Table Of Contents
Category
Artificial Intelligence
AIML
IoT
Blockchain
Healthcare & Medicine
1. Introduction to AI in Genomics
Artificial Intelligence (AI) is revolutionizing the field of genomics by enhancing the analysis and interpretation of genomic data. The integration of AI technologies into genomics is enabling researchers and healthcare professionals to make more informed decisions, leading to improved patient outcomes and advancements in personalized medicine.
- AI algorithms can process vast amounts of genomic data quickly and accurately, allowing for more efficient research and development cycles.
- Machine learning models can identify patterns and correlations that may not be evident through traditional analysis, providing insights that drive innovation in treatment strategies.
- AI tools are increasingly being used to predict disease susceptibility, treatment responses, and potential drug interactions, ultimately leading to better patient management and care.
The application of AI in genomics is not just limited to data analysis; it also encompasses various aspects of research and clinical practice. By leveraging AI, researchers can accelerate the discovery of new biomarkers and therapeutic targets, ultimately leading to more effective treatments and a higher return on investment (ROI) for healthcare organizations.
- AI can assist in the identification of genetic variants associated with diseases, streamlining the diagnostic process and reducing time to treatment.
- Natural language processing (NLP) can be used to extract relevant information from scientific literature and clinical records, enhancing the knowledge base available to researchers and clinicians.
- AI-driven platforms can facilitate collaboration among researchers by providing shared access to genomic data, fostering innovation and accelerating breakthroughs in the field.
As the field of genomics continues to evolve, the role of AI will become increasingly significant. The combination of AI and genomics holds the potential to transform healthcare by enabling precision medicine, where treatments are tailored to the individual characteristics of each patient. The emergence of AI in genomics is also reflected in the growing number of AI genomics companies that are developing innovative solutions. At Rapid Innovation, we are committed to helping our clients harness the power of AI in genomics to achieve their business goals efficiently and effectively, ultimately driving greater ROI and improving patient outcomes. The integration of artificial intelligence in clinical and genomic diagnostics is paving the way for a new era in healthcare, where AI in genomics market dynamics will continue to evolve.
1.1. Evolution of Genomic Data Processing
The evolution of genomic data processing has been a remarkable journey, driven by advancements in technology and an increasing understanding of genetics.
- Early sequencing methods, such as Sanger sequencing, laid the groundwork for genomic research but were time-consuming and costly.
- The introduction of next-generation sequencing (NGS) revolutionized the field, allowing for rapid sequencing of entire genomes at a fraction of the cost. This shift enabled large-scale genomic studies and personalized medicine.
- The Human Genome Project, completed in 2003, was a landmark achievement that provided a reference genome and spurred the development of bioinformatics tools for gene raw data analysis.
- As genomic data grew exponentially, the need for efficient data processing became critical. High-throughput sequencing technologies generated terabytes of data, necessitating robust computational frameworks.
- Cloud computing and big data technologies emerged, allowing researchers to store, manage, and analyze vast amounts of genomic data more effectively.
- The integration of machine learning and artificial intelligence into genomic data processing has further enhanced the ability to interpret complex datasets, leading to breakthroughs in understanding genetic diseases and drug development. At Rapid Innovation, we leverage these advancements to provide tailored AI solutions that optimize genomic data processing, ensuring our clients achieve greater efficiency and ROI. Additionally, understanding the importance of data quality is crucial for successful AI implementations in this field.
1.2. Current Challenges in Genomic Analysis
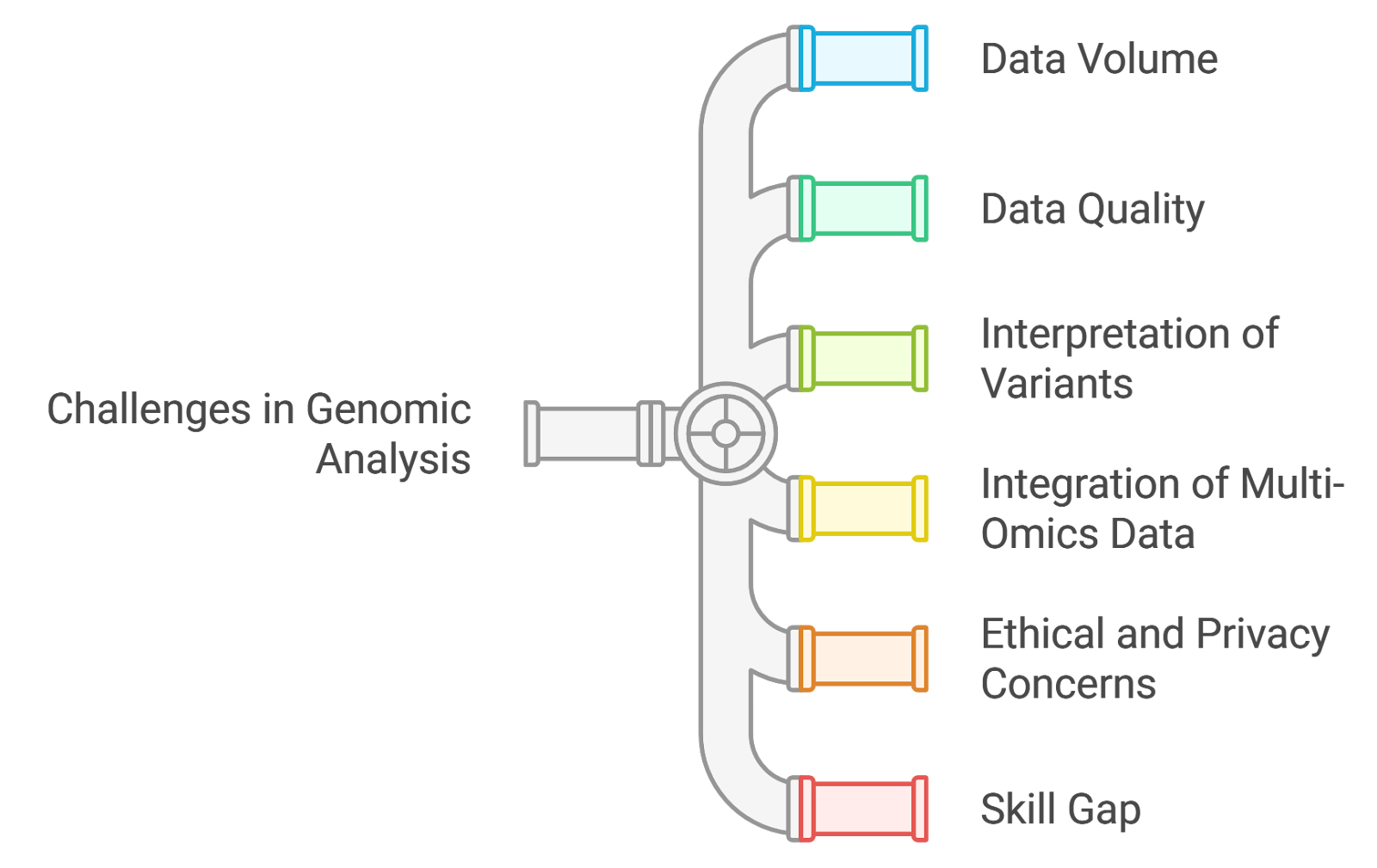
‍
Despite the advancements in genomic data processing, several challenges persist in the field of genomic analysis.
- Data Volume: The sheer volume of genomic data generated poses significant storage and processing challenges. Managing terabytes of data requires sophisticated infrastructure and resources.
- Data Quality: Ensuring the accuracy and reliability of genomic data is crucial. Errors in sequencing can lead to incorrect interpretations and conclusions, impacting research and clinical outcomes.
- Interpretation of Variants: Identifying and interpreting genetic variants remains a complex task. Many variants have unknown significance, making it difficult to determine their role in diseases.
- Integration of Multi-Omics Data: Combining genomic data with other omics data (like transcriptomics and proteomics) is essential for a comprehensive understanding of biological systems. However, integrating these diverse datasets presents technical and analytical challenges.
- Ethical and Privacy Concerns: The use of genomic data raises ethical issues, particularly regarding consent, data sharing, and privacy. Ensuring that individuals' genetic information is protected is paramount.
- Skill Gap: There is a shortage of trained professionals who can analyze and interpret genomic data effectively. Bridging this skill gap is essential for advancing genomic research and applications. Rapid Innovation addresses this challenge by providing consulting services that equip organizations with the necessary expertise and tools to navigate the complexities of genomic analysis.
1.3. Role of AI Agents in Modern Genomics
Artificial intelligence (AI) agents are playing an increasingly vital role in modern genomics, transforming how researchers analyze and interpret genomic data.
- Data Analysis: AI algorithms can process vast amounts of genomic data quickly and accurately, identifying patterns and correlations that may be missed by traditional methods.
- Predictive Modeling: Machine learning models can predict disease susceptibility based on genetic information, enabling personalized medicine approaches tailored to individual patients.
- Variant Classification: AI tools assist in the classification of genetic variants, helping researchers determine their potential impact on health and disease. This is particularly useful in clinical genomics.
- Drug Discovery: AI is being used to identify potential drug targets and predict the efficacy of new compounds based on genomic data, accelerating the drug discovery process.
- Automation: AI agents can automate repetitive tasks in genomic analysis, freeing up researchers to focus on more complex problems and enhancing overall productivity.
- Enhanced Collaboration: AI platforms facilitate collaboration among researchers by providing shared tools and resources, fostering innovation and accelerating discoveries in genomics.
The integration of AI in genomics is not just a trend; it is reshaping the landscape of genetic research and clinical applications, paving the way for more effective and personalized healthcare solutions. At Rapid Innovation, we are committed to harnessing the power of AI to help our clients overcome challenges in genomic analysis, ultimately driving greater ROI and advancing the field of genomics.
1.4. Overview of Key Technologies and Frameworks
Artificial Intelligence (AI) has become a transformative force in various fields, including genomics. The integration of AI technologies and frameworks is crucial for enhancing genomic research and applications. Here are some key technologies and frameworks that are shaping the landscape of AI in genomics:
- Machine Learning (ML): This subset of AI focuses on algorithms that allow computers to learn from and make predictions based on data. In genomics, ML is used for tasks such as gene expression analysis, variant calling, and predicting disease susceptibility. Rapid Innovation leverages ML to develop tailored solutions that help clients achieve significant improvements in their genomic research outcomes. Companies specializing in AI genomics are increasingly utilizing these techniques to drive innovation.
- Deep Learning (DL): A more advanced form of ML, deep learning utilizes neural networks with many layers. It excels in processing large datasets, making it ideal for analyzing complex genomic data, such as whole-genome sequencing. Our expertise in DL enables us to assist clients in extracting deeper insights from their genomic data, ultimately leading to better decision-making. The application of deep learning in genomics is a key area of focus for AI in genomics.
- Natural Language Processing (NLP): NLP techniques are employed to extract meaningful information from unstructured data sources, such as scientific literature and clinical notes. This helps in identifying relevant genomic information and trends. Rapid Innovation utilizes NLP to enhance data interpretation, allowing clients to stay ahead in their research endeavors. The integration of NLP in genomics is essential for understanding the vast amount of literature related to artificial intelligence in clinical and genomic diagnostics.
- Bioinformatics Tools: Various bioinformatics frameworks, such as Bioconductor and Galaxy, provide essential tools for data analysis and visualization in genomics. These platforms facilitate the integration of AI algorithms with genomic data. We assist clients in implementing these tools effectively, ensuring they maximize their research capabilities. The use of bioinformatics tools is critical for companies focused on AI in genomics.
- Cloud Computing: The scalability and flexibility of cloud computing enable researchers to store and analyze vast amounts of genomic data efficiently. Platforms like AWS and Google Cloud offer specialized services for genomic data processing. Rapid Innovation helps clients harness cloud solutions to optimize their data management and analysis processes, leading to enhanced operational efficiency. The cloud computing landscape is vital for the growth of AI in genomics.
- Data Integration Frameworks: Tools like Apache Spark and TensorFlow Extended (TFX) allow for the integration of diverse data sources, enhancing the ability to analyze genomic data alongside clinical and environmental data. Our expertise in these frameworks enables clients to create comprehensive datasets that drive more accurate insights. The integration of data from various sources is essential for the success of AI in genomics.
- Genomic Databases: Resources such as The Cancer Genome Atlas (TCGA) and the Genome Aggregation Database (gnomAD) provide extensive datasets that can be leveraged by AI algorithms for research and clinical applications. Rapid Innovation guides clients in utilizing these databases effectively, ensuring they derive maximum value from their genomic research. The availability of genomic databases is a cornerstone for AI in genomics, enabling researchers to access critical information.
These technologies and frameworks collectively enhance the capabilities of AI in genomics, leading to improved diagnostics, personalized medicine, and a deeper understanding of genetic diseases. The intersection of artificial intelligence and genomics is paving the way for innovative solutions in healthcare.
2. Fundamental Components of AI Agents in Genomics
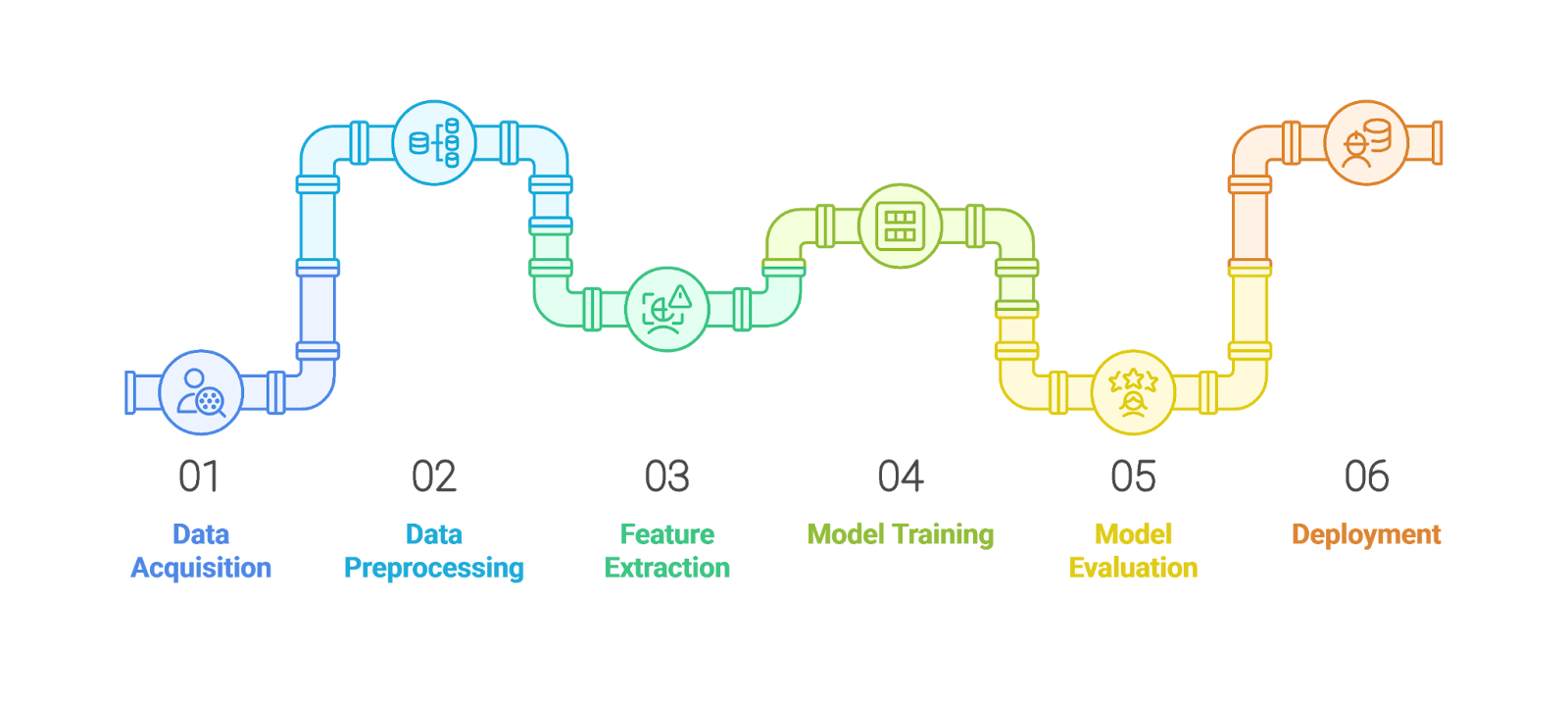
‍
AI agents in genomics are designed to perform specific tasks that enhance genomic research and clinical applications. Understanding the fundamental components of these agents is essential for leveraging their capabilities effectively. The key components include:
- Data Acquisition: This involves collecting genomic data from various sources, including sequencing technologies, clinical databases, and public repositories. High-quality data is crucial for training AI models. The role of AI in genomics is to streamline this process and ensure data integrity.
- Data Preprocessing: Raw genomic data often contains noise and inconsistencies. Preprocessing steps, such as normalization, filtering, and transformation, are necessary to prepare the data for analysis. Effective preprocessing is vital for the success of AI in genomics applications.
- Feature Extraction: Identifying relevant features from genomic data is critical for model performance. Techniques such as dimensionality reduction and selection algorithms help in isolating significant variables that influence outcomes. The application of machine learning and genomics is particularly relevant in this phase.
- Model Training: AI agents utilize machine learning algorithms to learn patterns from the processed data. This phase involves selecting appropriate models, tuning hyperparameters, and validating performance using training and test datasets. The training of models is a crucial step in the development of AI in genomics.
- Model Evaluation: After training, models must be evaluated to ensure their accuracy and reliability. Metrics such as precision, recall, and F1-score are commonly used to assess model performance in genomic applications. The evaluation process is essential for ensuring the effectiveness of AI in genomics.
- Deployment: Once validated, AI models can be deployed in clinical settings or research environments. This may involve integrating the models into existing workflows or developing user-friendly interfaces for researchers and clinicians. The deployment of AI solutions in genomics is a key factor in translating research into practice.
- Continuous Learning: AI agents should be designed to adapt and improve over time. Continuous learning mechanisms allow models to update their knowledge base as new genomic data becomes available, ensuring they remain relevant and effective. This adaptability is crucial for the ongoing evolution of AI in genomics.
2.1. Data Processing Architecture
The data processing architecture for AI agents in genomics is a critical component that determines how genomic data is handled, analyzed, and utilized. A well-structured architecture ensures efficient data flow and processing, enabling the effective application of AI techniques. Key elements of this architecture include:
- Data Ingestion: This is the initial step where genomic data is collected from various sources. It can include high-throughput sequencing data, clinical records, and public genomic databases. The role of AI in genomics is to facilitate this ingestion process.
- Data Storage: Efficient storage solutions are necessary to handle the large volumes of genomic data. Options include relational databases, NoSQL databases, and cloud storage solutions that provide scalability and accessibility. The storage of genomic data is a foundational aspect of AI in genomics.
- Data Processing Pipeline: A robust processing pipeline is essential for transforming raw data into usable formats. This pipeline typically includes: Â
- Data Cleaning: Removing errors and inconsistencies from the data.
- Data Transformation: Converting data into a suitable format for analysis, such as converting sequence data into numerical representations.
- Data Integration: Combining data from multiple sources to create a comprehensive dataset for analysis. The integration of diverse data sources is a hallmark of AI in genomics.
- Computational Resources: High-performance computing resources, including GPUs and distributed computing frameworks, are often required to process large genomic datasets efficiently. The computational demands of AI in genomics necessitate robust infrastructure.
- Analysis and Modeling: This stage involves applying AI algorithms to the processed data. It includes model training, evaluation, and optimization to ensure accurate predictions and insights. The analysis phase is where the true power of AI in genomics is realized.
- Visualization and Reporting: Effective visualization tools are necessary for interpreting genomic data and AI model outputs. Dashboards and reporting tools help researchers and clinicians understand the results and make informed decisions. Visualization is a critical component of communicating findings in AI and genomics.
- Feedback Loop: Incorporating a feedback mechanism allows for continuous improvement of the data processing architecture. This can involve updating algorithms based on new findings or user feedback to enhance performance. The feedback loop is essential for the iterative nature of AI in genomics.
By establishing a comprehensive data processing architecture, AI agents in genomics can effectively analyze complex datasets, leading to significant advancements in genomic research and personalized medicine. Rapid Innovation is committed to providing the expertise and solutions necessary for clients to achieve these advancements efficiently and effectively, including the exploration of AI genomics companies and the market for AI in genomics. For more insights on model development, you can refer to best practices for transformer model development.
2.1.1. Raw Data Preprocessing
Raw data preprocessing is a critical step in data analysis and machine learning. It involves transforming raw data into a clean and usable format, which is essential for ensuring the accuracy and reliability of the results derived from data analysis. At Rapid Innovation, we leverage our expertise in AI to streamline this process, enabling clients to achieve greater ROI through enhanced data quality.
- Data Cleaning: This involves identifying and correcting errors or inconsistencies in the data. Common tasks include: Â
- Removing duplicates
- Filling in missing values
- Correcting typos or formatting issues
- Data Transformation: This step modifies the data into a suitable format for analysis. Techniques include: Â
- Aggregating data to summarize information
- Encoding categorical variables into numerical formats
- Scaling numerical values to a standard range
- Data Integration: Combining data from different sources can provide a more comprehensive view. This may involve: Â
- Merging datasets from various databases
- Ensuring consistency in data formats across sources
- Data Reduction: Reducing the volume of data while maintaining its integrity is crucial. Methods include: Â
- Dimensionality reduction techniques like PCA (Principal Component Analysis)
- Sampling methods to select a representative subset of the data
Effective raw data preprocessing, including data preprocessing techniques in machine learning, can significantly enhance the quality of insights derived from data analysis, leading to better decision-making and ultimately driving business success.
2.1.2. Quality Control Systems
Quality control systems are essential for maintaining the integrity and reliability of data throughout its lifecycle. These systems ensure that data meets predefined standards and is suitable for analysis. Rapid Innovation implements robust quality control measures to help clients maintain high data standards, which is vital for informed decision-making.
- Data Validation: This process checks the accuracy and quality of data before it is used. Key aspects include: Â
- Implementing validation rules to catch errors
- Using automated tools to flag inconsistencies
- Monitoring and Auditing: Regular monitoring of data processes helps identify issues early. This includes: Â
- Conducting periodic audits of data sources
- Tracking changes and updates to data over time
- Error Reporting and Correction: Establishing a system for reporting and correcting errors is vital. This can involve: Â
- Creating a feedback loop for users to report discrepancies
- Implementing corrective actions to address identified issues
- Standard Operating Procedures (SOPs): Developing SOPs for data handling ensures consistency and quality. This includes: Â
- Defining roles and responsibilities for data management
- Outlining procedures for data entry, processing, and storage
Implementing robust quality control systems can lead to higher data accuracy, which is crucial for effective analysis and decision-making.
2.1.3. Data Normalization Techniques
Data normalization techniques are essential for preparing data for analysis, particularly in machine learning. Normalization ensures that different features contribute equally to the analysis, preventing bias towards certain variables. At Rapid Innovation, we utilize these techniques to enhance the performance of AI models, ensuring our clients achieve optimal results.
- Min-Max Normalization: This technique rescales the data to a fixed range, typically [0, 1]. It is useful for: Â
- Ensuring that all features have the same scale
- Making it easier to compare different features
- Z-Score Normalization: Also known as standardization, this method transforms data to have a mean of 0 and a standard deviation of 1. Benefits include: Â
- Reducing the impact of outliers
- Making the data distribution more Gaussian-like
- Decimal Scaling: This technique involves moving the decimal point of values to normalize the data. It is particularly useful for: Â
- Simplifying the representation of large numbers
- Ensuring that all values fall within a specific range
- Robust Scaler: This method uses the median and interquartile range for normalization, making it less sensitive to outliers. It is beneficial for: Â
- Maintaining the integrity of data with extreme values
- Providing a more accurate representation of the central tendency
Utilizing appropriate data normalization techniques, including data preprocessing algorithms, can enhance the performance of machine learning models and improve the interpretability of results, ultimately leading to greater ROI for our clients.
2.2. Machine Learning Components
Machine learning is a subset of artificial intelligence that enables systems to learn from data, identify patterns, and make decisions with minimal human intervention. The components of machine learning can be broadly categorized into various types, each serving a unique purpose in the data analysis process. Understanding these components is crucial for anyone looking to leverage machine learning in their projects, and Rapid Innovation is here to guide you through this journey to achieve your business goals efficiently and effectively.
- Data: The foundation of any machine learning model. The quality and quantity of data significantly impact the model's performance. At Rapid Innovation, we emphasize the importance of data quality and assist clients in curating and cleaning their datasets to maximize model efficacy.
- Algorithms: The mathematical procedures that process data and learn from it. Different algorithms are suited for different types of problems. Our team at Rapid Innovation employs a tailored approach, selecting the most appropriate algorithms based on the specific needs of your project to ensure optimal results. This includes techniques such as PCA (Principal Component Analysis) algorithm in machine learning, which is essential for dimensionality reduction.
- Models: The output of the training process, representing the learned patterns from the data. We help clients develop robust models that can adapt to changing data landscapes, ensuring long-term value and return on investment (ROI). For instance, we utilize PCA in machine learning python to enhance model performance.
- Features: The individual measurable properties or characteristics used by the model to make predictions. Our experts work closely with clients to identify and engineer the most relevant features, enhancing model performance and accuracy. This includes understanding the applications of PCA in machine learning to select the right features.
- Training and Testing: The process of training a model on a dataset and then testing its performance on unseen data. Rapid Innovation employs rigorous training and testing methodologies to validate model performance, ensuring that our clients can trust the insights generated.
2.2.1. Supervised Learning Models
Supervised learning is a type of machine learning where the model is trained on labeled data. This means that the input data is paired with the correct output, allowing the model to learn the relationship between the two. Supervised learning is widely used for classification and regression tasks.
- Classification: The task of predicting a categorical label, such as determining whether an email is spam or not. Rapid Innovation has successfully implemented classification models for various clients, enhancing their operational efficiency.
- Regression: The task of predicting a continuous value, for instance, predicting house prices based on various features like size and location. Our regression models have helped clients in real estate and finance make data-driven decisions that lead to increased profitability.
- Algorithms: Common algorithms include: Â
- Linear Regression
- Decision Trees
- Support Vector Machines (SVM)
- Neural Networks
- Applications: Supervised learning is used in various fields, including: Â
- Healthcare for disease diagnosis
- Finance for credit scoring
- Marketing for customer segmentation
The effectiveness of supervised learning models largely depends on the quality of the labeled data. A well-labeled dataset can significantly enhance the model's accuracy and reliability, and at Rapid Innovation, we ensure that our clients have access to high-quality data for their projects.
2.2.2. Unsupervised Learning Approaches
Unsupervised learning, in contrast to supervised learning, deals with unlabeled data. The model attempts to learn the underlying structure of the data without any explicit instructions on what to predict. This approach is particularly useful for exploratory data analysis and pattern recognition.
- Clustering: The process of grouping similar data points together. Common algorithms include: Â
- K-Means
- Hierarchical Clustering
- DBSCAN
- Dimensionality Reduction: Techniques used to reduce the number of features in a dataset while preserving its essential characteristics. Popular methods include: Â
- Principal Component Analysis (PCA)
- Kernel PCA in machine learning
- t-Distributed Stochastic Neighbor Embedding (t-SNE)
- Anomaly Detection: Identifying unusual data points that do not fit the expected pattern. This is crucial in fraud detection and network security, areas where Rapid Innovation has delivered significant value to clients.
- Applications: Unsupervised learning is widely applied in various domains, such as: Â
- Market basket analysis in retail
- Customer segmentation in marketing
- Image classification using PCA python
- Image compression in computer vision
Unsupervised learning is particularly valuable when labeled data is scarce or expensive to obtain. It allows organizations to uncover hidden patterns and insights from their data, leading to more informed decision-making. At Rapid Innovation, we leverage these techniques to help our clients gain a competitive edge in their respective markets, including the use of eigenvectors and eigenvalues in machine learning for deeper insights. For a comprehensive understanding of machine learning.
2.2.3. Deep Learning Architectures
Deep learning architectures are a subset of machine learning that utilize neural networks with multiple layers to analyze various types of data. These architectures have gained significant traction in recent years due to their ability to model complex patterns and relationships in large datasets, providing businesses with the tools to enhance decision-making and operational efficiency.
- Convolutional Neural Networks (CNNs): Â
- Primarily used for image processing tasks.
- Effective in identifying spatial hierarchies in data.
- Commonly applied in genomics for analyzing genomic sequences and images from microscopy, enabling researchers to derive insights that can lead to breakthroughs in personalized medicine. Architectures such as vgg16 architecture and vgg19 are popular examples of CNNs used in various applications.
- Recurrent Neural Networks (RNNs): Â
- Designed for sequential data analysis.
- Useful in tasks where context and order matter, such as time series data or natural language.
- In genomics, RNNs can be employed to predict gene expression levels based on previous sequences, allowing for more accurate forecasting of biological behaviors. The architecture of recurrent neural network is particularly suited for these tasks.
- Generative Adversarial Networks (GANs): Â
- Comprise two neural networks, a generator and a discriminator, that work against each other.
- Effective in generating new data samples that resemble the training data.
- In genomics, GANs can be used to synthesize new genomic sequences for research purposes, thus accelerating the pace of discovery and innovation. Variational autoencoder architecture is another generative model that can be utilized in similar contexts.
- Transformer Models: Â
- Utilize self-attention mechanisms to process data in parallel.
- Highly effective in natural language processing tasks.
- Their application in genomics is emerging, particularly in understanding complex relationships in genomic data, which can lead to more informed research outcomes. Deep learning architectures like inception v3 architecture are also being explored for their potential in genomic applications.
Deep learning architectures such as residual networks and resnet18 architecture are transforming the landscape of genomic research by enabling more accurate predictions and insights from vast amounts of data, ultimately helping organizations achieve greater ROI through enhanced research capabilities and faster time-to-market for new therapies.
2.3. Natural Language Processing for Genomic Literature
Natural Language Processing (NLP) is a field of artificial intelligence that focuses on the interaction between computers and human language. In the context of genomic literature, NLP plays a crucial role in extracting meaningful information from vast amounts of unstructured text data, thereby streamlining research processes.
- Information Extraction: Â
- NLP techniques can identify and extract relevant information from scientific papers, such as gene names, mutations, and disease associations. This helps researchers quickly gather insights from literature without manually reading each paper, saving time and resources.
- Text Mining: Â
- NLP enables the analysis of large volumes of genomic literature to identify trends, relationships, and gaps in research. Text mining tools can summarize findings, making it easier for researchers to stay updated on the latest developments and focus on high-impact areas.
- Sentiment Analysis: Â
- NLP can assess the sentiment of research articles, helping to gauge the scientific community's perception of specific genomic studies or findings. This can inform funding decisions and research directions, ensuring that investments are aligned with promising areas of study.
- Ontology Development: Â
- NLP aids in the creation of ontologies that standardize terminology in genomics, facilitating better data sharing and collaboration. Ontologies help in organizing knowledge and improving the interoperability of genomic databases, which is essential for collaborative research efforts.
By leveraging NLP, researchers can enhance their understanding of genomic literature, leading to more informed decisions and innovative discoveries that drive business growth and improve patient outcomes.
2.4. Visualization and Reporting Systems
Visualization and reporting systems are essential tools in genomics, enabling researchers to interpret complex data and communicate findings effectively. These systems transform raw data into visual formats that are easier to understand and analyze, thereby enhancing stakeholder engagement and decision-making.
- Data Visualization: Â
- Graphical representations of genomic data, such as heatmaps, scatter plots, and genome browsers, help researchers identify patterns and anomalies. Visualization tools can illustrate relationships between genes, mutations, and phenotypes, making it easier to draw conclusions that can inform strategic initiatives.
- Interactive Dashboards: Â
- These systems allow users to explore genomic data dynamically, providing filters and options to customize views. Interactive dashboards can facilitate real-time data analysis and collaboration among researchers, promoting a culture of innovation and agility.
- Reporting Tools: Â
- Automated reporting systems can generate comprehensive reports summarizing genomic findings, methodologies, and conclusions. These reports can be tailored for different audiences, including researchers, clinicians, and policymakers, ensuring that insights are communicated effectively.
- Integration with Other Systems: Â
- Visualization and reporting systems can integrate with databases and analytical tools, streamlining workflows. This integration enhances data accessibility and promotes collaboration across research teams, ultimately driving advancements in genomics research and personalized medicine.
Effective visualization and reporting systems are vital for translating complex genomic data into actionable insights, ultimately helping organizations achieve their business goals efficiently and effectively.
3. Core AI Technologies in Genomic Processing
The integration of artificial intelligence (AI) in genomic processing has revolutionized the field of genomics. Core AI technologies, particularly deep learning, have enabled researchers to analyze vast amounts of genomic data efficiently. This section delves into the significance of deep neural networks and their specific application through convolutional neural networks in genomic processing, particularly in the context of ai in genomic processing.
3.1. Deep Neural Networks
Deep neural networks (DNNs) are a class of machine learning algorithms that mimic the human brain's structure and function. They consist of multiple layers of interconnected nodes (neurons) that process data in a hierarchical manner. DNNs are particularly effective in handling complex datasets, making them ideal for genomic processing.
DNNs can learn intricate patterns in genomic data, which traditional algorithms may overlook. They are capable of performing tasks such as classification, regression, and clustering, which are essential in genomics. Additionally, DNNs can process unstructured data, such as DNA sequences, allowing for more comprehensive analyses.
The application of DNNs in genomics has led to significant advancements, including improved accuracy in predicting genetic disorders, enhanced understanding of gene expression and regulation, and accelerated drug discovery processes by identifying potential drug targets. At Rapid Innovation, we leverage DNNs to help our clients achieve greater ROI by streamlining their genomic research and development processes, ultimately leading to faster and more accurate results.
3.1.1. Convolutional Neural Networks
Convolutional neural networks (CNNs) are a specialized type of deep neural network designed to process structured grid data, such as images. In genomic processing, CNNs have gained traction due to their ability to analyze and interpret complex biological data, including DNA sequences and protein structures, which is a key aspect of ai in genomic processing.
CNNs utilize convolutional layers to automatically extract features from input data, reducing the need for manual feature engineering. They excel in recognizing spatial hierarchies, making them suitable for tasks like identifying motifs in DNA sequences.
Key applications of CNNs in genomic processing include:
- Genomic Sequence Analysis: CNNs can analyze DNA sequences to identify patterns associated with specific traits or diseases. This capability is crucial for personalized medicine and understanding genetic predispositions.
- Protein Structure Prediction: CNNs can predict the three-dimensional structure of proteins based on their amino acid sequences. This is vital for drug design and understanding biological functions.
- Image Analysis in Genomics: CNNs are also used in analyzing genomic images, such as those from microscopy or sequencing technologies. They can help in identifying cellular structures or anomalies in tissue samples.
The effectiveness of CNNs in genomic processing is supported by their ability to handle large datasets and their robustness in feature extraction. As genomic data continues to grow exponentially, the role of CNNs in this field is expected to expand, leading to more breakthroughs in understanding genetic information and its implications for health and disease. Rapid Innovation is committed to harnessing the power of CNNs to provide our clients with innovative solutions that enhance their genomic research capabilities and drive significant returns on investment.
3.1.2. Recurrent Neural Networks
Recurrent Neural Networks (RNNs) are a class of artificial neural networks designed for processing sequential data. Unlike traditional feedforward neural networks, RNNs have connections that loop back on themselves, allowing them to maintain a form of memory. This characteristic makes RNNs particularly effective for tasks involving time series data, natural language processing, and other sequential inputs.
- Key Features of RNNs: Â
- Memory: RNNs can remember previous inputs due to their internal state, which is updated at each time step.
- Sequence Processing: They excel in tasks where the order of data points is crucial, such as speech recognition and language translation.
- Variable Input Length: RNNs can handle input sequences of varying lengths, making them versatile for different applications.
- Types of RNNs: Â
- Vanilla RNNs: The basic form of RNNs, which can struggle with long-term dependencies due to issues like vanishing gradients.
- Long Short-Term Memory (LSTM): A specialized type of RNN designed to remember information for longer periods, effectively addressing the vanishing gradient problem.
- Gated Recurrent Units (GRU): A simpler alternative to LSTMs, GRUs also manage long-term dependencies but with fewer parameters.
RNNs have been widely used in various applications, including text generation, sentiment analysis, and time series forecasting. At Rapid Innovation, we leverage RNNs to develop tailored solutions that enhance user engagement and improve predictive capabilities, ultimately driving greater ROI for our clients. Additionally, RNNs can be applied in advanced analytics, such as predictive analytics and geo spatial analytics, to extract insights from complex datasets.
3.1.3. Transformer Models
Transformer models have revolutionized the field of natural language processing (NLP) and beyond. Introduced in the paper "Attention is All You Need," transformers utilize a mechanism called self-attention, allowing them to weigh the importance of different words in a sentence regardless of their position. This architecture has led to significant improvements in performance across various tasks.
- Key Features of Transformer Models: Â
- Self-Attention Mechanism: This allows the model to focus on relevant parts of the input sequence, enhancing context understanding.
- Parallelization: Unlike RNNs, transformers can process entire sequences simultaneously, leading to faster training times.
- Scalability: Transformers can be scaled up with more layers and parameters, resulting in models like BERT and GPT that achieve state-of-the-art results.
- Applications of Transformer Models: Â
- Language Translation: Transformers have set new benchmarks in translating text between languages.
- Text Summarization: They can condense lengthy articles into concise summaries while retaining essential information.
- Question Answering: Transformers excel in understanding context and providing accurate answers to user queries.
The impact of transformer models extends beyond NLP, influencing fields such as computer vision and reinforcement learning, showcasing their versatility and effectiveness. Rapid Innovation harnesses the power of transformer models to create advanced applications that enhance customer experiences and streamline operations, leading to improved business outcomes.
3.2. Advanced Analytics
Advanced analytics refers to the use of sophisticated techniques and tools to analyze data and extract valuable insights. This approach goes beyond traditional data analysis methods, incorporating machine learning, predictive modeling, and statistical algorithms to uncover patterns and trends. Advanced analytics tools are essential for organizations looking to implement advanced marketing analytics and big data advanced analytics strategies.
- Key Components of Advanced Analytics: Â
- Predictive Analytics: This involves using historical data to forecast future outcomes, helping organizations make informed decisions.
- Prescriptive Analytics: This goes a step further by recommending actions based on predictive insights, optimizing decision-making processes.
- Descriptive Analytics: This provides insights into past performance, helping organizations understand what happened and why.
- Benefits of Advanced Analytics: Â
- Improved Decision-Making: Organizations can make data-driven decisions, reducing reliance on intuition.
- Enhanced Operational Efficiency: By identifying inefficiencies and bottlenecks, businesses can streamline operations.
- Competitive Advantage: Companies leveraging advanced analytics can gain insights that lead to innovative products and services.
- Applications of Advanced Analytics: Â
- Customer Segmentation: Businesses can analyze customer data to identify distinct segments, tailoring marketing strategies accordingly.
- Fraud Detection: Advanced analytics can help detect unusual patterns indicative of fraudulent activities in real-time.
- Supply Chain Optimization: Organizations can forecast demand and optimize inventory levels, reducing costs and improving service levels.
Incorporating advanced analytics into business strategies can lead to significant improvements in performance and profitability, making it an essential component of modern data-driven organizations. At Rapid Innovation, we empower our clients to leverage advanced analytics for strategic decision-making, ultimately enhancing their operational effectiveness and driving higher returns on investment. This includes utilizing data and advanced analytics to inform business strategies and improve outcomes in various sectors, including healthcare and marketing.
3.2.1. Predictive Modeling
Predictive modeling is a statistical technique that uses historical data to forecast future outcomes. It is widely used across various industries, including finance, healthcare, marketing, and more. The primary goal of predictive modeling is to identify patterns and trends that can help organizations make informed decisions.
- Key components of predictive modeling include: Â
- Data collection: Gathering relevant historical data is crucial for building an effective model.
- Feature selection: Identifying the most significant variables that influence the outcome.
- Model selection: Choosing the appropriate algorithm, such as regression analysis, decision trees, or neural networks.
- Validation: Testing the model against a separate dataset to ensure its accuracy and reliability.
- Applications of predictive modeling: Â
- Customer segmentation: Businesses can predict customer behavior and tailor marketing strategies accordingly, leading to improved engagement and higher conversion rates.
- Risk assessment: Financial institutions use predictive models to evaluate the creditworthiness of applicants, reducing default rates and enhancing profitability.
- Healthcare outcomes: Predictive modeling helps in forecasting patient outcomes and optimizing treatment plans, ultimately improving patient care and reducing costs.
At Rapid Innovation, we leverage advanced predictive analytics modeling and predictive modeling techniques to help our clients achieve greater ROI by enabling data-driven decision-making and enhancing operational efficiency. Our expertise in machine learning ensures that organizations can harness the full potential of their data through applied predictive modeling and predictive modeling methods.
3.2.2. Pattern Recognition
Pattern recognition is a branch of machine learning that focuses on identifying regularities and patterns in data. It plays a crucial role in various applications, from image and speech recognition to fraud detection and medical diagnosis.
- Key aspects of pattern recognition include: Â
- Data preprocessing: Cleaning and transforming raw data into a suitable format for analysis.
- Feature extraction: Identifying the most relevant features that represent the data effectively.
- Classification: Assigning labels to data points based on learned patterns, using algorithms like support vector machines or neural networks.
- Common applications of pattern recognition: Â
- Image recognition: Used in facial recognition systems and autonomous vehicles, enhancing security and user experience.
- Speech recognition: Powers virtual assistants like Siri and Google Assistant, streamlining user interactions and improving accessibility.
- Medical diagnosis: Assists in identifying diseases from medical images or patient data, leading to timely interventions and better health outcomes.
At Rapid Innovation, we implement cutting-edge pattern recognition solutions that empower businesses to automate processes, enhance customer experiences, and drive innovation. Our tailored approaches ensure that clients can effectively adapt to changing market demands.
3.2.3. Anomaly Detection
Anomaly detection, also known as outlier detection, is the process of identifying rare items, events, or observations that differ significantly from the majority of the data. It is crucial for various applications, including fraud detection, network security, and fault detection in manufacturing.
- Key elements of anomaly detection include: Â
- Data collection: Gathering a comprehensive dataset that includes both normal and anomalous instances.
- Model training: Using historical data to train models that can distinguish between normal and abnormal behavior.
- Evaluation: Assessing the model's performance using metrics like precision, recall, and F1 score.
- Applications of anomaly detection: Â
- Fraud detection: Financial institutions use anomaly detection to identify unusual transactions that may indicate fraud, protecting assets and maintaining trust.
- Network security: Monitoring network traffic to detect potential security breaches or attacks, safeguarding sensitive information.
- Predictive maintenance: Identifying equipment failures before they occur by detecting anomalies in sensor data, reducing downtime and maintenance costs.
Anomaly detection techniques can be categorized into supervised, unsupervised, and semi-supervised methods. Each approach has its advantages and is chosen based on the specific requirements of the application. The ability to detect anomalies effectively is vital for maintaining security and operational efficiency in various domains. At Rapid Innovation, we provide robust anomaly detection solutions that enhance risk management and operational resilience for our clients.
3.3. Integration with Bioinformatics Tools
The integration of bioinformatics tools integration with various biological and medical research processes is crucial for advancing our understanding of complex biological systems. This integration allows researchers to analyze large datasets efficiently and derive meaningful insights.
- Bioinformatics tools facilitate the analysis of genomic, proteomic, and metabolomic data, enabling researchers to identify patterns and correlations that would be difficult to discern manually.
- These tools often include software for sequence alignment, gene expression analysis, and structural bioinformatics, which are essential for interpreting biological data.
- By integrating bioinformatics tools with laboratory techniques, researchers can streamline workflows, reduce errors, and enhance reproducibility in experiments.
- The use of databases and algorithms in bioinformatics allows for the storage and retrieval of vast amounts of biological data, making it easier to conduct comparative studies and meta-analyses.
- Collaborative platforms that combine bioinformatics with machine learning and artificial intelligence are emerging, providing powerful resources for predictive modeling and hypothesis generation. Rapid Innovation specializes in developing such platforms, ensuring that our clients can leverage cutting-edge technology to enhance their research capabilities, AI agents for patient care.
4. Key Benefits and Advantages
The integration of bioinformatics tools into research and clinical practices offers numerous benefits that enhance the overall effectiveness of scientific inquiry and medical diagnostics.
- Enhanced data management capabilities allow for the organization and analysis of large datasets.
- Improved collaboration among researchers through shared platforms and tools.
- Accelerated discovery of new biomarkers and therapeutic targets, leading to advancements in personalized medicine.
4.1. Improved Accuracy and Precision
One of the most significant advantages of integrating bioinformatics tools is the improved accuracy and precision in data analysis and interpretation.
- Bioinformatics tools utilize sophisticated algorithms that minimize human error, leading to more reliable results.
- High-throughput sequencing technologies generate vast amounts of data, and bioinformatics tools are essential for processing and analyzing this data accurately.
- The ability to perform complex statistical analyses helps in identifying significant biological signals amidst noise, enhancing the reliability of findings.
- Integration with machine learning techniques allows for the development of predictive models that can forecast outcomes with high accuracy. Rapid Innovation's expertise in AI ensures that our clients can harness these advanced techniques effectively.
- Improved accuracy in bioinformatics can lead to better clinical decision-making, as healthcare providers can rely on precise data for diagnosis and treatment planning.
By leveraging bioinformatics tools integration, researchers and clinicians can achieve a higher level of accuracy and precision, ultimately leading to more effective interventions and a deeper understanding of biological processes. Rapid Innovation is committed to helping clients navigate this complex landscape, ensuring they achieve greater ROI through innovative solutions tailored to their specific needs.
4.2. Accelerated Processing Speed
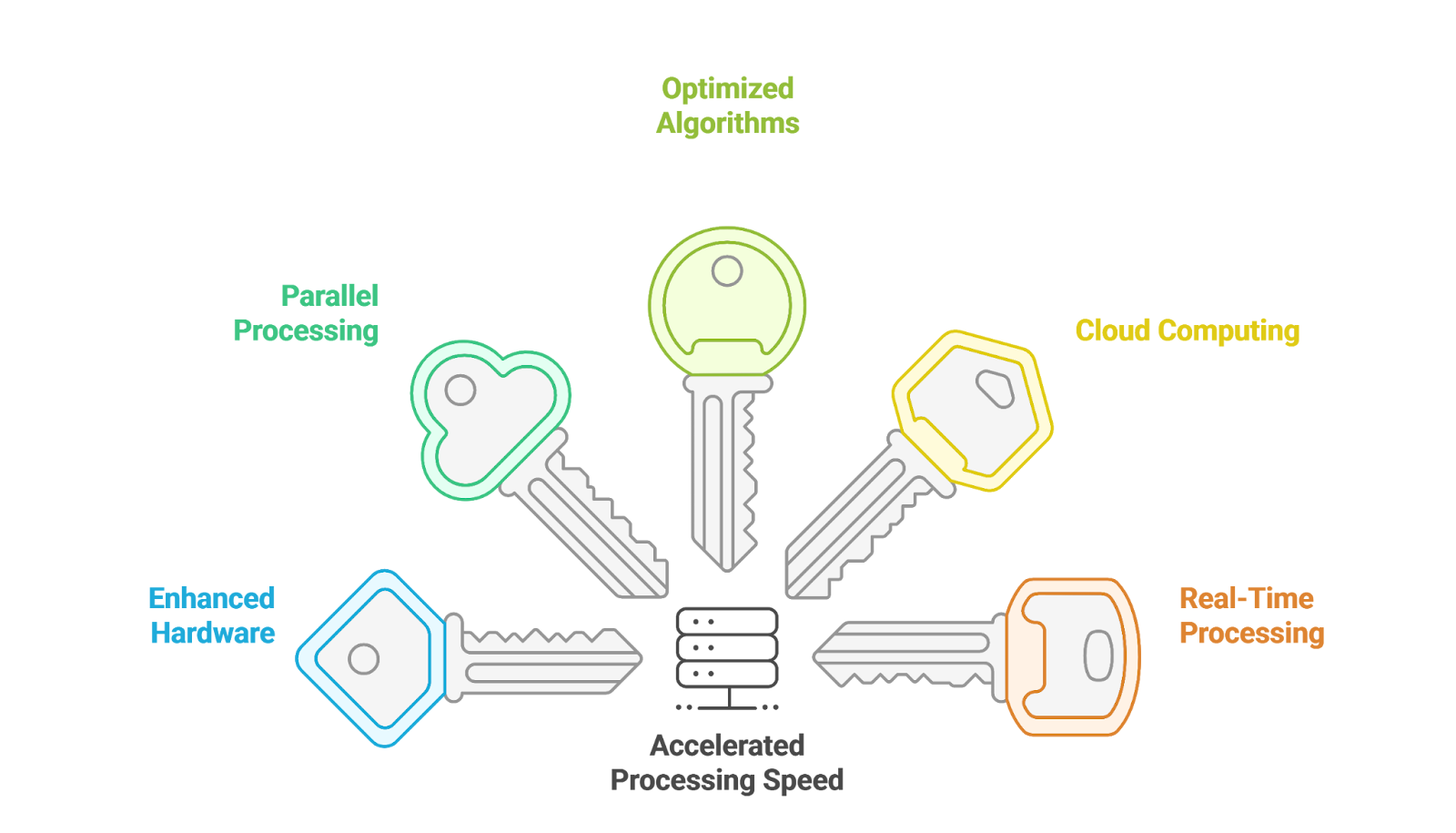
‍
Accelerated processing speed is a critical factor in enhancing the efficiency of various systems, particularly in computing and data management. This speed is achieved through advancements in technology, including hardware improvements and optimized software algorithms. At Rapid Innovation, we leverage these advancements to help our clients achieve their business goals effectively.
- Enhanced hardware capabilities, such as multi-core processors and solid-state drives (SSDs), significantly boost processing speed, allowing businesses to handle larger datasets and complex computations with ease. Â
- Parallel processing allows multiple tasks to be executed simultaneously, reducing the time required for data processing. This is particularly beneficial for industries that rely on real-time analytics, such as finance and e-commerce. Â
- Optimized algorithms can streamline operations, leading to faster data retrieval and processing times. Our team specializes in developing custom algorithms tailored to specific business needs, ensuring maximum efficiency. Â
- Technologies like cloud computing enable on-demand resources, allowing for rapid scaling of processing power as needed. This flexibility is essential for businesses experiencing fluctuating workloads. Â
- Real-time data processing is becoming increasingly important in industries like finance and healthcare, where timely information is crucial. Rapid Innovation implements solutions that facilitate real-time insights, empowering clients to make informed decisions swiftly.
4.3. Cost Reduction
Cost reduction is a primary goal for businesses looking to improve their bottom line. By implementing efficient systems and technologies, organizations can significantly lower their operational costs. Rapid Innovation focuses on delivering solutions that drive cost efficiency for our clients.
- Automation of repetitive tasks reduces the need for manual labor, leading to lower labor costs. Our AI-driven automation tools help businesses streamline operations and minimize human error. Â
- Cloud services often provide a more cost-effective solution compared to traditional on-premises infrastructure, as they eliminate the need for extensive hardware investments. We guide clients in selecting the right cloud solutions that align with their budget and operational needs. Â
- Energy-efficient technologies can lead to substantial savings on utility bills, especially in data centers and large-scale operations. Our consulting services include recommendations for energy-efficient practices, such as energy savings solutions llc and energy efficiency solutions llc, that can enhance sustainability and reduce costs. Â
- Outsourcing non-core functions can help businesses focus on their primary objectives while reducing overhead costs. We assist clients in identifying functions that can be outsourced effectively, allowing them to concentrate on growth. Â
- Streamlined supply chain management can minimize waste and improve resource allocation, further driving down costs. Our blockchain solutions enhance transparency and efficiency in supply chains, leading to significant cost savings.
4.4. Scalability and Flexibility
Scalability and flexibility are essential attributes for modern businesses, allowing them to adapt to changing market demands and growth opportunities. Rapid Innovation provides scalable and flexible solutions that empower organizations to thrive in dynamic environments.
- Scalable solutions enable organizations to increase or decrease resources based on current needs without significant downtime or investment. Our cloud-based solutions are designed to grow with your business. Â
- Cloud computing offers unparalleled flexibility, allowing businesses to access resources and services from anywhere, at any time. We help clients implement cloud strategies that enhance operational agility. Â
- Modular systems can be easily expanded or modified, ensuring that businesses can adapt to new technologies or market conditions. Our development approach emphasizes modularity, allowing for seamless upgrades and integrations. Â
- The ability to quickly pivot strategies in response to market changes is crucial for maintaining a competitive edge. We provide strategic consulting that helps clients navigate market shifts effectively, including insights from corporate energy efficiency and business efficiency solutions. Â
- Flexible work environments, supported by remote access technologies, can enhance employee satisfaction and productivity, leading to better overall performance. Our solutions facilitate remote work capabilities, ensuring teams remain connected and productive regardless of location. Â
At Rapid Innovation, we are committed to helping our clients achieve greater ROI through our expertise in AI and blockchain technologies. Our tailored solutions are designed to enhance efficiency, reduce costs, and provide the scalability needed for future growth, including energy saving solutions for hotels and commercial energy efficiency solutions.
4.5. Enhanced Pattern Discovery
Enhanced pattern discovery refers to the advanced techniques and methodologies used to identify trends, correlations, and anomalies within large datasets. This process is crucial for businesses and researchers who seek to derive actionable insights from complex information.
- Utilizes machine learning algorithms to analyze data more efficiently.
- Employs data mining techniques, including pattern discovery in data mining, to uncover hidden patterns that traditional methods might miss.
- Involves the use of visualization tools to present data in an easily interpretable format.
- Supports predictive analytics, allowing organizations to forecast future trends based on historical data.
- Enhances decision-making by providing a deeper understanding of customer behavior and market dynamics.
The significance of enhanced pattern discovery lies in its ability to transform raw data into valuable insights. For instance, businesses can identify customer preferences, optimize marketing strategies, and improve product offerings. This capability is increasingly important in a data-driven world where organizations must adapt quickly to changing market conditions. At Rapid Innovation, we leverage our expertise in AI to implement enhanced pattern discovery solutions that drive greater ROI for our clients by enabling them to make informed decisions based on data-driven insights.
4.6. Automated Quality Control
Automated quality control (AQC) is a systematic approach that leverages technology to monitor and ensure the quality of products and services throughout the production process. This method reduces human error and increases efficiency, leading to higher standards of quality.
- Integrates sensors and IoT devices to collect real-time data on production processes.
- Utilizes machine learning algorithms to analyze data and detect anomalies or defects.
- Implements automated testing procedures to ensure products meet specified standards.
- Reduces the need for manual inspections, saving time and resources.
- Enhances traceability by maintaining detailed records of quality checks and outcomes.
The benefits of automated quality control are substantial. Companies can achieve consistent quality, reduce waste, and improve customer satisfaction. By automating quality checks, organizations can also respond more swiftly to issues, minimizing the impact on production and delivery timelines. Rapid Innovation's AQC solutions empower businesses to maintain high-quality standards while optimizing operational efficiency, ultimately leading to increased profitability.
5. Primary Use Cases
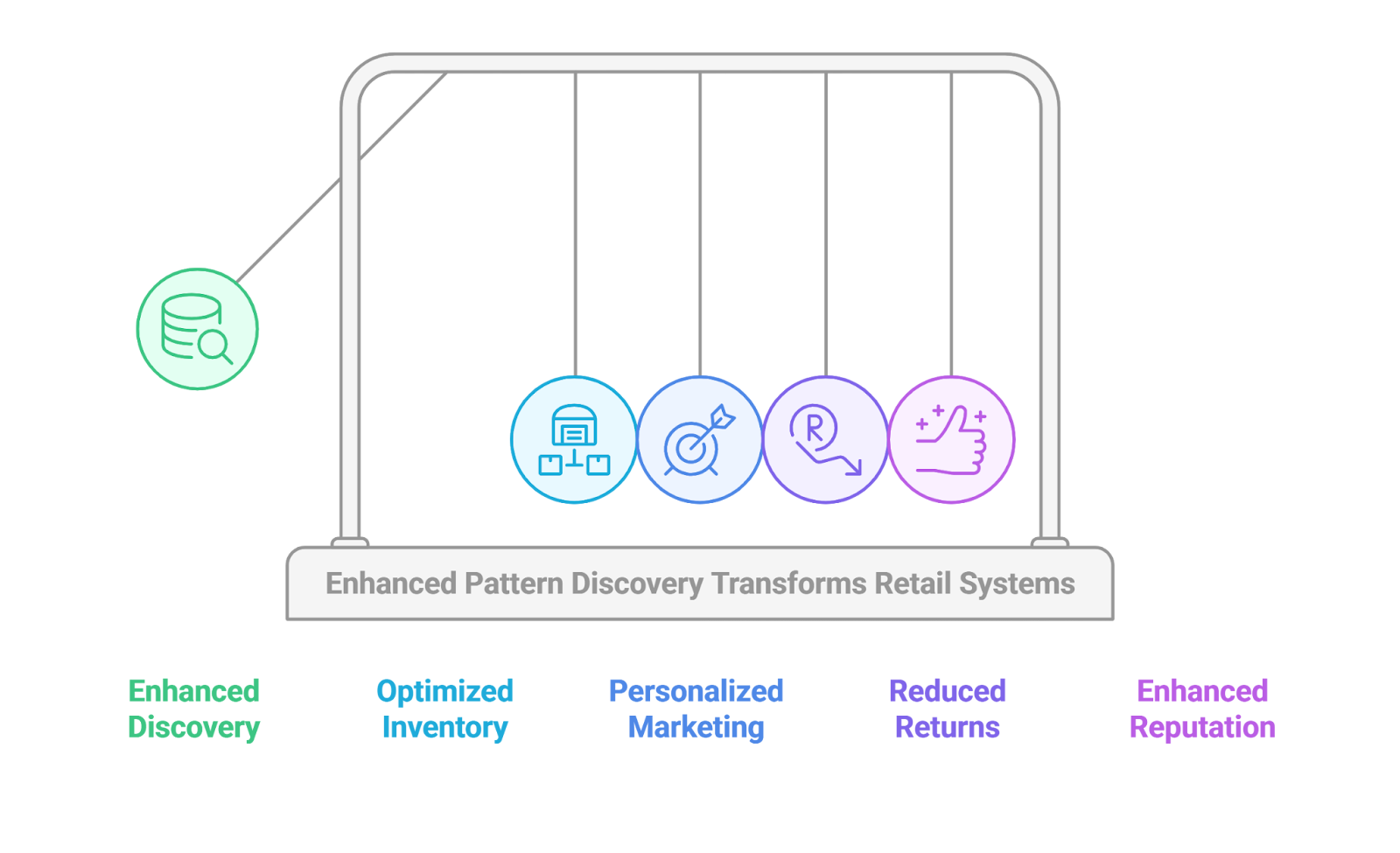
‍
The primary use cases for enhanced pattern discovery and automated quality control span various industries, showcasing their versatility and effectiveness in improving operations and outcomes.
- Retail and E-commerce: Enhanced pattern discovery helps retailers analyze customer purchasing behavior, optimize inventory management, and personalize marketing efforts. Automated quality control ensures that products meet quality standards before reaching consumers, reducing returns and enhancing brand reputation.
- Manufacturing: In manufacturing, enhanced pattern discovery can identify inefficiencies in production processes, leading to improved operational efficiency. Automated quality control systems monitor production lines in real-time, detecting defects early and ensuring that only high-quality products are shipped.
- Healthcare: Enhanced pattern discovery is used to analyze patient data, leading to better diagnosis and treatment plans. Automated quality control in healthcare ensures that medical devices and pharmaceuticals meet stringent regulatory standards, safeguarding patient safety.
- Finance: Financial institutions utilize enhanced pattern discovery techniques to detect fraudulent activities and assess risk. Automated quality control processes help maintain compliance with regulations and ensure the accuracy of financial reporting.
- Telecommunications: Enhanced pattern discovery aids in analyzing customer usage patterns, enabling service providers to tailor offerings and improve customer satisfaction. Automated quality control ensures that network services meet performance standards, reducing downtime and enhancing user experience.
These use cases illustrate the transformative potential of enhanced pattern discovery and automated quality control across various sectors. By leveraging these technologies, organizations can drive innovation, improve efficiency, and deliver superior products and services. At Rapid Innovation, we are committed to helping our clients harness the power of AI and blockchain to achieve their business goals effectively and efficiently.
5.1. Sequence Analysis
Sequence analysis is a critical component of genomics and molecular biology, focusing on the examination of nucleotide sequences in DNA and RNA. This process allows researchers to understand genetic information, identify mutations, and explore gene functions. Sequence analysis can be performed using various techniques and tools, enabling scientists to derive meaningful insights from biological data.
- Helps in identifying genetic variations.
- Aids in understanding evolutionary relationships.
- Facilitates the discovery of new genes and regulatory elements.
5.1.1. DNA Sequencing
DNA sequencing is the process of determining the precise order of nucleotides within a DNA molecule. This technique has revolutionized genetics, enabling researchers to decode the genetic blueprint of organisms. There are several methods of DNA sequencing, including Sanger sequencing and next-generation sequencing (NGS).
- Sanger Sequencing: Developed in the 1970s, it uses chain-terminating inhibitors to produce fragments of varying lengths. It is ideal for sequencing small DNA fragments and is often used for targeted sequencing.
- Next-Generation Sequencing (NGS): This method allows for massive parallel sequencing, enabling the analysis of millions of DNA fragments simultaneously. It provides high-throughput capabilities, making it suitable for whole-genome sequencing and large-scale studies.
- Applications of DNA Sequencing: Â
- Identifying genetic disorders and mutations.
- Understanding cancer genomics.
- Exploring microbial diversity and evolution.
The advancements in DNA sequencing technologies have significantly reduced costs and increased accessibility, leading to a surge in genomic research. According to a report by the National Human Genome Research Institute, the cost of sequencing a human genome has dropped from approximately $100 million in 2001 to around $1,000 in recent years.
5.1.2. RNA Sequencing
RNA sequencing (RNA-seq) is a powerful technique used to analyze the transcriptome, which is the complete set of RNA transcripts produced by the genome at any given time. RNA-seq provides insights into gene expression levels, alternative splicing events, and non-coding RNA functions.
- Key Features of RNA Sequencing: Â
- High sensitivity and specificity in detecting low-abundance transcripts.
- Ability to capture a wide range of RNA types, including mRNA, lncRNA, and miRNA.
- Steps in RNA Sequencing: Â
- RNA extraction: Isolating RNA from cells or tissues.
- Library preparation: Converting RNA into complementary DNA (cDNA) and preparing it for sequencing.
- Sequencing: Using NGS platforms to read the cDNA fragments.
- Data analysis: Employing bioinformatics tools to interpret the sequencing data and quantify gene expression.
- Applications of RNA Sequencing: Â
- Identifying differentially expressed genes in various conditions.
- Understanding the regulatory mechanisms of gene expression.
- Investigating the role of non-coding RNAs in cellular processes.
RNA-seq has become a standard method in genomics, providing a comprehensive view of gene expression and regulation. A study published in Nature Biotechnology highlighted that RNA-seq can detect over 90% of expressed genes in a given sample, making it a reliable tool for transcriptomic analysis.
In summary, both DNA and RNA sequencing are integral to sequence analysis, offering valuable insights into genetic and transcriptomic landscapes. Techniques such as T7 RNA polymerase promoter sequence and T7 polymerase promoter sequence are essential for understanding transcription processes. Additionally, the conversion of DNA to RNA sequence and the reverse process of RNA to DNA sequence are fundamental in molecular biology. These techniques continue to evolve, driving advancements in personalized medicine, evolutionary biology, and biotechnology.
At Rapid Innovation, we leverage our expertise in AI and blockchain to enhance the efficiency and accuracy of sequence analysis. By integrating AI algorithms, we can automate data analysis processes, leading to faster and more reliable results. Additionally, utilizing blockchain technology ensures the integrity and security of genomic data, providing clients with a robust framework for managing sensitive information. Our solutions not only streamline research workflows but also maximize ROI by reducing time-to-insight and enhancing data trustworthiness.
5.1.3. Protein Structure Prediction
Protein structure prediction is a crucial aspect of bioinformatics and molecular biology. Understanding the three-dimensional structure of proteins is essential for elucidating their function and role in biological processes. The prediction of protein structures can be achieved through various computational methods, which can be broadly categorized into:
- Homology Modeling: This method relies on the known structures of homologous proteins. By aligning the target protein sequence with a template protein of known structure, researchers can predict the 3D structure of the target. This approach is effective when there is a high degree of sequence similarity.
- Ab Initio Methods: These methods predict protein structures from scratch, without relying on templates. They use physical principles and statistical potentials to explore possible conformations. While ab initio methods can be computationally intensive, they are essential for predicting the structures of novel proteins.
- Threading: This technique involves fitting the target sequence into a known structure by optimizing the alignment. It is particularly useful for proteins with low sequence similarity to known structures.
- Machine Learning Approaches: Recent advancements in artificial intelligence have led to the development of machine learning models that can predict protein structures with remarkable accuracy. For instance, AlphaFold, developed by DeepMind, has shown unprecedented success in predicting protein structures based on amino acid sequences. The AlphaFold database provides valuable resources for researchers, while tools like AlphaFold 2 and AlphaFold multimer enhance the capabilities of protein structure prediction.
The implications of accurate protein structure prediction are vast, impacting drug design, understanding disease mechanisms, and developing therapeutic interventions. As research continues to evolve, the integration of experimental data with computational predictions will enhance our understanding of protein dynamics and interactions. At Rapid Innovation, we leverage AI-driven solutions, including AI protein folding techniques, to optimize these predictions, enabling our clients to accelerate their research and development processes, ultimately leading to greater ROI through faster time-to-market for new therapeutics. Additionally, the role of generative AI in accelerating drug discovery and personalized medicine is becoming increasingly significant, further enhancing the capabilities of protein structure prediction.
5.2. Disease Research
Disease research is a multidisciplinary field that aims to understand the underlying mechanisms of diseases, identify potential therapeutic targets, and develop effective treatments. This area of research encompasses various approaches, including genomics, proteomics, and systems biology. Key aspects of disease research include:
- Genomic Studies: Analyzing the genetic basis of diseases helps identify mutations and variations that contribute to disease susceptibility and progression.
- Biomarker Discovery: Identifying biomarkers can aid in early diagnosis, prognosis, and monitoring of disease progression.
- Therapeutic Development: Understanding disease mechanisms allows researchers to develop targeted therapies that can improve patient outcomes.
- Clinical Trials: Testing new treatments in clinical settings is essential for validating the efficacy and safety of therapeutic interventions.
Advancements in technology, such as next-generation sequencing and high-throughput screening, have accelerated disease research, enabling researchers to uncover complex interactions within biological systems. Rapid Innovation employs cutting-edge AI and blockchain technologies to enhance data integrity and streamline the research process, ensuring that our clients can achieve their business goals efficiently.
5.2.1. Cancer Genomics
Cancer genomics is a specialized field within disease research that focuses on the genetic alterations associated with cancer. By studying the genomic landscape of tumors, researchers can gain insights into the mechanisms driving cancer development and progression. Key components of cancer genomics include:
- Mutational Analysis: Identifying mutations in oncogenes and tumor suppressor genes helps understand the genetic basis of cancer. For example, mutations in the TP53 gene are commonly associated with various cancers.
- Copy Number Variations: Changes in the number of copies of specific genes can contribute to cancer. Analyzing these variations can reveal potential therapeutic targets.
- Gene Expression Profiling: Understanding how gene expression changes in cancer cells compared to normal cells can provide insights into tumor biology and potential treatment strategies.
- Personalized Medicine: Cancer genomics enables the development of personalized treatment plans based on the unique genetic profile of an individual's tumor. This approach can lead to more effective therapies with fewer side effects.
- Clinical Applications: Genomic data can inform clinical decisions, such as selecting targeted therapies or determining prognosis. For instance, the presence of specific mutations can guide the use of targeted therapies like EGFR inhibitors in lung cancer.
The integration of cancer genomics with other omics technologies, such as proteomics and metabolomics, is paving the way for a more comprehensive understanding of cancer biology and the development of innovative therapeutic strategies. As research progresses, the potential for improving patient outcomes through genomics-based approaches continues to expand. Rapid Innovation is committed to supporting this evolution by providing advanced AI solutions that enhance data analysis and interpretation, ultimately driving better clinical outcomes and maximizing ROI for our clients.
5.2.2. Rare Disease Analysis
Rare diseases, often defined as conditions affecting fewer than 200,000 individuals in the United States, present unique challenges in diagnosis and treatment. The analysis of rare diseases is crucial for understanding their etiology, improving patient outcomes, and developing targeted therapies.
- Understanding the genetic basis: Many rare diseases have a genetic component, making genetic testing and analysis essential. Identifying mutations can lead to better diagnostic tools and potential treatments. Rapid Innovation leverages AI algorithms to analyze genetic data, enabling more accurate identification of mutations and facilitating the development of personalized treatment plans.
- Data collection and sharing: Collaboration among researchers, healthcare providers, and patients is vital. Platforms like the National Organization for Rare Disorders (NORD) facilitate data sharing, which can accelerate research efforts. Rapid Innovation can implement blockchain solutions to ensure secure and transparent data sharing, enhancing collaboration while maintaining patient privacy.
- Patient registries: Establishing registries helps in tracking disease prevalence, patient demographics, and treatment responses. This data is invaluable for clinical trials and understanding disease progression. Our expertise in AI can optimize the analysis of registry data, providing insights that drive better clinical decision-making.
- Innovative research methods: Utilizing advanced technologies such as CRISPR and next-generation sequencing can enhance our understanding of rare diseases and lead to novel therapeutic approaches. Rapid Innovation supports clients in integrating AI-driven analytics to streamline research processes and improve outcomes.
- Regulatory support: Agencies like the FDA provide incentives for developing treatments for rare diseases, including orphan drug designation, which can expedite the approval process. Our consulting services can guide clients through regulatory pathways, ensuring compliance and maximizing the potential for successful drug approval.
5.2.3. Pathogen Detection
Pathogen detection is a critical aspect of public health, especially in the context of infectious diseases. Rapid and accurate identification of pathogens can significantly impact treatment outcomes and control measures.
- Diagnostic technologies: Advances in molecular diagnostics, such as PCR (Polymerase Chain Reaction) and next-generation sequencing, allow for the rapid detection of pathogens at a genetic level. Rapid Innovation employs AI to enhance diagnostic accuracy and speed, ensuring timely interventions.
- Surveillance systems: Implementing robust surveillance systems helps in monitoring outbreaks and understanding pathogen spread. This includes both human and environmental monitoring. Our blockchain solutions can provide secure and immutable records of surveillance data, facilitating better public health responses.
- Point-of-care testing: Developing portable diagnostic tools enables healthcare providers to detect pathogens quickly, even in remote areas. This is crucial for timely intervention and treatment. Rapid Innovation can assist in the development of AI-powered point-of-care devices that deliver rapid results.
- Bioinformatics: Analyzing genomic data from pathogens can provide insights into their evolution, resistance patterns, and potential vulnerabilities, aiding in the development of targeted therapies. Our AI capabilities can process vast amounts of genomic data, identifying trends and patterns that inform treatment strategies.
- Global collaboration: International partnerships and data sharing among countries enhance the ability to respond to emerging infectious diseases and improve global health security. Rapid Innovation can facilitate these collaborations through secure blockchain networks that promote data integrity and trust.
5.3. Drug Discovery and Development
The drug discovery and development process is a complex and lengthy journey that transforms scientific research into effective therapies. This process involves several stages, each critical for ensuring the safety and efficacy of new medications.
- Target identification: The first step involves identifying biological targets associated with diseases. This can include proteins, genes, or pathways that play a role in disease progression. Rapid Innovation utilizes AI to analyze biological data, accelerating the identification of promising drug targets.
- High-throughput screening: Utilizing automated systems to test thousands of compounds against the identified targets allows researchers to identify potential drug candidates quickly. Our expertise in AI can optimize screening processes, increasing efficiency and reducing costs.
- Preclinical studies: Before human trials, drugs undergo rigorous testing in laboratory and animal models to assess their safety, efficacy, and pharmacokinetics. Rapid Innovation can support clients in designing and analyzing preclinical studies using advanced AI models.
- Clinical trials: This phase is divided into three main stages (Phase I, II, and III) to evaluate the drug's safety and effectiveness in humans. Each phase has specific objectives and participant criteria. Our consulting services can help streamline trial design and patient recruitment, enhancing the likelihood of success.
- Regulatory approval: After successful clinical trials, drug developers submit their findings to regulatory agencies like the FDA for approval. This process ensures that only safe and effective drugs reach the market. Rapid Innovation provides guidance on regulatory strategies, helping clients navigate complex approval processes.
- Post-marketing surveillance: Once a drug is approved, ongoing monitoring is essential to identify any long-term effects or rare side effects that may not have been evident during clinical trials. Our AI solutions can assist in analyzing post-marketing data, ensuring ongoing safety and efficacy.
The drug discovery process is not only time-consuming but also costly, with estimates suggesting that it can take over a decade and billions of dollars to bring a new drug to market. Rapid Innovation is committed to leveraging AI and blockchain technologies to streamline this process, ultimately enhancing ROI for our clients.
5.3.1. Target Identification
Target identification is a crucial step in drug discovery and development. It involves pinpointing specific biological molecules, such as proteins or genes, that are implicated in a disease process. The goal is to find targets that can be modulated by drugs to achieve a therapeutic effect. Identifying targets requires a deep understanding of the underlying biology of diseases, including studying pathways, cellular interactions, and genetic factors. Modern techniques allow researchers to screen thousands of compounds against potential targets quickly, accelerating the identification of promising drug candidates. Additionally, computational methods are increasingly used to analyze biological data, helping to predict which targets are most likely to yield effective treatments. Once potential targets are identified, they must be validated through experimental studies to confirm their role in the disease and their suitability for drug development.
- Understanding disease mechanisms: Identifying targets requires a deep understanding of the underlying biology of diseases. This includes studying pathways, cellular interactions, and genetic factors.
- High-throughput screening: Modern techniques allow researchers to screen thousands of compounds against potential targets quickly. This accelerates the identification of promising drug candidates, including small molecule drug discovery and antibody drug discovery.
- Bioinformatics tools: Computational methods are increasingly used to analyze biological data, helping to predict which targets are most likely to yield effective treatments. AI in drug discovery and development plays a significant role in this process.
- Validation: Once potential targets are identified, they must be validated through experimental studies to confirm their role in the disease and their suitability for drug development.
At Rapid Innovation, we leverage advanced AI algorithms and blockchain technology to enhance the target identification process. Our AI-driven platforms can analyze vast datasets to uncover hidden patterns and relationships, leading to more accurate target identification. Furthermore, utilizing blockchain ensures the integrity and traceability of data throughout the research process, fostering collaboration and trust among stakeholders. For more information on how we can assist you, learn more about generative AI in drug discovery.
5.3.2. Drug Response Prediction
Drug response prediction is the process of forecasting how a patient will respond to a specific medication. This is essential for optimizing treatment plans and minimizing adverse effects. The field of pharmacogenomics studies how genes affect a person's response to drugs, as variations in genetic makeup can influence drug metabolism, efficacy, and toxicity. Advanced algorithms in machine learning analyze large datasets to identify patterns that predict drug responses, incorporating genetic, clinical, and demographic data. Identifying biomarkers can help predict which patients are likely to benefit from a particular treatment, leading to more targeted therapies and improved outcomes. Furthermore, data from clinical trials can be used to refine predictions about drug responses, helping to tailor treatments to individual patients.
- Pharmacogenomics: This field studies how genes affect a person's response to drugs. Variations in genetic makeup can influence drug metabolism, efficacy, and toxicity.
- Machine learning models: Advanced algorithms analyze large datasets to identify patterns that predict drug responses. These models can incorporate genetic, clinical, and demographic data, enhancing drug discovery and development.
- Biomarkers: Identifying biomarkers can help predict which patients are likely to benefit from a particular treatment. This can lead to more targeted therapies and improved outcomes.
- Clinical trials: Data from clinical trials can be used to refine predictions about drug responses, helping to tailor treatments to individual patients.
Rapid Innovation employs cutting-edge machine learning techniques to enhance drug response prediction. By integrating diverse data sources, we can create robust predictive models that help healthcare providers make informed decisions, ultimately improving patient outcomes and maximizing ROI in drug development.
5.3.3. Personalized Medicine
Personalized medicine, also known as precision medicine, is an innovative approach to healthcare that tailors treatment to the individual characteristics of each patient. This strategy enhances the effectiveness of therapies and reduces the risk of adverse effects. By analyzing a patient's genetic makeup, healthcare providers can identify the most effective treatments based on their unique genetic variations. Personalized medicine often involves the use of targeted therapies that specifically address the molecular changes in a patient's disease, leading to better outcomes. Involving patients in their treatment plans fosters better adherence and satisfaction, empowering them to make informed decisions about their healthcare. Although personalized medicine may require upfront investment in genetic testing and analysis, it can lead to long-term savings by reducing trial-and-error prescribing and minimizing adverse drug reactions.
- Genetic profiling: By analyzing a patient's genetic makeup, healthcare providers can identify the most effective treatments based on their unique genetic variations.
- Targeted therapies: Personalized medicine often involves the use of targeted therapies that specifically address the molecular changes in a patient's disease, leading to better outcomes. This includes advancements in antibody drug discovery and biotech drug development.
- Patient engagement: Involving patients in their treatment plans fosters better adherence and satisfaction. Personalized approaches can empower patients to make informed decisions about their healthcare.
- Cost-effectiveness: Although personalized medicine may require upfront investment in genetic testing and analysis, it can lead to long-term savings by reducing trial-and-error prescribing and minimizing adverse drug reactions.
At Rapid Innovation, we are committed to advancing personalized medicine through our AI and blockchain solutions. By harnessing the power of data analytics and secure data sharing, we enable healthcare providers to deliver tailored treatments that enhance patient care and drive efficiency in the healthcare system.
In conclusion, target identification, drug response prediction, and personalized medicine are interconnected components of modern drug development and healthcare. By leveraging advancements in technology and biology, including pharmaceutical drug discovery and drug design and development, these approaches aim to improve patient outcomes and revolutionize the way diseases are treated. Rapid Innovation stands at the forefront of this transformation, providing innovative solutions that help clients achieve their business goals efficiently and effectively.
5.4. Population Genetics
Population genetics is a branch of genetics that studies the distribution and change in frequency of alleles within populations. It combines principles from genetics, evolutionary biology, and ecology to understand how genetic variation is influenced by factors such as natural selection, genetic drift, mutation, and gene flow. This field is crucial for understanding the genetic structure of populations and how they evolve over time. It focuses on allele frequency and genetic diversity, examines the impact of evolutionary processes on populations, and utilizes mathematical models to predict genetic variation, including mathematical population genetics.
5.4.1. Ancestry Analysis
Ancestry analysis is a method used to trace lineage and understand the genetic heritage of individuals. This analysis can reveal information about an individual's ancestral origins, migration patterns, and genetic relationships with others. It utilizes DNA testing to provide insights into ancestry, can identify ethnic backgrounds and geographical origins, and helps in understanding familial connections and historical migrations, including ancient population genetics.
Ancestry analysis often employs autosomal DNA, mitochondrial DNA, and Y-chromosome DNA to provide a comprehensive view of an individual's ancestry. Autosomal DNA tests analyze chromosomes inherited from both parents, while mitochondrial DNA is passed down from mothers to their children, and Y-chromosome DNA is inherited by males from their fathers.
- Autosomal DNA: Provides a broad view of ancestry from both sides of the family Â
- Mitochondrial DNA: Traces maternal lineage Â
- Y-chromosome DNA: Traces paternal lineage Â
The results of ancestry analysis can be used for various purposes, including genealogical research, understanding population history, and even medical insights related to inherited conditions. Companies like AncestryDNA and 23andMe offer services that allow individuals to explore their genetic backgrounds and connect with relatives, contributing to human population genetics.
5.4.2. Genetic Variation Studies
Genetic variation studies focus on the differences in DNA sequences among individuals within a population. These variations can be single nucleotide polymorphisms (SNPs), insertions, deletions, or larger structural changes in the genome. Understanding genetic variation is essential for several reasons: it helps identify the genetic basis of diseases, informs conservation efforts for endangered species, and aids in understanding evolutionary processes, including conservation and the genetics of populations.
Genetic variation is crucial for the adaptability and survival of populations. High levels of genetic diversity can enhance a population's ability to adapt to changing environments, resist diseases, and recover from population declines. Conversely, low genetic diversity can lead to inbreeding and increased vulnerability to extinction.
- SNPs: The most common type of genetic variation, influencing traits and disease susceptibility Â
- Structural variations: Larger changes in the genome that can affect gene function and regulation Â
Researchers often use various techniques to study genetic variation, including genome-wide association studies (GWAS), which link specific genetic variants to traits or diseases. These studies have provided insights into complex conditions such as diabetes, heart disease, and various cancers, and are part of the broader field of population genetics evolution.
- GWAS: Identifies associations between genetic variants and traits Â
- Next-generation sequencing: Allows for comprehensive analysis of genetic variation Â
In summary, population genetics, through ancestry analysis and genetic variation studies, plays a vital role in understanding the genetic makeup of populations and their evolutionary trajectories. This knowledge has significant implications for health, conservation, and our understanding of human history, including the contributions of figures like David Reich in genetics.
At Rapid Innovation, we leverage advanced AI algorithms and blockchain technology to enhance the accuracy and efficiency of genetic research. By utilizing AI-driven data analysis, we can help clients in the healthcare and biotechnology sectors achieve greater ROI through improved genetic insights and personalized medicine solutions. Our blockchain solutions ensure secure and transparent data management, fostering trust and collaboration among researchers and stakeholders in the field of population genetics, including molecular population genetics and conservation and the genomics of populations.
5.4.3. Evolution Studies
Evolution studies focus on understanding the changes and adaptations that occur within species over time. This field of study is crucial for grasping the complexities of biological diversity and the mechanisms driving evolution. Evolutionary biology examines how species evolve through natural selection, genetic drift, and gene flow. Researchers utilize fossil records, comparative anatomy, and molecular biology to trace evolutionary lineages, including the study of biological evolution and early human evolution.
Key concepts include: - Speciation: the process by which new species arise. - Adaptation: traits that enhance survival and reproduction in specific environments. - Phylogenetics: the study of evolutionary relationships among species, which includes evolutionary relationships among early human ancestors.
Evolution studies have practical applications in fields such as medicine, agriculture, and conservation. Understanding evolutionary processes can help in predicting how species might respond to environmental changes, including climate change. This is particularly relevant in the context of molecular phylogeny and evolution, as well as biogeography and evolution, which explore how geographical factors influence evolutionary processes.
6. Implementation Strategies
Implementation strategies are essential for translating theoretical concepts into practical applications. These strategies ensure that projects, policies, or programs are executed effectively and efficiently.
- Clear objectives: Define specific, measurable goals to guide the implementation process.
- Stakeholder engagement: Involve all relevant parties, including community members, experts, and policymakers, to foster collaboration and support.
- Resource allocation: Identify and allocate necessary resources, including funding, personnel, and technology.
- Training and capacity building: Equip team members with the skills and knowledge required for successful implementation.
- Monitoring and evaluation: Establish metrics to assess progress and make adjustments as needed.
6.1. Infrastructure Requirements
Infrastructure requirements refer to the physical and organizational structures needed to support the implementation of strategies effectively. Adequate infrastructure is vital for ensuring that projects can be executed smoothly.
- Physical infrastructure: Â
- Facilities: Buildings and spaces where activities will take place.
- Transportation: Roads, bridges, and public transit systems to facilitate movement of people and goods.
- Utilities: Access to essential services such as water, electricity, and internet connectivity.
- Technological infrastructure: Â
- Software and hardware: Tools and systems necessary for data management, communication, and project execution.
- Cybersecurity: Measures to protect sensitive information and ensure data integrity.
- Human infrastructure: Â
- Skilled workforce: Trained personnel who can operate and maintain the infrastructure.
- Leadership: Strong management to guide the implementation process and address challenges.
- Financial infrastructure: Â
- Funding sources: Identifying grants, investments, or partnerships to support the initiative, including those from the society for the study of evolution.
- Budgeting: Developing a financial plan to allocate resources effectively.
By addressing these infrastructure requirements, organizations can enhance their capacity to implement strategies successfully and achieve desired outcomes.
At Rapid Innovation, we leverage our expertise in AI and Blockchain to optimize these implementation strategies. For instance, our AI-driven analytics can help define clear objectives by analyzing historical data and predicting future trends, ensuring that your goals are not only specific but also achievable. Additionally, our Blockchain solutions can enhance stakeholder engagement by providing transparent and secure communication channels, fostering trust and collaboration among all parties involved. By integrating advanced technologies into your infrastructure, we can help you achieve greater ROI and drive your business forward efficiently and effectively.
6.1.1. Computing Resources
Computing resources refer to the hardware and software components that are essential for processing data and executing applications. These resources are critical for businesses and organizations that rely on technology to operate efficiently. At Rapid Innovation, we leverage advanced computing resources to enhance our AI and Blockchain solutions, ensuring that our clients achieve their business goals effectively.
- Types of Computing Resources: Â
- Central Processing Units (CPUs): The brain of the computer, responsible for executing instructions. Our AI algorithms benefit from high-performance CPUs to process large datasets quickly.
- Graphics Processing Units (GPUs): Specialized processors designed to handle complex graphics and parallel processing tasks. We utilize GPUs for training machine learning models, significantly reducing the time to achieve accurate results.
- Memory (RAM): Temporary storage that allows for quick access to data and applications. Adequate RAM is crucial for running multiple applications simultaneously, especially in data-intensive environments.
- Virtual Machines (VMs): Software emulations of physical computers that allow multiple operating systems to run on a single physical machine. This flexibility enables us to test and deploy our solutions in various environments efficiently.
- Cloud Computing: Â
- Offers scalable computing resources on-demand. Rapid Innovation partners with leading cloud providers to ensure our clients can scale their operations without upfront capital investment.
- Providers like Amazon Web Services (AWS) and Microsoft Azure allow businesses to pay for only what they use, optimizing costs and improving ROI. Cloud computing services include various types of cloud computing service, such as cloud computing storage and cloud computing service, which enhance resource pooling in cloud computing.
- Performance Metrics: Â
- Throughput: The amount of data processed in a given time. We focus on optimizing throughput to enhance the performance of our AI applications.
- Latency: The time taken to process a request. Our solutions are designed to minimize latency, ensuring real-time data processing for critical applications.
- Scalability: The ability to increase resources as demand grows. Our architecture is built to scale seamlessly, accommodating the evolving needs of our clients, including high performance computing cluster and hpc computing cluster.
6.1.2. Storage Solutions
Storage solutions encompass the various methods and technologies used to store and manage data. With the exponential growth of data, effective storage solutions are vital for ensuring data accessibility, security, and integrity. Rapid Innovation implements robust storage solutions to support our AI and Blockchain projects, ensuring that our clients can manage their data efficiently.
- Types of Storage Solutions: Â
- Hard Disk Drives (HDDs): Traditional spinning disks that offer large storage capacities at a lower cost. Suitable for archiving large datasets.
- Solid State Drives (SSDs): Faster than HDDs, SSDs use flash memory to provide quicker data access and improved performance. We recommend SSDs for applications requiring high-speed data retrieval.
- Network Attached Storage (NAS): A dedicated file storage device that connects to a network, allowing multiple users to access data. This is ideal for collaborative projects.
- Cloud Storage: Services like Google Drive and Dropbox provide off-site storage solutions that are accessible from anywhere, enhancing data accessibility for our clients. Cloud computing and storage solutions are essential for managing large datasets effectively.
- Data Management: Â
- Backup Solutions: Regular backups are essential to prevent data loss. Options include local backups and cloud-based solutions, which we implement to safeguard our clients' data.
- Data Redundancy: Techniques like RAID (Redundant Array of Independent Disks) ensure data is duplicated across multiple drives for reliability, minimizing the risk of data loss.
- Security Considerations: Â
- Encryption: Protects data by converting it into a secure format. We prioritize encryption in our Blockchain solutions to ensure data integrity and confidentiality.
- Access Controls: Ensures that only authorized users can access sensitive data, a critical aspect of our security framework.
6.1.3. Network Architecture
Network architecture refers to the design and layout of a computer network, including its hardware, software, protocols, and transmission systems. A well-structured network architecture is crucial for ensuring efficient communication and data transfer. At Rapid Innovation, we design network architectures that support our AI and Blockchain solutions, ensuring seamless connectivity and data flow.
- Components of Network Architecture: Â
- Routers: Devices that forward data packets between networks, directing traffic efficiently. We optimize router configurations to enhance data transfer speeds.
- Switches: Connect devices within a network, allowing them to communicate with each other. Our network designs ensure minimal latency and maximum throughput.
- Firewalls: Security devices that monitor and control incoming and outgoing network traffic based on predetermined security rules. We implement robust firewall solutions to protect our clients' networks.
- Types of Network Architectures: Â
- Client-Server Architecture: A centralized model where clients request resources from a server. This model is often used in our enterprise solutions.
- Peer-to-Peer (P2P) Architecture: A decentralized model where each device can act as both a client and a server. This architecture is beneficial for Blockchain applications, enhancing resilience and security.
- Cloud-Based Architecture: Utilizes cloud services to provide scalable and flexible networking solutions, aligning with our commitment to delivering cost-effective solutions. Hybrid cloud network solutions are also considered to enhance flexibility.
- Network Protocols: Â
- Transmission Control Protocol/Internet Protocol (TCP/IP): The fundamental suite of protocols for internet communication. We ensure our solutions are compliant with these standards for interoperability.
- Hypertext Transfer Protocol (HTTP): Used for transferring web pages on the internet. Our web-based applications leverage HTTP for seamless user experiences.
- File Transfer Protocol (FTP): A standard network protocol used for transferring files between a client and server, facilitating efficient data exchange.
- Performance and Reliability: Â
- Bandwidth: The maximum rate of data transfer across a network. We design networks with sufficient bandwidth to support high-demand applications, including high performance computing storage.
- Latency: The delay before a transfer of data begins following an instruction. Our network solutions are optimized to minimize latency, ensuring real-time performance.
- Redundancy: Implementing backup connections to ensure network reliability in case of failure. This is a critical component of our network design, ensuring uninterrupted service for our clients, including managing cloud computing resources and utilizing virtual lab cloud computing solutions.
6.2. Data Management
Data management is a critical aspect of any organization, ensuring that data is collected, stored, and utilized effectively. Proper data management enhances decision-making, improves operational efficiency, and ensures compliance with regulations. It encompasses various processes, including data collection, storage, retrieval, and analysis. Effective data management strategies, such as master data management and energy data management, can lead to better insights and a competitive advantage in the market.
- Ensures data integrity and accuracy.
- Facilitates compliance with legal and regulatory requirements.
- Enhances data accessibility for stakeholders.
- Supports data-driven decision-making.
At Rapid Innovation, we leverage advanced AI algorithms to automate data management processes, ensuring that your organization can focus on strategic initiatives while we handle the complexities of data integrity and compliance. Our solutions, including data management software and digital asset management software, not only streamline data workflows but also enhance the accuracy of insights derived from your data, ultimately leading to greater ROI.
6.2.1. Data Collection Protocols
Data collection protocols are essential for gathering accurate and relevant data. These protocols outline the methods and procedures for collecting data, ensuring consistency and reliability. A well-defined data collection protocol can significantly impact the quality of the data collected.
To ensure effective data collection, it is important to:
- Define objectives: Clearly outline the purpose of data collection to ensure alignment with organizational goals.
- Choose appropriate methods: Select methods such as surveys, interviews, or observational studies based on the data type and objectives.
- Standardize procedures: Develop standardized procedures to minimize variability in data collection, ensuring consistency across different data collectors.
- Train personnel: Provide training for staff involved in data collection to ensure they understand the protocols and can execute them effectively.
- Monitor and evaluate: Regularly assess the data collection process to identify areas for improvement and ensure adherence to protocols.
Rapid Innovation employs AI-driven analytics to refine data collection protocols, enabling organizations to gather high-quality data that aligns with their strategic objectives. This approach not only enhances the reliability of the data but also accelerates the decision-making process, leading to improved business outcomes.
6.2.2. Storage and Retrieval Systems
Storage and retrieval systems are vital for managing data efficiently. These systems ensure that data is stored securely and can be retrieved quickly when needed. The choice of storage and retrieval systems, such as dataset management software and data center infrastructure management solutions, can significantly affect an organization’s ability to access and utilize data effectively.
To optimize storage and retrieval, organizations should:
- Choose the right storage solution: Options include cloud storage, on-premises servers, or hybrid solutions, depending on the organization’s needs and budget.
- Implement data security measures: Protect sensitive data through encryption, access controls, and regular security audits to prevent unauthorized access.
- Organize data systematically: Use a logical structure for data storage, such as categorizing by department, project, or data type, to facilitate easy retrieval.
- Utilize metadata: Implement metadata tagging to enhance data discoverability and improve search capabilities within the storage system.
- Regularly back up data: Establish a routine for data backups to prevent loss due to system failures or cyberattacks, ensuring business continuity.
At Rapid Innovation, we integrate blockchain technology into storage and retrieval systems to enhance data security and integrity. By utilizing decentralized storage solutions, we ensure that your data is not only secure but also easily accessible, providing a robust framework for data management that drives efficiency and ROI.
By focusing on effective data management, including master data governance and customer data management platforms, organizations can harness the power of their data, leading to improved performance and strategic advantages. Rapid Innovation is committed to helping you achieve these goals through our tailored AI and blockchain solutions, ensuring that your data management processes are both efficient and effective.
6.2.3. Security Measures
In today's digital landscape, security measures are paramount for protecting sensitive data and maintaining user trust. Implementing robust security protocols is essential for any organization, especially those handling personal or financial information.
- Data Encryption: Encrypting data both at rest and in transit ensures that unauthorized users cannot access sensitive information. This includes using protocols like
SSL/TLS
for data in transit andAES
for data at rest. - Access Control: Implementing strict access controls helps limit who can view or manipulate data. Role-based access control (RBAC) is a common method that assigns permissions based on user roles, ensuring that only authorized personnel can access critical systems.
- Regular Security Audits: Conducting regular security audits and vulnerability assessments helps identify potential weaknesses in the system. This proactive approach allows organizations to address issues before they can be exploited. Regular security audits are particularly important for businesses implementing cybersecurity measures for businesses.
- Multi-Factor Authentication (MFA): MFA adds an extra layer of security by requiring users to provide two or more verification factors to gain access. This significantly reduces the risk of unauthorized access.
- Incident Response Plan: Having a well-defined incident response plan ensures that organizations can quickly respond to security breaches. This plan should include steps for containment, eradication, recovery, and communication. Organizations should also consider preventive measures for ransomware attacks as part of their incident response strategy.
- Compliance with Regulations: Adhering to industry regulations such as
GDPR
,HIPAA
, orPCI-DSS
is crucial for maintaining security standards. Compliance not only protects data but also helps avoid legal repercussions. This is especially relevant for organizational security measures that focus on data privacy.
6.3. Integration with Existing Systems
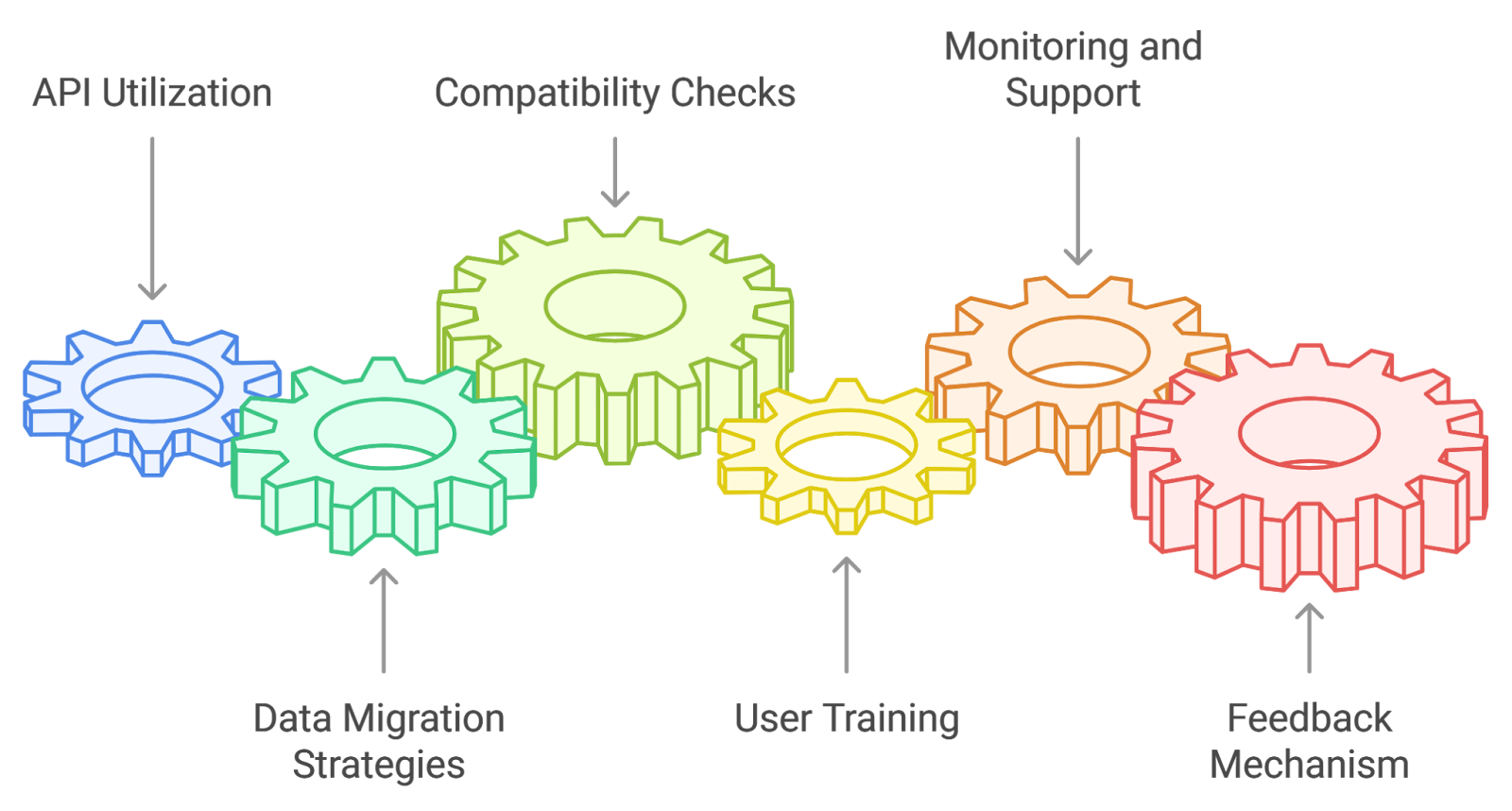
‍
Integrating new systems with existing infrastructure is a critical step in ensuring seamless operations and maximizing efficiency. Proper integration can lead to improved data flow, reduced redundancy, and enhanced user experience.
- API Utilization: Application Programming Interfaces (APIs) facilitate communication between different software applications. Utilizing APIs allows for smooth data exchange and integration with existing systems without significant overhauls.
- Data Migration Strategies: When integrating new systems, a well-planned data migration strategy is essential. This includes mapping data fields, ensuring data integrity, and minimizing downtime during the transition.
- Compatibility Checks: Before integration, it’s important to assess the compatibility of new systems with existing software and hardware. This helps identify potential issues early in the process.
- User Training: Providing training for users on the new system is vital for successful integration. This ensures that employees are comfortable with the new tools and can utilize them effectively.
- Monitoring and Support: Post-integration monitoring is crucial to identify any issues that may arise. Providing ongoing support helps users adapt to the new system and addresses any concerns promptly.
- Feedback Mechanism: Establishing a feedback mechanism allows users to report issues or suggest improvements. This can lead to continuous enhancement of the integrated systems.
6.4. Scaling Considerations
As organizations grow, their systems must be able to scale accordingly. Proper scaling considerations ensure that systems can handle increased loads without compromising performance or user experience.
- Cloud Solutions: Leveraging cloud computing can provide the flexibility needed for scaling. Cloud services allow organizations to easily adjust resources based on demand, ensuring optimal performance.
- Load Balancing: Implementing load balancing distributes incoming traffic across multiple servers. This prevents any single server from becoming overwhelmed, enhancing reliability and performance.
- Modular Architecture: Designing systems with a modular architecture allows for easier scaling. Organizations can add or remove components as needed without disrupting the entire system.
- Performance Monitoring: Regularly monitoring system performance helps identify bottlenecks and areas that require scaling. Tools that provide real-time analytics can assist in making informed decisions.
- Cost Management: Scaling can lead to increased costs, so it’s important to have a clear understanding of the financial implications. Budgeting for scaling initiatives ensures that organizations can grow sustainably.
- Future-Proofing: Considering future growth during the design phase can save time and resources later. This includes anticipating potential increases in user load and data volume, allowing for proactive scaling solutions.
At Rapid Innovation, we leverage our expertise in AI and Blockchain to enhance these security measures, ensuring that our clients not only meet compliance standards but also build a resilient infrastructure that fosters trust and efficiency. By integrating advanced AI algorithms for threat detection and utilizing Blockchain for immutable data integrity, we help organizations achieve greater ROI while safeguarding their assets. This includes implementing organizational security measures and policies in the mobile computing era, as well as ensuring effective organizational measures for handling mobile devices.
7. Challenges and Solutions
In the rapidly evolving landscape of technology, organizations face numerous challenges that can hinder their growth and efficiency. Addressing these challenges requires innovative solutions and strategic planning.
7.1 Technical Challenges
Technical challenges encompass a wide range of issues that organizations encounter when implementing new technologies or maintaining existing systems. These challenges can affect productivity, data integrity, and overall operational efficiency. Some of the key technical challenges include rapid technological advancements that can lead to outdated systems, integration issues between new and legacy systems that can create bottlenecks, increasingly sophisticated cybersecurity threats requiring constant vigilance, and skills gaps in the workforce that can hinder the effective use of new technologies.
7.1.1 Data Volume Management
Data volume management is a critical aspect of technical challenges faced by organizations today. With the exponential growth of data generated from various sources, managing this data effectively is essential for informed decision-making and operational efficiency. Organizations often struggle with data overload, which can lead to analysis paralysis. Additionally, finding cost-effective and scalable storage solutions is a challenge, as traditional storage methods may not suffice for large datasets. Ensuring data quality is crucial, as poor data quality can lead to misguided strategies and decisions. Furthermore, the demand for real-time processing is increasing, but many organizations lack the infrastructure to support it.
To address these challenges, organizations can implement several solutions:
- Data Governance Framework: Establishing a robust data governance framework can help manage data quality and compliance. This includes defining data ownership, data standards, and data lifecycle management, which is essential for master data governance.
- Cloud Storage Solutions: Utilizing cloud storage can provide scalable options for data storage, allowing organizations to expand their capacity as needed without significant upfront investment. This is particularly relevant for energy data management and engineering data management.
- Data Analytics Tools: Investing in advanced data analytics tools can help organizations process large volumes of data efficiently. These tools can provide insights that drive better decision-making, especially when integrated with data management software.
- Automated Data Management: Implementing automated data management solutions can streamline data processing, reduce manual errors, and improve overall efficiency. This is crucial for dataset management software and master data management.
- Training and Development: Upskilling employees in data management and analytics can bridge the skills gap and empower teams to leverage data effectively. This is vital for ensuring effective data security management and digital asset management software usage.
By addressing data volume management challenges with these solutions, organizations can enhance their operational efficiency and make data-driven decisions that propel growth. At Rapid Innovation, we specialize in providing tailored AI and Blockchain solutions that not only address these technical challenges but also drive greater ROI for our clients. Our expertise in advanced data analytics and cloud solutions ensures that organizations can harness the power of their data effectively, leading to improved decision-making and strategic growth. Additionally, we offer specialized services in ChatGPT applications development to further enhance organizational capabilities.
7.1.2. Processing Speed Optimization
Processing speed optimization is crucial in enhancing the performance of systems, especially in data-intensive applications. It involves improving the efficiency of algorithms and the overall system architecture to ensure faster data processing and response times. Key strategies include:
- Hardware Upgrades: Investing in high-performance hardware, such as SSDs, multi-core processors, and increased RAM, can significantly boost processing speeds, allowing businesses to handle larger datasets and more complex computations efficiently.
- Algorithm Efficiency: Optimizing algorithms to reduce time complexity can lead to faster execution. Techniques like dynamic programming and divide-and-conquer can be effective, enabling organizations to achieve quicker insights and decision-making.
- Parallel Processing: Utilizing multi-threading and distributed computing allows tasks to be executed simultaneously, drastically reducing processing time. This is particularly beneficial for applications requiring real-time data analysis.
- Data Structures: Choosing the right data structures can enhance speed. For instance, using hash tables for quick lookups instead of arrays can improve performance, leading to more responsive applications.
- Caching Mechanisms: Implementing caching strategies can minimize redundant data processing by storing frequently accessed data in memory, which can significantly reduce load times and improve user experience.
- Code Optimization: Refactoring code to eliminate bottlenecks and using efficient programming practices can lead to significant speed improvements, ensuring that applications run smoothly and efficiently. Processing speed optimization is essential for maintaining competitive advantage in today's fast-paced digital landscape.
7.1.3. Algorithm Accuracy
Algorithm accuracy is a critical factor in determining the reliability and effectiveness of computational models. It refers to how closely the output of an algorithm matches the expected results. To ensure high accuracy, consider the following:
- Validation Techniques: Employing cross-validation methods helps in assessing the accuracy of algorithms by testing them on different subsets of data, ensuring robust performance across various scenarios.
- Error Metrics: Utilizing metrics such as precision, recall, and F1 score provides insights into the performance of algorithms, especially in classification tasks, allowing businesses to fine-tune their models for better outcomes.
- Data Quality: Ensuring high-quality, clean data is essential for accurate algorithm performance. Poor data can lead to misleading results, impacting business decisions and strategies.
- Model Training: Properly training models with diverse datasets can enhance their ability to generalize and improve accuracy, which is vital for applications in dynamic environments.
- Regular Updates: Continuously updating algorithms with new data and retraining them can help maintain accuracy over time, ensuring that models remain relevant and effective.
- Bias Mitigation: Addressing biases in data and algorithms is crucial to ensure fair and accurate outcomes, fostering trust and reliability in AI-driven solutions.
7.2. Ethical Considerations

‍
Ethical considerations in technology and data processing are increasingly important as systems become more integrated into daily life. These considerations ensure that technology is used responsibly and equitably. Important aspects include:
- Data Privacy: Protecting user data and ensuring that personal information is handled with care is paramount. Organizations must comply with regulations like GDPR to maintain user trust.
- Transparency: Providing clear information about how algorithms work and the data they use fosters trust among users and stakeholders, which is essential for the adoption of AI and blockchain technologies.
- Bias and Fairness: Algorithms must be designed to minimize bias and ensure fairness, particularly in sensitive areas like hiring, lending, and law enforcement, to promote equitable outcomes.
- Accountability: Establishing accountability for algorithmic decisions is essential. Organizations should have mechanisms in place to address errors and grievances, ensuring responsible use of technology.
- Informed Consent: Users should be informed about how their data will be used and have the option to consent to its use, reinforcing ethical standards in data handling.
- Impact Assessment: Conducting ethical impact assessments can help identify potential negative consequences of deploying certain technologies, guiding organizations in making responsible decisions.
At Rapid Innovation, we leverage our expertise in AI and blockchain to help clients navigate these complexities, ensuring that their systems are not only efficient and effective but also ethical and responsible. By optimizing processing speeds and enhancing algorithm accuracy, we empower businesses to achieve greater ROI while adhering to ethical standards.
7.2.1. Privacy Concerns
Privacy concerns have become increasingly significant in today's digital landscape. With the rise of technology and data collection, individuals are more aware of how their personal information is used and shared. Personal data is often collected without explicit consent, leading to a lack of trust between users and organizations. Many companies track user behavior online, raising questions about how this data is utilized and who has access to it. The potential for data breaches exposes sensitive information, including financial details and personal identifiers, which can lead to identity theft. Regulations like the General Data Protection Regulation (GDPR) and the California Consumer Privacy Act (CCPA) have been implemented to protect user privacy, but compliance varies across organizations. Users often feel powerless in controlling their data, leading to a demand for more transparency and user-friendly privacy policies. Concerns about AI and privacy, as well as AI privacy issues, have emerged, particularly regarding AI privacy concerns and the implications of big data and privacy issues. At Rapid Innovation, we leverage blockchain technology to create decentralized solutions that empower users with greater control over their personal data, enhancing trust and compliance with privacy regulations. Additionally, discussions around biometric privacy concerns and chatgpt data privacy are becoming increasingly relevant in this context. For organizations looking to enhance their privacy measures, our AI as a Service solutions can provide innovative approaches to data management and security.
7.2.2. Data Security
Data security is a critical aspect of protecting sensitive information from unauthorized access and breaches. As cyber threats continue to evolve, organizations must adopt robust security measures to safeguard their data. Implementing strong encryption methods can protect data both in transit and at rest, making it difficult for unauthorized users to access sensitive information. Regular software updates and patches are essential to fix vulnerabilities that could be exploited by cybercriminals. Organizations should conduct regular security audits and risk assessments to identify potential weaknesses in their data protection strategies. Employee training on data security best practices can help mitigate risks associated with human error, which is often a significant factor in data breaches. Utilizing multi-factor authentication (MFA) adds an extra layer of security, ensuring that only authorized users can access sensitive data. Rapid Innovation employs advanced AI algorithms to monitor and detect anomalies in data access patterns, providing an additional layer of security to protect against potential breaches.
7.2.3. Ethical Use Guidelines
Ethical use guidelines are essential for ensuring that data is collected, stored, and utilized responsibly. These guidelines help organizations navigate the complex landscape of data ethics and maintain public trust. Organizations should establish clear policies regarding data collection, ensuring that users are informed about what data is being collected and how it will be used. Consent should be obtained from users before collecting their data, and they should have the option to withdraw consent at any time. Data should be anonymized whenever possible to protect individual identities, especially when used for research or analysis. Organizations must be transparent about their data-sharing practices, informing users if their data will be shared with third parties. Regularly reviewing and updating ethical guidelines is crucial to adapt to changing technologies and societal expectations regarding data use. At Rapid Innovation, we assist organizations in developing comprehensive ethical frameworks that align with industry standards, ensuring responsible data practices that foster trust and accountability.
7.3. Regulatory Compliance
Regulatory compliance refers to the adherence to laws, regulations, guidelines, and specifications relevant to an organization’s business processes. In many industries, especially finance, healthcare, and manufacturing, compliance is critical for operational integrity and public trust.
- Organizations must stay updated on local, national, and international regulations, including compliance regulations by industry.
- Non-compliance can lead to severe penalties, including fines, legal action, and reputational damage.
- Compliance frameworks often include:
- Data protection regulations (e.g., GDPR, HIPAA)
- Environmental regulations (e.g., EPA standards)
- Financial regulations (e.g., Sarbanes-Oxley Act, sox regulatory compliance)
- Regular audits and assessments are essential to ensure compliance with the regulations.
- Training employees on compliance matters is crucial for fostering a culture of accountability.
- Technology can aid compliance efforts through automated reporting and monitoring systems, including regtech solutions.
At Rapid Innovation, we leverage advanced AI solutions to help organizations automate compliance monitoring and reporting, ensuring they remain aligned with regulatory requirements. By implementing AI-driven analytics, we enable clients to identify compliance gaps proactively, reducing the risk of penalties and enhancing their reputation in the market.
The landscape of regulatory compliance is constantly evolving, making it essential for organizations to be proactive rather than reactive. Companies that prioritize compliance not only mitigate risks but also enhance their credibility and customer trust, particularly in areas like banking compliance regulations and pci regulatory compliance. For more information on how AI can assist in compliance monitoring, visit AI agents for compliance monitoring.
7.4. Resource Limitations
Resource limitations refer to the constraints that organizations face in terms of finances, personnel, technology, and time. These limitations can significantly impact an organization’s ability to achieve its goals and maintain operational efficiency.
- Common types of resource limitations include:
- Financial constraints that restrict investment in new projects or technologies.
- Limited human resources, leading to overworked staff and potential burnout.
- Insufficient technological infrastructure to support business operations.
- Time constraints that hinder project completion and strategic planning.
- Resource limitations can lead to:
- Decreased productivity and efficiency.
- Inability to meet customer demands or service levels.
- Challenges in innovation and growth.
- Organizations can address resource limitations by:
- Prioritizing projects based on strategic importance.
- Leveraging technology to automate processes and reduce manual workload.
- Exploring partnerships or outsourcing to fill gaps in expertise or capacity, especially in areas like industrial regulatory compliance.
- Implementing cost-control measures to optimize financial resources.
At Rapid Innovation, we understand the challenges posed by resource limitations. Our AI and blockchain solutions are designed to optimize operational efficiency, allowing organizations to automate routine tasks and focus on strategic initiatives. By utilizing our expertise, clients can maximize their resources, enhance productivity, and drive innovation.
Understanding and managing resource limitations is crucial for organizations aiming to sustain growth and competitiveness in a challenging business environment.
8. Future Perspectives
The future perspectives of any industry or organization are shaped by emerging trends, technological advancements, and changing consumer behaviors. As we look ahead, several key areas are likely to influence the landscape.
- Technological advancements will continue to drive innovation:
- Artificial intelligence and machine learning will enhance decision-making processes.
- Automation will streamline operations and reduce costs.
- Blockchain technology may revolutionize data security and transparency.
- Sustainability will become a central focus:
- Organizations will increasingly adopt eco-friendly practices to meet consumer demand.
- Regulatory pressures will push for greener operations and supply chains, including compliance with environmental regulations.
- The workforce will evolve:
- Remote work and flexible arrangements will become more common.
- Upskilling and reskilling will be essential to keep pace with technological changes.
- Consumer behavior will shift:
- Personalization and customer experience will be prioritized.
- Digital channels will dominate, necessitating a strong online presence.
Organizations that proactively adapt to these future perspectives will be better positioned to thrive in an ever-changing environment. Embracing innovation, sustainability, and a customer-centric approach will be key to long-term success. Rapid Innovation is committed to guiding clients through these transitions, ensuring they leverage the latest technologies to achieve their business goals efficiently and effectively.
8.1. Emerging Technologies
Emerging technologies are reshaping industries and driving innovation across various sectors. These technologies are characterized by their potential to create significant economic and social impacts. As businesses and governments invest in these advancements, they are paving the way for new solutions to complex problems. Key areas of focus include quantum computing and advanced artificial intelligence (AI) models. Rapid advancements in technology, including new technology and new advanced technology, are leading to new applications and solutions. Industries such as healthcare, finance, and manufacturing are particularly affected. The integration of these technologies, such as emerging technologies and new technologies 2023, can enhance efficiency and productivity, and at Rapid Innovation, we specialize in harnessing these advancements to help our clients achieve their business goals effectively and efficiently.
8.1.1. Quantum Computing Integration
Quantum computing represents a paradigm shift in computational power. Unlike classical computers, which use bits as the smallest unit of data, quantum computers use qubits. This allows them to process vast amounts of information simultaneously, making them exceptionally powerful for specific tasks. Quantum computers can solve complex problems much faster than traditional computers. They have the potential to revolutionize fields such as cryptography, drug discovery, and optimization. Companies like IBM, Google, and Microsoft are leading the charge in quantum research and development.
At Rapid Innovation, we assist organizations in integrating quantum computing into their existing systems, which can lead to enhanced data security through quantum encryption methods, improved algorithms for machine learning and AI applications, and breakthroughs in materials science and chemical simulations. As organizations begin to adopt quantum technologies, they must also consider the challenges, such as the need for specialized skills and knowledge to operate quantum systems, the high costs associated with developing and maintaining quantum infrastructure, and the current limitations in qubit stability and error rates. Our consulting services can help navigate these challenges, ensuring a smoother transition and maximizing ROI.
8.1.2. Advanced AI Models
Advanced AI models are transforming how businesses operate and make decisions. These models leverage machine learning, deep learning, and natural language processing to analyze data and generate insights. The evolution of AI has led to more sophisticated algorithms that can learn from vast datasets and improve over time. AI models can automate routine tasks, freeing up human resources for more strategic work. They enable predictive analytics, helping organizations anticipate market trends and customer needs. Industries such as retail, finance, and healthcare are increasingly relying on AI for decision-making.
Key advancements in AI models include the development of generative models, which can create new content, such as images and text; enhanced natural language processing capabilities, allowing for better human-computer interaction; and improved computer vision technologies that enable machines to interpret and understand visual data. The integration of advanced AI models can lead to increased operational efficiency through automation and optimization, enhanced customer experiences via personalized recommendations and services, and better risk management through predictive analytics and real-time data processing.
At Rapid Innovation, we provide tailored AI solutions that address specific business needs, ensuring that our clients can leverage these technologies, including new AI technology and emerging IT technologies, to drive growth and improve their bottom line. However, the adoption of advanced AI models also presents challenges, including ethical considerations surrounding data privacy and algorithmic bias, the need for transparency in AI decision-making processes, and the potential for job displacement as automation becomes more prevalent. Our expertise in AI ethics and governance helps organizations implement responsible AI practices, mitigating risks while maximizing benefits.
In conclusion, the integration of emerging technologies like quantum computing and advanced AI models, along with new technological advancements and latest technology trends, is set to redefine industries and create new opportunities. Organizations that embrace these advancements will be better positioned to thrive in an increasingly competitive landscape, and Rapid Innovation is here to guide them on this transformative journey.
8.1.3. Novel Sequencing Technologies
Novel sequencing technologies have revolutionized the field of genomics, enabling researchers to decode DNA and RNA with unprecedented speed and accuracy. These advancements have led to significant improvements in various applications, including personalized medicine, agricultural biotechnology, and environmental monitoring.
- Next-Generation Sequencing (NGS): NGS has become the gold standard in genomic research. It allows for massive parallel sequencing, meaning millions of DNA fragments can be sequenced simultaneously. This technology has drastically reduced the cost and time required for sequencing, enabling organizations to achieve greater efficiency and return on investment (ROI) in their research initiatives. Techniques such as next generation dna sequencing and dna sequencing ngs are widely utilized in this domain.
- Single-Cell Sequencing: This innovative approach allows researchers to analyze the genetic material of individual cells, providing insights into cellular heterogeneity. It is crucial for understanding complex biological systems, such as tumors or immune responses, and can lead to more targeted and effective therapeutic strategies. Single cell genome sequencing is a key method in this area.
- Long-Read Sequencing: Technologies like PacBio and Oxford Nanopore offer long-read sequencing capabilities, essential for resolving complex genomic regions that short-read technologies struggle with. This is particularly useful for studying structural variants and repetitive regions in genomes, allowing for more comprehensive genomic analyses that can drive innovation in drug development and personalized therapies. PacBio dna sequencing and pacific biosciences dna sequencing are notable examples of long-read sequencing technologies.
- Synthetic Biology Applications: Novel sequencing technologies are paving the way for advancements in synthetic biology. By enabling precise manipulation of genetic material, researchers can design and construct new biological parts, devices, and systems, which can lead to the development of novel bioproducts and solutions that meet specific market needs. Optical genome mapping is one such technique that enhances these capabilities. Additionally, Rapid Innovation offers metaverse healthcare solutions that can further enhance the application of these technologies in healthcare settings.
8.2. Research Directions
The field of genomics is rapidly evolving, and several research directions are emerging as key areas of focus. These directions aim to address current challenges and leverage new technologies for a better understanding and application of genomic data.
- Integration of Multi-Omics Data: Researchers are increasingly looking to integrate genomics with other omics data, such as proteomics and metabolomics. This holistic approach can provide a more comprehensive understanding of biological systems and disease mechanisms, enhancing the ability to develop targeted interventions.
- Artificial Intelligence and Machine Learning: The application of AI and machine learning in genomics is gaining traction. These technologies can help analyze vast datasets, identify patterns, and predict outcomes, thus enhancing the interpretation of genomic information. Rapid Innovation specializes in implementing AI solutions that can streamline data analysis processes, leading to faster insights and improved decision-making for our clients.
- CRISPR and Gene Editing: The continued exploration of CRISPR technology is a significant research direction. Scientists are investigating its potential for therapeutic applications, including gene therapy for genetic disorders and cancer treatment. Rapid Innovation can assist organizations in navigating the complexities of gene editing technologies, ensuring compliance with regulatory standards while maximizing research potential.
- Population Genomics: Understanding genetic diversity within and between populations is crucial for conservation efforts and public health. Research in this area focuses on how genetic variation influences traits and disease susceptibility, providing valuable insights for healthcare and environmental strategies.
8.3. Industry Trends
The genomics industry is witnessing several trends that are shaping its future. These trends reflect the growing importance of genomic data in various sectors, including healthcare, agriculture, and biotechnology.
- Personalized Medicine: There is a strong shift towards personalized medicine, where treatments are tailored to individual genetic profiles. This trend is driven by advancements in genomic sequencing and the increasing availability of genetic testing. Rapid Innovation can help healthcare providers implement genomic data into their practices, enhancing patient outcomes and operational efficiency.
- Direct-to-Consumer Genetic Testing: The rise of direct-to-consumer genetic testing services has made genomic information more accessible to the public. Companies like 23andMe and AncestryDNA are popularizing genetic testing for health insights and ancestry information, creating new market opportunities for businesses in the genomics space.
- Genomic Data Privacy: As genomic data becomes more prevalent, concerns about data privacy and security are rising. The industry is focusing on developing robust frameworks to protect individuals' genetic information while enabling research and innovation. Rapid Innovation offers blockchain solutions that can enhance data security and integrity, ensuring compliance with privacy regulations.
- Biobanking and Data Sharing: The establishment of biobanks and data-sharing initiatives is on the rise. These resources facilitate large-scale genomic studies and promote collaboration among researchers, ultimately accelerating discoveries in genomics. Rapid Innovation can assist organizations in developing secure and efficient data-sharing platforms that foster collaboration while protecting sensitive information.
- Regulatory Developments: As the genomics industry grows, regulatory bodies are adapting to ensure safety and efficacy in genetic testing and therapies. This includes guidelines for the ethical use of genomic data and oversight of gene editing technologies. Rapid Innovation provides consulting services to help clients navigate the regulatory landscape, ensuring compliance and facilitating innovation in their genomic projects.
8.4. Potential Applications
The potential applications of various technologies and methodologies are vast and varied, impacting numerous sectors. Here are some key areas where these applications can be observed:
- Healthcare: Telemedicine platforms enable remote consultations, improving access to healthcare. Wearable devices monitor patient vitals in real-time, enhancing chronic disease management. AI-driven diagnostics assist in early disease detection, leading to better patient outcomes. Rapid Innovation leverages AI to develop tailored healthcare solutions that enhance patient engagement and streamline operations, ultimately driving better ROI for healthcare providers. Additionally, technology applications in healthcare finance education are crucial for training professionals in managing healthcare costs and financial planning. For more insights on the role of AI in healthcare.
- Finance: Blockchain technology ensures secure transactions and enhances transparency in financial operations. Machine learning algorithms analyze market trends, aiding in investment decisions. Robo-advisors provide personalized financial advice based on individual risk profiles. By integrating blockchain solutions, Rapid Innovation helps financial institutions reduce fraud and operational costs, leading to significant returns on investment.
- Education: E-learning platforms offer flexible learning opportunities, catering to diverse learning styles. Virtual reality (VR) creates immersive learning experiences, particularly in fields like medicine and engineering. Data analytics helps educators tailor curricula to meet student needs effectively. Rapid Innovation's AI-driven educational tools enhance learning outcomes and operational efficiency, providing educational institutions with a competitive edge. Furthermore, technology applications in healthcare finance education can help students understand the financial aspects of healthcare systems.
- Manufacturing: Automation and robotics streamline production processes, increasing efficiency and reducing costs. IoT devices monitor equipment health, predicting maintenance needs and minimizing downtime. 3D printing enables rapid prototyping, allowing for quicker product development cycles. Rapid Innovation's expertise in AI and IoT allows manufacturers to optimize their operations, resulting in higher productivity and lower costs.
- Agriculture: Precision farming techniques optimize resource use, improving crop yields and sustainability. Drones monitor crop health and assess land conditions, providing valuable data for farmers. Genetic engineering enhances crop resilience to pests and climate change. Rapid Innovation employs AI and data analytics to empower farmers with actionable insights, leading to improved yields and profitability.
- Transportation: Autonomous vehicles promise to reduce accidents and improve traffic flow. Smart logistics systems optimize supply chain management, reducing costs and delivery times. Electric vehicles contribute to sustainability efforts, reducing carbon emissions. By implementing AI-driven logistics solutions, Rapid Innovation helps transportation companies enhance efficiency and reduce operational costs, driving greater ROI.
These applications illustrate the transformative potential of technology across various industries, driving innovation and improving efficiency.
9. Best Practices and Guidelines
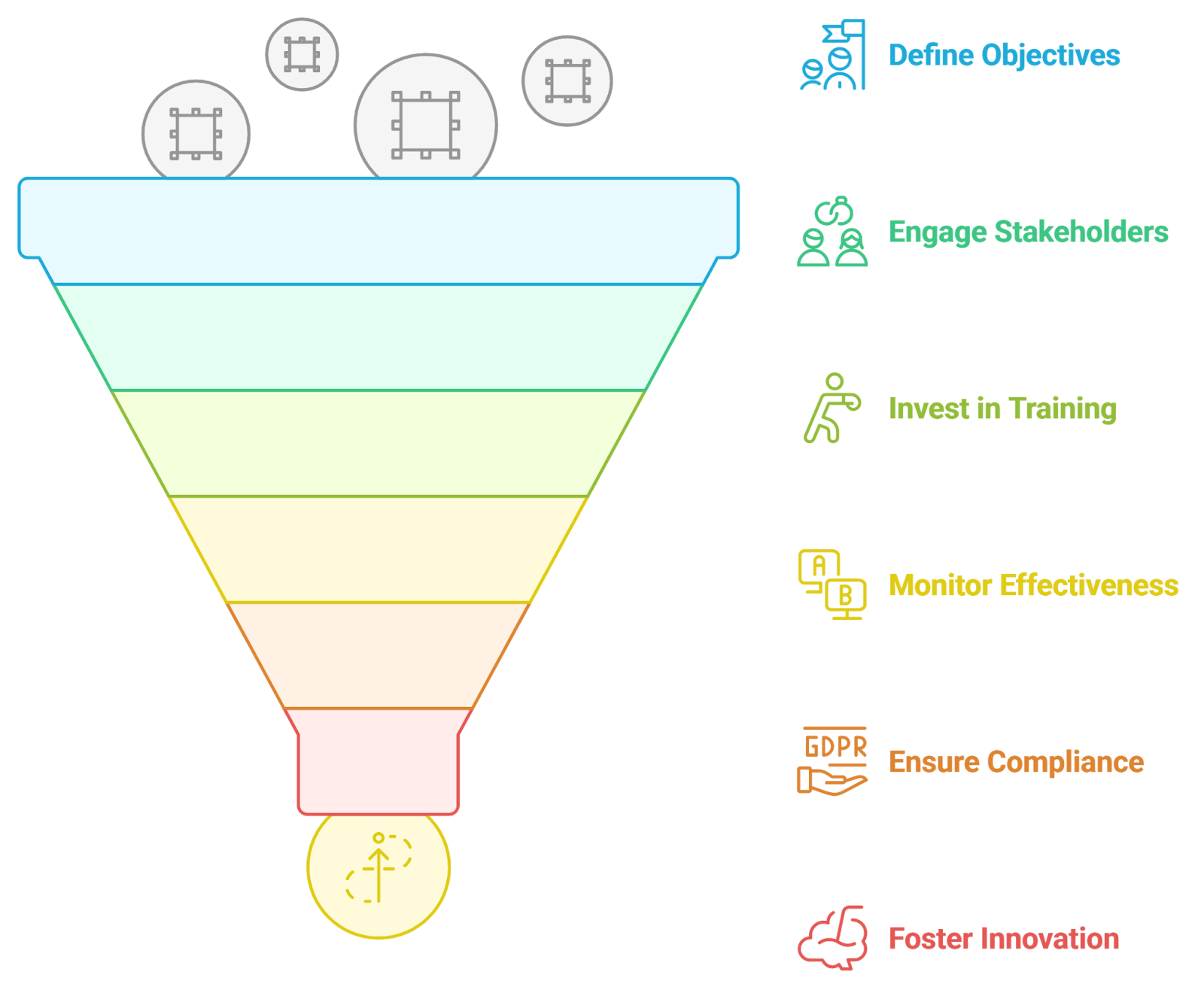
‍
Implementing best practices and guidelines is crucial for ensuring the successful adoption of new technologies and methodologies. Here are some essential considerations:
- Establish Clear Objectives: Define specific goals for technology implementation and align objectives with organizational strategy to ensure relevance.
- Engage Stakeholders: Involve key stakeholders early in the process to gather insights and foster buy-in. Maintain open communication to address concerns and expectations.
- Invest in Training: Provide comprehensive training programs for employees to enhance their skills and encourage continuous learning to keep pace with technological advancements.
- Monitor and Evaluate: Regularly assess the effectiveness of implemented technologies using metrics and KPIs to measure success and identify areas for improvement.
- Ensure Compliance: Stay updated on relevant regulations and standards to ensure compliance. Implement data protection measures to safeguard sensitive information.
- Foster a Culture of Innovation: Encourage experimentation and creativity within the organization, and recognize and reward innovative ideas and solutions.
By adhering to these best practices, organizations can maximize the benefits of new technologies while minimizing risks.
9.1. Data Quality Standards
Data quality standards are essential for ensuring the reliability and accuracy of data used in decision-making processes. High-quality data leads to better insights and outcomes. Here are key components of data quality standards:
- Accuracy: Data must be correct and free from errors. Regular audits and validation processes help maintain accuracy.
- Completeness: Ensure that all necessary data is collected and available for analysis. Identify and address gaps in data collection processes.
- Consistency: Data should be consistent across different systems and platforms. Implement standard formats and protocols to ensure uniformity.
- Timeliness: Data must be up-to-date and available when needed. Establish processes for regular data updates and maintenance.
- Relevance: Data should be pertinent to the specific context and objectives. Regularly review data sources to ensure ongoing relevance.
- Accessibility: Ensure that data is easily accessible to authorized users. Implement user-friendly interfaces and tools for data retrieval.
By adhering to these data quality standards, organizations can enhance their data management practices, leading to more informed decision-making and improved operational efficiency.
9.2. Model Selection Criteria
Choosing the right model is crucial in any data-driven project. The model selection criteria, such as aic akaike and aic criteria, help in identifying the most suitable algorithm for a specific problem. Here are some key factors to consider:
- Performance Metrics: Evaluate models based on accuracy, precision, recall, F1 score, and AUC-ROC. These metrics provide insights into how well the model performs on the given dataset, ensuring that Rapid Innovation can deliver solutions that meet client expectations.
- Complexity: Consider the complexity of the model. Simpler models are often preferred for their interpretability and ease of use, while complex models may provide better performance but can be harder to understand and maintain. Rapid Innovation emphasizes a balance between complexity and usability to enhance client engagement.
- Overfitting and Underfitting: Assess the model's ability to generalize to unseen data. A model that performs well on training data but poorly on validation data may be overfitting. Conversely, a model that performs poorly on both may be underfitting. Our team at Rapid Innovation employs rigorous testing to ensure models are robust and reliable.
- Computational Efficiency: Analyze the computational resources required for training and inference. Some models may require significant processing power and time, which can be a limiting factor in real-time applications. Rapid Innovation focuses on optimizing models to ensure they are efficient and cost-effective for our clients.
- Scalability: Ensure that the model can handle increasing amounts of data without a significant drop in performance. This is particularly important for applications that expect to grow over time. Rapid Innovation designs scalable solutions that adapt to evolving business needs.
- Domain Knowledge: Incorporate insights from the specific domain to guide model selection. Certain models may be more suitable for particular types of data or problems. Our expertise in various industries allows Rapid Innovation to tailor solutions that align with client objectives. This includes understanding information criteria and statistical modeling, as well as model selection criteria in econometrics. For more information on building domain-specific models, visit this link.
9.3. Validation Protocols
Validation protocols are essential for assessing the reliability and robustness of a model. They help ensure that the model performs well on unseen data. Key validation protocols include:
- Train-Test Split: Divide the dataset into two parts: one for training the model and the other for testing its performance. A common split is 70% for training and 30% for testing.
- Cross-Validation: Use techniques like k-fold cross-validation to evaluate the model's performance. This involves splitting the data into k subsets and training the model k times, each time using a different subset for testing.
- Leave-One-Out Cross-Validation (LOOCV): A special case of cross-validation where one observation is used for testing, and the rest for training. This is useful for small datasets but can be computationally expensive.
- Stratified Sampling: Ensure that each class is represented proportionally in both training and testing datasets. This is particularly important for imbalanced datasets.
- Performance Monitoring: Continuously monitor the model's performance over time. This can involve setting up a feedback loop to retrain the model as new data becomes available, ensuring that Rapid Innovation's solutions remain effective and relevant.
9.4. Documentation Requirements
Proper documentation is vital for maintaining transparency and reproducibility in data science projects. Documentation requirements include:
- Project Overview: Provide a clear description of the project, including objectives, scope, and the problem being addressed.
- Data Sources: Document all data sources used in the project, including how the data was collected, any preprocessing steps taken, and any transformations applied.
- Model Details: Include information about the selected model, including the algorithm used, hyperparameters, and the rationale behind the choice, such as aic model selection and aic bic model selection.
- Validation Results: Record the results of validation protocols, including performance metrics and any insights gained from the validation process.
- Version Control: Use version control systems to track changes in code, data, and documentation. This helps in maintaining a history of the project and facilitates collaboration.
- User Guide: Create a user guide that explains how to use the model, including any dependencies, installation instructions, and examples of input and output.
- Future Work: Document any limitations of the current model and suggest areas for future improvement or research. This can guide subsequent projects and help in building on existing work, ensuring that Rapid Innovation continues to provide cutting-edge solutions to our clients, including insights from multimodel inference understanding aic and bic in model selection.
9.5. Training and Support
Training and support are critical components in ensuring the successful implementation and utilization of any system or technology, particularly in the realms of AI and Blockchain. Organizations must prioritize these elements to maximize the benefits of their investments. Comprehensive training programs, including AI and blockchain training support, should be designed to cater to various user levels, from beginners to advanced users. Ongoing support is essential to address any issues that may arise post-implementation. This can include:
- Helpdesk services to assist users with technical queries
- Online resources such as FAQs and tutorials tailored to AI and Blockchain applications
- Regular updates and maintenance to ensure systems remain cutting-edge
User feedback should be actively sought to improve training materials and support services. Training can be delivered through various formats, including:
- In-person workshops focusing on hands-on experience with AI algorithms or Blockchain protocols
- Webinars that provide insights into the latest trends and best practices
- E-learning modules that allow users to learn at their own pace
Organizations should consider the following when developing training and support strategies:
- Assessing the specific needs of users to tailor content effectively
- Setting clear objectives for training sessions to ensure alignment with business goals
- Evaluating the effectiveness of training through assessments or surveys to measure ROI
- Providing access to a community forum can foster peer support and knowledge sharing among users, enhancing the learning experience.
10. Case Studies
Case studies serve as valuable tools for understanding the practical applications and outcomes of a particular system or technology, especially in AI and Blockchain. They provide real-world examples that can guide decision-making and strategy development. Case studies can highlight:
- Successful implementations of AI-driven solutions or Blockchain systems
- Challenges faced and how they were overcome, providing insights for future projects
- Measurable outcomes and benefits achieved, demonstrating ROI
They can be used to demonstrate the effectiveness of a product or service to potential clients or stakeholders. Organizations can leverage case studies to:
- Build credibility and trust in their AI and Blockchain capabilities
- Showcase expertise in a specific area, reinforcing their position in the market
- Identify best practices and lessons learned to refine future strategies
10.1. Research Institutions
Research institutions play a pivotal role in advancing knowledge and innovation across various fields, including AI and Blockchain. They often serve as testing grounds for new technologies and methodologies. These institutions typically focus on:
- Conducting rigorous scientific research that can lead to breakthroughs in AI algorithms or Blockchain applications
- Collaborating with industry partners to translate research into practical solutions
- Training the next generation of researchers and professionals in cutting-edge technologies
Research institutions can benefit from case studies by:
- Analyzing the impact of their research on society, particularly in the context of AI ethics or Blockchain transparency
- Demonstrating the value of their findings to funding bodies and policymakers, securing future investments
- Sharing successful projects to attract new partnerships and collaborations, enhancing their research capabilities
They often publish their findings in academic journals, which can further enhance their reputation and visibility in the research community. Engaging with the broader community through outreach programs can also help research institutions disseminate knowledge and foster public interest in science and technology.
10.2. Clinical Applications
Clinical applications of various medical technologies and treatments are crucial for improving patient outcomes and enhancing healthcare delivery. These applications span a wide range of areas, including diagnostics, therapeutics, and patient management.
- Diagnostics: Advanced imaging techniques, such as MRI and CT scans, allow for early detection of diseases. Additionally, molecular diagnostics enable personalized medicine by identifying specific genetic markers. Rapid Innovation leverages AI algorithms to enhance diagnostic accuracy, enabling healthcare providers to make informed decisions quickly, ultimately leading to better patient outcomes. The integration of IoT medical devices and electronic health record software solutions further enhances diagnostic capabilities.
- Therapeutics: Targeted therapies, such as monoclonal antibodies, are designed to treat specific types of cancer, improving efficacy and reducing side effects. Furthermore, gene therapy offers potential cures for genetic disorders by correcting defective genes. By utilizing blockchain technology, Rapid Innovation ensures the integrity and traceability of therapeutic data, fostering trust and compliance in clinical trials. Connected medical devices and robotics medical devices are also playing a significant role in therapeutic applications.
- Patient Management: Telemedicine has revolutionized patient care by allowing remote consultations, which is especially beneficial for patients in rural areas. Moreover, electronic health records (EHRs) facilitate better coordination among healthcare providers. Rapid Innovation's AI-driven patient management systems optimize scheduling and resource allocation, enhancing operational efficiency and patient satisfaction. The use of IoT applications in healthcare, such as remote patient health monitoring systems and smart healthcare devices, is transforming patient management strategies.
The integration of technology in clinical applications not only enhances the quality of care but also streamlines processes, making healthcare more efficient. For those looking to develop innovative solutions in this space, Rapid Innovation offers expertise in blockchain app development tailored for the healthcare industry.
10.3. Pharmaceutical Industry

‍
The pharmaceutical industry plays a pivotal role in the development and distribution of medications that improve health outcomes globally. This sector is characterized by extensive research and development (R&D) efforts, regulatory compliance, and market dynamics.
- Research and Development: Pharmaceutical companies invest heavily in R&D to discover new drugs. The average cost to develop a new drug can exceed $2.6 billion, according to some estimates. Rapid Innovation employs AI to analyze vast datasets, accelerating drug discovery and reducing time-to-market, thereby increasing ROI for pharmaceutical clients. The use of IoT healthcare devices can also enhance data collection during clinical trials.
- Regulatory Compliance: The industry is heavily regulated to ensure the safety and efficacy of medications. Agencies like the FDA in the United States and EMA in Europe oversee the approval process for new drugs. Our blockchain solutions provide a secure and transparent framework for managing compliance documentation, ensuring that all regulatory requirements are met efficiently.
- Market Dynamics: The pharmaceutical market is influenced by factors such as patent laws, competition, and pricing strategies. Generic drugs play a significant role in making medications more affordable. Rapid Innovation's market analysis tools utilize AI to predict market trends, enabling clients to make strategic decisions that enhance their competitive edge. The emergence of digital healthcare apps and virtual healthcare apps is also reshaping market dynamics.
The pharmaceutical industry is essential for advancing medical science and providing innovative solutions to health challenges.
10.4. Biotechnology Companies
Biotechnology companies are at the forefront of developing innovative solutions that leverage biological systems and organisms. These companies focus on various applications, including drug development, agricultural biotechnology, and environmental solutions.
- Drug Development: Biotech firms often specialize in creating biologics, which are products derived from living organisms. This includes monoclonal antibodies and vaccines, which have become critical in treating diseases and preventing outbreaks. Rapid Innovation's AI-driven analytics can optimize clinical trial designs, improving success rates and reducing costs. The integration of IoT medical sensors can also enhance monitoring during drug development.
- Agricultural Biotechnology: Companies in this sector develop genetically modified organisms (GMOs) to enhance crop yields, improve resistance to pests, and reduce the need for chemical pesticides. This contributes to food security and sustainable agriculture. Our blockchain solutions can track the supply chain of biotech products, ensuring transparency and safety from farm to table.
- Environmental Solutions: Biotechnology is also applied in environmental management, such as bioremediation, where microorganisms are used to clean up contaminated environments. Rapid Innovation's AI models can predict the effectiveness of various bioremediation strategies, helping clients choose the most efficient solutions.
Biotechnology companies are essential for driving innovation and addressing some of the most pressing challenges in healthcare, agriculture, and environmental sustainability. Rapid Innovation is committed to empowering these companies with cutting-edge AI and blockchain solutions that enhance their operational efficiency and return on investment.
10.5. Lessons Learned
In any project or endeavor, reflecting on the lessons learned in project management is crucial for continuous improvement and future success. This process involves analyzing what worked well, what didn’t, and how these insights can be applied moving forward. Here are some key lessons learned that can be beneficial across various fields:
- Importance of Planning
Comprehensive planning sets the foundation for success. Anticipating potential challenges can mitigate risks, and a well-structured timeline helps keep the project on track. At Rapid Innovation, we leverage AI-driven analytics to enhance our planning processes, ensuring that our clients can foresee potential obstacles and allocate resources effectively. Utilizing a lessons learned template project management can further streamline this process. - Effective Communication
Clear communication among team members fosters collaboration. Regular updates and feedback loops enhance transparency, while utilizing various communication tools can cater to different preferences. Our blockchain solutions facilitate secure and transparent communication channels, ensuring that all stakeholders are aligned and informed throughout the project lifecycle. This aligns with the project manager lessons learned approach, emphasizing the importance of communication. - Flexibility and Adaptability
Being open to change allows teams to pivot when necessary. Adapting to unforeseen circumstances can lead to innovative solutions, and embracing a growth mindset encourages resilience. Rapid Innovation’s agile methodologies enable us to quickly adjust our strategies in response to market shifts, maximizing our clients' ROI. Lessons learned examples for IT projects often highlight the need for adaptability. - Stakeholder Engagement
Involving stakeholders early in the process ensures their needs are met. Regular engagement helps build trust and buy-in, and feedback from stakeholders can provide valuable insights for improvement. Our approach integrates stakeholder feedback into the development process, ensuring that the final product aligns with client expectations and market demands. This is a key aspect of lessons learned in project management template. - Resource Management
Efficient use of resources, including time and budget, is essential. Identifying resource constraints early can prevent delays, and prioritizing tasks based on resource availability can enhance productivity. By utilizing AI tools for resource allocation, we help our clients optimize their investments and achieve greater returns. The lessons learned project template can assist in tracking resource management effectively. For accurate project estimation, consider partnering with an AI project estimation company. - Team Dynamics
Understanding team members' strengths and weaknesses can optimize performance. Fostering a positive team culture encourages collaboration and creativity, while conflict resolution strategies are vital for maintaining harmony. At Rapid Innovation, we emphasize team synergy, ensuring that our diverse skill sets in AI and blockchain work together seamlessly to deliver exceptional results. Examples of lessons learned project management often include insights on team dynamics. - Documentation and Record-Keeping
Keeping detailed records of processes and decisions aids future projects. Documentation serves as a reference for best practices and lessons learned, and regularly updating project documentation ensures that all team members have access to the latest information, enhancing overall project efficiency. Our blockchain solutions provide immutable records, ensuring that all project documentation is secure and easily retrievable for future reference. This practice is essential for capturing lesson learned from a project and ensuring that they are not lost.
In conclusion, applying these lessons learned examples in project management can significantly enhance the success of future projects. By systematically documenting and analyzing lessons learned, organizations can foster a culture of continuous improvement and innovation.