Table Of Contents
Category
Artificial Intelligence
AIML
IoT
FinTech
Healthcare & Medicine
1. Introduction to AI-Powered Fraud Detection
Artificial Intelligence (AI) has revolutionized various sectors, and AI in fraud detection in finance is no exception. With the increasing sophistication of fraudulent activities, traditional methods of fraud detection are often inadequate. AI-powered systems leverage advanced algorithms and machine learning techniques to enhance financial security and protect against fraud.
AI systems analyze vast amounts of data in real-time, identifying patterns and anomalies that may indicate fraudulent behavior. These systems can learn from historical data, improving their accuracy over time and adapting to new fraud tactics. By automating the detection process, AI reduces the time and resources needed to identify and respond to potential fraud.
At Rapid Innovation, we specialize in implementing AI-driven fraud detection solutions tailored to meet the unique needs of our clients. Our expertise allows us to help financial institutions not only enhance their security measures but also achieve greater ROI by minimizing losses associated with AI fraud detection.
The integration of artificial intelligence fraud detection in banking not only enhances security but also improves customer trust and satisfaction. Financial institutions can provide a safer environment for transactions, which is crucial in today’s digital economy. As cyber threats continue to evolve, the need for robust AI solutions in fraud detection becomes increasingly important. AI-powered fraud detection and prevention strategies are essential for staying ahead of potential threats.
Refer to the image for a visual representation of AI-powered fraud detection systems and their functionalities:

1.1. Evolution of Fraud Detection Systems
Fraud detection systems have undergone significant evolution over the years, adapting to the changing landscape of financial transactions and criminal tactics.
- Early Detection Methods: Initially, fraud detection relied heavily on manual processes. Analysts would review transactions and look for anomalies, which was time-consuming and often ineffective.
- Introduction of Automated Systems: With the advent of technology, automated systems began to emerge in the late 20th century. These systems utilized basic algorithms to flag suspicious activities based on predefined rules.
- Data Analytics Revolution: The 2000s saw a shift towards data analytics, where large datasets could be analyzed to identify patterns indicative of fraud. This era marked the beginning of more sophisticated detection methods, including fraud detection systems used by banks.
- Machine Learning and AI: In recent years, machine learning algorithms have transformed fraud detection. These systems learn from historical data, improving their accuracy over time and adapting to new fraud tactics. Rapid Innovation leverages these advanced AI techniques to provide clients with tailored fraud detection solutions that enhance their operational efficiency and reduce losses, including ai fraud detection solutions.
- Real-Time Monitoring: Modern systems now offer real-time monitoring capabilities, allowing for immediate action on suspicious transactions. This has significantly reduced the window of opportunity for fraudsters, enabling businesses to protect their assets more effectively through fraud monitoring systems and fraud prevention systems.
1.2. The Role of AI in Modern Fraud Prevention
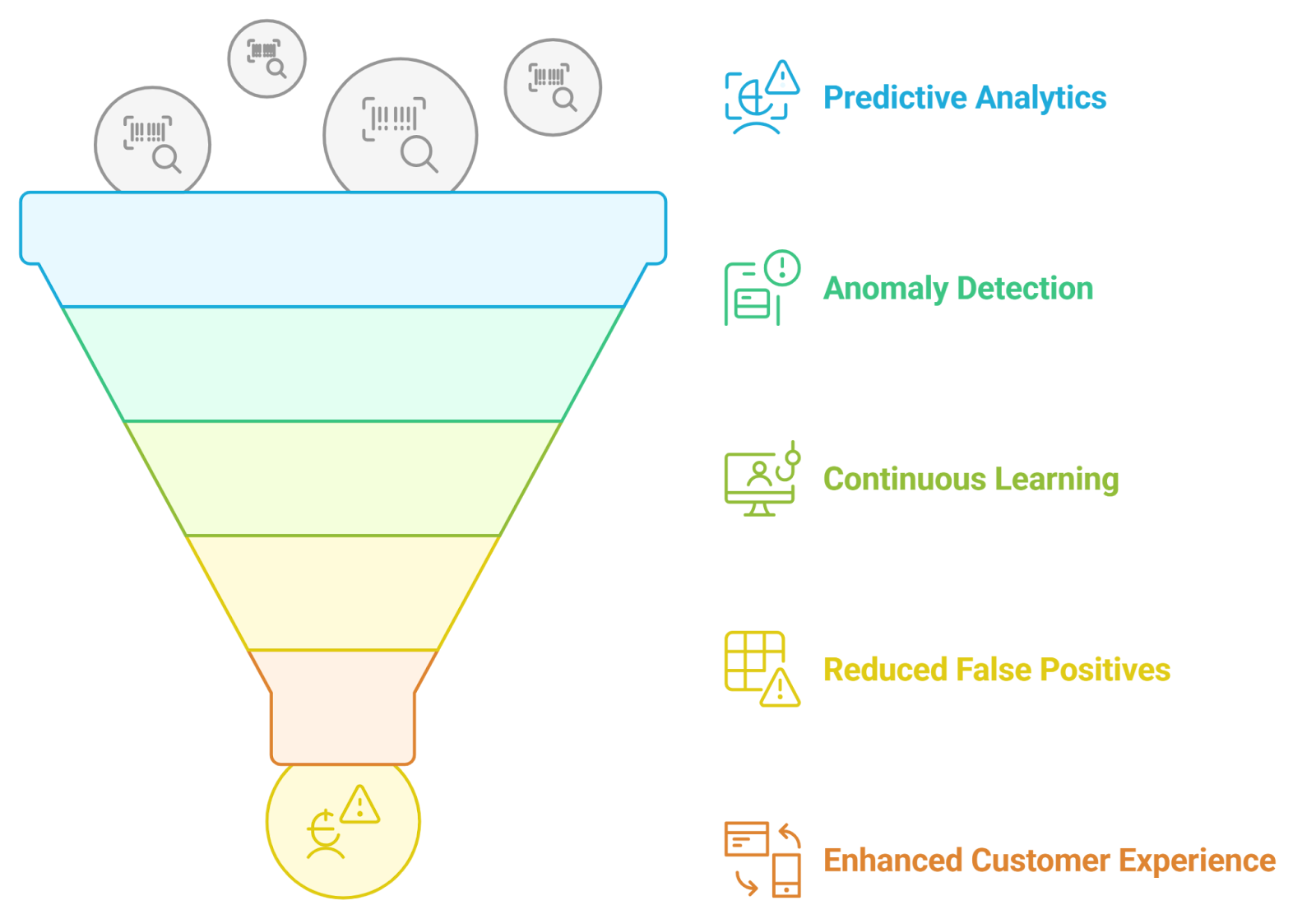
‍
Artificial Intelligence (AI) plays a crucial role in enhancing fraud prevention strategies across various sectors, particularly in finance.
- Predictive Analytics: AI algorithms analyze historical transaction data to predict potential fraud. By identifying patterns and trends, these systems can flag transactions that deviate from normal behavior. Rapid Innovation employs predictive analytics to help clients anticipate and mitigate risks before they escalate, utilizing fraud detection and prevention solutions.
- Anomaly Detection: AI excels at detecting anomalies in large datasets. It can identify unusual spending patterns or transactions that do not fit a user's profile, allowing for quick intervention. Our solutions empower clients to respond swiftly to potential threats, minimizing financial impact through anti fraud systems.
- Continuous Learning: AI systems continuously learn from new data, improving their detection capabilities. This adaptability is essential in staying ahead of evolving fraud tactics. Rapid Innovation ensures that our clients benefit from the latest advancements in AI, keeping their fraud prevention measures robust and effective, including enterprise fraud management software.
- Reduced False Positives: Traditional systems often generated numerous false positives, leading to customer dissatisfaction. AI reduces these occurrences by accurately distinguishing between legitimate and fraudulent transactions, enhancing client trust and satisfaction, particularly in credit card fraud detection systems.
- Enhanced Customer Experience: By streamlining the fraud detection process, AI allows for a smoother customer experience. Legitimate transactions are less likely to be flagged, reducing friction in the user journey. Rapid Innovation focuses on integrating AI solutions that not only protect businesses but also enhance the overall customer experience, including online fraud detection solutions.
1.3. Types of Financial Fraud
Financial fraud encompasses a wide range of illicit activities aimed at deceiving individuals or organizations for monetary gain. Understanding the various types of financial fraud is essential for effective prevention.
- Credit Card Fraud: This involves unauthorized use of a credit card to make purchases. It can occur through stolen card information or phishing scams.
- Identity Theft: Fraudsters steal personal information to impersonate individuals, often leading to unauthorized transactions or opening accounts in the victim's name.
- Investment Fraud: This type includes Ponzi schemes, where returns are paid to earlier investors using the capital from newer investors, rather than from profit earned.
- Insurance Fraud: Individuals may submit false claims or exaggerate damages to receive undeserved payouts from insurance companies.
- Mortgage Fraud: This occurs when false information is provided to secure a mortgage, often involving inflated property values or misrepresented income.
- Online Scams: With the rise of the internet, various scams such as phishing, advance-fee fraud, and fake online stores have become prevalent, targeting unsuspecting consumers.
Understanding these types of fraud is crucial for developing effective strategies to combat them and protect consumers and businesses alike. Rapid Innovation is committed to providing comprehensive solutions, including fraud management solutions and fraud management software, that address these challenges, ensuring our clients can navigate the complexities of financial fraud with confidence.
Refer to the image for a visual representation of the evolution of fraud detection systems.

1.4. Challenges in Traditional Fraud Detection
Traditional fraud detection methods often struggle to keep pace with the evolving tactics of fraudsters. These challenges can significantly hinder the effectiveness of fraud prevention strategies.
- Static Rules and Thresholds: Many traditional systems rely on predefined rules and thresholds. This approach can lead to high false positive rates, where legitimate transactions are flagged as fraudulent, causing inconvenience to customers.
- Limited Data Analysis: Traditional methods often analyze only a narrow set of data points. This limited scope can miss critical patterns and anomalies that indicate fraudulent behavior, particularly in areas like fraud detection using AI.
- Inability to Adapt: Fraud tactics are constantly changing. Traditional systems may not adapt quickly enough to new schemes, leaving organizations vulnerable to emerging threats, especially in the context of AI in fraud detection.
- Resource Intensive: Manual review processes are often required to investigate flagged transactions. This can be time-consuming and resource-intensive, leading to delays in response and increased operational costs, which can be mitigated by AI-based fraud detection.
- Lack of Real-Time Detection: Many traditional systems do not provide real-time detection capabilities. This delay can allow fraudulent transactions to be completed before they are identified and stopped, a challenge that AI in banking fraud detection aims to address.
- Data Silos: Organizations often have data spread across various systems, making it difficult to get a comprehensive view of transactions. This fragmentation can hinder effective fraud detection, particularly in banking fraud detection using machine learning.
- Human Error: Relying on human judgment for fraud detection can introduce errors. Analysts may overlook critical signs of fraud or misinterpret data, leading to incorrect conclusions, which can be reduced through AI and fraud detection technology.
2. Core Technologies and Frameworks
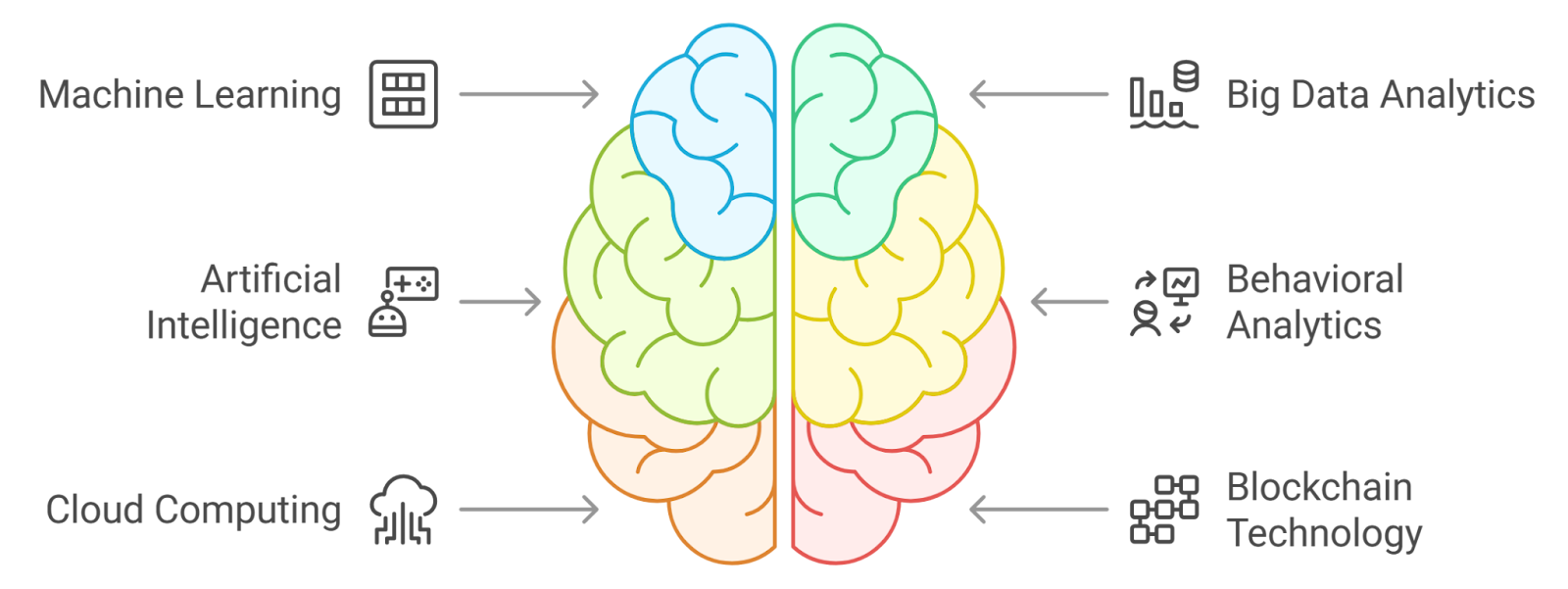
‍
The landscape of fraud detection is rapidly evolving, driven by advancements in technology. Core technologies and frameworks play a crucial role in enhancing the effectiveness of fraud detection systems.
- Machine Learning: Machine learning algorithms can analyze vast amounts of data to identify patterns and anomalies that may indicate fraud. These algorithms can learn from historical data, improving their accuracy over time, especially in applications like fraud detection using AI.
- Big Data Analytics: The ability to process and analyze large datasets in real-time allows organizations to detect fraudulent activities more effectively. Big data technologies enable the integration of diverse data sources for comprehensive analysis, which is essential for AI-based fraud detection in banking.
- Artificial Intelligence (AI): AI enhances fraud detection by automating processes and providing predictive insights. AI systems can adapt to new fraud patterns, making them more resilient against evolving threats, particularly in fraud detection artificial intelligence applications.
- Behavioral Analytics: This technology focuses on understanding user behavior to identify deviations that may signal fraud. By analyzing user interactions, organizations can detect unusual patterns that warrant further investigation, a key aspect of AI and fraud detection.
- Cloud Computing: Cloud-based solutions offer scalability and flexibility, allowing organizations to deploy advanced fraud detection systems without significant upfront investment. This technology also facilitates real-time data sharing and collaboration, crucial for effective fraud detection in banking.
- Blockchain Technology: Blockchain provides a secure and transparent way to record transactions. Its decentralized nature can help prevent fraud by ensuring data integrity and traceability, which is increasingly relevant in the context of fraud detection using artificial intelligence.
2.1. Machine Learning Foundations
Machine learning is a cornerstone of modern fraud detection systems. Understanding its foundations is essential for leveraging its capabilities effectively.
- Supervised Learning: This approach involves training algorithms on labeled datasets, where the outcome is known. Supervised learning is particularly useful for fraud detection, as it can classify transactions as legitimate or fraudulent based on historical data, including in areas like AI for fraud.
- Unsupervised Learning: In contrast, unsupervised learning deals with unlabeled data. It identifies patterns and anomalies without prior knowledge of outcomes. This method is valuable for detecting new types of fraud that have not been previously encountered, which is critical for fraud detection using machine learning in banking.
- Reinforcement Learning: This technique involves training algorithms through trial and error. The system learns to make decisions based on feedback from its actions, which can be beneficial in adapting to changing fraud patterns, particularly in AI-based fraud detection.
- Feature Engineering: The process of selecting and transforming variables (features) to improve model performance is crucial in machine learning. Effective feature engineering can enhance the ability of algorithms to detect fraud by highlighting relevant patterns, especially in fraud detection technology.
- Model Evaluation: Evaluating the performance of machine learning models is essential to ensure their effectiveness. Metrics such as precision, recall, and F1 score help assess how well the model identifies fraudulent transactions while minimizing false positives.
- Data Preprocessing: Preparing data for machine learning involves cleaning, normalizing, and transforming it into a suitable format. Proper preprocessing is vital for achieving accurate and reliable results in fraud detection.
- Continuous Learning: Machine learning models should be regularly updated with new data to maintain their effectiveness. Continuous learning allows systems to adapt to evolving fraud tactics and improve their detection capabilities over time.
At Rapid Innovation, we leverage these advanced technologies to help our clients overcome the challenges of traditional fraud detection. By implementing AI-driven solutions and blockchain technology, we enable organizations to achieve greater ROI through enhanced fraud prevention strategies, real-time detection, and reduced operational costs. Our expertise in machine learning and big data analytics ensures that our clients can stay ahead of fraudsters and protect their assets effectively through AI and fraud detection.
Refer to the image for a visual representation of the challenges in traditional fraud detection and the core technologies that can enhance fraud prevention strategies.
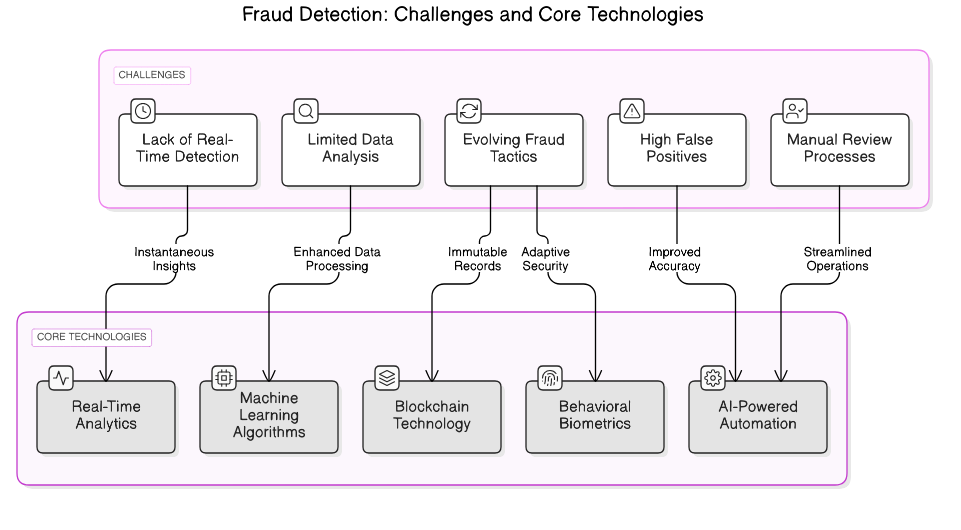
2.1.1. Supervised Learning Methods
Supervised learning is a type of machine learning where the model is trained on labeled data. This means that the input data is paired with the correct output, allowing the algorithm to learn the relationship between the two. Supervised learning is widely used in various applications, including:
- Classification: This involves categorizing data into predefined classes. For example, email filtering can classify messages as spam or not spam. This is a key aspect of machine learning classification.
- Regression: This method predicts continuous outcomes. For instance, predicting house prices based on features like size, location, and number of bedrooms.
- Common Algorithms: Some popular supervised learning algorithms include: Â
- Linear Regression
- Decision Trees
- Support Vector Machines (SVM)
- Neural Networks
- Supervised machine learning algorithms are also widely utilized in various domains.
Supervised learning is particularly effective when there is a large amount of labeled data available. The performance of these models can be evaluated using metrics such as accuracy, precision, recall, and F1 score. The key challenge in supervised learning is obtaining high-quality labeled data, which can be time-consuming and expensive. At Rapid Innovation, we leverage supervised learning techniques, including methods of machine learning and supervised machine learning example, to help clients optimize their decision-making processes, enhance customer targeting, and improve operational efficiencies, ultimately leading to greater ROI. Additionally, our expertise in adaptive AI development allows us to create tailored solutions that adapt to changing data and environments, further enhancing the effectiveness of supervised learning applications.
2.1.2. Unsupervised Learning Approaches
Unsupervised learning is a machine learning paradigm where the model is trained on data without labeled responses. The goal is to identify patterns or structures within the data. This approach is useful in scenarios where labeled data is scarce or unavailable. Key aspects of unsupervised learning include:
- Clustering: This technique groups similar data points together. For example, customer segmentation in marketing can help identify distinct groups based on purchasing behavior. Clustering machine learning is a common application in this area.
- Dimensionality Reduction: This method reduces the number of features in a dataset while preserving its essential characteristics. Techniques like Principal Component Analysis (PCA) are commonly used for this purpose.
- Anomaly Detection: Unsupervised learning can identify unusual data points that do not conform to expected patterns. This is useful in fraud detection and network security.
Common algorithms used in unsupervised learning include K-means clustering, hierarchical clustering, and Gaussian mixture models. The evaluation of unsupervised learning models can be challenging since there are no predefined labels. Techniques such as silhouette scores and the Davies-Bouldin index can help assess the quality of clustering. Rapid Innovation employs unsupervised learning methods, including example of unsupervised machine learning, to uncover hidden insights in client data, enabling them to make informed strategic decisions and enhance their market positioning.
2.1.3. Deep Learning Applications
Deep learning is a subset of machine learning that utilizes neural networks with many layers (deep networks) to model complex patterns in large datasets. It has gained significant attention due to its success in various applications, particularly in fields such as:
- Image Recognition: Deep learning models, especially Convolutional Neural Networks (CNNs), excel in tasks like facial recognition, object detection, and image classification.
- Natural Language Processing (NLP): Recurrent Neural Networks (RNNs) and Transformers are widely used for tasks such as language translation, sentiment analysis, and chatbots.
- Speech Recognition: Deep learning has revolutionized speech-to-text applications, enabling more accurate and efficient voice recognition systems.
Deep learning requires substantial computational power and large datasets for training. However, its ability to automatically extract features from raw data makes it a powerful tool for solving complex problems. The performance of deep learning models can be evaluated using metrics like accuracy, loss, and confusion matrices. As deep learning continues to evolve, its applications are expanding into areas such as healthcare, autonomous vehicles, and finance. At Rapid Innovation, we harness the power of deep learning to develop cutting-edge solutions that drive innovation and deliver measurable results for our clients, ensuring they stay ahead in a competitive landscape.
Refer to the image for a visual representation of the concepts discussed in 2.1.1 Supervised Learning Methods:

2.2. Natural Language Processing
Natural Language Processing (NLP) is a critical area of artificial intelligence that focuses on the interaction between computers and humans through natural language. It enables machines to understand, interpret, and respond to human language in a valuable way. NLP applications include chatbots, sentiment analysis, and language translation. It utilizes algorithms and models to process and analyze large amounts of textual data. Key techniques in NLP include tokenization, part-of-speech tagging, and named entity recognition. Machine learning and deep learning play significant roles in enhancing NLP capabilities, and NLP is widely used in customer service, content moderation, and social media monitoring.
At Rapid Innovation, we leverage NLP to help businesses enhance customer engagement and streamline operations. For example, our custom chatbot solutions can significantly reduce response times and improve customer satisfaction, leading to higher retention rates and increased sales. The growth of natural language programming and natural language processing has been fueled by the increasing availability of data and advancements in computational power. According to a report, the NLP market is expected to reach $43 billion by 2025, reflecting its growing importance in various industries.
Natural language analysis and natural language recognition are also key components of NLP, allowing for deeper insights into user interactions. The combination of nlp language processing and natural language programming language techniques enhances the overall effectiveness of NLP applications. Furthermore, defining nlp is essential for understanding its scope and potential in various fields. For more information on how AI agents can be utilized in marketing applications.
2.3. Network Analysis
Network Analysis is a method used to study the relationships and structures within networks, whether they are social, biological, or technological. It provides insights into how entities interact and the patterns that emerge from these interactions. It involves the use of graph theory to represent and analyze networks. Key metrics include centrality, density, and clustering coefficients. Applications range from social network analysis to fraud detection and epidemiology. Network analysis helps identify influential nodes, community structures, and information flow. Tools like Gephi and Cytoscape are commonly used for visualizing and analyzing networks.
At Rapid Innovation, we utilize network analysis to help clients optimize their marketing strategies and enhance operational efficiency. For instance, by analyzing social media networks, we can identify key influencers and tailor campaigns that resonate with target audiences. The importance of network analysis has surged with the rise of social media and interconnected systems. For instance, studies show that understanding social networks can lead to better marketing strategies and improved public health interventions.
2.4. Behavioral Analytics
Behavioral Analytics focuses on understanding user behavior through data analysis. It examines how individuals interact with products, services, or systems, providing insights that can drive business decisions. It involves collecting data from various sources, including web analytics, mobile apps, and customer feedback. Key metrics include user engagement, conversion rates, and churn rates. Behavioral analytics helps in personalizing user experiences and improving customer retention. Techniques such as cohort analysis and funnel analysis are commonly employed. Industries like e-commerce, finance, and healthcare leverage behavioral analytics for strategic insights.
At Rapid Innovation, we empower businesses to harness behavioral analytics to drive growth. By analyzing user interactions, we help clients tailor their offerings to meet customer needs, ultimately increasing conversion rates and revenue. The rise of big data has made behavioral analytics more accessible and impactful. Research indicates that companies using behavioral analytics can increase their revenue by up to 15% by better understanding customer needs and preferences.
2.5. Time Series Analysis
Time series analysis is a statistical technique that deals with time-ordered data points. It is widely used in various fields such as finance, economics, and environmental science to identify trends, seasonal patterns, and cyclical behaviors. The primary goal of time series analysis is to understand the underlying structure of the data and make forecasts based on historical patterns.
- Components of Time Series: Â
- Trend: The long-term movement in the data, indicating a general direction (upward or downward).
- Seasonality: Regular fluctuations that occur at specific intervals, such as monthly or quarterly.
- Cyclic Patterns: Long-term oscillations that are not fixed in frequency, often influenced by economic or environmental factors.
- Irregular Variations: Random, unpredictable variations that cannot be attributed to trend, seasonality, or cycles.
- Methods of Time Series Analysis: Â
- Decomposition: Breaking down the time series into its components (trend, seasonality, and irregular).
- Smoothing Techniques: Methods like moving averages or exponential smoothing to reduce noise and highlight trends.
- ARIMA Models: Autoregressive Integrated Moving Average models are used for forecasting by capturing different aspects of the time series data.
- Applications: Â
- Financial Forecasting: Predicting stock prices, interest rates, and economic indicators.
- Sales Forecasting: Estimating future sales based on historical data to optimize inventory and marketing strategies.
- Weather Prediction: Analyzing historical weather data to forecast future conditions.
Time series analysis is crucial for making informed decisions based on historical data trends. It allows businesses and researchers to anticipate future events and adjust strategies accordingly. At Rapid Innovation, we leverage advanced time series analysis techniques, including regression analysis of time series and models for time series analysis, to help our clients optimize their operations, enhance decision-making, and ultimately achieve greater ROI.
3. AI Agent Architecture for Fraud Detection
AI agent architecture for fraud detection involves the design and implementation of intelligent systems that can identify fraudulent activities in real-time. These systems leverage machine learning algorithms, data analytics, and pattern recognition to detect anomalies and prevent fraud.
- Key Features of AI Agent Architecture: Â
- Real-time Processing: Ability to analyze data as it is generated, allowing for immediate detection of suspicious activities.
- Scalability: The architecture should handle large volumes of data from various sources without compromising performance.
- Adaptability: The system must learn from new data and adapt its algorithms to improve detection accuracy over time.
- Types of AI Techniques Used: Â
- Supervised Learning: Training models on labeled datasets to classify transactions as legitimate or fraudulent.
- Unsupervised Learning: Identifying patterns in unlabeled data to detect anomalies that may indicate fraud.
- Deep Learning: Utilizing neural networks to analyze complex data patterns and improve detection capabilities.
- Integration with Existing Systems: Â
- The AI agent architecture should seamlessly integrate with existing financial systems, databases, and transaction processing systems to enhance overall security.
3.1. System Components
The system components of an AI agent architecture for fraud detection are essential for its functionality and effectiveness. Each component plays a specific role in ensuring that the system can accurately identify and respond to fraudulent activities.
- Data Collection Module: Â
- Gathers data from various sources, including transaction records, user behavior, and external databases.
- Ensures data is collected in real-time for immediate analysis.
- Data Preprocessing Module: Â
- Cleans and transforms raw data into a usable format.
- Handles missing values, outliers, and normalization to prepare data for analysis.
- Feature Engineering Module: Â
- Extracts relevant features from the preprocessed data that can enhance the model's predictive power.
- Involves techniques such as dimensionality reduction and variable selection.
- Model Training and Evaluation Module: Â
- Trains machine learning models using historical data to recognize patterns associated with fraud.
- Evaluates model performance using metrics like accuracy, precision, recall, and F1 score.
- Anomaly Detection Module: Â
- Continuously monitors transactions in real-time to identify deviations from established patterns.
- Utilizes algorithms that can flag suspicious activities for further investigation.
- Alerting and Reporting Module: Â
- Generates alerts for detected fraud cases and provides detailed reports for analysis.
- Facilitates communication with relevant stakeholders for timely action.
- Feedback Loop: Â
- Incorporates feedback from investigations and outcomes to refine models and improve detection accuracy.
- Ensures the system evolves with changing fraud patterns and tactics.
The integration of these components creates a robust AI agent architecture capable of effectively detecting and preventing fraud in various sectors, including banking, e-commerce, and insurance. By leveraging advanced technologies, organizations can enhance their security measures and protect against financial losses. Rapid Innovation specializes in developing such AI-driven solutions, enabling our clients to safeguard their assets and maximize their return on investment.
3.1.1. Data Ingestion Layer
The Data Ingestion Layer is a critical component in any data architecture, serving as the entry point for data into a system. This layer is responsible for collecting, importing, and processing data from various sources, ensuring that it is available for further analysis and processing. In the context of big data ingestion layer, this function becomes even more vital due to the volume and variety of data being handled.
- Functions of the Data Ingestion Layer: Â
- Data Collection: Gathers data from multiple sources, including databases, APIs, and real-time data streams.
- Data Transformation: Converts data into a suitable format for processing, which may involve cleaning, filtering, or enriching the data.
- Data Storage: Temporarily stores the ingested data in a staging area before it is processed or moved to a data warehouse.
- Types of Data Ingestion: Â
- Batch Ingestion: Involves collecting data at scheduled intervals. This method is suitable for large volumes of data that do not require real-time processing.
- Real-time Ingestion: Captures data continuously as it is generated. This approach is essential for applications that require immediate insights, such as fraud detection or monitoring systems.
- Tools and Technologies: Â
- Apache Kafka: A distributed streaming platform that excels in real-time data ingestion, enabling organizations to process data streams efficiently.
- Apache NiFi: A data integration tool that automates the flow of data between systems, ensuring seamless data movement and transformation.
- AWS Glue: A fully managed ETL service that simplifies data ingestion and transformation, allowing businesses to focus on deriving insights rather than managing infrastructure.
The ingestion layer in big data architecture plays a crucial role in ensuring that data is efficiently collected and made available for processing. For more insights on data science and programming, you can explore R Programming for Data Science.
3.1.2. Processing Engine
The Processing Engine is the core component that handles the transformation and processing of ingested data. It applies various algorithms and business logic to convert raw data into meaningful insights.
- Key Functions of the Processing Engine: Â
- Data Transformation: Applies transformations to the data, such as aggregations, joins, and filtering, to prepare it for analysis.
- Data Enrichment: Enhances the data by adding additional context or information, which can improve the quality of insights derived from the data.
- Scalability: The processing engine must be able to scale horizontally to handle increasing data volumes and processing demands.
- Types of Processing: Â
- Batch Processing: Processes large volumes of data at once, typically on a scheduled basis. This method is efficient for complex computations that do not require immediate results.
- Stream Processing: Processes data in real-time as it arrives. This is crucial for applications that need instant feedback, such as online recommendation systems.
- Popular Processing Engines: Â
- Apache Spark: A powerful open-source engine for large-scale data processing, supporting both batch and stream processing, enabling organizations to achieve faster insights.
- Apache Flink: A stream processing framework that provides high throughput and low latency for real-time data processing, ideal for applications requiring immediate action.
- Google Cloud Dataflow: A fully managed service for stream and batch processing that simplifies the development of data pipelines, allowing businesses to focus on their core objectives.
3.1.3. Analysis Module
The Analysis Module is where the processed data is examined to extract insights and support decision-making. This module employs various analytical techniques and tools to derive value from the data.
- Functions of the Analysis Module: Â
- Data Visualization: Presents data in graphical formats, making it easier to identify trends, patterns, and anomalies.
- Statistical Analysis: Applies statistical methods to understand data distributions, correlations, and relationships between variables.
- Predictive Analytics: Uses historical data to build models that forecast future outcomes, helping organizations make informed decisions.
- Types of Analysis: Â
- Descriptive Analysis: Summarizes historical data to understand what has happened in the past.
- Diagnostic Analysis: Investigates data to determine the causes of past outcomes.
- Prescriptive Analysis: Recommends actions based on data insights, guiding decision-makers on the best course of action.
- Tools for Analysis: Â
- Tableau: A leading data visualization tool that allows users to create interactive and shareable dashboards, enhancing data storytelling.
- R and Python: Programming languages widely used for statistical analysis and machine learning, empowering organizations to leverage advanced analytics.
- Microsoft Power BI: A business analytics tool that provides interactive visualizations and business intelligence capabilities, facilitating data-driven decision-making.
By effectively utilizing the Data Ingestion Layer, Processing Engine, and Analysis Module, organizations can create a robust data architecture that supports data-driven decision-making and enhances overall business performance. At Rapid Innovation, we specialize in implementing these components to help our clients achieve greater ROI through efficient data management and insightful analytics.
3.1.4. Alert Generation System
An Alert Generation System is a critical component in various applications, particularly in security, monitoring, and data analysis. This system is designed to detect anomalies or specific conditions and generate alerts to notify users or systems of potential issues, such as in a bridge monitoring and alert generation system using IoT.
- Functions of an Alert Generation System: Â
- Monitors data streams for predefined thresholds or patterns.
- Utilizes algorithms to identify unusual behavior or events.
- Sends notifications via email, SMS, or in-app alerts to relevant stakeholders.
- Key Features: Â
- Customizable alert settings to suit different user needs.
- Integration with other systems for comprehensive monitoring.
- Historical data analysis to improve alert accuracy over time.
- Benefits: Â
- Enhances response times to critical incidents.
- Reduces the risk of overlooking important events.
- Improves overall operational efficiency by automating alert processes.
At Rapid Innovation, we leverage advanced AI algorithms to enhance the effectiveness of our Alert Generation Systems, including alert generation systems for various applications. By employing machine learning techniques, we can fine-tune alert parameters based on historical data, ensuring that our clients receive timely and relevant notifications. This capability not only minimizes false positives but also maximizes the potential for swift action, ultimately leading to improved ROI.
3.2. Integration Frameworks
Integration Frameworks are essential for connecting various systems, applications, and data sources. They facilitate seamless communication and data exchange, enabling organizations to leverage their existing technology investments effectively.
- Types of Integration Frameworks: Â
- Middleware solutions that act as a bridge between disparate systems.
- API-based frameworks that allow for direct communication between applications.
- Event-driven architectures that respond to real-time data changes.
- Key Characteristics: Â
- Scalability to accommodate growing data and user demands.
- Flexibility to integrate with various technologies and platforms.
- Robust security measures to protect data during transmission.
- Advantages: Â
- Streamlines business processes by automating data flows.
- Enhances data accuracy and consistency across systems.
- Reduces operational costs by minimizing manual intervention.
Rapid Innovation specializes in creating tailored Integration Frameworks that align with our clients' unique business needs. By ensuring seamless integration of AI and blockchain technologies, we empower organizations to optimize their operations and achieve greater efficiency, leading to a significant return on investment.
3.3. Real-time Processing Capabilities
Real-time Processing Capabilities refer to the ability of a system to process data as it is generated or received. This capability is crucial for applications that require immediate insights and actions based on current data.
- Core Components: Â
- Stream processing engines that handle continuous data streams.
- In-memory databases that allow for rapid data retrieval and analysis.
- Event processing systems that trigger actions based on real-time events.
- Key Benefits: Â
- Enables timely decision-making by providing up-to-the-minute data.
- Improves customer experiences through instant responses and interactions.
- Supports proactive measures by identifying issues before they escalate.
- Use Cases: Â
- Fraud detection in financial transactions.
- Real-time monitoring of network security threats.
- Instant analytics for customer behavior in e-commerce platforms.
At Rapid Innovation, our expertise in real-time processing capabilities allows us to deliver solutions that not only meet but exceed client expectations. By harnessing the power of AI and blockchain, we provide our clients with the tools they need to make informed decisions quickly, thereby enhancing their operational efficiency and driving higher ROI.
3.4. Scalability Considerations
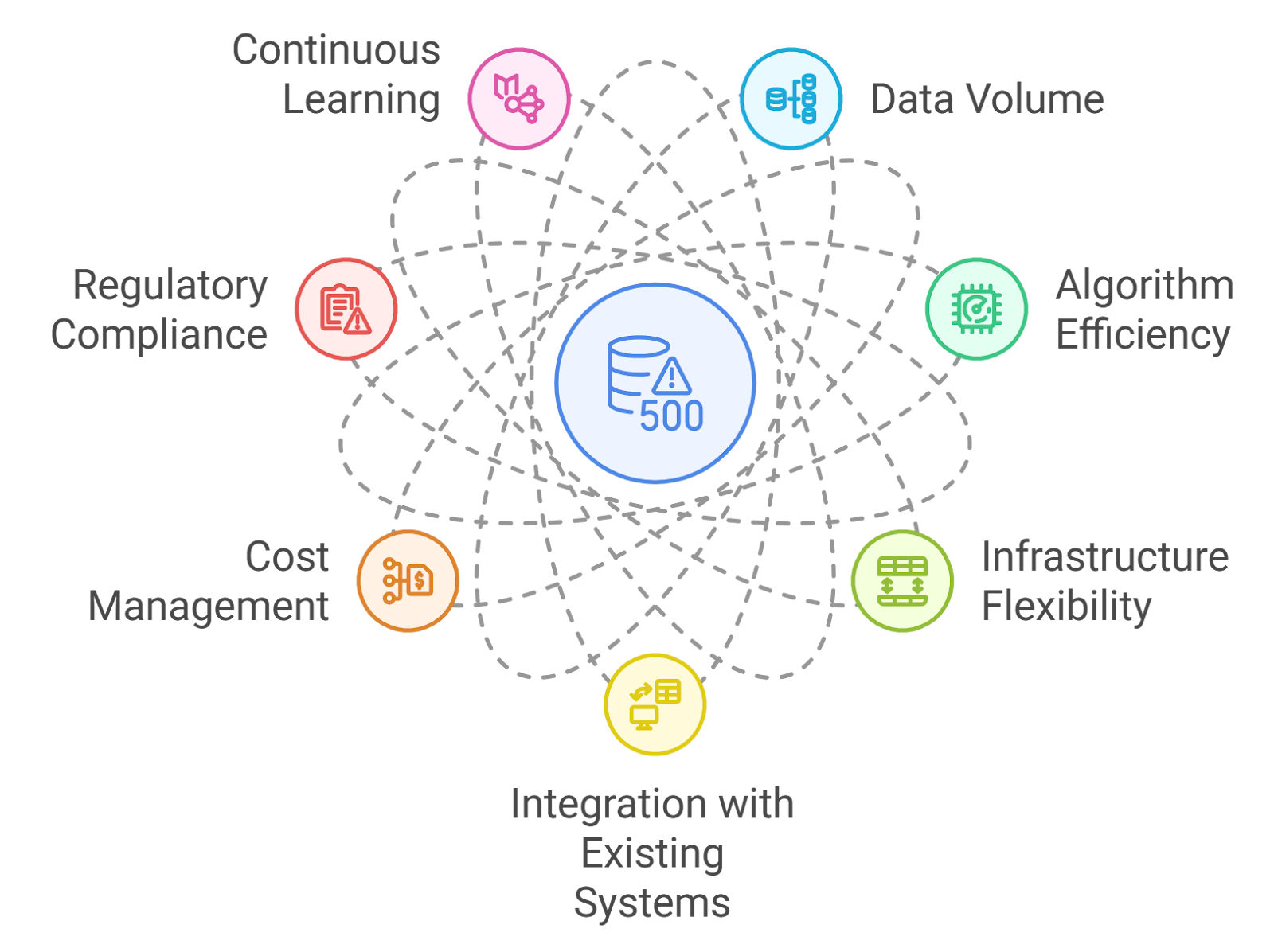
‍
Scalability is a critical factor in the design and implementation of AI fraud detection solutions. As businesses grow and transaction volumes increase, the ability of the fraud detection system to adapt and maintain performance becomes essential. Here are some key considerations regarding scalability:
- Data Volume: The amount of data processed by AI systems can grow exponentially. A scalable system should efficiently handle large datasets without compromising speed or accuracy. This often involves leveraging distributed computing and cloud-based solutions, which Rapid Innovation can implement to ensure your system remains robust as your data needs expand.
- Algorithm Efficiency: The algorithms used in fraud detection must be optimized for performance. As the volume of transactions increases, the algorithms should be able to process data in real-time or near-real-time to identify fraudulent activities promptly. Rapid Innovation specializes in developing and fine-tuning these algorithms to enhance detection capabilities and reduce false positives.
- Infrastructure Flexibility: A scalable AI fraud detection system should be built on flexible infrastructure that can be easily expanded. This includes the ability to add more servers, increase storage capacity, and enhance processing power as needed. Our team at Rapid Innovation can design infrastructure solutions that grow with your business, ensuring seamless scalability.
- Integration with Existing Systems: Scalability also involves ensuring that the AI system can integrate seamlessly with existing IT infrastructure. This includes compatibility with databases, payment gateways, and other financial systems. Rapid Innovation excels in creating tailored integration solutions that enhance your current systems without disruption.
- Cost Management: As systems scale, costs can increase significantly. It’s important to consider cost-effective solutions that allow for growth without excessive expenditure. This may involve using open-source tools or cloud services that offer pay-as-you-go pricing. Rapid Innovation can help you identify and implement cost-effective strategies that maximize your ROI while scaling your AI fraud detection capabilities.
- Regulatory Compliance: As businesses expand into new markets, they must comply with various regulations regarding data protection and fraud prevention. A scalable AI system should be adaptable to meet these regulatory requirements across different jurisdictions. Our expertise ensures that your systems remain compliant as you grow, mitigating risks associated with regulatory changes.
- Continuous Learning: A scalable AI fraud detection system should incorporate machine learning techniques that allow it to learn from new data continuously. This ensures that the system remains effective as fraud tactics evolve. Rapid Innovation focuses on developing systems that not only adapt but also improve over time, providing you with a competitive edge in fraud prevention.
4. Types of AI Fraud Detection Agents
AI fraud detection agents are specialized systems designed to identify and prevent fraudulent activities across various platforms. These agents utilize machine learning, data analytics, and other AI technologies to monitor transactions and flag suspicious behavior. Here are some common types of AI fraud detection agents:
4.1. Transaction Monitoring Agents
Transaction monitoring agents are a fundamental component of AI fraud detection systems. They continuously analyze transactions in real-time to identify patterns indicative of fraud. Key features of transaction monitoring agents include:
- Real-Time Analysis: These agents monitor transactions as they occur, allowing for immediate detection of suspicious activities. This is crucial for preventing fraud before it can cause significant damage.
- Pattern Recognition: Transaction monitoring agents use machine learning algorithms to recognize patterns associated with fraudulent behavior. They analyze historical transaction data to establish a baseline of normal activity, making it easier to identify anomalies.
- Risk Scoring: Each transaction is assigned a risk score based on various factors, such as transaction amount, location, and user behavior. High-risk transactions can be flagged for further investigation or automatically blocked.
- Alerts and Notifications: When suspicious transactions are detected, transaction monitoring agents generate alerts for compliance teams or automated systems to take action. This ensures that potential fraud is addressed promptly.
- Integration with Other Systems: These agents can be integrated with other fraud detection tools and systems, such as identity verification and customer profiling, to enhance overall fraud prevention efforts.
- Adaptive Learning: Transaction monitoring agents can adapt to new fraud patterns by continuously learning from new data. This helps them stay effective against evolving fraud tactics.
- Regulatory Compliance: Many transaction monitoring agents are designed to help organizations comply with regulatory requirements, such as Anti-Money Laundering (AML) and Know Your Customer (KYC) regulations.
- User Behavior Analytics: By analyzing user behavior, these agents can identify deviations from typical patterns, which may indicate fraudulent activity. This includes monitoring login locations, device types, and transaction frequencies.
- Cost-Effectiveness: Implementing transaction monitoring agents can be a cost-effective way to reduce fraud losses. By catching fraudulent transactions early, businesses can save money and protect their reputation.
Transaction monitoring agents play a vital role in the overall strategy for fraud detection and prevention. Their ability to analyze vast amounts of data in real-time makes them indispensable in today’s digital landscape. Rapid Innovation is committed to providing cutting-edge solutions that empower businesses to effectively combat fraud while achieving their operational goals. For more insights on the advantages and future of generative AI in finance and banking applications.
4.2. Identity Verification Agents
Identity verification agents play a crucial role in ensuring that individuals are who they claim to be. This process is essential in various sectors, including finance, healthcare, and e-commerce, where security and trust are paramount.
- These agents utilize advanced technologies such as biometric recognition, facial recognition, and document scanning to authenticate identities.
- They often cross-reference user-provided information with databases to confirm identity, reducing the risk of fraud.
- Identity verification agents can operate in real-time, providing instant results that enhance user experience while maintaining security.
- Many organizations are adopting multi-factor authentication (MFA) methods, which combine something the user knows (like a password) with something the user has (like a smartphone) to bolster security.
- Compliance with regulations such as Know Your Customer (KYC) and Anti-Money Laundering (AML) is facilitated by these agents, ensuring that businesses adhere to legal standards.
At Rapid Innovation, we leverage our expertise in AI and blockchain to develop robust identity verification solutions that not only enhance security but also streamline compliance processes. By integrating blockchain technology, we ensure that identity data is immutable and secure, further reducing the risk of identity fraud and enhancing trust among users.
4.3. Behavioral Pattern Analysis Agents
Behavioral pattern analysis agents focus on monitoring and analyzing user behavior to detect anomalies that may indicate fraudulent activities. This technology is increasingly important in cybersecurity and fraud prevention.
- These agents utilize machine learning algorithms to establish a baseline of normal user behavior, which helps in identifying deviations.
- By analyzing factors such as login times, transaction patterns, and device usage, they can flag suspicious activities for further investigation.
- Behavioral analysis can be applied in various contexts, including online banking, e-commerce, and social media platforms, enhancing security measures.
- The use of artificial intelligence (AI) in behavioral analysis allows for continuous learning and adaptation, improving the accuracy of fraud detection over time.
- Organizations can reduce false positives and enhance user experience by implementing behavioral pattern analysis, as it allows for more nuanced security measures.
Rapid Innovation employs advanced AI techniques to create behavioral pattern analysis agents that adapt to evolving user behaviors, ensuring that our clients can effectively combat fraud while maintaining a seamless user experience.
4.4. Document Verification Agents
Document verification agents are essential for validating the authenticity of documents presented by individuals or organizations. This process is vital in sectors such as banking, insurance, and legal services.
- These agents employ technologies like Optical Character Recognition (OCR) and machine learning to analyze documents for signs of forgery or tampering.
- They can verify various types of documents, including passports, driver's licenses, and utility bills, ensuring that the information provided is accurate and legitimate.
- Document verification agents often integrate with government databases to cross-check the validity of documents against official records.
- The automation of document verification processes significantly reduces the time and resources required for manual checks, enhancing operational efficiency.
- By ensuring that only legitimate documents are accepted, organizations can mitigate risks associated with identity theft and fraud.
At Rapid Innovation, we harness the power of AI and blockchain to develop document verification agents that not only automate the verification process but also ensure the integrity and security of the data involved. Our solutions help organizations minimize risks and enhance operational efficiency, ultimately leading to greater ROI.
4.5. Network Analysis Agents
Network analysis agents are specialized software programs designed to monitor, analyze, and manage network traffic and performance. These network analysis agents play a crucial role in ensuring the security and efficiency of network operations, which is essential for businesses aiming to optimize their IT infrastructure.
- Functionality: Â
- Collect data on network performance metrics such as bandwidth usage, latency, and packet loss.
- Identify anomalies and potential security threats by analyzing traffic patterns.
- Provide real-time alerts and reports to network administrators for proactive management.
- Types of Network Analysis Agents: Â
- Passive agents: Monitor network traffic without altering it, providing insights into normal operations and potential issues.
- Active agents: Generate traffic to test network performance and identify bottlenecks or vulnerabilities.
- Benefits: Â
- Enhanced visibility into network operations, allowing for quicker identification of issues.
- Improved security posture by detecting unusual activities that may indicate cyber threats.
- Optimization of network resources, leading to better performance and user experience.
- Applications: Â
- Used in enterprise networks to ensure smooth operations and security.
- Employed in cloud environments to monitor virtualized resources and services.
- Integral to the functioning of Internet of Things (IoT) devices, ensuring they communicate effectively and securely.
At Rapid Innovation, we leverage advanced network analysis agents to help our clients achieve greater ROI by ensuring their network infrastructure is both secure and efficient. By implementing these network analysis agents, businesses can reduce downtime, enhance security measures, and optimize resource allocation, ultimately leading to improved operational performance. For more insights on the use cases, benefits, and challenges of AI agents in network intelligence.
4.6. Multi-agent Systems
Multi-agent systems (MAS) consist of multiple interacting intelligent agents that work together to solve complex problems or perform tasks. These systems are designed to mimic collaborative behavior found in natural ecosystems, where individual agents contribute to a collective goal.
- Characteristics: Â
- Autonomy: Each agent operates independently, making its own decisions based on its environment and objectives.
- Communication: Agents can exchange information and coordinate actions to achieve common goals.
- Adaptability: Agents can learn from their experiences and adapt their strategies over time.
- Types of Agents: Â
- Reactive agents: Respond to environmental stimuli without internal deliberation.
- Deliberative agents: Engage in reasoning and planning to make informed decisions.
- Applications: Â
- Used in robotics for coordinated tasks, such as swarm robotics where multiple robots work together to complete a mission.
- Employed in simulations for traffic management, where agents represent vehicles that interact to optimize flow.
- Applied in distributed systems for resource allocation, where agents negotiate and collaborate to share resources efficiently.
- Benefits: Â
- Scalability: Multi-agent systems can easily expand by adding more agents to handle increased complexity.
- Robustness: The failure of one agent does not compromise the entire system, enhancing reliability.
- Flexibility: Agents can be designed to perform various tasks, making MAS adaptable to different domains.
At Rapid Innovation, we harness the power of multi-agent systems to create solutions that enhance collaboration and efficiency across various sectors. By deploying MAS, our clients can streamline operations, improve decision-making processes, and ultimately drive higher returns on investment.
5. Detection Methodologies
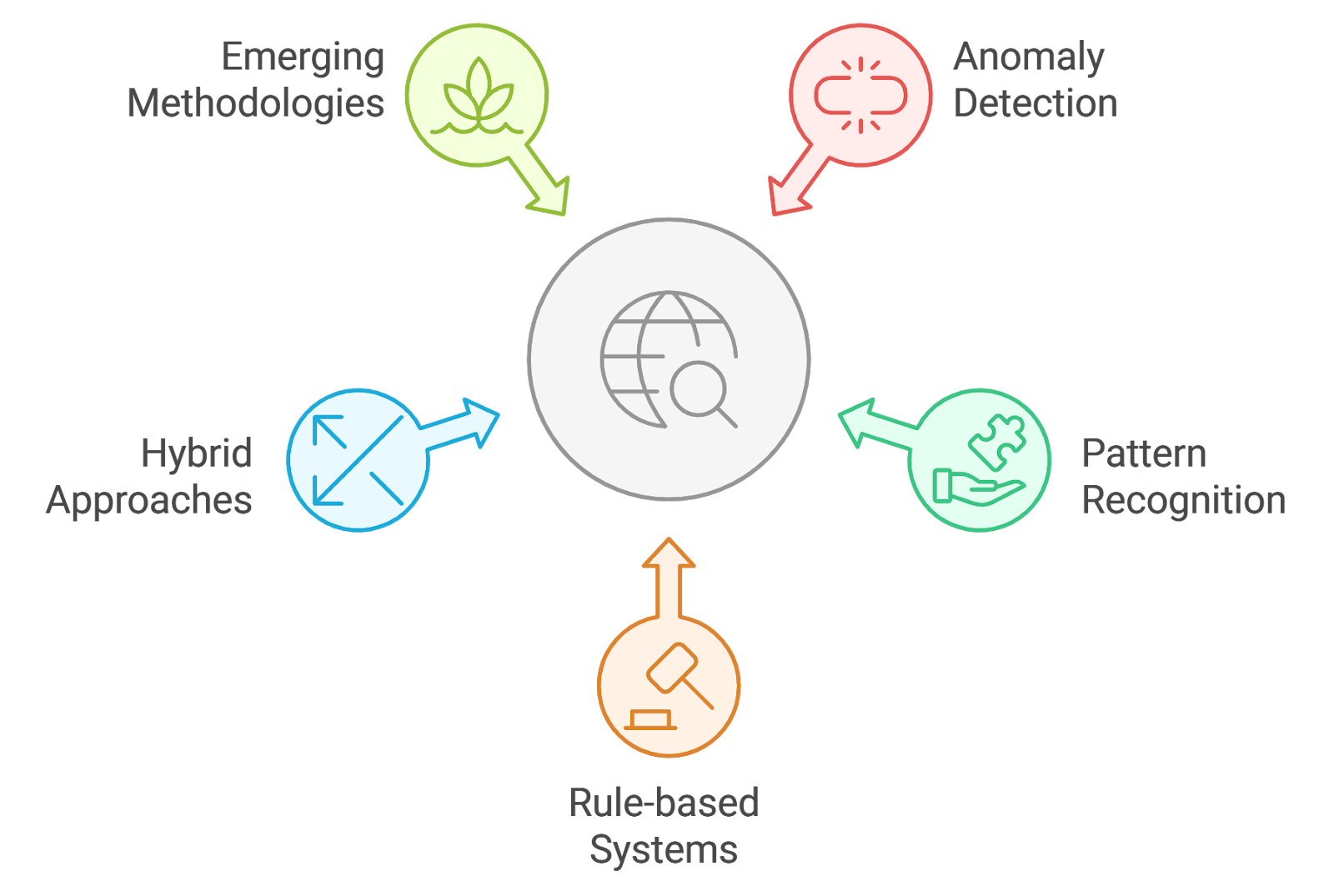
‍
Detection methodologies are systematic approaches used to identify and analyze threats, vulnerabilities, and anomalies within systems and networks. These methodologies are essential for maintaining security and ensuring the integrity of data and operations.
- Types of Detection Methodologies: Â
- Signature-based detection: Relies on known patterns or signatures of malicious activity to identify threats. This method is effective for known threats but may struggle with new or evolving attacks.
- Anomaly-based detection: Establishes a baseline of normal behavior and identifies deviations from this baseline as potential threats. This approach is useful for detecting unknown threats but may generate false positives.
- Heuristic-based detection: Utilizes algorithms and rules to identify suspicious behavior based on experience and expert knowledge. This method balances the strengths of both signature and anomaly-based detection.
- Key Components: Â
- Data collection: Gathering relevant data from various sources, including network traffic, system logs, and user behavior.
- Analysis: Employing algorithms and techniques to process the collected data and identify potential threats.
- Response: Implementing measures to mitigate identified threats, such as alerting administrators or blocking malicious activity.
- Challenges: Â
- Evolving threats: Cyber threats are constantly changing, making it difficult for detection methodologies to keep up.
- False positives: High rates of false positives can lead to alert fatigue, causing security teams to overlook genuine threats.
- Resource limitations: Effective detection often requires significant computational resources, which may not be available in all environments.
- Best Practices: Â
- Regularly update detection signatures and algorithms to stay ahead of emerging threats.
- Combine multiple detection methodologies for a more comprehensive security posture.
- Conduct regular training and simulations for security teams to improve response times and effectiveness.
At Rapid Innovation, we implement robust detection methodologies tailored to our clients' specific needs, ensuring they can effectively identify and respond to potential threats. By integrating these methodologies into their security frameworks, businesses can enhance their overall security posture and protect their valuable assets, leading to a more secure and profitable operation.
5.1. Anomaly Detection
Anomaly detection is a critical process in data analysis that identifies patterns in data that do not conform to expected behavior. This technique is widely used across various fields, including finance, healthcare, cybersecurity, and manufacturing. The primary goal of anomaly detection is to uncover rare events or observations that raise suspicions by differing significantly from the majority of the data. Anomaly detection helps in fraud detection, network security, fault detection, and monitoring environmental disturbances. It can be categorized into supervised, unsupervised, and semi-supervised learning methods. The effectiveness of anomaly detection systems relies on the quality of the data and the algorithms used.
At Rapid Innovation, we leverage advanced anomaly detection techniques, including anomaly detection using Python and scikit learn outlier detection, to help our clients enhance their operational efficiency and mitigate risks. By implementing tailored solutions, we enable organizations to identify and address anomalies in real-time, ultimately leading to greater ROI.
5.1.1. Statistical Methods
Statistical methods for anomaly detection involve using statistical tests and models to identify outliers in data. These methods are based on the assumption that the data follows a certain distribution, and deviations from this distribution are considered anomalies. Common statistical techniques include:
- Z-score: Measures how many standard deviations an element is from the mean. A high Z-score indicates an anomaly.
- Grubbs' Test: Identifies outliers in a univariate dataset assuming a normal distribution.
- Tukey's Fences: Uses interquartile ranges to detect outliers in a dataset.
The advantages of statistical methods are:
- Simplicity: Easy to implement and understand.
- Interpretability: Results are straightforward and can be easily communicated.
- Efficiency: Often computationally less intensive than machine learning methods.
However, there are limitations to statistical methods:
- Assumption of distribution: Many statistical methods assume that the data follows a specific distribution, which may not always be the case.
- Sensitivity to noise: Statistical methods can be affected by noise in the data, leading to false positives or negatives.
- Limited to linear relationships: These methods may struggle with complex, non-linear relationships in data.
5.1.2. Machine Learning Approaches
Machine learning approaches to anomaly detection leverage algorithms that can learn from data and identify patterns without explicit programming. These methods can handle large datasets and complex relationships, making them suitable for various applications. Key machine learning techniques include:
- Supervised Learning: Requires labeled data to train models. Algorithms like Support Vector Machines (SVM) and Decision Trees can be used to classify normal and anomalous data points.
- Unsupervised Learning: Does not require labeled data. Techniques such as clustering (e.g., K-means, DBSCAN) and dimensionality reduction (e.g., PCA) can help identify anomalies based on the structure of the data. This includes methods like cluster based anomaly detection and graph based anomaly detection.
- Semi-supervised Learning: Combines both labeled and unlabeled data, often using a small amount of labeled data to guide the learning process.
The advantages of machine learning approaches are:
- Flexibility: Can adapt to various types of data and distributions.
- Scalability: Capable of processing large volumes of data efficiently.
- Improved accuracy: Often more accurate than traditional statistical methods, especially in complex datasets.
Nonetheless, there are limitations to machine learning approaches:
- Data requirements: Requires a significant amount of data for training, especially for supervised methods.
- Complexity: More complex to implement and interpret compared to statistical methods.
- Risk of overfitting: Models may become too tailored to the training data, leading to poor performance on unseen data.
In conclusion, both statistical methods and machine learning approaches, including anomaly detection using machine learning and neural network anomaly detection, play vital roles in anomaly detection. The choice between these methods depends on the specific use case, the nature of the data, and the desired outcomes. Understanding the strengths and weaknesses of each approach can help organizations effectively implement anomaly detection systems tailored to their needs. At Rapid Innovation, we are committed to guiding our clients through this process, ensuring they achieve their business goals efficiently and effectively.
5.1.3. Deep Learning Models
Deep learning models are a subset of machine learning that utilize neural networks with many layers (hence "deep") to analyze various forms of data. These models have gained immense popularity due to their ability to automatically learn representations from raw data, making them particularly effective for complex tasks.
- Neural Networks: Deep learning models are primarily based on artificial neural networks, which mimic the way the human brain processes information. Each layer of the network extracts features from the input data, allowing the model to learn hierarchical representations. This includes various architectures such as convolutional neural networks (CNNs) and recurrent neural networks (RNNs).
- Applications: Deep learning is widely used in various fields, including: Â
- Image and speech recognition
- Natural language processing
- Autonomous vehicles
- Medical diagnosis
At Rapid Innovation, we leverage deep learning models, including convolutional neural networks and recurrent neural networks, to help our clients enhance their operational efficiency and drive innovation. For example, in the healthcare sector, we have developed deep learning solutions that assist in medical diagnosis, enabling healthcare providers to achieve faster and more accurate patient assessments, ultimately leading to improved patient outcomes and reduced costs.
- Performance: Deep learning models often outperform traditional machine learning algorithms, especially in tasks involving large datasets. For instance, they can achieve higher accuracy in image classification tasks compared to conventional methods.
- Frameworks: Popular frameworks for building deep learning models include TensorFlow, PyTorch, and Keras. These tools provide pre-built functions and libraries that simplify the development process, allowing Rapid Innovation to deliver tailored solutions efficiently.
- Challenges: Despite their advantages, deep learning models require significant computational resources and large amounts of labeled data for training. Overfitting is also a common issue, where the model performs well on training data but poorly on unseen data. At Rapid Innovation, we address these challenges by implementing robust data management strategies and utilizing advanced techniques to ensure our models generalize well.
5.2. Pattern Recognition
Pattern recognition is a field of artificial intelligence that focuses on the identification and classification of patterns in data. It plays a crucial role in various applications, from image processing to speech recognition.
- Definition: Pattern recognition involves the classification of input data into categories based on its features. This can be achieved through various techniques, including statistical methods, machine learning, and deep learning.
- Techniques: Common techniques used in pattern recognition include: Â
- Supervised learning: The model is trained on labeled data, learning to classify new data based on the patterns it has learned.
- Unsupervised learning: The model identifies patterns in data without prior labeling, often used for clustering similar data points.
- Feature extraction: This process involves identifying the most relevant features in the data to improve classification accuracy.
- Applications: Pattern recognition is utilized in numerous domains, such as: Â
- Facial recognition systems
- Handwriting recognition
- Medical image analysis
- Fraud detection in finance
Rapid Innovation employs advanced pattern recognition techniques to help businesses automate processes and enhance decision-making. For instance, we have developed fraud detection systems for financial institutions that utilize pattern recognition to identify suspicious transactions in real-time, significantly reducing losses and improving security.
- Importance: The ability to recognize patterns is essential for machines to interpret and respond to complex data. This capability enhances automation and decision-making processes across various industries.
5.3. Rule-based Systems
Rule-based systems are a type of artificial intelligence that uses predefined rules to make decisions or solve problems. These systems are particularly effective in environments where the rules are clear and well-defined.
- Structure: A rule-based system consists of a knowledge base and an inference engine. The knowledge base contains the rules and facts, while the inference engine applies the rules to the facts to derive conclusions.
- Types of Rules: Rules in a rule-based system can be: Â
- If-Then rules: These rules specify a condition and the corresponding action to take when the condition is met.
- Production rules: These are a set of rules that define the actions to be taken based on specific conditions.
- Applications: Rule-based systems are commonly used in: Â
- Expert systems for medical diagnosis
- Decision support systems in business
- Automated customer service systems
- Advantages: Â
- Transparency: The decision-making process is clear and understandable, as it follows explicit rules.
- Easy to update: New rules can be added or existing rules modified without overhauling the entire system.
- Limitations: Â
- Rigidity: Rule-based systems may struggle with ambiguous or complex situations that do not fit neatly into predefined rules.
- Maintenance: As the number of rules increases, managing and maintaining the system can become challenging.
At Rapid Innovation, we design rule-based systems that enhance operational efficiency and support decision-making in various industries. Our expertise ensures that these systems are not only effective but also adaptable to changing business needs.
In summary, deep learning models, including convolutional neural networks, recurrent neural networks, deep neural networks, and deep belief networks, pattern recognition, and rule-based systems are integral components of artificial intelligence, each with unique characteristics and applications. Understanding these concepts is essential for leveraging AI technologies effectively, and Rapid Innovation is here to guide you in achieving your business goals through innovative AI solutions.
5.4. Hybrid Approaches
Hybrid approaches in various fields combine elements from different methodologies to create a more effective solution. This is particularly relevant in areas like project management, software development, and data analysis. By integrating the strengths of multiple methods, hybrid approaches can address complex challenges more efficiently.
- Flexibility: Hybrid approaches allow teams to adapt to changing project requirements and environments. This flexibility can lead to better outcomes as teams can select the most suitable techniques for specific tasks. At Rapid Innovation, we leverage hybrid methodologies, such as hybrid agile and waterfall, to tailor our AI and Blockchain solutions, ensuring they meet the unique needs of our clients.
- Enhanced Collaboration: By merging different methodologies, teams can foster collaboration among members with diverse skill sets. This can lead to innovative solutions and improved problem-solving capabilities. Our interdisciplinary teams at Rapid Innovation work together to combine AI and Blockchain expertise, driving creative solutions that enhance client ROI.
- Risk Mitigation: Combining methodologies can help identify and mitigate risks more effectively. For instance, using both agile waterfall hybrid techniques can provide a structured framework while allowing for iterative improvements. Rapid Innovation employs this hybrid approach to minimize risks in AI and Blockchain projects, ensuring smoother implementation and greater success rates.
- Improved Resource Allocation: Hybrid approaches can optimize resource use by leveraging the strengths of various methodologies. This can lead to more efficient project execution and better overall performance. By utilizing hybrid strategies, such as hybrid project management methodologies, Rapid Innovation maximizes resource efficiency, ultimately delivering higher returns on investment for our clients.
5.5. Emerging Methodologies
Emerging methodologies are innovative approaches that are gaining traction in various industries. These methodologies often arise in response to the evolving needs of businesses and advancements in technology.
- Agile Methodology: Agile continues to evolve, with frameworks like Scrum and Kanban becoming increasingly popular. These methodologies emphasize iterative development, customer collaboration, and responsiveness to change. Rapid Innovation adopts Agile practices in our AI and Blockchain projects to ensure we remain aligned with client expectations and market dynamics.
- DevOps: This methodology integrates software development and IT operations, promoting a culture of collaboration and continuous improvement. DevOps aims to shorten development cycles and improve deployment frequency. At Rapid Innovation, we implement DevOps principles to enhance our AI and Blockchain development processes, resulting in faster delivery and improved quality.
- Design Thinking: This user-centered approach focuses on understanding user needs and creating solutions that address those needs effectively. Design thinking encourages creativity and innovation, making it a valuable methodology in product development. Rapid Innovation employs design thinking to ensure our AI and Blockchain solutions are not only technically sound but also user-friendly and impactful.
- Lean Startup: This methodology emphasizes rapid prototyping and validated learning. It encourages startups to test their ideas quickly and pivot based on feedback, reducing the risk of failure. Rapid Innovation supports clients in adopting Lean Startup principles, particularly in AI and Blockchain initiatives, to foster innovation while minimizing investment risks.
6. Industry-Specific Applications

‍
Industry-specific applications of methodologies are tailored to meet the unique challenges and requirements of different sectors. By customizing approaches, organizations can achieve better results and drive innovation.
- Healthcare: In healthcare, methodologies like Lean and Six Sigma are used to improve patient care and operational efficiency. These approaches help reduce waste, streamline processes, and enhance patient outcomes. Rapid Innovation applies AI and Blockchain technologies to optimize healthcare operations, ensuring better patient care and resource management.
- Manufacturing: The manufacturing sector often employs methodologies such as Total Quality Management (TQM) and Just-In-Time (JIT) to optimize production processes. These methodologies focus on quality control and minimizing inventory costs. Our AI solutions at Rapid Innovation help manufacturers enhance quality control and predictive maintenance, leading to significant cost savings.
- Information Technology: In IT, Agile and DevOps methodologies are widely adopted to enhance software development and deployment. These approaches facilitate faster delivery of high-quality software products. Rapid Innovation integrates AI and Blockchain into IT projects, improving efficiency and security in software development.
- Education: In the education sector, blended learning combines traditional teaching methods with online resources. This hybrid approach caters to diverse learning styles and enhances student engagement. Rapid Innovation leverages AI to personalize learning experiences, making education more accessible and effective.
- Finance: In finance, methodologies like Agile are increasingly used for project management and product development. This allows financial institutions to respond quickly to market changes and customer needs. Rapid Innovation utilizes Blockchain technology to enhance transparency and security in financial transactions, driving greater trust and efficiency in the sector.
6.1. Banking and Financial Services
The banking and financial services sector is a cornerstone of the global economy, providing essential services that facilitate transactions, savings, and investments. This sector has undergone significant transformation due to technological advancements and changing consumer preferences.
- Digital Banking: The rise of online banking has made financial services more accessible. Customers can manage their accounts, transfer funds, and apply for loans from the comfort of their homes. Rapid Innovation leverages AI to enhance user interfaces and streamline processes, ensuring a seamless digital banking experience that drives customer engagement and retention. Institutions like bb&t corporation and wells fargo advisors llc are leading the way in digital banking innovations.
- Fintech Innovations: Financial technology companies are disrupting traditional banking by offering innovative solutions such as peer-to-peer lending, mobile payment systems, and robo-advisors. Rapid Innovation collaborates with fintech startups to integrate blockchain technology, ensuring secure and transparent transactions that reduce costs and improve user experiences. Companies like ally financial credit card and synchrony bank credit card are examples of fintech innovations enhancing customer experiences.
- Regulatory Changes: Governments and regulatory bodies are adapting to the rapid changes in the financial landscape. New regulations aim to protect consumers while fostering innovation. Rapid Innovation provides consulting services to help financial institutions navigate these complexities, ensuring compliance while leveraging new technologies for growth. Institutions such as comerica incorporated and synovus financial are actively engaging with regulatory changes to enhance their services.
- Cybersecurity: As banking becomes increasingly digital, the importance of cybersecurity cannot be overstated. Financial institutions are investing heavily in security measures to protect sensitive customer data from breaches and fraud. Rapid Innovation employs advanced AI algorithms to detect anomalies and prevent fraud in real-time, safeguarding customer trust and institutional integrity. Companies like synchrony bank and financial first bank are prioritizing cybersecurity to protect their clients.
- Customer Experience: Banks are focusing on improving customer experience through personalized services, chatbots, and mobile apps that cater to individual needs. Rapid Innovation utilizes AI-driven analytics to understand customer behavior, enabling banks to offer tailored solutions that enhance satisfaction and loyalty. Institutions such as td auto financing and sun trust banking are implementing strategies to improve customer interactions and service delivery. For more insights on how AI is transforming banking.
6.2. E-commerce and Retail
E-commerce has revolutionized the retail landscape, providing consumers with the convenience of shopping from anywhere at any time. The growth of online shopping has reshaped consumer behavior and business strategies.
- Market Growth: The global e-commerce market is projected to reach trillions of dollars in the coming years, driven by increasing internet penetration and smartphone usage. Rapid Innovation helps e-commerce businesses scale by implementing AI solutions that optimize inventory management and demand forecasting.
- Omnichannel Retailing: Retailers are adopting omnichannel strategies, integrating online and offline experiences to meet customer expectations. This approach allows consumers to shop seamlessly across various platforms. Rapid Innovation assists retailers in creating cohesive customer journeys through data integration and AI-driven insights.
- Personalization: E-commerce platforms are leveraging data analytics to offer personalized shopping experiences. Recommendations based on browsing history and preferences enhance customer satisfaction and loyalty. Rapid Innovation employs machine learning algorithms to analyze customer data, enabling retailers to deliver highly personalized marketing campaigns.
- Mobile Commerce: With the rise of mobile devices, mobile commerce is becoming a significant part of e-commerce. Retailers are optimizing their websites and apps for mobile users to capture this growing market segment. Rapid Innovation develops mobile solutions that enhance user experience and drive conversions.
- Sustainability: Consumers are increasingly concerned about sustainability. E-commerce businesses are responding by adopting eco-friendly practices, such as sustainable packaging and carbon-neutral shipping options. Rapid Innovation supports retailers in implementing blockchain technology to ensure transparency in their supply chains, promoting sustainability and ethical practices.
6.3. Insurance
The insurance industry plays a vital role in risk management, providing individuals and businesses with financial protection against unforeseen events. The sector is evolving rapidly due to technological advancements and changing consumer expectations.
- Insurtech: The emergence of insurtech companies is transforming the insurance landscape. These startups are using technology to streamline processes, improve customer service, and offer innovative products. Rapid Innovation partners with insurtech firms to develop AI-driven solutions that enhance underwriting and claims processing.
- Data Analytics: Insurers are harnessing big data and analytics to assess risk more accurately. This enables them to offer personalized policies and pricing based on individual customer profiles. Rapid Innovation provides advanced analytics tools that empower insurers to make data-driven decisions, improving profitability and customer satisfaction.
- Customer-Centric Models: The insurance industry is shifting towards a more customer-centric approach. Companies are focusing on enhancing customer experience through digital platforms, self-service options, and transparent communication. Rapid Innovation helps insurers implement AI chatbots and digital interfaces that improve customer interactions and streamline service delivery.
- Regulatory Compliance: The insurance sector is subject to stringent regulations. Companies must navigate complex compliance requirements while adapting to new technologies and market dynamics. Rapid Innovation offers consulting services to ensure that insurers remain compliant while leveraging technology for operational efficiency.
- Emerging Risks: As the world changes, new risks emerge. Insurers are developing products to address challenges such as cyber threats, climate change, and health crises, ensuring they remain relevant in a rapidly evolving landscape. Rapid Innovation assists insurers in creating innovative products that address these emerging risks, utilizing AI and blockchain for enhanced risk assessment and management.
6.4. Healthcare
The healthcare sector is undergoing a significant transformation driven by technology, data analytics, and patient-centered care. The integration of digital tools and innovative practices is reshaping how healthcare providers deliver services and engage with patients.
- Telemedicine: The rise of telemedicine has made healthcare more accessible. Patients can consult with healthcare professionals remotely, reducing the need for in-person visits. This is particularly beneficial for those in rural areas or with mobility issues. Rapid Innovation can assist healthcare providers in developing robust telemedicine platforms that enhance patient experience and streamline operations.
- Electronic Health Records (EHR): EHR systems, including electronic health record ehr software and electronic health record ehr system, streamline patient data management, allowing for better coordination among healthcare providers. This leads to improved patient outcomes and reduced errors in treatment. Our expertise in AI can help optimize EHR systems, ensuring that data is not only stored but also analyzed for actionable insights.
- Wearable Technology: Devices like smartwatches and fitness trackers monitor health metrics in real-time. This data can be shared with healthcare providers, enabling proactive health management. Rapid Innovation can support the integration of wearable technology with healthcare systems, facilitating real-time data sharing and improving patient monitoring.
- Artificial Intelligence (AI): AI is being used for predictive analytics, helping to identify potential health risks before they become critical. This technology can also assist in diagnosing diseases more accurately. By leveraging AI, Rapid Innovation can help healthcare organizations implement advanced analytics solutions that enhance diagnostic accuracy and patient care.
- Patient Engagement: Digital platforms enhance patient engagement by providing access to health information and personalized care plans. This empowers patients to take an active role in their health. Our team can develop tailored patient engagement solutions that foster communication and improve health outcomes.
- Health Information Technology: The integration of health information technology, including health information tech and health information and technology, is crucial for improving healthcare delivery. Digital health records and medical devices companies near me are also essential components in this evolving landscape.
6.5. Telecommunications
The telecommunications industry is a cornerstone of modern communication, enabling connectivity across the globe. With the advent of 5G technology and advancements in mobile networks, the sector is poised for rapid growth and innovation.
- 5G Technology: The rollout of 5G networks promises faster data speeds and lower latency. This will enhance mobile experiences and enable new applications, such as augmented reality (AR) and virtual reality (VR). Rapid Innovation can assist telecom companies in developing applications that leverage 5G capabilities for enhanced user experiences.
- Internet of Things (IoT): Telecommunications companies are increasingly investing in IoT solutions, connecting devices and systems for smarter homes and cities. This integration improves efficiency and convenience in daily life. Our expertise in blockchain can enhance IoT security, ensuring that data transmitted between devices is secure and tamper-proof.
- Cloud Services: The shift to cloud-based services allows for scalable and flexible telecommunications solutions. Businesses can leverage these services for better communication and collaboration. Rapid Innovation can help telecom providers migrate to cloud solutions that optimize performance and reduce costs.
- Cybersecurity: As telecommunications networks expand, so do the risks associated with cyber threats. Companies are prioritizing cybersecurity measures to protect sensitive data and maintain customer trust. Our team can implement advanced cybersecurity protocols to safeguard telecommunications infrastructure.
- Regulatory Challenges: The telecommunications sector faces ongoing regulatory scrutiny. Compliance with government regulations is essential for maintaining operational integrity and ensuring fair competition. Rapid Innovation can provide consulting services to help telecom companies navigate regulatory landscapes effectively.
6.6. Government and Public Sector
The government and public sector play a crucial role in society, providing essential services and maintaining order. The integration of technology is enhancing the efficiency and effectiveness of public services.
- E-Government: Digital platforms are transforming how citizens interact with government services. E-government initiatives streamline processes, making it easier for citizens to access information and services online. Rapid Innovation can develop e-government solutions that improve service delivery and citizen engagement.
- Data Analytics: Governments are utilizing data analytics to improve decision-making and resource allocation. By analyzing data trends, public sector organizations can better address community needs. Our AI-driven analytics solutions can help government agencies derive insights from vast amounts of data, leading to more informed policy decisions.
- Smart Cities: The concept of smart cities involves using technology to improve urban living. This includes smart transportation systems, energy-efficient buildings, and enhanced public safety measures. Rapid Innovation can assist in the development of smart city initiatives that leverage IoT and AI for improved urban management.
- Citizen Engagement: Technology facilitates better communication between governments and citizens. Social media and mobile apps allow for real-time feedback and engagement, fostering a more participatory democracy. Our solutions can enhance citizen engagement platforms, ensuring that feedback is effectively captured and addressed.
- Cybersecurity in Government: Protecting sensitive information is paramount for government agencies. Investments in cybersecurity measures are essential to safeguard data and maintain public trust. Rapid Innovation can provide comprehensive cybersecurity strategies tailored to the unique needs of government entities.
7. Implementation Strategy
An effective implementation strategy is crucial for the success of any project, particularly in technology-driven initiatives. This strategy outlines the steps necessary to ensure that the project meets its objectives and delivers value to stakeholders. A well-defined strategy implementation plan is essential for guiding the project from conception to completion.
7.1 System Requirements Analysis
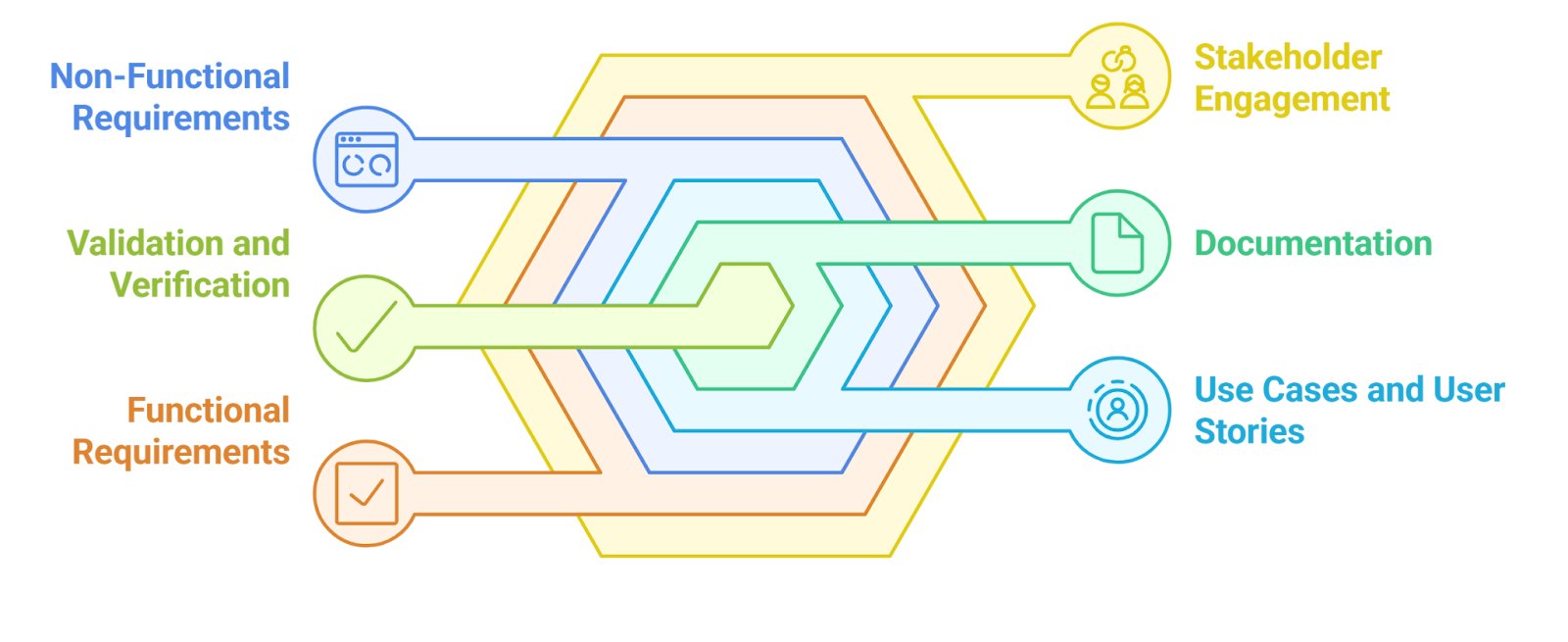
‍
System requirements analysis is the process of identifying and documenting the needs and expectations of stakeholders regarding a system. This phase is essential for ensuring that the final product aligns with user requirements and business goals.
- Stakeholder Engagement: Involve all relevant stakeholders, including end-users, management, and technical teams, to gather comprehensive requirements. At Rapid Innovation, we prioritize stakeholder engagement to ensure that every voice is heard, leading to a more tailored solution.
- Functional Requirements: Define what the system should do, including specific features and functionalities, such as user interfaces, data processing capabilities, and integration with other systems. Our expertise in AI and Blockchain allows us to create innovative functionalities that drive efficiency.
- Non-Functional Requirements: Identify performance metrics such as reliability, scalability, security, and usability, which are critical for the system's overall effectiveness. We leverage our knowledge in Blockchain to enhance security and reliability in your projects.
- Use Cases and User Stories: Develop use cases and user stories to illustrate how users will interact with the system. This helps in visualizing requirements and ensuring they are user-centric. Our team excels in crafting user stories that resonate with end-users, ensuring a seamless experience.
- Documentation: Create detailed documentation of all requirements, serving as a reference throughout the project lifecycle. This documentation should be clear and accessible to all stakeholders. We ensure that our documentation is comprehensive, facilitating smooth communication among all parties involved.
- Validation and Verification: Regularly review and validate requirements with stakeholders to ensure they remain relevant and achievable, helping to identify any necessary changes early in the process. Our iterative approach ensures that we adapt to changes swiftly, minimizing risks.
7.2 Data Preparation and Quality
Data preparation and quality are critical components of any implementation strategy, especially in data-driven projects. High-quality data is essential for accurate analysis and decision-making.
- Data Collection: Gather data from various sources, ensuring that it is relevant to the project objectives. This may include internal databases, external APIs, and user-generated content. Our expertise in AI enables us to efficiently collect and analyze vast amounts of data.
- Data Cleaning: Identify and rectify errors in the data, such as duplicates, missing values, and inconsistencies, which are vital for maintaining data integrity. We utilize advanced algorithms to automate data cleaning processes, ensuring high-quality datasets.
- Data Transformation: Convert data into a suitable format for analysis, which may involve normalizing data, aggregating information, or creating new variables that enhance the dataset's usability. Our team is skilled in transforming data to meet specific analytical needs.
- Data Quality Assessment: Evaluate the quality of the data using metrics such as accuracy, completeness, consistency, and timeliness. This assessment helps in identifying areas that require improvement. We implement robust quality checks to ensure that your data is always reliable.
- Data Governance: Establish data governance policies to ensure ongoing data quality, including defining roles and responsibilities for data management and implementing processes for regular data audits. Our approach to data governance ensures compliance and security, particularly in Blockchain applications.
- Documentation of Data Sources: Maintain a record of all data sources and their characteristics, which is essential for transparency and for future reference during data analysis. We emphasize thorough documentation to support ongoing data management efforts.
By focusing on system requirements analysis and data preparation and quality, organizations can lay a solid foundation for successful project implementation. These steps not only enhance the likelihood of meeting project goals but also ensure that the final product is robust, reliable, and user-friendly. At Rapid Innovation, we are committed to helping you achieve greater ROI through our tailored AI solutions and Blockchain solutions, utilizing effective change implementation strategies and strategic planning and implementation practices. Additionally, understanding the critical role of data quality in AI implementations is essential for ensuring the success of your projects.
7.3. Model Development
Model development is a critical phase in the data science workflow, where the goal is to create a predictive model that can effectively analyze data and provide insights. This process involves several key steps, including feature engineering and model selection. Each of these steps plays a vital role in ensuring that the final model is both accurate and reliable. Various methodologies, such as the waterfall model software engineering and agile development model in software engineering, can also influence the model development process.
7.3.1. Feature Engineering
Feature engineering is the process of transforming raw data into meaningful features that can improve the performance of machine learning models. It involves selecting, modifying, or creating new variables that can help the model learn patterns more effectively. Effective feature engineering can significantly impact the performance of machine learning models, making it a crucial step in model development.
- Importance of Feature Engineering: Â
- Enhances model accuracy by providing relevant information.
- Reduces overfitting by simplifying the model.
- Improves interpretability of the model results.
- Techniques in Feature Engineering: Â
- Normalization and Scaling: Adjusting the range of features to ensure they contribute equally to the model.
- Encoding Categorical Variables: Converting categorical data into numerical format using techniques like one-hot encoding or label encoding.
- Creating Interaction Features: Combining two or more features to capture relationships that may not be evident when considered separately.
- Dimensionality Reduction: Techniques like PCA (Principal Component Analysis) can help reduce the number of features while retaining essential information.
- Tools for Feature Engineering: Â
- Libraries such as Pandas and Scikit-learn in Python provide functions for data manipulation and feature transformation.
- Automated feature engineering tools like Featuretools can help streamline the process.
At Rapid Innovation, we leverage advanced feature engineering techniques to ensure that our clients' models are not only accurate but also robust. By tailoring features to the specific needs of the business, we help clients achieve greater ROI through improved decision-making capabilities.
7.3.2. Model Selection
Model selection is the process of choosing the most appropriate machine learning algorithm for a given problem. This step is essential because different algorithms have varying strengths and weaknesses, and the right choice can lead to better predictive performance. Choosing the right model is crucial for achieving optimal results in predictive analytics. Approaches like the software development spiral model and the prototyping model in software engineering can also guide this selection process.
- Factors to Consider in Model Selection: Â
- Nature of the Data: The type of data (e.g., structured vs. unstructured) can influence the choice of model.
- Problem Type: Whether the task is classification, regression, or clustering will determine the suitable algorithms.
- Performance Metrics: Understanding which metrics (e.g., accuracy, precision, recall) are most relevant for the specific application can guide model selection.
- Common Machine Learning Algorithms: Â
- Linear Regression: Suitable for regression tasks with a linear relationship.
- Decision Trees: Useful for both classification and regression, providing interpretable results.
- Random Forest: An ensemble method that improves accuracy by combining multiple decision trees.
- Support Vector Machines (SVM): Effective for high-dimensional spaces and classification tasks.
- Neural Networks: Powerful for complex problems, especially in image and speech recognition.
- Model Evaluation Techniques: Â
- Cross-Validation: A technique to assess how the results of a statistical analysis will generalize to an independent dataset.
- Grid Search: A method for hyperparameter tuning to find the best parameters for a model.
- Learning Curves: Graphs that show the relationship between the size of the training set and the model's performance.
By carefully considering the factors involved and utilizing appropriate evaluation techniques, data scientists can enhance the effectiveness of their models. At Rapid Innovation, we guide our clients through the model selection process, ensuring that they choose the most effective algorithms tailored to their specific business challenges. This strategic approach not only optimizes performance but also maximizes return on investment, enabling our clients to achieve their business goals efficiently and effectively. Additionally, methodologies like the agile v model and cleanroom software engineering can further refine the model development process.
7.3.3. Training Protocols
Training protocols are essential for ensuring that machine learning models are developed effectively and efficiently. These protocols outline the procedures and methodologies used to train models, ensuring consistency and reproducibility.
- Data Preparation: Clean and preprocess data to remove noise and irrelevant information. Normalize or standardize data to ensure uniformity across features. Split data into training, validation, and test sets to evaluate model performance accurately.
- Model Selection: Choose appropriate algorithms based on the problem type (e.g., classification, regression). Consider factors such as model complexity, interpretability, and computational efficiency.
- Hyperparameter Tuning: Use techniques like grid search or random search to find optimal hyperparameters. Implement cross-validation to assess model performance on different subsets of data.
- Training Techniques: Utilize techniques such as batch training, online learning, or transfer learning based on the dataset size and model requirements. Monitor training progress using metrics like loss and accuracy to avoid overfitting.
- Documentation: Maintain detailed records of training processes, including configurations, datasets used, and results. Ensure that protocols are easily accessible for future reference or audits.
7.4. Testing and Validation
Testing and validation are critical steps in the machine learning lifecycle, ensuring that models perform well on unseen data. This phase helps in assessing the model's generalization capabilities and reliability.
- Validation Techniques: Use k-fold cross-validation to evaluate model performance across different data splits. Implement stratified sampling to maintain the distribution of classes in classification tasks.
- Performance Metrics: Choose appropriate metrics based on the problem type. For classification, use accuracy, precision, recall, and F1-score. For regression, use mean absolute error (MAE), mean squared error (MSE), and R-squared.
- Error Analysis: Analyze misclassifications or prediction errors to identify patterns and areas for improvement. Use confusion matrices to visualize performance and understand model weaknesses.
- Robustness Testing: Test models against adversarial examples or noisy data to evaluate robustness. Conduct stress testing to assess performance under extreme conditions or edge cases.
- Documentation of Results: Keep comprehensive records of testing outcomes, including metrics and visualizations. Share findings with stakeholders to inform decision-making and model adjustments.
7.5. Deployment Strategies
Deployment strategies are crucial for transitioning machine learning models from development to production. Effective deployment ensures that models deliver value in real-world applications.
- Deployment Environments: Choose between cloud-based, on-premises, or hybrid deployment based on organizational needs and infrastructure. Consider scalability, security, and compliance requirements when selecting the environment.
- Continuous Integration/Continuous Deployment (CI/CD): Implement CI/CD pipelines to automate the deployment process, ensuring rapid and reliable updates. Use version control systems to manage model versions and track changes over time.
- Monitoring and Maintenance: Set up monitoring systems to track model performance in real-time, identifying drift or degradation. Schedule regular maintenance checks to update models and retrain them as necessary.
- User Feedback Loop: Establish mechanisms for collecting user feedback to improve model performance and user experience. Use feedback to inform future iterations and enhancements of the model.
- Documentation and Training: Provide comprehensive documentation for end-users and stakeholders to facilitate understanding and usage. Conduct training sessions to ensure that users are equipped to leverage the model effectively.
By following these structured approaches in machine learning training protocols, testing and validation, and deployment strategies, organizations can enhance the effectiveness and reliability of their machine learning initiatives. At Rapid Innovation, we leverage these methodologies to help our clients achieve greater ROI by ensuring that their AI solutions are robust, scalable, and aligned with their business objectives. Our expertise in AI and Blockchain development allows us to provide tailored solutions that drive efficiency and effectiveness in achieving business goals. For more information on how to build an AI app, check out our step-by-step guide.
7.6. Monitoring and Maintenance
Monitoring and maintenance are critical components of any system, especially in the context of machine learning and data-driven applications. Continuous oversight ensures that models perform optimally and adapt to changing data patterns.
- Performance Tracking: Regularly assess the model's performance using metrics such as accuracy, precision, recall, and F1 score. This helps identify any degradation in performance over time.
- Data Drift Detection: Monitor for data drift, which occurs when the statistical properties of the input data change. Implement techniques to detect shifts in data distributions, as this can significantly impact model accuracy.
- Model Retraining: Establish a schedule for retraining models with new data. This is essential to maintain relevance and accuracy, especially in dynamic environments.
- Logging and Alerts: Set up logging mechanisms to capture model predictions and outcomes. Create alerts for significant deviations in performance metrics, enabling quick responses to potential issues.
- Resource Management: Regularly check system resources such as CPU, memory, and storage. Ensure that the infrastructure can handle the model's demands, especially during peak usage times.
- Version Control: Maintain version control for models and datasets. This allows for easy rollback to previous versions if a new model underperforms or introduces errors.
- User Feedback: Incorporate user feedback into the monitoring process. This qualitative data can provide insights into model performance that quantitative metrics may miss.
8. Performance Optimization

‍
Performance optimization is essential for enhancing the efficiency and effectiveness of machine learning models. It involves refining algorithms and processes to achieve better results with fewer resources.
- Algorithm Selection: Choose the right algorithm based on the problem type and data characteristics. Different algorithms have varying strengths and weaknesses, so selecting the most suitable one is crucial.
- Feature Engineering: Improve model performance by creating new features or modifying existing ones. This can involve techniques like normalization, encoding categorical variables, or generating interaction terms.
- Hyperparameter Tuning: Optimize hyperparameters to enhance model performance. Techniques such as grid search, random search, or Bayesian optimization can help identify the best parameter settings. Automated hyperparameter optimization can also streamline this process.
- Ensemble Methods: Use ensemble techniques like bagging, boosting, or stacking to combine multiple models. This often leads to improved accuracy and robustness.
- Model Compression: Implement model compression techniques to reduce the size of the model without sacrificing performance. This is particularly important for deploying models in resource-constrained environments.
- Parallel Processing: Leverage parallel processing to speed up training and inference times. Utilizing multiple cores or distributed computing can significantly enhance performance.
- Benchmarking: Regularly benchmark model performance against industry standards or competing models. This helps identify areas for improvement and ensures that the model remains competitive.
8.1. Model Tuning
Model tuning is a vital aspect of performance optimization, focusing on adjusting model parameters to achieve the best possible results. It involves a systematic approach to refining models based on performance metrics.
- Understanding Hyperparameters: Hyperparameters are settings that govern the training process, such as learning rate, batch size, and number of epochs. Understanding their impact is crucial for effective tuning, especially in machine learning hyperparameter optimization.
- Grid Search: This method involves exhaustively searching through a specified subset of hyperparameters. While thorough, it can be computationally expensive and time-consuming.
- Random Search: Unlike grid search, random search samples hyperparameters randomly. This can be more efficient and often yields better results in less time.
- Bayesian Optimization: This advanced technique uses probabilistic models to find the optimal hyperparameters. It is more efficient than grid and random search, especially for complex models, and is often used in hyperparameter optimization for machine learning models based on Bayesian optimization.
- Cross-Validation: Implement cross-validation to assess model performance on different subsets of data. This helps ensure that the model generalizes well to unseen data.
- Early Stopping: Monitor the model's performance during training and stop when performance begins to degrade. This prevents overfitting and saves computational resources.
- Regularization Techniques: Apply regularization methods like L1 (Lasso) and L2 (Ridge) to prevent overfitting. These techniques add a penalty for larger coefficients, promoting simpler models.
By focusing on monitoring, maintenance, performance optimization, and model tuning, organizations can ensure their machine learning models remain effective, efficient, and relevant in a rapidly changing data landscape. At Rapid Innovation, we leverage our expertise in AI and Blockchain to provide tailored solutions that enhance your business's operational efficiency and drive greater ROI. Our comprehensive approach ensures that your systems are not only optimized for performance but also resilient to the evolving demands of the market, including the best optimizer for convolutional neural networks and hyperparameter optimization in deep learning.
8.2. False Positive Reduction
False positives occur when a system incorrectly identifies a benign event as a threat. Reducing false positives is crucial for improving the efficiency of security systems and ensuring that resources are focused on genuine threats.
- Implement advanced algorithms: By leveraging machine learning and artificial intelligence, Rapid Innovation can help clients distinguish between legitimate and malicious activities with greater accuracy, thereby enhancing the overall security posture and contributing to false positive reduction.
- Regularly update threat intelligence: Keeping threat databases current allows systems to recognize new patterns and reduce the chances of false alarms. Rapid Innovation can assist in integrating real-time threat intelligence feeds to ensure that clients' systems are always up-to-date, further aiding in false positive reduction.
- Fine-tune detection parameters: Adjusting sensitivity settings based on the specific environment can help minimize false positives while maintaining detection efficacy. Our team can work with clients to customize these parameters for optimal performance, which is essential for effective false positive reduction.
- Conduct thorough testing: Regularly testing the system with various scenarios can help identify and rectify issues that lead to false positives. Rapid Innovation offers comprehensive testing services to ensure that security systems are robust and reliable, playing a key role in false positive reduction.
- User feedback: Incorporating feedback from users can provide insights into the accuracy of alerts and help refine detection mechanisms. We can implement user-friendly interfaces that facilitate feedback collection, enhancing the system's effectiveness and supporting false positive reduction. Additionally, our AI business automation solutions can further streamline these processes.
8.3. Real-time Processing Optimization
Real-time processing is essential for timely threat detection and response. Optimizing this process ensures that security systems can handle large volumes of data without delays.
- Utilize edge computing: Processing data closer to the source reduces latency and allows for quicker decision-making. Rapid Innovation can help clients implement edge computing solutions tailored to their specific needs.
- Implement efficient data pipelines: Streamlining data flow from collection to analysis can enhance processing speed and reduce bottlenecks. Our expertise in data architecture ensures that clients can achieve seamless data integration.
- Leverage parallel processing: Distributing tasks across multiple processors can significantly speed up data analysis and threat detection. Rapid Innovation can design systems that utilize parallel processing to maximize efficiency.
- Optimize algorithms: Using more efficient algorithms can reduce the computational load and improve processing times. Our team specializes in developing and optimizing algorithms that meet the unique requirements of each client.
- Monitor system performance: Regularly assessing system performance can help identify areas for improvement and ensure that real-time processing remains effective. We provide ongoing support and monitoring services to maintain optimal system performance.
8.4. Resource Utilization
Effective resource utilization is vital for maintaining the performance and cost-effectiveness of security systems. Proper management of resources ensures that systems can operate efficiently without unnecessary expenditure.
- Assess resource allocation: Regularly evaluate how resources are distributed across various tasks and adjust allocations based on current needs. Rapid Innovation can assist clients in conducting thorough resource assessments to optimize their operations.
- Automate routine tasks: Implementing automation for repetitive tasks can free up human resources for more complex issues, enhancing overall efficiency. Our automation solutions can streamline workflows and reduce operational costs.
- Use cloud resources: Leveraging cloud computing can provide scalable resources that adapt to changing demands without the need for significant upfront investment. Rapid Innovation offers cloud solutions that are flexible and cost-effective.
- Monitor resource usage: Keeping track of resource consumption can help identify inefficiencies and areas where optimization is possible. We provide analytics tools that enable clients to gain insights into their resource utilization.
- Implement load balancing: Distributing workloads evenly across servers can prevent any single resource from becoming a bottleneck, ensuring smoother operations. Our team can design load balancing strategies that enhance system reliability and performance.
8.5. Scalability Solutions
Scalability is a critical aspect of any business, particularly in the tech industry. It refers to the ability of a system, network, or process to handle a growing amount of work or its potential to accommodate growth. Here are some key scalability solutions that Rapid Innovation can implement to help clients achieve their business goals efficiently and effectively:
- Cloud Computing: Utilizing cloud services allows businesses to scale resources up or down based on demand. This flexibility helps manage costs while ensuring performance, enabling clients to respond swiftly to market changes.
- Microservices Architecture: Breaking down applications into smaller, independent services can enhance scalability. Each service can be developed, deployed, and scaled independently, improving overall system efficiency and allowing for faster innovation cycles.
- Load Balancing: Distributing workloads across multiple servers ensures no single server becomes a bottleneck. This enhances performance and reliability, especially during peak usage times, which is crucial for maintaining customer satisfaction.
- Database Sharding: This technique involves splitting a database into smaller, more manageable pieces, or shards. Each shard can be hosted on different servers, allowing for better performance and scalability, which is essential for data-intensive applications.
- Content Delivery Networks (CDNs): CDNs distribute content across various locations, reducing latency and improving load times for users. This is particularly beneficial for businesses with a global audience, ensuring a seamless user experience.
- Vertical and Horizontal Scaling: Vertical scaling involves adding more power (CPU, RAM) to an existing server, while horizontal scaling involves adding more servers to handle increased load. Both methods have their advantages depending on the business needs, and Rapid Innovation can help determine the best approach for each client.
- Performance Monitoring Tools: Implementing tools to monitor system performance can help identify bottlenecks and areas for improvement, ensuring that scalability solutions are effective and aligned with business objectives.
- Automated Scaling: Using automation tools to adjust resources based on real-time demand can optimize performance and cost-efficiency, allowing businesses to focus on growth without worrying about infrastructure limitations.
- API Management: Efficient API management can facilitate scalability by allowing different applications to communicate seamlessly, enabling businesses to expand their services without significant rework. This is particularly important in an increasingly interconnected digital landscape.
In the context of blockchain technology, scalability solutions are particularly relevant. The scalability of blockchain is a major concern, and various blockchain scalability solutions are being developed to address these challenges. For instance, the lightning network scalability is a promising approach to enhance transaction speeds and reduce costs in crypto transactions. Additionally, the scalability of cryptocurrency is a critical factor for its adoption, with many projects focusing on scalability in blockchain challenges and solutions.
Moreover, concepts like plasma scalable autonomous smart contracts and polygon scalability are gaining traction as innovative solutions to improve the scalability of blockchain networks. As businesses explore scalability in crypto, understanding the most scalable blockchain and most scalable cryptocurrency options will be essential for making informed decisions.
By implementing these scalability solutions, Rapid Innovation can help clients navigate the complexities of scaling in crypto and blockchain technology effectively, including scaling the future: empowering blockchain scalability and zk-rollup development.
9.2. Data Privacy Requirements
Data privacy requirements are essential for protecting individuals' personal information in an increasingly digital world. Organizations must adhere to various regulations and best practices to ensure that data is collected, stored, and processed responsibly.
- Compliance with regulations: Organizations must comply with laws such as the General Data Protection Regulation (GDPR) and the California Consumer Privacy Act (CCPA). These regulations set strict guidelines on how personal data should be handled, including gdpr compliance and ccpa compliance. Rapid Innovation can assist clients in navigating these complexities to ensure compliance, thereby minimizing legal risks and enhancing their reputation.
- Data minimization: Collect only the data necessary for a specific purpose. This reduces the risk of data breaches and ensures that individuals' privacy is respected. Rapid Innovation employs advanced AI techniques to help organizations identify and limit data collection to what is essential, optimizing their data management processes in line with gdpr requirements.
- User consent: Obtain explicit consent from users before collecting their data. This empowers individuals to control their information and understand how it will be used. Rapid Innovation can implement blockchain solutions that provide transparent consent management, ensuring that users' rights are upheld while enhancing trust, in accordance with gdpr compliance regulations.
- Secure data storage: Implement robust security measures to protect data from unauthorized access. This includes encryption, access controls, and regular security audits. Rapid Innovation leverages cutting-edge blockchain technology to create immutable records of data access and modifications, significantly enhancing data security and supporting gdpr data protection requirements.
- Transparency in data usage: Clearly communicate to users how their data will be used, shared, and stored. This builds trust and fosters a positive relationship between organizations and their customers. Rapid Innovation can help clients develop user-friendly interfaces and communication strategies that promote transparency, ultimately leading to improved customer loyalty and satisfaction, while ensuring data privacy compliance.
9.3. Model Transparency
Model transparency refers to the clarity and openness with which organizations communicate the workings of their algorithms and models. This is crucial for building trust and ensuring accountability in AI and machine learning applications.
- Explainability: Organizations should strive to make their models interpretable. This means providing insights into how decisions are made, which can help users understand the rationale behind outcomes. Rapid Innovation specializes in developing explainable AI models that enhance user understanding and trust, aligning with data privacy and compliance standards.
- Documentation: Maintain comprehensive documentation of model development processes, including data sources, algorithms used, and performance metrics. This aids in accountability and facilitates audits. Rapid Innovation emphasizes thorough documentation practices, ensuring that clients can easily demonstrate compliance and model integrity, including adherence to gdpr and compliance requirements.
- Stakeholder engagement: Involve stakeholders in discussions about model design and implementation. This can include users, regulatory bodies, and ethicists, ensuring diverse perspectives are considered. Rapid Innovation facilitates stakeholder workshops to gather insights and foster collaboration, leading to more robust and accepted AI solutions.
- Regular updates: Continuously monitor and update models to reflect changes in data and societal norms. This helps maintain relevance and accuracy in decision-making. Rapid Innovation employs agile methodologies to ensure that AI models are regularly refined and improved based on real-time feedback.
- Ethical implications: Consider the ethical implications of model decisions. Organizations should assess potential biases and their impact on different demographic groups. Rapid Innovation integrates ethical assessments into the model development lifecycle, helping clients mitigate risks associated with bias and discrimination.
9.4. Ethical Considerations
Ethical considerations are paramount in the development and deployment of technology, particularly in AI and data-driven applications. Organizations must navigate complex moral landscapes to ensure their practices align with societal values.
- Fairness: Strive for fairness in algorithms to prevent discrimination against any group. This involves identifying and mitigating biases in data and model outputs. Rapid Innovation employs advanced techniques to audit and enhance fairness in AI systems, ensuring equitable outcomes.
- Accountability: Establish clear lines of accountability for decisions made by AI systems. Organizations should be prepared to explain and justify their actions to stakeholders. Rapid Innovation helps clients create governance frameworks that define accountability structures for AI decision-making.
- Privacy: Respect individuals' privacy rights by implementing data protection measures and ensuring that personal information is not misused. Rapid Innovation's expertise in blockchain technology provides clients with innovative solutions for data privacy and protection, including personal information compliance.
- Social impact: Assess the broader social implications of technology deployment. Organizations should consider how their products affect communities and contribute to societal well-being. Rapid Innovation encourages clients to evaluate the social impact of their technologies, fostering responsible innovation, particularly in the context of data privacy compliance.
- Continuous ethical review: Regularly evaluate ethical practices and policies. This can involve creating ethics boards or committees to oversee technology development and deployment. Rapid Innovation supports clients in establishing ongoing ethical review processes, ensuring that their practices remain aligned with evolving societal values, including adherence to gdpr compliance and data privacy compliance.
9.5. Auditing and Documentation
Auditing and documentation are critical components of any effective management system. They ensure compliance, enhance accountability, and provide a clear record of processes and decisions. Auditing involves a systematic examination of records, processes, and systems to ensure they meet established standards and regulations. Documentation refers to the creation and maintenance of records that detail procedures, policies, and outcomes. Both practices help organizations identify areas for improvement and ensure that they are operating efficiently and effectively.
Key aspects of auditing include:
- Internal Audits: Conducted by employees within the organization to assess compliance with internal policies and external regulations, often guided by an internal audit checklist.
- External Audits: Performed by independent third parties to provide an unbiased evaluation of the organization’s practices, including a review of audit workpapers.
- Compliance Audits: Focus on adherence to laws, regulations, and guidelines relevant to the industry, which may involve substantive audit procedures.
Documentation should encompass:
- Standard Operating Procedures (SOPs): Detailed instructions on how to perform specific tasks, which may be outlined in an audit plan.
- Records of Audits: Documentation of findings, corrective actions, and follow-up activities, including audit papers and audit documentation.
- Policy Manuals: Comprehensive guides that outline the organization’s policies and procedures, including management letters of representation.
Effective auditing and documentation practices lead to:
- Improved transparency and accountability.
- Enhanced decision-making based on accurate data.
- Better risk management by identifying potential issues early, as highlighted in the report on internal control over financial reporting.
10. Risk Management

‍
Risk management is the process of identifying, assessing, and mitigating risks that could negatively impact an organization. It is essential for ensuring business continuity and protecting assets. A robust risk management framework helps organizations anticipate potential threats and develop strategies to address them. It involves a proactive approach to identifying risks before they materialize, allowing for timely interventions.
Key components of risk management include:
- Risk Identification: Recognizing potential risks that could affect the organization, including operational, financial, and reputational risks, which can be assessed using a financial audit checklist.
- Risk Assessment: Evaluating the likelihood and impact of identified risks to prioritize them effectively, often informed by an internal audit report.
- Risk Mitigation: Developing strategies to minimize the impact of risks, which may include implementing controls, transferring risk, or accepting it, as outlined in audit guidelines.
Benefits of effective risk management:
- Protects organizational assets and resources.
- Enhances decision-making by providing a clear understanding of potential risks.
- Improves stakeholder confidence and trust.
10.1. System Risk Assessment
System risk assessment is a critical process within the broader risk management framework. It involves evaluating the risks associated with specific systems, processes, or technologies. This assessment helps organizations understand vulnerabilities and potential threats to their systems and is essential for ensuring the integrity, confidentiality, and availability of information.
Key steps in conducting a system risk assessment include:
- Defining the Scope: Clearly outline the systems and processes to be assessed, including their boundaries and interfaces.
- Identifying Assets: Catalog the assets within the system, including hardware, software, data, and personnel.
- Identifying Threats and Vulnerabilities: Analyze potential threats (e.g., cyberattacks, natural disasters) and vulnerabilities (e.g., outdated software, lack of training).
- Assessing Risks: Evaluate the likelihood and impact of identified threats exploiting vulnerabilities, often using qualitative or quantitative methods, which may be documented in audit documentation.
- Developing Mitigation Strategies: Create a plan to address identified risks, which may include implementing security measures, conducting training, or developing contingency plans, as suggested in an audit plan example.
Benefits of system risk assessment:
- Provides a comprehensive understanding of risks associated with specific systems.
- Helps prioritize resources and efforts to address the most critical risks.
- Supports compliance with regulatory requirements and industry standards, including attestation in auditing.
In conclusion, effective auditing, documentation, and risk management practices are essential for organizations to operate efficiently and securely. By implementing these processes, organizations can enhance their resilience and ensure long-term success. At Rapid Innovation, we leverage our expertise in AI and Blockchain to streamline these processes, ensuring that our clients not only meet compliance standards but also achieve greater ROI through enhanced operational efficiency and risk mitigation strategies. Additionally, how generative AI enhances internal audit processes can further improve these practices.
10.2. Fraud Risk Scoring
Fraud risk scoring is a critical component in the fight against financial fraud. It involves assessing the likelihood of fraudulent activity based on various risk factors. Organizations utilize fraud risk scoring to prioritize their resources and focus on high-risk transactions or accounts.
- Risk factors can include: Â
- Transaction history
- Customer behavior patterns
- Geographic location
- Account age and activity level
- Scoring models often employ: Â
- Machine learning algorithms
- Historical data analysis
- Predictive analytics
- Benefits of fraud risk scoring: Â
- Enhances detection of potential fraud
- Reduces false positives in alerts
- Improves operational efficiency by focusing on high-risk areas
At Rapid Innovation, we leverage advanced AI techniques to develop tailored fraud risk scoring models that integrate seamlessly into your existing systems. By utilizing machine learning algorithms and predictive analytics, we help organizations achieve greater ROI through enhanced fraud detection capabilities, ultimately safeguarding customer trust and reducing financial losses. Our fraud scores and fraud risk scoring methodologies are designed to provide a comprehensive view of potential threats, including specific assessments like phone fraud risk scores. For more insights on personalized risk evaluation in insurance with AI agents.
10.3. Alert Management
Alert management is the process of handling alerts generated by fraud detection systems. Effective alert management is essential for minimizing the impact of fraud while ensuring that legitimate transactions are not hindered.
- Key components of alert management include: Â
- Triage: Prioritizing alerts based on risk levels and potential impact.
- Investigation: Assessing alerts to determine if they are genuine threats or false positives.
- Resolution: Taking appropriate action, which may involve blocking transactions, contacting customers, or escalating to law enforcement.
- Best practices for alert management: Â
- Establish clear criteria for alert prioritization.
- Utilize automated systems to streamline the triage process.
- Train staff on effective investigation techniques to enhance response times.
- Challenges in alert management: Â
- High volume of alerts can lead to alert fatigue.
- Balancing security with customer experience is crucial.
- Continuous improvement of alert systems is necessary to adapt to evolving fraud tactics.
By implementing a robust alert management system, organizations can respond swiftly to potential fraud, thereby minimizing losses and maintaining customer confidence. Rapid Innovation can assist in developing automated alert management solutions that enhance operational efficiency and improve response times.
10.4. Investigation Protocols
Investigation protocols are essential for systematically addressing suspected fraud cases. These protocols outline the steps that should be taken when an alert is triggered, ensuring a thorough and consistent approach to fraud investigation.
- Key elements of investigation protocols include: Â
- Initial assessment: Quickly evaluating the alert to determine its validity.
- Data collection: Gathering relevant information, such as transaction details, customer history, and communication logs.
- Analysis: Reviewing the collected data to identify patterns or anomalies that may indicate fraud.
- Steps in the investigation process: Â
- Document findings: Keeping detailed records of the investigation process and outcomes.
- Collaborate with stakeholders: Engaging with relevant departments, such as legal and compliance, to ensure a comprehensive approach.
- Report findings: Communicating results to management and, if necessary, law enforcement.
- Importance of investigation protocols: Â
- Ensures consistency in handling fraud cases.
- Reduces the risk of overlooking critical evidence.
- Enhances the organization’s ability to learn from past incidents and improve future fraud prevention strategies.
By establishing clear investigation protocols, organizations can effectively manage fraud cases, leading to better outcomes and a stronger defense against future threats. Rapid Innovation's expertise in AI and blockchain technology can further enhance these protocols, ensuring a more secure and efficient fraud investigation process.
10.5. Incident Response
Incident response is a critical component of cybersecurity, focusing on the processes and procedures that organizations implement to detect, respond to, and recover from security incidents. A well-defined incident response plan, such as a security incident response plan or an incident response playbook, can significantly reduce the impact of a security breach and help organizations maintain their reputation and trust with customers.
- Preparation: This involves establishing and training an incident response team, developing policies, and ensuring that necessary tools and resources are in place. Regular training and simulations can help prepare the team for real incidents. Organizations may refer to a sample incident response plan or an example of an incident response plan to guide their preparations.
- Detection and Analysis: Organizations must have systems in place to detect potential security incidents. This can include intrusion detection systems, security information and event management (SIEM) tools, and user behavior analytics. Once an incident is detected, it is crucial to analyze the nature and scope of the incident to understand its impact. Utilizing a computer security incident response plan can aid in this analysis.
- Containment: After identifying an incident, the next step is to contain it to prevent further damage. This may involve isolating affected systems, blocking malicious traffic, or implementing temporary fixes. The incident response procedures should outline specific containment strategies.
- Eradication: Once the incident is contained, organizations must remove the root cause of the incident. This could involve deleting malware, closing vulnerabilities, or applying patches to affected systems. A data breach response plan may provide additional guidance on eradication steps.
- Recovery: After eradication, systems must be restored to normal operations. This includes restoring data from backups, ensuring systems are secure, and monitoring for any signs of residual threats. An IT security incident response plan can help streamline the recovery process.
- Post-Incident Review: After the incident is resolved, conducting a post-incident review is essential. This helps identify what went well, what could be improved, and how to prevent similar incidents in the future. Documentation of the incident and response actions taken is crucial for learning and compliance. Organizations may refer to the NIST incident response plan for best practices in this review.
- Continuous Improvement: Incident response is not a one-time effort. Organizations should continuously update their incident response plans based on lessons learned from past incidents and evolving threats. This includes revising the security incident response policy and incident management procedures.
Effective incident response can minimize downtime, reduce financial losses, and enhance an organization’s overall security posture.
11. Advanced Features and Capabilities
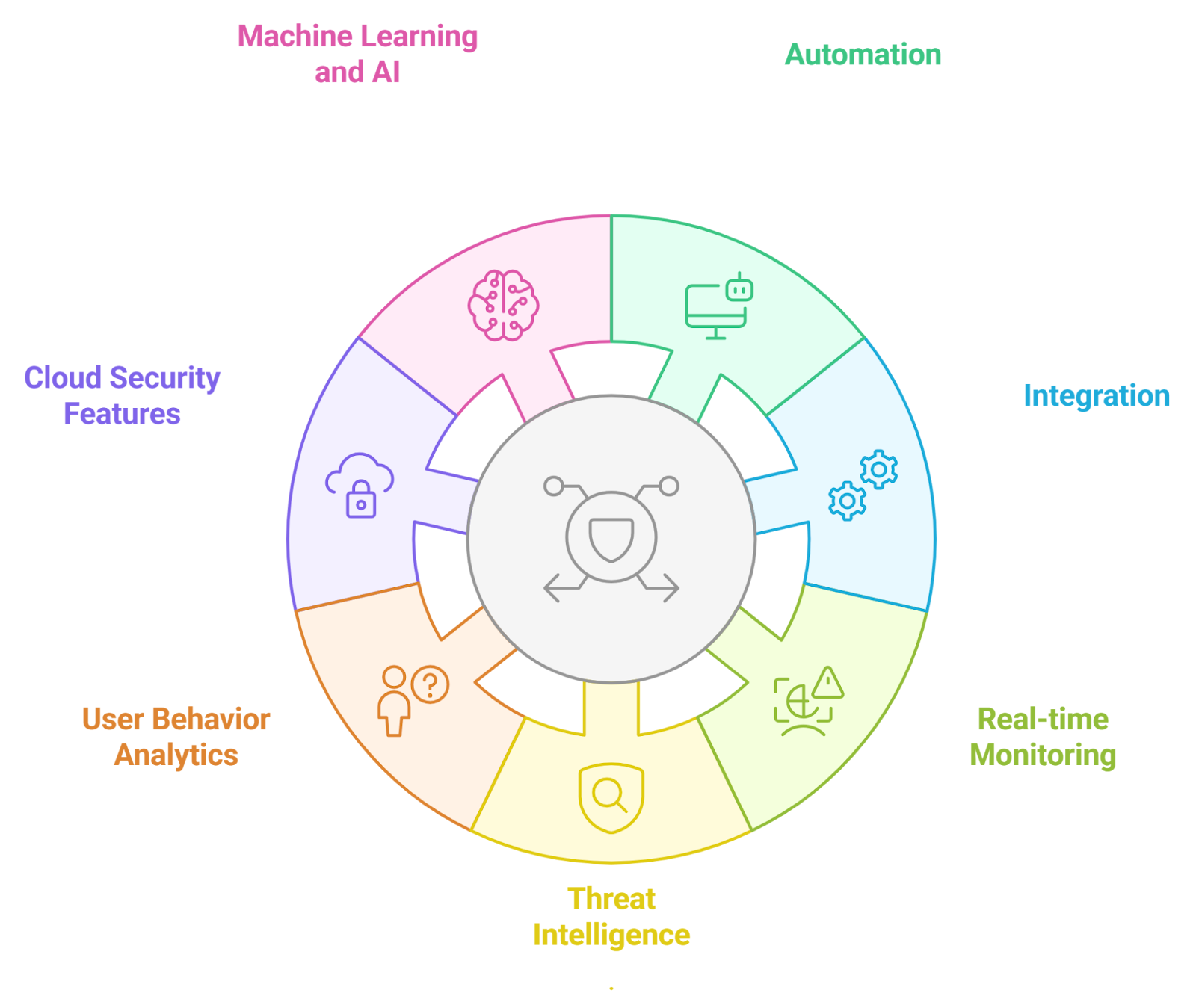
‍
Advanced features and capabilities in cybersecurity tools and systems are essential for organizations to stay ahead of evolving threats. These features enhance the effectiveness of security measures and provide organizations with the tools they need to protect their assets.
- Automation: Automating routine security tasks can significantly reduce the workload on security teams. This includes automated threat detection, incident response, and reporting, allowing teams to focus on more complex issues.
- Integration: Advanced security solutions often integrate with existing systems and tools, providing a unified view of security across the organization. This integration helps streamline processes and improve response times.
- Real-time Monitoring: Continuous monitoring of networks and systems allows organizations to detect threats as they occur. Real-time alerts enable swift action to mitigate potential damage.
- Threat Intelligence: Incorporating threat intelligence into security systems helps organizations stay informed about the latest threats and vulnerabilities. This proactive approach allows for better preparedness and response.
- User Behavior Analytics: Monitoring user behavior can help identify anomalies that may indicate a security breach. By analyzing patterns, organizations can detect insider threats and compromised accounts more effectively.
- Cloud Security Features: As more organizations move to the cloud, advanced security features tailored for cloud environments are essential. This includes data encryption, access controls, and compliance monitoring.
- Machine Learning and AI: Leveraging machine learning and artificial intelligence can enhance threat detection and response capabilities. These technologies can analyze vast amounts of data to identify patterns and predict potential threats.
11.1. Adaptive Learning Systems
Adaptive learning systems in cybersecurity are designed to evolve and improve over time based on new data and experiences. These systems utilize advanced algorithms and machine learning techniques to enhance their effectiveness in detecting and responding to threats.
- Dynamic Threat Detection: Adaptive learning systems can adjust their detection methods based on emerging threats. By continuously learning from new data, these systems can identify previously unknown threats more effectively.
- Personalized Security Measures: These systems can tailor security measures to individual users or devices based on their behavior and risk profiles. This personalized approach enhances security while minimizing disruptions to legitimate users.
- Feedback Loops: Adaptive learning systems incorporate feedback loops that allow them to learn from past incidents. This continuous improvement process helps refine detection algorithms and response strategies.
- Scalability: As organizations grow, adaptive learning systems can scale to accommodate increased data and user activity. This scalability ensures that security measures remain effective even in larger environments.
- Reduced False Positives: By learning from historical data, adaptive systems can reduce the number of false positives in threat detection. This leads to more accurate alerts and less time wasted on investigating benign activities.
- Integration with Other Technologies: Adaptive learning systems can work alongside other security technologies, such as SIEM and endpoint protection, to provide a comprehensive security solution.
- Proactive Defense: By anticipating potential threats and adjusting defenses accordingly, adaptive learning systems enable organizations to take a proactive stance in their cybersecurity efforts.
Incorporating adaptive learning systems into an organization’s cybersecurity strategy can significantly enhance its ability to respond to threats and protect sensitive data. At Rapid Innovation, we leverage our expertise in AI and blockchain technologies to develop tailored incident response solutions that not only enhance security but also drive greater ROI for our clients. By integrating advanced analytics and automated processes, we empower organizations to respond swiftly and effectively to incidents, ensuring business continuity and safeguarding their digital assets.
11.2. Collaborative Detection Networks
Collaborative Detection Networks (CDNs) represent a cutting-edge strategy for identifying and mitigating fraud across diverse platforms and organizations. By harnessing shared data and insights, CDNs significantly enhance the capability to detect fraudulent activities that may otherwise remain undetected in isolated systems.
- Enhanced data sharing: Organizations can share anonymized data to improve detection rates without compromising sensitive information, fostering a collaborative environment that strengthens overall security.
- Collective intelligence: By pooling resources and knowledge, organizations can develop more robust algorithms that adapt to emerging fraud patterns, leading to more effective fraud prevention strategies, including the use of fraud detection AI.
- Real-time monitoring: CDNs facilitate continuous monitoring of transactions, enabling immediate action against suspicious activities, which is crucial for minimizing potential losses. This is particularly relevant in the context of AI in fraud detection.
- Cross-industry collaboration: Different sectors can work together to identify fraud trends that may impact multiple industries, resulting in a more comprehensive understanding of fraud tactics and enhancing overall resilience.
- Improved accuracy: Collaborative efforts can reduce false positives, ensuring that legitimate transactions are not flagged unnecessarily, thus maintaining customer trust and satisfaction, which is essential in artificial intelligence fraud detection in banking.
11.3. Cross-channel Fraud Detection
Cross-channel Fraud Detection refers to the capability to monitor and analyze transactions across multiple channels, such as online, mobile, and in-store. This holistic approach is essential for identifying fraudulent activities that may span different platforms.
- Unified view of transactions: By integrating data from various channels, organizations can gain a complete picture of customer behavior and transaction patterns, allowing for more informed decision-making.
- Detection of sophisticated fraud schemes: Fraudsters often exploit multiple channels to execute their schemes. Cross-channel detection helps identify these complex tactics, enabling organizations to respond proactively, particularly through AI-based fraud detection in banking.
- Enhanced customer experience: By reducing false positives and improving detection accuracy, organizations can provide a smoother experience for legitimate customers, which is vital for maintaining loyalty.
- Real-time alerts: Cross-channel systems can trigger alerts when suspicious activities are detected, allowing for immediate investigation and response, thereby minimizing potential damage.
- Data analytics: Advanced analytics tools can identify anomalies across channels, helping organizations stay ahead of emerging fraud trends and adapt their strategies accordingly, including the use of fraud detection technology.
11.4. Predictive Analytics
Predictive Analytics involves utilizing historical data, statistical algorithms, and machine learning techniques to forecast future outcomes. In the context of fraud detection, predictive analytics can significantly enhance an organization's ability to identify potential fraud before it occurs.
- Data-driven decision-making: Organizations can leverage historical data to make informed decisions about risk management and fraud prevention strategies, leading to more effective resource allocation.
- Identification of risk patterns: Predictive models can uncover patterns and trends that indicate potential fraud, allowing organizations to proactively address vulnerabilities and strengthen their defenses, particularly in fraud detection in banking using machine learning.
- Improved resource allocation: By predicting where fraud is likely to occur, organizations can allocate resources more effectively to high-risk areas, maximizing their return on investment.
- Continuous learning: Machine learning algorithms can adapt and improve over time, enhancing the accuracy of predictions as new data becomes available, ensuring that organizations remain agile in their fraud prevention efforts.
- Cost savings: By preventing fraud before it occurs, organizations can save significant amounts of money that would otherwise be lost to fraudulent activities, ultimately contributing to a healthier bottom line.
At Rapid Innovation, we specialize in implementing these advanced technologies to help our clients achieve their business goals efficiently and effectively. By integrating AI and Blockchain solutions, we empower organizations to enhance their fraud detection capabilities, leading to greater ROI and a more secure operational environment, particularly through the use of fraud detection artificial intelligence.
11.5. Blockchain Integration
Blockchain technology is revolutionizing various industries by providing a decentralized and secure method for recording transactions. In the context of blockchain case management and reporting, blockchain integration can enhance data integrity, transparency, and efficiency.
- Data Security: Blockchain's cryptographic features ensure that data is secure and tamper-proof, which is crucial for sensitive information in blockchain case management systems.
- Transparency: All transactions recorded on a blockchain are visible to authorized users, promoting accountability and trust among stakeholders.
- Decentralization: By eliminating the need for a central authority, blockchain reduces the risk of data breaches and fraud.
- Smart Contracts: These self-executing contracts can automate processes within blockchain case management, reducing the time and effort required for manual interventions.
- Interoperability: Blockchain can facilitate seamless data sharing between different systems, improving collaboration among various entities involved in blockchain case management.
The integration of blockchain technology can lead to more efficient blockchain case management processes, ultimately improving outcomes for organizations and their clients. At Rapid Innovation, we leverage blockchain solutions to help clients streamline their operations, enhance security, and achieve greater ROI through improved data management and process automation. Additionally, we explore generative AI applications that can further enhance financial reporting and case management efficiency.
12. Case Management and Reporting
Case management and reporting are essential components of many organizations, particularly in sectors like healthcare, legal, and social services. Effective case management ensures that cases are handled efficiently, while reporting provides insights into performance and outcomes.
- Centralized Information: A robust case management system centralizes all relevant information, making it easily accessible to authorized personnel.
- Workflow Automation: Automating routine tasks can significantly reduce the administrative burden on staff, allowing them to focus on more critical aspects of case management.
- Performance Metrics: Reporting tools can track key performance indicators (KPIs), helping organizations assess their effectiveness and identify areas for improvement.
- Compliance and Auditing: Comprehensive reporting ensures that organizations meet regulatory requirements and can provide documentation for audits.
- Data Analysis: Advanced analytics can uncover trends and patterns in case data, enabling organizations to make informed decisions and improve service delivery.
By implementing effective case management and reporting systems, organizations can enhance their operational efficiency and improve client outcomes. Rapid Innovation specializes in developing tailored solutions that integrate AI and blockchain technologies, ensuring that our clients can maximize their performance and achieve their business goals.
12.1. Alert Triage
Alert triage is a critical process in case management, particularly in environments where timely responses are essential, such as healthcare and emergency services. It involves assessing alerts to determine their urgency and the appropriate response.
- Prioritization: Triage helps prioritize alerts based on severity, ensuring that the most critical cases receive immediate attention.
- Resource Allocation: By categorizing alerts, organizations can allocate resources more effectively, optimizing staff deployment and response times.
- Risk Management: Effective triage can mitigate risks by ensuring that potential issues are addressed before they escalate into more significant problems.
- Improved Communication: A structured triage process enhances communication among team members, ensuring everyone is aware of the status and urgency of cases.
- Feedback Loop: Continuous evaluation of the triage process can lead to improvements, as organizations learn from past experiences and adjust their protocols accordingly.
Implementing a robust alert triage system can significantly enhance the efficiency and effectiveness of case management, ultimately leading to better outcomes for clients and organizations alike. Rapid Innovation is committed to providing innovative solutions that empower organizations to optimize their alert triage processes and improve overall service delivery.
12.2. Investigation Workflow
An effective investigation workflow is crucial for ensuring that all aspects of an investigation are handled systematically and efficiently. This workflow typically includes several key stages:
- Preparation: Before initiating an investigation, it is essential to gather all necessary resources and information. This may involve assembling a team, defining roles, and establishing objectives.
- Data Collection: Collecting relevant data is a critical step. This can include interviews, document reviews, and physical evidence gathering. Ensuring that data is collected in a manner that maintains its integrity is vital.
- Analysis: Once data is collected, it must be analyzed to identify patterns, discrepancies, or evidence that supports or refutes claims. This stage often involves using analytical tools and methodologies, including AI-driven analytics that can enhance the speed and accuracy of data interpretation.
- Conclusion: After analysis, investigators draw conclusions based on the evidence. This step requires careful consideration to ensure that conclusions are logical and supported by the data.
- Review: A review process is essential to validate findings and ensure that all aspects of the investigation have been considered. Peer reviews or supervisory checks can enhance the credibility of the investigation.
- Follow-Up: After the investigation is concluded, follow-up actions may be necessary. This could involve implementing recommendations, monitoring compliance, or addressing any identified issues.
A well-defined investigation workflow not only enhances the quality of the investigation but also ensures that it adheres to legal and ethical standards.
12.3. Documentation Standards

‍
Documentation standards are critical in any investigation as they ensure that all findings, processes, and evidence are recorded accurately and consistently. Adhering to these standards can significantly impact the integrity and credibility of the investigation. Key aspects include:
- Clarity: Documentation should be clear and concise. Avoid jargon and ensure that all terms are defined. This helps in making the documentation accessible to all stakeholders.
- Consistency: Use a consistent format for all documents. This includes headings, fonts, and citation styles. Consistency aids in understanding and navigating the documentation.
- Completeness: Ensure that all relevant information is documented. This includes the context of the investigation, methodologies used, and all findings. Incomplete documentation can lead to misunderstandings or misinterpretations.
- Timeliness: Document findings and processes as they occur. Delayed documentation can result in lost details and may affect the accuracy of the information.
- Security: Protect sensitive information through secure storage and access controls. This is particularly important in investigations involving confidential or proprietary information, where blockchain technology can provide an immutable record of all documentation.
- Retention: Establish clear guidelines for how long documentation should be retained. This is often dictated by legal requirements or organizational policies.
By adhering to these documentation standards, organizations can ensure that their investigations are transparent, reliable, and defensible.
12.4. Reporting Systems
Reporting systems play a vital role in the communication of investigation findings. A well-structured reporting system ensures that information is conveyed effectively to all relevant parties. Key components include:
- Format: Reports should follow a standardized format that includes an executive summary, methodology, findings, conclusions, and recommendations. This structure helps readers quickly grasp the essential information.
- Audience: Tailor reports to the intended audience. Different stakeholders may require different levels of detail or focus on specific aspects of the investigation.
- Clarity and Precision: Use clear language and avoid ambiguity. Reports should be precise, with findings supported by evidence. This enhances the credibility of the report.
- Visual Aids: Incorporate charts, graphs, and tables where appropriate. Visual aids can help convey complex information more effectively and make reports more engaging.
- Timeliness: Reports should be generated and distributed promptly after the investigation concludes. Timely reporting ensures that findings are relevant and actionable.
- Feedback Mechanism: Establish a system for receiving feedback on reports. This can help improve future reporting processes and ensure that the needs of stakeholders are met.
By implementing effective reporting systems, organizations can enhance communication, facilitate decision-making, and promote accountability in the investigation process. Rapid Innovation leverages AI and blockchain technologies to streamline these investigation workflows, ensuring greater efficiency and a higher return on investment for our clients.
12.5. Performance Metrics
Performance metrics are essential tools for evaluating the effectiveness and efficiency of various processes, projects, or strategies within an organization. They provide quantifiable measures that help in assessing progress towards goals and objectives.
- Key performance indicators (KPIs) are specific metrics that align with strategic goals. They can include: Â
- Revenue growth
- Customer satisfaction scores
- Employee productivity rates
- Performance metrics can be categorized into different types: Â
- Financial Metrics: These include profit margins, return on investment (ROI), and cost per acquisition (CPA). They help organizations understand their financial health and operational efficiency.
- Operational Metrics: Metrics such as cycle time, throughput, and defect rates fall under this category. They provide insights into the efficiency of processes and workflows.
- Customer Metrics: These metrics focus on customer engagement and satisfaction, including Net Promoter Score (NPS) and customer retention rates.
- The importance of performance metrics includes: Â
- Informed Decision-Making: Metrics provide data-driven insights that help leaders make informed decisions. For instance, Rapid Innovation utilizes AI-driven analytics to offer clients real-time insights into their performance metrics, enabling them to pivot strategies effectively.
- Accountability: They establish clear expectations and accountability among team members. By implementing blockchain technology, organizations can ensure transparency in performance tracking, fostering a culture of accountability.
- Continuous Improvement: Regularly tracking performance metrics allows organizations to identify areas for improvement and implement necessary changes. Rapid Innovation assists clients in developing tailored dashboards that visualize performance metrics, facilitating ongoing enhancements.
- To effectively implement performance metrics, organizations should: Â
- Define clear objectives and align metrics accordingly, including key performance index (KPI) definitions.
- Use a balanced scorecard approach to ensure a comprehensive view of performance, incorporating key performance measures examples.
- Regularly review and adjust metrics to reflect changing business environments, including marketing KPIs and sales KPIs.
13. Future Trends and Innovation
The landscape of business and technology is constantly evolving, leading to new trends and innovations that can significantly impact organizations. Understanding these trends is crucial for staying competitive and relevant in the market.
- Key future trends include: Â
- Digital Transformation: Organizations are increasingly adopting digital technologies to enhance operations, improve customer experiences, and drive innovation.
- Sustainability: There is a growing emphasis on sustainable practices, with companies focusing on reducing their carbon footprint and promoting eco-friendly initiatives.
- Remote Work: The shift towards remote work is likely to continue, leading to changes in workplace dynamics and employee engagement strategies.
- Innovation plays a critical role in adapting to these trends: Â
- Companies are investing in research and development to create new products and services.
- Collaboration with startups and tech firms is becoming more common to leverage innovative solutions.
- Organizations are also focusing on fostering a culture of innovation among employees to encourage creative thinking.
13.1. Emerging Technologies
Emerging technologies are reshaping industries and creating new opportunities for businesses. Staying informed about these technologies is essential for organizations looking to innovate and maintain a competitive edge.
- Some notable emerging technologies include: Â
- Artificial Intelligence (AI): AI is transforming various sectors by automating processes, enhancing decision-making, and improving customer interactions. Rapid Innovation helps clients integrate AI solutions that optimize operations and drive significant ROI.
- Blockchain: This technology is revolutionizing data security and transparency, particularly in finance, supply chain management, and healthcare. By leveraging blockchain, Rapid Innovation enables clients to enhance trust and traceability in their transactions.
- Internet of Things (IoT): IoT devices are enabling real-time data collection and analysis, leading to improved operational efficiency and customer insights.
- The impact of these technologies can be seen in several areas: Â
- Enhanced Efficiency: Automation and data analytics streamline operations, reducing costs and increasing productivity.
- Improved Customer Experience: Personalized services and products driven by AI and data analytics enhance customer satisfaction.
- New Business Models: Emerging technologies are enabling innovative business models, such as subscription services and on-demand platforms.
- Organizations should consider the following strategies to leverage emerging technologies: Â
- Invest in training and development to equip employees with the necessary skills.
- Collaborate with technology providers to stay updated on the latest advancements.
- Experiment with pilot projects to test the feasibility of new technologies before full-scale implementation. Rapid Innovation can guide organizations through this process, ensuring they harness the full potential of these technologies for greater ROI.
13.2. Industry Evolution
The evolution of any industry is marked by significant changes in technology, consumer behavior, and market dynamics. In recent years, several key trends have shaped the landscape of various sectors:
- Technological Advancements: Rapid advancements in technology have transformed industries. Automation, artificial intelligence, and machine learning are now integral to operations, enhancing efficiency and productivity. At Rapid Innovation, we harness these technologies to develop tailored solutions that streamline processes and drive operational excellence for our clients.
- Consumer Expectations: Today's consumers demand personalized experiences and instant gratification. Businesses are adapting by leveraging data analytics to understand consumer preferences better. Our expertise in AI allows us to implement advanced analytics solutions that provide actionable insights, enabling businesses to meet and exceed customer expectations.
- Sustainability Focus: There is a growing emphasis on sustainability across industries. Companies are adopting eco-friendly practices and products to meet consumer demand for responsible business practices. Rapid Innovation supports clients in integrating sustainable technologies, including blockchain for transparent supply chains, ensuring they align with modern consumer values. For more on this topic, check out our article on leveraging generative AI for sustainable innovation in rapid prototyping and product development.
- Globalization: The interconnectedness of markets has led to increased competition and collaboration. Businesses are now operating on a global scale, requiring them to adapt to diverse cultural and regulatory environments. Our consulting services help clients navigate these complexities, ensuring compliance and strategic positioning in international markets.
- Regulatory Changes: Industries are also evolving due to changes in regulations. Compliance with new laws and standards is essential for businesses to maintain their market position. Rapid Innovation provides guidance on regulatory compliance, particularly in the realms of AI and blockchain, helping clients mitigate risks and enhance their operational frameworks.
These factors collectively contribute to the ongoing evolution of industries, necessitating continuous adaptation and innovation.
13.3. Research Directions
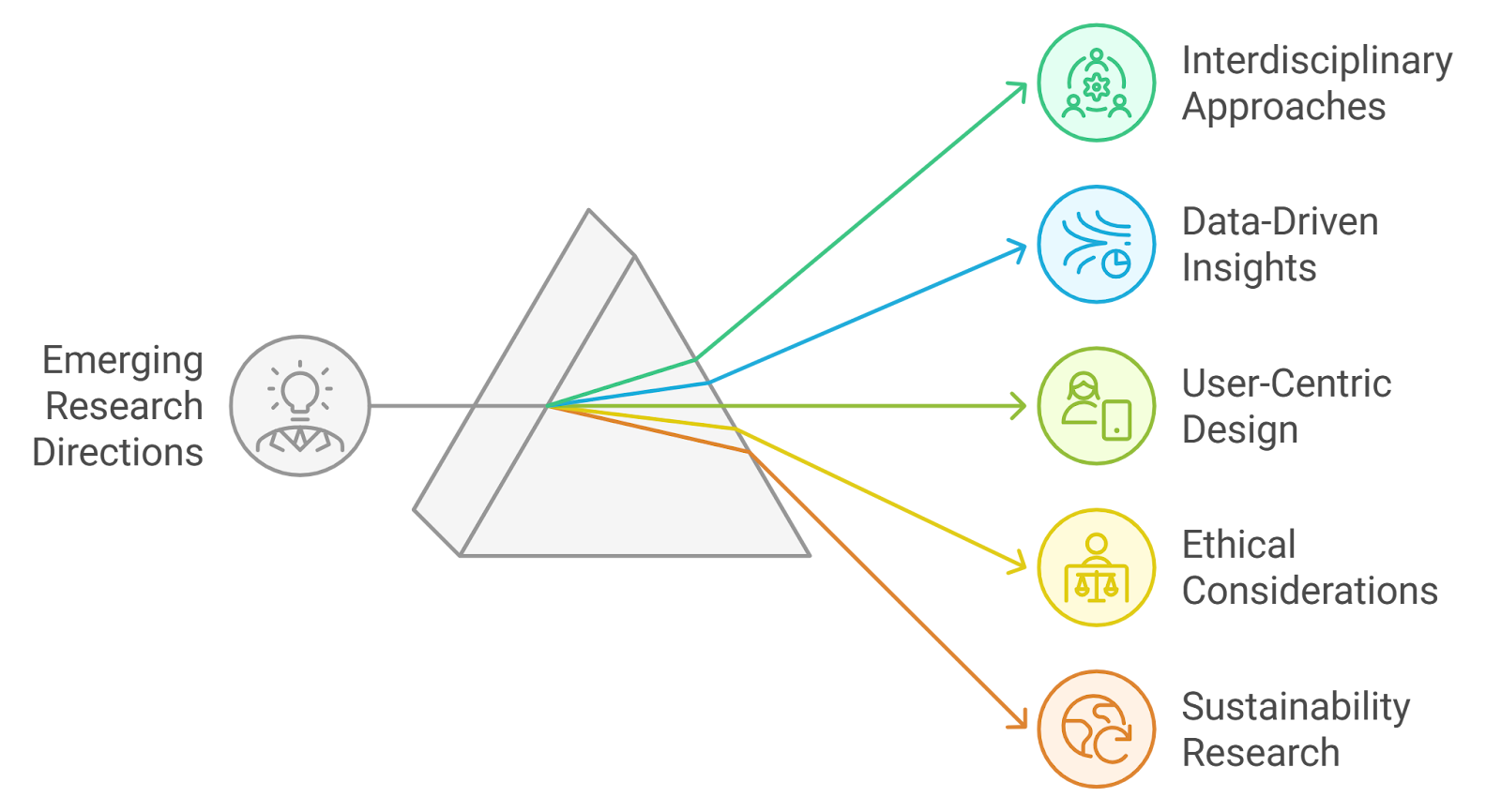
‍
Research plays a crucial role in driving innovation and understanding market dynamics. Several emerging research directions are shaping the future of various fields:
- Interdisciplinary Approaches: There is a growing trend towards interdisciplinary research, combining insights from different fields to address complex problems. This approach fosters innovation and leads to more comprehensive solutions. At Rapid Innovation, we advocate for interdisciplinary collaboration to develop cutting-edge AI and blockchain solutions.
- Data-Driven Insights: The rise of big data has opened new avenues for research. Analyzing large datasets can uncover patterns and trends that inform decision-making and strategy development. Our data analytics services empower clients to leverage their data effectively, driving informed business strategies.
- User-Centric Design: Research is increasingly focusing on user experience and design thinking. Understanding user needs and behaviors is essential for creating products and services that resonate with consumers. Rapid Innovation emphasizes user-centric design in our AI applications, ensuring that solutions are intuitive and impactful.
- Ethical Considerations: As technology advances, ethical considerations in research are becoming more prominent. Issues such as data privacy, algorithmic bias, and the societal impact of technology are critical areas of study. We prioritize ethical practices in our AI and blockchain projects, ensuring that our solutions uphold the highest standards of integrity.
- Sustainability Research: With the growing emphasis on sustainability, research is increasingly directed towards developing sustainable practices and technologies. This includes exploring renewable energy sources, waste reduction, and sustainable supply chains. Rapid Innovation is committed to advancing sustainability through innovative technology solutions that benefit both businesses and the environment.
These research directions are vital for fostering innovation and addressing the challenges faced by industries today.
13.4. Integration Possibilities
Integration across various sectors and technologies presents numerous opportunities for growth and efficiency. The potential for integration can be seen in several key areas:
- Technology Integration: The convergence of technologies such as IoT, AI, and blockchain is creating new possibilities for businesses. Integrating these technologies can enhance operational efficiency and improve customer experiences. Rapid Innovation specializes in creating integrated solutions that leverage these technologies to maximize ROI for our clients.
- Cross-Industry Collaboration: Businesses are increasingly collaborating across industries to leverage each other's strengths. For example, partnerships between tech companies and traditional industries can lead to innovative solutions and new market opportunities. We facilitate these collaborations, helping clients identify and engage with potential partners for mutual growth.
- Supply Chain Integration: Streamlining supply chains through integration can lead to significant cost savings and improved responsiveness. Technologies like RFID and blockchain can enhance transparency and traceability in supply chains. Our blockchain solutions provide clients with the tools to create more efficient and transparent supply chains, ultimately driving down costs and increasing trust.
- Data Integration: Combining data from various sources can provide a holistic view of operations and customer behavior. This integration enables better decision-making and more targeted marketing strategies. Rapid Innovation offers data integration services that empower clients to harness their data for strategic advantage.
- Customer Experience Integration: Creating a seamless customer experience across multiple touchpoints is essential for retaining customers. Integrating online and offline channels can enhance customer satisfaction and loyalty. Our AI-driven solutions help businesses create cohesive customer journeys that foster loyalty and drive revenue growth.
These integration possibilities highlight the importance of collaboration and technology in driving industry growth and innovation. At Rapid Innovation, we are dedicated to helping our clients navigate these opportunities to achieve their business goals efficiently and effectively.