Table Of Contents
Category
CRM
AIML
Blockchain
FinTech
Retail & Ecommerce
1. Introduction to CLV and AI Agents
Customer Lifetime Value (CLV) is a crucial metric that estimates the total revenue a business can expect from a single customer throughout their relationship. Understanding CLV helps businesses make informed decisions about marketing, sales, and customer service strategies. CLV is calculated by considering factors such as purchase frequency, average order value, and customer retention rate. It allows businesses to identify high-value customers and tailor their strategies accordingly. By focusing on CLV, companies can enhance customer satisfaction and loyalty, ultimately driving profitability.
AI agents, or artificial intelligence agents, are software programs that use machine learning algorithms to analyze data and make predictions. In the context of customer lifetime value AI agents, these tools can process vast amounts of customer data to predict future behaviors and potential value. AI agents can analyze historical purchase data, customer interactions, and demographic information. They can identify patterns and trends that may not be apparent through traditional analysis. By leveraging AI, businesses can gain deeper insights into customer behavior, leading to more accurate CLV predictions.
At Rapid Innovation, we specialize in integrating AI solutions that enhance customer lifetime value AI agents predictions, enabling our clients to make data-driven decisions that maximize their return on investment (ROI). For instance, by implementing AI agents, we have helped clients in retail optimize their marketing strategies, resulting in a significant increase in customer retention and overall sales.
The integration of AI agents in CLV prediction offers numerous benefits, but it also presents challenges that businesses must navigate. Understanding both aspects is essential for organizations looking to enhance their customer relationship management strategies. Rapid Innovation is here to guide you through these complexities, ensuring that your business can leverage AI effectively to achieve its goals.
Refer to the image for a visual representation of the concepts discussed in the introduction to CLV and AI agents:

1.1. Understanding Customer Lifetime Value
Customer Lifetime Value (CLV) is a crucial metric that estimates the total revenue a business can expect from a single customer throughout their relationship. Understanding CLV helps businesses make informed decisions regarding marketing strategies, customer service, and product development.
- CLV is calculated by considering:
- Average purchase value
- Purchase frequency
- Customer lifespan
A higher CLV indicates a more valuable customer, which can lead to:
- Increased marketing budgets for high-value segments
- Improved customer retention strategies
Businesses can use CLV to:
- Identify profitable customer segments
- Allocate resources effectively
- Enhance customer experience and loyalty
At Rapid Innovation, we leverage advanced AI and blockchain technologies to help businesses accurately calculate and analyze CLV. By integrating AI-driven analytics, we enable our clients to identify high-value customers and tailor their marketing strategies accordingly. This targeted approach not only maximizes profitability but also fosters long-term customer relationships, ultimately leading to increased revenue. Techniques such as customer lifetime value prediction, predictive CLV, and user lifetime value analysis are essential in this process. For more insights on how AI can enhance marketing applications, visit this link.
1.2. Evolution of CLV Prediction Methods
The methods for predicting Customer Lifetime Value have evolved significantly over the years, adapting to changes in technology and consumer behavior.
- Early methods relied on simple calculations based on historical data, such as:
- Average revenue per user (ARPU)
- Basic cohort analysis
As data analytics advanced, businesses began using more sophisticated techniques, including:
- Regression analysis to identify key factors influencing CLV
- Predictive modeling to forecast future customer behavior
The rise of big data and machine learning has further transformed CLV prediction by enabling:
- Real-time data analysis
- More accurate predictions based on complex algorithms
Current methods often incorporate:
- Customer segmentation
- Behavioral analytics
- Predictive analytics to refine CLV estimates
At Rapid Innovation, we utilize cutting-edge machine learning algorithms and blockchain technology to enhance the accuracy of CLV predictions. By employing real-time data analysis and predictive modeling, we empower our clients to make informed decisions that optimize their marketing efforts and improve customer engagement. Our approach includes customer lifetime value prediction using machine learning and customer lifetime value models that leverage data science.
1.3. Role of AI in Modern CLV Prediction
Artificial Intelligence (AI) plays a pivotal role in modern CLV prediction, enhancing the accuracy and efficiency of forecasting models.
- AI technologies enable businesses to:
- Analyze vast amounts of data quickly and accurately
- Identify patterns and trends that may not be apparent through traditional methods
Key applications of AI in CLV prediction include:
- Machine learning algorithms that adapt and improve over time
- Natural language processing (NLP) for analyzing customer feedback and sentiment
- Predictive analytics that forecast customer behavior based on historical data
The benefits of using AI for CLV prediction include:
- Increased precision in estimating customer value
- Enhanced ability to segment customers based on behavior and preferences
- Improved targeting of marketing campaigns, leading to higher conversion rates
AI can also help in:
- Automating data collection and analysis processes
- Providing real-time insights for timely decision-making
Incorporating AI into CLV prediction not only streamlines the process but also empowers businesses to make data-driven decisions that enhance customer relationships and drive revenue growth. At Rapid Innovation, we specialize in integrating AI solutions that refine CLV estimates, enabling our clients to stay ahead in a competitive market. As technology continues to advance, the role of AI in CLV prediction will likely expand, offering even more sophisticated tools for understanding customer value, including customer lifetime value prediction models and clv prediction techniques.
Refer to the image for a visual representation of Customer Lifetime Value (CLV) and its components.
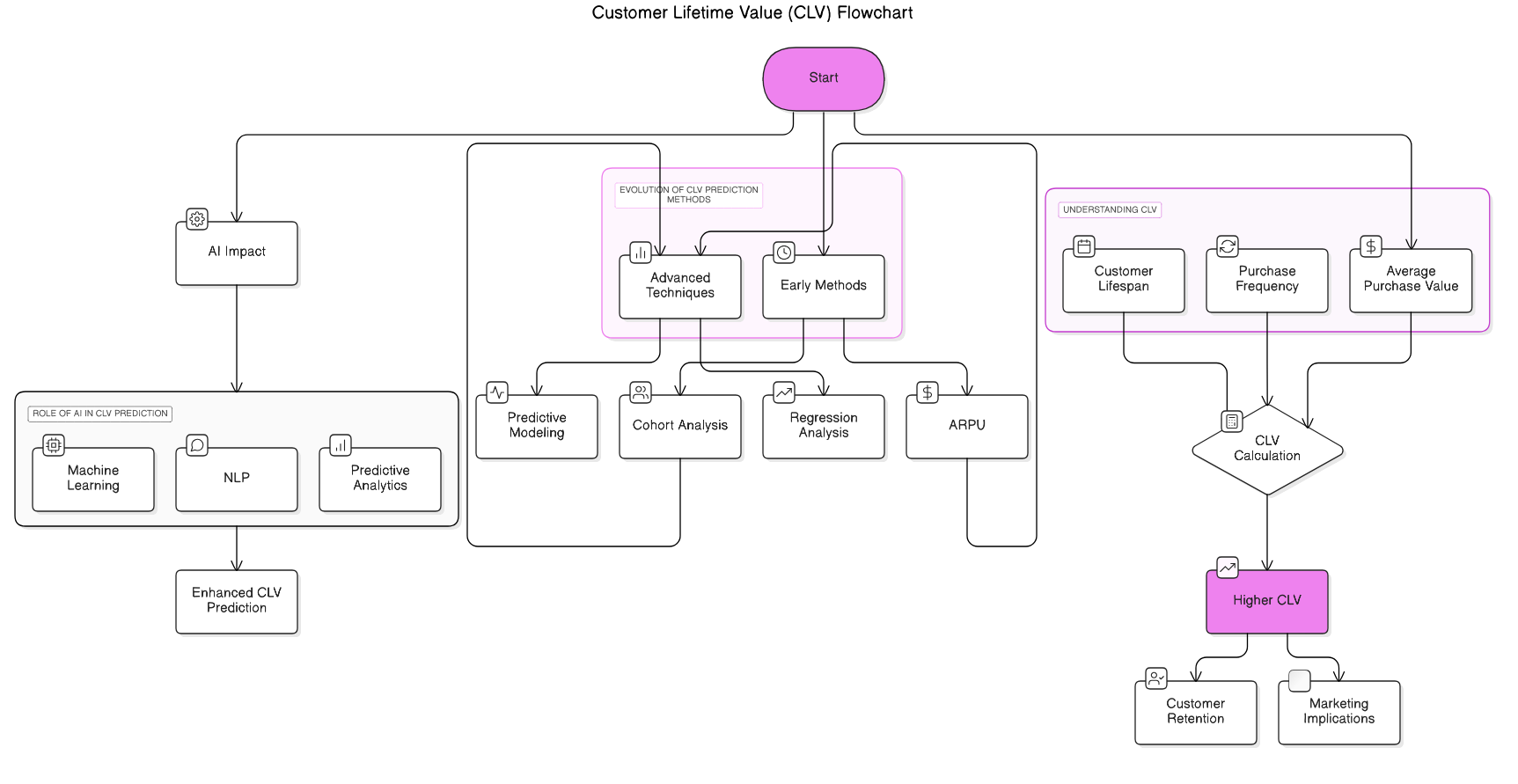
1.4. Business Impact and Significance
The business impact of Customer Lifetime Value (CLV) prediction is profound, influencing various aspects of a company's strategy and operations. Understanding CLV allows businesses to make informed decisions that can lead to increased profitability and customer satisfaction.
- Resource Allocation: Companies can allocate marketing budgets more effectively by identifying high-value customers through customer lifetime value prediction. This ensures that resources are directed towards retaining and acquiring customers who will generate the most revenue over time.
- Customer Segmentation: CLV prediction enables businesses to segment their customer base effectively. By understanding which segments are most profitable, companies can tailor their marketing strategies to meet the specific needs of these groups, enhancing their lifetime value prediction.
- Improved Customer Retention: By focusing on high CLV customers, businesses can implement targeted retention strategies. This can include personalized communication, loyalty programs, and exclusive offers that enhance customer satisfaction and loyalty, ultimately improving user lifetime value. For more insights on improving customer retention.
- Enhanced Product Development: Insights from CLV analysis can inform product development. Understanding customer preferences and behaviors allows businesses to create products that better meet the needs of their most valuable customers, leveraging customer lifetime value machine learning techniques.
- Strategic Pricing: Companies can adjust their pricing strategies based on CLV insights. For instance, they may choose to offer discounts to high CLV customers to encourage repeat purchases or implement premium pricing for products that appeal to this segment, informed by lifetime value prediction models.
- Long-term Planning: CLV prediction supports long-term business planning. By forecasting future revenue from existing customers, businesses can make strategic decisions regarding investments, expansions, and resource management, utilizing predictive CLV methodologies.
- Competitive Advantage: Organizations that effectively leverage CLV prediction can gain a competitive edge. By understanding their customers better than competitors, they can create more effective marketing campaigns and improve customer experiences, enhancing their customer lifetime value model machine learning capabilities.
2. Fundamentals of AI-Driven CLV Prediction
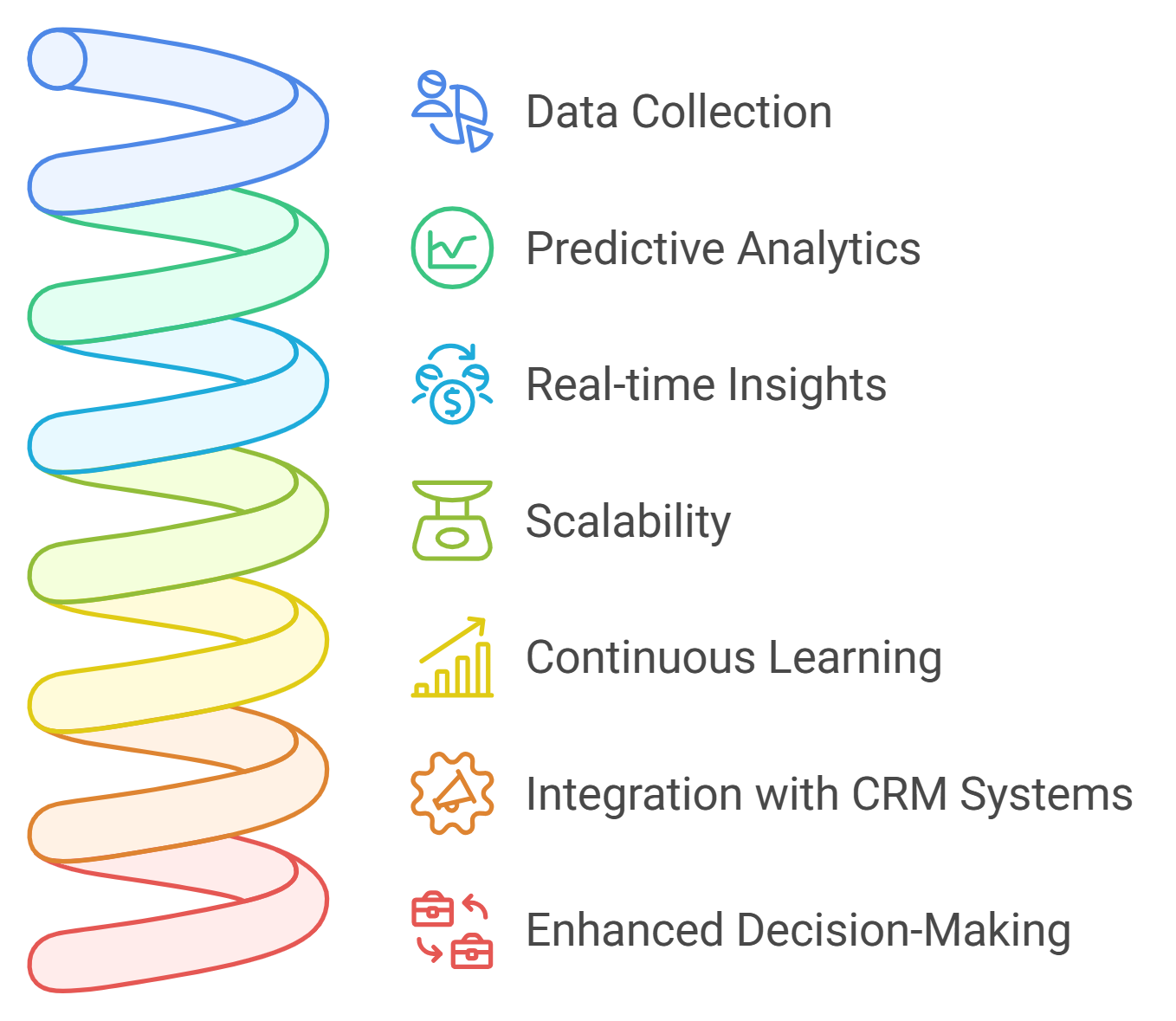
‍
AI-driven CLV prediction utilizes advanced algorithms and machine learning techniques to analyze customer data and forecast future value. This approach enhances the accuracy and efficiency of CLV calculations, providing businesses with actionable insights.
- Data Collection: AI systems gather vast amounts of data from various sources, including transaction history, customer interactions, and demographic information. This comprehensive data collection is crucial for accurate predictions in customer lifetime value prediction using machine learning.
- Predictive Analytics: Machine learning models analyze historical data to identify patterns and trends. These models can predict future customer behavior, allowing businesses to anticipate changes in CLV, thereby improving their lifetime value forecast.
- Real-time Insights: AI-driven systems can provide real-time insights, enabling businesses to make quick decisions based on the latest data. This agility is essential in today’s fast-paced market, particularly for customer lifetime value prediction in Python.
- Scalability: AI solutions can easily scale to accommodate growing datasets. As businesses expand, AI can continue to provide accurate CLV predictions without significant manual intervention, supporting customer lifetime value data science initiatives.
- Continuous Learning: AI models improve over time through continuous learning. As more data is collected, the algorithms refine their predictions, leading to increasingly accurate CLV estimates, which is vital for customer lifetime value machine learning applications.
- Integration with CRM Systems: AI-driven CLV prediction can be integrated with Customer Relationship Management (CRM) systems, allowing businesses to leverage customer insights directly within their existing workflows, enhancing their clv prediction model.
- Enhanced Decision-Making: By providing data-driven insights, AI enhances decision-making processes. Businesses can make informed choices regarding marketing strategies, customer engagement, and resource allocation, ultimately improving their customer lifetime value prediction machine learning efforts.
2.1. Core Components of CLV Calculation
Calculating Customer Lifetime Value involves several core components that contribute to an accurate assessment of a customer's worth over time. Understanding these components is essential for effective CLV prediction.
- Average Purchase Value: This is calculated by dividing total revenue by the number of purchases over a specific period. It helps businesses understand how much customers typically spend, which is crucial for customer value prediction.
- Purchase Frequency: This metric indicates how often a customer makes a purchase within a given timeframe. It is calculated by dividing the total number of purchases by the number of unique customers, aiding in lifetime value prediction.
- Customer Lifespan: This component estimates the average duration a customer remains active with a business. It can be determined by analyzing historical data on customer retention, which is essential for cltv prediction.
- Gross Margin: This is the difference between revenue and the cost of goods sold, expressed as a percentage of revenue. It provides insight into the profitability of each sale, impacting customer lifetime value calculations.
- Churn Rate: This metric measures the percentage of customers who stop doing business with a company over a specific period. A high churn rate can significantly impact CLV, making it vital for customer lifetime value prediction.
- Discount Rate: This is used to calculate the present value of future cash flows. It accounts for the time value of money, ensuring that future revenues are appropriately valued in customer lifetime value models.
- Customer Acquisition Cost (CAC): This is the cost associated with acquiring a new customer. Understanding CAC is crucial for determining the overall profitability of a customer over their lifetime, influencing customer lifetime value prediction.
- Retention Rate: This metric indicates the percentage of customers who continue to engage with a business over a specific period. A high retention rate typically correlates with a higher CLV, reinforcing the importance of customer lifetime value prediction.
By understanding these core components, businesses can develop more accurate CLV predictions, leading to better strategic decisions and improved customer relationships. Rapid Innovation leverages AI-driven methodologies to enhance these calculations, ensuring that clients can maximize their ROI through informed decision-making and strategic resource allocation in customer lifetime value prediction.
Refer to the image for a visual representation of the business impact and significance of Customer Lifetime Value (CLV) prediction.
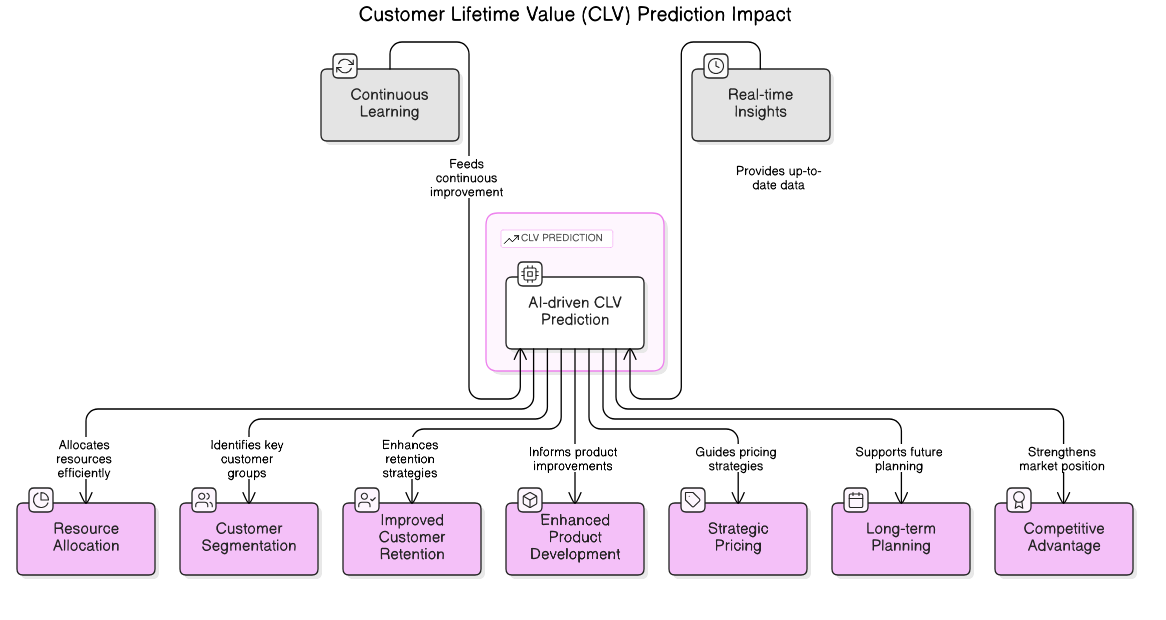
2.2. AI Technologies in CLV Prediction
Customer Lifetime Value (CLV) prediction is a crucial aspect of business strategy, allowing companies to understand the long-term value of their customers. At Rapid Innovation, we recognize that AI technologies for CLV prediction play a significant role in enhancing the accuracy and efficiency of CLV predictions. By leveraging advanced algorithms and models, businesses can make data-driven decisions that optimize customer relationships and maximize profitability. Our expertise in AI technologies enables us to analyze vast amounts of customer data, identify patterns and trends that traditional methods may overlook, and provide real-time insights, allowing for timely interventions.
2.2.1. Machine Learning Algorithms
Machine learning (ML) algorithms are a subset of AI that focuses on teaching computers to learn from data. In the context of CLV prediction, these algorithms can process historical customer data to forecast future behaviors and value.
- Regression Analysis: This technique helps in predicting continuous outcomes, such as the expected revenue from a customer over time. Linear regression is commonly used for its simplicity and interpretability.
- Classification Algorithms: These algorithms categorize customers based on their likelihood to churn or remain loyal. Techniques like logistic regression and decision trees are popular for this purpose.
- Clustering Techniques: Algorithms such as K-means clustering group customers based on similar characteristics, allowing businesses to tailor marketing strategies to different segments.
- Ensemble Methods: Techniques like Random Forest and Gradient Boosting combine multiple models to improve prediction accuracy. They reduce the risk of overfitting and enhance robustness.
- Survival Analysis: This method estimates the time until an event occurs, such as customer churn. It provides insights into customer retention and helps in developing strategies to prolong customer relationships.
Machine learning algorithms are particularly effective because they can adapt to new data, improving their predictions over time. They also allow for the integration of various data sources, such as transaction history, customer demographics, and engagement metrics. At Rapid Innovation, we implement these algorithms to help our clients achieve greater ROI by enhancing their customer engagement strategies.
2.2.2. Deep Learning Models
Deep learning, a more advanced subset of machine learning, utilizes neural networks with multiple layers to analyze complex data patterns. These models are particularly powerful for CLV prediction due to their ability to process unstructured data, such as text and images, alongside structured data.
- Neural Networks: These models mimic the human brain's structure and function, making them capable of identifying intricate patterns in large datasets. They are particularly useful for predicting CLV in industries with high variability in customer behavior.
- Recurrent Neural Networks (RNNs): RNNs are designed for sequential data, making them ideal for analyzing time-series data related to customer interactions. They can capture temporal dependencies, providing insights into how customer value evolves over time.
- Convolutional Neural Networks (CNNs): While primarily used in image processing, CNNs can also analyze customer data by identifying spatial hierarchies. They can be applied to customer reviews or social media content to gauge sentiment and predict CLV.
- Autoencoders: These unsupervised learning models are used for dimensionality reduction and feature extraction. They can help in identifying latent factors that influence customer value, leading to more accurate predictions.
- Transfer Learning: This technique allows models trained on one task to be adapted for another, reducing the need for extensive labeled data. It can be particularly beneficial in CLV prediction when historical data is limited.
Deep learning models excel in handling large datasets and can uncover hidden relationships that traditional models might miss. Their ability to learn from diverse data types enhances the precision of CLV predictions, enabling businesses to implement more effective customer retention strategies. Rapid Innovation leverages these advanced models to provide our clients with actionable insights that drive growth and improve their bottom line.
In conclusion, both machine learning algorithms and deep learning models are integral to the advancement of CLV prediction. By harnessing these AI technologies for CLV prediction, businesses can gain deeper insights into customer behavior, optimize marketing efforts, and ultimately drive growth. At Rapid Innovation, we are committed to helping our clients achieve their business goals efficiently and effectively through our tailored AI solutions.
Refer to the image below for a visual representation of AI technologies in CLV prediction.

2.2.3. Natural Language Processing
Natural Language Processing (NLP) is a crucial subfield of artificial intelligence that focuses on the interaction between computers and human language. It enables machines to understand, interpret, and generate human language in a valuable way. NLP combines linguistics, computer science, and machine learning to process and analyze large amounts of natural language data.
- Key components of NLP include: Â
- Tokenization: Breaking down text into smaller units, such as words or phrases.
- Part-of-Speech Tagging: Identifying the grammatical parts of speech in a sentence.
- Named Entity Recognition: Detecting and classifying key entities in text, such as names, dates, and locations.
- Sentiment Analysis: Determining the emotional tone behind a series of words, which is useful for understanding opinions and attitudes.
- Applications of NLP are vast and include: Â
- Chatbots and Virtual Assistants: Enhancing user interaction through natural conversation, which can lead to improved customer satisfaction and reduced operational costs.
- Text Analytics: Extracting insights from unstructured data, such as customer feedback, enabling businesses to make data-driven decisions that enhance product offerings and marketing strategies.
- Machine Translation: Automatically translating text from one language to another, improving global communication and expanding market reach.
NLP is increasingly important in various industries, including healthcare, finance, and marketing, as it helps organizations derive meaningful insights from textual data. The growth of NLP technologies is supported by advancements in deep learning and the availability of large datasets. At Rapid Innovation, we leverage natural language processing techniques to help clients streamline operations, enhance customer engagement, and ultimately achieve greater ROI.
2.3. Data Requirements and Sources
Data is the backbone of any analytical or predictive model. Understanding the data requirements and sources is essential for effective analysis and decision-making. The quality, quantity, and relevance of data significantly impact the outcomes of any project.
- Key data requirements include: Â
- Volume: Sufficient data size to ensure statistical significance and model robustness.
- Variety: Diverse data types (structured and unstructured) to capture different aspects of the problem.
- Velocity: The speed at which data is generated and processed, especially in real-time applications.
- Veracity: The accuracy and reliability of the data, which is crucial for building trust in the results.
- Common data sources include: Â
- Public Datasets: Open data repositories, such as government databases and research institutions, provide valuable datasets for analysis.
- Web Scraping: Extracting data from websites to gather real-time information on various topics.
- APIs: Application Programming Interfaces allow access to data from third-party services, such as social media platforms and financial markets.
- Surveys and Questionnaires: Collecting primary data directly from users or customers to gain insights into preferences and behaviors.
Organizations must ensure that they comply with data privacy regulations, such as GDPR, when collecting and using data. Proper data management practices are essential to maintain data integrity and security.
2.4. Prediction Methodologies
Prediction methodologies are techniques used to forecast future outcomes based on historical data. These methodologies leverage statistical models, machine learning algorithms, and data mining techniques to identify patterns and trends.
- Common prediction methodologies include: Â
- Regression Analysis: A statistical method used to model the relationship between a dependent variable and one or more independent variables. It helps in predicting continuous outcomes.
- Classification Algorithms: Techniques such as decision trees, support vector machines, and neural networks are used to categorize data into predefined classes. This is useful for tasks like spam detection and sentiment classification.
- Time Series Analysis: Analyzing data points collected or recorded at specific time intervals to identify trends, seasonal patterns, and cyclic behaviors. This is particularly useful in finance and economics.
- Ensemble Methods: Combining multiple models to improve prediction accuracy. Techniques like bagging and boosting help in reducing overfitting and enhancing model performance.
- Factors influencing the choice of prediction methodology include: Â
- Nature of the Data: The type of data (categorical, continuous, time-dependent) dictates the appropriate methodology.
- Problem Complexity: More complex problems may require advanced techniques, such as deep learning.
- Interpretability: Some applications require models that are easy to interpret, while others may prioritize accuracy over explainability.
Selecting the right prediction methodology is critical for achieving reliable and actionable insights. Continuous evaluation and refinement of models are necessary to adapt to changing data patterns and improve predictive performance. At Rapid Innovation, we assist clients in selecting and implementing the most effective methodologies, including natural language understanding and natural language generation, to maximize their return on investment.
3. Benefits of AI Agents in CLV Prediction
AI agents for CLV prediction are transforming the way businesses approach Customer Lifetime Value (CLV) prediction. By leveraging advanced algorithms and machine learning techniques, these agents provide significant advantages that can enhance business strategies and customer relationships.
3.1 Enhanced Prediction Accuracy
AI agents significantly improve the accuracy of CLV predictions through various methodologies:
- Data Analysis: AI can analyze vast amounts of data from multiple sources, including transaction history, customer behavior, and demographic information. This comprehensive analysis allows for more precise predictions.
- Machine Learning Models: By employing machine learning algorithms, AI agents can identify patterns and trends that may not be immediately apparent to human analysts. These models continuously learn from new data, refining their predictions over time.
- Segmentation: AI can segment customers into distinct groups based on their behaviors and preferences. This segmentation allows businesses to tailor their marketing strategies, leading to more accurate CLV predictions for each segment.
- Predictive Analytics: AI agents utilize predictive analytics to forecast future customer behavior based on historical data. This capability enables businesses to anticipate customer needs and adjust their strategies accordingly.
- Reduced Human Error: By automating the prediction process, AI minimizes the risk of human error, ensuring that the predictions are based on data-driven insights rather than subjective judgment.
The result is a more reliable and actionable understanding of customer value, which can lead to improved marketing strategies and increased profitability.
3.2 Real-time Customer Insights
AI agents for CLV prediction provide businesses with real-time insights into customer behavior, which is crucial for effective CLV prediction:
- Instant Data Processing: AI can process and analyze data in real-time, allowing businesses to respond quickly to changes in customer behavior. This immediacy helps in adjusting marketing strategies on the fly.
- Behavior Tracking: AI agents can track customer interactions across various channels, including social media, email, and websites. This tracking provides a holistic view of customer engagement, enabling businesses to understand what drives customer loyalty.
- Sentiment Analysis: By analyzing customer feedback and social media interactions, AI can gauge customer sentiment. Understanding how customers feel about a brand or product can significantly influence CLV predictions.
- Personalization: Real-time insights allow businesses to personalize their offerings and communications. Tailored experiences can enhance customer satisfaction and loyalty, ultimately increasing CLV.
- Proactive Engagement: With real-time insights, businesses can proactively engage with customers. For instance, if a customer shows signs of disengagement, targeted interventions can be implemented to retain them.
These real-time insights empower businesses to make informed decisions, enhancing their ability to predict and maximize customer lifetime value effectively. At Rapid Innovation, we harness these AI agents for CLV prediction to help our clients achieve greater ROI by optimizing their customer engagement strategies and driving sustainable growth. For more information on how AI predicts customer trends and behavior.
3.3. Automated Data Processing
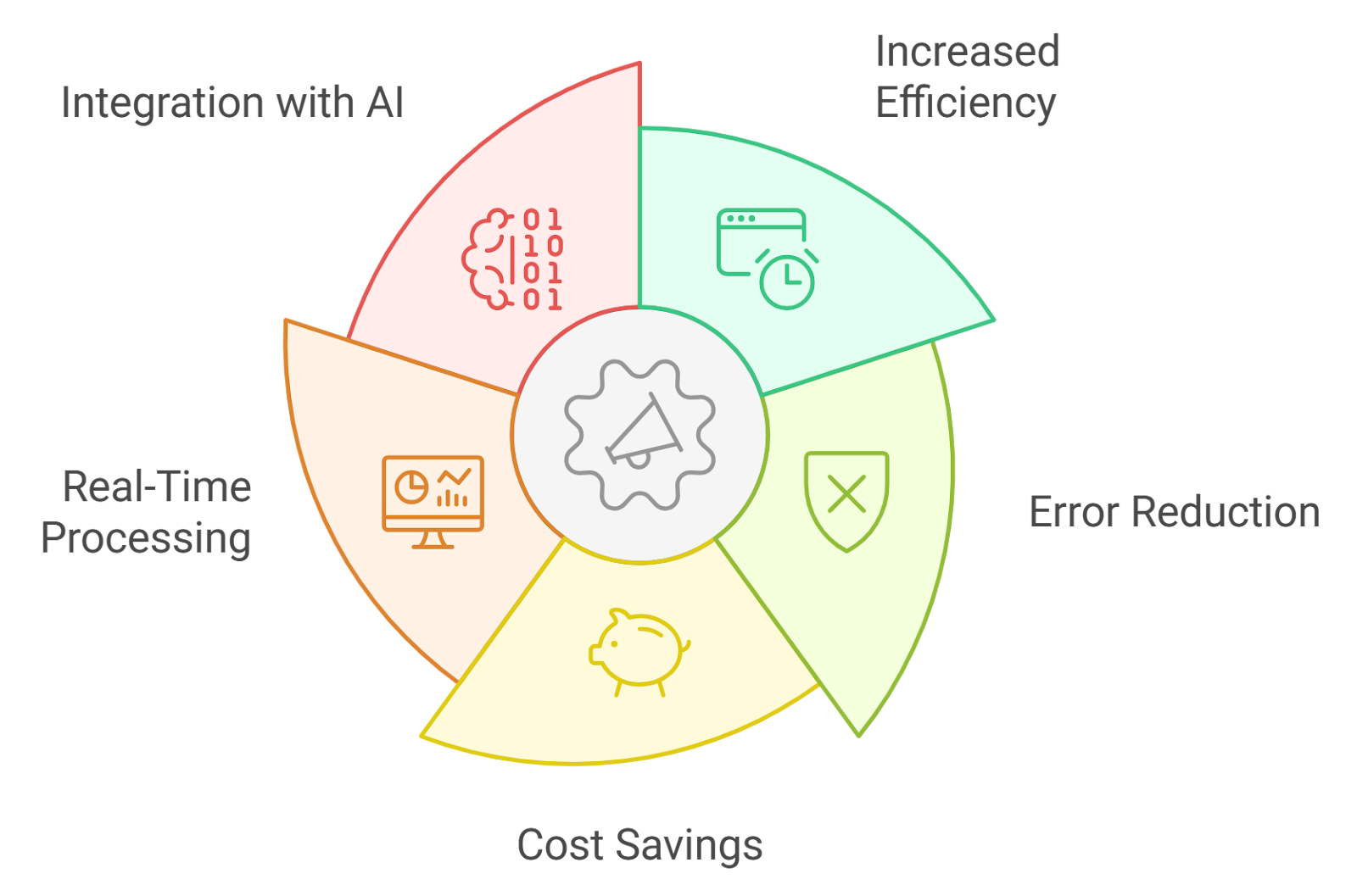
‍
Automated data processing refers to the use of technology to collect, process, and analyze data without human intervention. This approach streamlines operations, reduces errors, and enhances efficiency, ultimately contributing to greater ROI for businesses.
- Increased Efficiency: Automation speeds up data handling, allowing organizations to process large volumes of information quickly. Rapid Innovation implements tailored automated data processing tools that enable clients to focus on strategic initiatives rather than manual data tasks.
- Error Reduction: Automated systems minimize human errors, ensuring more accurate data processing. Our expertise in AI-driven automation ensures that clients experience fewer discrepancies, leading to more reliable outcomes.
- Cost Savings: By reducing the need for manual labor, businesses can save on operational costs. Rapid Innovation helps clients optimize their resources, translating to significant cost reductions and improved profitability through automated data processing software.
- Real-Time Processing: Automated systems can analyze data in real-time, providing immediate insights that can drive decision-making. We empower organizations with real-time analytics, enabling them to respond swiftly to market changes using intelligent document processing.
- Integration with AI: Many automated data processing systems leverage artificial intelligence to improve data accuracy and predictive capabilities. Rapid Innovation integrates AI into automated processes, enhancing decision-making and strategic planning, including automated sales order processing. For more insights, you can learn from real-world AI implementations.
3.4. Scalable Analysis Capabilities
Scalable analysis capabilities refer to the ability of a system to handle increasing amounts of data and complexity without compromising performance. This is crucial for businesses that anticipate growth or fluctuating data demands.
- Flexibility: Scalable systems can adapt to varying data loads, ensuring consistent performance regardless of volume. Rapid Innovation designs scalable solutions that grow alongside our clients' businesses, ensuring they remain agile.
- Cost-Effectiveness: Organizations can invest in scalable solutions that grow with their needs, avoiding the costs associated with frequent upgrades. Our approach allows clients to allocate budgets more effectively, maximizing their return on investment.
- Enhanced Performance: Scalable analysis tools can process data faster as they grow, maintaining efficiency even with larger datasets. We ensure that our clients' systems are equipped to handle increased data demands without sacrificing performance, utilizing automated data capture and automatic data capture techniques.
- Cloud Solutions: Many scalable analysis capabilities are offered through cloud platforms, allowing for easy expansion and access to advanced analytics tools. Rapid Innovation leverages cloud technology to provide clients with flexible and powerful analytical capabilities, including data extraction software.
- Future-Proofing: Investing in scalable systems prepares businesses for future data challenges, ensuring they remain competitive. Our forward-thinking solutions help clients stay ahead of industry trends and technological advancements, including the use of ETL automation and auto ETL processes.
3.5. Personalization Opportunities
Personalization opportunities involve tailoring products, services, and experiences to meet the individual preferences and needs of customers. This approach enhances customer satisfaction and loyalty, driving higher ROI.
- Improved Customer Experience: Personalization creates a more engaging experience, making customers feel valued and understood. Rapid Innovation helps clients implement personalized strategies that resonate with their target audience.
- Data-Driven Insights: By analyzing customer data, businesses can identify trends and preferences, allowing for targeted marketing strategies. Our expertise in data analytics enables clients to make informed decisions that enhance customer engagement.
- Increased Conversion Rates: Personalized recommendations can lead to higher sales, as customers are more likely to purchase products that align with their interests. We assist clients in developing tailored marketing campaigns that boost conversion rates.
- Enhanced Customer Retention: When customers receive personalized experiences, they are more likely to return, fostering long-term relationships. Rapid Innovation's solutions focus on building loyalty through personalized interactions.
- Use of AI and Machine Learning: Advanced technologies can analyze vast amounts of data to deliver personalized content and recommendations in real-time. Our integration of AI and machine learning ensures that clients can provide relevant experiences that drive customer satisfaction and retention, supported by automated data processing equipment and automatic data processing equipment.
3.6. Proactive Customer Retention
Proactive customer retention is a strategic approach that focuses on keeping customers engaged and satisfied before they consider leaving. This method emphasizes understanding customer needs and addressing potential issues before they escalate.
- Identify at-risk customers: Leverage advanced data analytics and AI algorithms to pinpoint customers who may be disengaging. By analyzing patterns such as decreased purchase frequency or negative feedback, businesses can take timely action.
- Personalized communication: Utilize AI-driven insights to tailor messages based on customer behavior and preferences. Personalized emails or offers can significantly enhance customer loyalty and engagement.
- Regular feedback loops: Implement surveys and feedback mechanisms powered by AI to gauge customer satisfaction. This allows businesses to address concerns promptly and adapt their strategies accordingly.
- Loyalty programs: Develop blockchain-based loyalty programs that reward repeat customers. Incentives such as discounts, exclusive access, or points systems can encourage continued patronage while ensuring transparency and security. For more insights on AI-driven loyalty programs, visit this link.
- Customer education: Provide resources that help customers maximize the value of your product or service. This can include tutorials, webinars, or FAQs, supported by AI to deliver personalized learning experiences.
- Community engagement: Foster a sense of community among customers through forums or social media groups. Engaged customers are less likely to leave, and blockchain can enhance trust and security in these interactions.
- Predictive analytics: Utilize predictive modeling and machine learning to forecast customer behavior and tailor retention strategies accordingly. This can help in anticipating churn and taking preemptive actions.
By implementing these strategies, businesses can create a proactive customer retention plan that not only reduces churn but also enhances overall customer satisfaction. This includes the use of customer retention strategies, client retention strategies, and retention marketing tactics to increase customer retention and improve customer retention in ecommerce.
4. Technical Architecture
Technical architecture refers to the structure and organization of technology systems that support business operations. It encompasses hardware, software, networks, and data management systems. A well-designed technical architecture is crucial for ensuring efficiency, scalability, and security.
- Scalability: The architecture should support growth, allowing for easy integration of new technologies and systems as the business expands.
- Flexibility: A flexible architecture can adapt to changing business needs and market conditions, ensuring long-term viability.
- Security: Implement robust security measures to protect sensitive data and maintain customer trust. This includes encryption, firewalls, and regular security audits.
- Integration: Ensure that different systems and applications can communicate effectively. This can be achieved through APIs and middleware solutions.
- Performance: Optimize the architecture for speed and efficiency. This includes selecting the right hardware and software solutions to meet performance requirements.
- Documentation: Maintain comprehensive documentation of the architecture to facilitate troubleshooting and onboarding of new team members.
A well-structured technical architecture lays the foundation for successful business operations and enhances the ability to respond to market demands.
4.1. Data Collection Systems
Data collection systems are essential for gathering, storing, and analyzing data to inform business decisions. These systems can vary in complexity and functionality, depending on the organization's needs.
- Types of data: Identify the types of data needed, such as customer demographics, purchase history, and engagement metrics. This will guide the design of the data collection system.
- Data sources: Utilize multiple sources for data collection, including: Â
- Customer relationship management (CRM) systems
- E-commerce platforms
- Social media analytics
- Data storage: Choose appropriate storage solutions, such as cloud-based systems or on-premises databases, to ensure data is easily accessible and secure.
- Data quality: Implement processes to ensure data accuracy and consistency. Regular audits and validation checks can help maintain high data quality.
- Real-time analytics: Incorporate tools that allow for real-time data analysis. This enables businesses to make informed decisions quickly and respond to trends as they arise.
- Compliance: Ensure that data collection practices comply with relevant regulations, such as GDPR or CCPA. This includes obtaining consent and providing transparency about data usage.
- User-friendly interfaces: Design intuitive interfaces for data entry and retrieval, making it easier for employees to use the system effectively.
By establishing robust data collection systems, organizations can harness the power of data to drive strategic decisions and improve overall performance, ultimately enhancing customer retention management strategies and client retention programs.
4.2. Processing Pipeline Design
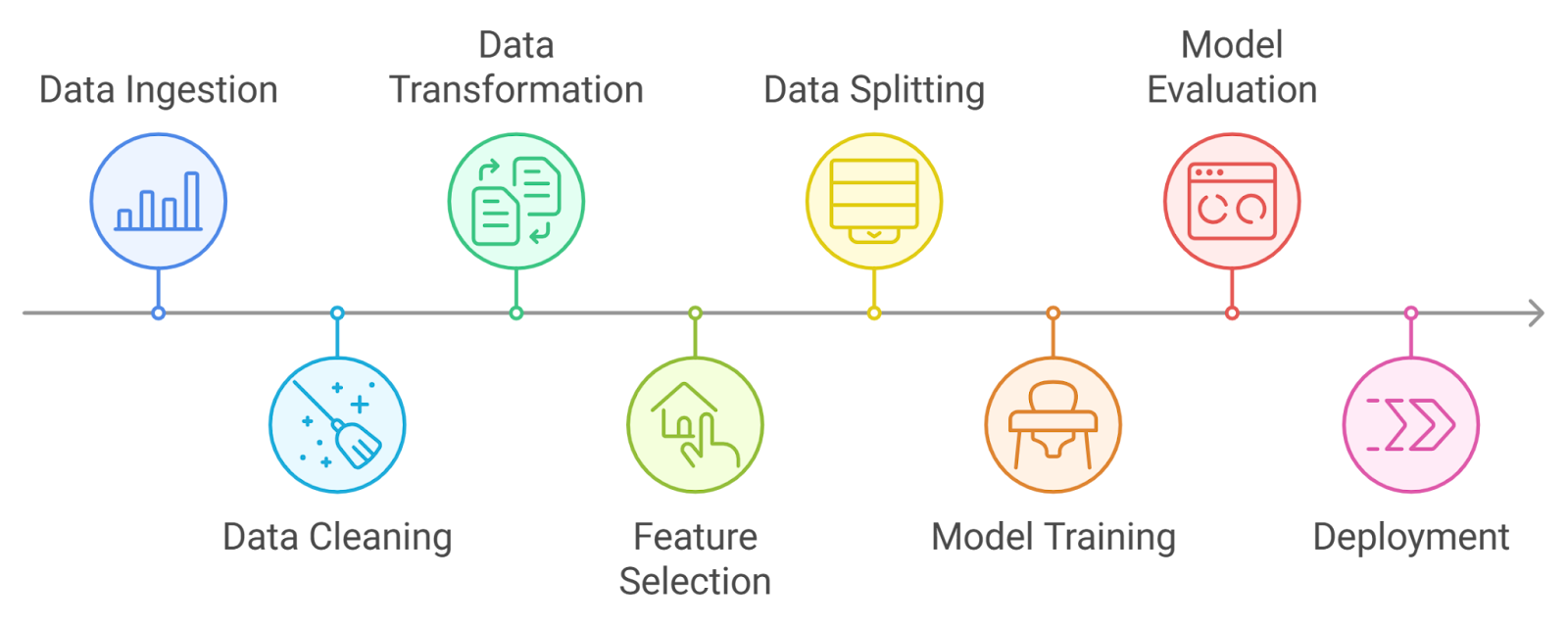
‍
A well-structured processing pipeline is crucial for the success of any machine learning project, including scikit learn pipeline, machine learning pipeline, and ml pipeline. It ensures that data flows smoothly from raw input to model training and evaluation. The design of a processing pipeline typically involves several key components:
- Data Ingestion: This is the first step where data is collected from various sources, including databases, APIs, or flat files. The ingestion process should be automated to handle real-time data efficiently, allowing businesses to make timely decisions based on the latest information. This is particularly important in contexts like sagemaker pipelines and azure ml pipeline.
- Data Cleaning: Raw data often contains noise, missing values, or inconsistencies. Data cleaning involves removing duplicates, filling in missing values, and correcting errors to ensure high-quality input for the model. This step is essential for maximizing the return on investment (ROI) by ensuring that the model is trained on reliable data, which is a key aspect of mlops pipeline.
- Data Transformation: This step involves converting data into a suitable format for analysis. Techniques such as normalization, standardization, and encoding categorical variables are commonly used to prepare data for machine learning algorithms. Proper transformation can enhance model performance, leading to better business outcomes, especially in machine learning data pipeline and data pipeline for machine learning.
- Feature Selection: Not all features contribute equally to model performance. Feature selection techniques help identify the most relevant features, reducing dimensionality and improving model efficiency. By focusing on impactful features, businesses can achieve greater ROI through more accurate predictions, which is crucial in building machine learning pipelines.
- Data Splitting: The dataset is typically divided into training, validation, and test sets. This ensures that the model can be trained on one subset of data while being evaluated on another, preventing overfitting and ensuring robust performance in real-world applications, a common practice in machine learning pipeline example.
- Model Training: Once the data is prepared, it is fed into the machine learning model for training. This step involves selecting the appropriate algorithm and tuning hyperparameters to optimize performance. Rapid Innovation leverages advanced algorithms to ensure that clients receive the best possible model for their specific needs, including deep learning pipeline and ml model pipeline.
- Model Evaluation: After training, the model is evaluated using metrics such as accuracy, precision, recall, and F1 score. This helps determine how well the model performs on unseen data, providing insights into its effectiveness and reliability, which is essential in ci cd machine learning and mlops ci cd.
- Deployment: Finally, the trained model is deployed into a production environment where it can make predictions on new data. Continuous monitoring is essential to ensure the model remains effective over time, allowing businesses to adapt to changing conditions and maintain a competitive edge, as seen in amazon sagemaker pipeline and databricks ml pipeline.
4.3. Model Architecture
Model architecture refers to the structure and design of a machine learning model. It encompasses the arrangement of layers, types of neurons, and the connections between them. The choice of model architecture significantly impacts the performance and efficiency of the model. Key considerations include:
- Type of Model: Different tasks require different types of models. For instance, convolutional neural networks (CNNs) are ideal for image processing, while recurrent neural networks (RNNs) are suited for sequential data like time series or natural language. Rapid Innovation helps clients select the most appropriate model type based on their specific use cases, including spark ml pipeline and spark machine learning pipeline.
- Layer Configuration: The number of layers and the type of each layer (e.g., convolutional, pooling, fully connected) play a critical role in how the model learns from data. Deeper architectures can capture more complex patterns but may also lead to overfitting. Our expertise ensures that clients benefit from optimal layer configurations tailored to their data, which is vital in mlops pipeline example.
- Activation Functions: Activation functions determine how the output of a neuron is calculated. Common choices include ReLU, sigmoid, and softmax. The choice of activation function can influence the model's ability to learn non-linear relationships, impacting overall performance.
- Regularization Techniques: To prevent overfitting, regularization techniques such as dropout, L1/L2 regularization, and batch normalization can be employed. These techniques help improve the model's generalization to new data, ensuring that clients achieve reliable results.
- Optimization Algorithms: The choice of optimization algorithm (e.g., Adam, SGD, RMSprop) affects how the model learns during training. Different algorithms have varying convergence rates and can impact the final performance of the model. Rapid Innovation utilizes state-of-the-art optimization techniques to enhance model training efficiency.
4.3.1. Feature Engineering
Feature engineering is the process of using domain knowledge to create new features or modify existing ones to improve model performance. It is a critical step in the machine learning pipeline and can significantly influence the outcome of the model. Key aspects of feature engineering include:
- Creating New Features: New features can be derived from existing data. For example, combining date and time into a single timestamp or extracting the day of the week from a date can provide additional insights that enhance model predictions.
- Transforming Features: Transformations such as logarithmic scaling or polynomial features can help in capturing non-linear relationships. These transformations can make the data more suitable for certain algorithms, leading to improved model performance.
- Encoding Categorical Variables: Categorical variables need to be converted into numerical formats for machine learning models. Techniques like one-hot encoding or label encoding are commonly used to achieve this, ensuring that the model can effectively process all types of data.
- Handling Missing Values: Missing data can skew results. Techniques such as imputation (filling in missing values with mean, median, or mode) or creating a separate category for missing values can be effective in maintaining data integrity.
- Feature Scaling: Normalizing or standardizing features ensures that they are on a similar scale, which is particularly important for algorithms sensitive to the scale of input data, such as k-nearest neighbors or gradient descent-based methods.
- Feature Selection: After creating and transforming features, it’s essential to evaluate their importance. Techniques like recursive feature elimination, feature importance from tree-based models, or statistical tests can help identify the most impactful features.
Effective feature engineering can lead to improved model accuracy and performance, making it a vital component of the machine learning process. Rapid Innovation's expertise in feature engineering ensures that clients can leverage their data to achieve optimal results and maximize ROI, particularly in contexts like ci cd for machine learning and ml pipeline spark.
4.3.2. Model Selection
Model selection is a critical step in the machine learning process, as it determines the algorithm that will be used to analyze data and make predictions. The choice of model can significantly impact the performance and accuracy of the results.
- Understand the problem type: Classification, regression, clustering, or reinforcement learning.
- Consider the data characteristics: Â
- Size of the dataset.
- Dimensionality and feature types (categorical, numerical).
- Evaluate model complexity: Â
- Simpler models (like linear regression) are easier to interpret but may underfit.
- Complex models (like deep learning) can capture intricate patterns but may overfit.
- Use cross-validation: Helps in assessing how the model generalizes to an independent dataset.
- Compare performance metrics: Â
- Accuracy, precision, recall, F1 score, and AUC-ROC for classification tasks.
- Mean squared error (MSE) or R-squared for regression tasks.
- Leverage domain knowledge: Incorporate insights from the specific field to guide model selection.
At Rapid Innovation, we assist clients in navigating this model selection process by providing tailored recommendations based on their unique business challenges and data characteristics. For instance, a retail client seeking to optimize inventory management may benefit from a regression model, while a financial services client might require a classification model to detect fraudulent transactions. Our expertise in model selection in machine learning ensures that clients select the most effective model to achieve their desired outcomes, ultimately leading to greater ROI. We also emphasize the importance of model evaluation and selection in machine learning to ensure optimal performance.
4.3.3. Training Procedures
Training procedures are essential for teaching the selected model to recognize patterns in the data. This phase involves several steps to ensure the model learns effectively.
- Data preprocessing: Â
- Clean the data by handling missing values and outliers.
- Normalize or standardize features to ensure uniformity.
- Split the dataset: Divide the data into training, validation, and test sets to evaluate model performance.
- Choose an optimization algorithm: Common choices include stochastic gradient descent (SGD), Adam, and RMSprop.
- Set hyperparameters: Determine values for learning rate, batch size, and number of epochs.
- Monitor training: Â
- Use techniques like early stopping to prevent overfitting.
- Track loss and accuracy metrics during training.
- Evaluate the model: Â
- After training, assess the model on the validation set to fine-tune hyperparameters.
- Finally, test the model on the test set to gauge its performance on unseen data.
At Rapid Innovation, we implement robust training procedures that ensure our clients' models are not only accurate but also resilient to overfitting. For example, in a project for a healthcare provider, we utilized advanced monitoring techniques to ensure the model maintained high accuracy while adapting to new patient data, thereby enhancing decision-making processes and improving patient outcomes.
4.4. Integration Points
Integration points refer to the stages where the machine learning model interacts with other systems or components. Proper integration is crucial for deploying the model effectively in real-world applications.
- Data ingestion: Ensure seamless data flow from various sources into the model for real-time predictions.
- API development: Create application programming interfaces (APIs) to allow other applications to access the model's predictions.
- User interface: Design intuitive interfaces for end-users to interact with the model outputs.
- Monitoring and maintenance: Implement systems to monitor model performance over time and retrain as necessary.
- Feedback loops: Establish mechanisms for users to provide feedback, which can be used to improve the model.
- Security and compliance: Ensure that data handling complies with regulations like GDPR and that the model is secure from vulnerabilities.
Rapid Innovation excels in establishing these integration points, ensuring that our clients can leverage their machine learning models effectively. For instance, we developed a comprehensive API for a logistics company that allowed real-time tracking of shipments, significantly enhancing operational efficiency and customer satisfaction. Our commitment to security and compliance further ensures that our clients can trust their data handling processes, thereby maximizing their return on investment. Additionally, we provide insights on choosing a machine learning model and automl model selection to streamline the process further.
4.5. Deployment Strategies
Deployment strategies are crucial for ensuring that software applications are released efficiently and effectively. The choice of deployment strategy can significantly impact the performance, reliability, and user experience of the application. Here are some common deployment strategies:
- Blue-Green Deployment: This strategy involves maintaining two identical environments, one active (blue) and one idle (green). When a new version of the application is ready, it is deployed to the green environment. Once testing is complete, traffic is switched from the blue to the green environment, minimizing downtime and risk.
- Canary Releases: In this approach, a new version of the application is rolled out to a small subset of users before a full-scale deployment. This allows developers to monitor the performance and gather feedback, ensuring that any issues can be addressed before the wider release.
- Rolling Deployment: This strategy involves gradually replacing instances of the previous version of the application with the new version. This method allows for continuous availability and reduces the risk of a complete failure, as only a portion of the application is updated at any given time.
- Recreate Deployment: In this strategy, the existing application is completely shut down before the new version is deployed. While this method is straightforward, it can lead to downtime, making it less favorable for applications requiring high availability.
- Shadow Deployment: This involves deploying the new version alongside the existing version without affecting the user experience. The new version processes real user requests, but the results are not visible to users. This allows for performance testing in a live environment.
Choosing the right deployment strategy depends on various factors, including application architecture, user base, and business requirements. Each strategy has its pros and cons, and understanding these can help teams make informed decisions. Additionally, organizations may consider various deployment methodologies, such as lean deployment strategy or hoshin strategy deployment, to align their deployment efforts with broader business goals.
5. Implementation Challenges
Implementing a new software application or system can present numerous challenges that organizations must navigate. These challenges can affect timelines, budgets, and overall project success. Here are some common implementation challenges:
- Resistance to Change: Employees may resist adopting new systems or processes, fearing disruption to their routines. Effective change management strategies, including training and communication, are essential to mitigate this resistance.
- Integration Issues: New applications often need to integrate with existing systems. Compatibility issues can arise, leading to delays and increased costs. Thorough planning and testing are crucial to ensure seamless integration.
- Resource Constraints: Limited resources, including time, budget, and personnel, can hinder implementation efforts. Organizations must allocate sufficient resources and prioritize tasks to ensure successful deployment.
- Lack of Clear Requirements: Ambiguous or poorly defined project requirements can lead to scope creep and misalignment between stakeholders. Engaging stakeholders early and often can help clarify expectations and requirements.
- Data Migration Challenges: Moving data from legacy systems to new applications can be complex. Data quality issues, such as inconsistencies and inaccuracies, can complicate this process, requiring careful planning and execution.
5.1. Data Quality Issues
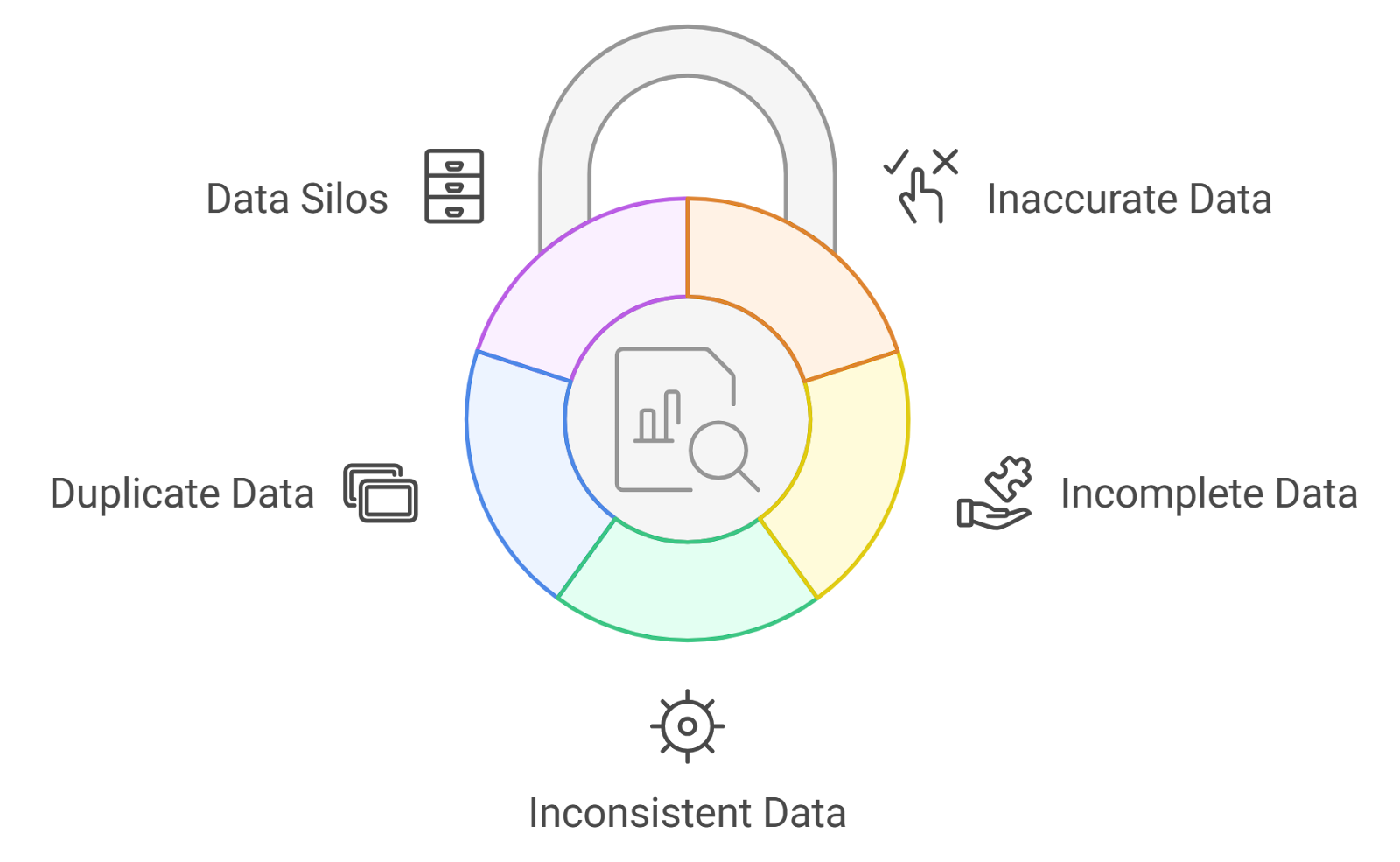
‍
Data quality is a critical aspect of any implementation project. Poor data quality can lead to inaccurate insights, ineffective decision-making, and ultimately, project failure. Here are some common data quality issues organizations face:
- Inaccurate Data: Data may be incorrect due to human error, outdated information, or system glitches. Regular audits and validation processes can help identify and rectify inaccuracies.
- Incomplete Data: Missing data can hinder analysis and decision-making. Organizations should establish protocols for data entry and ensure that all necessary fields are populated.
- Inconsistent Data: Data may be stored in different formats or structures across systems, leading to inconsistencies. Standardizing data formats and implementing data governance practices can help maintain consistency.
- Duplicate Data: Duplicate records can inflate data volumes and lead to confusion. Implementing deduplication processes and maintaining a single source of truth can help mitigate this issue.
- Data Silos: When data is isolated within departments or systems, it can limit visibility and collaboration. Encouraging cross-departmental data sharing and integration can help break down silos.
Addressing data quality issues is essential for successful implementation. Organizations should prioritize data management practices, including regular data cleansing, validation, and governance, to ensure high-quality data throughout the project lifecycle.
At Rapid Innovation, we leverage our expertise in AI and Blockchain to enhance deployment strategies, including application deployment strategies and strategy deployment software, and address implementation challenges effectively. By utilizing advanced analytics and smart contracts, we help clients streamline their processes, ensuring a higher return on investment (ROI) and improved operational efficiency. Our tailored solutions empower organizations to navigate the complexities of deployment and implementation, ultimately driving business success.
5.1.1. Data Completeness
Data completeness refers to the extent to which all required data is present and accounted for in a dataset. It is a critical aspect of data quality management, as incomplete data can lead to incorrect conclusions and poor decision-making. Ensuring data completeness involves several key practices:
- Identify required data fields: Clearly define which data points are necessary for analysis and decision-making. At Rapid Innovation, we assist clients in identifying these critical data fields, ensuring that they align with their business objectives.
- Regular audits: Conduct periodic checks to ensure that all required data is being collected and stored. Our team can implement automated auditing processes that leverage AI to enhance efficiency and accuracy.
- Data entry validation: Implement validation rules during data entry to prevent incomplete records from being saved. We can develop custom validation solutions that integrate seamlessly with existing systems.
- Use of default values: In cases where data may be missing, consider using default values to maintain dataset integrity. Our solutions can intelligently suggest default values based on historical data patterns.
- Monitor data sources: Keep track of data sources to ensure they are consistently providing complete information. Rapid Innovation employs blockchain technology to create immutable records of data sources, enhancing trust and reliability. For more insights on the importance of data quality in AI implementations, check out the critical role of data quality in AI implementations.
5.1.2. Data Accuracy
Data accuracy is the degree to which data correctly reflects the real-world entities or events it represents. Accurate data is essential for reliable analysis and informed decision-making. To enhance data accuracy, organizations can adopt the following strategies:
- Implement data validation checks: Use automated tools to verify data against predefined criteria or external sources. Our AI-driven validation tools can significantly reduce human error and improve data quality management.
- Regular updates: Ensure that data is regularly updated to reflect the most current information. We can help automate data refresh processes, ensuring that clients always work with the latest data.
- Cross-reference data: Compare data from multiple sources to identify discrepancies and correct inaccuracies. Our solutions can facilitate real-time cross-referencing, providing clients with a comprehensive view of their data landscape.
- Train staff: Provide training for employees on the importance of data accuracy and best practices for data entry. Rapid Innovation offers tailored training programs that empower teams to maintain high data quality standards.
- Use technology: Leverage advanced technologies like machine learning and artificial intelligence to identify and rectify inaccuracies in large datasets. Our expertise in AI allows us to develop sophisticated algorithms that enhance data accuracy.
5.1.3. Data Consistency
Data consistency refers to the uniformity of data across different datasets and systems. Inconsistent data can lead to confusion and misinterpretation, making it crucial for organizations to maintain consistency. Here are some effective methods to ensure data consistency:
- Standardize data formats: Establish standard formats for data entry, such as date formats or naming conventions, to ensure uniformity. We can assist in creating standardized templates that streamline data entry processes.
- Centralized data management: Use a centralized database to store data, reducing the chances of discrepancies across different systems. Our blockchain solutions can provide a single source of truth, enhancing data integrity.
- Regular synchronization: Ensure that data is regularly synchronized between systems to maintain consistency. Rapid Innovation can implement automated synchronization processes that keep all systems aligned.
- Data governance policies: Develop and enforce data governance policies that outline how data should be managed and maintained. We work with clients to establish robust governance frameworks that promote data consistency and data quality governance.
- Continuous monitoring: Implement monitoring tools to detect and address inconsistencies in real-time, ensuring data remains reliable and trustworthy. Our AI-powered monitoring solutions provide proactive alerts, enabling clients to address issues before they escalate.
5.2. Technical Challenges
In the realm of technology, various challenges can arise that hinder the smooth operation and implementation of systems. Understanding these technical challenges is crucial for organizations aiming to optimize their processes and enhance their technological infrastructure. Two significant technical challenges include system integration and scalability issues.
5.2.1. System Integration
System integration refers to the process of linking different computing systems and software applications physically or functionally to act as a coordinated whole. This is essential for organizations that rely on multiple systems to manage their operations. However, several challenges can arise during this process, including system integration challenges, sap integration challenges, and salesforce integration challenges:
- Compatibility: Different systems may use various protocols, data formats, and architectures, making it difficult to achieve seamless integration. Organizations often face issues when trying to connect legacy systems with modern applications, leading to system integration problems.
- Data Silos: When systems are not integrated, data can become siloed, leading to inefficiencies. Employees may struggle to access the information they need, resulting in delays and miscommunication. This is particularly evident in challenges of system integration.
- Complexity: The integration process can be complex, requiring specialized knowledge and skills. Organizations may need to invest in training or hire external experts, which can increase costs. This complexity is often highlighted in challenges in system integration.
- Maintenance: Once systems are integrated, ongoing maintenance is necessary to ensure they continue to function correctly. This can be resource-intensive and may require regular updates and monitoring, especially during salesforce data migration challenges.
- Security Risks: Integrating multiple systems can expose organizations to security vulnerabilities. Ensuring that data remains secure during the integration process is critical, as breaches can lead to significant financial and reputational damage.
At Rapid Innovation, we leverage our expertise in AI and Blockchain to facilitate seamless system integration. By utilizing AI-driven solutions, we can automate data synchronization and enhance compatibility between disparate systems. Our Blockchain technology ensures secure and transparent data sharing, effectively mitigating security risks and eliminating data silos. We also address specific challenges such as sap pi to po migration challenges and provide system integration challenges and solutions.
5.2.2. Scalability Issues
Scalability refers to the capability of a system to handle a growing amount of work or its potential to accommodate growth. As businesses expand, their technological infrastructure must also evolve. However, scalability issues can pose significant challenges:
- Resource Limitations: Many systems are designed with a specific capacity in mind. As demand increases, organizations may find that their existing resources—such as servers, storage, and bandwidth—are insufficient to meet new requirements.
- Performance Degradation: When systems are not scalable, performance can degrade as more users or processes are added. This can lead to slower response times and a poor user experience, ultimately affecting productivity.
- Cost Implications: Scaling a system often requires additional investments in hardware, software, and personnel. Organizations must carefully evaluate the cost-benefit ratio of scaling versus maintaining existing systems.
- Architectural Constraints: Some systems may have architectural limitations that hinder their ability to scale effectively. Organizations may need to redesign or replace these systems, which can be time-consuming and costly.
- Future-Proofing: As technology evolves, organizations must consider future scalability needs. Failing to plan for growth can result in systems that quickly become outdated or inadequate, necessitating further investments in the future.
At Rapid Innovation, we address scalability challenges by designing flexible and adaptive systems that can grow alongside your business. Our AI solutions enable predictive analytics to forecast resource needs, while our Blockchain infrastructure supports decentralized scaling, ensuring that your systems remain robust and efficient as demand increases.
Addressing these technical challenges requires a strategic approach, including thorough planning, investment in the right technologies, and ongoing evaluation of systems to ensure they meet the evolving needs of the organization. Rapid Innovation is committed to partnering with you to navigate these challenges and achieve greater ROI through innovative solutions, including addressing the challenges and issues of system integration.
5.2.3. Performance Optimization
Performance optimization is crucial for enhancing the efficiency and effectiveness of systems, applications, and processes. It involves various performance optimization strategies and techniques aimed at improving speed, responsiveness, and resource utilization. Key strategies include:
- Identify bottlenecks: Analyze system performance to pinpoint areas that slow down operations, which can include database queries, network latency, or inefficient algorithms. Rapid Innovation employs advanced analytics to identify these bottlenecks, enabling clients to streamline their operations and enhance overall performance.
- Implement caching: Use caching mechanisms to store frequently accessed data, reducing the need for repeated calculations or database calls. This can significantly speed up response times. Our team can design and implement tailored caching solutions that align with your specific application needs, resulting in improved user experiences and reduced operational costs.
- Optimize code: Review and refactor code to eliminate redundancies and improve execution speed. This may involve using more efficient algorithms or data structures. Rapid Innovation's experts leverage best practices in software development to ensure that your applications run efficiently, ultimately leading to higher ROI.
- Load balancing: Distribute workloads evenly across servers to prevent any single server from becoming a bottleneck, ensuring that resources are utilized effectively. Our solutions include intelligent load balancing strategies that adapt to real-time traffic, ensuring optimal performance and resource allocation.
- Monitor performance: Use monitoring tools to continuously track system performance, allowing for proactive identification of issues before they impact users. Rapid Innovation provides comprehensive monitoring solutions that empower organizations to maintain peak performance and quickly address potential issues.
- Scale resources: Adjust server capacity based on demand, which can involve vertical scaling (adding more power to existing servers) or horizontal scaling (adding more servers). Our cloud-based solutions enable seamless scaling, ensuring that your infrastructure can handle fluctuations in demand without compromising performance.
- Use content delivery networks (CDNs): CDNs can help deliver content more quickly to users by caching it at various locations around the globe, thereby reducing latency. Rapid Innovation can integrate CDN solutions that enhance content delivery speed, improving user satisfaction and engagement.
5.3. Organizational Challenges
Organizations often face various challenges that can hinder their performance and growth. These challenges can stem from internal processes, culture, or external market conditions. Some common challenges include:
- Resistance to change: Employees may resist new processes or technologies, leading to slow adoption and inefficiencies. Rapid Innovation assists organizations in change management, ensuring smooth transitions and fostering a culture of innovation.
- Communication barriers: Poor communication can result in misunderstandings and misalignment of goals across departments. Our consulting services focus on enhancing communication strategies, ensuring that all stakeholders are aligned and informed.
- Resource constraints: Limited budgets or personnel can restrict an organization’s ability to implement necessary changes or improvements. Rapid Innovation offers scalable solutions that maximize resource utilization, allowing organizations to achieve more with less.
- Lack of strategic vision: Without a clear direction, organizations may struggle to prioritize initiatives and allocate resources effectively. Our strategic consulting services help organizations define their vision and align their initiatives with business goals.
- Cultural issues: An organization's culture can impact employee engagement and productivity. A negative culture can lead to high turnover rates and low morale. Rapid Innovation works with organizations to cultivate a positive culture that promotes collaboration and innovation.
- Compliance and regulatory challenges: Organizations must navigate complex regulations, which can be time-consuming and costly. Our expertise in compliance solutions ensures that organizations can meet regulatory requirements efficiently, reducing the risk of penalties and enhancing operational integrity.
5.3.1. Skill Gap
The skill gap refers to the disparity between the skills required for a job and the actual skills possessed by employees. This gap can significantly impact an organization’s performance and competitiveness. Factors contributing to the skill gap include:
- Rapid technological advancements: As technology evolves, employees may lack the necessary skills to keep up with new tools and processes. Rapid Innovation provides training and development programs tailored to emerging technologies, ensuring that your workforce remains competitive.
- Insufficient training programs: Organizations may not invest enough in training and development, leaving employees unprepared for their roles. Our comprehensive training solutions equip employees with the skills they need to excel in their positions.
- Changing job requirements: Job roles are constantly evolving, and employees may not have the opportunity to acquire new skills that align with these changes. Rapid Innovation helps organizations identify skill gaps and implement targeted training initiatives to address them.
- Recruitment challenges: Finding candidates with the right skills can be difficult, leading to unfilled positions and increased workloads for existing staff. Our recruitment services focus on sourcing top talent with the necessary skills to drive your organization forward.
- Impact on productivity: A significant skill gap can lead to decreased productivity, as employees struggle to perform tasks effectively. By addressing skill gaps, Rapid Innovation helps organizations enhance productivity and achieve their business objectives.
- Employee morale: When employees feel unprepared or unsupported, it can lead to frustration and decreased job satisfaction. Our focus on employee development fosters a supportive environment that boosts morale and retention.
Addressing the skill gap is essential for organizations to remain competitive and ensure that their workforce is equipped to meet current and future challenges. Rapid Innovation is committed to providing the necessary tools and expertise to help organizations bridge this gap and achieve their strategic goals.
5.3.2. Change Management
Change management is a structured approach to transitioning individuals, teams, and organizations from a current state to a desired future state. It is essential for ensuring that changes are implemented smoothly and successfully, minimizing resistance and maximizing engagement.
- Importance of Change Management: Â
- Facilitates smooth transitions during organizational changes.
- Reduces resistance from employees by involving them in the process.
- Enhances communication and transparency throughout the organization.
- Key Components of Change Management: Â
- Planning: Develop a clear strategy that outlines the change management process, objectives, and expected outcomes.
- Communication: Keep all stakeholders informed about the changes, reasons behind them, and how they will be affected.
- Training and Support: Provide necessary training and resources to help employees adapt to new systems or processes.
- Monitoring and Feedback: Continuously assess the impact of changes and gather feedback to make necessary adjustments.
- Change Management Models: Â
- ADKAR Model: Focuses on Awareness, Desire, Knowledge, Ability, and Reinforcement.
- Kotter’s 8-Step Process: Emphasizes creating urgency, forming coalitions, and anchoring new approaches in the culture.
Effective change management can lead to improved employee morale, increased productivity, and a more agile organization. At Rapid Innovation, we leverage our expertise in AI and Blockchain to facilitate change management processes, ensuring that our clients can adapt to technological advancements and market shifts seamlessly. We also utilize frameworks such as the Prosci change management and engineering change management to enhance our approach. Additionally, we explore the use of AI agents for resource allocation to further streamline these processes.
5.3.3. Resource Allocation
Resource allocation is the process of distributing available resources—such as time, money, personnel, and technology—among various projects or departments within an organization. Proper resource allocation is crucial for maximizing efficiency and achieving strategic goals.
- Importance of Resource Allocation: Â
- Ensures that resources are used effectively to meet organizational objectives.
- Helps in prioritizing projects based on their importance and potential return on investment.
- Minimizes waste and redundancy by aligning resources with strategic goals.
- Key Considerations in Resource Allocation: Â
- Assessment of Needs: Evaluate the requirements of each project or department to determine the necessary resources.
- Prioritization: Rank projects based on urgency, impact, and alignment with organizational goals.
- Flexibility: Be prepared to reallocate resources as priorities change or new opportunities arise.
- Monitoring and Evaluation: Regularly review resource allocation to ensure it remains aligned with organizational objectives.
- Tools for Resource Allocation: Â
- Project Management Software: Tools like Asana, Trello, or Microsoft Project can help track resource allocation and project progress.
- Resource Management Tools: Software like Resource Guru or Float can assist in visualizing resource availability and allocation.
Effective resource allocation can lead to improved project outcomes, enhanced productivity, and better overall performance. Rapid Innovation employs advanced analytics and AI-driven insights to optimize resource allocation for our clients, ensuring that every investment yields maximum returns.
6. Best Practices and Solutions
Implementing best practices in change management and resource allocation can significantly enhance organizational effectiveness. Here are some recommended strategies:
- Best Practices in Change Management: Â
- Engage Stakeholders Early: Involve key stakeholders from the beginning to foster buy-in and reduce resistance.
- Create a Change Network: Establish a group of change champions who can advocate for the change and support their peers.
- Utilize Technology: Leverage digital tools for communication, training, and feedback to streamline the change process.
- Best Practices in Resource Allocation: Â
- Data-Driven Decision Making: Use analytics to inform resource allocation decisions, ensuring they are based on solid evidence.
- Regular Reviews: Conduct periodic assessments of resource allocation to identify areas for improvement and reallocation.
- Cross-Department Collaboration: Encourage collaboration between departments to share resources and expertise effectively.
- Solutions for Effective Change Management and Resource Allocation: Â
- Integrated Change Management Software: Tools like ChangeGear or ServiceNow can help manage change processes and track resource allocation.
- Training Programs: Invest in training programs that equip employees with the skills needed to adapt to changes and manage resources effectively.
- Feedback Mechanisms: Establish channels for employees to provide feedback on changes and resource allocation, fostering a culture of continuous improvement.
By adopting these best practices and solutions, organizations can navigate change more effectively and allocate resources in a way that maximizes their potential for success. Rapid Innovation is committed to guiding our clients through these processes, ensuring they achieve their business goals efficiently and effectively.
6.1. Data Management Strategies
Effective data management strategies, such as master data management strategy and enterprise data management strategy, are crucial for organizations to harness the full potential of their data. These strategies ensure that data is collected, stored, processed, and analyzed efficiently. At Rapid Innovation, we leverage our expertise in AI and Blockchain to enhance these strategies, ensuring our clients achieve greater ROI. Key components of data management strategies include:
- Data Governance: Establishing policies and procedures to manage data integrity, security, and compliance. This includes defining roles and responsibilities for data stewardship. Our consulting services can help organizations implement robust governance frameworks that align with industry standards, including enterprise data governance strategy.
- Data Quality: Implementing processes to ensure data accuracy, completeness, and consistency. Regular data cleansing and validation checks are essential to maintain high-quality data. We utilize AI-driven tools to automate data quality assessments, significantly reducing manual effort and improving accuracy. This is part of effective data management strategies.
- Data Storage Solutions: Choosing appropriate storage solutions, such as cloud storage or on-premises databases, based on the organization’s needs. This includes considering scalability, accessibility, and cost-effectiveness. Our team can guide clients in selecting the most suitable storage architecture, optimizing costs while ensuring performance, including cloud data management strategy.
- Data Integration: Utilizing tools and techniques to combine data from various sources, ensuring a unified view of information. This can involve ETL (Extract, Transform, Load) processes or data warehousing solutions. We employ advanced integration techniques, including Blockchain for secure and transparent data sharing across platforms, which is essential for a comprehensive data management strategy.
- Data Security: Protecting sensitive data through encryption, access controls, and regular security audits. Compliance with regulations like GDPR or HIPAA is also critical. Our Blockchain solutions provide an immutable ledger for data transactions, enhancing security and compliance, which is a key aspect of any data management strategy.
- Data Lifecycle Management: Managing data from creation to deletion, ensuring that data is archived or disposed of appropriately when it is no longer needed. We assist organizations in developing lifecycle management policies that maximize data utility while minimizing risks, aligning with master data governance strategy. Additionally, our services include Stable Diffusion Development to further enhance data management capabilities.
6.2. Model Development Guidelines
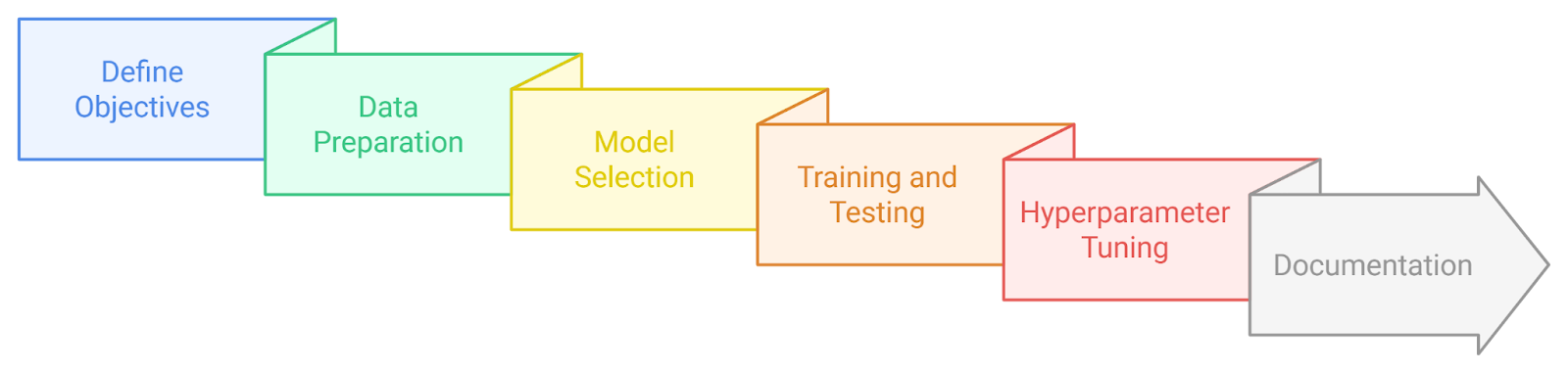
‍
Model development guidelines are essential for creating robust and reliable predictive models. These guidelines help ensure that models are built systematically and can be effectively deployed. At Rapid Innovation, we focus on integrating AI methodologies to enhance model performance. Key aspects include:
- Define Objectives: Clearly outline the goals of the model. Understanding the problem to be solved helps in selecting the right approach and metrics for evaluation.
- Data Preparation: Conduct thorough data preprocessing, including cleaning, normalization, and feature selection. This step is vital for improving model performance. Our AI tools streamline this process, ensuring high-quality input for model training, which is crucial for effective data management strategies.
- Model Selection: Choose the appropriate modeling techniques based on the nature of the data and the problem. Options may include regression, classification, clustering, or deep learning methods. We provide expert guidance in selecting the best-fit models tailored to specific business needs.
- Training and Testing: Split the dataset into training and testing sets to evaluate model performance. Cross-validation techniques can also be employed to ensure robustness. Our methodologies ensure that models are rigorously tested for reliability before deployment.
- Hyperparameter Tuning: Optimize model parameters to enhance performance. Techniques such as grid search or random search can be used to find the best configuration. We utilize automated tuning processes to save time and improve model accuracy.
- Documentation: Maintain comprehensive documentation throughout the model development process. This includes recording decisions made, methodologies used, and results obtained for future reference.
6.3. Quality Assurance Procedures
Quality assurance (QA) procedures are vital for ensuring that data and models meet established standards. Implementing QA procedures helps organizations maintain high levels of accuracy and reliability. At Rapid Innovation, we emphasize the importance of QA in both AI and Blockchain projects. Key elements include:
- Regular Audits: Conduct periodic audits of data and models to identify discrepancies or areas for improvement. This can involve reviewing data sources, processing methods, and model outputs. Our team conducts thorough audits to ensure compliance and performance standards are met.
- Validation Techniques: Use statistical methods to validate models, such as confusion matrices for classification tasks or R-squared values for regression models. This helps assess model performance against benchmarks.
- User Acceptance Testing (UAT): Involve end-users in testing the models to ensure they meet business requirements and are user-friendly. Feedback from users can provide valuable insights for further refinement.
- Continuous Monitoring: Implement systems for ongoing monitoring of data quality and model performance. This includes setting up alerts for anomalies or significant deviations from expected results. Our AI solutions enable real-time monitoring, ensuring prompt action can be taken when issues arise.
- Training and Support: Provide training for staff involved in data management and model development. Ensuring that team members are knowledgeable about QA procedures enhances overall quality.
- Feedback Loops: Establish mechanisms for collecting feedback on data and model performance. This can help identify issues early and facilitate continuous improvement. Our approach fosters a culture of continuous feedback, driving ongoing enhancements in data management and model development processes.
By integrating these strategies, including test data management strategy and metadata management strategy, Rapid Innovation empowers organizations to achieve their business goals efficiently and effectively, ultimately leading to greater ROI.
6.4. Performance Monitoring
Performance monitoring is a critical aspect of any operational strategy, ensuring that organizations can track their progress and make informed decisions. It involves the systematic collection, analysis, and reporting of performance data to assess how well an organization is achieving its objectives.
- Key Performance Indicators (KPIs): Establishing KPIs is essential for measuring success. These metrics should be specific, measurable, achievable, relevant, and time-bound (SMART). At Rapid Innovation, we assist clients in defining KPIs that align with their strategic goals, ensuring that they can effectively measure their performance.
- Data Collection: Utilize various tools and technologies to gather data, including software solutions, surveys, and direct observations. Our AI-driven analytics tools can automate data collection processes, providing real-time insights that enhance decision-making. Application performance monitoring tools can be integrated to ensure comprehensive data collection.
- Regular Reporting: Create a schedule for reporting performance data, which could be weekly, monthly, or quarterly, depending on the organization's needs. Rapid Innovation can help implement reporting frameworks that integrate seamlessly with existing systems, ensuring timely and accurate reporting. Application performance monitoring solutions can facilitate this process.
- Stakeholder Engagement: Involve stakeholders in the monitoring process, as their insights can provide valuable context and help identify areas for improvement. We facilitate stakeholder workshops to gather feedback and foster collaboration, enhancing the overall performance monitoring process.
- Actionable Insights: Analyze the data to derive actionable insights that can assist in making informed decisions and adjusting strategies as necessary. Our advanced AI algorithms can identify trends and anomalies, enabling organizations to respond proactively to performance issues. APM tools can be utilized to enhance this analysis.
- Benchmarking: Compare performance against industry standards or competitors to identify gaps and opportunities for improvement. Rapid Innovation leverages blockchain technology to ensure data integrity in benchmarking processes, providing clients with reliable comparisons. Tools like Datadog APM can be instrumental in this benchmarking process.
Effective performance monitoring not only helps in tracking progress but also fosters accountability within teams. By regularly reviewing performance data, organizations can quickly identify issues and implement corrective actions. For more insights on leveraging AI in business practices, check out the potential of business AI engineering best practices.
6.5. Continuous Improvement Framework
A continuous improvement framework is a structured approach to enhancing processes, products, or services over time. It emphasizes incremental improvements rather than large-scale changes, fostering a culture of ongoing enhancement.
- Plan-Do-Check-Act (PDCA) Cycle: This iterative process involves planning changes, implementing them, checking the results, and acting on what has been learned. Rapid Innovation can guide organizations through the PDCA cycle, ensuring that improvements are data-driven and aligned with business objectives.
- Employee Involvement: Encourage all employees to participate in the improvement process, as their firsthand experience can lead to valuable insights and innovative solutions. We provide training and workshops to empower employees, fostering a culture of continuous improvement.
- Training and Development: Invest in training programs to equip employees with the skills needed for continuous improvement, enhancing their ability to identify and implement changes. Our tailored training solutions focus on AI and blockchain technologies, ensuring that teams are well-equipped to drive innovation.
- Feedback Mechanisms: Establish channels for feedback from customers and employees, as this information is crucial for identifying areas that require improvement. Rapid Innovation can implement AI-driven feedback systems that analyze customer sentiment and employee engagement.
- Documentation: Keep detailed records of improvement initiatives and their outcomes to help track progress and learn from past experiences. We assist organizations in creating robust documentation practices that enhance knowledge sharing and accountability.
- Celebrate Successes: Recognize and celebrate improvements, no matter how small, to boost morale and encourage a culture of continuous improvement. Our team can help design recognition programs that align with organizational values and foster a positive work environment.
Implementing a continuous improvement framework can lead to increased efficiency, reduced waste, and enhanced customer satisfaction. Organizations that embrace this approach are better positioned to adapt to changing market conditions and customer needs.
7. Industry Applications and Use Cases
The principles of performance monitoring and continuous improvement are applicable across various industries. Understanding these applications can help organizations leverage these strategies effectively.
- Manufacturing: In manufacturing, performance monitoring can track production efficiency, quality control, and equipment effectiveness. Continuous improvement initiatives can lead to lean manufacturing practices, reducing waste and increasing productivity. Rapid Innovation can implement AI solutions that optimize production processes and enhance quality assurance.
- Healthcare: In the healthcare sector, performance monitoring is vital for patient care quality and operational efficiency. Continuous improvement frameworks can enhance patient outcomes and streamline processes, such as reducing wait times and improving service delivery. Our blockchain solutions can ensure secure and transparent patient data management.
- Retail: Retailers can use performance monitoring to analyze sales data, customer satisfaction, and inventory management. Continuous improvement efforts can focus on enhancing the customer experience, optimizing supply chains, and increasing sales conversions. Rapid Innovation's AI tools can provide predictive analytics to inform inventory decisions and marketing strategies.
- Information Technology: In IT, performance monitoring can assess system uptime, response times, and user satisfaction. Continuous improvement can lead to better software development practices, improved cybersecurity measures, and enhanced user experiences. We offer AI-driven monitoring solutions that provide real-time insights into system performance, including application performance monitoring solutions.
- Education: Educational institutions can apply performance monitoring to evaluate student performance, faculty effectiveness, and resource allocation. Continuous improvement initiatives can enhance curriculum development, teaching methods, and student engagement. Rapid Innovation can develop customized AI tools that support personalized learning experiences.
By understanding the industry-specific applications of performance monitoring and continuous improvement, organizations can tailor their strategies to meet unique challenges and opportunities. This adaptability is crucial for maintaining a competitive edge in today's dynamic business environment. Rapid Innovation is committed to helping clients achieve their business goals efficiently and effectively through innovative AI and blockchain solutions, including APM solutions and tools for server monitoring.
7.1. Retail and E-commerce
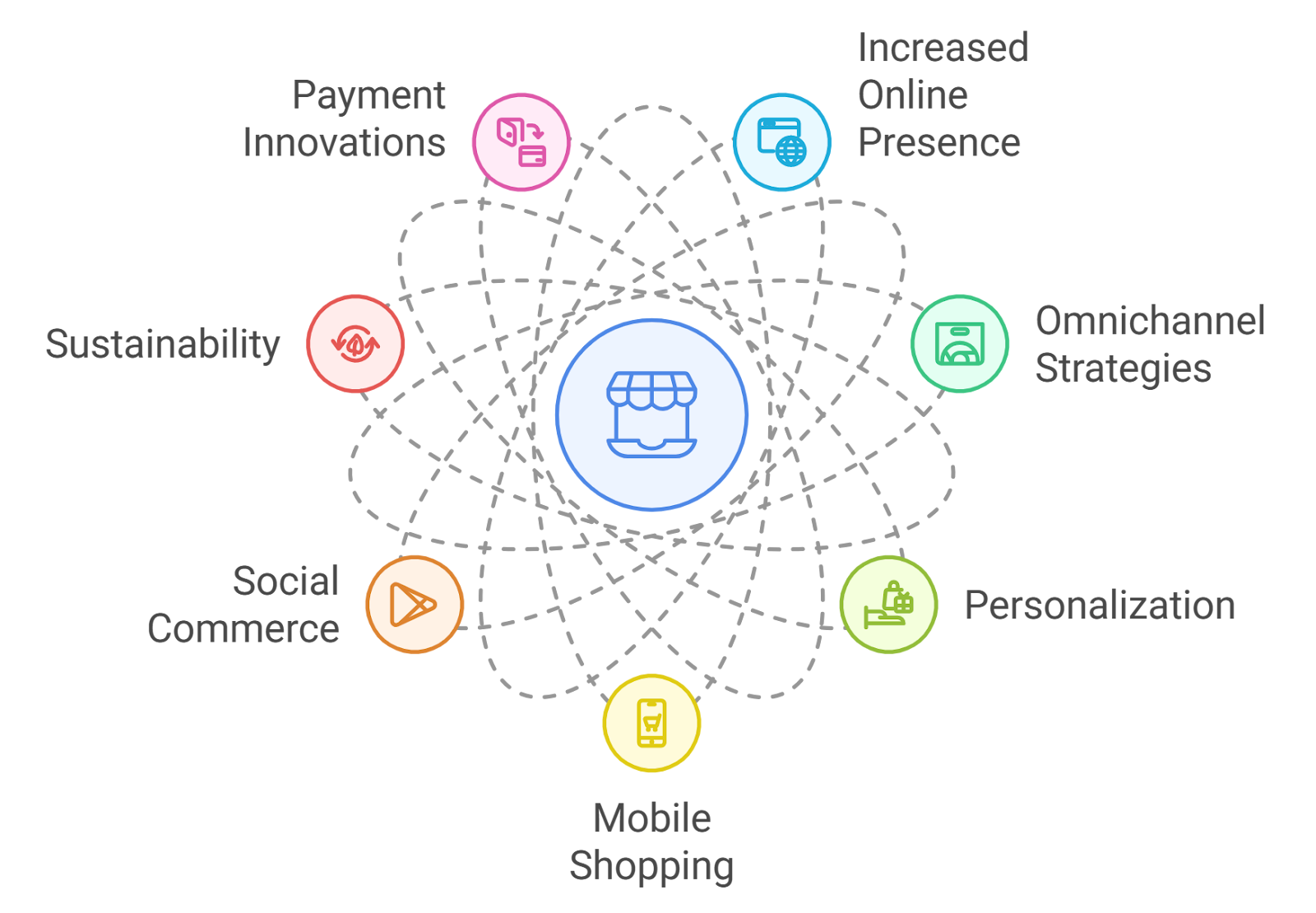
‍
The retail and e-commerce sectors have undergone significant transformations in recent years, driven by technological advancements and changing consumer behaviors. The rise of online shopping has reshaped how businesses operate and engage with customers.
- Increased Online Presence: Retailers are increasingly establishing an online presence to reach a broader audience. E-commerce platforms allow businesses to sell products 24/7, breaking geographical barriers. Rapid Innovation can assist in developing robust e-commerce solutions that enhance visibility and accessibility.
- Omnichannel Strategies: Many retailers are adopting omnichannel strategies, integrating online and offline experiences. This approach enhances customer convenience and satisfaction. Our expertise in AI can help analyze customer interactions across channels, optimizing the overall shopping experience.
- Personalization: E-commerce businesses leverage data analytics to offer personalized shopping experiences. Tailored recommendations and targeted marketing campaigns can significantly boost sales. Rapid Innovation employs advanced AI algorithms to analyze consumer behavior, enabling businesses to deliver highly personalized content and offers. This is particularly relevant in the context of ecommerce personalization trends and e commerce personalization trends.
- Mobile Shopping: The proliferation of smartphones has led to a surge in mobile shopping. Retailers must optimize their websites and apps for mobile users to capture this growing market. We can develop mobile-friendly platforms that enhance user experience and drive conversions. Mobile commerce trends indicate that this segment will continue to grow.
- Social Commerce: Social media platforms are becoming vital sales channels. Brands utilize social media to engage customers and drive traffic to their e-commerce sites. Our solutions can integrate social commerce features, allowing businesses to leverage social media for direct sales. The rise of social commerce platforms is a key factor in the future of ecommerce industry.
- Sustainability: Consumers are increasingly concerned about sustainability. Retailers that adopt eco-friendly practices can attract environmentally conscious shoppers. Rapid Innovation can help implement blockchain solutions to ensure transparency in supply chains, appealing to eco-conscious consumers.
- Payment Innovations: The introduction of various payment options, including digital wallets and buy-now-pay-later services, enhances the shopping experience and can lead to higher conversion rates. We can develop secure payment gateways that support diverse payment methods, improving customer satisfaction.
7.2. Financial Services
The financial services industry is experiencing a digital revolution, with technology reshaping how consumers manage their finances. Fintech companies are emerging as key players, offering innovative solutions that challenge traditional banking models.
- Digital Banking: Online banks and neobanks provide customers with convenient banking services without the need for physical branches. This trend is particularly appealing to tech-savvy consumers. Rapid Innovation can assist in developing user-friendly digital banking platforms that enhance customer engagement.
- Mobile Payments: The rise of mobile payment solutions, such as Apple Pay and Google Wallet, has made transactions faster and more secure. Consumers appreciate the convenience of paying with their smartphones. Our expertise in AI can enhance fraud detection mechanisms, ensuring secure transactions.
- Peer-to-Peer Lending: Fintech platforms facilitate peer-to-peer lending, allowing individuals to borrow and lend money directly. This model often offers lower interest rates compared to traditional banks. We can develop platforms that streamline the lending process, improving user experience and efficiency.
- Robo-Advisors: Automated investment platforms, or robo-advisors, provide personalized investment advice based on algorithms. They are accessible to a broader audience, making investing more inclusive. Rapid Innovation can create sophisticated robo-advisory solutions that cater to diverse investor needs.
- Blockchain Technology: Blockchain is revolutionizing the financial sector by enhancing security and transparency in transactions. Cryptocurrencies are gaining traction as alternative investment options. Our blockchain solutions can help financial institutions implement secure and transparent transaction systems.
- Regulatory Changes: The financial services industry is subject to evolving regulations. Companies must stay compliant while innovating to meet consumer demands. We can provide consulting services to navigate regulatory landscapes effectively.
- Cybersecurity: As digital transactions increase, so do cybersecurity threats. Financial institutions must invest in robust security measures to protect customer data and maintain trust. Rapid Innovation offers advanced cybersecurity solutions to safeguard sensitive financial information.
7.3. Subscription-based Businesses
Subscription-based business models have gained popularity across various industries, offering consumers convenience and value. This model allows businesses to generate recurring revenue while fostering customer loyalty.
- Predictable Revenue: Subscription models provide businesses with predictable revenue streams, making financial planning more manageable. This stability can be attractive to investors. Our analytics solutions can help businesses forecast revenue and optimize pricing strategies.
- Customer Retention: Subscriptions encourage customer loyalty, as consumers are more likely to stick with a service they are already paying for. Retaining existing customers is often more cost-effective than acquiring new ones. We can implement AI-driven retention strategies to minimize churn rates.
- Diverse Offerings: Subscription services span various industries, including entertainment (e.g., Netflix), food (e.g., meal kits), and software (e.g., Adobe Creative Cloud). This diversity allows businesses to cater to different consumer needs. Rapid Innovation can help develop tailored subscription models that align with market demands.
- Personalization: Many subscription services use data analytics to personalize offerings, enhancing customer satisfaction. Tailored recommendations can lead to higher engagement and retention rates. Our AI solutions can analyze user preferences to deliver personalized content and services.
- Flexibility: Subscription models often provide flexibility, allowing customers to choose plans that suit their needs. This adaptability can attract a broader audience. We can assist in designing flexible subscription frameworks that enhance customer experience.
- Churn Management: Managing customer churn is crucial for subscription businesses. Implementing strategies to retain subscribers, such as loyalty programs or exclusive content, can mitigate churn rates. Our data analytics can identify churn patterns and suggest effective retention strategies.
- Global Reach: The internet enables subscription-based businesses to reach a global audience. Companies can scale their operations without the limitations of physical locations. Rapid Innovation can support businesses in expanding their reach through scalable digital solutions.
- Ecommerce Trends: As the e-commerce industry continues to evolve, staying updated on ecommerce trends is essential for businesses to remain competitive. Understanding ecommerce industry trends and ecommerce statistics can provide valuable insights for strategic planning.
- Future of B2B Ecommerce: The future of b2b ecommerce is promising, with increasing digitalization and the rise of b2b commerce trends shaping the landscape. Companies must adapt to these changes to thrive in the competitive market.
- E Commerce Industry Growth: The e commerce industry growth is driven by factors such as mobile ecommerce trends and the increasing importance of social commerce in driving sales. Businesses must leverage these trends to maximize their potential in the market.
For more insights on subscription management, check out this complete guide on AI agents for subscription management.
7.4. B2B Services
B2B (Business-to-Business) services encompass a wide range of offerings designed to support the operational needs of businesses. These services are crucial for enhancing efficiency, productivity, and overall business performance.
- Types of B2B Services: Â
- Consulting Services: Expert advice in areas like management, IT, and marketing, where Rapid Innovation leverages its AI and Blockchain expertise to provide tailored solutions that drive strategic growth.
- Logistics and Supply Chain Management: Solutions for transportation, warehousing, and inventory management, enhanced by AI-driven analytics for optimized decision-making.
- Financial Services: Accounting, auditing, and financial planning tailored for businesses, utilizing Blockchain for secure and transparent transactions.
- Marketing Services: Digital marketing, SEO, and content creation aimed at business clients, where AI tools can analyze consumer behavior to improve targeting and engagement. This includes services from b2b digital marketing agencies and b2b marketing firms, as well as specialized offerings like b2b social media marketing and b2b lead generation.
- IT Services: Software development, cybersecurity, and IT support, with a focus on integrating AI and Blockchain technologies to enhance security and operational efficiency.
- Importance of B2B Services: Â
- Cost Efficiency: Streamlining operations can lead to significant cost savings, particularly through the automation capabilities of AI.
- Expertise Access: Businesses can leverage specialized knowledge without hiring full-time staff, allowing them to tap into Rapid Innovation's extensive experience in AI and Blockchain.
- Scalability: B2B services can grow with the business, adapting to changing needs, especially with the flexibility offered by cloud-based solutions.
- Focus on Core Activities: Outsourcing non-core functions allows businesses to concentrate on their primary objectives, while Rapid Innovation handles the complexities of technology integration.
- Trends in B2B Services: Â
- Digital Transformation: Increasing reliance on technology to enhance service delivery, where Rapid Innovation can guide businesses through the adoption of AI and Blockchain.
- Personalization: Tailoring services to meet specific client needs, utilizing AI to analyze data and deliver customized solutions.
- Sustainability: Growing demand for eco-friendly practices in service delivery, where Blockchain can provide transparency in supply chains to promote ethical sourcing.
7.5. Healthcare Services
Healthcare services are essential for maintaining and improving the health of individuals and communities. These services range from preventive care to complex medical treatments and are delivered through various channels.
- Types of Healthcare Services: Â
- Primary Care: General health services provided by family physicians and general practitioners.
- Specialty Care: Services provided by specialists, such as cardiologists and orthopedic surgeons.
- Emergency Services: Immediate care for acute medical conditions and injuries.
- Preventive Services: Screenings, vaccinations, and wellness checks aimed at preventing illness.
- Rehabilitation Services: Physical therapy and occupational therapy to aid recovery.
- Importance of Healthcare Services: Â
- Improved Health Outcomes: Access to quality healthcare leads to better health and longevity.
- Economic Impact: A healthy workforce contributes to increased productivity and economic growth.
- Public Health: Healthcare services play a vital role in controlling diseases and promoting community health.
- Trends in Healthcare Services: Â
- Telemedicine: Remote consultations and digital health solutions are becoming more prevalent.
- Patient-Centered Care: Emphasis on personalized treatment plans and patient engagement.
- Integration of Technology: Use of AI and data analytics to enhance service delivery and patient care, where Rapid Innovation can provide cutting-edge solutions.
8. Regulatory and Ethical Considerations
Regulatory and ethical considerations are critical in both B2B and healthcare services. They ensure that businesses operate within legal frameworks and adhere to ethical standards, promoting trust and accountability.
- Regulatory Considerations: Â
- Compliance: Businesses must comply with local, national, and international regulations.
- Data Protection: Adhering to laws like GDPR and HIPAA to protect sensitive information.
- Licensing and Accreditation: Ensuring that services are provided by qualified professionals and organizations.
- Ethical Considerations: Â
- Transparency: Maintaining open communication with clients and stakeholders.
- Fair Practices: Ensuring equitable treatment and avoiding discrimination in service delivery.
- Corporate Social Responsibility (CSR): Engaging in practices that benefit society and the environment.
- Importance of Regulatory and Ethical Considerations: Â
- Trust Building: Compliance and ethical behavior foster trust among clients and partners.
- Risk Management: Adhering to regulations reduces the risk of legal issues and penalties.
- Reputation Management: Ethical practices enhance a company's reputation and brand value.
In conclusion, understanding B2B services, including b2b lead generation, b2b online marketing, and b2b client services, healthcare services, and the associated regulatory considerations is essential for businesses to thrive in today's competitive landscape.
8.1. Data Privacy Compliance

‍
Data privacy compliance refers to the adherence to laws and regulations that govern the collection, storage, and processing of personal data. Organizations must ensure that they are compliant with various data protection laws, such as gdpr compliant regulations, to avoid legal repercussions and maintain consumer trust. Rapid Innovation can assist clients in navigating these complexities to achieve greater ROI through effective compliance strategies. Key aspects include:
- Understanding relevant regulations: Familiarize yourself with laws such as the General Data Protection Regulation (GDPR) in Europe, the California Consumer Privacy Act (CCPA) in the United States, and other regional data protection laws. Rapid Innovation offers consulting services to help organizations understand and implement these regulations effectively, including gdpr compliance regulations and ccpa compliance.
- Implementing data protection measures: Organizations should adopt robust data security practices, including encryption, access controls, and regular audits to protect personal data from breaches. Our development team can integrate advanced security features into your systems, ensuring gdpr compliance while enhancing data integrity.
- Obtaining consent: It is crucial to obtain explicit consent from individuals before collecting their data. This includes providing clear information about how their data will be used. Rapid Innovation can develop user-friendly consent management solutions that streamline this process, ensuring compliance with gdpr requirements.
- Data minimization: Collect only the data that is necessary for specific purposes. This reduces the risk of data breaches and ensures compliance with privacy regulations. Our expertise in data architecture can help clients design systems that prioritize data minimization, aligning with gdpr data protection requirements.
- Training employees: Regular training on data privacy policies and practices is essential for all employees to ensure they understand their responsibilities regarding data protection. Rapid Innovation offers tailored training programs to equip your team with the necessary knowledge and skills related to personal information compliance and data privacy compliance.
8.2. Ethical AI Usage
Ethical AI usage involves the responsible development and deployment of artificial intelligence technologies. As AI systems become more prevalent, organizations must consider the ethical implications of their use. Rapid Innovation is committed to guiding clients in implementing ethical AI practices to enhance their brand reputation and ROI. Key considerations include:
- Fairness: AI systems should be designed to avoid bias and discrimination. This involves using diverse datasets and regularly testing algorithms for fairness. Our team can help you develop AI models that are both effective and equitable.
- Accountability: Organizations must establish clear accountability for AI decisions. This includes identifying who is responsible for the outcomes of AI systems and ensuring that there are mechanisms for redress in case of harm. Rapid Innovation can assist in creating governance frameworks that promote accountability.
- Privacy: Ethical AI usage requires that personal data is handled with care. Organizations should implement privacy-preserving techniques, such as differential privacy, to protect individual data while still gaining insights from it. We can integrate these techniques into your AI solutions to ensure compliance and trust.
- Transparency: AI systems should be transparent in their operations. This means providing clear explanations of how decisions are made and ensuring that users understand the capabilities and limitations of AI technologies. Rapid Innovation emphasizes transparency in AI development, helping clients communicate effectively with stakeholders.
- Continuous monitoring: Regularly assess AI systems for ethical compliance and effectiveness. This includes monitoring for unintended consequences and making necessary adjustments to mitigate risks. Our ongoing support services ensure that your AI systems remain aligned with ethical standards.
8.3. Transparency Requirements
Transparency requirements refer to the obligations organizations have to disclose information about their data practices and AI systems. Transparency is crucial for building trust with consumers and stakeholders. Rapid Innovation can help clients meet these requirements efficiently, ultimately enhancing their market position. Key elements include:
- Clear communication: Organizations should provide clear and accessible information about their data collection practices, including what data is collected, how it is used, and who it is shared with. We can assist in crafting clear communication strategies that resonate with your audience.
- Algorithmic transparency: When using AI systems, organizations should disclose how algorithms work, including the data sources and decision-making processes involved. This helps users understand the rationale behind AI-driven decisions. Rapid Innovation can develop documentation and reporting tools that enhance algorithmic transparency.
- Reporting and documentation: Maintain thorough documentation of data processing activities and AI system operations. This includes keeping records of data sources, consent mechanisms, and any third-party data sharing. Our solutions streamline documentation processes, ensuring compliance and ease of access.
- User rights: Inform individuals of their rights regarding their personal data, including the right to access, rectify, or delete their information. Organizations should have processes in place to facilitate these rights. Rapid Innovation can implement user-friendly interfaces that empower individuals to exercise their rights effectively.
- Regular updates: Keep stakeholders informed about changes in data practices or AI systems. This includes notifying users of any significant changes to privacy policies or data handling procedures. Our communication solutions ensure that your stakeholders are always informed and engaged, supporting overall data privacy and compliance efforts.
8.4. Consumer Rights and Protections
Consumer rights and protections are essential components of a fair marketplace. They ensure that consumers are treated fairly and have access to safe products and services. Understanding these rights, including legal consumer rights, is crucial for both consumers and businesses.
- Right to Safety: Consumers have the right to be protected against products that are hazardous to health or life. This includes regulations on food safety, pharmaceuticals, and consumer goods.
- Right to Information: Consumers should receive accurate information about products and services, including pricing, ingredients, and potential risks. Transparency is key to informed decision-making.
- Right to Choose: Consumers should have access to a variety of products and services at competitive prices. This promotes healthy competition and innovation in the marketplace.
- Right to be Heard: Consumers have the right to voice their complaints and concerns. Businesses should have mechanisms in place to address consumer feedback effectively, including access to consumer rights contact information.
- Right to Privacy: With the rise of digital commerce, consumer privacy has become increasingly important. Regulations like GDPR in Europe protect consumers' personal data from misuse.
Understanding these rights helps consumers make informed choices and empowers them to seek redress when necessary. Resources such as consumer rights websites and consumer rights information can assist in this process. Businesses that prioritize consumer rights can build trust and loyalty, leading to long-term success. For instance, blockchain technology can enhance product quality and safety, further supporting consumer rights.
8.5. Industry-specific Regulations
Industry-specific regulations are tailored rules and guidelines that govern particular sectors. These regulations ensure that businesses operate within legal frameworks while protecting consumers and the environment.
- Financial Services: The financial industry is heavily regulated to protect consumers from fraud and ensure fair lending practices. Agencies like the Consumer Financial Protection Bureau (CFPB) enforce these regulations.
- Healthcare: Regulations in the healthcare sector focus on patient safety, data privacy, and the quality of care. The Health Insurance Portability and Accountability Act (HIPAA) is a key regulation that protects patient information.
- Telecommunications: The telecommunications industry is subject to regulations that promote competition and protect consumers from unfair practices. The Federal Communications Commission (FCC) oversees these regulations in the U.S.
- Environmental Regulations: Industries such as manufacturing and energy are subject to environmental regulations that aim to minimize pollution and protect natural resources. Compliance with these regulations is crucial for sustainable business practices.
- Food and Beverage: The food industry is regulated to ensure safety and quality. Agencies like the Food and Drug Administration (FDA) set standards for food production, labeling, and marketing.
Businesses must stay informed about industry-specific regulations to avoid legal issues and maintain compliance. Understanding consumer laws and rights is essential for businesses to navigate these regulations effectively. Adhering to these regulations not only protects consumers but also enhances a company's reputation.
9. Measuring Success and ROI
Measuring success and return on investment (ROI) is vital for businesses to assess their performance and make informed decisions. Understanding how to evaluate these metrics can lead to improved strategies and growth.
- Define Clear Objectives: Establish specific, measurable goals that align with your business strategy. This could include sales targets, customer acquisition rates, or brand awareness metrics.
- Use Key Performance Indicators (KPIs): Identify KPIs that reflect your objectives. Common KPIs include revenue growth, customer retention rates, and conversion rates.
- Analyze Financial Metrics: Calculate ROI by comparing the net profit from an investment to its cost. The formula is:
language="language-plaintext"``` -a1b2c3- Â ROI = (Net Profit / Cost of Investment) x 100
- Customer Feedback: Collect and analyze customer feedback to gauge satisfaction and identify areas for improvement. Surveys and reviews can provide valuable insights.
- Benchmarking: Compare your performance against industry standards or competitors. This helps identify strengths and weaknesses in your business strategy.
- Regular Reporting: Create regular reports to track progress over time. This allows for timely adjustments to strategies based on performance data.
By effectively measuring success and ROI, businesses can make data-driven decisions that enhance performance and drive growth. At Rapid Innovation, we leverage AI and Blockchain technologies to provide advanced analytics and insights, enabling our clients to optimize their operations and achieve greater ROI. For instance, our AI-driven analytics tools can help businesses identify trends and customer preferences, while our Blockchain solutions ensure transparency and security in transactions, further enhancing consumer trust and satisfaction. Additionally, businesses should be aware of the federal consumer rights and the main federal agency for handling consumer rights to ensure compliance and protect their customers.
9.1. Key Performance Indicators
Key Performance Indicators (KPIs) are essential metrics that help organizations measure their progress toward specific objectives. They provide a clear framework for evaluating success and making informed decisions.
- KPIs should be specific, measurable, achievable, relevant, and time-bound (SMART).
- Common KPIs include: Â
- Revenue growth
- Customer acquisition cost
- Customer lifetime value
- Employee productivity
- Net promoter score (NPS)
- KPIs can be categorized into: Â
- Financial KPIs: Focus on financial performance, such as profit margins and return on investment.
- Operational KPIs: Measure efficiency and effectiveness in processes, like production time and inventory turnover.
- Customer KPIs: Assess customer satisfaction and engagement, including retention rates and feedback scores.
Regularly reviewing KPIs allows organizations to adjust strategies and improve performance. Tools like dashboards and analytics software can help visualize KPI data for better decision-making. At Rapid Innovation, we leverage AI-driven analytics to provide real-time insights into your KPIs, enabling you to make data-informed decisions that align with your business goals. Understanding the meaning of KPIs and their role in performance measurement is crucial for effective management. For example, key performance indicators examples can include metrics like sales growth or customer satisfaction scores. Additionally, organizations may explore kpi key performance indicator frameworks to better define their objectives and track progress. Furthermore, organizations can assess the ROI of enterprise blockchain to measure the business impact of their initiatives.
9.2. ROI Calculation Methods
Return on Investment (ROI) is a critical metric that evaluates the profitability of an investment relative to its cost. Understanding ROI helps businesses make informed financial decisions and allocate resources effectively.
- Basic ROI formula: Â
- ROI = (Net Profit / Cost of Investment) x 100
- Different methods to calculate ROI include: Â
- Simple ROI: A straightforward calculation that provides a quick overview of profitability.
- Annualized ROI: Adjusts ROI to reflect annual performance, useful for comparing investments over different time frames.
- Adjusted ROI: Takes into account additional factors like risk and opportunity cost, providing a more comprehensive view.
- ROI can be applied to various contexts, such as: Â
- Marketing campaigns: Evaluating the effectiveness of advertising spend.
- Capital investments: Assessing the profitability of new equipment or technology.
- Employee training programs: Measuring the impact of training on productivity and performance.
At Rapid Innovation, we utilize advanced AI algorithms to optimize your ROI calculations, ensuring that you can accurately assess the profitability of your investments and make strategic decisions that enhance your financial performance.
9.3. Impact Assessment
Impact assessment is a systematic process used to evaluate the effects of a project, program, or policy on the environment, society, and economy. It helps organizations understand the broader implications of their actions and make data-driven decisions.
- Key components of impact assessment include: Â
- Baseline analysis: Establishing a reference point to measure changes over time.
- Stakeholder engagement: Involving affected parties to gather diverse perspectives and insights.
- Data collection: Utilizing qualitative and quantitative methods to gather relevant information.
- Types of impact assessments: Â
- Environmental impact assessment (EIA): Evaluates potential environmental effects of a project.
- Social impact assessment (SIA): Assesses the social consequences of a project on communities and individuals.
- Economic impact assessment: Analyzes the economic implications, such as job creation and local business growth.
- Benefits of conducting impact assessments: Â
- Informed decision-making: Provides evidence-based insights to guide project planning and execution.
- Risk management: Identifies potential negative impacts and allows for mitigation strategies.
- Enhanced accountability: Demonstrates commitment to responsible practices and stakeholder engagement.
Regular impact assessments can lead to continuous improvement and better alignment with organizational goals. By integrating blockchain technology, Rapid Innovation ensures transparency and traceability in your impact assessments, fostering trust and accountability among stakeholders. Understanding key point indicators and their relevance can further enhance the effectiveness of these assessments.
9.4. Benchmarking Strategies
Benchmarking strategies are essential for organizations aiming to improve their performance by comparing their processes and outcomes with industry standards or best practices. This systematic approach helps identify areas for improvement and fosters a culture of continuous enhancement.
- Types of Benchmarking: Â
- Internal Benchmarking: Comparing processes within the same organization to identify best practices.
- Competitive Benchmarking: Analyzing direct competitors to understand their strengths and weaknesses.
- Functional Benchmarking: Looking at similar functions in different industries to gain insights.
- Generic Benchmarking: Comparing processes that are similar regardless of the industry.
- Steps in Benchmarking: Â
- Identify Objectives: Clearly define what you want to achieve through benchmarking.
- Select Benchmarking Partners: Choose organizations or processes that excel in the areas you want to improve.
- Collect Data: Gather quantitative and qualitative data for comparison.
- Analyze Data: Evaluate the data to identify gaps and opportunities for improvement.
- Implement Changes: Develop action plans based on findings and implement necessary changes.
- Monitor Progress: Continuously track performance to ensure improvements are sustained.
- Benefits of Benchmarking: Â
- Enhances operational efficiency.
- Drives innovation by exposing organizations to new ideas.
- Improves customer satisfaction through better service delivery.
- Increases competitiveness by identifying best practices.
At Rapid Innovation, we leverage AI and blockchain technologies to enhance benchmarking processes. For instance, our AI-driven analytics tools can provide deeper insights into performance metrics, enabling organizations to make data-driven decisions that lead to greater ROI. Additionally, blockchain can ensure data integrity and transparency in benchmarking comparisons, fostering trust among stakeholders.
Benchmarking in strategic management is a critical aspect that organizations must consider to remain competitive. By implementing a benchmark pricing strategy, companies can align their pricing models with industry standards, ensuring they remain attractive to customers. Furthermore, benchmarking as a strategic management tool allows organizations to evaluate their performance against the best in the industry, leading to improved operational practices.
An example of strategic benchmarking can be seen in organizations that analyze their competitors' successful strategies to enhance their own. For instance, a benchmarking strategy example might involve a company studying the customer service practices of a leading competitor to identify areas for improvement. Similarly, an example of strategic benchmarking could involve a business assessing its supply chain efficiency against industry leaders to uncover potential enhancements.
For more insights on how AI can transform financial reporting and benchmarking, check out this article on generative AI and automated financial reporting applications.
9.5. Performance Reporting
Performance reporting is a critical component of organizational management, providing insights into how well an organization is achieving its goals. Effective performance reporting helps stakeholders make informed decisions and fosters accountability.
- Key Elements of Performance Reporting: Â
- Metrics and KPIs: Establish clear metrics and Key Performance Indicators (KPIs) to measure success.
- Data Visualization: Use charts, graphs, and dashboards to present data in an easily digestible format.
- Frequency of Reporting: Determine how often reports will be generated (e.g., weekly, monthly, quarterly).
- Stakeholder Engagement: Ensure reports are tailored to the needs of different stakeholders, including management, employees, and investors.
- Types of Performance Reports: Â
- Operational Reports: Focus on day-to-day operations and performance metrics.
- Financial Reports: Provide insights into the financial health of the organization.
- Strategic Reports: Assess progress towards long-term strategic goals.
- Best Practices for Performance Reporting: Â
- Ensure accuracy and reliability of data.
- Keep reports concise and focused on key insights.
- Encourage feedback from stakeholders to improve future reports.
- Use technology to automate reporting processes and enhance efficiency.
Rapid Innovation employs advanced AI algorithms to automate performance reporting, ensuring timely and accurate insights. Our solutions can help organizations visualize their performance data effectively, allowing for quick adjustments and improved decision-making.
10. Future Trends and Innovations
The landscape of business management is constantly evolving, driven by technological advancements and changing market dynamics. Understanding future trends and innovations is crucial for organizations to stay competitive and relevant.
- Digital Transformation: Â
- Organizations are increasingly adopting digital tools and technologies to streamline operations and enhance customer experiences.
- Automation and artificial intelligence (AI) are becoming integral in decision-making processes.
- Sustainability and Corporate Responsibility: Â
- There is a growing emphasis on sustainable practices and corporate social responsibility (CSR).
- Companies are integrating sustainability into their business models to meet consumer demand for ethical practices.
- Data-Driven Decision Making: Â
- The use of big data analytics is on the rise, enabling organizations to make informed decisions based on real-time data.
- Predictive analytics is helping businesses anticipate market trends and consumer behavior.
- Remote Work and Flexibility: Â
- The shift towards remote work is likely to continue, with organizations adopting flexible work arrangements.
- This trend is reshaping workplace culture and employee engagement strategies.
- Customer-Centric Approaches: Â
- Businesses are focusing more on personalized customer experiences, leveraging data to tailor products and services.
- Omnichannel strategies are being implemented to provide seamless interactions across various platforms.
- Agile Methodologies: Â
- Organizations are adopting agile practices to enhance responsiveness and adaptability in a fast-paced environment.
- This approach fosters collaboration and innovation, allowing teams to pivot quickly in response to changes.
By staying informed about these trends and innovations, organizations can position themselves for success in an ever-changing business landscape. Rapid Innovation is committed to guiding clients through these transformations, ensuring they harness the full potential of AI and blockchain technologies to achieve their business goals efficiently and effectively.
10.1. Emerging Technologies
Emerging technologies are reshaping industries and driving innovation across various sectors. These technologies are characterized by their potential to disrupt existing processes and create new opportunities. Key emerging technologies include:
- Artificial Intelligence (AI) and Machine Learning (ML): These technologies enable systems to learn from data, improving decision-making and automating tasks. At Rapid Innovation, we leverage AI and ML to develop tailored solutions that enhance operational efficiency and customer engagement. For instance, our AI-driven predictive analytics tools help clients in healthcare anticipate patient needs, leading to improved outcomes and reduced costs. This includes new AI technology and AI new technology that are at the forefront of innovation. As an AI consulting company, we can help you navigate this landscape effectively.
- Internet of Things (IoT): IoT connects devices and systems, allowing for real-time data collection and analysis. Rapid Innovation assists clients in implementing IoT solutions that transform their operations. For example, in the manufacturing sector, our IoT applications enable real-time monitoring of equipment, reducing downtime and optimizing production processes. Emerging tech in IoT is crucial for businesses looking to stay competitive.
- Blockchain: Originally developed for cryptocurrencies, blockchain technology is now being utilized for secure transactions and data integrity across various sectors, including finance, supply chain, and healthcare. Rapid Innovation specializes in blockchain development, helping clients enhance transparency and security in their operations. Our blockchain solutions have enabled businesses to streamline supply chain processes, resulting in significant cost savings and improved trust among stakeholders.
- Augmented Reality (AR) and Virtual Reality (VR): These technologies are enhancing user experiences in fields like gaming, education, and training. Rapid Innovation develops AR and VR applications that provide immersive training environments, allowing organizations to improve employee skills and knowledge retention effectively. The latest technologies in AR and VR are paving the way for innovative training solutions.
- Quantum Computing: Although still in its infancy, quantum computing has the potential to solve complex problems much faster than traditional computers, impacting fields such as cryptography, drug discovery, and optimization. Rapid Innovation is at the forefront of exploring quantum computing applications, preparing our clients for future advancements in this transformative technology. Emerging technologies like quantum computing are set to revolutionize various industries.
10.2. Advanced Analytics Capabilities
Advanced analytics capabilities are essential for organizations looking to leverage data for strategic decision-making. These capabilities go beyond traditional data analysis, providing deeper insights and predictive capabilities. Key components include:
- Predictive Analytics: This involves using statistical algorithms and machine learning techniques to identify the likelihood of future outcomes based on historical data. Rapid Innovation empowers businesses to anticipate customer behavior and optimize operations through our predictive analytics solutions, ultimately driving greater ROI. New technologies in predictive analytics are enhancing our capabilities.
- Prescriptive Analytics: This advanced form of analytics not only predicts outcomes but also recommends actions to achieve desired results. Our prescriptive analytics tools help organizations make informed decisions by evaluating various scenarios and their potential impacts, ensuring that clients can navigate complex business landscapes effectively.
- Real-time Analytics: The ability to analyze data as it is generated allows organizations to respond quickly to changing conditions. Rapid Innovation's real-time analytics solutions provide clients in industries like finance and e-commerce with timely insights that drive competitive advantage and enhance decision-making. The latest technology trends in real-time analytics are crucial for businesses.
- Data Visualization: Advanced analytics often includes sophisticated data visualization tools that help stakeholders understand complex data sets. Our data visualization solutions enable clients to reveal trends, patterns, and anomalies, facilitating better communication and strategic planning.
- Big Data Technologies: The rise of big data has necessitated the development of advanced analytics tools capable of processing vast amounts of information. Rapid Innovation utilizes technologies like Hadoop and Spark to help organizations analyze large data sets efficiently, unlocking valuable insights that drive business growth. New technologies in big data are essential for effective analysis.
10.3. Integration with Other Systems
Integration with other systems is crucial for maximizing the value of technology investments. Seamless integration allows organizations to streamline processes, enhance data sharing, and improve overall efficiency. Key aspects include:
- API Integration: Application Programming Interfaces (APIs) facilitate communication between different software applications. Rapid Innovation provides API integration services that enable organizations to connect disparate systems, allowing for data exchange and process automation, ultimately enhancing operational efficiency.
- Data Integration: Combining data from various sources into a unified view is essential for comprehensive analysis. Our data integration tools help organizations consolidate information from different databases, applications, and platforms, ensuring that clients have access to accurate and timely data for decision-making.
- Cloud Integration: As more organizations move to cloud-based solutions, integrating cloud applications with on-premises systems becomes vital. Rapid Innovation ensures that data flows smoothly between environments, enhancing collaboration and accessibility for our clients.
- Workflow Automation: Integrating systems can automate repetitive tasks, reducing manual effort and minimizing errors. Our workflow automation tools streamline processes across departments, improving productivity and allowing organizations to focus on strategic initiatives.
- Enhanced Customer Experience: Integration allows organizations to provide a more cohesive customer experience. By connecting customer relationship management (CRM) systems with marketing and sales platforms, Rapid Innovation helps businesses deliver personalized interactions and improve customer satisfaction, ultimately driving loyalty and revenue growth. New technologies in customer experience integration are vital for success.
10.4. Predictive Capabilities Evolution
Predictive capabilities have evolved significantly over the past few years, driven by advancements in technology and data analytics. Organizations are increasingly leveraging predictive analytics tools to enhance decision-making processes and improve operational efficiency.
- Enhanced Data Collection: The rise of IoT devices and big data has enabled organizations to collect vast amounts of data from various sources. This data serves as the foundation for predictive models, allowing Rapid Innovation to assist clients in harnessing this data for actionable insights.
- Machine Learning Integration: Machine learning algorithms are now widely used to analyze historical data and identify patterns. These patterns help in forecasting future trends and behaviors, enabling Rapid Innovation to develop tailored solutions that enhance predictive accuracy for our clients.
- Real-time Analytics: The ability to analyze data in real-time allows businesses to make timely decisions. This is particularly important in industries like finance and healthcare, where timely interventions can save costs and lives. Rapid Innovation empowers clients with real-time analytics solutions that drive efficiency and responsiveness.
- Improved Accuracy: As predictive models become more sophisticated, their accuracy improves. Organizations can now predict customer behavior, market trends, and operational risks with greater precision. Rapid Innovation leverages advanced algorithms to enhance the predictive capabilities of our clients, leading to better strategic decisions.
- User-friendly Tools: The development of user-friendly analytics tools has democratized access to predictive capabilities. Non-technical users can now leverage these tools to gain insights without needing extensive data science knowledge. Rapid Innovation focuses on creating intuitive interfaces that allow clients to easily interact with their data, including predictive analytics solutions and predictive marketing analytics.
10.5. Industry Transformation Outlook
The outlook for industry transformation is promising, as organizations continue to adapt to changing market dynamics and technological advancements. Several key trends are shaping this transformation:
- Digital Transformation: Companies are increasingly adopting digital technologies to streamline operations and enhance customer experiences. This includes the use of cloud computing, AI, and automation, areas where Rapid Innovation excels in providing comprehensive solutions, such as cloud-based predictive analytics and predictive analytics as a service.
- Sustainability Focus: There is a growing emphasis on sustainability across industries. Organizations are integrating sustainable practices into their operations to meet regulatory requirements and consumer expectations. Rapid Innovation supports clients in implementing sustainable technologies that align with their business goals.
- Workforce Evolution: The workforce is evolving with the integration of technology. Upskilling and reskilling employees are becoming essential to keep pace with industry changes. Rapid Innovation offers training and development programs to ensure that client teams are equipped with the necessary skills, including training on HR predictive analytics tools.
- Customer-Centric Approaches: Businesses are shifting towards customer-centric models, utilizing data analytics to understand customer preferences and tailor offerings accordingly. Rapid Innovation helps clients develop data-driven strategies that enhance customer engagement and satisfaction, leveraging tools like predictive sales analytics and predictive marketing automation.
- Collaboration and Partnerships: Companies are forming strategic partnerships to leverage complementary strengths. This collaboration fosters innovation and accelerates industry transformation, a principle that Rapid Innovation embodies in our approach to client relationships.
11. Implementation Roadmap

‍
An effective implementation roadmap is crucial for organizations looking to harness predictive capabilities and drive industry transformation. The following steps outline a structured approach:
- Define Objectives: Clearly outline the goals of implementing predictive analytics and industry transformation initiatives. This could include improving customer satisfaction, reducing costs, or enhancing operational efficiency.
- Assess Current Capabilities: Evaluate existing data infrastructure, analytics tools, and workforce skills. Identify gaps that need to be addressed to achieve the defined objectives.
- Develop a Data Strategy: Create a comprehensive data strategy that includes data collection, storage, and analysis. Ensure that data governance practices are in place to maintain data quality and security.
- Invest in Technology: Select the right tools and technologies that align with the organization’s goals. This may involve investing in advanced analytics platforms, machine learning tools, and cloud solutions, including options like SAP predictive analytics and AWS predictive analytics tools.
- Train and Upskill Employees: Provide training programs to equip employees with the necessary skills to utilize predictive analytics effectively. Encourage a culture of continuous learning and innovation.
- Monitor and Evaluate: Establish key performance indicators (KPIs) to measure the success of the implementation. Regularly review progress and make adjustments as needed to stay on track.
- Foster a Collaborative Environment: Encourage collaboration across departments to ensure that insights from predictive analytics are shared and utilized effectively. This can enhance decision-making and drive innovation.
By following this roadmap, organizations can successfully implement predictive capabilities and navigate the ongoing transformation in their respective industries, with Rapid Innovation as a trusted partner in their journey.
11.1. Assessment Phase
The assessment phase is a critical step in any project, particularly in software development and project management. This phase involves evaluating the current situation, identifying needs, and determining the feasibility of the project.
- Identify stakeholders: Engage with all relevant parties, including clients, team members, and end-users, to gather insights and expectations. At Rapid Innovation, we prioritize stakeholder engagement to ensure that all voices are heard, which leads to more tailored solutions.
- Define objectives: Clearly outline the goals of the project, ensuring they align with stakeholder needs and organizational strategy. Our expertise in AI and Blockchain allows us to set objectives that leverage these technologies for maximum impact.
- Conduct a SWOT analysis: Assess the project's strengths, weaknesses, opportunities, and threats to understand its potential impact. This analysis helps us identify how AI and Blockchain can enhance project outcomes.
- Gather requirements: Collect detailed requirements through interviews, surveys, and workshops to ensure a comprehensive understanding of what is needed. Our team utilizes advanced data analytics to refine these requirements, ensuring they are actionable and aligned with business goals.
- Analyze risks: Identify potential risks and challenges that could affect the project timeline, budget, or quality. We employ predictive analytics to foresee risks and develop mitigation strategies, enhancing project resilience. This includes conducting a project risk assessment and utilizing risk analysis in project management to ensure comprehensive coverage.
- Evaluate resources: Assess the availability of resources, including personnel, technology, and budget, to determine if the project is feasible. Our extensive network in the AI and Blockchain domains ensures that we can mobilize the right resources efficiently. We also consider the project management maturity model to gauge our readiness.
This phase sets the foundation for the entire project, ensuring that all stakeholders are aligned and that the project has a clear direction.
11.2. Planning and Design
Once the assessment phase is complete, the planning and design phase begins. This stage is crucial for outlining how the project will be executed and what the final product will look like.
- Develop a project plan: Create a detailed project plan that includes timelines, milestones, and deliverables. This plan serves as a roadmap for the project, ensuring that AI and Blockchain components are integrated seamlessly.
- Design architecture: Establish the technical architecture of the project, including system components, data flow, and integration points. Our expertise in Blockchain architecture ensures that your project is built on a secure and scalable foundation.
- Create wireframes and prototypes: Develop visual representations of the product to help stakeholders visualize the end result and provide feedback early in the process. This iterative design process is crucial for aligning expectations and refining functionalities.
- Allocate resources: Assign team members to specific tasks based on their skills and expertise, ensuring that the right people are in the right roles. Our team is composed of specialists in AI and Blockchain, ensuring that your project benefits from top-tier talent.
- Set a budget: Estimate costs associated with the project, including labor, materials, and technology, to ensure financial feasibility. We leverage our industry insights to provide accurate budgeting that maximizes ROI.
- Establish communication protocols: Define how team members will communicate throughout the project, including regular meetings and reporting structures. Effective communication is key to project success, and we implement best practices to keep all stakeholders informed.
Effective planning and design are essential for minimizing risks and ensuring that the project stays on track. This includes conducting a project management risk assessment and utilizing a PMO maturity model to guide our processes.
11.3. Development Process
The development process is where the actual creation of the product takes place. This phase involves coding, testing, and refining the product based on the requirements gathered in earlier phases.
- Follow an agile methodology: Implement an agile approach to development, allowing for flexibility and iterative progress. This enables teams to adapt to changes and feedback quickly, ensuring that the final product is aligned with user needs.
- Code development: Write the code according to the specifications outlined in the planning phase. Ensure that coding standards and best practices are followed to maintain quality. Our developers are well-versed in the latest technologies, ensuring high-quality code.
- Continuous integration: Use continuous integration tools to automate the testing and deployment process, ensuring that code changes are integrated smoothly and efficiently. This practice reduces time-to-market and enhances product reliability.
- Testing: Conduct various types of testing, including unit testing, integration testing, and user acceptance testing, to identify and fix issues before the product is released. Our rigorous testing protocols ensure that your product meets the highest standards of quality.
- Gather feedback: Involve stakeholders in the testing process to gather feedback and make necessary adjustments. This ensures that the final product meets user expectations and business objectives.
- Documentation: Maintain thorough documentation throughout the development process, including code comments, user manuals, and design specifications. This aids in future maintenance and updates, ensuring long-term project success.
The development process is vital for transforming ideas into a functional product, and careful attention to detail during this phase can significantly impact the project's success. At Rapid Innovation, we are committed to delivering solutions that not only meet but exceed client expectations, driving greater ROI through the strategic use of AI and Blockchain technologies. We also conduct performance evaluation in project management to ensure continuous improvement.
11.4. Testing and Validation
Testing and validation are critical phases in the software development lifecycle. They ensure that the application meets the specified requirements and functions correctly in various scenarios, ultimately leading to greater ROI for businesses.
- Types of Testing: Â
- Unit Testing: Focuses on individual components to ensure they work as intended, allowing for early detection of issues that could escalate costs if left unresolved.
- Integration Testing: Checks the interaction between different modules to identify interface defects, ensuring seamless functionality across the application.
- System Testing: Validates the complete and integrated software product to ensure compliance with requirements, reducing the risk of post-deployment failures.
- User Acceptance Testing (UAT): Involves end-users to confirm that the software meets their needs and expectations, enhancing user satisfaction and adoption rates.
- Validation Techniques: Â
- Manual Testing: Testers execute test cases manually to identify defects, providing a thorough examination of the application.
- Automated Testing: Utilizes scripts and tools to perform tests, increasing efficiency and coverage, which is particularly beneficial for large-scale applications.
- Performance Testing: Assesses the application's responsiveness, stability, and scalability under various conditions, ensuring it can handle user demands effectively.
- Importance of Testing: Â
- Reduces the risk of defects in production, which can lead to costly downtime and loss of customer trust.
- Enhances user satisfaction by ensuring a reliable product, ultimately driving customer loyalty and repeat business.
- Saves costs associated with post-deployment fixes, allowing organizations to allocate resources more effectively.
- Best Practices: Â
- Develop a comprehensive test plan outlining objectives, resources, and timelines to ensure all aspects of the application are covered, including deployment testing in software testing.
- Use version control to manage test scripts and results, facilitating collaboration and tracking changes.
- Continuously integrate testing into the development process to catch issues early, reducing the overall cost of development.
11.5. Deployment Strategy
A well-defined deployment strategy is essential for the successful release of software applications. It outlines how the software will be delivered to users and ensures a smooth transition from development to production, maximizing the return on investment.
- Deployment Models: Â
- Big Bang Deployment: All components are deployed at once, suitable for smaller projects where rapid delivery is essential.
- Phased Deployment: Gradual rollout of features or components, allowing for easier troubleshooting and minimizing disruption to users.
- Continuous Deployment: Automated deployment process that releases updates frequently, ensuring users always have the latest version and reducing the time to market.
- Key Considerations: Â
- Environment Setup: Ensure that the production environment mirrors the testing environment to avoid discrepancies that could lead to failures.
- Rollback Plan: Prepare a strategy to revert to the previous version in case of deployment failures, safeguarding against potential losses.
- User Communication: Inform users about the deployment schedule and any expected downtime, fostering transparency and trust.
- Tools and Technologies: Â
- CI/CD Tools: Continuous Integration and Continuous Deployment tools like Jenkins, GitLab CI, or CircleCI streamline the deployment process, enhancing efficiency.
- Containerization: Technologies like Docker and Kubernetes facilitate consistent deployment across different environments, reducing compatibility issues.
- Best Practices: Â
- Conduct thorough testing before deployment to minimize risks and ensure a smooth launch, including testing software before deployment.
- Monitor the application post-deployment to quickly identify and resolve issues, maintaining user satisfaction.
- Gather user feedback to inform future updates and improvements, aligning the product with user needs.
11.6. Maintenance and Updates
Maintenance and updates are vital for the longevity and performance of software applications. They ensure that the software remains functional, secure, and relevant to users' needs, ultimately contributing to sustained ROI.
- Types of Maintenance: Â
- Corrective Maintenance: Fixes defects and issues identified after deployment, ensuring the application remains reliable.
- Adaptive Maintenance: Modifies the software to accommodate changes in the environment or technology, keeping it up-to-date with industry standards.
- Perfective Maintenance: Enhances the software by adding new features or improving existing functionalities, increasing user engagement.
- Importance of Regular Updates: Â
- Security: Regular updates patch vulnerabilities, protecting the application from threats and safeguarding user data.
- Performance: Updates can optimize the software, improving speed and efficiency, which enhances user experience.
- User Experience: New features and enhancements keep the application engaging and relevant, driving user retention.
- Maintenance Strategies: Â
- Scheduled Maintenance: Regularly planned updates and checks to ensure the software remains in good condition, preventing unexpected failures.
- On-Demand Maintenance: Addressing issues as they arise, providing immediate fixes to critical problems, thus minimizing downtime.
- Best Practices: Â
- Document all changes and updates for future reference and compliance, ensuring accountability and traceability.
- Use monitoring tools to track application performance and user behavior, enabling proactive maintenance.
- Engage with users to gather feedback on potential improvements and issues, fostering a user-centric approach to development.
By implementing effective testing, deployment, and maintenance strategies, including canary testing software and continuous delivery reliable software releases, organizations can ensure their software applications are robust, user-friendly, and secure, ultimately achieving their business goals efficiently and effectively. Rapid Innovation is committed to guiding clients through these processes, leveraging our expertise in AI and Blockchain to enhance their operational efficiency and drive greater ROI.