Table Of Contents
Category
Artificial Intelligence
AIML
IoT
CRM
Security
1. Introduction to AI-Powered Damage Evaluation
Artificial Intelligence (AI) is revolutionizing various industries, and AI damage evaluation is no exception. AI-powered damage evaluation refers to the use of advanced algorithms and machine learning techniques to assess and quantify damage in various contexts, such as insurance claims, property assessments, and disaster recovery. This innovative approach enhances accuracy, speeds up the evaluation process, and reduces human error. As businesses and organizations increasingly adopt AI technologies, the landscape of damage evaluation is transforming, leading to more efficient and reliable outcomes.
1.1. Evolution of Damage Assessment Methods
The methods of damage assessment have evolved significantly over the years, transitioning from manual processes to automated systems.
- Traditional Methods: Initially, damage assessment relied heavily on manual inspections and subjective evaluations by experts. These methods were time-consuming and often led to inconsistencies in assessments due to human bias.
- Technological Advancements: The introduction of digital tools, such as drones and imaging technology, marked a significant shift. These tools allowed for more precise measurements and visual documentation of damage.
- Data-Driven Approaches: The rise of big data analytics enabled the collection and analysis of vast amounts of information related to damage events. This data-driven approach improved the accuracy of assessments and facilitated better decision-making.
- Integration of AI: The latest evolution involves the integration of AI technologies, which can analyze data patterns and predict damage outcomes with remarkable precision. AI algorithms can process images, assess structural integrity, and even predict future risks based on historical data.
1.2. Role of AI in Modern Damage Evaluation
AI plays a crucial role in modern damage evaluation, offering numerous advantages over traditional methods.
- Enhanced Accuracy: AI algorithms can analyze images and data with a level of precision that surpasses human capabilities, leading to more accurate assessments and reducing the likelihood of errors.
- Speed and Efficiency: AI-powered systems can process large volumes of data quickly, significantly reducing the time required for damage evaluations. This efficiency is particularly beneficial in emergency situations where timely assessments are critical.
- Predictive Analytics: AI can utilize historical data to predict potential damage scenarios, allowing organizations to prepare and mitigate risks effectively. This proactive approach can save time and resources in the long run.
- Cost-Effectiveness: By automating the evaluation process, AI reduces the need for extensive manpower, leading to cost savings for businesses and organizations. This efficiency can also translate to faster claim processing in the insurance industry.
- Improved Decision-Making: AI provides data-driven insights that enhance decision-making processes. Stakeholders can make informed choices based on accurate assessments and predictive analytics.
- Real-Time Monitoring: AI technologies enable real-time monitoring of assets and properties, allowing for immediate damage assessments after incidents. This capability is particularly valuable in industries such as construction and insurance.
In conclusion, the integration of AI in damage evaluation represents a significant advancement in the field. By leveraging technology, organizations can achieve more accurate, efficient, and cost-effective assessments, ultimately leading to better outcomes in damage management and recovery. At Rapid Innovation, we specialize in implementing AI solutions tailored to your specific needs, ensuring that your organization can harness the full potential of AI-powered damage evaluation to achieve greater ROI and operational efficiency.
Refer to the image for a visual representation of the evolution and role of AI in damage evaluation:
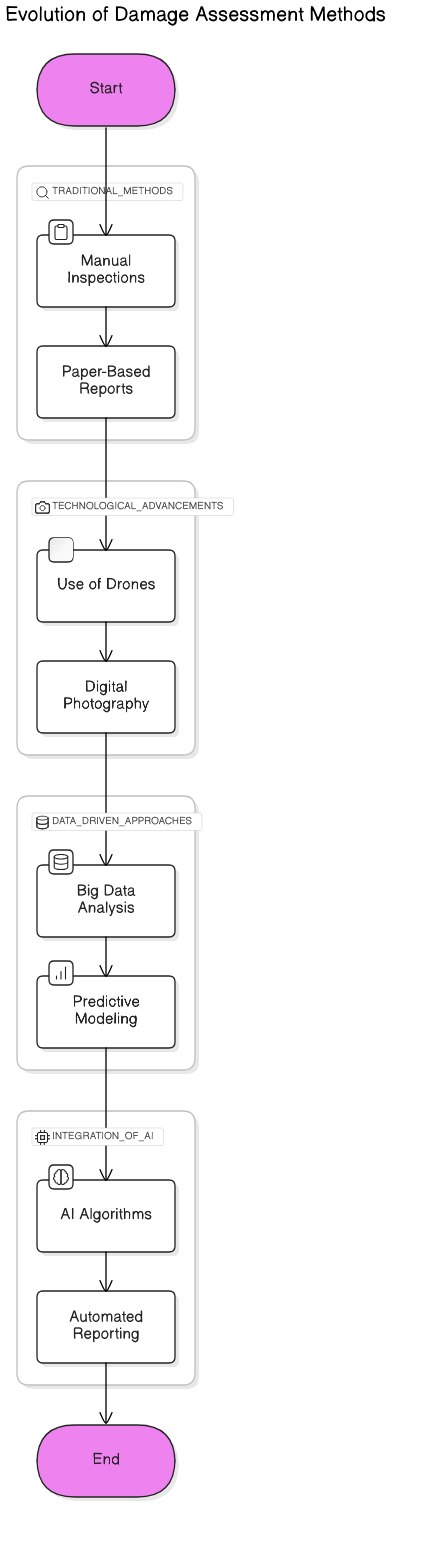
1.3. Key Stakeholders and Use Cases
In any industry, identifying key stakeholders is crucial for understanding the ecosystem and driving innovation. Stakeholders can vary widely depending on the sector, but they generally include:
- Customers: The end-users of products or services who drive demand and influence market trends. Their feedback is essential for product development and improvement.
- Suppliers: Entities that provide raw materials, components, or services necessary for production. Strong relationships with suppliers can lead to better pricing and quality control.
- Regulatory Bodies: Government agencies that enforce laws and regulations. Compliance with these regulations is vital for operational legitimacy and market access.
- Investors: Individuals or institutions that provide capital for business operations. Their expectations for returns can shape strategic decisions and growth trajectories.
- Employees: The workforce that drives the company’s operations. Employee satisfaction and engagement are critical for productivity and retention.
- Competitors: Other businesses in the same market. Understanding competitors’ strategies can help in positioning and differentiation.
Use cases for these stakeholders can include:
- Product Development: Engaging customers in the design process to create products that meet their needs, leveraging AI for insights and feedback analysis.
- Supply Chain Management: Collaborating with suppliers to optimize logistics and reduce costs, utilizing blockchain for transparency and efficiency.
- Regulatory Compliance: Working with regulatory bodies to ensure adherence to industry standards, employing AI to monitor compliance in real-time.
- Investment Strategies: Aligning business goals with investor expectations to secure funding, using data analytics to present compelling ROI forecasts.
- Employee Training: Implementing programs that enhance employee skills and job satisfaction, supported by AI-driven personalized learning paths.
- Market Analysis: Conducting competitive analysis to identify market gaps and opportunities, utilizing big data analytics for informed decision-making.
In this context, stakeholder management becomes essential. A stakeholder management plan can help in defining stakeholder management processes and ensuring effective stakeholder engagement. The stakeholder engagement strategy should include a stakeholder engagement assessment matrix to evaluate the level of involvement required for each stakeholder. Additionally, a stakeholder involvement plan can outline how to effectively communicate with stakeholders and keep them informed throughout the project lifecycle.
1.4. Current Industry Challenges
The landscape of any industry is fraught with challenges that can hinder growth and innovation. Some of the most pressing challenges include:
- Technological Disruption: Rapid advancements in technology can render existing business models obsolete. Companies must adapt quickly to stay relevant.
- Regulatory Compliance: Navigating complex regulations can be time-consuming and costly. Non-compliance can lead to severe penalties and reputational damage.
- Supply Chain Issues: Global supply chain disruptions, often exacerbated by geopolitical tensions or natural disasters, can impact production timelines and costs.
- Talent Acquisition and Retention: Finding and keeping skilled employees is increasingly difficult in a competitive job market. Companies must invest in employee development and workplace culture.
- Sustainability Pressures: Growing consumer demand for sustainable practices forces companies to rethink their operations and supply chains.
- Market Volatility: Economic fluctuations can affect consumer spending and investment, leading to uncertainty in business planning.
Addressing these challenges requires strategic planning, investment in technology, and a focus on building resilient business models. Understanding the meaning of stakeholder management and the importance of stakeholder involvement strategy can provide a framework for navigating these challenges effectively.
2. Core Technologies and Components
Core technologies and components are the backbone of any industry, enabling efficiency, innovation, and competitiveness. Key technologies include:
- Artificial Intelligence (AI): AI can automate processes, analyze data, and enhance decision-making. It is increasingly used in customer service, predictive analytics, and personalized marketing.
- Internet of Things (IoT): IoT devices collect and exchange data, providing real-time insights into operations. This technology is vital for smart manufacturing and supply chain optimization.
- Blockchain: This technology offers secure and transparent transaction records. It is particularly useful in supply chain management and financial services.
- Cloud Computing: Cloud services provide scalable resources and storage solutions, enabling businesses to operate more flexibly and cost-effectively.
- Big Data Analytics: Analyzing large datasets helps companies understand market trends, customer behavior, and operational efficiencies.
- Cybersecurity Solutions: As digital threats grow, robust cybersecurity measures are essential to protect sensitive data and maintain customer trust.
Components that support these technologies include:
- Software Platforms: Applications that facilitate data management, customer relationship management (CRM), and enterprise resource planning (ERP).
- Hardware Infrastructure: Servers, networking equipment, and IoT devices that support technology deployment.
- Data Management Systems: Tools for storing, processing, and analyzing data to derive actionable insights.
Investing in these core technologies and components is essential for businesses aiming to thrive in a competitive landscape. Rapid Innovation is positioned to assist clients in leveraging these technologies to achieve greater ROI and navigate industry challenges effectively. Understanding the stakeholder management meaning and the various aspects of stakeholder communication can further enhance the effectiveness of these initiatives. For instance, our expertise in AI Copilot Development can significantly improve product development and operational efficiency.
Refer to the image for a visual representation of key stakeholders and use cases in the industry.

2.1. Computer Vision Systems
Computer vision systems are a subset of artificial intelligence that enable machines to interpret and understand visual information from the world. These systems utilize algorithms and models to process images and videos, allowing computers to perform tasks that typically require human vision. The applications of computer vision are vast, ranging from autonomous vehicles to medical imaging and facial recognition, including computer vision technology and computer vision in manufacturing.
- Key components of computer vision systems include:
- Image acquisition
- Image processing
- Feature extraction
- Object detection
- Image classification
2.1.1. Image Recognition
Image recognition is a critical aspect of computer vision that involves identifying and classifying objects within an image. This technology has advanced significantly due to deep learning techniques, particularly convolutional neural networks (CNNs). Image recognition systems can analyze visual data and make decisions based on the content of the images, including applications in facial recognition open cv and opencv facial recognition.
- Applications of image recognition include: Â
- Facial recognition for security systems
- Object detection in autonomous vehicles
- Medical image analysis for disease detection
- Image tagging in social media platforms
- Benefits of image recognition: Â
- Increased accuracy in identifying objects
- Automation of repetitive tasks
- Enhanced user experience in applications like augmented reality
- Challenges in image recognition: Â
- Variability in lighting and angles
- Occlusion of objects
- Need for large datasets for training models
Recent advancements in image recognition have led to impressive accuracy rates, with some systems achieving over 95% accuracy in specific tasks. Rapid Innovation leverages these advancements to help clients implement image recognition solutions that enhance operational efficiency and drive greater ROI, including computer vision software and computer vision image recognition.
2.1.2. 3D Reconstruction
3D reconstruction is the process of capturing the shape and appearance of real objects to create a three-dimensional model. This technology is essential in various fields, including robotics, virtual reality, and computer graphics. 3D reconstruction can be achieved through different methods, such as stereo vision, structure from motion, and depth sensing, which are integral to computer vision 3D.
- Key techniques in 3D reconstruction: Â
- Stereo vision: Uses two or more images to estimate depth.
- Structure from motion: Analyzes motion between images to reconstruct 3D structures.
- Depth sensors: Devices like LiDAR and time-of-flight cameras measure distance to create 3D maps.
- Applications of 3D reconstruction: Â
- Creating realistic environments in video games and simulations
- Architectural modeling and design
- Medical imaging for surgical planning
- Cultural heritage preservation through digital archiving
- Benefits of 3D reconstruction: Â
- Enhanced visualization of complex structures
- Improved accuracy in measurements and modeling
- Ability to simulate real-world scenarios for training and analysis
- Challenges in 3D reconstruction: Â
- Computational intensity and processing time
- Difficulty in capturing fine details
- Variability in object textures and materials
3D reconstruction technologies are continually evolving, with recent developments enabling real-time processing and higher fidelity models. Rapid Innovation is at the forefront of these advancements, providing clients with tailored 3D reconstruction solutions that not only meet their specific needs but also maximize their return on investment, including machine vision software open source and edge computer vision.
Refer to the image for a visual representation of the components and processes involved in computer vision systems.

2.1.3. Depth Sensing
Depth sensing is a crucial technology that enables machines to perceive the world in three dimensions. It involves measuring the distance between the sensor and objects in the environment, providing valuable spatial information. This technology is widely used in various applications, including robotics, augmented reality, and autonomous vehicles.
- Depth sensors can be categorized into several types: Â
- Stereo vision: Utilizes two cameras to capture images from different angles, mimicking human binocular vision.
- Time-of-flight (ToF): Measures the time it takes for a light signal to travel to an object and back, calculating distance based on this time. This is a common feature in tof depth sensors.
- Structured light: Projects a known pattern onto a scene and analyzes the deformation of the pattern to determine depth.
- Applications of depth sensing include: Â
- Robotics: Enhances navigation and obstacle avoidance by providing spatial awareness, which is essential for efficient operation in dynamic environments. Depth sensors play a key role in this area.
- Augmented reality (AR): Enables realistic interaction between virtual objects and the real world by understanding the environment's depth, thereby improving user experience and engagement. Depth sensing cameras are often used in AR applications.
- Autonomous vehicles: Assists in safe navigation by detecting distances to other vehicles, pedestrians, and obstacles, significantly reducing the risk of accidents. Lidar depth sensors are particularly effective in this context.
Depth sensing technology is continually evolving, with advancements leading to improved accuracy and reduced costs. As a result, it is becoming increasingly accessible for various industries, enhancing the capabilities of machines and devices. At Rapid Innovation, we leverage depth sensing technology, including 3D depth sensors and depth sensing technology, to develop tailored solutions that help our clients achieve their business goals efficiently and effectively, ultimately driving greater ROI. For a comprehensive understanding of how machine learning integrates with depth sensing.
2.2. Machine Learning Models
Machine learning models are algorithms that enable computers to learn from data and make predictions or decisions without being explicitly programmed. These models are essential in analyzing complex datasets and extracting meaningful insights, making them invaluable in various fields, including finance, healthcare, and engineering.
- Key components of machine learning models include: Â
- Data: The foundation of any machine learning model, consisting of features (input variables) and labels (output variables).
- Training: The process of feeding data into the model to adjust its parameters and improve its accuracy.
- Evaluation: Assessing the model's performance using metrics such as accuracy, precision, and recall.
- Types of machine learning models: Â
- Supervised learning: Involves training a model on labeled data, where the desired output is known.
- Unsupervised learning: Deals with unlabeled data, focusing on finding patterns or groupings within the data.
- Reinforcement learning: Involves training an agent to make decisions by rewarding desired actions and penalizing undesired ones.
Machine learning models are transforming industries by automating processes, enhancing decision-making, and improving efficiency. As the volume of data continues to grow, the importance of these models will only increase. Rapid Innovation specializes in developing and implementing machine learning solutions that empower businesses to harness their data effectively, leading to improved operational efficiency and increased ROI.
2.2.1. Supervised Learning for Damage Classification
Supervised learning is a subset of machine learning where models are trained on labeled datasets. In the context of damage classification, supervised learning algorithms can effectively identify and categorize damage in various materials or structures, such as buildings, vehicles, and machinery.
The process of supervised learning for damage classification typically involves several steps:
- Data collection: Gathering labeled data that includes examples of damaged and undamaged instances.
- Feature extraction: Identifying relevant features from the data that will help the model distinguish between different types of damage.
- Model training: Using algorithms like decision trees, support vector machines, or neural networks to train the model on the labeled data.
- Model evaluation: Testing the model on a separate dataset to assess its accuracy and ability to generalize to new data.
The benefits of using supervised learning for damage classification are significant:
- High accuracy: Supervised learning models can achieve high levels of accuracy when trained on sufficient and representative data.
- Automation: These models can automate the damage assessment process, reducing the need for manual inspections and saving time and resources.
- Scalability: Once trained, supervised learning models can be deployed across various applications, making them versatile tools for damage classification.
Common applications of supervised learning in damage classification include:
- Infrastructure monitoring: Assessing the condition of bridges, roads, and buildings to identify potential hazards.
- Insurance claims: Automating the evaluation of damage in vehicles or properties for faster claims processing.
- Manufacturing: Detecting defects in products during the production process to ensure quality control.
Supervised learning for damage classification is a powerful approach that leverages the capabilities of machine learning to enhance safety, efficiency, and decision-making across multiple industries. At Rapid Innovation, we harness these advanced machine learning techniques to provide our clients with innovative solutions that drive operational excellence and maximize ROI.
Refer to the image for a visual representation of depth sensing technology and its applications.

2.2.2. Unsupervised Learning for Anomaly Detection
Unsupervised learning is a powerful approach in machine learning that does not require labeled data. It is particularly useful for anomaly detection, where the goal is to identify unusual patterns or outliers in data. This method is essential in various fields, including finance, healthcare, and cybersecurity.
- Definition: Unsupervised learning algorithms analyze data without prior labels, allowing them to discover hidden structures.
- Techniques: Common techniques include clustering, dimensionality reduction, and density estimation.
- Clustering: Algorithms like K-means and DBSCAN group similar data points, making it easier to spot anomalies that do not fit into any cluster. This is a key aspect of outlier detection.
- Dimensionality Reduction: Techniques such as PCA (Principal Component Analysis) reduce the number of features, helping to visualize data and identify outliers. This is particularly useful in anomaly detection using Python.
- Density Estimation: Methods like Gaussian Mixture Models (GMM) estimate the probability distribution of data points, allowing for the identification of low-density regions that may indicate anomalies. Statistical anomaly detection is often employed in this context.
- Applications: Unsupervised learning for anomaly detection is widely used in fraud detection, network security, and fault detection in manufacturing processes. Techniques such as scikit learn outlier detection are commonly utilized. At Rapid Innovation, we leverage these techniques to help clients enhance their security measures and operational efficiency, ultimately leading to greater ROI.
2.2.3. Deep Learning Architectures
Deep learning architectures are a subset of machine learning that utilize neural networks with multiple layers to model complex patterns in data. These architectures have gained popularity due to their ability to handle large datasets and extract intricate features.
- Types of Architectures: Â
- Convolutional Neural Networks (CNNs): Primarily used for image processing, CNNs excel at recognizing spatial hierarchies in images.
- Recurrent Neural Networks (RNNs): Ideal for sequential data, RNNs are used in natural language processing and time series analysis.
- Autoencoders: These are used for unsupervised learning tasks, particularly in anomaly detection, by learning efficient representations of data.
- Advantages: Â
- Feature Extraction: Deep learning models automatically learn features from raw data, reducing the need for manual feature engineering.
- Scalability: They can handle vast amounts of data, making them suitable for big data applications.
- Performance: Deep learning architectures often outperform traditional machine learning models in tasks like image and speech recognition.
- Challenges: Â
- Data Requirements: They typically require large datasets to train effectively.
- Computational Resources: Training deep learning models can be resource-intensive, requiring powerful hardware.
- Interpretability: Deep learning models are often seen as "black boxes," making it difficult to understand their decision-making processes.
At Rapid Innovation, we assist clients in implementing deep learning solutions tailored to their specific needs, ensuring they can harness the full potential of their data for improved decision-making and increased ROI.
2.3. Sensor Technologies
Sensor technologies play a crucial role in the collection of data for various applications, including IoT (Internet of Things), environmental monitoring, and industrial automation. These technologies enable the real-time gathering of information, which can be analyzed for insights and decision-making.
- Types of Sensors: Â
- Temperature Sensors: Used in HVAC systems, weather stations, and industrial processes to monitor temperature changes.
- Pressure Sensors: Essential in automotive and aerospace applications, these sensors measure pressure levels in various environments.
- Motion Sensors: Commonly used in security systems and smart homes, they detect movement and can trigger alarms or notifications.
- Applications: Â
- Smart Cities: Sensors are used to monitor traffic, air quality, and energy consumption, contributing to urban planning and sustainability.
- Healthcare: Wearable sensors track vital signs, enabling remote patient monitoring and personalized healthcare.
- Manufacturing: Sensors in production lines help monitor equipment health, predict failures, and optimize processes.
- Challenges: Â
- Data Management: The vast amount of data generated by sensors requires efficient storage and processing solutions.
- Interoperability: Different sensor technologies may not easily communicate with each other, leading to integration challenges.
- Security: As sensors become more connected, they are vulnerable to cyber threats, necessitating robust security measures.
In conclusion, unsupervised learning for anomaly detection, deep learning architectures, and sensor technologies are integral components of modern data analysis and machine learning applications. Each area presents unique opportunities and challenges, driving innovation across various industries. Rapid Innovation is committed to helping clients navigate these complexities, ensuring they achieve their business goals efficiently and effectively while maximizing their return on investment.
2.3.1. LiDAR Systems
LiDAR (Light Detection and Ranging) systems are advanced remote sensing technologies that utilize laser light to measure distances and create high-resolution maps. These systems are widely used in various fields, including geography, forestry, and urban planning, with numerous lidar applications.
- How it works: LiDAR emits laser pulses towards the ground and measures the time it takes for the light to return after hitting an object. This data is then used to calculate distances and create detailed 3D models of the terrain.
- Applications: Â
- Topographic mapping
- Vegetation analysis
- Infrastructure monitoring
- Application of lidar in civil engineering
- Advantages: Â
- High accuracy and precision
- Ability to penetrate vegetation
- Rapid data collection over large areas
- Limitations: Â
- High initial costs
- Requires skilled personnel for data interpretation
- Recent advancements: The integration of LiDAR with UAVs (drones) has revolutionized data collection, making it more accessible and efficient. At Rapid Innovation, we leverage these advancements to provide clients with tailored solutions that enhance operational efficiency and reduce costs, ultimately leading to greater ROI. The applications of lidar technology are expanding, particularly in civil engineering and urban planning. Additionally, the use of AI agents for IoT sensor integration is becoming increasingly relevant in optimizing these systems.
2.3.2. Thermal Imaging
Thermal imaging technology detects infrared radiation emitted by objects, allowing for the visualization of temperature differences. This technology is crucial in various sectors, including security, building inspections, and medical diagnostics.
- How it works: Thermal cameras capture the infrared radiation and convert it into an electronic signal, which is then processed to create a thermal image. The image displays temperature variations, with warmer areas appearing brighter.
- Applications: Â
- Building diagnostics (insulation and energy loss detection)
- Firefighting (locating hotspots)
- Medical imaging (detecting fevers or inflammation)
- Advantages: Â
- Non-invasive and safe
- Provides real-time data
- Effective in low-light conditions
- Limitations: Â
- Limited range compared to visible light cameras
- Requires calibration for accurate readings
- Recent trends: The use of thermal imaging in smart home technology is on the rise, enhancing security and energy efficiency. Rapid Innovation can assist clients in integrating thermal imaging solutions into their operations, leading to improved safety and reduced energy costs.
2.3.3. Acoustic Sensors
Acoustic sensors are devices that detect sound waves and convert them into electrical signals. These sensors are increasingly used in environmental monitoring, industrial applications, and security systems.
- How it works: Acoustic sensors capture sound waves through microphones or piezoelectric materials. The captured sound is then analyzed to determine its frequency, amplitude, and other characteristics.
- Applications: Â
- Wildlife monitoring (tracking animal movements)
- Structural health monitoring (detecting cracks or failures)
- Security systems (intrusion detection)
- Advantages: Â
- Can operate in various environmental conditions
- Capable of detecting a wide range of frequencies
- Relatively low cost compared to other sensor types
- Limitations: Â
- Susceptible to background noise
- Limited range for certain applications
- Emerging technologies: The integration of machine learning with acoustic sensors is enhancing their ability to analyze complex sound patterns, improving accuracy in various applications. At Rapid Innovation, we harness the power of AI to optimize the performance of acoustic sensors, enabling clients to achieve more accurate monitoring and analysis, thus maximizing their return on investment. The uses of lidar technology are also being explored in conjunction with acoustic sensors for enhanced environmental monitoring.
2.4. Data Processing Pipeline
A data processing pipeline is a series of data processing steps that transform raw data into a usable format. This pipeline is crucial for ensuring that data is clean, organized, and ready for analysis. The stages of a typical data processing pipeline include:
- Data Collection: Gathering data from various sources, such as databases, APIs, or user inputs. This can involve both structured data (like spreadsheets) and unstructured data (like text or images). This process is often part of an etl pipeline or an aws etl pipeline.
- Data Cleaning: Removing inaccuracies, duplicates, and irrelevant information. This step is essential to ensure the quality of the data, as poor-quality data can lead to misleading results.
- Data Transformation: Converting data into a suitable format for analysis. This may involve normalization, aggregation, or encoding categorical variables. An etl data pipeline is commonly used for this purpose.
- Data Storage: Storing the processed data in a database or data warehouse. This allows for efficient retrieval and management of data for future analysis.
- Data Analysis: Applying statistical methods or machine learning algorithms to extract insights from the data. This step is where the actual value of the data is realized. Data analysis can be part of a data analysis pipeline or a data processing pipeline.
- Data Visualization: Presenting the analyzed data in a visual format, such as charts or graphs, to make it easier to understand and communicate findings.
- Data Monitoring and Maintenance: Continuously monitoring the data pipeline for performance and making necessary adjustments to ensure it runs smoothly. This is crucial for maintaining an effective data pipeline management system.
The effectiveness of a data processing pipeline can significantly impact the quality of insights derived from data. Organizations that implement robust data processing pipelines can make more informed decisions and drive better business outcomes. At Rapid Innovation, we specialize in designing and implementing customized data processing pipelines, including python etl pipelines and data ingestion pipelines, that align with your business objectives, ensuring you achieve greater ROI through data-driven insights.
3. Industry-Specific Applications
Industry-specific applications of data processing and analysis are becoming increasingly important as businesses seek to leverage data for competitive advantage. Different industries utilize data in unique ways to address their specific challenges and opportunities. Some key applications include:
- Healthcare: Analyzing patient data to improve treatment outcomes and operational efficiency.
- Finance: Using data analytics for risk assessment, fraud detection, and investment strategies.
- Retail: Personalizing customer experiences through data-driven marketing and inventory management.
- Manufacturing: Implementing predictive maintenance and quality control through data analysis.
- Telecommunications: Enhancing customer service and network optimization using data insights.
These applications demonstrate the versatility of data processing across various sectors, highlighting the importance of tailored solutions to meet industry-specific needs.
3.1. Automotive Damage Assessment

‍
Automotive damage assessment is a critical application of data processing in the automotive industry. This process involves evaluating the extent of damage to vehicles after accidents or incidents. The integration of technology and data analytics has transformed how damage assessments are conducted. Key aspects include:
- Image Analysis: Utilizing computer vision and machine learning algorithms to analyze images of damaged vehicles. This technology can identify and quantify damage more accurately than traditional methods.
- Cost Estimation: Automating the estimation of repair costs based on the assessed damage, which can streamline the claims process for insurance companies and improve customer satisfaction.
- Data Integration: Combining data from various sources, such as repair history, parts availability, and labor costs, to provide a comprehensive assessment.
- Real-Time Reporting: Offering instant reports to stakeholders, including insurance adjusters and repair shops, which can expedite decision-making.
- Predictive Analytics: Using historical data to predict future repair needs and costs, helping businesses manage resources more effectively.
- Fraud Detection: Analyzing patterns in damage claims to identify potential fraud, protecting insurance companies from losses.
The use of data processing in automotive damage assessment not only enhances accuracy but also improves efficiency in the claims process. As technology continues to evolve, the automotive industry will likely see further advancements in damage assessment methodologies, leading to better outcomes for all parties involved. Rapid Innovation is committed to leveraging these advancements to help our clients optimize their operations and achieve significant returns on their investments.
3.1.1. Collision Analysis
Collision analysis is a critical process in understanding the dynamics of vehicle collisions. It involves examining the circumstances surrounding a vehicle collision to determine the cause and impact of the incident. This analysis is essential for insurance claims, legal proceedings, and improving vehicle safety standards. Key components of collision analysis include:
- Data Collection: Gathering data from the accident scene, including photographs, witness statements, and police reports.
- Vehicle Dynamics: Analyzing the speed, direction, and impact angles of the vehicles involved.
- Crash Reconstruction: Utilizing computer simulations and physical evidence to recreate the accident scenario, often enhanced by AI algorithms that can predict outcomes based on various parameters.
- Injury Assessment: Evaluating the injuries sustained by occupants to understand the collision's severity, which can be further analyzed using AI-driven predictive models to assess long-term impacts.
- Expert Testimony: Engaging accident reconstruction experts to provide insights in legal cases, supported by data analytics to strengthen arguments.
Collision analysis not only helps in determining liability but also aids in identifying trends that can lead to improved road safety measures, ultimately contributing to a reduction in accident rates and enhancing overall public safety.
3.1.2. Paint and Surface Damage
Paint and surface damage assessment is crucial in vehicle repair and restoration. This evaluation helps in determining the extent of damage and the necessary steps for repair. Understanding the types of paint and surface damage can also provide insights into the accident's severity. The assessment includes:
- Types of Damage: Identifying scratches, dents, and chips in the paintwork.
- Paint Layer Examination: Analyzing the layers of paint to determine if the damage penetrated to the metal.
- Color Matching: Ensuring that any repairs match the original paint color for aesthetic consistency, potentially utilizing AI tools for precise color matching.
- Corrosion Risk: Assessing the potential for rust and corrosion due to exposed metal surfaces.
- Repair Techniques: Exploring options such as touch-up paint, repainting, or professional detailing.
Proper assessment of paint and surface damage is essential for maintaining the vehicle's value and appearance, as well as ensuring long-term durability.
3.1.3. Structural Integrity Evaluation
Structural integrity evaluation is a vital aspect of vehicle safety assessment following a vehicle collision. This process involves examining the vehicle's frame and body to ensure it can withstand future impacts and provide adequate protection to occupants. The evaluation includes:
- Frame Inspection: Checking for bends, cracks, or misalignments in the vehicle's frame.
- Material Analysis: Evaluating the materials used in the vehicle's construction for strength and durability, potentially leveraging blockchain technology for traceability of material quality.
- Safety Features: Assessing the functionality of safety features such as airbags and crumple zones.
- Repair Feasibility: Determining whether the vehicle can be safely repaired or if it is a total loss.
- Regulatory Compliance: Ensuring that any repairs meet safety standards set by regulatory bodies.
A thorough structural integrity evaluation is essential for ensuring that a vehicle remains safe for operation after a vehicle collision, protecting both the driver and passengers. By integrating AI and blockchain technologies, Rapid Innovation can enhance the accuracy and reliability of these assessments, ultimately leading to greater ROI for clients in the automotive industry.
3.2. Property Damage Evaluation
Property damage evaluation is a critical process that assesses the extent of damage to a property, often following events such as natural disasters, accidents, or other unforeseen incidents. This evaluation is essential for insurance claims, restoration efforts, and ensuring the safety of occupants. The evaluation process typically involves two main components: natural disaster assessment and building structural analysis.
3.2.1. Natural Disaster Assessment
Natural disaster assessment focuses on evaluating the impact of natural events such as hurricanes, floods, earthquakes, and wildfires on properties. This assessment is crucial for understanding the extent of damage and determining the necessary recovery steps.
- Types of Natural Disasters: Â
- Hurricanes can cause wind damage and flooding.
- Earthquakes may lead to structural failures.
- Floods can result in water damage and mold growth.
- Assessment Process: Â
- Initial inspections are conducted to identify visible damage.
- The use of drones and satellite imagery can provide a broader view of affected areas, enhancing the accuracy of assessments.
- Detailed reports are generated, documenting the type and extent of damage.
- Data Collection: Â
- Gathering data on the disaster's intensity and duration.
- Utilizing historical data to predict potential future risks.
- Engaging with local authorities and emergency services for accurate information.
- Impact on Property Value: Â
- Properties in disaster-prone areas may experience a decrease in market value.
- Insurance premiums may rise due to increased risk assessments.
3.2.2. Building Structural Analysis
Building structural analysis is a systematic evaluation of a building's integrity and safety following damage. This analysis is essential to ensure that the structure can withstand future stresses and is safe for occupancy.
- Key Components of Structural Analysis: Â
- Foundation Assessment: Evaluating the foundation for cracks, settling, or shifting.
- Load-Bearing Walls: Inspecting walls that support the structure for any signs of damage.
- Roof Integrity: Checking for leaks, sagging, or missing shingles that could compromise the building.
- Methods of Analysis: Â
- Visual inspections by qualified engineers or inspectors.
- Non-destructive testing methods, such as ultrasonic testing, to assess material integrity.
- Load testing to determine if the structure can support expected loads.
- Safety Considerations: Â
- Identifying hazards that could pose risks to occupants.
- Ensuring compliance with local building codes and regulations.
- Recommending repairs or reinforcements to enhance structural integrity.
- Documentation and Reporting: Â
- Creating detailed reports that outline findings and recommendations.
- Providing documentation for insurance claims and legal purposes.
- Offering guidance on necessary repairs and timelines for restoration.
In conclusion, property damage evaluation through natural disaster assessment and building structural analysis is vital for ensuring safety, facilitating recovery, and maintaining property value. Proper evaluation helps property owners make informed decisions about repairs and future risk management. At Rapid Innovation, we leverage advanced AI technologies and blockchain solutions to streamline the property damage evaluation process, ensuring accuracy and efficiency, ultimately leading to greater ROI for our clients. For more insights on personalized risk evaluation in insurance with AI agents.
3.2.3. Interior Damage Documentation
Interior damage documentation is a critical process in assessing the extent of damage within a structure, particularly after events such as natural disasters, fires, or vandalism. This documentation serves multiple purposes, including insurance claims, legal proceedings, and restoration planning.
- Visual Evidence: Photographs and videos should be taken to capture the condition of the interior spaces. This includes: Â
- Walls, ceilings, and floors
- Fixtures and fittings
- Any visible mold or water damage
- Detailed Descriptions: Each documented area should include: Â
- A written description of the damage
- The location within the building
- The estimated size and severity of the damage
- Inventory of Damaged Items: Create a list of all damaged items, including: Â
- Furniture
- Appliances
- Personal belongings
- Professional Assessments: In some cases, it may be necessary to involve professionals such as: Â
- Structural engineers
- Restoration specialists
- Insurance adjusters
- Documentation Tools: Utilize various tools and technologies to enhance documentation, such as: Â
- Drones for aerial views
- 3D scanning for detailed modeling
- Mobile apps for real-time reporting
Proper interior damage documentation not only aids in recovery efforts but also ensures that all necessary information is available for future reference. Rapid Innovation can assist in this process by leveraging AI-driven image recognition and data analysis tools to streamline documentation, ensuring accuracy and efficiency, ultimately leading to a greater return on investment (ROI) for clients.
3.3. Infrastructure Assessment
Infrastructure assessment is essential for maintaining the safety and functionality of public and private structures. This process involves evaluating the condition of various infrastructure components to identify any necessary repairs or upgrades.
- Types of Infrastructure: Key areas of focus include: Â
- Roads and highways
- Bridges and tunnels
- Utilities such as water, gas, and electricity
- Assessment Techniques: Various methods can be employed to assess infrastructure, including: Â
- Visual inspections
- Non-destructive testing
- Load testing for structural integrity
- Data Collection: Collecting data is crucial for a comprehensive assessment. This can involve: Â
- Surveys and questionnaires
- Historical data analysis
- Geographic Information Systems (GIS) for mapping
- Prioritization of Repairs: After assessment, prioritize repairs based on: Â
- Safety concerns
- Cost-effectiveness
- Impact on the community
- Regulatory Compliance: Ensure that all assessments meet local, state, and federal regulations, which may include: Â
- Building codes
- Environmental regulations
- Safety standards
Regular infrastructure assessments help prevent catastrophic failures and ensure that communities remain safe and functional. Rapid Innovation employs blockchain technology to create immutable records of assessments, enhancing transparency and trust among stakeholders, which can lead to improved funding opportunities and project approvals.
3.3.1. Bridge and Road Inspection
Bridge and road inspections are vital components of infrastructure assessment, focusing specifically on the safety and usability of these critical transportation networks. Regular inspections help identify potential issues before they escalate into serious problems.
- Inspection Frequency: The frequency of inspections can vary based on: Â
- The age of the structure
- Traffic volume
- Environmental conditions
- Inspection Methods: Common methods for inspecting bridges and roads include: Â
- Visual inspections by trained personnel
- Use of drones for hard-to-reach areas
- Advanced technologies like ultrasonic testing for material integrity
- Key Inspection Elements: Inspectors should focus on several key areas, such as: Â
- Structural components (beams, girders, and supports)
- Surface conditions (cracks, potholes, and erosion)
- Safety features (guardrails, signage, and lighting)
- Documentation and Reporting: After inspections, it is essential to document findings thoroughly: Â
- Create detailed reports outlining the condition of the structure
- Include photographs and diagrams for clarity
- Recommend necessary repairs or maintenance
- Long-term Planning: Use inspection data to inform long-term infrastructure planning, including: Â
- Budgeting for repairs and upgrades
- Scheduling future inspections
- Implementing preventative maintenance programs
Regular bridge and road inspections are crucial for ensuring the safety of travelers and the longevity of infrastructure. By integrating AI analytics and blockchain for data integrity, Rapid Innovation enhances the inspection process, ensuring timely interventions and maximizing ROI for infrastructure projects.
3.3.2. Pipeline Damage Detection
Pipeline damage detection is crucial for maintaining the integrity and safety of pipeline systems. These systems transport essential resources like oil, gas, and water, and any damage can lead to significant environmental hazards and economic losses. Advanced technologies are employed to monitor pipelines continuously. Sensors and IoT devices are strategically placed along pipelines to detect leaks or structural weaknesses. Data analytics plays a vital role in interpreting sensor data to identify potential issues before they escalate. Machine learning algorithms can analyze historical data to predict failure points and optimize maintenance schedules. Regular inspections using drones and robotic systems enhance the ability to detect damage in hard-to-reach areas. Real-time monitoring systems can alert operators immediately when anomalies are detected, allowing for swift action.
The integration of AI in pipeline damage detection not only improves accuracy but also reduces operational costs. By leveraging predictive maintenance strategies, companies can minimize downtime and extend the lifespan of their infrastructure. At Rapid Innovation, we specialize in implementing these advanced AI solutions, ensuring that our clients achieve greater ROI through enhanced operational efficiency and reduced risk. For more information on how AI can enhance grid management.
3.3.3. Power Grid Infrastructure
Power grid infrastructure is the backbone of energy distribution, ensuring that electricity reaches homes and businesses efficiently. As the demand for energy increases, the need for a robust and resilient power grid becomes more critical. Smart grid technology enhances the efficiency of power distribution. Real-time data collection allows for better demand forecasting and load balancing. The integration of renewable energy sources, such as solar and wind, requires advanced grid management systems. Cybersecurity measures are essential to protect the grid from potential threats and attacks. Energy storage solutions, like batteries, help manage supply and demand fluctuations. Automated systems can quickly isolate faults, reducing the duration of outages.
Investing in modernizing power grid infrastructure is essential for sustainability and reliability. The transition to smart grids not only improves operational efficiency but also supports the integration of clean energy technologies. Rapid Innovation offers consulting and development services to help clients navigate this transition, ensuring they maximize their investments in smart grid technologies.
4. AI Agent Architecture
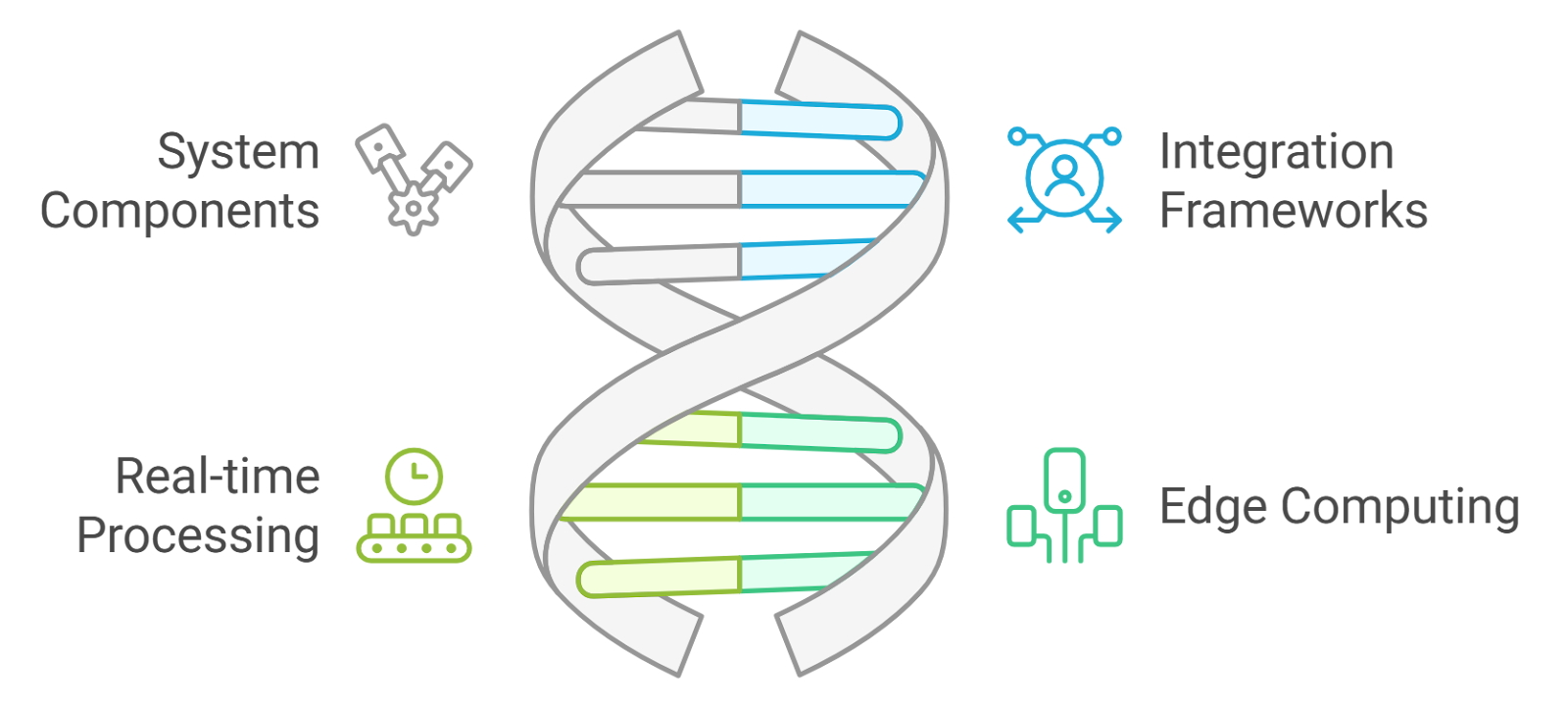
‍
AI agent architecture refers to the design and structure of systems that utilize artificial intelligence to perform tasks autonomously. This architecture is fundamental in developing intelligent systems capable of learning, reasoning, and decision-making. Modular design allows for flexibility and scalability in AI applications. Components typically include perception, reasoning, learning, and action modules. Perception modules gather data from the environment through sensors and cameras. Reasoning modules analyze the data to make informed decisions based on predefined rules or learned experiences. Learning modules enable the system to adapt and improve over time through machine learning techniques. Action modules execute decisions, interacting with the environment or other systems.
The effectiveness of AI agent architecture lies in its ability to process vast amounts of data and derive actionable insights. This architecture is widely used in various applications, including robotics, autonomous vehicles, and smart home systems. By optimizing AI agent architecture, developers can create more efficient and capable intelligent systems that enhance productivity and innovation. At Rapid Innovation, we leverage our expertise in AI agent architecture to deliver tailored solutions that drive business success for our clients.
4.1. System Components
In any robust system, especially those dealing with data processing and analytics, the architecture is typically composed of several key components. Understanding these components is crucial for optimizing performance and ensuring seamless operation. The two primary components of such a system are the Data Collection Module and the Analysis Engine.
4.1.1. Data Collection Module
The Data Collection Module is the foundational element of any data-driven system. It is responsible for gathering data from various sources, ensuring that the information is accurate, timely, and relevant. This module plays a critical role in the overall effectiveness of the system.
- Data Sources: The module can collect data from multiple sources, including: Â
- IoT devices
- Social media platforms
- Databases
- APIs
- User inputs
- Data Types: It handles various types of data, such as: Â
- Structured data (e.g., databases)
- Unstructured data (e.g., text, images)
- Semi-structured data (e.g., JSON, XML)
- Data Quality: Ensuring data quality is paramount. The module typically includes: Â
- Validation checks to ensure accuracy
- Cleaning processes to remove duplicates and errors
- Standardization to maintain consistency across datasets.
- Real-time vs. Batch Processing: The module can operate in different modes: Â
- Real-time data collection for immediate analysis, including realtime data processing and realtime data integration
- Batch processing for periodic data aggregation
- Scalability: As data volumes grow, the module must be scalable to handle increased loads without compromising performance.
- Security: Data collection must adhere to security protocols to protect sensitive information, including: Â
- Encryption during transmission
- Access controls to limit data exposure
4.1.2. Analysis Engine
The Analysis Engine is the core component that processes the collected data, transforming it into actionable insights. This engine employs various algorithms and methodologies to analyze data, making it a critical part of any data analytics system.
- Data Processing: The engine processes data through: Â
- Statistical analysis to identify trends and patterns
- Machine learning algorithms for predictive analytics
- Natural language processing for text analysis
- Visualization: The engine often includes tools for data visualization, allowing users to: Â
- Create dashboards
- Generate reports
- Use graphs and charts to represent data findings
- Performance Optimization: To ensure efficient processing, the engine may utilize: Â
- Parallel processing to handle large datasets
- Caching mechanisms to speed up repeated queries
- Integration: The Analysis Engine must integrate seamlessly with other system components, including: Â
- Data storage solutions (e.g., databases, data lakes)
- User interfaces for end-user interaction
- Feedback Loop: A well-designed engine incorporates a feedback mechanism to: Â
- Continuously improve algorithms based on new data
- Adjust models to enhance accuracy over time
- Scalability and Flexibility: The engine should be scalable to accommodate growing data needs and flexible enough to adapt to new analytical methods or technologies, such as OLAP and OLTP systems.
In summary, the Data Collection Module and the Analysis Engine are essential components of a data-driven system. Together, they ensure that data is accurately collected, processed, and transformed into valuable insights, driving informed decision-making and strategic planning. At Rapid Innovation, we leverage these components to help our clients achieve greater ROI by implementing tailored solutions that enhance data integrity, optimize analytics, and ultimately support their business objectives, including data migration from legacy systems and data warehouse extract transform load processes.
4.1.3. Reporting System
A robust reporting system is essential for organizations to make informed decisions based on data analysis. It serves as a bridge between raw data and actionable insights. An effective reporting system not only enhances transparency but also fosters accountability within the organization. By leveraging advanced analytics, businesses can uncover hidden insights that drive strategic initiatives and ultimately achieve greater ROI.
- Provides comprehensive data visualization tools to present information clearly, enabling stakeholders to grasp complex data quickly. Â
- Enables users to generate customized reports tailored to specific needs, ensuring that decision-makers have the most relevant information at their fingertips. Â
- Supports various formats for report generation, including PDF, Excel, and web-based dashboards, allowing for flexibility in data presentation. Â
- Facilitates real-time data access, allowing for timely decision-making that can significantly impact business outcomes. Â
- Incorporates automated reporting features to reduce manual effort and errors, streamlining the reporting process and enhancing productivity, such as those found in automated reporting systems and safety incident management software. Â
- Ensures data accuracy and integrity through validation processes, which is crucial for maintaining trust in the data-driven decision-making process. Â
- Offers historical data analysis to identify trends and patterns over time, empowering organizations to make proactive strategic decisions, similar to the capabilities of hr reporting software and epic reporting. Â
4.2. Integration Frameworks
Integration frameworks are critical for ensuring seamless communication between different systems and applications within an organization. They enable data sharing and process automation, enhancing overall operational efficiency. By implementing a robust integration framework, organizations can streamline workflows, reduce operational silos, and improve data accessibility across departments, leading to increased productivity and ROI.
- Supports various integration methods, including API, ETL (Extract, Transform, Load), and middleware solutions, allowing for versatile data connectivity. Â
- Facilitates interoperability between legacy systems and modern applications, ensuring that organizations can leverage existing investments while adopting new technologies. Â
- Allows for real-time data synchronization across platforms, ensuring consistency and accuracy in data reporting. Â
- Provides a centralized hub for managing data flows and integrations, simplifying the oversight of complex data environments. Â
- Enhances scalability by allowing organizations to add new applications without disrupting existing processes, thus supporting growth initiatives. Â
- Reduces integration complexity through pre-built connectors and templates, accelerating deployment times and reducing costs. Â
- Promotes data governance by ensuring compliance with regulatory standards, which is essential for maintaining operational integrity. Â
4.3. Real-time Processing Capabilities
Real-time processing capabilities are essential for organizations that require immediate insights and actions based on data. This capability allows businesses to respond swiftly to changing conditions and customer needs. With real-time processing capabilities, organizations can gain a competitive edge by leveraging data to drive innovation and improve operational efficiency, ultimately enhancing ROI.
- Enables instant data ingestion from various sources, including IoT devices, social media, and transactional systems, ensuring that organizations have access to the latest information. Â
- Supports real-time analytics, allowing organizations to derive insights as data is generated, which is crucial for timely decision-making. Â
- Facilitates immediate decision-making, which is crucial in industries like finance, healthcare, and e-commerce, where rapid responses can lead to significant advantages. Â
- Enhances customer experience by providing timely responses and personalized services, fostering customer loyalty and satisfaction. Â
- Reduces latency in data processing, ensuring that information is always up-to-date and actionable. Â
- Integrates with machine learning algorithms to enable predictive analytics in real-time, allowing organizations to anticipate trends and make informed decisions. Â
- Supports event-driven architectures, allowing systems to react to specific triggers or events, further enhancing operational responsiveness. Â
Additionally, tools like powerscribe, home inspection reporting software, and oracle business intelligence publisher can further enhance the reporting capabilities within organizations.
4.4. Edge Computing Implementation
Edge computing is a distributed computing paradigm that brings computation and data storage closer to the location where it is needed. This approach reduces latency, enhances speed, and improves the overall efficiency of data processing. Implementing edge computing involves several key steps:
- Infrastructure Setup: Establishing the necessary hardware and software infrastructure at the edge, which includes edge devices like IoT sensors, gateways, and local servers that can process data near the source.
- Data Management: Developing a robust data management strategy to handle data generated at the edge. This includes data filtering, aggregation, and storage solutions that minimize bandwidth usage and ensure quick access to relevant data.
- Application Development: Creating applications that can leverage edge computing capabilities. These applications should be designed to operate in real-time, processing data locally and only sending essential information to the cloud for further analysis. The implementation of IoT with edge devices is crucial in this step to enhance functionality.
- Security Measures: Implementing security protocols to protect data at the edge, including encryption, secure access controls, and regular updates to safeguard against vulnerabilities.
- Integration with Cloud Services: Ensuring seamless integration between edge devices and cloud services. This allows for a hybrid approach where critical data is processed locally, while less urgent data can be sent to the cloud for deeper analysis.
- Monitoring and Maintenance: Establishing a system for monitoring edge devices and applications to ensure they are functioning correctly. Regular maintenance and updates are crucial to keep the system secure and efficient.
5. Data Collection and Processing
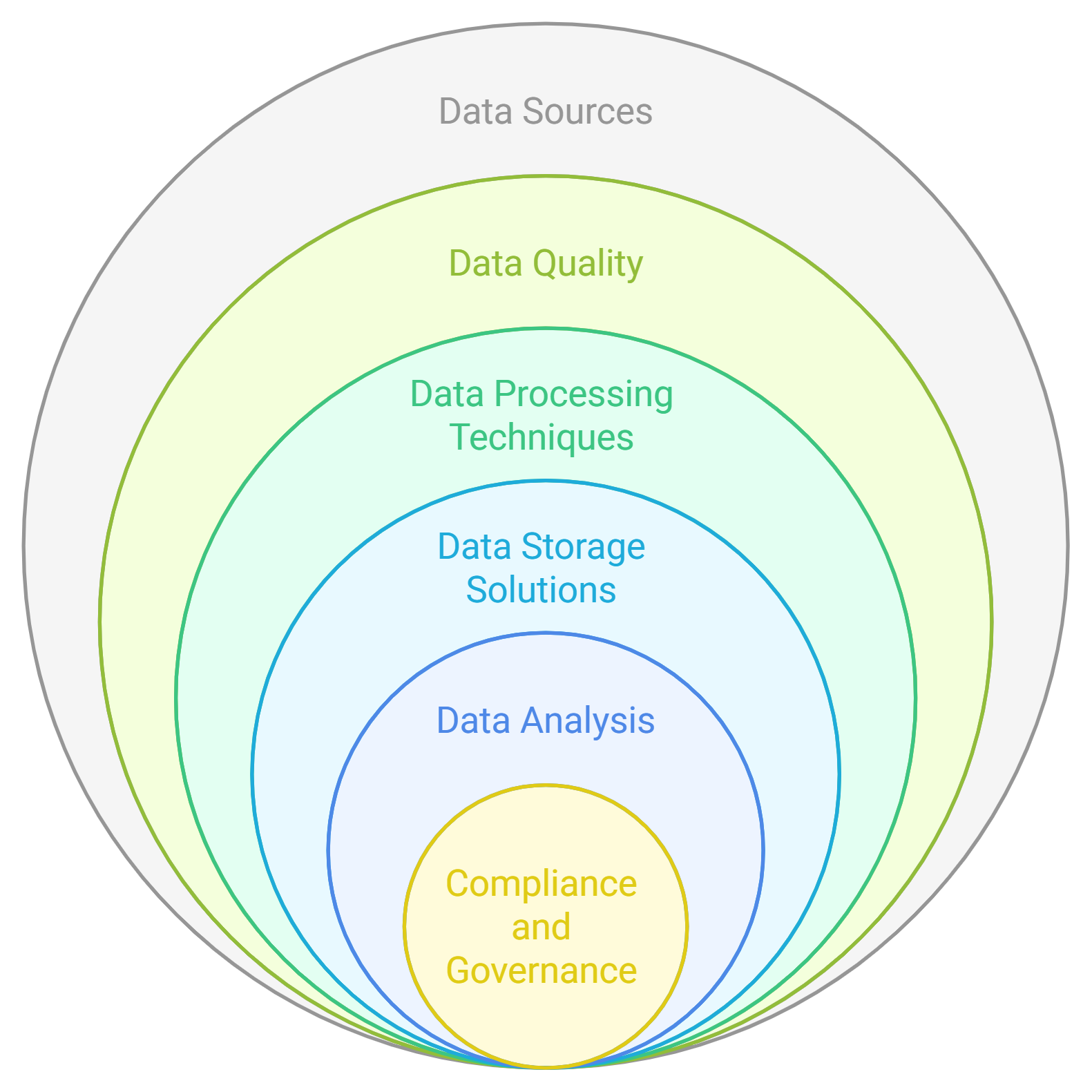
‍
Data collection and processing are fundamental components of any data-driven strategy. This process involves gathering data from various sources, processing it for analysis, and deriving insights that can inform decision-making. Key aspects include:
- Data Sources: Identifying and utilizing various data sources, such as IoT devices, social media, and enterprise systems. Each source can provide valuable information that contributes to a comprehensive data set.
- Data Quality: Ensuring the quality of collected data is paramount. This involves validating data accuracy, consistency, and completeness to ensure reliable analysis.
- Data Processing Techniques: Employing various data processing techniques, such as batch processing and real-time processing, depending on the use case. Real-time processing is essential for applications requiring immediate insights, while batch processing is suitable for large volumes of data.
- Data Storage Solutions: Choosing appropriate storage solutions that can handle the volume and velocity of data being collected. Options include cloud storage, on-premises databases, and hybrid solutions.
- Data Analysis: Utilizing analytical tools and techniques to extract insights from the processed data. This can involve statistical analysis, machine learning algorithms, and data visualization tools.
- Compliance and Governance: Adhering to data governance policies and regulations, such as GDPR or HIPAA, to ensure data privacy and security throughout the collection and processing stages.
5.1. Image and Video Capture
Image and video capture are critical components of data collection, especially in fields like surveillance, healthcare, and marketing. The process involves several key considerations:
- Capture Devices: Utilizing high-quality cameras and sensors for capturing images and videos. The choice of device can significantly impact the quality of the data collected.
- Resolution and Quality: Ensuring that the images and videos captured are of high resolution and quality, which is essential for accurate analysis and interpretation of the data.
- Storage and Management: Implementing effective storage solutions for the large volumes of image and video data generated, including cloud storage options that allow for easy access and retrieval.
- Processing Techniques: Applying image and video processing techniques to enhance the quality of the captured data, which can include noise reduction, image stabilization, and color correction.
- Real-time Processing: For applications requiring immediate insights, real-time processing of video feeds is crucial. This can involve using edge computing to analyze video data on-site, reducing latency.
- Data Annotation: Annotating images and videos for training machine learning models. This process involves labeling data to help algorithms learn and improve their accuracy.
- Privacy Considerations: Addressing privacy concerns related to image and video capture, including obtaining consent from individuals being recorded and ensuring compliance with relevant regulations.
By focusing on these aspects, organizations can effectively implement edge computing, streamline data collection and processing, and enhance their image and video capture capabilities. Rapid Innovation is well-equipped to assist clients in these areas, leveraging our expertise in AI and blockchain to drive efficiency and maximize ROI. Through tailored solutions, we help businesses harness the power of adaptive AI development implementation and data analytics to achieve their strategic objectives.
5.2. Sensor Data Integration
Sensor data integration is a crucial process in the realm of data analytics, particularly in industries such as IoT, healthcare, and smart cities. This process involves combining data from multiple sensors to create a comprehensive dataset that can be analyzed for insights.
- Types of Sensors: Various sensors, including temperature, humidity, motion, and pressure sensors, generate sensor data that can be integrated.
- Data Fusion: This technique combines sensor data from different sources to improve accuracy and reliability. For instance, merging data from GPS and accelerometers can enhance location tracking, which is vital for applications like fleet management and logistics optimization.
- Real-time Processing: Integrating sensor data in real-time allows for immediate analysis and response, which is vital in applications like autonomous vehicles and industrial automation. Rapid Innovation leverages advanced AI algorithms to facilitate real-time sensor data processing, enabling clients to make informed decisions swiftly.
- Standardization: To effectively integrate sensor data, it is essential to standardize formats and protocols. This ensures that data from different sensors can be easily combined and analyzed, leading to more coherent insights and better decision-making.
- Challenges: Issues such as data redundancy, latency, and varying data quality can complicate sensor data integration. Addressing these challenges is key to successful implementation. Rapid Innovation employs robust methodologies to tackle these issues, ensuring that clients can achieve greater ROI through efficient sensor data integration.
5.3. Data Preprocessing
Data preprocessing is a fundamental step in data analysis that prepares raw data for further analysis. This stage is critical for ensuring the quality and usability of the data.
- Data Cleaning: This involves removing inaccuracies, duplicates, and irrelevant information from the dataset. Techniques include identifying and correcting errors, removing outliers, and filling in missing values, which are essential for maintaining data integrity.
- Data Transformation: This step modifies the data into a suitable format for analysis. Common transformations include normalization (scaling data to a specific range) and encoding categorical variables (converting categories into numerical values). These transformations enhance the performance of AI models developed by Rapid Innovation.
- Data Reduction: Reducing the volume of data while maintaining its integrity is essential for efficient processing. Techniques include dimensionality reduction (using methods like PCA - Principal Component Analysis - to reduce the number of features) and aggregation (summarizing data points to create a more manageable dataset).
- Feature Engineering: Creating new features from existing data can enhance model performance. This may involve combining multiple features and extracting date and time components, which can lead to more accurate predictions and insights.
- Importance: Proper data preprocessing can significantly improve the accuracy of predictive models and the overall quality of insights derived from the data, ultimately driving better business outcomes for our clients.
5.4. Quality Assurance Methods
Quality assurance (QA) methods are essential for ensuring the reliability and accuracy of data throughout its lifecycle. Implementing robust QA practices can prevent errors and enhance data integrity.
- Data Validation: This process checks the accuracy and quality of data before it is used. Techniques include range checks (ensuring data falls within specified limits) and consistency checks (verifying that data is consistent across different sources).
- Automated Testing: Utilizing automated tools to test data quality can save time and reduce human error. This includes regular audits of data quality and automated scripts to identify anomalies, ensuring that clients can trust the data they are working with.
- Statistical Methods: Employing statistical techniques to assess data quality can provide insights into potential issues. Common methods include descriptive statistics (analyzing mean, median, and mode to understand data distribution) and control charts (monitoring data over time to identify trends and variations).
- Documentation and Standards: Establishing clear documentation and standards for data collection and processing helps maintain quality. This includes creating data dictionaries and defining data entry protocols, which are crucial for compliance and operational efficiency.
- Continuous Improvement: QA should be an ongoing process. Regularly reviewing and updating QA methods ensures that data quality remains high as systems and technologies evolve, allowing Rapid Innovation to provide clients with cutting-edge solutions that adapt to their changing needs.
5.5. Data Storage and Management
Data storage and management are critical components in any organization, especially in the age of big data. Effective data storage ensures that information is easily accessible, secure, and organized. Here are some key aspects to consider:
- Types of Data Storage: Â
- On-Premises Storage: Physical servers located within the organization, providing direct control over data.
- Cloud Storage: Data stored on remote servers accessed via the internet, offering scalability and flexibility to adapt to changing business needs, including cloud storage management and cloud file management.
- Hybrid Solutions: A combination of on-premises and cloud storage, allowing for a tailored approach that meets specific organizational requirements, such as hybrid cloud storage management.
- Data Management Practices: Â
- Data Governance: Establishing policies and standards for data management to ensure data quality and compliance with regulations, including electronic data management systems.
- Data Backup and Recovery: Regularly backing up data to prevent loss and implementing recovery plans for emergencies, ensuring business continuity, which can involve cloud backup management.
- Data Lifecycle Management: Managing data from creation to deletion, ensuring that data is archived or disposed of appropriately to optimize storage costs, relevant in data storage management solutions.
- Security Measures: Â
- Encryption: Protecting data by converting it into a secure format that can only be read with a decryption key, safeguarding sensitive information.
- Access Controls: Implementing user permissions to restrict access to sensitive data, ensuring that only authorized personnel can view or modify information.
- Regular Audits: Conducting audits to ensure compliance with data management policies and identify potential vulnerabilities, enhancing overall security posture.
- Emerging Technologies: Â
- Artificial Intelligence: Utilizing AI for data organization, predictive analytics, and anomaly detection, enabling organizations to derive insights and make informed decisions, which can be enhanced through big data storage and management.
- Blockchain: Ensuring data integrity and security through decentralized storage solutions, providing a transparent and tamper-proof method of managing data.
Effective data storage and management not only enhance operational efficiency but also support informed decision-making and strategic planning, ultimately leading to greater ROI for organizations, including the use of electronic document records management systems and information storage management.
6. Analysis and Assessment Techniques
Analysis and assessment techniques are essential for interpreting data and deriving actionable insights. These techniques help organizations understand trends, make predictions, and evaluate performance. Key techniques include:
- Descriptive Analysis: Â
- Summarizes historical data to identify patterns and trends.
- Utilizes statistical measures such as mean, median, and mode.
- Predictive Analysis: Â
- Uses historical data to forecast future outcomes.
- Employs machine learning algorithms to identify potential risks and opportunities.
- Prescriptive Analysis: Â
- Recommends actions based on data analysis.
- Combines predictive analytics with optimization techniques to suggest the best course of action.
- Qualitative Analysis: Â
- Focuses on non-numerical data to understand underlying motivations and behaviors.
- Involves methods such as interviews, focus groups, and content analysis.
- Quantitative Analysis: Â
- Involves numerical data to quantify relationships and test hypotheses.
- Utilizes statistical tools and software for data analysis.
- Visualization Techniques: Â
- Employs charts, graphs, and dashboards to present data in an easily digestible format.
- Enhances understanding and communication of complex data sets.
By implementing these analysis and assessment techniques, organizations can make data-driven decisions that enhance performance and drive growth, ultimately maximizing their return on investment.
6.1. Damage Classification Algorithms
Damage classification algorithms are vital in various fields, including engineering, healthcare, and environmental science. These algorithms help in identifying and categorizing damage based on specific criteria. Key points include:
- Types of Damage Classification Algorithms: Â
- Supervised Learning Algorithms:
- Require labeled data for training.
- Common algorithms include Support Vector Machines (SVM), Decision Trees, and Neural Networks.
- Unsupervised Learning Algorithms:
- Analyze data without pre-existing labels.
- Techniques like clustering (e.g., K-means) are used to identify patterns in data.
- Supervised Learning Algorithms:
- Applications: Â
- Structural Health Monitoring: Used to assess the integrity of buildings and bridges by classifying damage types.
- Medical Imaging: Algorithms classify types of injuries or diseases based on imaging data (e.g., X-rays, MRIs).
- Environmental Assessment: Classifies damage to ecosystems or habitats due to natural disasters or human activities.
- Performance Metrics: Â
- Accuracy: Measures the proportion of correctly classified instances.
- Precision and Recall: Evaluate the algorithm's ability to identify relevant instances while minimizing false positives and negatives.
- F1 Score: A balance between precision and recall, providing a single metric for performance evaluation.
- Challenges: Â
- Data Quality: Poor quality data can lead to inaccurate classifications.
- Overfitting: Algorithms may perform well on training data but poorly on unseen data.
- Scalability: Ensuring algorithms can handle large datasets efficiently.
By leveraging damage classification algorithms, organizations can enhance their ability to assess and respond to various types of damage, ultimately improving safety and operational efficiency, and driving better business outcomes, including the management of research data and the use of electronic database management systems.
6.2. Severity Assessment Methods
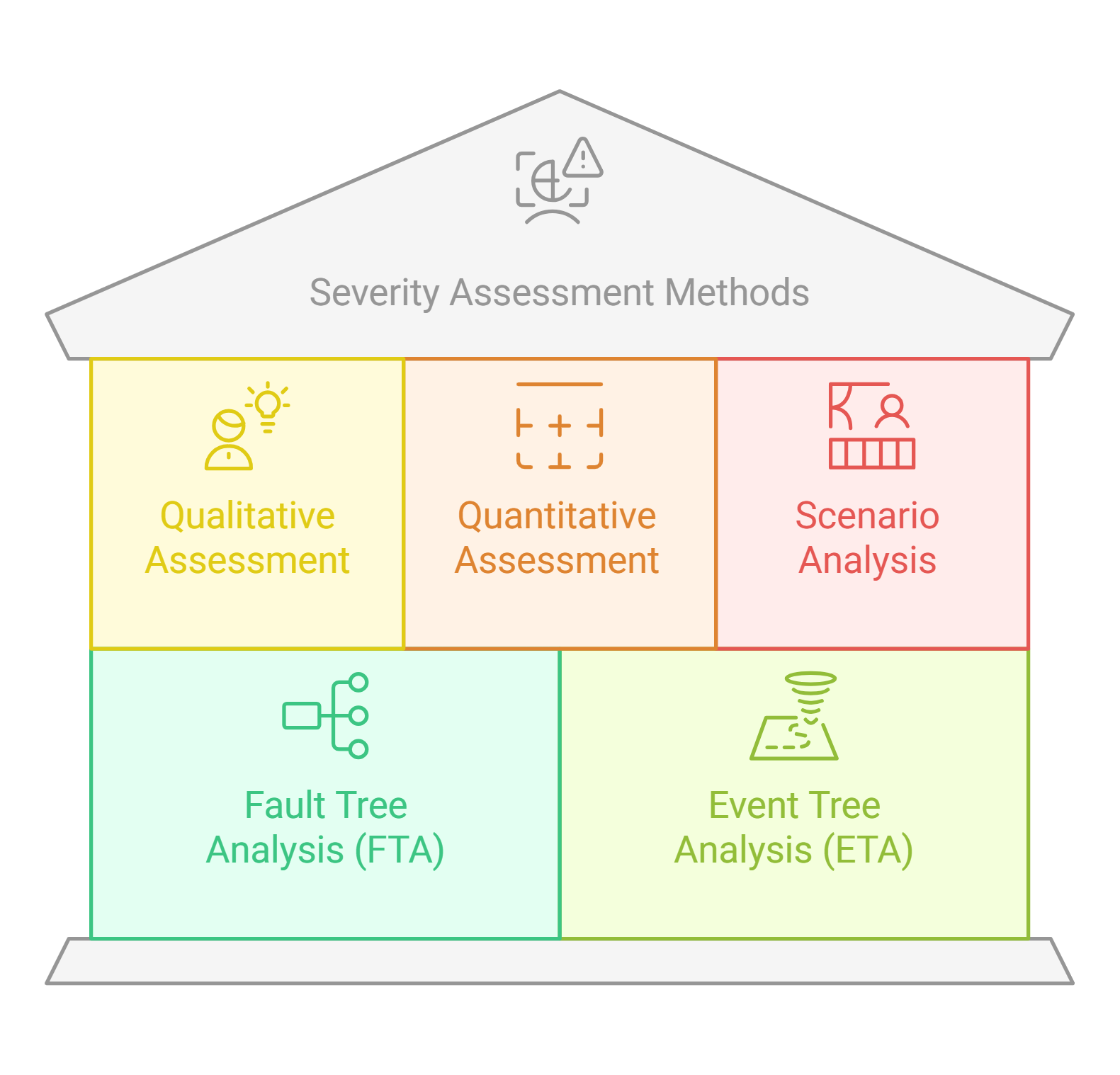
‍
Severity assessment methods are crucial in evaluating the potential impact of risks on projects, systems, or processes. These methods help organizations prioritize risks based on their severity, allowing for more effective risk management strategies.
- Qualitative Assessment: This method involves subjective judgment to categorize risks based on their potential impact and likelihood. Common tools include risk matrices and expert opinions, often utilized in qualitative risk assessment and qualitative risk analysis.
- Quantitative Assessment: This approach uses numerical data to assess risk severity. Techniques such as statistical analysis, simulations, and historical data analysis are employed to quantify risks and their potential impacts, aligning with quantitative risk assessment and quantitative risk analysis.
- Scenario Analysis: This method involves creating detailed scenarios to understand how different risks could affect outcomes. It helps in visualizing the severity of risks under various conditions, which can be enhanced through probabilistic risk assessment techniques.
- Fault Tree Analysis (FTA): FTA is a top-down approach that identifies potential failures and their causes. It helps in assessing the severity of risks by mapping out the relationships between different failure modes, similar to bow tie analysis.
- Event Tree Analysis (ETA): ETA is a bottom-up approach that evaluates the consequences of an initiating event. It helps in understanding the severity of risks by analyzing the potential outcomes of specific events, often supported by risk analysis monte carlo simulations. For more insights on the impact of AI on finance and risk management, check out this article on AI's impact on finance.
6.3. Cost Estimation Models
Cost estimation models are essential for predicting the financial implications of projects and risks. Accurate cost estimation helps organizations allocate resources effectively and make informed decisions.
- Analogous Estimating: This model uses historical data from similar projects to estimate costs. It is quick and easy but may lack precision if the projects differ significantly.
- Parametric Estimating: This method uses statistical relationships between historical data and other variables to estimate costs. It is more accurate than analogous estimating and can be applied to various project types.
- Bottom-Up Estimating: This approach involves estimating costs for individual components of a project and aggregating them to get a total cost. It is time-consuming but provides a detailed and accurate estimate.
- Three-Point Estimation: This model considers uncertainty by providing three estimates: optimistic, pessimistic, and most likely. It helps in understanding the range of potential costs and aids in risk management.
- Monte Carlo Simulation: This advanced technique uses computer algorithms to simulate different scenarios and their financial impacts. It provides a probabilistic analysis of costs, helping organizations prepare for uncertainties.
6.4. Risk Analysis Framework
A risk analysis framework provides a structured approach to identifying, assessing, and managing risks. It helps organizations develop strategies to mitigate risks and enhance decision-making.
- Risk Identification: This initial step involves recognizing potential risks that could impact the project or organization. Techniques include brainstorming sessions, checklists, and expert interviews, which can incorporate financial risk analysis methods.
- Risk Assessment: This phase evaluates the identified risks based on their likelihood and impact. It often employs severity assessment methods to prioritize risks for further analysis, including fmea risk assessment and risk assessment techniques.
- Risk Response Planning: After assessing risks, organizations develop strategies to address them. This may include risk avoidance, mitigation, transfer, or acceptance.
- Risk Monitoring and Review: Continuous monitoring of risks is essential to ensure that risk management strategies remain effective. Regular reviews help in identifying new risks and adjusting strategies accordingly.
- Communication and Reporting: Effective communication of risks and their management strategies is vital. Regular reporting to stakeholders ensures transparency and fosters a culture of risk awareness within the organization.
At Rapid Innovation, we leverage advanced AI and Blockchain technologies to enhance these risk management processes. By integrating AI-driven analytics, we can provide deeper insights into risk severity and cost estimation, enabling organizations to make data-driven decisions that maximize ROI. Our Blockchain solutions ensure transparency and traceability in risk management, fostering trust among stakeholders and enhancing overall project success. For more information on how we can assist with AI project estimation, visit our AI project estimation services.
6.5. Predictive Maintenance Integration
Predictive maintenance integration is a proactive approach that leverages data analytics and machine learning to anticipate equipment failures before they occur. This strategy is essential for organizations aiming to enhance operational efficiency and reduce downtime.
- Utilizes real-time data from sensors and IoT devices to monitor equipment health. Â
- Employs advanced algorithms to analyze historical data and predict potential failures. Â
- Reduces maintenance costs by addressing issues before they escalate into major problems. Â
- Enhances equipment lifespan through timely interventions. Â
- Supports decision-making by providing insights into maintenance schedules and resource allocation. Â
By integrating predictive maintenance into existing systems, organizations can optimize their maintenance strategies, leading to improved productivity and reduced operational risks. This integration often involves collaboration between IT and operational technology teams to ensure seamless data flow and analysis. At Rapid Innovation, we specialize in implementing these advanced predictive maintenance solutions, including IoT predictive maintenance and AWS predictive maintenance, ensuring that our clients achieve greater ROI through reduced downtime and enhanced operational efficiency. Our expertise in enterprise AI development allows us to deliver tailored solutions that meet the unique needs of your organization. Additionally, we provide insights into what AI can do in predictive maintenance.
7. Reporting and Documentation
Effective reporting and documentation are critical components of any maintenance strategy. They provide a clear record of maintenance activities, equipment performance, and compliance with industry standards.
- Ensures transparency in maintenance operations, allowing stakeholders to track progress and outcomes. Â
- Facilitates compliance with regulatory requirements by maintaining accurate records. Â
- Supports continuous improvement by analyzing past maintenance activities and outcomes. Â
- Enhances communication among team members and departments through standardized reporting formats. Â
- Provides valuable insights for future planning and resource allocation. Â
Robust reporting and documentation practices not only improve accountability but also help organizations identify trends and areas for improvement in their maintenance processes.
7.1. Automated Report Generation
Automated report generation streamlines the process of creating maintenance reports, saving time and reducing the potential for human error. This technology is increasingly being adopted in various industries to enhance efficiency and accuracy.
- Generates reports in real-time, providing up-to-date information on equipment status and maintenance activities. Â
- Reduces manual effort, allowing maintenance teams to focus on critical tasks rather than paperwork. Â
- Customizes reports based on specific metrics and KPIs relevant to the organization. Â
- Integrates with existing maintenance management systems for seamless data extraction and reporting. Â
- Enhances data accuracy by minimizing the risk of errors associated with manual data entry. Â
By implementing automated report generation, organizations can ensure that they have timely and accurate information at their fingertips, enabling better decision-making and improved maintenance outcomes. Rapid Innovation's expertise in AI-driven solutions allows us to tailor these automated reporting systems to meet the unique needs of our clients, ultimately driving efficiency and effectiveness in their operations.
7.2. Visual Documentation
Visual documentation services are a critical component in various fields, including construction, insurance, and environmental monitoring. It involves capturing images, videos, and other visual media to provide a clear representation of a situation, project, or condition.
- Enhances communication: Visual documentation helps convey complex information quickly and effectively. It allows stakeholders to understand the context without needing extensive explanations, thereby streamlining project discussions and decision-making.
- Provides evidence: In legal and insurance contexts, visual documentation services serve as crucial evidence. It can substantiate claims, demonstrate compliance, or illustrate the state of a project at a specific time, ensuring that all parties have a clear understanding of the facts.
- Facilitates monitoring: Regular visual documentation can track changes over time, making it easier to identify trends, issues, or improvements. This is particularly useful in construction projects where progress needs to be monitored closely, allowing for timely interventions and adjustments.
- Supports training: Visual aids can be used in training sessions to illustrate best practices, safety protocols, or operational procedures, enhancing learning and retention. This can lead to a more skilled workforce and reduced operational risks.
7.3. Damage Progression Tracking
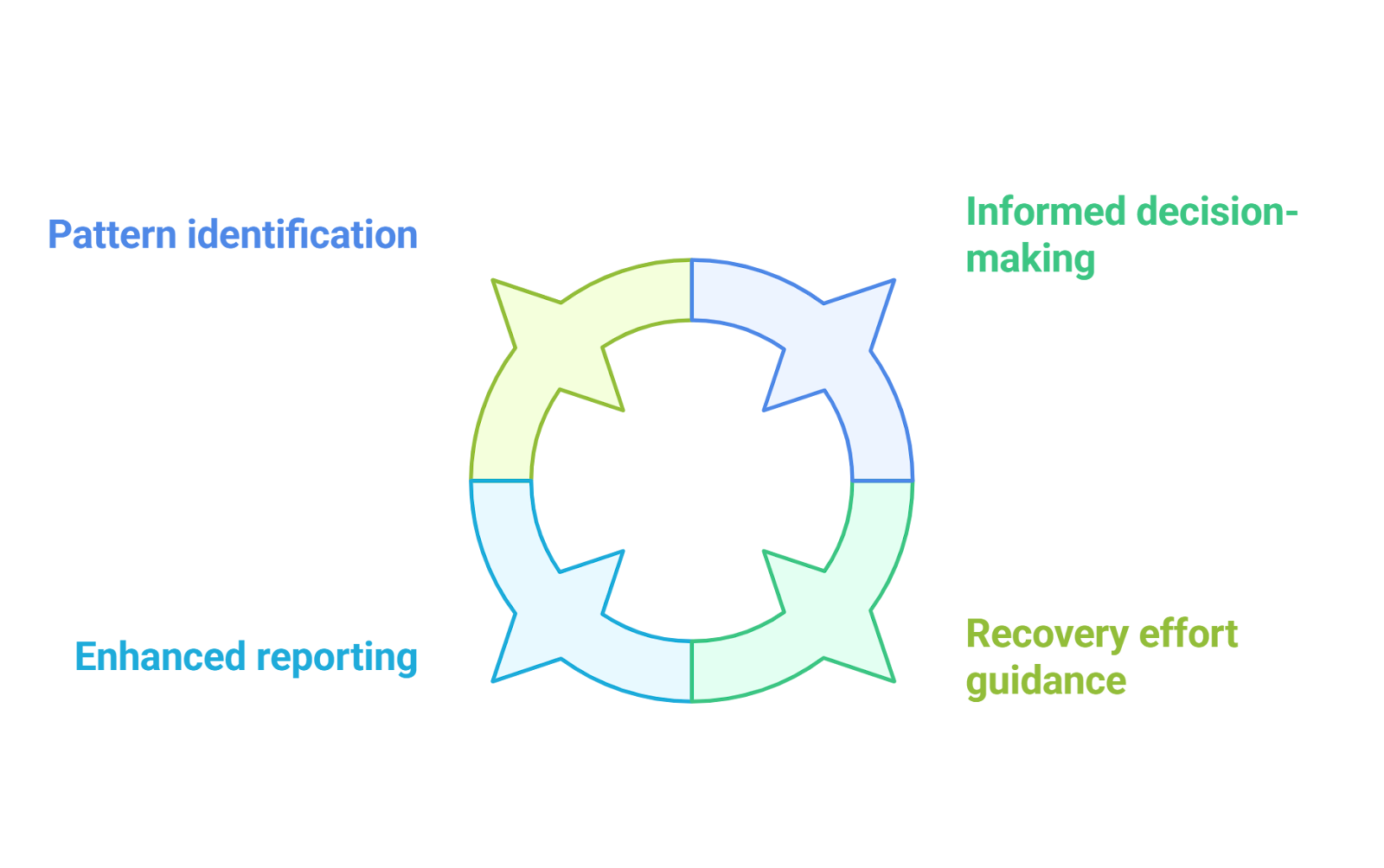
‍
Damage progression tracking is essential for assessing the impact of incidents, whether they are natural disasters, accidents, or wear and tear over time. This process involves systematically documenting the extent and nature of damage as it evolves.
- Identifies patterns: By tracking damage progression, organizations can identify patterns that may indicate underlying issues, such as structural weaknesses or recurring environmental impacts. This proactive approach can lead to more effective maintenance strategies.
- Informs decision-making: Accurate tracking allows for informed decisions regarding repairs, resource allocation, and risk management. It helps prioritize actions based on the severity and urgency of the damage, ultimately leading to more efficient use of resources.
- Aids in recovery efforts: In the aftermath of a disaster, tracking damage progression can guide recovery efforts, ensuring that resources are directed where they are most needed. This can significantly reduce downtime and associated costs.
- Enhances reporting: Detailed records of damage progression can improve reporting to stakeholders, including insurance companies, regulatory bodies, and the public, fostering transparency and trust. This can also facilitate smoother claims processes and regulatory compliance.
7.4. Compliance Documentation
Compliance documentation is vital for organizations to demonstrate adherence to laws, regulations, and industry standards. This documentation serves as proof that an organization is operating within the legal framework and following best practices.
- Ensures accountability: Proper compliance documentation holds organizations accountable for their actions. It provides a clear record of policies, procedures, and actions taken to meet regulatory requirements, which is essential for maintaining stakeholder confidence.
- Facilitates audits: Well-organized compliance documentation simplifies the audit process. It allows auditors to quickly verify compliance and identify any areas needing improvement, thereby reducing the time and resources spent on audits.
- Reduces risk: By maintaining thorough compliance documentation, organizations can mitigate risks associated with non-compliance, such as fines, legal issues, and reputational damage. This proactive approach can lead to significant cost savings.
- Supports continuous improvement: Regularly reviewing compliance documentation can highlight areas for improvement, enabling organizations to enhance their processes and stay ahead of regulatory changes. This commitment to improvement can also enhance overall operational efficiency and effectiveness.
At Rapid Innovation, we leverage advanced AI and Blockchain technologies to enhance these visual documentation services and other documentation processes, ensuring that our clients achieve greater ROI through improved efficiency, accuracy, and compliance. By integrating AI-driven analytics and Blockchain's immutable record-keeping, we empower organizations to make data-driven decisions, streamline operations, and maintain transparency, ultimately driving business success.
7.5. Stakeholder Communication
Effective stakeholder communication is crucial for the success of any project or organization. It involves the systematic dissemination of information to all parties involved, ensuring that everyone is informed and engaged.
- Clear messaging: Use straightforward language to convey messages. Avoid jargon that may confuse stakeholders.
- Regular updates: Schedule consistent updates to keep stakeholders informed about project progress, changes, and challenges.
- Feedback mechanisms: Establish channels for stakeholders to provide feedback. This can include surveys, meetings, or digital platforms.
- Tailored communication: Different stakeholders may require different types of information. Customize your communication strategy to meet their needs, especially when addressing key stakeholders in a project.
- Transparency: Be open about challenges and setbacks. This builds trust and encourages collaboration, particularly in stakeholder public relations.
- Use of technology: Leverage tools like email newsletters, project management software, and social media to enhance communication with stakeholders in project management.
- Documentation: Keep records of all communications for future reference and accountability, which is essential in stakeholder management communication.
Effective stakeholder communication not only fosters a positive relationship but also enhances project outcomes by ensuring that all parties are aligned and informed. At Rapid Innovation, we utilize advanced AI-driven communication tools to streamline stakeholder interactions, ensuring that our clients receive timely updates and feedback, ultimately leading to improved project efficiency and ROI. This approach is particularly beneficial when identifying key stakeholders examples and understanding their roles in communication.
8. Integration with Business Systems
Integrating various business systems is essential for streamlining operations and improving efficiency. This process involves connecting different software applications and databases to work together seamlessly.
- Improved data accuracy: Integration reduces the chances of errors that can occur when data is manually transferred between systems.
- Enhanced collaboration: Teams can work more effectively when systems are interconnected, allowing for real-time data sharing.
- Cost savings: By automating processes through integration, organizations can reduce operational costs and improve resource allocation.
- Scalability: Integrated systems can easily adapt to changing business needs, allowing for growth without significant overhauls.
- Better decision-making: Access to consolidated data enables informed decision-making, as stakeholders can analyze comprehensive information.
- Customer experience: Integration can lead to improved customer service by providing a unified view of customer interactions across platforms.
Investing in system integration can lead to significant long-term benefits, making it a critical component of modern business strategy. Rapid Innovation specializes in integrating AI and blockchain technologies into existing business systems, enhancing operational efficiency and driving greater ROI for our clients.
8.1. Claims Management Systems
Claims management systems are specialized software solutions designed to streamline the process of handling claims, particularly in industries like insurance and healthcare. These systems automate and manage the entire claims lifecycle.
- Automation: Claims management systems automate repetitive tasks, reducing the time and effort required to process claims.
- Improved accuracy: By minimizing manual data entry, these systems help reduce errors and ensure that claims are processed correctly.
- Enhanced tracking: Users can easily track the status of claims in real-time, providing transparency and accountability.
- Data analytics: Advanced claims management systems offer analytics tools that help organizations identify trends and improve decision-making.
- Compliance: These systems help ensure that claims processing adheres to industry regulations and standards, reducing the risk of non-compliance.
- Customer satisfaction: By speeding up the claims process and improving communication, organizations can enhance customer satisfaction and loyalty.
Implementing a robust claims management system can significantly improve operational efficiency and customer experience, making it a vital investment for organizations dealing with claims. Rapid Innovation's expertise in AI and blockchain can further enhance these systems, ensuring secure, efficient, and transparent claims processing.
8.2. Enterprise Resource Planning

‍
Enterprise Resource Planning (ERP) systems are integrated software solutions that help organizations manage and automate core business processes. They provide a unified platform for various functions, including finance, human resources, supply chain, and manufacturing.
- Streamlined Operations: ERP systems centralize data, allowing for improved communication and collaboration across departments. This leads to more efficient workflows and reduced operational costs. At Rapid Innovation, we leverage AI algorithms to optimize these workflows further, ensuring that your organization can adapt quickly to changing demands.
- Real-time Data Access: With ERP, businesses can access real-time data analytics, enabling informed decision-making and timely responses to market changes. Our expertise in AI-driven analytics enhances this capability, providing predictive insights that empower your business to stay ahead of the competition.
- Scalability: ERP solutions can grow with a business, accommodating increased data volume and additional functionalities as needed. Rapid Innovation ensures that your ERP system is not only scalable but also integrates seamlessly with emerging technologies, including blockchain for enhanced security and transparency.
- Compliance and Risk Management: ERP systems often include features that help organizations comply with industry regulations and manage risks effectively. Our consulting services can guide you in implementing blockchain solutions that provide immutable records, further strengthening your compliance efforts.
- Enhanced Reporting: Advanced reporting tools within ERP systems allow for detailed insights into business performance, helping to identify trends and areas for improvement. By incorporating AI, we can help you automate reporting processes, saving time and resources while delivering actionable insights.
Incorporating enterprise resource planning systems, enterprise resource management, and ERP software into your operations can significantly enhance your business efficiency and effectiveness. By utilizing enterprise resource planning software, organizations can streamline their processes and improve overall performance. Additionally, our AI in customer support solutions can further enhance your customer interactions and support capabilities.
8.5. Third-party Integration
Third-party integration refers to the process of connecting external applications, services, or platforms with your existing systems to enhance functionality and streamline operations. This integration is crucial for businesses looking to leverage additional features without developing everything in-house.
- Benefits of Third-party Integration  Â
- Enhanced Functionality: Integrating with third-party tools can provide advanced features such as payment processing, customer relationship management (CRM), and analytics. Â
- Cost Efficiency: Utilizing existing solutions can save time and resources compared to building custom software from scratch. Â
- Scalability: Third-party services often offer scalable solutions that can grow with your business needs. Â
- Focus on Core Competencies: By integrating with specialized services, businesses can focus on their core functions while relying on experts for other areas. Â
- Common Third-party Integrations  Â
- Payment Gateways: Services like PayPal, Stripe, and Square allow businesses to process transactions securely. Â
- CRM Systems: Tools like Salesforce and HubSpot help manage customer relationships and sales processes, including salesforce third party integration and salesforce integration with third party applications. Â
- Marketing Automation: Platforms such as Mailchimp and Marketo enable businesses to automate marketing campaigns and track performance. Â
- Considerations for Integration  Â
- Compatibility: Ensure that the third-party service is compatible with your existing systems, such as third party api integration and third party software integration. Â
- Security: Evaluate the security measures of the third-party provider to protect sensitive data. Â
- Support and Maintenance: Consider the level of support offered by the third-party service for troubleshooting and updates. Â
9. Challenges and Solutions
While third-party integration offers numerous benefits, it also presents challenges that businesses must navigate. Identifying these challenges and implementing effective solutions is essential for successful integration.
- Common Challenges  Â
- Data Security: Integrating with external services can expose sensitive data to potential breaches. Â
- System Compatibility: Different systems may not communicate effectively, leading to integration issues, such as those encountered in third party integration examples. Â
- Vendor Reliability: Relying on third-party services means depending on their uptime and performance. Â
- Solutions to Overcome Challenges  Â
- Conduct Thorough Research: Before integrating, research potential third-party providers to assess their reliability and security measures, including third party integration services. Â
- Implement Robust Security Protocols: Use encryption and secure APIs to protect data during transmission, especially when dealing with api for third party integration. Â
- Regular Monitoring and Maintenance: Continuously monitor the integration for performance issues and conduct regular updates to ensure compatibility. Â
9.1. Technical Challenges
Technical challenges are often the most complex aspects of third-party integration. These challenges can arise from various factors, including system architecture, data formats, and network issues.
- Common Technical Challenges  Â
- API Limitations: Many third-party services have limitations on their APIs, which can restrict functionality, as seen in third party api limitations. Â
- Data Format Incompatibility: Different systems may use varying data formats, making it difficult to exchange information seamlessly, particularly in third party app integration. Â
- Latency Issues: Integrating with external services can introduce latency, affecting the performance of your application. Â
- Solutions to Technical Challenges  Â
- Use Middleware Solutions: Middleware can help bridge the gap between different systems, facilitating smoother data exchange, such as in workday cloud connect for third party payroll. Â
- Standardize Data Formats: Implementing standard data formats (like JSON or XML) can help ensure compatibility between systems, including integration with third party api. Â
- Optimize Network Performance: Invest in network infrastructure to reduce latency and improve the speed of data transfer. Â
By addressing these technical challenges proactively, businesses can ensure a smoother integration process and maximize the benefits of third-party services, including sap integration with third party software and jira third party integration.
At Rapid Innovation, we specialize in facilitating seamless third-party integrations tailored to your business needs. Our expertise in AI and Blockchain technologies allows us to enhance your operational efficiency and drive greater ROI through strategic integrations. Whether it's implementing secure payment gateways or optimizing CRM systems like salesforce third party integration or hubspot third party integrations, we ensure that your integrations are not only effective but also secure and scalable. For more information on AI as a Service.
9.1.1. Accuracy and Reliability
Accuracy and reliability are critical components in any system, especially in fields such as data analysis, machine learning, and artificial intelligence. These factors determine how well a system performs its intended functions and how trustworthy its outputs are.
- Accuracy refers to the closeness of a measured value to a standard or known value. In data-driven systems, high accuracy means that the predictions or classifications made by the system closely match the actual outcomes.
- Reliability, on the other hand, is the consistency of a system's performance over time. A reliable system produces stable results under varying conditions and can be depended upon to function correctly.
- Factors affecting accuracy and reliability include: Â
- Quality of input data: Poor quality or biased data can lead to inaccurate results.
- Algorithm effectiveness: The choice of algorithms and models can significantly impact performance.
- Environmental conditions: External factors such as hardware limitations or network issues can affect system reliability.
At Rapid Innovation, we emphasize the importance of system accuracy and reliability in our AI and blockchain solutions. By leveraging advanced data preprocessing techniques and selecting the most effective algorithms, we ensure that our systems deliver high-quality outputs. Our rigorous testing and validation processes help clients achieve greater ROI by minimizing errors and enhancing decision-making capabilities.
9.1.2. Edge Cases
Edge cases refer to scenarios that occur at the extreme ends of input ranges or conditions, which may not be well-handled by a system. These cases are crucial to consider during the design and testing phases, as they can reveal vulnerabilities or limitations in the system.
- Edge cases can include:
- Unusual input values: Inputs that are at the maximum or minimum limits of what the system is designed to handle.
- Rare events: Situations that occur infrequently but can have significant impacts on system performance.
- Unexpected user behavior: Actions taken by users that deviate from the norm, potentially leading to system failures.
Addressing edge cases is vital for several reasons: - They help identify weaknesses in the system that could lead to failures or inaccuracies. - Testing for edge cases can improve the robustness of the system, ensuring it performs well under a variety of conditions. - Understanding edge cases can enhance user experience by preventing frustrating errors or crashes.
At Rapid Innovation, we incorporate edge case testing into our development processes, ensuring that our AI and blockchain solutions can handle a wide range of scenarios effectively. This proactive approach not only enhances system reliability but also contributes to a better user experience, ultimately driving higher ROI for our clients.
9.1.3. System Limitations
Every system has inherent limitations that can affect its performance and usability. Understanding these limitations is essential for users and developers alike, as it helps set realistic expectations and guides future improvements.
- Common system limitations include:
- Processing power: Limited computational resources can restrict the complexity of tasks a system can perform.
- Data constraints: Insufficient or low-quality data can hinder the system's ability to learn and make accurate predictions.
- Algorithmic constraints: Some algorithms may not be suitable for certain types of data or problems, leading to suboptimal performance.
Recognizing system limitations is important for several reasons: - It helps users understand the boundaries of what the system can achieve, preventing misuse or over-reliance on its capabilities. - Developers can prioritize areas for improvement, focusing on enhancing performance where it is most needed. - Awareness of limitations can guide users in making informed decisions about how to integrate the system into their workflows.
By acknowledging and addressing system limitations, organizations can better leverage technology to meet their goals while minimizing risks associated with overestimating capabilities. At Rapid Innovation, we work closely with our clients to identify these limitations and develop tailored solutions that maximize their potential for success.
9.2. Implementation Challenges
Implementing new systems, processes, or technologies often comes with a set of challenges that organizations must navigate, such as challenges implementing electronic health records and challenges in implementing ehr. These challenges can hinder the effectiveness of the implementation and impact overall productivity. Understanding these challenges is crucial for successful implementation.
- Resistance to change from employees
- Lack of adequate resources
- Insufficient planning and strategy
- Technical difficulties and integration issues, including emr implementation challenges
- Inadequate communication throughout the organization
9.2.1. Training Requirements
Training is a critical component of any implementation process. Without proper training, employees may struggle to adapt to new systems or processes, leading to decreased efficiency and productivity. To ensure effective training:
- Identify specific training needs based on the new system or process.
- Develop a comprehensive training program that includes:
- Hands-on workshops
- Online tutorials
- User manuals and documentation
- Schedule training sessions to accommodate all employees.
- Utilize experienced trainers or external consultants to enhance learning.
- Monitor and evaluate the effectiveness of training through feedback and assessments.
Investing in training not only helps employees feel more confident but also ensures that the organization maximizes the benefits of the new implementation. At Rapid Innovation, we leverage our expertise in AI and Blockchain to create tailored training programs that empower your workforce, ensuring they are well-equipped to utilize new technologies effectively.
9.2.2. Change Management
Change management is essential for guiding an organization through the transition period associated with new implementations. Effective change management strategies can minimize resistance and foster a culture of adaptability. Key strategies include:
- Communicate the reasons for the change clearly to all stakeholders.
- Involve employees in the change process to gain their buy-in.
- Establish a change management team to oversee the implementation.
- Provide ongoing support and resources to help employees adjust.
- Monitor the progress of the implementation and make adjustments as needed.
By prioritizing change management, organizations can create a smoother transition, reduce anxiety among employees, and ultimately achieve a successful implementation. Rapid Innovation specializes in developing change management frameworks that align with your business goals, ensuring that the integration of AI and Blockchain technologies is seamless and effective, leading to greater ROI.
Additionally, organizations may face barriers to implementing ehr, software implementation challenges, and specific challenges related to workday implementation challenges, sap implementation challenges, oracle cloud implementation challenges, and s4 hana implementation challenges. Understanding these various implementation challenges, including the top 10 ehr implementation challenges and how to overcome them, is vital for a successful transition. For more insights on overcoming these challenges, check out the potential of business AI engineering best practices.
9.2.3. Cost Considerations
Cost considerations are crucial in any business decision-making process. Understanding the financial implications of a project or investment can significantly influence its success. Here are some key aspects to consider:
- Initial Investment: Assess the upfront costs required for the project, including equipment, technology, and human resources. This initial outlay can impact cash flow and budgeting. At Rapid Innovation, we help clients identify the most cost-effective technologies, particularly in AI and Blockchain, to optimize their initial investments.
- Operational Costs: Evaluate ongoing expenses such as maintenance, utilities, and staffing. These costs can accumulate over time and affect profitability. Our solutions often include automation and smart contracts that can reduce operational costs significantly.
- Return on Investment (ROI): Calculate the expected ROI to determine if the project is financially viable. A positive ROI indicates that the benefits outweigh the costs. Rapid Innovation employs advanced analytics to project ROI accurately, ensuring our clients make informed decisions.
- Hidden Costs: Be aware of potential hidden costs, such as regulatory fees, training expenses, or unexpected repairs. These can significantly alter the overall budget. We assist clients in identifying and mitigating these hidden costs through comprehensive project assessments.
- Cost-Benefit Analysis: Conduct a thorough cost-benefit analysis to weigh the financial pros and cons of the project. This analysis helps in making informed decisions. Our team utilizes AI-driven tools to enhance the accuracy of these analyses.
- Funding Sources: Explore various funding options, including loans, grants, or investor contributions. Understanding available financial resources can ease the burden of initial costs. Rapid Innovation can connect clients with potential investors interested in AI and Blockchain projects.
- Long-term Financial Planning: Consider the long-term financial implications of the project. Sustainable financial planning can help ensure the project's success over time. We guide clients in developing strategies that align with their long-term business goals, leveraging AI and Blockchain for sustained growth. For more insights on AI applications in legal contexts, check out our AI agents for legal applications.
9.3. Legal and Ethical Considerations
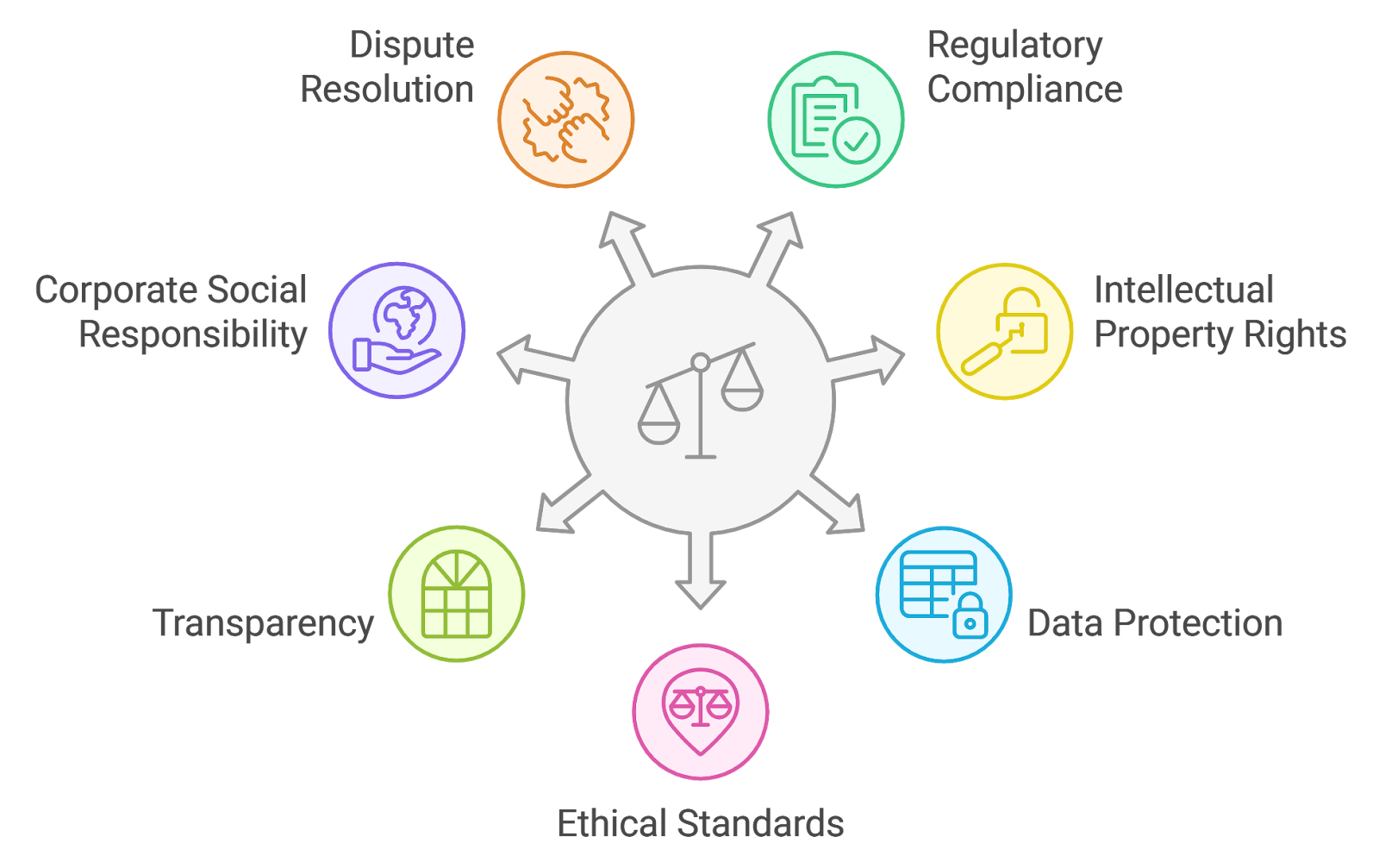
‍
Legal and ethical considerations are paramount in any business operation. They ensure compliance with laws and regulations while maintaining a company's integrity. Here are some essential points to consider:
- Regulatory Compliance: Ensure that all business practices adhere to local, state, and federal regulations. Non-compliance can lead to legal penalties and damage to reputation.
- Intellectual Property Rights: Protect intellectual property through patents, trademarks, and copyrights. This safeguards innovations and prevents unauthorized use.
- Data Protection: Implement robust data protection measures to comply with privacy laws, such as GDPR. Protecting customer data is not only a legal requirement but also builds trust.
- Ethical Standards: Establish a code of ethics that guides employee behavior and decision-making. This promotes a culture of integrity and accountability.
- Transparency: Maintain transparency in business operations, especially in financial reporting and communications with stakeholders. This fosters trust and credibility.
- Corporate Social Responsibility (CSR): Engage in CSR initiatives that benefit the community and environment. Ethical business practices can enhance brand reputation and customer loyalty.
- Dispute Resolution: Have a clear process for resolving disputes, whether with employees, customers, or suppliers. This can prevent legal issues and maintain positive relationships.
9.3.1. Liability Issues
Liability issues are a significant concern for businesses, as they can lead to costly legal battles and damage to reputation. Understanding these issues is essential for risk management. Here are some key points to consider:
- Types of Liability: Familiarize yourself with different types of liability, including product liability, professional liability, and general liability. Each type has specific legal implications.
- Insurance Coverage: Invest in appropriate insurance coverage to protect against potential liabilities. This can include general liability insurance, professional liability insurance, and workers' compensation.
- Negligence: Understand the concept of negligence, which occurs when a party fails to exercise reasonable care, leading to harm. Businesses must take proactive measures to prevent negligence claims.
- Contractual Liability: Be aware of contractual obligations and the potential liabilities that arise from breaching contracts. Clear contracts can help mitigate risks.
- Employee Training: Provide training to employees on safety protocols and legal compliance. Well-informed employees can help reduce the risk of liability claims.
- Legal Counsel: Consult with legal professionals to navigate complex liability issues. Having expert advice can help in making informed decisions and minimizing risks.
- Crisis Management: Develop a crisis management plan to address potential liability issues swiftly. Being prepared can mitigate damage and protect the company's reputation.
9.3.2. Privacy Concerns
Privacy concerns are increasingly significant in today's digital landscape, especially with the rise of data-driven technologies. As organizations collect vast amounts of personal data, the potential for misuse or unauthorized access grows. Key aspects of privacy concerns include:
- Data Breaches: High-profile data breaches have exposed sensitive information, leading to financial loss and reputational damage for companies. According to a report, the average cost of a data breach is approximately $4.24 million (source: IBM). Rapid Innovation can help mitigate these risks by implementing robust AI-driven security measures that proactively identify vulnerabilities and protect sensitive data.
- User Consent: Many users are unaware of how their data is collected and used. Clear consent mechanisms are essential to ensure users understand what they are agreeing to when providing personal information. Our blockchain solutions can enhance transparency in data handling, allowing users to track how their data is used and providing them with greater control.
- Surveillance: The increasing use of surveillance technologies raises ethical questions about privacy. Many individuals feel uncomfortable with constant monitoring, whether through social media, smart devices, or public surveillance systems. Rapid Innovation advocates for ethical AI practices that prioritize user privacy while still enabling organizations to leverage data for insights.
- Data Minimization: Organizations are encouraged to adopt data minimization practices, collecting only the data necessary for their operations. This approach not only protects user privacy but also reduces the risk of data breaches. Our consulting services can guide businesses in developing data strategies that align with these principles, ensuring compliance with GDPR requirements and enhancing user trust.
- Anonymization: Techniques such as data anonymization can help protect individual identities while still allowing organizations to analyze trends and patterns in data. Rapid Innovation employs advanced AI algorithms to anonymize data effectively, enabling businesses to gain insights without compromising user privacy.
9.3.3. Regulatory Compliance
Regulatory compliance is crucial for organizations handling personal data. Various laws and regulations govern how data should be collected, stored, and processed. Key points regarding regulatory compliance include:
- GDPR: The General Data Protection Regulation (GDPR) is a comprehensive data protection law in the European Union. It mandates strict guidelines for data collection and processing, emphasizing user consent and the right to access personal data. Rapid Innovation assists clients in navigating GDPR compliance through tailored solutions that ensure adherence to these regulations, including GDPR compliance services.
- CCPA: The California Consumer Privacy Act (CCPA) provides California residents with rights regarding their personal information. It requires businesses to disclose data collection practices and allows users to opt-out of data selling. Our expertise in blockchain technology can help organizations implement transparent data practices that align with CCPA requirements, ensuring compliance with both CCPA and GDPR.
- Fines and Penalties: Non-compliance with data protection regulations can result in significant fines. For instance, GDPR violations can lead to fines of up to €20 million or 4% of a company's global annual revenue, whichever is higher. Rapid Innovation's proactive compliance strategies can help mitigate these risks and protect your bottom line.
- Data Protection Officers: Many organizations are required to appoint Data Protection Officers (DPOs) to oversee compliance efforts and ensure that data handling practices align with legal requirements. Our consulting services can assist in establishing effective DPO roles and responsibilities within your organization, ensuring compliance with data protection acts.
- Regular Audits: Conducting regular audits helps organizations identify compliance gaps and implement necessary changes to adhere to regulations. Rapid Innovation offers comprehensive audit services that leverage AI to streamline the compliance assessment process, ensuring adherence to GDPR compliance regulations.
10. Future Developments
The future of data privacy and regulatory compliance is likely to evolve significantly as technology advances. Anticipated developments include:
- Enhanced AI Regulations: As artificial intelligence becomes more prevalent, regulations will likely focus on ethical AI use, ensuring transparency and accountability in automated decision-making processes. Rapid Innovation is committed to leading the charge in developing AI solutions that prioritize ethical considerations.
- Increased User Control: Future regulations may empower users with greater control over their data, allowing them to manage permissions and access more easily. Our blockchain solutions can facilitate this empowerment by providing users with secure, decentralized access to their personal information, aligning with personal information compliance standards.
- Global Standards: The push for global data protection standards may lead to harmonized regulations across countries, simplifying compliance for multinational organizations. Rapid Innovation stays ahead of regulatory trends to help clients navigate these changes effectively, including compliance with GDPR and CCPA.
- Privacy-First Technologies: Innovations in privacy-first technologies, such as decentralized data storage and blockchain, may provide users with more secure ways to manage their personal information. Rapid Innovation specializes in developing these technologies, ensuring that our clients can offer enhanced privacy protections to their users.
- Evolving Threat Landscape: As cyber threats continue to evolve, organizations will need to adapt their security measures and compliance strategies to protect against new vulnerabilities. Our AI-driven security solutions are designed to evolve alongside these threats, providing ongoing protection for your data.
- Focus on Ethical Data Use: There will likely be a growing emphasis on ethical data use, with organizations being held accountable for how they leverage personal information for business purposes. Rapid Innovation is dedicated to fostering ethical practices in AI and blockchain development, helping clients build trust with their users while achieving their business goals, including compliance with GDPR and CCPA.
10.1. Emerging Technologies
Emerging technologies are reshaping industries and driving innovation across various sectors. These technologies are characterized by their potential to disrupt existing markets and create new opportunities.
- Artificial Intelligence (AI) and Machine Learning (ML): AI and ML are transforming how businesses operate by enabling data-driven decision-making. They enhance customer experiences through personalized recommendations and automate routine tasks, increasing efficiency. At Rapid Innovation, we leverage AI and ML to develop tailored solutions that help clients optimize their operations, leading to significant cost savings and improved ROI. New AI technology and advancements in artificial intelligence are at the forefront of this transformation.
- Blockchain: Originally developed for cryptocurrencies, blockchain technology is now being utilized in supply chain management, healthcare, and finance. Its decentralized nature ensures transparency and security, making it ideal for tracking transactions and ensuring data integrity. Rapid Innovation assists clients in implementing blockchain solutions that enhance trust and accountability in their operations, ultimately driving greater customer satisfaction and loyalty.
- Internet of Things (IoT): IoT connects devices and systems, allowing for real-time data collection and analysis. This technology is pivotal in smart homes, industrial automation, and healthcare, where it can monitor patient health and optimize resource usage. Our expertise in IoT enables clients to harness data effectively, leading to improved operational efficiency and better decision-making. Emerging technologies in IoT are paving the way for smarter solutions.
- Augmented Reality (AR) and Virtual Reality (VR): AR and VR are revolutionizing training, education, and entertainment. They provide immersive experiences that enhance learning and engagement, making them valuable tools in various industries. Rapid Innovation develops AR and VR applications that help clients create engaging customer experiences, resulting in increased brand loyalty and sales. New tech technologies in AR and VR are enhancing user experiences. For more insights on the future of these technologies, check out this article on AR vs VR: Which Reality Will Define Tomorrow.
- Quantum Computing: Although still in its infancy, quantum computing promises to solve complex problems much faster than traditional computers. This technology could revolutionize fields such as cryptography, drug discovery, and materials science. At Rapid Innovation, we are exploring quantum computing applications that can provide our clients with a competitive edge in their respective markets. Emerging technologies in quantum computing are set to change the landscape of computing.
10.2. Industry Trends
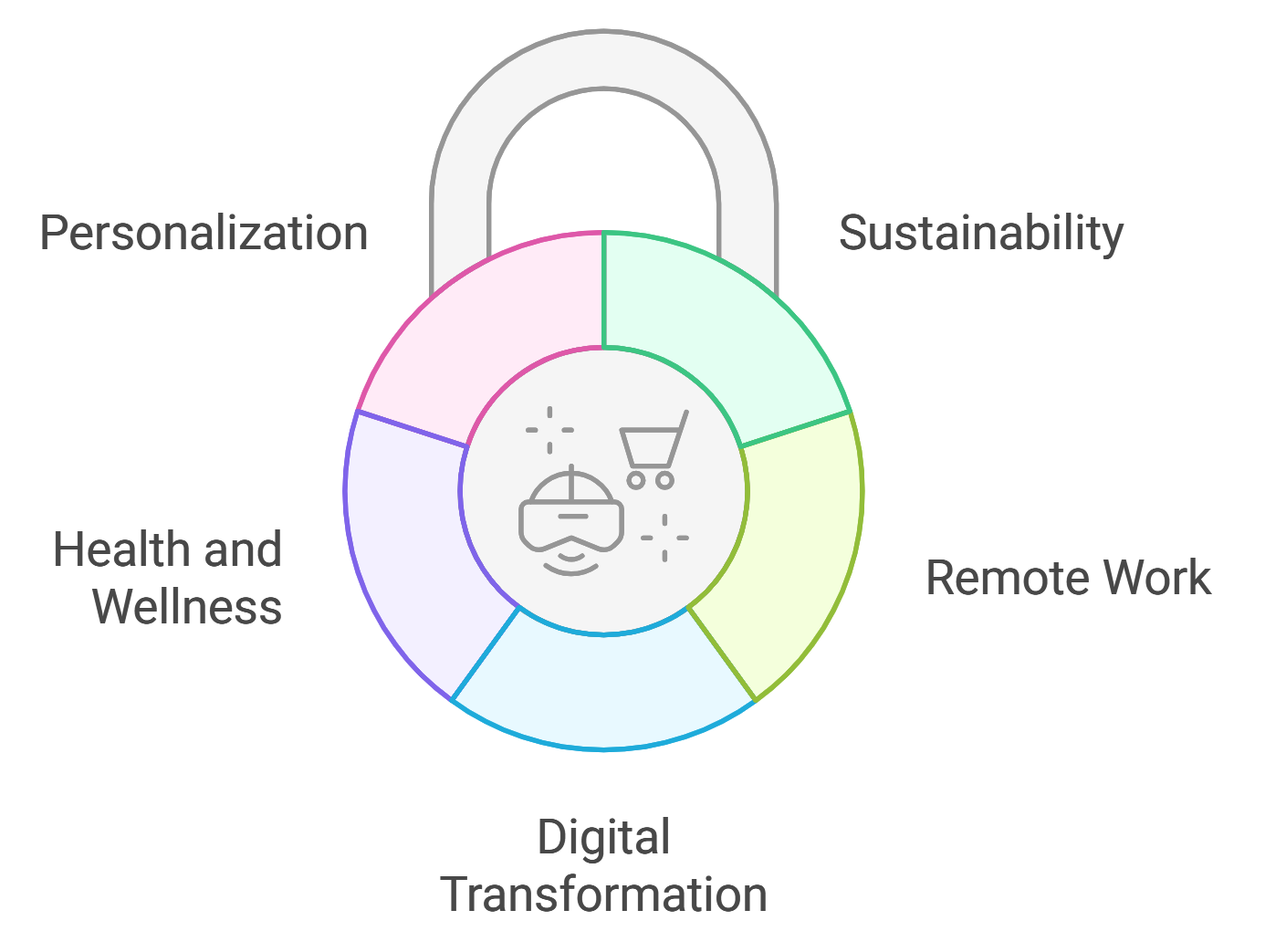
‍
Industry trends reflect the evolving landscape of business practices and consumer behavior. Staying informed about these trends is crucial for organizations aiming to remain competitive.
- Sustainability: There is a growing emphasis on sustainable practices across industries. Companies are adopting eco-friendly materials, reducing waste, and implementing energy-efficient processes to meet consumer demand for responsible products. Rapid Innovation helps clients integrate sustainable technologies into their operations, enhancing their brand reputation and attracting environmentally conscious consumers.
- Remote Work: The COVID-19 pandemic accelerated the shift to remote work. Many organizations are now adopting hybrid work models, allowing employees to work from home while maintaining some in-office presence. This trend is reshaping workplace culture and employee engagement. Our solutions facilitate seamless collaboration and communication, ensuring that businesses can thrive in this new work environment.
- Digital Transformation: Businesses are increasingly investing in digital technologies to enhance operations and customer experiences. This includes adopting cloud computing, e-commerce platforms, and advanced analytics to drive growth and efficiency. Rapid Innovation partners with clients to implement digital transformation strategies that streamline processes and improve overall performance. The latest technologies are crucial in this transformation.
- Health and Wellness: The focus on health and wellness has intensified, with consumers seeking products and services that promote well-being. This trend is evident in the rise of fitness apps, mental health resources, and organic food options. We assist clients in developing health-focused solutions that cater to this growing market, driving customer engagement and loyalty. Emerging technologies in healthcare are playing a significant role in this trend.
- Personalization: Consumers expect personalized experiences tailored to their preferences. Companies are leveraging data analytics to deliver targeted marketing, customized products, and individualized customer service. Rapid Innovation employs advanced analytics and AI to help clients create personalized experiences that resonate with their customers, leading to increased sales and customer retention.
10.3. Research Directions
Research directions guide the exploration of new ideas and innovations in various fields. Identifying key areas for research can lead to breakthroughs and advancements.
- Interdisciplinary Research: Combining knowledge from different fields can lead to innovative solutions. For example, integrating AI with healthcare research can improve diagnostics and treatment options. Rapid Innovation fosters interdisciplinary collaboration to drive innovation and create impactful solutions for our clients.
- Cybersecurity: As technology advances, so do the threats to data security. Research in cybersecurity focuses on developing robust systems to protect sensitive information from cyberattacks and data breaches. We prioritize cybersecurity in our solutions, ensuring that our clients' data remains secure and their operations uninterrupted.
- Renewable Energy: With the urgent need to combat climate change, research in renewable energy sources such as solar, wind, and bioenergy is critical. Innovations in energy storage and efficiency are also key areas of focus. Rapid Innovation supports clients in adopting renewable energy technologies, helping them reduce their carbon footprint and operational costs.
- Biotechnology: Advances in biotechnology are paving the way for breakthroughs in medicine, agriculture, and environmental sustainability. Research in gene editing, synthetic biology, and personalized medicine holds great promise for the future. Our expertise in biotechnology enables us to assist clients in developing innovative solutions that address pressing challenges in health and sustainability.
- Social Impact: Research that examines the social implications of technology is increasingly important. Understanding how emerging technologies affect society can guide ethical practices and policy development. Rapid Innovation is committed to ensuring that our solutions not only drive business success but also contribute positively to society. Emerging technologies in social impact are essential for responsible innovation.
10.4. Potential Applications
The potential applications of various technologies and methodologies are vast and varied, impacting numerous sectors. Understanding these applications can help organizations leverage them effectively.
- Healthcare: Technologies like telemedicine and AI-driven diagnostics are revolutionizing patient care by enabling remote consultations and faster diagnosis, which improves patient outcomes. Rapid Innovation can assist healthcare providers in integrating AI solutions that enhance patient engagement and streamline operations, ultimately leading to improved ROI.
- Education: E-learning platforms and virtual classrooms are making education more accessible, allowing for personalized learning experiences that cater to diverse learning styles. By leveraging AI, Rapid Innovation can help educational institutions create adaptive learning systems that optimize student performance and retention rates. Tools like adobe creative cloud and blackboard mobile app can enhance the learning experience further.
- Manufacturing: Automation and robotics are streamlining production processes, enhancing efficiency, reducing human error, and lowering operational costs. Rapid Innovation specializes in implementing AI-driven automation solutions that can significantly boost productivity and reduce waste, resulting in higher profit margins.
- Finance: Fintech innovations, such as blockchain and mobile banking, are transforming financial transactions by offering increased security, transparency, and convenience for users. Rapid Innovation can guide financial institutions in adopting blockchain technology to enhance transaction security and reduce operational costs, leading to greater customer trust and loyalty.
- Agriculture: Precision farming technologies, including drones and IoT sensors, are optimizing crop yields by providing real-time data on soil health and weather conditions, enabling better decision-making. Rapid Innovation can help agricultural businesses implement AI and IoT solutions that maximize yield and minimize resource usage, driving profitability.
- Transportation: Autonomous vehicles and smart traffic management systems are reshaping urban mobility, promising to reduce congestion and improve safety on the roads. Rapid Innovation can assist municipalities in deploying AI-based traffic management systems that enhance urban mobility and reduce operational costs.
- Retail: E-commerce and AI-driven customer service tools are enhancing the shopping experience by providing personalized recommendations and streamlining inventory management. Rapid Innovation can help retailers implement AI solutions that improve customer engagement and optimize supply chain operations, resulting in increased sales and customer satisfaction. Technologies like duo mobile can also enhance security in retail transactions.
11. Implementation Guide
Implementing new technologies or methodologies requires a structured approach to ensure success. An effective implementation guide can help organizations navigate this process.
- Define Objectives: Clearly outline what you aim to achieve with the new technology, which could include improving efficiency, reducing costs, or enhancing customer satisfaction.
- Conduct Research: Investigate the available technologies and methodologies that align with your objectives. Look for case studies and best practices from similar organizations, including tools like adobe acrobat creative cloud and canvas mobile app.
- Engage Stakeholders: Involve key stakeholders early in the process, as their insights and buy-in are crucial for successful implementation.
- Develop a Roadmap: Create a detailed plan that outlines the steps needed for implementation, including timelines, resources required, and key milestones.
- Pilot Testing: Before a full rollout, conduct a pilot test to identify potential issues, allowing for adjustments based on real-world feedback.
- Training and Support: Provide comprehensive training for users to ensure they are comfortable with the new technology. Ongoing support is essential for addressing any challenges that arise, especially when using complex software like adobe creative cloud software.
- Monitor and Evaluate: After implementation, continuously monitor the performance of the new technology. Evaluate its impact against the defined objectives and make necessary adjustments.
11.1. Planning and Assessment
Effective planning and assessment are critical components of any successful implementation strategy. This phase sets the foundation for the entire process.
- Identify Needs: Assess the current state of your organization to identify gaps and areas for improvement, which will help in determining the specific needs that the new technology should address.
- Set Clear Goals: Establish measurable goals that align with your organization's overall strategy. These goals should be specific, achievable, and time-bound.
- Resource Allocation: Determine the resources required for implementation, including budget, personnel, and technology. Ensure that you have the necessary support to execute the plan, including tools like nyit application portal and payne tech application.
- Risk Assessment: Identify potential risks associated with the implementation and develop strategies to mitigate these risks to ensure a smooth transition.
- Stakeholder Analysis: Understand the interests and influence of various stakeholders to help manage expectations and gain support throughout the process.
- Timeline Development: Create a realistic timeline for the implementation process, including key milestones and deadlines to keep the project on track.
- Feedback Mechanisms: Establish channels for feedback during the planning phase, allowing for adjustments based on stakeholder input and changing circumstances.
11.2. Pilot Program Design
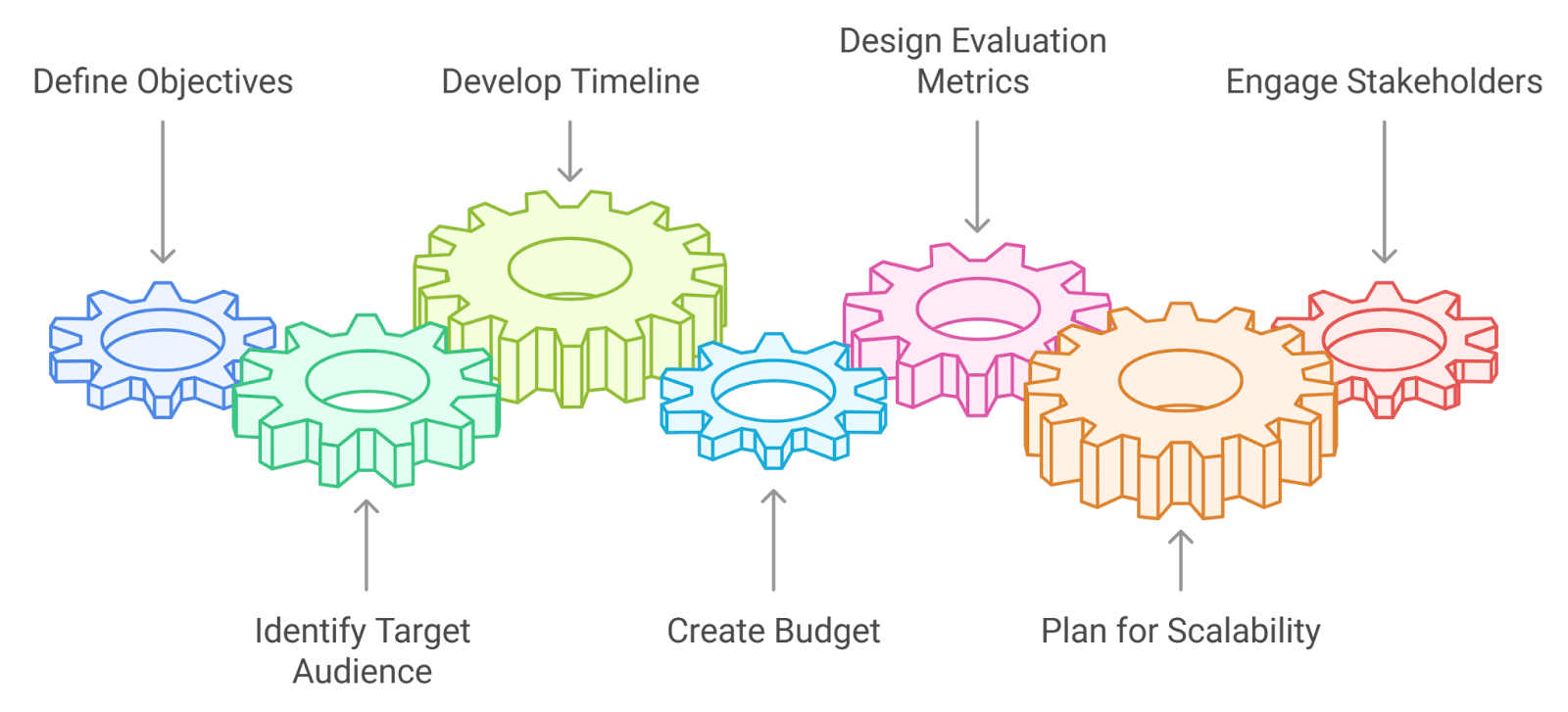
‍
A pilot program design is a crucial step in testing new initiatives before full-scale implementation. The design of a pilot program should be methodical and strategic to ensure that it effectively evaluates the feasibility and impact of the proposed initiative.
- Define objectives: Clearly outline what the pilot aims to achieve. This could include testing new processes, technologies, or services, particularly in the realms of AI and Blockchain, where innovation is rapid and requires careful validation.
- Identify target audience: Select a specific group that will participate in the pilot. This group should represent the broader population that will eventually use the program, ensuring that insights gained are applicable across the intended user base.
- Develop a timeline: Establish a clear timeline for the pilot program design, including start and end dates, as well as key milestones. This is essential for maintaining momentum and ensuring timely evaluations.
- Create a budget: Allocate resources for the pilot, including funding for materials, personnel, and any necessary technology. This is particularly important in AI and Blockchain projects, where specialized skills and tools may be required.
- Design evaluation metrics: Determine how success will be measured. This could include quantitative metrics (e.g., usage rates, cost savings) and qualitative feedback (e.g., user satisfaction), which are critical for assessing the impact of AI-driven solutions or Blockchain implementations.
- Plan for scalability: Consider how the pilot can be expanded if successful. This includes identifying potential barriers to scaling and strategies to overcome them, ensuring that the transition from pilot to full implementation is seamless.
- Engage stakeholders: Involve key stakeholders throughout the pilot program design process to ensure buy-in and support. This could include team members, management, and end-users, all of whom play a vital role in the success of AI and Blockchain initiatives.
11.3. Deployment Strategy
A well-thought-out deployment strategy is essential for the successful rollout of any new program or initiative. This strategy should encompass all aspects of the deployment process to ensure a smooth transition.
- Develop a communication plan: Clearly communicate the goals, benefits, and timeline of the deployment to all stakeholders. This helps manage expectations and fosters support, particularly in complex AI and Blockchain projects.
- Train staff: Provide comprehensive training for all personnel involved in the deployment. This ensures that everyone understands their roles and responsibilities, which is crucial for the effective use of new technologies.
- Implement a phased rollout: Consider deploying the program in phases rather than all at once. This allows for adjustments based on initial feedback and reduces the risk of widespread issues, especially in dynamic environments like AI and Blockchain.
- Monitor resources: Ensure that all necessary resources, including technology and personnel, are in place before the deployment begins. This is vital for maintaining operational efficiency.
- Establish support systems: Create channels for users to report issues or seek assistance during the deployment phase. This could include help desks, FAQs, or user forums, which are essential for user engagement and satisfaction.
- Collect feedback: Actively solicit feedback from users during the deployment. This information is invaluable for making real-time adjustments and improvements, ensuring that the program meets user needs.
11.4. Performance Monitoring
Performance monitoring is vital for assessing the effectiveness of a pilot program or new initiative. It involves tracking key metrics and gathering feedback to ensure that the program meets its objectives.
- Define key performance indicators (KPIs): Identify specific metrics that will be used to measure success. These could include user engagement, cost-effectiveness, and overall satisfaction, particularly in the context of AI and Blockchain applications.
- Use data analytics: Leverage data analytics tools to track performance in real-time. This allows for quick identification of trends and issues, enabling proactive management of the program.
- Conduct regular reviews: Schedule periodic reviews to assess performance against the established KPIs. This helps ensure that the program remains on track and aligned with business goals.
- Gather user feedback: Regularly solicit input from users to understand their experiences and identify areas for improvement. This feedback loop is essential for continuous enhancement of AI and Blockchain solutions.
- Adjust strategies as needed: Be prepared to make changes based on performance data and user feedback. Flexibility is key to optimizing the program and maximizing ROI.
- Report findings: Share performance results with stakeholders to maintain transparency and demonstrate the program's impact. This can help secure ongoing support and funding, which is crucial for the sustainability of innovative initiatives.
By focusing on these critical areas—pilot program design, deployment strategy, and performance monitoring—organizations can enhance their chances of successfully implementing new initiatives and achieving desired outcomes. Rapid Innovation is committed to guiding clients through these processes, leveraging our expertise in AI and Blockchain to ensure efficient and effective solutions that drive greater ROI.
11.5. Continuous Improvement
Continuous improvement is a systematic, ongoing effort to enhance products, services, or processes. It is a fundamental aspect of quality management and operational excellence. Organizations that embrace continuous improvement foster a culture of innovation and adaptability, which can lead to increased efficiency, reduced waste, and improved customer satisfaction.
- Definition: Continuous improvement, often referred to as "Kaizen," is derived from Japanese philosophy and means "change for the better." It emphasizes small, incremental changes rather than large-scale transformations. This concept is also known as the continuous improvement process.
- Importance: Â
- Enhances efficiency: Streamlining processes leads to better performance, which is crucial in the fast-paced world of AI and Blockchain development.
- Reduces costs: Eliminating waste contributes to lower operational expenses, allowing organizations to allocate resources more effectively.
- Improves product quality: Continuous efforts lead to higher customer satisfaction, particularly in technology-driven sectors where quality is paramount.
- Encourages employee engagement: Empowering employees fosters a sense of ownership, which is essential for driving innovation.
- Key Principles: Â
- Focus on customer needs: Understanding customer requirements is crucial for improvement, especially in AI and Blockchain solutions where user experience is key.
- Data-driven decision-making: Use metrics and analytics to identify areas for improvement, leveraging AI tools to gain insights from data.
- Employee involvement: Engage all employees in the improvement process to leverage their insights and expertise, fostering a collaborative environment.
- Incremental changes: Implement small changes regularly rather than waiting for a major overhaul, which can be particularly effective in agile development environments.
- Tools and Techniques: Â
- Plan-Do-Check-Act (PDCA) Cycle: A four-step model for continuous improvement that encourages iterative testing and refinement, applicable in both AI model training and Blockchain protocol development. This cycle is also known as the Deming cycle.
- Six Sigma: A data-driven approach that aims to reduce defects and improve quality, ensuring that AI algorithms and Blockchain transactions are reliable.
- Lean Management: Focuses on maximizing value by minimizing waste, which is essential in optimizing development processes. Lean process improvement is a key aspect of this methodology.
- Root Cause Analysis: Identifies the underlying causes of problems to prevent recurrence, crucial for maintaining the integrity of AI systems and Blockchain networks.
- Implementation Steps: Â
- Identify areas for improvement: Conduct assessments to pinpoint inefficiencies or quality issues, utilizing AI analytics for deeper insights.
- Set measurable goals: Establish clear, achievable objectives to guide improvement efforts, ensuring alignment with business goals.
- Develop an action plan: Outline specific steps, responsibilities, and timelines for implementation, integrating Blockchain for transparency.
- Monitor progress: Regularly review performance metrics to assess the effectiveness of changes, using AI tools for real-time tracking.
- Foster a culture of feedback: Encourage open communication and feedback to continuously refine processes, enhancing team collaboration.
- Challenges: Â
- Resistance to change: Employees may be hesitant to adopt new practices, particularly in traditional industries.
- Lack of management support: Leadership commitment is essential for successful implementation, especially in tech-driven transformations.
- Insufficient training: Employees need proper training to understand and apply improvement techniques, particularly in complex fields like AI and Blockchain.
- Benefits: Â
- Increased productivity: Streamlined processes lead to faster output, enabling quicker time-to-market for AI and Blockchain solutions.
- Enhanced quality: Continuous monitoring and improvement result in higher quality products and services, crucial for maintaining competitive advantage.
- Greater employee satisfaction: Involvement in improvement initiatives can boost morale and job satisfaction, fostering a motivated workforce.
- Real-World Examples: Â
- Toyota Production System: A hallmark of continuous improvement, focusing on efficiency and quality, which can inspire tech firms to adopt similar methodologies.
- Starbucks: Implements continuous improvement in customer service and product offerings based on customer feedback, a practice that can be mirrored in tech industries to enhance user experience.
Continuous improvement is not a one-time effort but a long-term commitment to excellence. By fostering a culture that values ongoing enhancement, organizations can remain competitive and responsive to changing market demands, particularly in the rapidly evolving fields of AI and Blockchain. At Rapid Innovation, we leverage these principles, including kaizen and continuous improvement methodologies, to help our clients achieve greater ROI through efficient and effective development and consulting solutions. For more insights, check out learning from real-world AI implementations.