Table Of Contents
Category
Artificial Intelligence
FinTech
1. Introduction to AI in Loan Processing
Artificial Intelligence (AI) is revolutionizing various sectors, and the financial industry is no exception. In loan processing, AI in loan processing is becoming increasingly prevalent, streamlining operations and enhancing decision-making. The integration of AI in loan approval processes offers numerous advantages, but it also presents certain challenges that need to be addressed.
AI technologies, such as machine learning and natural language processing, are being utilized to analyze vast amounts of data quickly and accurately. These technologies assist in assessing creditworthiness, predicting loan defaults, and personalizing loan offers for customers, ultimately leading to a more tailored experience for borrowers.
The use of AI can significantly reduce the time taken for loan approvals, which traditionally involves extensive paperwork and manual reviews. By automating these processes, Rapid Innovation helps financial institutions achieve faster turnaround times, thereby improving customer satisfaction and retention.
The growing reliance on AI in loan processing is driven by the need for efficiency, accuracy, and improved customer experience. As financial institutions seek to remain competitive, understanding the role of AI agents in loan approval becomes essential. Rapid Innovation is committed to guiding clients through the implementation of AI solutions that not only enhance operational efficiency but also drive greater ROI by optimizing resource allocation and minimizing risks associated with loan defaults. For more insights on AI applications in finance.
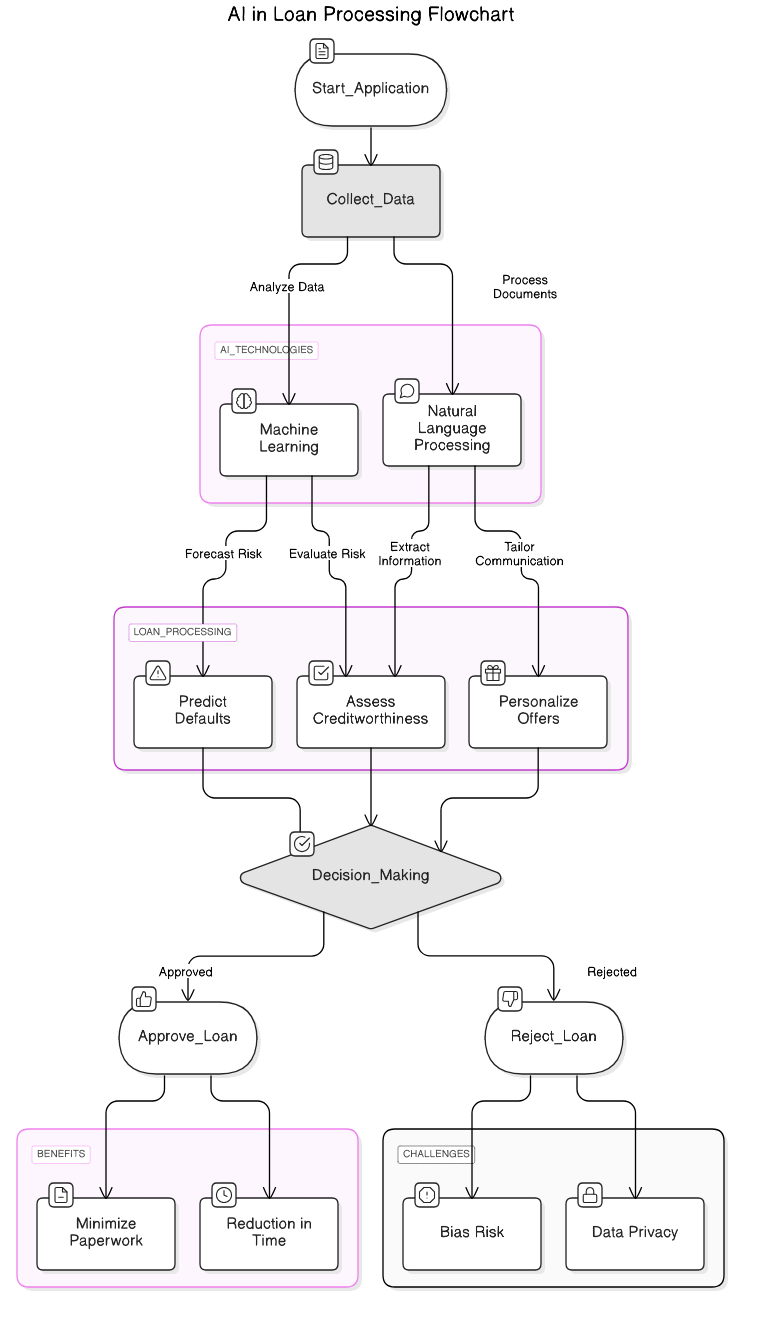
Refer to the image for a visual representation of the role of AI in loan processing.
1.1. Evolution of Loan Approval Systems
The evolution of loan approval systems has undergone significant transformations over the years, driven by technological advancements and changing consumer expectations.
- Early loan approval processes were manual, requiring extensive paperwork and in-person meetings.
- The introduction of computers in the 1980s streamlined data entry and record-keeping, but the process remained largely paper-based.
- The 1990s saw the rise of online applications, allowing borrowers to submit requests from the comfort of their homes.
- The 2000s introduced automated underwriting systems, which utilized algorithms to assess creditworthiness more efficiently.
- Recent advancements in big data and machine learning have further revolutionized loan approval, enabling lenders to analyze vast amounts of data quickly and accurately.
- Today, many lenders use AI-driven systems that can provide instant approvals, reducing the time from application to funding significantly.
At Rapid Innovation, we leverage these advancements to help our clients implement AI loan processing systems that enhance efficiency and customer satisfaction, ultimately leading to greater ROI. Additionally, the role of AI in credit scoring and lending decision-making is becoming increasingly important as lenders seek to improve their assessment processes.
1.2. Traditional vs AI-Powered Loan Processing
The contrast between traditional loan processing methods and AI-powered systems is stark, with each approach offering distinct advantages and challenges.
Traditional Loan Processing:
- Relies heavily on human judgment and manual data entry.
- Involves lengthy approval times, often taking days or weeks.
- Prone to human error, which can lead to inconsistencies in decision-making.
- Limited ability to analyze large datasets, restricting the assessment of borrower risk.
AI-Powered Loan Processing:
- Utilizes algorithms and machine learning to analyze borrower data quickly.
- Can provide instant loan approvals, enhancing customer satisfaction.
- Reduces human error by automating data analysis and decision-making processes.
- Capable of assessing a broader range of data points, including alternative credit data, which can help underserved populations gain access to credit.
The shift towards AI-powered loan processing is reshaping the lending landscape, making it more efficient and inclusive. Rapid Innovation specializes in developing tailored AI solutions that empower lenders to transition from traditional methods to AI-driven processes, maximizing their operational efficiency and return on investment.
1.3. Regulatory Framework and Compliance Overview
The regulatory framework governing loan approval systems is crucial for ensuring consumer protection and maintaining the integrity of the financial system.
- Regulations vary by country but generally include guidelines on fair lending practices, data privacy, and anti-discrimination laws.
- In the United States, the Equal Credit Opportunity Act (ECOA) prohibits lenders from discriminating against applicants based on race, gender, or other protected characteristics.
- The Dodd-Frank Act introduced additional regulations aimed at increasing transparency and accountability in the lending process.
- Compliance with the General Data Protection Regulation (GDPR) in Europe mandates that lenders protect consumer data and obtain consent for data usage.
- Lenders must also stay updated on evolving regulations related to AI and machine learning, as these technologies raise new ethical and compliance challenges.
Understanding the regulatory landscape is essential for lenders to navigate compliance effectively while leveraging innovative technologies in loan processing. At Rapid Innovation, we provide consulting services to help our clients ensure compliance with these regulations while implementing cutting-edge AI loan processing solutions that enhance their loan processing capabilities.
Refer to the image below for a visual representation of the evolution of loan approval systems:

1.4. Current Market Landscape
The current market landscape for AI loan agents is rapidly evolving, driven by advancements in technology and changing consumer expectations. The integration of artificial intelligence in the financial sector has transformed how loans are processed, approved, and managed. Key trends include the increased adoption of AI loan agents by financial institutions to enhance customer experience, a growing demand for personalized loan products tailored to individual needs, and competitive pressure among lenders to streamline operations and reduce costs. Additionally, regulatory changes are encouraging the use of technology in lending practices, while there is a rise of fintech companies leveraging AI to disrupt traditional banking models.
According to recent studies, the global AI in the fintech market is expected to grow significantly, with projections indicating a compound annual growth rate (CAGR) of over 23% from 2021 to 2028. This growth is fueled by the need for efficiency, accuracy, and improved customer service in loan processing.
The market is characterized by a mix of established banks and emerging fintech startups, each vying for a share of the growing demand for AI-driven solutions. Traditional banks are increasingly partnering with technology firms to enhance their offerings, while fintech companies are innovating rapidly to capture market share. At Rapid Innovation, we leverage our expertise in AI and blockchain to help clients navigate this evolving landscape, ensuring they remain competitive and achieve greater ROI through tailored solutions.
2. Core Technologies Powering AI Loan Agents
AI loan agents rely on a variety of core technologies that enable them to function effectively and efficiently. These technologies are essential for automating processes, analyzing data, and providing personalized services to borrowers. The key technologies include:
- Natural Language Processing (NLP): Enables AI loan agents to understand and respond to customer inquiries in a conversational manner.
- Data Analytics: Helps in assessing borrower profiles and predicting loan performance based on historical data.
- Robotic Process Automation (RPA): Automates repetitive tasks, such as document verification and data entry, to speed up the loan approval process.
These technologies work in tandem to create a seamless experience for both lenders and borrowers, reducing the time and effort required to secure a loan.
2.1. Machine Learning Algorithms
Machine learning algorithms are at the heart of AI loan agents, enabling them to learn from data and improve their decision-making processes over time. These algorithms analyze vast amounts of data to identify patterns and make predictions about loan approvals and risk assessments. The main types of machine learning algorithms used include:
- Supervised Learning: Involves training algorithms on labeled datasets to predict outcomes, such as whether a loan should be approved based on a borrower's credit history.
- Unsupervised Learning: Used to identify hidden patterns in data without predefined labels, helping lenders discover new customer segments or risk factors.
- Reinforcement Learning: Allows AI systems to learn optimal strategies through trial and error, improving their performance in real-time loan processing scenarios.
The application of machine learning in loan processing has led to significant improvements in accuracy and efficiency. For instance, lenders can now assess creditworthiness more effectively, reducing the risk of defaults and enhancing profitability.
As machine learning continues to evolve, its integration into AI loan agents will likely lead to even more sophisticated models that can adapt to changing market conditions and borrower behaviors. This adaptability is crucial in a dynamic financial landscape where consumer preferences and regulatory requirements are constantly shifting. At Rapid Innovation, we are committed to helping our clients harness these technologies to drive efficiency and maximize their return on investment.
Refer to the image below for a visual representation of the current market landscape for AI loan agents.

2.1.1. Supervised Learning Models
Supervised learning is a type of machine learning where models are trained on labeled datasets. This means that the input data is paired with the correct output, allowing the model to learn the relationship between the two. Supervised learning is widely used in various applications, including designing machine learning systems, due to its effectiveness in making predictions based on historical data.
- Key Characteristics: Â
- Requires labeled data for training.
- The model learns to map inputs to outputs.
- Performance can be evaluated using metrics like accuracy, precision, and recall.
- Common Algorithms: Â
- Linear Regression: Used for predicting continuous values.
- Logistic Regression: Suitable for binary classification tasks.
- Decision Trees: Useful for both classification and regression tasks.
- Support Vector Machines (SVM): Effective in high-dimensional spaces.
- Neural Networks: Capable of capturing complex patterns in data.
- Applications: Â
- Email filtering: Classifying emails as spam or not spam.
- Credit scoring: Predicting the likelihood of a borrower defaulting on a loan.
- Medical diagnosis: Assisting in identifying diseases based on patient data.
- Machine learning applications in various sectors, including healthcare and finance.
At Rapid Innovation, we leverage supervised learning models to help clients enhance their decision-making processes and operational efficiencies. For instance, in the financial sector, our predictive models can significantly improve credit scoring accuracy, leading to better risk management and increased ROI. We also focus on machine learning for systems that optimize operational workflows, including our expertise in AI deep learning applications.
2.1.2. Unsupervised Learning Applications
Unsupervised learning is a type of machine learning that deals with unlabeled data. Unlike supervised learning, the model is not provided with the correct output during training. Instead, it tries to identify patterns and structures within the data on its own. This approach is particularly useful for exploratory data analysis and discovering hidden insights, such as manifold learning.
- Key Characteristics: Â
- Does not require labeled data.
- Focuses on finding patterns and groupings in data.
- Performance evaluation can be subjective, often relying on visual inspection.
- Common Algorithms: Â
- K-Means Clustering: Groups data into k distinct clusters based on similarity.
- Hierarchical Clustering: Builds a tree of clusters for better data organization.
- Principal Component Analysis (PCA): Reduces dimensionality while preserving variance.
- t-Distributed Stochastic Neighbor Embedding (t-SNE): Visualizes high-dimensional data in lower dimensions.
- Applications: Â
- Market segmentation: Identifying distinct customer groups for targeted marketing.
- Anomaly detection: Detecting unusual patterns that may indicate fraud or errors.
- Recommendation systems: Suggesting products based on user behavior and preferences.
- Machine learning with applications in customer insights and behavior analysis.
Unsupervised learning applications are vital in scenarios where labeled data is scarce or unavailable. They enable organizations to gain insights from large datasets, leading to informed decision-making. At Rapid Innovation, we assist clients in implementing unsupervised learning techniques to uncover valuable insights, such as customer segmentation, which can drive targeted marketing strategies and improve customer engagement.
2.1.3. Deep Learning Integration
Deep learning is a subset of machine learning that utilizes neural networks with many layers (hence "deep") to analyze various forms of data. It has gained significant traction due to its ability to handle large datasets and extract complex features automatically. Deep learning integration is transforming industries by enabling advanced applications that were previously unattainable, including deep learning applications in computer vision.
- Key Characteristics: Â
- Utilizes multi-layered neural networks.
- Capable of automatic feature extraction.
- Requires substantial computational power and large datasets for training.
- Common Architectures: Â
- Convolutional Neural Networks (CNNs): Primarily used for image processing tasks.
- Recurrent Neural Networks (RNNs): Effective for sequential data, such as time series or natural language.
- Generative Adversarial Networks (GANs): Used for generating new data samples that resemble the training data.
- Applications: Â
- Image recognition: Identifying objects within images for applications like facial recognition.
- Natural language processing: Enabling machines to understand and generate human language.
- Autonomous vehicles: Processing sensor data to navigate and make driving decisions.
- Deep learning computer vision applications that enhance image analysis capabilities.
Deep learning integration is revolutionizing fields such as healthcare, finance, and entertainment by providing powerful tools for data analysis and prediction. Its ability to learn from vast amounts of data makes it a cornerstone of modern artificial intelligence. At Rapid Innovation, we harness deep learning technologies to develop solutions that enhance operational efficiencies and drive innovation, ultimately leading to greater ROI for our clients, including machine learning in drug discovery and other innovative applications.
Refer to the image for a visual representation of the concepts discussed in the Supervised Learning Models section.
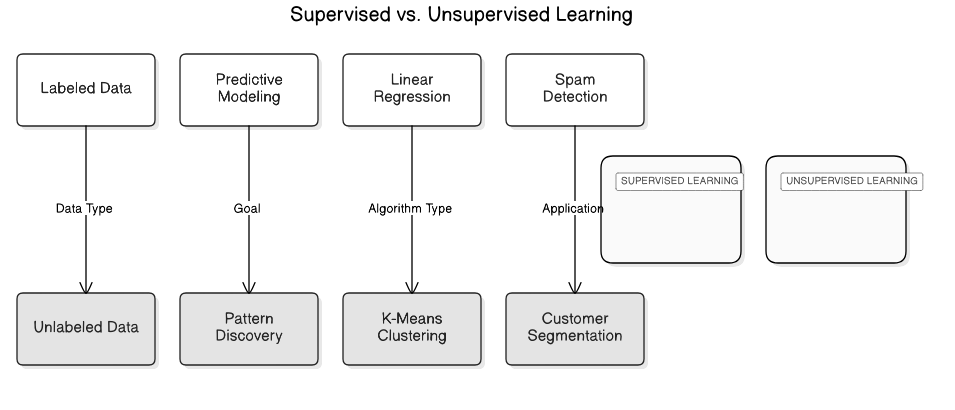
2.2. Natural Language Processing
Natural Language Processing (NLP) is a branch of artificial intelligence that focuses on the interaction between computers and humans through natural language. It enables machines to understand, interpret, and respond to human language in a valuable way. NLP is essential for various applications, including chatbots, sentiment analysis, and language translation. Natural language programming is a related concept that emphasizes the use of natural language for programming tasks.
- Key components of NLP include: Â
- Tokenization: Breaking down text into smaller units, such as words or phrases.
- Part-of-Speech Tagging: Identifying the grammatical parts of speech in a sentence.
- Named Entity Recognition: Detecting and classifying key entities in text, such as names, dates, and locations.
- Sentiment Analysis: Determining the emotional tone behind a series of words, which is crucial for understanding customer feedback.
- NLP techniques are widely used in: Â
- Chatbots: Enhancing customer service by providing instant responses to inquiries, thereby improving customer engagement and satisfaction.
- Search Engines: Improving search results by understanding user intent, which can lead to higher conversion rates.
- Content Recommendation: Analyzing user preferences to suggest relevant articles or products, ultimately driving sales and user retention.
- Challenges in NLP include: Â
- Ambiguity: Words can have multiple meanings depending on context, which can complicate understanding.
- Sarcasm and Irony: Understanding nuanced language can be difficult for machines, potentially leading to misinterpretations.
- Language Variability: Different dialects and slang can complicate processing, requiring tailored solutions for diverse user bases.
NLP continues to evolve with advancements in machine learning and deep learning, making it a critical area for businesses looking to leverage data-driven insights. At Rapid Innovation, we harness NLP technologies to help clients enhance customer interactions, streamline operations, and ultimately achieve greater ROI. The field of natural language analysis is also gaining traction, focusing on extracting insights from text data. For more information on NLP, you can visit this link.
2.3. Computer Vision for Document Processing
Computer Vision is a field of artificial intelligence that enables machines to interpret and make decisions based on visual data. In document processing, computer vision plays a crucial role in automating the extraction and analysis of information from various document types, such as invoices, contracts, and forms.
- Key applications of computer vision in document processing include: Â
- Optical Character Recognition (OCR): Converting different types of documents, such as scanned paper documents or PDFs, into editable and searchable data, which enhances accessibility and usability.
- Image Classification: Automatically categorizing documents based on their content, which streamlines organization and retrieval, saving time and resources.
- Data Extraction: Identifying and extracting specific data points from documents, such as dates, amounts, and names, to facilitate accurate record-keeping and reporting.
- Benefits of using computer vision in document processing: Â
- Increased Efficiency: Automating data entry reduces manual labor and speeds up processing times, allowing staff to focus on higher-value tasks.
- Improved Accuracy: Advanced algorithms minimize human error in data extraction, leading to more reliable data management.
- Cost Savings: Reducing the need for manual processing can lead to significant cost reductions, enhancing overall profitability.
- Challenges faced in computer vision for document processing: Â
- Quality of Input: Poor quality scans can hinder OCR accuracy, necessitating high-quality document management practices.
- Complex Layouts: Documents with intricate designs may complicate data extraction, requiring sophisticated algorithms to navigate.
- Language Variability: Different languages and fonts can affect recognition rates, necessitating adaptable solutions.
As technology advances, computer vision continues to enhance document processing capabilities, making it an invaluable tool for businesses aiming to optimize their workflows. Rapid Innovation leverages computer vision to help clients automate processes, reduce costs, and improve accuracy, ultimately driving better business outcomes.
2.4. Predictive Analytics Frameworks
Predictive analytics frameworks are systems that utilize statistical algorithms and machine learning techniques to analyze historical data and predict future outcomes. These frameworks are essential for businesses looking to make data-driven decisions and improve operational efficiency.
- Key components of predictive analytics frameworks include: Â
- Data Collection: Gathering relevant data from various sources, including databases, APIs, and user interactions, to create a comprehensive dataset.
- Data Preprocessing: Cleaning and transforming data to ensure accuracy and consistency, which is crucial for reliable predictions.
- Model Building: Developing predictive models using techniques such as regression analysis, decision trees, and neural networks, tailored to specific business needs.
- Model Evaluation: Assessing the performance of predictive models using metrics like accuracy, precision, and recall to ensure effectiveness.
- Applications of predictive analytics frameworks span various industries: Â
- Retail: Forecasting sales trends and optimizing inventory management, leading to reduced waste and increased sales.
- Healthcare: Predicting patient outcomes and improving treatment plans, enhancing patient care and operational efficiency.
- Finance: Assessing credit risk and detecting fraudulent transactions, safeguarding assets and improving financial decision-making.
- Benefits of implementing predictive analytics frameworks: Â
- Enhanced Decision-Making: Data-driven insights lead to more informed business strategies, improving overall performance.
- Risk Management: Identifying potential risks allows businesses to take proactive measures, reducing losses and enhancing stability.
- Customer Insights: Understanding customer behavior helps tailor marketing efforts and improve customer satisfaction, driving loyalty and revenue.
- Challenges in predictive analytics include: Â
- Data Quality: Inaccurate or incomplete data can lead to misleading predictions, necessitating robust data governance.
- Model Complexity: Developing and maintaining sophisticated models requires expertise and resources, which can be a barrier for some organizations.
- Interpretability: Understanding how models arrive at predictions can be difficult, especially with complex algorithms, requiring clear communication of insights.
Predictive analytics frameworks are becoming increasingly vital as organizations seek to harness the power of data to drive growth and innovation. At Rapid Innovation, we specialize in developing tailored predictive analytics solutions that empower clients to make informed decisions, mitigate risks, and maximize ROI. Additionally, the definition of NLP is crucial for understanding its role in the broader context of natural language programming and processing.
3. Key Use Cases
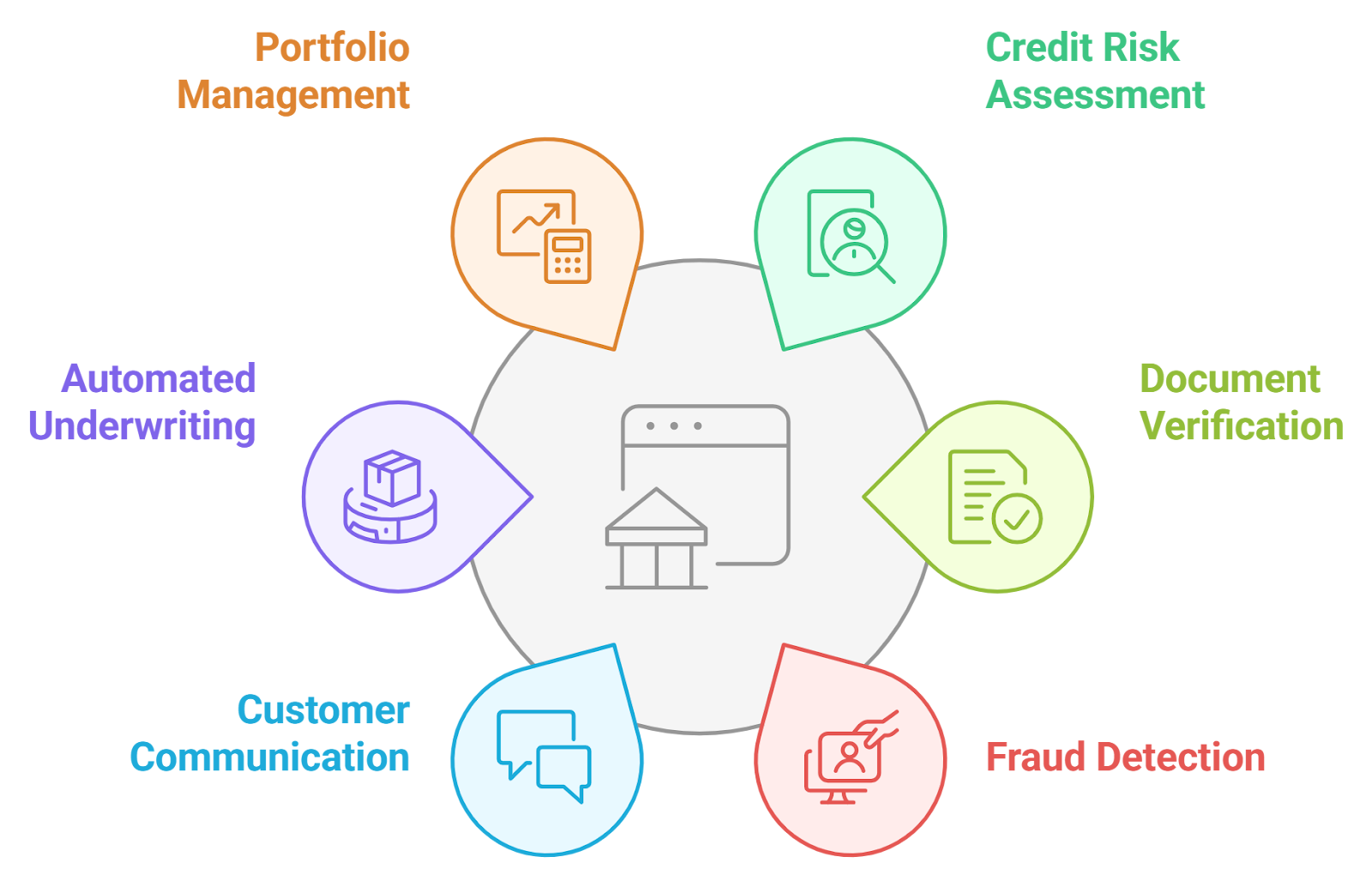
‍
3.1. Credit Risk Assessment
Credit risk assessment is a critical process for financial institutions, enabling them to evaluate the likelihood that a borrower will default on a loan. This assessment helps lenders make informed decisions about extending credit, setting interest rates, and managing their overall risk exposure. The use of advanced analytics and machine learning has transformed credit risk assessment, allowing for more accurate predictions and better risk management.
- Enhanced decision-making: By leveraging data analytics, lenders can make more informed decisions regarding creditworthiness, including customer credit risk assessment and assessing credit worthiness.
- Improved risk management: Financial institutions can better manage their portfolios by identifying high-risk borrowers early in the process, utilizing credit risk analysis and credit risk evaluation techniques.
- Increased efficiency: Automated credit risk assessment processes reduce the time and resources needed for manual evaluations, streamlining the credit risk assessment process.
3.1.1. Traditional Credit Scoring
Traditional credit scoring has been the cornerstone of credit risk assessment for decades. It typically relies on a borrower’s credit history, which is compiled into a credit report. The most common scoring models include FICO and VantageScore, which assign a numerical score based on various factors, such as credit history, credit utilization, and recent inquiries.
- Credit history: This includes the length of time accounts have been open, payment history, and the types of credit used.
- Credit utilization: This measures the amount of credit being used compared to the total available credit, typically expressed as a percentage.
- Recent inquiries: New credit applications can negatively impact a credit score, as they may indicate financial distress.
Traditional credit scoring has its limitations, including limited data sources, lack of inclusivity, and slow adaptation.
- Limited data sources: Traditional models often rely solely on credit history, which may not provide a complete picture of a borrower’s financial behavior, particularly in corporate credit assessment and credit assessments for SMEs.
- Lack of inclusivity: Individuals with limited credit histories, such as young adults or those new to the country, may struggle to obtain credit, highlighting the need for alternative credit risk assessment models.
- Slow adaptation: Traditional scoring models may not quickly adapt to changes in a borrower’s financial situation, leading to outdated assessments.
As the financial landscape evolves, there is a growing need for alternative credit scoring methods that incorporate a broader range of data sources and provide a more holistic view of a borrower’s creditworthiness. Rapid Innovation is at the forefront of this transformation, utilizing AI-driven analytics and blockchain technology to create more inclusive and adaptive credit assessment solutions. By integrating diverse data sources and employing machine learning algorithms, we help financial institutions enhance their credit risk assessment processes, including credit risk analysis models and loan risk assessment, ultimately leading to greater ROI and improved customer satisfaction.
3.1.2. Alternative Data Analysis
Alternative data analysis refers to the process of utilizing non-traditional data sources to gain insights into consumer behavior, creditworthiness, and risk assessment. This approach has gained traction in various industries, particularly in finance and lending, as it provides a more comprehensive view of an individual's or business's financial health.
- Types of alternative data include: Â
- Social media activity
- Online purchase history
- Utility payment records
- Mobile phone usage patterns
- Employment history
- Benefits of alternative data analysis: Â
- Enhanced credit scoring: Traditional credit scores may not reflect the true financial situation of individuals with limited credit history. Alternative data can fill this gap, allowing lenders to make more informed decisions.
- Improved risk assessment: By analyzing diverse data points, companies can better predict the likelihood of default or late payments.
- Broader access to credit: Alternative data can help underserved populations gain access to financial products, promoting financial inclusion.
- Challenges in alternative data analysis: Â
- Data privacy concerns: The use of personal data raises ethical questions and regulatory challenges.
- Data quality and reliability: Not all alternative data sources are accurate or consistent, which can lead to misleading conclusions.
- Integration with traditional data: Combining alternative data with existing systems can be complex and resource-intensive.
At Rapid Innovation, we leverage advanced AI algorithms to analyze alternative data, enabling our clients to enhance their credit scoring models and improve risk assessment processes. By integrating alternative data into their decision-making frameworks, businesses can achieve greater ROI through more accurate lending practices and expanded customer bases. This includes exploring alternative to NVivo for data analysis, which can provide innovative solutions for data analytics.
3.1.3. Behavioral Scoring
Behavioral scoring is a method used to evaluate an individual's or business's creditworthiness based on their past behaviors and actions rather than solely relying on traditional credit scores. This approach leverages data analytics to assess risk and predict future behavior.
- Key components of behavioral scoring: Â
- Transaction history: Analyzing spending patterns, payment timeliness, and transaction types.
- Engagement metrics: Evaluating how often a customer interacts with financial institutions or services.
- Risk indicators: Identifying red flags such as frequent overdrafts or missed payments.
- Advantages of behavioral scoring: Â
- More accurate risk assessment: By focusing on actual behavior, lenders can make better-informed decisions about creditworthiness.
- Dynamic scoring: Behavioral scores can be updated in real-time, reflecting changes in a consumer's financial situation.
- Customization: Lenders can tailor their offerings based on individual behavioral profiles, improving customer satisfaction.
- Limitations of behavioral scoring: Â
- Data dependency: The effectiveness of behavioral scoring relies heavily on the availability and quality of data.
- Potential biases: If not carefully managed, behavioral scoring can inadvertently reinforce existing biases in lending practices.
- Regulatory scrutiny: As with alternative data, behavioral scoring must navigate complex regulations regarding data usage and consumer privacy.
Rapid Innovation employs machine learning techniques to develop robust behavioral scoring models that provide our clients with a more nuanced understanding of their customers. This leads to improved risk management and ultimately enhances profitability.
3.2. Document Verification
Document verification is a critical process in various industries, particularly in finance, insurance, and legal sectors, where confirming the authenticity of documents is essential for compliance and risk management. This process involves validating the identity and credentials of individuals or entities through their submitted documents.
- Common types of documents verified: Â
- Government-issued identification (e.g., passports, driver's licenses)
- Financial statements (e.g., bank statements, tax returns)
- Employment verification documents (e.g., pay stubs, employment letters)
- Importance of document verification: Â
- Fraud prevention: Verifying documents helps mitigate the risk of identity theft and fraudulent activities.
- Regulatory compliance: Many industries are required to adhere to strict regulations that mandate thorough verification processes.
- Trust building: Ensuring the authenticity of documents fosters trust between businesses and their customers.
- Methods of document verification: Â
- Manual verification: Involves human review of documents, which can be time-consuming and prone to errors.
- Automated verification: Utilizing technology such as Optical Character Recognition (OCR) and machine learning algorithms to streamline the verification process.
- Third-party verification services: Engaging specialized firms that focus on document verification to enhance accuracy and efficiency.
- Challenges in document verification: Â
- Document forgery: As technology advances, so do the methods used to create counterfeit documents, making verification increasingly complex.
- Data privacy: Handling sensitive personal information requires strict adherence to data protection regulations.
- Integration with existing systems: Implementing document verification solutions can be challenging, especially for organizations with legacy systems.
At Rapid Innovation, we utilize blockchain technology to enhance document verification processes, ensuring data integrity and security. By implementing decentralized verification systems, we help our clients reduce fraud risk and comply with regulatory requirements, ultimately leading to increased trust and efficiency in their operations. Additionally, we explore alternative data analytics to further improve our verification processes.
3.2.1. ID Verification
ID verification is a crucial step in various processes, particularly in financial services, online transactions, and identity management. It ensures that the individual is who they claim to be, thereby reducing the risk of fraud and identity theft. The process typically involves checking government-issued identification documents such as passports, driver's licenses, or national ID cards. Advanced technologies like biometric verification (fingerprints, facial recognition) are increasingly being used to enhance security. Many organizations utilize third-party services to streamline ID verification, including services like irs identity verification and id verification irs, ensuring compliance with regulations like Know Your Customer (KYC) and Anti-Money Laundering (AML). The verification process may include cross-referencing the provided ID with databases to confirm authenticity, such as through the irs identity verification service. In some cases, additional steps like video verification or live photo submissions may be required to further validate identity.
- The process typically involves checking government-issued identification documents such as passports, driver's licenses, or national ID cards.
- Advanced technologies like biometric verification (fingerprints, facial recognition) are increasingly being used to enhance security.
- Many organizations utilize third-party services to streamline ID verification, ensuring compliance with regulations like Know Your Customer (KYC) and Anti-Money Laundering (AML).
- The verification process may include cross-referencing the provided ID with databases to confirm authenticity.
- In some cases, additional steps like video verification or live photo submissions may be required to further validate identity.
At Rapid Innovation, we leverage AI-driven solutions to enhance the ID verification process, ensuring a seamless and secure experience for our clients. By integrating advanced biometric technologies, we help organizations reduce fraud risk while maintaining compliance with regulatory standards, ultimately leading to greater ROI. Our services also include identity verification online and online identity verification service to cater to diverse client needs.
3.2.2. Income Documentation
Income documentation is essential for various financial applications, including loans, mortgages, and credit approvals. It provides lenders and financial institutions with a clear picture of an applicant's financial stability and ability to repay debts. Common forms of income documentation include pay stubs, tax returns, bank statements, and employment verification letters. Self-employed individuals may need to provide additional documentation, such as profit and loss statements or 1099 forms. Lenders often require documentation for a specific period, typically the last two years, to assess income consistency. Accurate income documentation helps in determining the debt-to-income ratio, which is a critical factor in loan approval. Digital platforms are increasingly offering automated income verification services, making the process faster and more efficient.
- Common forms of income documentation include pay stubs, tax returns, bank statements, and employment verification letters.
- Self-employed individuals may need to provide additional documentation, such as profit and loss statements or 1099 forms.
- Lenders often require documentation for a specific period, typically the last two years, to assess income consistency.
- Accurate income documentation helps in determining the debt-to-income ratio, which is a critical factor in loan approval.
- Digital platforms are increasingly offering automated income verification services, making the process faster and more efficient.
Rapid Innovation employs AI technologies to automate income documentation processes, significantly reducing the time and effort required for verification. Our solutions not only enhance accuracy but also improve the overall customer experience, leading to increased efficiency and higher returns on investment for our clients.
3.2.3. Asset Verification
Asset verification is the process of confirming the ownership and value of an individual's or entity's assets. This step is vital in various financial transactions, including loan applications, investment assessments, and estate planning. Common assets that may require verification include real estate, vehicles, bank accounts, and investment portfolios. Lenders often request documentation such as property deeds, vehicle titles, and account statements to validate asset ownership. The valuation of assets can be conducted through appraisals, market analysis, or financial statements. Asset verification helps lenders assess the overall financial health of an applicant, providing insights into their net worth and liquidity. In the digital age, many financial institutions are leveraging technology to automate asset verification, reducing the time and effort involved in the process.
- Common assets that may require verification include real estate, vehicles, bank accounts, and investment portfolios.
- Lenders often request documentation such as property deeds, vehicle titles, and account statements to validate asset ownership.
- The valuation of assets can be conducted through appraisals, market analysis, or financial statements.
- Asset verification helps lenders assess the overall financial health of an applicant, providing insights into their net worth and liquidity.
- In the digital age, many financial institutions are leveraging technology to automate asset verification, reducing the time and effort involved in the process.
At Rapid Innovation, we utilize blockchain technology to enhance asset verification processes, ensuring transparency and security in transactions. By providing immutable records of asset ownership, we help our clients build trust with stakeholders and streamline their operations, ultimately driving greater ROI. Our services also include identity verification companies and online id verification service to meet the diverse needs of our clients.
3.3. Fraud Detection
Fraud detection is a critical component in various industries, particularly in finance and insurance. The rise of digital transactions has led to an increase in fraudulent activities, making it essential for organizations to implement robust fraud detection systems, including fraud detection systems used by banks.
- Machine Learning Algorithms: At Rapid Innovation, we leverage advanced machine learning algorithms that analyze vast amounts of data to identify patterns and anomalies indicative of fraudulent behavior. By continuously learning from new data, these systems can adapt to evolving fraud tactics, ensuring that our clients stay one step ahead of potential threats. Our ai fraud detection solutions are designed to enhance this capability.
- Real-Time Monitoring: Our solutions include the implementation of real-time monitoring systems, such as fraud monitoring systems, enabling organizations to detect suspicious activities as they occur. This immediate response capability can prevent further losses and protect customer information, ultimately safeguarding the organization’s reputation and financial health.
- Multi-Factor Authentication: We recommend adding layers of security, such as multi-factor authentication, to significantly reduce the risk of fraud. This method requires users to provide multiple forms of verification before accessing sensitive information, enhancing overall security.
- Data Analytics: Utilizing our expertise in advanced data analytics, we help organizations sift through large datasets to identify potential fraud. By analyzing transaction histories and customer behaviors, our clients can flag unusual activities for further investigation, leading to more informed decision-making. This is particularly important in the context of credit card fraud detection systems.
- Collaboration with Law Enforcement: We encourage establishing partnerships with law enforcement agencies to enhance fraud detection efforts. By sharing information and resources, organizations can lead more effective investigations and prosecutions, thereby contributing to a safer business environment. This collaboration is crucial for effective fraud management solutions. For more insights on this topic, you can read about AI anomaly detection.
3.4. Customer Communication
Effective customer communication is vital for building trust and maintaining strong relationships with clients. In today's fast-paced digital world, organizations must adopt various strategies to ensure clear and timely communication.
- Omnichannel Communication: Rapid Innovation assists organizations in providing multiple channels for communication, such as email, chat, and social media, allowing customers to reach out in their preferred way. This flexibility enhances customer satisfaction and engagement.
- Personalization: We emphasize the importance of tailoring communication to individual customer needs and preferences, significantly improving the customer experience. Personalized messages resonate more with clients and foster loyalty.
- Proactive Communication: Keeping customers informed about changes, updates, or potential issues can prevent misunderstandings and build trust. Our strategies for proactive communication demonstrate that organizations value their customers.
- Feedback Mechanisms: Implementing feedback systems, such as surveys or reviews, allows customers to voice their opinions. This feedback can be invaluable for improving services and addressing concerns promptly.
- Crisis Communication: Having a clear crisis communication plan in place ensures that organizations can respond quickly and effectively during emergencies. Transparent communication during crises can mitigate damage and maintain customer trust.
3.5. Automated Underwriting
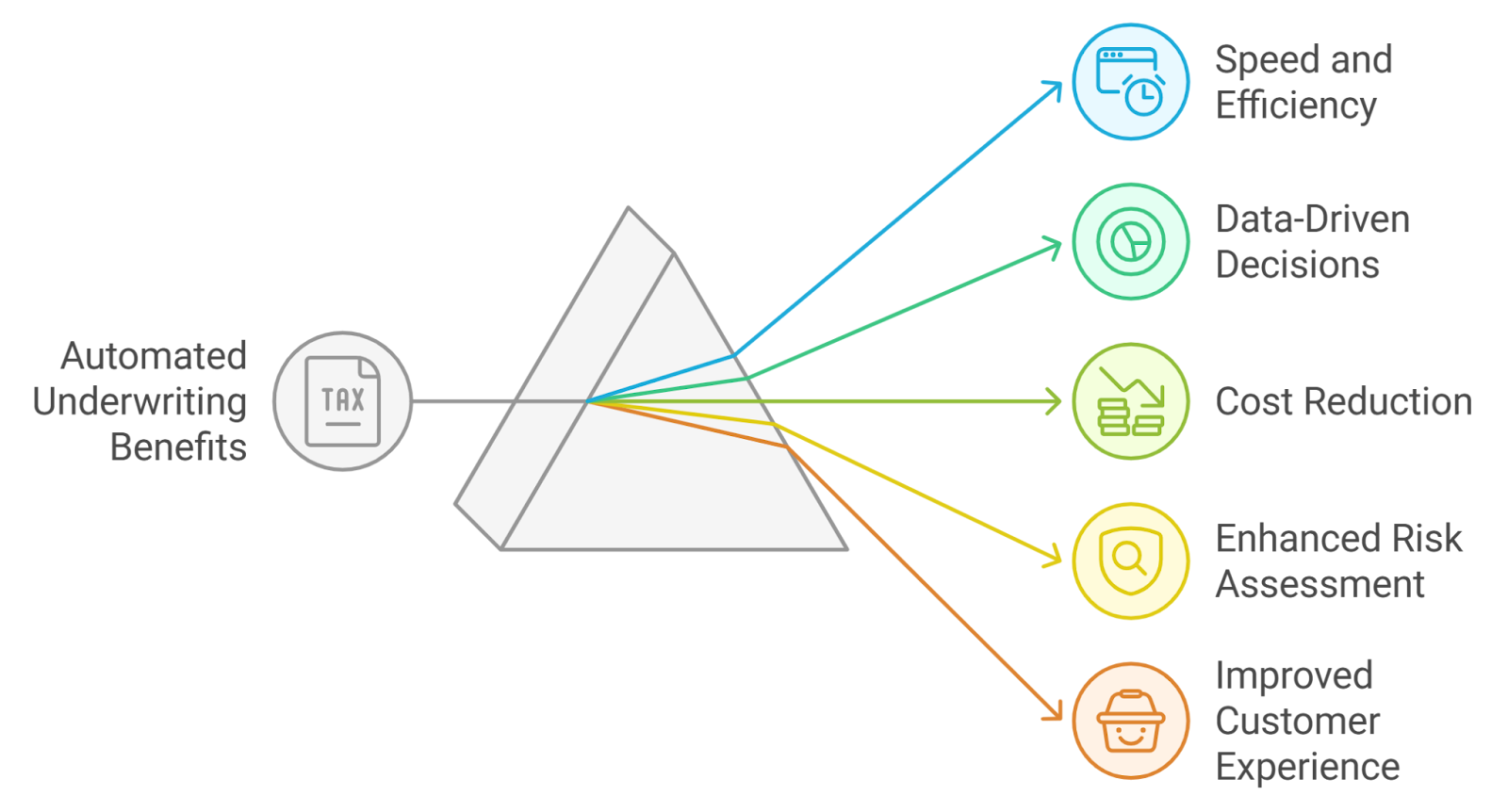
‍
Automated underwriting is revolutionizing the insurance and lending industries by streamlining the decision-making process. This technology leverages data and algorithms to assess risk and determine eligibility more efficiently.
- Speed and Efficiency: Rapid Innovation's automated underwriting solutions significantly reduce the time required to process applications. This efficiency allows organizations to serve customers faster and improve overall satisfaction.
- Data-Driven Decisions: By utilizing extensive data sources, our automated underwriting systems can make informed decisions based on a comprehensive analysis of risk factors. This data-driven approach enhances accuracy and reduces human error.
- Cost Reduction: Automating the underwriting process can lead to significant cost savings for organizations. By minimizing manual labor and reducing processing times, companies can allocate resources more effectively.
- Enhanced Risk Assessment: Our advanced algorithms analyze various risk factors, including credit scores, financial history, and market trends. This thorough assessment helps organizations make better-informed decisions regarding approvals and premiums.
- Improved Customer Experience: With faster processing times and more accurate assessments, customers benefit from a smoother application experience. This improvement can lead to higher retention rates and increased customer loyalty, ultimately driving greater ROI for our clients.
3.6. Portfolio Management
Portfolio management is a critical aspect of financial services, particularly in investment and asset management. It involves the strategic allocation of assets to achieve specific investment goals while managing risk. Effective portfolio management requires a deep understanding of market trends, asset classes, and individual client needs.
- Diversification: A well-managed portfolio spreads investments across various asset classes, such as stocks, bonds, and real estate, to minimize risk. This approach helps in mitigating losses during market downturns.
- Risk Assessment: Portfolio managers continuously assess the risk associated with different investments. They use various metrics, such as the Sharpe ratio and beta, to evaluate performance relative to risk.
- Performance Monitoring: Regularly reviewing portfolio performance is essential. Managers analyze returns against benchmarks and adjust strategies as needed to align with client objectives.
- Rebalancing: Over time, asset values fluctuate, which can lead to an unbalanced portfolio. Rebalancing involves realigning the portfolio to maintain the desired level of risk and return.
- Client Communication: Effective communication with clients about portfolio performance, market conditions, and strategy adjustments is vital for maintaining trust and transparency.
Incorporating technology, such as AI and machine learning, can enhance portfolio management by providing data-driven insights and automating routine tasks. This allows portfolio managers to focus on strategic decision-making and client relationships. At Rapid Innovation, we leverage our expertise in AI to develop tailored solutions that optimize portfolio management processes, ultimately leading to greater ROI for our clients.
The role of a chartered investment manager is crucial in this process, as they are responsible for managing investments and ensuring that the portfolio aligns with the client's financial goals. Additionally, project portfolio management (PPM) techniques can be applied to enhance the efficiency of managing multiple investment projects simultaneously. This includes the integration of project and portfolio management strategies to ensure that all investments are aligned with the overall financial objectives.
Furthermore, the concept of portfolio at risk is essential for understanding the potential downsides of investment strategies. Discretionary fund management allows portfolio managers to make investment decisions on behalf of clients, which can lead to more dynamic and responsive portfolio management.
In summary, effective portfolio management encompasses a range of strategies and practices, including project portfolio, financial portfolio, and portfolio mgmt, all aimed at optimizing investment outcomes while managing risk.
4.1.1. Processing Speed
Processing speed refers to the rate at which a system can execute tasks and deliver results. In today's fast-paced digital environment, organizations prioritize processing speed optimization to enhance productivity and efficiency. Faster processing speeds lead to quicker decision-making, while improved processing speeds can significantly reduce wait times for users. High-speed processing is essential for real-time data analysis and reporting. Additionally, technologies such as cloud computing and edge computing contribute to enhanced processing speeds. Businesses can leverage advanced algorithms and machine learning to optimize processing times, which is a core competency of Rapid Innovation.
Investing in high-performance hardware and software solutions can further boost processing speeds. For instance, utilizing solid-state drives (SSDs) instead of traditional hard drives can lead to substantial improvements in data retrieval times. Additionally, optimizing code and employing parallel processing techniques can also enhance overall system performance. Rapid Innovation specializes in implementing these advanced technologies to ensure that our clients achieve optimal processing speeds, ultimately leading to greater operational efficiency and ROI. Our expertise extends to providing AI business automation solutions that can further enhance processing capabilities.
4.1.2. Cost Reduction
Cost reduction is a critical objective for businesses aiming to improve their bottom line. By implementing strategies that lower operational costs, organizations can allocate resources more effectively and increase profitability. Streamlining processes can lead to significant savings in labor costs. Automation of repetitive tasks reduces the need for manual intervention, cutting down on errors and time. Utilizing cloud services can lower IT infrastructure costs by eliminating the need for on-premises hardware. Bulk purchasing and negotiating with suppliers can lead to lower material costs, while implementing energy-efficient practices can reduce utility expenses.
According to a report by McKinsey, companies that focus on cost reduction can improve their profit margins by up to 20%. By continuously evaluating and optimizing expenditures, businesses can maintain a competitive edge while ensuring sustainable growth. Rapid Innovation assists clients in identifying cost-saving opportunities through AI-driven analytics and blockchain solutions that enhance transparency and efficiency in supply chain management.
4.1.3. Resource Optimization
Resource optimization involves the strategic allocation and management of resources to maximize efficiency and effectiveness. This practice is essential for organizations looking to enhance productivity while minimizing waste. Identifying underutilized resources can lead to better allocation and reduced costs. Implementing project management tools can help track resource usage and improve planning. Regularly reviewing processes can uncover inefficiencies and areas for improvement. Training employees to use resources effectively can enhance overall productivity, and utilizing data analytics can provide insights into resource performance and help in making informed decisions.
Effective resource optimization not only improves operational efficiency but also contributes to sustainability efforts. By minimizing waste and maximizing the use of available resources, organizations can reduce their environmental impact while achieving their business objectives. Rapid Innovation employs cutting-edge AI and blockchain technologies to facilitate resource optimization, ensuring that our clients can achieve their goals while maintaining a commitment to sustainability.
4.2. Risk Management
Risk management is a critical component of any successful organization. It involves identifying, assessing, and prioritizing risks followed by coordinated efforts to minimize, monitor, and control the probability or impact of unfortunate events. Effective risk management helps organizations safeguard their assets, enhance decision-making, and ensure compliance with regulations.
- Identifying potential risks
- Assessing the likelihood and impact of risks
- Prioritizing risks based on their severity
- Implementing strategies to mitigate a risk
- Monitoring and reviewing risk management processes regularly
4.2.1. Enhanced Accuracy
Enhanced accuracy in risk management refers to the ability to make informed decisions based on precise data and analysis. Accurate risk assessment is essential for organizations to understand potential threats and opportunities. This can be achieved through:
- Utilization of advanced analytics and data modeling
- Implementation of real-time monitoring systems
- Regular updates to risk assessment frameworks
- Training staff on data interpretation and risk analysis
- Leveraging technology for predictive analytics
At Rapid Innovation, we harness the power of AI to enhance data accuracy and predictive capabilities. By integrating machine learning algorithms, we enable organizations to analyze vast datasets, identify patterns, and forecast potential risks with greater precision. This proactive approach allows our clients to implement more effective risk mitigation strategies, ultimately contributing to their overall stability and success. Additionally, we focus on risk management strategies that align with organizational goals and objectives. For more insights on this topic, check out our article on the future of personalized risk evaluation in insurance with AI agents.
4.2.2. Consistent Decision Making
Consistent decision-making is vital for effective risk management. It ensures that all stakeholders are aligned and that decisions are made based on established criteria and processes. Key elements include:
- Development of standardized risk assessment protocols
- Establishment of clear communication channels among teams
- Regular training sessions to reinforce decision-making frameworks
- Use of decision support systems to guide choices
- Continuous evaluation of decision outcomes to refine processes
When organizations adopt a consistent approach to decision-making, they can reduce uncertainty and enhance their ability to respond to risks. This consistency fosters trust among stakeholders and promotes a culture of accountability, ultimately leading to better organizational performance. At Rapid Innovation, we assist clients in developing robust decision support systems powered by blockchain technology, ensuring transparency and traceability in decision-making processes. This not only enhances accountability but also builds confidence among stakeholders, driving better organizational outcomes. Our focus on risk handling strategies and strategic risk management ensures that organizations are well-prepared to navigate uncertainties.
4.2.3. Real-time Risk Monitoring
Real-time risk monitoring is a crucial component in today's financial landscape, especially for institutions that deal with large volumes of transactions. This process involves continuously assessing and analyzing risk factors as they occur, allowing organizations to respond swiftly to potential threats.
- Enhances decision-making: By having access to real-time data, organizations can make informed decisions quickly, reducing the likelihood of losses. Rapid Innovation leverages AI algorithms to provide clients with actionable insights, ensuring they can navigate risks effectively.
- Improves compliance: Real-time monitoring helps ensure that organizations adhere to regulatory requirements, minimizing the risk of penalties. Our blockchain solutions facilitate transparent and immutable record-keeping, enhancing compliance efforts.
- Detects fraud: Continuous monitoring allows for the immediate identification of suspicious activities, enabling rapid intervention. Utilizing machine learning, Rapid Innovation develops systems that can detect anomalies in transaction patterns, significantly reducing fraud risk.
- Utilizes advanced technologies: Machine learning and artificial intelligence play significant roles in real-time risk monitoring, analyzing vast amounts of data to identify patterns and anomalies. Our expertise in these technologies empowers clients to implement robust risk management frameworks.
- Increases operational efficiency: By automating risk assessments, organizations can allocate resources more effectively and focus on strategic initiatives. Rapid Innovation's solutions streamline processes, allowing clients to enhance their operational capabilities.
4.3. Customer Experience
Customer experience (CX) is a vital aspect of any business, particularly in the financial sector. A positive customer experience can lead to increased loyalty, higher retention rates, and ultimately, greater profitability.
- Personalization: Tailoring services and communications to individual customer preferences enhances satisfaction and engagement. Rapid Innovation employs AI-driven analytics to help clients understand customer behavior and preferences, enabling personalized interactions.
- Omnichannel support: Providing seamless interactions across various platforms (online, mobile, in-person) ensures customers can access services conveniently. Our solutions integrate various channels, ensuring a cohesive customer experience.
- Feedback mechanisms: Actively seeking customer feedback helps organizations understand pain points and areas for improvement. We assist clients in implementing AI tools that analyze customer feedback, driving continuous improvement.
- Speed and efficiency: Streamlined processes and quick responses to inquiries contribute to a more satisfying customer journey. Rapid Innovation's automation solutions enhance response times, ensuring customers receive timely assistance.
- Trust and transparency: Clear communication regarding fees, policies, and procedures fosters trust and strengthens customer relationships. Our blockchain technology ensures transparency in transactions, building customer confidence.
4.3.1. Faster Approval Times
Faster approval times are essential for enhancing customer experience in the financial industry. Customers expect quick responses, especially when applying for loans or credit.
- Streamlined processes: Implementing automated systems can significantly reduce the time taken to process applications. Rapid Innovation designs tailored automation solutions that expedite application processing.
- Digital documentation: Allowing customers to submit documents electronically speeds up the verification process. Our platforms facilitate secure digital submissions, enhancing the customer experience.
- Real-time data access: Utilizing real-time data for credit assessments enables quicker decision-making. We integrate advanced data analytics to provide clients with immediate insights, accelerating approval times.
- Enhanced communication: Keeping customers informed throughout the approval process helps manage expectations and reduces anxiety. Our solutions include automated communication tools that keep customers updated in real-time.
- Competitive advantage: Organizations that can offer faster approval times are more likely to attract and retain customers in a crowded market. Rapid Innovation empowers clients to differentiate themselves through efficient processes and superior customer service.
Incorporating real-time risk monitoring into these processes ensures that organizations can maintain a proactive stance against potential threats while enhancing customer experience. For more information on how we can assist with AI-driven solutions, check out our AI customer care solutions.
4.3.2. 24/7 Availability
In today's fast-paced digital world, 24/7 availability is crucial for businesses aiming to provide exceptional customer service. This constant accessibility ensures that customers can reach out for support or information at any time, enhancing their overall experience. Customers expect immediate responses, regardless of the hour. Businesses can leverage AI-driven chatbots and automated systems to provide instant replies, while human support can be scheduled around the clock to handle complex inquiries. For instance, companies like Verizon customer service 24 7 and AT&T prepaid customer service number 24 7 exemplify this commitment to constant availability. This availability can lead to increased customer satisfaction and loyalty. Additionally, companies that offer 24/7 support, such as directv customer service number 24 7, often see a boost in sales, as customers can make purchases at their convenience.
Having a 24/7 support system not only meets customer expectations but also positions a business as reliable and customer-centric. Rapid Innovation can assist in implementing these AI solutions, ensuring that your business remains accessible and responsive, ultimately driving greater ROI.
4.3.3. Personalized Services
Personalized services are essential for creating a unique customer experience. By tailoring services to individual preferences and needs, businesses can foster stronger relationships with their customers. Utilizing customer data allows businesses to understand preferences and behaviors. Personalized recommendations can enhance the shopping experience, leading to higher conversion rates. Furthermore, communication can be customized based on customer history, making interactions more relevant. Loyalty programs can be tailored to reward individual customer behaviors, increasing engagement. Ultimately, personalized services can differentiate a brand in a competitive market.
Investing in personalized services can lead to increased customer retention and satisfaction, ultimately driving business growth. Rapid Innovation leverages advanced AI analytics to help businesses harness customer data effectively, ensuring that your offerings resonate with your target audience.
4.4. Scalability and Flexibility
Scalability and flexibility are vital for businesses looking to adapt to changing market conditions and customer demands. These attributes allow companies to grow without compromising service quality. Scalable solutions enable businesses to expand their operations seamlessly. Cloud-based technologies provide the flexibility to adjust resources based on demand. Additionally, businesses can quickly pivot their strategies in response to market trends. Flexibility in service offerings allows companies to cater to diverse customer needs, while scalable systems can help manage increased workloads during peak times without sacrificing performance. For example, companies like Square customer service number 24 7 and Verizon 24 7 customer support demonstrate the importance of being adaptable.
Emphasizing scalability and flexibility ensures that a business can thrive in a dynamic environment, maintaining a competitive edge while meeting customer expectations. Rapid Innovation specializes in developing blockchain solutions that enhance scalability and security, enabling your business to adapt and grow efficiently.
5. Implementation Challenges
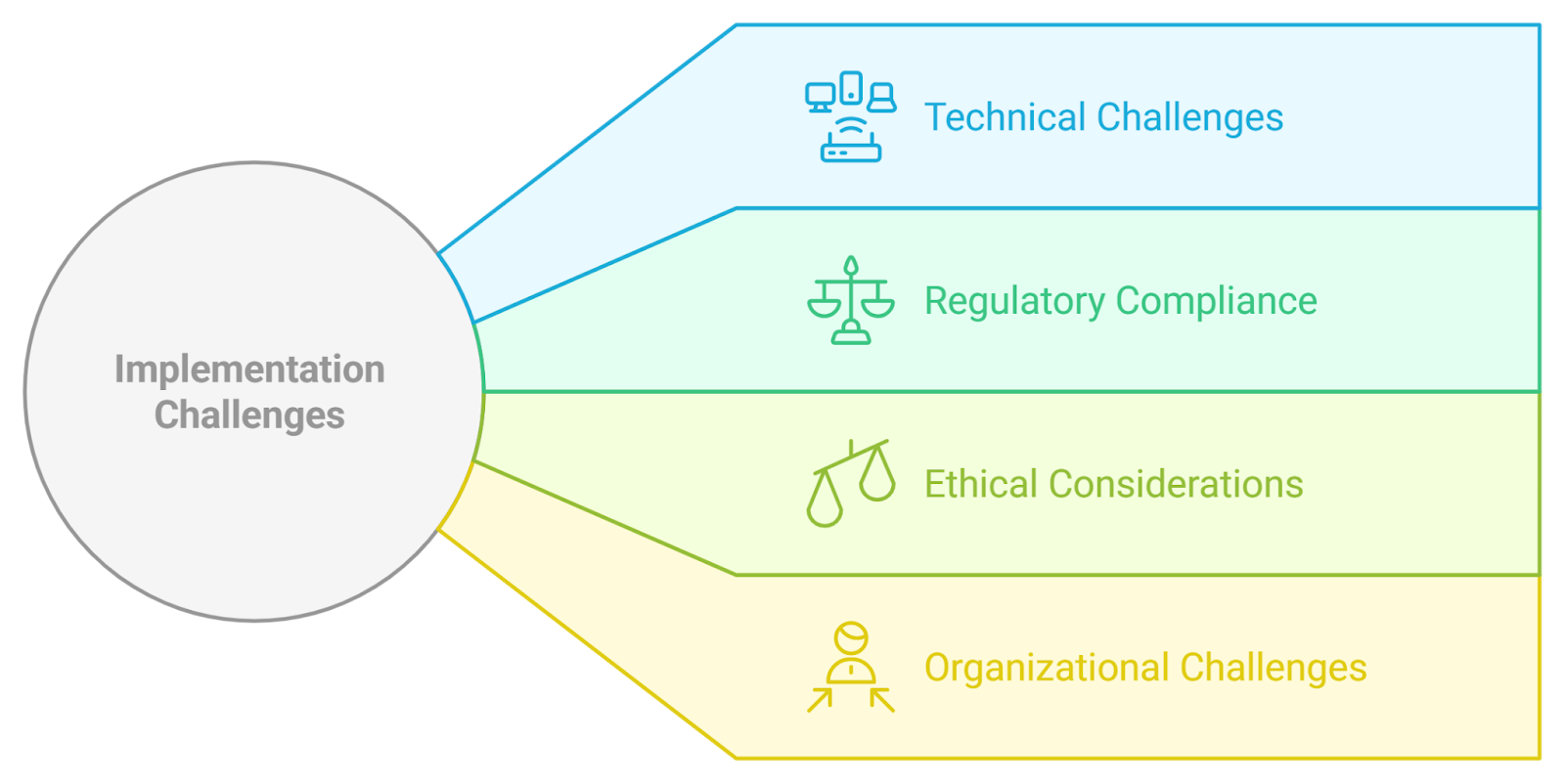
‍
Implementing new systems or processes often comes with a variety of challenges that can hinder progress and affect outcomes. Understanding these challenges is crucial for successful implementation, especially when it comes to challenges implementing electronic health records, challenges in implementing ehr, and emr implementation challenges.
5.1. Technical Challenges
Technical challenges are among the most significant hurdles organizations face during implementation. These challenges can stem from outdated technology, lack of integration, or insufficient technical expertise. Addressing these issues is essential for ensuring a smooth transition and achieving desired results. Legacy systems may not support new technologies, leading to compatibility issues. Integration of various systems can be complex and time-consuming. Additionally, insufficient technical skills within the team can slow down the implementation process, particularly in software implementation challenges.
5.1.1. Data Quality and Availability
Data quality and availability are critical components of any successful implementation. Poor data quality can lead to incorrect insights, while a lack of data availability can hinder decision-making processes. Inaccurate data can result from human error, outdated information, or inconsistent data entry practices. Data silos within an organization can limit access to necessary information, making it difficult to obtain a comprehensive view. Furthermore, organizations may struggle with data governance, leading to compliance issues and potential legal ramifications, which can be exacerbated by barriers to implementing ehr.
To address these challenges, organizations should focus on:
- Establishing clear data management policies to ensure data accuracy and consistency.
- Investing in data integration tools to break down silos and improve accessibility.
- Conducting regular data audits to identify and rectify quality issues.
At Rapid Innovation, we leverage our expertise in AI and Blockchain to help organizations overcome these technical challenges. For instance, our AI-driven data management solutions can enhance data quality by automating data entry and validation processes, significantly reducing human error. Additionally, our Blockchain technology can ensure data integrity and transparency, addressing governance concerns effectively.
By prioritizing data quality and availability, organizations can enhance their implementation efforts and drive better outcomes, ultimately achieving greater ROI with the support of Rapid Innovation's tailored solutions. This is particularly important when considering top 10 ehr implementation challenges and how to overcome them, as well as the broader context of implementation challenges like workday implementation challenges and sap implementation challenges.
5.1.2. System Integration
System integration is a critical aspect of modern technology infrastructure, ensuring that various subsystems work together seamlessly. This process involves combining different computing systems and software applications physically or functionally to act as a coordinated whole. Effective system integration enhances operational efficiency by streamlining processes, facilitates data sharing across different platforms to reduce redundancy, supports real-time data access essential for decision-making, improves user experience by providing a unified interface, and reduces operational costs by minimizing the need for multiple systems.
At Rapid Innovation, we leverage our expertise in AI and Blockchain to implement robust system integration solutions that not only connect disparate systems but also enhance data integrity and security. For instance, by utilizing blockchain technology, we can ensure that data shared across systems is tamper-proof and verifiable, thereby increasing trust among stakeholders. Additionally, our AI-driven middleware solutions can intelligently route data and automate workflows, further optimizing operational efficiency. We also specialize in integrating platforms such as salesforce crm, sage intacct, and shopify erp integration to create a cohesive operational environment.
Effective system integration requires careful planning and execution. Organizations often utilize middleware solutions to connect disparate systems, allowing them to communicate and share data effectively. Additionally, APIs (Application Programming Interfaces) play a vital role in enabling integration by allowing different software applications to interact. Solutions like integration software and system integration software are essential for achieving seamless connectivity. Furthermore, our expertise in enterprise building integrator and data integration software ensures that organizations can effectively manage their integration needs.
5.1.3. Model Maintenance
Model maintenance is essential for ensuring that predictive models remain accurate and relevant over time. As data evolves, models must be updated to reflect new trends and patterns. This process involves regular monitoring of model performance to identify any degradation, retraining models with new data to improve accuracy, validating models to ensure they meet performance standards, documenting changes made to models for transparency, and supporting compliance with industry standards and regulations.
At Rapid Innovation, we implement a comprehensive model maintenance framework that includes automated monitoring and retraining processes powered by AI. This proactive approach helps in mitigating risks associated with outdated models and ensures that the insights derived from them remain actionable, ultimately driving greater ROI for our clients. Our model maintenance strategies also encompass integration with systems like netsuite integrations and paycom netsuite integration to ensure that all data sources are accurately reflected in the models.
Organizations should establish a robust model maintenance framework that includes scheduled reviews and updates. This proactive approach helps in mitigating risks associated with outdated models and ensures that the insights derived from them remain actionable.
5.2. Regulatory Compliance
Regulatory compliance refers to the adherence to laws, regulations, guidelines, and specifications relevant to an organization’s business processes. It is crucial for maintaining operational integrity and avoiding legal penalties. Compliance ensures that organizations meet industry standards and legal requirements, protects consumer rights and enhances trust in the organization, reduces the risk of financial penalties and legal issues, promotes ethical business practices and corporate governance, and facilitates smoother audits and inspections by regulatory bodies.
At Rapid Innovation, we understand the complexities of regulatory compliance in the rapidly evolving landscape of AI and Blockchain. Our solutions are designed to help organizations stay informed about the regulatory landscape, which can vary significantly across industries and regions. Implementing compliance management systems can help streamline the process of monitoring and adhering to regulations. Regular training and awareness programs for employees are also essential to foster a culture of compliance within the organization. By integrating compliance into the core of your business processes, we help you mitigate risks and enhance operational integrity, ultimately leading to improved business outcomes. Our experience with systems integrations, such as sage and intacct marketplace and sugarcrm crm, further supports our commitment to compliance and operational excellence.
5.2.1. Fair Lending Laws
Fair lending laws are designed to ensure that all individuals have equal access to credit and financial services, regardless of their race, color, religion, national origin, sex, marital status, age, or because they receive public assistance. These laws are crucial in promoting fairness and preventing discrimination in lending practices. The primary legislation governing fair lending includes the Equal Credit Opportunity Act (ECOA) and the Fair Housing Act (FHA). ECOA prohibits lenders from discriminating against applicants based on protected characteristics, while FHA focuses on preventing discrimination in housing-related transactions, including mortgage lending. Regulatory bodies, such as the Consumer Financial Protection Bureau (CFPB) and the Department of Housing and Urban Development (HUD), enforce these laws. Lenders must conduct regular audits and assessments to ensure compliance with fair lending laws, as non-compliance can lead to severe penalties, including fines and loss of lending licenses.
At Rapid Innovation, we leverage AI-driven analytics to help financial institutions assess their lending practices against fair lending laws. By utilizing machine learning algorithms, we can identify patterns of potential discrimination in lending decisions, enabling our clients to proactively address compliance issues and enhance their reputation. This not only mitigates the risk of penalties but also fosters trust with consumers, ultimately leading to greater ROI.
- The primary legislation governing fair lending includes the Equal Credit Opportunity Act (ECOA) and the Fair Housing Act (FHA).
- ECOA prohibits lenders from discriminating against applicants based on protected characteristics.
- FHA focuses on preventing discrimination in housing-related transactions, including mortgage lending.
- Regulatory bodies, such as the Consumer Financial Protection Bureau (CFPB) and the Department of Housing and Urban Development (HUD), enforce these laws.
- Lenders must conduct regular audits and assessments to ensure compliance with fair lending laws, including fair lending risk assessment and fair lending compliance.
- Non-compliance can lead to severe penalties, including fines and loss of lending licenses, making a fair lending compliance program essential.
5.2.2. Privacy Regulations
Privacy regulations are essential for protecting consumers' personal information in the financial sector. These regulations dictate how financial institutions collect, use, and share customer data, ensuring that individuals' privacy rights are respected. The Gramm-Leach-Bliley Act (GLBA) requires financial institutions to explain their information-sharing practices to customers and to safeguard sensitive data. The Fair Credit Reporting Act (FCRA) regulates the collection and use of consumer credit information, ensuring accuracy and privacy. Institutions must implement robust data protection measures, including encryption and secure storage, to prevent data breaches. Consumers have the right to access their personal information and request corrections if inaccuracies are found. Non-compliance with privacy regulations can result in significant fines and reputational damage for financial institutions.
At Rapid Innovation, we utilize blockchain technology to enhance data privacy and security for financial institutions. By implementing decentralized data storage solutions, we ensure that sensitive customer information is protected against unauthorized access and breaches. This not only helps our clients comply with privacy regulations but also builds consumer confidence, leading to increased customer loyalty and higher returns on investment.
- The Gramm-Leach-Bliley Act (GLBA) requires financial institutions to explain their information-sharing practices to customers and to safeguard sensitive data.
- The Fair Credit Reporting Act (FCRA) regulates the collection and use of consumer credit information, ensuring accuracy and privacy.
- Institutions must implement robust data protection measures, including encryption and secure storage, to prevent data breaches.
- Consumers have the right to access their personal information and request corrections if inaccuracies are found.
- Non-compliance with privacy regulations can result in significant fines and reputational damage for financial institutions.
5.2.3. Model Governance
Model governance refers to the framework and processes that financial institutions use to manage and validate their predictive models, particularly those used in risk assessment, credit scoring, and pricing. Effective model governance is critical for ensuring that models are accurate, reliable, and compliant with regulatory standards. A robust model governance framework includes model development, validation, implementation, and ongoing monitoring. Institutions should establish clear roles and responsibilities for model risk management, including a dedicated model risk management team. Regular validation of models is necessary to ensure they perform as expected and remain relevant in changing market conditions. Documentation is crucial; institutions must maintain comprehensive records of model development processes, assumptions, and validation results. Regulatory bodies, such as the Office of the Comptroller of the Currency (OCC), provide guidelines for model risk management that institutions must follow. Failure to adhere to model governance standards can lead to inaccurate risk assessments and potential regulatory scrutiny.
Rapid Innovation offers advanced AI solutions that streamline model governance processes. Our AI tools can automate model validation and monitoring, ensuring that predictive models remain compliant and effective in real-time. By reducing the manual effort involved in model governance, we help our clients save time and resources, ultimately leading to improved operational efficiency and a higher return on investment.
- A robust model governance framework includes model development, validation, implementation, and ongoing monitoring.
- Institutions should establish clear roles and responsibilities for model risk management, including a dedicated model risk management team.
- Regular validation of models is necessary to ensure they perform as expected and remain relevant in changing market conditions.
- Documentation is crucial; institutions must maintain comprehensive records of model development processes, assumptions, and validation results.
- Regulatory bodies, such as the Office of the Comptroller of the Currency (OCC), provide guidelines for model risk management that institutions must follow.
- Failure to adhere to model governance standards can lead to inaccurate risk assessments and potential regulatory scrutiny.
5.3. Ethical Considerations
Ethical considerations are crucial in any field, especially in technology and data-driven industries. As organizations increasingly rely on algorithms and artificial intelligence (AI), it is essential to address the ethical implications of these technologies, including the ethical considerations of AI. Ethical considerations encompass various aspects, including bias, fairness, and transparency. These elements are vital for building trust and ensuring that technology serves all segments of society equitably.
5.3.1. Bias and Fairness
Bias in algorithms can lead to unfair treatment of individuals or groups, often perpetuating existing societal inequalities. It is essential to identify and mitigate bias to ensure fairness in AI systems, which is a key aspect of ethical considerations in AI.
- Types of Bias: Â
- Data bias occurs when the training data reflects historical prejudices or imbalances.
- Algorithmic bias arises from the design of the algorithm itself, which may favor certain outcomes over others.
- Impact of Bias: Â
- Biased algorithms can lead to discriminatory practices in hiring, lending, law enforcement, and more. For instance, studies have shown that facial recognition systems misidentify individuals from minority groups at higher rates than those from majority groups.
- Mitigation Strategies: Â
- Regular audits of algorithms to identify and rectify biases.
- Diverse data sets that represent various demographics to train AI systems.
- Involving stakeholders from different backgrounds in the development process to ensure a broader perspective.
- Fairness Metrics: Â
- Implementing fairness metrics can help assess the performance of algorithms across different demographic groups.
- Techniques such as equal opportunity and demographic parity can be used to evaluate fairness.
5.3.2. Transparency
Transparency in AI and algorithmic decision-making is essential for accountability and trust. Stakeholders need to understand how decisions are made and the factors influencing those decisions, which ties into the ethical considerations in machine learning.
- Importance of Transparency: Â
- Transparency helps users comprehend the workings of AI systems, fostering trust and acceptance.
- It allows for scrutiny and accountability, enabling stakeholders to challenge decisions that may be unjust or biased.
- Methods to Enhance Transparency: Â
- Clear documentation of algorithms, including their purpose, data sources, and decision-making processes.
- User-friendly explanations of how AI systems operate, making it easier for non-experts to understand.
- Regulatory Frameworks: Â
- Governments and organizations are increasingly advocating for regulations that mandate transparency in AI systems.
- The European Union's General Data Protection Regulation (GDPR) emphasizes the right to explanation, requiring organizations to provide clear information about automated decision-making processes.
- Challenges to Transparency: Â
- Proprietary algorithms may limit the ability to disclose inner workings, raising concerns about accountability.
- The complexity of AI systems can make it difficult to provide straightforward explanations.
- Tools for Transparency: Â
- Techniques such as explainable AI (XAI) aim to make AI systems more interpretable.
- Visualization tools can help stakeholders understand how algorithms arrive at specific decisions.
By addressing bias and fairness, as well as enhancing transparency, organizations can navigate the ethical landscape of AI and technology more effectively. These considerations are not just about compliance; they are about fostering a more equitable and trustworthy technological future. At Rapid Innovation, we prioritize these ethical considerations in our AI and Blockchain solutions, including the potential benefits and ethical considerations of artificial intelligence, ensuring that our clients achieve their business goals while upholding the highest standards of integrity and responsibility. Additionally, we recognize the importance of conversational AI social and ethical considerations, as well as medical ethics considerations on artificial intelligence, to ensure a comprehensive approach to ethical AI development.
5.3.3. Accountability
Accountability in an organization refers to the obligation of individuals or teams to report on their activities, accept responsibility for their actions, and disclose results in a transparent manner. It is a critical component of effective governance and management, particularly in the context of AI and Blockchain initiatives.
- Promotes trust: When employees know they are accountable, they are more likely to act responsibly and ethically, fostering a culture that is essential for the successful implementation of AI and Blockchain technologies.
- Enhances performance: Clear accountability structures can lead to improved performance as individuals understand their roles and responsibilities, especially in complex projects that involve AI algorithms or Blockchain protocols.
- Encourages ownership: Employees who are held accountable are more likely to take ownership of their tasks and outcomes, which is crucial in fast-paced environments where innovation is key.
- Fosters a culture of feedback: Accountability encourages open communication and constructive feedback, which can lead to continuous improvement in processes and technologies, particularly in iterative AI development cycles.
- Supports decision-making: When accountability is established, it becomes easier to make informed decisions based on clear data and results, enabling organizations to leverage AI insights and Blockchain transparency effectively.
Organizations can implement organizational accountability through various means, such as performance reviews, regular check-ins, and transparent reporting systems. Establishing clear expectations and consequences for actions is essential for fostering a culture of accountability.
5.4. Organizational Challenges
Organizational challenges refer to the obstacles that companies face in achieving their goals and objectives. These challenges can stem from various sources, including internal processes, external market conditions, and workforce dynamics.
- Resistance to change: Employees may resist new initiatives or changes in processes, leading to stagnation, particularly when adopting advanced technologies like AI and Blockchain.
- Communication barriers: Poor communication can result in misunderstandings and misalignment of goals, which can be detrimental when integrating new technologies that require cross-functional collaboration.
- Resource constraints: Limited financial or human resources can hinder project execution and innovation, especially in tech-driven initiatives that require specialized skills.
- Cultural differences: In diverse workplaces, varying cultural backgrounds can lead to conflicts or misinterpretations, impacting team dynamics and project outcomes.
- Technological advancements: Keeping up with rapid technological changes can be overwhelming for organizations, particularly in the fast-evolving fields of AI and Blockchain.
Addressing these challenges requires a strategic approach, including effective leadership, employee engagement, and continuous training. Organizations must be proactive in identifying potential obstacles and developing solutions to overcome them.
5.4.1. Change Management
Change management is the structured approach to transitioning individuals, teams, and organizations from a current state to a desired future state. It is essential for ensuring that changes are implemented smoothly and effectively, particularly in the context of AI and Blockchain transformations.
- Importance of change management: Â
- Minimizes resistance: A well-planned change management strategy can reduce employee resistance to change, facilitating smoother adoption of AI and Blockchain technologies.
- Enhances engagement: Involving employees in the change process fosters a sense of ownership and commitment, which is vital for the success of innovative projects.
- Improves outcomes: Effective change management can lead to better project outcomes and higher success rates, particularly in complex technology implementations.
- Key components of change management: Â
- Communication: Clear and consistent communication is vital to inform employees about the change and its benefits, especially when introducing new AI tools or Blockchain systems.
- Training: Providing training and resources helps employees adapt to new processes or technologies, ensuring they are equipped to leverage AI and Blockchain effectively.
- Support systems: Establishing support systems, such as mentoring or coaching, can ease the transition for employees, fostering a culture of continuous learning.
- Change management models: Â
- Kotter’s 8-Step Process: This model outlines steps for successful change, including creating urgency and building a guiding coalition, which can be particularly effective in tech-driven environments.
- ADKAR Model: Focuses on individual change and includes Awareness, Desire, Knowledge, Ability, and Reinforcement, ensuring that all employees are aligned with the organization's vision.
Implementing effective change management practices can significantly enhance an organization's ability to adapt to new challenges and opportunities. By prioritizing change management, organizations can ensure a smoother transition and maintain productivity during periods of change, ultimately leading to greater ROI in their AI and Blockchain initiatives.
5.4.2. Skill Gap
The skill gap refers to the disparity between the skills that employers need and the skills that job seekers possess. This gap can significantly hinder organizational growth and innovation.
- Rapid technological advancements often outpace the current workforce's skills, particularly in fields like AI and Blockchain, where new tools and methodologies emerge frequently.
- Many industries, such as technology and healthcare, are experiencing a shortage of qualified candidates, making it challenging for organizations to leverage cutting-edge solutions.
- Continuous learning and upskilling are essential to bridge this gap. Rapid Innovation offers tailored training programs, including gap negotiation training and gap partnership negotiation training, that equip employees with the necessary skills in AI and Blockchain technologies.
- Employers may need to invest in training programs, such as skill gap training programs, to develop their workforce's capabilities, ensuring they can effectively implement innovative solutions.
- Collaboration with educational institutions can help align curricula with industry needs, fostering a pipeline of talent proficient in the latest technologies.
- The rise of remote work has also changed the skill requirements, emphasizing digital literacy and communication skills, which are critical for successful collaboration in tech-driven environments.
Addressing the skill gap is crucial for maintaining competitiveness in the market. Organizations that prioritize skill development can enhance employee satisfaction and retention, ultimately leading to better performance and productivity. For more insights on bridging the skill gap, check out successful AI integration strategies.
5.4.3. Cultural Resistance
Cultural resistance refers to the reluctance of individuals or groups to adopt new ideas, practices, or technologies within an organization. This resistance can stem from various factors, including:
- Fear of change: Employees may feel threatened by new processes or technologies that could alter their roles, particularly in the context of AI and Blockchain integration.
- Lack of trust: If employees do not trust leadership or the rationale behind changes, they may resist. Building trust is essential for successful technology adoption.
- Established norms: Long-standing practices can create a comfort zone, making it difficult for employees to embrace new methods, especially when transitioning to innovative solutions.
- Insufficient communication: A lack of clear communication about the benefits and necessity of changes can lead to misunderstandings and resistance.
To overcome cultural resistance, organizations should focus on:
- Engaging employees in the change process to foster a sense of ownership, particularly when implementing AI and Blockchain solutions.
- Providing training and resources to ease the transition, ensuring that employees feel confident in their ability to adapt.
- Communicating the benefits of change clearly and consistently, highlighting how new technologies can enhance productivity and efficiency.
- Celebrating small wins to build momentum and encourage acceptance, reinforcing the positive impact of new initiatives.
Addressing cultural resistance is vital for the successful implementation of new strategies and technologies, ensuring that the workforce is aligned with organizational goals.
6. Implementation Strategy

‍
An effective implementation strategy is essential for translating plans into action. This strategy outlines the steps necessary to achieve organizational objectives while addressing potential challenges.
- Define clear goals: Establish specific, measurable, achievable, relevant, and time-bound (SMART) objectives to guide the implementation process.
- Develop a detailed action plan: Outline the tasks, timelines, and responsibilities for each phase of the implementation, particularly for AI and Blockchain projects.
- Allocate resources: Ensure that adequate resources, including budget, personnel, and technology, are available to support the implementation.
- Foster stakeholder engagement: Involve key stakeholders throughout the process to gain buy-in and support, which is crucial for successful technology adoption.
- Monitor progress: Regularly assess the implementation's progress against established goals and make adjustments as needed.
- Provide training and support: Equip employees with the necessary skills and knowledge to adapt to changes effectively, particularly in the context of new technologies.
- Evaluate outcomes: After implementation, assess the results to determine the effectiveness of the strategy and identify areas for improvement.
A well-structured implementation strategy not only facilitates smooth transitions but also enhances the likelihood of achieving desired outcomes, ultimately driving organizational success. Rapid Innovation is committed to guiding organizations through this process, ensuring they harness the full potential of AI and Blockchain technologies to achieve greater ROI.
6.1. Readiness Assessment
A readiness assessment is a critical step in preparing for any significant project, especially in the context of technology implementation or organizational change. This process evaluates the current state of an organization to determine its preparedness for new initiatives, including a readiness assessment for technology implementation.
- Identify existing capabilities: Assess the current skills, resources, and technologies available within the organization. Rapid Innovation can assist in identifying these capabilities, ensuring that your team is equipped to leverage AI and Blockchain technologies effectively. Â
- Evaluate organizational culture: Understand how the company culture may impact the adoption of new systems or processes. Our consultants can provide insights into fostering a culture that embraces innovation and technology. Â
- Analyze stakeholder engagement: Determine the level of support and involvement from key stakeholders, including leadership and team members. Engaging stakeholders early in the process can enhance buy-in and facilitate smoother implementation. Â
- Assess current processes: Review existing workflows and identify areas that may require adjustment or improvement. Rapid Innovation can help streamline these processes, integrating AI and Blockchain solutions to enhance efficiency. Â
- Identify potential risks: Recognize any barriers or challenges that could hinder the successful implementation of new initiatives. Our expertise allows us to foresee potential pitfalls and develop strategies to mitigate them. Â
Conducting a thorough readiness assessment helps organizations identify gaps and areas for improvement, ensuring a smoother transition to new systems or processes. For more insights, you can learn from real-world AI implementations.
6.2. Data Preparation
Data preparation is a vital step in any data-driven project, ensuring that the information used is accurate, relevant, and ready for analysis. This process involves several key activities that help organizations make informed decisions based on reliable data.
- Data collection: Gather data from various sources, including databases, spreadsheets, and external systems. Rapid Innovation employs advanced data collection techniques to ensure comprehensive data gathering. Â
- Data cleaning: Identify and rectify errors, inconsistencies, and duplicates in the data to enhance its quality. Our AI-driven tools can automate this process, significantly reducing the time and effort required. Â
- Data transformation: Convert data into a suitable format for analysis, which may involve normalization, aggregation, or encoding. We ensure that your data is primed for insightful analysis, particularly in AI applications. Â
- Data integration: Combine data from different sources to create a unified dataset that provides a comprehensive view of the information. Our expertise in Blockchain technology can facilitate secure and efficient data integration. Â
- Data validation: Ensure that the prepared data meets the required standards and is ready for analysis or reporting. Rapid Innovation emphasizes the importance of data integrity, which is crucial for accurate decision-making. Â
Effective data preparation is essential for achieving accurate insights and making data-driven decisions. Organizations that invest time in this process are more likely to succeed in their analytical endeavors.
6.3. System Architecture
System architecture refers to the conceptual model that defines the structure, behavior, and various views of a system. It serves as a blueprint for both the system and the project developing it. Understanding system architecture is crucial for ensuring that technology solutions align with business goals.
- Define system components: Identify the various elements of the system, including hardware, software, and network resources. Rapid Innovation can help design a robust architecture that incorporates the latest AI and Blockchain technologies. Â
- Establish relationships: Determine how different components interact with each other and the data flow between them. Our expertise ensures that these interactions are optimized for performance and security. Â
- Consider scalability: Design the architecture to accommodate future growth and changes in user demand. We focus on creating scalable solutions that can evolve with your business needs. Â
- Ensure security: Implement security measures to protect sensitive data and maintain system integrity. Rapid Innovation prioritizes security, especially in Blockchain implementations, to safeguard your assets. Â
- Optimize performance: Design the system for efficiency, ensuring that it can handle the required workload without compromising speed or reliability. Our solutions are tailored to maximize performance while minimizing costs. Â
A well-defined system architecture is essential for successful technology implementation, as it provides a clear framework for development and helps mitigate potential issues during the project lifecycle.
6.4. Integration Framework
An integration framework is essential for ensuring that various systems and applications work together seamlessly. It provides a structured approach to connect disparate systems, enabling data exchange and process automation.
- Key components of an integration framework include: Â
- Middleware: Acts as a bridge between different applications, facilitating communication and data transfer.
- APIs (Application Programming Interfaces): Allow different software systems to interact with each other, enabling functionalities like data retrieval and updates.
- Data Transformation Tools: Convert data formats to ensure compatibility between systems.
- Benefits of a robust integration framework: Â
- Improved Efficiency: Automates repetitive tasks, reducing manual intervention and errors, which can lead to significant cost savings and increased productivity.
- Enhanced Data Accuracy: Ensures that data is consistent across systems, minimizing discrepancies that can affect decision-making and operational efficiency.
- Scalability: Supports the addition of new applications and services without significant rework, allowing businesses to adapt quickly to changing market demands.
- Common integration patterns include: Â
- Point-to-Point Integration: Direct connections between systems, suitable for simple environments, often used in smaller organizations or specific use cases.
- Hub-and-Spoke Model: Centralized integration point that connects multiple systems, ideal for complex architectures, enabling better management and monitoring of data flows.
- Service-Oriented Architecture (SOA): Promotes the use of services to facilitate integration, enhancing flexibility and reusability, which is particularly beneficial for enterprises looking to innovate rapidly.
- Examples of integration frameworks include: Â
- SAP Application Integration Framework: A solution designed to integrate SAP applications with other systems.
- Maximo Integration Framework: A framework that facilitates integration with IBM Maximo.
- D365 Integration Framework: A framework for integrating Microsoft Dynamics 365 applications.
- API Integration Framework: A structured approach to integrating various APIs for seamless data exchange.
- Enterprise Integration Framework: A comprehensive framework for integrating enterprise-level applications.
At Rapid Innovation, we offer specialized services in Stable Diffusion Development to help businesses implement effective integration frameworks tailored to their unique needs. Additionally, we explore the role of AI agents in software testing to enhance the integration process.
6.5. Testing and Validation
Testing and validation are critical steps in the software development lifecycle, ensuring that applications function as intended and meet user requirements.
- Types of testing include: Â
- Unit Testing: Focuses on individual components to verify their correctness, ensuring that each part of the application performs as expected.
- Integration Testing: Assesses the interaction between integrated components to identify issues, crucial for maintaining system integrity.
- System Testing: Evaluates the complete system's compliance with specified requirements, ensuring that the application meets business objectives.
- User Acceptance Testing (UAT): Conducted by end-users to validate the system against their needs, ensuring that the final product aligns with user expectations.
- Key aspects of validation: Â
- Requirements Verification: Ensures that the software meets all specified requirements, reducing the risk of project failure.
- Performance Testing: Assesses the system's responsiveness and stability under various conditions, critical for maintaining user satisfaction.
- Security Testing: Identifies vulnerabilities and ensures data protection, particularly important in today's data-driven landscape.
- Best practices for effective testing and validation: Â
- Automate Testing: Use automated testing tools to increase efficiency and coverage, allowing teams to focus on more complex testing scenarios.
- Continuous Testing: Integrate testing into the development process to catch issues early, facilitating a more agile development environment.
- Document Results: Maintain thorough documentation of test cases, results, and any identified defects, which is essential for compliance and future reference.
6.6. Deployment Models
Deployment models define how applications are delivered and managed in a computing environment. Choosing the right deployment model is crucial for meeting business needs and ensuring optimal performance.
- Common deployment models include: Â
- On-Premises: Software is installed and runs on local servers. This model offers greater control but requires significant infrastructure investment, making it suitable for organizations with strict regulatory requirements.
- Cloud-Based: Applications are hosted on cloud servers, providing flexibility and scalability. This model reduces the need for physical hardware and allows for easy access from anywhere, making it ideal for businesses looking to innovate rapidly.
- Hybrid: Combines on-premises and cloud solutions, allowing organizations to leverage the benefits of both environments. This model is ideal for businesses with varying workloads and security requirements, enabling a tailored approach to deployment.
- Factors to consider when selecting a deployment model: Â
- Cost: Evaluate the total cost of ownership, including hardware, software, and maintenance, to ensure alignment with budget constraints.
- Scalability: Determine how easily the model can accommodate growth and changing demands, which is essential for long-term planning.
- Security: Assess the security measures in place for each model, especially for sensitive data, to mitigate risks associated with data breaches.
- Trends in deployment models: Â
- Containerization: Using containers to package applications for consistent deployment across environments, enhancing portability and efficiency.
- Microservices Architecture: Breaking applications into smaller, independent services that can be deployed and scaled individually, promoting agility and faster time-to-market.
- Serverless Computing: Running applications without managing servers, allowing developers to focus on code rather than infrastructure, which can lead to reduced operational costs and increased innovation.
At Rapid Innovation, we leverage these frameworks, including the well architected framework for Salesforce, testing methodologies, and deployment models to help our clients achieve their business goals efficiently and effectively, ultimately driving greater ROI through tailored solutions in AI and Blockchain development.
6.7. Monitoring and Maintenance
Monitoring and maintenance are critical components in ensuring the longevity and efficiency of systems, particularly in technology-driven environments. Regular monitoring helps identify potential issues before they escalate, while maintenance ensures that systems operate at optimal performance levels.
- Continuous Monitoring: Â
- Implement real-time monitoring tools to track system performance.
- Use analytics to gather data on system usage and identify trends.
- Set up alerts for unusual activity or performance dips.
- Scheduled Maintenance: Â
- Establish a routine maintenance schedule to perform updates and patches.
- Conduct regular system audits to ensure compliance with industry standards.
- Review and optimize system configurations periodically.
- Performance Metrics: Â
- Define key performance indicators (KPIs) to measure system effectiveness.
- Analyze metrics such as uptime, response time, and error rates.
- Use this data to inform future upgrades and maintenance strategies.
- User Feedback: Â
- Encourage users to report issues or suggest improvements.
- Implement a feedback loop to address concerns promptly.
- Use surveys to gauge user satisfaction and system usability.
- Documentation: Â
- Maintain detailed records of all monitoring and maintenance activities.
- Document changes made to the system for future reference.
- Ensure that all team members have access to up-to-date documentation.
- Risk Management: Â
- Identify potential risks associated with system failures.
- Develop contingency plans to mitigate these risks.
- Regularly review and update risk management strategies.
7. Industry-Specific Applications
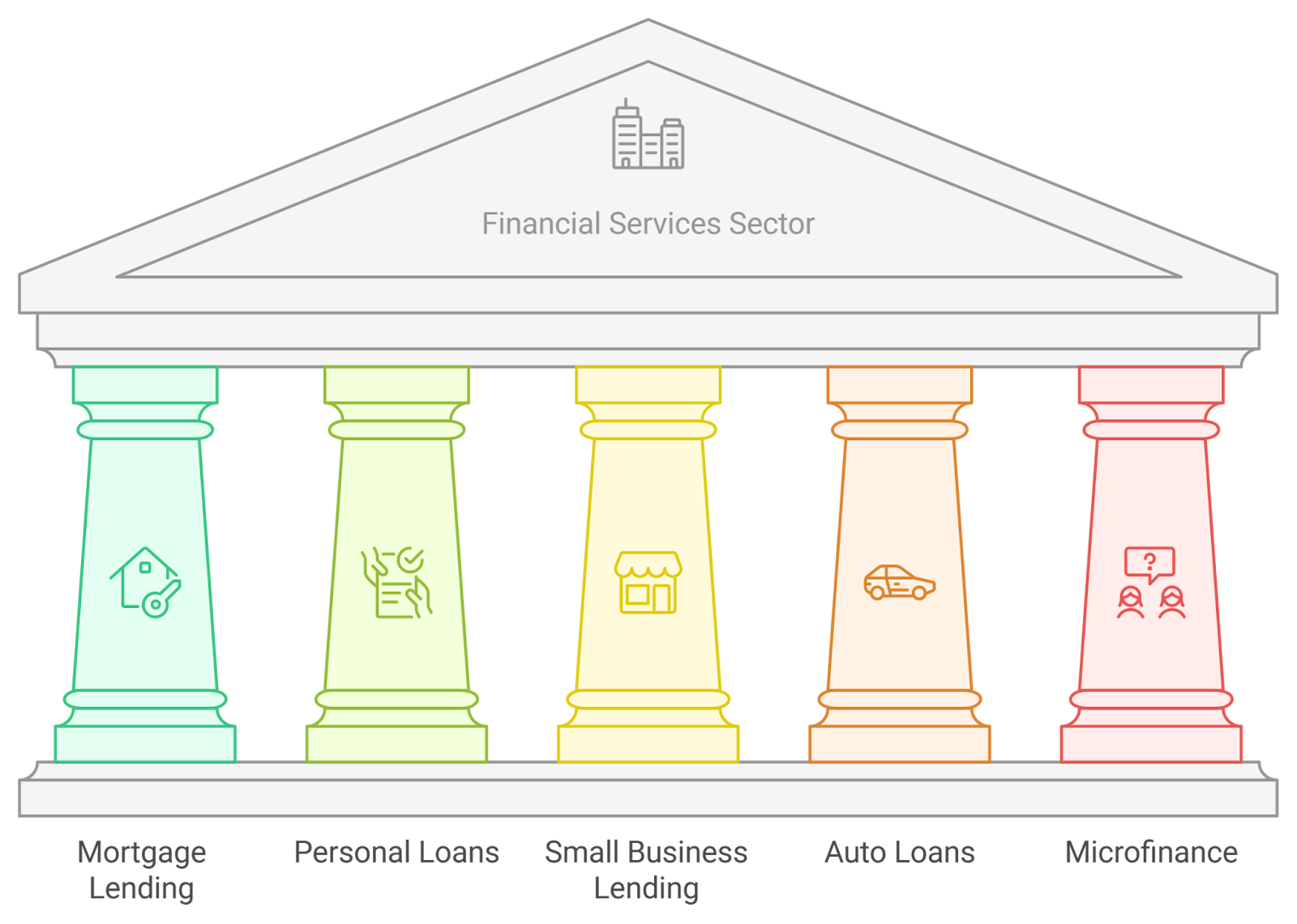
‍
Industry-specific applications refer to software solutions tailored to meet the unique needs of particular sectors. These applications enhance efficiency, streamline processes, and improve overall productivity. Understanding the specific requirements of an industry is crucial for developing effective solutions.
- Customization: Â
- Tailor applications to address the specific challenges of the industry.
- Incorporate industry regulations and compliance requirements into the software.
- Ensure that the user interface is intuitive for industry professionals.
- Integration: Â
- Facilitate seamless integration with existing systems and tools.
- Use APIs to connect with third-party applications commonly used in the industry.
- Ensure data consistency across platforms to enhance decision-making.
- Scalability: Â
- Design applications that can grow with the business.
- Allow for easy upgrades and additional features as needed.
- Ensure that the application can handle increased user loads without performance issues.
- Training and Support: Â
- Provide comprehensive training for users to maximize application effectiveness.
- Offer ongoing support to address any technical issues that arise.
- Create a knowledge base with resources and FAQs for users.
7.1. Mortgage Lending
Mortgage lending is a specialized area within the financial services industry that involves providing loans to individuals or businesses for purchasing real estate. The process is complex and requires a deep understanding of both the financial and regulatory landscapes.
- Application Processing: Â
- Streamline the application process with user-friendly online forms.
- Implement automated systems to verify applicant information quickly.
- Use algorithms to assess creditworthiness and risk levels.
- Compliance and Regulation: Â
- Stay updated on federal and state regulations affecting mortgage lending.
- Ensure that all lending practices comply with the Truth in Lending Act (TILA) and the Real Estate Settlement Procedures Act (RESPA).
- Regularly review compliance protocols to avoid legal issues.
- Risk Assessment: Â
- Utilize data analytics to evaluate borrower risk profiles.
- Implement predictive modeling to forecast potential defaults.
- Adjust lending criteria based on market trends and economic indicators.
- Customer Relationship Management (CRM): Â
- Use CRM systems to manage client interactions and track leads.
- Automate follow-ups and reminders for important milestones in the lending process.
- Personalize communication to enhance customer experience.
- Technology Integration: Â
- Leverage technology such as blockchain for secure transactions.
- Use artificial intelligence (AI) to improve decision-making processes.
- Implement mobile applications to provide clients with easy access to their loan information.
- Market Trends: Â
- Monitor housing market trends to adjust lending strategies accordingly.
- Analyze interest rate fluctuations and their impact on mortgage demand.
- Stay informed about emerging technologies that could disrupt the mortgage industry.
- Education and Resources: Â
- Provide educational resources for borrowers to understand the mortgage process.
- Offer tools such as mortgage calculators to help clients make informed decisions.
- Host webinars or workshops to discuss market trends and lending options.
At Rapid Innovation, we leverage our expertise in AI and Blockchain to enhance monitoring and maintenance processes, including the use of remote maintenance software, ensuring that our clients achieve greater ROI through efficient system management and tailored industry-specific applications. By integrating advanced technologies, we help businesses streamline operations, reduce risks, and improve overall productivity, while also considering factors such as prtg maintenance cost in our strategies.
7.2. Personal Loans
Personal loans are unsecured loans that individuals can use for various purposes, such as consolidating debt, financing a large purchase, or covering unexpected expenses. They typically have fixed interest rates and repayment terms, making them a popular choice for borrowers seeking predictable monthly payments.
- Types of Personal Loans: Â
- Unsecured personal loans: No collateral is required, but they may have higher interest rates.
- Secured personal loans: Require collateral, which can lower the interest rate.
- Personalized loans: Tailored to meet specific borrower needs.
- Application Process: Â
- Check your credit score: A higher score can lead to better loan terms.
- Compare lenders: Look for competitive interest rates and fees, including options for personal loans and p loan.
- Gather documentation: Common requirements include proof of income, employment verification, and identification.
- Benefits: Â
- Flexibility: Funds can be used for various purposes, including bills consolidation loans.
- Quick access to cash: Many lenders offer fast approval and funding, including loans near me.
- Fixed payments: Easier budgeting with predictable monthly payments.
- Considerations: Â
- Interest rates: Can vary significantly based on creditworthiness.
- Fees: Be aware of origination fees or prepayment penalties.
- Impact on credit score: Applying for multiple loans can temporarily lower your score, especially with no credit check loans.
7.3. Small Business Lending
Small business lending refers to the various financing options available to entrepreneurs and small business owners. These loans can help businesses cover startup costs, expand operations, or manage cash flow.
- Types of Small Business Loans: Â
- Traditional bank loans: Often have lower interest rates but stricter qualification criteria.
- SBA loans: Backed by the Small Business Administration, these loans offer favorable terms.
- Alternative lenders: Online lenders may provide faster access to funds but at higher interest rates.
- Application Process: Â
- Prepare a business plan: A solid plan can help demonstrate your business's potential.
- Gather financial documents: Lenders typically require tax returns, profit and loss statements, and bank statements.
- Understand your credit score: A good credit score can improve your chances of approval.
- Benefits: Â
- Access to capital: Helps businesses invest in growth opportunities.
- Builds credit history: Timely repayments can improve your business credit score.
- Diverse options: Various loan types cater to different business needs.
- Considerations: Â
- Interest rates: Can vary widely based on the lender and your creditworthiness.
- Repayment terms: Understand the loan's duration and monthly payment obligations.
- Personal guarantees: Some lenders may require personal guarantees, putting personal assets at risk.
7.4. Auto Loans
Auto loans are specifically designed to help individuals purchase vehicles. These loans can be secured or unsecured, with the vehicle often serving as collateral for secured loans.
- Types of Auto Loans: Â
- New car loans: Typically offered for purchasing new vehicles, often with lower interest rates.
- Used car loans: Financing options for pre-owned vehicles, which may have slightly higher rates.
- Lease buyout loans: For those looking to purchase a leased vehicle at the end of the lease term.
- Application Process: Â
- Check your credit score: A higher score can lead to better loan terms.
- Research lenders: Compare rates from banks, credit unions, and dealerships.
- Get pre-approved: Pre-approval can give you a better idea of your budget.
- Benefits: Â
- Fixed interest rates: Many auto loans come with fixed rates, making budgeting easier.
- Flexible terms: Loan terms can range from 36 to 72 months or more.
- Potential tax benefits: In some cases, interest on auto loans may be tax-deductible.
- Considerations: Â
- Total cost of ownership: Factor in insurance, maintenance, and fuel costs.
- Loan-to-value ratio: Lenders may consider the vehicle's value compared to the loan amount.
- Early repayment penalties: Some loans may charge fees for paying off the loan early.
At Rapid Innovation, we leverage AI and Blockchain technologies to enhance the lending process for personal loans, small business lending, and auto loans. By utilizing AI algorithms, we can analyze creditworthiness more accurately, leading to better loan terms for borrowers. Our Blockchain solutions ensure secure and transparent transactions, reducing the risk of fraud and enhancing trust between lenders and borrowers. This innovative approach not only streamlines the application process but also helps clients achieve greater ROI by minimizing operational costs and improving customer satisfaction, including options like payday loans near me and private student loan solutions.
7.5. Microfinance
Microfinance is a financial service that provides small loans, savings, and other financial products to individuals or small businesses that lack access to traditional banking services. It plays a crucial role in promoting financial inclusion, particularly in developing countries.
- Micro lending institutions offer services tailored to low-income clients, enabling them to start or expand small businesses.
- The loans are typically small, ranging from $100 to $5,000, and are often provided without the need for collateral.
- Microfinance not only helps individuals improve their economic situation but also empowers women, as a significant portion of microloans are given to female entrepreneurs.
- The repayment rates for microloans are generally high, often exceeding 90%, demonstrating the effectiveness of this model.
- In addition to loans, many microfinancing institutions provide financial education and training, helping clients manage their finances better.
- The microfinance sector has evolved with technology, leading to the rise of digital microfinance platforms that streamline the lending process and reduce costs.
At Rapid Innovation, we leverage AI and blockchain technology to enhance the microfinance sector. By implementing AI-driven analytics, we help microloan companies better assess creditworthiness and tailor financial products to meet the specific needs of their clients. Our blockchain solutions ensure secure and transparent transactions, reducing the risk of fraud and enhancing trust among borrowers and lenders.
Microfinance has shown promising results in poverty alleviation and economic development, but it also faces challenges such as high-interest rates and over-indebtedness among borrowers. As the sector continues to grow, it is essential to balance profitability with social impact.
8. Future Trends and Innovations
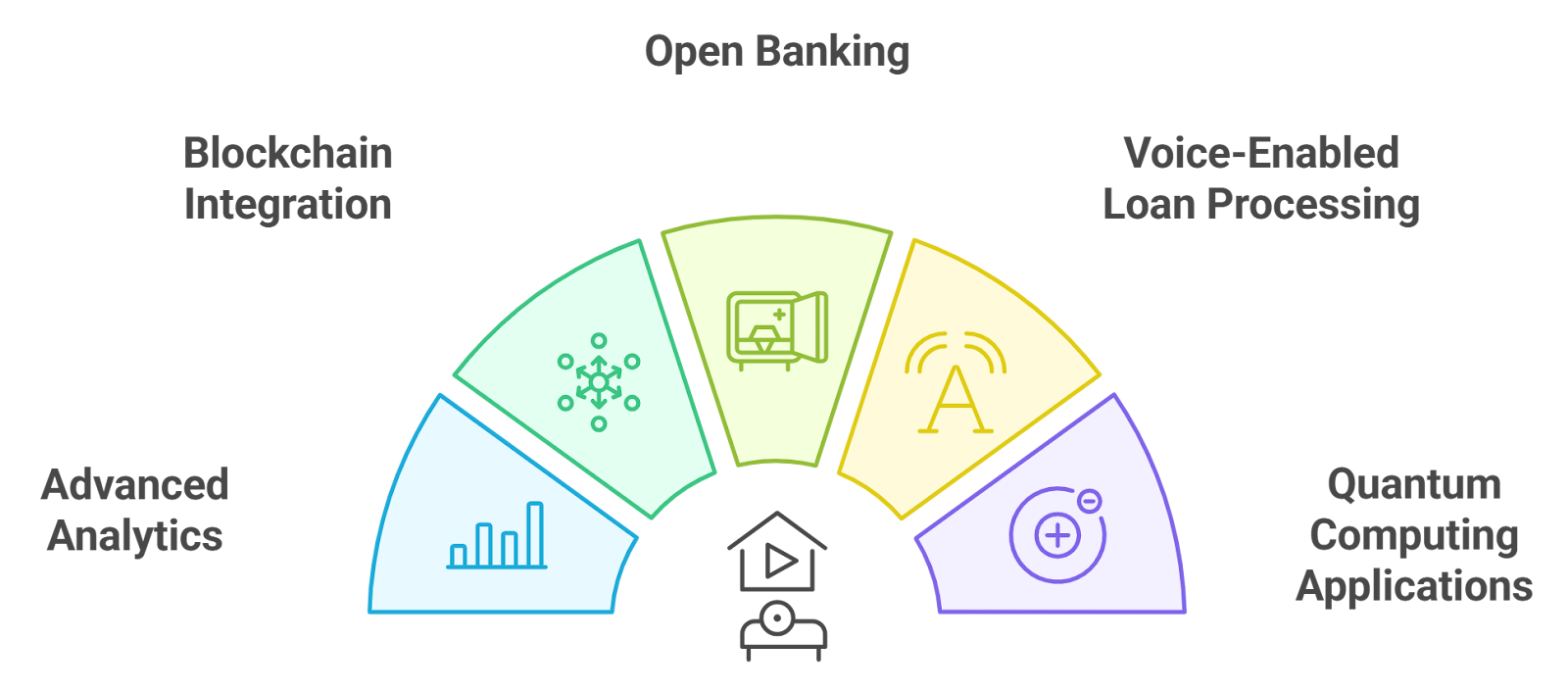
‍
The future of finance is rapidly evolving, driven by technological advancements and changing consumer behaviors. Several trends and innovations are shaping the financial landscape, making it more accessible and efficient.
- The rise of fintech companies is disrupting traditional banking models, offering innovative solutions that cater to the needs of consumers and businesses.
- Digital currencies and blockchain technology are gaining traction, providing secure and transparent transaction methods.
- Artificial intelligence (AI) and machine learning are being integrated into financial services, enhancing risk assessment and customer service.
- Sustainability and ethical investing are becoming increasingly important, with more investors seeking socially responsible options.
- Regulatory changes are also influencing the financial sector, as governments adapt to new technologies and consumer demands.
These trends indicate a shift towards a more inclusive and technology-driven financial ecosystem, where consumers have greater control over their financial decisions.
8.1. Advanced Analytics
Advanced analytics refers to the use of sophisticated data analysis techniques to gain insights and make informed decisions. In the financial sector, advanced analytics is transforming how institutions operate and interact with customers.
- Predictive analytics helps financial institutions anticipate customer needs and behaviors, allowing for personalized services and targeted marketing.
- Risk management is enhanced through advanced analytics, enabling organizations to identify potential risks and mitigate them effectively.
- Customer segmentation becomes more precise, allowing for tailored products and services that meet specific demographic needs.
- Real-time data analysis supports quicker decision-making, improving operational efficiency and responsiveness to market changes.
- Fraud detection systems leverage advanced analytics to identify unusual patterns and prevent financial crimes.
The integration of advanced analytics in finance not only improves operational efficiency but also enhances customer experience, making it a vital component of future financial innovations. As organizations continue to harness the power of data, the financial landscape will become increasingly data-driven and customer-centric. At Rapid Innovation, we are committed to helping our clients navigate these changes and achieve greater ROI through our expertise in AI and blockchain technologies.
Additionally, microlending organizations and microfinance banks play a significant role in this evolving landscape, providing essential services to underserved populations. The collaboration between banking and microfinance is crucial for fostering economic growth and development in emerging markets.
8.2. Blockchain Integration
Blockchain technology is revolutionizing various sectors, including finance, by providing a decentralized and secure method for transactions. Its integration into financial services offers numerous benefits:
- Enhanced Security: Blockchain's cryptographic nature ensures that data is secure and tamper-proof, reducing the risk of fraud.
- Transparency: All transactions are recorded on a public ledger, allowing for greater transparency and traceability.
- Reduced Costs: By eliminating intermediaries, blockchain can lower transaction fees and operational costs.
- Faster Transactions: Blockchain enables real-time processing of transactions, significantly speeding up the settlement process.
- Smart Contracts: These self-executing contracts, with the terms directly written into code, can automate and streamline various processes.
At Rapid Innovation, we leverage blockchain technology to help financial institutions enhance their operational efficiency and security. For instance, our clients have successfully implemented blockchain solutions for cross-border payments, resulting in reduced transaction times and costs. By integrating blockchain, we empower organizations to transform traditional banking practices, ultimately leading to greater ROI. We also explore applications of blockchain in finance, such as blockchain financial services and blockchain applications in financial services, to provide tailored solutions. Our expertise includes blockchain development for finance projects and understanding the benefits of blockchain in financial services, ensuring our clients stay ahead in the competitive landscape. You can read more about this in our article on Aptos: The Crypto Frontier.
8.3. Open Banking
Open banking is a financial services model that allows third-party developers to build applications and services around financial institutions. This approach fosters innovation and enhances customer experience through several key features:
- Customer Control: Open banking gives customers control over their financial data, allowing them to share it with authorized third parties.
- Improved Services: By leveraging customer data, banks and fintech companies can offer personalized financial products and services.
- Increased Competition: Open banking encourages competition among financial institutions, leading to better rates and services for consumers.
- API Utilization: Application Programming Interfaces (APIs) enable seamless data sharing between banks and third-party providers, facilitating new service offerings.
- Enhanced Financial Management: Customers can access a consolidated view of their finances, making it easier to manage budgets and track spending.
The implementation of open banking is gaining momentum globally, driven by regulatory changes and consumer demand for more innovative financial solutions. As a result, it is reshaping the landscape of banking and finance. Rapid Innovation assists clients in navigating this transformation by developing tailored open banking solutions that enhance customer engagement and drive business growth.
8.4. Voice-Enabled Loan Processing
Voice-enabled technology is transforming the way consumers interact with financial institutions, particularly in the loan processing sector. This innovation offers several advantages:
- Convenience: Customers can initiate loan applications and inquiries using voice commands, making the process more user-friendly.
- Speed: Voice recognition technology can expedite the loan application process, reducing the time it takes to complete forms and gather information.
- Accessibility: Voice-enabled systems can assist individuals with disabilities or those who prefer verbal communication, broadening access to financial services.
- Enhanced Customer Experience: By providing a more interactive and engaging experience, voice technology can improve customer satisfaction and loyalty.
- Data Collection: Voice interactions can be analyzed to gather insights into customer preferences and behaviors, helping institutions tailor their offerings.
As voice technology continues to evolve, its integration into loan processing is expected to grow, making financial services more accessible and efficient for consumers. Rapid Innovation is at the forefront of this trend, helping financial institutions implement voice-enabled solutions that enhance operational efficiency and customer satisfaction, ultimately leading to improved ROI. We also recognize the role of blockchain fintech examples in enhancing these processes, ensuring that our clients benefit from the latest technological advancements in the financial sector.
8.5. Quantum Computing Applications
Quantum computing is a revolutionary technology that leverages the principles of quantum mechanics to process information in ways that classical computers cannot. Its applications span various fields, offering solutions to complex problems that were previously unsolvable.
- Cryptography: Quantum computers can break traditional encryption methods, leading to the development of quantum-resistant algorithms. This is crucial for securing sensitive data against future quantum attacks, ensuring that businesses can protect their intellectual property and customer information.
- Drug Discovery: Quantum computing can simulate molecular interactions at an unprecedented scale, significantly speeding up the drug discovery process. This can lead to the development of new medications and therapies, allowing pharmaceutical companies to bring products to market faster and more cost-effectively.
- Optimization Problems: Industries such as logistics, finance, and manufacturing can benefit from quantum algorithms that solve optimization problems more efficiently than classical methods. This can lead to cost savings and improved operational efficiency, enabling businesses to allocate resources more effectively.
- Artificial Intelligence: Quantum computing can enhance machine learning algorithms, allowing for faster data processing and improved pattern recognition. This can lead to advancements in AI applications across various sectors, driving innovation and competitive advantage. The intersection of quantum computing and AI is particularly promising, as quantum computing for AI can unlock new capabilities in data analysis and decision-making.
- Financial Modeling: Quantum computers can analyze complex financial models and risk assessments more effectively, providing better insights for investment strategies and market predictions. This can help financial institutions make more informed decisions, ultimately increasing their ROI. Quantum computing finance applications are expected to revolutionize how financial data is processed and analyzed.
- Climate Modeling: Quantum computing can improve simulations of climate systems, helping researchers understand climate change and develop strategies for mitigation. This can support organizations in their sustainability efforts and compliance with environmental regulations.
- Material Science: The ability to model and simulate materials at the quantum level can lead to the discovery of new materials with unique properties, impacting industries like electronics and energy. This can foster innovation and lead to the development of next-generation products.
- Telecommunications: Quantum key distribution (QKD) offers a method for secure communication, ensuring that data transmitted over networks remains confidential. This is essential for businesses that rely on secure data transmission to maintain customer trust and regulatory compliance.
As quantum technology continues to evolve, its applications are expected to expand, potentially transforming industries and creating new opportunities. The exploration of quantum computing applications, such as quantum computing transforming blockchain security and scalability in 2024, will further enhance our understanding and capabilities in this field.
9. Best Practices and Guidelines

‍
Implementing best practices and guidelines is essential for organizations to maximize the benefits of technology while minimizing risks. These practices ensure that systems are efficient, secure, and compliant with regulations.
- Establish Clear Objectives: Define the goals and objectives of technology implementation to align with business strategies.
- Regular Training: Provide ongoing training for employees to keep them updated on the latest technologies and best practices.
- Risk Assessment: Conduct regular risk assessments to identify vulnerabilities and implement appropriate mitigation strategies.
- Data Privacy Compliance: Ensure compliance with data protection regulations such as GDPR and CCPA to protect user data and maintain trust.
- Documentation: Maintain thorough documentation of processes, policies, and procedures to facilitate transparency and accountability.
- Continuous Improvement: Regularly review and update practices to adapt to changing technologies and business environments.
- Stakeholder Engagement: Involve stakeholders in the decision-making process to ensure that their needs and concerns are addressed.
9.1. Data Management
Effective data management is crucial for organizations to harness the power of data while ensuring its security and integrity. Proper data management practices can lead to better decision-making and improved operational efficiency.
- Data Governance: Establish a data governance framework that defines roles, responsibilities, and processes for managing data assets.
- Data Quality: Implement measures to ensure data accuracy, consistency, and completeness. Regular data cleansing and validation processes are essential.
- Data Security: Protect sensitive data through encryption, access controls, and regular security audits to prevent unauthorized access and data breaches.
- Data Lifecycle Management: Manage data throughout its lifecycle, from creation and storage to archiving and deletion, ensuring compliance with regulations.
- Backup and Recovery: Implement robust backup and recovery solutions to safeguard data against loss due to hardware failures, cyberattacks, or natural disasters.
- Data Integration: Utilize data integration tools to consolidate data from various sources, providing a unified view for analysis and reporting.
- Analytics and Reporting: Leverage data analytics tools to extract insights from data, enabling informed decision-making and strategic planning.
- User Training: Educate employees on data management best practices to foster a culture of data responsibility and awareness.
By adhering to these data management practices, organizations can optimize their data usage, enhance security, and drive better business outcomes. At Rapid Innovation, we are committed to helping our clients implement these best practices, leveraging our expertise in AI and Blockchain to ensure that they achieve their business goals efficiently and effectively.
9.2. Model Development
Model development is a critical phase in various industries, particularly in finance, healthcare, and technology. It involves creating predictive models that can analyze data and provide insights for decision-making. The process typically includes several key steps:
- Data Collection: Gathering relevant data from various sources is essential. This data can include historical records, market trends, and user behavior. At Rapid Innovation, we leverage advanced AI tools to automate data collection, ensuring that our clients have access to the most comprehensive datasets for their model development, including insights from software development life cycle models.
- Data Preprocessing: Cleaning and transforming the data to ensure accuracy and consistency. This step may involve handling missing values, normalizing data, and removing outliers. Our expertise in AI allows us to implement sophisticated preprocessing techniques that enhance data quality, leading to more reliable model outcomes, similar to the iterative development model used in agile methodology.
- Feature Selection: Identifying the most relevant variables that will contribute to the model's predictive power. This can significantly enhance the model's performance. Rapid Innovation employs machine learning algorithms to optimize feature selection, ensuring that our clients' models are both efficient and effective, akin to the feature selection process in the agile software development model.
- Model Selection: Choosing the appropriate algorithm or technique for the model. Common methods include regression analysis, decision trees, and machine learning algorithms. Our team of experts at Rapid Innovation assists clients in selecting the best-suited algorithms based on their specific business needs and objectives, often drawing from various sdlc models and methodologies.
- Training the Model: Using a portion of the data to train the model, allowing it to learn patterns and relationships within the data. We utilize cutting-edge AI techniques to enhance the training process, ensuring that models are robust and capable of delivering high accuracy, similar to the training processes in the waterfall development model.
- Validation and Testing: Evaluating the model's performance using a separate dataset to ensure it generalizes well to new data. Metrics such as accuracy, precision, and recall are often used. Rapid Innovation emphasizes rigorous validation processes to guarantee that our clients' models perform optimally in real-world scenarios, akin to the validation steps in the spiral development model.
- Deployment: Implementing the model in a real-world environment where it can be used for decision-making. Our team ensures a seamless deployment process, integrating models into existing systems to maximize their utility, similar to the deployment strategies in rapid application development.
- Monitoring and Maintenance: Continuously tracking the model's performance and making necessary adjustments to improve accuracy over time. Rapid Innovation provides ongoing support and maintenance, allowing clients to adapt their models to changing conditions and maintain high performance, reflecting the iterative development practices found in agile methodology.
Effective model development can lead to better predictions, improved efficiency, and enhanced decision-making capabilities, ultimately driving greater ROI for our clients, much like the benefits realized through effective sdlc and agile methodology integration.
9.3. Risk Mitigation
Risk mitigation is a proactive approach to identifying, assessing, and minimizing potential risks that could negatively impact an organization. It is essential for maintaining operational stability and achieving strategic objectives. Key components of risk mitigation include:
- Risk Identification: Recognizing potential risks that could affect the organization. This can involve brainstorming sessions, expert consultations, and reviewing historical data.
- Risk Assessment: Evaluating the likelihood and impact of identified risks. This helps prioritize which risks need immediate attention.
- Developing Mitigation Strategies: Creating plans to reduce or eliminate risks. Strategies may include implementing safety protocols, diversifying investments, purchasing insurance, and establishing contingency plans.
- Monitoring Risks: Continuously tracking identified risks and emerging threats. This ensures that the organization can respond quickly to changes in the risk landscape.
- Training and Awareness: Educating employees about potential risks and the importance of following mitigation strategies. This fosters a culture of risk awareness within the organization.
By effectively managing risks, organizations can protect their assets, enhance their reputation, and ensure long-term sustainability.
9.4. Compliance Management
Compliance management involves ensuring that an organization adheres to laws, regulations, and internal policies. It is crucial for maintaining legal and ethical standards while minimizing the risk of penalties and reputational damage. Key aspects of compliance management include:
- Understanding Regulations: Staying informed about relevant laws and regulations that apply to the organization. This can include industry-specific regulations, data protection laws, and labor laws.
- Policy Development: Creating clear policies and procedures that align with regulatory requirements. These policies should be communicated effectively to all employees.
- Training and Education: Providing regular training sessions to employees on compliance matters. This helps ensure that everyone understands their responsibilities and the importance of compliance.
- Monitoring and Auditing: Regularly reviewing processes and practices to ensure compliance. This can involve internal audits, risk assessments, and compliance checks.
- Reporting and Documentation: Maintaining accurate records of compliance efforts and any incidents that occur. This documentation is essential for demonstrating compliance to regulators and stakeholders.
- Continuous Improvement: Regularly updating compliance programs to reflect changes in regulations and organizational practices. This ensures that the organization remains compliant in a dynamic environment.
Effective compliance management not only helps avoid legal issues but also builds trust with customers and stakeholders, enhancing the organization's overall reputation.
9.5. Performance Monitoring
Performance monitoring is a critical aspect of any business strategy, particularly in the financial sector. It involves the continuous assessment of various metrics to ensure that organizational goals are being met effectively and efficiently.
- Key Performance Indicators (KPIs) are essential for tracking progress. Common KPIs in banking include: Â
- Return on Assets (ROA)
- Return on Equity (ROE)
- Net Interest Margin (NIM)
- Customer Satisfaction Scores
- Regularly reviewing these KPIs helps identify trends and areas for improvement. This can lead to: Â
- Enhanced decision-making
- Increased operational efficiency
- Better customer service
- Performance monitoring tools can include: Â
- Dashboards that provide real-time data visualization
- Automated reporting systems that streamline data collection
- Benchmarking against industry standards to gauge performance
- The importance of data analytics cannot be overstated. By leveraging big data, banks can: Â
- Predict customer behavior
- Identify potential risks
- Optimize product offerings
- Compliance with regulatory requirements is another critical aspect of performance monitoring. Banks must ensure they are: Â
- Adhering to financial regulations
- Conducting regular audits
- Reporting accurately to regulatory bodies
- Continuous improvement is a goal of performance monitoring. By analyzing performance data, banks can: Â
- Implement corrective actions
- Innovate new services
- Enhance customer engagement strategies
10. Case Studies
Case studies provide valuable insights into how different organizations implement strategies and overcome challenges. They serve as real-world examples that can guide other businesses in their decision-making processes.
- Case studies can highlight: Â
- Successful implementations of new technologies
- Effective customer engagement strategies
- Lessons learned from failures
- They often include: Â
- Background information on the organization
- The challenges faced
- The solutions implemented
- The outcomes achieved
- Analyzing case studies can help organizations: Â
- Understand industry trends
- Identify best practices
- Avoid common pitfalls
10.1. Traditional Bank Implementation
Traditional banks have been adapting to the rapidly changing financial landscape by implementing various strategies to enhance their operations and customer service.
- The shift towards digital banking has prompted traditional banks to: Â
- Invest in technology to improve online services
- Develop mobile banking applications
- Enhance cybersecurity measures
- A notable example of traditional bank implementation is the integration of Artificial Intelligence (AI) and machine learning. This has led to: Â
- Improved fraud detection systems
- Personalized customer experiences through tailored product recommendations
- Streamlined operations via automated processes
- Traditional banks are also focusing on customer relationship management (CRM) systems to: Â
- Better understand customer needs
- Enhance communication channels
- Foster long-term relationships with clients
- The implementation of omnichannel banking strategies allows banks to: Â
- Provide a seamless customer experience across various platforms
- Increase customer engagement through multiple touchpoints
- Gather valuable data on customer preferences
- Regulatory compliance remains a significant focus for traditional banks. They must: Â
- Ensure adherence to laws and regulations
- Conduct regular risk assessments
- Implement robust reporting systems
- Case studies of traditional banks that have successfully navigated these changes can provide insights into: Â
- Effective change management strategies
- The importance of employee training and development
- The role of customer feedback in shaping services
By examining these implementations, other financial institutions can learn valuable lessons and apply them to their own operations, ensuring they remain competitive in an evolving market.
At Rapid Innovation, we leverage our expertise in AI and Blockchain to enhance performance monitoring in banking systems for our clients. By integrating advanced analytics and real-time data visualization tools, we empower banks to make informed decisions that drive greater ROI and operational efficiency. Our tailored solutions ensure compliance with regulatory standards while fostering continuous improvement, ultimately leading to enhanced customer satisfaction and loyalty.
10.2. FinTech Startup Approach
The FinTech startup approach is characterized by innovation, agility, and a customer-centric focus. These startups leverage technology to disrupt traditional financial services, offering solutions that are often faster, cheaper, and more accessible.
- Emphasis on Technology: FinTech startups utilize advanced technologies such as artificial intelligence, blockchain, and big data analytics to enhance their services. This tech-driven approach allows for real-time processing and improved customer experiences. At Rapid Innovation, we specialize in integrating AI and blockchain solutions that optimize operational efficiency and drive significant ROI for our clients. For instance, companies like niyo solutions funding are at the forefront of utilizing technology to provide innovative financial services.
- Customer-Centric Solutions: Understanding customer needs is paramount. FinTech startups often conduct extensive market research to identify pain points in existing financial services and develop tailored solutions, which could include mobile banking apps, robo-advisors, or peer-to-peer lending platforms. Our consulting services help clients design and implement these customer-centric solutions effectively. The success of platforms like signzy digital kyc illustrates the importance of addressing customer needs in the digital identity verification space.
- Regulatory Navigation: FinTech startups must navigate complex regulatory environments. Many employ compliance experts to ensure they meet legal requirements while still innovating. This balance is crucial for building trust with customers and investors. Rapid Innovation offers guidance on regulatory compliance, ensuring that our clients can innovate without compromising on legal standards.
- Agile Development: The ability to pivot quickly in response to market changes is a hallmark of FinTech startups. They often use agile methodologies to develop and iterate on their products, allowing for rapid deployment and feedback incorporation. Our development teams at Rapid Innovation are adept at agile practices, enabling clients to bring their products to market swiftly and efficiently.
- Collaboration with Traditional Institutions: Many FinTech startups partner with established financial institutions to leverage their resources and customer base. This collaboration can lead to innovative products that benefit both parties. For example, razorpay buys payments tech startup izealiant technologies for undisclosed sum highlights how collaboration can enhance service offerings. Rapid Innovation facilitates these partnerships, helping startups connect with traditional institutions to enhance their service offerings.
10.3. Alternative Lending Platforms
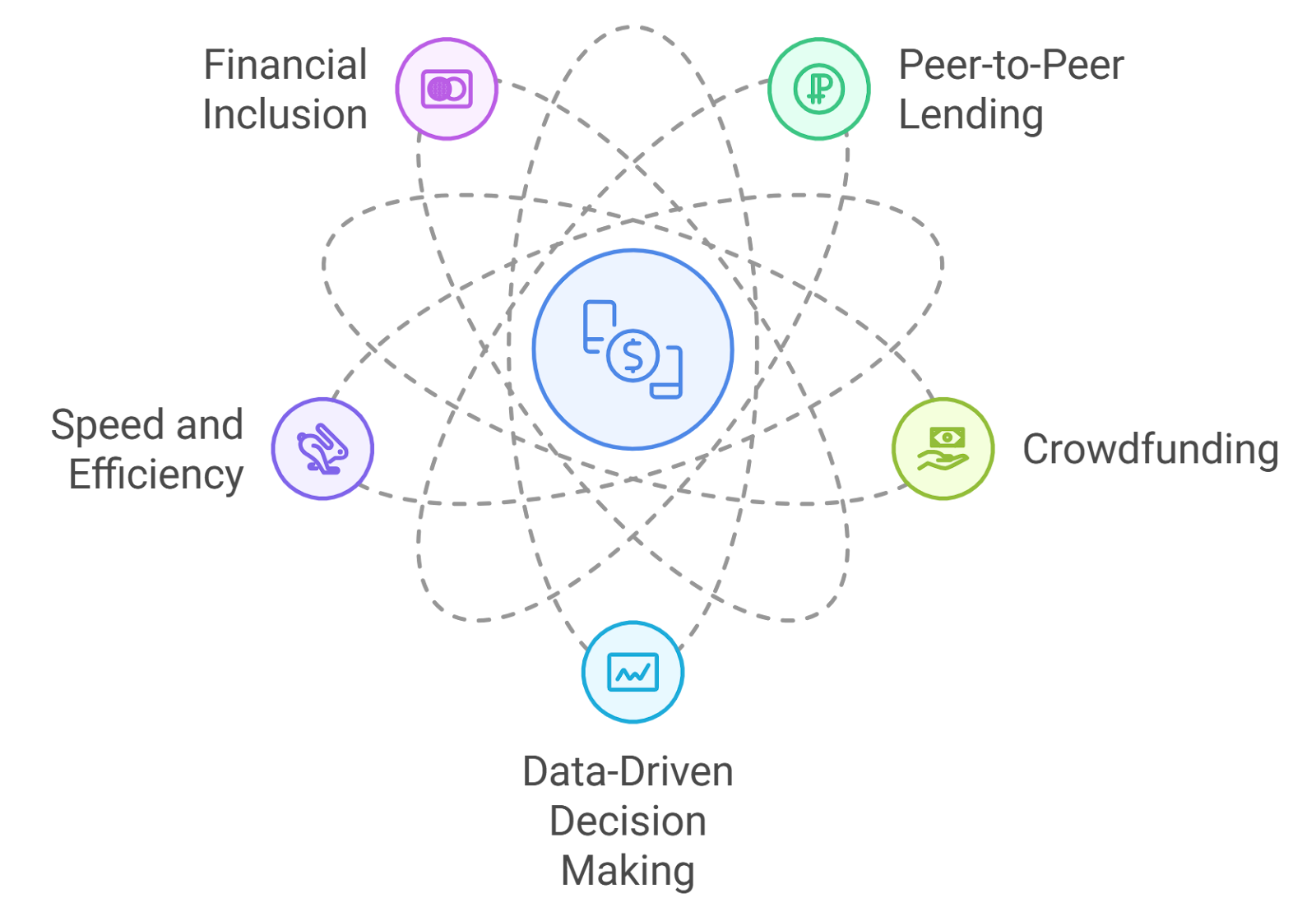
‍
Alternative lending platforms have emerged as a significant component of the FinTech landscape, providing borrowers with options outside traditional banks. These platforms utilize technology to streamline the lending process, making it more accessible and efficient.
- Peer-to-Peer Lending: This model connects borrowers directly with individual lenders, bypassing traditional financial institutions. It often results in lower interest rates for borrowers and higher returns for lenders.
- Crowdfunding: Platforms like Kickstarter and Indiegogo allow entrepreneurs to raise funds from a large number of people, often in exchange for early access to products or equity stakes. This democratizes access to capital for startups.
- Data-Driven Decision Making: Alternative lending platforms use data analytics to assess creditworthiness. By analyzing non-traditional data points, such as social media activity or transaction history, they can offer loans to individuals who may not qualify through traditional means. Rapid Innovation employs advanced AI algorithms to enhance data analysis capabilities, enabling more accurate credit assessments.
- Speed and Efficiency: The application process for alternative lending is typically faster than traditional banks. Many platforms offer instant approvals and fund disbursement, catering to the needs of borrowers who require quick access to funds.
- Financial Inclusion: Alternative lending platforms play a crucial role in promoting financial inclusion. They provide access to credit for underserved populations, including those with limited credit histories or lower income levels.
10.4. Results and Lessons Learned
The rise of FinTech startups and alternative lending platforms has yielded valuable insights and lessons for the financial industry.
- Importance of Trust: Building trust with customers is essential. Startups that prioritize transparency in their operations and communicate clearly about fees and terms tend to foster stronger relationships with users.
- Adaptability is Key: The financial landscape is constantly evolving. Successful FinTech companies demonstrate adaptability by staying ahead of regulatory changes and technological advancements.
- Customer Feedback Drives Innovation: Engaging with customers and incorporating their feedback into product development can lead to more successful offerings. Startups that actively seek user input often create solutions that better meet market demands.
- Data Security is Crucial: As FinTech companies handle sensitive financial information, robust data security measures are non-negotiable. Companies that prioritize cybersecurity can build a reputation for reliability and safety. Rapid Innovation emphasizes the importance of security in our solutions, ensuring that our clients can protect their data effectively.
- Collaboration Over Competition: The FinTech ecosystem thrives on collaboration. Startups that partner with established financial institutions or other tech companies can leverage shared resources and expertise to enhance their offerings.
- Focus on Financial Literacy: Educating customers about financial products and services can empower them to make informed decisions. Startups that provide educational resources often see higher customer satisfaction and loyalty. Rapid Innovation supports our clients in developing educational tools that enhance user understanding and engagement.