Table Of Contents
Category
Security
Surveillance
Blockchain
Artificial Intelligence
1. Introduction to AI in Cybersecurity
Artificial Intelligence (AI) is revolutionizing the field of cybersecurity by enhancing threat detection and response capabilities. As cyber threats become increasingly sophisticated, traditional security measures often fall short. AI offers a proactive approach to identifying and mitigating risks, making it an essential tool for organizations of all sizes. AI systems can analyze vast amounts of data in real-time, identifying patterns and anomalies that may indicate a security breach. Additionally, machine learning algorithms enable AI to learn from past incidents, improving its ability to predict and respond to future threats. Furthermore, AI can automate routine security tasks, allowing cybersecurity professionals to focus on more complex issues.
The integration of AI in cybersecurity is not just a trend; it is becoming a necessity. According to a report by Cybersecurity Ventures, global spending on AI in cybersecurity is expected to reach $46 billion by 2027. This growth reflects the increasing recognition of AI's potential to enhance security measures. At Rapid Innovation, we leverage AI technologies to help organizations stay ahead of cybercriminals by providing insights that are not easily discernible through manual analysis. Our AI-driven solutions enable clients to respond to threats in real-time, significantly reducing the potential damage caused by cyberattacks. Moreover, our tools assist in compliance with regulations by ensuring that security protocols are consistently followed.
AI in cybersecurity encompasses various applications, including AI used in cyber security, AI for network security, and AI cybersecurity solutions. Companies specializing in artificial intelligence security, such as darktrace cyber security and dark trace cyber security, are at the forefront of this technological shift. The rise of generative AI cybersecurity is also noteworthy, as it introduces new methods for threat detection and response.
In summary, AI is transforming cybersecurity by providing advanced threat detection and response capabilities. As organizations continue to face evolving cyber threats, the adoption of AI technologies, including AI and network security, will be crucial in safeguarding sensitive information and maintaining operational integrity. Rapid Innovation is committed to helping clients harness the power of AI to achieve their cybersecurity goals efficiently and effectively, ultimately leading to greater ROI. For more information, check out our post on AI agents for cybersecurity defense.
Refer to the image for a visual representation of how AI is enhancing cybersecurity capabilities:

1.1. Evolution of Cybersecurity Threats
Cybersecurity threats have evolved significantly over the years, adapting to technological advancements and changing user behaviors. Early threats were primarily viruses and worms, which spread through infected files and email attachments. The rise of the internet in the 1990s led to the emergence of more sophisticated attacks, such as denial-of-service (DoS) attacks, which aimed to overwhelm systems and disrupt services. In the 2000s, the focus shifted to more targeted attacks, including phishing schemes that exploited human psychology to gain sensitive information. The advent of social media and mobile devices introduced new vulnerabilities, leading to threats like ransomware, which encrypts user data and demands payment for its release. Today, threats are increasingly complex, involving advanced persistent threats (APTs) that utilize stealthy techniques to infiltrate networks and remain undetected for long periods.
The landscape of cybersecurity threats continues to evolve, with cybercriminals leveraging emerging technologies such as the Internet of Things (IoT) and cloud computing to exploit weaknesses in systems. According to a report by Cybersecurity Ventures, global cybercrime costs are expected to reach $10.5 trillion annually by 2025, highlighting the urgent need for robust cybersecurity measures. At Rapid Innovation, we understand these evolving threats, including cyber threats and cybersecurity breaches, and offer tailored AI and blockchain solutions to help organizations fortify their defenses and achieve greater ROI through enhanced security.
1.2. The Role of Artificial Intelligence
Artificial Intelligence (AI) plays a crucial role in modern cybersecurity, enhancing the ability to detect, respond to, and mitigate threats. AI algorithms can analyze vast amounts of data in real-time, identifying patterns and anomalies that may indicate a security breach. Machine learning, a subset of AI, enables systems to learn from past incidents and improve their threat detection capabilities over time. AI-driven security solutions can automate routine tasks, such as monitoring network traffic and responding to alerts, allowing cybersecurity professionals to focus on more complex issues. Predictive analytics powered by AI can forecast potential threats based on historical data, enabling organizations to proactively strengthen their defenses. AI can also enhance incident response by providing actionable insights and recommendations during a security event, reducing response times and minimizing damage.
The integration of AI in cybersecurity not only improves efficiency but also helps organizations stay ahead of evolving threats, including cybersecurity threats and cyber security services. According to a study by McKinsey, companies that adopt AI in their cybersecurity strategies can reduce the time to detect and respond to incidents by up to 90%. At Rapid Innovation, we leverage AI technologies to provide our clients with cutting-edge security solutions that drive efficiency and effectiveness, ultimately leading to a higher return on investment.
1.3. Types of AI Agents in Security
Various types of AI agents are employed in cybersecurity, each serving distinct functions to enhance security measures.
- Anomaly Detection Agents: These agents monitor network traffic and user behavior to identify deviations from established patterns, signaling potential threats. They are particularly effective in detecting insider threats and zero-day vulnerabilities.
- Automated Response Agents: These agents can take immediate action in response to detected threats, such as isolating affected systems or blocking malicious IP addresses. This rapid response capability is crucial in minimizing damage during a security incident, especially in the context of managed cyber security services.
- Threat Intelligence Agents: These agents gather and analyze data from various sources, including dark web forums and threat databases, to provide insights into emerging threats and vulnerabilities. They help organizations stay informed about the latest cyber threats and trends, including cybersecurity for small businesses and top cyber security companies.
- Phishing Detection Agents: These specialized agents use natural language processing (NLP) to analyze emails and messages for signs of phishing attempts. By identifying suspicious content, they can help prevent users from falling victim to scams.
- Vulnerability Assessment Agents: These agents scan systems and applications for known vulnerabilities, providing organizations with a comprehensive view of their security posture. They help prioritize remediation efforts based on the severity of identified vulnerabilities, which is essential for cybersecurity for business.
The deployment of these AI agents enhances an organization's overall security strategy, enabling a more proactive and efficient approach to cybersecurity. As cyber threats continue to evolve, including recent cyber attacks and cyber security detection, the role of AI in security will become increasingly vital in safeguarding sensitive information and maintaining operational integrity. Rapid Innovation is committed to helping clients implement these advanced AI solutions, ensuring they are well-equipped to face the challenges of modern cybersecurity.
Refer to the image for a visual representation of the evolution of cybersecurity threats and the role of AI in enhancing security measures:

1.4. Current Landscape and Challenges
The current landscape of technology is characterized by rapid advancements and a growing reliance on digital solutions across various sectors. However, this evolution comes with its own set of challenges that organizations must navigate, including technology challenges, problems in technology today, and specific issues like iot privacy issues and security and privacy issues in iot.
- Data Privacy and Security: With the increase in data generation, concerns about privacy and security have escalated. Organizations face the challenge of protecting sensitive information while complying with regulations such as GDPR and CCPA. Breaches can lead to significant financial and reputational damage. Rapid Innovation offers robust AI-driven security solutions that help organizations safeguard their data and ensure compliance, ultimately reducing the risk of breaches and enhancing trust.
- Integration of Legacy Systems: Many organizations still rely on outdated systems that are not compatible with modern technologies. Integrating these legacy systems with new solutions can be complex and costly, hindering innovation. Rapid Innovation specializes in developing tailored integration strategies that bridge the gap between legacy systems and cutting-edge technologies, enabling seamless transitions and improved operational efficiency.
- Talent Shortage: There is a significant shortage of skilled professionals in fields like data science, cybersecurity, and artificial intelligence. This gap makes it difficult for organizations to implement advanced technologies effectively. Rapid Innovation addresses this challenge by providing consulting services and training programs that empower organizations to build in-house expertise and leverage AI and blockchain technologies effectively.
- Rapid Technological Change: The pace of technological change can be overwhelming. Organizations must continuously adapt to new tools and platforms, which can strain resources and lead to implementation challenges. Rapid Innovation helps clients navigate this landscape by offering strategic guidance and implementation support, ensuring that they remain competitive and agile in a fast-evolving market. This includes addressing challenges of implementing new technology in an organization and technology problems in society.
- Ethical Considerations: As technology evolves, ethical dilemmas arise, particularly in areas like AI and machine learning. Issues such as bias in algorithms and the potential for job displacement require careful consideration and proactive management. Rapid Innovation emphasizes ethical AI practices, helping organizations develop transparent and fair algorithms that align with their values and societal expectations.
- Cost of Implementation: The financial investment required for adopting new technologies can be a barrier for many organizations, especially small and medium-sized enterprises. Budget constraints can limit their ability to innovate and compete. Rapid Innovation offers cost-effective solutions and flexible pricing models that enable organizations to access advanced technologies without compromising their budgets. This is particularly relevant for technology challenges for small business and technology challenges for nonprofits.
- User Adoption: Even the best technologies can fail if users do not adopt them. Organizations must invest in training and change management to ensure that employees are comfortable with new tools and processes. Rapid Innovation provides comprehensive training and support services to facilitate user adoption, ensuring that organizations maximize the value of their technology investments. This is crucial in addressing challenges with technology in the classroom and educational technology problems.
2. Foundational Technologies
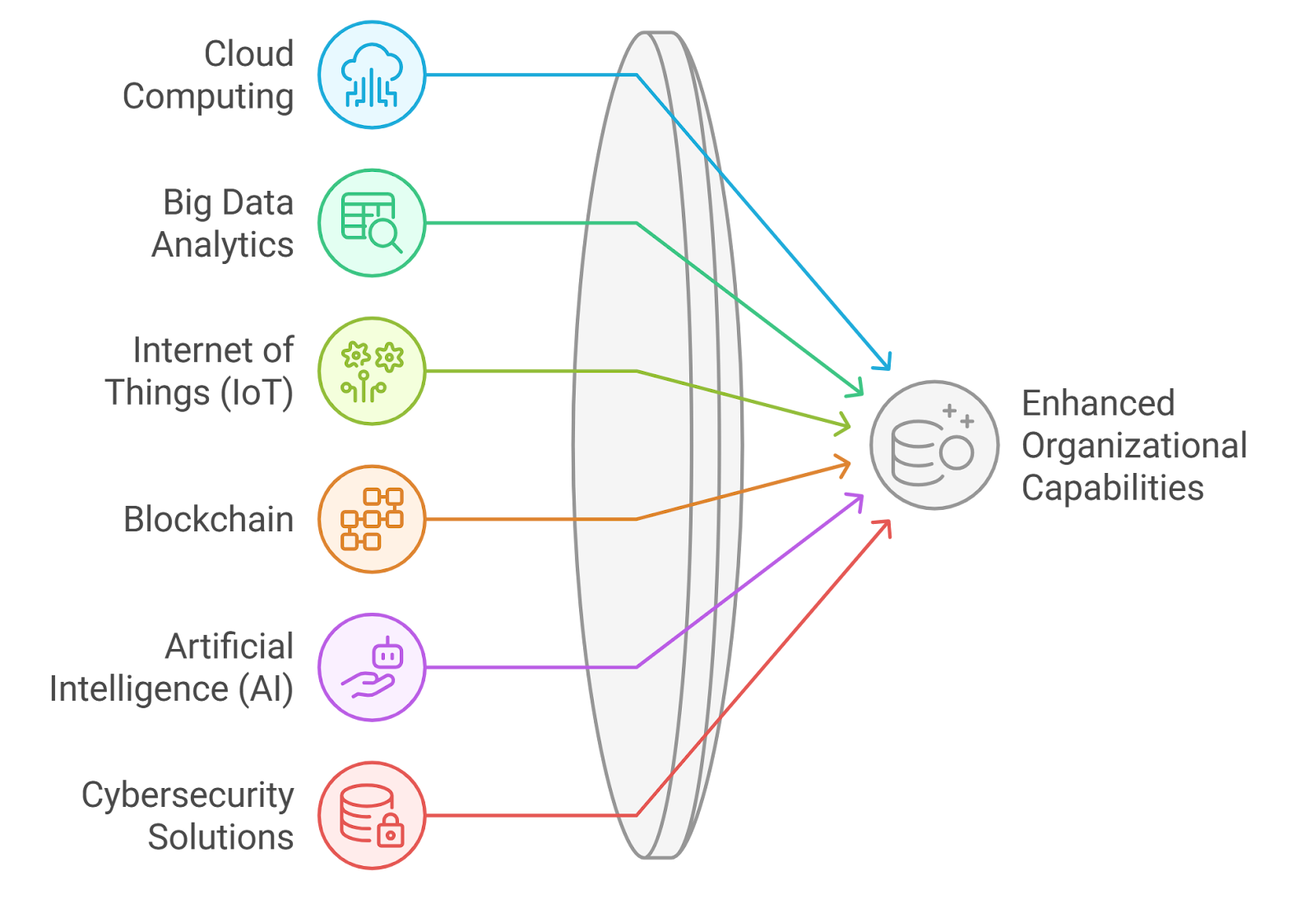
‍
Foundational technologies are the building blocks that enable the development and implementation of advanced solutions across various industries. These technologies provide the necessary infrastructure and capabilities for innovation.
- Cloud Computing: Cloud services offer scalable resources and flexibility, allowing organizations to store and process data without the need for extensive on-premises infrastructure. This technology supports remote work and collaboration.
- Big Data Analytics: The ability to analyze large volumes of data helps organizations gain insights into customer behavior, market trends, and operational efficiency. Big data analytics tools enable data-driven decision-making.
- Internet of Things (IoT): IoT connects devices and sensors to the internet, facilitating real-time data collection and monitoring. This technology is transforming industries such as manufacturing, healthcare, and agriculture, but also raises iot privacy issues and security and privacy issues in iot.
- Blockchain: Blockchain technology provides a secure and transparent way to record transactions. It is particularly useful in industries that require trust and traceability, such as finance and supply chain management. Rapid Innovation leverages blockchain to enhance transparency and security in transactions, driving greater efficiency and trust in business operations.
- Artificial Intelligence (AI): AI encompasses various technologies, including machine learning and natural language processing, that enable machines to perform tasks that typically require human intelligence. AI is revolutionizing customer service, healthcare, and many other sectors. Rapid Innovation harnesses AI to create intelligent solutions that optimize processes and enhance customer experiences.
- Cybersecurity Solutions: As digital threats increase, robust cybersecurity measures are essential. Foundational technologies in this area include firewalls, encryption, and intrusion detection systems to protect sensitive data, addressing 5g security threats and 5g security challenges.
2.1. Machine Learning Algorithms
Machine learning algorithms are a subset of artificial intelligence that enable systems to learn from data and improve their performance over time without being explicitly programmed. These algorithms are crucial for various applications, from predictive analytics to natural language processing.
- Supervised Learning: This approach involves training a model on labeled data, where the desired output is known. Common algorithms include linear regression, decision trees, and support vector machines. Supervised learning is widely used in applications like spam detection and image recognition.
- Unsupervised Learning: In unsupervised learning, models are trained on data without labeled outputs. The goal is to identify patterns or groupings within the data. Algorithms such as k-means clustering and hierarchical clustering are commonly used for market segmentation and anomaly detection.
- Reinforcement Learning: This type of learning involves training algorithms through trial and error, where an agent learns to make decisions by receiving rewards or penalties. Reinforcement learning is often applied in robotics, gaming, and autonomous systems, such as those seen in the darpa autonomous vehicle challenge and darpa driverless car challenge.
- Deep Learning: A subset of machine learning, deep learning uses neural networks with multiple layers to analyze complex data. It excels in tasks such as image and speech recognition. Deep learning has driven advancements in AI applications, including self-driving cars and virtual assistants.
- Natural Language Processing (NLP): NLP algorithms enable machines to understand and interpret human language. Applications include chatbots, sentiment analysis, and language translation. NLP is essential for enhancing user interactions with technology.
- Model Evaluation and Tuning: To ensure the effectiveness of machine learning algorithms, it is crucial to evaluate their performance using metrics such as accuracy, precision, and recall. Techniques like cross-validation and hyperparameter tuning help optimize models for better results.
- Ethical Considerations in Machine Learning: As machine learning becomes more prevalent, ethical concerns arise, particularly regarding bias in algorithms and data privacy. Organizations must implement practices to ensure fairness and transparency in their machine learning applications. Rapid Innovation is committed to promoting ethical AI practices, ensuring that our solutions are not only effective but also responsible and equitable. For more insights on AI subfields, you can explore key concepts and technologies in AI.
Refer to the image for a visual representation of the current landscape and challenges in technology.

2.1.1. Supervised Learning
Supervised learning is a type of machine learning where the model is trained on a labeled dataset. This means that each training example is paired with an output label, allowing the algorithm to learn the relationship between the input data and the corresponding output. The process involves feeding the model a set of input-output pairs, where the model makes predictions based on the input data and is then corrected by comparing its predictions to the actual output.
Common algorithms used in supervised learning include: - Linear regression - Decision trees - Support vector machines (SVM) - Neural networks
Applications of supervised learning are vast and include: - Image classification (e.g., identifying objects in photos) - Spam detection in emails - Predictive analytics in finance
At Rapid Innovation, we leverage supervised learning to help clients enhance their decision-making processes and operational efficiencies. For instance, in the finance sector, we can develop predictive models that analyze historical data to forecast market trends, enabling businesses to make informed investment decisions and achieve greater ROI.
Supervised learning is particularly effective when: - A large amount of labeled data is available. - The relationship between input and output is relatively straightforward.
In the context of supervised machine learning, techniques such as machine learning classification and methods of machine learning are often employed to improve model accuracy. Additionally, feature engineering for machine learning plays a crucial role in enhancing the performance of these models.
2.1.2. Unsupervised Learning
Unsupervised learning, in contrast to supervised learning, deals with unlabeled data. The model is tasked with identifying patterns and structures within the data without any explicit guidance on what to look for. The primary goal is to explore the underlying structure of the data.
Common techniques include: - Clustering (e.g., K-means, hierarchical clustering) - Dimensionality reduction (e.g., Principal Component Analysis, t-SNE) - Anomaly detection
Key applications of unsupervised learning include: - Customer segmentation in marketing - Market basket analysis in retail - Image compression
At Rapid Innovation, we utilize unsupervised learning to help clients uncover hidden insights from their data. For example, in marketing, we can implement customer segmentation techniques that allow businesses to tailor their strategies to specific customer groups, ultimately leading to improved customer engagement and increased sales.
Unsupervised learning is beneficial when: - The dataset lacks labels or annotations. - The goal is to discover hidden patterns or groupings in the data.
Methods in machine learning, such as clustering machine learning and example of unsupervised machine learning, are essential for extracting valuable insights from unstructured data. Additionally, non supervised machine learning approaches can be explored to further enhance data analysis.
2.1.3. Reinforcement Learning
Reinforcement learning (RL) is a type of machine learning where an agent learns to make decisions by taking actions in an environment to maximize cumulative rewards. Unlike supervised learning, RL does not rely on labeled input-output pairs but instead learns from the consequences of its actions. The agent interacts with the environment and receives feedback in the form of rewards or penalties.
The learning process involves: - Exploration: Trying new actions to discover their effects. - Exploitation: Using known actions that yield high rewards.
Key components of reinforcement learning include: - The agent: The learner or decision-maker. - The environment: The context in which the agent operates. - The reward signal: Feedback that guides the agent's learning.
Applications of reinforcement learning are diverse and include: - Game playing (e.g., AlphaGo, OpenAI's Dota 2 bot) - Robotics (e.g., teaching robots to navigate complex environments) - Autonomous vehicles (e.g., optimizing driving strategies)
At Rapid Innovation, we harness the power of reinforcement learning to develop intelligent systems that adapt and optimize their performance over time. For instance, in the realm of autonomous vehicles, we can create algorithms that enable vehicles to learn from their surroundings and improve their navigation strategies, leading to safer and more efficient transportation solutions.
Reinforcement learning is particularly effective when: - The problem involves sequential decision-making. - The environment is dynamic and requires adaptation over time.
Incorporating ensemble learning methods can also enhance the performance of reinforcement learning models, making them more robust in complex environments.
Refer to the image for a visual representation of supervised learning concepts:

2.2. Deep Learning Applications
Deep learning, a subset of machine learning, has revolutionized various industries by enabling systems to learn from vast amounts of data. Its applications are diverse and impactful, transforming how we interact with technology and driving significant ROI for businesses.
- Healthcare: Deep learning algorithms analyze medical images, such as X-rays and MRIs, to assist in diagnosing diseases. For instance, convolutional neural networks (CNNs) can detect tumors with high accuracy, leading to earlier interventions and improved patient outcomes. This is a key area in deep learning for computer vision.
- Finance: In the financial sector, deep learning models predict stock prices and assess credit risk. By analyzing historical data and market trends, these models enable financial institutions to make informed decisions, optimize investment strategies, and reduce potential losses.
- Autonomous Vehicles: Self-driving cars utilize deep learning for object detection, lane recognition, and decision-making. These systems process data from cameras and sensors in real-time, enhancing safety and efficiency in transportation, which can lead to reduced operational costs.
- Speech Recognition: Virtual assistants like Siri and Alexa rely on deep learning to understand and respond to voice commands. Recurrent neural networks (RNNs) are often used for this purpose, improving user experience and driving engagement with technology.
- Gaming: Deep learning enhances the gaming experience by creating intelligent non-player characters (NPCs) that adapt to player behavior, making games more engaging and increasing player retention.
The versatility of deep learning continues to expand, with ongoing research leading to new applications across various fields, including deep learning applications in image processing and machine learning for computer vision. At Rapid Innovation, we leverage these advancements to help our clients achieve their business goals efficiently and effectively, ensuring a greater return on investment. For more information on natural language processing and its usesk.
2.3. Natural Language Processing
Natural Language Processing (NLP) is a branch of artificial intelligence that focuses on the interaction between computers and human language. It enables machines to understand, interpret, and generate human language in a valuable way, providing businesses with tools to enhance customer engagement and operational efficiency.
- Sentiment Analysis: Businesses use NLP to analyze customer feedback and social media posts to gauge public sentiment about products or services. This helps in making data-driven marketing decisions that can lead to increased sales and customer satisfaction.
- Chatbots and Virtual Assistants: NLP powers chatbots that provide customer support and virtual assistants that help users with tasks. These systems can understand context and respond appropriately, reducing operational costs and improving customer service.
- Machine Translation: Tools like Google Translate utilize NLP to convert text from one language to another, making communication across language barriers easier and facilitating global business operations.
- Text Summarization: NLP algorithms can condense large volumes of text into concise summaries, saving time for users who need quick insights from lengthy documents, thereby enhancing productivity.
- Information Retrieval: Search engines leverage NLP to improve the accuracy of search results by understanding user queries and providing relevant information, which can drive traffic and engagement.
NLP continues to evolve, with advancements in deep learning techniques enhancing its capabilities and applications. Rapid Innovation harnesses these technologies to empower our clients, ensuring they stay ahead in a competitive landscape.
2.4. Computer Vision in Security
Computer vision, a field of artificial intelligence, enables machines to interpret and understand visual information from the world. In security, its applications are critical for enhancing safety and surveillance, providing businesses with robust solutions to protect their assets.
- Facial Recognition: This technology identifies individuals by analyzing facial features. It is widely used in security systems for access control and monitoring public spaces, improving security measures and reducing unauthorized access.
- Surveillance Systems: Computer vision enhances traditional surveillance by enabling real-time analysis of video feeds. It can detect unusual behavior or identify potential threats automatically, allowing for quicker response times and increased safety.
- Intrusion Detection: Systems equipped with computer vision can monitor restricted areas and alert security personnel in case of unauthorized access, minimizing risks and potential losses.
- License Plate Recognition: This application is used in traffic management and law enforcement to identify vehicles by reading their license plates, aiding in tracking stolen cars or monitoring traffic violations, thus enhancing public safety.
- Crowd Monitoring: Computer vision can analyze crowd behavior in public spaces, helping to identify potential security risks or emergencies, which is crucial for event management and public safety.
The integration of computer vision in security systems not only improves response times but also enhances overall safety measures in various environments. At Rapid Innovation, we specialize in developing tailored solutions that leverage these technologies, ensuring our clients achieve their security objectives efficiently and effectively.
3. AI Agent Architecture for Cybersecurity
The integration of AI in cybersecurity has revolutionized how organizations protect their digital assets. AI agents are designed to analyze vast amounts of data, identify threats, and respond to incidents in real-time. The architecture of these AI agents consists of several core components that work together to enhance security measures.
3.1 Core Components
The core components of an AI agent architecture for cybersecurity include:
- Data Collection Modules
- Data Processing Units
- Threat Intelligence Systems
- Response Mechanisms
- User Interface and Reporting Tools
Each of these components plays a crucial role in ensuring that the AI agent can effectively monitor, analyze, and respond to cybersecurity threats.
3.1.1 Data Collection Modules
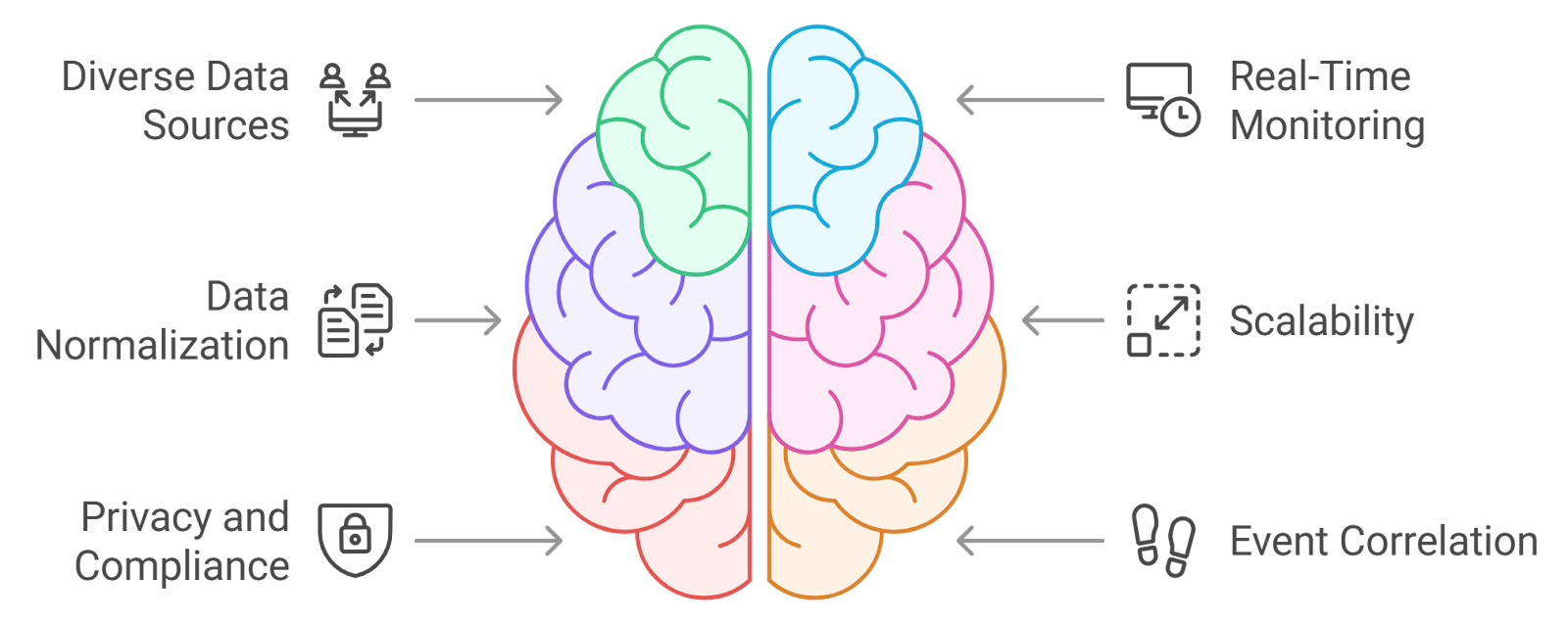
‍
Data collection modules are the foundational elements of AI agent architecture for cybersecurity. They are responsible for gathering data from various sources to provide a comprehensive view of the cybersecurity landscape. Key aspects of data collection modules include:
- Diverse Data Sources: Collect data from network traffic, system logs, user behavior, and external threat intelligence feeds. Integrate with existing security tools like firewalls, intrusion detection systems (IDS), and antivirus software.
- Real-Time Monitoring: Enable continuous data collection to ensure that the AI agent has the most up-to-date information. Use techniques like packet sniffing and log aggregation to capture relevant data in real-time.
- Data Normalization: Standardize data from different sources to ensure consistency and facilitate analysis. Employ data transformation techniques to convert raw data into a usable format.
- Scalability: Design modules to handle large volumes of data as organizations grow and their networks expand. Utilize cloud-based solutions to enhance storage and processing capabilities.
- Privacy and Compliance: Implement measures to ensure that data collection adheres to privacy regulations such as GDPR and HIPAA. Anonymize sensitive data to protect user privacy while still allowing for effective analysis.
- Event Correlation: Analyze collected data to identify patterns and correlations that may indicate potential threats. Use machine learning algorithms to enhance the accuracy of threat detection.
The effectiveness of data collection modules directly impacts the overall performance of the AI agent architecture for cybersecurity. By ensuring comprehensive and accurate data collection, organizations can significantly improve their ability to detect and respond to cybersecurity threats. At Rapid Innovation, we leverage our expertise in AI to develop tailored solutions that enhance your cybersecurity posture, ultimately leading to greater ROI and a more secure digital environment.
3.1.2. Analysis Engines
Analysis engines are critical components in data processing and analytics. They are designed to interpret, process, and derive insights from large volumes of data. These engines utilize various algorithms and methodologies to transform raw data into meaningful information, enabling organizations to make informed decisions and achieve their business goals efficiently.
- Types of Analysis Engines: Â
- Batch Processing Engines: Handle large datasets in bulk, processing data at scheduled intervals. Examples include Apache Hadoop and Apache Spark, which can be leveraged by Rapid Innovation to optimize data workflows and enhance reporting capabilities for clients. Additionally, data processing engines like the snowflake processing engine can be utilized for efficient batch processing.
- Stream Processing Engines: Process data in real-time, allowing for immediate insights. Examples include Apache Flink and Apache Kafka, which can be utilized to provide clients with real-time analytics, improving responsiveness to market changes. Event processing engines also play a significant role in this category.
- Machine Learning Engines: Focus on predictive analytics and pattern recognition. Tools like TensorFlow and Scikit-learn fall into this category, enabling Rapid Innovation to develop tailored machine learning models that drive better decision-making for clients. Data mining engines can also be integrated to enhance these capabilities.
- Key Features: Â
- Scalability: Ability to handle increasing amounts of data without performance degradation, ensuring that clients can grow their data operations without disruption. Big data processing engines are essential for this scalability.
- Flexibility: Support for various data formats and sources, including structured and unstructured data, allowing Rapid Innovation to cater to diverse client needs. Data transformation engines are crucial in this aspect.
- Speed: Efficient processing capabilities to deliver insights quickly, especially in time-sensitive applications, enhancing clients' operational efficiency. In-memory data engines can significantly improve processing speed.
- Use Cases: Â
- Business Intelligence: Organizations use analysis engines to generate reports and dashboards for decision-making, a service Rapid Innovation can provide to help clients visualize their data effectively. Tools like the salesforce data processing engine can enhance these capabilities.
- Fraud Detection: Financial institutions employ these engines to analyze transaction patterns and identify anomalies, a critical service that Rapid Innovation can implement to safeguard client operations. Data mining in search engine analytics can also be applied here.
- Customer Insights: Retailers leverage analysis engines to understand consumer behavior and preferences, enabling Rapid Innovation to assist clients in tailoring their marketing strategies for better ROI. The integration of data ingestion engines can further enhance customer insights.
3.1.3. Response Systems
Response systems are designed to react to the insights generated by analysis engines. They play a crucial role in automating actions based on data-driven decisions. These systems ensure that organizations can respond promptly to changing conditions or insights, ultimately enhancing operational efficiency.
- Types of Response Systems: Â
- Automated Response Systems: Trigger predefined actions based on specific data inputs. For example, an e-commerce platform may automatically adjust prices based on inventory levels, a solution Rapid Innovation can implement to optimize client pricing strategies. The use of automated systems can be enhanced by integrating with data processing engines.
- Interactive Response Systems: Allow users to engage with the system for real-time decision-making. Chatbots and virtual assistants are common examples, which Rapid Innovation can develop to enhance customer engagement for clients. These systems can utilize event processing engines for improved interaction.
- Feedback Loops: Systems that not only respond but also learn from the outcomes of their actions to improve future responses, enabling continuous improvement in client operations.
- Key Features: Â
- Real-Time Processing: Ability to act on data as it is generated, ensuring timely responses that Rapid Innovation can leverage to enhance client service delivery. The integration of stream processing engines is vital for real-time capabilities.
- Integration with Analysis Engines: Seamless communication with analysis engines to utilize insights effectively, ensuring that clients can act on data-driven insights promptly. This can be facilitated by using a combination of data processing engines and response systems.
- User-Friendly Interfaces: Simplified interfaces for users to interact with the system and customize responses, making it easier for clients to manage their operations.
- Use Cases: Â
- Customer Support: Automated chatbots provide immediate assistance based on customer inquiries, a service Rapid Innovation can implement to improve client customer service. The integration of salesforce data processing engine can enhance customer support capabilities.
- Marketing Automation: Systems that adjust marketing campaigns in real-time based on user engagement metrics, allowing Rapid Innovation to help clients maximize their marketing ROI. The use of data transformation engines can optimize marketing strategies.
- Supply Chain Management: Automated systems that reorder stock based on predictive analytics of demand, a solution Rapid Innovation can provide to enhance client inventory management. The application of data ingestion engines can streamline supply chain processes.
3.2. Integration Frameworks
Integration frameworks are essential for connecting various systems, applications, and data sources within an organization. They facilitate the seamless flow of information, ensuring that different components can work together effectively, which is crucial for achieving business objectives.
- Types of Integration Frameworks: Â
- Enterprise Service Bus (ESB): A middleware solution that enables communication between different applications. Examples include MuleSoft and Apache Camel, which Rapid Innovation can implement to streamline client operations. The integration of various data processing engines can enhance ESB functionalities.
- API Management Platforms: Tools that help create, manage, and secure APIs for data exchange. Examples include Apigee and AWS API Gateway, enabling Rapid Innovation to enhance client data accessibility and security.
- Data Integration Tools: Solutions that focus on consolidating data from multiple sources into a unified view. Examples include Talend and Informatica, which Rapid Innovation can utilize to provide clients with comprehensive data insights. The use of elastic search etl can also improve data integration processes.
- Key Features: Â
- Interoperability: Ability to connect disparate systems and applications, regardless of their underlying technologies, ensuring that Rapid Innovation can provide tailored solutions for diverse client environments.
- Data Transformation: Capabilities to convert data formats and structures to ensure compatibility, allowing Rapid Innovation to facilitate seamless data integration for clients. The use of data transformation engines is critical in this aspect.
- Monitoring and Management: Tools to oversee integration processes, ensuring data flows smoothly and efficiently, which is vital for maintaining operational integrity for clients.
- Use Cases: Â
- Cloud Integration: Organizations use integration frameworks to connect on-premises systems with cloud applications, a service Rapid Innovation can provide to enhance client flexibility and scalability. The integration of snowflake processing engine can facilitate cloud data operations.
- Data Warehousing: Integration frameworks help consolidate data from various sources into a central repository for analysis, enabling Rapid Innovation to assist clients in making data-driven decisions. The use of big data processing engines can enhance data warehousing capabilities.
- Microservices Architecture: Frameworks facilitate communication between microservices, enabling agile development and deployment, which Rapid Innovation can implement to enhance client software development processes. The integration of various data processing engines can support microservices architecture effectively.
3.3. Scalability Considerations
Scalability is a critical aspect of system design, especially for applications that anticipate growth in user base or data volume. It refers to the ability of a system to handle increased load without compromising performance. Here are key considerations for scalability:
- Horizontal vs. Vertical Scaling: Â
- Horizontal scaling involves adding more machines or nodes to distribute the load, which is particularly beneficial for cloud-based applications.
- Vertical scaling means upgrading existing hardware to improve performance, which can be effective for legacy systems.
- Load Balancing: Â
- Implementing load balancers can help distribute incoming traffic evenly across servers, ensuring no single server becomes a bottleneck. This is essential for maintaining high availability and performance.
- Microservices Architecture: Â
- Adopting a microservices architecture allows individual components of an application to scale independently, improving resource utilization and enabling faster deployment cycles.
- Database Scalability: Â
- Consider using database sharding or replication to manage large datasets effectively.
- NoSQL databases often provide better scalability for unstructured data, making them ideal for applications that require flexibility and speed.
- Caching Strategies: Â
- Implement caching mechanisms to reduce database load and improve response times.
- Use tools like Redis or Memcached for efficient data retrieval, which can significantly enhance user experience.
- Cloud Solutions: Â
- Leverage cloud services that offer auto-scaling features to automatically adjust resources based on demand, ensuring that your application can handle traffic spikes without manual intervention. Explore how AI agents for biometric authentication can enhance security and scalability.
3.4. Performance Optimization
Performance optimization is essential for ensuring that applications run efficiently and provide a seamless user experience. Here are several strategies to enhance performance:
- Code Optimization: Â
- Regularly review and refactor code to eliminate inefficiencies.
- Use profiling tools to identify bottlenecks in the code, which can lead to significant performance improvements.
- Database Optimization: Â
- Optimize database queries by using indexing and avoiding unnecessary joins.
- Regularly analyze and clean up the database to maintain performance, ensuring that data retrieval is swift and efficient.
- Content Delivery Networks (CDNs): Â
- Utilize CDNs to cache static content closer to users, reducing latency and improving load times, which is crucial for global applications.
- Asynchronous Processing: Â
- Implement asynchronous processing for tasks that do not require immediate user feedback, such as sending emails or processing data, allowing the application to remain responsive.
- Image and Asset Optimization: Â
- Compress images and minify CSS/JavaScript files to reduce load times.
- Use responsive images to serve appropriate sizes based on user devices, enhancing the mobile experience.
- Monitoring and Analytics: Â
- Continuously monitor application performance using tools like Google Analytics or New Relic.
- Analyze user behavior to identify areas for improvement, enabling data-driven decisions for optimization.
4. Threat Detection and Prevention
Threat detection and prevention are vital components of cybersecurity, aimed at identifying and mitigating potential security risks. Here are essential strategies for effective threat management:
- Intrusion Detection Systems (IDS): Â
- Deploy IDS to monitor network traffic for suspicious activities and potential threats.
- Use both network-based and host-based IDS for comprehensive coverage, ensuring that all entry points are monitored.
- Regular Security Audits: Â
- Conduct regular security audits to identify vulnerabilities in systems and applications.
- Use automated tools to scan for known vulnerabilities, allowing for proactive risk management.
- User Behavior Analytics (UBA): Â
- Implement UBA to detect anomalies in user behavior that may indicate a security breach.
- Analyze patterns to identify potential insider threats, enhancing overall security posture.
- Firewalls and Access Controls: Â
- Use firewalls to create a barrier between trusted and untrusted networks.
- Implement strict access controls to limit user permissions based on roles, reducing the risk of unauthorized access.
- Threat Intelligence: Â
- Leverage threat intelligence feeds to stay updated on emerging threats and vulnerabilities.
- Share information with industry peers to enhance collective security, fostering a collaborative approach to cybersecurity.
- Incident Response Plan: Â
- Develop and regularly update an incident response plan to ensure quick action in the event of a security breach.
- Conduct drills to prepare the team for real-world scenarios, ensuring readiness and resilience.
- Employee Training: Â
- Regularly train employees on security best practices and phishing awareness.
- Foster a culture of security within the organization to reduce human error, which is often the weakest link in security.
At Rapid Innovation, we leverage our expertise in AI and Blockchain to implement these strategies effectively, ensuring that our clients achieve their business goals efficiently and effectively while maximizing ROI.
4.1. Real-time Monitoring Systems
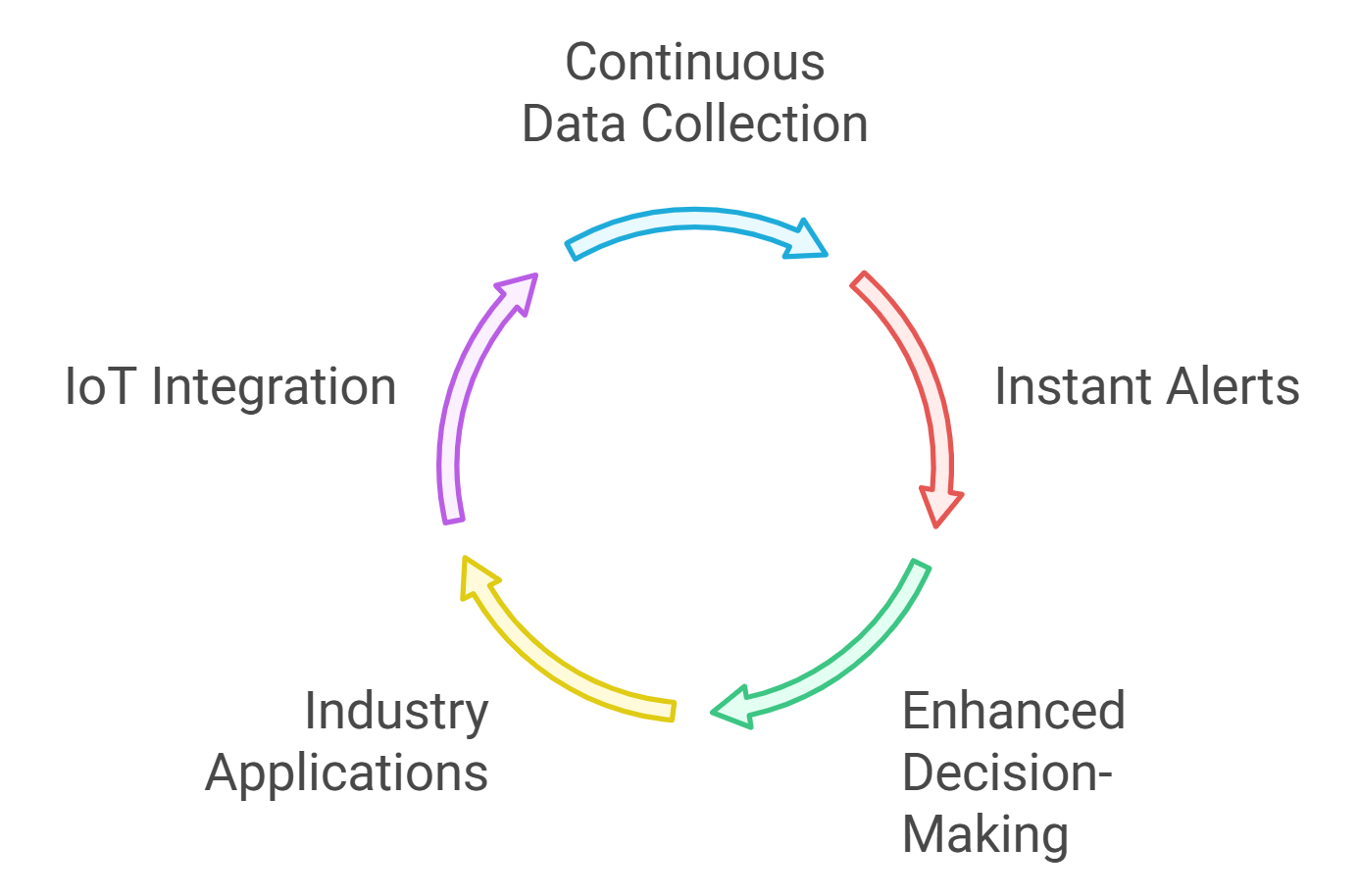
‍
Real-time monitoring systems are essential tools in various industries, providing immediate insights into operations, performance, and security. These systems collect and analyze data as it is generated, allowing organizations to respond swiftly to any changes or issues.
- Continuous data collection: Real-time monitoring systems gather data from multiple sources, including sensors, devices, and applications. Rapid Innovation leverages advanced AI algorithms to enhance data collection processes, ensuring comprehensive insights that drive operational efficiency. This includes solutions for real time network monitoring and real time monitoring systems that cater to specific industry needs.
- Instant alerts: These systems can send notifications to relevant personnel when predefined thresholds are breached, enabling quick action. By integrating blockchain technology, we ensure that alerts are secure and tamper-proof, enhancing trust in the data being acted upon. Real time security monitoring is a critical component of this process.
- Enhanced decision-making: By providing up-to-the-minute information, organizations can make informed decisions that improve efficiency and reduce risks. Our solutions empower clients to utilize predictive analytics, allowing them to anticipate issues before they arise. This is particularly important in real time network performance monitoring, where timely data can prevent outages.
- Applications across industries: Real-time monitoring is utilized in sectors such as healthcare, finance, manufacturing, and IT, ensuring optimal performance and security. Rapid Innovation has successfully implemented these systems for clients, resulting in significant ROI through improved operational performance. Our real time monitoring solutions have been tailored to meet the unique demands of various sectors.
- Integration with IoT: Many real-time monitoring systems leverage the Internet of Things (IoT) to connect devices and gather data seamlessly. Our expertise in IoT integration allows clients to create a cohesive ecosystem that maximizes data utility and operational insights. This includes linux real time network monitor solutions that enhance data collection from various devices. Additionally, our computer vision software development services can further enhance the capabilities of these systems. For more information on how AI can improve anomaly detection in real-time monitoring systems, check out this introduction to AI anomaly detection.
4.2. Behavioral Analysis
Behavioral analysis involves examining patterns of behavior to identify trends, anomalies, and potential risks. This technique is widely used in cybersecurity, marketing, and customer service to enhance understanding and improve outcomes.
- User behavior tracking: Organizations can monitor how users interact with their systems, identifying common patterns and deviations. Rapid Innovation employs machine learning models to analyze user behavior, enabling clients to optimize user experiences and increase engagement.
- Fraud detection: By analyzing behavioral patterns, companies can detect unusual activities that may indicate fraudulent behavior. Our AI-driven solutions provide real-time fraud detection capabilities, significantly reducing financial losses for our clients.
- Personalization: Behavioral analysis helps businesses tailor their offerings to individual preferences, enhancing customer satisfaction and loyalty. We assist clients in implementing personalized marketing strategies that drive customer retention and increase sales.
- Predictive analytics: By understanding past behaviors, organizations can predict future actions, allowing for proactive strategies. Our advanced analytics tools empower clients to make data-driven decisions that align with their business goals.
- Machine learning integration: Advanced algorithms can analyze vast amounts of data, improving the accuracy of behavioral insights over time. Rapid Innovation's expertise in machine learning ensures that our clients benefit from continuous improvement in their analytical capabilities.
4.3. Anomaly Detection
Anomaly detection is a critical process in identifying unusual patterns or outliers in data that may indicate problems or opportunities. This technique is vital for maintaining system integrity and security.
- Definition of anomalies: Anomalies are data points that deviate significantly from the expected norm, which can signal issues such as fraud, system failures, or security breaches. Our solutions are designed to detect these anomalies in real-time, allowing for immediate corrective actions.
- Techniques used: Various methods, including statistical analysis, machine learning, and artificial intelligence, are employed to detect anomalies effectively. Rapid Innovation utilizes cutting-edge AI techniques to enhance the accuracy and speed of anomaly detection processes.
- Real-world applications: Anomaly detection is used in finance for fraud detection, in IT for network security, and in manufacturing for quality control. Our clients have experienced substantial ROI through the implementation of our anomaly detection systems, which have minimized risks and improved operational efficiency.
- Importance of timely detection: Early identification of anomalies can prevent significant losses and enhance operational efficiency. Rapid Innovation's solutions are designed to provide timely insights, ensuring that our clients can act swiftly to mitigate risks.
- Continuous improvement: As systems learn from past anomalies, they become better at detecting future issues, leading to improved accuracy and reduced false positives. Our commitment to continuous improvement ensures that our clients remain at the forefront of their industries, leveraging the latest advancements in AI and blockchain technology.
4.4. Pattern Recognition
Pattern recognition is a crucial aspect of cybersecurity, enabling systems to identify and classify data based on established patterns. This technology is essential for detecting anomalies and potential threats in vast amounts of data. It utilizes algorithms to analyze data sets and identify trends or irregularities, and it helps in recognizing known attack patterns, such as malware signatures or phishing attempts. Additionally, machine learning models can improve over time, adapting to new threats and refining their detection capabilities. This approach is effective in real-time monitoring, allowing for immediate responses to suspicious activities, and it can be applied in various domains, including network security, fraud detection, and user behavior analytics.
At Rapid Innovation, we harness the power of cybersecurity pattern recognition to enhance our clients' security frameworks, enabling them to proactively identify and mitigate risks. By leveraging our expertise in MLOps consulting services, organizations can significantly enhance their security posture and reduce the risk of successful cyberattacks.
4.5. Predictive Threat Analysis
Predictive threat analysis involves using data analytics and machine learning to anticipate potential cyber threats before they occur. This proactive approach allows organizations to strengthen their defenses and mitigate risks effectively. It analyzes historical data to identify trends and predict future attack vectors, and it employs statistical models to assess the likelihood of various threats based on current data. This method enables organizations to allocate resources more efficiently by focusing on high-risk areas, integrates threat intelligence feeds to stay updated on emerging threats and vulnerabilities, and facilitates the development of incident response plans tailored to predicted threats.
By implementing predictive threat analysis, businesses can stay one step ahead of cybercriminals, significantly reducing the chances of a successful attack. Rapid Innovation's tailored solutions in predictive analytics empower our clients to allocate their resources effectively, ensuring a robust defense against evolving threats.
4.6. Zero-Day Attack Detection
Zero-day attacks exploit vulnerabilities in software that are unknown to the vendor and have no available patches. Detecting these attacks is particularly challenging due to their stealthy nature. It involves monitoring for unusual behavior that may indicate an exploit is in progress and utilizes advanced threat detection techniques, such as behavioral analysis and anomaly detection. This process requires continuous updates to threat databases to recognize new vulnerabilities as they emerge, employs sandboxing techniques to analyze suspicious files in a controlled environment, and collaboration with security researchers and vendors is essential for timely identification and mitigation.
Effective zero-day attack detection is vital for organizations to protect sensitive data and maintain operational integrity in an increasingly complex threat landscape. At Rapid Innovation, we provide cutting-edge solutions that enhance our clients' capabilities to detect and respond to zero-day threats, ensuring their data remains secure and their operations uninterrupted.
5. Incident Response and Management
Incident response and management is a critical aspect of cybersecurity, ensuring that organizations can effectively handle security breaches and minimize damage. A well-structured incident response plan can significantly reduce recovery time and costs associated with cyber incidents, including crisis incident management and security incident management.
5.1. Automated Response Systems
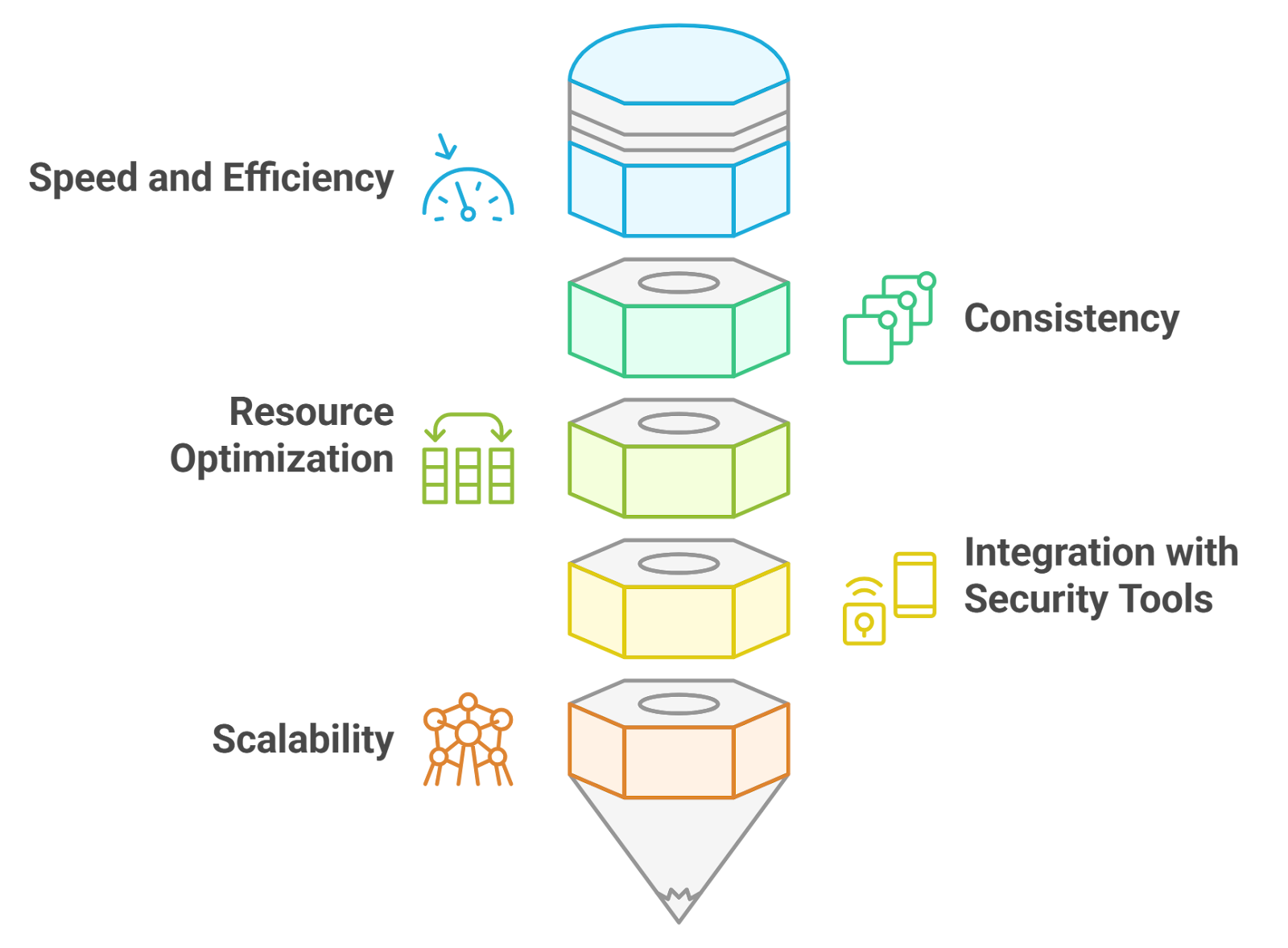
‍
Automated response systems are essential tools in modern incident response strategies. They help organizations react swiftly to security incidents, reducing the time between detection and response, which is crucial for effective incident response management.
- Speed and Efficiency: Automated systems can respond to threats in real-time, allowing for immediate action without human intervention. This is crucial in minimizing the impact of an incident, especially during a crisis incident.
- Consistency: Automation ensures that responses are consistent and follow predefined protocols, reducing the risk of human error during high-pressure situations, such as security incident response.
- Resource Optimization: By automating routine tasks, security teams can focus on more complex issues that require human expertise. This leads to better resource allocation and improved overall security posture, enhancing automated incident management.
- Integration with Security Tools: Automated response systems can integrate with various security tools, such as intrusion detection systems (IDS) and security information and event management (SIEM) solutions, to provide a comprehensive response strategy for incident handling in cyber security.
- Scalability: As organizations grow, automated systems can scale to handle increased volumes of data and incidents without a proportional increase in resources, making them ideal for security incident management.
5.2. Threat Prioritization
Threat prioritization is a vital component of incident response, allowing organizations to focus their efforts on the most significant risks. By assessing and categorizing threats, organizations can allocate resources more effectively and respond to incidents that pose the greatest danger, including those identified in their incident management plan.
- Risk Assessment: Organizations should conduct regular risk assessments to identify potential threats and vulnerabilities. This helps in understanding which assets are most at risk and require immediate attention, forming the basis of best practice incident management.
- Impact Analysis: Evaluating the potential impact of different threats helps prioritize responses. High-impact threats that could lead to significant data loss or operational disruption should be addressed first, particularly in the context of security incident response management.
- Threat Intelligence: Utilizing threat intelligence feeds can provide insights into emerging threats and vulnerabilities. This information can help organizations prioritize their response efforts based on current trends in cyber threats, enhancing their overall incident response management.
- Business Context: Understanding the business context is crucial for effective threat prioritization. Organizations should consider factors such as the criticality of assets, regulatory requirements, and potential reputational damage when assessing threats, especially in crisis incident management.
- Continuous Monitoring: Ongoing monitoring of the threat landscape allows organizations to adjust their prioritization strategies in real-time. This ensures that they remain agile and responsive to new threats as they emerge, which is essential for effective incident management policies.
At Rapid Innovation, we leverage our expertise in AI and Blockchain to enhance incident response and management strategies for our clients. By implementing automated response systems powered by AI, we enable organizations to achieve faster response times and reduce the impact of security incidents. Our approach to threat prioritization, informed by advanced analytics and threat intelligence, ensures that our clients can focus on the most critical risks, ultimately leading to greater ROI and a more resilient security posture in the realm of cyber security incident management.
5.3. Incident Classification
Incident classification is a critical step in the incident response process. It involves categorizing incidents based on their nature, severity, and potential impact on the organization. Proper classification helps in prioritizing response efforts and allocating resources effectively. This includes frameworks such as security incident classification NIST, which provides guidelines for categorizing incidents.
- Types of incidents may include: Â
- Malware infections
- Data breaches
- Denial of Service (DoS) attacks
- Insider threats
- Classification criteria often include: Â
- Severity level (low, medium, high)
- Affected systems or data
- Potential business impact
- Benefits of effective incident classification: Â
- Streamlined response efforts
- Improved communication among teams
- Enhanced reporting and analysis capabilities
Organizations can utilize frameworks such as the Common Vulnerability Scoring System (CVSS) to aid in classification. By assigning scores to vulnerabilities, teams can better understand the urgency of incidents and respond accordingly. At Rapid Innovation, we leverage AI-driven analytics to enhance incident classification, enabling organizations to achieve greater ROI by reducing response times and improving resource allocation. This is particularly important in the context of information security incident classification, which helps organizations understand the specific nature of incidents they face. For more information on how AI can assist in incident resolution workflows.
5.4. Response Orchestration
Response orchestration refers to the coordinated approach to managing and automating incident response activities. It involves integrating various tools, processes, and teams to ensure a swift and effective response to security incidents.
- Key components of response orchestration include: Â
- Automation of repetitive tasks
- Integration of security tools (SIEM, SOAR, etc.)
- Real-time communication among stakeholders
- Benefits of response orchestration: Â
- Reduced response times
- Minimized human error
- Enhanced collaboration across teams
- Best practices for effective orchestration: Â
- Develop clear incident response playbooks
- Regularly test and update response plans
- Utilize threat intelligence to inform decisions
By implementing response orchestration, organizations can create a more agile and efficient incident response framework, ultimately reducing the impact of security incidents. Rapid Innovation's expertise in AI and Blockchain allows us to provide tailored solutions that enhance orchestration capabilities, ensuring that our clients can respond to incidents with speed and precision.
5.5. Recovery Procedures
Recovery procedures are essential for restoring normal operations after a security incident. These procedures outline the steps necessary to recover affected systems, data, and services while minimizing downtime and data loss.
- Key elements of recovery procedures include: Â
- Data backup and restoration processes
- System reconfiguration and hardening
- Validation of system integrity before going live
- Steps in the recovery process: Â
- Assess the extent of the damage
- Implement data recovery strategies
- Communicate with stakeholders about recovery status
- Importance of recovery procedures: Â
- Ensures business continuity
- Reduces the risk of future incidents
- Helps maintain customer trust and confidence
Organizations should regularly review and test their recovery procedures to ensure they are effective and up-to-date. This proactive approach can significantly enhance resilience against future incidents. At Rapid Innovation, we assist organizations in developing robust recovery strategies that not only restore operations but also fortify their defenses against future threats, ultimately driving greater ROI. This is especially relevant in the context of incident.
6. Specialized AI Security Agents
In the realm of cybersecurity, specialized AI security agents are becoming increasingly vital. These agents leverage artificial intelligence to enhance security measures, detect threats, and respond to incidents more effectively than traditional methods. Two primary categories of these agents are network security agents and endpoint protection agents.
6.1 Network Security Agents

‍
Network security agents focus on protecting the integrity, confidentiality, and availability of data as it travels across networks. They utilize AI algorithms to monitor network traffic, identify anomalies, and respond to potential threats in real-time.
- Traffic Analysis: AI-driven network security agents analyze vast amounts of network traffic data to identify patterns and detect unusual behavior. They can differentiate between normal and malicious traffic, allowing for quicker responses to potential threats.
- Intrusion Detection and Prevention: These agents employ machine learning models to recognize known attack signatures and detect new, unknown threats. They can automatically block suspicious activities, reducing the risk of data breaches.
- Automated Response: In the event of a detected threat, network security agents can initiate automated responses, such as isolating affected systems or alerting security personnel. This rapid response capability minimizes damage and helps maintain network integrity.
- Scalability: AI network security agents can scale to accommodate growing network demands, making them suitable for organizations of all sizes. They can adapt to new threats as they emerge, ensuring ongoing protection.
- Cost Efficiency: By automating many security processes, these agents can reduce the need for extensive human intervention, leading to cost savings. Organizations can allocate resources more effectively, focusing on strategic initiatives rather than routine monitoring.
6.2 Endpoint Protection Agents
Endpoint protection agents are designed to secure individual devices connected to a network, such as computers, smartphones, and tablets. These agents utilize AI to provide comprehensive security solutions that protect against a wide range of threats.
- Threat Detection: AI algorithms analyze endpoint behavior to identify potential threats, including malware, ransomware, and phishing attacks. They can detect anomalies in user behavior, flagging suspicious activities for further investigation.
- Real-Time Monitoring: Endpoint protection agents continuously monitor devices for signs of compromise, ensuring immediate detection and response to threats. This proactive approach helps prevent data loss and system damage.
- Automated Remediation: Upon detecting a threat, these agents can automatically quarantine infected files or roll back changes made by malicious software. This minimizes downtime and helps maintain business continuity.
- User Education: Many endpoint protection solutions include features that educate users about security best practices, helping to reduce the risk of human error. By fostering a culture of security awareness, organizations can enhance their overall security posture.
- Integration with Other Security Solutions: Endpoint protection agents can integrate with other security tools, such as firewalls and SIEM (Security Information and Event Management) systems, to provide a comprehensive security framework. This integration allows for better visibility and coordination in threat detection and response.
In conclusion, specialized AI security agents, including network security agents and endpoint protection agents, play a crucial role in modern cybersecurity strategies. By leveraging advanced AI technologies, these agents enhance threat detection, automate responses, and ultimately protect organizations from an ever-evolving landscape of cyber threats. At Rapid Innovation, we harness these cutting-edge AI security agents to help our clients achieve greater ROI by ensuring robust security measures that safeguard their digital assets while optimizing operational efficiency. For more information on AI agents for network monitoring.
6.3. Cloud Security Agents
Cloud security agents are essential tools designed to protect data and applications hosted in cloud environments. As businesses increasingly migrate to the cloud, the need for robust security measures becomes paramount. Cloud security agents help mitigate risks associated with data breaches, unauthorized access, and compliance violations.
- Data Encryption: Cloud security agents often provide encryption services to protect sensitive data both at rest and in transit. This ensures that even if data is intercepted, it remains unreadable without the proper decryption keys.
- Access Control: These agents implement strict access control measures, allowing organizations to define who can access specific data and applications. Role-based access control (RBAC) is commonly used to enforce these policies.
- Threat Detection: Cloud security agents utilize advanced threat detection techniques, including machine learning and behavioral analysis, to identify and respond to potential security threats in real-time. Rapid Innovation leverages AI algorithms to enhance threat detection capabilities, ensuring that clients can proactively address vulnerabilities before they are exploited. Additionally, AI agents for proactive system monitoring can further enhance the security landscape.
- Compliance Management: Many cloud security agents assist organizations in maintaining compliance with industry regulations such as GDPR, HIPAA, and PCI-DSS. They provide audit trails and reporting features to demonstrate compliance efforts, which is crucial for businesses aiming to avoid penalties and maintain customer trust.
- Integration with Existing Security Tools: Cloud security agents can often integrate with existing security solutions, providing a unified approach to security management across on-premises and cloud environments. Rapid Innovation ensures seamless integration, allowing clients to enhance their security posture without disrupting existing workflows.
6.4. Email Security Agents
Email security agents are critical for protecting organizations from email-based threats, which are among the most common vectors for cyberattacks. These agents help safeguard sensitive information and maintain the integrity of communication channels.
- Spam Filtering: Email security agents employ sophisticated algorithms to filter out spam and phishing emails, reducing the risk of users falling victim to malicious attacks.
- Malware Protection: These agents scan incoming and outgoing emails for malware, ensuring that harmful attachments or links do not compromise the organization's network.
- Data Loss Prevention (DLP): Email security agents often include DLP features that monitor outgoing emails for sensitive information, preventing unauthorized sharing of confidential data.
- Encryption: Many email security solutions offer encryption options for emails, ensuring that sensitive communications remain secure during transmission.
- User Training and Awareness: Some email security agents provide training resources to educate employees about recognizing phishing attempts and other email threats, fostering a culture of security awareness.
6.5. Web Application Security Agents
Web application security agents are specialized tools designed to protect web applications from various threats, including SQL injection, cross-site scripting (XSS), and other vulnerabilities. As web applications become increasingly integral to business operations, securing them is crucial.
- Vulnerability Scanning: Web application security agents regularly scan applications for known vulnerabilities, helping organizations identify and remediate security weaknesses before they can be exploited.
- Web Application Firewalls (WAF): Many web application security agents include WAF capabilities, which filter and monitor HTTP traffic to and from web applications. This helps block malicious requests and protect against common web attacks.
- Real-time Monitoring: These agents provide real-time monitoring of web applications, allowing organizations to detect and respond to security incidents as they occur.
- Compliance Support: Web application security agents often assist with compliance requirements by providing features that help organizations adhere to standards such as OWASP Top Ten and PCI-DSS.
- Integration with DevOps: Many modern web application security agents integrate seamlessly with DevOps processes, enabling security to be embedded into the software development lifecycle (SDLC) and ensuring that security is a priority from the outset. Rapid Innovation's expertise in both AI and blockchain technologies allows us to enhance web application security, ensuring that our clients can innovate while maintaining robust security measures.
6.6. IoT Security Agents
IoT security agents are specialized software or hardware solutions designed to protect Internet of Things (IoT) devices and networks from various cyber threats. As the number of connected devices continues to grow, so does the need for robust security measures, including iot security solutions and secure iot solution. IoT security agents play a crucial role in ensuring the integrity, confidentiality, and availability of data transmitted across these devices.
- Continuous monitoring: IoT security agents provide real-time monitoring of device activity, identifying unusual patterns that may indicate a security breach.
- Threat detection: They utilize advanced algorithms and machine learning to detect potential threats, such as malware or unauthorized access attempts. Rapid Innovation leverages AI-driven analytics to enhance threat detection capabilities, ensuring timely identification of vulnerabilities. This includes solutions from internet of things security companies and iot cybersecurity solutions.
- Automated responses: Upon detecting a threat, IoT security agents can automatically initiate predefined responses, such as isolating affected devices or alerting administrators. This automation minimizes response time, allowing organizations to mitigate risks effectively.
- Device authentication: These agents ensure that only authorized devices can connect to the network, reducing the risk of unauthorized access. Rapid Innovation implements robust authentication protocols to fortify device security, including secure remote access iot and secure remote management iot device.
- Data encryption: IoT security agents often implement encryption protocols to protect data in transit and at rest, safeguarding sensitive information from interception. Our blockchain solutions can further enhance data integrity and security through decentralized verification, particularly in the context of cloud and iot security.
- Compliance management: They help organizations adhere to industry regulations and standards by providing necessary security controls and reporting capabilities. Rapid Innovation assists clients in navigating compliance requirements, ensuring their IoT deployments meet regulatory standards, including pki iot.
The implementation of IoT security agents is essential for organizations looking to secure their IoT ecosystems effectively. As cyber threats evolve, these agents must also adapt to provide comprehensive protection, including industrial internet of things security and iot cloud security.
7. Advanced Defense Mechanisms
Advanced defense mechanisms are sophisticated strategies and technologies designed to enhance cybersecurity posture against increasingly complex threats. These mechanisms go beyond traditional security measures, incorporating innovative approaches to detect, prevent, and respond to cyber incidents.
- Layered security: Employing multiple security layers ensures that if one layer is breached, others remain intact to protect critical assets.
- Behavioral analysis: Advanced defense mechanisms utilize behavioral analytics to identify anomalies in user and device behavior, allowing for early detection of potential threats.
- Threat intelligence: Integrating threat intelligence feeds helps organizations stay informed about emerging threats and vulnerabilities, enabling proactive defense strategies.
- Incident response automation: Automating incident response processes reduces the time it takes to react to security incidents, minimizing potential damage.
- Cloud security: As organizations increasingly adopt cloud services, advanced defense mechanisms must include robust cloud security measures to protect data and applications hosted in the cloud, including iot and cloud security.
By implementing advanced defense mechanisms, organizations can significantly enhance their cybersecurity resilience, ensuring they are better prepared to face evolving threats.
7.1. Deception Technology

‍
Deception technology is an innovative cybersecurity strategy that involves creating decoys and traps to mislead attackers and detect intrusions. This proactive approach aims to confuse and delay cybercriminals, allowing organizations to gather intelligence on their tactics and techniques.
- Honeypots: These are decoy systems designed to attract attackers, providing a controlled environment to study their behavior without risking actual assets.
- Deceptive endpoints: Organizations can deploy fake endpoints that appear legitimate, luring attackers into engaging with them instead of real systems.
- Threat intelligence gathering: By monitoring interactions with deception technologies, organizations can gain valuable insights into attacker methodologies and tools.
- Early detection: Deception technology can alert security teams to unauthorized access attempts, enabling quicker responses to potential breaches.
- Reduced attack surface: By diverting attackers to decoys, organizations can protect their critical assets and reduce the likelihood of successful attacks.
Deception technology is becoming an essential component of modern cybersecurity strategies, providing organizations with a unique way to enhance their defenses and stay one step ahead of cyber threats. Rapid Innovation is committed to integrating these advanced technologies into our clients' security frameworks, ensuring they remain resilient against evolving cyber threats, including iot cyber security companies and splunk iot.
7.2. Adaptive Security Architecture
Adaptive Security Architecture (ASA) is a proactive approach to cybersecurity that emphasizes the need for systems to evolve in response to changing threats. This architecture is designed to be flexible and responsive, allowing organizations to adjust their security measures based on real-time data and threat intelligence. Key components of ASA include:
- Continuous monitoring: ASA relies on constant surveillance of network traffic and user behavior to identify anomalies and potential threats.
- Risk assessment: Regular evaluations of vulnerabilities and risks help organizations prioritize their security efforts and allocate resources effectively.
- Dynamic policy enforcement: Security policies can be adjusted on-the-fly based on the current threat landscape, ensuring that defenses are always aligned with the latest risks.
- Integration with existing systems: ASA can work alongside traditional security measures, enhancing their effectiveness without requiring a complete overhaul of existing infrastructure.
- User-centric approach: By focusing on user behavior and access patterns, ASA can better protect sensitive data and resources from insider threats and external attacks.
At Rapid Innovation, we leverage ASA to help our clients create a resilient cybersecurity framework that adapts to their unique operational environments. By implementing continuous monitoring and dynamic policy enforcement, we enable organizations to respond to threats in real-time, ultimately leading to a greater return on investment (ROI) through reduced risk exposure and enhanced operational efficiency. Our approach aligns with principles of information security architecture and cybersecurity architecture, ensuring a comprehensive defense strategy. Additionally, we offer services such as smart contract development to further enhance security measures in blockchain applications.
7.3. Autonomous Defense Systems
Autonomous Defense Systems (ADS) represent a significant advancement in cybersecurity technology. These systems utilize artificial intelligence (AI) and machine learning (ML) to automatically detect, respond to, and mitigate threats without human intervention. The main features of ADS include:
- Real-time threat detection: ADS can analyze vast amounts of data to identify potential threats in real-time, significantly reducing response times.
- Automated response: Once a threat is detected, ADS can initiate predefined responses, such as isolating affected systems or blocking malicious traffic, without waiting for human approval.
- Learning capabilities: These systems continuously learn from new threats and adapt their defenses accordingly, improving their effectiveness over time.
- Reduced human error: By automating many security processes, ADS minimizes the risk of human error, which is a common vulnerability in traditional security practices.
- Scalability: ADS can easily scale to accommodate growing networks and increasing data volumes, making them suitable for organizations of all sizes.
At Rapid Innovation, we integrate ADS into our clients' cybersecurity strategies, enabling them to harness the power of AI and ML for enhanced threat detection and response. This not only streamlines security operations but also significantly improves ROI by minimizing downtime and potential losses from security breaches. Our solutions are informed by the latest trends in cybersecurity architecture and the principles of cybersecurity mesh architecture.
7.4. Security Automation and Orchestration
Security Automation and Orchestration (SAO) refers to the use of technology to automate security tasks and streamline security operations. This approach enhances efficiency and effectiveness in managing security incidents and vulnerabilities. Key aspects of SAO include:
- Incident response automation: SAO can automate repetitive tasks involved in incident response, such as data collection and analysis, allowing security teams to focus on more complex issues.
- Integration of tools: By orchestrating various security tools and technologies, SAO creates a cohesive security environment that improves visibility and control over security operations.
- Improved collaboration: SAO facilitates better communication and collaboration among security teams, enabling them to respond more effectively to incidents.
- Enhanced threat intelligence: Automated systems can quickly gather and analyze threat intelligence from multiple sources, providing security teams with actionable insights.
- Cost efficiency: By reducing the time and resources required for manual security tasks, SAO can lead to significant cost savings for organizations.
Rapid Innovation employs SAO to help clients optimize their security operations, ensuring that they can respond to incidents swiftly and effectively. By automating routine tasks and integrating various security tools, we enable organizations to achieve greater efficiency and cost savings, ultimately enhancing their overall security posture and ROI. Our methodologies are aligned with the principles of cybersecurity architecture framework and the concepts of trust architectures.
Incorporating Adaptive Security Architecture, Autonomous Defense Systems, and Security Automation and Orchestration into an organization's cybersecurity strategy can significantly enhance its ability to defend against evolving threats. These approaches not only improve the effectiveness of security measures but also enable organizations to respond more swiftly and efficiently to incidents, ultimately leading to a more robust security posture. At Rapid Innovation, we are committed to helping our clients navigate the complexities of cybersecurity, ensuring they achieve their business goals efficiently and effectively through a comprehensive cybersecurity mesh architecture.
7.5. Threat Hunting
Threat hunting is a proactive cybersecurity practice that involves actively searching for potential threats within an organization's network before they can cause harm. Unlike traditional security measures that rely on automated alerts, threat hunting requires skilled analysts to investigate and identify anomalies that may indicate a breach or malicious activity.
- Focus on Indicators of Compromise (IoCs): Threat hunters look for specific signs that suggest a security incident, such as unusual login attempts, unexpected file changes, or abnormal network traffic patterns.
- Utilize Threat Intelligence: Incorporating threat intelligence feeds can enhance the hunting process by providing context about known threats and vulnerabilities, allowing organizations to stay ahead of potential attacks.
- Employ Advanced Tools: Tools like SIEM (Security Information and Event Management) systems, EDR (Endpoint Detection and Response), and machine learning algorithms can help in identifying patterns and anomalies, thereby improving the efficiency of threat detection. Cybersecurity threat hunting techniques are essential in this context.
- Continuous Improvement: Threat hunting is an iterative process. Findings from hunts should feed back into the security posture, improving detection capabilities and response strategies, ultimately leading to a more resilient security framework. The CISA threat hunting framework can be a valuable resource for organizations looking to enhance their practices.
- Collaboration: Effective threat hunting often involves collaboration between different teams, including incident response, IT, and threat intelligence, to share insights and improve overall security. This collaborative approach enhances the organization's ability to respond to threats swiftly and effectively. Certified cyber threat hunting professionals can play a crucial role in this process.
8. Data Management and Analysis
Data management and analysis are critical components of any organization’s strategy, especially in the context of cybersecurity. Proper data management ensures that data is collected, stored, and processed efficiently, while analysis helps in deriving actionable insights from that data.
- Importance of Data Governance: Establishing clear policies and procedures for data management helps ensure compliance with regulations and protects sensitive information, which is essential for maintaining trust and integrity.
- Data Quality: High-quality data is essential for accurate analysis. Organizations should implement processes to clean and validate data regularly, ensuring that decisions are based on reliable information.
- Scalability: As organizations grow, their data needs will evolve. Scalable data management solutions can accommodate increasing volumes of data without compromising performance, allowing businesses to adapt to changing demands.
- Integration: Seamless integration of data from various sources allows for a more comprehensive analysis, enabling organizations to make informed decisions based on a holistic view of their data landscape.
8.1. Data Collection Strategies
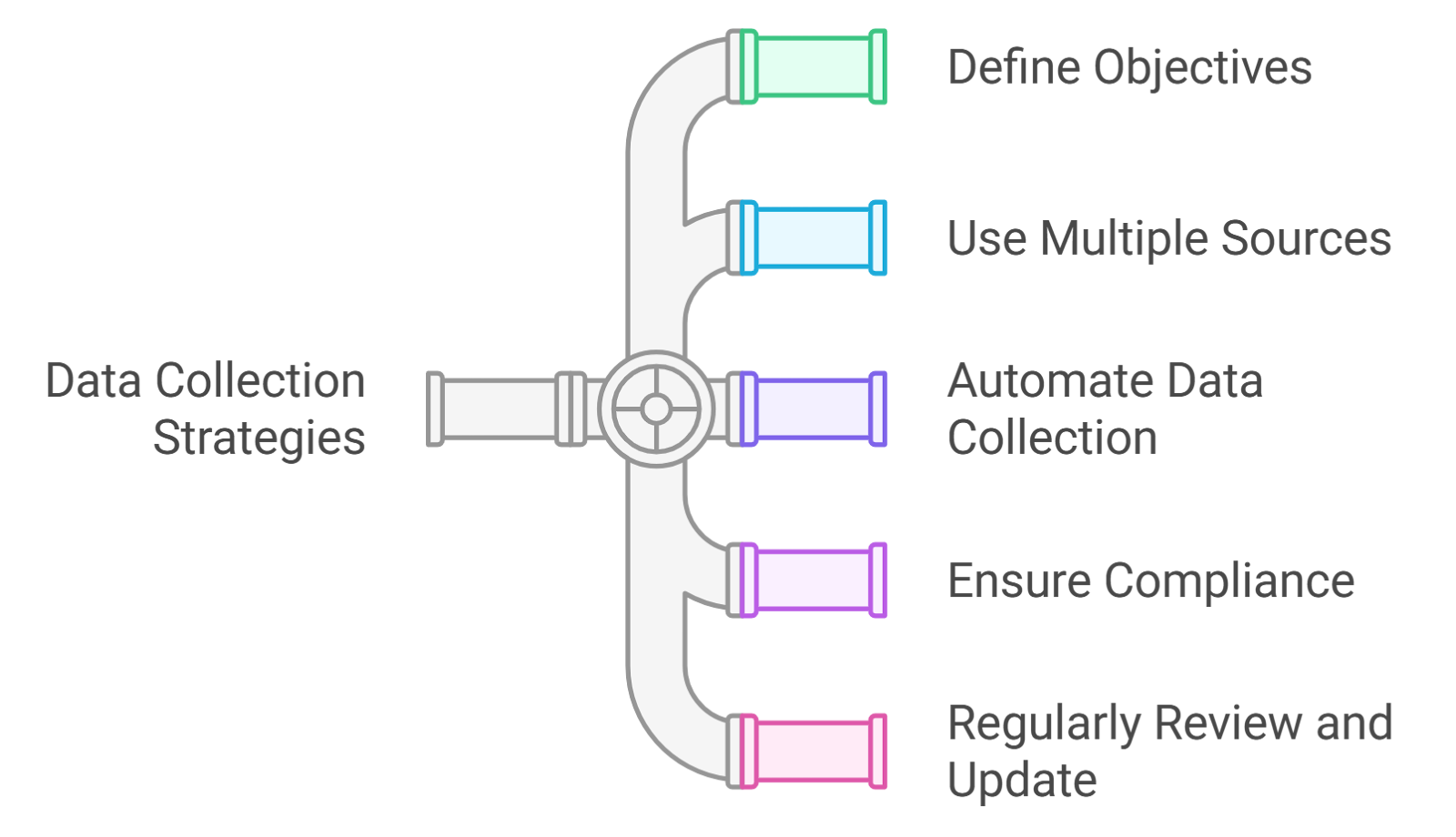
‍
Effective data collection strategies are vital for ensuring that organizations gather relevant and useful data for analysis. The right approach can significantly enhance the quality of insights derived from the data.
- Define Objectives: Clearly outline what you want to achieve with data collection. This could range from improving customer experience to enhancing security measures.
- Use Multiple Sources: Collect data from various sources, including internal systems, external APIs, and user interactions, to create a well-rounded dataset that supports diverse analytical needs.
- Automate Data Collection: Implementing automated tools can streamline the data collection process, reducing the risk of human error and ensuring timely updates, which is crucial for maintaining data relevance.
- Ensure Compliance: Adhere to data protection regulations such as GDPR or CCPA when collecting personal data to avoid legal repercussions and safeguard customer trust.
- Regularly Review and Update: Data collection strategies should be dynamic. Regularly assess and update your methods to adapt to changing business needs and technological advancements, ensuring that your organization remains competitive and compliant.
At Rapid Innovation, we leverage our expertise in AI and Blockchain to enhance these processes, providing tailored solutions that drive efficiency and maximize ROI for our clients. By integrating advanced technologies into threat hunting, including threat hunting in cyber security and practical cyber threat hunting approaches, we empower organizations to not only protect their assets but also to harness their data for strategic advantage.
8.2. Data Processing Pipeline
A data processing pipeline is a series of data processing steps that transform raw data into a format suitable for analysis and modeling. This pipeline is crucial in machine learning and data science as it ensures that data is clean, consistent, and ready for use.
- Data Collection: The first step involves gathering data from various sources, which can include databases, APIs, or web scraping. The quality and relevance of the data collected are vital for the success of the project. At Rapid Innovation, we leverage advanced data collection techniques to ensure that our clients have access to high-quality datasets that align with their business objectives. This can include implementing an etl pipeline or an aws etl pipeline to streamline the data collection process.
- Data Cleaning: This step addresses issues such as missing values, duplicates, and outliers. Techniques like imputation, normalization, and standardization are often employed to ensure data integrity. Our expertise in data cleaning helps clients maintain the accuracy of their datasets, leading to more reliable insights and decision-making. We often utilize data processing pipelines to automate this cleaning process.
- Data Transformation: Data may need to be transformed into a suitable format. This can include converting categorical variables into numerical ones, scaling features, or aggregating data. Rapid Innovation utilizes state-of-the-art transformation techniques to prepare data for effective analysis, enhancing the overall performance of machine learning models. This is a critical part of the etl data pipeline.
- Data Integration: If data comes from multiple sources, it needs to be integrated into a single dataset. This may involve merging datasets and ensuring that they are compatible. Our team specializes in seamless data integration, allowing clients to consolidate their data for comprehensive analysis and reporting. We often design data ingestion pipelines to facilitate this integration.
- Data Storage: Once processed, data should be stored in a way that allows for easy access and retrieval. This can involve using databases, data lakes, or cloud storage solutions. Rapid Innovation provides tailored storage solutions that ensure data is both secure and readily accessible, facilitating efficient data management. We also consider the design of the data pipeline for machine learning to ensure optimal storage solutions.
- Data Pipeline Automation: Automating the data processing pipeline can save time and reduce errors. Tools like Apache Airflow or Luigi can help manage and schedule data workflows. We implement automation strategies that streamline data processing, enabling clients to focus on deriving insights rather than managing data workflows. Our automated etl pipeline solutions are designed to enhance efficiency.
8.3. Feature Engineering
Feature engineering is the process of selecting, modifying, or creating new features from raw data to improve the performance of machine learning models. It plays a critical role in determining the success of predictive models.
- Feature Selection: This involves identifying the most relevant features that contribute to the model's predictive power. Techniques like Recursive Feature Elimination (RFE) or using algorithms like Random Forest can help in this process. At Rapid Innovation, we apply rigorous feature selection methods to enhance model accuracy and efficiency.
- Feature Creation: New features can be created from existing data. For example, combining date and time into a single timestamp or creating interaction terms between features can provide additional insights. Our team excels in innovative feature creation, ensuring that models are equipped with the most informative variables.
- Encoding Categorical Variables: Categorical data needs to be converted into numerical format. Techniques such as one-hot encoding or label encoding are commonly used to achieve this. We ensure that categorical variables are effectively encoded, maximizing the model's predictive capabilities.
- Scaling Features: Normalizing or standardizing features ensures that they are on a similar scale, which can improve model performance. Common methods include Min-Max scaling and Z-score normalization. Rapid Innovation employs best practices in feature scaling to optimize model training and performance.
- Dimensionality Reduction: Techniques like Principal Component Analysis (PCA) can reduce the number of features while retaining essential information, making models more efficient and easier to interpret. Our expertise in dimensionality reduction helps clients simplify their models without sacrificing accuracy.
- Feature Importance Analysis: After model training, analyzing feature importance can provide insights into which features are most influential, guiding future feature engineering efforts. We leverage feature importance analysis to inform our clients' strategic decisions and enhance their models continuously.
8.4. Model Training and Validation
Model training and validation are critical steps in the machine learning workflow, ensuring that models are both accurate and generalizable to new data.
- Model Selection: Choosing the right algorithm is essential. Options include linear regression, decision trees, support vector machines, and neural networks, among others. The choice depends on the problem type and data characteristics. Rapid Innovation assists clients in selecting the most suitable algorithms based on their specific needs and data profiles.
- Training the Model: The selected model is trained using a training dataset. This involves feeding the model input features and corresponding target values, allowing it to learn patterns in the data. Our rigorous training processes ensure that models are well-prepared to make accurate predictions.
- Hyperparameter Tuning: Models often have hyperparameters that need to be optimized for better performance. Techniques like Grid Search or Random Search can be used to find the best combination of hyperparameters. We implement advanced tuning strategies to enhance model performance, ensuring clients achieve optimal results.
- Validation Techniques: To assess model performance, various validation techniques can be employed: Â
- Cross-Validation: This method involves splitting the dataset into multiple subsets to ensure that the model is tested on different data portions.
- Train-Test Split: The dataset is divided into a training set and a testing set, allowing for an evaluation of the model's performance on unseen data. Our validation techniques provide clients with confidence in their models' reliability and effectiveness.
- Performance Metrics: Evaluating model performance is crucial. Common metrics include accuracy, precision, recall, F1 score, and area under the ROC curve (AUC-ROC). The choice of metric depends on the specific problem and business objectives. Rapid Innovation helps clients select the most relevant performance metrics to align with their business goals.
- Model Refinement: Based on validation results, models may need to be refined. This can involve revisiting feature engineering, adjusting hyperparameters, or even selecting a different model altogether. Our iterative refinement process ensures that models continuously improve and adapt to changing data landscapes.
- Deployment: Once validated, the model can be deployed into a production environment, where it can make predictions on new data. Continuous monitoring is essential to ensure that the model remains effective over time. Rapid Innovation provides comprehensive deployment solutions, ensuring that clients can leverage their models for real-time decision-making and sustained business growth. We also consider the use of gcp etl pipeline and azure etl pipeline for deployment strategies.
8.5. Performance Metrics
Performance metrics are essential for evaluating the effectiveness of any strategy or initiative. They provide quantifiable measures that help organizations assess their progress toward goals. In the context of business, performance metrics can be categorized into several key areas:
- Financial Metrics: These include revenue growth, profit margins, and return on investment (ROI). Financial metrics help organizations understand their economic health and make informed decisions. For instance, Rapid Innovation leverages AI-driven analytics to optimize financial performance, enabling clients to identify cost-saving opportunities and enhance profitability. Key performance indicators (KPIs) in this area might include kpi meaning in business and kpi business meaning.
- Operational Metrics: These metrics focus on the efficiency of processes, with examples including cycle time, throughput, and defect rates. Monitoring operational metrics can lead to improved productivity and reduced costs. By implementing blockchain solutions, Rapid Innovation helps clients streamline operations, ensuring transparency and traceability that reduce inefficiencies. Specific operational KPIs could include kpi of business and business management kpi.
- Customer Metrics: This category includes customer satisfaction scores, Net Promoter Score (NPS), and customer retention rates. These metrics are crucial for understanding customer loyalty and the overall customer experience. Rapid Innovation utilizes AI to analyze customer feedback and behavior, allowing clients to tailor their offerings and improve customer satisfaction. Relevant KPIs might include kpis for business development and company kpi examples.
- Employee Metrics: Employee engagement scores, turnover rates, and productivity levels are vital for assessing workforce effectiveness. High employee engagement often correlates with better performance and lower turnover. Rapid Innovation supports organizations in implementing AI-based tools that enhance employee engagement and productivity through personalized development programs. Employee-related KPIs could include kpi for construction company and kpis for accounts receivable.
- Market Metrics: Market share, brand awareness, and competitive positioning are important for understanding an organization’s standing in the industry. These metrics can guide marketing strategies and product development. Rapid Innovation employs data analytics to help clients gain insights into market trends, enabling them to make informed decisions that enhance their competitive edge. Market KPIs may include retail store kpis and retail industry kpis.
Establishing clear performance metrics allows organizations to track progress, identify areas for improvement, and make data-driven decisions. Regularly reviewing these metrics ensures that strategies remain aligned with organizational goals. Examples of business kpi examples and kpi of company can further illustrate the importance of these metrics. For more insights on the benefits and developments of multi-agent AI.
9. Implementation Strategies
Implementation strategies are critical for translating plans into actionable steps. A well-defined strategy ensures that resources are allocated effectively and that all stakeholders are aligned. Key components of successful implementation strategies include:
- Clear Objectives: Establishing specific, measurable, achievable, relevant, and time-bound (SMART) objectives is essential. Clear objectives guide the implementation process and provide a benchmark for success.
- Resource Allocation: Identifying and allocating the necessary resources—such as budget, personnel, and technology—is crucial. Proper resource allocation ensures that the implementation process runs smoothly.
- Stakeholder Engagement: Engaging stakeholders throughout the implementation process fosters collaboration and buy-in. Regular communication and feedback loops can help address concerns and adapt strategies as needed.
- Training and Development: Providing training for employees ensures they have the skills and knowledge required to execute the strategy effectively. Continuous development opportunities can enhance employee performance and satisfaction.
- Monitoring and Evaluation: Establishing a framework for monitoring progress and evaluating outcomes is vital. Regular assessments allow organizations to make adjustments and improve the implementation process.
By focusing on these components, organizations can enhance their chances of successful implementation and achieve their strategic goals.
9.1. Assessment and Planning
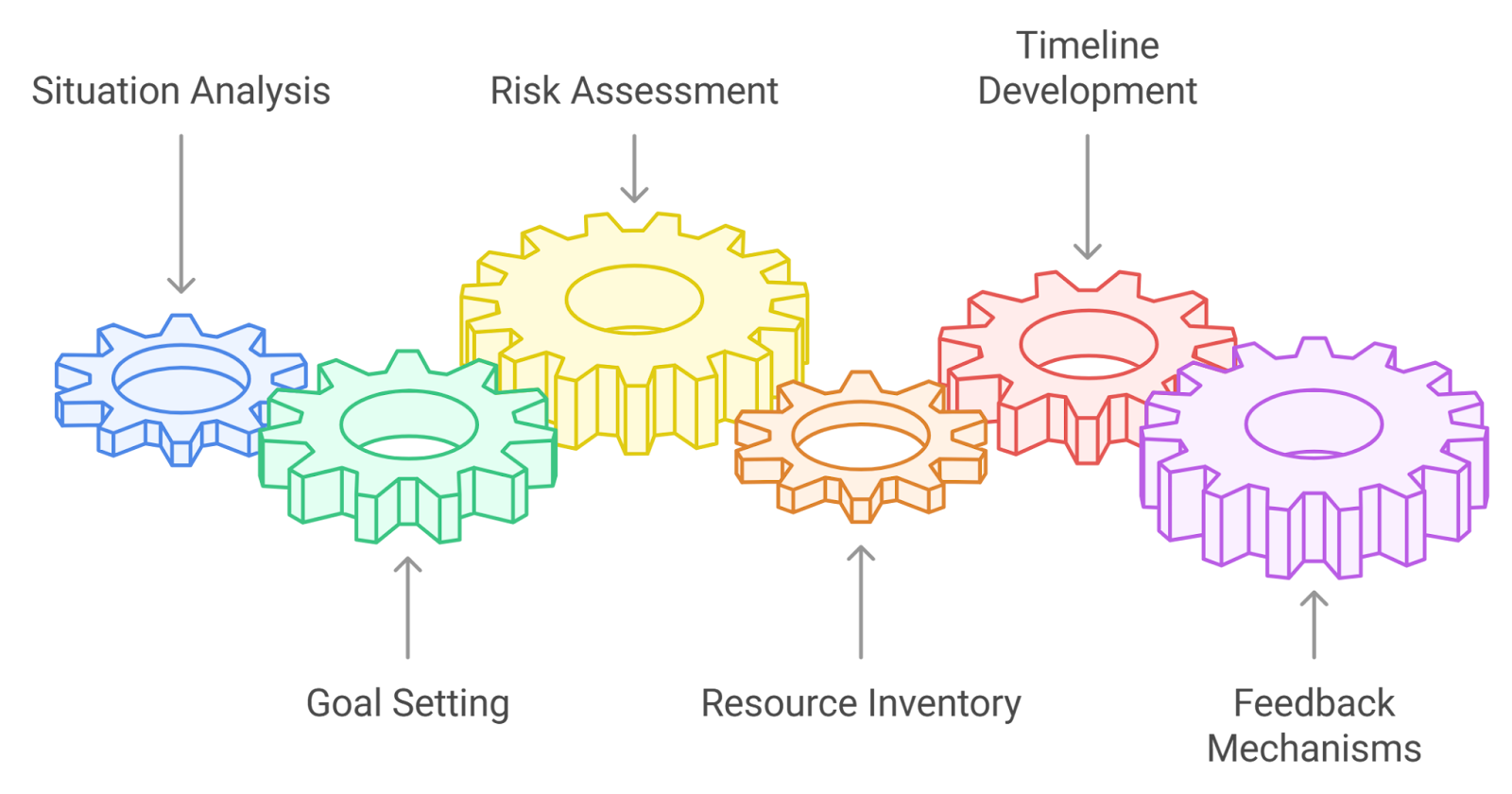
‍
Assessment and planning are foundational steps in the implementation process. They involve evaluating current conditions and developing a roadmap for future actions. Key aspects of assessment and planning include:
- Situation Analysis: Conducting a thorough analysis of the current environment is essential. This includes assessing internal strengths and weaknesses, as well as external opportunities and threats (SWOT analysis). Understanding the landscape helps in making informed decisions.
- Goal Setting: Based on the situation analysis, organizations should set clear and achievable goals that align with the overall mission and vision of the organization, providing direction for the implementation process.
- Risk Assessment: Identifying potential risks and challenges is crucial for effective planning. Organizations should develop strategies to mitigate these risks, ensuring that they are prepared for unforeseen circumstances.
- Resource Inventory: Assessing available resources, including human, financial, and technological assets, helps organizations understand what is at their disposal. This inventory informs resource allocation during implementation.
- Timeline Development: Creating a realistic timeline for implementation is essential. This timeline should include key milestones and deadlines, allowing organizations to track progress and make necessary adjustments.
- Feedback Mechanisms: Establishing feedback mechanisms ensures that stakeholders can provide input throughout the assessment and planning process. This collaborative approach can lead to more effective strategies and greater buy-in.
By focusing on assessment and planning, organizations can lay a solid foundation for successful implementation, ensuring that their strategies are well-informed and aligned with their goals.
9.2. Deployment Models
Deployment models refer to the various ways in which cloud services can be deployed and utilized. Understanding these models is crucial for organizations looking to leverage cloud technology effectively. The primary deployment models include:
- Public Cloud: Services are offered over the public internet and shared across multiple organizations. This model is cost-effective and scalable, making it ideal for small to medium-sized businesses. Providers like Amazon Web Services (AWS) and Microsoft Azure exemplify this model.
- Private Cloud: This model is dedicated to a single organization, providing enhanced security and control. It can be hosted on-premises or by a third-party provider. Private clouds are suitable for businesses with strict regulatory requirements or sensitive data.
- Hybrid Cloud: A combination of public and private clouds, hybrid models allow data and applications to be shared between them. This flexibility enables organizations to scale resources as needed while maintaining control over sensitive information.
- Multi-Cloud: Involves using services from multiple cloud providers. This approach can enhance redundancy and avoid vendor lock-in, allowing organizations to choose the best services for their needs.
Choosing the right deployment model depends on factors such as budget, security requirements, and scalability needs. Organizations must evaluate their specific requirements to determine the most suitable model for their cloud strategy. At Rapid Innovation, we assist clients in selecting the optimal deployment model that aligns with their business objectives, ensuring a greater return on investment (ROI) through tailored solutions. This includes understanding cloud deployment models, cloud computing deployment models, and deployment models for cloud computing. Additionally, our expertise in generative AI consulting can further enhance your cloud strategy.
9.3. Integration with Existing Infrastructure
Integrating cloud services with existing infrastructure is a critical step for organizations transitioning to the cloud. Successful integration ensures that businesses can leverage their current systems while taking advantage of cloud capabilities. Key considerations include:
- Assessment of Current Infrastructure: Evaluate existing hardware, software, and network capabilities to identify compatibility with cloud services. This assessment helps in understanding what can be migrated and what needs to be upgraded.
- APIs and Middleware: Utilize application programming interfaces (APIs) and middleware to facilitate communication between cloud services and on-premises systems. This integration layer is essential for seamless data flow and operational efficiency.
- Data Migration Strategies: Develop a clear plan for migrating data to the cloud. This may involve choosing between a lift-and-shift approach or re-architecting applications for cloud-native environments. Data integrity and security during migration are paramount.
- Security and Compliance: Ensure that the integration adheres to security protocols and compliance regulations. This includes implementing encryption, access controls, and regular audits to protect sensitive information.
- Training and Change Management: Provide training for staff to adapt to new cloud technologies and processes. Change management strategies can help ease the transition and ensure that employees are comfortable with the new systems.
Effective integration can lead to improved operational efficiency, reduced costs, and enhanced agility in responding to market changes. Rapid Innovation specializes in creating seamless integration strategies that maximize the value of cloud investments, including deployment in cloud computing and cloud computing deployment methods.
9.4. Testing and Validation
Testing and validation are essential components of any cloud deployment strategy. They ensure that cloud services function as intended and meet organizational requirements. Key aspects of testing and validation include:
- Functional Testing: Verify that all features and functionalities of the cloud service work as expected. This includes testing user interfaces, APIs, and integrations with existing systems.
- Performance Testing: Assess the performance of cloud applications under various load conditions. This testing helps identify potential bottlenecks and ensures that the system can handle expected traffic.
- Security Testing: Conduct thorough security assessments to identify vulnerabilities in the cloud environment. This includes penetration testing, vulnerability scanning, and compliance checks to ensure adherence to security standards.
- User Acceptance Testing (UAT): Involve end-users in the testing process to validate that the cloud solution meets their needs. UAT helps gather feedback and make necessary adjustments before full deployment.
- Continuous Monitoring: Implement monitoring tools to track the performance and security of cloud services post-deployment. Continuous monitoring allows for quick identification and resolution of issues.
- Documentation and Reporting: Maintain detailed documentation of testing processes, results, and any issues encountered. This documentation is vital for compliance and future reference.
Thorough testing and validation not only enhance the reliability of cloud services but also build confidence among stakeholders in the organization's cloud strategy. At Rapid Innovation, we prioritize rigorous testing protocols to ensure that our clients' cloud solutions deliver optimal performance and security, ultimately driving greater ROI. This includes understanding the nuances of SaaS deployment, community cloud example, and hybrid cloud deployment.
9.5. Maintenance and Updates
Regular maintenance and updates, including system maintenance before updates, are crucial for the longevity and performance of any system, software, or application. This process ensures that the technology remains functional, secure, and relevant in a rapidly changing digital landscape.
- Importance of Maintenance  Â
- Prevents system failures and downtime. Â
- Enhances performance and efficiency. Â
- Addresses bugs and vulnerabilities promptly. Â
- Types of Maintenance  Â
- Corrective Maintenance: Fixes issues that arise after deployment. Â
- Adaptive Maintenance: Modifies the system to accommodate changes in the environment or technology. Â
- Perfective Maintenance: Improves system performance or adds new features based on user feedback. Â
- Update Strategies  Â
- Scheduled Updates: Regularly planned updates to ensure consistency. Â
- Emergency Updates: Immediate fixes for critical vulnerabilities or issues. Â
- User-Initiated Updates: Allowing users to manually check for and install updates. Â
- System Maintenance and Updates: Integrating maintenance tasks with update processes to streamline operations. Â
- Best Practices for Maintenance  Â
- Regularly back up data to prevent loss. Â
- Monitor system performance and user feedback. Â
- Document all changes and updates for future reference. Â
- Tools for Maintenance  Â
- Use automated tools for monitoring and updating systems. Â
- Implement version control systems to manage changes effectively. Â
- User Education  Â
- Inform users about the importance of updates. Â
- Provide clear instructions on how to perform updates. Â
At Rapid Innovation, we understand that effective maintenance and updates are essential for maximizing the return on investment (ROI) for our clients. By leveraging our expertise in AI and Blockchain, we can implement automated maintenance solutions that not only reduce operational costs but also enhance system reliability and performance. For instance, our AI-driven monitoring tools can predict potential system failures before they occur, allowing for proactive maintenance that minimizes downtime and maximizes productivity. If you're looking to enhance your development team, consider our Action Transformer Developers for specialized support. Additionally, you can explore our insights on AI agents for maintenance tracking to further enhance your maintenance strategies.
10. Ethical and Legal Considerations
In the digital age, ethical and legal considerations are paramount for businesses and organizations. These factors influence how data is collected, stored, and used, ensuring that practices align with societal norms and legal requirements.
- Understanding Ethical Standards  Â
- Ethical considerations involve the moral implications of technology use. Â
- Organizations must balance profit motives with social responsibility. Â
- Legal Frameworks  Â
- Compliance with laws such as GDPR, HIPAA, and CCPA is essential. Â
- Organizations must stay updated on changing regulations to avoid legal repercussions. Â
- Impact on Business Practices  Â
- Ethical and legal considerations can affect brand reputation. Â
- Non-compliance can lead to fines, lawsuits, and loss of customer trust. Â
10.1. Privacy Concerns

‍
Privacy concerns are a significant aspect of ethical and legal considerations in technology. As data collection becomes more pervasive, individuals are increasingly aware of how their information is used.
- Data Collection Practices  Â
- Organizations often collect personal data for various purposes, including marketing and service improvement. Â
- Transparency in data collection is crucial to build trust with users. Â
- User Consent  Â
- Obtaining informed consent from users is a legal requirement in many jurisdictions. Â
- Users should have the option to opt-in or opt-out of data collection practices. Â
- Data Security Measures  Â
- Implement robust security protocols to protect user data from breaches. Â
- Regularly audit data storage and access practices to ensure compliance. Â
- User Rights  Â
- Users have the right to access, correct, and delete their personal information. Â
- Organizations must provide clear channels for users to exercise these rights. Â
- Impact of Data Breaches  Â
- Data breaches can lead to significant financial and reputational damage. Â
- Organizations must have a response plan in place to address breaches promptly. Â
- Emerging Technologies and Privacy  Â
- Technologies like AI and IoT raise new privacy concerns. Â
- Organizations must consider the implications of these technologies on user privacy. Â
By addressing maintenance and updates, ethical and legal considerations, and privacy concerns, organizations can create a more secure and trustworthy environment for users. At Rapid Innovation, we are committed to helping our clients navigate these complexities, ensuring that their technology solutions are not only effective but also ethically sound and compliant with legal standards.
10.2. Regulatory Compliance
Regulatory compliance refers to the adherence to laws, regulations, guidelines, and specifications relevant to an organization’s business processes. In the context of artificial intelligence (AI), regulatory compliance is crucial for ensuring that AI systems operate within legal frameworks and ethical boundaries.
- Organizations must stay updated on local, national, and international regulations that govern AI usage, including ai regulatory compliance. Rapid Innovation assists clients in navigating these complex regulatory landscapes, ensuring that their AI solutions are compliant and up-to-date.
- Compliance with regulations such as the General Data Protection Regulation (GDPR) in Europe is essential for companies operating in or with clients in the EU. Our team provides consulting services to help organizations implement necessary changes to meet these compliance requirements effectively, including artificial intelligence regulatory compliance.
- Regulatory bodies are increasingly focusing on AI, leading to the development of specific guidelines and frameworks to ensure responsible AI deployment. Rapid Innovation helps clients understand and integrate these guidelines into their AI strategies, including ai for regulatory compliance.
- Non-compliance can result in significant penalties, including fines and reputational damage. We work with organizations to develop robust compliance programs that mitigate these risks, emphasizing the importance of regulatory compliance ai.
- Companies should implement compliance programs that include regular audits, training, and updates to policies as regulations evolve. Rapid Innovation offers tailored compliance solutions that include training sessions and audit services to ensure ongoing adherence, particularly in the context of ai consulting services and regulatory compliance.
10.3. Ethical AI Usage
Ethical AI usage involves the responsible development and deployment of AI technologies, ensuring that they align with moral principles and societal values. This is increasingly important as AI systems become more integrated into daily life.
- Organizations should establish ethical guidelines that govern AI development and usage. Rapid Innovation collaborates with clients to create comprehensive ethical frameworks tailored to their specific needs.
- Transparency in AI algorithms is vital; stakeholders should understand how decisions are made. We emphasize the importance of transparency in our AI solutions, helping clients communicate their processes clearly to stakeholders.
- Bias in AI systems can lead to unfair treatment of individuals or groups, making it essential to implement measures to identify and mitigate bias. Our team employs advanced techniques to detect and reduce bias in AI models, ensuring fair outcomes.
- Stakeholder engagement is crucial; involving diverse groups in the development process can help ensure that AI systems are fair and inclusive. Rapid Innovation facilitates stakeholder workshops to gather diverse perspectives during the development phase.
- Continuous monitoring and evaluation of AI systems can help identify ethical concerns and allow for timely adjustments. We provide ongoing support and monitoring services to help clients maintain ethical standards in their AI applications.
10.4. Data Protection Standards
Data protection standards are frameworks and regulations designed to safeguard personal and sensitive information from unauthorized access, breaches, and misuse. In the realm of AI, data protection is particularly important due to the vast amounts of data processed by AI systems.
- Organizations must comply with data protection laws such as GDPR, which sets strict guidelines for data collection, storage, and processing. Rapid Innovation offers expertise in implementing these regulations effectively within AI systems.
- Implementing robust data encryption and anonymization techniques can help protect sensitive information. Our development team integrates state-of-the-art security measures into AI solutions to ensure data protection.
- Regular data audits and assessments are necessary to ensure compliance with data protection standards. We provide comprehensive audit services to help organizations assess their data protection practices.
- Employee training on data protection best practices is essential to minimize the risk of human error leading to data breaches. Rapid Innovation offers training programs designed to educate employees on data protection protocols.
- Organizations should have clear data retention policies to ensure that data is not kept longer than necessary, reducing the risk of exposure. We assist clients in developing and implementing effective data retention strategies that align with regulatory requirements.
10.5. Accountability Framework
An accountability framework is essential for organizations to ensure that all members are responsible for their actions and decisions. This framework outlines the roles, responsibilities, and expectations for individuals and teams, promoting transparency and trust within the organization. The SASB sustainability framework can serve as a guide for integrating sustainability into accountability practices.
- Establish clear roles and responsibilities: Define who is accountable for specific tasks and outcomes. This clarity helps prevent confusion and overlaps in duties, particularly in the context of SASB industry standards.
- Set measurable goals: Use Key Performance Indicators (KPIs) to track progress and performance. This allows for objective assessment of individual and team contributions, including SASB metrics that align with sustainability goals.
- Implement regular reviews: Schedule periodic evaluations to assess performance against established goals. This can include one-on-one meetings, team reviews, and feedback sessions, ensuring that SASB disclosures are considered in the evaluation process.
- Foster a culture of accountability: Encourage open communication and ownership of tasks. Recognize and reward individuals who demonstrate accountability in their work, particularly in relation to the SASB framework of accountability.
- Utilize technology: Leverage project management tools and software to track progress and document responsibilities. This can enhance visibility and accountability across the organization, especially when integrating the SASB framework ESG into operations.
An effective accountability framework not only improves performance but also enhances employee engagement and satisfaction. When individuals understand their roles and feel responsible for their contributions, they are more likely to be motivated and committed to the organization's success, particularly in sectors defined by SASB sectors.
11. Operational Challenges

‍
Operational challenges refer to the difficulties organizations face in their day-to-day operations. These challenges can hinder efficiency, productivity, and overall performance. Identifying and addressing these challenges is crucial for maintaining a competitive edge.
- Resource allocation: Ensuring that resources, including personnel, finances, and technology, are allocated effectively can be a significant challenge. Misallocation can lead to bottlenecks and inefficiencies.
- Process inefficiencies: Outdated or poorly designed processes can slow down operations. Regularly reviewing and optimizing workflows is essential to enhance productivity.
- Change management: Implementing new technologies or processes can meet resistance from employees. Effective change management strategies are necessary to facilitate smooth transitions.
- Compliance and regulations: Keeping up with industry regulations and compliance requirements can be daunting. Organizations must stay informed and adapt their operations accordingly, including adherence to the SASB accountability framework.
- Communication barriers: Poor communication can lead to misunderstandings and errors. Establishing clear communication channels is vital for operational success.
Addressing these operational challenges requires a proactive approach, including continuous improvement initiatives and employee training programs.
11.1. False Positives Management
False positives occur when a system incorrectly identifies a benign event as a threat or issue. This is particularly relevant in fields such as cybersecurity, fraud detection, and quality control. Managing false positives is crucial to ensure that resources are not wasted on unnecessary investigations.
- Analyze data accuracy: Regularly review the data inputs and algorithms used in detection systems. Ensuring high-quality data can reduce the likelihood of false positives.
- Implement threshold adjustments: Fine-tune the sensitivity of detection systems. Adjusting thresholds can help balance the trade-off between catching true threats and minimizing false alarms.
- Utilize machine learning: Incorporate machine learning algorithms that can learn from past data and improve accuracy over time. These systems can adapt to new patterns and reduce false positives.
- Establish a review process: Create a systematic approach for reviewing flagged incidents. This can involve a dedicated team to assess alerts and determine their validity.
- Provide training: Educate employees on recognizing and responding to false positives. Training can help staff differentiate between genuine threats and false alarms, improving overall response efficiency.
Effectively managing false positives not only saves time and resources but also enhances the credibility of detection systems. By minimizing unnecessary alerts, organizations can focus on genuine threats and improve their operational efficiency.
At Rapid Innovation, we leverage our expertise in AI and Blockchain to help organizations implement robust accountability frameworks, including the SASB sustainability framework, and address operational challenges effectively. By utilizing advanced machine learning algorithms, we can enhance data accuracy and reduce false positives, ultimately driving greater ROI for our clients. Our tailored solutions ensure that organizations can navigate their operational hurdles while fostering a culture of accountability and transparency.
11.2. Resource Optimization
Resource optimization is a critical aspect of any organization aiming to enhance efficiency and productivity. It involves the strategic allocation and management of resources—be it human, financial, or technological—to achieve maximum output with minimal waste.
- Efficient use of resources can lead to significant cost savings.
- Identifying underutilized assets helps in reallocating them effectively.
- Implementing resource optimization strategies can improve project timelines and deliverables.
- Techniques such as Lean Management and Six Sigma can be employed to streamline processes.
- Utilizing technology, such as AI and data analytics, can provide insights into resource allocation and usage patterns.
At Rapid Innovation, we leverage AI-driven analytics to help organizations identify inefficiencies and optimize their resource allocation. For example, by implementing machine learning algorithms, we can analyze historical data to predict future resource needs, ensuring that our clients are not over or under-resourced. Organizations that focus on resource optimization often see improved performance metrics and a stronger competitive edge in their industry. For instance, companies that adopt resource optimization strategies can reduce operational costs by up to 30%. Additionally, our expertise in project estimation ensures that resources are allocated effectively from the outset, further enhancing project success. Furthermore, our insights on AI agents for resource allocation can provide organizations with innovative solutions to optimize their resource management.
11.3. Skills Gap and Training
The skills gap refers to the disparity between the skills required for a job and the skills possessed by the workforce. This gap can hinder organizational growth and innovation. Addressing the skills gap through targeted training programs is essential for maintaining a competitive workforce.
- Regular training and development programs can help bridge the skills gap.
- Upskilling and reskilling initiatives are crucial in adapting to technological advancements.
- Conducting skills assessments allows organizations to identify gaps and tailor training accordingly.
- Collaboration with educational institutions can create a pipeline of skilled talent.
- Investing in employee development can lead to higher job satisfaction and retention rates.
At Rapid Innovation, we offer tailored training programs that focus on AI and blockchain technologies, ensuring that your workforce is equipped with the necessary skills to thrive in a rapidly evolving landscape. According to a report by the World Economic Forum, 54% of all employees will require significant reskilling by 2022. This statistic underscores the importance of continuous learning and development in the workplace.
11.4. Cost Considerations
Cost considerations are vital for any business strategy, influencing decisions on resource allocation, project management, and overall financial health. Understanding the various cost factors can help organizations make informed choices that align with their financial goals.
- Analyzing fixed and variable costs is essential to determine overall expenditure.
- Budgeting and forecasting are crucial for anticipating future costs and revenues.
- Cost-benefit analysis can aid in evaluating the financial viability of projects.
- Considering both direct and indirect costs is important when making decisions.
- Implementing cost control measures can enhance profitability and sustainability.
Effective cost management can lead to improved financial performance. At Rapid Innovation, we utilize blockchain technology to enhance transparency and traceability in financial transactions, which can significantly reduce fraud and errors, leading to cost savings. According to a study by Deloitte, organizations that prioritize cost management can achieve up to 20% higher profitability. This highlights the importance of integrating cost considerations into strategic planning.
11.5. Integration Complexity
Integration complexity refers to the challenges organizations face when trying to incorporate new technologies into their existing systems. As businesses increasingly adopt advanced technologies like artificial intelligence (AI), machine learning, and cloud computing, the complexity of integration can significantly impact operational efficiency and overall success.
- Diverse Systems: Organizations often operate with a mix of legacy systems and modern applications, making integration a daunting task. Each system may have different data formats, protocols, and interfaces, complicating the integration process.
- Data Silos: Many organizations struggle with data silos, where information is isolated within departments. This fragmentation can hinder the seamless flow of data necessary for effective integration, leading to inefficiencies and missed opportunities.
- Resource Allocation: Integrating new technologies often requires significant time and resources. Organizations may need to invest in training staff, hiring specialists, or upgrading infrastructure, which can strain budgets and timelines.
- Change Management: The human element of integration cannot be overlooked. Employees may resist changes to their workflows, leading to potential disruptions. Effective change management strategies are essential to ensure smooth transitions.
- Security Concerns: Integrating new technologies can introduce vulnerabilities. Organizations must ensure that security measures are in place to protect sensitive data during and after the integration process.
- Compliance Issues: Different technologies may have varying compliance requirements. Organizations must navigate these regulations to avoid legal repercussions and ensure that their integrations meet industry standards.
Addressing integration complexity requires a strategic approach, including thorough planning, stakeholder engagement, and leveraging integration platforms that can simplify the process. Rapid Innovation specializes in providing tailored solutions that help organizations navigate these technology integration challenges, ensuring a smoother integration of AI and blockchain technologies. By understanding the challenges and proactively addressing them, organizations can enhance their ability to integrate new technologies effectively, ultimately leading to greater ROI.
12. Future Trends and Innovation

‍
The landscape of technology is constantly evolving, and staying ahead of future trends is crucial for organizations aiming to maintain a competitive edge. Several key trends are shaping the future of technology and innovation.
- Artificial Intelligence Advancements: AI continues to evolve, with advancements in natural language processing, computer vision, and automation. These developments are enabling more sophisticated applications across various industries.
- Sustainability and Green Technology: As environmental concerns grow, organizations are increasingly focusing on sustainable practices. Innovations in renewable energy, waste reduction, and eco-friendly materials are becoming essential components of business strategies.
- Remote Work Technologies: The shift to remote work has accelerated the development of tools and technologies that facilitate collaboration and productivity. Innovations in virtual reality (VR) and augmented reality (AR) are enhancing remote work experiences.
- Cybersecurity Innovations: With the rise in cyber threats, organizations are investing in advanced cybersecurity measures. Innovations in AI-driven security solutions and zero-trust architectures are becoming critical for protecting sensitive data.
- Blockchain Technology: Blockchain is gaining traction beyond cryptocurrencies. Its potential for enhancing transparency, security, and efficiency in various sectors, including supply chain management and finance, is driving innovation.
- Internet of Things (IoT): The proliferation of IoT devices is creating new opportunities for data collection and analysis. Organizations are leveraging IoT to improve operational efficiency and enhance customer experiences.
12.1. Quantum-resistant AI
As quantum computing technology advances, the need for quantum-resistant AI becomes increasingly important. Quantum computers have the potential to break traditional encryption methods, posing significant risks to data security. Quantum-resistant AI refers to the development of artificial intelligence systems that can withstand the challenges posed by quantum computing.
- Enhanced Security Protocols: Quantum-resistant AI focuses on creating new encryption algorithms that are secure against quantum attacks. These protocols are essential for protecting sensitive data in various applications, from finance to healthcare.
- Improved Data Privacy: As quantum computing threatens existing privacy measures, quantum-resistant AI aims to ensure that personal and sensitive information remains secure. This is particularly crucial in industries that handle large volumes of confidential data.
- Accelerated Problem Solving: Quantum computing can solve complex problems at unprecedented speeds. Quantum-resistant AI can leverage these capabilities to enhance decision-making processes and optimize operations across various sectors.
- Collaboration Between Fields: The intersection of quantum computing and AI is fostering collaboration between researchers and technologists. This interdisciplinary approach is driving innovation and leading to the development of new solutions that address emerging challenges.
- Future-proofing Technology: As organizations invest in AI technologies, ensuring that these systems are quantum-resistant is vital for long-term sustainability. This proactive approach can help organizations mitigate risks associated with future technological advancements.
In conclusion, the integration of quantum-resistant AI into existing systems is essential for organizations looking to safeguard their data and maintain a competitive edge in an increasingly complex technological landscape. Rapid Innovation is committed to helping clients navigate these advancements, ensuring they are well-prepared for the future.
12.2. Advanced Attribution Techniques
Advanced attribution techniques are essential for understanding the effectiveness of marketing campaigns and customer interactions. These methods go beyond traditional attribution models, providing deeper insights into consumer behavior and the impact of various touchpoints.
- Multi-Touch Attribution (MTA): This technique assigns value to multiple interactions a customer has with a brand before making a purchase, helping marketers understand the full customer journey.
- Algorithmic Attribution: Utilizing machine learning algorithms, this method analyzes vast amounts of data to determine the most influential touchpoints. It can adapt over time, improving accuracy as more data is collected.
- Data-Driven Attribution: This approach uses statistical models to evaluate the contribution of each channel based on actual conversion data, providing a more precise understanding of how different channels work together.
- Customer Journey Mapping: By visualizing the customer journey, businesses can identify key moments that influence decisions, which helps in optimizing marketing strategies.
- Predictive Analytics: Leveraging historical data, predictive analytics forecasts future customer behavior, allowing marketers to tailor their strategies accordingly.
These advanced attribution techniques enable businesses to allocate resources more effectively, optimize marketing spend, and ultimately drive better ROI. At Rapid Innovation, we leverage these advanced attribution techniques to help our clients enhance their marketing strategies, ensuring they achieve maximum return on investment through data-driven insights.
12.3. Autonomous Security Systems
Autonomous security systems represent a significant advancement in the field of security technology. These systems utilize artificial intelligence and machine learning to enhance safety and efficiency.
- Real-Time Monitoring: Autonomous security systems can continuously monitor environments using cameras and sensors, providing instant alerts for any suspicious activity.
- Automated Response: These systems can take immediate action in response to threats, such as locking doors or alerting authorities, without human intervention.
- Facial Recognition: Advanced algorithms enable these systems to identify individuals in real-time, enhancing security in public spaces and restricted areas.
- Predictive Threat Analysis: By analyzing patterns and behaviors, autonomous systems can predict potential security threats before they occur, allowing for proactive measures.
- Integration with IoT Devices: These systems can connect with various Internet of Things (IoT) devices, creating a comprehensive security network that enhances overall safety.
The implementation of autonomous security systems not only improves security but also reduces the need for human oversight, allowing security personnel to focus on more complex tasks. Rapid Innovation specializes in developing these systems, ensuring that our clients benefit from cutting-edge technology to safeguard their assets.
12.4. Cross-platform Integration
Cross-platform integration is crucial for businesses looking to streamline operations and enhance user experiences across different platforms. This approach ensures that various systems and applications work together seamlessly.
- Unified User Experience: By integrating platforms, businesses can provide a consistent experience for users, regardless of the device or application they are using.
- Data Synchronization: Cross-platform integration allows for real-time data sharing between systems, ensuring that all platforms have access to the most current information.
- Improved Collaboration: Teams can collaborate more effectively when tools and applications are integrated, leading to increased productivity and efficiency.
- Enhanced Customer Insights: By combining data from multiple platforms, businesses can gain a holistic view of customer behavior, enabling more targeted marketing strategies.
- Scalability: Cross-platform integration supports business growth by allowing new applications and systems to be added without disrupting existing operations.
Investing in cross-platform integration not only enhances operational efficiency but also improves customer satisfaction by providing a seamless experience across all touchpoints. At Rapid Innovation, we provide tailored solutions that ensure your systems are integrated effectively, driving growth and enhancing user engagement.
12.5. AI-powered Threat Intelligence
AI-powered threat intelligence refers to the use of artificial intelligence technologies to enhance the detection, analysis, and response to cybersecurity threats. This approach leverages machine learning, natural language processing, and data analytics to provide organizations with actionable insights into potential threats.
- Enhanced Detection: AI algorithms can analyze vast amounts of data in real-time, identifying patterns and anomalies that may indicate a security breach. This capability allows for quicker detection of threats compared to traditional methods.
- Predictive Analytics: By utilizing historical data, AI can predict potential future attacks. This proactive approach helps organizations to strengthen their defenses before an attack occurs.
- Automated Response: AI can automate responses to certain types of threats, reducing the time it takes to mitigate risks. For example, if a phishing attempt is detected, AI can automatically quarantine the affected email.
- Continuous Learning: AI systems continuously learn from new data, improving their accuracy and effectiveness over time. This adaptability is crucial in the ever-evolving landscape of cyber threats.
- Integration with Existing Systems: AI-powered threat intelligence can be integrated with existing security systems, enhancing their capabilities without requiring a complete overhaul.
- Cost Efficiency: By automating threat detection and response, organizations can reduce the need for extensive human resources, leading to cost savings.
- Real-time Threat Intelligence Sharing: AI can facilitate the sharing of threat intelligence across organizations, creating a collaborative defense against cyber threats.
13. Case Studies and Best Practices
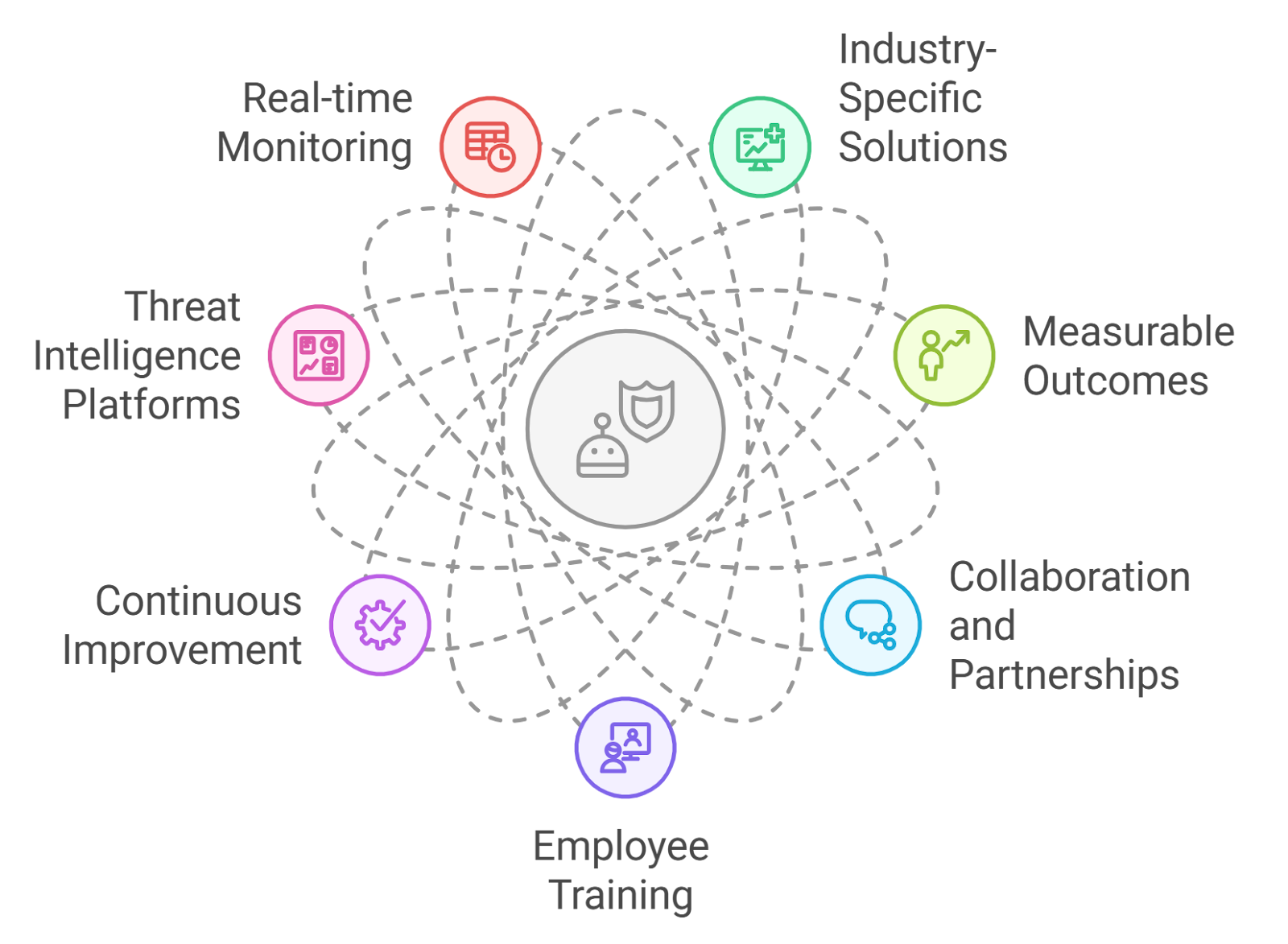
‍
Case studies and best practices provide valuable insights into how organizations successfully implement AI-powered threat intelligence. By examining real-world examples, businesses can learn from the experiences of others and apply similar strategies to enhance their cybersecurity posture.
- Industry-Specific Solutions: Different industries face unique threats. Case studies often highlight tailored solutions that address specific vulnerabilities, such as healthcare data breaches or financial fraud.
- Measurable Outcomes: Successful implementations often showcase quantifiable results, such as reduced incident response times or decreased breach occurrences. These metrics help organizations understand the potential ROI of investing in AI-powered threat intelligence.
- Collaboration and Partnerships: Many case studies emphasize the importance of collaboration between organizations and cybersecurity vendors. Partnerships can lead to more robust threat intelligence sharing and improved security measures.
- Employee Training: Best practices often include training employees on recognizing threats and understanding the importance of cybersecurity. A well-informed workforce can act as a first line of defense against attacks.
- Continuous Improvement: Organizations that regularly assess and update their threat intelligence strategies tend to be more resilient. Best practices encourage ongoing evaluation and adaptation to new threats.
- Use of Threat Intelligence Platforms: Many successful case studies highlight the implementation of dedicated threat intelligence platforms that aggregate data from various sources, providing a comprehensive view of the threat landscape.
- Real-time Monitoring: Organizations that employ real-time monitoring and analysis of their networks can respond more swiftly to potential threats, minimizing damage.
13.1. Enterprise Implementation Examples
Enterprise implementation examples illustrate how large organizations have successfully integrated AI-powered threat intelligence into their cybersecurity frameworks. These examples serve as a guide for other enterprises looking to enhance their security measures.
- Financial Sector: A major bank implemented an AI-driven threat intelligence system that analyzed transaction data for signs of fraud. By using machine learning algorithms, the bank reduced false positives by 30% and improved fraud detection rates significantly.
- Healthcare Industry: A large healthcare provider adopted AI-powered threat intelligence to protect sensitive patient data. The system monitored network traffic and identified unusual access patterns, leading to a 40% decrease in data breaches.
- Retail Sector: A global retail chain utilized AI to analyze customer transaction data and detect potential cyber threats. The implementation resulted in faster incident response times and a notable reduction in successful phishing attacks.
- Government Agencies: A government agency integrated AI threat intelligence to monitor and respond to cyber threats targeting national security. The system provided real-time alerts and automated responses, enhancing the agency's overall security posture.
- Technology Companies: A leading tech firm employed AI to analyze software vulnerabilities in real-time. This proactive approach allowed the company to patch vulnerabilities before they could be exploited, significantly reducing the risk of cyberattacks.
- Telecommunications: A major telecom provider used AI to monitor network traffic for signs of Distributed Denial of Service (DDoS) attacks. The system enabled the provider to mitigate attacks quickly, ensuring uninterrupted service for customers.
- Manufacturing: A manufacturing company implemented AI-driven threat intelligence to protect its operational technology (OT) systems. By monitoring for anomalies, the company was able to prevent potential disruptions to production.
These enterprise implementation examples highlight the versatility and effectiveness of AI-powered threat intelligence across various sectors, demonstrating its potential to enhance cybersecurity measures significantly. At Rapid Innovation, we leverage our expertise in AI-powered threat intelligence to help organizations implement these advanced solutions, ensuring they achieve greater ROI and a robust security posture.
13.2. Success Stories
Success stories in various industries highlight the transformative power of innovative strategies and technologies, including success stories in technology. These narratives not only inspire but also provide valuable insights into effective practices.
- Case Studies: Many organizations have documented their success stories, showcasing how they overcame challenges through strategic planning and execution. For instance, a tech startup that implemented agile methodologies saw a 40% increase in productivity within six months. At Rapid Innovation, we have helped similar startups integrate AI-driven project management tools, resulting in enhanced efficiency and a significant reduction in time-to-market.
- Customer Testimonials: Positive feedback from clients can serve as powerful endorsements. Companies that prioritize customer satisfaction often report higher retention rates and increased referrals. Our clients have expressed how our blockchain solutions have not only streamlined their operations but also built trust with their customers through enhanced transparency.
- Quantifiable Results: Success stories often include metrics that demonstrate the impact of specific initiatives. For example, a retail chain that adopted an omnichannel approach experienced a 30% boost in sales. Rapid Innovation has empowered retailers with AI analytics to optimize inventory management, leading to improved sales forecasting and reduced stockouts. Additionally, our work with top AI cryptocurrencies has provided insights into the evolving landscape of digital assets.
- Industry Recognition: Awards and accolades can validate a company's success. Recognition from industry bodies can enhance credibility and attract new business opportunities. Our innovative AI and blockchain solutions have garnered industry awards, showcasing our commitment to excellence and our clients' success.
13.3. Lessons Learned
Lessons learned from both successes and failures are crucial for continuous improvement. Analyzing these experiences can lead to better decision-making and strategic planning. Organizations that adapt to changing market conditions tend to thrive, as flexibility in operations allows businesses to pivot when necessary. Utilizing analytics to inform decisions can lead to more effective strategies, and companies that leverage data often outperform their competitors. Successful projects often involve cross-functional teams, as collaboration fosters innovation and ensures diverse perspectives are considered. Understanding potential risks and having contingency plans can mitigate negative impacts, positioning companies that proactively manage risks to handle crises more effectively. Furthermore, organizations that prioritize learning and development create a culture of innovation, and investing in employee training can lead to improved performance and job satisfaction.
13.4. Industry-specific Applications
Different industries can leverage unique applications of technology and strategies to enhance their operations. Understanding these applications can lead to tailored solutions that drive success.
- Healthcare: Telemedicine has revolutionized patient care, allowing for remote consultations and improved access to healthcare services. This application has become increasingly vital, especially during the COVID-19 pandemic. Rapid Innovation has developed AI-powered telehealth platforms that enhance patient engagement and streamline care delivery.
- Manufacturing: The adoption of Industry 4.0 technologies, such as IoT and automation, has streamlined production processes. These innovations lead to increased efficiency and reduced operational costs. Our blockchain solutions ensure secure and transparent supply chain management, further optimizing manufacturing operations.
- Retail: E-commerce platforms have transformed the retail landscape, enabling businesses to reach a global audience. Personalization through data analytics enhances customer experience and drives sales. Rapid Innovation's AI algorithms help retailers analyze consumer behavior, enabling targeted marketing strategies that boost conversion rates.
- Finance: Fintech solutions, including mobile banking and blockchain technology, have disrupted traditional banking. These innovations offer greater convenience and security for consumers. Our blockchain applications enhance transaction security and reduce fraud, providing clients with peace of mind.
- Education: Online learning platforms have made education more accessible. The integration of technology in classrooms enhances engagement and facilitates personalized learning experiences. Rapid Innovation has partnered with educational institutions to implement AI-driven learning management systems that adapt to individual student needs, improving educational outcomes.
13.5. ROI Analysis
Return on Investment (ROI) analysis is a critical component of evaluating the effectiveness of any business initiative or marketing strategy, including the role of a roi business analyst. It helps organizations determine the profitability of their investments and make informed decisions moving forward.
- Definition: ROI is a financial metric that compares the gain or loss from an investment relative to its cost. It is expressed as a percentage and calculated using the formula:
language="language-plaintext"ROI = (Net Profit / Cost of Investment) x 100
- Importance of ROI Analysis: Â
- Assists in budget allocation by identifying which projects yield the highest returns, particularly in AI and Blockchain initiatives where technology investments can be substantial.
- Helps in justifying expenditures to stakeholders and securing future funding, especially when demonstrating the potential of AI-driven solutions or Blockchain applications.
- Provides insights into the effectiveness of marketing campaigns, product launches, or operational changes, allowing organizations to pivot strategies based on data-driven results, including roi in business analysis.
- Steps in Conducting ROI Analysis: Â
- Identify the investment costs, including direct and indirect expenses associated with AI and Blockchain projects.
- Measure the returns generated from the investment, which can include increased sales, cost savings, or enhanced productivity through automation and decentralized systems.
- Calculate the ROI using the formula mentioned above.
- Analyze the results to determine if the investment was worthwhile and if similar strategies should be pursued in the future.
- Challenges in ROI Analysis: Â
- Difficulty in quantifying intangible benefits, such as brand awareness or customer satisfaction, particularly in emerging technologies like AI and Blockchain.
- Variability in timeframes for realizing returns, which can complicate comparisons, especially in long-term projects.
- The need for accurate data collection and analysis to ensure reliable results, emphasizing the importance of robust data management systems.
14. Performance Measurement and Optimization
Performance measurement and optimization are essential for ensuring that business strategies are effective and aligned with organizational goals. This process involves tracking key metrics, analyzing performance data, and making necessary adjustments to improve outcomes.
- Importance of Performance Measurement: Â
- Provides a clear understanding of how well a business is performing against its objectives, particularly in the context of AI and Blockchain implementations.
- Identifies areas for improvement and helps in setting realistic goals, ensuring that technology investments are aligned with business outcomes.
- Facilitates data-driven decision-making, leading to better resource allocation and maximizing ROI.
- Key Components of Performance Measurement: Â
- Data Collection: Gathering relevant data from various sources, including sales reports, customer feedback, and operational metrics, particularly from AI analytics and Blockchain transactions.
- Analysis: Evaluating the collected data to identify trends, patterns, and areas of concern, leveraging AI tools for deeper insights.
- Reporting: Communicating findings to stakeholders through dashboards, reports, or presentations, ensuring transparency and accountability.
- Optimization Strategies: Â
- Continuous Improvement: Implementing a culture of ongoing assessment and refinement of processes, particularly in AI model training and Blockchain protocol enhancements.
- A/B Testing: Experimenting with different approaches to determine which yields better results, especially in marketing strategies powered by AI.
- Feedback Loops: Establishing mechanisms for regular feedback from customers and employees to inform adjustments, ensuring that solutions remain relevant and effective.
14.1. Key Performance Indicators
Key Performance Indicators (KPIs) are quantifiable metrics that organizations use to measure their success in achieving specific objectives. KPIs provide a clear framework for assessing performance and guiding strategic decisions, including roi analysis for business.
- Characteristics of Effective KPIs: Â
- Specific: Clearly defined and focused on a particular area of performance.
- Measurable: Quantifiable to track progress over time.
- Achievable: Realistic and attainable within the given timeframe.
- Relevant: Aligned with the organization's goals and objectives, particularly in the context of AI and Blockchain initiatives.
- Time-bound: Set within a specific timeframe for evaluation.
- Types of KPIs: Â
- Financial KPIs: Metrics such as revenue growth, profit margins, and return on investment, crucial for assessing the financial impact of AI and Blockchain projects.
- Customer KPIs: Metrics that assess customer satisfaction, retention rates, and net promoter scores, particularly relevant in user-centric AI applications.
- Operational KPIs: Metrics that evaluate efficiency, productivity, and process effectiveness, essential for optimizing AI algorithms and Blockchain operations.
- Implementing KPIs: Â
- Define Objectives: Clearly outline what the organization aims to achieve with its AI and Blockchain strategies.
- Select Relevant KPIs: Choose metrics that align with the defined objectives, ensuring they reflect the unique aspects of technology-driven initiatives.
- Set Targets: Establish benchmarks for success to measure against.
- Monitor and Review: Regularly track performance and adjust strategies as needed, leveraging AI analytics for real-time insights.
- Benefits of Using KPIs: Â
- Enhances accountability by assigning responsibility for specific metrics.
- Provides a clear focus for teams and individuals, aligning efforts with organizational goals.
- Facilitates communication and collaboration across departments by sharing performance data.
By effectively utilizing ROI analysis, performance measurement, and KPIs, organizations can optimize their strategies, improve decision-making, and ultimately drive better business outcomes, particularly in the rapidly evolving fields of AI and Blockchain.
14.2. Monitoring and Reporting
Monitoring and reporting are essential components of any effective management system. They ensure that organizations can track their performance, identify areas for improvement, and make informed decisions. At Rapid Innovation, we leverage AI-driven analytics to enhance monitoring processes, enabling clients to gain deeper insights into their operations, including insights from the gartner magic quadrant application performance monitoring and gartner apm magic quadrant.
- Purpose of Monitoring: Â
- To assess the effectiveness of processes and systems.
- To ensure compliance with regulations and standards.
- To identify trends and patterns that may require attention.
- Key Elements of Monitoring: Â
- Data Collection: Gathering relevant data from various sources, including operational metrics, customer feedback, and financial reports. Our AI solutions automate data collection, ensuring accuracy and timeliness.
- Performance Indicators: Establishing key performance indicators (KPIs) to measure success. These can include productivity rates, customer satisfaction scores, and financial performance metrics. We help clients define and track KPIs that align with their strategic goals, including those highlighted in the gartner apm report.
- Regular Reviews: Conducting periodic reviews to analyze the collected data and assess performance against established benchmarks. Our blockchain technology ensures data integrity during these reviews.
- Reporting: Â
- Frequency: Reports should be generated regularly (e.g., monthly, quarterly) to provide timely insights. We offer customizable reporting solutions that adapt to client needs, including insights from the gartner apm magic quadrant 2022.
- Stakeholder Communication: Sharing reports with stakeholders, including management, employees, and external partners, to ensure transparency and accountability. Our platforms facilitate seamless communication and collaboration.
- Actionable Insights: Reports should highlight not just the data but also actionable insights that can guide decision-making. Our AI algorithms analyze data trends to provide recommendations for improvement, including those derived from the application performance reporting metrics.
14.3. Continuous Improvement
Continuous improvement is a systematic approach to enhancing processes, products, or services over time. It focuses on incremental changes that lead to significant benefits. Rapid Innovation employs AI and blockchain technologies to foster a culture of continuous improvement among our clients.
- Principles of Continuous Improvement: Â
- Customer Focus: Understanding customer needs and expectations to drive improvements.
- Employee Involvement: Engaging employees at all levels to contribute ideas and solutions.
- Data-Driven Decisions: Utilizing data and metrics to identify areas for improvement and measure progress.
- Methods for Continuous Improvement: Â
- Plan-Do-Check-Act (PDCA): A cyclical process that involves planning changes, implementing them, checking results, and acting on what is learned. Our AI tools streamline this process, making it more efficient.
- Lean Methodology: Aiming to eliminate waste and enhance value by streamlining processes. We help clients implement lean practices supported by data analytics.
- Six Sigma: A data-driven approach that seeks to reduce defects and improve quality. Our expertise in AI allows for precise measurement and analysis of quality metrics.
- Benefits of Continuous Improvement: Â
- Increased efficiency and productivity.
- Enhanced customer satisfaction and loyalty.
- Greater employee engagement and morale.
14.4. Benchmarking
Benchmarking is the process of comparing an organization's performance metrics to industry bests or best practices from other organizations. It serves as a tool for identifying gaps and opportunities for improvement. Rapid Innovation assists clients in leveraging benchmarking to enhance their competitive edge, including insights from the gartner magic quadrant monitoring.
- Types of Benchmarking: Â
- Internal Benchmarking: Comparing performance across different departments or units within the same organization.
- Competitive Benchmarking: Analyzing performance against direct competitors to understand relative strengths and weaknesses. Our AI tools provide insights into competitor performance, including data from the gartner mq apm 2022.
- Functional Benchmarking: Looking at similar functions or processes in different industries to gain insights.
- Steps in the Benchmarking Process: Â
- Identify Metrics: Determine which performance metrics are relevant for comparison.
- Select Benchmarking Partners: Choose organizations or industry leaders known for their excellence in the identified metrics.
- Collect Data: Gather data on performance metrics from both your organization and benchmarking partners. Our blockchain solutions ensure secure and transparent data sharing.
- Analyze Results: Compare your performance against the benchmarks to identify gaps and areas for improvement. Our AI analytics provide deep insights into performance gaps, including those highlighted in the gartner apm magic quadrant 2021.
- Implement Changes: Develop and implement strategies to close the performance gaps identified through benchmarking.
- Benefits of Benchmarking: Â
- Provides a clear understanding of where an organization stands in relation to peers.
- Encourages a culture of learning and improvement.
- Helps set realistic performance goals based on industry standards, including insights from the 2021 gartner magic quadrant for application performance monitoring.
By integrating AI and blockchain technologies into these processes, Rapid Innovation empowers clients to achieve greater ROI and drive sustainable growth.
14.5. Quality Assurance
Quality assurance (QA) is a systematic process designed to determine whether a product or service meets specified requirements and standards. It plays a crucial role in various industries, including manufacturing, software development, and healthcare. The primary goal of QA is to enhance customer satisfaction by ensuring that products are reliable, safe, and of high quality.
- Definition and Importance: Quality assurance refers to the planned and systematic activities implemented in a quality system to ensure that quality requirements for a product or service will be fulfilled. It is essential for maintaining a competitive edge in the market, as high-quality products lead to increased customer loyalty and reduced costs associated with defects and rework.
- Key Components of Quality Assurance: Â
- Process Definition: Establishing clear processes and standards that guide the production or development of products, including qa and testing methodologies.
- Documentation: Maintaining comprehensive records of processes, procedures, and outcomes to ensure traceability and accountability.
- Training: Providing ongoing training for employees to ensure they understand quality standards and practices, including qa planning and quality assurance methodology.
- Audits and Reviews: Conducting regular audits to assess compliance with quality standards and identify areas for improvement.
- Quality Assurance vs. Quality Control: While quality assurance focuses on preventing defects through planned processes, quality control is about identifying defects in finished products. QA is proactive, aiming to improve processes, whereas QC is reactive, addressing issues after they occur. This distinction is crucial in software quality assurance (SQA) and software testing and quality assurance practices.
- Benefits of Quality Assurance: Â
- Increased Efficiency: Streamlined processes reduce waste and improve productivity, particularly in software testing and qa processes.
- Cost Reduction: Fewer defects lead to lower costs associated with returns, repairs, and customer complaints.
- Enhanced Reputation: Consistently high-quality products build brand trust and customer loyalty.
- Regulatory Compliance: Many industries have strict regulations; QA helps ensure compliance, avoiding legal issues.
- Implementing a Quality Assurance Program: Â
- Define Objectives: Clearly outline what the QA program aims to achieve.
- Develop a QA Plan: Create a detailed plan that includes processes, responsibilities, and timelines, including procedures for quality assurance.
- Engage Stakeholders: Involve all relevant parties, including management, employees, and customers, in the QA process.
- Monitor and Measure: Use key performance indicators (KPIs) to track the effectiveness of the QA program and make necessary adjustments.
- Tools and Techniques for Quality Assurance: Â
- Statistical Process Control (SPC): A method of monitoring and controlling processes using statistical methods.
- Failure Mode and Effects Analysis (FMEA): A systematic approach to identifying potential failure modes and their impact on product performance.
- Root Cause Analysis (RCA): A technique used to identify the underlying causes of defects or problems, which is essential in qa testing software.
- Challenges in Quality Assurance: Â
- Resistance to Change: Employees may resist new processes or standards, hindering implementation.
- Resource Allocation: Adequate resources, including time and budget, are necessary for effective QA.
- Keeping Up with Standards: Quality standards and regulations can change, requiring continuous updates to QA processes, especially in the context of software qc.
- Future Trends in Quality Assurance: Â
- Automation: Increasing use of automated tools and software to streamline QA processes, including qa & testing tools.
- Data Analytics: Leveraging big data to gain insights into quality trends and improve decision-making.
- Agile QA: Adopting agile methodologies to enhance flexibility and responsiveness in QA processes.
At Rapid Innovation, we understand that quality assurance is a vital aspect of any organization aiming to deliver high-quality products and services. By implementing effective QA practices, including quality assurance testing software, we help our clients enhance customer satisfaction, reduce costs, and maintain a strong market position. Our expertise in AI and Blockchain allows us to integrate advanced technologies into QA processes, ensuring that our clients achieve greater ROI through improved efficiency and reliability. For more information on how we can assist with your AI project estimation needs, visit our AI for Quality Assurance and our AI project estimation company.