Table Of Contents
Category
Artificial Intelligence
Blockchain
Security
Oil and Gas
1. Introduction to Grid Stability and AI Integration
Grid stability is a critical aspect of modern electrical systems, ensuring that the supply of electricity meets the demand in real-time. As renewable energy sources like wind and solar become more prevalent, maintaining grid stability has become increasingly complex. This is where Artificial Intelligence (AI) in grid stability comes into play, offering innovative solutions to enhance grid management.
- Definition of grid stability: Â
- The ability of the power system to maintain a balance between supply and demand.
- Involves frequency control, voltage regulation, and system reliability.
- Importance of grid stability: Â
- Prevents blackouts and system failures.
- Ensures a reliable power supply for consumers and industries.
- Supports the integration of renewable energy sources.
- Role of AI in grid stability: Â
- AI algorithms can analyze vast amounts of data from various sources, including weather forecasts, energy consumption patterns, and grid performance metrics. Machine learning models can predict demand fluctuations and optimize energy distribution accordingly. Additionally, AI can facilitate real-time monitoring and control of grid operations, enhancing responsiveness to disturbances. At Rapid Innovation, we leverage these AI capabilities to help clients improve their grid management systems, ultimately leading to greater operational efficiency and ROI.
- Key AI technologies used in grid management: Â
- Predictive analytics for demand forecasting.
- Reinforcement learning for optimizing grid operations.
- Neural networks for anomaly detection and fault prediction.
The integration of AI in grid stability efforts presents both significant benefits and challenges, which will be explored in the following sections. By partnering with Rapid Innovation, clients can harness these advanced technologies to achieve their business goals effectively and efficiently.
1.1. Understanding Power Grid Dynamics
Power grid dynamics refer to the behavior and interactions of various components within an electrical grid. This includes generation, transmission, distribution, and consumption of electricity. Understanding these dynamics is crucial for maintaining grid stability and reliability.
- The power grid consists of interconnected networks that deliver electricity from producers to consumers. Â
- Key components include power plants, transformers, transmission lines, and substations. Â
- Grid dynamics are influenced by factors such as load demand, generation capacity, and environmental conditions. Â
- Real-time monitoring and control are essential for managing fluctuations in supply and demand, which is where grid management software plays a vital role. Â
- The integration of renewable energy sources, like solar and wind, adds complexity to grid dynamics due to their variable nature. Â
- Effective grid management requires advanced forecasting techniques to predict demand and generation patterns, often facilitated by grid management systems. Â
1.2. Evolution of Grid Management Systems
Grid management systems have evolved significantly over the years, driven by technological advancements and the need for more efficient energy distribution.
- Early grid management relied on manual processes and basic monitoring tools. Â
- The introduction of Supervisory Control and Data Acquisition (SCADA) systems marked a significant advancement, allowing for real-time data collection and control. Â
- Modern grid management systems incorporate advanced analytics, automation, and communication technologies, including smart grid management software. Â
- Smart grid technologies enable two-way communication between utilities and consumers, enhancing demand response capabilities. Â
- The integration of distributed energy resources (DERs) has necessitated more sophisticated management systems to balance supply and demand, leading to the development of distributed grid management solutions. Â
- Regulatory changes and the push for sustainability have accelerated the adoption of innovative grid management solutions, including energy grid management software and outage management systems in smart grids. Â
1.3. Role of AI in Modern Grid Operations
Artificial Intelligence (AI) plays a transformative role in modern grid operations, enhancing efficiency, reliability, and decision-making processes.
- AI algorithms analyze vast amounts of data from various sources, improving predictive maintenance and fault detection. Â
- Machine learning models can forecast energy demand and generation, allowing for better resource allocation, which is crucial for power grid management. Â
- AI-driven optimization techniques help in managing grid operations, reducing operational costs, and improving service reliability. Â
- Intelligent automation systems can respond to grid disturbances in real-time, minimizing downtime and enhancing resilience, particularly in outage management systems for smart grids. Â
- AI applications in grid management include demand response programs, energy storage optimization, and grid security measures, all of which are integral to smart grid management systems. Â
- The use of AI is expected to grow, with projections indicating that AI in the energy sector could reach a market value of $7.78 billion by 2025.
At Rapid Innovation, we leverage our expertise in AI to develop tailored solutions that enhance grid operations. By implementing AI-driven analytics and optimization tools, we help clients achieve greater ROI through improved efficiency and reduced operational costs. Our consulting services guide organizations in integrating these advanced technologies into their existing systems, ensuring a seamless transition to smarter grid management.
1.4. Current Challenges in Grid Stability
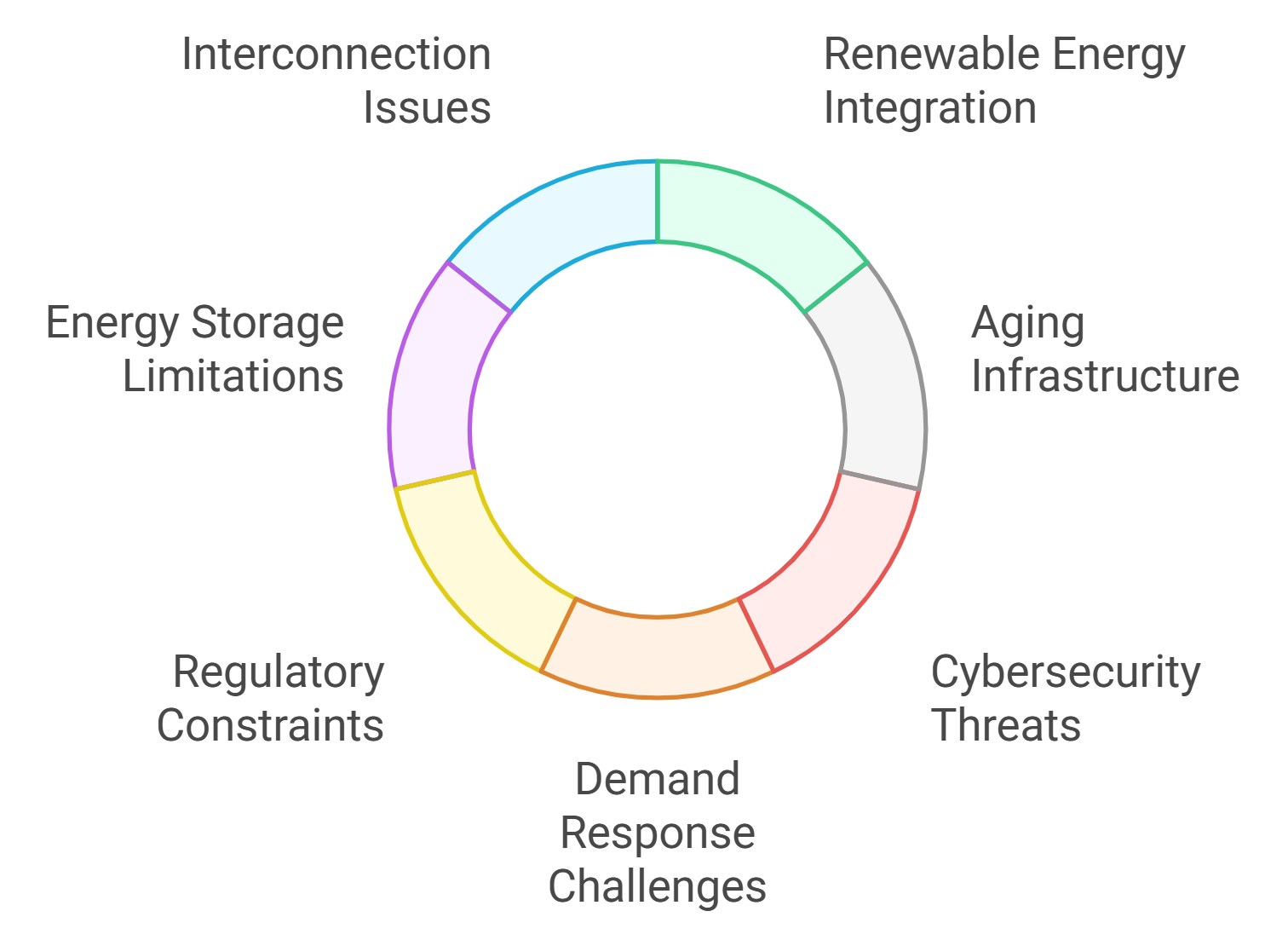
‍
Grid stability is a critical aspect of power systems, ensuring that electricity supply meets demand without interruptions. However, several grid stability challenges threaten this stability:
- Increased Renewable Energy Integration: The rise of renewable energy sources, such as wind and solar, introduces variability and unpredictability. Unlike traditional power plants, renewables depend on weather conditions, making it difficult to maintain a consistent power supply.
- Aging Infrastructure: Many power grids are built on outdated infrastructure that struggles to handle modern demands. Aging equipment can lead to failures and outages, impacting overall grid reliability.
- Cybersecurity Threats: As power systems become more digitized, they are increasingly vulnerable to cyberattacks. A successful breach can disrupt grid operations, leading to widespread outages and safety concerns.
- Demand Response Challenges: The growing demand for electricity, especially during peak hours, poses a challenge. Implementing effective demand response strategies is essential to balance supply and demand, but it can be complex to manage.
- Regulatory and Market Constraints: Regulatory frameworks often lag behind technological advancements. This can hinder the adoption of innovative solutions necessary for maintaining grid stability.
- Energy Storage Limitations: While energy storage technologies, such as batteries, are advancing, they still face challenges in terms of cost, capacity, and efficiency. These limitations can restrict the ability to store excess energy generated from renewables.
- Interconnection Issues: As grids become more interconnected, the complexity of managing these networks increases. Coordination between different regions and systems is essential to ensure stability but can be difficult to achieve.
Addressing these grid stability challenges requires a multifaceted approach, including investment in infrastructure, enhanced cybersecurity measures, and the integration of advanced technologies.
2. Fundamentals of AI Agents in Power Systems
Artificial Intelligence (AI) is transforming power systems by enhancing efficiency, reliability, and decision-making processes. AI agents are software programs that can perform tasks autonomously, learning from data and adapting to changing conditions. In power systems, AI agents play a crucial role in optimizing grid management.
- Data Analysis: AI agents can analyze vast amounts of data from various sources, including sensors and smart meters. This capability allows for real-time monitoring and predictive analytics, improving decision-making.
- Automation: AI agents can automate routine tasks, such as load forecasting and fault detection. This reduces the need for human intervention, allowing operators to focus on more complex issues.
- Optimization: AI algorithms can optimize energy distribution and consumption, ensuring that resources are used efficiently. This is particularly important in managing the integration of renewable energy sources.
- Predictive Maintenance: By analyzing historical data, AI agents can predict equipment failures before they occur. This proactive approach to maintenance can reduce downtime and extend the lifespan of grid infrastructure.
- Enhanced Security: AI can help identify and mitigate cybersecurity threats by monitoring network activity and detecting anomalies. This is crucial for protecting the integrity of power systems.
2.1. Types of AI Agents for Grid Management
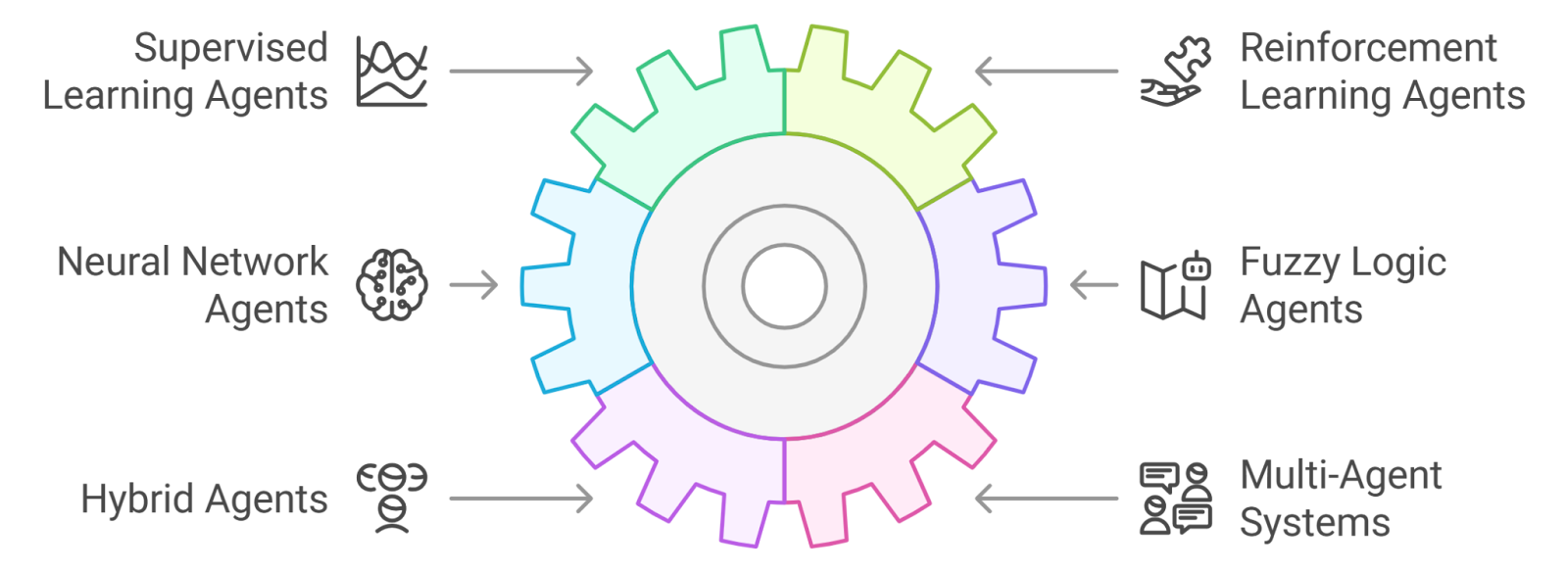
‍
AI agents can be categorized based on their functions and applications in grid management. Understanding these types can help utilities and operators implement the most effective solutions.
- Supervised Learning Agents: These agents are trained on labeled datasets to make predictions or classifications. They are useful for tasks such as load forecasting and demand prediction, where historical data can inform future trends.
- Reinforcement Learning Agents: These agents learn through trial and error, receiving feedback from their environment. They are particularly effective in optimizing grid operations, such as energy dispatch and demand response strategies.
- Neural Network Agents: Utilizing deep learning techniques, these agents can model complex relationships within data. They are beneficial for tasks like fault detection and predictive maintenance, where patterns may not be immediately apparent.
- Fuzzy Logic Agents: These agents use fuzzy logic to handle uncertainty and imprecision in data. They are useful in scenarios where traditional binary logic falls short, such as in managing the variability of renewable energy sources.
- Hybrid Agents: Combining multiple AI techniques, hybrid agents can leverage the strengths of different approaches. For example, a hybrid agent might use supervised learning for forecasting while employing reinforcement learning for real-time optimization.
- Multi-Agent Systems: These systems consist of multiple AI agents that can communicate and collaborate. They are effective in decentralized grid management, where different agents can manage local resources while coordinating with the overall grid.
By leveraging these various types of AI agents, power systems can enhance their operational efficiency, improve grid stability, and better integrate renewable energy sources. At Rapid Innovation, we specialize in developing tailored AI solutions that address these grid stability challenges, ensuring that our clients achieve greater ROI through improved grid management and operational resilience. For more information on the benefits and challenges of AI agents in energy management, visit this link.
2.1.1. Monitoring Agents
Monitoring agents are essential components in various systems, particularly in IT and network management. Their primary function is to observe and report on the status of different processes, applications, or systems.
- They continuously track performance metrics, such as CPU usage, memory consumption, and network traffic.
- Monitoring agents can detect anomalies or deviations from normal operating conditions, allowing for timely intervention.
- They often provide real-time alerts to system administrators, enabling quick responses to potential issues.
- These agents can be deployed across various environments, including cloud, on-premises, and hybrid systems.
- Common tools that utilize monitoring agents include Nagios, Zabbix, and Prometheus, as well as specific solutions like Datadog agent, Microsoft monitoring, Azure monitor agent, and Ninja RMM agent.
The data collected by monitoring agents can be invaluable for performance tuning and capacity planning. By analyzing historical data, organizations can make informed decisions about resource allocation and system upgrades. At Rapid Innovation, we leverage our expertise in AI to enhance monitoring capabilities, ensuring that our clients can achieve greater operational efficiency and ROI through proactive management. Tools such as Telegraf InfluxDB and NSClient++ are also utilized to gather and analyze monitoring data effectively.
2.1.2. Control Agents
Control agents play a crucial role in managing and regulating systems based on the data they receive from monitoring agents. Their primary function is to execute commands or adjustments to maintain optimal performance and ensure system stability.
- They can automate responses to specific conditions, such as scaling resources up or down based on demand.
- Control agents often work in conjunction with monitoring agents to create a feedback loop, where data informs actions.
- They can enforce policies and compliance measures, ensuring that systems adhere to organizational standards.
- Control agents are commonly used in cloud environments to manage resources dynamically, optimizing costs and performance.
- Examples of control agents include AWS Auto Scaling, Kubernetes controllers, and Ansible.
By implementing control agents, organizations can achieve greater efficiency and reduce the risk of human error in system management. This automation leads to improved uptime and better resource utilization. Rapid Innovation assists clients in integrating these control agents into their systems, ensuring that they can respond to changing demands swiftly and effectively.
2.1.3. Predictive Agents
Predictive agents utilize advanced algorithms and machine learning techniques to forecast future events or trends based on historical data. Their primary goal is to anticipate issues before they occur, allowing organizations to take proactive measures.
- They analyze patterns in data to identify potential failures or performance bottlenecks.
- Predictive agents can enhance decision-making by providing insights into future resource needs and system behavior.
- They are particularly valuable in industries such as finance, healthcare, and manufacturing, where timely interventions can prevent significant losses.
- These agents often integrate with monitoring and control agents to create a comprehensive management system.
- Tools that employ predictive analytics include IBM Watson, Microsoft Azure Machine Learning, and Google Cloud AI.
By leveraging predictive agents, organizations can shift from reactive to proactive management strategies, ultimately leading to improved operational efficiency and reduced downtime. At Rapid Innovation, we empower our clients to harness the power of predictive analytics, enabling them to make data-driven decisions that enhance their overall business performance and ROI.
2.1.4. Optimization Agents
Optimization agents are essential components in various systems, particularly in artificial intelligence and machine learning. They are designed to enhance performance by making decisions that lead to the best possible outcomes based on specific criteria.
- Definition: Optimization agents are algorithms or software that analyze data and make adjustments to improve efficiency and effectiveness.
- Functionality: They operate by evaluating multiple variables and constraints to find the optimal solution for a given problem.
- Applications: Commonly used in fields such as logistics, finance, and marketing, optimization agents help in: Â
- Resource allocation
- Route planning
- Inventory management
At Rapid Innovation, we leverage optimization agents to help our clients streamline operations and maximize their return on investment (ROI). For instance, in logistics, our optimization algorithms can significantly reduce transportation costs by determining the most efficient routes, leading to faster delivery times and lower fuel expenses.
- Types: Various types of optimization agents exist, including: Â
- Genetic algorithms
- Simulated annealing
- Gradient descent
- Benefits: The use of optimization agents can lead to: Â
- Cost reduction
- Time savings
- Improved decision-making processes
By implementing these agents, our clients have experienced substantial improvements in operational efficiency. For example, a retail client utilized our optimization solutions to manage inventory levels more effectively, resulting in a 20% reduction in holding costs.
- Challenges: Despite their advantages, optimization agents face challenges such as:
- Complexity in modeling real-world scenarios
- Computational limitations
- The need for high-quality data inputs High-quality data is essential for accurate analysis.
2.2. Core Technologies and Frameworks
Core technologies and frameworks form the backbone of modern software development and data processing. They provide the necessary tools and structures to build, deploy, and manage applications effectively.
- Definition: Core technologies refer to the fundamental tools and systems that enable software functionality, while frameworks offer a structured approach to application development.
- Key Technologies: Some of the most widely used core technologies include: Â
- Programming languages (e.g., Python, Java, JavaScript)
- Databases (e.g., SQL, NoSQL)
- Cloud computing platforms (e.g., AWS, Azure)
- Popular Frameworks: Frameworks streamline development processes and enhance productivity. Notable frameworks include: Â
- Django for Python web development
- React for building user interfaces
- TensorFlow for machine learning applications
At Rapid Innovation, we utilize these core technologies and frameworks to develop robust AI and blockchain solutions tailored to our clients' needs. This approach not only accelerates development cycles but also ensures that the applications we build are scalable and maintainable.
- Importance: Utilizing the right technologies and frameworks can lead to: Â
- Faster development cycles
- Enhanced scalability
- Improved maintainability of applications
- Trends: Emerging trends in core technologies and frameworks include: Â
- Microservices architecture
- Serverless computing
- Containerization with Docker and Kubernetes
2.3. Data Requirements and Sources
Data is the lifeblood of any analytical or machine learning project. Understanding data requirements and identifying reliable sources is crucial for successful outcomes.
- Definition: Data requirements refer to the specific types and quantities of data needed to achieve project goals, while data sources are the origins from which this data is obtained.
- Types of Data: Different types of data may be required, including: Â
- Structured data (e.g., databases, spreadsheets)
- Unstructured data (e.g., text, images, videos)
- Semi-structured data (e.g., JSON, XML)
- Data Quality: High-quality data is essential for accurate analysis. Key aspects of data quality include: Â
- Accuracy
- Completeness
- Consistency
At Rapid Innovation, we emphasize the importance of data quality in our projects. By ensuring that our clients have access to high-quality data, we help them achieve more accurate insights and better decision-making.
- Sources of Data: Common sources for obtaining data include: Â
- Public datasets (e.g., government databases, research institutions)
- Web scraping (extracting data from websites)
- APIs (application programming interfaces) for accessing data from other applications
- Data Privacy: It is crucial to consider data privacy and compliance with regulations such as GDPR when collecting and using data.
- Tools for Data Management: Various tools can assist in managing data requirements, including: Â
- Data warehousing solutions (e.g., Snowflake, Redshift)
- ETL (Extract, Transform, Load) tools (e.g., Apache NiFi, Talend)
- Data visualization tools (e.g., Tableau, Power BI)
By leveraging these tools, Rapid Innovation ensures that our clients can effectively manage their data, leading to enhanced analytical capabilities and improved business outcomes.
2.4. Integration with Existing Grid Infrastructure
Integrating AI integration in power systems with existing grid infrastructure is crucial for enhancing the efficiency and reliability of power systems. This integration involves several key components:
- Compatibility with Legacy Systems: AI solutions must be designed to work seamlessly with older grid technologies. This ensures that utilities can leverage existing investments while upgrading their systems.
- Data Interoperability: AI agents require access to vast amounts of data from various sources, including sensors, smart meters, and SCADA systems. Ensuring that these data sources can communicate effectively is essential for real-time decision-making.
- Scalability: The integration process should allow for scalability, enabling utilities to expand their AI capabilities as needed. This means that the infrastructure can accommodate additional AI tools and applications without significant overhauls.
- Cybersecurity Measures: As AI agents are integrated into the grid, robust cybersecurity protocols must be established to protect sensitive data and prevent unauthorized access. This is particularly important given the increasing number of cyber threats targeting energy infrastructure.
- Regulatory Compliance: Utilities must ensure that their AI integration efforts comply with local and national regulations. This includes adhering to standards for data privacy, grid reliability, and environmental impact.
- Stakeholder Engagement: Successful integration requires collaboration among various stakeholders, including utility companies, technology providers, and regulatory bodies. Engaging these groups early in the process can help identify potential challenges and streamline implementation.
- Pilot Programs: Before full-scale deployment, pilot programs can be beneficial. These allow utilities to test AI solutions in controlled environments, gather data, and refine their approaches based on real-world performance.
3. Key Benefits of AI Agents in Grid Stability
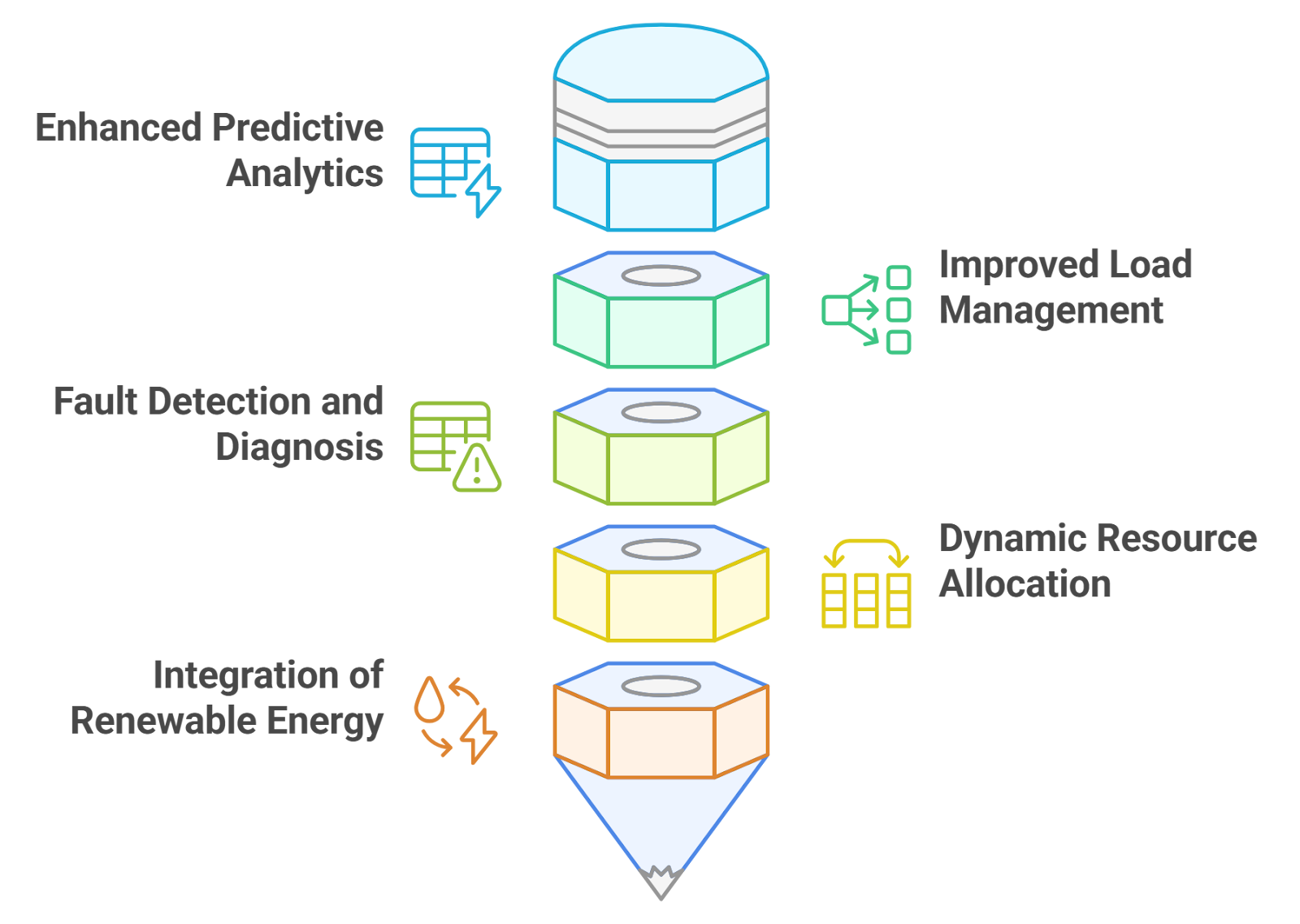
‍
AI agents play a pivotal role in enhancing grid stability, offering numerous benefits that contribute to a more resilient energy system. Some of the key advantages include:
- Enhanced Predictive Analytics: AI can analyze historical data to predict potential outages or disruptions, allowing utilities to take proactive measures.
- Improved Load Management: AI agents can optimize load distribution across the grid, ensuring that energy supply meets demand efficiently.
- Fault Detection and Diagnosis: AI systems can quickly identify faults in the grid, reducing response times and minimizing downtime.
- Dynamic Resource Allocation: AI can facilitate real-time adjustments in resource allocation, ensuring that energy is directed where it is needed most.
- Integration of Renewable Energy: AI helps manage the variability of renewable energy sources, ensuring that they can be effectively integrated into the grid without compromising stability.
3.1. Real-time Monitoring and Response
Real-time monitoring and response are critical components of maintaining grid stability, and AI agents excel in these areas. The benefits of real-time capabilities include:
- Instantaneous Data Analysis: AI agents can process vast amounts of data from sensors and smart devices in real-time, enabling immediate insights into grid performance.
- Automated Decision-Making: With AI, utilities can automate responses to certain conditions, such as rerouting power during an outage or adjusting load in response to demand spikes.
- Enhanced Situational Awareness: AI provides operators with a comprehensive view of the grid, allowing for better situational awareness and quicker decision-making.
- Predictive Maintenance: By continuously monitoring equipment health, AI can predict when maintenance is needed, reducing the likelihood of unexpected failures.
- Adaptive Control Systems: AI agents can adjust control strategies dynamically based on real-time conditions, improving overall grid resilience.
- Reduced Human Error: Automating monitoring and response processes minimizes the risk of human error, which can lead to costly mistakes in grid management.
- Faster Recovery from Disruptions: In the event of a disturbance, AI can quickly analyze the situation and implement recovery strategies, significantly reducing downtime.
By leveraging AI for real-time monitoring and response, utilities can enhance grid stability, improve operational efficiency, and ensure a reliable energy supply for consumers. At Rapid Innovation, we specialize in integrating these advanced AI solutions into existing infrastructures, helping our clients achieve greater ROI through improved efficiency and reduced operational costs.
3.2. Predictive Maintenance
Predictive maintenance is a proactive approach that leverages data analytics and machine learning to predict equipment failures before they occur. This strategy is essential in industries such as manufacturing, energy, and transportation, where equipment downtime can lead to significant financial losses.
- Utilizes sensors and IoT devices to collect real-time data on equipment performance, a key aspect of IoT predictive maintenance. Â
- Analyzes historical data to identify patterns and predict potential failures, which is crucial for effective predictive maintenance solutions. Â
- Reduces maintenance costs by addressing issues before they escalate, a benefit highlighted by companies specializing in predictive maintenance. Â
- Increases equipment lifespan and reliability through timely interventions, which is a primary goal of predictive maintenance companies. Â
- Enhances operational efficiency by minimizing unplanned downtime, a significant advantage of implementing IoT for predictive maintenance. Â
At Rapid Innovation, we specialize in implementing predictive maintenance solutions that can lead to substantial cost savings. According to a report by Deloitte, organizations that adopt predictive maintenance can reduce maintenance costs by up to 30% and increase productivity by 25%. By integrating our AI-driven analytics and machine learning capabilities, we empower clients to optimize their maintenance strategies, ultimately achieving greater ROI. Our expertise includes working with platforms like AWS predictive maintenance, IBM predictive maintenance, and SAP predictive maintenance, ensuring we provide tailored solutions that meet diverse client needs.
3.3. Load Forecasting and Balancing
Load forecasting and balancing are critical components of energy management systems. Accurate load forecasting helps utilities predict electricity demand, ensuring that supply meets demand efficiently.
- Involves analyzing historical consumption data to forecast future energy needs. Â
- Utilizes advanced algorithms and machine learning models for improved accuracy. Â
- Helps in optimizing energy generation and distribution, reducing waste. Â
- Supports grid stability by balancing supply and demand in real-time. Â
- Facilitates better planning for renewable energy sources, which can be variable. Â
Effective load forecasting can significantly enhance grid reliability. According to the U.S. Energy Information Administration, accurate load forecasting can improve operational efficiency by up to 15%. At Rapid Innovation, we leverage our expertise in AI to develop tailored forecasting models that help clients optimize their energy management, ensuring they meet demand while minimizing costs.
3.4. Renewable Energy Integration
Renewable energy integration refers to the process of incorporating renewable energy sources, such as solar and wind, into the existing energy grid. This transition is vital for reducing carbon emissions and promoting sustainable energy practices.
- Involves the use of smart grid technologies to manage variable energy sources. Â
- Requires advanced energy storage solutions to balance supply and demand. Â
- Promotes energy efficiency and reduces reliance on fossil fuels. Â
- Encourages the development of microgrids for localized energy generation. Â
- Supports government policies aimed at increasing renewable energy adoption. Â
The integration of renewable energy is not without challenges. However, studies show that with proper planning and technology, it is possible to achieve a high percentage of renewable energy in the grid. According to the International Renewable Energy Agency, countries can integrate up to 80% renewable energy into their grids without compromising reliability. At Rapid Innovation, we provide consulting and development services that facilitate this transition, helping clients navigate the complexities of renewable energy integration while maximizing their investment in sustainable practices.
3.5. Emergency Response Management
Emergency response management is a critical component of any organization’s operational strategy. It involves the planning, coordination, and execution of actions to address emergencies effectively, including natural disasters, industrial accidents, or public health crises.
- Establishing a clear emergency response plan is essential. This plan should outline roles, responsibilities, and procedures for various scenarios, ensuring that all team members are aligned and prepared. This includes understanding the incident command system roles and responsibilities, which are vital for effective coordination.
- Regular training and drills for staff ensure that everyone knows their role during an emergency. This preparedness can significantly reduce response times and improve outcomes, ultimately safeguarding both personnel and assets. Training should also cover the hospital incident command system to ensure readiness in healthcare settings.
- Communication is key during emergencies. Organizations should have a reliable communication system in place to disseminate information quickly to all stakeholders, minimizing confusion and enhancing coordination. This is particularly important in emergency response planning and during hazardous materials incidents.
- Collaboration with local emergency services and agencies can enhance response efforts. Building relationships with these entities can facilitate quicker assistance and resource sharing, leveraging community resources for a more effective response. Engaging with ESF emergency management can provide additional support and resources.
- Continuous evaluation and improvement of emergency response plans are necessary. After each drill or actual event, organizations should review their performance and make necessary adjustments, ensuring that their strategies evolve with changing circumstances. This includes assessing the effectiveness of disaster response planning and the roles of hospital emergency response teams. For organizations looking to enhance their emergency response capabilities, exploring AI agents for incident resolution workflow can provide innovative tools and strategies.
3.6. Cost Optimization
Cost optimization is the process of reducing expenses while maintaining or improving the quality of services and products. It is vital for organizations looking to enhance profitability and efficiency.
- Conducting a thorough analysis of current expenditures helps identify areas where costs can be reduced, including reviewing supplier contracts, operational processes, and staffing levels. This analysis can reveal hidden inefficiencies that can be addressed.
- Implementing technology solutions can streamline operations and reduce labor costs. Automation of repetitive tasks can free up resources for more strategic initiatives, allowing organizations to focus on growth and innovation.
- Regularly benchmarking against industry standards can provide insights into potential savings. Understanding where your organization stands compared to competitors can highlight areas for improvement and drive competitive advantage.
- Encouraging a culture of cost awareness among employees can lead to innovative ideas for savings. Employees often have valuable insights into inefficiencies that can be addressed, fostering a collaborative environment for continuous improvement.
- Utilizing data analytics can help in making informed decisions about resource allocation. By analyzing spending patterns, organizations can identify trends and adjust their strategies accordingly, ensuring that resources are used effectively.
4. Technical Implementation
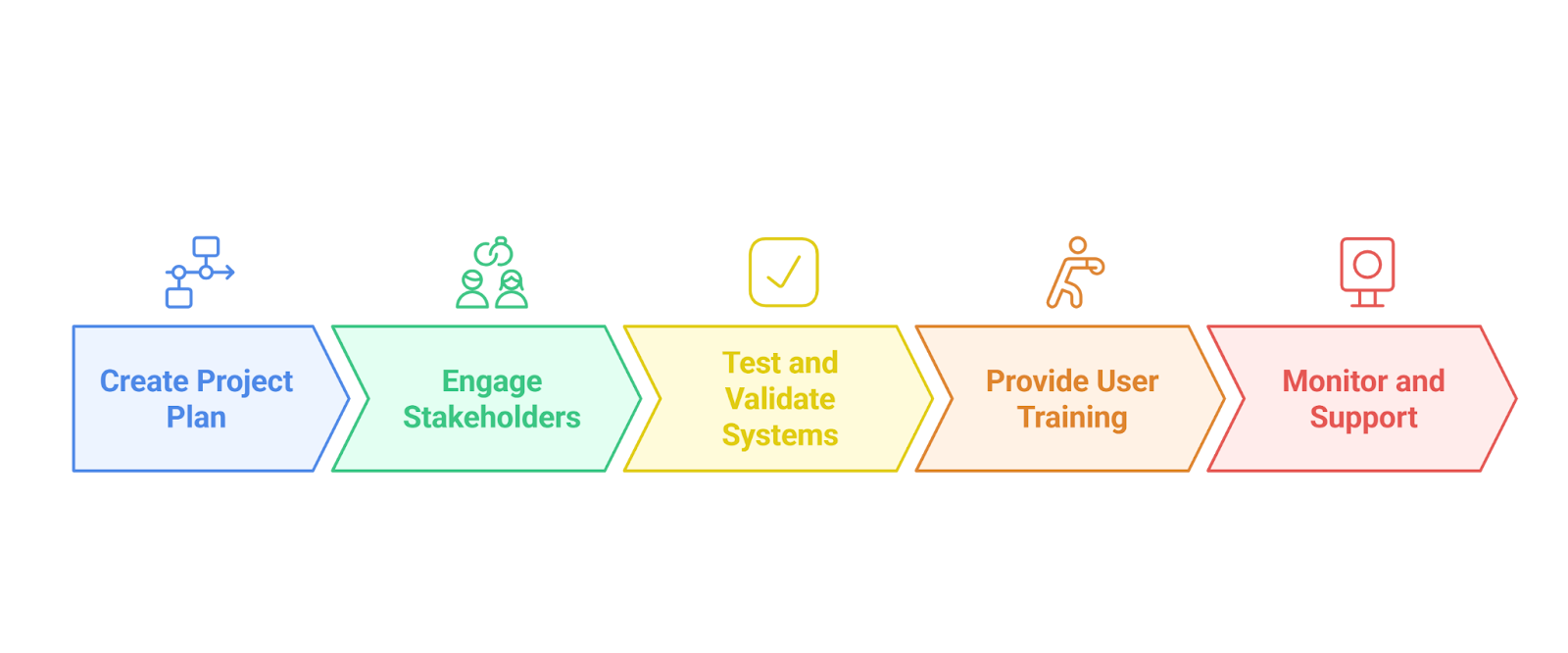
‍
Technical implementation refers to the process of putting technology solutions into practice within an organization. This phase is crucial for ensuring that systems and processes function as intended.
- A clear project plan is essential for successful technical implementation. This plan should outline timelines, milestones, and responsibilities for all team members involved, ensuring accountability and clarity throughout the process.
- Engaging stakeholders early in the process can help in identifying requirements and potential challenges. Their input can lead to a more tailored solution that meets the organization’s needs, enhancing buy-in and support.
- Testing and validation of systems before full-scale deployment is critical. This helps in identifying any issues that need to be addressed, ensuring a smoother transition and minimizing disruptions.
- Providing adequate training for users is vital for adoption. Employees should feel comfortable using new technologies to maximize their benefits, which can lead to increased productivity and satisfaction.
- Continuous monitoring and support after implementation can help in addressing any issues that arise. This ongoing support ensures that the technology remains effective and relevant to the organization’s goals, ultimately driving greater ROI and operational efficiency.
At Rapid Innovation, we leverage our expertise in AI and Blockchain to enhance these processes, ensuring that our clients not only meet their operational goals but also achieve significant returns on their investments through innovative solutions tailored to their unique challenges.
4.1. Agent Architecture Design
Agent architecture design is a critical aspect of developing intelligent systems. It involves structuring the components of an agent to ensure efficient operation, adaptability, and scalability. The design can significantly influence how agents interact with their environment, process information, and collaborate with other agents. Key considerations in agent architecture design include the choice between centralized and distributed approaches, as well as the communication protocols that facilitate interaction.
4.1.1. Centralized vs. Distributed Approaches
The choice between centralized and distributed architectures is fundamental in agent architecture design. Each approach has its advantages and disadvantages, impacting performance, scalability, and fault tolerance.
- Centralized Approaches: Â
- In a centralized architecture, a single agent or a central server manages all operations. This design simplifies coordination and control, making it easier to implement complex decision-making processes. Centralized systems can be more efficient in terms of resource allocation since all data is processed in one location. However, they can become bottlenecks, leading to performance issues as the number of agents increases. Centralized systems are also more vulnerable to single points of failure, which can compromise the entire system.
- Distributed Approaches: Â
- Distributed architectures consist of multiple agents that operate independently but can collaborate when necessary. This design enhances scalability, as new agents can be added without significantly affecting the overall system. Distributed systems are more resilient to failures; if one agent fails, others can continue functioning. They can also reduce latency since processing can occur closer to the data source. However, distributed systems can be more complex to manage, requiring robust coordination and communication mechanisms.
4.1.2. Communication Protocols
Communication protocols are essential for enabling agents to share information and coordinate their actions. The choice of protocol can significantly affect the efficiency and effectiveness of agent interactions.
- Types of Communication Protocols: Â
- Message Passing: Agents send and receive messages to communicate. This method is straightforward and allows for asynchronous communication.
- Remote Procedure Calls (RPC): This protocol allows agents to invoke functions on remote agents as if they were local, simplifying interaction.
- Publish/Subscribe: In this model, agents can subscribe to specific topics and receive updates when relevant information is published. This approach is useful for decoupling agents and enhancing scalability.
- Factors to Consider: Â
- Latency: The time it takes for messages to be sent and received can impact the responsiveness of the system.
- Bandwidth: The amount of data that can be transmitted affects the volume of information agents can share.
- Reliability: Protocols must ensure that messages are delivered accurately and in the correct order, especially in critical applications.
- Popular Protocols: Â
- Agent Communication Language (ACL): A standard for agent communication that allows for the exchange of messages in a structured format.
- Foundation for Intelligent Physical Agents (FIPA): A set of standards that define how agents should communicate and interact in a multi-agent system.
- WebSocket: A protocol that enables real-time communication between agents over the web, suitable for applications requiring low latency.
In conclusion, the design of agent architecture, including the choice between centralized and distributed approaches and the selection of communication protocols, plays a vital role in the performance and effectiveness of intelligent systems. Understanding these elements is crucial for developing robust and scalable agent-based applications. At Rapid Innovation, we leverage our expertise in AI and Blockchain to help clients implement the most suitable agent architecture designs tailored to their specific business needs, ultimately driving greater ROI through enhanced operational efficiency and adaptability.
4.1.3. Security Framework
A security framework is a structured approach that organizations use to manage and mitigate risks associated with information security. It provides guidelines, best practices, and standards to protect sensitive data and ensure compliance with regulations.
- Key components of a security framework include:
- Risk Assessment: Identifying and evaluating risks to determine their potential impact on the organization.
- Policies and Procedures: Establishing clear guidelines for data protection, incident response, and user access.
- Security Controls: Implementing technical measures such as firewalls, encryption, and intrusion detection systems to safeguard information.
- Compliance: Ensuring adherence to legal and regulatory requirements, such as GDPR or HIPAA.
- Training and Awareness: Educating employees about security best practices and potential threats.
Organizations often adopt established frameworks such as the NIST Cybersecurity Framework, NIST Cyber, NIST CSF, NIST CSF 2.0, or COBIT to create a robust security posture. These frameworks help in aligning security strategies with business objectives and improving overall resilience against cyber threats. The NIST Cybersecurity Framework and NIST 800 53 Rev 5 provide comprehensive guidelines for managing cybersecurity risks. Additionally, organizations may implement the CIS Critical Security Controls or the Critical Security Controls to enhance their security measures. At Rapid Innovation, we leverage our expertise in AI and Blockchain to enhance these security frameworks, ensuring that our clients not only meet compliance requirements but also protect their sensitive data against evolving cyber threats, including those outlined in the NIST 800 37 and NIST 800 30. For more information on how AI and machine learning can assist with regulatory compliance, visit this link.
4.2. Machine Learning Models
Machine learning models are algorithms that enable computers to learn from data and make predictions or decisions without being explicitly programmed. These models are essential in various applications, from finance to healthcare, and are categorized into different types based on their learning approach.
- Common types of machine learning models include:
- Supervised Learning: Models learn from labeled data, where the input-output pairs are known. They are used for classification and regression tasks.
- Unsupervised Learning: Models identify patterns in data without labeled outputs. They are often used for clustering and association tasks.
- Reinforcement Learning: Models learn by interacting with an environment and receiving feedback in the form of rewards or penalties.
Machine learning models are increasingly being integrated into business processes to enhance decision-making, automate tasks, and improve efficiency. The choice of model depends on the specific problem, the nature of the data, and the desired outcome.
4.2.1. Supervised Learning Applications
Supervised learning is a prominent machine learning approach where models are trained on labeled datasets. This method is widely used across various industries due to its effectiveness in making accurate predictions.
- Key applications of supervised learning include:
- Image Recognition: Identifying objects, faces, or scenes in images. This technology is used in social media tagging and autonomous vehicles.
- Spam Detection: Classifying emails as spam or not spam based on historical data. This application helps improve email security and user experience.
- Credit Scoring: Assessing the creditworthiness of individuals by analyzing historical financial data. Financial institutions use this to make lending decisions.
- Medical Diagnosis: Assisting healthcare professionals in diagnosing diseases by analyzing patient data and medical images.
- Customer Churn Prediction: Identifying customers likely to leave a service based on their behavior and usage patterns. This helps businesses retain customers through targeted interventions.
Supervised learning models, such as decision trees, support vector machines, and neural networks, are trained using algorithms that minimize prediction errors. The effectiveness of these models relies heavily on the quality and quantity of the training data. At Rapid Innovation, we harness the power of these machine learning models to drive greater ROI for our clients, enabling them to make data-driven decisions that enhance operational efficiency and customer satisfaction.
4.2.2. Reinforcement Learning in Grid Control
Reinforcement Learning (RL) is a subset of machine learning that focuses on how agents ought to take actions in an environment to maximize cumulative reward. In the context of grid control, reinforcement learning can significantly enhance the efficiency and reliability of power systems.
- Dynamic Decision Making: RL algorithms can adapt to changing conditions in real-time, making them ideal for grid control where demand and supply fluctuate.
- Optimal Resource Allocation: By learning from past experiences, reinforcement learning can optimize the distribution of resources, ensuring that energy is supplied where it is most needed.
- Improved Load Balancing: RL can assist in managing load distribution across the grid, reducing the risk of overloads and outages.
- Enhanced Predictive Maintenance: By analyzing patterns in grid performance, reinforcement learning can predict potential failures and suggest maintenance schedules, thereby minimizing downtime.
The application of reinforcement learning in grid control is still evolving, but its potential to transform energy management is significant. For instance, RL has been used to optimize the operation of microgrids, leading to improved energy efficiency and reduced operational costs.
4.2.3. Deep Learning for Pattern Recognition
Deep Learning (DL) is a powerful tool in the realm of artificial intelligence, particularly for pattern recognition tasks. In the energy sector, deep learning can analyze vast amounts of data to identify trends and anomalies, which is crucial for effective grid management.
- Image and Signal Processing: DL algorithms can process images and signals from various sensors in the grid, identifying patterns that may indicate faults or inefficiencies.
- Anomaly Detection: By training on historical data, DL models can recognize normal operating conditions and flag deviations, allowing for proactive maintenance and quicker response to issues.
- Forecasting Demand: DL can analyze historical consumption data to predict future energy demand, enabling better planning and resource allocation.
- Integration with IoT: The combination of DL and Internet of Things (IoT) devices allows for real-time data collection and analysis, enhancing the grid's responsiveness to changing conditions.
The use of deep learning in pattern recognition is transforming how energy companies approach grid management, leading to more informed decision-making and improved operational efficiency.
4.3. Integration with SCADA Systems
Supervisory Control and Data Acquisition (SCADA) systems are critical for monitoring and controlling industrial processes, including power generation and distribution. Integrating advanced technologies like reinforcement learning and deep learning with SCADA systems can significantly enhance grid management.
- Real-Time Monitoring: Integration allows for real-time data analysis, enabling operators to make informed decisions quickly.
- Enhanced Control Capabilities: By incorporating reinforcement learning and deep learning, SCADA systems can automate control processes, reducing the need for human intervention and minimizing errors.
- Improved Data Visualization: Advanced analytics can provide clearer insights into grid performance, helping operators to identify issues and trends more effectively.
- Increased Reliability: The combination of SCADA with machine learning techniques can lead to more reliable grid operations, as predictive analytics can foresee potential failures before they occur.
The integration of reinforcement learning and deep learning with SCADA systems represents a significant advancement in grid control technology, paving the way for smarter, more resilient energy systems. At Rapid Innovation, we leverage these advanced technologies to help our clients achieve greater operational efficiency and return on investment (ROI) in their energy management systems. By implementing tailored reinforcement learning and deep learning solutions, we empower organizations to optimize their grid operations, reduce costs, and enhance reliability, ultimately driving their business goals forward.
4.4. Edge Computing Implementation
Edge computing is a transformative technology that brings computation and data storage closer to the location where it is needed. This reduces latency, enhances speed, and improves the overall efficiency of data processing. Implementing edge computing involves several key steps:
- Infrastructure Setup: Organizations need to establish a robust infrastructure that includes edge devices, gateways, and local servers. This setup allows for data processing at the edge rather than relying solely on centralized cloud servers. Mobile edge computing is a key aspect of this infrastructure, especially with the advent of 5G technology.
- Data Management: Effective data management strategies must be developed to handle the influx of data generated at the edge. This includes data filtering, aggregation, and storage solutions that ensure only relevant data is sent to the cloud for further analysis. Examples of edge computing applications can help illustrate effective data management strategies.
- Security Measures: With edge computing, security becomes paramount. Implementing strong encryption protocols, secure access controls, and regular security audits can help protect sensitive data processed at the edge. Edge computing security is a critical consideration for organizations.
- Integration with Existing Systems: Edge computing solutions should seamlessly integrate with existing IT infrastructure. This may involve using APIs and middleware to ensure compatibility and smooth data flow between edge devices and central systems. The integration of edge computing and IoT is essential for maximizing the benefits of both technologies.
- Scalability Considerations: As the demand for edge computing grows, organizations must plan for scalability. This includes choosing hardware and software solutions that can easily expand to accommodate increased data loads and additional edge devices. Industrial edge computing solutions can provide insights into scalable architectures.
- Monitoring and Maintenance: Continuous monitoring of edge devices is essential to ensure optimal performance. Implementing remote management tools can help in diagnosing issues and performing maintenance without the need for physical access. Mobile edge computing 5G can enhance monitoring capabilities.
- Use Cases Identification: Identifying specific use cases for edge computing can drive its implementation. Industries such as manufacturing, healthcare, and smart cities can benefit significantly from edge computing solutions. Examples of edge computing in autonomous vehicles and healthcare monitoring illustrate its diverse applications.
5. Specific Applications

‍
Edge computing has a wide range of applications across various industries. By processing data closer to the source, organizations can achieve faster response times and improved operational efficiency. Some specific applications include:
- IoT Device Management: Edge computing enables real-time data processing from IoT devices, allowing for immediate insights and actions. The integration of IoT edge computing enhances the capabilities of smart devices.
- Autonomous Vehicles: In the automotive industry, edge computing supports the processing of data from sensors and cameras, which is crucial for the safe operation of autonomous vehicles. IIoT edge computing plays a significant role in this sector.
- Smart Cities: Edge computing facilitates the management of smart city applications, such as traffic monitoring and public safety systems, by processing data locally to enhance responsiveness. The relationship between edge computing and IoT is vital for smart city initiatives.
- Healthcare Monitoring: In healthcare, edge computing allows for real-time patient monitoring and data analysis, improving patient outcomes and operational efficiency. Mobile edge computing can enhance healthcare applications.
- Retail Analytics: Retailers can leverage edge computing to analyze customer behavior in real-time, optimizing inventory management and enhancing the shopping experience.
5.1. Frequency Regulation
Frequency regulation is a critical aspect of maintaining the stability of electrical grids. It involves balancing supply and demand to ensure that the frequency of the electricity supply remains within acceptable limits. Edge computing plays a significant role in enhancing frequency regulation through the following mechanisms:
- Real-Time Data Processing: Edge computing allows for the real-time processing of data from various sources, including smart meters and grid sensors. This enables utilities to monitor frequency fluctuations and respond promptly.
- Distributed Energy Resources (DER) Management: With the rise of renewable energy sources, managing DERs becomes essential. Edge computing can optimize the integration of these resources into the grid, ensuring that they contribute effectively to frequency regulation.
- Demand Response Programs: Edge computing supports demand response initiatives by enabling utilities to communicate with consumers in real-time. This allows for adjustments in energy consumption during peak periods, helping to stabilize frequency.
- Predictive Analytics: By utilizing edge computing, utilities can implement predictive analytics to forecast demand and supply trends. This foresight aids in proactive frequency management, reducing the risk of outages.
- Enhanced Communication: Edge computing facilitates faster communication between grid operators and distributed assets. This improved communication is vital for coordinating actions that maintain frequency stability.
- Cost Efficiency: Implementing edge computing for frequency regulation can lead to cost savings by reducing the need for expensive grid upgrades and enhancing the efficiency of existing resources.
- Regulatory Compliance: Edge computing can help utilities meet regulatory requirements for frequency control by providing accurate and timely data for reporting and compliance purposes.
By leveraging edge computing, utilities can enhance their frequency regulation capabilities, leading to a more stable and reliable electrical grid. At Rapid Innovation, we specialize in implementing edge computing solutions tailored to your specific business needs, ensuring you achieve greater ROI through improved operational efficiency and responsiveness. Additionally, our MLOps consulting services can further enhance your edge computing strategies. For more insights on the future of edge computing, check out our article on accelerating innovation in AI-driven edge computing.
5.2. Voltage Control
Voltage control is a critical aspect of electrical systems, ensuring that voltage levels remain within specified limits to maintain the stability and reliability of power supply. Effective voltage control helps prevent equipment damage, enhances system performance, and improves overall power quality.
- Voltage regulation is essential for maintaining the voltage within acceptable limits, typically ±5% of the nominal voltage.
- Various devices are used for voltage control, including transformers, capacitors, and voltage regulators.
- Automatic Voltage Regulators (AVRs) play a significant role in adjusting voltage levels in real-time, responding to load changes and fluctuations.
- Reactive power management is crucial for voltage control, as it helps balance the reactive power in the system, which directly affects voltage levels.
- Advanced technologies, such as smart grid solutions and voltage control solutions, enable better voltage control through real-time monitoring and automated adjustments. Rapid Innovation leverages AI algorithms to optimize these processes, ensuring that voltage levels are maintained efficiently, thus reducing operational costs and enhancing system reliability.
- Voltage control strategies can include load shedding, capacitor bank switching, and transformer tap changing to maintain voltage stability. By implementing these strategies, Rapid Innovation assists clients in achieving greater ROI through improved energy efficiency and reduced downtime.
5.3. Power Quality Management
Power quality management focuses on ensuring that the electrical power supplied to consumers is of high quality, free from disturbances that can affect the performance of electrical devices. Poor power quality can lead to equipment malfunctions, increased operational costs, and reduced system efficiency.
- Key power quality issues include voltage sags, swells, harmonics, flicker, and transients.
- Monitoring power quality involves using specialized equipment to measure and analyze various parameters, such as voltage, current, and frequency.
- Power quality standards, such as IEEE 519, provide guidelines for acceptable levels of harmonics and other disturbances in electrical systems.
- Solutions for improving power quality may include installing power conditioning equipment, such as filters, surge protectors, and uninterruptible power supplies (UPS). Rapid Innovation employs AI-driven analytics to identify and mitigate power quality issues proactively, ensuring optimal performance of electrical systems.
- Regular maintenance and monitoring can help identify potential power quality issues before they escalate, ensuring a reliable power supply.
- Educating consumers about the importance of power quality can lead to better practices and reduced disturbances in the electrical system.
5.4. Fault Detection and Isolation
Fault detection and isolation are vital for maintaining the safety and reliability of electrical systems. Quick identification and isolation of faults help prevent equipment damage, minimize downtime, and ensure the safety of personnel.
Faults can occur due to various reasons, including equipment failure, environmental factors, and human error. Advanced fault detection techniques, such as digital relays and smart sensors, enable real-time monitoring and quick identification of faults. The use of algorithms and machine learning can enhance fault detection capabilities, allowing for predictive maintenance and quicker response times. Rapid Innovation integrates AI solutions to improve fault detection accuracy, enabling clients to respond to issues before they escalate.
Isolation of faults is crucial to prevent the spread of issues throughout the electrical system, often achieved through circuit breakers and protective relays. Automated systems can facilitate rapid isolation of faults, reducing the risk of widespread outages and improving system resilience. Regular testing and maintenance of protective devices are essential to ensure their effectiveness in fault detection and isolation. By utilizing blockchain technology, Rapid Innovation can enhance the traceability and accountability of maintenance activities, further ensuring system reliability and safety.
5.5. Demand Response Optimization
Demand response optimization is a crucial strategy in energy management that aims to balance supply and demand in real-time. This approach allows utilities and grid operators to adjust the demand for power instead of adjusting the supply.
- Enhances grid reliability by reducing peak demand. Â
- Encourages consumers to shift their energy usage to off-peak times. Â
- Utilizes advanced technologies like smart meters and IoT devices for real-time data collection. Â
- Involves incentives for consumers to reduce or shift their electricity usage during peak periods. Â
- Can lead to significant cost savings for both utilities and consumers. Â
The optimization process often involves sophisticated algorithms and machine learning techniques to predict demand patterns and adjust accordingly. By analyzing historical data and current usage trends, utilities can create more efficient demand response optimization programs. At Rapid Innovation, we leverage our expertise in AI to develop tailored solutions that enhance demand response strategies, ultimately driving greater ROI for our clients. Our approach includes utilizing predictive analytics to forecast demand and optimize energy usage effectively, as well as employing AI agents for energy consumption tracking.
5.6. Renewable Energy Forecasting
Renewable energy forecasting is essential for integrating renewable sources like solar and wind into the energy grid. Accurate forecasting helps in predicting the availability of these energy sources, which can be variable and unpredictable.
- Involves the use of meteorological data and advanced modeling techniques. Â
- Helps grid operators plan for energy supply and demand effectively. Â
- Reduces reliance on fossil fuels by optimizing the use of renewable energy. Â
- Enhances the stability of the grid by anticipating fluctuations in energy production. Â
- Supports the development of energy storage solutions by predicting when excess energy will be available. Â
Forecasting methods can range from simple statistical models to complex machine learning algorithms. These models analyze various factors, including weather patterns, historical energy production data, and geographical considerations. Accurate forecasting can lead to better decision-making and improved energy management. Rapid Innovation employs advanced AI techniques to enhance forecasting accuracy, enabling our clients to optimize their renewable energy investments and achieve substantial returns.
6. Challenges and Limitations
Despite the advancements in demand response optimization and renewable energy forecasting, several challenges and limitations persist.
- Data Privacy: The collection of real-time data raises concerns about consumer privacy and data security. Â
- Technology Integration: Integrating new technologies with existing infrastructure can be complex and costly. Â
- Consumer Participation: Engaging consumers in demand response optimization programs can be challenging, as not all consumers are willing to adjust their usage patterns. Â
- Variability of Renewable Sources: The inherent unpredictability of renewable energy sources can complicate forecasting efforts. Â
- Regulatory Barriers: Existing regulations may not support innovative demand response strategies or renewable energy integration. Â
Addressing these challenges requires collaboration among stakeholders, including utilities, regulators, and consumers. Continuous advancements in technology and data analytics will also play a vital role in overcoming these limitations. At Rapid Innovation, we are committed to providing consulting and development solutions that help our clients navigate these challenges effectively, ensuring they can maximize their operational efficiency and ROI. to address data quality challenges effectively. We understand the challenges of data quality that organizations face, particularly in data warehouses where data quality challenges in data warehouse environments can be pronounced. Additionally, we recognize the data quality problems in data mining that can arise when extracting insights from large datasets.
To further support our clients, we provide a comprehensive list and describe the most common data quality problems, ensuring that organizations are well-equipped to tackle these issues head-on. By focusing on these data quality challenges, we empower organizations to make informed decisions and drive successful outcomes.
6.1.3. Real-time Processing Requirements
Real-time processing is crucial for many modern applications, especially in sectors like finance, healthcare, and e-commerce. The need for immediate data processing can significantly impact decision-making and operational efficiency.
- Immediate data availability: Organizations require instant access to data to make timely decisions. For instance, in stock trading, a delay of even a few seconds can lead to substantial financial losses. Rapid Innovation leverages AI algorithms to ensure that data is processed and made available in real-time, enabling clients to make informed decisions swiftly. This is particularly relevant in scenarios involving realtime data processing and real time data analysis.
- Continuous data streams: Many applications rely on continuous data input, such as IoT devices or social media feeds. These systems must process data in real-time to provide relevant insights. Our expertise in AI and blockchain allows us to create robust systems that can handle continuous data streams efficiently, ensuring that clients receive actionable insights without delay. This includes real time data integration and real time data ingestion.
- Low latency: Real-time processing demands low latency to ensure that data is processed and delivered without significant delays. This is particularly important in applications like autonomous vehicles, where split-second decisions can be life-saving. Rapid Innovation employs advanced technologies to minimize latency, enhancing the performance of critical applications. Our solutions also encompass real time stream processing and real time stream analytics.
- Scalability: As data volumes grow, systems must be able to scale efficiently to handle increased loads without compromising performance. Our solutions are designed with scalability in mind, allowing organizations to expand their operations seamlessly as their data needs evolve. This is essential for both batch and real time processing.
- Data accuracy: Real-time systems must ensure high data accuracy to avoid errors that could lead to incorrect conclusions or actions. Rapid Innovation implements rigorous data validation processes to maintain high accuracy levels, ensuring that our clients can trust the insights generated by their systems. This is particularly important in projects like aws real time data processing and etl real time projects.
6.1.4. Integration with Legacy Systems
Integrating new technologies with legacy systems presents unique challenges. Many organizations still rely on older systems that may not be compatible with modern applications.
- Compatibility issues: Legacy systems often use outdated technologies that may not support new software or hardware, leading to integration difficulties. Rapid Innovation specializes in bridging the gap between legacy systems and modern technologies, ensuring a smooth transition for our clients.
- Data silos: Legacy systems can create data silos, making it challenging to access and share information across the organization. This can hinder collaboration and decision-making. Our solutions focus on breaking down these silos, enabling seamless data sharing and enhancing organizational efficiency.
- High costs: Upgrading or replacing legacy systems can be expensive and time-consuming. Organizations must weigh the costs against the benefits of integration. Rapid Innovation provides cost-effective strategies for integration, helping clients maximize their ROI while minimizing disruption.
- Training requirements: Employees may need training to work with both legacy and new systems, which can lead to temporary productivity losses. We offer comprehensive training programs to ensure that staff are well-equipped to handle new technologies, facilitating a smoother transition.
- Incremental integration: Organizations may need to adopt a phased approach to integration, gradually connecting legacy systems with new technologies to minimize disruption. Our incremental integration strategies are designed to reduce risks and ensure that clients can adapt without significant operational interruptions.
6.2. Operational Challenges
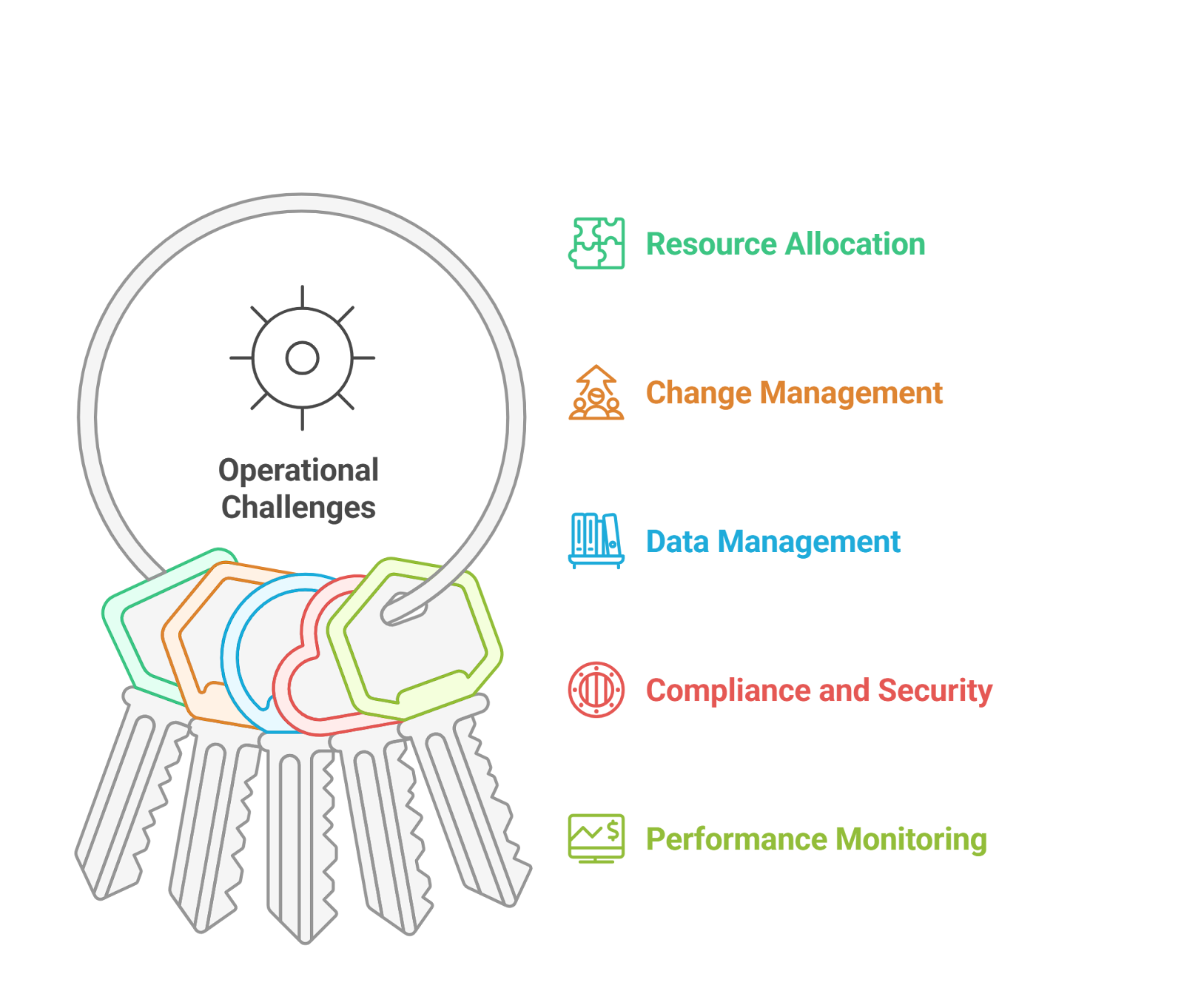
‍
Operational challenges can significantly impact an organization's efficiency and effectiveness. Addressing these challenges is essential for maintaining a competitive edge.
- Resource allocation: Organizations often struggle with allocating resources effectively, leading to bottlenecks and inefficiencies in operations. Rapid Innovation helps clients optimize resource allocation through AI-driven analytics, ensuring that resources are utilized effectively.
- Change management: Implementing new technologies or processes can meet resistance from employees, making change management a critical aspect of operational success. Our change management strategies are tailored to address employee concerns, fostering a culture of innovation and adaptability.
- Data management: As data volumes increase, managing and analyzing data becomes more complex. Organizations must invest in robust data management strategies to ensure data quality and accessibility. Rapid Innovation provides comprehensive data management solutions that enhance data quality and streamline access.
- Compliance and security: Organizations must navigate regulatory requirements and ensure data security, which can complicate operations and increase costs. Our blockchain expertise ensures that clients can maintain compliance and security while optimizing their operations.
- Performance monitoring: Continuous monitoring of operational performance is necessary to identify areas for improvement. Organizations must implement effective metrics and KPIs to track progress and make informed decisions. Rapid Innovation assists clients in establishing performance monitoring frameworks that drive continuous improvement and operational excellence.
6.2.1. Training Requirements
Training requirements are essential for ensuring that employees possess the necessary skills and knowledge to perform their jobs effectively. This is particularly important in industries that are rapidly evolving or heavily regulated, such as AI and Blockchain.
- Identify specific skills needed for each role, particularly those related to AI algorithms, data analysis, and blockchain protocols.
- Develop a comprehensive training program that includes: Â
- Onboarding for new employees, focusing on foundational knowledge in AI and Blockchain technologies, including management training for new managers.
- Ongoing training for existing staff to keep them updated on the latest advancements and best practices, such as corporate training programs for employees.
- Specialized training for advanced roles, such as data scientists or blockchain developers, to deepen their expertise, including employee development training and training plans for employees.
- Utilize various training methods, such as: Â
- E-learning modules for flexibility, allowing employees to learn at their own pace, including online training courses for employees.
- In-person workshops for hands-on experience with AI tools and blockchain applications, such as supervisor management training.
- Mentorship programs to foster knowledge sharing and practical insights from experienced professionals, including programs for employee development.
- Regularly assess training effectiveness through: Â
- Feedback surveys from participants to gauge satisfaction and areas for improvement, including employee training classes.
- Performance metrics to measure skill enhancement and application in real-world scenarios, such as training courses for employees.
- Adjusting training content based on industry changes and emerging technologies, including types of employee development programs.
- Ensure that training aligns with organizational goals and compliance requirements, particularly in the context of AI ethics and blockchain regulations. For more insights on best practices, refer to the potential of business AI engineering.
6.2.2. Change Management
Change management is a structured approach to transitioning individuals, teams, and organizations from a current state to a desired future state. Effective change management minimizes resistance and maximizes engagement, especially when integrating AI and Blockchain solutions.
- Establish a clear vision for the change initiative, outlining how AI and Blockchain will enhance operational efficiency and drive innovation.
- Communicate the reasons for the change to all stakeholders: Â
- Use multiple channels (emails, meetings, newsletters) to reach everyone and ensure transparency.
- Address potential concerns and benefits openly, emphasizing the value of AI and Blockchain in achieving business goals.
- Involve employees in the change process: Â
- Create change champions within teams to advocate for the initiative and facilitate adoption, including training manager training courses.
- Encourage feedback and suggestions to foster a sense of ownership and collaboration.
- Provide support throughout the transition: Â
- Offer resources such as FAQs, training sessions, and one-on-one coaching to ease the learning curve, including supervisory training for new supervisors.
- Monitor progress and adjust strategies as needed to ensure successful implementation.
- Evaluate the change process post-implementation: Â
- Measure success through key performance indicators (KPIs) related to AI and Blockchain performance.
- Conduct follow-up surveys to assess employee sentiment and identify areas for improvement.
6.2.3. Regulatory Compliance
Regulatory compliance refers to the adherence to laws, regulations, guidelines, and specifications relevant to an organization’s business processes. It is crucial for maintaining operational integrity and avoiding legal penalties, particularly in the AI and Blockchain sectors.
- Identify applicable regulations for your industry: Â
- Stay updated on local, national, and international laws governing AI ethics and blockchain transactions.
- Consult legal experts or compliance officers for guidance on navigating complex regulatory landscapes.
- Develop a compliance program that includes: Â
- Policies and procedures outlining compliance expectations specific to AI and Blockchain technologies.
- Regular training sessions to educate employees on compliance matters and ethical considerations, including employee diversity training.
- Implement monitoring and auditing processes: Â
- Conduct regular internal audits to assess compliance levels with AI and Blockchain regulations.
- Use technology solutions for real-time monitoring and reporting of compliance metrics.
- Establish a reporting mechanism for compliance issues: Â
- Encourage employees to report violations without fear of retaliation, fostering a culture of transparency.
- Investigate reported issues promptly and take corrective actions to mitigate risks.
- Review and update compliance programs regularly: Â
- Adapt to changes in regulations and industry standards, ensuring ongoing compliance.
- Engage in continuous improvement to enhance compliance efforts and align with best practices in AI and Blockchain.
6.3. Security Concerns
In today's digital landscape, security concerns are paramount for individuals and organizations alike. As technology evolves, so do the threats that accompany it. Understanding these security concerns is essential for safeguarding sensitive information and maintaining trust in digital systems.
6.3.1. Cybersecurity Risks
Cybersecurity risks encompass a wide range of threats that can compromise the integrity, confidentiality, and availability of information systems. These risks can arise from various sources, including malicious actors, software vulnerabilities, and human error.
- Malware Attacks: Malicious software, or malware, can infiltrate systems to steal data, disrupt operations, or gain unauthorized access. Common types of malware include viruses, worms, and ransomware.
- Phishing Scams: Cybercriminals often use phishing techniques to trick individuals into revealing sensitive information, such as passwords or credit card numbers. These scams typically involve deceptive emails or websites that appear legitimate.
- Denial of Service (DoS) Attacks: DoS attacks aim to overwhelm a system with traffic, rendering it unavailable to users. This can lead to significant downtime and financial losses for businesses.
- Insider Threats: Employees or contractors with access to sensitive information can pose a risk if they intentionally or unintentionally compromise security. This can include data theft or accidental data leaks.
- Unpatched Software: Failing to update software can leave systems vulnerable to exploitation. Cybercriminals often target known vulnerabilities in outdated software to gain access.
- IoT Vulnerabilities: The proliferation of Internet of Things (IoT) devices has introduced new security challenges. Many IoT devices lack robust security measures, making them easy targets for attackers.
Organizations must implement comprehensive cybersecurity strategies to mitigate these risks. This includes regular security assessments, employee training, and the use of advanced security technologies. Cyber security companies and cybersecurity managed services can provide essential support in developing and maintaining these strategies. Rapid Innovation specializes in developing AI-driven security solutions that can proactively identify and respond to these threats, ensuring that your organization remains resilient against cyberattacks. For more insights on AI in security, check out AI Knowledge Management in 2024.
6.3.2. Data Privacy
Data privacy refers to the proper handling, processing, and storage of personal information. With the increasing amount of data collected by businesses and governments, concerns about data privacy have become more pronounced.
- Regulatory Compliance: Organizations must comply with various data protection regulations, such as the General Data Protection Regulation (GDPR) and the California Consumer Privacy Act (CCPA). Non-compliance can result in hefty fines and reputational damage.
- Data Breaches: High-profile data breaches have highlighted the importance of data privacy. When sensitive information is exposed, it can lead to identity theft, financial loss, and a loss of trust in the affected organization.
- User Consent: Obtaining explicit consent from users before collecting their data is crucial. Organizations should clearly communicate how data will be used and provide users with the option to opt-out.
- Data Minimization: Collecting only the data necessary for a specific purpose can reduce the risk of exposure. Organizations should regularly review their data collection practices to ensure they align with this principle.
- Third-Party Risks: Sharing data with third-party vendors can introduce additional privacy risks. Organizations should conduct due diligence to ensure that third parties adhere to strict data privacy standards, especially when utilizing managed data security services.
- Data Encryption: Encrypting sensitive data can protect it from unauthorized access. Even if data is intercepted, encryption makes it difficult for attackers to decipher the information.
To address data privacy concerns, organizations should adopt a proactive approach that includes regular audits, employee training, and the implementation of robust data protection measures. Cyber security solutions and services, including cloud web security and cloud network security, can enhance data protection efforts. Rapid Innovation offers consulting services that help organizations navigate the complexities of data privacy regulations and implement effective data protection strategies, ultimately enhancing their reputation and trustworthiness in the market.
6.3.3. System Resilience
System resilience refers to the ability of a system to anticipate, prepare for, respond to, and recover from adverse conditions, disruptions, or threats. In the context of technology and infrastructure, resilience is crucial for maintaining operational continuity and ensuring that services remain available even in the face of challenges, particularly in areas such as supply chain risk management vulnerability and resilience in logistics.
- Key Components of System Resilience: Â
- Redundancy: Implementing backup systems and components to ensure that if one part fails, others can take over.
- Diversity: Utilizing a variety of technologies and approaches to reduce the risk of systemic failure.
- Adaptability: The ability of a system to adjust to changing conditions and recover from disruptions.
- Monitoring and Feedback: Continuous assessment of system performance to identify potential vulnerabilities and areas for improvement.
- Importance of System Resilience: Â
- Enhances operational reliability and service availability.
- Reduces downtime and associated costs.
- Builds trust with stakeholders and customers by ensuring consistent service delivery.
- Supports compliance with regulatory requirements and industry standards, which is essential in structural dynamics and resilience in supply chain risk management.
- Strategies to Improve System Resilience: Â
- Conduct regular stress tests to evaluate system performance under extreme conditions.
- Develop and implement incident response plans to address potential disruptions.
- Invest in training and awareness programs for staff to ensure they are prepared for emergencies.
- Utilize cloud services and distributed architectures to enhance flexibility and scalability.
7. Risk Management and Mitigation
Risk management and mitigation involve identifying, assessing, and prioritizing risks followed by coordinated efforts to minimize, monitor, and control the probability or impact of unfortunate events. Effective risk management is essential for organizations to safeguard their assets, reputation, and operational capabilities, particularly in the context of system resilience and risk management.
- Key Elements of Risk Management: Â
- Risk Identification: Recognizing potential risks that could impact the organization.
- Risk Analysis: Evaluating the likelihood and potential impact of identified risks.
- Risk Evaluation: Comparing estimated risks against risk criteria to determine their significance.
- Risk Treatment: Developing strategies to mitigate or eliminate risks.
- Benefits of Effective Risk Management: Â
- Protects organizational assets and resources.
- Enhances decision-making processes by providing a clear understanding of risks.
- Improves compliance with legal and regulatory requirements.
- Fosters a culture of risk awareness and proactive management.
- Common Risk Mitigation Strategies: Â
- Implementing controls and safeguards to reduce risk exposure.
- Transferring risk through insurance or outsourcing.
- Accepting risk when the costs of mitigation exceed potential losses.
- Regularly reviewing and updating risk management plans to adapt to new threats.
7.1. Risk Assessment Framework
A risk assessment framework provides a structured approach to identifying, analyzing, and managing risks within an organization. It serves as a guide for decision-makers to understand the risk landscape and prioritize actions based on potential impacts, including those related to system resilience and risk management.
- Components of a Risk Assessment Framework: Â
- Context Establishment: Defining the environment in which risks will be assessed, including internal and external factors.
- Risk Identification: Systematically identifying risks through various methods such as brainstorming, interviews, and historical data analysis.
- Risk Analysis: Assessing the likelihood and consequences of identified risks using qualitative and quantitative methods.
- Risk Evaluation: Prioritizing risks based on their significance and determining which risks require treatment.
- Importance of a Risk Assessment Framework: Â
- Provides a consistent approach to risk management across the organization.
- Facilitates communication and understanding of risks among stakeholders.
- Supports informed decision-making by highlighting critical risks and their potential impacts.
- Enhances the organization’s ability to respond to emerging threats and challenges, particularly in the context of system resilience and risk management.
- Best Practices for Implementing a Risk Assessment Framework: Â
- Involve stakeholders from various departments to gain diverse perspectives on risks.
- Use established standards and guidelines to ensure a comprehensive approach.
- Regularly review and update the framework to reflect changes in the risk environment.
- Document the risk assessment process and outcomes to support accountability and continuous improvement.
At Rapid Innovation, we leverage our expertise in AI and Blockchain to enhance system resilience and risk management for our clients. By integrating advanced AI algorithms, we can predict potential disruptions and automate responses, ensuring that your systems remain robust and responsive. Our Blockchain solutions provide an immutable record of transactions, enhancing transparency and trust, which are critical in risk management. Together, these technologies empower organizations to achieve greater ROI by minimizing downtime and optimizing operational efficiency, addressing the challenges of system resilience and risk management effectively. For more insights, check out the future of personalized risk evaluation in insurance with AI agents.
7.2. Failsafe Mechanisms
Failsafe mechanisms are critical components in any system designed to ensure safety and reliability. These mechanisms are put in place to prevent catastrophic failures and to maintain operational integrity even when unexpected issues arise. Failsafe mechanisms can include automatic shutdown systems that activate when a fault is detected. They often involve redundancy, where critical components have backups that can take over in case of a failure. These systems are designed to minimize human error by automating responses to specific failure conditions. Examples include circuit breakers in electrical systems that cut off power to prevent overloads and fires. In software, failsafe mechanisms can involve error handling routines that prevent crashes and maintain service availability. Implementing failsafe mechanisms is essential for industries such as aviation, healthcare, and manufacturing, where the cost of failure can be extremely high.
- Failsafe mechanisms can include automatic shutdown systems that activate when a fault is detected.
- They often involve redundancy, where critical components have backups that can take over in case of a failure.
- These systems are designed to minimize human error by automating responses to specific failure conditions.
- Examples include circuit breakers in electrical systems that cut off power to prevent overloads and fires.
- In software, failsafe mechanisms can involve error handling routines that prevent crashes and maintain service availability.
7.3. Backup Systems
Backup systems are essential for data protection and continuity of operations. They ensure that critical information is preserved and can be restored in the event of data loss or system failure. Regular backups should be scheduled to capture the most recent data changes. Backup systems can be local (on-site) or remote (cloud-based), providing flexibility in data recovery options. Incremental backups save only the changes made since the last backup, optimizing storage space and time. Full backups provide a complete snapshot of the data, which is useful for comprehensive recovery. Testing backup systems regularly is crucial to ensure that data can be restored quickly and accurately when needed. Incorporating robust backup systems is vital for businesses to protect against data breaches, hardware failures, and natural disasters.
- Regular backups should be scheduled to capture the most recent data changes.
- Backup systems can be local (on-site) or remote (cloud-based), providing flexibility in data recovery options.
- Incremental backups save only the changes made since the last backup, optimizing storage space and time.
- Full backups provide a complete snapshot of the data, which is useful for comprehensive recovery.
- Testing backup systems regularly is crucial to ensure that data can be restored quickly and accurately when needed.
7.4. Disaster Recovery Planning
Disaster recovery planning is a strategic approach to preparing for and responding to unexpected events that can disrupt business operations. A well-structured disaster recovery plan (DRP) outlines the processes and procedures to follow in the event of a disaster. A DRP should include risk assessments to identify potential threats and vulnerabilities. It should define recovery time objectives (RTO) and recovery point objectives (RPO) to set clear expectations for downtime and data loss. Regular training and drills for employees ensure that everyone knows their roles during a disaster. The plan should be regularly updated to reflect changes in technology, business processes, and personnel. Communication strategies are essential to keep stakeholders informed during a crisis. Effective disaster recovery planning helps organizations minimize downtime, protect critical assets, and maintain customer trust in the face of adversity.
- A DRP should include risk assessments to identify potential threats and vulnerabilities.
- It should define recovery time objectives (RTO) and recovery point objectives (RPO) to set clear expectations for downtime and data loss.
- Regular training and drills for employees ensure that everyone knows their roles during a disaster.
- The plan should be regularly updated to reflect changes in technology, business processes, and personnel.
- Communication strategies are essential to keep stakeholders informed during a crisis.
At Rapid Innovation, we understand the importance of these mechanisms in achieving business goals efficiently and effectively. By integrating advanced AI and Blockchain technologies, we can enhance the reliability and security of your systems, ensuring that your organization is well-prepared for any challenges that may arise. Our expertise in developing tailored solutions allows us to help clients achieve greater ROI by minimizing risks and maximizing operational efficiency.
7.5. Compliance and Auditing
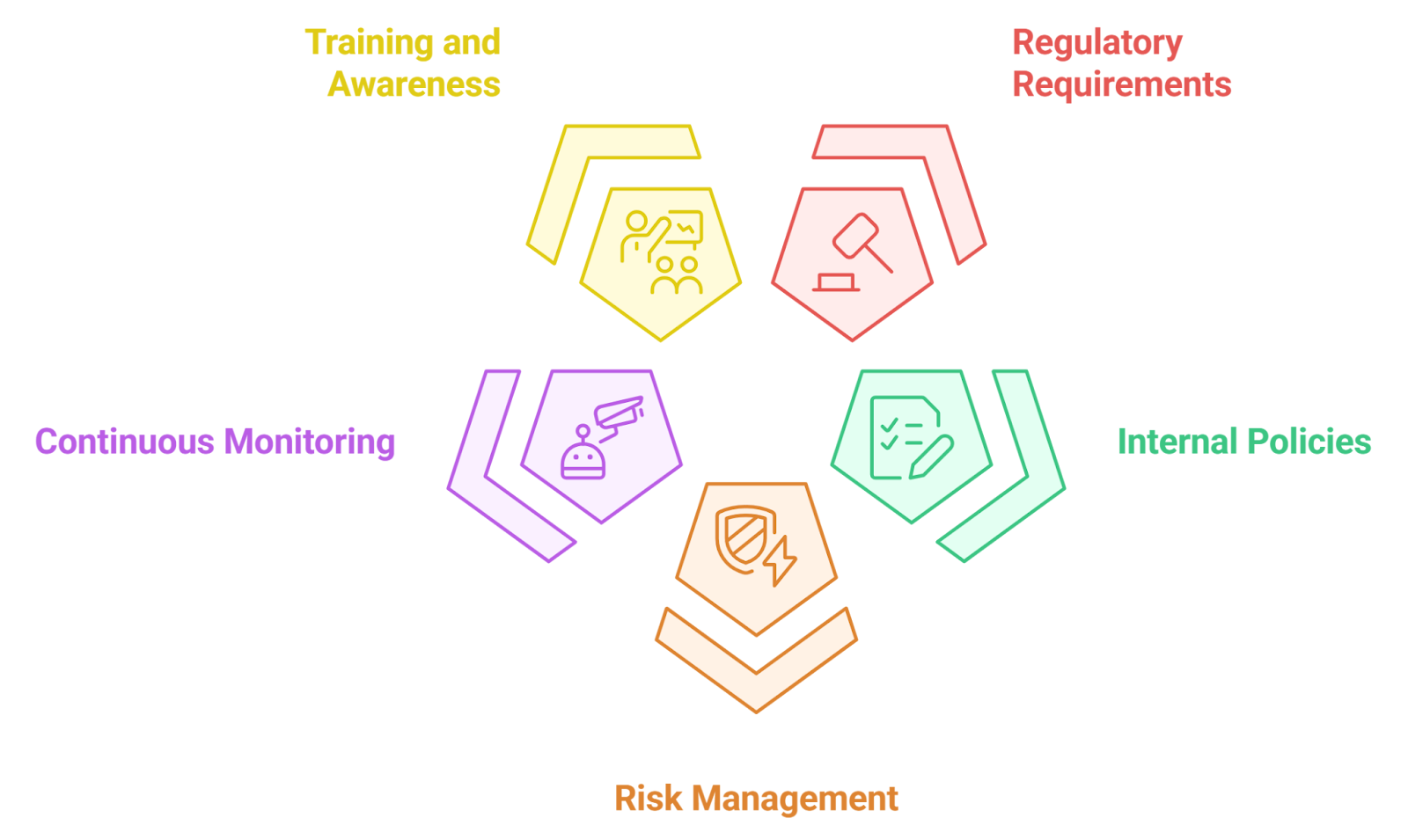
‍
Compliance and auditing are critical components of any organization’s operational framework. They ensure that businesses adhere to laws, regulations, and internal policies, thereby minimizing risks and enhancing accountability. Compliance refers to the process of conforming to established guidelines or specifications, which can be legal, regulatory, or internal. Auditing involves the systematic examination of records, processes, and systems to ensure compliance and identify areas for improvement, including soc2 audit, sox audit, and compliance audit.
Key aspects of compliance and auditing include:
- Regulatory Requirements: Organizations must stay updated on relevant laws and regulations, such as GDPR, HIPAA, or industry-specific standards. Non-compliance can lead to hefty fines and legal repercussions, particularly in areas like pci compliance audit and sarbane oxley audit.
- Internal Policies: Companies should develop and enforce internal policies that align with external regulations. Regular reviews and updates are essential to maintain relevance, especially in the context of sox and audit practices.
- Risk Management: Compliance and auditing help identify potential risks, allowing organizations to implement controls to mitigate them. Rapid Innovation leverages AI-driven analytics to assess risk factors in real-time, enabling proactive measures. This includes conducting soc 2 type 2 audits and understanding the implications of ssae16.
- Continuous Monitoring: Regular audits and compliance checks ensure ongoing adherence to policies and regulations, fostering a culture of accountability. Our blockchain solutions can provide immutable records of compliance activities, enhancing transparency, particularly in compliance & audit processes.
- Training and Awareness: Employees should be trained on compliance requirements and the importance of adhering to policies. This can reduce the likelihood of violations. Rapid Innovation offers tailored training programs that utilize AI to adapt to the specific needs of your workforce, including training on sox in audit practices.
Organizations often engage third-party auditors to provide an objective assessment of their compliance status. This external perspective can uncover issues that internal teams may overlook, especially in the context of a 133 audits or pci audit.
8. Economic Considerations
Economic considerations play a vital role in the decision-making process of any organization. They encompass the financial implications of various business strategies, including investments, operational costs, and potential returns.
- Cost-Benefit Analysis: Organizations must weigh the costs of implementing new initiatives against the expected benefits. This analysis helps in making informed decisions.
- Market Trends: Understanding economic trends can guide businesses in adjusting their strategies to remain competitive. This includes analyzing consumer behavior, pricing strategies, and market demand.
- Resource Allocation: Effective allocation of resources is crucial for maximizing profitability. Organizations must consider the economic impact of their resource distribution.
Key economic factors to consider include:
- Inflation Rates: Rising inflation can affect purchasing power and operational costs, influencing pricing strategies and profit margins.
- Interest Rates: Changes in interest rates can impact borrowing costs and investment decisions. Organizations must monitor these rates to make informed financial choices.
- Economic Growth: A growing economy can lead to increased consumer spending, while a recession may necessitate cost-cutting measures.
8.1. Implementation Costs
Implementation costs refer to the expenses associated with putting a new system, process, or initiative into action. Understanding these costs is essential for effective budgeting and financial planning.
- Direct Costs: These are the obvious expenses incurred during implementation, such as purchasing software, hiring staff, or acquiring equipment.
- Indirect Costs: These costs may not be immediately apparent but can significantly impact the overall budget. They include training, maintenance, and potential downtime during the transition.
- Opportunity Costs: Organizations must consider what they are giving up by allocating resources to a new initiative. This includes potential revenue from alternative projects.
Factors influencing implementation costs include:
- Project Scope: The larger and more complex the project, the higher the implementation costs are likely to be.
- Technology Requirements: Upgrading or integrating new technology can lead to significant expenses, including software licenses and hardware purchases.
- Change Management: Effective change management strategies can mitigate costs associated with employee resistance and training.
To manage implementation costs effectively, organizations should:
- Conduct a thorough cost analysis before initiating a project.
- Develop a detailed budget that includes all potential expenses.
- Monitor costs throughout the implementation process to identify and address any overruns promptly.
By understanding and managing compliance, auditing, economic considerations, and implementation costs, organizations can enhance their operational efficiency and ensure long-term success. Rapid Innovation is committed to guiding clients through these complexities, utilizing our expertise in AI and blockchain to deliver tailored solutions that drive greater ROI.
8.2. Operating Expenses
Operating expenses (OPEX) are the costs associated with running a business on a day-to-day basis. These expenses are crucial for maintaining operations and can significantly impact a company's profitability. Understanding and managing operating expenses management is essential for financial health. For more insights on how AI can enhance financial operations, check out this article on AI in banking and finance use cases and applications.
- Types of Operating Expenses:
- Fixed Costs: These remain constant regardless of production levels, such as rent and salaries.
- Variable Costs: These fluctuate with production volume, including raw materials and utility costs.
- **Semi-Variable Costs.
8.5. Funding and Investment Strategies
Funding and investment strategies are crucial for the growth and sustainability of any business. These strategies help organizations secure the necessary capital to expand operations, invest in new technologies, and enhance product offerings.
- Types of Funding Sources Â
- Equity Financing: Involves selling shares of the company to raise capital. This can be through venture capital, angel investors, or public offerings.
- Debt Financing: Involves borrowing money that must be repaid over time, often through loans or bonds.
- Grants and Subsidies: Non-repayable funds provided by governments or organizations to support specific projects or initiatives.
- Investment Strategies Â
- Diversification: Spreading investments across various sectors to minimize risk. This can include investing in different asset classes or geographic regions, such as private credit funds or small cap value stocks.
- Value Investing: Focusing on undervalued companies with strong fundamentals, aiming for long-term growth. This can include aggressive growth mutual funds or aggressive growth ETFs.
- Growth Investing: Targeting companies expected to grow at an above-average rate compared to their industry or the overall market, such as T Rowe large cap growth or quantitative funds.
- Crowdfunding: A modern approach where businesses raise small amounts of money from a large number of people, typically via online platforms. This method not only provides funding but also validates the business idea.
- Strategic Partnerships: Collaborating with other businesses can provide access to new markets, technologies, and resources, enhancing overall investment potential.
- Impact Investing: Investing in companies that generate social or environmental benefits alongside financial returns. This strategy is gaining traction as more investors seek to align their portfolios with their values.
- Exit Strategies: Planning for how investors will realize returns on their investments, whether through selling the company, merging, or going public.
9. Future Developments and Trends
The business landscape is constantly evolving, influenced by technological advancements, changing consumer preferences, and global economic shifts. Understanding future developments and trends is essential for businesses to remain competitive.
- Sustainability: Companies are increasingly focusing on sustainable practices, driven by consumer demand for environmentally friendly products and services. This trend is reshaping supply chains and product development.
- Remote Work: The rise of remote work has transformed workplace dynamics. Businesses are investing in technology to support remote collaboration and communication, leading to more flexible work environments.
- Digital Transformation: Organizations are adopting digital technologies to improve efficiency, enhance customer experiences, and streamline operations. This includes the use of artificial intelligence, big data, and cloud computing.
- Consumer Behavior Changes: Shifts in consumer preferences, particularly towards online shopping and personalized experiences, are prompting businesses to adapt their marketing and sales strategies.
- Regulatory Changes: Businesses must stay informed about evolving regulations, particularly in areas like data privacy, environmental standards, and labor laws, which can impact operations and investment strategies.
9.1. Emerging Technologies
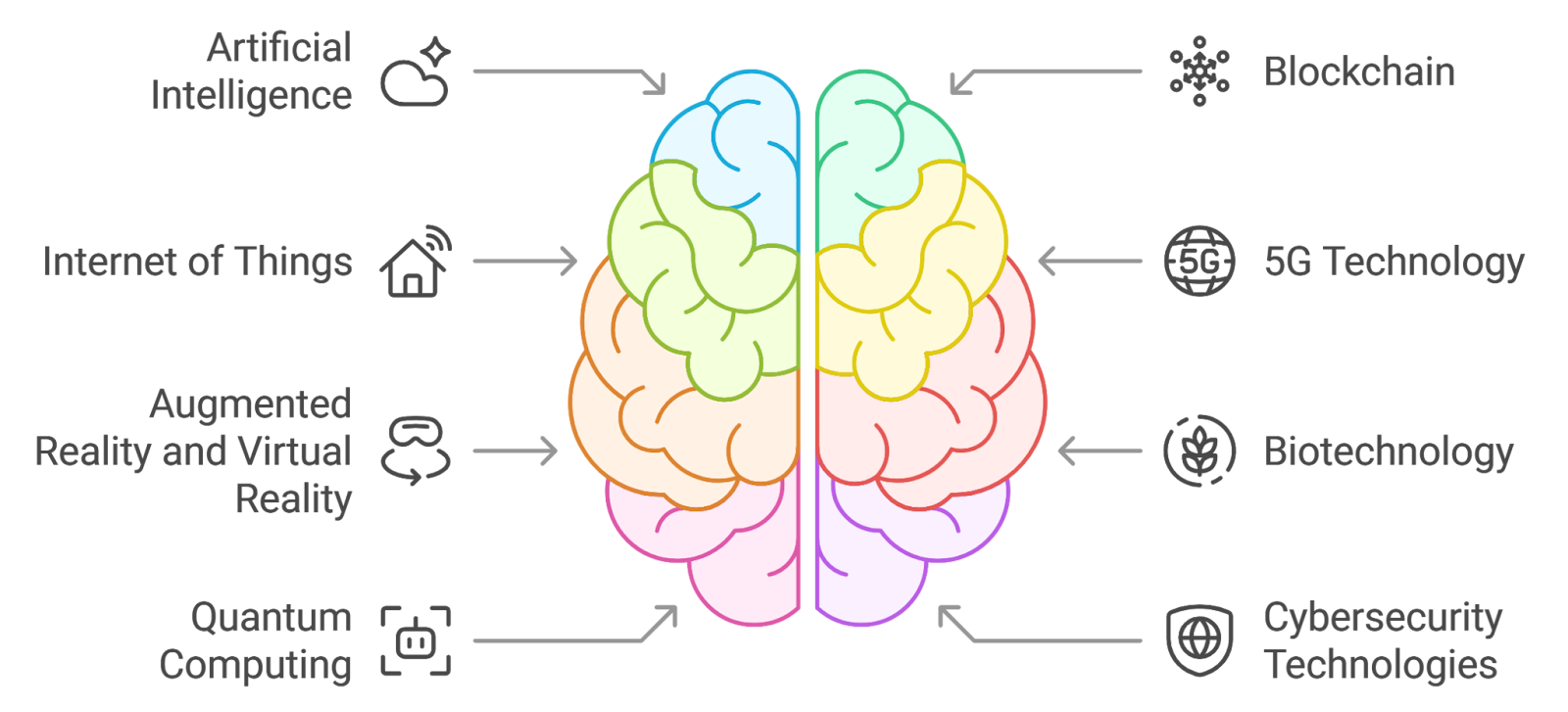
‍
Emerging technologies are reshaping industries and creating new opportunities for innovation and growth. Staying ahead of these trends is vital for businesses looking to maintain a competitive edge.
- Artificial Intelligence (AI): AI is revolutionizing various sectors, from healthcare to finance. Businesses are leveraging AI for data analysis, customer service automation, and predictive analytics. Rapid Innovation specializes in implementing AI solutions that enhance operational efficiency and drive significant ROI for our clients.
- Blockchain: This technology offers secure and transparent transaction methods, making it valuable for industries like finance, supply chain management, and healthcare. Blockchain can enhance trust and reduce fraud. At Rapid Innovation, we develop blockchain applications that streamline processes and improve data integrity, ultimately leading to better investment outcomes.
- Internet of Things (IoT): IoT connects devices and systems, enabling real-time data collection and analysis. This technology is transforming industries by improving operational efficiency and enhancing customer experiences.
- 5G Technology: The rollout of 5G networks is set to enhance connectivity and enable faster data transfer. This will support advancements in IoT, autonomous vehicles, and smart cities.
- Augmented Reality (AR) and Virtual Reality (VR): These technologies are changing how consumers interact with products and services. Businesses are using AR and VR for immersive marketing experiences and training programs. For instance, our Virtual Reality app development services can help businesses create engaging and interactive experiences.
- Biotechnology: Innovations in biotechnology are leading to breakthroughs in healthcare, agriculture, and environmental sustainability. This field is poised for significant growth as new applications are discovered.
- Quantum Computing: Although still in its infancy, quantum computing has the potential to solve complex problems much faster than traditional computers. This could revolutionize fields like cryptography, materials science, and drug discovery.
- Cybersecurity Technologies: As cyber threats become more sophisticated, businesses are investing in advanced cybersecurity measures to protect sensitive data and maintain customer trust.
By understanding and adapting to these emerging technologies, businesses can position themselves for future success and capitalize on new market opportunities. Rapid Innovation is committed to guiding our clients through these advancements, ensuring they achieve their business goals efficiently and effectively.
9.1.1. Quantum Computing Applications
Quantum computing represents a revolutionary shift in computational power and capabilities. Unlike classical computers that use bits as the smallest unit of data, quantum computers utilize qubits, which can exist in multiple states simultaneously. This unique property allows quantum computers to solve complex problems much faster than traditional systems. Key applications of quantum computing include:
- Cryptography: Quantum computing has the potential to break current encryption methods, leading to the development of quantum-resistant algorithms. This is crucial for securing sensitive data in finance, healthcare, and government sectors. At Rapid Innovation, we can assist clients in transitioning to quantum-resistant security protocols, ensuring their data remains protected against future threats.
- Drug Discovery: Quantum computers can simulate molecular interactions at an unprecedented scale, significantly speeding up the drug discovery process. This can lead to the development of new medications and treatments for various diseases. Our team can leverage quantum computing to enhance research and development efforts, ultimately reducing time-to-market for pharmaceutical innovations.
- Optimization Problems: Industries such as logistics, finance, and manufacturing can benefit from quantum computing's ability to solve optimization problems more efficiently, leading to cost savings and improved operational efficiency. Rapid Innovation can help clients implement quantum algorithms to optimize supply chains, resource allocation, and financial modeling.
- Artificial Intelligence: Quantum computing can enhance machine learning algorithms, allowing for faster data processing and improved predictive analytics. By integrating quantum computing with AI, we can help clients achieve more accurate insights and better decision-making capabilities.
9.1.2. Advanced AI Algorithms
The field of artificial intelligence (AI) is rapidly evolving, with advanced algorithms driving significant improvements in various applications. These algorithms enable machines to learn from data, make decisions, and perform tasks that traditionally required human intelligence. Some of the most notable advanced AI algorithms include:
- Deep Learning: This subset of machine learning uses neural networks with many layers to analyze data. Deep learning has revolutionized image and speech recognition, natural language processing, and autonomous systems. Rapid Innovation can implement deep learning solutions tailored to specific business needs, enhancing customer experiences and operational efficiency.
- Reinforcement Learning: This algorithm allows machines to learn optimal actions through trial and error. It is widely used in robotics, gaming, and autonomous vehicles, enabling systems to adapt to dynamic environments. Our expertise in reinforcement learning can help clients develop intelligent systems that improve over time, leading to better performance and ROI.
- Generative Adversarial Networks (GANs): GANs consist of two neural networks that compete against each other to generate realistic data. They are used in image generation, video creation, and even in art. Rapid Innovation can harness GANs to create innovative marketing content and enhance product design processes.
- Natural Language Processing (NLP): Advanced NLP algorithms enable machines to understand and generate human language. Applications include chatbots, translation services, and sentiment analysis. We can help clients implement NLP solutions that improve customer engagement and streamline communication processes.
9.1.3. IoT Integration
The Internet of Things (IoT) refers to the network of interconnected devices that communicate and exchange data. Integrating IoT with other technologies enhances efficiency, data collection, and automation across various sectors. Key areas of IoT integration include:
- Smart Homes: IoT devices like smart thermostats, lights, and security systems allow homeowners to control their environment remotely, improving energy efficiency and security. Rapid Innovation can assist in developing smart home solutions that enhance user experience and energy management.
- Healthcare Monitoring: Wearable devices and remote monitoring systems collect health data in real-time, enabling proactive healthcare management and personalized treatment plans. Our expertise in IoT can help healthcare providers implement effective monitoring solutions that improve patient outcomes.
- Industrial IoT (IIoT): In manufacturing, IoT sensors monitor equipment performance, predict maintenance needs, and optimize production processes, leading to reduced downtime and increased productivity. Rapid Innovation can support clients in deploying IIoT solutions that drive operational excellence and cost efficiency.
- Smart Cities: IoT integration in urban infrastructure can improve traffic management, waste management, and energy consumption, contributing to sustainable urban development. We can collaborate with municipalities to design and implement smart city initiatives that enhance quality of life and resource management.
At Rapid Innovation, our expertise in AI, blockchain, and IoT positions us to help clients achieve their business goals efficiently and effectively, ultimately driving greater ROI.
9.2. Smart Grid Evolution
The evolution of smart grids represents a significant shift in how electricity is generated, distributed, and consumed. Smart grids integrate advanced technologies, including AI and blockchain, to enhance the efficiency, reliability, and sustainability of energy systems.
- Enhanced Communication: Smart grids utilize two-way communication between utilities and consumers, allowing for real-time data exchange. This leads to better demand response and energy management, enabling businesses to optimize their energy usage and reduce costs.
- Integration of Renewable Energy: The evolution of smart grids facilitates the integration of renewable energy sources like solar and wind, which is crucial for reducing carbon emissions and promoting sustainable energy practices. Rapid Innovation can assist clients in implementing blockchain solutions to track and verify renewable energy credits, ensuring transparency and trust in sustainability efforts.
- Advanced Metering Infrastructure (AMI): Smart meters are a key component of smart grids, providing detailed information on energy usage. This empowers consumers to make informed decisions about their energy consumption. Our AI-driven analytics can help clients interpret this data to enhance operational efficiency and drive cost savings.
- Grid Resilience: Smart grids are designed to withstand and recover from disruptions, such as natural disasters or cyber-attacks. This resilience is vital for maintaining a stable energy supply. Rapid Innovation can provide consulting on blockchain-based solutions that enhance grid security and data integrity.
- Energy Storage Solutions: The incorporation of energy storage technologies, such as batteries, allows for better management of energy supply and demand, especially with intermittent renewable sources. Our expertise in AI can help clients optimize energy storage systems, maximizing ROI through improved energy management strategies. For instance, AI agents can be utilized to enhance the efficiency of energy systems.
9.3. Regulatory Landscape
The regulatory landscape surrounding smart grids is complex and varies significantly across regions. Governments and regulatory bodies play a crucial role in shaping the development and implementation of smart grid technologies.
- Policy Frameworks: Many countries have established policies to promote smart grid development, focusing on energy efficiency, renewable energy integration, and grid modernization. Rapid Innovation can guide clients through these frameworks to ensure compliance and capitalize on available incentives.
- Incentives and Funding: Regulatory bodies often provide financial incentives for utilities and consumers to adopt smart grid technologies, which can include grants, tax credits, and subsidies. Our consulting services can help clients identify and apply for these opportunities, enhancing their financial viability.
- Standards and Compliance: The establishment of technical standards is essential for ensuring interoperability among different smart grid components. Regulatory agencies often oversee the development and enforcement of these standards. Rapid Innovation can assist clients in navigating compliance requirements, ensuring their technologies meet industry standards.
- Consumer Protection: As smart grids collect vast amounts of data, regulations are needed to protect consumer privacy and data security, including guidelines on data usage and sharing. Our blockchain solutions can enhance data security and transparency, addressing regulatory concerns while building consumer trust.
- Stakeholder Engagement: Effective regulatory frameworks encourage collaboration among stakeholders, including utilities, technology providers, and consumers, to ensure that smart grid initiatives meet diverse needs. Rapid Innovation can facilitate stakeholder engagement strategies, ensuring that all voices are heard in the development process.
9.4. Research Directions
Research in the field of smart grids is rapidly evolving, driven by technological advancements and the need for sustainable energy solutions. Several key research directions are emerging.
- Cybersecurity: As smart grids become more interconnected, the need for robust cybersecurity measures is paramount. Research is focused on developing advanced security protocols to protect against cyber threats. Rapid Innovation can provide expertise in implementing AI-driven cybersecurity solutions tailored for smart grid applications.
- Artificial Intelligence and Machine Learning: These technologies are being explored to optimize grid operations, enhance predictive maintenance, and improve demand forecasting. AI can analyze vast amounts of data to identify patterns and make real-time decisions. Our AI solutions can help clients leverage this data for improved operational efficiency and cost savings.
- Energy Management Systems: Research is ongoing to develop sophisticated energy management systems that can optimize energy consumption at both the consumer and utility levels, including smart home technologies and demand response programs. Rapid Innovation can assist clients in designing and implementing these systems to maximize energy efficiency.
- Electric Vehicle Integration: With the rise of electric vehicles (EVs), research is focusing on how to integrate EV charging into the smart grid, including developing infrastructure and policies to support widespread EV adoption. Our blockchain solutions can facilitate secure transactions and data sharing between EVs and the grid, enhancing integration efforts.
- Microgrids and Decentralized Energy: The concept of microgrids, which can operate independently from the main grid, is gaining traction. Research is exploring how these systems can enhance energy resilience and support local energy generation. Rapid Innovation can help clients develop and implement smart micro grid solutions that align with their sustainability goals.
By focusing on these areas, researchers aim to address the challenges and opportunities presented by the evolution of smart grids, ultimately leading to a more efficient and sustainable energy future. Rapid Innovation is committed to helping clients navigate this landscape, leveraging our expertise in AI and blockchain to drive greater ROI and achieve their business objectives.
10. Implementation Strategy
An effective implementation strategy is crucial for the successful execution of any project or initiative. It outlines the steps necessary to bring a plan to fruition, ensuring that all stakeholders are aligned and that resources are utilized efficiently. This is often referred to as a strategy implementation plan.
10.1 Assessment and Planning
Assessment and planning are foundational steps in the implementation strategy. This phase involves evaluating the current situation, identifying needs, and setting clear objectives.
- Conduct a Needs Assessment: Gather data to understand the existing conditions and gaps by using surveys, interviews, and focus groups to collect qualitative and quantitative information. This process allows Rapid Innovation to tailor AI and Blockchain solutions that directly address client needs.
- Define Objectives: Establish SMART (Specific, Measurable, Achievable, Relevant, Time-bound) goals and ensure that objectives align with the overall vision of the project. For instance, a client aiming to enhance operational efficiency through AI can set specific targets for process automation.
- Identify Stakeholders: Recognize all parties involved, including team members, clients, and external partners. Engage stakeholders early to gather input and foster buy-in, ensuring that everyone is on the same page regarding the project’s goals.
- Resource Allocation: Assess available resources, including budget, personnel, and technology. Create a resource management plan to optimize the use of assets, ensuring that the right tools and technologies are in place for successful AI and Blockchain implementation.
- Risk Assessment: Identify potential risks that could impact the project and develop mitigation strategies to address these risks proactively. This is particularly important in Blockchain projects, where regulatory and security concerns may arise.
- Timeline Development: Create a detailed timeline that outlines key milestones and deadlines. Use project management tools to track progress and adjust as necessary, ensuring that the project stays on schedule.
- Communication Plan: Establish a communication strategy to keep stakeholders informed. Determine the frequency and channels of communication to ensure transparency, which is vital for maintaining trust and collaboration throughout the project.
10.2 Pilot Program Design
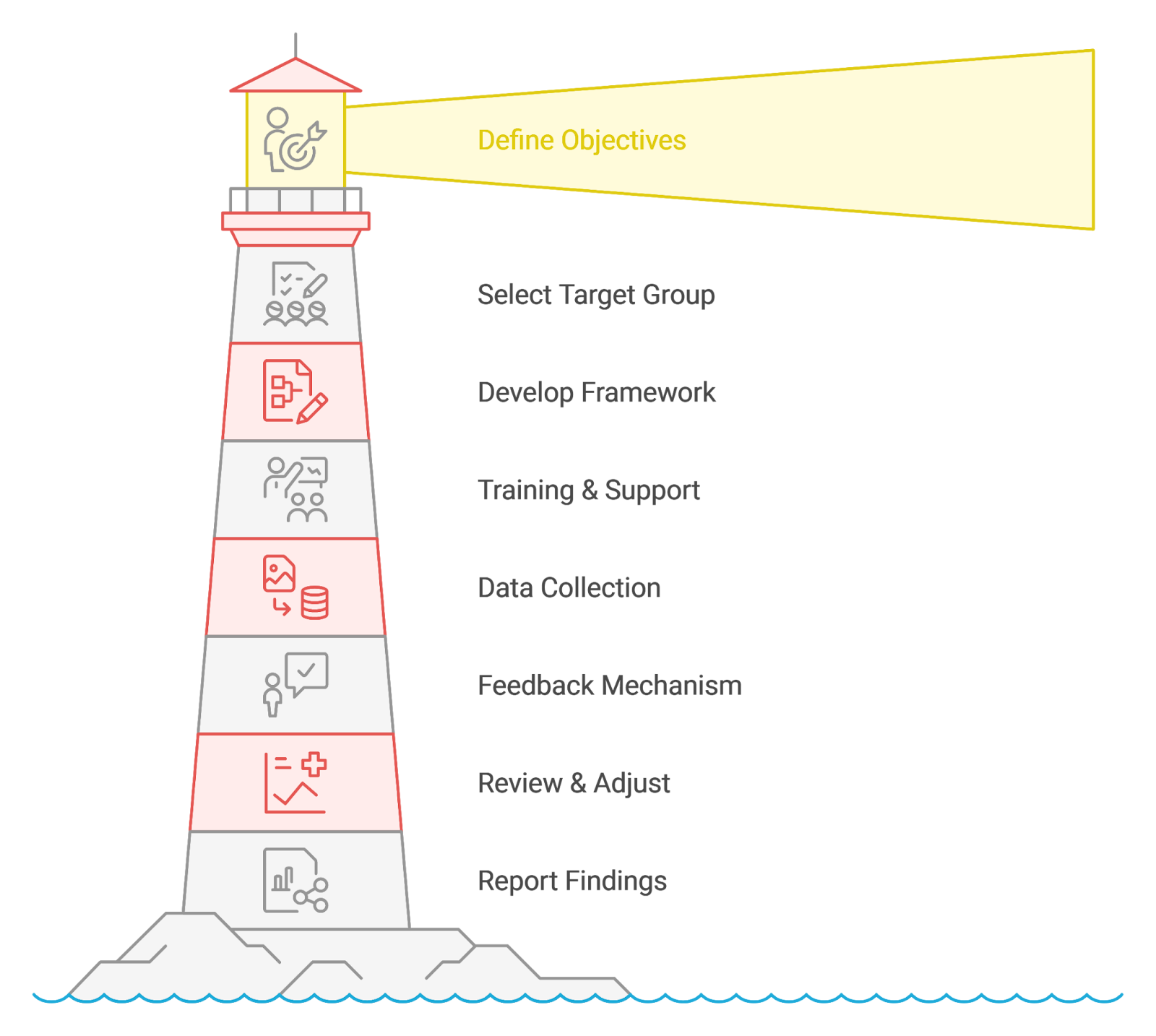
‍
Designing a pilot program is a critical step in testing the feasibility of the proposed initiative before full-scale implementation. A well-structured pilot can provide valuable insights and help refine the approach.
- Define Pilot Objectives: Clearly outline what the pilot aims to achieve, focusing on specific outcomes that will inform the larger implementation. For example, a pilot for an AI-driven customer service chatbot can measure response times and customer satisfaction.
- Select a Target Group: Identify a representative sample of the population that will be affected by the project, ensuring diversity in the sample to gather comprehensive feedback.
- Develop the Pilot Framework: Create a detailed plan that includes the scope, methodology, and evaluation criteria. Specify the duration of the pilot and the resources required, ensuring that the pilot is manageable and focused.
- Training and Support: Provide training for participants and stakeholders involved in the pilot, ensuring that support mechanisms are in place to address any challenges that arise. This is essential for the successful adoption of new technologies.
- Data Collection and Analysis: Establish methods for collecting data during the pilot phase, using both qualitative and quantitative metrics to evaluate success. This data will be crucial for assessing the effectiveness of AI algorithms or Blockchain transactions.
- Feedback Mechanism: Implement a system for gathering feedback from participants through surveys, interviews, and focus groups to assess experiences and outcomes. This feedback will guide necessary adjustments before full-scale implementation.
- Review and Adjust: Analyze the data collected to identify strengths and weaknesses, making necessary adjustments to the program based on feedback and findings. This iterative process is key to refining AI models and Blockchain applications.
- Report Findings: Document the results of the pilot program in a comprehensive report and share insights with stakeholders to inform the next steps in the implementation process.
By focusing on thorough assessment and careful pilot program design, organizations can significantly enhance the likelihood of successful implementation, ultimately achieving greater ROI through effective AI and Blockchain solutions. This aligns with the broader concepts of strategy formulation and implementation, as well as change implementation strategies that are essential for business strategy implementation.
10.3. Scaling Considerations
Scaling is a critical aspect of any business or technology initiative. It involves expanding operations, resources, or capabilities to meet increasing demand or to enhance performance. Here are some key considerations for scaling effectively:
- Infrastructure: Ensure that your infrastructure can handle increased loads. This may involve upgrading servers, optimizing databases, or utilizing cloud services for flexibility. Rapid Innovation can assist in designing a robust infrastructure that leverages AI and blockchain technologies to enhance scalability and performance.
- Team Capacity: Assess whether your current team can manage the increased workload. Hiring additional staff or training existing employees may be necessary. Our consulting services can help identify skill gaps and provide tailored training programs to empower your team.
- Process Optimization: Streamline processes to eliminate bottlenecks. This can include automating repetitive tasks or refining workflows to improve efficiency. By implementing AI-driven automation solutions, Rapid Innovation can help you achieve significant time savings and operational efficiency.
- Technology Stack: Evaluate your technology stack for scalability. Choose tools and platforms that can grow with your business, such as microservices architecture or serverless computing. Our expertise in blockchain can also provide decentralized solutions that enhance scalability and security.
- Customer Support: As you scale, customer support becomes crucial. Implement scalable support solutions, such as chatbots or helpdesk software, to maintain service quality. Rapid Innovation can develop AI-powered customer support systems that ensure prompt and effective responses to customer inquiries.
- Market Demand: Analyze market trends to anticipate demand. Use data analytics to make informed decisions about scaling operations. Our data analytics services can provide insights that help you align your scaling efforts with market opportunities.
- Financial Resources: Ensure you have the financial backing to support scaling efforts. This may involve securing investments or reallocating budgets. Rapid Innovation can assist in developing financial models that project ROI from scaling initiatives, ensuring you make informed investment decisions.
- Business Scaling Strategies: Implement effective business scaling strategies that align with your overall growth objectives. This may include diversifying your product offerings or expanding into new markets.
- Scalable Growth Strategy: Develop a scalable growth strategy that focuses on sustainable practices and long-term success. This involves creating systems and processes that can easily adapt to increased demand without compromising quality.
- Scaling Growth Strategy: Formulate a scaling growth strategy that emphasizes innovation and efficiency. This can help your organization respond quickly to market changes and capitalize on new opportunities. For comprehensive support in developing your enterprise AI solutions, consider our Enterprise AI Development services. Additionally, learn from real-world AI implementations to enhance your scaling strategies.
10.4. Performance Metrics
Performance metrics are essential for measuring the success of your initiatives and understanding areas for improvement. They provide insights into how well your business is performing and help guide decision-making. Key performance metrics to consider include:
- Key Performance Indicators (KPIs): Identify specific KPIs relevant to your goals. These could include sales growth, customer acquisition cost, or customer satisfaction scores.
- Operational Efficiency: Measure how efficiently resources are being used. Metrics like cycle time, throughput, and resource utilization can provide valuable insights.
- Customer Engagement: Track metrics related to customer interactions, such as Net Promoter Score (NPS), customer retention rate, and average response time.
- Financial Performance: Monitor financial metrics, including revenue growth, profit margins, and return on investment (ROI). These figures are crucial for assessing overall business health.
- Employee Performance: Evaluate employee productivity and satisfaction through metrics like employee turnover rate and performance reviews.
- Market Share: Analyze your market position relative to competitors. Understanding your market share can help identify growth opportunities.
10.5. Continuous Improvement Framework
A continuous improvement framework is vital for fostering a culture of ongoing enhancement within an organization. It encourages teams to regularly assess and refine processes, products, and services. Key components of a continuous improvement framework include:
- Plan-Do-Check-Act (PDCA): This iterative process involves planning changes, implementing them, checking results, and acting on what is learned. It promotes systematic improvement.
- Feedback Loops: Establish mechanisms for gathering feedback from employees, customers, and stakeholders. This information is crucial for identifying areas needing improvement.
- Training and Development: Invest in employee training to equip them with the skills necessary for continuous improvement. This can lead to innovative ideas and enhanced performance.
- Data-Driven Decision Making: Utilize data analytics to inform decisions. Analyzing performance metrics can highlight trends and areas for improvement.
- Cross-Functional Collaboration: Encourage collaboration across departments to share insights and best practices. This can lead to more comprehensive solutions and improvements.
- Recognition and Rewards: Implement a system to recognize and reward employees who contribute to continuous improvement efforts. This can motivate teams to engage in the process actively.
- Regular Reviews: Schedule regular reviews of processes and performance metrics to ensure that improvement initiatives are on track and effective.
11. Case Studies
Case studies provide valuable insights into real-world applications of theories and practices. They illustrate how concepts are implemented, the challenges faced, and the outcomes achieved. This section will explore successful implementations and the lessons learned from various case studies, particularly in the realms of AI and blockchain case studies, showcasing how Rapid Innovation can help clients achieve their business goals efficiently and effectively.
11.1 Successful Implementations
Successful implementations of strategies or technologies can serve as a blueprint for others. Here are some notable examples:
- Company A: Digital Transformation
Rapid Innovation assisted in implementing a comprehensive digital strategy that integrated AI-driven data analytics and cloud computing. This resulted in a 30% increase in operational efficiency and a 25% reduction in costs. Additionally, it enhanced customer engagement through personalized marketing campaigns powered by machine learning algorithms. - Company B: Blockchain for Sustainability Initiatives
Collaborating with Rapid Innovation, this company adopted a zero-waste policy and utilized blockchain technology to track and verify its renewable energy investments. This initiative achieved a 40% reduction in carbon footprint within three years and gained recognition as a leader in corporate social responsibility, significantly boosting brand loyalty. - Company C: Agile Methodology with AI Integration
Rapid Innovation guided this organization in transitioning to an agile project management approach in software development, enhanced by AI tools for project tracking and resource allocation. This improved project delivery times by 50% and increased team collaboration, fostering a culture of continuous improvement and innovation.
These successful implementations highlight the importance of strategic planning, stakeholder engagement, and adaptability in achieving desired outcomes, particularly when leveraging AI and blockchain technologies.
11.2 Lessons Learned
Every case study offers valuable lessons that can inform future practices. Here are some key takeaways from various implementations:
- Importance of Stakeholder Engagement
Engaging stakeholders early in the process can lead to better buy-in and smoother implementation. Regular communication helps address concerns and fosters collaboration, especially when introducing advanced technologies like AI and blockchain. - Flexibility and Adaptability
Being open to change and adapting strategies based on feedback can enhance success. Organizations that embrace a culture of flexibility are better equipped to navigate challenges, particularly in the fast-evolving tech landscape. - Data-Driven Decision Making
Utilizing data analytics to inform decisions can lead to more effective strategies. Continuous monitoring and evaluation of outcomes are essential for ongoing improvement, particularly when integrating AI solutions. - Training and Development
Investing in employee training ensures that teams are equipped with the necessary skills to leverage AI and blockchain technologies effectively. Ongoing development fosters a culture of learning and innovation. - Sustainability Focus
Incorporating sustainability into business practices can lead to long-term benefits. Companies that prioritize environmental responsibility, supported by technologies like blockchain for transparency, often see enhanced brand reputation.
These lessons learned from successful implementations can guide organizations in their future endeavors, helping them to avoid common pitfalls and capitalize on best practices, particularly in the context of AI and blockchain solutions offered by Rapid Innovation.
11.3. Best Practices

‍
Implementing best practices in any field is crucial for achieving optimal results and maintaining a competitive edge. Here are some key best practices to consider:
- Continuous Learning: Stay updated with the latest trends and technologies, particularly in AI and blockchain best practices. Regular training and workshops can enhance skills and knowledge, ensuring your team is equipped to leverage these advancements effectively.
- Data-Driven Decision Making: Utilize analytics and data to inform decisions. In the context of AI, this means harnessing machine learning algorithms to analyze customer behavior and market trends, which minimizes risks and maximizes opportunities.
- Collaboration and Communication: Foster a culture of open communication and teamwork. Effective collaboration can lead to innovative solutions, especially when integrating AI and blockchain technologies into your business processes.
- Customer-Centric Approach: Always prioritize the needs and feedback of customers. Understanding their preferences can guide product development and service enhancements, ensuring that your AI and blockchain solutions are aligned with market demands.
- Sustainability Practices: Incorporate eco-friendly practices into operations. This not only benefits the environment but also appeals to a growing base of environmentally conscious consumers, particularly in sectors utilizing blockchain for transparency in sustainable sourcing.
- Agile Methodologies: Adopt agile practices to enhance flexibility and responsiveness. This allows teams to adapt quickly to changes in the market or project requirements, which is essential when deploying AI and blockchain solutions.
- Regular Performance Reviews: Conduct frequent assessments of processes and outcomes. This helps identify areas for improvement and ensures alignment with strategic goals, particularly in the implementation of AI-driven analytics and blockchain systems.
- Invest in Technology: Leverage the latest technologies to streamline operations. Automation and advanced software, especially those powered by AI, can significantly enhance efficiency and accuracy in your business processes.
- Risk Management: Develop a robust risk management strategy. Identifying potential risks early, particularly in the context of blockchain transactions, can mitigate their impact on the organization.
- Networking and Partnerships: Build relationships with industry peers and stakeholders. Networking can open doors to new opportunities and collaborations, particularly in the rapidly evolving fields of AI and blockchain.
11.4. Future Opportunities
The future holds numerous opportunities across various sectors, driven by technological advancements and changing consumer behaviors. Here are some promising areas to explore:
- Artificial Intelligence and Machine Learning: The integration of AI and machine learning into business processes can enhance efficiency and decision-making. Companies that harness these technologies can gain a significant competitive advantage, leading to greater ROI.
- Remote Work Solutions: The shift towards remote work has created a demand for innovative tools and platforms that facilitate collaboration and productivity. Businesses can capitalize on this trend by developing or investing in remote work technologies.
- Sustainable Products and Services: As consumers become more environmentally conscious, there is a growing market for sustainable products. Companies that prioritize sustainability in their offerings can attract a loyal customer base.
- Health and Wellness: The focus on health and wellness continues to rise. Opportunities exist in developing products and services that promote physical and mental well-being.
- E-commerce Expansion: The e-commerce sector is booming, with more consumers shopping online than ever before. Businesses can explore new markets and enhance their online presence to tap into this growth.
- Blockchain Technology: Blockchain offers transparency and security in transactions. Industries such as finance, supply chain, and healthcare can benefit from implementing blockchain solutions, enhancing trust and efficiency.
- Personalization: Consumers increasingly expect personalized experiences. Companies that leverage data to tailor their offerings can improve customer satisfaction and loyalty.
- Cybersecurity Solutions: With the rise in digital transactions, the need for robust cybersecurity measures is paramount. There is a growing demand for innovative cybersecurity solutions to protect sensitive information.
- Smart Home Technology: The smart home market is expanding rapidly. Opportunities exist for businesses that develop products that enhance convenience, security, and energy efficiency in homes.
- Education Technology: The shift towards online learning has created opportunities in the education technology sector. Companies that provide innovative learning solutions can meet the evolving needs of students and educators.
At Rapid Innovation, we are committed to helping our clients navigate these best practices and future opportunities, ensuring they achieve their business goals efficiently and effectively through our AI and blockchain expertise.