes in data. Implement feedback loops to refine the model based on real-world performance. Anomaly detection for data quality is an ongoing process that requires vigilance.
5.1. Infrastructure Requirements
Setting up an effective anomaly detection system requires a robust infrastructure. Here are the essential components:
- Hardware Requirements: High-performance servers or cloud-based solutions are necessary to handle large datasets and complex computations. Consider using GPUs for deep learning models, as they significantly speed up training and inference times.
- Software Tools: Utilize programming languages and libraries suited for data analysis, such as Python with libraries like Scikit-learn, TensorFlow, or PyTorch. Data visualization tools (e.g., Tableau, Power BI) can help in understanding anomalies and presenting findings. Python outlier detection time series libraries are particularly useful for time-series analysis.
- Data Storage Solutions: Choose appropriate storage solutions based on data volume and access speed. Options include relational databases, NoSQL databases, or data lakes. Ensure that the storage solution can scale as data grows.
- Network Infrastructure: A reliable and fast network is crucial for transferring large datasets, especially in real-time anomaly detection scenarios. Implement security measures to protect sensitive data during transmission.
- Integration Capabilities: Ensure that the anomaly detection system can integrate with existing systems and workflows. This may involve APIs or middleware solutions. Consider compatibility with data sources, such as IoT devices, databases, or cloud services.
- Compliance and Security: Adhere to industry regulations regarding data privacy and security. Implement encryption and access controls to protect sensitive information. Regularly audit the system for vulnerabilities and compliance with standards.
By focusing on these infrastructure requirements, organizations can build a robust anomaly detection system that effectively identifies and responds to unusual patterns in data. At Rapid Innovation, we leverage our expertise in AI and Blockchain to help clients implement these systems efficiently, ensuring they achieve greater ROI through enhanced data insights and security measures.
5.2. Data Collection and Preparation
Data collection and preparation are critical steps in any data-driven project, particularly in machine learning and artificial intelligence. The quality and relevance of the data directly influence the performance of the model. This phase often involves data preparation for machine learning, which includes various techniques and methodologies.
- Identify the data sources:
- Internal databases
- Public datasets
- APIs from third-party services
- Data cleaning:
- Remove duplicates
- Handle missing values
- Correct inconsistencies in data formats
- Data transformation:
- Normalize or standardize numerical data
- Encode categorical variables
- Create new features through feature engineering
- Data splitting:
- Divide the dataset into training, validation, and test sets
- Common ratios include 70% training, 15% validation, and 15% test
- Documentation:
- Maintain clear records of data sources and transformations
- Ensure reproducibility for future analysis
Proper data collection and preparation set the foundation for effective model training and validation. According to a study, up to 80% of the time spent on data science projects is dedicated to data preparation steps for machine learning. At Rapid Innovation, we emphasize the importance of this phase, ensuring that our clients have high-quality data that leads to better model performance and, ultimately, greater ROI. For those interested in advanced techniques, our how to build an AI app: a step-by-step guide can provide significant enhancements.
5.3. Model Training and Validation
Model training and validation are essential for developing a robust machine learning model. This phase involves selecting the right algorithms, tuning hyperparameters, and evaluating model performance.
- Algorithm selection:
- Choose algorithms based on the problem type (e.g., classification, regression)
- Consider popular algorithms like decision trees, support vector machines, or neural networks
- Hyperparameter tuning:
- Use techniques like grid search or random search to find optimal hyperparameters
- Cross-validation can help assess model performance on different subsets of data
- Performance metrics:
- Use metrics such as accuracy, precision, recall, F1 score, and ROC-AUC for evaluation
- Choose metrics that align with business objectives
- Overfitting and underfitting:
- Monitor for overfitting by comparing training and validation performance
- Use techniques like regularization or dropout to mitigate overfitting
- Iterative process:
- Model training is often iterative; refine the model based on validation results
- Continuously assess and improve the model until satisfactory performance is achieved
Effective model training and validation can significantly enhance predictive accuracy. Research indicates that well-tuned models can improve performance by up to 30%. At Rapid Innovation, we leverage our expertise to ensure that our clients' models are not only accurate but also aligned with their business goals, maximizing their return on investment.
5.4. Deployment Considerations
Deployment is the final step in the machine learning lifecycle, where the trained model is integrated into a production environment. This phase requires careful planning to ensure the model operates effectively and efficiently.
- Environment setup:
- Choose between cloud-based or on-premises deployment
- Ensure compatibility with existing systems and infrastructure
- Monitoring and maintenance:
- Implement monitoring tools to track model performance in real-time
- Set up alerts for performance degradation or anomalies
- Scalability:
- Design the deployment architecture to handle varying loads
- Consider using containerization technologies like Docker for scalability
- Security:
- Ensure data privacy and compliance with regulations (e.g., GDPR)
- Implement authentication and authorization measures to protect sensitive data
- User feedback:
- Collect feedback from end-users to identify areas for improvement
- Use this feedback to iterate on the model and enhance its performance
Deployment is a crucial phase that can determine the success of a machine learning project. According to a report, 70% of machine learning models never make it to production due to deployment challenges. Rapid Innovation addresses these challenges head-on, ensuring that our clients' models are successfully deployed and maintained, leading to sustained business value and improved ROI.
5.5. Continuous Learning and Adaptation
Continuous learning and adaptation are crucial in today’s fast-paced environment, especially in industries driven by technology and innovation. Organizations must foster a culture that encourages ongoing education and flexibility to stay competitive. This includes:
- Emphasizing the importance of lifelong learning for employees.
- Encouraging organizations to invest in training programs and workshops, particularly in AI and Blockchain technologies.
- Promoting the use of online courses and certifications to enhance skills relevant to emerging technologies.
- Supporting the idea of learning from failures and successes alike, particularly in the context of innovative projects.
- Advocating for feedback mechanisms to improve processes and strategies, ensuring alignment with industry best practices.
- Highlighting the role of mentorship and peer learning in professional growth, especially in complex fields like AI and Blockchain.
- Stressing the need for adaptability in response to market changes and technological advancements.
By prioritizing continuous learning and adaptation, companies can ensure their workforce remains skilled and knowledgeable, ready to tackle new challenges and seize opportunities, ultimately leading to greater ROI.
6. Industry-Specific Applications
Industry-specific applications refer to tailored solutions designed to meet the unique needs of various sectors. These applications leverage technology to enhance efficiency, productivity, and overall performance. Key aspects include:
- Focusing on the unique challenges and requirements of each industry.
- Utilizing specialized software and tools, including AI-driven analytics and Blockchain solutions, to address specific operational needs.
- Enhancing decision-making through data analytics and insights derived from advanced technologies.
- Improving customer engagement and satisfaction with targeted solutions that leverage AI for personalization.
- Streamlining processes to reduce costs and increase profitability through automation and smart contracts in Blockchain.
- Fostering innovation by integrating emerging technologies, ensuring businesses remain competitive.
Understanding the nuances of each industry allows businesses to implement effective strategies that drive growth and success.
6.1. Manufacturing Maintenance
Manufacturing maintenance is a critical aspect of the production process, ensuring that machinery and equipment operate efficiently and reliably. Effective maintenance strategies can significantly reduce downtime and enhance productivity. This involves:
- Conducting regular inspections and preventive maintenance to avoid breakdowns.
- Utilizing predictive maintenance techniques powered by AI to anticipate equipment failures.
- Incorporating the Internet of Things (IoT) for real-time monitoring of machinery, enhancing operational visibility.
- Enhancing safety protocols to protect workers and equipment through data-driven insights.
- Reducing operational costs by minimizing unplanned downtime with proactive maintenance strategies.
- Supporting sustainability efforts through efficient resource management and waste reduction.
- Encouraging employee training on maintenance best practices, particularly in the context of new technologies.
By implementing robust manufacturing maintenance strategies, companies can optimize their operations, extend the lifespan of their equipment, and ultimately improve their bottom line, driving greater ROI through efficiency and innovation.
6.2. Energy and Utilities
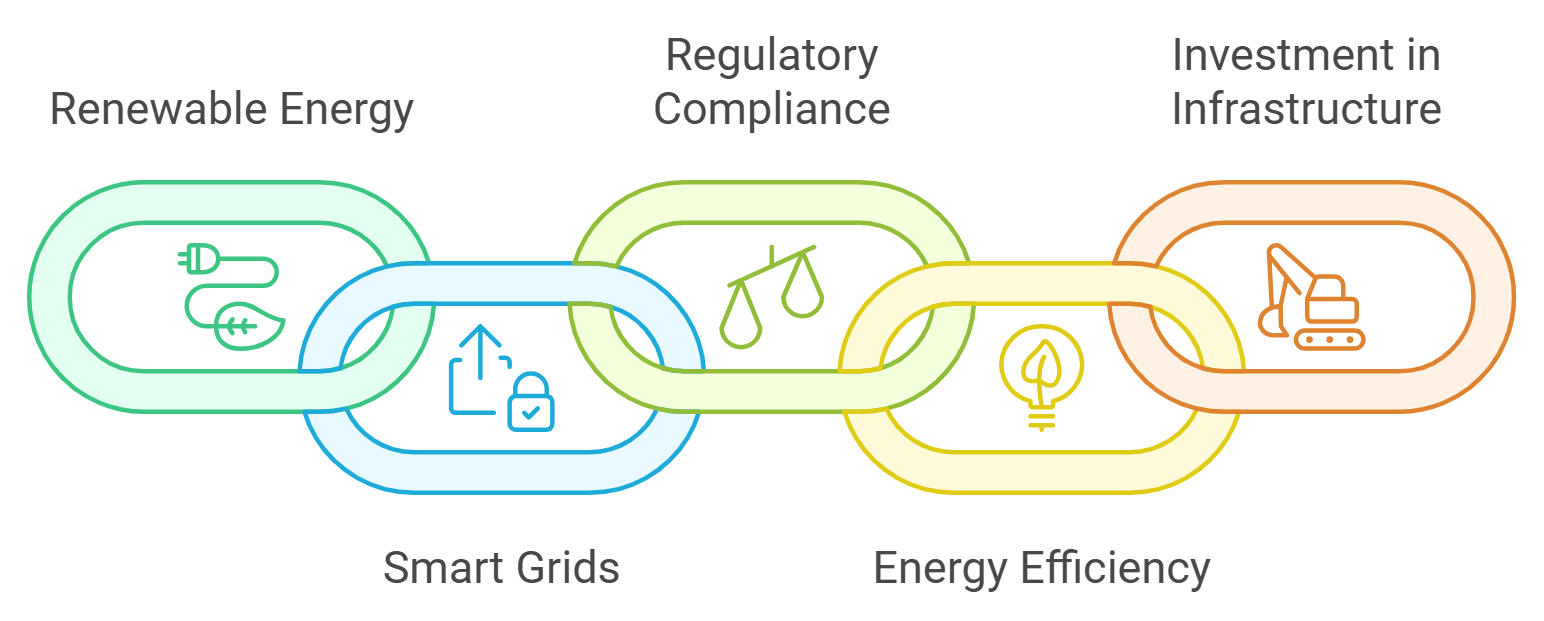
The energy and utilities sector is a critical component of the global economy, providing essential services that power homes, businesses, and industries. This sector encompasses a wide range of activities, including electricity generation, water supply, natural gas distribution, and waste management.
- Renewable Energy: The shift towards renewable energy sources, such as solar, wind, and hydroelectric power, is transforming the energy landscape. This transition is driven by the need for sustainable practices and reducing carbon emissions. Rapid Innovation leverages AI algorithms to optimize energy production and consumption, enabling clients to maximize their investments in renewable technologies.
- Smart Grids: The implementation of smart grid technology enhances the efficiency and reliability of energy distribution. Smart grids utilize digital communication to monitor and manage energy flows, leading to reduced outages and improved service delivery. Our blockchain solutions can facilitate secure data sharing among stakeholders, ensuring transparency and accountability in energy transactions.
- Regulatory Compliance: Energy and utility companies, such as Consumers Energy Company, Atmos Energy Corp, and FirstEnergy, must navigate complex regulatory environments. Compliance with environmental regulations and safety standards is crucial for operational success and public trust. Rapid Innovation provides consulting services to help clients implement AI-driven compliance monitoring systems, reducing the risk of non-compliance and associated penalties.
- Energy Efficiency: Increasing energy efficiency is a priority for both consumers and businesses. Implementing energy-saving technologies and practices can lead to significant cost savings and reduced environmental impact. Our AI solutions analyze consumption patterns, providing actionable insights that help clients optimize their energy usage and lower operational costs. Companies like Nevada Energy and Philadelphia Electric Company are leading the way in promoting energy efficiency initiatives.
- Investment in Infrastructure: Aging infrastructure poses challenges for the energy sector. Investment in modernizing facilities and expanding capacity is essential to meet growing demand and ensure reliable service. Rapid Innovation assists clients in developing blockchain-based asset management systems that enhance the tracking and maintenance of infrastructure, ultimately leading to improved ROI. Utilities such as West Penn Power Company and Piedmont Natural Gas Company are also focusing on infrastructure improvements to better serve their customers.
6.3. Transportation and Logistics
Transportation and logistics play a vital role in the global supply chain, facilitating the movement of goods and services across various modes of transport. This sector is essential for economic growth and development, impacting everything from local businesses to international trade.
- Supply Chain Optimization: Efficient logistics management is key to reducing costs and improving service delivery. Companies are increasingly adopting technology solutions, such as inventory management systems and route optimization software, to streamline operations. Rapid Innovation employs AI to analyze logistics data, enabling clients to make informed decisions that enhance efficiency and reduce expenses.
- E-commerce Growth: The rise of e-commerce has transformed transportation and logistics. Businesses must adapt to the demand for faster delivery times and improved customer service, leading to innovations in last-mile delivery solutions. Our blockchain technology ensures secure and transparent transactions, fostering trust between e-commerce platforms and logistics providers.
- Sustainability Initiatives: The transportation sector is under pressure to reduce its carbon footprint. Companies are exploring alternative fuels, electric vehicles, and sustainable packaging to minimize environmental impact. Rapid Innovation supports clients in implementing AI-driven sustainability strategies that optimize resource usage and reduce emissions.
- Global Trade Dynamics: Changes in trade policies and international relations can significantly affect transportation and logistics. Companies must stay informed about tariffs, trade agreements, and geopolitical developments to navigate these challenges effectively. Our consulting services provide clients with insights and strategies to adapt to evolving global trade landscapes.
- Technology Integration: The use of technology, such as IoT and blockchain, is revolutionizing logistics. These technologies enhance visibility, traceability, and security throughout the supply chain, leading to improved efficiency and reduced risks. Rapid Innovation specializes in integrating these technologies into existing logistics frameworks, ensuring clients remain competitive in a rapidly changing market.
6.4. Healthcare Equipment Management
Healthcare equipment management is a crucial aspect of the healthcare industry, ensuring that medical devices and equipment are available, safe, and effective for patient care. Proper management of healthcare equipment is essential for maintaining high standards of care and operational efficiency.
- Asset Tracking: Effective tracking of medical equipment is vital for optimizing usage and minimizing loss. Implementing asset management systems can help healthcare facilities monitor equipment location, usage, and maintenance schedules. Rapid Innovation utilizes AI to enhance asset tracking capabilities, ensuring that healthcare providers can efficiently manage their resources.
- Preventive Maintenance: Regular maintenance of medical equipment is essential to ensure reliability and compliance with safety standards. Preventive maintenance programs can reduce downtime and extend the lifespan of equipment. Our solutions leverage AI to predict maintenance needs, allowing healthcare facilities to proactively address issues before they impact patient care.
- Regulatory Compliance: Healthcare facilities must adhere to strict regulations regarding equipment safety and efficacy. Compliance with standards set by organizations such as the FDA and ISO is critical for patient safety and operational integrity. Rapid Innovation offers consulting services to help clients navigate regulatory requirements and implement compliance monitoring systems.
- Training and Education: Staff training on the proper use and maintenance of medical equipment is essential. Ongoing education ensures that healthcare professionals are knowledgeable about the latest technologies and best practices. We provide tailored training programs that incorporate AI-driven simulations, enhancing staff competency and confidence.
- Cost Management: Managing the costs associated with healthcare equipment is a significant challenge. Facilities must balance the need for advanced technology with budget constraints, often requiring strategic planning and investment in cost-effective solutions. Rapid Innovation assists clients in developing financial models that optimize equipment investments, ensuring sustainable growth and improved ROI.
6.5. Smart Building Maintenance
Smart building maintenance refers to the use of advanced technologies and data analytics to enhance the upkeep and management of buildings. This approach not only improves operational efficiency but also extends the lifespan of building systems and reduces costs.
- Predictive Maintenance:
- Utilizes IoT sensors to monitor equipment health in real-time.
- Predicts failures before they occur, allowing for timely interventions.
- Reduces downtime and maintenance costs, ultimately leading to a higher ROI for building owners. This is a key aspect of predictive maintenance smart buildings.
- Automated Systems:
- Employs automation for HVAC, lighting, and security systems.
- Enhances energy efficiency and occupant comfort, which can lead to increased tenant satisfaction and retention.
- Minimizes human error in maintenance tasks, ensuring consistent performance and reliability.
- Data-Driven Insights:
- Analyzes data collected from various building systems.
- Identifies patterns and trends for better decision-making, enabling building managers to allocate resources more effectively.
- Supports proactive maintenance strategies that can prevent costly repairs and extend the life of equipment.
- Sustainability:
- Integrates green technologies to reduce environmental impact, aligning with corporate sustainability goals.
- Monitors energy consumption and waste management, providing insights that can lead to significant cost savings.
- Promotes sustainable practices within building operations, enhancing the building's marketability.
- Enhanced Safety and Security:
- Implements smart surveillance and access control systems.
- Ensures a safer environment for occupants, which can reduce liability and insurance costs.
- Provides real-time alerts for any security breaches, enhancing overall building security.
- Cost Efficiency:
- Reduces operational costs through optimized resource management, allowing for better budget allocation.
- Lowers energy bills by improving system performance, contributing to a more favorable bottom line.
- Extends the life of building assets through regular monitoring, reducing the need for premature replacements.
7. Performance Metrics and Evaluation
Performance metrics and evaluation are essential for assessing the effectiveness of building operations and maintenance strategies. By establishing clear metrics, building managers can make informed decisions that enhance performance and sustainability.
- Importance of Performance Metrics:
- Provides a framework for measuring success.
- Identifies areas for improvement in building operations.
- Facilitates accountability among staff and stakeholders.
- Types of Performance Metrics:
- Operational Efficiency: Measures how effectively resources are utilized.
- Energy Consumption: Tracks energy usage to identify savings opportunities.
- Occupant Satisfaction: Assesses the comfort and satisfaction levels of building occupants.
- Data Collection Methods:
- Utilizes smart sensors and IoT devices for real-time data gathering.
- Employs surveys and feedback tools to gauge occupant experiences.
- Analyzes historical data for trend identification.
- Continuous Improvement:
- Encourages regular reviews of performance metrics.
- Implements changes based on data-driven insights.
- Fosters a culture of ongoing enhancement in building management.
7.1. Key Performance Indicators (KPIs)

Key Performance Indicators (KPIs) are specific metrics used to evaluate the success of building operations and maintenance. They provide quantifiable measures that help in tracking progress toward strategic goals.
- Energy Efficiency KPIs:
- Energy Use Intensity (EUI): Measures energy consumption per square foot.
- Carbon Footprint: Assesses the total greenhouse gas emissions.
- Renewable Energy Usage: Tracks the percentage of energy sourced from renewables.
- Operational KPIs:
- Maintenance Response Time: Measures the time taken to address maintenance requests.
- Equipment Downtime: Tracks the duration of equipment failures.
- Cost per Square Foot: Evaluates operational costs relative to building size.
- Occupant Experience KPIs:
- Occupant Satisfaction Score: Gathers feedback on comfort and amenities.
- Indoor Air Quality Index: Measures air quality levels within the building.
- Space Utilization Rate: Assesses how effectively spaces are used.
- Benchmarking:
- Compares KPIs against industry standards or similar buildings.
- Identifies best practices and areas for improvement.
- Supports strategic planning and resource allocation.
- Reporting and Visualization:
- Utilizes dashboards to present KPI data clearly.
- Facilitates easy interpretation of performance metrics.
- Enhances communication with stakeholders regarding building performance.
By focusing on smart building maintenance and performance metrics, building managers can create more efficient, sustainable, and occupant-friendly environments, ultimately driving greater ROI and operational success. Rapid Innovation is well-positioned to assist clients in implementing these advanced solutions, leveraging our expertise in AI and blockchain technologies to optimize building management processes, particularly in the realm of smart building predictive maintenance.
7.2. Economic Impact Assessment
Economic impact assessment (EIA) is a systematic approach to evaluating the economic effects of a project, policy, or program. It helps stakeholders understand the potential benefits and costs associated with an initiative, enabling them to make informed decisions that align with their business goals.
- Purpose of EIA:
- To gauge the overall economic benefits to a community or region.
- To identify potential job creation, income generation, and tax revenue increases.
- To assess the long-term sustainability of economic benefits.
- Key Components:
- Direct Impacts: Immediate effects on employment, income, and production.
- Indirect Impacts: Secondary effects resulting from the spending of income earned from direct impacts.
- Induced Impacts: Economic effects from increased household spending due to direct and indirect impacts.
- Methodologies:
- Input-output models to analyze inter-industry relationships.
- Cost-benefit analysis to compare the economic advantages and disadvantages.
- Economic multipliers to estimate the ripple effects of spending.
- Importance:
- Informs decision-makers about the viability of projects.
- Helps in resource allocation and prioritization of initiatives.
- Engages stakeholders by providing transparent economic forecasts.
At Rapid Innovation, we leverage advanced AI algorithms to enhance the accuracy of EIA, ensuring that our clients can anticipate the economic outcomes of their initiatives with greater precision. By integrating blockchain technology, we also provide a transparent and immutable record of the assessment process, fostering trust among stakeholders.
In addition, we utilize various tools such as economic impact analysis tools and economic impact measurement techniques to provide comprehensive insights. Our economic impact evaluation processes are designed to deliver reliable results, and we often refer to economic impact analysis examples to illustrate potential outcomes.
Furthermore, we conduct socio economic impact assessments to evaluate the broader implications of projects on communities. This includes socio economic impact analysis and socio economic impact studies that help in understanding the social dimensions of economic initiatives. We also provide socio economic assessment tools to aid stakeholders in their evaluations. For precise project estimations, consider our AI project estimation services and learn more about AI agent societies and simulations.
7.3. Reliability and Accuracy Metrics
Reliability and accuracy metrics are essential for evaluating the performance of models and assessments in various fields, including economic impact assessments. These metrics ensure that the data and predictions are trustworthy and can be used for informed decision-making.
- Key Metrics:
- Precision: The degree to which repeated measurements under unchanged conditions show the same results.
- Bias: The systematic error that leads to inaccurate results, which can skew the findings.
- Confidence Intervals: A range of values that is likely to contain the true value of an estimate, providing insight into the uncertainty of the results.
- Methods to Enhance Reliability:
- Use of robust data sources and methodologies.
- Regular calibration and validation of models against real-world outcomes.
- Peer review and stakeholder feedback to identify potential flaws.
- Importance of Metrics:
- Ensures that stakeholders can trust the findings of assessments.
- Facilitates better decision-making by providing a clear understanding of the potential risks and uncertainties.
- Enhances the credibility of the assessment process, leading to greater acceptance of the results.
At Rapid Innovation, we utilize AI-driven analytics to continuously monitor and improve the reliability of our assessments, ensuring that our clients receive the most accurate and actionable insights.
7.4. Cost-Benefit Analysis
Cost-benefit analysis (CBA) is a financial assessment tool used to evaluate the economic feasibility of a project or decision by comparing its costs and benefits. It is a critical component of economic impact assessments and helps stakeholders make informed choices.
- Components of CBA:
- Identification of Costs: All direct and indirect costs associated with the project, including initial investment, operational costs, and maintenance.
- Identification of Benefits: All direct and indirect benefits, such as increased revenue, job creation, and social benefits.
- Time Value of Money: Adjusting future costs and benefits to present value using discount rates to account for inflation and opportunity costs.
- Steps in Conducting CBA:
- Define the scope and objectives of the analysis.
- Gather data on costs and benefits.
- Calculate net present value (NPV) to determine the overall value of the project.
- Perform sensitivity analysis to assess how changes in assumptions affect outcomes.
- Importance of CBA:
- Provides a clear framework for comparing different projects or policies.
- Helps prioritize investments based on economic returns.
- Supports transparency and accountability in decision-making processes.
Rapid Innovation employs sophisticated AI models to streamline the CBA process, allowing clients to quickly assess the economic viability of their projects. By integrating blockchain technology, we ensure that all data used in the analysis is secure and verifiable, enhancing the overall integrity of the assessment.
In conclusion, economic impact assessment, reliability and accuracy metrics, and cost-benefit analysis are integral components of evaluating projects and policies. They provide a structured approach to understanding the economic implications, ensuring that decisions are based on sound data and analysis. At Rapid Innovation, our expertise in AI and blockchain empowers clients to achieve greater ROI through informed decision-making and strategic planning.
8. Challenges and Limitations
In the realm of data science and machine learning, various challenges and limitations can hinder the effectiveness and reliability of models. Understanding these challenges is crucial for practitioners aiming to develop robust solutions.
8.1 Data Quality and Availability
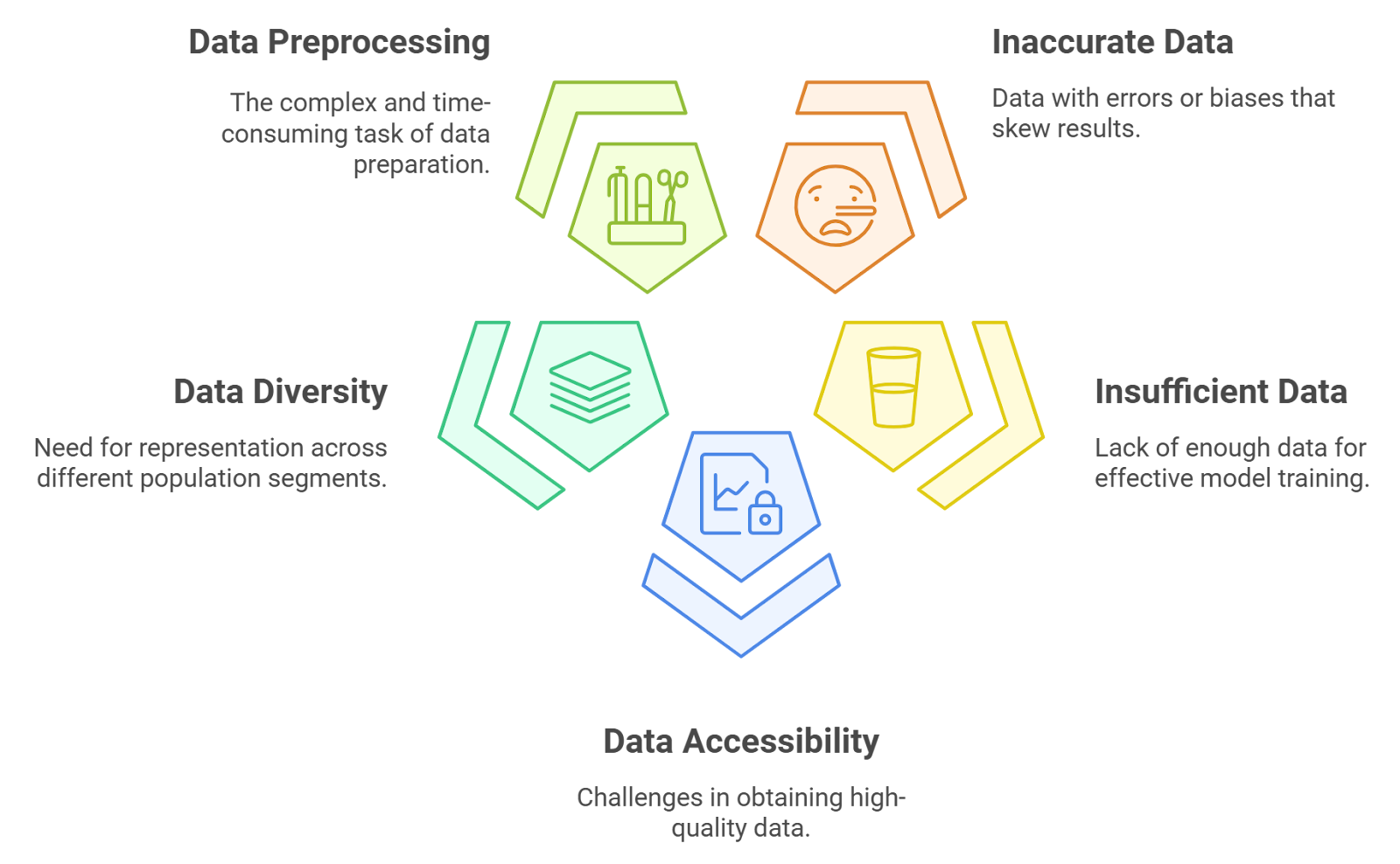
Data quality and availability are fundamental to the success of any data-driven project. Poor quality data can lead to inaccurate models and misleading insights. Issues related to data quality include:
- Inaccurate Data: Data may contain errors, inconsistencies, or biases that can skew results. For instance, if a dataset has missing values or incorrect entries, the model trained on this data may produce unreliable predictions.
- Insufficient Data: Many machine learning models require large datasets to learn effectively. In cases where data is scarce, models may overfit, meaning they perform well on training data but poorly on unseen data. This is a common issue faced in data science challenges.
- Data Accessibility: Accessing high-quality data can be a significant barrier. Organizations may face legal, ethical, or logistical challenges in obtaining the necessary data. For example, sensitive information may be restricted due to privacy laws, which can complicate challenges in data science projects.
- Data Diversity: A lack of diversity in the dataset can lead to biased models. If the training data does not represent the entire population, the model may not generalize well, resulting in poor performance in real-world applications. This is particularly relevant in common data science problems.
- Data Preprocessing: The process of cleaning and preparing data is often time-consuming and complex. Inadequate preprocessing can lead to models that do not perform as expected, which is a frequent issue in data science take home challenges.
At Rapid Innovation, we understand these challenges and offer tailored solutions to ensure data quality and availability. Our expertise in AI and data management allows us to implement robust data collection and validation processes, ultimately leading to more reliable machine learning models and greater ROI for our clients. As an AI as a Service company, we are committed to addressing these challenges effectively.
8.2 Model Interpretability
Model interpretability refers to the degree to which a human can understand the decisions made by a machine learning model. This is particularly important in fields where decisions have significant consequences, such as healthcare and finance. Key challenges related to model interpretability include:
- Complex Models: Many advanced machine learning models, such as deep learning networks, are often seen as "black boxes." Their internal workings are complex, making it difficult to understand how they arrive at specific predictions. This complexity is often encountered in data science coding challenges.
- Trust and Accountability: Stakeholders need to trust the model's predictions, especially in critical applications. If a model's decision-making process is opaque, it can lead to skepticism and reluctance to adopt the technology, which is a concern in challenges faced by data scientists.
- Regulatory Compliance: In some industries, regulations require that decisions made by algorithms be explainable. For instance, the General Data Protection Regulation (GDPR) in Europe emphasizes the right to explanation for automated decisions.
- Debugging and Improvement: Understanding how a model makes decisions is crucial for identifying errors and improving performance. Without interpretability, it can be challenging to diagnose issues or refine the model, which is a common problem in data scientist hiring tests.
- Ethical Considerations: Interpretability is also tied to ethical considerations. If a model makes biased or unfair decisions, understanding its reasoning can help identify and mitigate these issues.
To enhance model interpretability, practitioners can employ various techniques, such as using simpler models, feature importance analysis, or visualization tools. At Rapid Innovation, we prioritize model interpretability in our AI solutions, ensuring that our clients can trust and understand the decisions made by their models. This commitment not only fosters stakeholder confidence but also aligns with regulatory requirements, ultimately driving better business outcomes. Balancing model complexity with interpretability is a key challenge in the field of machine learning, and we are here to guide our clients through it, especially in the context of data science challenges for beginners and the broader landscape of data science problems in healthcare and retail.
8.3. Integration with Legacy Systems
Integrating new technologies with legacy systems is a critical challenge for many organizations. Legacy systems, often built on outdated technology, can hinder the adoption of modern solutions. However, successful integration can lead to improved efficiency and enhanced capabilities.
- Compatibility: Ensuring that new systems can communicate with legacy systems is essential. This may involve using middleware or APIs to bridge the gap, allowing for seamless data exchange and operational continuity. This is particularly relevant in the context of legacy application integration and integration of legacy platforms.
- Data Migration: Transferring data from legacy systems to new platforms can be complex. Organizations must ensure data integrity and security during this process, which is where Rapid Innovation's expertise in data management and migration strategies can significantly reduce risks and enhance reliability. Legacy data integration is a key aspect of this process.
- Incremental Upgrades: Instead of a complete overhaul, organizations can opt for incremental upgrades. This approach allows for gradual integration, minimizing disruption and enabling organizations to adapt to new technologies at a manageable pace. Mainframe modernization and integration can be part of this strategy.
- Training and Support: Employees may need training to adapt to new systems. Providing adequate support can ease the transition and improve user adoption, ensuring that teams are equipped to leverage new tools effectively. This is especially important when integrating Salesforce with legacy systems.
- Cost Considerations: Integrating legacy systems can be costly. Organizations should evaluate the return on investment (ROI) before proceeding with integration efforts. Rapid Innovation can assist in conducting thorough ROI analyses to ensure that integration efforts align with business objectives. Mulesoft legacy modernization can be a cost-effective solution in this regard.
8.4. Ethical and Security Considerations
As technology evolves, ethical and security considerations become increasingly important. Organizations must navigate these challenges to maintain trust and compliance.
- Data Privacy: Protecting user data is paramount. Organizations must adhere to regulations such as GDPR and CCPA to ensure data privacy, and Rapid Innovation can help implement robust data governance frameworks to safeguard sensitive information.
- Cybersecurity: With the rise of cyber threats, organizations must implement robust security measures. This includes regular security audits and employee training on best practices, areas where Rapid Innovation can provide tailored solutions to enhance organizational resilience.
- Ethical AI: As artificial intelligence becomes more prevalent, ethical considerations surrounding its use are critical. Organizations should ensure that AI systems are transparent and free from bias, and Rapid Innovation can guide the development of ethical AI frameworks that promote fairness and accountability.
- Accountability: Organizations must establish clear accountability for data breaches and ethical violations. This includes having policies in place to address any incidents that may arise, ensuring that organizations are prepared to respond effectively.
- Stakeholder Engagement: Engaging stakeholders in discussions about ethical practices can foster a culture of responsibility and transparency, which is essential for building trust in technology-driven initiatives.
9. Future Trends and Emerging Technologies
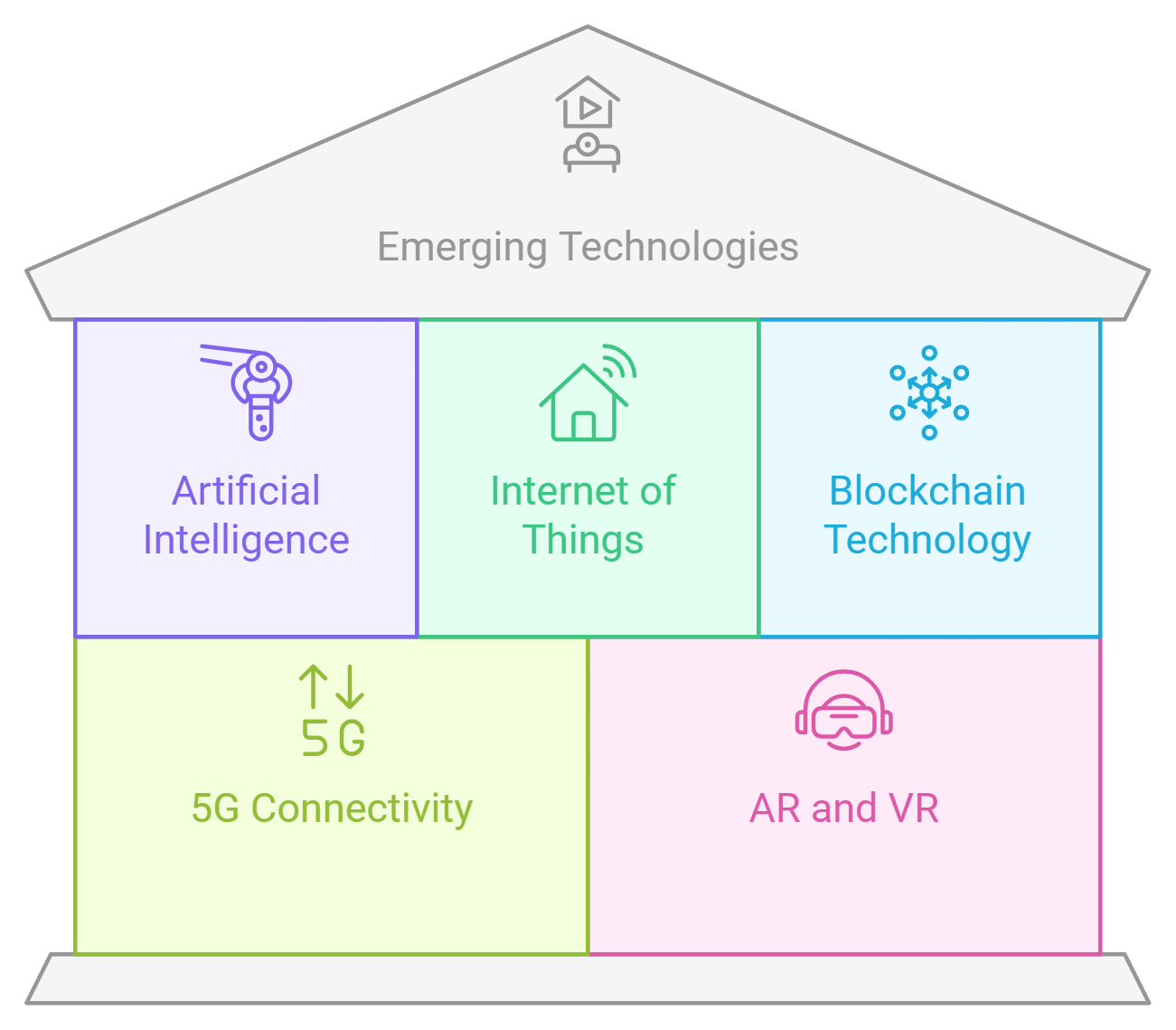
The landscape of technology is constantly evolving, with new trends and innovations shaping the future. Organizations must stay informed to remain competitive.
- Artificial Intelligence (AI): AI continues to advance, with applications in various sectors. From predictive analytics to natural language processing, AI is transforming how businesses operate, and Rapid Innovation is at the forefront of helping organizations harness these capabilities for improved decision-making and operational efficiency.
- Internet of Things (IoT): The IoT is expanding, connecting devices and enabling smarter operations. This trend is particularly significant in industries like manufacturing and healthcare, where Rapid Innovation can facilitate the integration of IoT solutions to optimize processes and enhance service delivery.
- Blockchain Technology: Blockchain offers secure and transparent transactions. Its potential applications range from supply chain management to digital identity verification, and Rapid Innovation specializes in developing blockchain solutions that enhance trust and traceability in business operations.
- 5G Connectivity: The rollout of 5G networks is set to revolutionize communication. With faster speeds and lower latency, 5G will enhance IoT capabilities and enable new applications, positioning organizations to leverage real-time data for strategic advantage.
- Augmented Reality (AR) and Virtual Reality (VR): AR and VR technologies are gaining traction in training, marketing, and entertainment. These immersive experiences can enhance user engagement and learning, and Rapid Innovation can assist in creating innovative AR and VR applications tailored to specific business needs.
By understanding these trends and technologies, organizations can better prepare for the future and leverage new opportunities for growth and innovation, with Rapid Innovation as a trusted partner in their digital transformation journey.
9.1. Edge AI and Distributed Intelligence
Edge AI refers to the deployment of artificial intelligence algorithms directly on devices at the edge of the network, rather than relying on centralized cloud computing. This approach offers several advantages:
- Reduced Latency: By processing data closer to where it is generated, Edge AI minimizes the time it takes to analyze and respond to data inputs. This is crucial for applications requiring real-time decision-making, such as autonomous vehicles and industrial automation.
- Bandwidth Efficiency: Transmitting large volumes of data to the cloud can be costly and slow. Edge AI reduces the amount of data sent over the network by processing it locally, which is particularly beneficial in environments with limited connectivity.
- Enhanced Privacy and Security: Keeping sensitive data on local devices reduces the risk of data breaches associated with cloud storage. Edge AI allows for better compliance with data protection regulations by minimizing data transfer.
- Scalability: Distributed intelligence enables systems to scale more effectively. As more devices are added to the network, they can operate independently while still contributing to the overall intelligence of the system.
- Energy Efficiency: Processing data locally can lead to lower energy consumption, which is essential for battery-powered devices and sustainability efforts.
At Rapid Innovation, we leverage Edge AI solutions to help clients optimize their operations, reduce costs, and enhance their service offerings. For instance, in the manufacturing sector, we have implemented Edge AI solutions that allow for real-time monitoring and predictive analytics, leading to significant reductions in downtime and maintenance costs. Additionally, we utilize the NVIDIA Jetson platform to enhance our Edge AI platform capabilities, ensuring robust performance and scalability. If you're looking to enhance your team with specialized skills, consider hiring generative AI engineers to drive your projects forward.
9.2. Advanced Machine Learning Techniques
Advanced machine learning techniques are transforming how data is analyzed and utilized across various industries. These techniques include:
- Deep Learning: Utilizing neural networks with multiple layers, deep learning excels in tasks such as image and speech recognition. It requires large datasets and significant computational power but can achieve remarkable accuracy.
- Reinforcement Learning: This technique involves training algorithms through trial and error, allowing them to learn optimal actions based on rewards. It is widely used in robotics, gaming, and autonomous systems.
- Transfer Learning: Transfer learning allows models trained on one task to be adapted for another, reducing the time and data required for training. This is particularly useful in scenarios where labeled data is scarce.
- Federated Learning: This decentralized approach enables multiple devices to collaboratively learn a shared model while keeping their data local. It enhances privacy and reduces the need for data centralization.
- Natural Language Processing (NLP): Advanced NLP techniques enable machines to understand and generate human language, facilitating applications like chatbots, sentiment analysis, and language translation.
At Rapid Innovation, we harness these advanced machine learning techniques to deliver tailored solutions that drive business growth. For example, our deep learning models have been successfully deployed in healthcare to improve diagnostic accuracy, resulting in better patient outcomes and increased operational efficiency. Our Edge ML platform further enhances these capabilities by enabling efficient model deployment at the edge.
9.3. Integration with IoT and Digital Twins
The integration of artificial intelligence with the Internet of Things (IoT) and digital twins is revolutionizing industries by creating smarter, more efficient systems. Key aspects include:
- Real-time Data Analysis: IoT devices generate vast amounts of data. AI algorithms can analyze this data in real-time, providing insights that drive immediate actions and improve operational efficiency.
- Predictive Maintenance: By leveraging AI and IoT data, organizations can predict equipment failures before they occur. This proactive approach reduces downtime and maintenance costs, enhancing overall productivity.
- Digital Twins: A digital twin is a virtual representation of a physical object or system. Integrating AI with digital twins allows for advanced simulations and scenario testing, enabling better decision-making and optimization of processes.
- Enhanced Automation: AI-driven IoT systems can automate complex processes, reducing the need for human intervention. This leads to increased efficiency and the ability to respond quickly to changing conditions.
- Improved Customer Experiences: The combination of AI, IoT, and digital twins enables businesses to offer personalized services and products. By analyzing user behavior and preferences, companies can tailor their offerings to meet customer needs effectively.
Rapid Innovation specializes in integrating AI with IoT and digital twins to help clients achieve greater ROI. For instance, we have developed digital twin solutions for smart cities that optimize resource management and enhance urban planning, leading to improved quality of life for residents and significant cost savings for municipalities. Our expertise in Edge AI platforms ensures that these solutions are not only innovative but also practical and efficient.
9.4. Quantum Computing in Maintenance
Quantum computing is revolutionizing various sectors, including maintenance in industries such as manufacturing, aerospace, and energy. By leveraging the principles of quantum mechanics, quantum computers can process vast amounts of data at unprecedented speeds, leading to significant improvements in maintenance strategies.
- Predictive Maintenance: Quantum computing can enhance predictive maintenance by analyzing complex datasets to identify patterns and predict equipment failures before they occur. This proactive approach minimizes downtime and reduces maintenance costs, ultimately leading to a higher return on investment (ROI) for organizations.
- Optimization of Resources: Quantum algorithms can optimize resource allocation for maintenance tasks, ensuring that the right personnel and tools are available at the right time. This improves efficiency and reduces waste, allowing companies to allocate resources more effectively and increase profitability.
- Real-time Data Processing: Quantum computers can handle real-time data from IoT devices, enabling immediate analysis and decision-making. This capability allows for rapid responses to maintenance needs, enhancing operational reliability and reducing the likelihood of costly disruptions.
- Simulation and Modeling: Quantum computing can simulate various maintenance scenarios, helping organizations understand potential outcomes and make informed decisions. This is particularly useful in industries where safety and reliability are critical, as it allows for better planning and risk management.
- Enhanced Data Security: Quantum encryption methods can secure sensitive maintenance data, protecting it from cyber threats. This is essential as industries increasingly rely on digital solutions for maintenance management, ensuring that organizations can maintain trust and compliance while safeguarding their assets.
10. Implementation Roadmap

An effective implementation roadmap is crucial for integrating new technologies, such as quantum computing, into maintenance processes. This roadmap outlines the steps necessary to ensure a smooth transition and maximize the benefits of the technology.
- Define Objectives: Clearly outline the goals of implementing quantum computing in maintenance. This could include reducing downtime, improving efficiency, or enhancing predictive capabilities, all of which contribute to achieving greater ROI.
- Stakeholder Engagement: Involve key stakeholders from various departments, including IT, operations, and maintenance teams. Their insights will help shape the implementation strategy and ensure alignment with organizational goals.
- Technology Assessment: Evaluate the current technology landscape to identify gaps and opportunities for integrating quantum computing. This assessment should include hardware, software, and data management systems.
- Pilot Projects: Initiate pilot projects to test quantum computing applications in maintenance. These projects will provide valuable insights and help refine the implementation strategy before a full-scale rollout.
- Training and Development: Invest in training programs for staff to ensure they are equipped to work with quantum computing technologies. This will facilitate a smoother transition and enhance overall productivity.
- Monitor and Evaluate: Establish metrics to monitor the performance of quantum computing applications in maintenance. Regular evaluations will help identify areas for improvement and ensure the technology is delivering the desired outcomes.
10.1. Assessment and Readiness Evaluation
Before implementing quantum computing in maintenance, organizations must conduct a thorough assessment and readiness evaluation. This process helps identify the current state of operations and the necessary steps for successful integration.
- Current State Analysis: Assess the existing maintenance processes, technologies, and data management practices. Understanding the current state will highlight areas that require improvement and inform the implementation strategy.
- Capability Assessment: Evaluate the organization's capabilities in terms of technology, personnel, and processes. This assessment will help determine whether the organization is ready to adopt quantum computing or if additional resources are needed.
- Risk Analysis: Identify potential risks associated with the implementation of quantum computing, including technical challenges, data security concerns, and the impact on existing workflows.
- Infrastructure Readiness: Review the current IT infrastructure to ensure it can support quantum computing technologies. This may involve upgrading hardware, software, or network capabilities.
- Cultural Readiness: Assess the organizational culture to determine if it is conducive to adopting new technologies. A culture that embraces innovation and change will facilitate a smoother transition to quantum computing.
- Stakeholder Buy-in: Ensure that key stakeholders are on board with the implementation plan. Their support is crucial for overcoming resistance and ensuring the success of the initiative.
At Rapid Innovation, we specialize in guiding organizations through the complexities of integrating advanced technologies like quantum computing maintenance into their maintenance processes. Our expertise in AI and blockchain further enhances our ability to deliver tailored solutions that drive efficiency, security, and ROI for our clients.
10.2. Pilot Project Development
Pilot project development is a crucial step in testing new ideas, products, or processes before full-scale implementation. It allows organizations to evaluate the feasibility and effectiveness of a project in a controlled environment, ultimately leading to greater ROI.
- Define objectives: Clearly outline what you aim to achieve with the pilot project development. This could include testing a new technology, assessing user engagement, or measuring operational efficiency. For instance, Rapid Innovation can assist in defining specific KPIs that align with your business goals.
- Select a target group: Choose a representative sample of users or stakeholders who will participate in the pilot. This group should reflect the broader audience for the project, ensuring that insights gained are applicable across the organization.
- Develop a project plan: Create a detailed plan that includes timelines, resources, and responsibilities. This plan should also outline the metrics for success and how data will be collected. Rapid Innovation can provide expertise in project management methodologies tailored to AI and Blockchain initiatives.
- Implement the pilot: Execute the project according to the plan. Ensure that all participants are informed and trained on their roles. Our team can facilitate training sessions to ensure smooth execution.
- Monitor and evaluate: Collect data throughout the pilot project development to assess performance against the defined objectives. Use surveys, interviews, and analytics to gather insights. Rapid Innovation employs advanced analytics tools to provide real-time feedback and insights.
- Adjust and iterate: Based on the evaluation, make necessary adjustments to improve the project before scaling it up. This may involve refining processes, enhancing user experience, or addressing unforeseen challenges. Our iterative approach ensures that your project evolves based on data-driven insights.
10.3. Scalability Strategies
Scalability strategies are essential for organizations looking to grow and expand their operations without compromising quality or performance. Effective scalability ensures that a business can handle increased demand efficiently, and Rapid Innovation is here to guide you through this process.
- Assess current infrastructure: Evaluate your existing systems, processes, and resources to identify areas that can support growth. This includes technology, workforce, and supply chain capabilities. Our experts can conduct a thorough assessment to pinpoint opportunities for improvement.
- Automate processes: Implement automation tools to streamline operations. Automation can reduce manual errors, increase efficiency, and free up staff for more strategic tasks. Rapid Innovation specializes in AI-driven automation solutions that enhance productivity.
- Standardize procedures: Develop standardized processes and protocols to ensure consistency across the organization. This makes it easier to replicate successful practices as the business scales. Our team can help create documentation and training materials to support this standardization.
- Invest in technology: Leverage technology solutions that can grow with your business. Cloud computing, for instance, allows for flexible resource allocation and can easily accommodate increased demand. Rapid Innovation can recommend and implement scalable technology solutions tailored to your needs.
- Foster a culture of innovation: Encourage employees to contribute ideas for improvement and growth. A culture that embraces change can adapt more readily to new challenges and opportunities. We can facilitate workshops to inspire creativity and innovation within your team.
- Monitor performance metrics: Regularly track key performance indicators (KPIs) to assess scalability efforts. This data-driven approach helps identify areas for improvement and informs strategic decisions. Our analytics solutions provide comprehensive dashboards for real-time monitoring.
10.4. Change Management
Change management is the structured approach to transitioning individuals, teams, and organizations from a current state to a desired future state. It is vital for ensuring that changes are implemented smoothly and effectively, and Rapid Innovation can support you throughout this journey.
- Communicate effectively: Clear communication is essential during any change initiative. Keep all stakeholders informed about the reasons for the change, the benefits, and how it will impact them. Our communication strategies ensure that everyone is aligned and informed.
- Involve stakeholders: Engage employees and other stakeholders in the change process. Their input can provide valuable insights and foster a sense of ownership. Rapid Innovation can facilitate stakeholder engagement sessions to gather feedback and insights.
- Provide training and support: Equip employees with the necessary skills and knowledge to adapt to the change. This may include workshops, online courses, or one-on-one coaching. Our training programs are designed to empower your team with the skills needed for successful adoption.
- Address resistance: Anticipate and address resistance to change. Understand the concerns of those affected and provide reassurance and support to ease their transition. We can help develop strategies to mitigate resistance and foster acceptance.
- Monitor progress: Regularly assess the implementation of the change initiative. Use feedback mechanisms to gather insights and make adjustments as needed. Our monitoring tools provide ongoing support to ensure successful change management.
- Celebrate successes: Recognize and celebrate milestones and achievements throughout the change process. This helps to maintain morale and encourages continued engagement. Rapid Innovation can assist in creating recognition programs that highlight achievements and foster a positive culture.
10.5. Continuous Improvement Framework

The Continuous Improvement Framework (CIF) is a structured approach that organizations use to enhance their processes, products, and services over time. This framework is essential for fostering a culture of ongoing development and efficiency, particularly in the realms of AI and Blockchain, where rapid technological advancements necessitate constant adaptation.
- Focus on incremental changes rather than radical shifts, allowing organizations to integrate new technologies seamlessly.
- Utilize methodologies such as Lean, Six Sigma, and Kaizen to drive improvements, ensuring that AI and Blockchain implementations are optimized for performance and cost-effectiveness. This aligns with the principles of the continuous improvement itil framework.
- Encourage employee involvement at all levels to identify areas for enhancement, leveraging diverse perspectives to innovate solutions, as seen in the continuous quality improvement framework.
- Implement regular training and development programs to equip staff with the necessary skills, particularly in understanding AI algorithms and Blockchain protocols, which is a key aspect of the continuous improvement governance framework.
- Use data-driven decision-making to assess performance and identify improvement opportunities, enabling organizations to harness the full potential of AI analytics and Blockchain transparency, similar to the itil service improvement model.
- Establish clear metrics and KPIs to measure progress and success, ensuring that AI and Blockchain initiatives align with business objectives, as outlined in the framework for process improvement.
- Foster an environment where feedback is welcomed and acted upon, promoting a culture of continuous learning and adaptation, which is crucial in the steps of continuous improvement framework.
- Regularly review and adjust processes to ensure they remain effective and relevant, particularly in the fast-evolving landscape of technology, as emphasized in the continuous improvement framework steps.
The CIF is not a one-time initiative but a continuous cycle of planning, executing, checking, and acting (PDCA). This cyclical nature ensures that organizations remain agile and responsive to changes in the market and customer needs, particularly in the context of AI and Blockchain innovations. Examples of continuous improvement framework examples can provide further insights into practical applications, including AI development guides for businesses.
11. Case Studies
Case studies provide real-world examples of how organizations have successfully implemented strategies and frameworks to achieve their goals. They serve as valuable learning tools for other businesses looking to replicate success, especially in the fields of AI and Blockchain.
- Highlight specific challenges faced by organizations.
- Detail the strategies and frameworks employed to overcome these challenges, including the itil service improvement plan and the itil continuous improvement model.
- Showcase measurable outcomes and improvements resulting from the initiatives, reflecting the impact of the cqi framework and baldrige continuous improvement principles.
- Provide insights into the lessons learned and best practices developed through the process, including the commercial continuous improvement assessment framework.
Case studies can cover various industries, showcasing the versatility of different approaches and the universal principles of success.
11.1. Industrial Success Stories
Industrial success stories illustrate how companies in various sectors have effectively applied continuous improvement principles to achieve remarkable results. These stories often highlight innovative practices and the impact of strategic initiatives.
- Toyota: Pioneered the Lean manufacturing approach, significantly reducing waste and improving efficiency. Their commitment to continuous improvement has made them a leader in the automotive industry.
- General Electric: Implemented Six Sigma to enhance quality and reduce defects, resulting in billions of dollars in savings and improved customer satisfaction.
- Boeing: Adopted a continuous improvement culture in their production processes, leading to faster turnaround times and increased product quality.
These success stories demonstrate the power of continuous improvement in driving operational excellence and achieving competitive advantage. By learning from these examples, other organizations can adapt similar strategies to their unique contexts, particularly in leveraging AI and Blockchain technologies for enhanced performance and ROI. For expert guidance in this area, consider partnering with an AI technology consulting company to enhance your continuous improvement efforts.
11.2. Lessons Learned
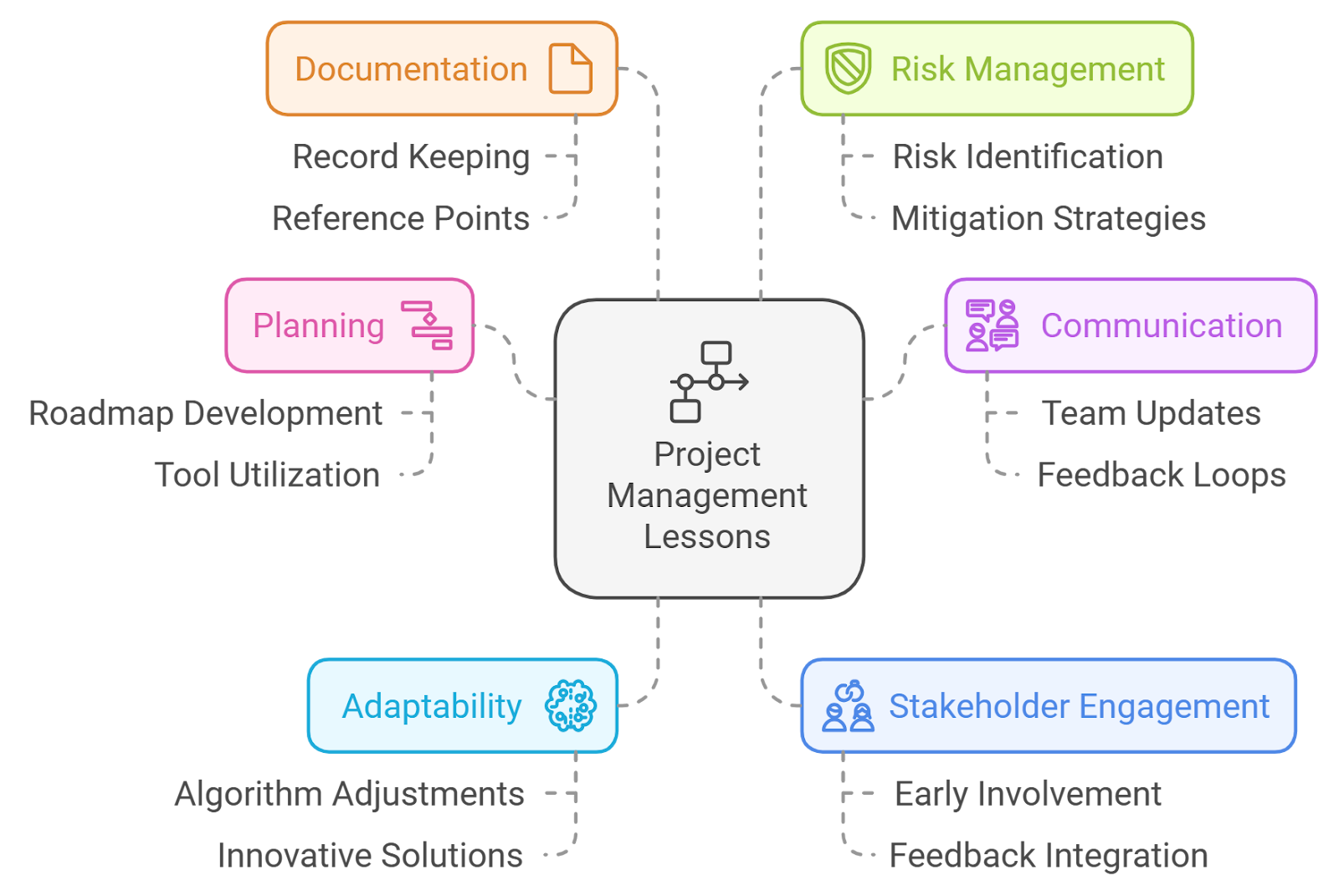
In any project or initiative, reflecting on the lessons learned is crucial for future success. This process involves analyzing what worked well, what didn’t, and how these insights can be applied moving forward.
- Importance of Planning: Effective planning is essential. Projects that lack a clear roadmap often face delays and budget overruns. A well-structured plan helps in setting realistic timelines and expectations, particularly in AI and Blockchain projects where technical complexities can arise. Tools like insight for jira and smartsheet work insights can aid in this planning phase.
- Communication is Key: Open lines of communication among team members and stakeholders can prevent misunderstandings and ensure everyone is on the same page. Regular updates and feedback loops are vital for maintaining alignment, especially when integrating AI solutions or Blockchain technologies that require cross-functional collaboration. Utilizing platforms such as asana insights and wrike marketing insights can enhance communication efforts.
- Flexibility and Adaptability: The ability to pivot in response to unforeseen challenges is critical. Projects often encounter unexpected obstacles, and being adaptable can lead to innovative solutions. For instance, in AI development, adjusting algorithms based on real-time data can significantly enhance outcomes. This adaptability can be supported by tools like mavenlink insights and project management insights.
- Stakeholder Engagement: Involving stakeholders early in the process can lead to better outcomes. Their insights and feedback can help shape the project and ensure it meets the needs of all parties involved. This is particularly important in Blockchain initiatives, where stakeholder buy-in is essential for network success. Capital insight project management can provide valuable stakeholder engagement strategies.
- Documentation: Keeping thorough records of decisions, changes, and outcomes can provide valuable insights for future projects. This documentation serves as a reference point for what strategies were effective and which were not, aiding in the iterative processes common in AI and Blockchain development. Utilizing tools like project insight pricing can streamline documentation efforts.
- Risk Management: Identifying potential risks early on and developing mitigation strategies can save time and resources. A proactive approach to risk management can prevent small issues from escalating into major problems, especially in the rapidly evolving fields of AI and Blockchain.
11.3. Comparative Analysis
Comparative analysis involves evaluating different projects, strategies, or methodologies to identify strengths and weaknesses. This process can provide valuable insights that inform future decisions.
- Benchmarking: Comparing performance metrics against industry standards or competitors can highlight areas for improvement. This can include analyzing cost efficiency, time management, and quality of output, particularly in AI model performance or Blockchain transaction speeds. Tools like smartsheet insights can assist in benchmarking efforts.
- Identifying Best Practices: By examining successful projects, organizations can identify best practices that can be adopted in their own initiatives. This can lead to improved processes and outcomes, especially in the context of AI algorithms or Blockchain implementations. Insights from project management insights can be particularly useful here.
- Understanding Market Trends: A comparative analysis can reveal shifts in market trends and consumer preferences. Staying informed about these changes can help organizations adapt their strategies accordingly, particularly in the fast-paced AI and Blockchain sectors.
- SWOT Analysis: Conducting a SWOT (Strengths, Weaknesses, Opportunities, Threats) analysis can provide a comprehensive view of where a project stands in relation to others. This can help in strategic planning and resource allocation, ensuring that AI and Blockchain projects are aligned with organizational goals.
- Learning from Failures: Analyzing projects that did not meet their objectives can provide critical lessons. Understanding the reasons behind failures can help prevent similar mistakes in the future, particularly in complex AI and Blockchain projects where the stakes are high.
- Data-Driven Decisions: Utilizing data from comparative analysis can lead to more informed decision-making. This can enhance the effectiveness of strategies and improve overall project outcomes, especially in data-intensive fields like AI and Blockchain.
12. Conclusion
In conclusion, the insights gained from lessons learned and comparative analysis are invaluable for driving future success. By focusing on effective planning, communication, and adaptability, organizations can enhance their project management practices.
- Continuous Improvement: Emphasizing a culture of continuous improvement ensures that organizations are always learning and evolving. This mindset fosters innovation and resilience, particularly in the dynamic fields of AI and Blockchain.
- Strategic Decision-Making: Utilizing comparative analysis allows organizations to make data-driven decisions that align with their goals. This strategic approach can lead to better resource allocation and project outcomes.
- Future Readiness: By applying the lessons learned and insights gained from comparative analysis, organizations can better prepare for future challenges. This proactive stance can enhance their competitive edge in the market.
- Collaboration and Engagement: Encouraging collaboration among team members and stakeholders can lead to richer insights and more effective solutions. Engaged teams are often more motivated and productive.
- Embracing Change: Organizations that are willing to embrace change and adapt to new information are more likely to succeed in a dynamic environment. Flexibility is key to navigating uncertainties.
By integrating these principles into their operations, organizations can not only improve their current projects but also set a solid foundation for future endeavors, particularly in the innovative realms of AI and Blockchain.
12.1. Key Takeaways

- Understanding the core principles of a subject is essential for effective application, particularly in AI and Blockchain, where foundational knowledge drives successful implementation.
- Data-driven decision-making enhances the quality of outcomes in various fields, allowing organizations to leverage AI analytics for improved insights and strategic direction.
- Collaboration among teams leads to innovative solutions and improved performance, especially when integrating diverse expertise in AI and Blockchain technologies, including blockchain integration with AI.
- Continuous learning and adaptation are crucial in a rapidly changing environment, ensuring that teams stay ahead of technological advancements and market demands.
- Stakeholder engagement is vital for successful project implementation and sustainability, particularly in Blockchain projects where transparency and trust are paramount.
- Technology integration can streamline processes and increase efficiency, enabling businesses to automate workflows and reduce operational costs through AI solutions, particularly in the context of AI and blockchain a disruptive integration.
- Clear communication fosters transparency and trust within organizations, essential for aligning teams on AI and Blockchain initiatives.
- Measuring success through key performance indicators (KPIs) helps in tracking progress, allowing organizations to assess the ROI of their AI and Blockchain investments.
- Emphasizing customer feedback can lead to better product development and service delivery, particularly in AI-driven applications that require user-centric design.
- Sustainability practices are becoming increasingly important for long-term viability, with AI and Blockchain offering innovative solutions to enhance environmental responsibility.
12.2. Strategic Recommendations
- Invest in training programs to enhance team skills and knowledge, particularly in AI and Blockchain technologies, to ensure your workforce is equipped for future challenges.
- Utilize analytics tools to gather insights and inform strategic decisions, leveraging AI capabilities to drive data-informed strategies.
- Foster a culture of open communication to encourage idea sharing and collaboration, which is essential for driving innovation in AI and Blockchain projects.
- Develop a robust stakeholder engagement plan to ensure all voices are heard, particularly in Blockchain initiatives where community involvement is critical.
- Leverage technology to automate repetitive tasks and improve operational efficiency, utilizing AI to enhance productivity and reduce manual errors.
- Establish clear KPIs to measure success and adjust strategies as needed, ensuring that AI and Blockchain initiatives are aligned with business objectives.
- Encourage innovation by providing resources and time for creative projects, particularly in exploring new applications of AI and Blockchain, including AI and blockchain integration.
- Implement feedback loops to continuously improve products and services, ensuring that AI solutions evolve based on user experiences and needs.
- Prioritize sustainability initiatives to align with consumer expectations and regulatory requirements, utilizing Blockchain for transparent supply chain practices.
- Regularly review and adjust strategies based on market trends and performance data, ensuring that your organization remains agile in the face of technological advancements.
12.3. Future Outlook
The integration of artificial intelligence and machine learning will transform industries, leading to more personalized experiences. Remote work is likely to remain prevalent, necessitating new management strategies and tools. Sustainability will become a central focus for businesses, influencing consumer choices and investment strategies. The demand for data privacy and security will increase, prompting organizations to adopt stricter measures. Collaboration tools will evolve, enhancing remote teamwork and communication. The gig economy will continue to grow, changing traditional employment models and workforce dynamics. Health and wellness will gain prominence in workplace culture, impacting employee satisfaction and productivity. Digital transformation will accelerate, requiring businesses to adapt quickly to technological advancements. Consumer behavior will shift towards more ethical and sustainable practices, influencing product development. Organizations that embrace change and innovation will be better positioned for success in the future, particularly those leveraging AI integration strategies to drive efficiency and transparency.