Table Of Contents
Category
Security
CRM
Oil and Gas
IoT
Blockchain
1. Introduction to AI in Cybersecurity
Artificial Intelligence (AI) is revolutionizing various sectors, and cybersecurity is no exception. The integration of AI into cybersecurity strategies, such as ai cybersecurity and ai and cybersecurity, is becoming increasingly vital as cyber threats evolve in complexity and frequency. AI agents are designed to enhance the capabilities of cybersecurity systems, providing a proactive approach to threat detection and response.
AI can analyze vast amounts of data quickly, identifying patterns and anomalies that may indicate a security breach. Machine learning algorithms enable systems to learn from past incidents, improving their ability to predict and prevent future attacks. Additionally, AI-driven tools can automate routine security tasks, allowing human analysts to focus on more complex issues, which is particularly relevant in ai in cybersecurity.
The growing reliance on digital infrastructure has made organizations more vulnerable to cyberattacks. According to a report, cybercrime is projected to cost the world $10.5 trillion annually by 2025. This alarming statistic underscores the need for advanced cybersecurity measures, where AI plays a crucial role, including frameworks like nist risk management frameworks.
At Rapid Innovation, we leverage cutting-edge AI technologies, such as natural language processing and deep learning, to enhance threat intelligence, automate incident response, and improve overall security posture for our clients. Our expertise in artificial intelligence in security allows us to tailor solutions that not only address immediate security concerns but also contribute to long-term business resilience. We also focus on ai cybersecurity companies and artificial intelligence security companies to stay ahead in the field. However, while the benefits of AI in cybersecurity are significant, there are also challenges that organizations must navigate to effectively implement these technologies, including ai used in cyber security and ai for network security.
1.1. Evolution of Cybersecurity Threats
Cybersecurity threats have evolved significantly over the years, adapting to technological advancements and changing user behaviors. Early threats were primarily simple viruses and worms, often spread through floppy disks and email attachments. The rise of the internet in the 1990s led to more sophisticated attacks, including denial-of-service (DoS) attacks and the emergence of malware. In the 2000s, cybercriminals began to leverage social engineering tactics, such as phishing, to exploit human vulnerabilities. Today, threats include advanced persistent threats (APTs), ransomware, and state-sponsored attacks, which are more organized and targeted. The proliferation of IoT devices has expanded the attack surface, making networks more vulnerable to breaches. Additionally, cybercriminals now utilize artificial intelligence to automate attacks, making them faster and harder to detect.
The evolution of these threats highlights the need for robust cybersecurity measures that can adapt to new challenges. At Rapid Innovation, we understand these complexities and offer tailored AI cybersecurity solutions to help organizations fortify their defenses against evolving threats, ensuring a more secure operational environment.
1.2. The Need for AI-Driven Defense
As cyber threats become more sophisticated, traditional cybersecurity measures are often insufficient. AI-driven defense mechanisms can analyze vast amounts of data in real-time, identifying patterns and anomalies that may indicate a security breach. Machine learning algorithms can improve over time, learning from past incidents to enhance threat detection and response. AI can automate repetitive tasks, allowing cybersecurity professionals to focus on more complex issues. Predictive analytics powered by AI can help organizations anticipate potential threats before they occur. The speed of AI-driven responses can significantly reduce the time it takes to mitigate attacks, minimizing damage and recovery costs. With the increasing complexity of cyber threats, AI provides a scalable solution that can adapt to evolving security landscapes.
The integration of AI into cybersecurity strategies is becoming essential for organizations aiming to protect their digital assets effectively. Rapid Innovation specializes in implementing AI-driven cyber security solutions that not only enhance security but also improve operational efficiency, ultimately leading to greater ROI for our clients.
1.3. Current State of Cybersecurity AI
The current state of cybersecurity AI reflects a rapidly growing field that is becoming integral to modern security frameworks. Many organizations are investing in AI technologies to enhance their cybersecurity posture, with the global AI in cybersecurity market expected to reach $38.2 billion by 2026. AI tools are being used for threat intelligence, automating incident response, and improving vulnerability management. Companies are leveraging natural language processing (NLP) to analyze unstructured data, such as security logs and threat reports, for better insights. Despite advancements, challenges remain, including the potential for AI systems to be manipulated by cybercriminals and the need for skilled professionals to interpret AI-generated insights. Collaboration between AI and human expertise is crucial, as human intuition and experience can complement AI's analytical capabilities. Regulatory frameworks are also evolving to address the ethical implications of AI in cybersecurity, ensuring responsible use of technology.
The current landscape of cybersecurity AI is dynamic, with ongoing innovations aimed at enhancing security measures against increasingly complex threats. At Rapid Innovation, we are at the forefront of these advancements, providing our clients with cutting-edge AI-driven cyber solutions that not only address current security challenges but also position them for future success in an ever-evolving digital landscape.
1.4. Key Stakeholders and Their Roles
In any cybersecurity initiative, various cybersecurity stakeholders play crucial roles in ensuring the effectiveness and security of systems. Understanding these roles is essential for a cohesive approach to cybersecurity.
- IT Security Team: Responsible for implementing security measures, monitoring network traffic, responding to incidents, and conducting regular security audits and vulnerability assessments.
- Management: Provides strategic direction, allocates resources, ensures compliance with regulations and policies, and supports the cybersecurity team through training and awareness programs.
- End Users: Often the first line of defense against cyber threats, end users must be educated on best practices for security and are responsible for reporting suspicious activities.
- Third-Party Vendors: Provide tools and services that enhance cybersecurity, must adhere to security standards and protocols, and collaborate with internal teams to ensure seamless integration.
- Regulatory Bodies: Establish guidelines and compliance requirements, monitor organizations for adherence to cybersecurity laws, and provide frameworks for risk management and incident response.
- Incident Response Teams: Specialized groups that handle security breaches, develop and implement incident response plans, and conduct post-incident analysis to improve future responses.
Understanding the roles of these stakeholders helps organizations create a robust cybersecurity framework, ensuring that all aspects of security are covered and that there is a clear line of communication and responsibility.
2. Understanding AI Agents in Cybersecurity
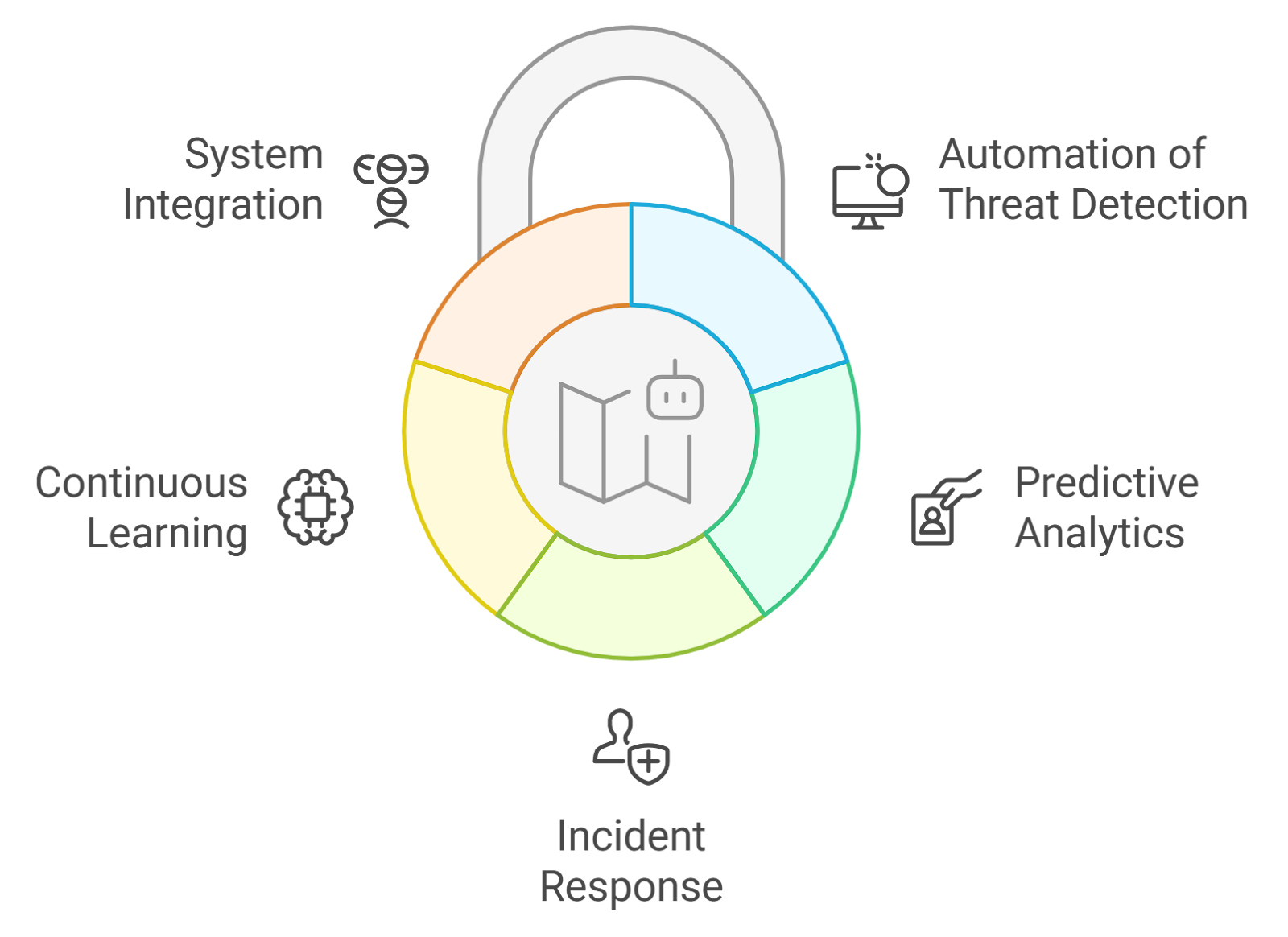
‍
AI agents are becoming increasingly important in the field of cybersecurity. They leverage machine learning and artificial intelligence to enhance security measures, automate responses, and analyze vast amounts of data for potential threats.
- Automation of Threat Detection: AI agents can analyze network traffic in real-time, identify anomalies that may indicate a security breach, and reduce the time taken to detect threats compared to traditional methods.
- Predictive Analytics: AI agents can predict potential vulnerabilities based on historical data, helping organizations proactively address security gaps and enhancing overall security posture.
- Incident Response: AI agents can automate responses to certain types of incidents, isolate affected systems to prevent further damage, and minimize the impact of security breaches through rapid response capability.
- Continuous Learning: AI agents improve over time by learning from new data, adapting to evolving threats and changing environments, which enhances their effectiveness.
- Integration with Existing Systems: AI agents can be integrated into existing security frameworks, complementing human efforts rather than replacing them, leading to a more comprehensive security strategy.
2.1. Types of AI Agents
AI agents in cybersecurity can be categorized into several types, each serving distinct functions and purposes. Understanding these types helps organizations choose the right AI solutions for their specific needs.
- Reactive Agents: Respond to specific inputs or events, operate based on predefined rules and protocols, and are useful for immediate threat detection and response.
- Proactive Agents: Anticipate potential threats before they occur, use predictive analytics to identify vulnerabilities, and help organizations stay ahead of cyber threats.
- Autonomous Agents: Operate independently without human intervention, capable of making decisions based on real-time data, and are ideal for environments requiring constant monitoring.
- Collaborative Agents: Work alongside human teams to enhance decision-making, provide insights and recommendations based on data analysis, and foster a partnership between AI and human expertise.
- Hybrid Agents: Combine features of both reactive and proactive agents, adapt to various situations and environments, and offer flexibility in addressing diverse cybersecurity challenges.
- Intelligent Agents: Utilize advanced machine learning algorithms, continuously learn from new data and experiences, and enhance their capabilities over time, improving threat detection and response.
By understanding the different types of AI agents, organizations can better implement AI-driven solutions in their cybersecurity strategies, ensuring a more robust defense against evolving cyber threats.
At Rapid Innovation, we leverage our expertise in AI and blockchain to help clients enhance their cybersecurity frameworks, ensuring that they not only meet compliance requirements but also achieve greater ROI through efficient and effective security measures.
2.1.1. Monitoring Agents
Monitoring agents are essential components in cybersecurity and IT management. They continuously observe and collect data from various systems, networks, and applications to ensure optimal performance and security.
- They track system performance metrics, such as CPU usage, memory consumption, and network traffic. Â
- Monitoring agents can detect anomalies that may indicate security breaches or system failures. Â
- They provide real-time alerts to administrators, enabling quick responses to potential issues. Â
- These cybersecurity monitoring agents can be deployed on servers, workstations, and network devices, ensuring comprehensive coverage. Â
- They often integrate with centralized management systems for streamlined monitoring and reporting. Â
The effectiveness of monitoring agents lies in their ability to provide actionable insights. By analyzing the data collected, organizations can identify trends, optimize resource allocation, and enhance overall system reliability. At Rapid Innovation, we leverage advanced AI algorithms to enhance the capabilities of monitoring agents, enabling our clients to achieve greater operational efficiency and reduce downtime, ultimately leading to a higher return on investment (ROI). For more information on how AI can improve anomaly detection in business.
2.1.2. Detection Agents
Detection agents play a critical role in identifying potential threats and vulnerabilities within an organization's IT infrastructure. They utilize various techniques to analyze data and detect suspicious activities.
- Detection agents can employ signature-based detection, which identifies known threats by comparing data against a database of signatures. Â
- They may also use anomaly-based detection, which establishes a baseline of normal behavior and flags deviations from this norm. Â
- These agents are crucial for identifying malware, unauthorized access attempts, and other security incidents. Â
- They often work in conjunction with monitoring agents to provide a comprehensive view of the security landscape. Â
- Detection agents can be configured to operate in real-time, providing immediate alerts when a threat is detected. Â
The implementation of detection agents is vital for proactive cybersecurity measures. By identifying threats early, organizations can mitigate risks and prevent potential damage. Rapid Innovation employs cutting-edge AI techniques to enhance detection capabilities, ensuring that our clients can respond to threats swiftly and effectively, thereby minimizing potential losses and maximizing ROI.
2.1.3. Response Agents
Response agents are the frontline defenders in cybersecurity, tasked with taking action when a threat is detected. Their primary goal is to contain and remediate incidents to minimize damage and restore normal operations.
- Response agents can automate responses to certain types of incidents, such as isolating affected systems or blocking malicious IP addresses. Â
- They often work in tandem with detection agents to ensure a swift response to identified threats. Â
- These agents can execute predefined playbooks that outline specific steps to take during an incident, ensuring consistency and efficiency. Â
- They may also provide forensic capabilities, allowing organizations to analyze the incident and understand its impact. Â
- Response agents are essential for incident management, helping organizations recover quickly and effectively from security breaches. Â
The role of response agents is crucial in maintaining an organization's security posture. By ensuring rapid and effective responses to incidents, they help protect sensitive data and maintain business continuity. At Rapid Innovation, we integrate AI-driven response strategies that not only enhance the speed of incident resolution but also improve the overall security framework, leading to a more resilient organization and a significant increase in ROI.
2.1.4. Prediction Agents
Prediction agents are advanced systems designed to analyze data and forecast future outcomes based on historical patterns. These agents utilize various algorithms and statistical methods to make informed predictions, which can be applied across multiple domains such as finance, healthcare, marketing, and more. They leverage large datasets to identify trends and correlations, operate in real-time to provide immediate insights and recommendations, and often incorporate machine learning techniques to improve accuracy over time. Common applications include stock market predictions, customer behavior forecasting, and risk assessment in insurance. The effectiveness of prediction agents is often measured by their accuracy and the speed at which they can process data.
The rise of big data has significantly enhanced the capabilities of prediction agents, allowing them to analyze vast amounts of information quickly. As a result, businesses can make data-driven decisions that lead to improved outcomes and competitive advantages. At Rapid Innovation, we harness the power of prediction agents to help our clients optimize their operations, enhance customer experiences, and ultimately achieve greater ROI. Our expertise in adaptive AI development ensures that we can create tailored solutions that meet the unique needs of each client.
2.2. Core Technologies
Core technologies refer to the foundational tools and systems that enable the development and functioning of various applications, particularly in the realm of artificial intelligence (AI) and machine learning (ML). These technologies are essential for building robust, scalable, and efficient solutions.
- Core technologies include programming languages, frameworks, and libraries that facilitate AI development. Â
- They encompass data storage and processing systems, such as databases and cloud computing platforms. Â
- Core technologies also involve hardware components, including GPUs and TPUs, which are crucial for training complex models. Â
- Security and privacy technologies are vital to protect sensitive data used in AI applications. Â
- Integration with existing systems and APIs is necessary for seamless functionality. Â
Understanding these core technologies is crucial for developers and organizations looking to implement AI solutions effectively. They provide the necessary infrastructure to support innovative applications and ensure that they operate efficiently and securely. Rapid Innovation leverages these core technologies to deliver tailored solutions that align with our clients' business objectives.
2.2.1. Machine Learning Models
Machine learning models are algorithms that enable computers to learn from data and make predictions or decisions without being explicitly programmed. These models are at the heart of many AI applications and can be categorized into several types based on their learning approach.
- Supervised learning models require labeled data to train, allowing them to make predictions based on input-output pairs. Â
- Unsupervised learning models analyze data without labels, identifying patterns and groupings within the data. Â
- Reinforcement learning models learn through trial and error, receiving feedback from their actions to improve performance over time. Â
- Common machine learning algorithms include decision trees, neural networks, support vector machines, and ensemble methods. Â
- The choice of model depends on the specific problem, the nature of the data, and the desired outcome. Â
Machine learning models are continually evolving, with advancements in deep learning and neural networks leading to significant improvements in performance and capabilities. These models are widely used in applications such as image recognition, natural language processing, and predictive analytics, making them integral to the future of technology and business. At Rapid Innovation, we specialize in developing and implementing machine learning models that drive efficiency and innovation for our clients, ensuring they stay ahead in a competitive landscape.
2.2.2. Natural Language Processing
Natural Language Processing (NLP) is a crucial subfield of artificial intelligence that focuses on the interaction between computers and humans through natural language. The goal of NLP is to enable machines to understand, interpret, and respond to human language in a valuable way.
- NLP encompasses various tasks, including: Â
- Text analysis
- Sentiment analysis
- Language translation
- Speech recognition
- Chatbots and virtual assistants
- Techniques used in NLP include: Â
- Tokenization: Breaking down text into smaller units, such as words or phrases.
- Named Entity Recognition (NER): Identifying and classifying key entities in text, such as names, dates, and locations.
- Part-of-Speech Tagging: Assigning grammatical categories to words, helping to understand sentence structure.
- NLP applications are widespread: Â
- Customer service automation through chatbots.
- Content recommendation systems that analyze user preferences.
- Language translation services.
At Rapid Innovation, we leverage NLP to enhance customer engagement and streamline operations. For example, our custom chatbot solutions can significantly reduce response times and improve customer satisfaction, leading to a higher return on investment (ROI) for our clients. The rise of deep learning has significantly advanced NLP capabilities, allowing for more sophisticated models that can understand context and nuances in language. For instance, models like BERT and GPT-3 have revolutionized how machines process and generate human language. Additionally, techniques such as natural language programming and natural language analysis are becoming increasingly important in the field of NLP, further enhancing its applications in areas like natural language recognition and natural language processing techniques.
2.2.3. Deep Learning Systems
Deep Learning Systems are a subset of machine learning that utilize neural networks with many layers (hence "deep") to analyze various forms of data. These systems are particularly effective in handling large datasets and complex patterns.
- Key characteristics of deep learning include: Â
- Use of artificial neural networks that mimic the human brain's structure.
- Ability to learn from vast amounts of unstructured data, such as images, audio, and text.
- Automatic feature extraction, reducing the need for manual feature engineering.
- Common architectures in deep learning: Â
- Convolutional Neural Networks (CNNs): Primarily used for image processing and computer vision tasks.
- Recurrent Neural Networks (RNNs): Effective for sequential data, such as time series or natural language.
- Generative Adversarial Networks (GANs): Used for generating new data samples that resemble existing data.
- Applications of deep learning systems are diverse: Â
- Image recognition in social media platforms.
- Voice assistants that understand and respond to user commands.
- Autonomous vehicles that interpret sensor data to navigate safely.
At Rapid Innovation, we harness deep learning to develop advanced predictive analytics solutions that help businesses make data-driven decisions. By implementing deep learning models, our clients have seen improved accuracy in forecasting and enhanced operational efficiency, ultimately leading to greater ROI. The performance of deep learning systems has been enhanced by advancements in hardware, such as GPUs, and the availability of large datasets, enabling breakthroughs in various fields.
2.2.4. Reinforcement Learning
Reinforcement Learning (RL) is a type of machine learning where an agent learns to make decisions by taking actions in an environment to maximize cumulative rewards. Unlike supervised learning, where the model learns from labeled data, RL focuses on learning from the consequences of actions.
- Core components of reinforcement learning include: Â
- Agent: The learner or decision-maker.
- Environment: The context in which the agent operates.
- Actions: The choices available to the agent.
- Rewards: Feedback from the environment based on the agent's actions.
- The learning process involves: Â
- Exploration: The agent tries new actions to discover their effects.
- Exploitation: The agent uses known actions that yield high rewards.
- Balancing exploration and exploitation is crucial for effective learning.
- Applications of reinforcement learning are extensive: Â
- Game playing, such as AlphaGo, which defeated human champions in complex games.
- Robotics, where RL helps robots learn tasks through trial and error.
- Personalized recommendations in online platforms, optimizing user engagement.
At Rapid Innovation, we apply reinforcement learning to optimize business processes and enhance user experiences. For instance, our RL-based recommendation systems can adapt to user behavior in real-time, leading to increased engagement and higher conversion rates. Reinforcement learning has gained popularity due to its ability to solve complex problems that require sequential decision-making, making it a powerful tool in AI development.
2.3. Architecture and Integration
The architecture of AI agents is crucial for their functionality and effectiveness in various applications. A well-structured architecture allows for seamless integration with existing systems, enhancing overall performance. This includes various types of agent architecture in AI, such as BDI architecture in artificial intelligence and logic-based agent architecture in AI.
- Modular Design: AI agents often utilize a modular architecture, which allows different components to function independently while still communicating effectively. This modularity facilitates easier updates and maintenance, enabling Rapid Innovation to tailor solutions to specific client needs.
- Data Processing Layers: The architecture typically includes multiple layers for data processing. These layers can include: Â
- Data Ingestion: Collects data from various sources, such as sensors, databases, or user inputs.
- Data Processing: Analyzes and processes the ingested data using algorithms and machine learning models.
- Decision Making: Based on the processed data, the AI agent makes decisions or predictions that align with business objectives.
- Integration with Existing Systems: AI agents must integrate smoothly with current IT infrastructures. This can involve: Â
- APIs: Application Programming Interfaces allow AI agents to communicate with other software systems, ensuring that Rapid Innovation's solutions can be easily incorporated into clients' existing frameworks.
- Middleware: Acts as a bridge between different applications, ensuring data flows seamlessly and enhancing operational efficiency.
- Scalability: The architecture should support scalability, allowing organizations to expand their AI capabilities as needed without significant overhauls. This flexibility is essential for clients looking to grow their operations.
- Security Considerations: Given the sensitive nature of data processed by AI agents, security must be a priority. This includes: Â
- Data Encryption: Protecting data at rest and in transit to safeguard client information.
- Access Controls: Ensuring that only authorized users can access sensitive information, thereby enhancing trust in Rapid Innovation's solutions.
- Cloud and Edge Computing: Many AI agents leverage cloud computing for storage and processing power, while edge computing can be used for real-time data processing closer to the source, reducing latency and improving responsiveness.
3. Key Benefits of AI Agents

‍
AI agents offer numerous advantages across various sectors, enhancing efficiency, accuracy, and decision-making processes. They can automate repetitive tasks, freeing up human resources for more complex activities. By analyzing vast amounts of data quickly, AI agents provide insights that can lead to better decision-making. Implementing AI agents can lead to significant cost savings by reducing labor costs and minimizing errors. Unlike human workers, AI agents can operate continuously, providing support and services around the clock. Additionally, AI agents can analyze user behavior and preferences, allowing for tailored experiences in customer service and marketing.
3.1. Real-time Threat Detection
One of the most critical applications of AI agents is in the realm of cybersecurity, particularly in real-time threat detection. AI agents can monitor networks and systems continuously, identifying anomalies that may indicate a security threat. Upon detecting a potential threat, AI agents can initiate immediate responses, such as isolating affected systems or alerting security personnel. These agents utilize advanced machine learning algorithms to learn from past incidents, improving their ability to detect new threats over time. They can also analyze user behavior patterns to identify deviations that may signal a security breach.
AI agents can be integrated with existing security infrastructure, enhancing the overall security posture of an organization. By leveraging sophisticated algorithms, they can minimize false positives, ensuring that security teams focus on genuine threats. As organizations grow, AI agents can scale to monitor larger networks without a proportional increase in resources. Implementing AI for real-time threat detection can reduce the costs associated with data breaches and security incidents.
In conclusion, the architecture and integration of AI agents, including the architecture of intelligent agents in AI, play a vital role in their effectiveness, while their ability to provide real-time threat detection offers significant benefits in cybersecurity. Rapid Innovation is committed to helping clients harness these capabilities to achieve their business goals efficiently and effectively.
3.2. Automated Response Capabilities
Automated response capabilities are essential in modern systems, particularly in cybersecurity and customer service. These capabilities allow organizations to react swiftly to incidents or inquiries without human intervention, enhancing efficiency and reducing response times. Automated systems, such as automated IVR systems and automated voice response systems, can initiate responses instantly, addressing issues like security breaches or customer queries without delay. They ensure uniformity in handling situations, reducing the risk of human error and maintaining service quality. By automating routine tasks, organizations can allocate human resources to more complex issues, improving overall productivity. Many automated systems leverage artificial intelligence to improve decision-making processes, allowing for more sophisticated responses based on historical data and patterns. Additionally, automated systems can operate around the clock, providing support and monitoring without the limitations of human work hours.
- Immediate Action: Automated systems can initiate responses instantly, addressing issues like security breaches or customer queries without delay.
- Consistency: Automated responses ensure uniformity in handling situations, reducing the risk of human error and maintaining service quality.
- Resource Optimization: By automating routine tasks, organizations can allocate human resources to more complex issues, improving overall productivity.
- Integration with AI: Many automated systems leverage artificial intelligence to improve decision-making processes, allowing for more sophisticated responses based on historical data and patterns.
- 24/7 Availability: Automated systems can operate around the clock, providing support and monitoring without the limitations of human work hours.
3.3. Pattern Recognition and Analysis
Pattern recognition and analysis are critical components in various fields, including data science, cybersecurity, and market research. These processes involve identifying trends and anomalies within large datasets, enabling organizations to make informed decisions. Advanced algorithms can sift through vast amounts of data to uncover hidden patterns, which can be crucial for predictive analytics. By recognizing deviations from established patterns, organizations can identify potential threats or issues before they escalate. In customer service, pattern recognition helps in understanding customer behavior, allowing for personalized marketing strategies and improved user experiences. Many systems utilize machine learning techniques to enhance pattern recognition, continuously improving their accuracy as they process more data. The ability to analyze patterns in real-time allows organizations to respond quickly to emerging trends or threats, maintaining a competitive edge.
- Data Mining: Advanced algorithms can sift through vast amounts of data to uncover hidden patterns, which can be crucial for predictive analytics.
- Anomaly Detection: By recognizing deviations from established patterns, organizations can identify potential threats or issues before they escalate.
- Behavioral Insights: In customer service, pattern recognition helps in understanding customer behavior, allowing for personalized marketing strategies and improved user experiences.
- Machine Learning: Many systems utilize machine learning techniques to enhance pattern recognition, continuously improving their accuracy as they process more data.
- Real-time Analysis: The ability to analyze patterns in real-time allows organizations to respond quickly to emerging trends or threats, maintaining a competitive edge.
3.4. Scalability and Coverage
Scalability and coverage are vital for organizations looking to grow and adapt to changing market demands. These concepts refer to the ability of a system to expand its capabilities and the extent of its operational reach. Scalable systems can adjust resources based on demand, ensuring that performance remains consistent even during peak times. Coverage refers to the geographical and operational extent of a system, allowing organizations to serve a broader audience without compromising service quality. Scalable solutions often lead to cost savings, as organizations can invest in additional resources only when necessary, avoiding over-provisioning. As business needs evolve, scalable systems can incorporate new technologies and processes, ensuring long-term viability. With proper scalability, organizations can maintain high performance levels, even as user numbers and data volumes increase.
- Flexible Infrastructure: Scalable systems can adjust resources based on demand, ensuring that performance remains consistent even during peak times.
- Global Reach: Coverage refers to the geographical and operational extent of a system, allowing organizations to serve a broader audience without compromising service quality.
- Cost Efficiency: Scalable solutions often lead to cost savings, as organizations can invest in additional resources only when necessary, avoiding over-provisioning.
- Adaptability: As business needs evolve, scalable systems can incorporate new technologies and processes, ensuring long-term viability.
- Enhanced Performance: With proper scalability, organizations can maintain high performance levels, even as user numbers and data volumes increase.
At Rapid Innovation, we leverage these advanced capabilities in AI and blockchain to help our clients achieve their business goals efficiently and effectively. By implementing automated response systems, including automated IVR and automated voice response, we enable organizations to enhance their operational efficiency and reduce costs, ultimately leading to greater ROI. Our expertise in pattern recognition and analysis allows us to provide actionable insights that drive strategic decision-making, while our scalable solutions ensure that our clients can grow and adapt to market changes without compromising service quality.
3.5. Predictive Security Measures
Predictive security measures involve the use of advanced analytics and machine learning to anticipate potential security threats before they occur. This proactive approach is essential in today’s digital landscape, where cyber threats are becoming increasingly sophisticated.
- Utilizes data analysis to identify patterns and trends in security incidents. Â
- Employs algorithms to predict potential vulnerabilities and attack vectors. Â
- Integrates threat intelligence feeds to stay updated on emerging threats. Â
- Enhances incident response times by preparing for likely scenarios. Â
- Reduces the overall risk by addressing vulnerabilities before they can be exploited. Â
Organizations can leverage predictive security measures to create a more resilient security posture. By investing in tools that analyze historical data and current threat landscapes, businesses can better allocate resources and prioritize security initiatives. This not only helps in mitigating risks but also in fostering a culture of security awareness among employees. At Rapid Innovation, we harness the power of AI to develop tailored predictive security solutions that align with your business objectives, ultimately driving greater ROI.
3.6. Resource Optimization
Resource optimization in security refers to the efficient allocation and management of security resources, including personnel, technology, and budget. This is crucial for organizations aiming to maximize their security investments while minimizing waste.
- Involves assessing current security tools and processes for effectiveness. Â
- Encourages the consolidation of security solutions to reduce complexity. Â
- Focuses on automating repetitive tasks to free up human resources for strategic initiatives. Â
- Implements risk-based prioritization to allocate resources where they are needed most. Â
- Utilizes metrics and KPIs to measure the effectiveness of security investments. Â
By optimizing resources, organizations can ensure that they are not only protected against threats but also able to respond effectively when incidents occur. This approach leads to improved operational efficiency and can significantly lower the total cost of ownership for security solutions. Rapid Innovation assists clients in streamlining their security frameworks, ensuring that every dollar spent contributes to a stronger security posture and enhanced business performance.
3.7. Continuous Learning and Adaptation
Continuous learning and adaptation are vital components of a robust security strategy. The threat landscape is constantly evolving, and organizations must be agile enough to adapt to new challenges.
- Encourages ongoing training and education for security personnel. Â
- Promotes a culture of knowledge sharing and collaboration among teams. Â
- Utilizes feedback loops to learn from past incidents and improve future responses. Â
- Adopts new technologies and methodologies as they become available. Â
- Engages in regular security assessments and audits to identify areas for improvement. Â
Organizations that prioritize continuous learning and adaptation are better equipped to handle emerging threats. By fostering an environment where learning is encouraged, businesses can stay ahead of cybercriminals and ensure that their security measures remain effective over time. This proactive stance not only enhances security but also builds trust with customers and stakeholders. At Rapid Innovation, we emphasize the importance of continuous improvement, helping clients to stay ahead of the curve through innovative training programs and adaptive security solutions.
4. Implementation Challenges
Implementing new systems or processes often comes with a variety of challenges that can hinder progress and effectiveness. Understanding these challenges is crucial for successful implementation, particularly when it comes to challenges implementing electronic health records and emr implementation challenges.
4.1 Technical Challenges
Technical challenges are among the most significant hurdles organizations face during implementation. These challenges can stem from outdated technology, lack of integration, or insufficient technical expertise. Addressing these issues is essential for ensuring a smooth transition and achieving desired outcomes. Key technical challenges include:
- Legacy systems may not support new technologies.
- Integration between different systems can be complex, especially in the context of challenges in implementing ehr.
- Technical expertise may be lacking within the organization, which is a common barrier to implementing ehr.
4.1.1 Data Quality and Availability
Data quality and availability are critical components of any successful implementation. Poor data quality can lead to incorrect insights, while unavailability can stall processes altogether. Organizations must prioritize data management to overcome these challenges. The main issues related to data quality and availability include:
- Inaccurate Data: Data may be outdated, incomplete, or incorrect, leading to flawed decision-making.
- Data Silos: Information may be trapped in separate systems, making it difficult to access and analyze.
- Data Governance: Lack of clear policies on data management can result in inconsistencies and compliance issues.
To address these issues, organizations should consider the following strategies:
- Data Cleaning: Regularly audit and clean data to ensure accuracy and relevance.
- Centralized Data Management: Implement systems that allow for centralized access to data across departments.
- Training and Awareness: Educate staff on the importance of data quality and how to maintain it.
At Rapid Innovation, we leverage our expertise in AI and Blockchain to help organizations navigate these technical challenges effectively. For instance, our AI-driven data management solutions can automate data cleaning processes, ensuring that your organization has access to accurate and relevant information. Additionally, our Blockchain technology can facilitate seamless integration between disparate systems, breaking down data silos and enhancing data governance. By focusing on data quality and availability, organizations can mitigate technical challenges and enhance the overall effectiveness of their implementation efforts, ultimately achieving greater ROI.
Moreover, organizations should be aware of the top 10 ehr implementation challenges and how to overcome them, as well as the specific problems of policy implementation that can arise during the process. Understanding software implementation challenges and workday implementation challenges can also provide valuable insights for a smoother transition.
4.1.2. Integration with Legacy Systems
Integrating new technologies with legacy systems can be a complex and challenging process. Legacy systems often use outdated technology, making it difficult to connect them with modern applications. This integration is crucial for organizations looking to leverage new capabilities while still utilizing existing infrastructure, such as through legacy application integration and mainframe modernization and integration.
- Compatibility Issues: Legacy systems may not support modern APIs or data formats, leading to compatibility challenges, particularly when integrating with solutions like salesforce integration with legacy systems.
- Data Migration: Transferring data from legacy systems to new platforms can be time-consuming and prone to errors, especially in legacy data integration scenarios.
- Cost Implications: Integration efforts can incur significant costs, including software, hardware, and labor, particularly in projects involving erp legacy system integration.
- Training Requirements: Employees may need training to understand both the legacy and new systems, which can slow down the integration process, especially when dealing with mulesoft legacy modernization.
- Risk of Downtime: Integrating new systems can lead to temporary downtime, affecting business operations, particularly when integrating the legacy platforms.
Organizations must carefully plan their integration strategies to minimize disruptions and ensure a smooth transition. Rapid Innovation specializes in developing tailored middleware solutions and custom APIs that effectively bridge the gap between old and new technologies, ensuring a seamless integration process that enhances operational efficiency and maximizes ROI, including solutions for legacy api salesforce and legacy system in salesforce. Additionally, our expertise in large language model development can further enhance integration capabilities.
4.1.3. Performance Overhead
Performance overhead refers to the additional resources required to run a system or application beyond the basic operational needs. This can significantly impact the efficiency and speed of applications, especially in environments where performance is critical.
- Resource Consumption: New technologies may require more CPU, memory, or storage, leading to increased operational costs.
- Latency Issues: Additional layers of processing can introduce latency, affecting user experience and application responsiveness.
- Scalability Challenges: As systems grow, performance overhead can hinder scalability, making it difficult to accommodate increased loads.
- Monitoring Needs: Organizations may need to invest in monitoring tools to track performance metrics and identify bottlenecks.
- Balancing Act: Finding the right balance between functionality and performance is essential to avoid excessive overhead.
To mitigate performance overhead, organizations can optimize their applications through code refactoring, load balancing, and efficient resource allocation. Rapid Innovation employs advanced performance testing methodologies and optimization techniques to help clients identify and address performance bottlenecks, ensuring that their systems operate at peak efficiency and deliver a strong return on investment.
4.1.4. Model Training and Updates
Model training and updates are critical components of machine learning and artificial intelligence systems. These processes ensure that models remain accurate and relevant over time, adapting to new data and changing conditions.
- Data Quality: The effectiveness of model training heavily relies on the quality of the data used. Poor-quality data can lead to inaccurate models.
- Frequency of Updates: Regular updates are necessary to maintain model performance, especially in dynamic environments where data patterns change frequently.
- Computational Resources: Training models can be resource-intensive, requiring significant computational power and time.
- Version Control: Managing different versions of models is essential to track improvements and ensure that the best-performing model is in use.
- Continuous Learning: Implementing continuous learning strategies allows models to adapt in real-time, improving their accuracy and relevance.
Organizations should establish a robust framework for model training and updates, including automated pipelines for data ingestion, model training, and deployment. Rapid Innovation provides comprehensive solutions for model training and updates, ensuring that clients' AI systems are consistently optimized and capable of responding effectively to evolving business needs, thereby enhancing overall performance and ROI.
4.2. Organizational Challenges
Organizations today face a myriad of challenges that can hinder their growth and efficiency. These challenges often stem from internal processes, employee engagement, and the rapid pace of technological advancements. Addressing these issues is crucial for maintaining a competitive edge in the market.
- Increased competition necessitates constant innovation.
- Employee retention is becoming more difficult in a dynamic job market.
- Adapting to technological changes requires ongoing investment and training.
4.2.1. Skill Gap and Training
One of the most pressing organizational challenges is the skill gap among employees. As industries evolve, the demand for specific skills often outpaces the supply of qualified workers. This gap can lead to decreased productivity and hinder an organization's ability to innovate. Rapid technological advancements require continuous learning, and many employees may lack the necessary skills for new tools and processes. Therefore, organizations must invest in training programs to upskill their workforce.
To address the skill gap, organizations can implement various strategies:
- Conduct regular skills assessments to identify gaps.
- Develop tailored training programs that align with business goals.
- Encourage a culture of continuous learning through workshops and online courses.
At Rapid Innovation, we leverage AI-driven analytics to conduct skills assessments, enabling organizations to pinpoint specific areas for improvement. Our tailored training programs incorporate the latest advancements in AI and Blockchain, ensuring that employees are equipped with the skills necessary to thrive in a rapidly changing environment. Investing in employee training not only enhances skills but also boosts morale and retention rates. According to a report, companies that invest in employee training see a 24% higher profit margin compared to those that do not.
4.2.2. Change Management
Change management is another critical organizational challenge. As businesses adapt to new technologies, market demands, and internal restructuring, managing change effectively becomes essential. Poor change management can lead to employee resistance, decreased productivity, and ultimately, failure to achieve desired outcomes. Employees may feel uncertain or anxious about changes, and a lack of clear communication can lead to misunderstandings. Additionally, resistance to change can stem from fear of job loss or increased workload.
To facilitate effective change management, organizations should consider the following approaches:
- Develop a clear change management strategy that outlines objectives and processes.
- Communicate openly with employees about the reasons for change and expected outcomes.
- Involve employees in the change process to foster a sense of ownership and reduce resistance.
At Rapid Innovation, we assist organizations in developing robust change management strategies that incorporate AI and Blockchain technologies. By utilizing AI for predictive analytics, we help organizations anticipate potential challenges, including challenges in organizational change and challenges of change in organization, and streamline the transition process. Successful change management can lead to improved organizational performance and employee satisfaction. Research indicates that organizations with effective change management practices are 6 times more likely to achieve their desired outcomes.
By addressing the skill gap and implementing effective change management strategies, organizations can navigate the complexities of the modern business landscape and position themselves for long-term success. Rapid Innovation is committed to empowering organizations with the tools and expertise needed to achieve greater ROI and sustainable growth. Common organizational problems, such as organizational culture problems and challenges of organizational culture, can be mitigated through proactive measures. Additionally, organizations with communication problems must prioritize clear and open dialogue to foster a healthier work environment. For more information on how we can help with AI development for businesses.
4.2.3. Budget Constraints
Budget constraints are a significant factor that can impact various projects and initiatives within an organization. These limitations can affect decision-making processes, resource allocation, and overall project feasibility. Limited financial resources can lead to reduced scope of projects, delayed timelines, and compromised quality of deliverables. Organizations often face challenges in balancing operational costs, capital expenditures, and unexpected expenses.
At Rapid Innovation, we understand the importance of maximizing ROI while navigating budget constraints. Our AI-driven analytics tools can help organizations identify cost-saving opportunities and prioritize projects that align with strategic goals. By leveraging our expertise in blockchain technology, we can also facilitate transparent budgeting processes, ensuring that every dollar spent is accounted for and directed towards high-impact initiatives.
Effective budget management strategies, such as the best money management strategy, include prioritizing essential projects, implementing cost-saving measures, and regularly reviewing and adjusting budgets based on performance. Stakeholders must communicate openly about budget constraints to set realistic expectations, foster collaboration, and identify alternative funding sources or partnerships. Additionally, strategies to manage money effectively can help organizations navigate financial limitations and enhance project outcomes. A strategy to monitor actual expenditure is also crucial in maintaining financial discipline and ensuring that resources are utilized efficiently. For organizations looking to develop innovative solutions, partnering with a crypto token development company can be a strategic move to enhance their project outcomes. Furthermore, understanding the landscape of regulations and compliance best practices is essential for organizations to effectively manage their budgets while adhering to legal requirements.
4.2.4. Regulatory Compliance
Regulatory compliance refers to the adherence to laws, regulations, guidelines, and specifications relevant to an organization’s operations. Non-compliance can lead to severe penalties, including fines and reputational damage. Key aspects of regulatory compliance include understanding applicable laws and regulations, implementing policies and procedures to ensure compliance, and providing regular training for employees on compliance matters.
Organizations must stay updated on changes in regulations, industry standards, and best practices for compliance management. The benefits of maintaining regulatory compliance include enhanced reputation and trust with stakeholders, reduced risk of legal issues, and improved operational efficiency. Compliance audits and assessments are essential for identifying gaps in compliance, ensuring continuous improvement, and demonstrating accountability to regulators and stakeholders.
Rapid Innovation offers consulting services that help organizations navigate the complex landscape of regulatory compliance, particularly in the realms of AI and blockchain. Our solutions ensure that your technology implementations not only meet regulatory standards but also enhance operational efficiency and stakeholder trust.
4.3. Security Challenges
Security challenges encompass a wide range of threats that organizations face in protecting their assets, data, and operations. These challenges can arise from both internal and external sources. Common security challenges include cybersecurity threats, such as hacking and phishing, physical security risks, including theft and vandalism, and insider threats from employees or contractors.
Organizations must adopt a multi-layered security approach that includes implementing robust cybersecurity measures, conducting regular security assessments and audits, and providing ongoing training for employees on security best practices. The rise of remote work has introduced new security vulnerabilities, such as insecure home networks, increased reliance on cloud services, and challenges in monitoring employee activity.
To mitigate security challenges, organizations should develop a comprehensive security policy, invest in advanced security technologies, and foster a culture of security awareness among employees. Rapid Innovation specializes in integrating AI and blockchain solutions that enhance security protocols, ensuring that your organization is well-equipped to handle emerging threats while maintaining compliance and operational integrity.
4.3.1. AI Model Vulnerabilities
AI models, particularly those based on machine learning, can exhibit various vulnerabilities, including ai model vulnerabilities, that can compromise their effectiveness and reliability. Understanding these vulnerabilities is crucial for developing robust AI systems, and at Rapid Innovation, we leverage our expertise to help clients navigate these challenges effectively.
- Data Sensitivity: AI models are highly sensitive to the data they are trained on. If the training data is biased or unrepresentative, the model may produce skewed results, leading to ethical concerns and misinformed decisions. Rapid Innovation assists clients in curating high-quality datasets, ensuring that the training data is diverse and representative, which ultimately enhances model performance and trustworthiness.
- Overfitting: When a model learns too much from the training data, it may perform poorly on unseen data. This phenomenon, known as overfitting, can make the model less generalizable and more vulnerable to unexpected inputs. Our team employs techniques such as cross-validation and regularization to mitigate overfitting, ensuring that our clients' models maintain high accuracy across various scenarios.
- Lack of Interpretability: Many AI models, especially deep learning models, operate as "black boxes." This lack of transparency can make it difficult to understand how decisions are made, leading to trust issues among users and stakeholders. Rapid Innovation emphasizes the importance of model interpretability and provides solutions that enhance transparency, allowing clients to explain AI-driven decisions to their stakeholders effectively.
- Dependency on Feature Engineering: AI models often rely on specific features extracted from the data. If these features are not well-defined or are manipulated, the model's performance can degrade significantly. Our experts at Rapid Innovation work closely with clients to identify and engineer relevant features, optimizing model performance and ensuring that the AI systems are robust against variations in input data.
- Model Drift: Over time, the data distribution may change, leading to a decline in model performance. This phenomenon, known as model drift, necessitates regular updates and retraining of the model to maintain accuracy. Rapid Innovation offers ongoing support and monitoring services to help clients adapt their models to evolving data landscapes, ensuring sustained performance and relevance.
4.3.2. Adversarial Attacks
Adversarial attacks are deliberate attempts to deceive AI models by introducing subtle perturbations to the input data. These attacks can exploit the vulnerabilities of AI systems, leading to incorrect predictions or classifications.
- Types of Attacks: Â
- Evasion Attacks: These involve modifying input data to evade detection or mislead the model. For example, altering an image slightly can cause a model to misclassify it. Rapid Innovation implements advanced detection mechanisms to identify and mitigate such attacks, enhancing the security of our clients' AI systems.
- Poisoning Attacks: In this scenario, attackers inject malicious data into the training set, which can corrupt the model's learning process and lead to poor performance. Our team develops robust training protocols that minimize the risk of poisoning attacks, ensuring the integrity of the training data.
- Model Inversion: Attackers can infer sensitive information about the training data by querying the model, potentially exposing private data. Rapid Innovation employs privacy-preserving techniques to safeguard sensitive information, protecting our clients from potential data breaches.
- Impact on Security: Adversarial attacks can have severe implications, especially in critical applications like autonomous driving, facial recognition, and cybersecurity. A successful attack can lead to catastrophic failures or breaches. We prioritize security in our AI solutions, providing clients with comprehensive strategies to defend against adversarial threats.
- Defense Mechanisms: Â
- Adversarial Training: This involves training the model on both clean and adversarial examples to improve its robustness. Rapid Innovation integrates adversarial training into our development processes, enhancing model resilience.
- Input Sanitization: Filtering or preprocessing inputs to remove potential adversarial perturbations can help mitigate risks. Our team implements input sanitization techniques to ensure that only valid data is processed by the models.
- Model Regularization: Techniques like dropout and weight decay can enhance model robustness against adversarial attacks. We apply these techniques to strengthen our clients' models against potential vulnerabilities.
4.3.3. False Positives/Negatives
False positives and false negatives are critical metrics in evaluating the performance of AI models, particularly in classification tasks. Understanding these concepts is essential for improving model accuracy and reliability.
- False Positives: This occurs when a model incorrectly identifies a negative instance as positive. For example, in a spam detection system, a legitimate email may be flagged as spam. This can lead to user frustration and loss of trust in the system, as well as increased operational costs due to unnecessary actions taken on false alarms. Rapid Innovation helps clients fine-tune their models to minimize false positives, enhancing user satisfaction and operational efficiency.
- False Negatives: Conversely, false negatives happen when a model fails to identify a positive instance, such as a spam email being classified as legitimate. This can result in security risks, especially in applications like fraud detection or malware identification, and missed opportunities for businesses, as potential leads or customers may be overlooked. Our team focuses on optimizing recall to reduce false negatives, ensuring that clients do not miss critical opportunities.
4.3.4. Privacy Concerns
- Â
Privacy concerns are increasingly significant in today's digital landscape, especially with the rise of data collection and surveillance technologies. These concerns can be categorized into several key areas:
- Data Collection: Organizations often collect vast amounts of personal data, sometimes without explicit consent. This raises questions about how this data is used and who has access to it. Rapid Innovation can assist organizations in implementing AI-driven data management solutions, including data privacy compliance software, that ensure compliance with privacy regulations while optimizing data usage for business insights.
- Surveillance: The use of surveillance technologies, such as cameras and tracking software, can infringe on individual privacy. Many people are unaware of the extent to which they are being monitored, leading to a sense of vulnerability. By leveraging blockchain technology, Rapid Innovation can help create transparent systems that allow users to understand and control how their data is being collected and used.
- Data Breaches: High-profile data breaches have exposed sensitive information, leading to identity theft and financial loss. The frequency of these breaches has heightened public awareness and concern regarding data security. Rapid Innovation offers robust cybersecurity solutions, including AI-based threat detection and response systems, to help organizations proactively safeguard their data.
- Regulatory Compliance: Laws such as the General Data Protection Regulation (GDPR) and the California Consumer Privacy Act (CCPA) aim to protect consumer privacy. Organizations must navigate these regulations to avoid penalties and maintain consumer trust. Rapid Innovation provides consulting services to help businesses understand and implement compliance strategies effectively, including the use of GDPR compliance software and CCPA compliance software, ensuring they meet regulatory requirements while enhancing customer trust.
- User Awareness: Many users lack understanding of their rights regarding data privacy. Educating users about their rights and how to protect their information is crucial in addressing privacy concerns. Rapid Innovation can develop AI-driven educational platforms that empower users with knowledge about their data rights and privacy protection measures, utilizing privacy management software to facilitate this education.
- Ethical Considerations: Companies must consider the ethical implications of their data practices. Transparency in data handling and a commitment to user privacy can enhance trust and loyalty. Rapid Innovation advocates for ethical AI and blockchain practices, helping organizations build trust with their customers through responsible data management, including the implementation of best data privacy management software.
5. Use Cases and Applications
The applications of technology are vast and varied, impacting numerous sectors. Understanding these use cases can help organizations leverage technology effectively.
5.1. Network Security
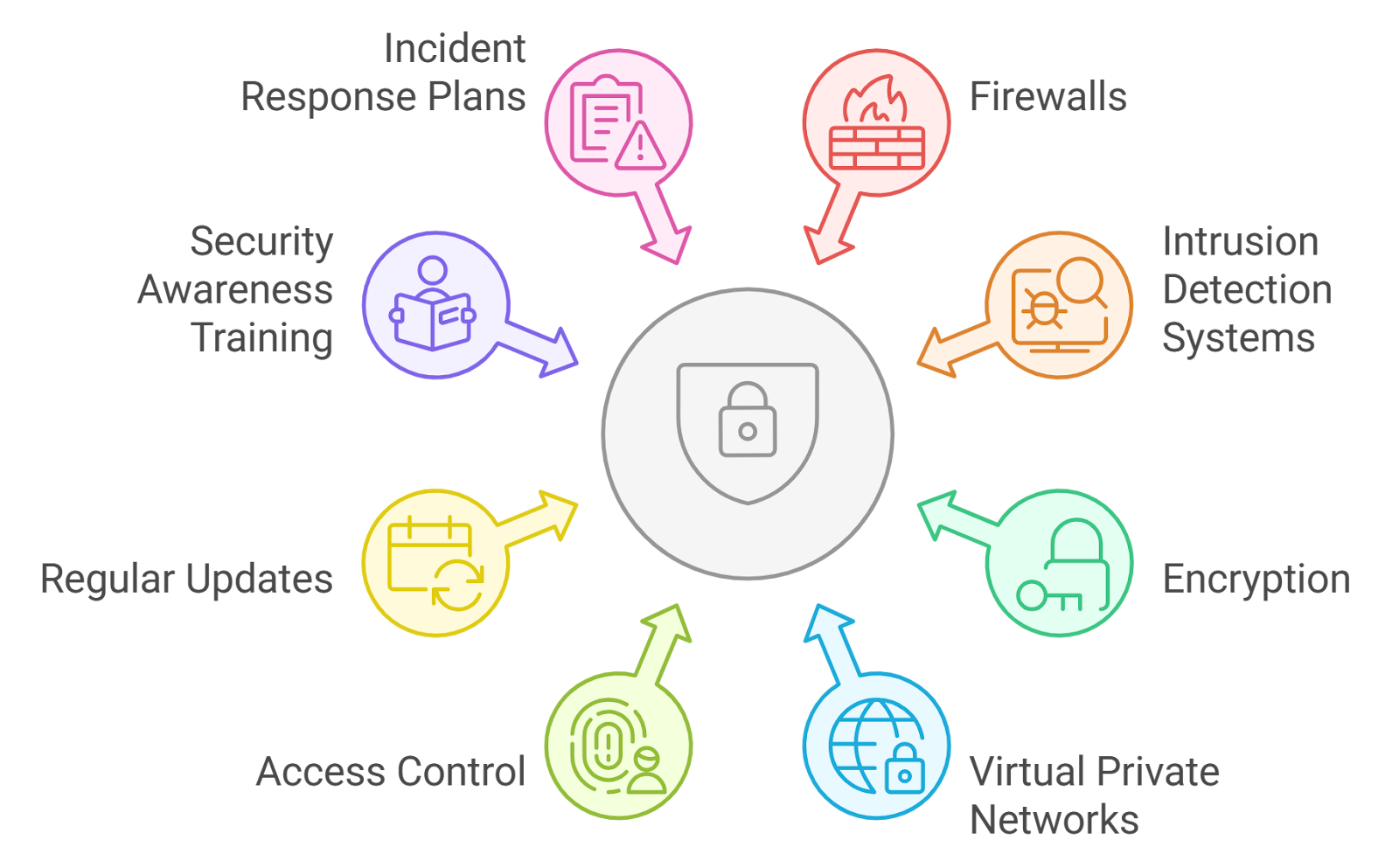
‍
Network security is a critical aspect of information technology, focusing on protecting networks from unauthorized access, misuse, or damage. Key components of network security include:
- Firewalls: These act as barriers between trusted internal networks and untrusted external networks. They monitor and control incoming and outgoing network traffic based on predetermined security rules.
- Intrusion Detection Systems (IDS): IDS monitor network traffic for suspicious activity and potential threats. They can alert administrators to potential breaches, allowing for quick responses.
- Encryption: Encrypting data ensures that even if it is intercepted, it cannot be read without the appropriate decryption key. This is essential for protecting sensitive information during transmission.
- Virtual Private Networks (VPNs): VPNs create secure connections over the internet, allowing users to access private networks remotely while maintaining privacy and security.
- Access Control: Implementing strict access controls ensures that only authorized users can access sensitive data and systems. This can include multi-factor authentication and role-based access controls.
- Regular Updates and Patching: Keeping software and systems updated is crucial for protecting against vulnerabilities. Regular patching helps mitigate risks associated with outdated software.
- Security Awareness Training: Educating employees about security best practices can significantly reduce the risk of human error, which is often a leading cause of security breaches.
- Incident Response Plans: Having a well-defined incident response plan ensures that organizations can quickly and effectively respond to security incidents, minimizing damage and recovery time.
By focusing on these aspects of network security, organizations can better protect their data and maintain the integrity of their networks. Rapid Innovation is committed to providing tailored solutions that enhance security and privacy, ultimately driving greater ROI for our clients, including the deployment of privacy compliance solutions and data privacy platforms.
5.2. Endpoint Protection
Endpoint protection is a critical component of cybersecurity that focuses on securing endpoints, such as laptops, desktops, and mobile devices, from threats. As the number of devices connected to corporate networks increases, so does the potential attack surface for cybercriminals.
- Endpoint protection solutions, including symantec endpoint protection and antivirus endpoint protection, typically include antivirus software, anti-malware tools, and firewalls.
- These solutions work to detect, block, and respond to threats in real-time, ensuring that endpoints are safeguarded against malware, ransomware, and other cyber threats.
- Advanced endpoint protection, such as norton endpoint protection and systematic endpoint protection, often incorporates machine learning and artificial intelligence to identify and mitigate threats based on behavior rather than relying solely on known signatures. Rapid Innovation leverages AI algorithms to enhance threat detection capabilities, allowing organizations to respond swiftly and effectively to emerging threats.
- Regular updates and patches are essential to maintain the effectiveness of endpoint protection solutions, as new vulnerabilities are discovered frequently. Our consulting services can help organizations establish a robust patch management strategy to ensure timely updates.
- Organizations should also implement policies for device management, ensuring that only authorized devices can access sensitive data. Rapid Innovation can assist in developing comprehensive device management policies tailored to your organization's specific needs, including endpoint security management.
5.3. Cloud Security
Cloud security refers to the set of policies, technologies, and controls designed to protect data, applications, and infrastructure associated with cloud computing. As businesses increasingly migrate to the cloud, ensuring robust security measures is paramount.
- Cloud security encompasses various aspects, including data protection, identity and access management, and compliance with regulations.
- Organizations must ensure that data stored in the cloud is encrypted both at rest and in transit to prevent unauthorized access. Rapid Innovation can implement advanced encryption techniques to safeguard your data effectively.
- Multi-factor authentication (MFA) is a critical component of cloud security, adding an extra layer of protection against unauthorized access. Our team can help integrate MFA solutions that align with your organizational requirements.
- Regular audits and assessments of cloud security practices help identify vulnerabilities and ensure compliance with industry standards. Rapid Innovation offers comprehensive security assessments to help organizations stay ahead of potential threats.
- It is essential to choose reputable cloud service providers that offer strong security measures and transparent policies regarding data handling and privacy. We can guide you in selecting the right cloud service provider that meets your security needs.
5.4. Application Security
Application security involves measures taken to improve the security of applications by identifying, fixing, and preventing security vulnerabilities. As applications become more complex and interconnected, the need for robust application security practices has never been greater.
- Secure coding practices are fundamental to application security, ensuring that developers write code that is resistant to common vulnerabilities such as SQL injection and cross-site scripting (XSS). Rapid Innovation provides training and resources to help your development team adopt secure coding practices.
- Regular security testing, including static and dynamic analysis, helps identify vulnerabilities before applications are deployed. Our automated testing solutions can streamline this process, ensuring thorough assessments.
- Implementing a secure software development lifecycle (SDLC) ensures that security is integrated into every phase of application development. Rapid Innovation can assist in establishing an SDLC that prioritizes security without compromising development speed.
- Organizations should also conduct regular penetration testing to simulate attacks and identify weaknesses in their applications. Our expert team can perform penetration tests tailored to your applications, providing actionable insights for improvement.
- Continuous monitoring of applications post-deployment is crucial to detect and respond to any emerging threats or vulnerabilities. Rapid Innovation offers ongoing monitoring solutions that leverage AI to identify and address potential security issues in real-time.
5.5. Identity and Access Management
Identity and Access Management (IAM) is a critical component of cybersecurity that focuses on ensuring that the right individuals have the appropriate access to technology resources. IAM encompasses policies, processes, and technologies that help organizations manage digital identities and control user access to sensitive information, including solutions like google privileged access management and privileged identity management.
- Centralized user management: IAM systems allow organizations to manage user identities from a single platform, streamlining the process of adding, modifying, or removing user access. Rapid Innovation can implement tailored IAM solutions, such as identity management and access management, that enhance operational efficiency and reduce administrative overhead, ultimately leading to a greater return on investment (ROI).
- Role-based access control (RBAC): This approach assigns permissions based on user roles, ensuring that individuals only have access to the information necessary for their job functions. By leveraging RBAC, organizations can minimize the risk of data breaches and ensure compliance with industry regulations, which can significantly enhance their bottom line.
- Multi-factor authentication (MFA): MFA adds an extra layer of security by requiring users to provide two or more verification factors to gain access, significantly reducing the risk of unauthorized access. Rapid Innovation can help clients implement MFA solutions that not only bolster security but also instill confidence in customers and stakeholders.
- Single sign-on (SSO): SSO enables users to log in once and gain access to multiple applications, improving user experience while maintaining security. By streamlining access, organizations can enhance productivity and reduce the time spent on password management, leading to improved operational efficiency. Solutions like microsoft identity manager can be integrated to facilitate SSO.
- Compliance and auditing: IAM solutions help organizations comply with regulations by providing detailed logs of user access and activities, making it easier to conduct audits and identify potential security breaches. Rapid Innovation's expertise in IAM can assist clients in navigating complex compliance landscapes, ultimately reducing the risk of costly penalties. This includes utilizing cloud based identity management solutions and cloud based IAM solutions. For more insights on how decentralized identity systems are transforming digital security and privacy, visit this link.
5.6. Threat Hunting
Threat hunting is a proactive cybersecurity practice that involves actively searching for signs of malicious activity within an organization's network. Unlike traditional security measures that rely on automated alerts, threat hunting requires skilled analysts to investigate potential threats before they can cause harm.
- Continuous monitoring: Threat hunters continuously analyze network traffic, logs, and endpoint data to identify anomalies that may indicate a security breach. Rapid Innovation employs advanced AI-driven analytics to enhance monitoring capabilities, allowing organizations to detect threats in real-time and respond swiftly.
- Hypothesis-driven approach: Threat hunters develop hypotheses based on known attack patterns and behaviors, allowing them to focus their investigations on specific areas of concern. This targeted approach not only improves detection rates but also optimizes resource allocation, leading to a more effective security posture.
- Use of advanced tools: Threat hunting often involves the use of sophisticated tools and technologies, such as machine learning and artificial intelligence, to enhance detection capabilities. Rapid Innovation integrates cutting-edge technologies into threat hunting practices, ensuring that clients stay ahead of emerging threats.
- Collaboration with incident response teams: Threat hunters work closely with incident response teams to share findings and improve overall security posture. By fostering collaboration, organizations can enhance their incident response capabilities and reduce the impact of security incidents.
- Reducing dwell time: By identifying threats early, organizations can significantly reduce the time attackers remain undetected within their systems, minimizing potential damage. Rapid Innovation's proactive threat hunting services empower clients to mitigate risks effectively, ultimately protecting their assets and reputation.
5.7. Incident Response
Incident response refers to the systematic approach an organization takes to prepare for, detect, and respond to cybersecurity incidents. A well-defined incident response plan is essential for minimizing the impact of security breaches and ensuring a swift recovery.
- Preparation: Organizations must establish an incident response team, develop policies, and conduct training to ensure readiness for potential incidents. Rapid Innovation can assist in creating comprehensive incident response plans tailored to specific organizational needs, ensuring preparedness for any eventuality.
- Detection and analysis: This phase involves identifying and assessing security incidents through monitoring tools, alerts, and user reports to determine the scope and severity of the threat. By leveraging AI-driven analytics, Rapid Innovation enhances detection capabilities, allowing organizations to respond more effectively.
- Containment: Once an incident is confirmed, immediate actions are taken to contain the threat, preventing further damage and protecting critical assets. Rapid Innovation's incident response strategies focus on minimizing downtime and safeguarding vital resources.
- Eradication: After containment, the root cause of the incident is identified and eliminated to prevent recurrence. Our expertise ensures that organizations can effectively address vulnerabilities, reducing the likelihood of future incidents.
- Recovery: This phase focuses on restoring affected systems and services to normal operation while ensuring that vulnerabilities are addressed. Rapid Innovation supports clients in implementing recovery strategies that prioritize business continuity and resilience.
- Post-incident review: After an incident, organizations conduct a thorough review to analyze the response, identify lessons learned, and improve future incident response efforts. Rapid Innovation facilitates these reviews, helping clients refine their incident response processes and enhance overall security posture, including the integration of IAM identity access management and cloud IAM solutions.
6. Implementation Strategies
Effective cybersecurity implementation strategies are crucial for the successful deployment of any project, especially in the realm of cybersecurity. These strategies ensure that all aspects of the project are considered, from initial assessments to ongoing evaluations.
6.1 Assessment and Planning
Assessment and planning form the foundation of any cybersecurity implementation strategy. This phase involves a thorough evaluation of the current environment, identifying gaps, and establishing a roadmap for improvement. Key activities in this phase include:
- Conduct a comprehensive analysis of existing systems and processes.
- Identify key stakeholders and their roles in the implementation process.
- Set clear objectives and goals that align with the overall business strategy.
- Develop a timeline for implementation, including milestones and deadlines.
- Allocate resources effectively, ensuring that both human and financial resources are in place.
A well-structured assessment and planning phase can significantly reduce risks and enhance the effectiveness of the cybersecurity implementation strategy.
6.1.1 Security Posture Evaluation
Security posture evaluation is a critical component of the assessment phase. It involves analyzing an organization’s current security measures and identifying vulnerabilities that could be exploited by cyber threats. This evaluation includes:
- Review existing security policies and procedures to ensure they are up-to-date and effective.
- Conduct vulnerability assessments and penetration testing to identify weaknesses in the system.
- Evaluate the effectiveness of current security tools and technologies.
- Assess employee awareness and training regarding security best practices.
- Benchmark against industry standards and regulations to ensure compliance.
By conducting a thorough security posture evaluation, organizations can gain insights into their strengths and weaknesses, allowing them to prioritize areas for improvement. This proactive approach helps in mitigating risks and enhancing overall security.
Incorporating these cybersecurity implementation strategies into the implementation process not only strengthens the security framework but also fosters a culture of continuous improvement within the organization. At Rapid Innovation, we leverage our expertise in AI and Blockchain to enhance these strategies, ensuring that our clients achieve greater ROI through optimized processes and robust security measures. By integrating advanced technologies, we help organizations not only meet their immediate cybersecurity needs but also position them for future growth and resilience.
6.1.2. Requirements Analysis
Requirements analysis is a critical phase in any project, particularly in software development and system implementation. This process involves gathering, analyzing, and documenting the needs and expectations of stakeholders to ensure that the final product meets their requirements.
- Identify stakeholders: Engage with all relevant parties, including end-users, project sponsors, and technical teams, to gather diverse perspectives.
- Elicit requirements: Use techniques such as interviews, surveys, and workshops to collect detailed information about what stakeholders need from the system. This includes understanding business analyst job requirements and the qualifications required for business analyst roles.
- Categorize requirements: Classify requirements into functional (what the system should do) and non-functional (how the system should perform) categories for better organization. This may involve requirements analysis techniques and requirement elicitation techniques.
- Validate requirements: Ensure that the gathered requirements are feasible, clear, and aligned with business objectives through stakeholder reviews and feedback.
- Document requirements: Create a comprehensive requirements specification document that serves as a reference throughout the project lifecycle. This document should reflect the best business analysis certification practices and the skills required for business analysts.
Effective requirements analysis helps in minimizing misunderstandings and scope creep, ultimately leading to a more successful project outcome. According to a study, projects with well-defined requirements are 30% more likely to succeed. At Rapid Innovation, we leverage advanced AI tools to automate parts of this analysis, ensuring that we capture stakeholder needs accurately and efficiently, which translates to a higher return on investment (ROI) for our clients. Learn more about AI agents for resource allocation.
6.1.3. Resource Planning
Resource planning is essential for ensuring that a project has the necessary human, financial, and material resources to achieve its objectives. This process involves identifying, allocating, and managing resources effectively throughout the project lifecycle.
- Assess resource needs: Determine the types and quantities of resources required based on the project scope and timeline.
- Create a resource allocation plan: Develop a detailed plan that outlines how resources will be distributed across various tasks and phases of the project.
- Monitor resource utilization: Regularly track resource usage to ensure that they are being used efficiently and effectively.
- Adjust as necessary: Be prepared to reallocate resources in response to changes in project scope, timelines, or unexpected challenges.
- Communicate with stakeholders: Keep all relevant parties informed about resource availability and any changes to the resource plan.
Proper resource planning can significantly impact project success. Research indicates that projects with effective resource management are 25% more likely to be completed on time and within budget. Rapid Innovation employs blockchain technology to enhance transparency and traceability in resource management, ensuring that all stakeholders have real-time access to resource allocation and utilization data, thereby maximizing ROI.
6.2. Deployment Approaches
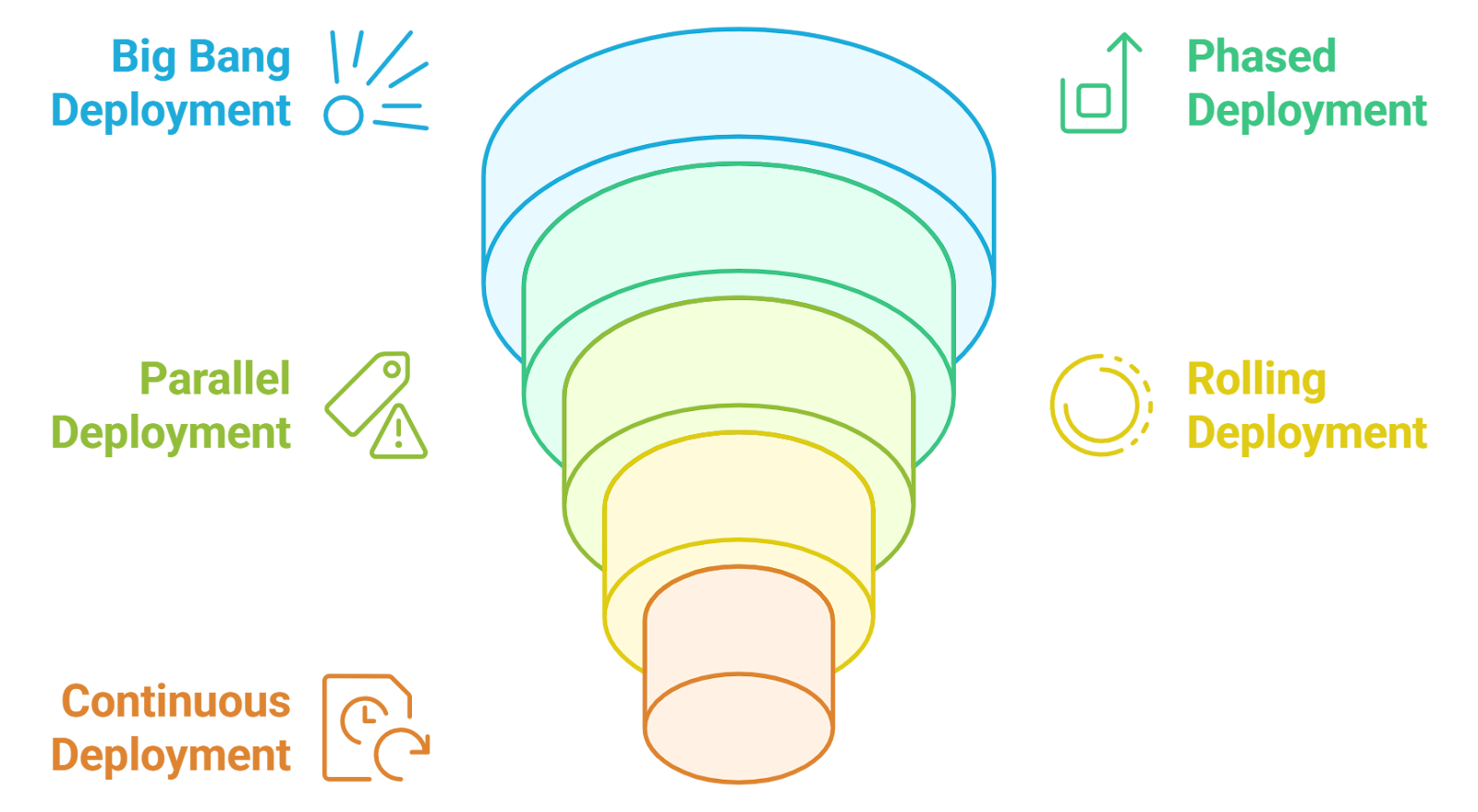
‍
Deployment approaches refer to the strategies and methodologies used to release a software product or system into a live environment. Choosing the right deployment approach is crucial for minimizing risks and ensuring a smooth transition.
- Big Bang Deployment: This approach involves deploying the entire system at once. It is suitable for smaller projects but can be risky for larger systems due to potential disruptions.
- Phased Deployment: In this method, the system is deployed in stages or phases. This allows for testing and feedback at each stage, reducing the risk of major failures.
- Parallel Deployment: This approach runs the new system alongside the existing one for a period. It allows users to transition gradually while ensuring that the new system is functioning correctly.
- Rolling Deployment: This method involves deploying the system to a subset of users or locations first, then gradually expanding to the entire user base. It helps in identifying issues early on.
- Continuous Deployment: In agile environments, continuous deployment allows for frequent updates and releases. This approach emphasizes automation and rapid feedback loops.
Selecting the appropriate deployment approach depends on various factors, including project size, complexity, and organizational readiness. A well-planned deployment strategy can enhance user acceptance and minimize downtime. According to industry reports, organizations that adopt phased or rolling deployment strategies experience 40% fewer post-deployment issues. Rapid Innovation's expertise in AI and blockchain ensures that we can tailor deployment strategies to meet the unique needs of our clients, ultimately driving greater efficiency and ROI.
6.2.1. Phased Implementation
Phased implementation is a strategic approach to deploying new systems, processes, or technologies in manageable segments. This method allows organizations to minimize risks and ensure smoother transitions. By breaking down the implementation into distinct phases, organizations can focus on one aspect at a time, making it easier to manage resources and timelines. Each phase can include feedback mechanisms, allowing teams to assess performance and make necessary adjustments before moving on to the next stage. Additionally, phased implementation helps identify potential issues early in the process, reducing the likelihood of large-scale failures. Organizations can allocate resources more effectively, ensuring that each phase has the necessary support without overwhelming the team. Furthermore, this approach allows for targeted training sessions, ensuring that employees are well-prepared for each new phase.
- Step-by-step approach: By breaking down the implementation into distinct phases, organizations can focus on one aspect at a time, making it easier to manage resources and timelines.
- Feedback loops: Each phase can include feedback mechanisms, allowing teams to assess performance and make necessary adjustments before moving on to the next stage.
- Risk management: Phased implementation helps identify potential issues early in the process, reducing the likelihood of large-scale failures.
- Resource allocation: Organizations can allocate resources more effectively, ensuring that each phase has the necessary support without overwhelming the team.
- Training opportunities: Phased implementation allows for targeted training sessions, ensuring that employees are well-prepared for each new phase. For more insights on effective strategies, check out successful AI integration strategies.
6.2.2. Pilot Programs
Pilot programs are small-scale trials designed to test new initiatives before full-scale implementation. They provide valuable insights and help organizations refine their strategies. These programs serve as a testing ground for new ideas, allowing organizations to evaluate their feasibility and effectiveness in a controlled environment. They generate data that can inform decision-making, helping organizations understand potential challenges and successes. Involving stakeholders in pilot programs fosters buy-in and support for the initiative, as they can see firsthand the benefits and challenges. Feedback from pilot programs can lead to iterative improvements, ensuring that the final implementation is more effective and aligned with organizational goals. By identifying issues early, pilot programs can save organizations money in the long run by preventing costly mistakes during full-scale implementation.
- Testing ground: Pilot programs serve as a testing ground for new ideas, allowing organizations to evaluate their feasibility and effectiveness in a controlled environment.
- Data collection: These programs generate data that can inform decision-making, helping organizations understand potential challenges and successes.
- Stakeholder engagement: Involving stakeholders in pilot programs fosters buy-in and support for the initiative, as they can see firsthand the benefits and challenges.
- Iterative improvements: Feedback from pilot programs can lead to iterative improvements, ensuring that the final implementation is more effective and aligned with organizational goals.
- Cost-effectiveness: By identifying issues early, pilot programs can save organizations money in the long run by preventing costly mistakes during full-scale implementation.
6.2.3. Integration Methods
Integration methods refer to the various techniques used to combine new systems or processes with existing ones. Effective integration is crucial for ensuring seamless operations and maximizing the benefits of new initiatives. Application Programming Interfaces (APIs) allow different software systems to communicate and share data, facilitating smoother integration. Middleware acts as a bridge between different applications, enabling them to work together without requiring significant changes to existing systems. Proper data migration is essential for integration, ensuring that information is transferred accurately and securely from old systems to new ones. Training users on new integration methods is vital for ensuring that they can effectively utilize the combined systems and processes. Ongoing monitoring of integrated systems helps identify issues early, allowing for timely adjustments and improvements.
- API integration: Application Programming Interfaces (APIs) allow different software systems to communicate and share data, facilitating smoother integration.
- Middleware solutions: Middleware acts as a bridge between different applications, enabling them to work together without requiring significant changes to existing systems.
- Data migration strategies: Proper data migration is essential for integration, ensuring that information is transferred accurately and securely from old systems to new ones.
- User training: Training users on new integration methods is vital for ensuring that they can effectively utilize the combined systems and processes.
- Continuous monitoring: Ongoing monitoring of integrated systems helps identify issues early, allowing for timely adjustments and improvements.
At Rapid Innovation, we leverage these methodologies to ensure that our clients can implement AI and Blockchain solutions effectively, leading to greater ROI and enhanced operational efficiency. By utilizing phased implementation strategies, pilot programs, and robust integration methods, we help organizations navigate the complexities of technology adoption while minimizing risks and maximizing benefits. Additionally, our expertise as an AI agent development company ensures that we can provide tailored solutions to meet your specific needs.
6.3. Performance Monitoring
Performance monitoring is a critical aspect of maintaining the efficiency and effectiveness of any system, application, or process. It involves the continuous observation and analysis of performance metrics to ensure that everything operates within desired parameters.
- Key performance indicators (KPIs) should be established to measure success.
- Regularly track metrics such as response time, throughput, and resource utilization.
- Utilize monitoring tools like New Relic, Datadog, or Prometheus for real-time insights, including application performance monitoring and APM tools.
- Set up alerts for anomalies or performance degradation to enable quick responses.
- Analyze historical data to identify trends and predict future performance issues.
- Conduct regular reviews of performance reports to assess system health and make informed decisions.
Effective performance monitoring not only helps in identifying issues but also aids in capacity planning and resource allocation. By understanding how systems perform under various loads, organizations can better prepare for peak usage times and avoid potential bottlenecks. At Rapid Innovation, we leverage advanced AI algorithms to enhance performance monitoring, enabling our clients to achieve greater ROI by proactively addressing performance issues before they impact business operations. This includes utilizing application performance management tools and application monitoring tools to ensure optimal performance. For more insights on maintaining best practices, visit this link.
6.4. Optimization and Tuning
Optimization and tuning are essential processes that enhance the performance of systems and applications. These practices involve adjusting configurations, refining code, and improving resource allocation to achieve better efficiency.
- Identify bottlenecks through performance monitoring data, including insights from application performance monitoring solutions.
- Optimize database queries to reduce load times and improve response rates.
- Fine-tune server configurations to maximize resource utilization, utilizing server monitor tools and software for server monitoring.
- Implement caching strategies to decrease latency and improve user experience.
- Regularly review and refactor code to eliminate inefficiencies.
- Use load testing tools to simulate high traffic and identify weaknesses.
Optimization is an ongoing process that requires regular assessment and adjustment. By continuously tuning systems, organizations can ensure they meet performance expectations and provide a seamless experience for users. Rapid Innovation employs cutting-edge blockchain technology to optimize data integrity and security, ensuring that our clients not only enhance performance but also maintain trust and transparency in their operations.
7. Best Practices and Guidelines
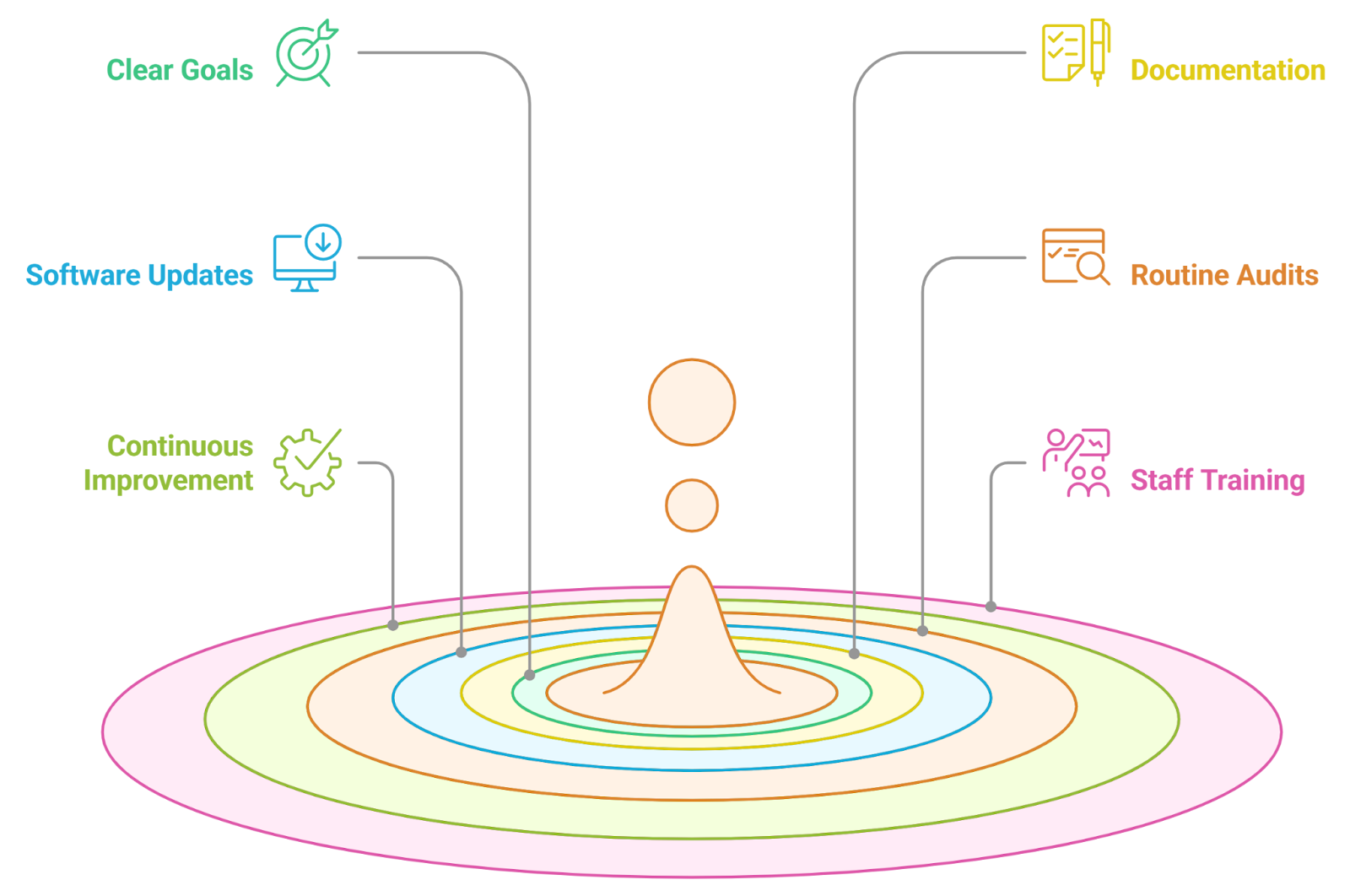
‍
Implementing best practices and guidelines is crucial for achieving optimal performance and reliability in any system. These practices help streamline processes, reduce errors, and enhance overall efficiency.
- Establish clear performance goals and objectives.
- Document all configurations and changes for future reference.
- Regularly update software and systems to benefit from the latest features and security patches, including application performance monitoring tools.
- Conduct routine audits to ensure compliance with performance standards.
- Foster a culture of continuous improvement by encouraging feedback and suggestions from team members.
- Train staff on best practices for performance monitoring and optimization, including the use of APM tooling and application performance tools.
By adhering to these best practices, organizations can create a robust framework that supports sustained performance and reliability. This proactive approach not only mitigates risks but also positions the organization for future growth and success. At Rapid Innovation, we guide our clients in implementing these best practices, ensuring they harness the full potential of AI and blockchain technologies to drive efficiency and achieve their business goals.
7.1. Data Management
Data management is a critical aspect of any data-driven project, especially in fields like machine learning and artificial intelligence. Effective data management ensures that data is collected, stored, processed, and utilized efficiently, ultimately leading to greater ROI for businesses.
- Data Collection: Â
- Gather data from reliable sources to ensure quality, which is essential for accurate model predictions.
- Use automated tools for data scraping and collection to save time and reduce manual errors, allowing your team to focus on strategic initiatives.
- Data Storage: Â
- Choose appropriate storage solutions, such as cloud storage or on-premises databases, tailored to your business needs.
- Implement data warehousing for structured data analysis, enabling faster insights and decision-making.
- Data Processing: Â
- Clean and preprocess data to remove inconsistencies and errors, ensuring that your models are trained on high-quality data.
- Use data transformation techniques to convert raw data into a usable format, enhancing the efficiency of your analytics processes.
- Data Governance: Â
- Establish policies for data usage, access, and sharing to maintain data integrity and security.
- Ensure compliance with regulations like GDPR and HIPAA to protect sensitive information, thereby reducing the risk of costly penalties.
- Data Quality: Â
- Regularly assess data quality through validation checks to maintain the reliability of your insights.
- Implement data profiling to understand data characteristics and improve accuracy, which can lead to better business outcomes.
- Machine Learning Data Management: Â
- Integrate machine learning data management practices to streamline the handling of datasets specifically for machine learning applications.
- Utilize master data management machine learning techniques to ensure consistency and accuracy across your data assets.
- Dataset Management for Machine Learning: Â
- Focus on dataset management for machine learning to optimize the organization and accessibility of data used in model training.
- Implement machine learning metadata management to track data lineage and enhance the understanding of data sources.
7.2. Model Training and Validation
Model training and validation are essential steps in developing machine learning models. These processes ensure that the model performs well on unseen data and generalizes effectively, ultimately driving business success.
- Model Training: Â
- Split the dataset into training and testing sets to evaluate model performance accurately.
- Use various algorithms and techniques, such as supervised and unsupervised learning, to train models that align with your business objectives.
- Hyperparameter Tuning: Â
- Optimize model parameters to improve performance, ensuring that your models deliver the best possible results.
- Use techniques like grid search or random search for effective tuning, maximizing the efficiency of your model development process.
- Cross-Validation: Â
- Implement k-fold cross-validation to assess model stability and reliability. This technique helps in reducing overfitting and ensures that the model performs well across different subsets of data, enhancing its robustness.
- Performance Metrics: Â
- Evaluate model performance using metrics like accuracy, precision, recall, and F1 score, selecting the right metrics based on your specific use case and business objectives.
- This targeted approach allows for more informed decision-making and resource allocation.
- Model Validation: Â
- Test the model on a separate validation dataset to ensure it generalizes well, reducing the risk of deploying ineffective models.
- Conduct A/B testing to compare the performance of different models in real-world scenarios, providing insights into which model best meets your business needs.
7.3. Security Controls
Security controls are vital for protecting data and ensuring the integrity of machine learning models. Implementing robust security measures helps mitigate risks associated with data breaches and unauthorized access, safeguarding your business's reputation and assets.
- Data Encryption: Â
- Use encryption techniques to protect sensitive data both at rest and in transit, ensuring that your data remains secure from unauthorized access.
- Implement end-to-end encryption to safeguard data throughout its lifecycle, enhancing trust with your clients.
- Access Control: Â
- Establish strict access controls to limit who can view or manipulate data, reducing the risk of internal threats.
- Use role-based access control (RBAC) to assign permissions based on user roles, ensuring that only authorized personnel have access to sensitive information.
- Regular Audits: Â
- Conduct regular security audits to identify vulnerabilities in the system, allowing for proactive measures to be taken.
- Implement penetration testing to simulate attacks and assess security measures, ensuring that your defenses are robust.
- Incident Response Plan: Â
- Develop a comprehensive incident response plan to address potential security breaches, minimizing the impact on your business.
- Ensure that all team members are trained on the response procedures, fostering a culture of security awareness.
- Compliance and Regulations: Â
- Stay updated on relevant security regulations and compliance requirements to avoid legal repercussions.
- Implement necessary measures to adhere to standards like ISO 27001 and NIST, reinforcing your commitment to data security.
By focusing on data management, including machine learning in data management and data management for machine learning, model training and validation, and security controls, organizations can enhance their data-driven initiatives while ensuring the safety and integrity of their data and models. Rapid Innovation is dedicated to helping clients navigate these complexities, ultimately driving greater ROI and achieving business goals efficiently and effectively.
7.4. Compliance and Governance
Compliance and governance are critical components of any organization’s operational framework, particularly in industries that are heavily regulated. Ensuring adherence to laws, regulations, and internal policies is essential for maintaining trust and integrity.
- Regulatory Requirements: Organizations must comply with various regulations such as GDPR, HIPAA, and PCI-DSS. Non-compliance can lead to hefty fines and legal repercussions. Rapid Innovation assists clients in navigating these complex regulatory landscapes by implementing AI-driven compliance solutions that automate monitoring and reporting, thereby reducing the risk of non-compliance. This includes utilizing governance risk & compliance (GRC) frameworks to ensure comprehensive adherence.
- Risk Management: Effective governance frameworks help identify, assess, and mitigate risks. This proactive approach minimizes potential threats to the organization. Our blockchain solutions enhance transparency and traceability, allowing organizations to better manage risks associated with data integrity and security. The integration of governance risk and compliance (GRC) strategies is essential in this process.
- Policy Development: Establishing clear policies and procedures is vital. These documents guide employees on compliance expectations and promote a culture of accountability. Rapid Innovation collaborates with clients to develop tailored policies that align with their specific operational needs and regulatory requirements, including governance risk and compliance (GRC) policies.
- Audits and Assessments: Regular audits help ensure compliance with internal and external standards. They also provide insights into areas needing improvement. Our AI tools can streamline the audit process, providing real-time insights and analytics that help organizations stay ahead of compliance requirements, including governance risk compliance software.
- Training and Awareness: Continuous training programs for employees on compliance issues foster a culture of awareness and responsibility. Rapid Innovation offers customized training solutions that leverage AI to deliver engaging and effective compliance training tailored to the unique needs of each organization, including training on governance risk & compliance (GRC) practices. For more information on AI agents for compliance monitoring, visit this link.
7.5. Incident Response Integration
Incident response integration is essential for organizations to effectively manage and mitigate security incidents. A well-structured incident response plan ensures that teams can act swiftly and efficiently when a breach occurs.
- Preparedness: Organizations should develop and regularly update incident response plans, which includes defining roles and responsibilities for team members. Rapid Innovation helps clients create comprehensive incident response strategies that incorporate AI for predictive analysis and threat detection, ensuring alignment with governance risk and compliance (GRC) standards.
- Detection and Analysis: Implementing monitoring tools helps in the early detection of incidents. Analyzing the nature and scope of incidents is crucial for an effective response. Our AI solutions provide advanced analytics that enhance the detection and analysis of potential security threats, including those related to governance risk and compliance (GRC).
- Containment and Eradication: Quick containment of incidents prevents further damage. Following containment, organizations must eradicate the root cause to prevent recurrence. Rapid Innovation's blockchain technology ensures that once an incident is contained, the integrity of data is maintained, allowing for a thorough investigation, which is critical in governance risk and compliance (GRC) contexts.
- Recovery: After an incident, organizations should focus on restoring systems and services. This phase also includes assessing the impact and making necessary adjustments. Our team assists clients in developing recovery plans that leverage AI to optimize system restoration processes, ensuring compliance with governance risk and compliance (GRC) requirements.
- Continuous Improvement: Post-incident reviews are vital for learning from incidents. Organizations should refine their incident response plans based on these evaluations. Rapid Innovation provides tools that facilitate continuous improvement by analyzing past incidents and suggesting enhancements to response strategies, particularly in the realm of governance risk and compliance (GRC).
7.6. Team Collaboration
Team collaboration is essential for fostering a productive work environment. Effective collaboration enhances communication, innovation, and overall team performance.
- Communication Tools: Utilizing tools like Slack, Microsoft Teams, or Zoom facilitates real-time communication among team members, regardless of location. Rapid Innovation integrates AI-driven communication tools that enhance collaboration and streamline workflows, including those related to governance risk and compliance (GRC) initiatives.
- Shared Goals: Establishing common objectives aligns team efforts and encourages collaboration. Clear goals help teams stay focused and motivated. Our consulting services assist organizations in defining and aligning their strategic goals with team objectives, including those related to governance risk and compliance (GRC).
- Cross-Functional Teams: Encouraging collaboration across different departments can lead to innovative solutions and a more comprehensive understanding of projects. Rapid Innovation promotes the formation of cross-functional teams that leverage diverse expertise to drive innovation, particularly in governance risk and compliance (GRC) areas.
- Regular Meetings: Scheduling regular check-ins and brainstorming sessions promotes open dialogue and allows teams to address challenges collectively. Our solutions include AI tools that facilitate effective meeting management and follow-up processes, ensuring that governance risk and compliance (GRC) topics are consistently addressed.
- Feedback Culture: Creating an environment where team members feel comfortable providing and receiving feedback fosters continuous improvement and strengthens relationships. Rapid Innovation emphasizes the importance of feedback loops in team dynamics, helping organizations cultivate a culture of open communication and growth, especially in the context of governance risk and compliance (GRC).
8. Future Trends and Evolution
The future of technology is constantly evolving, driven by innovation and the need for efficiency. As we look ahead, several trends are emerging that will shape various industries and our daily lives.
8.1 Emerging Technologies
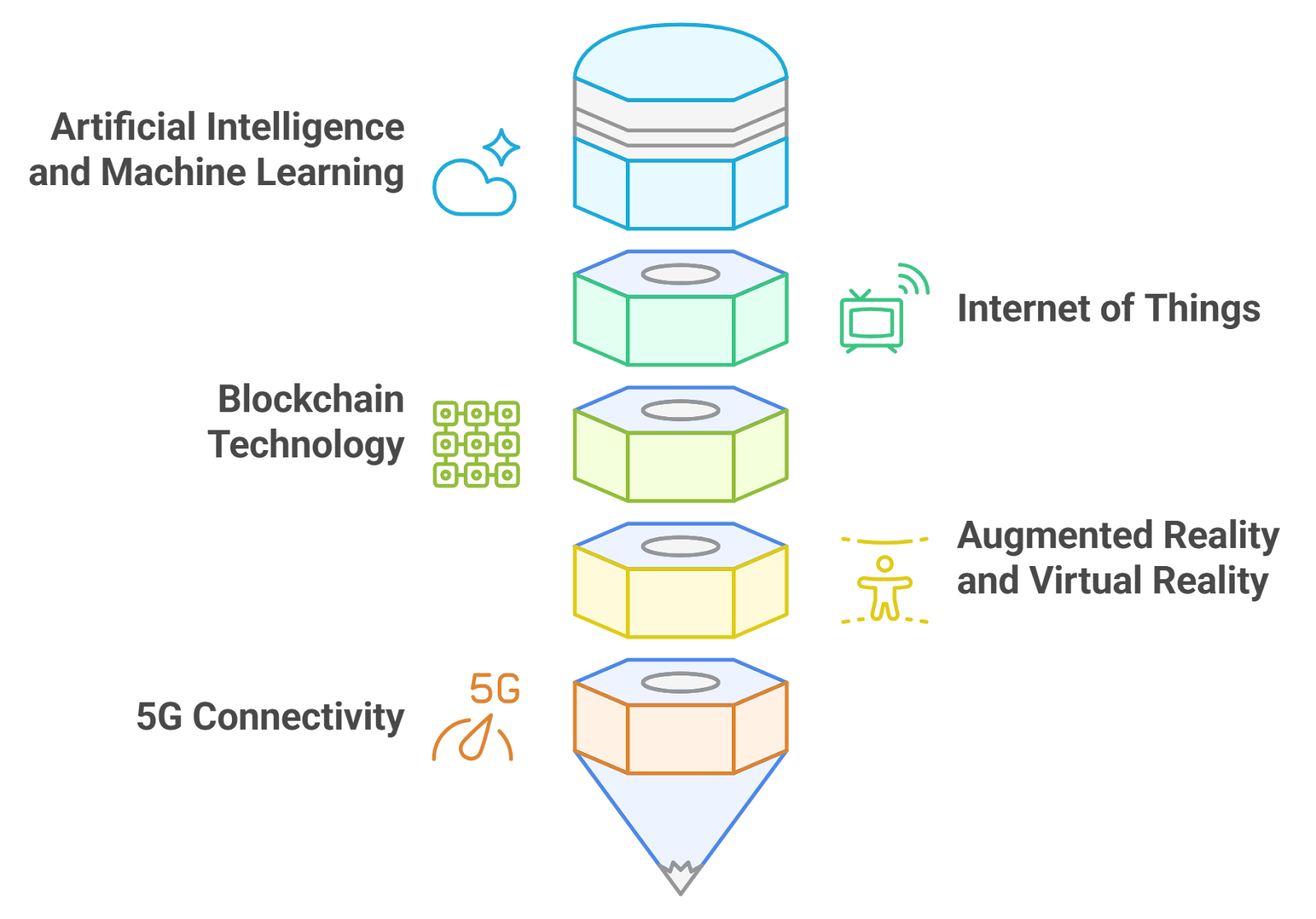
‍
Emerging technologies are at the forefront of this evolution, offering new solutions and capabilities. These technologies are not only transforming existing processes but also creating entirely new markets. Key areas of focus include:
- Artificial Intelligence (AI) and Machine Learning (ML): Rapid Innovation leverages AI and ML to enhance data analysis, enabling businesses to make informed decisions faster and achieve greater ROI through predictive analytics and automation. New AI technology is continuously being developed, enhancing capabilities in various sectors.
- Internet of Things (IoT): By connecting devices, we help clients create smarter homes and cities, optimizing resource management and improving user experiences. Emerging tech in IoT is paving the way for innovative applications.
- Blockchain technology: Our expertise in blockchain provides clients with secure and transparent transactions, enhancing trust and efficiency in their operations. New technologies in blockchain are emerging, offering even more robust solutions.
- Augmented Reality (AR) and Virtual Reality (VR): We assist businesses in utilizing AR and VR to transform the landscape of entertainment and education, creating immersive experiences that engage users. The latest technologies in AR and VR are revolutionizing user interaction.
- 5G connectivity: The rollout of 5G is set to enhance connectivity, allowing for faster data transfer and improved user experiences, which we integrate into our solutions for maximum impact. Emerging technologies in 5G are expected to further enhance these capabilities.
Each of these technologies has the potential to revolutionize how we interact with the world. The rollout of 5G is set to enhance connectivity, allowing for faster data transfer and improved user experiences.
8.1.1 Quantum Computing Impact
Quantum computing is one of the most exciting emerging technologies, promising to solve complex problems that are currently beyond the reach of classical computers. Its impact is expected to be profound across various sectors:
- Healthcare: Quantum computing can accelerate drug discovery and personalized medicine by simulating molecular interactions at unprecedented speeds, enabling healthcare providers to deliver tailored treatments. New technology in healthcare is being driven by these advancements.
- Finance: It can optimize trading strategies and risk assessments, leading to more efficient markets and improved financial decision-making. New tech technologies are emerging to support these financial innovations.
- Cryptography: Quantum computers could break traditional encryption methods, prompting the need for new security protocols, which we can help clients navigate. Emerging IT technologies are crucial in developing these new security measures.
- Logistics: They can enhance supply chain management by solving optimization problems more efficiently, reducing costs and improving delivery times. The latest technological advancements in logistics are being influenced by quantum computing.
The potential of quantum computing is immense, with estimates suggesting it could outperform classical computers by a factor of millions for specific tasks. As research and development continue, industries are beginning to explore practical applications, leading to collaborations between tech companies and academic institutions.
In conclusion, the future trends in technology, particularly the rise of emerging technologies like quantum computing, are set to redefine our world. As these innovations develop, they will create new opportunities and challenges, requiring adaptation and foresight from businesses and individuals alike. Rapid Innovation is here to guide you through this transformation, ensuring that you harness these advancements to achieve your business goals efficiently and effectively. New battery technology and other innovations will continue to emerge, further shaping the landscape of technology.
8.1.2. Edge AI Security
Edge AI security refers to the integration of artificial intelligence (AI) at the edge of networks, where data is processed closer to the source rather than in a centralized data center. This approach enhances security by reducing latency and bandwidth usage while enabling real-time decision-making. However, it also introduces unique security challenges.
- Increased attack surface: With more devices connected at the edge, the potential entry points for cyberattacks multiply.
- Data privacy concerns: Sensitive data processed at the edge may be exposed to unauthorized access if not properly secured.
- Device vulnerabilities: Edge devices often have limited processing power and may not support robust security measures, making them susceptible to attacks.
To mitigate these risks, organizations should implement strong encryption protocols, regular software updates, and continuous monitoring of edge devices. Additionally, employing AI-driven security solutions can help detect anomalies and respond to threats in real-time. At Rapid Innovation, we specialize in developing tailored edge AI security solutions that not only enhance your security posture but also ensure compliance with data privacy regulations, ultimately leading to greater ROI.
8.1.3. Autonomous Security Systems
Autonomous security systems leverage AI and machine learning to automate security processes, reducing the need for human intervention. These systems can analyze vast amounts of data, identify threats, and respond to incidents more quickly than traditional security measures.
- Real-time threat detection: Autonomous systems can monitor networks continuously, identifying potential threats as they arise.
- Automated response: These systems can take immediate action, such as isolating affected devices or blocking malicious traffic, to minimize damage.
- Enhanced efficiency: By automating routine security tasks, organizations can allocate resources more effectively and focus on strategic initiatives.
However, the reliance on autonomous systems also raises concerns about accountability and the potential for false positives. Organizations must ensure that these systems are regularly updated and monitored to maintain their effectiveness and reliability. Rapid Innovation offers consulting and development services to implement autonomous security systems that are not only efficient but also tailored to your specific business needs, ensuring a higher return on investment.
8.2. Threat Landscape Evolution
The threat landscape is constantly evolving, driven by advancements in technology and changing tactics employed by cybercriminals. Understanding this evolution is crucial for organizations to stay ahead of potential threats.
- Increased sophistication of attacks: Cybercriminals are using more advanced techniques, such as AI-driven malware and social engineering, to bypass traditional security measures.
- Rise of ransomware: Ransomware attacks have surged, with attackers targeting critical infrastructure and demanding hefty ransoms for data recovery.
- Supply chain vulnerabilities: As organizations increasingly rely on third-party vendors, the risk of supply chain attacks has grown, making it essential to assess the security posture of all partners.
To combat these evolving threats, organizations should adopt a proactive security posture, including regular risk assessments, employee training, and the implementation of advanced security technologies. Staying informed about the latest trends and threats in the cybersecurity landscape is vital for maintaining robust defenses. At Rapid Innovation, we empower organizations to navigate this complex landscape with our expertise in AI and Blockchain, ensuring that your security measures are not only effective but also aligned with your business objectives for optimal ROI.
8.3. Regulatory Changes
Regulatory changes can significantly impact various industries, influencing operational practices, compliance requirements, and overall business strategies. Staying informed about these regulatory changes is crucial for organizations to maintain compliance and avoid penalties.
- New regulations can emerge from government bodies, affecting everything from data privacy to environmental standards.
- Companies must adapt to changes in labor laws, which can influence hiring practices and employee benefits.
- Financial regulations may alter how businesses report earnings or manage risk, impacting investment strategies.
- Organizations should invest in compliance training and resources to ensure all employees understand new regulations.
- Regular audits and assessments can help identify areas of non-compliance and mitigate risks associated with regulatory changes.
For example, the General Data Protection Regulation (GDPR) has reshaped how companies handle personal data in Europe, leading to increased scrutiny and the need for robust data protection measures. At Rapid Innovation, we leverage our expertise in AI to develop compliance monitoring systems that can automatically track regulatory changes and alert organizations, ensuring they remain compliant and avoid costly penalties. Additionally, our security token development services can help organizations navigate the complexities of regulatory compliance in the evolving digital landscape. Furthermore, understanding how generative AI is changing marketing in 2024 can also play a role in adapting to these regulatory changes.
8.4. Industry Developments
Industry developments encompass trends, innovations, and shifts that can redefine market dynamics. Keeping abreast of these changes is essential for businesses to remain competitive and relevant.
- Technological advancements can lead to new products and services, enhancing customer experiences.
- Market trends, such as the rise of remote work, can influence organizational structures and workforce management.
- Sustainability initiatives are becoming increasingly important, prompting companies to adopt eco-friendly practices.
- Collaborations and partnerships within industries can drive innovation and expand market reach.
- Consumer behavior shifts, driven by social media and digital platforms, require businesses to adapt their marketing strategies.
For instance, the rapid growth of e-commerce has transformed retail, compelling traditional brick-and-mortar stores to enhance their online presence and digital marketing efforts. Rapid Innovation assists clients in navigating these industry developments by integrating blockchain solutions that enhance transparency and trust in supply chains, ultimately improving customer satisfaction and loyalty.
9. Measuring Success and ROI
Measuring success and return on investment (ROI) is vital for understanding the effectiveness of business strategies and initiatives. Organizations must establish clear metrics to evaluate performance and make informed decisions.
- Define specific, measurable goals aligned with overall business objectives.
- Utilize key performance indicators (KPIs) to track progress and assess outcomes.
- Analyze financial metrics, such as revenue growth, profit margins, and cost savings, to gauge ROI.
- Implement customer feedback mechanisms to evaluate satisfaction and loyalty.
- Regularly review and adjust strategies based on performance data to optimize results.
For example, a marketing campaign can be assessed by tracking metrics like conversion rates, customer acquisition costs, and overall sales growth. By analyzing these factors, businesses can determine the effectiveness of their marketing efforts and make necessary adjustments for future campaigns. At Rapid Innovation, we utilize advanced analytics and AI-driven insights to help clients measure their ROI effectively, ensuring they achieve their business goals efficiently and effectively.
9.1. Key Performance Indicators
Key Performance Indicators (KPIs) are essential metrics that help organizations measure their performance against strategic goals. In the context of business operations, KPIs provide insights into efficiency, productivity, and overall success.
- KPIs should be specific, measurable, achievable, relevant, and time-bound (SMART).
- Common KPIs include: Â
- Revenue growth rate
- Customer acquisition cost
- Employee turnover rate
- KPIs can be categorized into: Â
- Financial KPIs: Focus on financial performance, such as profit margins and return on investment (ROI). For instance, Rapid Innovation can assist clients in optimizing their financial KPIs through AI-driven analytics that identify cost-saving opportunities and enhance revenue streams.
- Operational KPIs: Measure the efficiency of business processes, like production time and order fulfillment rates. By implementing blockchain solutions, we can streamline operations, ensuring transparency and reducing delays.
- Customer KPIs: Assess customer satisfaction and retention, including Net Promoter Score (NPS) and customer lifetime value (CLV). Our AI tools can analyze customer feedback and behavior, enabling businesses to tailor their offerings and improve retention rates.
- Regularly reviewing KPIs allows organizations to adjust strategies and improve performance. Understanding the meaning of KPIs and how they function is crucial for effective management.
- Tools like dashboards and reporting software can help visualize KPI data for better decision-making. Rapid Innovation offers customized dashboard solutions that integrate AI and blockchain data, providing real-time insights for informed decision-making. Examples of KPIs can also be found in various industries, showcasing their versatility.
9.2. Security Metrics
Security metrics are quantifiable measures used to assess the effectiveness of an organization's security posture. They help identify vulnerabilities, track incidents, and ensure compliance with regulations.
- Key security metrics include: Â
- Number of security incidents: Tracks the frequency of breaches or attacks.
- Time to detect and respond: Measures how quickly an organization identifies and mitigates threats. Rapid Innovation employs AI algorithms to enhance threat detection and response times, significantly reducing potential damage.
- Compliance status: Assesses adherence to industry standards and regulations, such as GDPR or HIPAA.
- Security metrics can be divided into: Â
- Leading indicators: Predict potential security issues, such as the number of phishing attempts.
- Lagging indicators: Reflect past performance, like the number of successful breaches.
- Regularly analyzing security metrics helps organizations strengthen their defenses and reduce risks. Our blockchain solutions provide immutable records of security incidents, enhancing accountability and compliance.
- Utilizing security information and event management (SIEM) tools can enhance the collection and analysis of security metrics. Rapid Innovation can integrate advanced SIEM tools with AI capabilities to provide comprehensive security insights.
9.3. Cost-Benefit Analysis
Cost-Benefit Analysis (CBA) is a systematic approach to evaluating the financial implications of a project or decision. It helps organizations weigh the expected benefits against the associated costs to determine feasibility and value.
- Steps in conducting a CBA include: Â
- Identifying costs: Consider both direct and indirect costs, such as implementation, maintenance, and training expenses. Rapid Innovation can assist in accurately forecasting these costs through our expertise in AI and blockchain technologies.
- Estimating benefits: Quantify the expected advantages, including increased revenue, cost savings, and improved efficiency. Our clients have seen significant ROI through the implementation of AI-driven solutions that optimize operations and reduce waste.
- Comparing costs and benefits: Calculate the net present value (NPV) or return on investment (ROI) to assess overall value.
- CBA can be applied to various scenarios, including: Â
- New technology investments
- Process improvements
- Risk management initiatives
- A well-executed CBA provides a clear rationale for decision-making and helps prioritize projects based on their potential return. Rapid Innovation's expertise ensures that our clients can make informed decisions that align with their strategic goals.
- Engaging stakeholders in the CBA process can enhance buy-in and ensure all perspectives are considered. Our collaborative approach ensures that all relevant stakeholders are involved, leading to more comprehensive and effective decision-making. Understanding key performance metrics examples can also aid in this process.
9.4. Impact Assessment
Impact assessment is a critical process that evaluates the potential effects of a project, policy, or program on the environment, society, and economy. This assessment helps stakeholders understand the implications of their actions and make informed decisions.
- Purpose of Impact Assessment: Â
- Identify potential negative and positive impacts.
- Facilitate stakeholder engagement and transparency.
- Ensure compliance with legal and regulatory requirements.
- Types of Impact Assessments: Â
- Environmental Impact Assessment (EIA): Focuses on ecological consequences.
- Social Impact Assessment (SIA): Evaluates effects on communities and social structures.
- Economic Impact Assessment: Analyzes financial implications and economic benefits.
- Key Steps in Impact Assessment: Â
- Screening: Determine if an assessment is necessary.
- Scoping: Identify key issues and stakeholders.
- Assessment: Analyze potential impacts using qualitative and quantitative methods, including environmental impact analysis process and visual impact assessment.
- Mitigation: Propose measures to minimize negative impacts.
- Monitoring: Track the actual impacts post-implementation, including the impact of environmental impact assessment.
- Benefits of Impact Assessment: Â
- Enhances project design and implementation.
- Reduces risks and liabilities.
- Promotes sustainable development by balancing economic growth with environmental protection.
At Rapid Innovation, we leverage advanced AI and blockchain technologies to enhance the impact assessment process. For instance, AI algorithms can analyze vast datasets to predict potential impacts more accurately, while blockchain can ensure transparency and traceability in stakeholder engagement. This includes the eia environmental impact assessment and the environmental impact assessment act.
9.5. Continuous Improvement
Continuous improvement is an ongoing effort to enhance products, services, or processes. It is a fundamental principle in quality management and operational excellence, aiming to increase efficiency and effectiveness.
- Core Principles of Continuous Improvement: Â
- Customer Focus: Prioritize customer needs and feedback.
- Employee Involvement: Engage employees at all levels in the improvement process.
- Data-Driven Decision Making: Use data and metrics to guide improvements.
- Common Methodologies: Â
- Plan-Do-Check-Act (PDCA): A cyclical model for testing changes and implementing improvements.
- Lean: Focuses on eliminating waste and optimizing processes.
- Six Sigma: Aims to reduce defects and improve quality through statistical analysis.
- Benefits of Continuous Improvement: Â
- Increases operational efficiency and reduces costs.
- Enhances product quality and customer satisfaction.
- Fosters a culture of innovation and adaptability.
- Implementation Strategies: Â
- Establish clear goals and objectives.
- Train employees on improvement methodologies.
- Regularly review processes and outcomes for further enhancements.
Rapid Innovation employs AI-driven analytics to identify areas for improvement, enabling businesses to make informed decisions that lead to greater ROI. By integrating blockchain technology, we ensure that the processes are not only efficient but also secure and transparent.
10. Case Studies
Case studies provide real-world examples of how organizations implement strategies, face challenges, and achieve results. They serve as valuable learning tools for understanding best practices and lessons learned.
- Importance of Case Studies: Â
- Illustrate practical applications of theories and concepts.
- Offer insights into problem-solving and decision-making processes.
- Highlight successes and failures, providing a balanced view.
- Key Elements of a Case Study: Â
- Background Information: Context about the organization and the issue at hand.
- Challenges Faced: Description of the specific problems or obstacles encountered.
- Strategies Implemented: Overview of the approaches taken to address the challenges, including environmental assessment eia and impact and risk analysis.
- Results Achieved: Analysis of the outcomes, including metrics and qualitative feedback.
- Examples of Case Studies: Â
- Toyota Production System: Demonstrates lean manufacturing principles and continuous improvement.
- Starbucks: Showcases customer experience enhancement and brand loyalty strategies.
- Unilever: Highlights sustainability initiatives and their impact on business growth.
- Benefits of Analyzing Case Studies: Â
- Provides practical insights that can be applied to similar situations.
- Encourages critical thinking and analysis of different approaches.
- Serves as a source of inspiration for innovation and improvement.
At Rapid Innovation, we compile and analyze case studies that showcase the successful application of AI and blockchain technologies, providing our clients with actionable insights to drive their business forward, including examples of environmental impact assessment and eia assessment.
10.1. Enterprise Implementation Examples
Enterprise implementation refers to the process of integrating new systems, technologies, or methodologies within a large organization. Here are some notable examples:
- SAP ERP Implementation at Coca-Cola: Coca-Cola implemented SAP ERP to streamline its operations and improve data management. This integration allowed for better inventory control and enhanced reporting capabilities, leading to increased efficiency across its global operations.
- Salesforce at American Express: American Express adopted Salesforce to enhance customer relationship management (CRM). This implementation enabled the company to provide personalized services and improve customer engagement, resulting in higher customer satisfaction rates.
- Microsoft Azure at GE: General Electric (GE) transitioned to Microsoft Azure for its cloud computing needs. This move facilitated better data analytics and improved operational efficiency, allowing GE to leverage IoT technologies effectively.
These examples illustrate how large enterprises can successfully implement new technologies to enhance their operations and drive growth. At Rapid Innovation, we specialize in guiding organizations through similar implementations, including enterprise resource planning implementation and risk management in ERP implementation, leveraging our expertise in AI and Blockchain to ensure that your enterprise solutions are not only effective but also tailored to your specific business goals. If you're looking to enhance your team with cutting-edge technology, consider our services to hire generative AI engineers for your projects.
10.2. Success Stories
Success stories in enterprise implementation highlight the positive outcomes that organizations experience after adopting new systems or processes. Here are a few notable success stories:
- Netflix's Transition to Cloud: Netflix migrated its entire infrastructure to the cloud, which allowed for scalable streaming services. This transition not only improved service reliability but also enabled Netflix to expand its global reach, resulting in a significant increase in subscribers.
- Walmart's Use of Big Data: Walmart implemented big data analytics to optimize its supply chain and inventory management. By analyzing customer purchasing patterns, Walmart reduced stockouts and improved product availability, leading to increased sales and customer satisfaction.
- Zara's Fast Fashion Model: Zara adopted a unique supply chain model that emphasizes rapid production and distribution. By leveraging technology for real-time inventory management, Zara can quickly respond to fashion trends, resulting in a competitive edge in the retail market.
These success stories demonstrate how effective implementation can lead to significant improvements in efficiency, customer satisfaction, and overall business performance. Rapid Innovation can help your organization achieve similar success by integrating AI-driven insights and Blockchain technology to enhance transparency and security in your operations, including enterprise software implementation and ERP SAP implementation.
10.3. Lessons Learned
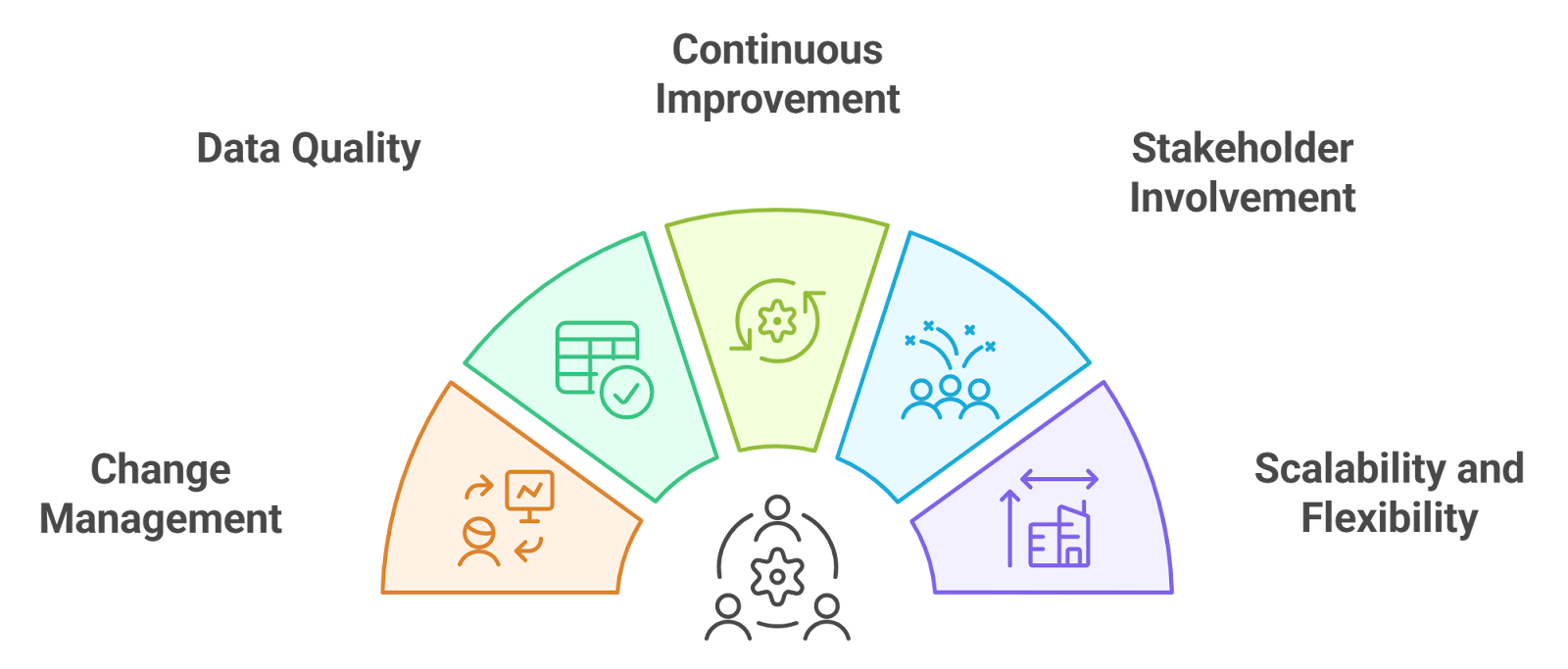
‍
The journey of enterprise implementation is often fraught with challenges. However, organizations can glean valuable lessons from their experiences. Here are some key lessons learned:
- Importance of Change Management: Successful implementation requires effective change management strategies. Engaging employees early in the process and providing adequate training can help mitigate resistance and ensure a smoother transition.
- Data Quality Matters: High-quality data is crucial for the success of any new system. Organizations must prioritize data cleansing and validation before implementation to avoid issues that can arise from inaccurate or incomplete data.
- Continuous Improvement: Implementation is not a one-time event but an ongoing process. Organizations should regularly assess the effectiveness of the new system and be open to making adjustments based on feedback and performance metrics.
- Stakeholder Involvement: Involving key stakeholders throughout the implementation process can lead to better outcomes. Their insights and feedback can help tailor the system to meet the specific needs of the organization.
- Scalability and Flexibility: Choosing systems that are scalable and flexible is essential for long-term success. As organizations grow and evolve, their systems should be able to adapt to changing needs without requiring a complete overhaul.
These lessons learned can guide organizations in future implementations, including risk in ERP implementation and examples of ERP implementation, helping them to avoid common pitfalls and achieve their desired outcomes. At Rapid Innovation, we are committed to ensuring that your enterprise implementation is not only successful but also aligned with your strategic objectives, maximizing your return on investment through innovative AI and Blockchain solutions.
10.4. Failed Implementations Analysis
Failed implementations can significantly impact an organization’s resources, reputation, and overall success. Analyzing these failures is crucial for understanding what went wrong and how to avoid similar pitfalls in the future. Here are key aspects to consider in a failed implementations analysis:
- Identifying the Causes of Failure
Many projects fail due to poorly defined goals and objectives, leading to a lack of clear direction and alignment among teams. Insufficient planning can also result in overlooked risks and challenges; thus, a comprehensive project plan is essential for anticipating potential issues. Additionally, employees may resist new systems or processes, resulting in poor adoption rates. Understanding the human element is vital for successful implementation. - Impact on Stakeholders
Failed implementations can lead to significant financial losses, including wasted resources and lost opportunities. Furthermore, when projects fail, it can cause frustration and decreased morale among employees, negatively affecting productivity and engagement levels. Organizations may also suffer reputational harm, making it difficult to regain trust from clients, partners, and stakeholders. - Lessons Learned from Failures
Involving stakeholders early in the process can help identify potential issues and foster buy-in. Continuous communication through regular updates and open lines can mitigate misunderstandings and resistance. Moreover, being willing to adjust plans based on feedback and changing circumstances can enhance the likelihood of success. - Case Studies of Failed Implementations
Many organizations have faced challenges when implementing Enterprise Resource Planning (ERP) systems, often due to inadequate training and lack of user involvement. Customer Relationship Management (CRM) systems can also fail if they do not align with business processes or if users are not adequately trained. Additionally, integrating new software with existing systems can lead to complications, especially if there is a lack of technical expertise. - Strategies for Improvement
Conducting thorough risk assessments can help organizations identify potential risks early and develop strategies to mitigate them. Investing in comprehensive training and ongoing support can enhance user adoption and satisfaction. Establishing clear metrics for success allows organizations to measure progress and make necessary adjustments. - Utilizing Technology for Better Outcomes
Leveraging project management tools can help teams stay organized and on track. Analyzing data from previous failed implementations can provide insights into what works and what doesn’t. Implementing feedback mechanisms can help organizations gather input from users and make real-time adjustments. At Rapid Innovation, we utilize advanced AI analytics to assess past project data, enabling us to provide tailored solutions that enhance the likelihood of successful implementations, including our expertise in AI agents for technical troubleshooting. - Conclusion
Analyzing failed implementations is essential for continuous improvement. By understanding the causes of failure and learning from past mistakes, organizations can enhance their chances of success in future projects. At Rapid Innovation, our expertise in AI and Blockchain allows us to guide clients through the complexities of implementation, ensuring that they achieve their business goals efficiently and effectively.