Table Of Contents
Category
FinTech
Healthcare & Medicine
Legal
CRM
Security
Blockchain
1. Introduction to AI-Powered Credit Limit Systems
Artificial Intelligence (AI) is revolutionizing the financial sector, particularly in the area of ai credit limit systems. Traditional methods of determining credit limits often rely on static algorithms and historical data, which can lead to inefficiencies and inaccuracies. AI-powered systems, on the other hand, leverage advanced machine learning techniques to analyze vast amounts of data in real-time, enabling more intelligent financial decision-making.
AI systems can process data from various sources, including:
- Credit scores
- Transaction histories
- Social media activity
- Economic indicators
These systems utilize predictive analytics to forecast a borrower’s creditworthiness, allowing lenders to make informed decisions about credit limits. The integration of AI in credit limit systems offers several advantages, such as:
- Enhanced accuracy in assessing risk
- Personalized credit limit recommendations
- Improved customer experience through faster decision-making
At Rapid Innovation, we specialize in developing and implementing ai credit limit systems tailored to meet the unique needs of financial institutions. Our expertise ensures that these systems are not only efficient but also scalable, allowing our clients to adapt to changing market conditions seamlessly.
AI-powered credit limit systems are designed to adapt and learn from new data, ensuring that recommendations remain relevant and accurate over time. This adaptability is crucial in a rapidly changing financial landscape, where consumer behavior and economic conditions can shift unexpectedly.
Moreover, these systems can help financial institutions reduce default rates by identifying potential risks before they materialize. By analyzing patterns and trends, AI can flag accounts that may require closer monitoring or intervention.
In summary, ai credit limit systems represent a significant advancement in the way financial institutions assess and manage credit risk. By harnessing the power of AI, lenders can make smarter, more informed decisions that benefit both their business and their customers. At Rapid Innovation, we are committed to helping our clients achieve greater ROI through innovative AI solutions that drive efficiency and effectiveness in their operations. For more information, visit our article on AI-based credit scoring use cases, types, and benefits.
Refer to the image for a visual representation of AI-powered credit limit systems:

1.1. Evolution of Credit Assessment
Credit assessment has undergone significant changes over the years, evolving from traditional methods to more sophisticated techniques. Early credit assessments relied heavily on personal relationships and subjective judgment, with lenders often basing decisions on character references and face-to-face interactions. The introduction of credit scoring systems in the late 20th century marked a pivotal shift, utilizing statistical models to evaluate creditworthiness based on historical data, which made the process more objective. The rise of credit bureaus, such as Experian and TransUnion, provided lenders with access to standardized credit reports, further streamlining the assessment process. In recent years, alternative data sources, including social media activity and payment histories for utilities, have emerged, allowing lenders to assess creditworthiness for individuals with limited credit histories. Additionally, the integration of machine learning algorithms has enhanced predictive accuracy, enabling lenders to make more informed decisions based on a broader range of data points. At Rapid Innovation, we leverage these advancements to develop tailored credit assessment AI solutions that enhance credit assessment processes, ultimately driving greater ROI for our clients. You can learn more about the role of AI in decision-making in credit scoring and lending.
1.2. Role of AI in Modern Credit Management
Artificial Intelligence (AI) is transforming credit management by automating processes and improving decision-making. AI algorithms analyze vast amounts of data quickly, identifying patterns that human analysts might miss, which enhances risk assessment and fraud detection. Machine learning models can adapt over time, learning from new data to refine credit scoring models and improve accuracy. AI-driven chatbots and virtual assistants streamline customer service by providing instant responses to inquiries and improving the overall customer experience. Predictive analytics powered by AI can forecast borrower behavior, helping lenders to proactively manage risk and tailor their offerings. Furthermore, the use of AI in credit management promotes financial inclusion by enabling lenders to assess creditworthiness for underserved populations who may lack traditional credit histories. Rapid Innovation specializes in implementing credit assessment AI-driven solutions that not only optimize credit management but also significantly increase operational efficiency and profitability for our clients.
1.3. Regulatory Framework and Compliance
The regulatory landscape surrounding credit assessment and management is crucial for maintaining consumer protection and market stability. Regulations such as the Fair Credit Reporting Act (FCRA) in the United States govern how credit information is collected, shared, and used, ensuring transparency and accuracy in credit reporting. Compliance with the General Data Protection Regulation (GDPR) in Europe mandates that lenders handle personal data responsibly, giving consumers rights over their information. Regulatory bodies, such as the Consumer Financial Protection Bureau (CFPB), oversee lending practices to prevent discrimination and ensure fair treatment of all borrowers. Lenders must also adhere to anti-money laundering (AML) and know your customer (KYC) regulations, which require thorough verification of borrower identities and sources of funds. As technology evolves, regulators are increasingly focusing on the implications of AI in credit assessment, emphasizing the need for ethical AI practices and accountability in automated decision-making processes. At Rapid Innovation, we guide our clients through the complexities of regulatory compliance, ensuring that their AI and blockchain solutions not only meet legal standards but also enhance their competitive edge in the market.
Refer to the image for a visual representation of the evolution of credit assessment.
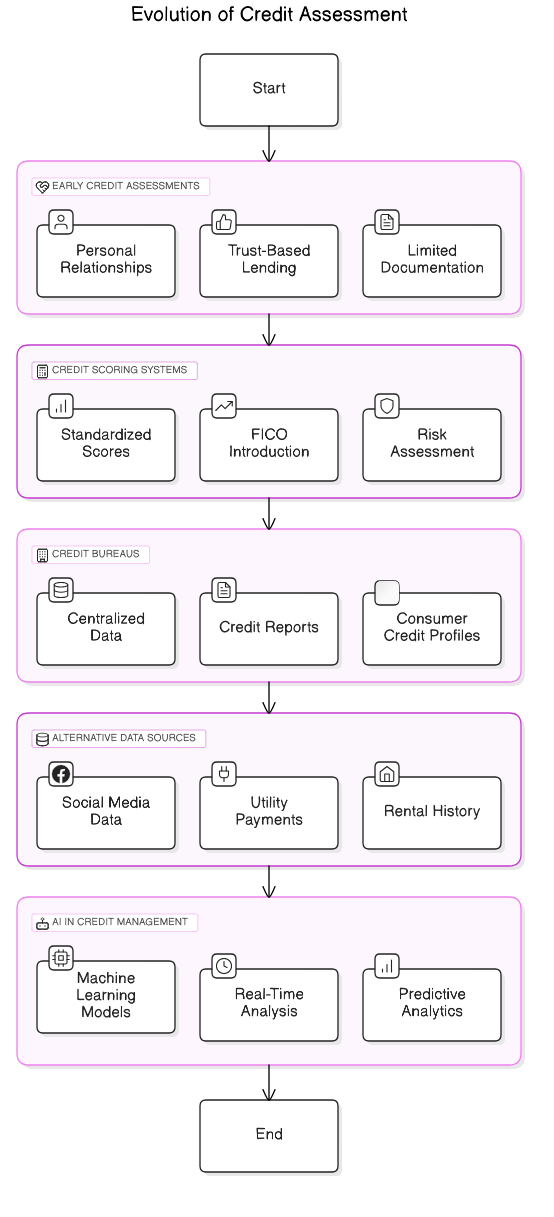
1.4. Current Industry Challenges
The industry faces a myriad of challenges that hinder growth and efficiency. Understanding these challenges is crucial for stakeholders aiming to navigate the complexities of the market.
- Data Overload: Organizations are inundated with vast amounts of data, making it difficult to extract actionable insights. According to a report, 2.5 quintillion bytes of data are created every day, and this number continues to grow.
- Skill Shortages: There is a significant gap in skilled professionals who can analyze and interpret data effectively. Many companies struggle to find talent proficient in data science, machine learning, and AI technologies.
- Integration Issues: Many businesses operate with legacy systems that are not compatible with modern technologies. This lack of integration can lead to inefficiencies and increased operational costs.
- Regulatory Compliance: As data privacy laws become more stringent, companies must navigate complex regulations. Non-compliance can result in hefty fines and damage to reputation.
- Cybersecurity Threats: With the rise of digital transformation, the risk of cyberattacks has increased. Organizations must invest in robust security measures to protect sensitive data.
- Customer Expectations: Today's consumers demand personalized experiences and instant responses. Meeting these expectations requires advanced technologies and strategies, including aidriven solutions.
1.5. The Promise of AI-Driven Solutions
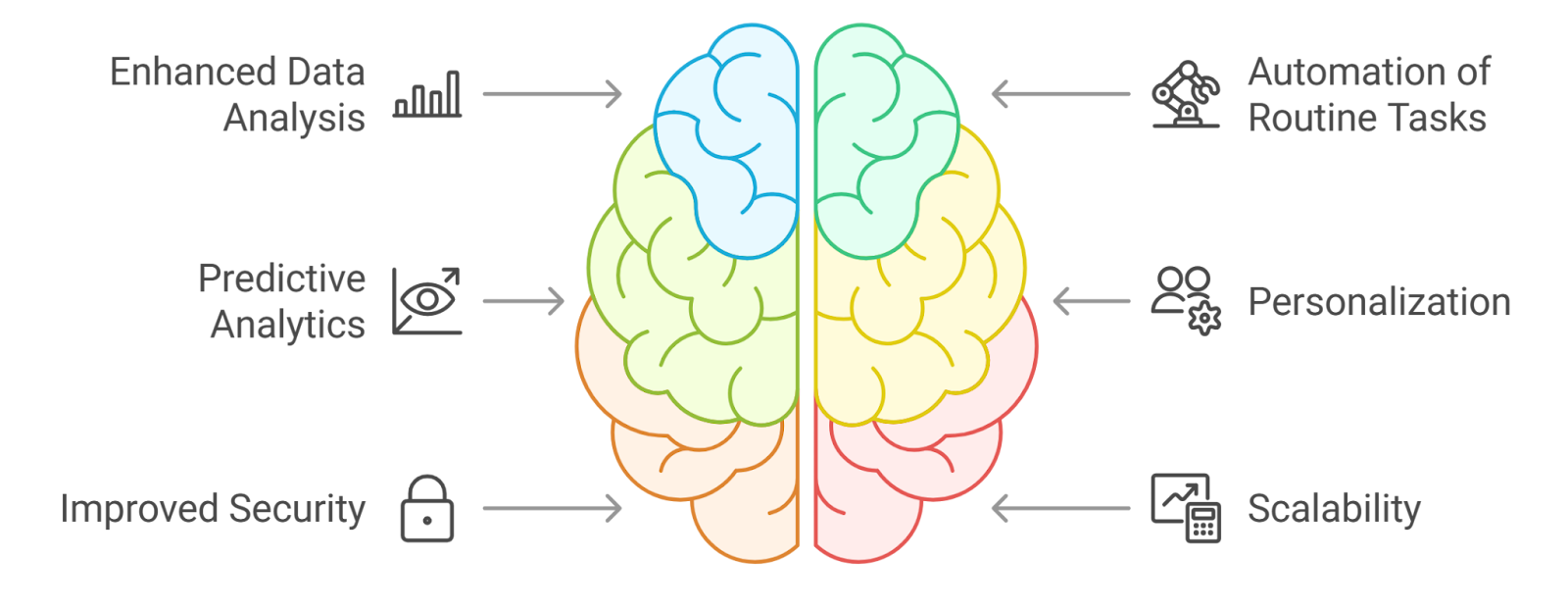
‍
AI-driven solutions offer transformative potential for addressing the challenges faced by industries today. By leveraging artificial intelligence, organizations can enhance efficiency, improve decision-making, and drive innovation.
- Enhanced Data Analysis: AI can process and analyze large datasets quickly, uncovering patterns and insights that would be impossible for humans to detect. This capability allows businesses to make data-driven decisions.
- Automation of Routine Tasks: AI technologies can automate repetitive tasks, freeing up human resources for more strategic initiatives. This leads to increased productivity and reduced operational costs.
- Predictive Analytics: AI can forecast trends and behaviors, enabling companies to anticipate customer needs and market shifts. This proactive approach can enhance competitiveness.
- Personalization: AI algorithms can analyze customer data to deliver personalized experiences, improving customer satisfaction and loyalty. Tailored recommendations can significantly boost sales.
- Improved Security: AI can enhance cybersecurity measures by identifying threats in real-time and responding to them more effectively. This proactive stance helps protect sensitive information.
- Scalability: AI solutions can easily scale with business growth, allowing organizations to adapt to changing demands without significant additional investment.
2. Core Technologies and Components
Understanding the core technologies and components that drive AI solutions is essential for organizations looking to implement these innovations effectively.
- Machine Learning (ML): A subset of AI, machine learning enables systems to learn from data and improve over time without explicit programming. It is widely used in predictive analytics and recommendation systems.
- Natural Language Processing (NLP): NLP allows machines to understand and interpret human language. This technology powers chatbots, virtual assistants, and sentiment analysis tools, enhancing customer interactions.
- Computer Vision: This technology enables machines to interpret and process visual information from the world. Applications include facial recognition, object detection, and autonomous vehicles.
- Robotic Process Automation (RPA): RPA automates rule-based tasks across various applications, streamlining workflows and reducing human error. It is particularly useful in finance, HR, and customer service.
- Big Data Technologies: Tools like Hadoop and Spark facilitate the storage and processing of large datasets. These technologies are crucial for managing the data that fuels AI algorithms.
- Cloud Computing: Cloud platforms provide the necessary infrastructure for deploying AI solutions at scale. They offer flexibility, cost-effectiveness, and accessibility, enabling organizations to leverage AI without heavy upfront investments.
- Internet of Things (IoT): IoT devices generate vast amounts of data that can be analyzed using AI. This integration allows for real-time monitoring and decision-making in various sectors, including manufacturing and healthcare.
- Deep Learning: A subset of machine learning, deep learning uses neural networks to analyze complex data patterns. It is particularly effective in image and speech recognition tasks.
- Data Management Tools: Effective data management is critical for AI success. Tools that ensure data quality, governance, and integration are essential for building reliable AI models.
- APIs and Integration Frameworks: Application Programming Interfaces (APIs) facilitate the integration of AI solutions with existing systems. They enable seamless communication between different software applications, enhancing functionality.
By understanding these core technologies and components, organizations can better position themselves to harness the power of AI and address the challenges they face in the current landscape. Rapid Innovation is committed to guiding clients through these complexities, ensuring they leverage AI and blockchain technologies, including aidriven solutions, to achieve greater ROI and operational efficiency.
Refer to the image for a visual representation of the current industry challenges and the promise of AI-driven solutions.

2.1. Machine Learning Foundations
Machine learning (ML) is a subset of artificial intelligence (AI) that focuses on the development of algorithms that allow computers to learn from and make predictions based on data. The foundations of machine learning are built on various mathematical and statistical principles, enabling machines to improve their performance on tasks through experience. Understanding these foundations is crucial for anyone looking to delve into the field of machine learning.
- Core concepts include:
- Algorithms: Step-by-step procedures for calculations.
- Data: The information used to train models.
- Features: Individual measurable properties or characteristics of the data.
- Labels: The output variable in supervised learning.
2.1.1. Supervised Learning Models
Supervised learning is a type of machine learning where the model is trained on a labeled dataset. This means that the input data is paired with the correct output, allowing the model to learn the relationship between the two. Supervised learning is widely used for classification and regression tasks.
- Key characteristics of supervised learning: Â
- Labeled Data: Each training example is paired with an output label.
- Training Phase: The model learns from the labeled data to make predictions.
- Evaluation: The model's performance is assessed using a separate test dataset.
- Common algorithms include: Â
- Linear Regression: Used for predicting continuous values, often implemented with scikit learn linear regression.
- Logistic Regression: Used for binary classification tasks, such as logistic regression model machine learning.
- Decision Trees: A flowchart-like structure for decision-making, also available in decision tree scikit learn.
- Support Vector Machines (SVM): Effective for high-dimensional spaces.
- Neural Networks: Inspired by the human brain, used for complex tasks, including neural network machine learning.
- Applications of supervised learning: Â
- Email filtering: Classifying emails as spam or not spam.
- Image recognition: Identifying objects within images.
- Medical diagnosis: Predicting diseases based on patient data.
At Rapid Innovation, we leverage supervised learning models, including classification random forest and random forest scikit learn, to help clients enhance their operational efficiency. For instance, by implementing advanced image recognition algorithms, we have assisted retail clients in automating inventory management, leading to significant cost savings and improved accuracy.
2.1.2. Unsupervised Learning Approaches
Unsupervised learning, in contrast to supervised learning, deals with unlabeled data. The model attempts to learn the underlying structure of the data without any explicit guidance on what the output should be. This approach is particularly useful for exploratory data analysis and pattern recognition.
- Key characteristics of unsupervised learning: Â
- Unlabeled Data: The model works with data that does not have predefined labels.
- Pattern Discovery: The focus is on finding hidden patterns or intrinsic structures.
- Clustering and Association: Common tasks in unsupervised learning.
- Common algorithms include: Â
- K-Means Clustering: Groups data into K distinct clusters based on similarity.
- Hierarchical Clustering: Builds a tree of clusters based on data similarity.
- Principal Component Analysis (PCA): Reduces dimensionality while preserving variance.
- t-Distributed Stochastic Neighbor Embedding (t-SNE): Visualizes high-dimensional data.
- Applications of unsupervised learning: Â
- Customer segmentation: Grouping customers based on purchasing behavior, often enhanced by ensemble learning in machine learning.
- Anomaly detection: Identifying unusual patterns in data, such as fraud.
- Market basket analysis: Discovering associations between products purchased together.
At Rapid Innovation, we utilize unsupervised learning techniques, including restricted boltzmann machine and boltzmann machine, to provide our clients with deep insights into customer behavior. For example, through customer segmentation, we have enabled businesses to tailor their marketing strategies, resulting in increased engagement and higher return on investment.
Understanding both supervised and unsupervised learning is essential for leveraging machine learning effectively. Each approach has its unique strengths and applications, making them suitable for different types of problems in various industries. By partnering with Rapid Innovation, clients can harness the power of machine learning, including deep learning models and probabilistic machine learning, to achieve their business goals efficiently and effectively. Additionally, our expertise in adaptive AI development allows us to create tailored solutions that meet the specific needs of our clients.
Refer to the image for a visual representation of the concepts discussed in 2.1 Machine Learning Foundations:
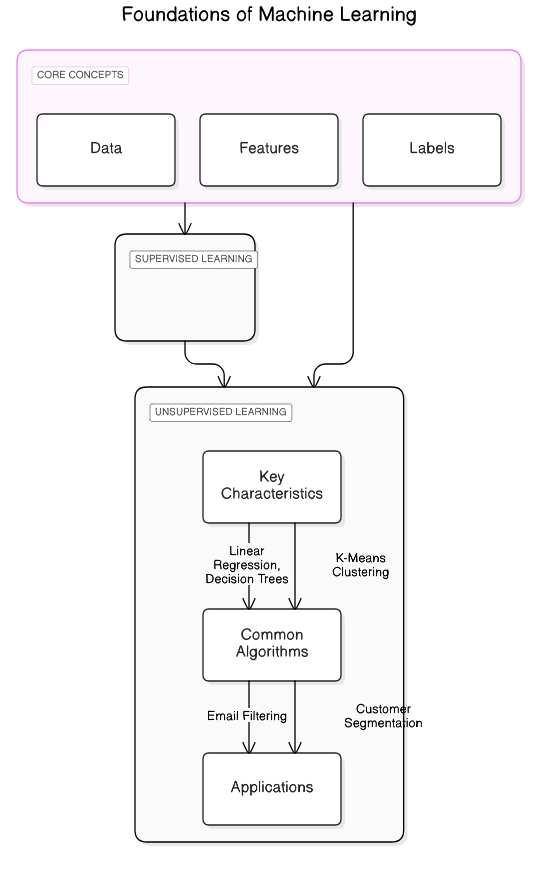
2.1.3. Reinforcement Learning Applications
Reinforcement Learning (RL) is a subset of machine learning where an agent learns to make decisions by taking actions in an environment to maximize cumulative rewards. This approach has gained traction across various industries due to its ability to solve complex problems efficiently and effectively.
- Gaming: RL has revolutionized the gaming industry. Algorithms like Deep Q-Networks (DQN) have been used to train AI agents that can outperform human players in games like Go and Dota 2. These systems learn strategies through trial and error, refining their approach based on the outcomes of their actions. Rapid Innovation can assist gaming companies in implementing RL algorithms to enhance player engagement and retention, ultimately driving greater ROI. Multi agent reinforcement learning techniques can further enhance the gaming experience by allowing multiple agents to interact and compete.
- Robotics: In robotics, RL is employed to teach robots how to perform tasks such as walking, grasping objects, or navigating environments. By simulating various scenarios, robots can learn optimal movements and actions, improving their efficiency and adaptability in real-world applications. Rapid Innovation can help organizations develop custom RL solutions that optimize robotic processes, leading to cost savings and improved operational efficiency. Applications of deep reinforcement learning in robotics can lead to more sophisticated and capable robotic systems.
- Finance: In the financial sector, RL is used for algorithmic trading, portfolio management, and risk assessment. By analyzing market conditions and historical data, RL models can make informed decisions that maximize returns while minimizing risks. Rapid Innovation can provide tailored RL models that enhance trading strategies, resulting in increased profitability for financial institutions. Reinforcement learning use cases in finance include fraud detection reinforcement learning, which can help identify and mitigate fraudulent activities.
- Healthcare: RL applications in healthcare include personalized treatment plans and optimizing resource allocation in hospitals. By analyzing patient data and treatment outcomes, RL can help in making better clinical decisions. Rapid Innovation can collaborate with healthcare providers to implement RL solutions that improve patient outcomes and reduce operational costs. Reinforcement learning in computer vision can also be applied to analyze medical images for better diagnosis.
- Autonomous Vehicles: RL plays a crucial role in the development of self-driving cars. These vehicles learn to navigate complex environments by continuously adjusting their actions based on feedback from their surroundings. Rapid Innovation can support automotive companies in developing advanced RL systems that enhance the safety and efficiency of autonomous driving technologies. Learning to communicate with deep multi agent reinforcement learning can further improve the coordination between multiple autonomous vehicles.
2.2. Data Processing Systems
Data processing systems are essential for managing, analyzing, and interpreting large volumes of data. These systems enable organizations to derive insights and make data-driven decisions.
- Batch Processing: This method involves processing large datasets in batches. It is efficient for tasks that do not require real-time analysis. Technologies like Apache Hadoop and Apache Spark are commonly used for batch processing, allowing for the handling of vast amounts of data. Rapid Innovation can help businesses implement robust batch processing solutions that streamline data management and analysis.
- Stream Processing: Unlike batch processing, stream processing deals with real-time data. It processes data as it arrives, making it suitable for applications like fraud detection and real-time analytics. Tools such as Apache Kafka and Apache Flink are popular for stream processing. Rapid Innovation can assist organizations in deploying effective stream processing systems that enhance decision-making and responsiveness.
- Data Warehousing: Data warehousing systems consolidate data from multiple sources into a single repository. This allows for efficient querying and reporting. Solutions like Amazon Redshift and Google BigQuery are widely used for data warehousing, enabling businesses to analyze historical data effectively. Rapid Innovation can guide companies in designing and implementing data warehousing solutions that improve data accessibility and insights.
- ETL Processes: Extract, Transform, Load (ETL) processes are crucial for data integration. They involve extracting data from various sources, transforming it into a suitable format, and loading it into a data warehouse. Tools like Talend and Informatica facilitate ETL processes, ensuring data quality and consistency. Rapid Innovation can optimize ETL workflows, ensuring seamless data integration and enhanced data quality.
- Data Lakes: Data lakes store vast amounts of unstructured and structured data. They provide flexibility for data scientists and analysts to explore and analyze data without predefined schemas. Technologies like Amazon S3 and Azure Data Lake Storage are commonly used for building data lakes. Rapid Innovation can help organizations establish data lakes that support advanced analytics and machine learning initiatives.
2.3. Natural Language Processing
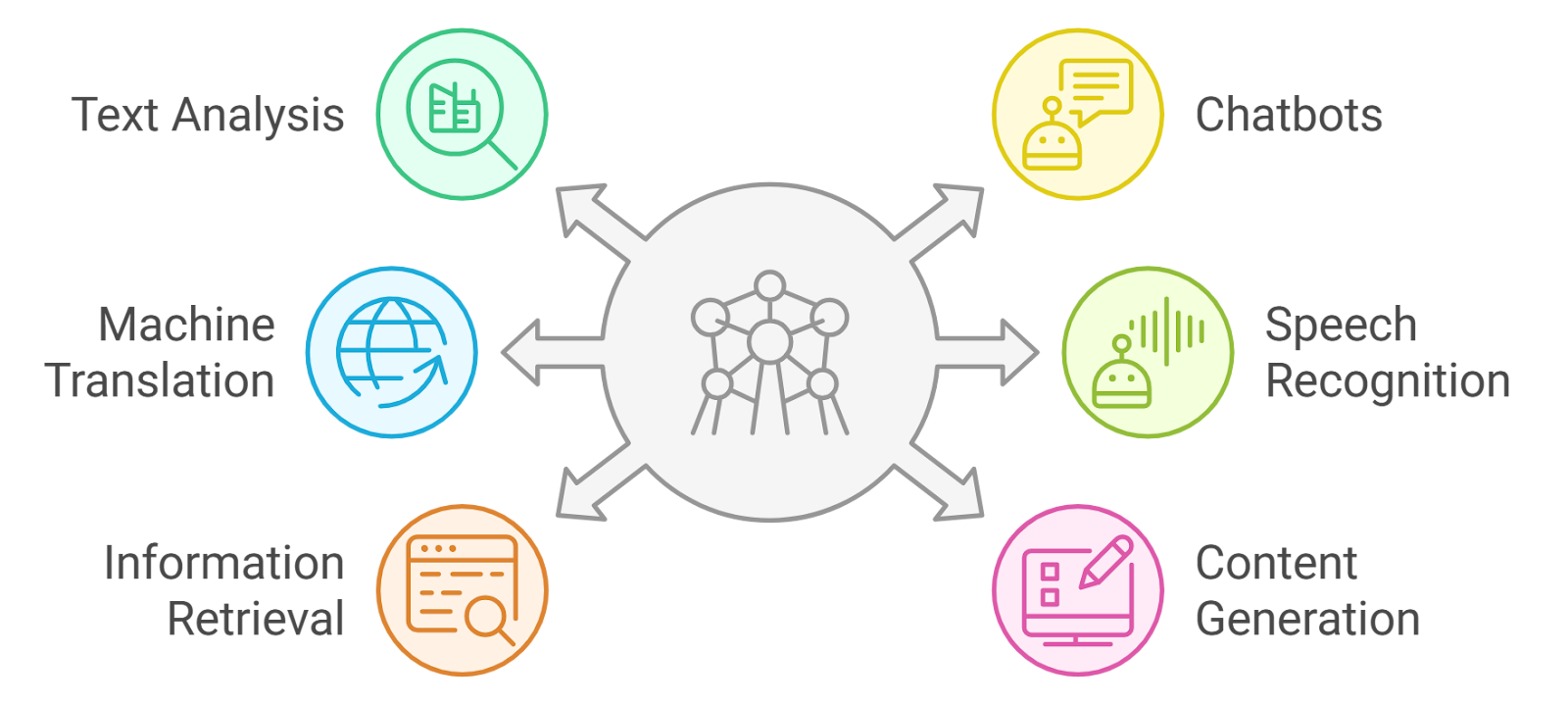
‍
Natural Language Processing (NLP) is a field of artificial intelligence that focuses on the interaction between computers and human language. NLP enables machines to understand, interpret, and respond to human language in a meaningful way.
- Text Analysis: NLP techniques are used for text analysis, which includes sentiment analysis, topic modeling, and keyword extraction. Businesses leverage these techniques to gain insights from customer feedback, social media, and reviews. Rapid Innovation can implement NLP solutions that enhance customer understanding and drive marketing strategies.
- Chatbots and Virtual Assistants: NLP powers chatbots and virtual assistants like Siri and Alexa. These systems use NLP to understand user queries and provide relevant responses, enhancing customer service and user experience. Rapid Innovation can develop custom chatbots that improve customer engagement and operational efficiency.
- Machine Translation: NLP is integral to machine translation services like Google Translate. These systems analyze and translate text from one language to another, making communication across languages more accessible. Rapid Innovation can assist businesses in implementing NLP-driven translation solutions that expand their global reach.
- Speech Recognition: NLP enables speech recognition technologies, allowing users to interact with devices using voice commands. Applications range from voice-activated assistants to transcription services, improving accessibility and convenience. Rapid Innovation can help organizations integrate speech recognition capabilities that enhance user experience and accessibility.
- Information Retrieval: NLP enhances information retrieval systems, enabling users to search and retrieve relevant information from large datasets. Search engines like Google utilize NLP algorithms to improve search accuracy and relevance. Rapid Innovation can optimize information retrieval systems, ensuring users can access critical data efficiently.
- Content Generation: NLP is also used for content generation, where algorithms can create human-like text. This application is useful in marketing, journalism, and content creation, allowing for automated report generation and personalized content delivery. Rapid Innovation can develop NLP solutions that streamline content creation processes, saving time and resources while enhancing quality.
2.4. Real-time Decision Engines
Real-time decision engines are sophisticated systems designed to analyze data and make decisions instantly. These engines leverage advanced algorithms and machine learning techniques to process vast amounts of information in real-time, enabling organizations to respond quickly to changing conditions.
- Key Features: Â
- Instantaneous Analysis: Capable of processing data as it arrives, allowing for immediate decision-making.
- Adaptive Learning: Continuously learns from new data inputs, improving accuracy over time.
- Scalability: Can handle increasing volumes of data without compromising performance.
- Applications: Â
- Fraud Detection: Financial institutions use real-time decision engines to identify and prevent fraudulent transactions as they occur. Rapid Innovation can implement tailored solutions that enhance detection rates and reduce false positives, ultimately protecting client assets and improving customer trust.
- Dynamic Pricing: E-commerce platforms adjust prices based on real-time market conditions and consumer behavior. Our expertise in AI allows us to develop algorithms that optimize pricing strategies, maximizing revenue while remaining competitive.
- Personalized Marketing: Retailers deliver tailored promotions to customers based on their current shopping behavior. By leveraging machine learning, Rapid Innovation helps clients create personalized marketing campaigns that significantly increase conversion rates.
- Benefits: Â
- Enhanced Agility: Organizations can pivot quickly in response to market changes, allowing them to seize new opportunities and mitigate risks effectively.
- Improved Customer Experience: Real-time insights lead to more relevant interactions with customers, fostering loyalty and satisfaction.
- Increased Efficiency: Automating decision-making processes reduces the time and resources spent on manual analysis, allowing teams to focus on strategic initiatives.
2.5. Integration Frameworks
Integration frameworks are essential for connecting various systems and applications within an organization. They facilitate seamless data exchange and communication, ensuring that different components work together effectively.
- Key Features: Â
- Interoperability: Supports multiple protocols and standards, allowing diverse systems to communicate.
- Modularity: Components can be added or removed without disrupting the entire system.
- Centralized Management: Provides a single point of control for monitoring and managing integrations.
- Applications: Â
- Enterprise Resource Planning (ERP): Integration frameworks connect ERP systems with other business applications, streamlining operations. Rapid Innovation can help clients implement robust integration solutions that enhance data flow and operational efficiency.
- Customer Relationship Management (CRM): Ensures that CRM systems are updated with real-time data from various sources. Our expertise ensures that clients can leverage their CRM systems to gain actionable insights and improve customer engagement.
- Cloud Services: Facilitates the integration of on-premises systems with cloud-based applications, enabling organizations to harness the power of cloud computing while maintaining existing infrastructure.
- Benefits: Â
- Increased Efficiency: Reduces manual data entry and the risk of errors, leading to more reliable operations.
- Enhanced Collaboration: Teams can access and share information across different platforms easily, fostering a culture of collaboration and innovation.
- Cost Savings: Streamlined processes lead to reduced operational costs, allowing organizations to allocate resources more effectively.
3. Data Sources and Input Variables
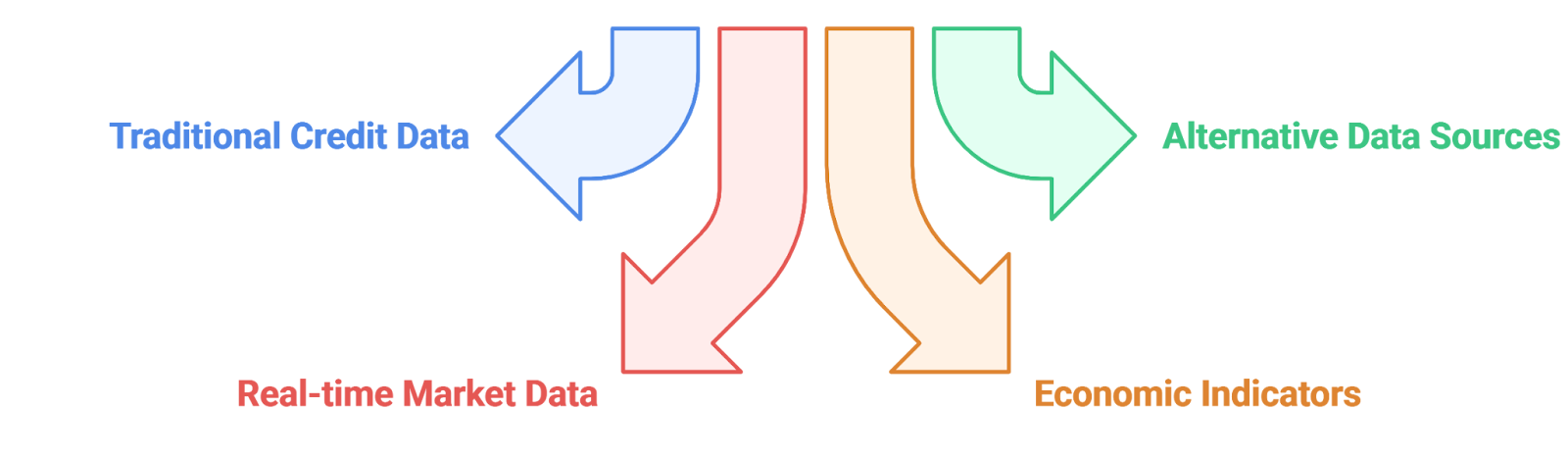
‍
Data sources and input variables are critical components in any data-driven decision-making process. They provide the raw information needed for analysis and insights.
- Types of Data Sources: Â
- Structured Data: Organized data, such as databases and spreadsheets, that can be easily analyzed.
- Unstructured Data: Information that does not have a predefined format, such as emails, social media posts, and videos.
- Semi-structured Data: Data that does not fit neatly into a database but contains tags or markers to separate elements, like XML or JSON files.
- Input Variables: Â
- Demographic Information: Age, gender, income level, and location of customers can influence decision-making.
- Behavioral Data: Insights into customer behavior, such as purchase history and website interactions, help tailor marketing strategies.
- Environmental Factors: External variables like economic conditions, weather patterns, and market trends can impact business decisions.
- Importance of Data Quality: Â
- Accuracy: Ensures that decisions are based on reliable information.
- Timeliness: Data must be current to reflect the latest trends and conditions.
- Relevance: Input variables should be pertinent to the specific analysis being conducted.
- Data Integration: Â
- Combining Sources: Merging data from various sources enhances the richness of insights.
- Data Cleansing: Removing duplicates and correcting errors improves overall data quality.
- Real-time Updates: Ensuring that data is continuously updated allows for more accurate decision-making.
By understanding the significance of real-time decision engines, integration frameworks, and data sources, organizations can enhance their operational efficiency and make informed decisions that drive success. Rapid Innovation is committed to providing the tools and expertise necessary to help clients achieve their business goals efficiently and effectively.
3.1. Traditional Credit Data
Traditional credit data refers to the information collected and maintained by credit bureaus that helps lenders assess the creditworthiness of individuals. This data is crucial for determining loan approvals, interest rates, and credit limits. Traditional credit data primarily includes credit history, payment behavior, and other financial activities that reflect an individual's ability to manage debt responsibly.
- Traditional credit data is often used by lenders to evaluate risk.
- It typically includes information from banks, credit card companies, and other financial institutions.
- The data is compiled into credit reports, which are used to generate credit scores.
3.1.1. Credit Bureau Reports
Credit bureau reports are comprehensive documents that provide a detailed overview of an individual's credit history. These reports are generated by credit bureaus, which are organizations that collect and maintain credit information. The three major credit bureaus in the United States are Experian, TransUnion, and Equifax.
- Credit bureau reports include personal information such as name, address, and Social Security number.
- They detail credit accounts, including credit cards, mortgages, and loans, along with their current status.
- The reports also contain public records, such as bankruptcies, liens, and judgments, which can significantly impact creditworthiness.
- Lenders use these reports to make informed decisions about extending credit.
Credit bureau reports are essential for consumers as well. They allow individuals to monitor their credit status, identify errors, and understand factors affecting their credit scores. Regularly reviewing credit reports can help consumers take proactive steps to improve their credit health.
3.1.2. Payment History
Payment history is a critical component of traditional credit data and is one of the most significant factors influencing credit scores. It reflects an individual's track record of making timely payments on credit accounts, including loans, credit cards, and other financial obligations.
- Payment history accounts for approximately 35% of a credit score, making it the most influential factor.
- A positive payment history demonstrates reliability and responsibility, while late payments, defaults, or delinquencies can severely damage credit scores.
- Lenders often look for a consistent pattern of on-time payments when evaluating credit applications.
Maintaining a good payment history is essential for anyone looking to build or maintain a strong credit profile. Strategies to ensure a positive payment history include:
- Setting up automatic payments to avoid missed deadlines.
- Keeping track of due dates using calendars or financial apps.
- Communicating with lenders if financial difficulties arise to explore options for deferment or restructuring.
At Rapid Innovation, we leverage AI and blockchain technologies to enhance the management and analysis of traditional credit data. By utilizing AI algorithms, we can help clients analyze vast amounts of credit data more efficiently, identifying patterns and insights that lead to better decision-making. Additionally, blockchain technology can provide a secure and transparent way to store and share credit data, ensuring that all parties involved have access to accurate and tamper-proof information.
Understanding the importance of payment history and credit bureau reports can empower consumers to take control of their financial futures. By actively managing their credit data, individuals can improve their chances of securing favorable loan terms and interest rates, ultimately leading to greater ROI for both consumers and lenders alike. For more information on how we can assist with AI banking solutions, visit our AI in Loan Underwriting: Use Cases, Best Practices, and Future.
3.1.3. Existing Credit Utilization
Credit utilization refers to the ratio of your current credit card balances to your credit limits. It is a critical factor in determining your credit score and overall creditworthiness. Understanding existing credit utilization is essential for both consumers and lenders.
- A lower credit utilization ratio (generally below 30%) is favorable and indicates responsible credit management.
- High credit utilization can negatively impact credit scores, making it harder to secure loans or favorable interest rates.
- Lenders often assess credit utilization to gauge risk; a high ratio may suggest financial distress or over-reliance on credit.
- Monitoring existing credit utilization can help consumers make informed decisions about spending and repayment strategies.
- Tools like credit monitoring services can provide insights into credit utilization trends over time.
3.2. Alternative Data Sources
Alternative data sources are non-traditional data points that can provide insights into an individual's creditworthiness. These sources are increasingly being used by lenders to assess risk, especially for those with limited credit histories.
- Alternative data can include utility payments, rental history, and even social media activity.
- Utilizing alternative data can help expand access to credit for underbanked populations who may not have traditional credit scores.
- Lenders can gain a more comprehensive view of a borrower's financial behavior, leading to better lending decisions.
- The use of alternative data can also enhance the speed of credit assessments, allowing for quicker loan approvals.
- Regulatory considerations are important; lenders must ensure compliance with laws governing data usage and consumer privacy.
3.2.1. Transaction Patterns
Transaction patterns refer to the spending behaviors and financial habits observed through an individual's bank account transactions. Analyzing these patterns can provide valuable insights into a person's financial stability and creditworthiness.
- Regular income deposits can indicate financial stability and the ability to repay loans.
- Spending habits, such as consistent payment of bills and discretionary spending, can reveal a borrower’s financial discipline.
- Lenders can use transaction data to identify trends, such as seasonal spending spikes or changes in income, which may affect repayment ability.
- Machine learning algorithms can analyze transaction patterns to predict future behavior and assess risk more accurately.
- Understanding transaction patterns can also help consumers manage their finances better, leading to improved credit health.
At Rapid Innovation, we leverage advanced AI and blockchain technologies to enhance the analysis of credit utilization analysis and alternative data sources. By implementing machine learning algorithms, we can help lenders make more informed decisions based on transaction patterns, ultimately leading to greater ROI. Our solutions enable clients to streamline their credit assessment processes, reduce risk, and expand access to credit for underserved populations, thereby achieving their business goals efficiently and effectively.
3.2.2. Digital Footprint Analysis
Digital footprint analysis involves examining the online traces left by individuals or organizations. This analysis is crucial for understanding consumer behavior, preferences, and trends.
- Types of Digital Footprints: Â
- Active Footprints: Information shared intentionally, such as social media posts, blog comments, and online reviews.
- Passive Footprints: Data collected without direct input, like browsing history, cookies, and location tracking.
- Importance of Digital Footprint: Â
- Helps businesses tailor marketing strategies based on consumer behavior.
- Provides insights into brand perception and customer engagement.
- Aids in identifying potential risks and vulnerabilities in online presence.
- Tools for Analysis: Â
- Google Analytics: Tracks website traffic and user behavior.
- Social Media Analytics: Platforms like Facebook Insights and Twitter Analytics provide data on engagement and reach.
- SEO Tools: Tools like SEMrush and Ahrefs help analyze online visibility and keyword performance.
- Challenges: Â
- Privacy concerns: Users are increasingly aware of their digital footprints and may limit data sharing.
- Data accuracy: Not all data collected is reliable or relevant, making it essential to filter and analyze effectively.
3.2.3. Social Data Indicators
Social data indicators are metrics derived from social media platforms that provide insights into audience engagement, sentiment, and overall brand performance.
- Key Metrics: Â
- Engagement Rate: Measures interactions (likes, shares, comments) relative to total followers.
- Reach and Impressions: Indicates how many people see content and how often it appears.
- Sentiment Analysis: Evaluates public perception of a brand or topic through positive, negative, or neutral sentiments.
- Benefits of Analyzing Social Data: Â
- Identifies trends and patterns in consumer behavior.
- Helps in crafting targeted marketing campaigns based on audience preferences.
- Enables real-time feedback, allowing brands to adjust strategies promptly.
- Tools for Social Data Analysis: Â
- Hootsuite: Offers comprehensive analytics for multiple social media platforms.
- Sprout Social: Provides in-depth reports on engagement and audience demographics.
- Brandwatch: Specializes in social listening and sentiment analysis.
- Challenges: Â
- Data Overload: The vast amount of data can be overwhelming, making it difficult to extract actionable insights.
- Algorithm Changes: Social media platforms frequently update their algorithms, affecting visibility and engagement metrics.
3.3. Real-time Market Data
Real-time market data refers to the immediate availability of information regarding market conditions, trends, and consumer behavior. This data is essential for businesses to make informed decisions quickly.
- Sources of Real-time Market Data: Â
- Financial Markets: Stock exchanges provide live updates on stock prices, trading volumes, and market indices.
- Social Media: Platforms like Twitter and Facebook offer insights into trending topics and consumer sentiment.
- E-commerce: Online retailers provide data on sales trends, inventory levels, and customer preferences.
- Importance of Real-time Data: Â
- Enhances decision-making: Businesses can respond swiftly to market changes and consumer demands.
- Improves competitive advantage: Companies that leverage real-time data can outperform competitors by anticipating market shifts.
- Facilitates agile marketing: Real-time insights allow for immediate adjustments in marketing strategies.
- Tools for Accessing Real-time Data: Â
- Bloomberg Terminal: Provides comprehensive financial data and analytics.
- Google Trends: Offers insights into search trends and consumer interests.
- Tableau: Visualizes real-time data for better understanding and analysis.
- Challenges: Â
- Data Accuracy: Ensuring the reliability of real-time data can be challenging due to the rapid pace of information flow.
- Integration: Combining real-time data with historical data for comprehensive analysis can be complex.
At Rapid Innovation, we leverage advanced AI and blockchain technologies to enhance digital footprint analysis, social media footprint analysis, social data indicators, and real-time market data. By utilizing AI algorithms, we can provide deeper insights into consumer behavior and preferences, enabling businesses to make data-driven decisions that lead to greater ROI. Our blockchain solutions ensure data integrity and security, addressing privacy concerns while maximizing the value derived from digital footprints and social data. Through our expertise, we empower clients to navigate the complexities of the digital landscape effectively and efficiently. For more information on how AI is transforming the field of neuromarketing.
3.4. Economic Indicators
Economic indicators are essential statistics that provide insights into the overall health and performance of an economy. They help businesses, investors, and policymakers make informed decisions. Understanding these indicators is crucial for analyzing economic trends and forecasting future performance.
- Types of Economic Indicators: Â
- Leading Indicators: These predict future economic activity. Examples include stock market performance, new housing starts, and consumer confidence indicator, consumer confidence index, and consumer sentiment index.
- Lagging Indicators: These reflect the economy's past performance. Common examples are unemployment rates and corporate profits.
- Coincident Indicators: These occur simultaneously with economic events, such as domestic GDP, GDP gross, and growth national product.
- Key Economic Indicators: Â
- Gross Domestic Product (GDP): Measures the total value of goods and services produced in a country. A growing GDP indicates a healthy economy, while a declining GDP may signal a recession. GDP per capita explanation and GDP capita meaning are also important for understanding economic performance on an individual level.
- Unemployment Rate: Indicates the percentage of the labor force that is unemployed and actively seeking employment. High unemployment can indicate economic distress.
- Inflation Rate: Measures the rate at which the general level of prices for goods and services rises. Moderate inflation is normal, but hyperinflation can be detrimental to an economy.
- Importance of Economic Indicators: Â
- Decision-Making: Businesses use these indicators to make strategic decisions regarding investments, hiring, and expansion. The economic misery index and big mac economic index can also provide unique insights into consumer behavior and purchasing power.
- Policy Formulation: Governments rely on economic indicators to shape fiscal and monetary policies.
- Market Analysis: Investors analyze these indicators to gauge market conditions and make investment choices. The michigan consumer sentiment index and university of michigan consumer sentiment index are valuable tools for understanding consumer confidence.
Understanding economic indicators is vital for anyone involved in economic planning or investment. They provide a snapshot of economic conditions and help predict future trends.
4. AI Agent Architecture
AI agent architecture refers to the design and structure of artificial intelligence systems that enable them to perform tasks autonomously. This architecture is crucial for developing intelligent agents capable of learning, reasoning, and interacting with their environment.
- Core Components of AI Agent Architecture: Â
- Perception: The ability of an AI agent to sense its environment through various inputs, such as cameras, microphones, and sensors.
- Reasoning: The process by which an AI agent interprets data, makes decisions, and solves problems based on its knowledge and experience.
- Action: The execution of decisions made by the AI agent, which can involve physical actions or digital responses.
- Types of AI Agent Architectures: Â
- Reactive Agents: These agents respond to specific stimuli without internal memory. They are simple and operate based on predefined rules.
- Deliberative Agents: These agents maintain an internal model of the world and can plan and reason about future actions.
- Hybrid Agents: Combining reactive and deliberative approaches, these agents can adapt to changing environments while also planning for future actions.
- Applications of AI Agent Architecture: Â
- Robotics: AI agents in robots can navigate and perform tasks in dynamic environments.
- Virtual Assistants: AI agents like Siri and Alexa use natural language processing to understand and respond to user queries.
- Autonomous Vehicles: AI agents in self-driving cars analyze data from sensors to make real-time driving decisions.
The architecture of AI agents is fundamental to their functionality and effectiveness in various applications. A well-designed architecture enhances the agent's ability to learn and adapt to new situations.
4.1. System Components
The system components of AI agent architecture are the building blocks that enable the agent to function effectively. Each component plays a specific role in the overall operation of the AI system.
- Key System Components: Â
- Sensors: Devices that collect data from the environment. They can include cameras, microphones, and other input devices that provide information for processing.
- Actuators: Mechanisms that allow the AI agent to take action in the environment. This can include motors, speakers, or any output device that enables interaction.
- Processing Unit: The core of the AI agent, where data is analyzed, decisions are made, and actions are determined. This unit often includes CPUs, GPUs, or specialized AI chips.
- Supporting Components: Â
- Knowledge Base: A repository of information that the AI agent uses to make informed decisions. This can include databases, ontologies, and machine learning models.
- Communication Interface: Allows the AI agent to interact with other systems or agents. This can involve APIs, messaging protocols, or network connections.
- Learning Mechanism: The component that enables the AI agent to learn from experience and improve its performance over time. This can include supervised learning, unsupervised learning, or reinforcement learning techniques.
- Integration of Components: Â
- Modularity: Each component can be developed and updated independently, allowing for flexibility and scalability in the AI system.
- Interoperability: Components must work together seamlessly to ensure the AI agent can function effectively in its environment.
- Real-Time Processing: The system must be capable of processing data and making decisions quickly to respond to dynamic conditions.
Understanding the system components of AI agent architecture is essential for developing robust and efficient AI systems. Each component contributes to the overall capability of the agent, enabling it to perform complex tasks autonomously.
At Rapid Innovation, we leverage our expertise in AI and Blockchain to help clients navigate these economic indicators effectively. By integrating advanced AI solutions, we enable businesses to analyze data trends and make informed decisions that align with their strategic goals, ultimately driving greater ROI. Our blockchain solutions enhance transparency and security in transactions, further supporting informed decision-making in a rapidly changing economic landscape. For more insights, visit AI Agent Societies and Simulations.
4.1.1. Data Ingestion Layer
The Data Ingestion Layer is a critical component in any data architecture, serving as the entry point for data into a system. This layer is responsible for collecting, importing, and processing data from various sources, ensuring that it is available for further analysis and processing.
- Collects data from multiple sources, including: Â
- Databases
- APIs
- IoT devices
- Social media platforms
- Supports various data formats, such as: Â
- Structured data (e.g., SQL databases)
- Semi-structured data (e.g., JSON, XML)
- Unstructured data (e.g., text files, images)
- Ensures data quality and integrity through: Â
- Validation checks
- Data cleansing processes
- Utilizes tools and technologies like: Â
- Apache Kafka for real-time data streaming
- Apache NiFi for data flow automation
- AWS Glue for serverless data integration
- Enables scalability to handle large volumes of data, accommodating growth in data sources and types.
At Rapid Innovation, we leverage our expertise in AI and Blockchain to enhance the Data Ingestion Layer, ensuring that our clients can efficiently gather and process data from diverse sources. By implementing robust data ingestion strategies, including a big data ingestion layer, we help organizations achieve greater ROI through improved data accessibility and quality. The ingestion layer in big data architecture is essential for managing the influx of data from various channels.
4.1.2. Processing Engine
The Processing Engine is the core of data transformation and analysis. It takes the ingested data and processes it to derive insights, perform calculations, and prepare it for decision-making.
- Performs data transformation tasks, including: Â
- Aggregation
- Filtering
- Enrichment
- Supports batch processing and real-time processing, allowing for: Â
- Scheduled data processing (e.g., nightly batch jobs)
- Continuous data processing (e.g., real-time analytics)
- Utilizes frameworks and technologies such as: Â
- Apache Spark for distributed data processing
- Apache Flink for stream processing
- Hadoop for large-scale data processing
- Ensures efficient resource management through: Â
- Load balancing
- Parallel processing
- Provides capabilities for machine learning and advanced analytics, enabling organizations to: Â
- Build predictive models
- Conduct data mining
Rapid Innovation employs advanced processing techniques to transform raw data into actionable insights. Our AI-driven analytics solutions empower clients to make data-informed decisions, ultimately leading to enhanced operational efficiency and increased profitability.
4.1.3. Decision Layer
The Decision Layer is where insights derived from processed data are translated into actionable decisions. This layer is crucial for business intelligence and strategic planning.
- Integrates with visualization tools to present data insights, including: Â
- Dashboards
- Reports
- Interactive visualizations
- Supports decision-making processes by providing: Â
- Key performance indicators (KPIs)
- Trend analysis
- Predictive analytics
- Facilitates collaboration among stakeholders by: Â
- Sharing insights across departments
- Enabling data-driven discussions
- Utilizes decision support systems (DSS) to enhance decision-making, which may include: Â
- Scenario analysis
- What-if modeling
- Ensures compliance and governance by: Â
- Implementing data security measures
- Adhering to regulatory requirements (e.g., GDPR, HIPAA)
At Rapid Innovation, we understand the importance of the Decision Layer in driving business success. By integrating AI and Blockchain technologies, we provide our clients with powerful decision-making tools that enhance strategic planning and foster collaboration across teams. This ultimately leads to improved business outcomes and a higher return on investment.
Each of these layers plays a vital role in the overall data architecture, ensuring that organizations can effectively manage, process, and utilize their data for informed decision-making. The ingestion layer in big data is particularly significant as it lays the foundation for the entire data pipeline.
4.1.4. Output Interface
The output interface is a critical component of any system, as it defines how data is presented to users or other systems. A well-designed output interface enhances user experience and ensures that information is conveyed clearly and effectively.
- User-Friendly Design: The output interface should be intuitive, allowing users to easily navigate and understand the information presented. This includes using clear labels, logical layouts, and consistent design elements, which are key aspects of output and user interface design.
- Data Formats: The output can be delivered in various formats, such as HTML, PDF, or CSV. Choosing the right format depends on the target audience and the type of data being presented.
- Real-Time Updates: For systems that require timely information, the output interface should support real-time data updates. This ensures users have access to the most current information without needing to refresh or reload the interface.
- Accessibility: The output interface must be accessible to all users, including those with disabilities. Implementing features like screen reader compatibility and keyboard navigation can enhance accessibility.
- Customization Options: Providing users with the ability to customize their output view can improve satisfaction. Options may include filtering data, changing display formats, or selecting specific data points to view, which is an important aspect of user interface input and output design.
4.2. Integration Points
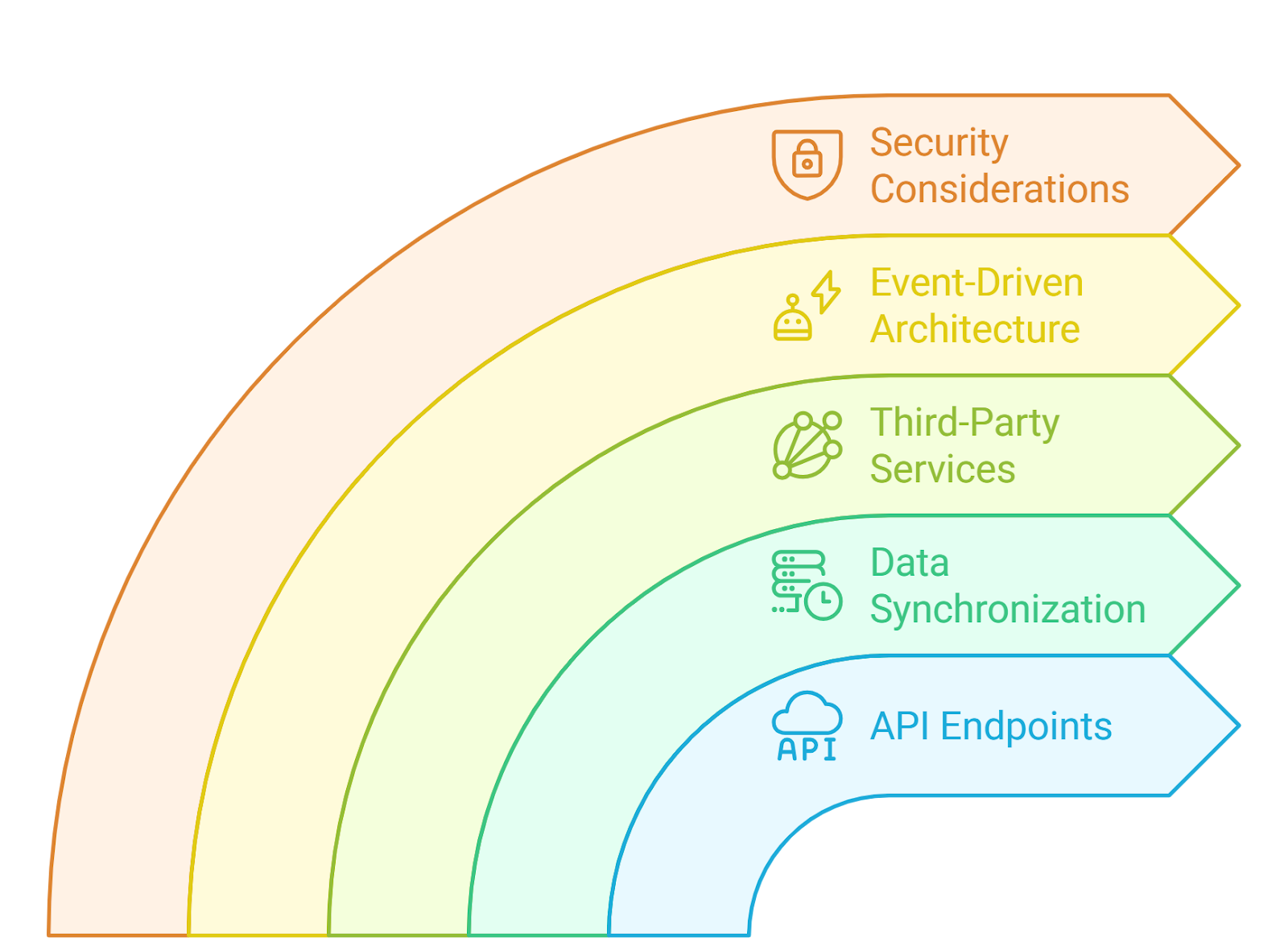
‍
Integration points are essential for ensuring that different systems or components can communicate and work together effectively. Identifying and implementing these points can significantly enhance the functionality and efficiency of a system.
- API Endpoints: Application Programming Interfaces (APIs) serve as integration points that allow different software applications to communicate. Well-documented APIs enable seamless data exchange and functionality sharing.
- Data Synchronization: Integration points should facilitate data synchronization between systems. This ensures that all systems have access to the same information, reducing discrepancies and improving decision-making.
- Third-Party Services: Many systems rely on third-party services for additional functionality, such as payment processing or data analytics. Identifying integration points with these services is crucial for expanding system capabilities.
- Event-Driven Architecture: Implementing an event-driven architecture can enhance integration by allowing systems to respond to events in real-time. This approach can improve responsiveness and reduce latency in data processing.
- Security Considerations: When establishing integration points, it is vital to consider security measures. This includes using secure protocols, authentication methods, and data encryption to protect sensitive information.
4.3. Scalability Considerations
Scalability is a fundamental aspect of system design, ensuring that a system can handle increased loads without compromising performance. Proper scalability considerations can future-proof a system and accommodate growth.
- Horizontal vs. Vertical Scaling: Understanding the difference between horizontal and vertical scaling is essential. Horizontal scaling involves adding more machines to handle increased load, while vertical scaling involves upgrading existing machines. Each approach has its advantages and challenges.
- Load Balancing: Implementing load balancing can distribute incoming traffic across multiple servers, preventing any single server from becoming a bottleneck. This enhances performance and reliability.
- Database Scalability: As data volume grows, database scalability becomes crucial. Techniques such as sharding, replication, and using NoSQL databases can help manage large datasets effectively.
- Performance Monitoring: Regularly monitoring system performance can identify potential scalability issues before they become critical. Tools that provide insights into resource usage and traffic patterns can help in proactive scaling.
- Future Growth Projections: Anticipating future growth is vital for scalability planning. Understanding user trends and potential increases in data volume can guide infrastructure investments and design choices.
At Rapid Innovation, we leverage our expertise in AI and Blockchain to enhance these aspects of system design, ensuring that our clients achieve greater ROI through efficient and effective solutions. By implementing advanced AI algorithms, we can optimize output interfaces for better user engagement, while our Blockchain solutions ensure secure and reliable integration points, ultimately leading to scalable systems that can grow with your business needs.
4.4. Security Architecture
Security architecture is a comprehensive framework that outlines the security measures and protocols necessary to protect an organization's information systems. It serves as a blueprint for managing security risks and ensuring the integrity, confidentiality, and availability of data, including zero trust architecture and enterprise security architecture.
- Key components of security architecture include: Â
- Security Policies: These are formalized rules and guidelines that dictate how security measures, such as those in information security architecture, should be implemented and maintained.
- Security Controls: These are the technical and administrative safeguards put in place to protect information systems from threats, including those identified in network security architecture.
- Network Security: This involves protecting the integrity and usability of network and data, including firewalls, intrusion detection systems, and secure communication protocols, as well as the principles of secure architecture.
- Identity and Access Management (IAM): This ensures that only authorized users have access to specific data and systems, utilizing methods like multi-factor authentication and role-based access control, which are critical in a zero trust network architecture.
- Data Protection: This includes encryption, data masking, and secure data storage practices to protect sensitive information from unauthorized access, aligning with cloud security architecture.
- Incident Response: A well-defined plan for responding to security breaches or incidents, including detection, containment, eradication, and recovery processes, is essential in any cybersecurity mesh architecture.
A robust security architecture not only protects against external threats but also addresses internal vulnerabilities. It is essential for organizations to regularly review and update their security architecture, including enterprise IT security architecture, to adapt to evolving threats and compliance requirements. Rapid Innovation leverages advanced AI algorithms to enhance security measures, enabling real-time threat detection and response, thereby increasing the overall security posture of our clients, particularly in the context of SASE architecture and NIST zero trust frameworks.
5. Risk Assessment Methodologies

‍
Risk assessment methodologies are systematic approaches used to identify, evaluate, and prioritize risks associated with an organization's operations. These methodologies help organizations make informed decisions about risk management and resource allocation.
- Common risk assessment methodologies include: Â
- Qualitative Risk Assessment: This approach relies on subjective judgment to evaluate risks based on their likelihood and impact. It often uses risk matrices to categorize risks as low, medium, or high.
- Quantitative Risk Assessment: This method uses numerical data and statistical analysis to assess risks. It often involves calculating potential losses and the probability of occurrence, providing a more objective view of risk.
- Hybrid Approaches: Combining qualitative and quantitative methods, hybrid approaches leverage the strengths of both to provide a comprehensive risk assessment.
- Frameworks and Standards: Organizations may adopt established frameworks such as NIST, ISO 31000, or FAIR to guide their risk assessment processes, including those related to cybersecurity mesh architecture.
Effective risk assessment methodologies enable organizations to:
- Identify vulnerabilities and threats
- Prioritize risks based on their potential impact
- Develop strategies to mitigate or transfer risks
- Ensure compliance with regulatory requirements
5.1. Traditional Credit Scoring
Traditional credit scoring is a method used by lenders to evaluate the creditworthiness of potential borrowers. It relies on historical financial data to predict the likelihood of a borrower defaulting on a loan.
- Key elements of traditional credit scoring include: Â
- Credit History: This includes the borrower's past borrowing and repayment behavior, which is a significant factor in determining credit scores.
- Credit Utilization: This measures the amount of credit being used compared to the total available credit. A lower utilization ratio is generally viewed favorably.
- Length of Credit History: A longer credit history can positively impact a credit score, as it provides more data on the borrower's financial behavior.
- Types of Credit: A mix of credit types, such as credit cards, mortgages, and installment loans, can enhance a credit score.
- Recent Inquiries: Multiple recent credit inquiries can negatively affect a credit score, as they may indicate financial distress.
Traditional credit scoring models, such as FICO and VantageScore, typically range from 300 to 850, with higher scores indicating lower risk. These scores are crucial for lenders in making decisions about loan approvals, interest rates, and credit limits.
- Limitations of traditional credit scoring include: Â
- Lack of Inclusivity: Many individuals, particularly those with limited credit history, may be excluded from the credit system.
- Potential Bias: Traditional scoring models may inadvertently perpetuate biases based on socioeconomic factors.
- Static Nature: Credit scores may not reflect real-time changes in a borrower's financial situation, leading to outdated assessments.
As the financial landscape evolves, alternative credit scoring methods are emerging to address these limitations, providing a more comprehensive view of creditworthiness. Rapid Innovation is at the forefront of this transformation, utilizing AI-driven analytics to create more inclusive and dynamic credit scoring models that better reflect an individual's financial behavior.
5.2. AI-Enhanced Risk Models
AI-enhanced risk models leverage advanced algorithms and machine learning techniques to improve the accuracy and efficiency of risk assessment in various industries, particularly in finance and insurance. These models analyze vast amounts of data to identify patterns and predict potential risks, including applications in ai risk assessment and ai risk analysis.
- Improved accuracy: AI models can process large datasets, leading to more precise risk predictions. Â
- Real-time analysis: Continuous data monitoring allows for immediate updates to risk assessments. Â
- Predictive analytics: Machine learning algorithms can forecast future risks based on historical data. Â
- Customization: AI can tailor risk models to specific industries or organizations, enhancing relevance. Â
- Cost efficiency: Automating risk assessment reduces the need for extensive manual analysis, saving time and resources. Â
At Rapid Innovation, we implement AI-enhanced risk models to help our clients achieve greater ROI by enabling them to make informed decisions based on accurate risk assessments, ultimately leading to reduced losses and optimized resource allocation. Our expertise in ai in risk assessment ensures that our clients are equipped with the best tools for their needs. For more insights on this topic, check out the future of personalized risk evaluation in insurance with AI agents.
5.3. Dynamic Risk Assessment
Dynamic risk assessment refers to the ongoing evaluation of risks as conditions change. Unlike traditional static assessments, which are often conducted at set intervals, dynamic assessments adapt to new information and evolving circumstances.
- Continuous monitoring: Organizations can track risk factors in real-time, allowing for timely interventions. Â
- Flexibility: Dynamic assessments can adjust to changes in the business environment, regulatory landscape, or market conditions. Â
- Enhanced decision-making: Real-time data enables better-informed decisions regarding risk mitigation strategies. Â
- Integration with technology: Utilizing tools like IoT and AI, organizations can gather and analyze data more effectively. Â
- Improved resilience: By staying updated on risks, organizations can respond more swiftly to potential threats. Â
Dynamic risk assessment is essential for organizations aiming to maintain a competitive edge in a rapidly changing environment. Rapid Innovation assists clients in implementing dynamic risk assessment frameworks that enhance their agility and responsiveness to emerging risks, thereby maximizing their operational efficiency.
5.4. Behavioral Scoring
Behavioral scoring is a technique used to evaluate the likelihood of an individual or entity engaging in certain behaviors based on historical data. This method is particularly prevalent in credit scoring, fraud detection, and customer relationship management.
- Data-driven insights: Behavioral scoring relies on data analytics to assess patterns in behavior. Â
- Risk identification: Organizations can identify high-risk individuals or transactions more effectively. Â
- Personalization: Insights from behavioral scoring can help tailor products and services to meet customer needs. Â
- Fraud prevention: By analyzing behavioral patterns, organizations can detect anomalies that may indicate fraudulent activity. Â
- Enhanced customer relationships: Understanding customer behavior allows for improved engagement and retention strategies. Â
At Rapid Innovation, we leverage behavioral scoring to empower our clients with actionable insights that enhance their risk management and customer relationship strategies, ultimately driving higher customer satisfaction and loyalty.
5.5. Industry-Specific Risk Factors
In any industry, understanding the unique risk factors is crucial for effective management and strategic planning. Industry-specific risk factors can significantly impact operations, profitability, and long-term sustainability. Here are some key considerations:
- Regulatory Compliance: Different industries face varying levels of regulatory scrutiny. For example, the healthcare sector must comply with HIPAA regulations, while financial services are governed by SEC rules. Non-compliance can lead to hefty fines and reputational damage. Rapid Innovation assists clients in navigating these complexities by leveraging AI-driven compliance solutions that automate monitoring and reporting, ensuring adherence to regulations while minimizing risks. This is particularly relevant when considering types of risk in healthcare.
- Market Volatility: Industries such as technology and commodities are often subject to rapid changes in market conditions. Fluctuations in demand, supply chain disruptions, or shifts in consumer preferences can create significant risks. Our blockchain solutions provide transparent and immutable records that enhance supply chain visibility, enabling clients to respond swiftly to market changes and optimize inventory management.
- Technological Advancements: Industries that rely heavily on technology, like manufacturing and retail, must continuously adapt to new innovations. Failure to keep pace can result in obsolescence and loss of competitive advantage. Rapid Innovation offers AI consulting services that help organizations identify and implement cutting-edge technologies, ensuring they remain competitive and efficient. This is also a concern in the risk in IT industry.
- Environmental Factors: Industries such as agriculture and energy are particularly vulnerable to environmental changes. Climate change, natural disasters, and resource scarcity can disrupt operations and affect profitability. Our AI models can predict environmental impacts, allowing clients to develop proactive strategies that mitigate risks and enhance sustainability.
- Labor Market Dynamics: Industries like hospitality and construction often face challenges related to labor availability and wage fluctuations. Economic downturns can exacerbate these issues, leading to increased operational costs. Rapid Innovation employs AI-driven workforce management solutions that optimize labor allocation and reduce costs, helping clients maintain operational efficiency. This is especially pertinent when considering financial risks in construction projects.
- Cybersecurity Threats: As industries become more digitized, the risk of cyberattacks increases. Sectors like finance and healthcare are prime targets for hackers, necessitating robust cybersecurity measures. Our blockchain technology enhances data security through decentralized systems, providing clients with a resilient infrastructure that protects sensitive information from breaches. This highlights the importance of understanding examples of risks in healthcare and operational risk in the airline industry.
- Industry Risk Factors: Each industry has its own set of risk factors that can affect its stability and growth. For instance, the healthcare industry faces unique challenges such as examples of poor risk management in healthcare and factors that influence risk management in the health care industry. Additionally, understanding industry risk rating and industry-specific risk premium can help organizations better assess their risk exposure.
6. Implementation Framework
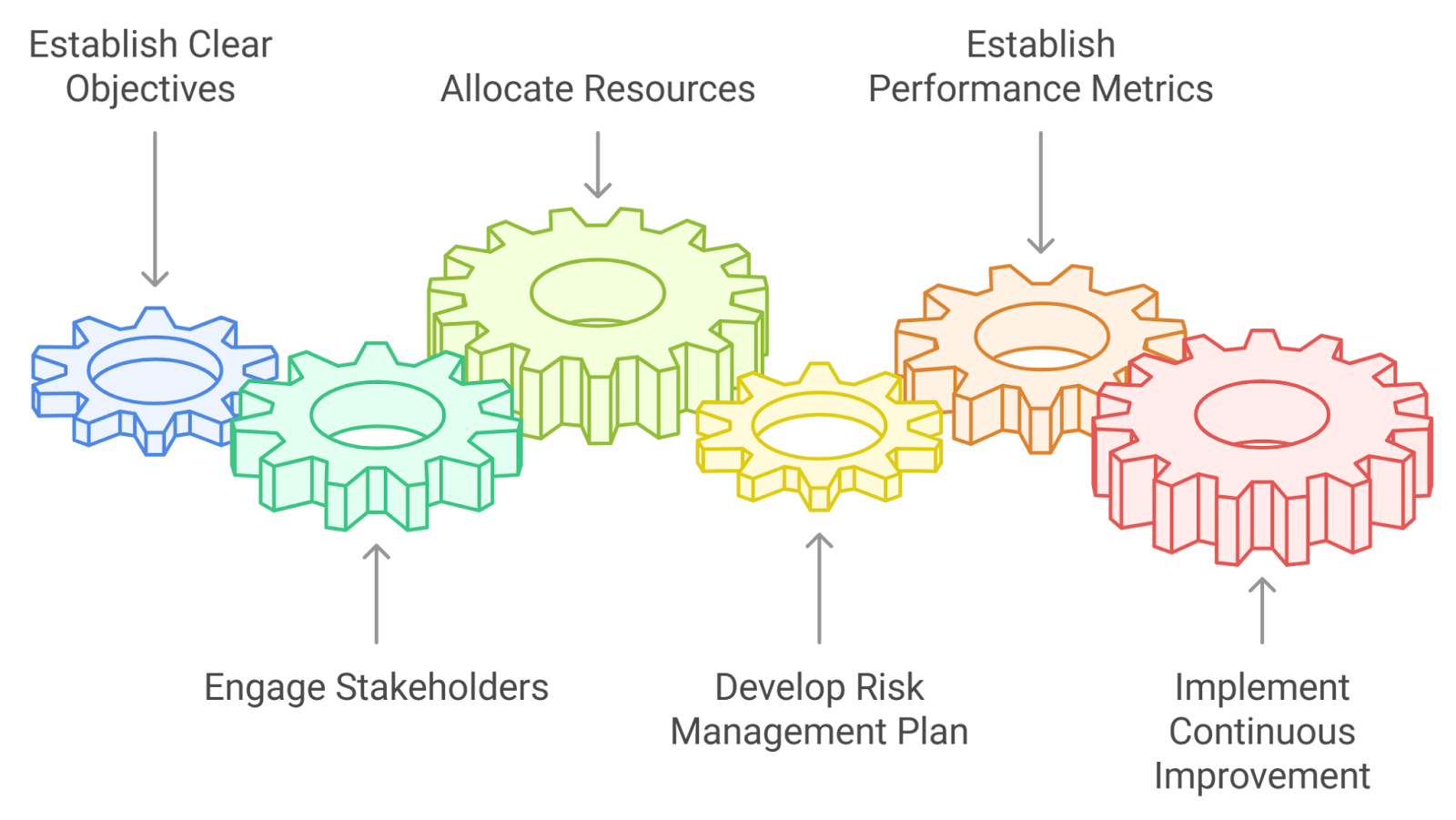
‍
An effective implementation framework is essential for translating strategic plans into actionable steps. This framework provides a structured approach to ensure that initiatives are executed efficiently and effectively. Key components of an implementation framework include:
- Clear Objectives: Establishing specific, measurable, achievable, relevant, and time-bound (SMART) objectives is crucial. This clarity helps align team efforts and resources toward common goals.
- Stakeholder Engagement: Involving key stakeholders throughout the implementation process fosters buy-in and support. Regular communication and feedback loops can enhance collaboration and address concerns early.
- Resource Allocation: Identifying and allocating the necessary resources—financial, human, and technological—is vital for successful implementation. This includes budgeting for potential contingencies.
- Risk Management: Developing a risk management plan to identify, assess, and mitigate potential risks is essential. This proactive approach helps minimize disruptions and ensures smoother execution.
- Performance Metrics: Establishing key performance indicators (KPIs) allows organizations to track progress and measure success. Regularly reviewing these metrics can inform necessary adjustments to the implementation strategy.
- Continuous Improvement: An effective implementation framework should include mechanisms for continuous feedback and improvement. This iterative process allows organizations to adapt to changing circumstances and enhance overall performance.
6.1. System Requirements
Defining system requirements is a critical step in the implementation framework. These requirements outline the necessary specifications and functionalities needed to support the organization's objectives. Key aspects to consider include:
- Functional Requirements: These specify what the system should do, including features and capabilities. For example, a customer relationship management (CRM) system may require functionalities like lead tracking, reporting, and integration with other tools.
- Non-Functional Requirements: These address how the system performs, including aspects like reliability, scalability, and security. Ensuring that the system can handle increased loads and protect sensitive data is essential.
- User Requirements: Understanding the needs and preferences of end-users is crucial for system adoption. Conducting user surveys or focus groups can provide valuable insights into desired features and usability.
- Technical Requirements: These outline the technical specifications necessary for system implementation, including hardware, software, and network infrastructure. Ensuring compatibility with existing systems is vital to avoid integration issues.
- Compliance Requirements: Depending on the industry, certain compliance standards may dictate specific system functionalities. For instance, financial systems may need to adhere to PCI DSS standards for payment processing.
- Budget Constraints: Establishing a budget for system implementation is essential. This includes costs for software licenses, hardware, training, and ongoing maintenance. A clear budget helps prioritize features and manage expectations.
- Timeline: Setting a realistic timeline for system implementation is crucial. This includes milestones for development, testing, and deployment. A well-defined timeline helps keep the project on track and ensures timely delivery.
By addressing these system requirements, organizations can ensure that their implementation framework is robust and aligned with their strategic goals. Rapid Innovation is committed to guiding clients through this process, leveraging our expertise in AI and blockchain to enhance operational efficiency and drive greater ROI.
6.2. Data Preparation
Data preparation is a crucial step in the machine learning pipeline. It involves cleaning, transforming, and organizing raw data into a format suitable for analysis and model training. Proper data preparation can significantly enhance the performance of machine learning models, ultimately leading to greater ROI for businesses.
- Data Cleaning: This step involves identifying and correcting errors or inconsistencies in the dataset. Common tasks include: Â
- Removing duplicates.
- Handling missing values (e.g., imputation or deletion).
- Correcting data types (e.g., converting strings to dates).
- Data Transformation: Transforming data helps in making it more suitable for modeling. Key techniques include: Â
- Normalization or standardization to scale features.
- Encoding categorical variables (e.g., one-hot encoding).
- Feature extraction to create new variables from existing ones.
- Data Splitting: The dataset is typically divided into training, validation, and test sets. This ensures that the model can be trained on one subset and evaluated on another, preventing overfitting.
- Exploratory Data Analysis (EDA): EDA helps in understanding the underlying patterns in the data. Techniques include: Â
- Visualizations (e.g., histograms, scatter plots).
- Summary statistics (e.g., mean, median, mode).
Effective data preparation lays the foundation for successful model training and can lead to improved accuracy and reliability of predictions. At Rapid Innovation, we leverage our expertise in AI to ensure that your data is meticulously prepared, enabling your models to perform at their best and drive significant business outcomes. This includes data preparation for machine learning, data preparation techniques in machine learning, and dataset preparation for machine learning.
6.3. Model Training
Model training is the process of teaching a machine learning algorithm to recognize patterns in data. This step is essential for developing a predictive model that can make accurate forecasts based on new data.
- Choosing the Right Algorithm: Selecting an appropriate algorithm is critical. Common algorithms include: Â
- Linear regression for continuous outcomes.
- Decision trees for classification tasks.
- Neural networks for complex data patterns.
- Hyperparameter Tuning: Hyperparameters are settings that govern the training process. Tuning these parameters can significantly affect model performance. Techniques include: Â
- Grid search for exhaustive parameter testing.
- Random search for a more efficient approach.
- Bayesian optimization for intelligent parameter selection.
- Training the Model: During training, the model learns from the training dataset. Key aspects include: Â
- Feeding the model input features and corresponding labels.
- Adjusting weights and biases through optimization algorithms (e.g., gradient descent).
- Monitoring Performance: It's essential to track the model's performance during training. Metrics such as accuracy, precision, recall, and F1 score help in evaluating how well the model is learning.
Model training is a dynamic process that requires continuous evaluation and adjustment to achieve optimal results. Rapid Innovation employs advanced techniques and best practices to ensure that your models are not only trained effectively but also aligned with your business objectives, maximizing your return on investment. This includes machine learning data preparation steps and data preparation for machine learning python.
6.4. Validation Processes
Validation processes are critical for assessing the performance and generalizability of a machine learning model. These processes help ensure that the model performs well not just on the training data but also on unseen data.
- Cross-Validation: This technique involves partitioning the training data into multiple subsets. The model is trained on some subsets and validated on others. Common methods include: Â
- K-fold cross-validation, where the data is divided into K subsets.
- Stratified K-fold, which maintains the distribution of classes in each fold.
- Holdout Validation: In this method, the dataset is split into training and validation sets. The model is trained on the training set and evaluated on the validation set. This approach is straightforward but may lead to variability in results based on how the data is split.
- Performance Metrics: Evaluating model performance is essential. Common metrics include: Â
- Accuracy for overall correctness.
- Precision and recall for class-specific performance.
- ROC-AUC for binary classification tasks.
- Overfitting and Underfitting: Validation processes help identify issues like overfitting (model performs well on training data but poorly on validation data) and underfitting (model fails to capture the underlying trend). Techniques to mitigate these issues include: Â
- Regularization methods (e.g., L1 and L2 regularization).
- Using more data for training.
Validation processes are vital for ensuring that the model is robust and can make reliable predictions in real-world scenarios. At Rapid Innovation, we prioritize rigorous validation techniques to ensure that your AI solutions are not only effective but also scalable, providing you with a competitive edge in your industry. This includes data preparation for deep learning and data preparation for neural network applications.
6.5. Deployment Strategies
Deployment strategies are crucial for ensuring that software applications are released efficiently and effectively. The choice of deployment strategy can significantly impact the performance, reliability, and user experience of the application. Here are some common deployment strategies:
- Blue-Green Deployment: This strategy involves maintaining two identical environments, one active (blue) and one idle (green). New versions of the application are deployed to the green environment. Once testing is complete, traffic is switched from blue to green, minimizing downtime and risk.
- Canary Releases: In this approach, a new version of the application is rolled out to a small subset of users before a full-scale deployment. This allows for monitoring of the new version's performance and user feedback, reducing the risk of widespread issues.
- Rolling Deployment: This strategy involves gradually replacing instances of the previous version of the application with the new version. This method allows for continuous availability and can be rolled back easily if issues arise.
- Recreate Deployment: In this method, the existing application is completely shut down before the new version is deployed. While this is straightforward, it can lead to downtime, making it less favorable for applications requiring high availability.
- Shadow Deployment: This strategy involves deploying the new version alongside the existing version without affecting the user experience. The new version processes real user requests, but the results are not visible to users. This allows for performance testing in a live environment.
Choosing the right deployment strategy depends on various factors, including application architecture, user base, and business requirements. Each strategy has its pros and cons, and understanding these can help teams make informed decisions. At Rapid Innovation, we leverage our expertise in AI and Blockchain to tailor deployment strategies, including lean deployment strategy and application deployment strategies, that align with your specific business goals, ensuring a seamless transition and maximizing your return on investment. Additionally, methodologies such as hoshin strategy deployment and danaher policy deployment can be integrated into the deployment process to enhance strategic alignment. Understanding the types of deployment strategies available, such as a3 strategy deployment and api deployment strategy, can further refine your approach. Each deployment strategie has its unique characteristics, and incorporating policy strategy and goal deployment can ensure that your deployment aligns with broader organizational objectives. For developers facing technical challenges in AI agent deployment.
6.6. Monitoring and Maintenance
Monitoring and maintenance are essential components of the software lifecycle, ensuring that applications run smoothly and efficiently after deployment. Effective monitoring helps identify issues before they escalate, while maintenance ensures that the application remains up-to-date and secure. Key aspects include:
- Performance Monitoring: Tools like New Relic or Datadog can track application performance metrics, such as response times, error rates, and resource usage. This data helps identify bottlenecks and optimize performance.
- Error Tracking: Implementing error tracking tools like Sentry or Rollbar allows teams to capture and analyze errors in real-time. This helps in quickly addressing issues and improving the overall user experience.
- User Feedback: Gathering user feedback through surveys or monitoring user behavior can provide insights into how the application is performing from the end-user perspective. This information is invaluable for making improvements.
- Regular Updates: Keeping the application updated with the latest security patches and features is crucial. Regular maintenance schedules should be established to ensure that updates are applied without disrupting service.
- Backup and Recovery: Implementing a robust backup and recovery plan is essential for data integrity. Regular backups ensure that data can be restored in case of failures or data loss.
- Scalability Monitoring: As user demand grows, monitoring scalability is vital. Tools that analyze traffic patterns can help predict when additional resources are needed to maintain performance.
By focusing on these aspects of monitoring and maintenance, organizations can ensure their applications remain reliable, secure, and user-friendly. Rapid Innovation employs advanced monitoring solutions that utilize AI to predict potential issues and automate maintenance tasks, thereby enhancing operational efficiency and ensuring a higher ROI for our clients.
7. Advanced Features and Capabilities

‍
In today's competitive landscape, advanced features and capabilities can set an application apart from its competitors. These features enhance user experience, improve functionality, and provide additional value. Some notable advanced features include:
- Artificial Intelligence and Machine Learning: Integrating AI and ML can provide personalized experiences, automate tasks, and analyze data for insights. This can lead to improved decision-making and enhanced user engagement.
- API Integrations: Offering robust API integrations allows applications to connect with other services and platforms, enhancing functionality and enabling seamless data exchange. This is crucial for creating a cohesive ecosystem.
- Real-time Data Processing: Applications that can process data in real-time provide users with immediate insights and updates. This is particularly important for industries like finance and e-commerce, where timely information is critical.
- Mobile Optimization: With the increasing use of mobile devices, ensuring that applications are optimized for mobile is essential. This includes responsive design, fast loading times, and mobile-specific features.
- Cloud Capabilities: Leveraging cloud technology allows for scalability, flexibility, and cost-effectiveness. Cloud-based applications can easily adapt to changing user demands and provide access from anywhere.
- Security Features: Advanced security features, such as multi-factor authentication, encryption, and regular security audits, are essential for protecting user data and maintaining trust.
- User Analytics: Implementing user analytics tools can provide insights into user behavior, preferences, and engagement levels. This data can inform future development and marketing strategies.
By incorporating these advanced features and capabilities, organizations can enhance their applications, meet user expectations, and stay ahead in a rapidly evolving market. Rapid Innovation is committed to helping clients integrate these advanced functionalities, ensuring that their applications not only meet current demands but also anticipate future trends, thereby maximizing their competitive edge and ROI.
7.1. Real-time Limit Adjustments
Real-time limit adjustments are crucial for businesses that need to manage risk effectively while ensuring customer satisfaction. This feature allows organizations to modify transaction limits instantly based on various factors, such as customer behavior, transaction history, and external market conditions.
- Enhances customer experience by allowing higher limits for trusted customers. Â
- Reduces the risk of fraud by lowering limits for suspicious activities. Â
- Utilizes machine learning algorithms to analyze data and make informed decisions. Â
- Provides flexibility in managing credit limits, especially in industries like finance and e-commerce. Â
- Enables businesses to respond quickly to changing market dynamics or customer needs. Â
By implementing real-time limit adjustments, companies can maintain a balance between security and customer service, ultimately leading to increased trust and loyalty. Rapid Innovation specializes in developing tailored solutions that leverage AI technologies to facilitate real-time limit adjustments, ensuring that businesses can operate efficiently while minimizing risk.
7.2. Fraud Detection Integration
Fraud detection integration is essential for safeguarding businesses against financial losses and reputational damage. This process involves incorporating advanced technologies and analytics to identify and mitigate fraudulent activities in real-time.
- Employs machine learning models to detect unusual patterns in transaction data. Â
- Utilizes behavioral analytics to understand customer habits and flag anomalies. Â
- Integrates with existing systems to provide a seamless experience for users. Â
- Offers multi-layered security measures, including biometric authentication and geolocation tracking. Â
- Reduces false positives, ensuring legitimate transactions are not unnecessarily blocked. Â
With effective fraud detection integration, businesses can protect themselves and their customers, fostering a secure environment for transactions. Rapid Innovation's expertise in AI and blockchain technologies enables us to create robust fraud detection systems that adapt to evolving threats, ensuring a higher return on investment for our clients.
7.3. Customer Segmentation
Customer segmentation is a strategic approach that involves dividing a customer base into distinct groups based on shared characteristics. This practice allows businesses to tailor their marketing efforts and improve customer engagement.
- Enables personalized marketing campaigns that resonate with specific customer groups. Â
- Increases customer retention by addressing the unique needs of different segments. Â
- Utilizes data analytics to identify trends and preferences within each segment. Â
- Supports targeted product development based on the demands of specific demographics. Â
- Enhances customer service by allowing businesses to anticipate and meet customer expectations. Â
By leveraging customer segmentation, organizations can optimize their marketing strategies, leading to improved sales and customer satisfaction. Rapid Innovation employs advanced AI-driven analytics to help businesses effectively segment their customers, ensuring that marketing efforts are both efficient and impactful.
7.4. Predictive Analytics
Predictive analytics is a powerful tool that leverages historical data, statistical algorithms, and machine learning techniques to identify the likelihood of future outcomes. In various industries, predictive analytics is transforming decision-making processes by providing insights that were previously unattainable.
- Enhances decision-making: By analyzing trends and patterns, organizations can make informed decisions that align with their strategic goals. Rapid Innovation employs advanced predictive analytics to help clients optimize their strategies, leading to improved ROI.
- Improves customer experience: Businesses can anticipate customer needs and preferences, leading to personalized services and increased satisfaction. Our AI-driven solutions enable clients to tailor their offerings, enhancing customer loyalty and retention.
- Optimizes operations: Predictive analytics can forecast demand, allowing companies to manage inventory and resources more effectively. Rapid Innovation assists clients in streamlining their operations, resulting in cost savings and increased efficiency.
- Reduces risks: By identifying potential issues before they arise, organizations can mitigate risks and avoid costly mistakes. Our predictive models empower clients to proactively address challenges, safeguarding their investments.
- Drives innovation: Insights gained from predictive analytics can inspire new products and services, keeping companies competitive in their markets. Rapid Innovation collaborates with clients to harness these insights, fostering a culture of innovation.
Industries such as healthcare, finance, and retail are increasingly adopting predictive analytics to enhance their operations. For instance, in healthcare, predictive analytics in healthcare can forecast patient admissions, enabling better resource allocation. In finance, predictive analytics helps in fraud detection by identifying unusual transaction patterns. Retailers use it to optimize pricing strategies and inventory management. Additionally, predictive modeling and analytics are being utilized to create predictive analytical models that drive business strategies. Tools like power bi predictive analytics and azure predictive analytics are becoming essential for organizations looking to leverage data effectively.
7.5. Automated Documentation
Automated documentation refers to the use of technology to create, manage, and store documents without manual intervention. This process streamlines workflows, reduces errors, and saves time, making it an essential component of modern business operations.
- Increases efficiency: Automation speeds up the documentation process, allowing employees to focus on more strategic tasks. Rapid Innovation's automated solutions enhance productivity, enabling clients to achieve their business objectives more effectively.
- Reduces errors: Automated systems minimize human error, ensuring that documents are accurate and consistent. Our technology ensures high-quality documentation, reducing the risk of costly mistakes.
- Enhances compliance: Automated documentation can help organizations adhere to regulatory requirements by maintaining accurate records and audit trails. Rapid Innovation supports clients in navigating compliance challenges, ensuring they meet industry standards.
- Facilitates collaboration: Cloud-based automated documentation tools enable real-time collaboration among team members, regardless of their location. Our solutions promote seamless communication, enhancing teamwork and project outcomes.
- Saves costs: By reducing the need for physical storage and manual labor, organizations can significantly cut down on operational costs. Rapid Innovation's automation strategies lead to substantial cost savings for our clients.
Many industries benefit from automated documentation. In healthcare, electronic health records (EHR) systems streamline patient documentation, improving care coordination. In finance, automated reporting tools help ensure compliance with regulations while providing timely insights. Legal firms utilize document automation to generate contracts and agreements quickly, reducing turnaround times.
8. Compliance and Ethics
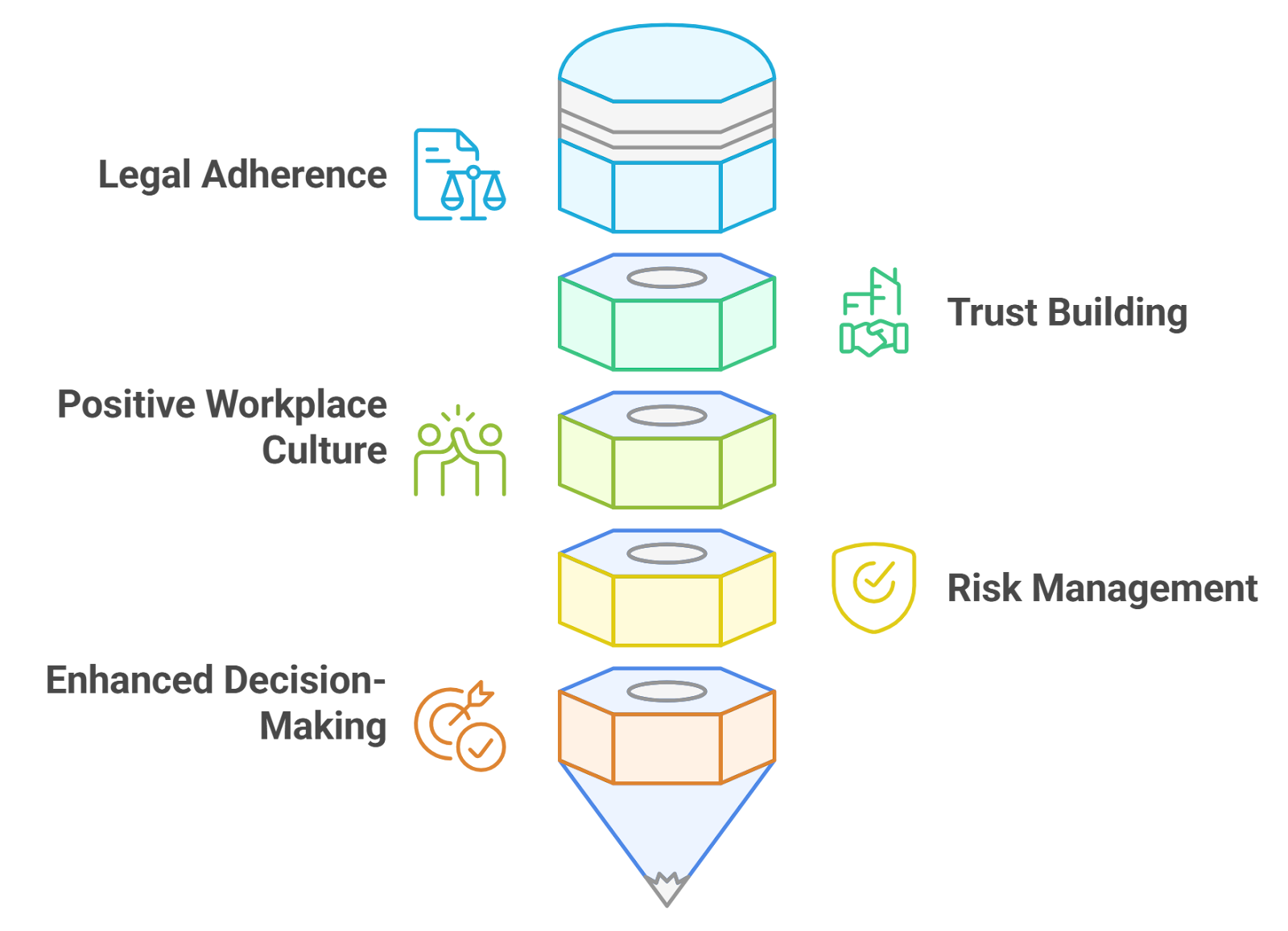
‍
Compliance and ethics are critical components of any organization, ensuring that businesses operate within legal frameworks and uphold moral standards. A strong compliance and ethics program fosters a culture of integrity and accountability, which is essential for long-term success.
- Legal adherence: Compliance ensures that organizations follow laws and regulations relevant to their industry, reducing the risk of legal penalties. Rapid Innovation helps clients implement robust compliance frameworks, safeguarding their operations.
- Builds trust: A commitment to ethical practices enhances an organization’s reputation, fostering trust among customers, employees, and stakeholders. Our consulting services guide clients in establishing ethical standards that resonate with their values.
- Promotes a positive workplace culture: An ethical environment encourages employees to act responsibly and make decisions that align with the organization's values. Rapid Innovation supports clients in cultivating a culture of integrity, enhancing employee engagement.
- Risk management: A robust compliance program identifies potential risks and implements measures to mitigate them, protecting the organization from financial and reputational damage. Our expertise in risk assessment empowers clients to navigate challenges effectively.
- Enhances decision-making: Ethical considerations in decision-making processes lead to more sustainable and socially responsible outcomes. Rapid Innovation assists clients in integrating ethical frameworks into their strategic planning.
Organizations can implement various strategies to strengthen their compliance and ethics programs. These include:
- Developing clear policies and procedures that outline expected behaviors and compliance requirements.
- Providing regular training and resources to employees to ensure they understand their responsibilities.
- Establishing a reporting mechanism for employees to voice concerns or report unethical behavior without fear of retaliation.
- Conducting regular audits and assessments to evaluate the effectiveness of compliance programs and identify areas for improvement.
In conclusion, predictive analytics, automated documentation, and a strong focus on compliance and ethics are essential for organizations aiming to thrive in today’s competitive landscape. By leveraging these tools and principles, businesses can enhance their operations, foster trust, and ensure long-term success. Rapid Innovation is committed to partnering with clients to achieve these goals efficiently and effectively, driving greater ROI and sustainable growth.
8.1. Regulatory Requirements
Regulatory requirements are essential for ensuring that businesses operate within the legal framework set by governments and international bodies. These requirements vary significantly across different sectors and regions, impacting how companies manage their operations, products, and services. Compliance with these regulations, including regulatory compliance and compliance with the regulations, is crucial for maintaining market integrity, protecting consumers, and ensuring fair competition.
Regulatory requirements can include: - Licensing and permits - Reporting and disclosure obligations - Health and safety standards - Environmental regulations - Data protection laws
Understanding and adhering to these requirements is vital for businesses to avoid legal penalties and maintain their reputation, particularly in areas such as legal compliance and compliance & regulatory.
8.1.1. Global Standards
Global standards are established guidelines and criteria that organizations across various industries must follow to ensure quality, safety, and efficiency. These standards are often developed by international organizations and are designed to facilitate trade, enhance consumer protection, and promote sustainable practices.
Key global standards include: - ISO (International Organization for Standardization) standards, which cover a wide range of industries, including quality management (ISO 9001) and environmental management (ISO 14001). - IEC (International Electrotechnical Commission) standards, focusing on electrical and electronic technologies. - WHO (World Health Organization) guidelines for health and safety in various sectors.
Adhering to global standards can provide several benefits, such as improved product quality and safety, enhanced customer satisfaction, and increased market access and competitiveness. Companies that comply with these standards often gain a competitive edge, as they demonstrate their commitment to quality and safety, including compliance regulations by industry.
8.1.2. Regional Regulations
Regional regulations refer to the specific laws and guidelines that govern business operations within a particular geographic area. These regulations can vary widely from one region to another, reflecting local economic, social, and environmental conditions. Businesses must be aware of and comply with these regulations to operate legally and effectively in their respective markets, including banking compliance regulations and industry compliance regulations.
Common types of regional regulations include: - Labor laws, which govern employee rights, wages, and working conditions. - Environmental regulations, which set limits on emissions and waste management practices. - Consumer protection laws, which ensure that products are safe and accurately marketed.
Understanding regional regulations is crucial for businesses because non-compliance can lead to significant fines and legal issues. Adapting to local regulations can enhance a company's reputation and customer trust, while compliance can open up opportunities for government contracts and partnerships, particularly in areas like industrial regulatory compliance and regulatory requirements.
In summary, both global standards and regional regulations play a critical role in shaping the operational landscape for businesses. Companies that prioritize compliance with these requirements, including sox regulatory compliance and pci regulatory compliance, are better positioned for long-term success and sustainability.
At Rapid Innovation, we leverage our expertise in AI and Blockchain to help businesses navigate these regulatory landscapes efficiently. By implementing AI-driven compliance solutions, we enable organizations to automate reporting and monitoring processes, reducing the risk of non-compliance and associated penalties, including regulatory risk. Additionally, our Blockchain solutions enhance transparency and traceability, ensuring that businesses can demonstrate adherence to both global standards and regional regulations effectively. This strategic approach not only mitigates risks but also drives greater ROI by streamlining operations and fostering trust with stakeholders, particularly in the realm of regtech and aws regulatory compliance.
8.1.3. Industry Guidelines
Industry guidelines are essential frameworks that help organizations navigate the complexities of their respective sectors. These guidelines are often developed by regulatory bodies, industry associations, and other stakeholders to ensure industry guidelines compliance, promote best practices, and enhance consumer protection.
- Establish clear standards for operations and practices. Â
- Promote transparency and accountability within the industry. Â
- Encourage ethical behavior among organizations and their employees. Â
- Provide a basis for evaluating performance and compliance. Â
- Facilitate communication and collaboration among industry players. Â
Organizations must stay updated on these guidelines to avoid legal repercussions and maintain a competitive edge. Adhering to industry guidelines can also enhance a company's reputation, fostering trust among consumers and stakeholders. At Rapid Innovation, we leverage our expertise in AI and Blockchain to help clients not only comply with these guidelines but also to implement innovative solutions that streamline operations and enhance transparency, such as AI agents for loan approval.
8.2. Fair Lending Practices
Fair lending practices are crucial for ensuring that all individuals have equal access to credit and financial services, regardless of their background. These practices are designed to prevent discrimination and promote equity in lending.
- Ensure that lending decisions are based on objective criteria, such as creditworthiness and income. Â
- Provide clear and transparent information about loan terms and conditions. Â
- Offer equal treatment to all applicants, regardless of race, gender, or socioeconomic status. Â
- Regularly review lending policies to identify and eliminate potential biases. Â
- Educate staff on fair lending laws and the importance of equitable treatment. Â
By implementing fair lending practices, financial institutions can build stronger relationships with their communities and contribute to economic growth. Compliance with fair lending regulations not only protects consumers but also enhances the institution's reputation and reduces the risk of legal challenges. Rapid Innovation can assist in developing AI-driven solutions that analyze lending patterns and ensure compliance with fair lending practices, ultimately leading to greater ROI.
8.3. Bias Detection and Mitigation
Bias detection and mitigation are critical components in promoting fairness and equity in various sectors, particularly in lending and hiring practices. Identifying and addressing biases can help organizations create a more inclusive environment.
- Utilize data analytics to identify patterns of bias in decision-making processes. Â
- Implement training programs to raise awareness about unconscious biases among employees. Â
- Regularly audit algorithms and models used in decision-making to ensure they are free from bias. Â
- Establish clear protocols for reporting and addressing instances of bias. Â
- Foster a culture of inclusivity where diverse perspectives are valued and considered. Â
By actively working to detect and mitigate bias, organizations can enhance their credibility and foster a more equitable environment. This not only benefits the organization but also contributes to a more just society. At Rapid Innovation, we employ advanced AI techniques to help organizations identify and rectify biases in their processes, ensuring a fairer approach that can lead to improved performance and stakeholder trust.
8.4. Explainability Requirements
Explainability in artificial intelligence (AI) and machine learning (ML) is crucial for building trust and ensuring accountability. As AI systems become more complex, understanding their decision-making processes becomes increasingly important.
- Explainability refers to the ability to describe how an AI model makes decisions in a way that is understandable to humans.
- Regulatory bodies and industry standards are increasingly emphasizing the need for explainable AI, particularly in sectors like finance, healthcare, and autonomous vehicles.
- Explainable AI can help identify biases in algorithms, ensuring fair treatment across different demographic groups.
- Techniques for achieving explainability include: Â
- Model-agnostic methods, such as LIME (Local Interpretable Model-agnostic Explanations) and SHAP (SHapley Additive exPlanations), which provide insights into model predictions.
- Visualization tools that help users understand the features influencing model outcomes.
- Simplifying complex models into more interpretable forms, such as decision trees or linear models, when feasible.
- Organizations should establish clear guidelines for explainability, ensuring that stakeholders can comprehend AI decisions and their implications. At Rapid Innovation, we assist clients in implementing these techniques, enhancing transparency and trust in their AI solutions, ultimately leading to improved stakeholder engagement and greater ROI. This includes the use of explainable AI examples and the integration of explainability in AI practices, such as model explainability and machine learning explainability.
8.5. Privacy Considerations
Privacy considerations are paramount in the development and deployment of AI systems. As AI often relies on large datasets, protecting personal information is essential to maintain user trust and comply with regulations.
- Data privacy laws, such as the General Data Protection Regulation (GDPR) in Europe and the California Consumer Privacy Act (CCPA) in the U.S., impose strict requirements on data handling and user consent.
- Key privacy considerations include: Â
- Data minimization: Collect only the data necessary for the intended purpose.
- Anonymization: Remove personally identifiable information (PII) from datasets to protect individual identities.
- Secure data storage and transmission: Implement encryption and other security measures to safeguard data from unauthorized access.
- Organizations should conduct regular privacy impact assessments to identify potential risks and ensure compliance with relevant regulations.
- Transparency is vital; users should be informed about how their data is collected, used, and shared. Rapid Innovation helps clients navigate these privacy challenges, ensuring compliance and fostering user trust, which is essential for achieving long-term business success.
9. Testing and Validation
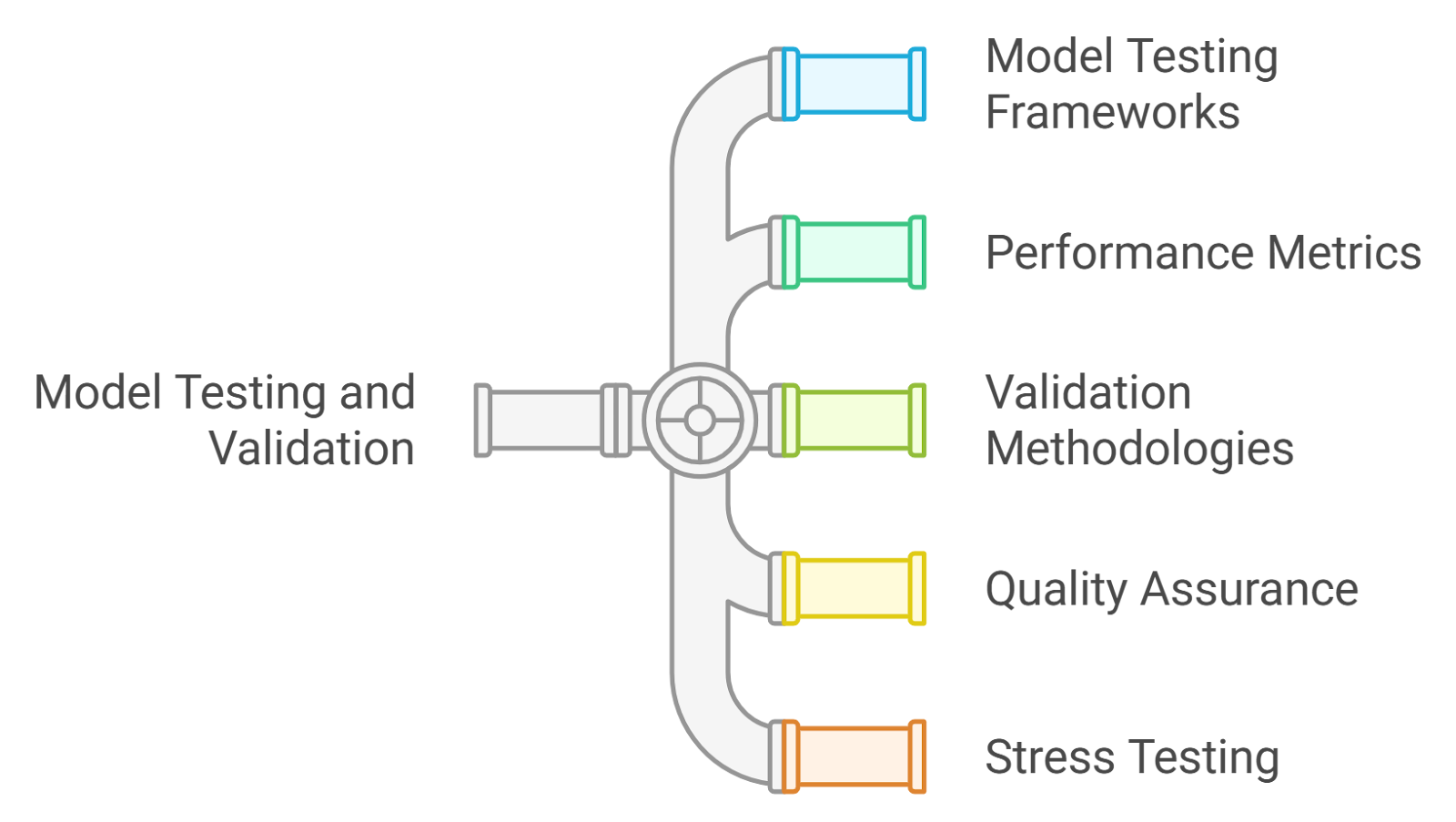
‍
Testing and validation are critical steps in the AI development lifecycle, ensuring that models perform as intended and meet quality standards.
- Testing involves evaluating the model's performance on various datasets to identify strengths and weaknesses.
- Validation ensures that the model generalizes well to unseen data, preventing overfitting and ensuring reliability in real-world applications.
- Key components of testing and validation include: Â
- Cross-validation: A technique that divides the dataset into multiple subsets to train and test the model, providing a more robust evaluation.
- Performance metrics: Use metrics such as accuracy, precision, recall, and F1 score to assess model performance quantitatively.
- Stress testing: Evaluate how the model performs under extreme conditions or with noisy data to ensure robustness.
- Continuous monitoring is essential post-deployment to track model performance and make necessary adjustments.
- Incorporating user feedback can also enhance the validation process, ensuring that the model meets user needs and expectations. At Rapid Innovation, we emphasize rigorous testing and validation processes, ensuring that our clients' AI solutions are not only effective but also reliable, leading to enhanced performance and greater ROI. This includes the application of explainable artificial intelligence (XAI) principles and the use of LIME and SHAP for model evaluation.
9.1. Model Testing Frameworks
Model testing frameworks are essential tools in the development and evaluation of machine learning models. They provide a structured approach to assess the performance and reliability of models before deployment.
- Frameworks help in automating the testing process, ensuring consistency and repeatability.
- They allow for the integration of various testing methodologies, including unit tests, integration tests, and end-to-end tests.
- Popular frameworks include:
- PyTest: A robust testing framework for Python that supports fixtures and parameterized testing.
- unittest: A built-in Python module that provides a simple way to create and run tests.
- TensorFlow Model Analysis: Specifically designed for evaluating TensorFlow models, it provides insights into model performance across different slices of data.
- These frameworks often support continuous integration (CI) practices, enabling developers to run tests automatically with each code change.
- They facilitate the identification of issues such as overfitting, underfitting, and data leakage.
At Rapid Innovation, we leverage these frameworks to ensure that our machine learning models are rigorously tested, including techniques for machine learning model testing and testing machine learning algorithms, leading to higher reliability and performance. By implementing automated testing processes, we help clients reduce time-to-market and enhance the overall quality of their AI solutions, ultimately driving greater ROI. Additionally, we explore the role of AI agents in software testing to further enhance our testing capabilities.
9.2. Performance Metrics
Performance metrics are critical for evaluating the effectiveness of machine learning models. They provide quantitative measures that help in understanding how well a model performs on a given task.
- Common performance metrics include:
- Accuracy: The ratio of correctly predicted instances to the total instances.
- Precision: The ratio of true positive predictions to the total predicted positives, indicating the quality of positive predictions.
- Recall (Sensitivity): The ratio of true positive predictions to the total actual positives, reflecting the model's ability to identify relevant instances.
- F1 Score: The harmonic mean of precision and recall, providing a balance between the two.
- ROC-AUC: The area under the receiver operating characteristic curve, measuring the model's ability to distinguish between classes.
- The choice of metrics depends on the specific problem and the business objectives. For instance, in medical diagnosis, recall may be prioritized to ensure that most positive cases are identified.
- It's essential to analyze metrics in conjunction with each other to get a comprehensive view of model performance. Relying on a single metric can be misleading.
At Rapid Innovation, we assist clients in selecting the most relevant performance metrics tailored to their specific business goals, including accuracy testing in machine learning and test accuracy machine learning. By providing detailed analyses of these metrics, we empower organizations to make informed decisions, optimize their models, and ultimately achieve a higher return on investment.
9.3. Validation Methodologies
Validation methodologies are crucial for ensuring that machine learning models generalize well to unseen data. They help in assessing the robustness and reliability of models before they are deployed in real-world applications.
- Common validation methodologies include:
- Train-Test Split: Dividing the dataset into two parts, one for training the model and the other for testing its performance.
- K-Fold Cross-Validation: The dataset is divided into 'k' subsets. The model is trained on 'k-1' subsets and tested on the remaining subset, repeating this process 'k' times to ensure every data point is used for both training and testing.
- Stratified K-Fold Cross-Validation: Similar to K-Fold but ensures that each fold has the same proportion of classes as the entire dataset, which is particularly useful for imbalanced datasets.
- Leave-One-Out Cross-Validation (LOOCV): A special case of K-Fold where 'k' equals the number of data points. Each instance is used once as a test set while the rest serve as the training set.
- These methodologies help in identifying issues such as overfitting and underfitting, ensuring that the model performs well on unseen data.
- It's important to choose the right validation methodology based on the dataset size, model complexity, and specific use case to achieve reliable results.
Rapid Innovation employs these validation methodologies to ensure that our clients' machine learning models are robust and reliable. By carefully selecting the appropriate validation techniques, including testing machine learning models and testing deep learning models, we help organizations mitigate risks associated with model deployment, leading to improved performance and increased ROI.
9.4. Quality Assurance
Quality Assurance (QA) is a systematic process that ensures products and services meet specified requirements and standards. In the context of software development, QA plays a crucial role in delivering high-quality applications that satisfy user needs and function correctly.
- QA involves various activities, including: Â
- Requirement analysis to ensure clarity and completeness.
- Test planning to define the scope and approach of testing.
- Test case design to create detailed scenarios for validation.
- Test execution to run the tests and document results.
- Defect tracking to identify, report, and resolve issues.
- The importance of QA cannot be overstated: Â
- It helps in identifying defects early in the development cycle, reducing costs associated with fixing issues later.
- QA enhances user satisfaction by ensuring that the final product is reliable and performs as expected.
- It contributes to compliance with industry standards and regulations, which is essential for many businesses.
- Various QA methodologies exist, including: Â
- Manual testing, where testers execute test cases without automation, such as qa manual testing.
- Automated testing, which uses scripts and tools to perform tests efficiently, including quality assurance automation.
- Continuous testing, integrated into the DevOps pipeline to ensure ongoing quality.
- Effective QA practices lead to: Â
- Improved product quality and performance, as seen in software quality assurance.
- Reduced time to market due to fewer defects.
- Enhanced team collaboration and communication, particularly in software quality assurance engineers.
At Rapid Innovation, we leverage advanced AI-driven testing tools to enhance our QA processes. By automating repetitive tasks and utilizing machine learning algorithms, we can identify potential defects more efficiently, ultimately leading to a higher return on investment (ROI) for our clients. Our approach includes qa testing and software quality control to ensure comprehensive coverage.
9.5. Stress Testing
Stress Testing is a type of performance testing that evaluates how a system behaves under extreme conditions. The goal is to determine the system's breaking point and ensure it can handle high loads without failure.
- Key aspects of stress testing include: Â
- Simulating high traffic or data loads to assess system performance.
- Identifying bottlenecks and weaknesses in the application.
- Evaluating system recovery and stability after stress conditions.
- Stress testing is essential for several reasons: Â
- It helps ensure that applications can handle peak usage times, such as during sales events or product launches.
- It provides insights into how the system behaves under stress, allowing for better resource allocation and optimization.
- It aids in identifying potential failure points, enabling proactive measures to enhance system resilience.
- Common techniques used in stress testing are: Â
- Load testing, which gradually increases the load on the system until it reaches its limits.
- Spike testing, which suddenly increases the load to simulate unexpected traffic surges.
- Endurance testing, which evaluates system performance over an extended period under a sustained load.
- The benefits of stress testing include: Â
- Increased confidence in system reliability and performance.
- Enhanced user experience by minimizing downtime and performance issues.
- Better preparedness for unexpected traffic scenarios.
At Rapid Innovation, we employ sophisticated stress testing methodologies to ensure that our clients' applications can withstand high traffic and data loads. This proactive approach not only enhances system reliability but also contributes to greater customer satisfaction and retention, ultimately driving ROI.
10. Business Impact Analysis
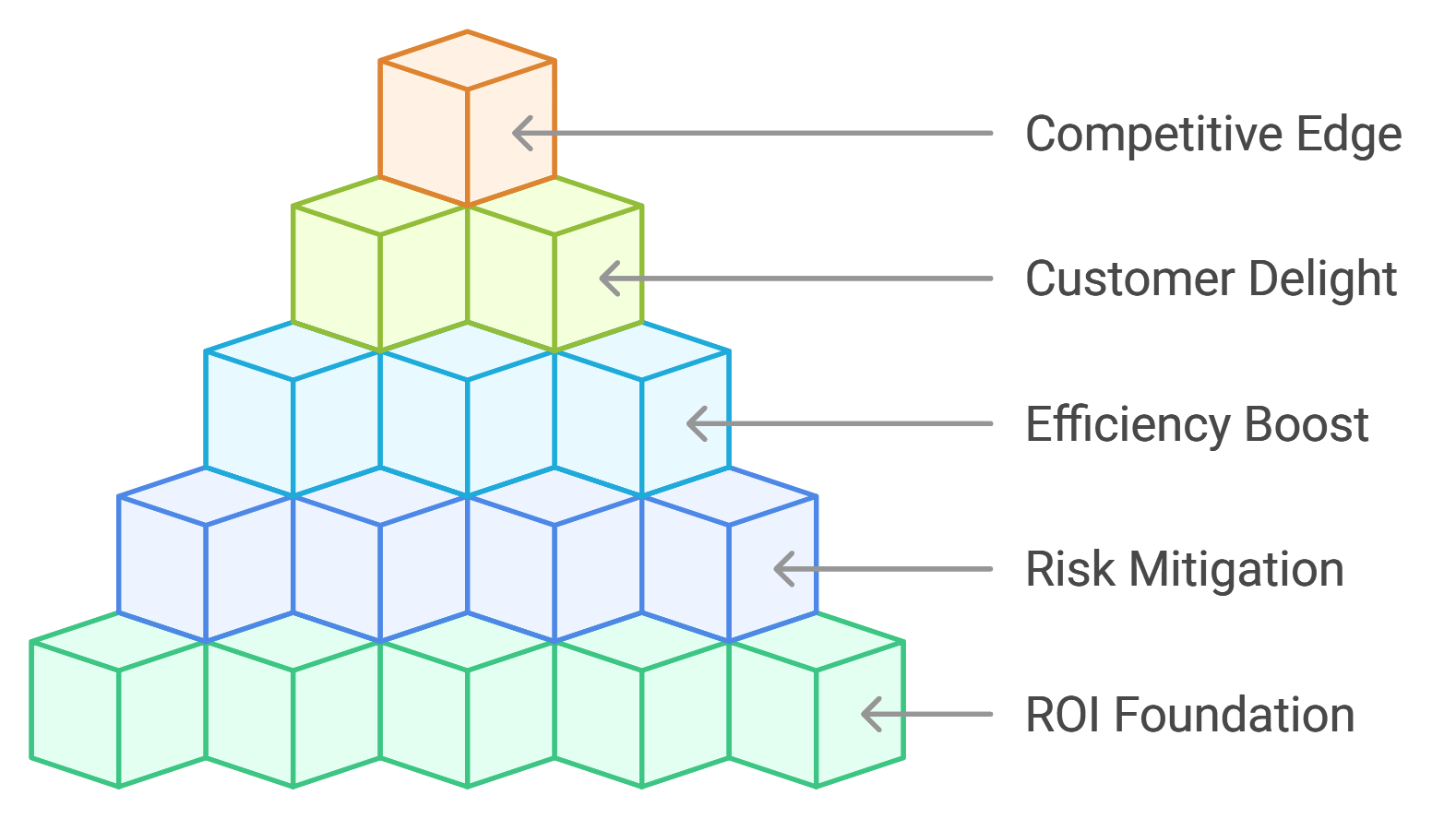
‍
Business Impact Analysis (BIA) is a critical process that helps organizations identify and evaluate the potential effects of disruptions on business operations. It is an essential component of risk management and disaster recovery planning.
- The BIA process typically involves: Â
- Identifying critical business functions and processes.
- Assessing the potential impact of disruptions on these functions.
- Determining recovery time objectives (RTO) and recovery point objectives (RPO) for each function.
- Prioritizing recovery efforts based on the analysis.
- The significance of BIA includes: Â
- Understanding the financial implications of disruptions, which can inform resource allocation and risk mitigation strategies.
- Enhancing organizational resilience by preparing for potential threats and minimizing downtime.
- Supporting compliance with regulatory requirements that mandate business continuity planning.
- Key components of a successful BIA are: Â
- Stakeholder engagement to gather insights and perspectives from various departments.
- Data collection through surveys, interviews, and document reviews to ensure comprehensive analysis.
- Scenario analysis to evaluate different types of disruptions, such as natural disasters, cyberattacks, or supply chain failures.
- The outcomes of a BIA can lead to: Â
- Development of effective business continuity plans that outline recovery strategies.
- Improved communication and coordination among teams during a crisis.
- Enhanced decision-making capabilities by providing a clear understanding of risks and impacts.
By implementing robust QA processes, including software quality assurance sqa and quality assurance and testing, conducting thorough stress testing, and performing comprehensive business impact analyses, organizations can significantly improve their operational resilience and ensure long-term success. At Rapid Innovation, our expertise in AI and Blockchain technologies allows us to provide tailored solutions that enhance these processes, ultimately driving greater ROI for our clients.
10.1. ROI Measurements
Return on Investment (ROI) is a critical metric for evaluating the effectiveness of investments in various business initiatives. It helps organizations determine the profitability of their investments and make informed decisions.
- ROI is calculated by dividing the net profit from an investment by the initial cost of the investment, then multiplying by 100 to get a percentage.
- A positive ROI indicates that the investment has generated more income than it cost, while a negative ROI suggests a loss.
- Businesses often use ROI to assess marketing campaigns, technology implementations, and employee training programs. For instance, Rapid Innovation has helped clients implement AI-driven marketing strategies that have resulted in a significant increase in customer engagement and sales, thereby enhancing their ROI.
- It is essential to consider both direct and indirect benefits when calculating ROI, as some investments may yield long-term gains that are not immediately apparent. Our blockchain solutions, for example, can streamline supply chain processes, leading to cost savings and improved transparency over time.
- Regularly measuring ROI can help organizations identify successful strategies and areas needing improvement. Rapid Innovation provides analytics tools that enable clients to track their ROI effectively, ensuring they can pivot strategies as needed for optimal performance.
- Understanding ROI meaning is crucial for stakeholders to grasp the financial implications of their investments.
- Calculating ROI accurately is vital for determining return on investment in various projects.
- Organizations often seek to find ROI in their initiatives to ensure they are making sound financial decisions.
- Best investment ROI practices involve thorough analysis and strategic planning to maximize returns.
- Estimating return on investment can help businesses forecast potential gains and losses before committing resources.
- Calculating return on investment should be a regular practice to maintain financial health and growth. Rapid Innovation offers AI business automation solutions that can significantly enhance ROI through efficient process automation. Additionally, AI agents for investment strategy can provide valuable insights to improve investment decisions.
10.2. Risk Reduction Metrics
Risk reduction metrics are essential for organizations to evaluate the effectiveness of their risk management strategies. These metrics help in quantifying the potential risks and the impact of mitigation efforts.
- Common risk reduction metrics include the number of incidents reported, the severity of incidents, and the financial impact of risks.
- Organizations often use risk assessments to identify vulnerabilities and prioritize risk mitigation efforts. Rapid Innovation employs advanced AI algorithms to predict potential risks, allowing clients to proactively address vulnerabilities before they escalate.
- Key Performance Indicators (KPIs) related to risk management can include the percentage of risks mitigated, the time taken to resolve incidents, and the cost of risk management initiatives. Our blockchain solutions enhance data integrity, reducing the likelihood of fraud and associated risks.
- By tracking these metrics, businesses can make data-driven decisions to enhance their risk management frameworks. Rapid Innovation assists clients in developing comprehensive risk management strategies that leverage both AI and blockchain technologies.
- Effective risk reduction not only protects assets but also improves stakeholder confidence and can lead to better financial performance. Our clients have reported increased investor trust and market competitiveness as a result of implementing our risk management solutions.
10.3. Operational Efficiency
Operational efficiency refers to the ability of an organization to deliver products or services in the most cost-effective manner while maintaining high quality. It is a crucial aspect of business performance.
- Key indicators of operational efficiency include production costs, cycle times, and resource utilization rates.
- Streamlining processes through automation and technology can significantly enhance operational efficiency. Rapid Innovation has successfully implemented AI-driven automation solutions that have reduced operational costs for clients while improving service delivery times.
- Regularly reviewing workflows and eliminating bottlenecks can lead to improved productivity and reduced waste. Our consulting services guide organizations in identifying inefficiencies and implementing tailored solutions that drive operational excellence.
- Employee training and development play a vital role in fostering a culture of efficiency, as skilled employees are better equipped to identify and implement improvements. Rapid Innovation offers training programs focused on AI and blockchain technologies, empowering teams to leverage these tools effectively.
- Organizations that prioritize operational efficiency often experience increased customer satisfaction, reduced operational costs, and enhanced competitiveness in the market. By partnering with Rapid Innovation, clients have achieved measurable improvements in their operational metrics, leading to sustainable growth and profitability.
10.4. Customer Satisfaction
Customer satisfaction is a critical component of any successful business strategy. It reflects how well a company's products or services meet or exceed customer expectations. High levels of customer satisfaction can lead to increased loyalty, repeat business, and positive word-of-mouth referrals.
- Understanding customer needs is essential for enhancing satisfaction. Regular surveys and feedback mechanisms can help identify areas for improvement, allowing Rapid Innovation to tailor its AI and Blockchain solutions to better meet client expectations. Implementing customer survey strategy can provide valuable insights into customer preferences.
- Providing excellent customer service is a key factor. Quick response times, knowledgeable staff, and effective problem resolution contribute significantly to customer satisfaction. Rapid Innovation ensures that clients receive timely support and guidance throughout their project lifecycle, which is part of an action plan to increase customer satisfaction.
- Personalization can enhance the customer experience. Tailoring products, services, and communications to individual preferences can make customers feel valued. By leveraging AI, Rapid Innovation can help clients create personalized experiences for their end-users, driving higher satisfaction rates. This aligns with customer satisfaction marketing strategy.
- Consistency in product quality and service delivery is crucial. Customers expect the same level of quality every time they interact with a brand. Rapid Innovation emphasizes quality assurance in its development processes, ensuring that clients receive reliable and high-quality solutions, which is a key aspect of improve customer satisfaction strategy.
- Monitoring customer satisfaction metrics, such as Net Promoter Score (NPS) and Customer Satisfaction Score (CSAT), can provide insights into customer perceptions and areas needing attention. Rapid Innovation utilizes data analytics to track these metrics, enabling continuous improvement in service delivery. Different strategies to measure consumer satisfaction can be employed to enhance this process, including customer care AI solutions.
10.5. Market Competitiveness
Market competitiveness refers to a company's ability to maintain an edge over its rivals in the marketplace. It involves understanding market dynamics, customer preferences, and the competitive landscape.
- Analyzing competitors is vital. Regularly assessing competitors' strengths and weaknesses can help identify opportunities for differentiation. Rapid Innovation assists clients in conducting competitive analysis to refine their strategies and offerings.
- Innovation plays a significant role in competitiveness. Companies that continuously innovate their products or services can attract and retain customers more effectively. Rapid Innovation leverages cutting-edge AI and Blockchain technologies to help clients stay ahead of the curve.
- Pricing strategies are essential. Competitive pricing can attract price-sensitive customers, while premium pricing can position a brand as high-quality. Rapid Innovation provides insights into pricing models that align with market trends and customer expectations.
- Building a strong brand identity can enhance market competitiveness. A well-recognized brand can foster customer loyalty and trust. Rapid Innovation helps clients develop their brand identity through strategic marketing and technology integration.
- Leveraging technology can improve operational efficiency and customer engagement, giving companies a competitive advantage. Rapid Innovation's expertise in AI and Blockchain enables clients to implement innovative solutions that enhance their operational capabilities.
11. Operational Considerations
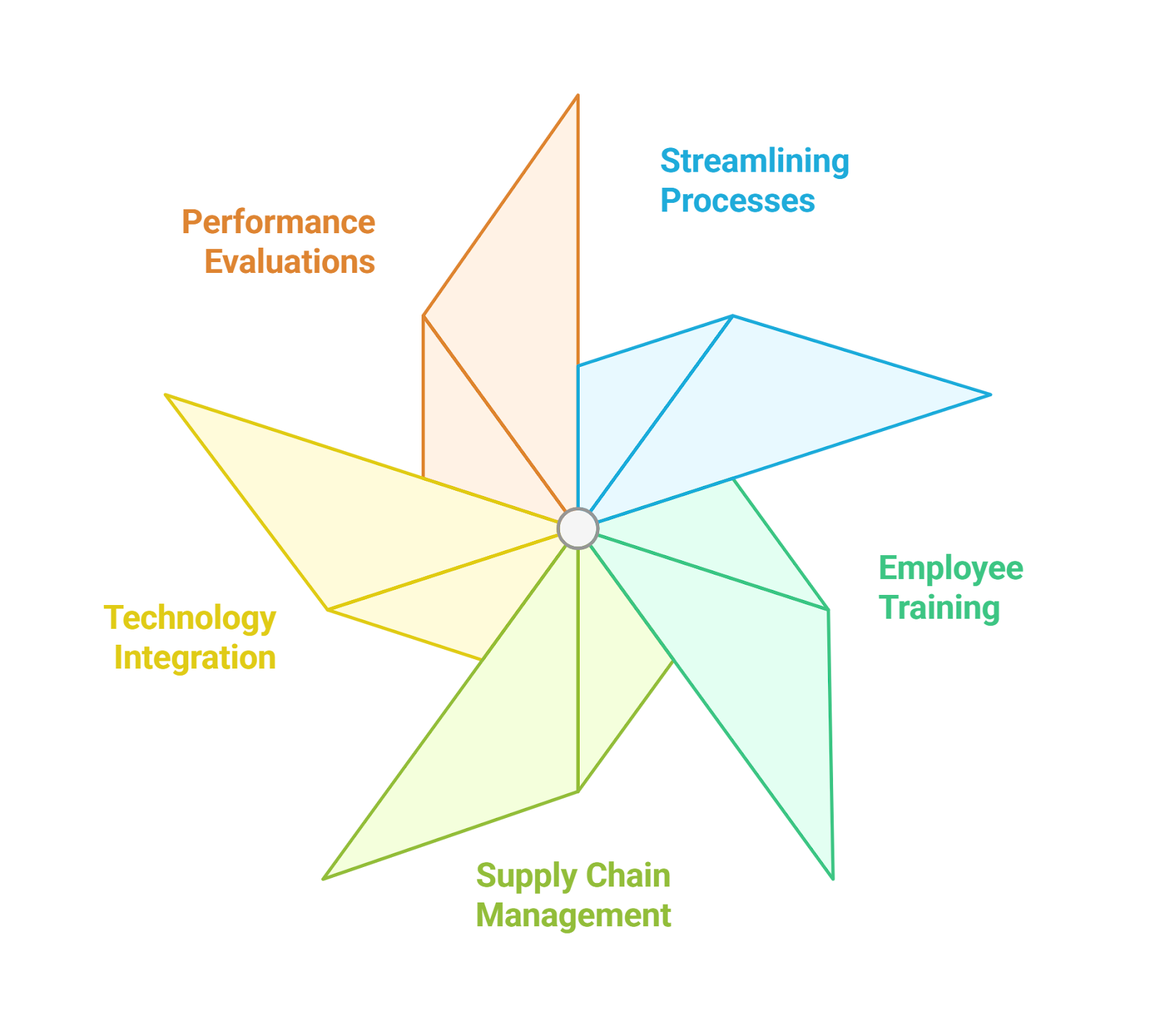
‍
Operational considerations encompass the internal processes and systems that support a business's overall strategy. Efficient operations are crucial for delivering products and services effectively while maintaining quality and cost-effectiveness.
- Streamlining processes can enhance efficiency. Identifying bottlenecks and implementing process improvements can lead to faster service delivery and reduced costs. Rapid Innovation employs AI-driven analytics to optimize client operations.
- Employee training and development are vital. A well-trained workforce is more productive and can provide better customer service. Rapid Innovation offers training programs focused on AI and Blockchain technologies to empower client teams.
- Supply chain management is critical. Effective coordination with suppliers and logistics partners ensures timely delivery of products and minimizes disruptions. Rapid Innovation can assist clients in integrating Blockchain solutions for enhanced supply chain transparency and efficiency.
- Technology integration can improve operational efficiency. Utilizing software solutions for inventory management, customer relationship management (CRM), and data analytics can optimize operations. Rapid Innovation specializes in integrating AI and Blockchain technologies to streamline these processes.
- Regular performance evaluations can help identify areas for improvement. Key performance indicators (KPIs) should be established to measure operational success and guide decision-making. Rapid Innovation collaborates with clients to define and track KPIs that align with their strategic objectives, which is part of customer satisfaction & customer retention strategies.
11.1. Integration with Existing Systems
Integrating new systems with existing infrastructure is crucial for seamless operations. This process involves ensuring that new software or hardware can communicate effectively with current systems. At Rapid Innovation, we leverage our expertise in AI and Blockchain to facilitate this integration, ensuring that our clients can achieve their business goals efficiently.
- Compatibility: Assess the compatibility of new systems with existing software and hardware. This includes checking APIs, data formats, and communication protocols. Our team utilizes advanced AI algorithms to analyze compatibility, ensuring a smooth integration process.
- Data Migration: Plan for data migration to ensure that existing data is transferred accurately and securely to the new system. This may involve data cleansing and validation. We employ Blockchain technology to enhance data integrity during migration, providing our clients with a secure and transparent process.
- Workflow Alignment: Ensure that the new system aligns with current workflows to minimize disruption. This may require mapping out existing processes and identifying areas for improvement. Our consultants work closely with clients to optimize workflows, leveraging AI to identify inefficiencies and recommend enhancements.
- Testing: Conduct thorough testing to identify any integration issues before full deployment. This includes unit testing, system testing, and user acceptance testing. Our rigorous testing protocols ensure that all systems function seamlessly together, reducing downtime and increasing ROI.
- Documentation: Maintain clear documentation of the integration process, including configurations and troubleshooting steps, to assist future maintenance and upgrades. We provide comprehensive documentation that serves as a valuable resource for ongoing support and system enhancements.
11.2. Staff Training Requirements
Effective staff training is essential for the successful adoption of new systems. Proper training ensures that employees are equipped with the necessary skills to utilize the new technology effectively. Rapid Innovation offers tailored training solutions that align with our clients' specific needs, including workday integration training, sap integration suite training, and system integration training.
- Training Needs Assessment: Identify the specific training needs of different staff members based on their roles and responsibilities. This can be done through surveys or interviews. Our team conducts thorough assessments to ensure that training is relevant and impactful.
- Training Formats: Utilize various training formats such as workshops, online courses, and hands-on sessions to cater to different learning styles. We incorporate AI-driven learning platforms that adapt to individual learning paces, enhancing the training experience. Options include workday integration course and salesforce integration training.
- Ongoing Support: Provide ongoing support and resources, such as user manuals and FAQs, to help staff navigate the new system post-training. Our commitment to support ensures that employees feel confident in using new technologies, including premium learning salesforce and cloud platform integration training.
- Feedback Mechanism: Implement a feedback mechanism to gather insights from staff about the training process and areas for improvement. This continuous feedback loop allows us to refine training programs and maximize effectiveness.
- Continuous Learning: Encourage a culture of continuous learning by offering refresher courses and updates on system enhancements. We promote ongoing education to keep staff informed about the latest advancements in AI and Blockchain technologies, including ims lead auditor training and oracle ics certification.
11.3. Change Management
Change management is a structured approach to transitioning individuals, teams, and organizations from a current state to a desired future state. It is vital for minimizing resistance and ensuring a smooth transition. Rapid Innovation employs strategic change management practices to support our clients through this process.
- Communication Strategy: Develop a clear communication strategy to inform all stakeholders about the changes, benefits, and impacts. Regular updates can help alleviate concerns. Our approach ensures that all parties are informed and engaged throughout the transition.
- Stakeholder Engagement: Involve key stakeholders early in the process to gain their support and address any potential resistance. This can include managers, team leaders, and end-users. We facilitate workshops and discussions to foster collaboration and buy-in.
- Training and Resources: Provide adequate training and resources to help staff adapt to the changes. This includes access to training materials and support channels, such as peoplesoft integration broker training and ims lead auditor course online. Our comprehensive training programs are designed to empower employees during transitions.
- Monitoring and Evaluation: Establish metrics to monitor the effectiveness of the change management process. Regular evaluations can help identify areas needing adjustment. We utilize data analytics to track progress and make informed decisions.
- Celebrate Success: Recognize and celebrate milestones and successes throughout the change process to boost morale and encourage continued engagement. Acknowledging achievements fosters a positive environment and reinforces commitment to the new systems.
At Rapid Innovation, our expertise in AI and Blockchain positions us to help clients navigate these critical processes, ultimately leading to greater ROI and enhanced operational efficiency.
11.4. Support and Maintenance
Support and maintenance are critical components of any system or software lifecycle. They ensure that the system remains functional, secure, and up-to-date.
- Ongoing Support: This includes technical assistance for users, troubleshooting issues, and providing solutions to problems that may arise during the use of the system. Support can be offered through various channels such as phone, email, or live chat, ensuring that clients receive timely assistance. This may also involve hardware maintenance and network maintenance support to address any underlying issues.
- Regular Updates: Software and systems require regular updates to fix bugs, patch security vulnerabilities, and introduce new features. These updates help maintain the system's performance and security, ultimately leading to a more reliable user experience. Regular hardware maintenance is also essential to ensure that physical components are functioning optimally.
- Performance Monitoring: Continuous monitoring of system performance is essential. This involves tracking metrics such as uptime, response time, and user satisfaction to ensure the system operates efficiently. Rapid Innovation employs advanced analytics to provide insights that can drive improvements, including network maintenance and support to enhance connectivity.
- Documentation: Comprehensive documentation is vital for both users and support teams. It should include user manuals, troubleshooting guides, and FAQs to facilitate easier use and maintenance of the system, thereby reducing the need for support interventions. Documentation should also cover third party hardware maintenance procedures to assist in external support scenarios.
- User Training: Providing training sessions for users can enhance their understanding of the system, leading to better utilization and fewer support requests. This proactive approach helps clients maximize their investment in technology, including IT hardware maintenance training for staff.
- Feedback Mechanism: Establishing a feedback loop allows users to report issues and suggest improvements. This can help in refining the system and enhancing user experience, ensuring that the solutions provided by Rapid Innovation evolve with client needs. Feedback on server support and maintenance can be particularly valuable for ongoing improvements, including the use of AI agents for technical troubleshooting.
11.5. Disaster Recovery
Disaster recovery (DR) is a crucial aspect of business continuity planning. It involves strategies and processes to recover from disruptive events, ensuring minimal downtime and data loss.
- Risk Assessment: Identifying potential risks and vulnerabilities is the first step in disaster recovery planning. This includes natural disasters, cyberattacks, and hardware failures, allowing organizations to prepare effectively. Regular assessments of third party hardware maintenance contracts can also mitigate risks.
- Backup Solutions: Regular data backups are essential. Organizations should implement automated backup solutions to ensure that data is consistently saved and can be restored quickly, safeguarding against data loss. This may include server maintenance support to ensure backup systems are operational.
- Recovery Plan: A well-documented recovery plan outlines the steps to be taken in the event of a disaster. This plan should include roles and responsibilities, communication strategies, and recovery timelines, ensuring a coordinated response. The plan should also address hardware and maintenance contingencies.
- Testing the Plan: Regularly testing the disaster recovery plan is vital to ensure its effectiveness. Simulated disaster scenarios can help identify weaknesses and areas for improvement, allowing organizations to refine their strategies. Testing should include scenarios involving network maintenance support.
- Redundancy: Implementing redundancy in critical systems and data storage can help ensure that operations can continue even if one component fails, enhancing overall resilience. This is particularly important for data center hardware maintenance.
- Cloud Solutions: Utilizing cloud services for disaster recovery can provide flexibility and scalability. Cloud-based DR solutions often offer automated backups and quick recovery options, making them an attractive choice for modern businesses, especially those relying on server support and maintenance.
12. Future Developments
Future developments in technology and systems are essential for staying competitive and meeting evolving user needs. Organizations must be proactive in planning for these advancements.
- Emerging Technologies: Keeping an eye on emerging technologies such as artificial intelligence, machine learning, and blockchain can provide opportunities for innovation and efficiency. Rapid Innovation leverages these technologies to create solutions that drive business growth, including advancements in hardware maintenance.
- User-Centric Design: Future developments should focus on enhancing user experience. This includes intuitive interfaces, personalized features, and accessibility improvements, ensuring that technology serves the user effectively.
- Integration Capabilities: As businesses adopt more tools and platforms, ensuring that systems can integrate seamlessly with other applications will be crucial for operational efficiency. Rapid Innovation specializes in creating interoperable solutions that enhance productivity, including integration with existing hardware and maintenance systems.
- Sustainability Initiatives: Future developments should also consider environmental impact. Implementing sustainable practices in technology can enhance brand reputation and meet regulatory requirements, aligning with corporate social responsibility goals.
- Agile Methodologies: Adopting agile methodologies in development processes allows for quicker iterations and responsiveness to user feedback, leading to more effective solutions that meet client expectations.
- Cybersecurity Enhancements: As cyber threats evolve, future developments must prioritize robust security measures to protect sensitive data and maintain user trust. Rapid Innovation is committed to integrating advanced security protocols in all solutions, including those related to network maintenance and support.
- Data Analytics: Leveraging data analytics for decision-making can provide insights into user behavior and system performance, guiding future enhancements and strategies. This data-driven approach enables organizations to make informed decisions that enhance ROI, particularly in the context of IT hardware maintenance.
12.1. Emerging Technologies
Emerging technologies are reshaping industries and creating new opportunities for businesses. These innovations often lead to increased efficiency, enhanced customer experiences, and new business models. Key emerging technologies include:
- Artificial Intelligence (AI) and Machine Learning: AI is revolutionizing data analysis, enabling businesses to make informed decisions based on predictive analytics. Machine learning algorithms can automate processes, improving operational efficiency. At Rapid Innovation, we leverage new AI technology to develop tailored solutions that enhance decision-making and drive greater ROI for our clients.
- Internet of Things (IoT): IoT connects devices and systems, allowing for real-time data collection and analysis. This technology is particularly impactful in sectors like manufacturing, healthcare, and smart cities, where it enhances monitoring and control. Our expertise in IoT solutions helps clients optimize operations and reduce costs through improved data insights.
- Blockchain: Originally developed for cryptocurrencies, blockchain technology is now being utilized for secure transactions and data integrity across various industries, including finance, supply chain, and healthcare. Rapid Innovation specializes in blockchain development, enabling businesses to enhance transparency, security, and efficiency in their operations, ultimately leading to a higher return on investment.
- Augmented Reality (AR) and Virtual Reality (VR): These technologies are transforming customer engagement and training processes. Retailers use AR to enhance shopping experiences, while VR is employed in simulations for training purposes. Our team at Rapid Innovation creates immersive AR and VR solutions that elevate customer interactions and improve training outcomes.
- 5G Technology: The rollout of 5G networks is set to enhance connectivity, enabling faster data transfer and supporting the growth of IoT devices. This technology will facilitate advancements in telemedicine, autonomous vehicles, and smart infrastructure. Rapid Innovation is at the forefront of integrating 5G capabilities into our solutions, ensuring clients can capitalize on the benefits of enhanced connectivity.
12.2. Market Trends
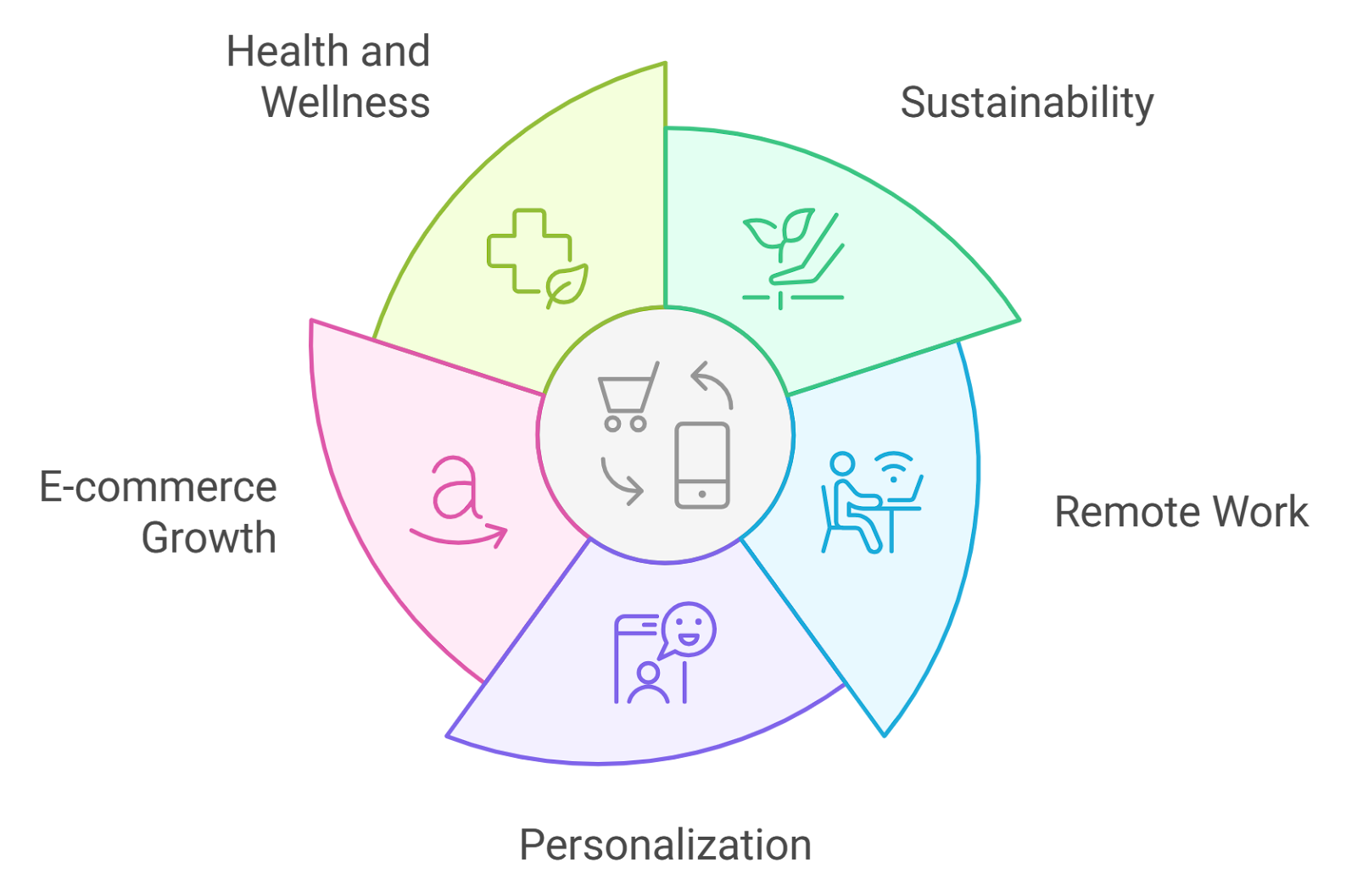
‍
Market trends reflect the evolving preferences and behaviors of consumers and businesses. Understanding these trends is crucial for companies to remain competitive. Some notable market trends include:
- Sustainability: Consumers are increasingly prioritizing eco-friendly products and practices. Businesses are responding by adopting sustainable practices, such as reducing waste and sourcing materials responsibly. Rapid Innovation assists clients in implementing sustainable technologies that not only meet regulatory requirements but also resonate with environmentally conscious consumers.
- Remote Work: The COVID-19 pandemic accelerated the shift towards remote work. Companies are now investing in technology to support hybrid work environments, leading to changes in office space design and employee engagement strategies. Our solutions help organizations adapt to this new landscape, enhancing collaboration and productivity.
- Personalization: Customers expect personalized experiences tailored to their preferences. Businesses are leveraging data analytics to create targeted marketing campaigns and customized product offerings. Rapid Innovation employs advanced AI techniques to help clients deliver personalized experiences that drive customer loyalty and increase sales.
- E-commerce Growth: Online shopping continues to rise, driven by convenience and changing consumer habits. Companies are enhancing their digital presence and optimizing their supply chains to meet the demand for fast delivery. Our expertise in e-commerce solutions enables clients to streamline operations and improve customer satisfaction.
- Health and Wellness: There is a growing focus on health and wellness, influencing product development across various sectors. Brands are incorporating health-conscious ingredients and promoting mental well-being in their marketing strategies. Rapid Innovation supports clients in developing health-focused solutions that align with market demands, including emerging technologies in healthcare.
12.3. Regulatory Evolution
Regulatory evolution refers to the changes in laws and regulations that impact industries and businesses. Staying informed about these changes is essential for compliance and strategic planning. Key areas of regulatory evolution include:
- Data Privacy: With the rise of digital technologies, data privacy regulations are becoming more stringent. Laws like the General Data Protection Regulation (GDPR) in Europe and the California Consumer Privacy Act (CCPA) in the U.S. are setting standards for data protection and consumer rights. Rapid Innovation helps clients navigate these regulations, ensuring compliance while maximizing data utility.
- Environmental Regulations: Governments are implementing stricter environmental regulations to combat climate change. Businesses are required to adopt sustainable practices and report their environmental impact, influencing operational strategies. Our consulting services guide clients in adopting eco-friendly technologies that align with regulatory expectations.
- Financial Regulations: The financial sector is experiencing increased scrutiny and regulation, particularly in areas like anti-money laundering (AML) and consumer protection. Companies must adapt to these regulations to avoid penalties and maintain trust. Rapid Innovation provides solutions that enhance compliance and operational efficiency in the financial sector.
- Health and Safety Regulations: The pandemic has led to heightened health and safety regulations across industries. Businesses must implement measures to protect employees and customers, impacting operational procedures and costs. Our expertise in technology solutions supports clients in meeting these evolving health and safety standards.
- Technology Regulations: As emerging technologies like AI and blockchain gain traction, regulatory bodies are developing frameworks to address ethical concerns, security, and accountability. Companies must navigate these evolving regulations to leverage technology responsibly. Rapid Innovation stays ahead of regulatory changes, ensuring our clients can innovate while remaining compliant with the latest technological advancements and emerging it technologies.
12.4. Innovation Opportunities
Innovation opportunities refer to the potential areas where new ideas, products, or processes can be developed to meet market demands or improve existing solutions. Identifying these opportunities is crucial for businesses aiming to stay competitive and relevant in their industries. Here are some key aspects to consider when exploring innovation opportunities:
- Market Gaps: Â
- Analyze current market trends to identify unmet needs.
- Look for customer pain points that existing products do not address.
- Conduct surveys and focus groups to gather insights directly from consumers.
- Technological Advancements: Â
- Stay updated on emerging technologies that can enhance product offerings.
- Explore how artificial intelligence, machine learning, and automation can streamline operations. For instance, Rapid Innovation has helped clients implement AI-driven analytics to optimize supply chain management, resulting in significant cost savings and improved efficiency.
- Investigate the potential of blockchain technology in various sectors, such as finance and supply chain. Our expertise in blockchain has enabled clients to enhance transparency and security in transactions, leading to increased trust and customer satisfaction. Additionally, consider reviewing the business roadmap for custom blockchain development to understand strategic implementation.
- Sustainability Initiatives: Â
- Consider eco-friendly alternatives to traditional products and processes.
- Develop solutions that reduce waste and promote recycling.
- Explore renewable energy sources and their applications in your industry.
- Collaboration and Partnerships: Â
- Engage with startups and innovators to co-develop new solutions.
- Form strategic alliances with other companies to leverage complementary strengths.
- Participate in innovation hubs or incubators to foster creativity and collaboration.
- Customer-Centric Design: Â
- Implement design thinking methodologies to create user-focused products.
- Gather feedback throughout the development process to ensure alignment with customer needs.
- Utilize prototyping and testing to refine ideas before full-scale production.
- Global Market Expansion: Â
- Identify opportunities in emerging markets where demand is growing.
- Adapt products and services to meet local preferences and regulations.
- Leverage digital marketing strategies to reach a broader audience.
- Regulatory Changes: Â
- Monitor changes in regulations that may create new opportunities for innovation, such as the WIOA (Workforce Innovation and Opportunity Act).
- Develop solutions that help businesses comply with new laws and standards.
- Explore how regulatory frameworks can be leveraged to gain a competitive advantage.
- Data-Driven Insights: Â
- Utilize big data analytics to uncover trends and consumer behavior. Rapid Innovation has successfully implemented data analytics solutions that have allowed clients to make informed decisions, leading to increased ROI.
- Implement data-driven decision-making processes to guide innovation efforts.
- Explore predictive analytics to anticipate future market needs.
- Employee Engagement: Â
- Foster a culture of innovation within the organization by encouraging employee input.
- Provide training and resources to help employees develop their ideas, including WIOA training programs.
- Recognize and reward innovative contributions to motivate ongoing creativity.
- Digital Transformation: Â
- Embrace digital tools and platforms to enhance operational efficiency.
- Explore e-commerce opportunities to reach customers directly.
- Invest in cybersecurity measures to protect digital assets and build trust.
- Social Impact: Â
- Identify ways to create products or services that address social issues, potentially through WIOA approved programs.
- Engage in corporate social responsibility initiatives that align with your brand values.
- Collaborate with non-profits or community organizations to drive positive change.
- Continuous Improvement: Â
- Implement a feedback loop to regularly assess and improve existing products.
- Encourage a mindset of experimentation and learning from failures.
- Utilize agile methodologies to adapt quickly to changing market conditions.
By focusing on these areas, businesses can uncover numerous innovation opportunities that not only enhance their offerings but also contribute to long-term growth and sustainability. Rapid Innovation is committed to guiding clients through these opportunities, ensuring they achieve greater ROI and remain at the forefront of their industries, including leveraging WIOA funding and scholarships for workforce development.