Table Of Contents
Category
FinTech
1. Introduction to AI in Investment Recommendations
Artificial Intelligence (AI) is revolutionizing the financial sector, particularly in ai investment recommendations. By leveraging advanced algorithms and machine learning, AI agents can analyze vast amounts of data, identify patterns, and provide insights that were previously unattainable. This transformation is reshaping how investors make decisions, leading to more informed and strategic choices.
- AI agents can process data at unprecedented speeds, allowing for real-time analysis.
- They utilize historical data, market trends, and economic indicators to generate ai investment recommendations.
- The integration of AI in investment strategies enhances accuracy and reduces human error.
At Rapid Innovation, we harness the power of AI to help our clients achieve greater ROI through tailored ai investment recommendations. Our expertise in developing AI-driven platforms enables businesses to make data-informed decisions that align with their financial objectives.
The rise of AI in investment recommendations is driven by several factors:
- Increased data availability: The financial market generates massive amounts of data daily, which AI can analyze efficiently.
- Demand for personalized investment strategies: Investors seek tailored ai investment recommendations that align with their financial goals and risk tolerance.
- Enhanced predictive capabilities: AI can forecast market movements and trends, providing investors with a competitive edge.
As AI technology continues to evolve, its role in investment decision-making will likely expand, offering even more sophisticated tools for investors. The future of finance is increasingly intertwined with AI, making it essential for investors to understand and embrace these innovations. At Rapid Innovation, we are committed to guiding our clients through this transformative landscape, ensuring they leverage AI effectively to maximize their investment potential.
Refer to the image for a visual representation of how AI is transforming investment recommendations.
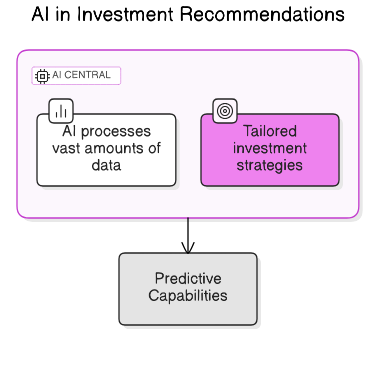
1.1. Defining AI Investment Agents
AI investment agents are sophisticated software systems that utilize artificial intelligence to assist in making investment decisions. These agents analyze vast amounts of data, identify patterns, and provide insights that can help investors optimize their portfolios.
- AI investment agents can process data at an unprecedented speed, allowing for real-time analysis of market trends. This capability enables clients to make informed decisions quickly, enhancing their competitive edge in the market.
- They employ machine learning algorithms to improve their predictions over time, adapting to new information and changing market conditions. Rapid Innovation leverages these algorithms to ensure that our clients benefit from the most accurate forecasts available.
- These AI investment agents can operate autonomously or assist human advisors, providing a hybrid approach to investment management. This flexibility allows our clients to choose the level of automation that best suits their operational needs.
- They can analyze various data sources, including financial reports, news articles, and social media sentiment, to gauge market sentiment and potential investment opportunities. By integrating these insights, Rapid Innovation helps clients uncover hidden opportunities and mitigate risks effectively. For more information on the applications of AI in banking and finance.
1.2. Evolution of Investment Decision Technologies
The evolution of investment decision technologies has transformed the financial landscape significantly. From traditional methods to advanced AI-driven solutions, the journey has been marked by several key developments.
- Early investment strategies relied heavily on human intuition and experience, often leading to inconsistent results. Rapid Innovation recognizes this limitation and offers AI solutions that enhance decision-making accuracy.
- The introduction of quantitative analysis in the 1980s marked a shift towards data-driven decision-making, utilizing statistical models to inform investment choices. Our firm builds on this foundation by incorporating advanced AI techniques that provide deeper insights.
- The rise of algorithmic trading in the 2000s allowed for automated trading strategies, executing trades at high speeds based on predefined criteria. Rapid Innovation develops custom algorithmic trading solutions that optimize execution and reduce transaction costs for our clients.
- Today, AI and machine learning are at the forefront, enabling predictive analytics and personalized investment strategies that were previously unimaginable. Our expertise in these areas allows us to deliver tailored solutions that drive greater ROI for our clients.
1.3. Paradigm Shift in Financial Advisory
The financial advisory landscape is undergoing a paradigm shift, driven by advancements in technology and changing consumer expectations. This shift is characterized by a move towards more personalized, data-driven advisory services.
- Traditional financial advisors often provided generic advice based on broad market trends, but AI investment agents can tailor recommendations to individual investor profiles. Rapid Innovation empowers clients with customized strategies that align with their unique goals.
- The integration of AI allows for continuous monitoring of investments, providing real-time insights and adjustments based on market fluctuations. Our solutions ensure that clients remain agile and responsive to market changes, maximizing their investment potential.
- Clients now expect transparency and accessibility, leading to the rise of robo-advisors that offer low-cost, automated investment management services. Rapid Innovation's offerings include robust robo-advisory platforms that enhance client engagement and satisfaction.
- This shift is not only enhancing the client experience but also enabling financial advisors to focus on higher-value tasks, such as strategic planning and relationship building. By automating routine processes, we help our clients achieve operational efficiency and drive growth.
At Rapid Innovation, we are committed to leveraging AI investment agents and blockchain technologies to help our clients achieve their business goals efficiently and effectively, ultimately leading to greater ROI.
Refer to the image based on the provided information about AI investment agents and their evolution in the financial advisory landscape:
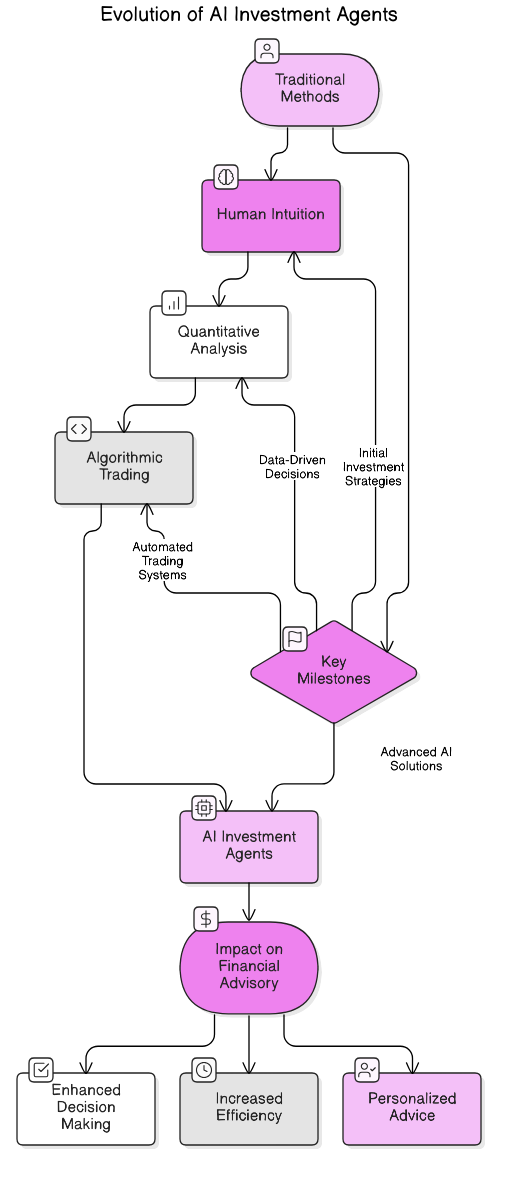
1.4. Key Challenges in Traditional Investment Approaches
Traditional investment approaches have long been the backbone of financial markets, but they face several key challenges that can hinder their effectiveness and adaptability in today's fast-paced environment.
- Market Volatility: Traditional investments are often susceptible to market fluctuations. Economic downturns, geopolitical tensions, and unexpected events can lead to significant losses, making it difficult for investors to react quickly enough to mitigate risks. This is particularly relevant for strategies like day trading for beginners, where rapid market changes can impact returns.
- High Fees and Commissions: Many traditional investment vehicles, such as mutual funds and managed portfolios, come with high management fees and commissions. These costs can erode returns over time, complicating investors' efforts to achieve their financial goals. This is a concern for those exploring alternative investing options or dividend investing.
- Limited Access to Information: Investors relying on traditional methods may not have access to real-time data and analytics. This lack of information can lead to suboptimal decision-making, as investors may miss out on critical market trends or opportunities, such as those presented by crypto arbitrage strategies or micro strategy stock.
- Behavioral Biases: Human emotions often influence investment decisions, leading to irrational behavior. Fear and greed can cause investors to buy high and sell low, undermining their long-term strategies. This is especially true in high-stakes environments like short trading stocks or option trading for beginners.
- Regulatory Constraints: Traditional investment approaches are often subject to stringent regulations that can limit flexibility. Compliance with these regulations can be time-consuming and costly for investment firms, impacting strategies like tax loss harvesting or dollar cost averaging.
- Inefficiencies in Asset Allocation: Traditional methods may not always optimize asset allocation effectively. Investors may struggle to diversify their portfolios adequately, exposing them to unnecessary risks. This is a critical issue for those considering equity investment strategies or value investing. For insights on how new approaches are addressing these challenges, you can read about how STOs are transforming fundraising and investment.
1.5. Ethical Considerations in AI-Driven Investments
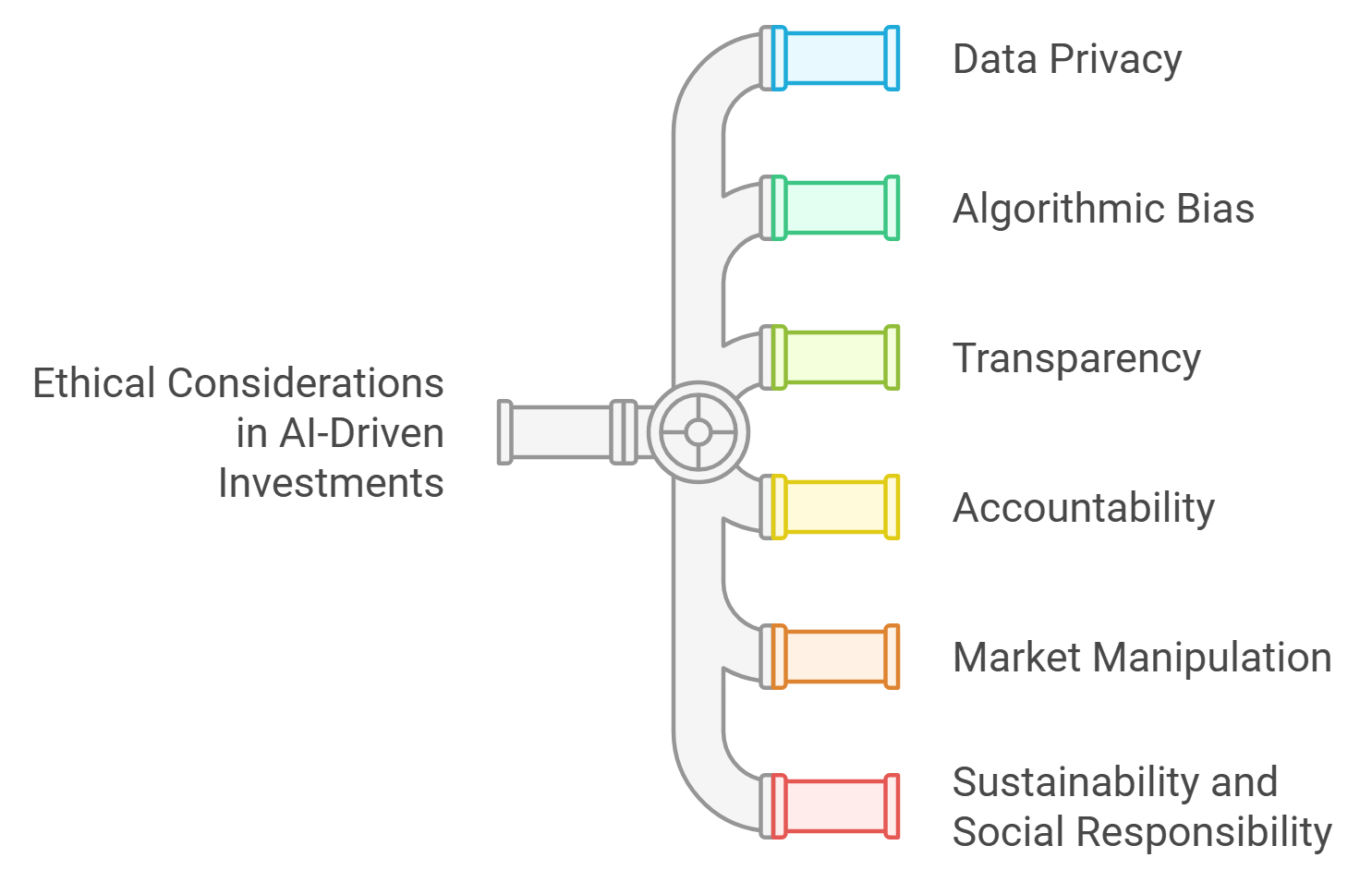
‍
As artificial intelligence (AI) becomes increasingly integrated into investment strategies, ethical considerations must be addressed to ensure responsible and fair practices.
- Data Privacy: AI-driven investment platforms often rely on vast amounts of data, including personal information. Ensuring the privacy and security of this data is paramount to maintain investor trust.
- Algorithmic Bias: AI systems can inadvertently perpetuate biases present in the data they are trained on. This can lead to unfair treatment of certain groups or individuals, raising ethical concerns about equity in investment opportunities.
- Transparency: The complexity of AI algorithms can make it challenging for investors to understand how decisions are made. Ensuring transparency in AI-driven investment processes is essential to build trust and accountability.
- Accountability: When AI systems make investment decisions, determining accountability for losses or unethical behavior becomes complicated. Clear guidelines must be established to address who is responsible for the outcomes of AI-driven investments.
- Market Manipulation: The speed and efficiency of AI can lead to concerns about market manipulation. If not regulated properly, AI-driven trading strategies could exploit market inefficiencies in ways that harm other investors.
- Sustainability and Social Responsibility: Investors increasingly seek to align their portfolios with ethical and sustainable practices. AI-driven investment strategies must consider environmental, social, and governance (ESG) factors to meet these demands.
2. Foundational Technologies
The landscape of investment is rapidly evolving, driven by foundational technologies that enhance efficiency, accuracy, and accessibility.
- Blockchain Technology: Blockchain provides a decentralized ledger that enhances transparency and security in transactions. It can streamline processes such as clearing and settlement, reducing costs and time delays. Rapid Innovation leverages blockchain to create secure and efficient investment solutions that minimize risks and enhance trust.
- Artificial Intelligence and Machine Learning: AI and machine learning algorithms analyze vast datasets to identify patterns and trends. These technologies enable predictive analytics, helping investors make informed decisions based on data-driven insights. At Rapid Innovation, we implement AI solutions that optimize investment strategies, leading to greater ROI for our clients.
- Big Data Analytics: The ability to process and analyze large volumes of data allows investors to gain deeper insights into market trends and consumer behavior. This can lead to more informed investment strategies and better risk management. Our expertise in big data analytics empowers clients to harness data for strategic decision-making.
- Robo-Advisors: These automated platforms use algorithms to provide investment advice and portfolio management services. They offer low-cost alternatives to traditional financial advisors, making investing more accessible to a broader audience. Rapid Innovation develops customized robo-advisory solutions that cater to diverse investor needs.
- Cloud Computing: Cloud technology enables scalable storage and processing power, allowing investment firms to manage and analyze data more efficiently. This flexibility supports real-time decision-making and enhances collaboration among teams. We utilize cloud solutions to enhance operational efficiency for our clients.
- Internet of Things (IoT): IoT devices collect real-time data from various sources, providing valuable insights into market conditions. This data can inform investment strategies and enhance risk assessment. Rapid Innovation integrates IoT data into investment frameworks to provide clients with a competitive edge.
- Cybersecurity Solutions: As investments become increasingly digital, robust cybersecurity measures are essential to protect sensitive financial information. Advanced security technologies help safeguard against data breaches and fraud. Our cybersecurity solutions ensure that client investments are secure and compliant with regulations.
- Augmented and Virtual Reality: These technologies can enhance investor experiences by providing immersive environments for analyzing data and visualizing market trends. They can also facilitate remote collaboration among investment teams. Rapid Innovation explores AR and VR applications to enrich client engagement and decision-making processes.
By leveraging these foundational technologies, investors can navigate the complexities of modern financial markets more effectively, ultimately leading to better investment outcomes. Rapid Innovation is committed to helping clients achieve their business goals efficiently and effectively through innovative AI and blockchain solutions.
Refer to the image for a visual representation of the key challenges in traditional investment approaches.

2.1. Machine Learning Fundamentals
Machine learning (ML) is a subset of artificial intelligence (AI) that focuses on the development of algorithms that allow computers to learn from and make predictions based on data. Understanding the fundamentals of machine learning is crucial for anyone looking to delve into this field, and at Rapid Innovation, we leverage these principles to help our clients achieve their business goals efficiently and effectively.
- Types of Machine Learning: Â
- Supervised Learning: Involves training a model on a labeled dataset, where the input data is paired with the correct output. Common algorithms include linear regression, decision trees, and support vector machines. Rapid Innovation utilizes supervised learning to enhance predictive analytics for clients, enabling them to make data-driven decisions that lead to greater ROI. This is a key aspect of machine learning fundamentals.
- Unsupervised Learning: In this approach, the model is trained on data without labeled responses. It aims to find hidden patterns or intrinsic structures in the input data. Examples include clustering algorithms like K-means and hierarchical clustering. By applying unsupervised learning, we help clients uncover valuable insights from their data, driving innovation and strategic planning. Understanding the fundamentals of AI and ML is essential here.
- Reinforcement Learning: This type of learning is based on the idea of agents taking actions in an environment to maximize cumulative rewards. It is widely used in robotics and game playing. Our expertise in reinforcement learning allows us to develop intelligent systems that adapt and optimize processes, ultimately enhancing operational efficiency. This is part of the fundamentals of reinforcement learning.
- Key Concepts: Â
- Features and Labels: Features are the input variables used for making predictions, while labels are the output variables that the model aims to predict. Understanding these concepts is essential for our clients to effectively utilize machine learning models. This is a fundamental concept in machine learning fundamentals.
- Training and Testing: The dataset is typically split into a training set to train the model and a testing set to evaluate its performance. Rapid Innovation emphasizes the importance of this process to ensure robust model performance and reliability. This is a critical aspect of machine learning fundamentals.
- Overfitting and Underfitting: Overfitting occurs when a model learns the training data too well, capturing noise instead of the underlying pattern. Underfitting happens when a model is too simple to capture the data's complexity. We guide our clients in balancing these aspects to achieve optimal model performance. Understanding these concepts is part of the fundamentals of machine learning and deep learning.
- Applications: Â
- Image recognition
- Fraud detection
- Predictive analytics
At Rapid Innovation, we harness the power of machine learning to deliver tailored solutions that drive measurable results for our clients. Our machine learning fundamentals course is designed to equip individuals with the necessary skills to excel in this field.
2.2. Deep Learning Architectures
Deep learning is a specialized area of machine learning that uses neural networks with many layers (hence "deep") to analyze various forms of data. It has gained immense popularity due to its effectiveness in handling large datasets and complex problems.
- Types of Neural Networks: Â
- Convolutional Neural Networks (CNNs): Primarily used for image processing tasks, CNNs excel at recognizing patterns and features in visual data. They utilize convolutional layers to automatically detect features. Rapid Innovation employs CNNs to develop advanced image recognition systems that enhance user experiences and operational efficiencies. This is a fundamental aspect of deep learning fundamentals.
- Recurrent Neural Networks (RNNs): Designed for sequential data, RNNs are effective in tasks like language modeling and time series prediction. They maintain a memory of previous inputs, making them suitable for tasks where context is important. Our expertise in RNNs allows us to create predictive models that can forecast trends and behaviors, providing clients with a competitive edge. Understanding the fundamentals of deep learning is crucial here.
- Generative Adversarial Networks (GANs): Comprising two neural networks, a generator and a discriminator, GANs are used to generate new data samples that resemble a given dataset. They are popular in image generation and enhancement. We leverage GANs to create innovative solutions that push the boundaries of traditional data generation.
- Training Deep Learning Models: Â
- Backpropagation: A key algorithm used for training neural networks, backpropagation calculates the gradient of the loss function and updates the weights to minimize errors. Our team at Rapid Innovation ensures that models are trained effectively to achieve high accuracy. This is a fundamental technique in deep learning fundamentals.
- Activation Functions: Functions like ReLU (Rectified Linear Unit) and sigmoid are used to introduce non-linearity into the model, allowing it to learn complex patterns. We guide clients in selecting the right activation functions to optimize their models.
- Challenges: Â
- Requires large amounts of labeled data
- Computationally intensive, often needing GPUs for training
- Risk of overfitting if not properly regularized
2.3. Natural Language Processing
Natural Language Processing (NLP) is a field at the intersection of computer science, artificial intelligence, and linguistics. It focuses on enabling machines to understand, interpret, and respond to human language in a valuable way.
- Core Tasks in NLP: Â
- Text Classification: Assigning predefined categories to text, such as spam detection in emails or sentiment analysis in reviews. Rapid Innovation utilizes text classification to help clients enhance customer engagement and satisfaction. This is a key application of machine learning fundamentals.
- Named Entity Recognition (NER): Identifying and classifying key entities in text, such as names, dates, and locations. Our NER solutions enable clients to extract valuable insights from unstructured data, driving informed decision-making. Understanding the fundamentals of NLP is essential here.
- Machine Translation: Automatically translating text from one language to another, as seen in tools like Google Translate. We develop advanced translation systems that facilitate global communication for our clients.
- Techniques and Models: Â
- Tokenization: The process of breaking down text into smaller units, such as words or phrases, to facilitate analysis. Our expertise in tokenization ensures that clients' NLP models are built on a solid foundation.
- Word Embeddings: Techniques like Word2Vec and GloVe convert words into numerical vectors, capturing semantic relationships between them. We implement these techniques to enhance the understanding of context in client applications.
- Transformers: A revolutionary architecture that has transformed NLP; models like BERT and GPT utilize attention mechanisms to process text more effectively. Rapid Innovation leverages transformers to create state-of-the-art NLP solutions that meet our clients' needs.
- Applications: Â
- Chatbots and virtual assistants
- Content recommendation systems
- Sentiment analysis for market research
Understanding these foundational concepts in machine learning, deep learning architectures, and natural language processing is essential for leveraging AI technologies effectively. Each area offers unique tools and methodologies that can be applied across various industries, driving innovation and efficiency. At Rapid Innovation, we are committed to helping our clients harness these technologies to achieve greater ROI and business success. Our offerings include courses on AI ML basics and fundamentals of AI and ML to further enhance understanding in these areas.
Refer to the image for a visual representation of the concepts discussed in the Machine Learning Fundamentals section.

2.4. Predictive Analytics
Predictive analytics is a branch of advanced analytics that uses historical data, statistical algorithms, and machine learning techniques to identify the likelihood of future outcomes. This approach is widely used across various industries to enhance decision-making processes.
- Key components of predictive analytics include: Â
- Data collection: Gathering relevant data from various sources, including databases, social media, and IoT devices.
- Data processing: Cleaning and organizing data to ensure accuracy and reliability.
- Model building: Developing statistical models that can predict future trends based on historical data.
- Validation: Testing the model against new data to ensure its predictive power.
- Applications of predictive analytics: Â
- Marketing: Businesses can predict customer behavior, allowing for targeted marketing strategies that enhance customer engagement and conversion rates.
- Healthcare: Predictive models can forecast patient outcomes, helping in resource allocation and treatment planning, ultimately improving patient care and operational efficiency. This includes applications of predictive analytics in healthcare and specific hr predictive analytics tools.
- Finance: Financial institutions use predictive analytics to assess credit risk and detect fraudulent activities, leading to better risk management and reduced losses. Predictive modeling applications are also prevalent in this sector.
At Rapid Innovation, we leverage predictive analytics to empower our clients with data-driven insights that enhance operational efficiency and drive growth. By utilizing our expertise in AI, we help businesses anticipate trends and make informed choices that lead to greater ROI. Our services include predictive analytics applications and the application of predictive analysis tailored to specific business needs, such as crm predictive modeling.
2.5. Big Data Integration
Big data integration refers to the process of combining data from various sources into a unified view, enabling organizations to analyze and derive insights from large volumes of data. This integration is crucial for businesses looking to harness the power of big data for strategic decision-making.
- Key aspects of big data integration include: Â
- Data sources: Integrating data from diverse sources such as databases, cloud storage, and real-time data streams.
- Data formats: Handling various data formats, including structured, semi-structured, and unstructured data.
- Data quality: Ensuring the accuracy, consistency, and completeness of integrated data.
- Benefits of big data integration: Â
- Enhanced insights: A unified view of data allows for more comprehensive analysis and better decision-making, leading to improved business strategies.
- Improved efficiency: Streamlining data processes reduces redundancy and saves time, allowing organizations to focus on core activities.
- Real-time analytics: Organizations can access and analyze data in real-time, leading to quicker responses to market changes and opportunities.
Big data integration is essential for organizations aiming to leverage data for competitive advantage. At Rapid Innovation, we specialize in integrating diverse data sources to unlock valuable insights that drive innovation and growth, ultimately enhancing our clients' ROI.
2.6. Neural Network Approaches
Neural network approaches are a subset of machine learning techniques inspired by the human brain's structure and function. These models are designed to recognize patterns and make predictions based on complex data inputs.
- Key features of neural networks include: Â
- Layers: Neural networks consist of input, hidden, and output layers, where each layer processes data and passes it to the next.
- Activation functions: These functions determine the output of each neuron, enabling the network to learn complex patterns.
- Training: Neural networks are trained using large datasets, adjusting weights and biases to minimize prediction errors.
- Applications of neural network approaches: Â
- Image recognition: Neural networks excel in identifying objects and patterns in images, making them valuable in fields like healthcare and security.
- Natural language processing: These models are used in chatbots and virtual assistants to understand and respond to human language, enhancing customer interactions.
- Financial forecasting: Neural networks can analyze market trends and predict stock prices, aiding investors in making informed decisions.
Neural network approaches are revolutionizing various industries by providing powerful tools for data analysis and prediction. Their ability to learn from vast amounts of data makes them indispensable in today's data-driven world. At Rapid Innovation, we harness the power of neural networks to deliver innovative solutions that drive efficiency and profitability for our clients. This includes applications of predictive modeling and predictive analytics applications examples that showcase our capabilities.
3. AI Agent Capabilities

‍
AI agents are increasingly becoming integral to various industries, leveraging advanced algorithms and machine learning to enhance decision-making processes. Their capabilities span a wide range of applications, particularly in artificial intelligence industry overview, market trend analysis, and risk assessment, which are essential for achieving business goals efficiently and effectively.
3.1. Market Trend Analysis
Market trend analysis involves examining historical data to identify patterns and predict future movements in the market. AI agents excel in this area due to their ability to process vast amounts of data quickly and accurately.
AI agents can analyze large datasets from multiple sources, including social media, news articles, and financial reports, to identify emerging trends. By utilizing machine learning algorithms, they can forecast market trends based on historical data, helping businesses make informed decisions. Additionally, AI can gauge public sentiment by analyzing social media posts and news articles, providing insights into consumer behavior and market dynamics. They can continuously monitor market conditions, allowing businesses to react swiftly to changes and capitalize on opportunities. Furthermore, AI tools can assess competitors' strategies and performance, helping businesses understand their position in the market.
The effectiveness of AI in market trend analysis is evident, with studies showing that companies using AI-driven analytics can improve their forecasting accuracy by up to 30% (source: McKinsey). At Rapid Innovation, we leverage these capabilities to help our clients optimize their strategies, ensuring they stay ahead of the competition and maximize their return on investment.
3.2. Risk Assessment
Risk assessment is crucial for businesses to identify potential threats and mitigate them effectively. AI agents enhance this process by providing deeper insights and more accurate evaluations.
AI agents can aggregate data from various sources, including financial records, market trends, and regulatory changes, to provide a comprehensive risk profile. They can create predictive models that simulate various scenarios, helping businesses understand potential risks and their impacts. Machine learning algorithms can identify unusual patterns in data that may indicate fraud or operational risks, allowing for timely intervention. Moreover, AI agents improve over time by learning from new data, ensuring that risk assessments remain relevant and accurate. They can also help businesses stay compliant with regulations by monitoring changes in laws and assessing their potential impact on operations.
The integration of AI in risk assessment has shown to reduce risk-related losses by up to 25% in some industries (source: Deloitte). Rapid Innovation employs these advanced AI capabilities to assist our clients in navigating risks effectively, ultimately leading to enhanced operational resilience and improved financial performance.
In conclusion, AI agents are transforming market trend analysis and risk assessment, providing businesses with the tools they need to navigate complex environments effectively. Their capabilities not only enhance decision-making but also drive competitive advantage in an increasingly data-driven world. At Rapid Innovation, we are committed to harnessing the power of AI to help our clients achieve their business goals and realize greater ROI. For more information on our services, visit our AI agent development company.
3.3. Portfolio Optimization
Portfolio optimization is a crucial aspect of investment management that aims to maximize returns while minimizing risk. This process involves selecting the right mix of assets to achieve the desired financial goals. A well-optimized portfolio includes a variety of asset classes, such as stocks, bonds, and real estate, to spread risk. Investors must evaluate their risk tolerance and adjust their portfolios accordingly, utilizing tools like the Sharpe ratio to measure risk-adjusted returns. Strategic asset allocation is essential, as it involves determining the percentage of the portfolio to invest in different asset classes based on market conditions and individual goals. Regularly rebalancing the portfolio ensures that it remains aligned with the investor's risk profile and investment objectives, which may involve selling overperforming assets and buying underperforming ones. Many investors now utilize algorithms and software for portfolio optimization, including stock optimizers and advanced techniques like markowitz portfolio optimization, which can analyze vast amounts of data to suggest the best asset combinations. At Rapid Innovation, we leverage advanced AI algorithms to enhance portfolio optimization, enabling our clients to achieve greater ROI through data-driven insights and automated rebalancing strategies. Our approach also incorporates efficient portfolio strategies and the sharpe ratio portfolio optimization to ensure optimal performance. Additionally, we offer crypto market-making services to further enhance investment strategies in the digital asset space.
3.4. Real-Time Investment Recommendations
Real-time investment recommendations are increasingly important in today's fast-paced financial markets. These recommendations provide investors with timely insights to make informed decisions. Real-time data analysis allows investors to track market trends and identify potential investment opportunities as they arise. Many platforms offer automated alerts for price changes, news events, or technical indicators, enabling investors to act quickly. Advanced technologies like AI and machine learning are used to analyze historical data and predict future market movements, providing investors with actionable insights. Real-time recommendations can be tailored to individual investment strategies, risk tolerance, and financial goals, enhancing the relevance of the advice. Additionally, platforms that incorporate social trading allow investors to follow and replicate the strategies of successful traders in real-time, providing additional insights and recommendations.
3.5. Sentiment Analysis
Sentiment analysis is a powerful tool used to gauge market sentiment and investor behavior. By analyzing public opinion, investors can make more informed decisions. Sentiment analysis often utilizes data from social media, news articles, and financial reports to assess the overall mood of the market. Advanced algorithms process large volumes of text data to identify positive, negative, or neutral sentiments regarding specific assets or the market as a whole. By understanding market sentiment, investors can anticipate price movements and adjust their strategies accordingly; for example, a surge in positive sentiment may indicate a potential rise in stock prices. Sentiment analysis can also help in risk management by identifying potential market downturns based on negative sentiment trends. Many traders incorporate sentiment analysis into their trading strategies, using it alongside technical and fundamental analysis to enhance decision-making. Rapid Innovation employs cutting-edge sentiment analysis tools to provide our clients with deeper insights into market dynamics, ultimately leading to more informed investment decisions and improved ROI.
3.6. Personalized Investment Strategies
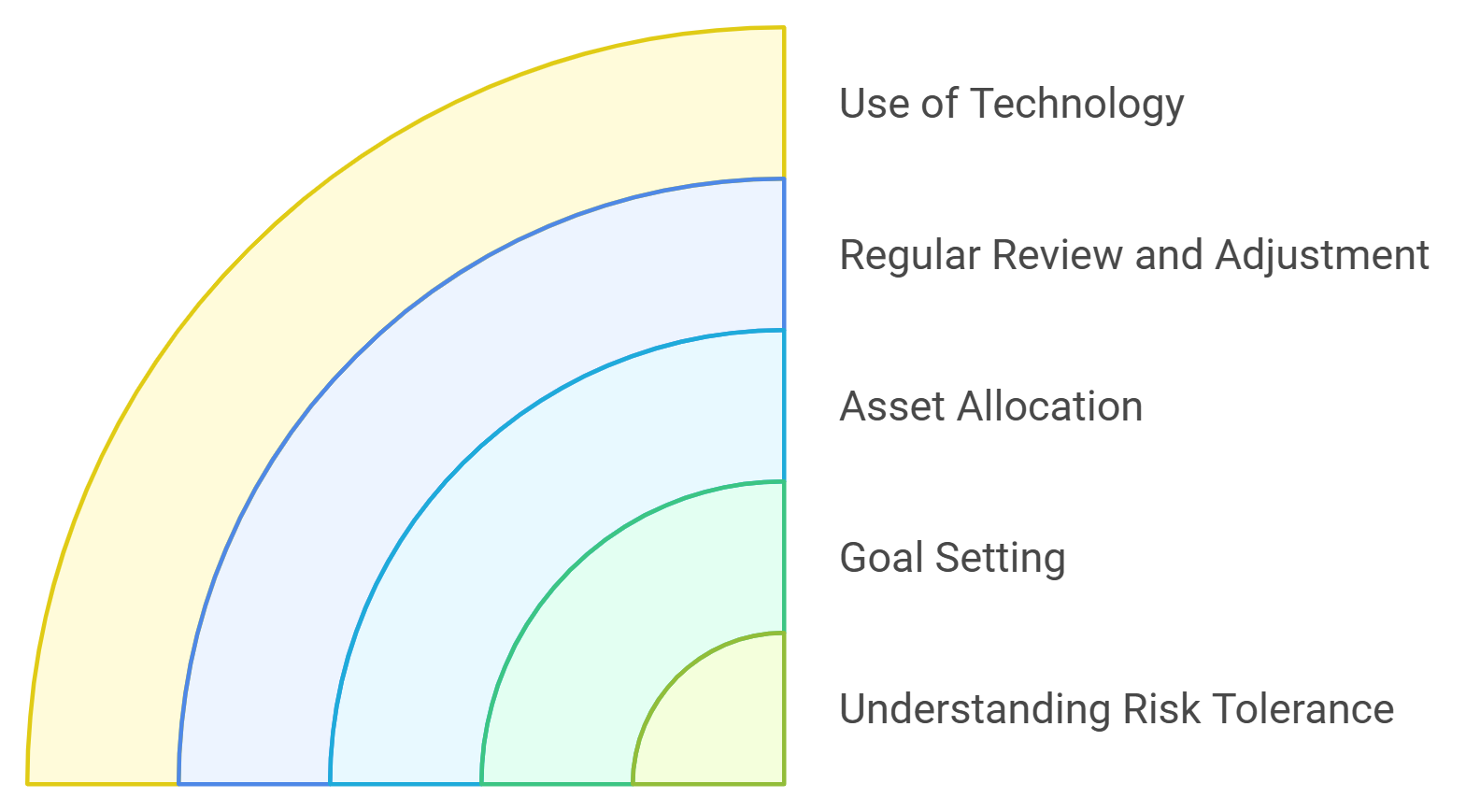
‍
Personalized investment strategies are tailored approaches that consider an individual’s financial goals, risk tolerance, and investment horizon. These strategies are designed to optimize returns while minimizing risks based on the unique circumstances of each investor.
- Understanding Risk Tolerance: Assessing how much risk an investor is willing to take is crucial. This can be influenced by age, income, and financial obligations. Tools like risk assessment questionnaires can help gauge an investor's comfort level with market fluctuations.
- Goal Setting: Investors should clearly define their financial goals, whether it's saving for retirement, funding education, or purchasing a home. Strategies can be aligned with short-term, medium-term, or long-term objectives.
- Asset Allocation: A personalized strategy often includes a diversified portfolio that balances equities, bonds, and alternative investments. For example, the best asset allocation for a Roth IRA might include a mix of stocks and bonds tailored to the investor's age and risk tolerance. The allocation can be adjusted based on market conditions and personal circumstances.
- Regular Review and Adjustment: Investment strategies should not be static. Regular reviews allow for adjustments based on performance and changing life situations. Rebalancing the portfolio ensures that it remains aligned with the investor's goals and risk tolerance. This is particularly important for personalized investment strategies, such as those offered by personal capital investment strategy tools.
- Use of Technology: Rapid Innovation leverages advanced AI algorithms and blockchain technology to provide personalized recommendations through robo-advisors and investment platforms. These tools analyze market data and individual preferences, enabling investors to make informed decisions without needing extensive financial knowledge. This is especially beneficial for young adults seeking investing strategies that fit their unique situations, such as the best investment strategy for young adults.
3.7. Cross-Asset Class Recommendations
Cross-asset class recommendations involve strategies that span multiple asset classes, such as equities, fixed income, real estate, and commodities. This approach aims to enhance portfolio diversification and optimize returns.
- Diversification Benefits: Investing across different asset classes can reduce overall portfolio risk. When one asset class underperforms, others may compensate. Historical data shows that a diversified portfolio can lead to more stable returns over time.
- Market Conditions: Cross-asset class strategies can be adjusted based on prevailing market conditions. For instance, during economic downturns, investors might shift towards safer assets like bonds or gold. Understanding macroeconomic indicators can help in making informed cross-asset class decisions.
- Tactical Asset Allocation: This strategy involves actively adjusting the mix of asset classes based on market forecasts and economic trends. Investors can capitalize on short-term opportunities while maintaining a long-term investment strategy. This is similar to the tactical asset allocation approach used in the Dave Ramsey mutual fund strategy.
- Thematic Investing: Cross-asset class recommendations can also focus on specific themes, such as sustainability or technology, allowing investors to align their portfolios with their values. This approach can include investments in stocks, bonds, and real estate that support a particular theme.
- Professional Guidance: Financial advisors can provide insights into cross-asset class strategies, helping investors navigate complex markets. Utilizing their expertise can lead to more informed investment decisions and better portfolio performance. This is particularly relevant for those exploring personalized investment strategies or seeking guidance on day trading Dave Ramsey style.
4. Technical Architecture
Technical architecture refers to the underlying framework that supports investment strategies and portfolio management. It encompasses the systems, tools, and processes that facilitate efficient investment operations.
- Data Management: A robust technical architecture must include effective data management systems to handle vast amounts of financial data. This includes data collection, storage, and analysis to inform investment decisions.
- Integration of Tools: Investment platforms should integrate various tools for analysis, trading, and reporting. This ensures seamless operations and enhances user experience. APIs (Application Programming Interfaces) can facilitate the integration of different financial services and data sources.
- Security Measures: Given the sensitive nature of financial data, strong security protocols are essential. This includes encryption, secure access controls, and regular security audits. Compliance with regulations such as GDPR and FINRA is crucial to protect investor information.
- Scalability: The architecture should be scalable to accommodate growth in user base and data volume. This ensures that the system can handle increased demand without compromising performance. Cloud-based solutions can offer flexibility and scalability for investment firms.
- User Interface and Experience: A user-friendly interface is vital for both investors and financial advisors. It should provide easy access to information and tools necessary for making informed decisions. Continuous feedback from users can help improve the interface and overall experience.
- Analytics and Reporting: Advanced analytics tools can provide insights into portfolio performance and market trends. This helps investors make data-driven decisions. Customizable reporting features allow users to generate reports that meet their specific needs.
- Continuous Improvement: The technical architecture should be regularly updated to incorporate new technologies and methodologies. Staying current with industry trends ensures that the investment platform remains competitive and effective. Rapid Innovation is committed to integrating cutting-edge AI and blockchain solutions to enhance the efficiency and effectiveness of investment operations.
4.1. Data Collection Mechanisms
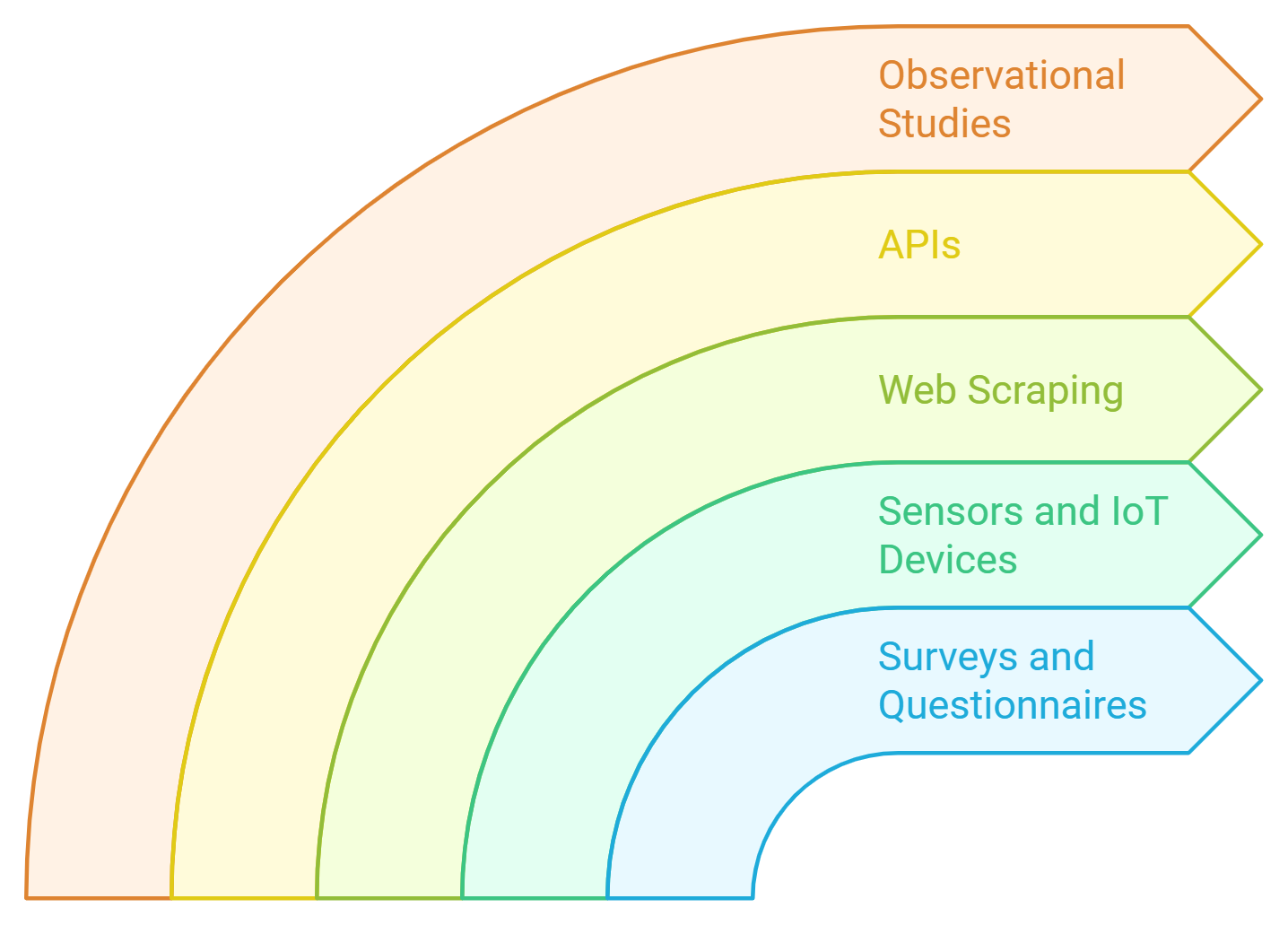
‍
Data collection mechanisms are essential for gathering relevant information that can be analyzed to derive insights. These mechanisms can vary widely depending on the type of data needed and the context in which it is collected.
- Surveys and Questionnaires: These tools are commonly used to gather quantitative and qualitative data from a specific population. They can be distributed online or in person, allowing for a broad reach. Rapid Innovation can assist in designing and implementing these surveys to ensure that the data collected aligns with your business objectives.
- Sensors and IoT Devices: In the age of the Internet of Things (IoT), sensors collect real-time data from various environments. This data can include temperature, humidity, and even user interactions. By leveraging our expertise in IoT, Rapid Innovation can help you integrate these devices into your operations, enabling you to make data-driven decisions in real-time.
- Web Scraping: This technique involves extracting data from websites and is particularly useful for gathering large datasets from online sources, such as social media or e-commerce platforms. Rapid Innovation can develop custom web scraping solutions tailored to your specific needs, allowing you to gain insights from vast amounts of online data.
- APIs: Application Programming Interfaces (APIs) allow for the automated collection of data from other software applications, which is particularly useful for integrating data from multiple sources. Our team can help you design and implement APIs that streamline your data collection processes, enhancing your operational efficiency.
- Observational Studies: Researchers can collect data through direct observation, which is particularly useful in fields like social sciences and healthcare. Rapid Innovation can assist in structuring these studies to ensure that the data collected is both relevant and actionable.
4.2. Information Processing Frameworks
Information processing frameworks are structured approaches to handling and analyzing data. These frameworks help organizations make sense of the vast amounts of data they collect.
- Data Warehousing: This involves storing large volumes of data in a centralized repository, facilitating easy access and analysis, and allowing for better decision-making. Rapid Innovation can help you design a data warehousing solution that meets your specific business needs, ensuring that your data is organized and accessible.
- ETL Processes: Extract, Transform, Load (ETL) processes are crucial for preparing data for analysis. Data is extracted from various sources, transformed into a suitable format, and then loaded into a data warehouse. Our expertise in ETL processes can help you streamline your data preparation, leading to more efficient analysis.
- Data Lakes: Unlike data warehouses, data lakes store raw data in its native format, allowing for more flexibility in data analysis, as users can access and analyze data without predefined schemas. Rapid Innovation can assist in setting up a data lake that supports your analytical needs, enabling you to derive insights from diverse data sources.
- Machine Learning Frameworks: These frameworks, such as TensorFlow and PyTorch, provide tools for building and deploying machine learning models, enabling organizations to automate data analysis and derive insights from complex datasets. Our team can help you implement machine learning solutions that drive innovation and improve decision-making.
- Data Visualization Tools: Tools like Tableau and Power BI help in presenting data in a visual format, making it easier for stakeholders to understand trends and patterns. Rapid Innovation can assist in creating compelling visualizations that communicate your data insights effectively.
4.3. Decision-Making Algorithms
Decision-making algorithms are mathematical models that help organizations make informed choices based on data analysis. These algorithms can significantly enhance the efficiency and accuracy of decision-making processes.
- Predictive Analytics: Algorithms in this category analyze historical data to predict future outcomes. Businesses often use predictive analytics for sales forecasting and customer behavior analysis. Rapid Innovation can help you implement predictive analytics solutions that enhance your strategic planning and operational efficiency.
- Classification Algorithms: These algorithms categorize data into predefined classes. For example, spam detection in emails uses classification algorithms to filter unwanted messages. Our expertise in classification algorithms can help you automate processes and improve data management.
- Clustering Algorithms: Clustering algorithms group similar data points together, which is useful in market segmentation, where businesses can identify distinct customer groups based on purchasing behavior. Rapid Innovation can assist in applying clustering techniques to better understand your customer base and tailor your marketing strategies.
- Reinforcement Learning: This type of algorithm learns optimal actions through trial and error. It is commonly used in robotics and game development, where the algorithm improves its performance over time. Our team can help you explore reinforcement learning applications that drive innovation in your business processes.
- Decision Trees: These algorithms use a tree-like model of decisions and their possible consequences. They are easy to interpret and are widely used in various fields, including finance and healthcare. Rapid Innovation can assist in developing decision tree models that enhance your decision-making capabilities, leading to improved outcomes.
4.4. Recommendation Generation
Recommendation generation is a crucial aspect of many digital platforms, particularly in e-commerce, streaming services, and social media. The goal is to provide personalized suggestions to users based on their preferences and behaviors.
- Algorithms analyze user data, including past purchases, browsing history, and ratings, to generate tailored recommendations. At Rapid Innovation, we leverage advanced algorithms, including recommendation engine algorithms, to ensure that our clients can deliver highly relevant suggestions to their users, enhancing their overall experience.
- Collaborative filtering is a popular method, where the system identifies patterns among users with similar tastes to suggest items they might like. By implementing collaborative filtering, we help clients increase user engagement and drive sales through targeted recommendations, similar to those used by platforms like Movielens and Netflix.
- Content-based filtering focuses on the attributes of items themselves, recommending similar products based on user preferences. Our expertise in content-based filtering allows us to create systems that understand product features, leading to more accurate suggestions, akin to the content-based filtering used in recommendation systems like YouTube recommender.
- Hybrid models combine both collaborative and content-based filtering to enhance accuracy and user satisfaction. Rapid Innovation specializes in developing hybrid models that maximize the effectiveness of recommendation systems, resulting in higher conversion rates for our clients, similar to the approaches used by Amazon recommender and Spotify recommender.
- Machine learning techniques, such as neural networks, can improve the sophistication of recommendations by learning complex patterns in user behavior. Our team utilizes state-of-the-art machine learning techniques, including recommendation machine learning and recommendation system machine learning, to refine recommendation systems, ensuring they evolve with user preferences.
- The effectiveness of recommendation systems can significantly impact user engagement and retention, leading to increased sales and customer loyalty. By partnering with Rapid Innovation, organizations can achieve greater ROI through enhanced user experiences driven by intelligent recommendation systems, including AI recommender solutions and AI-powered product recommendations in e-commerce.
4.5. Continuous Learning Models
Continuous learning models are designed to adapt and evolve over time, ensuring that systems remain relevant and effective as user preferences and external conditions change.
- These models utilize real-time data to update their algorithms, allowing them to learn from new information continuously. Rapid Innovation implements continuous learning models that empower our clients to stay ahead of market trends and user demands.
- Techniques such as online learning enable systems to adjust their parameters incrementally, rather than requiring retraining from scratch. Our solutions incorporate online learning techniques, ensuring that clients can maintain system performance without significant downtime.
- Continuous learning is essential in dynamic environments where user behavior can shift rapidly, such as in social media or news platforms. We help organizations adapt to these changes by integrating continuous learning into their systems, enhancing responsiveness and relevance.
- Reinforcement learning is another approach, where models learn optimal actions through trial and error, receiving feedback from their environment. Our expertise in reinforcement learning allows us to develop systems that optimize user interactions, leading to improved outcomes.
- The integration of continuous learning can lead to improved accuracy in predictions and recommendations, enhancing user experience. By leveraging continuous learning, Rapid Innovation ensures that our clients can provide timely and relevant content to their users.
- Organizations that implement continuous learning models can respond more effectively to market trends and user needs, maintaining a competitive edge. Our tailored solutions enable clients to harness the power of continuous learning for sustained growth and profitability.
4.6. Feedback Loop Integration
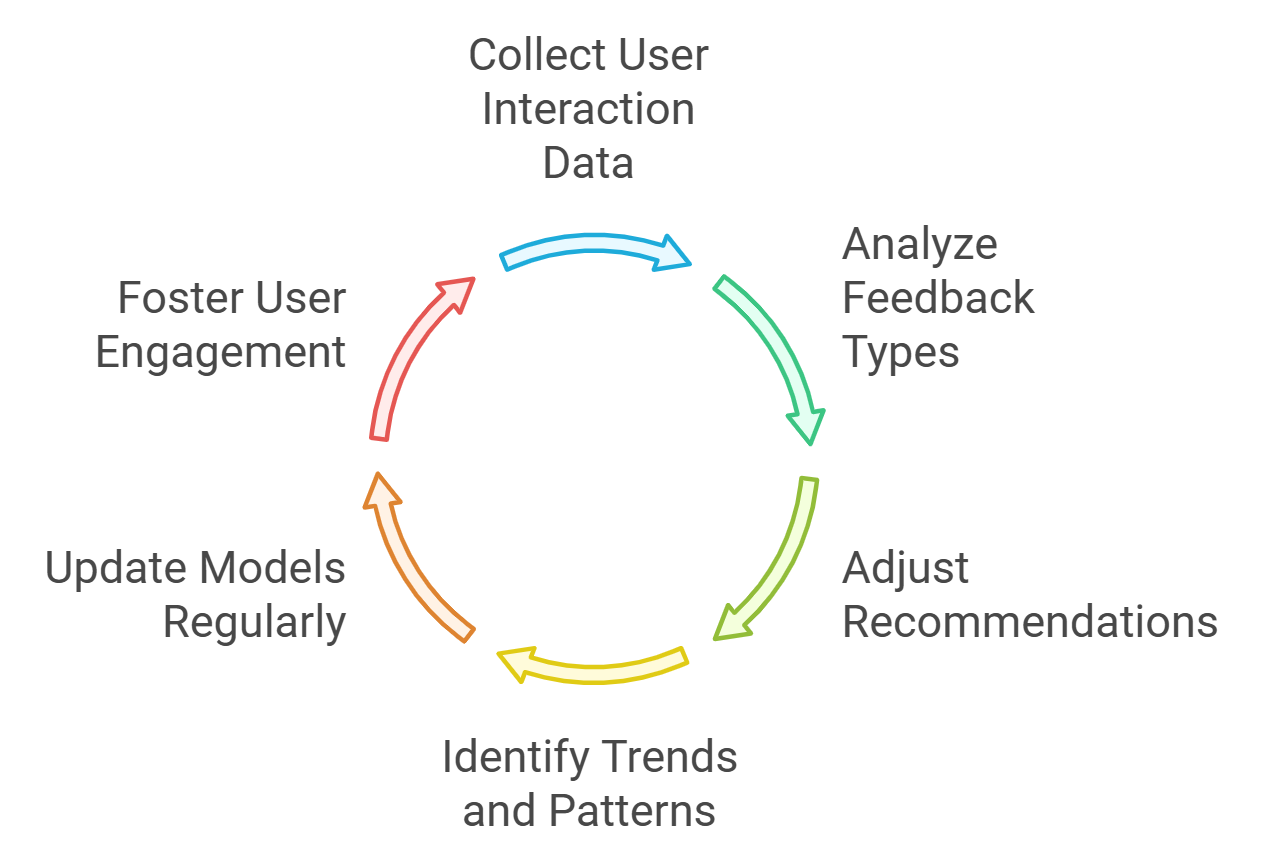
‍
Feedback loop integration is vital for refining and improving recommendation systems and continuous learning models. It involves collecting user feedback and using it to enhance the system's performance.
- User interactions, such as clicks, purchases, and ratings, provide valuable data that can inform future recommendations. Rapid Innovation emphasizes the importance of user interaction data in shaping effective recommendation strategies for our clients.
- Explicit feedback, like user ratings, and implicit feedback, such as browsing behavior, both play a role in understanding user preferences. We design systems that effectively capture and analyze both types of feedback, ensuring a comprehensive understanding of user needs.
- Incorporating feedback loops allows systems to adjust recommendations based on user satisfaction, leading to a more personalized experience. Our approach to feedback loop integration ensures that clients can continuously refine their offerings based on real-time user input.
- A well-designed feedback loop can help identify trends and patterns, enabling proactive adjustments to the recommendation algorithms. Rapid Innovation assists organizations in establishing robust feedback mechanisms that drive ongoing improvements in their systems.
- Regularly updating models based on feedback ensures that they remain aligned with user expectations and preferences. Our commitment to model updates guarantees that clients can maintain relevance in a fast-paced digital landscape.
- Organizations that effectively integrate feedback loops can foster greater user engagement and loyalty, as users feel their preferences are being acknowledged and catered to. By partnering with Rapid Innovation, businesses can enhance user satisfaction and drive long-term loyalty through effective feedback integration, similar to the practices seen in leading recommendation systems.
5. Data Sources and Integration
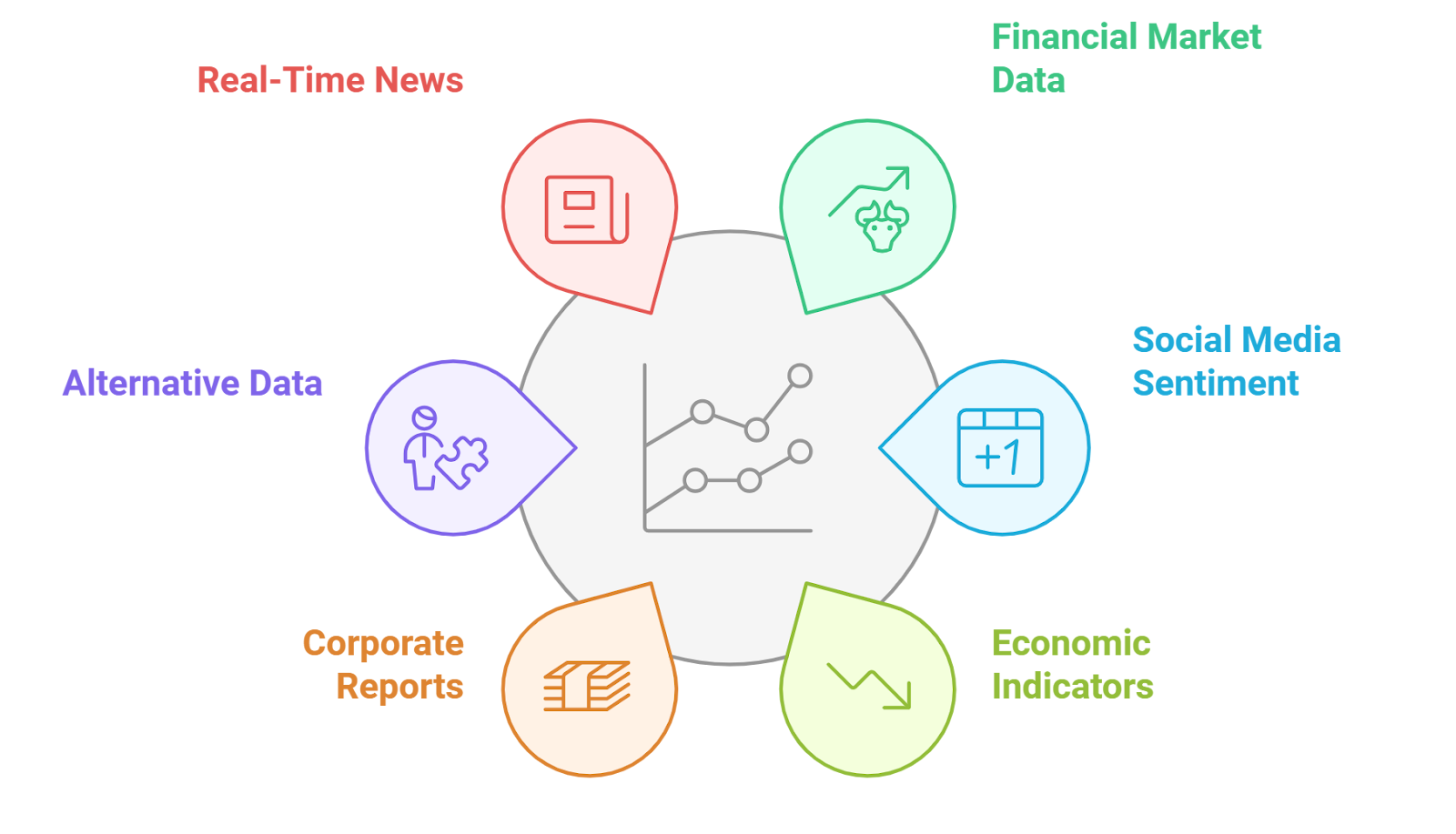
‍
Data sources and integration are crucial components in the realm of data analytics, especially when it comes to making informed decisions in various sectors such as finance, marketing, and social research. The ability to gather, analyze, and integrate diverse data sources can significantly enhance the quality of insights derived from the data, particularly in data integration for financial analysis.
5.1. Financial Market Data
Financial market data refers to the information related to the trading of financial instruments such as stocks, bonds, commodities, and currencies. This data is essential for investors, analysts, and financial institutions to make informed decisions.
- Types of financial market data include: Â
- Price Data: Historical and real-time prices of financial instruments.
- Volume Data: The number of shares or contracts traded during a specific period.
- Market Capitalization: The total market value of a company's outstanding shares.
- Economic Indicators: Data such as GDP growth rates, unemployment rates, and inflation rates that influence market conditions.
- Sources of financial market data: Â
- Stock Exchanges: Organizations like the New York Stock Exchange (NYSE) and NASDAQ provide real-time trading data.
- Financial News Services: Bloomberg and Reuters offer comprehensive market data and analysis.
- Data Aggregators: Platforms like Yahoo Finance and Google Finance compile data from various sources for easy access.
- Importance of financial market data: Â
- Investment Decisions: Investors rely on accurate data to assess the performance of assets and make buy/sell decisions.
- Risk Management: Financial institutions use data to evaluate risks and develop strategies to mitigate them.
- Market Trends: Analyzing historical data helps identify trends and forecast future market movements.
Integrating financial market data with other data sources can provide a more holistic view of market dynamics. For instance, combining price data with economic indicators can help analysts understand the broader economic context affecting market performance. At Rapid Innovation, we leverage advanced AI algorithms to automate the integration of these data sources, enabling clients to make timely and informed investment decisions that drive greater ROI.
5.2. Social Media Sentiment Analysis
Social media sentiment analysis involves evaluating and interpreting the emotions expressed in social media content. This analysis is increasingly important for businesses and organizations looking to understand public opinion and consumer behavior.
- Key components of social media sentiment analysis: Â
- Text Mining: Extracting relevant information from social media posts, comments, and reviews.
- Natural Language Processing (NLP): Utilizing algorithms to analyze and interpret human language in social media content.
- Sentiment Scoring: Assigning a score to posts based on the sentiment expressed, categorizing them as positive, negative, or neutral.
- Sources for social media sentiment analysis: Â
- Social Media Platforms: Twitter, Facebook, Instagram, and LinkedIn are rich sources of user-generated content.
- Review Sites: Websites like Yelp and TripAdvisor provide valuable insights into consumer opinions and experiences.
- News Articles and Blogs: Online articles and blogs can also be analyzed for sentiment regarding brands or products.
- Benefits of social media sentiment analysis: Â
- Brand Monitoring: Companies can track public perception of their brand and respond to customer feedback in real-time.
- Market Research: Understanding consumer sentiment helps businesses tailor their products and marketing strategies.
- Crisis Management: Rapid sentiment analysis can help organizations identify and address potential PR crises before they escalate.
Integrating social media sentiment analysis with financial market data can provide unique insights. For example, a surge in positive sentiment about a company on social media may correlate with an increase in its stock price, offering valuable information for investors. Rapid Innovation employs cutting-edge AI techniques to analyze sentiment data, allowing clients to align their marketing strategies with real-time consumer perceptions, ultimately enhancing their market positioning and ROI.
In conclusion, leveraging diverse data sources such as financial market data and social media sentiment analysis can significantly enhance decision-making processes across various sectors. By integrating these data types, organizations can gain a comprehensive understanding of market trends and consumer behavior, ultimately leading to more informed strategies and actions. Rapid Innovation is committed to helping clients harness the power of data integration for financial analysis to achieve their business goals efficiently and effectively.
5.3. Economic Indicators
Economic indicators are vital statistics that provide insights into the overall health of an economy. They help investors, policymakers, and businesses make informed decisions. Key economic indicators include:
- Gross Domestic Product (GDP): Measures the total value of goods and services produced in a country. A growing GDP indicates a healthy economy, while a declining GDP may signal economic trouble. This includes metrics such as domestic GDP and GDP gross.
- Unemployment Rate: Represents the percentage of the labor force that is unemployed and actively seeking employment. A high unemployment rate can indicate economic distress, while a low rate suggests a robust job market.
- Inflation Rate: Tracks the rate at which the general level of prices for goods and services rises, eroding purchasing power. Moderate inflation is normal, but hyperinflation can destabilize an economy.
- Consumer Confidence Index (CCI): Gauges how optimistic or pessimistic consumers are regarding their expected financial situation. High consumer confidence typically leads to increased spending, driving economic growth. Related metrics include the consumer confidence indicator and consumer confidence.
- Interest Rates: Set by central banks, these rates influence borrowing costs. Lower interest rates can stimulate economic activity, while higher rates may slow it down.
- Genuine Progress Indicator: This alternative measure assesses the economic well-being of a nation, taking into account factors such as environmental health and social equity.
- Growth National Product: Similar to GDP, this indicator measures the economic performance of a country, focusing on the growth aspect.
Understanding these indicators can help stakeholders anticipate economic trends and adjust their strategies accordingly. At Rapid Innovation, we leverage AI and blockchain technologies to analyze these economic indicators, enabling our clients to make data-driven decisions that align with market conditions and enhance their ROI and explore concepts like AI agent societies and simulations.
5.4. Corporate Financial Reports
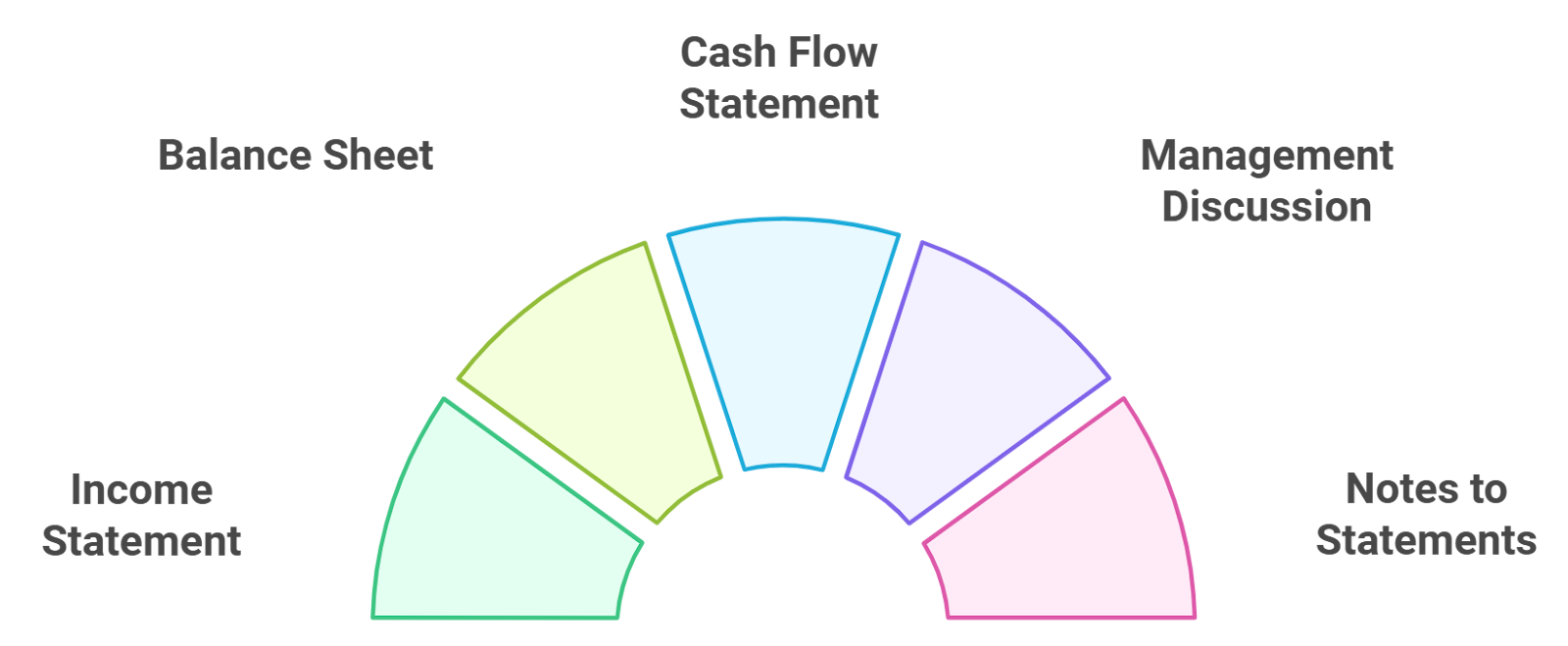
‍
Corporate financial reports are essential documents that provide a comprehensive overview of a company's financial performance and position. These reports are crucial for investors, analysts, and stakeholders to assess a company's health. Key components of corporate financial reports include:
- Income Statement: Shows the company's revenues, expenses, and profits over a specific period. It helps stakeholders understand how well the company generates profit from its operations.
- Balance Sheet: Provides a snapshot of a company's assets, liabilities, and equity at a specific point in time. It helps assess the company's financial stability and liquidity.
- Cash Flow Statement: Details the inflow and outflow of cash within the company. It highlights how well the company manages its cash to fund operations and growth.
- Management Discussion and Analysis (MD&A): Offers insights from management regarding the financial results, including challenges faced and future outlook. This section provides qualitative context to the quantitative data.
- Notes to Financial Statements: These notes provide additional details and context about the figures presented in the financial statements, including accounting policies and potential risks.
Regularly reviewing corporate financial reports can help investors make informed decisions about buying, holding, or selling stocks. Rapid Innovation assists clients in automating the analysis of these reports using AI, allowing for quicker insights and improved decision-making processes.
5.5. Alternative Data Sources
Alternative data sources are non-traditional data sets that can provide valuable insights into market trends and consumer behavior. These data sources are increasingly used by investors and analysts to gain a competitive edge. Key types of alternative data include:
- Social Media Sentiment: Analyzing social media platforms can reveal public sentiment about brands, products, or market trends. This data can help predict stock movements based on consumer opinions.
- Web Traffic Analytics: Monitoring website traffic and engagement metrics can provide insights into a company's performance and consumer interest. Increased web traffic often correlates with higher sales.
- Satellite Imagery: Companies use satellite images to assess economic activity, such as monitoring retail parking lots or agricultural yields. This data can provide real-time insights into supply and demand.
- Credit Card Transaction Data: Analyzing anonymized credit card transactions can help gauge consumer spending patterns and trends, offering a glimpse into a company's sales performance before official reports are released.
- Job Listings and Hiring Trends: Tracking job postings can indicate a company's growth trajectory and hiring intentions, which can be a precursor to future performance.
Utilizing alternative data sources can enhance traditional analysis methods, providing a more comprehensive view of market dynamics. Rapid Innovation employs advanced AI algorithms to process and analyze these alternative data sources, enabling clients to uncover actionable insights that drive strategic decisions and maximize ROI.
5.6. Real-Time News Integration
Real-time news integration is a crucial aspect of modern trading and investment strategies. It involves the immediate incorporation of news events into trading decisions, allowing investors to react swiftly to market-moving information.
- Enhances decision-making: By accessing real-time news integration, traders can make informed decisions based on the latest developments in the market. Rapid Innovation leverages AI algorithms to filter and analyze news, providing clients with actionable insights that enhance their trading strategies.
- Reduces information lag: Traditional methods of news dissemination can lead to delays, but real-time news integration ensures that traders receive updates as they happen. Our solutions utilize blockchain technology to ensure the integrity and timeliness of news data, minimizing the risk of misinformation.
- Improves market responsiveness: Investors can capitalize on short-term price movements triggered by breaking news, such as earnings reports, geopolitical events, or economic indicators. Rapid Innovation's AI-driven analytics can predict market reactions to news events, allowing clients to position themselves advantageously.
- Utilizes technology: Many trading platforms now offer integrated news feeds, allowing users to monitor relevant news alongside their trading activities. Our development team can create customized platforms that seamlessly integrate real-time news integration tailored to specific trading strategies.
- Supports algorithmic trading: Automated trading systems can be programmed to react to specific news events, executing trades based on predefined criteria. Rapid Innovation specializes in developing sophisticated algorithmic trading systems that utilize real-time news data to optimize trading performance.
Incorporating real-time news integration into trading strategies can significantly enhance performance and profitability. By staying informed, traders can better navigate the complexities of the financial markets. For those interested in expanding their capabilities, our security token development services can provide valuable support.
6. Investment Strategy Methodologies
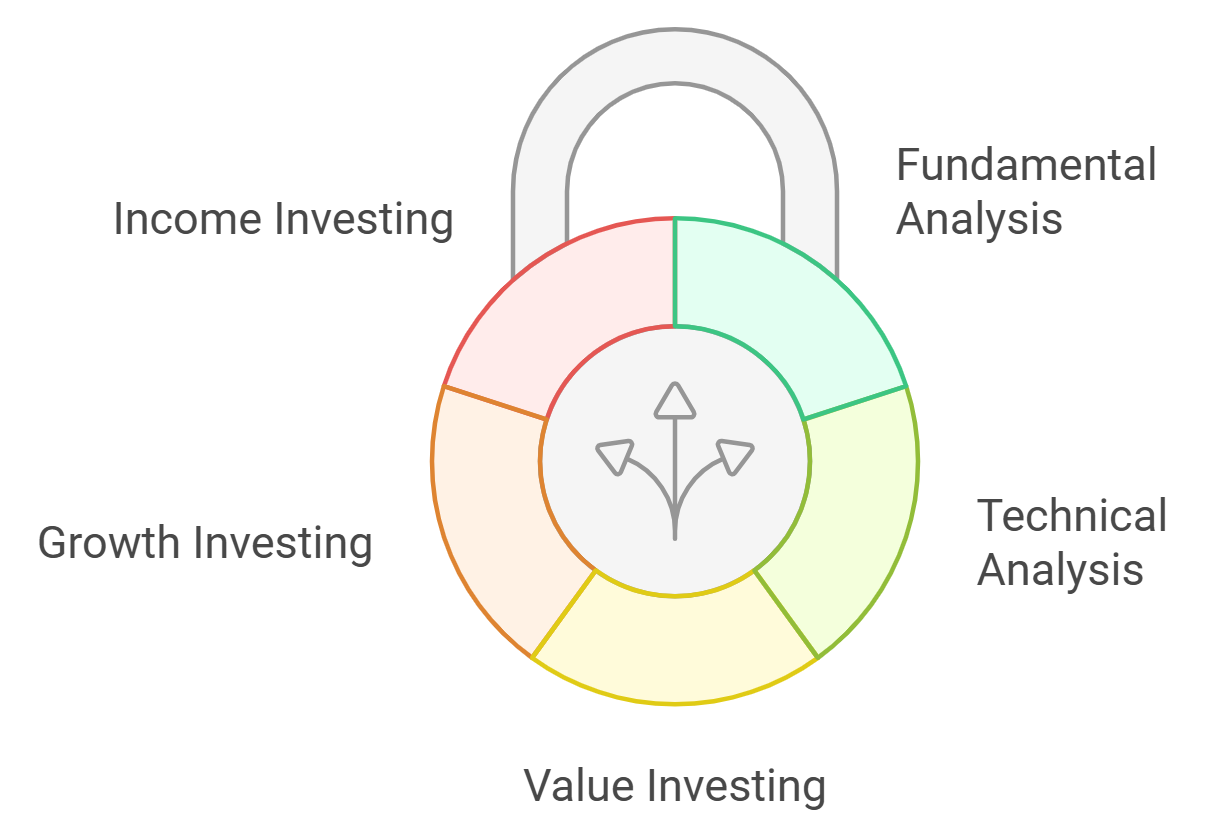
‍
Investment strategy methodologies refer to the various approaches investors use to allocate their capital and manage their portfolios. These methodologies can vary widely, depending on the investor's goals, risk tolerance, and market outlook.
- Fundamental analysis: This approach involves evaluating a company's financial health, industry position, and economic factors to determine its intrinsic value. Rapid Innovation can assist clients in developing AI models that automate fundamental analysis, providing deeper insights and faster evaluations.
- Technical analysis: Investors using this methodology analyze price charts and trading volumes to identify patterns and trends that can inform their trading decisions. Our blockchain solutions can enhance the transparency and security of technical analysis data, ensuring that clients have access to reliable information.
- Value investing: This strategy focuses on identifying undervalued stocks that have the potential for long-term growth. Rapid Innovation's AI tools can help clients identify these opportunities by analyzing vast datasets to uncover hidden value.
- Growth investing: Investors look for companies with strong earnings growth potential, often willing to pay a premium for stocks they believe will appreciate significantly. Our consulting services can guide clients in developing growth-focused investment strategies backed by data-driven insights.
- Income investing: This methodology emphasizes generating regular income through dividends or interest payments, often appealing to retirees or conservative investors. Rapid Innovation can help clients create portfolios that balance income generation with growth potential.
Each investment strategy methodology has its strengths and weaknesses, and investors often combine multiple approaches to create a diversified portfolio that aligns with their financial objectives.
6.1. Quantitative Analysis
Quantitative analysis is a systematic approach to evaluating investments using mathematical and statistical models. This methodology relies on numerical data to identify trends, assess risk, and make informed investment decisions.
- Data-driven insights: Quantitative analysis uses historical data to identify patterns and correlations that can inform future performance. Rapid Innovation employs advanced AI techniques to enhance data analysis, providing clients with deeper insights into market trends.
- Risk assessment: By employing statistical models, investors can quantify the risk associated with specific investments, helping them make more informed decisions. Our blockchain solutions ensure that risk assessment data is secure and immutable, enhancing trust in the analysis.
- Algorithmic trading: Many quantitative analysts develop algorithms that execute trades based on predefined criteria, allowing for faster and more efficient trading. Rapid Innovation specializes in creating custom algorithmic trading solutions that leverage real-time data for optimal execution.
- Backtesting: This process involves testing investment strategies against historical data to evaluate their effectiveness before implementation. Our AI-driven backtesting tools allow clients to simulate various strategies and refine their approaches based on empirical evidence.
- Portfolio optimization: Quantitative analysis can help investors construct portfolios that maximize returns while minimizing risk through diversification. Rapid Innovation's expertise in AI and blockchain enables us to develop sophisticated portfolio optimization models tailored to client needs.
Quantitative analysis is increasingly popular among institutional investors and hedge funds, as it allows for a more objective and systematic approach to investment decision-making. By leveraging data and technology, investors can enhance their strategies and improve overall performance. Rapid Innovation is committed to helping clients achieve greater ROI through innovative solutions in AI and blockchain.
6.2. Fundamental Investment Approaches
Fundamental investment approaches focus on analyzing a company's financial health and overall economic conditions to make informed investment decisions. This method involves evaluating various factors that can influence a company's value, including:
- Financial Statements: Investors examine balance sheets, income statements, and cash flow statements to assess profitability, debt levels, and revenue growth. At Rapid Innovation, we leverage AI-driven analytics to automate the analysis of these financial documents, providing clients with real-time insights that enhance decision-making and improve ROI.
- Valuation Ratios: Key ratios such as Price-to-Earnings (P/E), Price-to-Book (P/B), and Dividend Yield help investors determine if a stock is undervalued or overvalued. Our blockchain solutions can ensure the integrity and transparency of these financial metrics, fostering trust among stakeholders.
- Economic Indicators: Macroeconomic factors like GDP growth, unemployment rates, and inflation can impact market performance and investment strategies. By utilizing AI algorithms, we can predict economic trends and their potential impact on investments, allowing clients to adjust their strategies proactively.
- Industry Analysis: Understanding the competitive landscape and market trends within specific sectors can provide insights into potential investment opportunities. Our AI tools can analyze vast amounts of industry data, identifying emerging trends and opportunities that clients can capitalize on, such as micro strategy stock and alternative investing options.
- Management Quality: Evaluating the experience and track record of a company's management team can influence investment decisions, as strong leadership often correlates with better performance. We assist clients in assessing management quality through data-driven insights, enhancing their investment strategies.
Fundamental analysis is particularly useful for long-term investors who seek to identify stocks that are likely to appreciate over time based on their intrinsic value, including strategies like dividend investing and value investing.
6.3. Technical Analysis
Technical analysis is a method used to evaluate securities by analyzing statistics generated by market activity, such as past prices and volume. This approach is based on the belief that historical price movements can help predict future price behavior. Key components of technical analysis include:
- Charts and Patterns: Investors use various chart types (line, bar, candlestick) to visualize price movements and identify patterns that may indicate future trends. Our AI-powered charting tools can enhance pattern recognition, providing clients with actionable insights, especially in day trading stocks.
- Indicators and Oscillators: Tools like Moving Averages, Relative Strength Index (RSI), and Bollinger Bands help traders assess market momentum and potential reversal points. Rapid Innovation's AI algorithms can optimize the use of these indicators, improving the accuracy of trading signals, which is crucial for option trading for beginners.
- Support and Resistance Levels: Identifying price levels where a stock tends to stop and reverse can help traders make informed entry and exit decisions. Our advanced analytics can automate the identification of these levels, streamlining the trading process for clients engaged in short trading stocks.
- Volume Analysis: Examining trading volume alongside price movements can provide insights into the strength of a trend, as higher volume often indicates stronger conviction among traders. We utilize AI to analyze volume trends, helping clients make more informed trading decisions, particularly in the context of crypto arbitrage strategies.
- Market Sentiment: Understanding the overall mood of the market can help traders gauge potential price movements, as fear and greed often drive market behavior. Our sentiment analysis tools leverage AI to assess market sentiment, providing clients with a competitive edge in their investment strategies.
Technical analysis is favored by short-term traders and those looking to capitalize on market fluctuations, including strategies like dollar cost averaging and tax loss harvesting.
6.4. Hybrid Investment Strategies

‍
Hybrid investment strategies combine elements of both fundamental and technical analysis to create a more comprehensive approach to investing. This method allows investors to leverage the strengths of both strategies while mitigating their weaknesses. Key aspects of hybrid investment strategies include:
- Balanced Approach: Investors can use fundamental analysis to identify undervalued stocks and technical analysis to determine optimal entry and exit points. Rapid Innovation's integrated solutions enable clients to seamlessly combine these approaches for enhanced investment outcomes, including strategies like equity investment strategies and investment ideas.
- Risk Management: By incorporating both strategies, investors can better manage risk, as fundamental analysis provides a long-term perspective while technical analysis offers short-term insights. Our AI-driven risk assessment tools help clients identify and mitigate potential risks effectively, particularly in the context of shorting a stock.
- Diversification: Hybrid strategies can lead to a more diversified portfolio, as investors can select a mix of assets based on both fundamental value and technical trends. We assist clients in creating diversified portfolios that align with their investment goals, including options like cd ladders and bito stock price.
- Flexibility: Investors can adapt their strategies based on market conditions, switching between fundamental and technical analysis as needed. Our blockchain solutions provide real-time data access, allowing clients to make timely adjustments to their strategies.
- Enhanced Decision-Making: Combining both approaches can lead to more informed investment decisions, as investors can consider a wider range of factors before making trades. Rapid Innovation empowers clients with AI-driven insights that enhance their decision-making processes, making it easier to implement strategies like arbitrage strategy and investment strategies.
Hybrid investment strategies appeal to a broad range of investors, from those seeking long-term growth to those looking for short-term gains.
6.5. Risk-Adjusted Recommendations
Risk-adjusted recommendations are essential for investors seeking to optimize their portfolios while managing potential risks. These recommendations take into account the volatility and uncertainty associated with various investment options, allowing for a more informed decision-making process.
- Risk assessment involves evaluating the potential downsides of an investment, including market fluctuations and economic changes.
- Tools such as the Sharpe Ratio and Sortino Ratio help investors understand the return per unit of risk taken.
- Diversification strategies can mitigate risks by spreading investments across different asset classes, sectors, or geographical regions.
- Incorporating historical data and predictive analytics can enhance the accuracy of risk assessments.
- Regularly updating risk profiles based on market conditions ensures that recommendations remain relevant and effective.
By focusing on risk-adjusted returns, investors can align their strategies with their risk tolerance and investment goals, leading to more sustainable financial growth. At Rapid Innovation, we leverage our expertise in AI and data analytics to provide tailored risk-adjusted investment recommendations that help our clients achieve greater ROI while effectively managing their investment risks. For those interested in real estate, our AI real estate solutions can further enhance investment strategies. Additionally, insights on generative AI in asset management can provide further context on the evolving landscape of investment strategies.
6.6. Multi-Dimensional Investment Modeling
Multi-dimensional investment modeling is a sophisticated approach that allows investors to analyze various factors influencing investment performance. This method goes beyond traditional models by incorporating multiple variables and scenarios.
- It considers economic indicators, market trends, and individual asset performance to create a comprehensive view of potential outcomes.
- Scenario analysis helps investors understand how different conditions, such as interest rate changes or geopolitical events, can impact their portfolios.
- Sensitivity analysis identifies which variables have the most significant effect on investment returns, enabling better risk management.
- Visualization tools can present complex data in an easily digestible format, aiding in decision-making.
- This modeling approach supports strategic asset allocation by providing insights into how different investments interact with one another.
By utilizing multi-dimensional investment modeling, investors can make more informed decisions, ultimately leading to improved portfolio performance. Rapid Innovation employs advanced modeling techniques to assist clients in navigating complex investment landscapes, ensuring they are well-equipped to optimize their asset allocations.
7. Advanced AI Capabilities

‍
Advanced AI capabilities are transforming the investment landscape by providing tools that enhance analysis, forecasting, and decision-making processes. These technologies leverage machine learning and data analytics to deliver insights that were previously unattainable.
- Predictive analytics can forecast market trends and asset performance, allowing investors to make proactive decisions.
- Natural language processing (NLP) enables the analysis of news articles, social media, and financial reports to gauge market sentiment.
- Automated trading systems can execute trades based on predefined criteria, increasing efficiency and reducing human error.
- AI-driven risk management tools can identify potential threats and suggest mitigation strategies in real-time.
- Portfolio optimization algorithms can analyze vast amounts of data to recommend the best asset allocation strategies.
By integrating advanced AI capabilities into investment strategies, investors can enhance their ability to navigate complex markets and achieve their financial objectives. At Rapid Innovation, we harness these advanced AI technologies to empower our clients, enabling them to make data-driven decisions that lead to improved investment outcomes.
7.1. Predictive Portfolio Management
Predictive portfolio management leverages advanced analytics and machine learning algorithms to forecast market trends and asset performance. This approach allows investors to make informed decisions based on data-driven insights rather than relying solely on intuition or historical performance.
- Utilizes big data analytics to assess market conditions.
- Employs machine learning models to predict asset price movements.
- Enhances risk management by identifying potential downturns early.
- Integrates various data sources, including economic indicators, social media sentiment, and historical price data.
- Provides real-time insights, enabling quicker decision-making.
By implementing predictive portfolio management, investors can optimize their portfolios for better returns while minimizing risks. This method is particularly beneficial in volatile markets where traditional strategies may fall short. At Rapid Innovation, we harness the power of AI to develop tailored predictive portfolio management models that align with your investment goals, ensuring you stay ahead of market trends. Additionally, our expertise extends to cryptocurrency exchange development, providing innovative solutions for modern investors. For more insights on the intersection of AI and trading, check out our article on the intersection of artificial intelligence with margin trading exchanges.
7.2. Autonomous Trading Strategies
Autonomous trading strategies refer to automated systems that execute trades based on predefined algorithms without human intervention. These strategies are designed to capitalize on market inefficiencies and execute trades at optimal times.
- Operates 24/7, allowing for continuous market engagement.
- Reduces emotional bias in trading decisions.
- Can process vast amounts of data in real-time, identifying trading opportunities faster than human traders.
- Utilizes high-frequency trading (HFT) techniques to exploit small price discrepancies.
- Adapts to changing market conditions through machine learning algorithms.
The rise of autonomous trading strategies has transformed the trading landscape, making it more efficient and accessible. Investors can benefit from these systems by implementing them in their trading practices, allowing for a more systematic approach to investing. Rapid Innovation specializes in developing robust autonomous trading systems that enhance your trading capabilities and maximize your ROI.
7.3. Dynamic Asset Allocation
Dynamic asset allocation is an investment strategy that involves adjusting the proportions of various asset classes in a portfolio based on changing market conditions and economic forecasts. This approach aims to maximize returns while managing risk effectively.
- Allows for flexibility in response to market fluctuations.
- Involves continuous monitoring of economic indicators and market trends.
- Can enhance portfolio performance by reallocating assets to outperforming sectors.
- Reduces exposure to underperforming assets, thereby minimizing losses.
- Often employs quantitative models to guide allocation decisions.
Dynamic asset allocation is particularly useful in uncertain economic environments, where static strategies may not yield optimal results. By actively managing asset allocation, investors can better navigate market volatility and enhance their overall investment outcomes. At Rapid Innovation, we utilize advanced analytics and AI-driven insights to support your dynamic asset allocation strategies, ensuring you make informed decisions that align with your financial objectives.
7.4. Macro-Economic Trend Prediction
Macro-economic trend prediction involves analyzing various economic indicators to forecast future economic conditions. This process is crucial for businesses, investors, and policymakers as it helps them make informed decisions. Key components of macro-economic trend prediction include:
- Economic Indicators: These are statistics that provide insight into the economic performance of a country. Common indicators include GDP growth rates, unemployment rates, inflation rates, and consumer confidence indices.
- Data Analysis: Utilizing historical data and statistical models, analysts can identify patterns and trends. Techniques such as regression analysis and time series forecasting are often employed to predict future economic conditions. At Rapid Innovation, we leverage advanced AI algorithms to enhance the accuracy of these predictions, enabling clients to make data-driven decisions that align with their business goals.
- Global Influences: Economic trends are not isolated; they are influenced by global events. Factors such as international trade agreements, geopolitical tensions, and global market fluctuations can significantly impact local economies. Our blockchain solutions can provide transparent tracking of these global influences, ensuring that businesses remain informed and agile in their strategies.
- Policy Implications: Understanding macro-economic trends allows governments to implement effective fiscal and monetary policies. For instance, if inflation is predicted to rise, central banks may consider increasing interest rates to stabilize the economy. Rapid Innovation can assist policymakers by providing predictive analytics tools that simulate the potential impacts of various policy decisions.
- Investment Strategies: Investors use macro-economic predictions to adjust their portfolios. For example, if a recession is anticipated, investors might shift towards more stable, defensive stocks. Our consulting services can guide clients in developing robust investment strategies that capitalize on macro-economic insights, ultimately leading to greater ROI. Additionally, insights on how AI predicts customer trends and behavior can also play a significant role in shaping investment strategies.
7.5. Behavioral Finance Insights

‍
Behavioral finance explores the psychological factors that influence investors' decisions and market outcomes. It challenges the traditional notion of rational decision-making in finance. Key insights from behavioral finance include:
- Cognitive Biases: Investors often fall prey to cognitive biases, such as overconfidence, anchoring, and loss aversion. These biases can lead to irrational investment decisions, impacting market efficiency. Rapid Innovation employs AI-driven behavioral analysis tools to help clients recognize and mitigate these biases in their investment strategies.
- Market Sentiment: Investor sentiment plays a significant role in market movements. Positive or negative news can lead to herd behavior, where investors collectively buy or sell assets, causing price fluctuations. Our solutions can analyze sentiment data from various sources, providing clients with actionable insights to navigate market volatility.
- Emotional Factors: Emotions like fear and greed can drive market trends. For instance, during market downturns, fear may lead to panic selling, while during bull markets, greed can result in excessive risk-taking. By integrating AI with behavioral finance principles, we help clients develop strategies that account for emotional influences, leading to more rational decision-making.
- Framing Effects: The way information is presented can influence decision-making. For example, investors may react differently to a 10% loss versus a 10% gain, even if the underlying situation is the same. Our consulting services can assist clients in effectively framing their investment narratives to enhance decision-making processes.
- Long-Term Implications: Understanding behavioral finance can help investors develop strategies to mitigate biases, leading to more rational decision-making and improved investment outcomes. Rapid Innovation's expertise in AI can facilitate the development of personalized investment strategies that align with individual investor profiles.
7.6. Scenario Simulation and Stress Testing
Scenario simulation and stress testing are essential tools for assessing the resilience of financial systems and institutions under various conditions. These methods help identify vulnerabilities and prepare for potential economic shocks. Key aspects include:
- Scenario Analysis: This involves creating hypothetical scenarios to evaluate how different factors could impact financial performance. Scenarios may include economic downturns, interest rate changes, or geopolitical events. Rapid Innovation utilizes AI to simulate a wide range of scenarios, providing clients with comprehensive insights into potential outcomes.
- Stress Testing: Financial institutions conduct stress tests to evaluate their ability to withstand adverse conditions. This process assesses the impact of extreme but plausible events on capital adequacy and liquidity. Our blockchain solutions can enhance the transparency and reliability of stress testing processes, ensuring that clients meet regulatory requirements effectively.
- Regulatory Requirements: Many regulatory bodies require banks and financial institutions to perform regular stress tests. These tests ensure that institutions maintain sufficient capital buffers to absorb losses during economic crises. Rapid Innovation offers consulting services to help clients navigate these regulatory landscapes with confidence.
- Risk Management: Scenario simulation and stress testing are integral to risk management frameworks. By understanding potential risks, organizations can develop strategies to mitigate them and enhance their overall stability. Our AI-driven risk assessment tools empower clients to proactively manage risks and optimize their operational resilience.
- Decision-Making: The insights gained from these analyses inform strategic decision-making. Organizations can adjust their business models, investment strategies, and operational practices based on the outcomes of scenario simulations and stress tests. Rapid Innovation's expertise ensures that clients are equipped with the necessary tools and insights to make informed decisions that drive growth and profitability.
8. Implementation Strategies
Implementation strategies are crucial for the successful deployment of any project, especially in technology-driven environments. These strategies ensure that the project aligns with organizational goals and operates efficiently. Two key components of implementation strategies are technology infrastructure and data preparation and normalization.
8.1 Technology Infrastructure

‍
Technology infrastructure refers to the foundational technology components that support the deployment and operation of systems and applications. A robust technology infrastructure, including elements like hyperconvergence infrastructure and data center infrastructure management, is essential for ensuring that projects run smoothly and can scale as needed.
- Hardware Components: This includes servers, storage devices, and networking equipment. Choosing the right hardware is vital for performance and reliability, particularly in AI and blockchain applications where processing power and speed are critical.
- Software Platforms: The selection of operating systems, databases, and application frameworks can significantly impact the efficiency of the project. Open-source solutions can offer flexibility, while proprietary software may provide better support. Rapid Innovation leverages cutting-edge software platforms tailored to AI and blockchain to enhance project outcomes.
- Cloud Services: Utilizing cloud infrastructure, such as cloud compute platforms, can enhance scalability and reduce costs. Services like AWS, Azure, and Google Cloud provide on-demand resources that can be adjusted based on project needs, allowing clients to optimize their investments and achieve greater ROI.
- Network Security: Implementing robust security measures is essential to protect sensitive data and maintain compliance with regulations. This includes firewalls, encryption, and intrusion detection systems, which are particularly important in blockchain projects where data integrity is paramount.
- Integration Capabilities: Ensuring that different systems can communicate effectively is crucial. APIs and middleware can facilitate seamless integration between various applications and services, enabling clients to maximize the utility of their existing technology stack.
- Monitoring and Maintenance: Establishing a system for ongoing monitoring and maintenance helps identify issues before they escalate. Tools for performance monitoring and automated backups are essential for long-term success, ensuring that AI models and blockchain networks operate optimally.
A well-planned technology infrastructure not only supports current project requirements but also allows for future growth and adaptation, ultimately driving better business outcomes.
8.2 Data Preparation and Normalization
Data preparation and normalization are critical steps in ensuring that data is clean, consistent, and ready for analysis. Proper data handling can significantly enhance the quality of insights derived from the data.
The process begins with Data Collection, which involves gathering data from various sources, including databases, APIs, and user-generated content. Ensuring that data is collected in a structured manner is essential for later stages. Next, Data Cleaning is performed to identify and correct errors or inconsistencies in the data, addressing common issues such as duplicate entries, missing values, and incorrect formats. Tools like OpenRefine can assist in this process.
Following cleaning, Data Transformation is crucial, as it involves converting data into a suitable format for analysis, which may include changing data types, aggregating data, or creating new calculated fields. Normalization is the next step, where data is organized to reduce redundancy and improve integrity, often by structuring it into tables and defining relationships. Techniques such as Min-Max scaling or Z-score normalization can be applied to ensure that data is on a similar scale.
After preparation, Data Validation ensures that the data meets required standards for accuracy and completeness, which can involve cross-referencing with trusted sources or using automated validation tools. Finally, Documentation is essential for transparency and reproducibility, including noting the sources of data, the cleaning methods used, and any transformations applied.
Effective data preparation and normalization lead to higher-quality data, which in turn results in more reliable analysis and decision-making. Rapid Innovation's expertise in these areas empowers clients to harness the full potential of their data, driving informed decisions and maximizing ROI. This is particularly relevant in the context of information technology infrastructure and its infrastructure, where data integrity and management are paramount. For more insights on this topic, visit the critical role of data quality in AI implementations.
8.3. Model Training Methodologies
Model training methodologies are crucial in developing effective machine learning models. These methodologies dictate how data is processed, how models learn from data, and how they are validated. Here are some key methodologies:
- Supervised Learning: This involves training a model on labeled data, where the input data is paired with the correct output. The model learns to map inputs to outputs, making it suitable for tasks like classification and regression. Rapid Innovation employs supervised learning to help clients enhance customer segmentation and improve targeted marketing strategies, leading to increased conversion rates and ROI.
- Unsupervised Learning: In this approach, the model is trained on data without labels. It identifies patterns and structures within the data, making it useful for clustering and association tasks. By utilizing unsupervised learning, Rapid Innovation assists clients in discovering hidden insights in their data, enabling them to make data-driven decisions that optimize operational efficiency.
- Semi-Supervised Learning: This methodology combines both labeled and unlabeled data. It is particularly useful when acquiring labeled data is expensive or time-consuming, allowing the model to leverage the strengths of both supervised and unsupervised learning. Rapid Innovation can implement semi-supervised learning to maximize the value of existing data, reducing costs while improving model accuracy.
- Reinforcement Learning: Here, an agent learns to make decisions by taking actions in an environment to maximize cumulative rewards. This methodology is widely used in robotics, gaming, and autonomous systems. Rapid Innovation leverages reinforcement learning to develop intelligent systems that adapt and optimize processes in real-time, enhancing productivity and reducing operational costs for clients.
- Transfer Learning: This technique involves taking a pre-trained model and fine-tuning it on a new, but related, task. It significantly reduces training time and improves performance, especially when data is scarce. Rapid Innovation utilizes transfer learning to accelerate project timelines and deliver high-performing models, ensuring clients achieve faster time-to-market and better ROI.
- Cross-Validation: This is a technique used to assess how the results of a statistical analysis will generalize to an independent dataset. It helps in preventing overfitting and ensures that the model performs well on unseen data. By implementing cross-validation, Rapid Innovation ensures that the models developed for clients are robust and reliable, ultimately leading to sustained business success.
In addition to these methodologies, organizations often adopt specific frameworks such as methodology for machine learning projects or machine learning project methodology to guide their development processes. The choice of methodology can also depend on the specific goals of the project, such as whether it focuses on machine learning research methodology or deep learning methodologies. Understanding the methodology of machine learning and deep learning methodology is essential for successful implementation.
8.4. Deployment Approaches
Once a model is trained, the next step is deployment. Deployment approaches determine how the model will be integrated into production environments. Here are some common deployment strategies:
- Batch Deployment: In this approach, predictions are made on a batch of data at scheduled intervals. It is suitable for scenarios where real-time predictions are not critical, such as monthly sales forecasts.
- Real-Time Deployment: This method allows for immediate predictions as data is received. It is essential for applications like fraud detection or recommendation systems, where timely responses are crucial. Rapid Innovation specializes in real-time deployment, enabling clients to respond swiftly to market changes and customer needs, thereby enhancing their competitive edge.
- Cloud Deployment: Deploying models in the cloud offers scalability and flexibility. Cloud platforms provide resources that can be adjusted based on demand, making it easier to manage large-scale applications. Rapid Innovation assists clients in leveraging cloud deployment to ensure their solutions are scalable and cost-effective, maximizing their return on investment.
- On-Premises Deployment: Some organizations prefer to deploy models on their own servers for security and compliance reasons. This approach requires more maintenance but offers greater control over data and infrastructure.
- Edge Deployment: This involves deploying models on edge devices, such as IoT devices or mobile phones. It reduces latency and bandwidth usage, making it ideal for applications that require quick responses in remote locations.
- Containerization: Using technologies like Docker, models can be packaged with their dependencies, ensuring consistency across different environments. This approach simplifies deployment and scaling.
8.5. Performance Monitoring
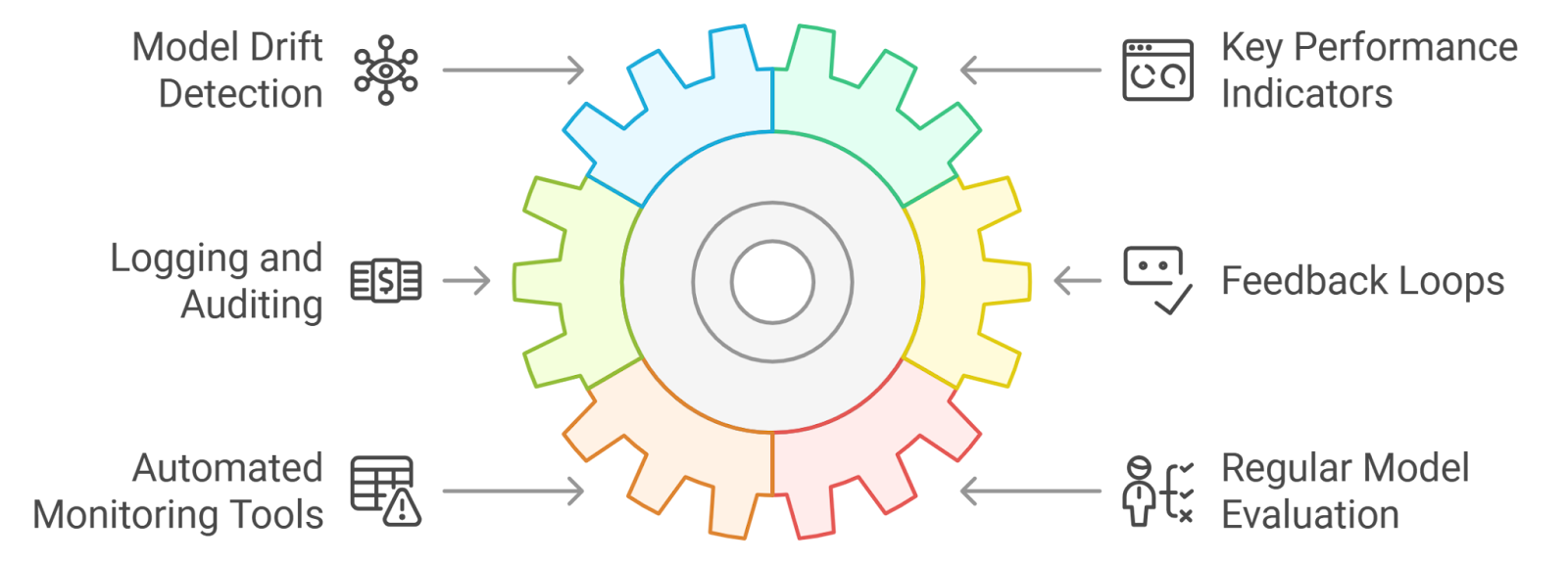
‍
Performance monitoring is essential to ensure that machine learning models continue to perform well after deployment. It involves tracking various metrics and making adjustments as necessary. Key aspects of performance monitoring include:
- Model Drift Detection: Over time, the data distribution may change, leading to model drift. Monitoring tools can detect these changes, allowing for timely retraining or adjustments to the model. Rapid Innovation implements robust monitoring solutions to ensure that clients' models remain accurate and effective over time.
- Key Performance Indicators (KPIs): Establishing KPIs helps in evaluating model performance. Common KPIs include accuracy, precision, recall, F1 score, and AUC-ROC. Regularly reviewing these metrics ensures the model meets business objectives.
- Logging and Auditing: Keeping detailed logs of model predictions and performance metrics is crucial for accountability and troubleshooting. It helps in understanding how the model behaves under different conditions.
- Feedback Loops: Implementing feedback mechanisms allows users to provide input on model predictions. This feedback can be used to improve the model and adapt it to changing requirements.
- Automated Monitoring Tools: Utilizing automated tools can streamline the monitoring process. These tools can alert data scientists and engineers when performance drops below a certain threshold, enabling quick responses.
- Regular Model Evaluation: Periodically re-evaluating the model against a validation dataset helps in assessing its ongoing effectiveness. This practice ensures that the model remains relevant and accurate over time. Rapid Innovation emphasizes continuous performance monitoring to help clients maintain high standards of model efficacy, ultimately driving better business outcomes.
8.6. Continuous Improvement Frameworks
Continuous improvement frameworks are essential for organizations aiming to enhance their processes, products, and services consistently. These frameworks provide structured methodologies that help teams identify areas for improvement, implement changes, and measure the effectiveness of those changes over time.
- Key principles of continuous improvement include: Â
- Customer Focus: Understanding customer needs and expectations is crucial for driving improvements.
- Data-Driven Decision Making: Utilizing data analytics to inform decisions ensures that changes are based on evidence rather than assumptions. At Rapid Innovation, we leverage advanced AI analytics to provide actionable insights that guide your improvement initiatives.
- Employee Involvement: Engaging employees at all levels fosters a culture of improvement and innovation. Our consulting services help organizations create an environment where every team member feels empowered to contribute.
- Incremental Changes: Small, manageable changes are often more effective and less disruptive than large-scale transformations. We assist clients in implementing these changes through tailored AI solutions that streamline processes.
- Popular continuous improvement frameworks include: Â
- Plan-Do-Check-Act (PDCA): A cyclical model that encourages iterative testing and refinement of processes.
- Lean: Focuses on eliminating waste and maximizing value for customers. Our blockchain solutions can enhance transparency and traceability, further supporting Lean initiatives.
- Six Sigma: Aims to reduce defects and variability in processes through statistical analysis. Rapid Innovation employs AI-driven analytics to support Six Sigma methodologies, ensuring data accuracy and process optimization.
- Kaizen: A Japanese term meaning "change for better," emphasizing continuous, incremental improvements.
- Benefits of implementing continuous improvement frameworks: Â
- Enhanced efficiency and productivity.
- Improved quality of products and services.
- Increased employee engagement and morale.
- Greater customer satisfaction and loyalty.
Organizations that adopt continuous improvement frameworks can create a culture of excellence, leading to sustainable growth and competitive advantage. Examples of continuous improvement frameworks include the continuous improvement governance framework and the process improvement framework. The continuous improvement framework steps guide organizations through the process, while the driver framework for continuous improvement helps identify key factors that influence success. Additionally, the itil service improvement plan and itil service improvement plan template provide structured approaches for IT service management, and the process improvement framework example illustrates practical applications of these concepts.
9. Risk Management
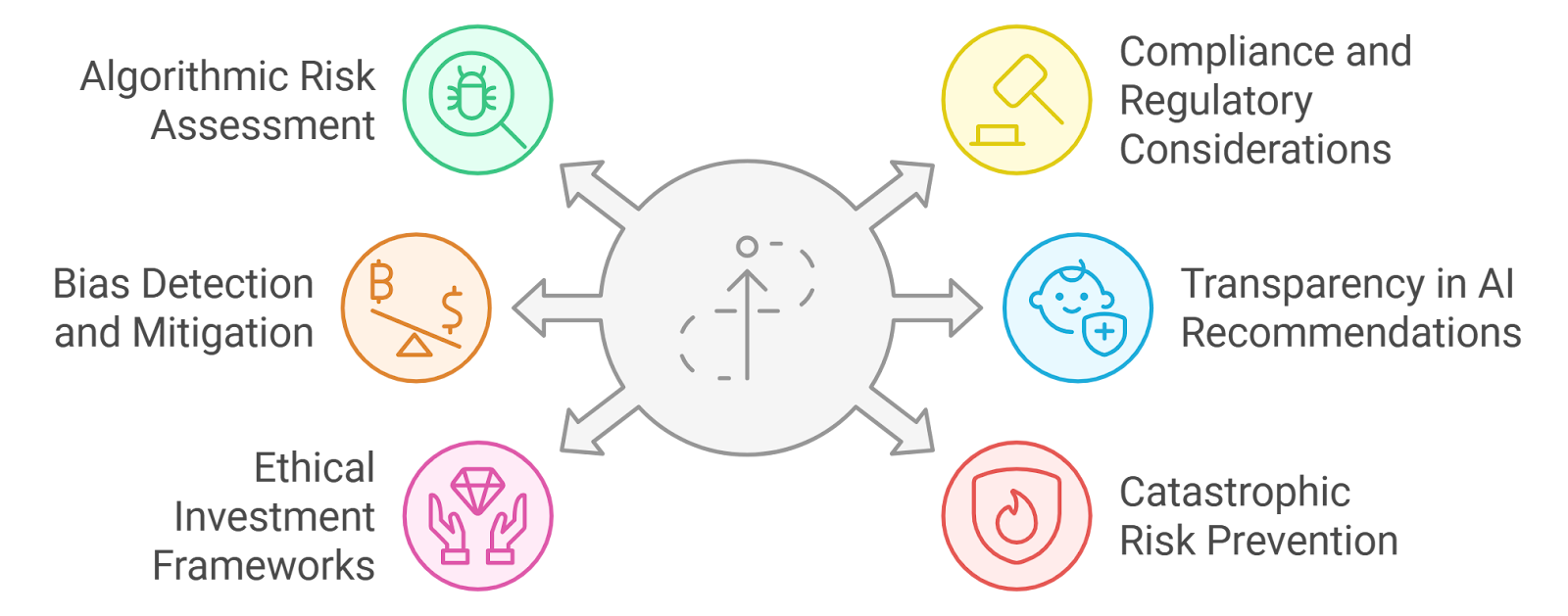
‍
Risk management is a critical component of organizational strategy, focusing on identifying, assessing, and mitigating risks that could impact the achievement of objectives. Effective risk management helps organizations navigate uncertainties and make informed decisions.
- Key elements of risk management include: Â
- Risk Identification: Recognizing potential risks that could affect the organization, including operational, financial, strategic, and compliance risks.
- Risk Assessment: Evaluating the likelihood and impact of identified risks to prioritize them effectively. Our AI solutions can automate this process, providing real-time risk assessments that enhance decision-making.
- Risk Mitigation: Developing strategies to minimize the impact of risks, which may include avoidance, reduction, sharing, or acceptance. Rapid Innovation's blockchain technology can provide secure and transparent methods for risk sharing.
- Monitoring and Review: Continuously tracking risks and the effectiveness of mitigation strategies to adapt to changing circumstances.
- Benefits of a robust risk management framework: Â
- Improved decision-making processes.
- Enhanced organizational resilience.
- Protection of assets and resources.
- Increased stakeholder confidence.
Organizations that prioritize risk management can better anticipate challenges and seize opportunities, ultimately leading to long-term success.
9.1. Algorithmic Risk Assessment
Algorithmic risk assessment involves using algorithms and data analytics to evaluate and quantify risks associated with various activities or decisions. This approach leverages technology to enhance traditional risk management practices, providing more accurate and timely insights.
- Key components of algorithmic risk assessment include: Â
- Data Collection: Gathering relevant data from various sources, including historical data, market trends, and operational metrics. Our AI capabilities enable efficient data collection and integration from diverse sources.
- Model Development: Creating algorithms that can analyze data to identify patterns and predict potential risks. Rapid Innovation specializes in developing custom algorithms tailored to your specific risk landscape.
- Risk Scoring: Assigning scores to different risks based on their likelihood and potential impact, allowing for prioritization.
- Continuous Learning: Algorithms can improve over time by learning from new data and outcomes, enhancing their predictive capabilities.
- Advantages of algorithmic risk assessment: Â
- Increased accuracy in risk evaluation.
- Faster decision-making processes.
- Ability to analyze large volumes of data efficiently.
- Enhanced ability to identify emerging risks.
- Applications of algorithmic risk assessment include: Â
- Financial services for credit scoring and fraud detection.
- Healthcare for patient risk stratification.
- Supply chain management for assessing supplier risks.
By integrating algorithmic risk assessment into their risk management strategies, organizations can gain a competitive edge and make more informed decisions in an increasingly complex environment. Rapid Innovation is here to support you in harnessing these advanced methodologies to achieve greater ROI and drive your business forward.
9.2. Compliance and Regulatory Considerations
Compliance and regulatory considerations are critical in the development and deployment of artificial intelligence (AI) systems. Organizations must navigate a complex landscape of laws and regulations that govern data usage, privacy, and ethical standards. Key aspects include:
- Data Protection Laws: Compliance with regulations such as the General Data Protection Regulation (GDPR) in Europe and the California Consumer Privacy Act (CCPA) in the United States is essential. These laws dictate how personal data should be collected, processed, and stored, ensuring that organizations prioritize user privacy and data security.
- Industry-Specific Regulations: Different industries have specific regulations. For instance, healthcare organizations must comply with the Health Insurance Portability and Accountability Act (HIPAA), which mandates strict data privacy and security measures. Rapid Innovation assists clients in understanding and implementing these regulations to avoid costly penalties and enhance their operational integrity.
- Accountability and Liability: Organizations must establish clear accountability frameworks. This includes defining who is responsible for AI decisions and ensuring that there are mechanisms for redress in case of harm caused by AI systems. Rapid Innovation helps clients create robust governance structures that clarify roles and responsibilities, thereby minimizing legal risks.
- Ethical Guidelines: Many organizations are adopting ethical guidelines for AI development. These guidelines often emphasize fairness, accountability, and transparency, aligning with broader societal values. Rapid Innovation collaborates with clients to develop ethical AI frameworks that resonate with their corporate values and stakeholder expectations, including artificial intelligence regulatory compliance.
- Regular Audits and Assessments: Conducting regular audits of AI systems can help ensure compliance with regulatory requirements and ethical standards. This includes assessing algorithms for bias and effectiveness. Rapid Innovation offers comprehensive audit services that not only ensure compliance but also enhance the overall performance of AI systems, leading to greater ROI. This is particularly important in the context of AI compliance and regulation.
9.3. Bias Detection and Mitigation
Bias in AI systems can lead to unfair outcomes and perpetuate existing inequalities. Therefore, bias detection and mitigation are crucial steps in the AI development process. Key strategies include:
- Diverse Data Sets: Using diverse and representative data sets can help reduce bias. This involves ensuring that the data reflects various demographics and does not favor one group over another. Rapid Innovation emphasizes the importance of data diversity in its AI solutions, ensuring equitable outcomes for all users.
- Algorithmic Audits: Regularly auditing algorithms for bias is essential. This can involve testing AI systems against different demographic groups to identify any disparities in outcomes. Rapid Innovation provides clients with tailored audit services that identify and rectify biases, enhancing the fairness of AI applications.
- Bias Mitigation Techniques: Implementing techniques such as re-weighting, data augmentation, or adversarial debiasing can help mitigate bias in AI models. These methods aim to adjust the training process to minimize biased outcomes. Rapid Innovation employs state-of-the-art techniques to ensure that AI models are not only effective but also equitable.
- Stakeholder Engagement: Engaging with diverse stakeholders, including affected communities, can provide valuable insights into potential biases and their impacts. This collaborative approach can lead to more equitable AI solutions. Rapid Innovation facilitates stakeholder engagement sessions to gather insights that inform the development of fair AI systems.
- Continuous Monitoring: Bias detection should not be a one-time effort. Continuous monitoring of AI systems in real-world applications is necessary to identify and address any emerging biases over time. Rapid Innovation implements ongoing monitoring solutions that help clients maintain the integrity of their AI systems.
9.4. Transparency in AI Recommendations
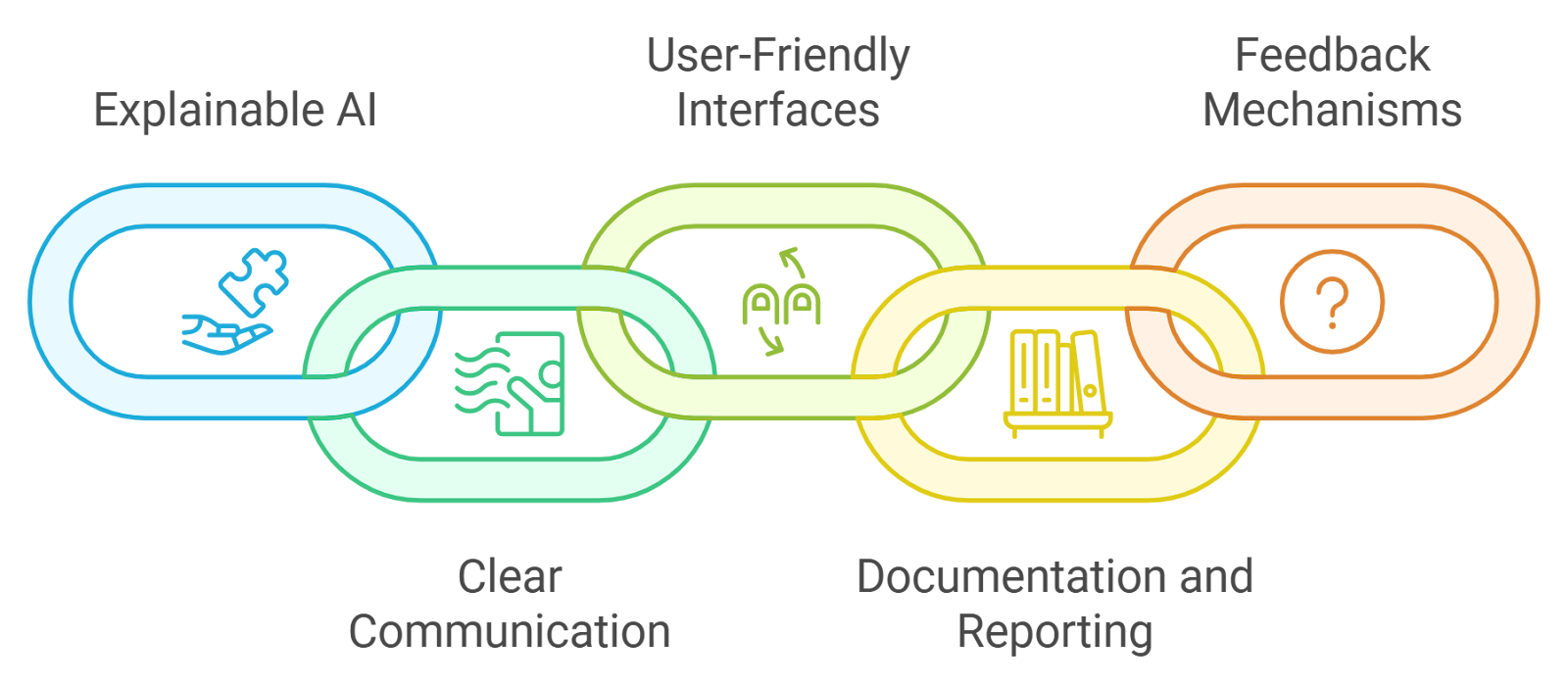
‍
Transparency in AI recommendations is vital for building trust and ensuring accountability. Users need to understand how AI systems arrive at their conclusions. Key elements include:
- Explainable AI (XAI): Developing explainable AI models allows users to comprehend the reasoning behind AI decisions. This can involve using techniques that provide insights into how inputs are transformed into outputs. Rapid Innovation specializes in creating XAI solutions that enhance user understanding and trust.
- Clear Communication: Organizations should communicate the capabilities and limitations of AI systems clearly. This includes informing users about the data used, the algorithms applied, and the potential biases involved. Rapid Innovation assists clients in crafting clear communication strategies that foster transparency and user confidence.
- User-Friendly Interfaces: Designing user interfaces that present AI recommendations in an understandable manner can enhance transparency. Visualizations and simplified explanations can help users grasp complex AI processes. Rapid Innovation focuses on creating intuitive interfaces that make AI insights accessible to all users.
- Documentation and Reporting: Maintaining thorough documentation of AI systems, including their design, data sources, and decision-making processes, is essential. This documentation can serve as a reference for audits and regulatory compliance. Rapid Innovation provides comprehensive documentation services that support clients in meeting regulatory requirements.
- Feedback Mechanisms: Implementing feedback mechanisms allows users to report issues or concerns regarding AI recommendations. This can help organizations identify areas for improvement and enhance the overall transparency of their AI systems. Rapid Innovation encourages the establishment of feedback loops that drive continuous improvement in AI solutions.
9.5. Ethical Investment Frameworks
Ethical investment frameworks are essential for guiding investors toward making socially responsible choices. These frameworks help align investment strategies with personal values and societal goals, focusing on various criteria, including environmental sustainability, social justice, and corporate governance.
- Definition: Ethical investment involves selecting investments based on ethical guidelines, often excluding industries like tobacco, firearms, and fossil fuels.
- Types of Ethical Investments: Â
- Socially Responsible Investing (SRI): This approach considers both financial return and social/environmental good.
- Environmental, Social, and Governance (ESG): ESG criteria evaluate a company's operations and impact on the environment and society.
- Impact Investing: This strategy aims to generate measurable social or environmental impact alongside financial returns.
- Benefits: Â
- Aligns investments with personal values.
- Potential for long-term financial performance.
- Contributes to positive societal change.
- Challenges: Â
- Limited investment options in certain sectors.
- Difficulty in measuring impact and performance.
- Potential for lower short-term returns compared to traditional investments.
Investors can utilize various resources to identify ethical investment opportunities, such as ESG ratings and ethical investment funds. The growing demand for ethical investments has led to the development of numerous indices and funds that focus on sustainable practices. For more insights on the ethical considerations in investment, you can refer to ethics and privacy in AI app development.
9.6. Catastrophic Risk Prevention
Catastrophic risk prevention involves strategies and measures designed to mitigate the impact of extreme events that could lead to significant losses. These risks can stem from natural disasters, technological failures, or geopolitical tensions.
- Understanding Catastrophic Risks: Â
- Defined as low-probability but high-impact events.
- Examples include earthquakes, pandemics, and cyber-attacks.
- Prevention Strategies: Â
- Risk Assessment: Identifying potential risks and their impacts on assets and operations.
- Insurance Solutions: Utilizing insurance products specifically designed for catastrophic events.
- Crisis Management Plans: Developing comprehensive plans to respond to and recover from catastrophic events.
- Importance of Preparedness: Â
- Reduces potential financial losses.
- Enhances organizational resilience.
- Protects stakeholders and communities.
- Technological Innovations: Â
- Use of predictive analytics to forecast risks.
- Implementation of advanced monitoring systems for early detection.
- Development of robust infrastructure to withstand disasters.
Organizations and governments are increasingly recognizing the importance of catastrophic risk prevention. By investing in risk management strategies, they can safeguard their assets and ensure continuity in the face of unforeseen challenges.
10. Use Cases and Scenarios
Use cases and scenarios are practical applications of concepts, technologies, or strategies in real-world situations. They help illustrate the potential benefits and challenges of implementing specific solutions.
- Importance of Use Cases: Â
- Provide concrete examples of how theories or technologies can be applied.
- Help stakeholders understand the practical implications of decisions.
- Facilitate better planning and strategy development.
- Types of Use Cases: Â
- Business Applications: Demonstrating how a new technology can improve efficiency or reduce costs.
- Social Impact: Showcasing initiatives that address social issues, such as poverty or education.
- Environmental Solutions: Highlighting projects that promote sustainability and conservation.
- Scenario Planning: Â
- Involves creating detailed narratives about potential future events.
- Helps organizations prepare for various outcomes and uncertainties.
- Encourages proactive thinking and strategic flexibility.
- Benefits of Scenarios: Â
- Enhances decision-making by considering multiple perspectives.
- Identifies risks and opportunities in different contexts.
- Fosters innovation by exploring unconventional ideas.
By analyzing use cases and scenarios, organizations can better understand the implications of their choices and develop strategies that are both effective and sustainable. This approach not only aids in risk management but also promotes a culture of continuous improvement and adaptability.
At Rapid Innovation, we leverage our expertise in AI and Blockchain to enhance ethical investment frameworks and catastrophic risk prevention strategies. By integrating advanced analytics and decentralized technologies, we help clients achieve greater ROI while aligning their investments with ethical standards and mitigating potential risks. Our tailored solutions empower organizations to navigate complex landscapes, ensuring they remain resilient and socially responsible in their investment choices. For more information on our services, check out our AI insurance solutions.
10.1. Retail Investor Solutions
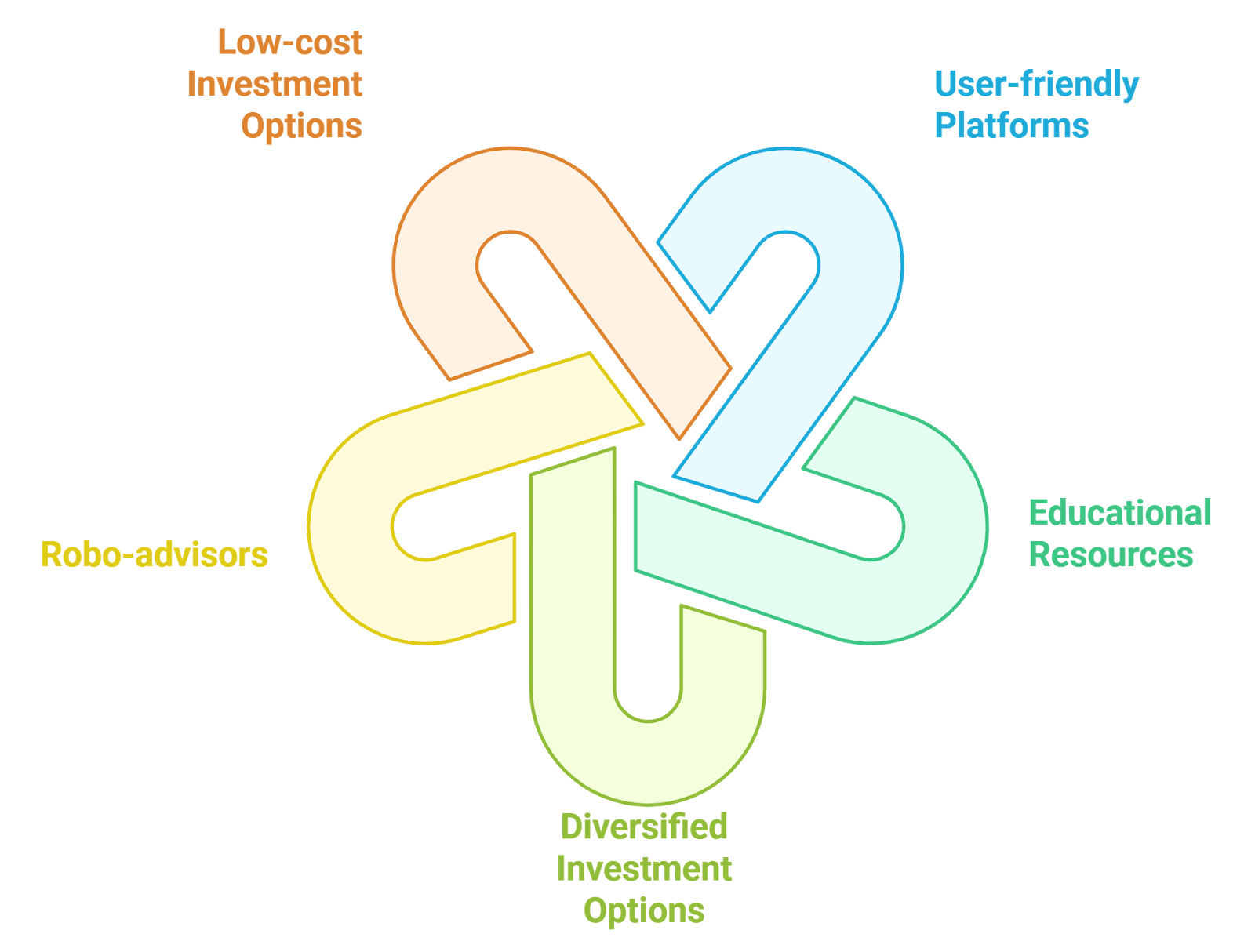
‍
Retail investor solutions are designed to cater to individual investors who are looking to grow their wealth through various investment avenues. These solutions provide tools, resources, and strategies tailored to meet the unique needs of retail investors.
- User-friendly platforms: Many financial institutions offer intuitive online platforms that allow retail investors to easily manage their portfolios, execute trades, and access market research. At Rapid Innovation, we leverage AI to enhance user experience, providing personalized insights and recommendations that empower investors to make informed decisions.
- Educational resources: Retail investor solutions often include educational materials such as webinars, articles, and tutorials to help investors understand market dynamics and investment strategies. Our AI-driven content curation ensures that investors receive the most relevant and timely information to enhance their investment knowledge.
- Diversified investment options: Retail investors can access a wide range of investment products, including stocks, bonds, mutual funds, and exchange-traded funds (ETFs), allowing for portfolio diversification. Rapid Innovation can assist in developing blockchain-based platforms that facilitate seamless access to diverse investment opportunities, ensuring transparency and security. For more information on innovative investment strategies, check out our comprehensive guide to real estate tokenization.
- Robo-advisors: Automated investment services, or robo-advisors, provide personalized investment advice based on individual risk tolerance and financial goals, making investing more accessible for retail investors. Our AI algorithms can optimize these robo-advisory services, delivering tailored investment strategies that adapt to market changes in real-time.
- Low-cost investment options: Many retail investor solutions focus on minimizing fees, offering low-cost index funds and commission-free trading to enhance returns. By integrating blockchain technology, we can help reduce transaction costs and improve the efficiency of trading processes, ultimately benefiting retail investors.
10.2. Institutional Investment Strategies
Institutional investment strategies are tailored for large organizations, such as pension funds, insurance companies, and endowments, that manage substantial assets. These strategies are designed to achieve specific financial goals while managing risk effectively.
- Asset allocation: Institutional investors often employ strategic asset allocation to diversify their portfolios across various asset classes, such as equities, fixed income, and alternative investments, to optimize returns. Rapid Innovation can provide AI-driven analytics to enhance asset allocation decisions, ensuring data-backed strategies.
- Risk management: Advanced risk management techniques, including hedging and derivatives, are commonly used to protect against market volatility and ensure the stability of returns. Our expertise in AI can help institutions develop predictive models that identify potential risks and suggest mitigation strategies.
- Active vs. passive management: Institutional investors may choose between active management, where fund managers make investment decisions based on research and analysis, and passive management, which aims to replicate market indices. We can assist in creating hybrid models that leverage AI for active management while maintaining the cost-effectiveness of passive strategies.
- Long-term focus: Institutional investors typically have a longer investment horizon, allowing them to withstand short-term market fluctuations and focus on sustainable growth. Our blockchain solutions can enhance transparency and trust in long-term investments, fostering stronger relationships between institutions and their stakeholders.
- ESG considerations: Environmental, social, and governance (ESG) factors are increasingly integrated into institutional investment strategies, reflecting a growing commitment to responsible investing. Rapid Innovation can help institutions implement AI tools that assess ESG metrics, ensuring compliance and alignment with ethical investment goals.
10.3. Wealth Management
Wealth management encompasses a comprehensive approach to managing an individual's or family's financial resources. It involves a range of services aimed at preserving and growing wealth over time.
- Personalized financial planning: Wealth management begins with a thorough assessment of an individual's financial situation, goals, and risk tolerance, leading to a customized financial plan. Our AI solutions can analyze vast amounts of data to create highly personalized financial strategies that adapt to changing circumstances.
- Investment management: Wealth managers actively manage investment portfolios, selecting appropriate assets and strategies to align with clients' objectives and market conditions. By utilizing AI-driven insights, we can enhance investment decision-making processes, leading to improved portfolio performance.
- Tax optimization: Wealth management services often include strategies to minimize tax liabilities, ensuring that clients retain more of their investment returns. Our blockchain technology can streamline tax reporting and compliance, making it easier for clients to manage their tax obligations efficiently.
- Estate planning: Wealth managers assist clients in developing estate plans that outline how their assets will be distributed upon death, helping to minimize estate taxes and ensure a smooth transfer of wealth. Rapid Innovation can provide secure blockchain solutions for estate planning, ensuring that clients' wishes are honored and assets are protected.
- Holistic approach: Wealth management considers all aspects of a client's financial life, including retirement planning, insurance needs, and philanthropic goals, to create a cohesive strategy for wealth preservation and growth. Our integrated AI and blockchain solutions enable a comprehensive view of clients' financial landscapes, facilitating informed decision-making and long-term success.
10.4. Pension Fund Management
Pension fund management is a critical aspect of financial planning, ensuring that individuals have sufficient resources for retirement. Effective management of pension funds involves several key components:
- Investment Strategy: Pension funds typically adopt a diversified investment strategy to balance risk and return. This may include a mix of equities, bonds, real estate, and alternative investments. At Rapid Innovation, we leverage AI-driven analytics to optimize investment strategies, enabling funds to identify high-potential assets and adjust allocations dynamically based on market conditions. Notable players in this space include Calpers AUM, CPPIB AUM, and OTPP AUM, which exemplify effective investment strategies.
- Risk Assessment: Understanding the risk tolerance of the fund is essential. This involves evaluating market conditions, economic indicators, and the demographic profile of the fund's beneficiaries. Our AI solutions can enhance risk assessment by providing predictive insights, allowing fund managers to make informed decisions that align with their risk appetite. This is particularly relevant for managing pension funds like the Stanbic IBTC Pension Scheme and ICICI Prudential Pension Fund.
- Regulatory Compliance: Pension funds must adhere to various regulations and standards set by government bodies. Compliance ensures the fund operates within legal frameworks and protects the interests of its members. Rapid Innovation offers blockchain-based solutions that enhance transparency and traceability, ensuring compliance with regulatory requirements while reducing administrative burdens. Pension management companies must stay updated on these regulations to maintain compliance.
- Performance Monitoring: Regular assessment of investment performance is crucial. This includes analyzing returns against benchmarks and making adjustments as necessary to optimize growth. Our advanced analytics tools provide real-time performance monitoring, enabling fund managers to react swiftly to market changes and optimize returns. Funds like BlackRock Pension Fund and HDFC Pension Management Co Ltd exemplify the importance of performance monitoring in achieving investment goals.
- Cost Management: Keeping operational costs low while maximizing returns is vital. This involves negotiating fees with fund managers and minimizing administrative expenses. By implementing AI-driven automation, Rapid Innovation helps pension funds streamline operations, reduce costs, and improve overall efficiency. Effective cost management is essential for all pension management, including those managed by companies like HDFC Pension Management Company Ltd.
Effective pension fund management not only secures the financial future of retirees but also contributes to overall economic stability.
10.5. Cryptocurrency and Alternative Investments
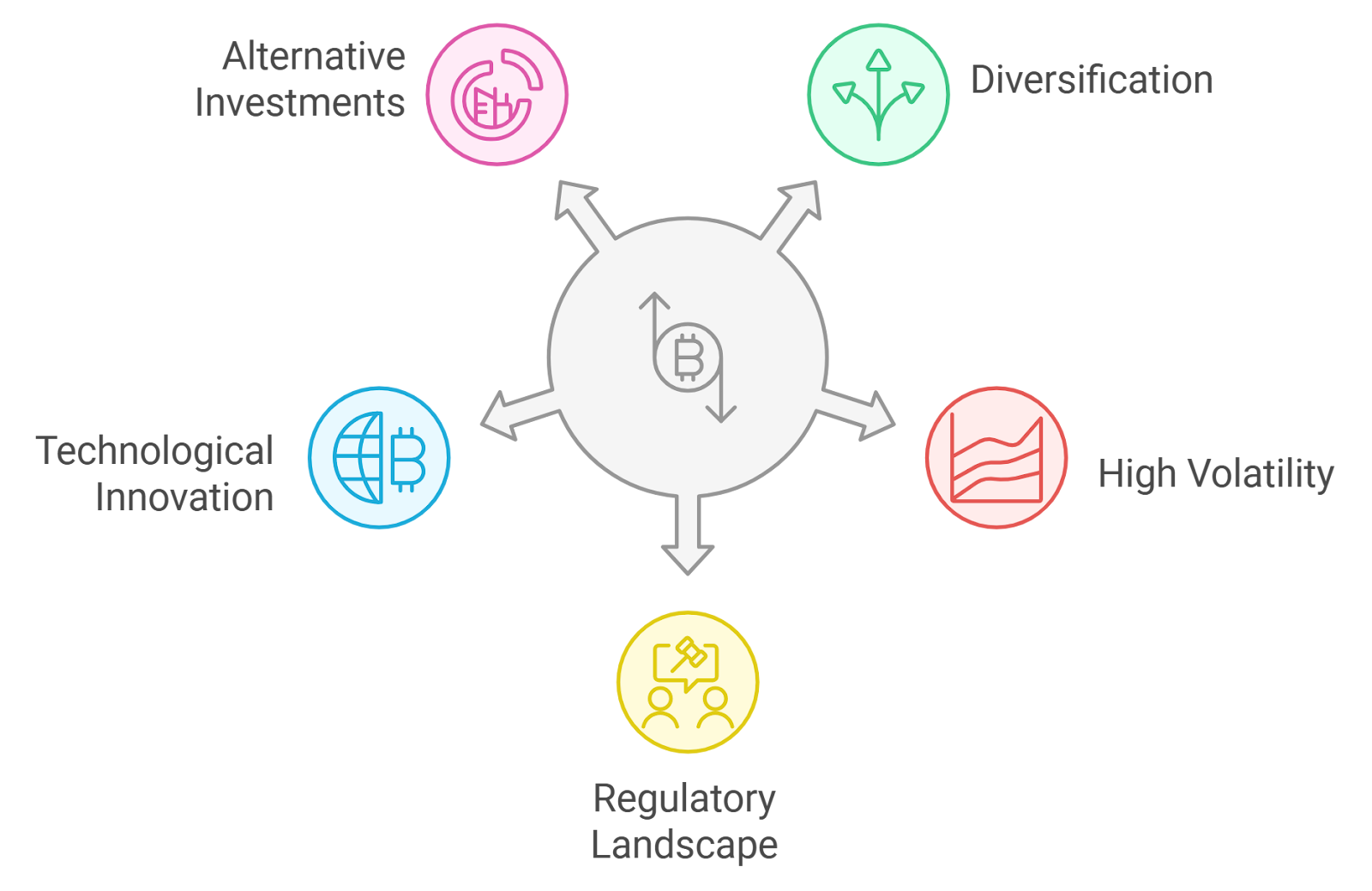
‍
Cryptocurrency and alternative investments have gained significant traction in recent years, attracting both institutional and retail investors. These assets offer unique opportunities and challenges:
- Diversification: Cryptocurrencies, such as Bitcoin and Ethereum, provide a new asset class that can enhance portfolio diversification. They often have low correlation with traditional assets like stocks and bonds. Rapid Innovation assists clients in integrating cryptocurrencies into their investment portfolios, utilizing AI to identify optimal entry and exit points.
- High Volatility: The cryptocurrency market is known for its price volatility. While this can lead to substantial gains, it also poses significant risks. Investors must be prepared for price fluctuations. Our risk management solutions leverage AI to analyze market trends and volatility, helping investors make informed decisions.
- Regulatory Landscape: The regulatory environment for cryptocurrencies is evolving. Investors should stay informed about regulations in their jurisdiction to ensure compliance and understand potential risks. Rapid Innovation provides consulting services to navigate the complex regulatory landscape, ensuring clients remain compliant while maximizing their investment potential.
- Technological Innovation: Blockchain technology, which underpins cryptocurrencies, is revolutionizing various sectors. Investing in blockchain-related projects can offer exposure to innovative solutions and growth potential. Our expertise in blockchain development enables clients to explore and invest in cutting-edge projects that align with their strategic goals.
- Alternative Investments: Beyond cryptocurrencies, alternative investments include real estate, hedge funds, private equity, and commodities. These assets can provide additional diversification and potential for higher returns. Rapid Innovation helps clients identify and evaluate alternative investment opportunities, utilizing data-driven insights to enhance decision-making.
Investors should conduct thorough research and consider their risk tolerance before venturing into cryptocurrency and alternative investments.
10.6. Cross-Border Investment Recommendations
Cross-border investments can offer significant opportunities for growth and diversification. However, they also come with unique challenges that investors must navigate:
- Market Research: Understanding the economic and political landscape of the target country is crucial. Investors should analyze market trends, currency stability, and regulatory environments. Rapid Innovation employs AI tools to conduct comprehensive market research, providing clients with actionable insights for informed investment decisions.
- Currency Risk: Investing in foreign markets exposes investors to currency fluctuations. Hedging strategies can mitigate this risk, but they require careful planning and execution. Our blockchain solutions facilitate secure and efficient currency transactions, helping clients manage currency risk effectively.
- Tax Implications: Different countries have varying tax laws that can impact investment returns. Investors should consult with tax professionals to understand the implications of cross-border investments. Rapid Innovation offers consulting services to navigate the complexities of international tax regulations, ensuring clients optimize their tax strategies.
- Cultural Considerations: Cultural differences can affect business practices and consumer behavior. Investors should be aware of these factors to make informed decisions. Our team provides insights into cultural dynamics, helping clients tailor their strategies to local markets.
- Legal Framework: Familiarity with the legal environment in the target country is essential. This includes understanding property rights, investment regulations, and dispute resolution mechanisms. Rapid Innovation assists clients in navigating legal complexities, ensuring compliance and protecting their investments.
Cross-border investments can enhance portfolio performance, but they require diligent research and strategic planning to navigate the complexities involved.
11. Performance Evaluation
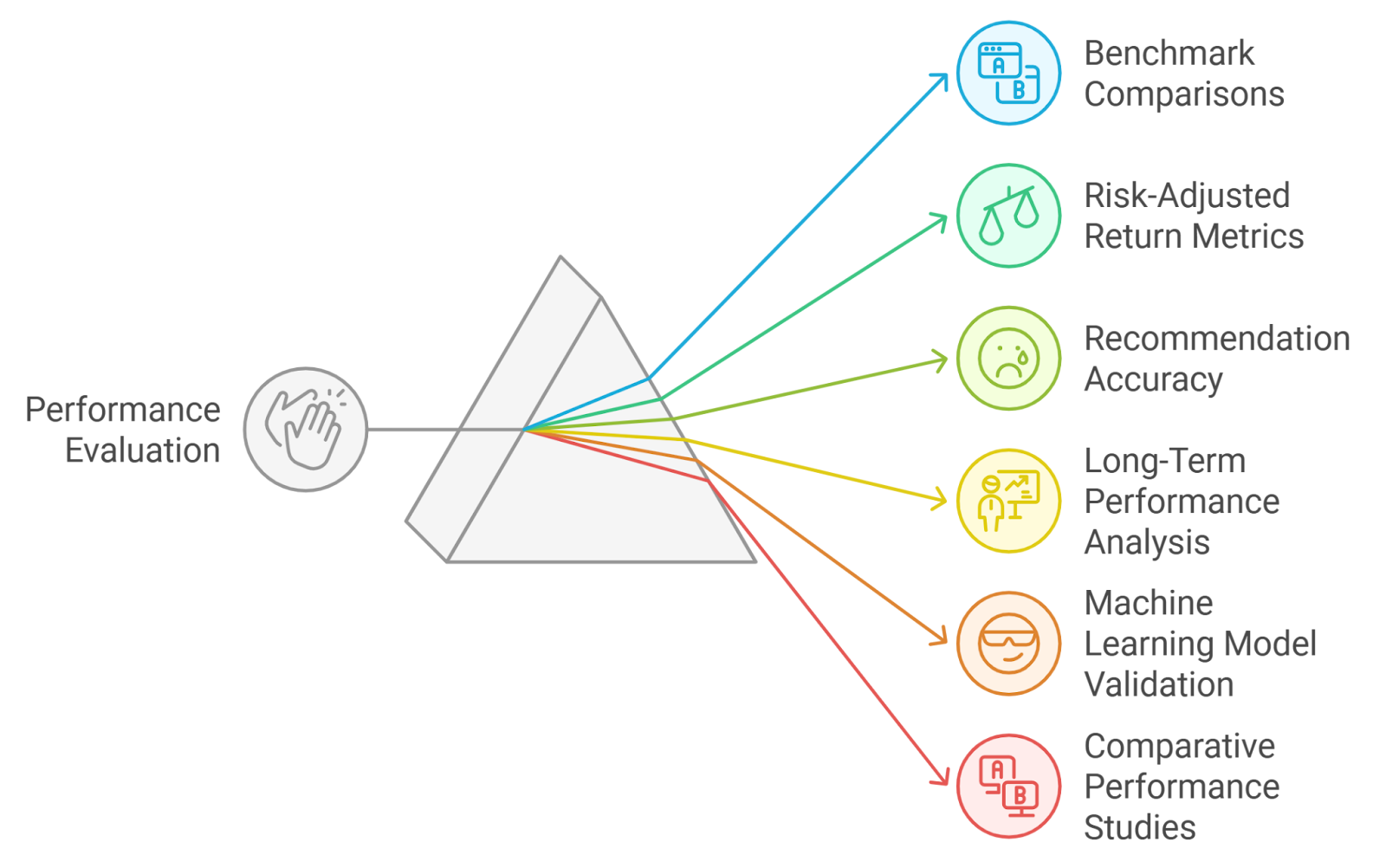
‍
Performance evaluation is a critical aspect of investment management, allowing investors to assess how well their portfolios are performing relative to their objectives and benchmarks. It involves analyzing various metrics and comparisons to determine the effectiveness of investment strategies, including mutual fund evaluation and investment performance evaluation.
11.1 Benchmark Comparisons
Benchmark comparisons are essential for evaluating the performance of an investment portfolio. A benchmark serves as a standard against which the performance of an investment can be measured.
- Definition of Benchmarks: Benchmarks can be indices, such as the S&P 500 or the Dow Jones Industrial Average, or they can be custom indices tailored to specific investment strategies.
- Purpose: The primary purpose of using benchmarks is to provide a point of reference. Investors can see if their portfolio is outperforming or underperforming relative to the benchmark.
- Types of Benchmarks: Â
- Market Indices: These are widely recognized indices that represent a specific segment of the market.
- Peer Group Comparisons: This involves comparing a portfolio's performance against similar funds or investment strategies, such as mutual fund performance analysis.
- Custom Benchmarks: Tailored benchmarks that reflect the specific investment goals and asset allocation of a portfolio.
- Performance Metrics: Common metrics used in benchmark comparisons include: Â
- Absolute Return: The total return of the portfolio over a specific period.
- Relative Return: The return of the portfolio compared to the benchmark return.
- Tracking Error: A measure of how closely a portfolio follows its benchmark, calculated as the standard deviation of the difference between the portfolio return and the benchmark return.
- Importance of Benchmarking: Â
- Helps in identifying strengths and weaknesses in investment strategies.
- Provides insights into market trends and performance drivers.
- Aids in making informed decisions about asset allocation and investment adjustments.
At Rapid Innovation, we leverage advanced AI algorithms to analyze benchmark comparisons, enabling our clients to gain deeper insights into their investment performance. By utilizing machine learning techniques, we can identify patterns and trends that may not be immediately apparent, allowing for more informed decision-making and ultimately enhancing ROI. This includes evaluating mutual funds performance and evaluating ETF performance.
11.2 Risk-Adjusted Return Metrics
Risk-adjusted return metrics are crucial for understanding the performance of an investment in relation to the risk taken to achieve that return. These metrics help investors evaluate whether the returns justify the risks involved.
- Definition: Risk-adjusted returns measure how much return an investment has generated relative to the amount of risk taken. This is important because higher returns often come with higher risks.
- Common Risk-Adjusted Metrics: Â
- Sharpe Ratio: This ratio measures the excess return per unit of risk. It is calculated by subtracting the risk-free rate from the portfolio return and dividing by the standard deviation of the portfolio returns. A higher Sharpe ratio indicates better risk-adjusted performance.
- Treynor Ratio: Similar to the Sharpe ratio, but it uses beta (a measure of systematic risk) instead of standard deviation. It assesses how much excess return is generated for each unit of market risk.
- Sortino Ratio: This ratio focuses only on downside risk, measuring the return relative to the risk of negative returns. It is particularly useful for investors who are more concerned about losses than volatility.
- Importance of Risk-Adjusted Metrics: Â
- Helps investors understand the trade-off between risk and return.
- Allows for better comparisons between different investments or portfolios with varying risk profiles, including evaluating stock performance.
- Aids in portfolio optimization by identifying investments that offer the best return for the level of risk taken.
- Application: Investors can use these metrics to: Â
- Make informed decisions about asset allocation.
- Identify underperforming investments that may need to be reevaluated or replaced, such as evaluating investment manager performance.
- Align investment strategies with risk tolerance and financial goals.
At Rapid Innovation, we utilize blockchain technology to enhance the transparency and security of performance evaluations. By implementing decentralized ledgers, we ensure that all performance data is immutable and verifiable, providing our clients with a trustworthy foundation for their investment decisions.
In conclusion, performance evaluation through benchmark comparisons and risk-adjusted return metrics is vital for effective investment management. By utilizing these tools, including mutual fund performance analysis pdf, investors can gain valuable insights into their portfolios, make informed decisions, and ultimately enhance their investment outcomes. Rapid Innovation is committed to helping clients achieve greater ROI through innovative AI and blockchain solutions tailored to their specific needs, including performance evaluation in portfolio management. For more information on the business impact of blockchain, visit the ROI of Enterprise Blockchain.
11.3. Recommendation Accuracy
Recommendation accuracy is a critical metric in evaluating the effectiveness of recommendation systems. It measures how well the system predicts user preferences and suggests relevant items. High recommendation accuracy leads to improved user satisfaction and engagement, ultimately driving greater ROI for businesses.
- Factors influencing recommendation accuracy include: Â
- Data quality: The accuracy of recommendations heavily relies on the quality of the data used for training the model. Clean, relevant, and comprehensive datasets yield better results. Rapid Innovation emphasizes the importance of data curation and preprocessing to ensure optimal model performance.
- Algorithm choice: Different algorithms, such as collaborative filtering, content-based filtering, and hybrid methods, can significantly impact accuracy. Selecting the right algorithm based on the context is essential. Our team at Rapid Innovation leverages advanced algorithms tailored to specific business needs, enhancing the relevance of recommendations.
- User behavior: Understanding user preferences and behavior patterns is crucial. Systems that adapt to changing user interests tend to perform better. Rapid Innovation employs machine learning techniques to analyze user behavior, allowing for dynamic adjustments to recommendations.
- Techniques to enhance recommendation accuracy: Â
- Regular updates: Continuously updating the model with new data helps maintain accuracy over time. Rapid Innovation implements automated data pipelines to ensure that models are always trained on the latest information.
- A/B testing: Implementing A/B tests allows for comparing different recommendation strategies to identify the most effective one. Our consulting services guide clients through the A/B testing process, ensuring data-driven decision-making.
- User feedback: Incorporating user feedback into the recommendation process can refine suggestions and improve accuracy. Rapid Innovation integrates feedback loops into systems, enabling continuous improvement of recommendations. For more insights, check out the best practices for transformer model development.
11.4. Long-Term Performance Analysis
Long-term performance analysis evaluates how well a recommendation system performs over an extended period. This analysis is vital for understanding the sustainability and effectiveness of the system in real-world applications, ultimately contributing to long-term business success.
- Key aspects of long-term performance analysis include: Â
- User retention: Monitoring how many users continue to engage with the system over time provides insights into its effectiveness. Rapid Innovation helps clients track user engagement metrics to inform strategic adjustments.
- Adaptability: A system that can adapt to changing user preferences and market trends is more likely to maintain its performance. Our solutions are designed with flexibility in mind, allowing for quick adaptations to evolving business landscapes.
- Consistency: Evaluating the consistency of recommendations over time helps identify any degradation in performance. Rapid Innovation employs robust monitoring tools to ensure that recommendation systems maintain high standards of accuracy.
- Methods for conducting long-term performance analysis: Â
- Historical data analysis: Analyzing historical user interaction data can reveal trends and patterns that inform future improvements. Our data scientists utilize advanced analytics to extract actionable insights from historical data.
- Performance metrics: Utilizing metrics such as precision, recall, and F1 score over time helps assess the system's effectiveness. Rapid Innovation provides comprehensive reporting tools to track these metrics and guide optimization efforts.
- User surveys: Gathering user feedback through surveys can provide qualitative insights into the system's long-term performance. We assist clients in designing effective survey strategies to capture user sentiment.
11.5. Machine Learning Model Validation
Machine learning model validation is a crucial step in ensuring that the recommendation system performs as expected. It involves assessing the model's accuracy, reliability, and generalizability to new data, which is essential for maximizing ROI.
- Important aspects of model validation include: Â
- Cross-validation: This technique involves dividing the dataset into multiple subsets to train and test the model. It helps ensure that the model performs well across different data samples. Rapid Innovation employs rigorous cross-validation techniques to enhance model robustness.
- Performance metrics: Common metrics for evaluating model performance include accuracy, precision, recall, and AUC-ROC. These metrics provide a comprehensive view of the model's effectiveness. Our team focuses on selecting the most relevant metrics based on client objectives, including recommendation system accuracy.
- Overfitting and underfitting: Identifying and addressing overfitting (where the model performs well on training data but poorly on new data) and underfitting (where the model fails to capture underlying patterns) is essential for robust model validation. Rapid Innovation implements strategies to mitigate these issues, ensuring reliable model performance.
- Best practices for machine learning model validation: Â
- Use a holdout dataset: Keeping a separate dataset for testing helps evaluate the model's performance on unseen data. Our validation frameworks are designed to incorporate holdout datasets effectively.
- Regular updates: Continuously validating and updating the model with new data ensures it remains relevant and accurate. Rapid Innovation emphasizes the importance of ongoing model maintenance to adapt to changing conditions.
- Ensemble methods: Combining multiple models can enhance performance and provide more reliable recommendations. Our expertise in ensemble techniques allows us to deliver superior recommendation systems that maximize client ROI, going beyond accuracy by evaluating recommender systems by coverage and serendipity.
11.6. Comparative Performance Studies
Comparative performance studies are essential for evaluating the effectiveness of different systems, processes, or interventions. These studies allow researchers and practitioners to assess how various approaches stack up against one another in terms of efficiency, outcomes, and overall impact. For instance, a comparative analysis of student performance in an online setting can provide insights into the effectiveness of digital learning environments.
- Purpose of Comparative Performance Studies: Â
- Identify best practices and benchmarks.
- Facilitate informed decision-making.
- Enhance accountability and transparency in performance evaluation.
- Key Components: Â
- Selection of appropriate metrics for comparison.
- Establishment of a control group or baseline for accurate assessment.
- Use of statistical methods to analyze data and draw conclusions.
- Types of Comparative Performance Studies: Â
- Cross-sectional studies that compare different groups at a single point in time.
- Longitudinal studies that track performance over time to observe changes and trends.
- Meta-analyses that synthesize findings from multiple studies to provide a comprehensive overview.
- Applications: Â
- In healthcare, comparing treatment outcomes across different hospitals or clinics.
- In education, evaluating the effectiveness of various teaching methods or curricula, such as comparative dramaturgy and performance research.
- In business, assessing the performance of different marketing strategies or operational processes, including a comparative analysis of lending performance of commercial banks.
- Challenges: Â
- Ensuring comparability between groups or systems being studied.
- Controlling for confounding variables that may skew results.
- Accessing reliable and comprehensive data for analysis, such as in a comparative analysis of mutual fund schemes.
12. Challenges and Limitations
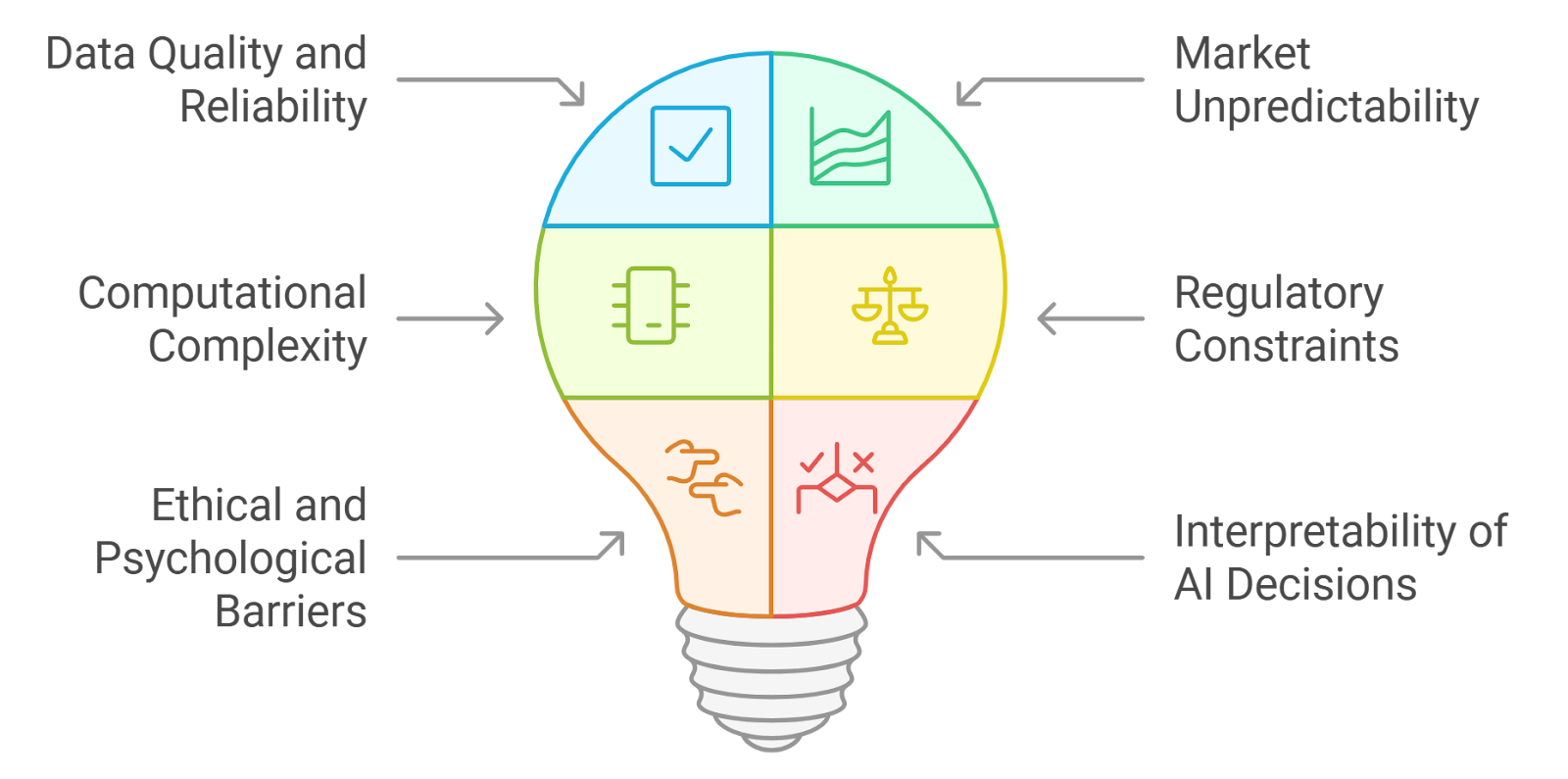
‍
Despite the value of comparative performance studies, several challenges and limitations can hinder their effectiveness. Understanding these obstacles is crucial for researchers and practitioners aiming to conduct meaningful evaluations.
- Common Challenges: Â
- Data availability and accessibility can limit the scope of studies.
- Variability in measurement tools and methodologies can lead to inconsistent results.
- Ethical considerations may restrict the ability to conduct certain types of comparisons.
- Limitations: Â
- Findings may not be generalizable to broader populations or contexts.
- Potential biases in data collection and analysis can affect the validity of conclusions.
- Resource constraints, including time and funding, can impact the depth and rigor of studies, such as in mutual funds comparative analysis.
12.1. Data Quality and Reliability
Data quality and reliability are critical factors in the success of comparative performance studies. High-quality data ensures that findings are accurate and trustworthy, while reliable data collection methods enhance the credibility of the research.
- Importance of Data Quality: Â
- Accurate data leads to valid conclusions and informed decision-making.
- Poor data quality can result in misleading findings and ineffective interventions.
- Factors Affecting Data Quality: Â
- Completeness: Ensuring that all necessary data points are collected.
- Consistency: Maintaining uniformity in data collection methods across different groups.
- Accuracy: Verifying that data is correct and free from errors.
- Strategies to Enhance Data Quality: Â
- Implementing standardized data collection protocols.
- Regularly training staff involved in data collection to minimize errors.
- Conducting audits and validation checks to ensure data integrity.
- Reliability of Data: Â
- Reliability refers to the consistency of data over time and across different contexts.
- High reliability indicates that repeated measurements yield similar results.
- Methods to Assess Reliability: Â
- Test-retest reliability: Measuring the same subjects at different times to check for consistency.
- Inter-rater reliability: Evaluating the agreement between different observers or data collectors.
- Internal consistency: Assessing the correlation between different items or measures within a single test.
- Challenges in Ensuring Data Quality and Reliability: Â
- Limited resources for comprehensive data collection and analysis.
- Variability in data sources, which can introduce discrepancies.
- The need for ongoing monitoring and evaluation to maintain data standards.
By addressing these challenges and focusing on data quality and reliability, researchers can enhance the effectiveness of comparative performance studies, leading to more accurate and actionable insights. At Rapid Innovation, we leverage advanced AI and Blockchain technologies to streamline data collection and analysis processes, ensuring that our clients achieve greater ROI through informed decision-making and enhanced operational efficiency.
12.2. Market Unpredictability
Market unpredictability refers to the inherent volatility and uncertainty present in financial markets. This unpredictability can stem from various factors, including economic indicators, geopolitical events, and changes in consumer behavior. Economic indicators such as inflation rates, unemployment figures, and GDP growth can significantly influence market trends. Geopolitical events, including elections, trade wars, and international conflicts, can create sudden shifts in market sentiment. Consumer behavior changes, driven by technological advancements or social trends, can also lead to unexpected market movements.
Investors and businesses must navigate this market unpredictability to make informed decisions. Strategies to manage market unpredictability include:
- Diversification of investment portfolios to spread risk.
- Utilizing hedging techniques to protect against adverse market movements.
- Staying informed about global events and economic indicators to anticipate potential market shifts.
At Rapid Innovation, we leverage AI-driven analytics to provide clients with real-time insights into market trends, enabling them to make data-informed decisions that mitigate risks associated with market unpredictability. Our solutions help businesses identify emerging opportunities, allowing them to adapt swiftly to changing market conditions and achieve greater ROI. For more information on how AI can assist in navigating regulatory compliance, visit our article on AI in Financial Regulatory Compliance.
12.3. Computational Complexity
Computational complexity refers to the challenges associated with processing and analyzing large volumes of data in financial markets. As technology advances, the amount of data generated continues to grow exponentially, leading to several issues. High-frequency trading generates vast amounts of data that require real-time analysis. Complex algorithms and models are needed to interpret this data effectively. Additionally, the need for speed in decision-making can lead to errors if systems are not adequately designed.
To address computational complexity, financial institutions and investors can adopt several strategies:
- Implementing advanced analytics and machine learning techniques to enhance data processing capabilities.
- Investing in robust IT infrastructure to support high-speed data analysis.
- Collaborating with data scientists and quantitative analysts to develop more effective models.
At Rapid Innovation, we specialize in developing AI and machine learning solutions that streamline data processing and enhance analytical capabilities. By tackling computational complexity, our clients can improve their decision-making processes and gain a competitive edge in the market, ultimately leading to increased ROI.
12.4. Regulatory Constraints
Regulatory constraints refer to the rules and regulations imposed by government bodies and financial authorities to ensure market integrity and protect investors. These constraints can significantly impact how businesses operate and make decisions in the financial sector. Regulations can dictate capital requirements, trading practices, and reporting standards. Compliance with these regulations often requires significant resources and can limit operational flexibility. Furthermore, changes in regulations can create uncertainty, affecting market dynamics and investor confidence.
To navigate regulatory constraints, organizations can consider the following approaches:
- Establishing a dedicated compliance team to monitor and adapt to regulatory changes.
- Engaging with regulatory bodies to stay informed about upcoming changes and participate in discussions.
- Implementing robust compliance programs to ensure adherence to regulations and minimize risks.
Rapid Innovation offers consulting services that help organizations understand and manage regulatory constraints effectively. By integrating blockchain technology, we provide transparent and secure solutions that enhance compliance efforts, thereby maintaining operational efficiency and fostering trust in the financial markets. Our expertise ensures that clients can navigate the regulatory landscape with confidence, ultimately supporting their business goals and enhancing ROI.
12.5. Ethical and Psychological Barriers
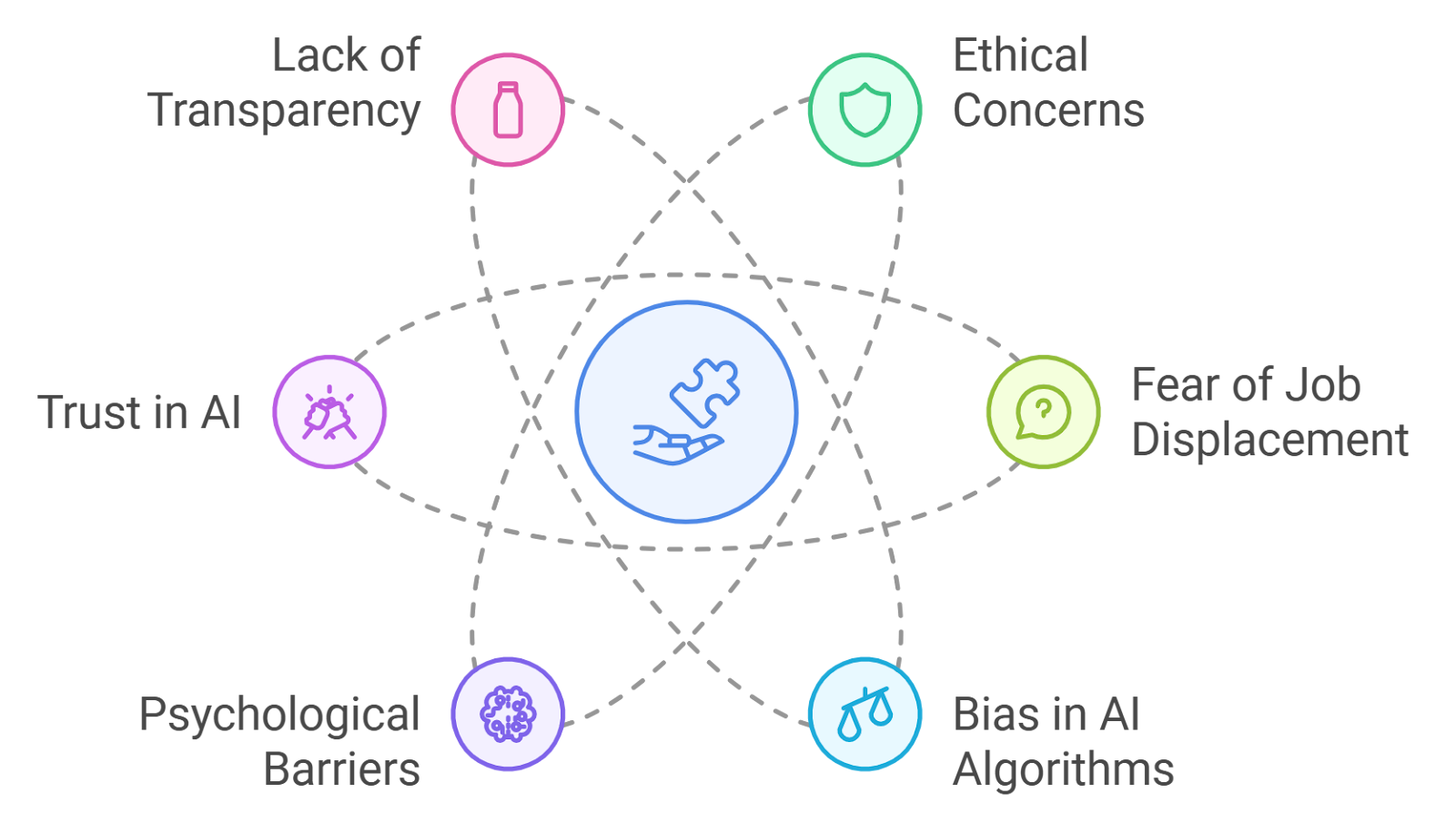
‍
Ethical and psychological barriers play a significant role in the adoption and implementation of artificial intelligence (AI) technologies. These barriers can hinder the effectiveness of AI systems and impact user trust and acceptance.
- Ethical concerns often arise from the potential misuse of AI, including issues related to privacy, surveillance, and data security. Rapid Innovation emphasizes the importance of ethical AI development, ensuring that our solutions prioritize user privacy and data protection.
- The fear of job displacement due to automation can create resistance among employees and stakeholders. We work closely with clients to implement AI solutions that augment human capabilities rather than replace them, fostering a collaborative environment.
- Bias in AI algorithms can lead to unfair treatment of individuals or groups, raising questions about accountability and fairness. Our team at Rapid Innovation is dedicated to developing unbiased AI systems through rigorous testing and validation processes.
- Psychological barriers include the fear of the unknown, where users may feel overwhelmed by the complexity of AI systems. We provide comprehensive training and support to help users navigate these technologies confidently.
- Trust in AI is crucial; if users do not understand how AI makes decisions, they may be reluctant to rely on it. We prioritize transparency in our AI solutions, offering clear insights into how decisions are made.
- The lack of transparency in AI processes can exacerbate these fears, leading to skepticism about the technology's reliability. Rapid Innovation advocates for open communication about AI capabilities and limitations to build trust with our clients.
Addressing these barriers, including barriers to AI adoption, requires a multi-faceted approach, including ethical guidelines, user education, and transparent communication about AI capabilities and limitations. For expert guidance in overcoming these challenges, consider partnering with an AI consulting company like Rapid Innovation.
12.6. Interpretability of AI Decisions
Interpretability refers to the degree to which a human can understand the cause of a decision made by an AI system. This is a critical aspect of AI, especially in high-stakes applications such as healthcare, finance, and criminal justice.
Many AI models, particularly deep learning algorithms, operate as "black boxes," making it difficult to trace how they arrive at specific conclusions. The lack of interpretability can lead to mistrust among users, as they may not feel comfortable relying on decisions that they cannot comprehend. Regulatory bodies are increasingly emphasizing the need for explainable AI, particularly in sectors where decisions can significantly impact individuals' lives. Techniques such as LIME (Local Interpretable Model-agnostic Explanations) and SHAP (SHapley Additive exPlanations) are being developed to enhance the interpretability of AI models. At Rapid Innovation, we integrate these techniques into our AI solutions, providing clear explanations for AI decisions to improve user trust and facilitate better decision-making processes. Organizations must prioritize interpretability to ensure ethical AI deployment and compliance with emerging regulations.
Future Trends and Outlook
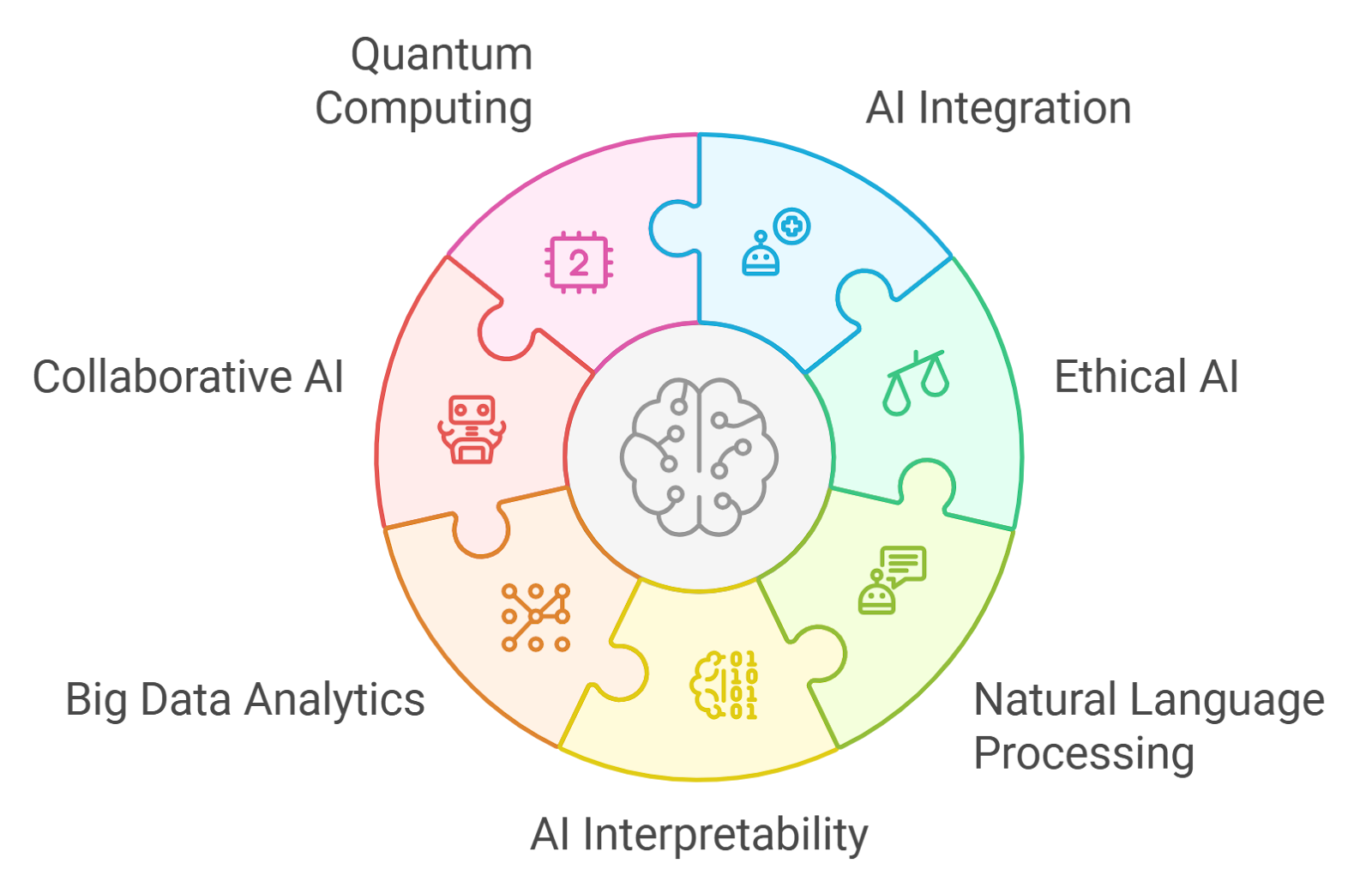
‍
The future of AI is poised for significant advancements, driven by ongoing research, technological innovations, and evolving societal needs.
- Increased integration of AI in various sectors, including healthcare, finance, and transportation, is expected to enhance efficiency and decision-making. Rapid Innovation is at the forefront of this integration, helping clients leverage AI to optimize their operations.
- The rise of ethical AI will lead to the development of frameworks and guidelines to ensure responsible AI usage. We are committed to guiding our clients through these frameworks to ensure compliance and ethical practices.
- Advances in natural language processing (NLP) will enable more intuitive human-AI interactions, making AI systems more accessible to non-technical users. Our NLP solutions are designed to enhance user experience and engagement.
- The focus on AI interpretability will grow, with more organizations seeking transparent AI solutions to build trust and comply with regulations. Rapid Innovation prioritizes interpretability in our AI offerings, ensuring that clients can confidently adopt these technologies.
- AI will increasingly leverage big data analytics, enabling more personalized and predictive services across industries. We help clients harness the power of big data to drive insights and improve decision-making.
- Collaborative AI, where humans and machines work together, will become more prevalent, enhancing productivity and creativity. Our solutions are designed to foster collaboration between AI systems and human users, maximizing efficiency.
- The emergence of quantum computing may revolutionize AI capabilities, allowing for faster processing and more complex problem-solving. Rapid Innovation is exploring the potential of quantum computing to enhance our AI solutions further.
As these trends unfold, the landscape of AI will continue to evolve, presenting both opportunities and challenges for businesses and society at large. Rapid Innovation is dedicated to helping clients navigate this landscape, ensuring they achieve their business goals efficiently and effectively, while also addressing the various AI adoption barriers that may arise.
13.1. Quantum Computing in Investments
Quantum computing is revolutionizing the investment landscape by providing unprecedented computational power. This technology leverages the principles of quantum mechanics to process information in ways that classical computers cannot. Quantum computers can analyze vast datasets at lightning speed, allowing investors to identify patterns and trends that would be impossible to detect with traditional computing. Additionally, quantum algorithms can improve risk modeling by simulating various market scenarios, helping investors make more informed decisions. They can also optimize investment portfolios by evaluating numerous combinations of assets simultaneously, leading to better returns. Furthermore, quantum algorithms can execute trades faster and more efficiently, capitalizing on market opportunities in real-time.
At Rapid Innovation, we harness the potential of quantum computing to help our clients in the investment sector achieve greater ROI. By integrating quantum algorithms into their investment strategies, we enable firms to make data-driven decisions that enhance their competitive edge. As the technology matures, it is expected to significantly impact hedge funds, asset management firms, and other investment entities. The potential for quantum computing in investments is vast, with estimates suggesting it could lead to a multi-trillion-dollar market by 2030. Investors are increasingly looking at quantum computing stocks, such as QUBT stock and D-Wave stock, as part of their portfolios.
13.2. Advanced Machine Learning Techniques
Advanced machine learning techniques are transforming the investment sector by enabling more accurate predictions and smarter decision-making processes. These techniques utilize algorithms that learn from data, improving their performance over time. Machine learning models can analyze historical data to forecast future market trends, helping investors make proactive decisions. By processing news articles, social media, and other text data, machine learning can gauge market sentiment, providing insights into public perception and potential market movements. Advanced algorithms can also identify unusual patterns in trading activity, helping to detect and prevent fraudulent transactions. Moreover, machine learning enables the development of sophisticated trading bots that can execute trades based on real-time data analysis, optimizing investment strategies.
At Rapid Innovation, we implement advanced machine learning solutions tailored to our clients' specific needs, ensuring they stay ahead in a rapidly evolving market. The integration of machine learning in investments is not just a trend; it is becoming a necessity for firms looking to maintain a competitive edge. The adoption of machine learning in finance is expected to grow significantly, with many firms already reaping the benefits. Investors are also exploring quantum computing penny stocks and companies investing in quantum computing as part of their advanced investment strategies.
13.3. Integrated AI Ecosystems
Integrated AI ecosystems represent a holistic approach to utilizing artificial intelligence across various functions within investment firms. This integration allows for seamless data sharing and collaboration among different AI applications. An integrated AI ecosystem can streamline data collection, storage, and analysis, ensuring that all relevant information is readily available for decision-making. By connecting various AI tools and platforms, investment firms can foster collaboration among teams, leading to more innovative solutions and strategies. Integrated systems can provide real-time analytics and insights, enabling investors to react swiftly to market changes and opportunities. As investment firms grow, integrated AI ecosystems can easily scale to accommodate increasing data volumes and complexity, ensuring that firms remain agile and responsive.
At Rapid Innovation, we specialize in developing integrated AI ecosystems that enhance operational efficiency and decision-making capabilities for investment firms. The shift towards integrated AI ecosystems is driven by the need for efficiency and effectiveness in investment management. Firms that adopt this approach are likely to see improved performance and a stronger competitive position in the market. Research indicates that companies leveraging integrated AI solutions can achieve up to a 30% increase in productivity. As the landscape evolves, investors are also keeping an eye on stocks related to quantum technology, such as Honeywell quantum stock and IonQ investment, to stay ahead of the curve.
13.4. Personalized Financial Intelligence
Personalized financial intelligence refers to the use of advanced technologies and data analytics to tailor financial advice and services to individual needs. This approach leverages artificial intelligence (AI) and machine learning to analyze a person's financial behavior, preferences, and goals.
- Customization: Financial services can be personalized based on individual risk tolerance, investment goals, and spending habits. Rapid Innovation employs AI algorithms to create bespoke personalized financial intelligence strategies that align with each client's unique profile, enhancing their investment potential. Â
- Real-time insights: Users receive immediate feedback on their financial decisions, helping them make informed choices. Our AI-driven platforms provide clients with real-time analytics, enabling them to adjust their strategies promptly for optimal results. Â
- Predictive analytics: By analyzing historical data, financial intelligence tools can forecast future trends and suggest optimal investment strategies. Rapid Innovation utilizes machine learning models to predict market movements, allowing clients to capitalize on emerging opportunities. Â
- User-friendly interfaces: Many platforms offer intuitive dashboards that simplify complex financial data, making it accessible to everyone. We prioritize user experience in our solutions, ensuring that clients can easily navigate their financial landscapes. Â
- Enhanced decision-making: Personalized insights empower users to take control of their financial futures, leading to better investment outcomes. Our consulting services guide clients in interpreting these insights, fostering informed decision-making that drives ROI.
The rise of personalized financial intelligence is transforming how individuals manage their finances, making it easier to achieve financial goals.
13.5. Global Economic Modeling
Global economic modeling involves the use of mathematical and statistical techniques to simulate and analyze economic systems on a worldwide scale. This modeling helps policymakers, businesses, and researchers understand complex economic interactions and predict future trends.
- Economic indicators: Models often incorporate key indicators such as GDP, inflation rates, and employment figures to assess economic health. Rapid Innovation's expertise in data analytics allows us to create robust models that provide actionable insights for our clients. Â
- Scenario analysis: By creating different scenarios, economists can evaluate the potential impact of policy changes, market shifts, or global events. Our team leverages advanced modeling techniques to help clients prepare for various economic conditions, ensuring strategic resilience. Â
- Interconnectedness: Global economic models highlight how economies are interlinked, showing how changes in one region can affect others. We assist clients in understanding these dynamics, enabling them to make informed decisions in a global context. Â
- Data-driven decisions: Policymakers can use these models to make informed decisions that promote economic stability and growth. Rapid Innovation supports clients in utilizing data-driven insights to enhance their strategic planning. Â
- Risk assessment: Economic modeling helps identify potential risks and vulnerabilities in the global economy, allowing for proactive measures. Our consulting services include risk assessment frameworks that empower clients to mitigate potential threats effectively.
As the world becomes increasingly interconnected, global economic modeling is essential for understanding and navigating the complexities of the modern economy.
13.6. Emerging Investment Technologies
Emerging investment technologies are reshaping the financial landscape by introducing innovative tools and platforms that enhance investment strategies. These technologies leverage advancements in AI, blockchain, and data analytics to create new opportunities for investors.
- Robo-advisors: Automated platforms provide personalized investment advice based on algorithms, making investing accessible to a broader audience. Rapid Innovation develops sophisticated robo-advisory solutions that cater to diverse investor profiles, maximizing engagement and returns. Â
- Blockchain technology: This decentralized ledger system enhances transparency and security in transactions, reducing fraud and increasing trust. Our blockchain solutions ensure secure and efficient transactions, fostering confidence among investors. Â
- Crowdfunding platforms: These allow individuals to invest in startups and projects, democratizing access to investment opportunities. We assist clients in launching crowdfunding initiatives that connect them with potential investors, driving growth and innovation. Â
- Artificial intelligence: AI-driven analytics can identify trends and patterns in market data, enabling more informed investment decisions. Rapid Innovation harnesses AI to provide clients with deep market insights, enhancing their investment strategies. Â
- Social trading: Platforms that allow users to follow and replicate the trades of successful investors are gaining popularity, fostering a community-driven approach to investing. We create social trading platforms that empower users to learn from each other, enhancing their investment acumen.
The integration of these emerging technologies is revolutionizing how individuals and institutions approach investing, making it more efficient and inclusive. Rapid Innovation is at the forefront of this transformation, helping clients leverage these advancements to achieve greater ROI.
14. Implementation Roadmap
An implementation roadmap is a strategic plan that outlines the steps necessary to achieve a specific goal or project. It serves as a guide to ensure that all stakeholders are aligned and that the project progresses smoothly. The roadmap typically includes various phases, timelines, and key milestones, such as the safe implementation roadmap and the scaled agile implementation roadmap.
14.1 Initial Assessment
The initial assessment is a critical first step in the implementation roadmap. It involves evaluating the current state of the organization, identifying needs, and determining the resources required for successful implementation. This process includes several key activities:
- Identify existing processes and systems.
- Assess the strengths and weaknesses of current operations.
- Gather input from stakeholders to understand their needs and expectations.
- Analyze market trends and the competitive landscape.
- Determine the scope of the project and set clear objectives.
Conducting a thorough initial assessment helps in identifying gaps and opportunities, which can inform the subsequent phases of the implementation roadmap, including the scaled agile framework implementation roadmap. This phase also aids in risk management by highlighting potential challenges early on. At Rapid Innovation, we leverage our expertise in AI and Blockchain to provide insights that enhance this assessment, ensuring that our clients are well-prepared to move forward.
14.2 Technology Selection
Technology selection is a pivotal aspect of the implementation roadmap. Choosing the right technology can significantly impact the success of the project. The following steps are essential in this phase:
- Evaluate available technologies that align with project goals, such as the power bi implementation roadmap or the salesforce implementation roadmap.
- Consider scalability, flexibility, and integration capabilities.
- Assess the total cost of ownership, including licensing, maintenance, and support.
- Review vendor reputation and customer feedback.
- Conduct pilot testing to validate technology choices, including options for devops implementation roadmap.
Selecting the appropriate technology ensures that the organization can effectively meet its objectives while optimizing resources. It also facilitates smoother integration with existing systems, enhancing overall efficiency. At Rapid Innovation, we guide our clients through this selection process, ensuring that they choose AI and Blockchain solutions that not only meet their current needs but also position them for future growth and greater ROI.
14.3. Pilot Program Design
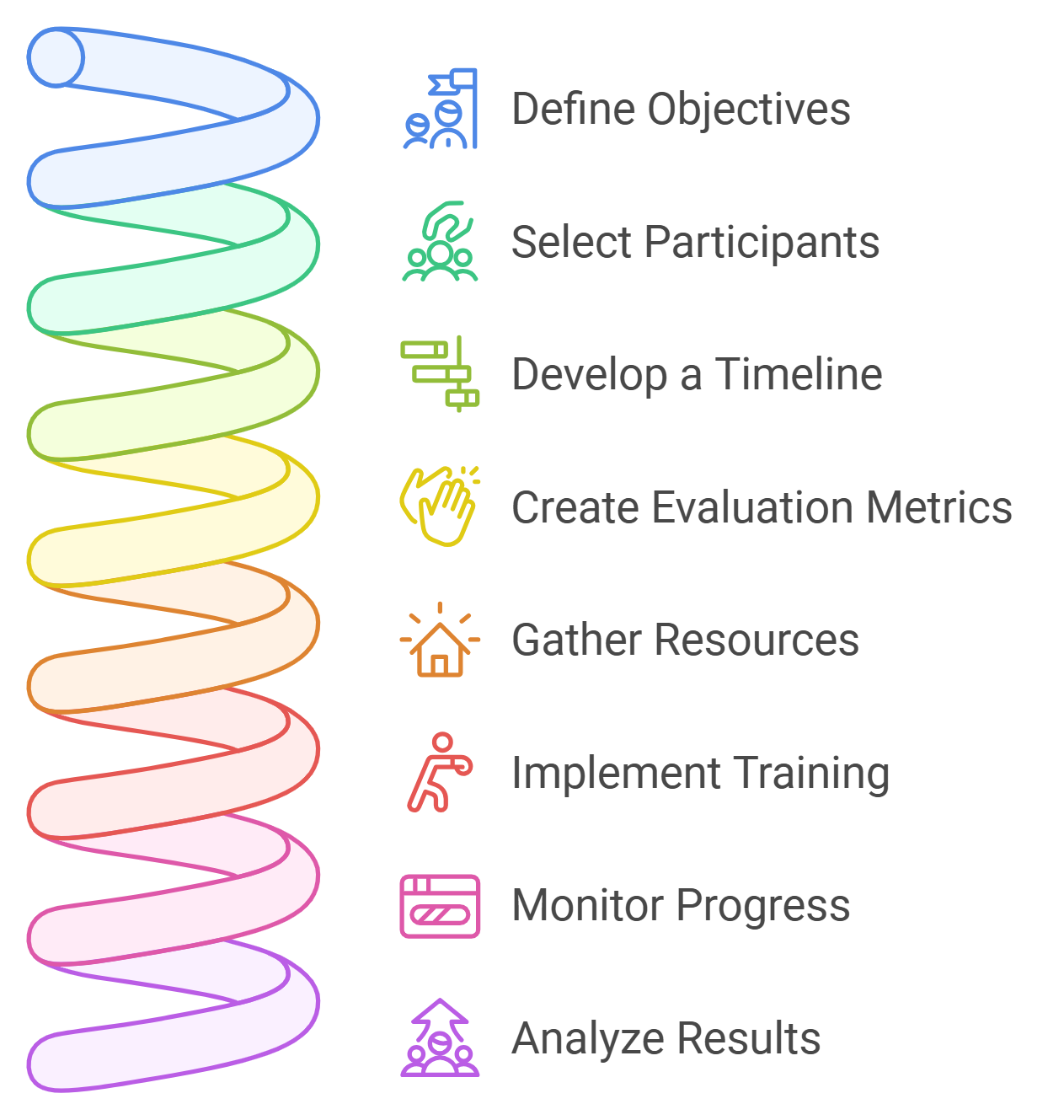
‍
A pilot program is a small-scale implementation of a project or initiative to test its feasibility, effectiveness, and potential for broader application. Designing a pilot program involves several key steps:
- Define Objectives: Clearly outline what the pilot aims to achieve. This could include testing new processes, technologies, or strategies, particularly in the realms of pilot program design, where innovation is rapid and requires careful validation.
- Select Participants: Choose a representative group of participants who will be involved in the pilot. This group should reflect the diversity of the larger population, ensuring that insights gained are applicable across various segments.
- Develop a Timeline: Establish a timeline for the pilot program, including start and end dates, milestones, and evaluation points. This structured approach is crucial for maintaining focus and ensuring timely feedback.
- Create Evaluation Metrics: Determine how success will be measured. This could include quantitative metrics (e.g., performance data) and qualitative feedback (e.g., participant satisfaction). In AI projects, metrics might focus on accuracy and efficiency, while Blockchain initiatives could emphasize security and transaction speed.
- Gather Resources: Identify the resources needed for the pilot, including personnel, technology, and budget. Leveraging Rapid Innovation's expertise in AI and Blockchain can help optimize resource allocation.
- Implement Training: Provide necessary training for participants to ensure they understand the pilot's objectives and their roles. This is especially important in technology-driven projects where user proficiency can significantly impact outcomes.
- Monitor Progress: Regularly check in on the pilot's progress, making adjustments as needed based on feedback and data collected. Continuous monitoring allows for agile responses to challenges that may arise.
- Analyze Results: After the pilot concludes, analyze the results against the defined objectives to determine its success and areas for improvement. This analysis can inform future projects and enhance overall ROI.
A well-designed pilot program can provide valuable insights and help mitigate risks before a full-scale rollout.
14.4. Scalability Considerations
Scalability is the ability of a system, process, or organization to grow and manage increased demand without compromising performance. When planning for scalability, consider the following factors:
- Infrastructure: Ensure that the existing infrastructure can support growth. This includes technology, facilities, and human resources, particularly in the context of AI and Blockchain solutions that may require robust systems.
- Process Efficiency: Streamline processes to eliminate bottlenecks. Efficient processes can handle increased volume without additional strain, which is essential for maintaining service quality as demand grows.
- Technology Solutions: Invest in scalable technology solutions that can grow with the organization. Cloud-based services, for example, can easily accommodate increased data and user demands, making them ideal for AI applications and Blockchain networks.
- Financial Resources: Assess the financial implications of scaling. Ensure that there is a budget in place to support growth initiatives, particularly in technology investments that can yield high returns.
- Market Demand: Analyze market trends and customer needs to ensure that scaling aligns with demand. This helps avoid overextending resources and ensures that growth is sustainable.
- Training and Development: Prepare staff for growth by investing in training and development. A skilled workforce is essential for managing increased workloads, especially in tech-driven environments.
- Feedback Mechanisms: Implement systems for ongoing feedback to identify areas for improvement as the organization scales. This iterative approach can enhance adaptability and responsiveness.
By addressing these considerations, organizations can effectively plan for growth and ensure that they are prepared to meet future demands.
14.5. Organizational Change Management
Organizational change management (OCM) is the process of managing the people side of change to achieve a required business outcome. Effective OCM involves several critical components:
- Communication: Develop a clear communication strategy to inform all stakeholders about the changes. Transparency helps build trust and reduces resistance, which is vital during transitions involving new technologies like AI and Blockchain.
- Stakeholder Engagement: Identify key stakeholders and involve them in the change process. Their input can provide valuable insights and foster buy-in, ensuring that the transition is smooth and well-received.
- Training and Support: Offer training programs to help employees adapt to new systems or processes. Ongoing support is crucial for easing the transition, particularly in complex technology implementations.
- Change Champions: Identify and empower change champions within the organization. These individuals can advocate for the change and help motivate others, facilitating a culture of innovation.
- Resistance Management: Anticipate resistance and develop strategies to address concerns. Understanding the reasons behind resistance can help in crafting effective responses, ensuring that the transition is as seamless as possible.
- Monitoring and Feedback: Establish mechanisms to monitor the change process and gather feedback. This allows for adjustments to be made in real-time, enhancing the overall effectiveness of the change initiative.
- Celebrating Success: Recognize and celebrate milestones and successes throughout the change process. This can boost morale and reinforce the benefits of the change, fostering a positive environment for future initiatives.
By focusing on these elements, organizations can navigate change more effectively, ensuring that transitions are smooth and that employees remain engaged and productive. Rapid Innovation is committed to guiding clients through these processes, leveraging our expertise in AI and Blockchain to drive successful outcomes.
14.6. Continuous Learning Strategy

‍
Continuous learning is an essential component of personal and professional development. It involves the ongoing, voluntary, and self-motivated pursuit of knowledge for personal or professional growth. A well-structured continuous learning strategy can significantly enhance an organization’s performance and employee satisfaction. Here are key elements to consider when developing a continuous learning strategy:
- Identify Learning Objectives
Define clear goals for what the organization aims to achieve through continuous learning. Align learning objectives with the overall business strategy to ensure relevance. Consider both individual and team learning needs. - Assess Current Skills and Knowledge Gaps
Conduct skills assessments to identify existing competencies and areas for improvement. Use surveys, interviews, and performance reviews to gather data on employee skills. Analyze the results to prioritize learning initiatives based on organizational needs. - Create a Learning Culture
Foster an environment that encourages curiosity and knowledge sharing. Promote the idea that learning is a lifelong journey, not just a one-time event. Recognize and reward employees who actively engage in learning activities. - Leverage Technology
Utilize e-learning platforms and Learning Management Systems (LMS) to provide accessible training resources. Incorporate mobile learning options to allow employees to learn on-the-go. Use data analytics to track learning progress and adapt strategies accordingly. - Offer Diverse Learning Opportunities
Provide a mix of formal and informal learning options, such as workshops, webinars, and mentorship programs. Encourage participation in industry conferences and networking events. Support self-directed learning through access to online courses and resources. - Encourage Collaboration and Knowledge Sharing
Create opportunities for employees to collaborate on projects and share insights. Establish communities of practice where employees can discuss challenges and solutions. Implement knowledge management systems to capture and disseminate valuable information. - Evaluate and Adapt Learning Programs
Regularly assess the effectiveness of learning initiatives through feedback and performance metrics. Be open to making adjustments based on employee input and changing business needs. Celebrate successes and learn from failures to continuously improve the learning strategy. - Support Leadership Development
Invest in leadership training to prepare future leaders within the organization. Encourage leaders to model continuous learning behaviors and support their teams in pursuing development opportunities. Create succession plans that include learning pathways for high-potential employees. - Promote Work-Life Balance
Recognize that employees have varying commitments outside of work, and offer flexible learning options. Encourage employees to integrate learning into their daily routines without overwhelming them. Provide resources that help employees manage their time effectively while pursuing learning goals. - Measure Impact on Performance
Establish key performance indicators (KPIs) to evaluate the impact of learning initiatives on business outcomes. Use metrics such as employee engagement, retention rates, and productivity levels to assess effectiveness. Share results with stakeholders to demonstrate the value of continuous learning investments.
By implementing a comprehensive continuous learning strategy, organizations can enhance employee engagement, improve retention rates, and drive innovation. Continuous learning not only benefits individual employees but also contributes to the overall success and adaptability of the organization in a rapidly changing business landscape.
At Rapid Innovation, we understand that integrating a continuous learning strategy with AI and Blockchain technologies can further amplify its effectiveness. For instance, utilizing AI-driven analytics can help identify skill gaps more accurately and tailor learning paths to individual needs, ensuring that your workforce is equipped with the latest knowledge and skills. Additionally, Blockchain can enhance the credibility of learning achievements through secure, verifiable credentials, fostering a culture of trust and recognition within your organization. By leveraging these technologies, we can help you achieve greater ROI and drive sustainable growth.