Table Of Contents
Category
Artificial Intelligence
AIML
IoT
Blockchain
Healthcare & Medicine
Customer Service
1. Introduction to AI in Claims Management
Artificial Intelligence (AI) is revolutionizing various industries, and the insurance sector is no exception. In claims management, AI claims management agents are transforming the way claims are processed, enhancing efficiency, accuracy, and customer satisfaction. AI technologies, such as machine learning and natural language processing, are being integrated into claims workflows. These technologies help automate repetitive tasks, allowing human agents to focus on more complex issues. Additionally, AI can analyze vast amounts of data quickly, leading to faster decision-making and reduced processing times.
The adoption of AI in claims management is not just a trend; it is becoming a necessity for insurance companies aiming to stay competitive. By leveraging AI, insurers can improve their operational efficiency and provide a better experience for policyholders. Rapid Innovation specializes in implementing AI solutions tailored to the unique needs of insurance firms, ensuring they achieve greater ROI through enhanced operational capabilities.
- AI agents can assist in fraud detection by identifying patterns and anomalies in claims data, significantly reducing losses for insurers.
- They can also enhance customer service through chatbots that provide instant responses to inquiries, improving customer satisfaction and retention.
- The use of AI in claims management can lead to significant cost savings for insurance companies by streamlining processes and reducing manual labor, allowing for reallocation of resources to more strategic initiatives.
As the insurance industry continues to evolve, the integration of AI in claims management will play a crucial role in shaping the future of insurance processing. Rapid Innovation is committed to guiding clients through this transformation, ensuring they harness the full potential of AI to meet their business goals efficiently and effectively.
Refer to the image for a visual representation of the role of AI in claims management:

1.1. The Evolution of Claims Processing
Claims processing has undergone significant changes over the years, evolving from manual, paper-based systems to sophisticated digital platforms.
- Early claims processing relied heavily on paper forms and manual data entry, which was time-consuming and prone to errors.
- The introduction of computers in the 1980s marked the beginning of automation in claims processing, allowing for faster data handling and improved accuracy.
- The 1990s saw the rise of electronic claims submission, which streamlined the process and reduced the need for physical paperwork.
- In the 2000s, the integration of customer relationship management (CRM) systems and data analytics began to enhance the efficiency of claims processing.
- Today, claims processing is increasingly reliant on cloud-based solutions and mobile technology, enabling real-time updates and access to information from anywhere. The emergence of automated claims and claims processing automation has further transformed the landscape, allowing for more efficient handling of insurance claims.
The evolution of claims processing has not only improved efficiency but also enhanced customer satisfaction by reducing turnaround times and increasing transparency. For organizations looking to enhance their claims processing capabilities, AI insurance solutions can provide innovative approaches to streamline operations and improve customer experiences.
1.2. Limitations of Traditional Claims Workflows
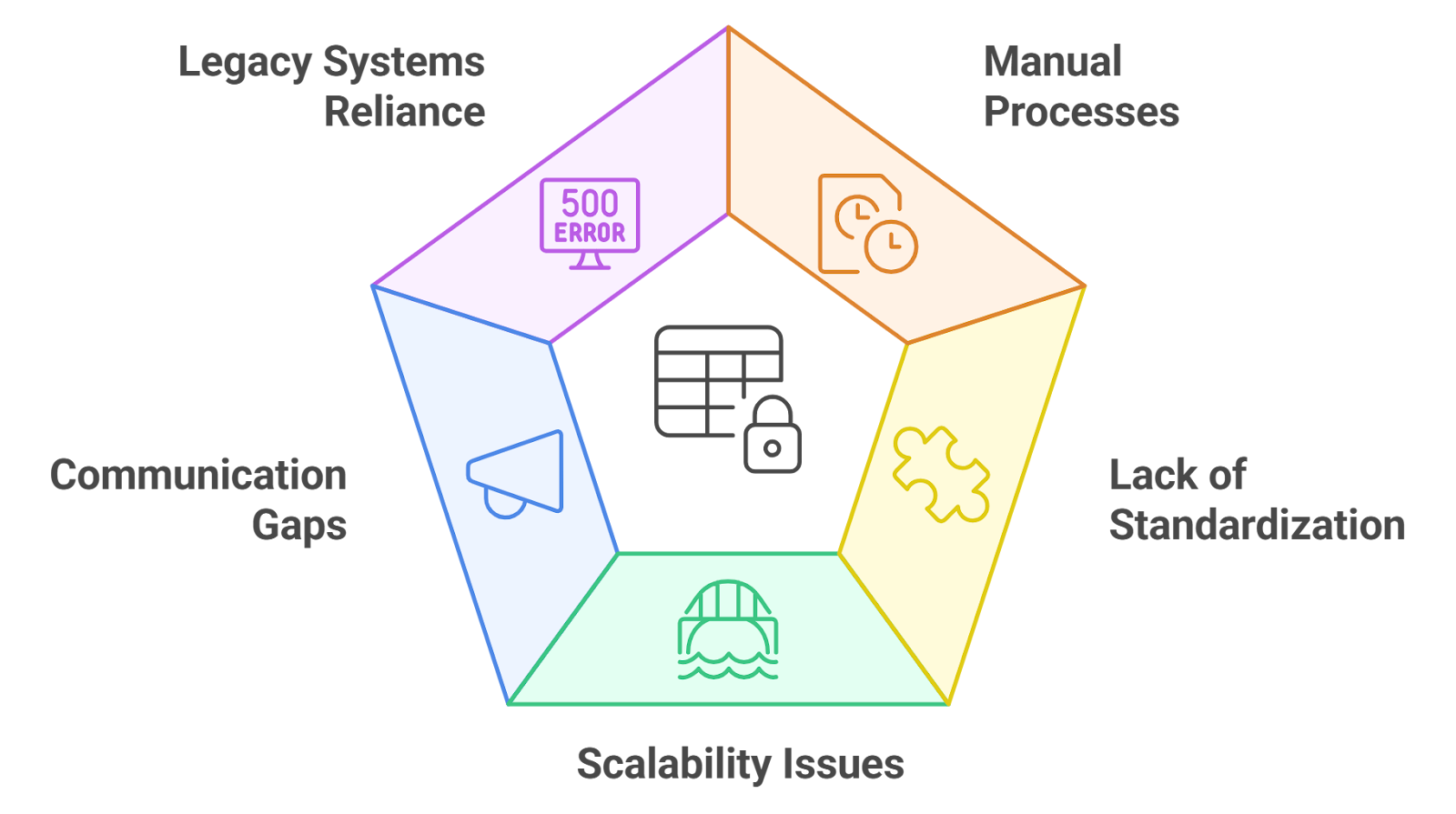
‍
Despite advancements, traditional claims workflows still face several limitations that hinder efficiency and effectiveness.
- Manual processes are still prevalent in many organizations, leading to delays and increased chances of human error.
- Lack of standardization across different systems can result in inconsistent data handling and processing times.
- Traditional workflows often struggle with scalability, making it difficult for organizations to handle spikes in claims volume, such as during natural disasters or pandemics.
- Communication gaps between departments can lead to mismanagement of claims and customer dissatisfaction.
- The reliance on legacy systems can limit the ability to implement new technologies and adapt to changing market demands. The need for automation of insurance claims and claims management automation has become increasingly apparent.
These limitations highlight the need for a more agile and integrated approach to claims processing.
1.3. AI's Transformative Potential
Artificial Intelligence (AI) is poised to revolutionize claims processing by addressing many of the limitations of traditional workflows.
- AI can automate repetitive tasks, such as data entry and document verification, significantly reducing processing times. This includes the automation of healthcare claims and insurance claim automation.
- Machine learning algorithms can analyze vast amounts of data to identify patterns and predict outcomes, improving decision-making in claims approval.
- Natural language processing (NLP) can enhance customer interactions by enabling chatbots to handle inquiries and provide real-time updates on claims status.
- AI-driven analytics can provide insights into claims trends, helping organizations to identify areas for improvement and optimize their workflows. The integration of AI in insurance claims processing, including ai insurance claims processing, can lead to more personalized customer experiences.
- The integration of AI can lead to more personalized customer experiences, as systems can tailor responses and solutions based on individual needs. RPA in insurance claims processing is another avenue that organizations are exploring to enhance efficiency.
By leveraging AI, organizations can not only streamline their claims processing but also enhance overall operational efficiency and customer satisfaction. At Rapid Innovation, we specialize in implementing AI solutions that drive greater ROI for our clients, ensuring they stay ahead in a competitive landscape. Our expertise in AI allows us to customize solutions that address specific business challenges, ultimately leading to improved performance and customer engagement.
Refer to the image for a visual representation of the evolution of claims processing.

1.4. Key Challenges in Insurance Claims Management
Insurance claims management is a critical aspect of the insurance industry, but it faces several challenges that can hinder efficiency and customer satisfaction.
- Complexity of Claims: Insurance claims can be intricate, involving multiple parties, extensive documentation, and various regulations. This complexity can lead to delays and errors in processing, particularly in systems like medical claims processing systems and claims management systems.
- Fraud Detection: Insurance fraud is a significant issue, costing the industry billions annually. Identifying fraudulent claims requires sophisticated tools and techniques, which can be resource-intensive, especially for companies focused on claims management healthcare and automated claims management.
- Customer Experience: Policyholders expect quick and transparent claims processing. Delays or lack of communication can lead to dissatisfaction and damage the insurer's reputation, particularly in sectors like healthcare claims processing companies and insurance claims management services.
- Data Management: Insurers often deal with vast amounts of data from various sources. Managing this data effectively to ensure accurate claims processing is a significant challenge, especially for those utilizing insurance claims management systems and property claims management.
- Regulatory Compliance: The insurance industry is heavily regulated. Keeping up with changing regulations and ensuring compliance can be daunting for claims managers, particularly in the context of workers compensation claims management and claims management in health insurance.
- Integration of Technology: Many insurance companies still rely on legacy systems that are not integrated with modern technologies. This can lead to inefficiencies and increased operational costs, particularly for organizations like claim management companies and outsourced claims management. For more insights on how AI can address these challenges, check out this guide to AI in insurance.
1.5. Paradigm Shift: From Manual to Intelligent Processing
The insurance industry is undergoing a significant transformation as it shifts from manual claims processing to intelligent processing powered by advanced technologies.
- Automation: Intelligent processing utilizes automation to streamline claims management. This reduces the time taken to process claims and minimizes human error, which is crucial for effective claims management in life insurance and auto claims management.
- Artificial Intelligence: AI technologies, such as machine learning and natural language processing, are being integrated into claims management systems. These technologies can analyze data patterns, predict outcomes, and assist in decision-making, enhancing the capabilities of systems like medical claims management and American claims management claims.
- Enhanced Data Analytics: Intelligent processing allows for better data analytics, enabling insurers to gain insights into claims trends and customer behavior. This can lead to more informed decision-making and improved risk assessment, particularly for catastrophe management solutions and TPA claims management.
- Improved Customer Interaction: Chatbots and virtual assistants powered by AI can provide real-time assistance to policyholders, enhancing the customer experience and reducing the workload on claims adjusters, which is vital for companies like Sedgwick insurance claim and Snapsheet claims.
- Cost Efficiency: By reducing manual processes and improving accuracy, intelligent processing can lead to significant cost savings for insurance companies. This can also translate to lower premiums for customers, benefiting those in the insurance claims management companies sector.
- Scalability: Intelligent processing systems can easily scale to handle increased claims volumes, making them more adaptable to market changes and customer demands, especially for organizations like Pinnacle claims management inc and Trident claims management.
2. Foundational AI Technologies
The foundation of intelligent processing in insurance claims management is built on several key AI technologies that enhance efficiency and accuracy.
- Machine Learning: This technology enables systems to learn from data and improve over time. In claims management, machine learning algorithms can identify patterns in claims data, helping to detect fraud and predict claim outcomes, particularly in automated claims management.
- Natural Language Processing (NLP): NLP allows machines to understand and interpret human language. In the context of insurance claims, NLP can be used to analyze customer communications, automate responses, and extract relevant information from documents, enhancing the capabilities of insurance claims management systems.
- Robotic Process Automation (RPA): RPA automates repetitive tasks, such as data entry and document processing. This technology can significantly reduce the time and effort required for manual claims processing, especially in medical claims processing systems.
- Predictive Analytics: This technology uses historical data to forecast future outcomes. In insurance, predictive analytics can help insurers assess risk more accurately and make better underwriting decisions, which is crucial for claims management in health insurance.
- Computer Vision: Computer vision technology can analyze images and videos, which is particularly useful in claims involving property damage. It can assess the extent of damage and streamline the claims evaluation process, benefiting property claims management.
- Blockchain: While still emerging, blockchain technology offers potential benefits for claims management by providing a secure and transparent way to store and share data. This can enhance trust and reduce fraud in the claims process, particularly for catastrophe management solutions.
By leveraging these foundational AI technologies, insurance companies can transform their claims management processes, leading to improved efficiency, reduced costs, and enhanced customer satisfaction. At Rapid Innovation, we specialize in implementing these advanced technologies to help our clients navigate the complexities of insurance claims management, ultimately driving greater ROI and operational excellence.
Refer to the image for a visual representation of the key challenges in insurance claims management.
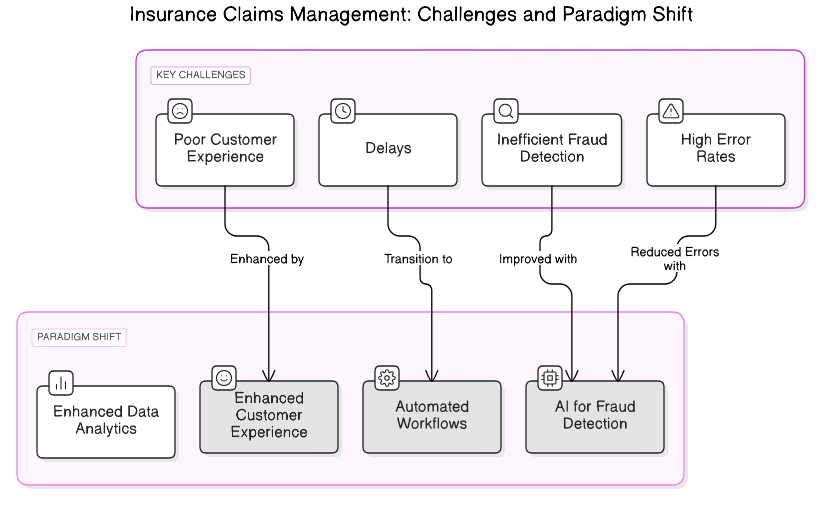
2.1. Machine Learning Fundamentals
Machine learning (ML) is a subset of artificial intelligence (AI) that focuses on the development of algorithms that allow computers to learn from and make predictions based on data. Understanding the fundamentals of machine learning is crucial for anyone looking to delve into this field, and at Rapid Innovation, we leverage these principles to help our clients achieve their business goals efficiently and effectively.
- Types of Machine Learning: Â
- Supervised Learning: Involves training a model on labeled data, where the outcome is known. Common algorithms include linear regression, decision trees, and support vector machines. For instance, we have implemented supervised learning models for clients in the finance sector to enhance fraud detection, resulting in significant cost savings and improved security.
- Unsupervised Learning: Deals with unlabeled data, aiming to find hidden patterns or intrinsic structures. Techniques include clustering and dimensionality reduction. Our work with e-commerce clients has utilized unsupervised learning to develop recommendation systems that increase customer engagement and sales.
- Reinforcement Learning: Involves training models to make sequences of decisions by rewarding desired behaviors and punishing undesired ones. This is often used in robotics and game playing. We have applied reinforcement learning in optimizing supply chain logistics, leading to enhanced operational efficiency.
- Key Concepts: Â
- Features and Labels: Features are the input variables used for prediction, while labels are the output variables.
- Training and Testing: The dataset is typically split into training and testing sets to evaluate the model's performance.
- Overfitting and Underfitting: Overfitting occurs when a model learns the training data too well, capturing noise rather than the underlying pattern. Underfitting happens when a model is too simple to capture the data's complexity. Our expertise in model evaluation ensures that our clients achieve the right balance, maximizing their return on investment.
- Applications: Â
- Fraud detection in finance
- Recommendation systems in e-commerce
- Predictive maintenance in manufacturing
- Machine learning applications in drug discovery
- Machine learning for signal processing
- Designing machine learning systems for embedded systems
- Computer vision software development
Understanding these foundational technologies—machine learning, natural language processing, and computer vision—provides a solid base for exploring advanced applications and innovations in artificial intelligence. At Rapid Innovation, we are committed to harnessing these technologies to drive greater ROI for our clients, ensuring they stay ahead in a competitive landscape. For a deeper exploration of these concepts and technologies, you can refer to our key concepts and technologies in AI.
Refer to the image for a visual representation of the concepts discussed in the Machine Learning Fundamentals section.
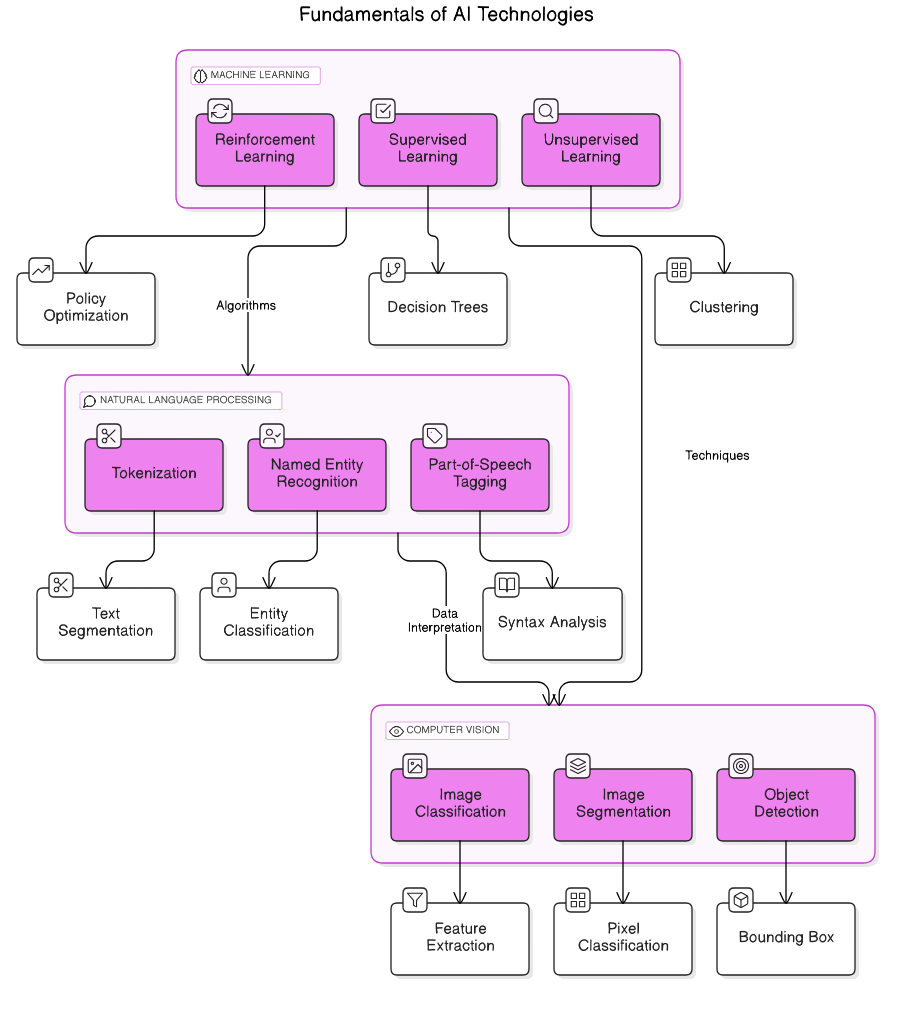
2.4. Predictive Analytics
Predictive analytics is a branch of advanced analytics that uses historical data, statistical algorithms, and machine learning techniques to identify the likelihood of future outcomes. This approach is widely used across various industries to enhance decision-making processes.
- Key components of predictive analytics include: Â
- Data collection: Gathering relevant data from various sources, including databases, sensors, and social media.
- Data processing: Cleaning and transforming data to ensure accuracy and usability.
- Model building: Developing statistical models that can predict future events based on historical data.
- Validation: Testing the model against new data to ensure its predictive power.
- Applications of predictive analytics are vast: Â
- In healthcare: It can predict patient outcomes and optimize treatment plans, leading to better resource allocation and improved patient care. Examples include predictive analytics applications in healthcare and the application of predictive analytics in healthcare.
- In finance: It helps in credit scoring and fraud detection, allowing institutions to minimize risk and enhance security.
- In marketing: Businesses can forecast customer behavior and tailor campaigns accordingly, resulting in higher conversion rates and customer loyalty. This includes the business application of predictive analytics and predictive modeling applications.
- The benefits of predictive analytics include: Â
- Improved decision-making through data-driven insights, enabling organizations to make informed choices that align with their strategic goals.
- Increased efficiency by anticipating trends and behaviors, allowing businesses to allocate resources more effectively.
- Enhanced customer satisfaction by personalizing experiences, which can lead to increased retention and revenue.
At Rapid Innovation, we leverage predictive analytics to help our clients achieve greater ROI by transforming raw data into actionable insights, ultimately driving business growth and operational efficiency. This includes the use of hr predictive analytics tools and crm predictive modeling to enhance our services.
2.5. Deep Learning Architectures
Deep learning architectures are a subset of machine learning that utilize neural networks with many layers (hence "deep") to analyze various forms of data. These architectures are particularly effective in handling large datasets and complex problems.
- Common types of deep learning architectures include: Â
- Convolutional Neural Networks (CNNs): Primarily used for image processing and computer vision tasks, enabling applications such as facial recognition and autonomous vehicles.
- Recurrent Neural Networks (RNNs): Ideal for sequential data, such as time series analysis and natural language processing, facilitating advancements in speech recognition and language translation.
- Generative Adversarial Networks (GANs): Used for generating new data samples that resemble existing data, which can be applied in creative fields like art and music generation.
- Advantages of deep learning architectures: Â
- High accuracy in tasks such as image recognition and language translation, leading to improved user experiences and operational outcomes.
- Ability to learn features automatically from raw data, reducing the need for manual feature extraction and expediting the development process.
- Scalability, allowing models to improve as more data becomes available, ensuring that businesses can adapt to changing market conditions.
- Challenges associated with deep learning include: Â
- The need for large amounts of labeled data for training, which can be resource-intensive.
- High computational power and resources required for model training, necessitating investment in infrastructure.
- Interpretability issues, as deep learning models can be seen as "black boxes," making it difficult to understand their decision-making processes.
Deep learning architectures are revolutionizing fields such as artificial intelligence, enabling advancements in automation, robotics, and more. At Rapid Innovation, we harness these architectures to develop cutting-edge solutions that drive innovation and efficiency for our clients.
2.6. Cognitive Computing Principles
Cognitive computing refers to systems that simulate human thought processes in complex situations. It combines artificial intelligence, machine learning, and natural language processing to create systems that can understand, learn, and interact with humans.
- Core principles of cognitive computing include: Â
- Learning: Systems can learn from data and experiences, improving their performance over time.
- Reasoning: Cognitive systems can analyze data and draw conclusions, mimicking human reasoning to provide insights that inform decision-making.
- Natural language processing: These systems can understand and generate human language, facilitating better communication and interaction with users.
- Applications of cognitive computing are diverse: Â
- In customer service: Chatbots and virtual assistants provide personalized support, enhancing customer engagement and satisfaction.
- In healthcare: Cognitive systems assist in diagnosing diseases and recommending treatments, leading to improved patient outcomes and operational efficiencies.
- In finance: They help in risk assessment and portfolio management, enabling financial institutions to make informed investment decisions.
- Benefits of cognitive computing: Â
- Enhanced decision-making through data analysis and insights, allowing organizations to respond proactively to challenges and opportunities.
- Improved efficiency by automating routine tasks, freeing up human resources for more strategic initiatives.
- Greater personalization in user experiences, which can drive customer loyalty and increase revenue.
Cognitive computing is paving the way for smarter systems that can augment human capabilities, leading to innovative solutions across various sectors. At Rapid Innovation, we are committed to integrating cognitive computing principles into our offerings, helping clients achieve their business goals effectively and efficiently.
3. AI Agent Capabilities in Claims Workflow
AI agents are transforming the claims workflow automation by enhancing efficiency, accuracy, and customer satisfaction. Their capabilities span various stages of the claims process, from initial intake to document processing.
3.1 Initial Claim Intake and Triage
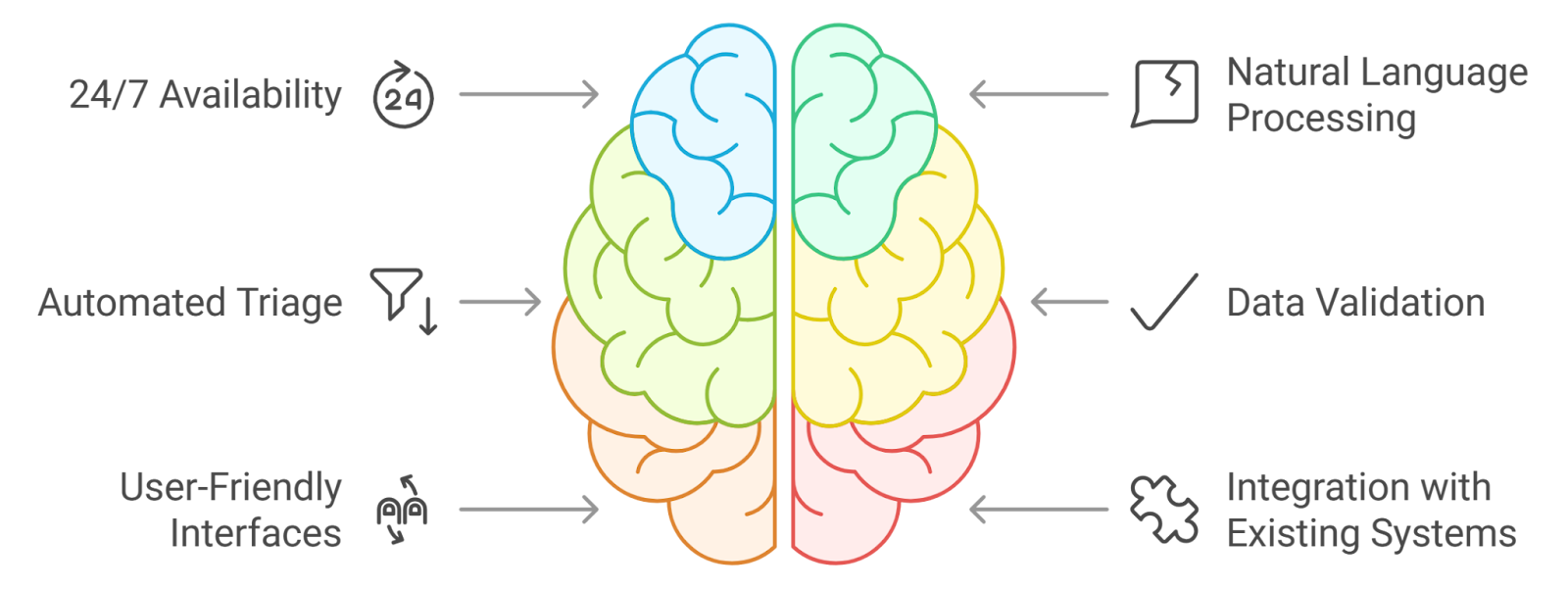
‍
The initial claim intake and triage process is crucial for setting the tone of the entire claims experience. AI agents streamline this phase through several key functionalities:
- 24/7 Availability: AI agents can operate around the clock, allowing claimants to submit their claims at any time. This flexibility improves customer satisfaction and reduces wait times.
- Natural Language Processing (NLP): AI agents utilize NLP to understand and interpret the claimant's language. This capability allows them to accurately capture the details of the claim, regardless of how the information is presented.
- Automated Triage: Once a claim is submitted, AI agents can assess its complexity and urgency. They categorize claims based on predefined criteria, ensuring that high-priority claims are escalated promptly.
- Data Validation: AI agents can automatically validate the information provided by claimants against existing databases. This reduces the likelihood of errors and ensures that only complete and accurate claims move forward in the process.
- User-Friendly Interfaces: Many AI agents are designed with intuitive interfaces that guide users through the claim submission process. This reduces confusion and enhances the overall user experience.
- Integration with Existing Systems: AI agents can seamlessly integrate with existing claims management systems, allowing for a smooth transition of data and minimizing disruptions in workflow.
By leveraging these capabilities, organizations can significantly enhance the initial claim intake and triage process, leading to faster resolutions and improved customer experiences.
3.2 Automated Document Processing
Automated document processing is another critical area where AI agents excel, particularly in the claims workflow automation. This capability encompasses several important functions:
- Document Recognition: AI agents can utilize Optical Character Recognition (OCR) technology to scan and interpret various document types, such as PDFs, images, and handwritten notes. This allows for the extraction of relevant information without manual input.
- Data Extraction: Once documents are recognized, AI agents can extract key data points, such as policy numbers, claimant details, and incident descriptions. This automation reduces the time spent on manual data entry and minimizes human error.
- Classification and Indexing: AI agents can automatically classify and index documents based on their content. This organization makes it easier for claims adjusters to retrieve and review necessary documents quickly.
- Fraud Detection: Advanced AI algorithms can analyze documents for signs of fraud, such as inconsistencies or anomalies in the data. This proactive approach helps organizations mitigate risks associated with fraudulent claims.
- Workflow Automation: AI agents can trigger specific workflows based on the documents processed. For example, if a claim requires additional documentation, the AI can automatically notify the claimant or escalate the issue to a human adjuster.
- Compliance and Audit Trails: Automated document processing ensures that all actions taken on documents are logged, creating a clear audit trail. This is essential for compliance with industry regulations and for maintaining transparency in the claims process.
By implementing automated document processing, organizations can significantly reduce the time and resources required to handle claims, leading to faster resolutions and improved operational efficiency. At Rapid Innovation, we harness these AI capabilities to help our clients achieve greater ROI by streamlining their claims workflow automation and enhancing customer satisfaction.
3.3. Damage Assessment and Evaluation
Damage assessment and evaluation are critical processes in understanding the extent of loss or harm caused by an incident, whether it be natural disasters, accidents, or other unforeseen events. This process involves a systematic approach to quantify and qualify the damage, which is essential for insurance claims, recovery efforts, and future risk management.
- Data Collection: Gathering accurate data is the first step in damage assessment. This includes photographs, videos, and written reports from witnesses or affected parties. Leveraging AI technologies, Rapid Innovation can automate data collection processes, ensuring accuracy and efficiency.
- Site Inspection: Physical inspections of the affected area help assess structural damage, environmental impact, and personal injuries. Trained professionals often conduct these inspections to ensure thorough evaluations. Utilizing drones and AI-powered imaging tools, Rapid Innovation enhances the precision of site inspections, providing real-time data analysis.
- Cost Estimation: After assessing the damage, estimating the repair or replacement costs is crucial. This may involve consulting with contractors, appraisers, or other experts to provide accurate figures. Rapid Innovation employs machine learning algorithms to analyze historical data, enabling more accurate cost predictions and improving budget planning.
- Impact Analysis: Evaluating the broader impact of the damage on the community, economy, and environment is essential. This includes understanding how the incident affects local businesses, infrastructure, and public services. By integrating blockchain technology, Rapid Innovation ensures transparent and tamper-proof records of impact assessments, facilitating better decision-making.
- Reporting: A comprehensive report detailing the findings of the assessment is created. This report serves as a basis for insurance claims, government aid, and recovery planning. Rapid Innovation can streamline the reporting process through automated documentation tools, ensuring timely and accurate submissions.
Effective damage assessment and evaluation can significantly influence recovery efforts and help mitigate future risks.
3.4. Fraud Detection Mechanisms
Fraud detection mechanisms are essential in various sectors, particularly in insurance, finance, and e-commerce. These mechanisms help identify and prevent fraudulent activities, protecting organizations from financial losses and reputational damage.
- Data Analytics: Advanced data analytics tools analyze patterns and anomalies in transactions. By using algorithms and machine learning, organizations can detect unusual behavior that may indicate fraud. Rapid Innovation's AI solutions enhance these capabilities, providing predictive insights that help organizations stay ahead of potential fraud.
- Identity Verification: Implementing robust identity verification processes, such as two-factor authentication and biometric checks, helps ensure that individuals are who they claim to be, reducing the risk of identity theft. Rapid Innovation can develop customized identity verification systems that leverage blockchain for secure and immutable identity management.
- Transaction Monitoring: Continuous monitoring of transactions allows organizations to flag suspicious activities in real-time. This can include large transactions, rapid changes in spending patterns, or transactions from unusual locations. Rapid Innovation's AI-driven monitoring systems provide organizations with real-time alerts, enabling swift action against potential fraud.
- Employee Training: Educating employees about common fraud schemes and red flags can enhance an organization’s ability to detect fraud early. Regular training sessions can keep staff informed about the latest trends in fraudulent activities. Rapid Innovation offers tailored training programs that incorporate AI insights to keep employees updated on emerging threats.
- Collaboration with Law Enforcement: Establishing partnerships with law enforcement agencies can enhance fraud detection efforts. Sharing information and resources can lead to more effective investigations and prosecutions. Rapid Innovation facilitates secure data sharing platforms using blockchain technology, ensuring that sensitive information is protected while fostering collaboration.
Implementing these fraud detection mechanisms can significantly reduce the risk of financial loss and enhance overall security.
3.5. Risk Profiling
Risk profiling is a systematic approach to identifying and assessing potential risks that an organization may face. This process helps organizations understand their vulnerabilities and develop strategies to mitigate those risks effectively.
- Risk Identification: The first step in risk profiling involves identifying potential risks, which can include operational, financial, strategic, and compliance risks. This can be achieved through brainstorming sessions, surveys, and historical data analysis. Rapid Innovation utilizes AI tools to analyze vast datasets, identifying risks that may not be immediately apparent.
- Risk Assessment: Once risks are identified, they must be assessed based on their likelihood and potential impact. This often involves qualitative and quantitative analysis to prioritize risks effectively. Rapid Innovation's advanced analytics capabilities allow organizations to visualize risk scenarios, aiding in informed decision-making.
- Risk Mitigation Strategies: Developing strategies to mitigate identified risks is crucial. This can include implementing controls, transferring risk through insurance, or developing contingency plans. Rapid Innovation collaborates with clients to design tailored risk mitigation strategies that leverage both AI and blockchain technologies for enhanced effectiveness.
- Continuous Monitoring: Risk profiles should be regularly updated to reflect changes in the organization’s environment, operations, and external factors. Continuous monitoring allows organizations to adapt their risk management strategies as needed. Rapid Innovation provides real-time monitoring solutions that utilize AI to detect emerging risks promptly.
- Stakeholder Involvement: Engaging stakeholders in the risk profiling process ensures that diverse perspectives are considered. This can lead to a more comprehensive understanding of risks and better-informed decision-making. Rapid Innovation emphasizes stakeholder collaboration, ensuring that all relevant parties contribute to the risk profiling process.
Effective risk profiling enables organizations to proactively manage risks, ensuring long-term sustainability and resilience.
3.6. Predictive Claim Outcome Modeling
Predictive claim outcome modeling is a powerful tool in the insurance industry that leverages data analytics and machine learning to forecast the results of claims. This approach helps insurers make informed decisions, streamline processes, and enhance customer satisfaction.
- Utilizes historical data: By analyzing past claims data, insurers can identify patterns and trends that predict future outcomes.
- Machine learning algorithms: These algorithms can process vast amounts of data to improve accuracy in predicting claim outcomes.
- Risk assessment: Predictive modeling aids in assessing the risk associated with a claim, allowing for better resource allocation.
- Fraud detection: By identifying anomalies in claims data, predictive models can help flag potentially fraudulent claims.
- Enhanced decision-making: Insurers can make quicker and more accurate decisions regarding claim approvals or denials.
- Improved customer experience: Faster processing times and accurate predictions lead to higher customer satisfaction.
At Rapid Innovation, we harness the power of predictive claim outcome modeling to help our clients in the insurance sector achieve greater ROI. By implementing tailored machine learning solutions, we enable insurers to optimize their claims processes, reduce operational costs, and enhance customer loyalty.
3.7. Intelligent Claims Routing
Intelligent claims routing refers to the automated process of directing claims to the appropriate adjusters or departments based on various criteria. This technology enhances efficiency and ensures that claims are handled by the most qualified personnel.
- Automated decision-making: Algorithms analyze claims data to determine the best route for each claim.
- Skill-based routing: Claims are assigned to adjusters based on their expertise, ensuring that complex claims are handled by specialists.
- Real-time processing: Intelligent routing allows for immediate assignment of claims, reducing wait times for customers.
- Enhanced collaboration: Teams can work together more effectively when claims are routed intelligently, leading to quicker resolutions.
- Data-driven insights: Continuous analysis of routing patterns can help improve the process over time.
- Increased productivity: By minimizing manual intervention, adjusters can focus on resolving claims rather than sorting them.
Rapid Innovation's intelligent claims routing solutions empower insurers to streamline their operations, leading to improved efficiency and reduced claim processing times. Our expertise in AI allows us to create customized routing algorithms that align with our clients' specific needs, ultimately driving higher profitability.
4. Technical Architecture of Claims AI Agents

‍
The technical architecture of claims AI agents is crucial for their functionality and effectiveness in the insurance sector. This architecture encompasses various components that work together to automate and optimize the claims process.
- Data ingestion layer: This layer collects data from multiple sources, including customer interactions, claims submissions, and external databases.
- Processing engine: The processing engine utilizes machine learning algorithms to analyze data, predict outcomes, and make routing decisions.
- User interface: A user-friendly interface allows claims adjusters and customers to interact with the AI agents seamlessly.
- Integration with existing systems: Claims AI agents must integrate with legacy systems to ensure a smooth transition and data flow.
- Feedback loop: Continuous learning is facilitated through a feedback mechanism that allows the AI to improve its predictions and routing over time.
- Security measures: Robust security protocols are essential to protect sensitive customer data and ensure compliance with regulations.
By implementing these components, insurers can create a comprehensive technical architecture that enhances the efficiency and effectiveness of claims processing through AI agents. At Rapid Innovation, we specialize in developing and integrating these advanced AI solutions, ensuring our clients can achieve their business goals efficiently and effectively.
4.1. Agent Design Principles
Agent design principles are fundamental guidelines that inform the development of intelligent agents. These principles ensure that agents are efficient, effective, and capable of adapting to various environments. Key design principles include:
- Autonomy: Agents should operate independently, making decisions based on their programming and the data they collect. This autonomy allows them to function without constant human intervention, enabling businesses to streamline operations and reduce overhead costs.
- Reactivity: Agents must be able to respond to changes in their environment in real-time. This involves monitoring conditions and adapting their actions accordingly to maintain effectiveness, which can significantly enhance customer service and operational efficiency.
- Proactivity: Beyond mere reactivity, agents should anticipate future events and take initiative. This proactive behavior enhances their ability to achieve goals and improve overall performance, leading to increased productivity and better resource allocation.
- Social Ability: Agents should be designed to interact with other agents and humans. This social capability is crucial for collaboration, negotiation, and information sharing, fostering a more integrated approach to problem-solving within organizations.
- Learning: Incorporating machine learning techniques allows agents to improve their performance over time. By analyzing past experiences, agents can adapt their strategies and enhance their decision-making processes, ultimately driving greater ROI for businesses.
- Scalability: The design should accommodate growth, allowing agents to handle increasing amounts of data and interactions without a decline in performance. This scalability is essential for businesses looking to expand their operations without compromising service quality.
- Robustness: Agents must be resilient to failures and capable of recovering from errors. This robustness ensures reliability in various operational conditions, which is critical for maintaining trust and satisfaction among clients and stakeholders.
4.2. Data Integration Frameworks
Data integration frameworks are essential for combining data from different sources into a unified view. These frameworks facilitate seamless data flow and enhance the ability to analyze and utilize information effectively. Key components of data integration frameworks include:
- Data Sources: Integration frameworks can connect to various data sources, including databases, APIs, and cloud services. This diversity allows for comprehensive data collection, enabling businesses to leverage all available information for informed decision-making.
- ETL Processes: Extract, Transform, Load (ETL) processes are critical for data integration. They involve extracting data from sources, transforming it into a suitable format, and loading it into a target system, ensuring that organizations can work with clean and relevant data.
- Data Quality Management: Ensuring data quality is vital for accurate analysis. Integration frameworks often include tools for data cleansing, validation, and enrichment, which are essential for maintaining the integrity of business intelligence efforts.
- Real-Time Integration: Many frameworks support real-time data integration, allowing organizations to access and analyze data as it is generated. This capability is crucial for time-sensitive decision-making, enabling businesses to respond swiftly to market changes.
- Interoperability: Effective data integration frameworks promote interoperability between different systems and applications. This ensures that data can flow freely across platforms, enhancing collaboration and operational efficiency.
- Scalability and Flexibility: Frameworks should be scalable to accommodate growing data volumes and flexible enough to adapt to changing business needs, ensuring that organizations can evolve without being hindered by their data infrastructure.
4.3. Multi-Modal Data Processing
Multi-modal data processing refers to the ability to analyze and interpret data from various modalities, such as text, images, audio, and video. This approach enhances the richness of data analysis and provides deeper insights. Key aspects of multi-modal data processing include:
- Diverse Data Types: Multi-modal processing involves handling different types of data, which can include structured data (like databases) and unstructured data (like social media posts or images). This versatility allows businesses to gain a holistic view of their operations and customer interactions.
- Integration of Modalities: Combining insights from various data modalities allows for a more comprehensive understanding of the subject matter. For example, analyzing text and images together can provide richer context in social media analysis, leading to more effective marketing strategies.
- Advanced Algorithms: Utilizing machine learning and deep learning algorithms is essential for effective multi-modal processing. These algorithms can learn patterns across different data types and improve predictive accuracy, enabling businesses to make data-driven decisions with confidence.
- Applications: Multi-modal data processing has applications in various fields, including healthcare (combining patient records with imaging data), marketing (analyzing customer feedback alongside sales data), and security (integrating video surveillance with audio analysis). This breadth of application demonstrates the versatility and value of multi-modal approaches.
- Challenges: Despite its advantages, multi-modal data processing presents challenges, such as data alignment, varying data quality, and the need for sophisticated models to interpret complex relationships between modalities. Addressing these challenges is crucial for maximizing the benefits of multi-modal data analysis.
- Future Trends: As technology advances, the integration of multi-modal data processing is expected to grow, driven by the increasing availability of diverse data sources and the demand for more nuanced insights. Rapid Innovation is poised to help organizations navigate these trends, ensuring they remain competitive in an evolving landscape.
4.4. Real-time Decision Support Systems
Real-time decision support systems (DSS) are crucial for organizations that require immediate insights to make informed decisions. These systems leverage data analytics, machine learning, and artificial intelligence to provide timely information that can influence operational strategies.
- Instant data processing: Real-time decision support systems can analyze vast amounts of data as it is generated, allowing organizations to respond quickly to changing conditions. For instance, a retail company can adjust inventory levels in real-time based on customer purchasing patterns, optimizing stock and reducing waste.
- Enhanced decision-making: By providing actionable insights, these systems help decision-makers evaluate options and outcomes more effectively. For example, a financial institution can utilize real-time decision support systems to assess risk and make informed lending decisions, ultimately improving profitability.
- Integration with IoT: Real-time decision support systems often integrate with Internet of Things (IoT) devices, enabling organizations to monitor processes and environments continuously. This integration allows manufacturers to track equipment performance and predict maintenance needs, reducing downtime and enhancing productivity.
- Predictive analytics: These systems can forecast trends and behaviors, allowing businesses to anticipate challenges and opportunities. For instance, a healthcare provider can predict patient admission rates, enabling better resource allocation and improved patient care.
- User-friendly interfaces: Many real-time decision support systems come with dashboards that present data visually, making it easier for users to interpret complex information. This accessibility empowers teams across the organization to make data-driven decisions swiftly. Additionally, organizations looking to enhance their machine learning operations can benefit from MLOps consulting services to streamline their processes and improve decision-making capabilities.
4.5. Cloud and Edge Computing Approaches
Cloud and edge computing are two complementary technologies that enhance data processing and storage capabilities. They play a significant role in modern IT infrastructure, especially for businesses that require scalability and flexibility.
- Cloud computing: This approach allows organizations to store and process data on remote servers, providing access to resources over the internet. Benefits include: Â
- Scalability: Businesses can easily scale their operations up or down based on demand. For example, an e-commerce platform can handle increased traffic during peak shopping seasons without investing in physical infrastructure.
- Cost-effectiveness: Organizations can reduce capital expenditures by using pay-as-you-go models, allowing them to allocate resources more efficiently.
- Collaboration: Cloud services facilitate collaboration among teams by providing shared access to data and applications, enhancing productivity and innovation.
- Edge computing: This technology processes data closer to the source, reducing latency and bandwidth usage. Key advantages include: Â
- Faster response times: By processing data locally, edge computing minimizes delays, which is critical for applications like autonomous vehicles and real-time analytics. For instance, smart traffic systems can make instantaneous adjustments to traffic signals based on real-time data.
- Improved reliability: Edge computing can continue to function even when connectivity to the cloud is disrupted, ensuring uninterrupted service for critical applications.
- Enhanced security: By keeping sensitive data closer to its source, organizations can reduce the risk of data breaches, particularly in industries like finance and healthcare.
4.6. Security and Compliance Mechanisms
Security and compliance are paramount in today’s digital landscape, especially as organizations increasingly rely on technology for their operations. Implementing robust security and compliance mechanisms is essential to protect sensitive data and adhere to regulations.
- Data encryption: Encrypting data both at rest and in transit ensures that unauthorized users cannot access sensitive information, safeguarding customer trust and organizational integrity.
- Access controls: Implementing strict access controls helps organizations manage who can view or manipulate data, reducing the risk of insider threats. This is particularly important in sectors like finance, where data sensitivity is high.
- Regular audits: Conducting regular security audits and compliance checks helps organizations identify vulnerabilities and ensure adherence to regulations, thereby mitigating risks associated with data breaches.
- Incident response plans: Having a well-defined incident response plan allows organizations to react swiftly to security breaches, minimizing potential damage and ensuring business continuity.
- Compliance with regulations: Organizations must stay updated on relevant regulations, such as GDPR or HIPAA, to avoid legal penalties and maintain customer trust. Rapid Innovation can assist clients in navigating these complexities, ensuring that their systems are compliant and secure.
By leveraging our expertise in AI and Blockchain technologies, Rapid Innovation empowers organizations to implement these advanced systems effectively, driving greater ROI and achieving business goals efficiently.
5. Implementation Strategies
Effective implementation strategies are crucial for the success of any project, especially in the context of technology and data management. These strategies ensure that organizations are prepared to adopt new systems and processes seamlessly. Below are two key components of implementation strategies: Organizational Readiness Assessment and Data Preparation and Normalization.
5.1 Organizational Readiness Assessment

‍
An Organizational Readiness Assessment is a systematic evaluation of an organization’s capacity to implement change. This assessment helps identify strengths, weaknesses, and areas that require attention before proceeding with a new initiative.
- Identify Stakeholders: Recognizing key stakeholders is essential, including leadership, employees, and any external partners who will be affected by the change.
- Evaluate Current Processes: Assess existing workflows and processes to understand how they align with the proposed changes, helping to identify gaps and areas for improvement.
- Assess Culture and Attitudes: Understanding the organizational culture and employee attitudes towards change can provide insights into potential resistance. Surveys and interviews can be effective tools for this assessment.
- Resource Availability: Evaluate the availability of resources, including financial, human, and technological resources, to ensure that the organization can support the implementation without overextending itself.
- Training Needs: Identify training requirements for staff to ensure they are equipped to handle new systems or processes. A well-planned training program can significantly enhance readiness.
- Change Management Plan: Develop a change management plan that outlines how the organization will communicate changes, manage resistance, and support employees throughout the transition.
Conducting a thorough Organizational Readiness Assessment can lead to a smoother implementation process and increase the likelihood of project success.
5.2 Data Preparation and Normalization
Data Preparation and Normalization are critical steps in ensuring that data is clean, consistent, and ready for analysis. Proper data management enhances the quality of insights derived from data, which is essential for informed decision-making.
- Data Collection: Gather data from various sources, ensuring that it is relevant and comprehensive. This may include internal databases, external datasets, and user-generated content.
- Data Cleaning: Remove inaccuracies, duplicates, and irrelevant information from the dataset. This step is vital to ensure that the data used for analysis is reliable.
- Data Transformation: Convert data into a suitable format for analysis, which may involve changing data types, aggregating data, or creating new calculated fields.
- Normalization: Standardize data to ensure consistency across different datasets. This includes: Â
- Adjusting scales: For example, converting all currency values to a single currency.
- Ensuring uniform naming conventions: Using consistent terminology for categories.
- Handling missing values appropriately: This may involve imputation or removal.
- Data Integration: Combine data from different sources to create a unified dataset, which is crucial for comprehensive analysis and reporting.
- Validation: After preparation and normalization, validate the data to ensure accuracy and completeness. This can involve cross-referencing with original sources or conducting statistical checks.
By focusing on Data Preparation and Normalization, organizations can enhance the quality of their data, leading to more accurate analyses and better decision-making outcomes. At Rapid Innovation, we leverage our expertise in AI and Blockchain to ensure that these implementation strategies, including strategy formulation and implementation, are not only effective but also tailored to meet the unique needs of each client, ultimately driving greater ROI and business success. Additionally, we provide implementation strategy examples and change implementation strategies to guide organizations through their journey. Our approach to strategic planning and implementation ensures that every aspect of the project is aligned with the overall business objectives, making the strategy and implementation process seamless.
5.3. AI Agent Training Methodologies
AI agent training methodologies are crucial for developing effective artificial intelligence systems. These methodologies encompass various techniques and approaches that enable AI agents to learn from data, adapt to new situations, and improve their performance over time.
- Supervised Learning: Â
- Involves training AI agents on labeled datasets.
- The model learns to map inputs to outputs based on examples.
- Commonly used in applications like image recognition and natural language processing.
- Unsupervised Learning: Â
- AI agents learn from unlabeled data.
- The focus is on identifying patterns and structures within the data.
- Useful for clustering and anomaly detection tasks.
- Reinforcement Learning: Â
- AI agents learn through trial and error.
- They receive feedback in the form of rewards or penalties based on their actions.
- This methodology is widely used in robotics and game playing.
- Transfer Learning: Â
- Involves taking a pre-trained model and fine-tuning it for a specific task.
- Reduces the amount of data and time needed for training.
- Particularly effective in domains where labeled data is scarce.
- Federated Learning: Â
- A decentralized approach where multiple devices collaboratively train a model without sharing raw data.
- Enhances privacy and security while leveraging diverse data sources.
- Gaining traction in industries like healthcare and finance.
5.4. Deployment Models
Deployment models refer to the various ways AI systems can be implemented and utilized in real-world applications. The choice of deployment model significantly impacts the performance, scalability, and accessibility of AI solutions.
- Cloud-Based Deployment: Â
- AI models are hosted on cloud platforms, allowing for easy access and scalability.
- Offers flexibility in resource allocation and cost management.
- Ideal for applications requiring high computational power and storage.
- Edge Computing: Â
- AI processing occurs closer to the data source, such as IoT devices.
- Reduces latency and bandwidth usage, enhancing real-time decision-making.
- Suitable for applications in autonomous vehicles and smart cities.
- Hybrid Deployment: Â
- Combines cloud and edge computing to leverage the benefits of both.
- Allows for data processing at the edge while utilizing cloud resources for heavy computations.
- Provides a balanced approach for various use cases.
5.4.1. On-Premise Solutions
On-premise solutions involve deploying AI systems within an organization’s own infrastructure. This model offers several advantages and challenges that organizations must consider.
- Data Security: Â
- Organizations maintain full control over their data, reducing the risk of breaches.
- Compliance with regulations is easier to manage when data is stored on-site.
- Customization: Â
- On-premise solutions can be tailored to meet specific organizational needs.
- Organizations can modify the AI system to align with their unique processes and workflows.
- Performance: Â
- Reduced latency as data processing occurs locally.
- High-speed access to data can enhance the performance of AI applications.
- Cost Considerations: Â
- Initial setup costs can be high due to hardware and software investments.
- Ongoing maintenance and updates require dedicated IT resources.
- Scalability Challenges: Â
- Scaling on-premise solutions can be more complex compared to cloud-based options.
- Organizations may face limitations in hardware capacity and resource allocation.
- Integration Complexity: Â
- Integrating on-premise AI solutions with existing systems can be challenging.
- Requires careful planning and execution to ensure compatibility and functionality.
At Rapid Innovation, we leverage these AI agent training methodologies and deployment models to help our clients achieve their business goals efficiently and effectively. By utilizing advanced techniques such as supervised learning, unsupervised learning, reinforcement learning, transfer learning, and federated learning, we can develop tailored AI solutions that drive greater ROI. Our expertise in deployment models ensures that we can implement these solutions in a manner that aligns with your organizational needs, whether through cloud, edge, or on-premise solutions. This strategic approach not only enhances performance but also optimizes resource allocation, ultimately leading to improved business outcomes. For more information on how we can assist you with adaptive AI development, visit our Adaptive AI Development page and learn more about our AI Agent Development services.
5.4.2. Cloud-Based Implementations
Cloud-based implementations refer to the deployment of applications and services over the internet, utilizing cloud computing resources. This approach offers numerous advantages for businesses looking to enhance their operational efficiency and scalability.
- Scalability: Cloud solutions can easily scale up or down based on demand, allowing businesses to adjust resources without significant upfront investments. Rapid Innovation leverages this scalability to help clients optimize their IT infrastructure, ensuring they can meet fluctuating market demands without incurring unnecessary costs.
- Cost-Effectiveness: By leveraging cloud services, organizations can reduce costs associated with hardware, maintenance, and energy consumption. Our team at Rapid Innovation assists clients in identifying the most cost-effective cloud solutions tailored to their specific needs, ultimately driving greater ROI.
- Accessibility: Cloud-based systems enable remote access, allowing employees to work from anywhere, which is particularly beneficial in today’s flexible work environment. Rapid Innovation implements cloud solutions that enhance workforce mobility, ensuring that teams remain productive regardless of their location.
- Collaboration: Cloud platforms facilitate real-time collaboration among teams, improving productivity and communication. We help organizations integrate collaborative tools that enhance teamwork and streamline project management, leading to faster project completion and improved outcomes.
- Security: Many cloud providers offer robust security measures, including data encryption and regular backups, to protect sensitive information. Rapid Innovation emphasizes security in our cloud implementations, ensuring that our clients' data is safeguarded against potential threats.
According to a report by Gartner, the global public cloud services market is expected to grow by 23% in 2021, highlighting the increasing reliance on cloud-based solutions such as cloud based data storage, cloud based hosting services, and cloud based backup services. Additionally, our expertise in generative AI consulting can further enhance your cloud-based strategies.
5.4.3. Hybrid Approaches
Hybrid approaches combine both on-premises and cloud-based solutions, allowing organizations to enjoy the benefits of both environments. This strategy is particularly useful for businesses with specific regulatory or operational requirements.
- Flexibility: Hybrid models provide the flexibility to choose where to store data and applications, enabling organizations to optimize performance and cost. Rapid Innovation guides clients in designing hybrid architectures that align with their operational goals and compliance needs.
- Enhanced Security: Sensitive data can be kept on-premises while less critical workloads can be moved to the cloud, striking a balance between security and accessibility. Our expertise in both cloud and on-premises solutions ensures that clients can maintain control over their most sensitive information.
- Improved Performance: By utilizing local resources for high-performance applications and cloud resources for scalability, businesses can enhance overall system performance. Rapid Innovation helps clients identify the right balance between local and cloud resources to maximize efficiency.
- Disaster Recovery: Hybrid approaches can improve disaster recovery strategies by allowing data to be backed up in the cloud while maintaining local copies. We assist organizations in developing comprehensive disaster recovery plans that leverage both cloud and on-premises resources.
- Gradual Transition: Organizations can gradually migrate to the cloud without disrupting existing operations, making it easier to adapt to new technologies. Rapid Innovation supports clients through each phase of their cloud journey, ensuring a smooth transition that minimizes operational impact.
According to a study by Flexera, 93% of enterprises have a multi-cloud strategy, indicating a strong trend towards hybrid approaches in IT infrastructure, including the use of cloud based aws and cloud based azure.
5.5. Continuous Learning and Adaptation
Continuous learning and adaptation are essential components of modern business strategies, particularly in rapidly changing environments. Organizations that prioritize these elements can remain competitive and responsive to market demands.
- Agility: Continuous learning fosters an agile mindset, enabling teams to quickly adapt to new information and changing circumstances. Rapid Innovation promotes agile methodologies in our projects, ensuring that our clients can pivot quickly in response to market shifts.
- Innovation: By encouraging a culture of learning, organizations can drive innovation, leading to the development of new products and services. Our focus on innovation helps clients harness emerging technologies, such as AI and blockchain, to create cutting-edge solutions.
- Employee Development: Investing in continuous learning opportunities for employees enhances skills and knowledge, contributing to overall organizational growth. Rapid Innovation offers training and development programs that empower teams to stay ahead in a competitive landscape.
- Feedback Loops: Implementing feedback mechanisms allows organizations to learn from successes and failures, refining processes and strategies over time. We help clients establish effective feedback systems that drive continuous improvement.
- Data-Driven Decisions: Leveraging data analytics for continuous improvement helps organizations make informed decisions based on real-time insights. Rapid Innovation utilizes advanced analytics to provide clients with actionable insights that enhance decision-making processes.
Research from McKinsey indicates that companies that embrace continuous learning are 2.5 times more likely to be agile and responsive to change, underscoring the importance of this approach in today’s business landscape, especially in the context of cloud based computing services and cloud based service providers.
6. Use Cases and Scenarios
Use cases and scenarios are essential in understanding how various industries can leverage technology and processes to improve efficiency, customer satisfaction, and overall performance. In the insurance sector, these use cases can significantly enhance operations, streamline claims processing, and provide better service to policyholders.
6.1 Property and Casualty Insurance
Property and casualty (P&C) insurance encompasses a wide range of coverage types, including homeowners, auto, and liability insurance. The use cases in this sector focus on risk assessment, claims processing, and customer engagement.
- Risk Assessment and Underwriting: Advanced analytics and machine learning models can analyze vast amounts of data to assess risk more accurately. Insurers can utilize geographic information systems (GIS) to evaluate risks related to natural disasters, such as floods or earthquakes. Predictive modeling helps in determining the likelihood of claims, allowing for more tailored premiums. Rapid Innovation can assist insurers in implementing these technologies, ensuring they achieve a competitive edge in risk management. Automated insurance solutions can further enhance this process.
- Claims Processing: Automation tools can streamline the claims process, significantly reducing the time taken to settle claims. Mobile applications enable policyholders to report claims instantly, upload photos, and track the status of their claims in real-time. Artificial intelligence (AI) can assist in fraud detection by analyzing patterns and flagging suspicious claims for further investigation. By integrating insurance technology solutions, Rapid Innovation helps clients enhance operational efficiency and improve customer satisfaction.
- Customer Engagement: Chatbots and virtual assistants can provide 24/7 customer support, answering queries and guiding users through the claims process. Personalized communication strategies can enhance customer relationships, ensuring that policyholders feel valued and informed. Data analytics can help insurers understand customer behavior, allowing for targeted marketing and product offerings. Rapid Innovation's expertise in AI solutions for insurance can empower insurers to create more engaging customer experiences.
6.2 Health Insurance Claims
Health insurance claims involve the process of submitting and processing claims for medical services rendered to policyholders. The use cases in this area focus on improving efficiency, reducing fraud, and enhancing patient experience.
- Claims Submission and Processing: Electronic claims submission systems allow healthcare providers to submit claims directly to insurers, reducing paperwork and processing time. Automated claims processing can minimize human error and speed up the approval process, leading to quicker reimbursements for providers. Integration with electronic health records (EHR) ensures that claims are accurate and based on verified patient data. Rapid Innovation can facilitate the implementation of these systems, driving efficiency and accuracy in claims management.
- Fraud Detection: Advanced analytics can identify unusual patterns in claims submissions, helping insurers detect potential fraud. Machine learning algorithms can analyze historical claims data to flag anomalies that may indicate fraudulent activity. Collaboration with law enforcement and regulatory bodies can enhance efforts to combat healthcare fraud. Rapid Innovation's AI solutions can bolster these fraud detection efforts, ultimately protecting insurers' bottom lines.
- Patient Experience: Mobile applications allow patients to track their claims status, view benefits, and understand their coverage in real-time. Telehealth services can simplify the claims process for virtual consultations, making it easier for patients to access care. Personalized communication regarding claims and benefits can improve patient satisfaction and trust in the insurer. By leveraging Rapid Innovation's expertise, health insurers can enhance the overall patient experience, leading to increased loyalty and retention.
In both property and casualty insurance and health insurance claims, leveraging technology and data analytics can lead to significant improvements in efficiency, customer satisfaction, and overall operational performance. By focusing on these use cases, insurers can better meet the needs of their policyholders while also enhancing their bottom line. Rapid Innovation is committed to providing tailored AI and blockchain solutions that empower clients to achieve their business goals effectively and efficiently. Additionally, the integration of insurance telematics and car insurance telematics can further optimize risk assessment and claims processing. Companies like Duck Creek Insurance Solutions and VertaFore acquired solutions are paving the way for innovative insurance technology platforms that enhance service delivery.
6.3. Auto Insurance Claims Processing
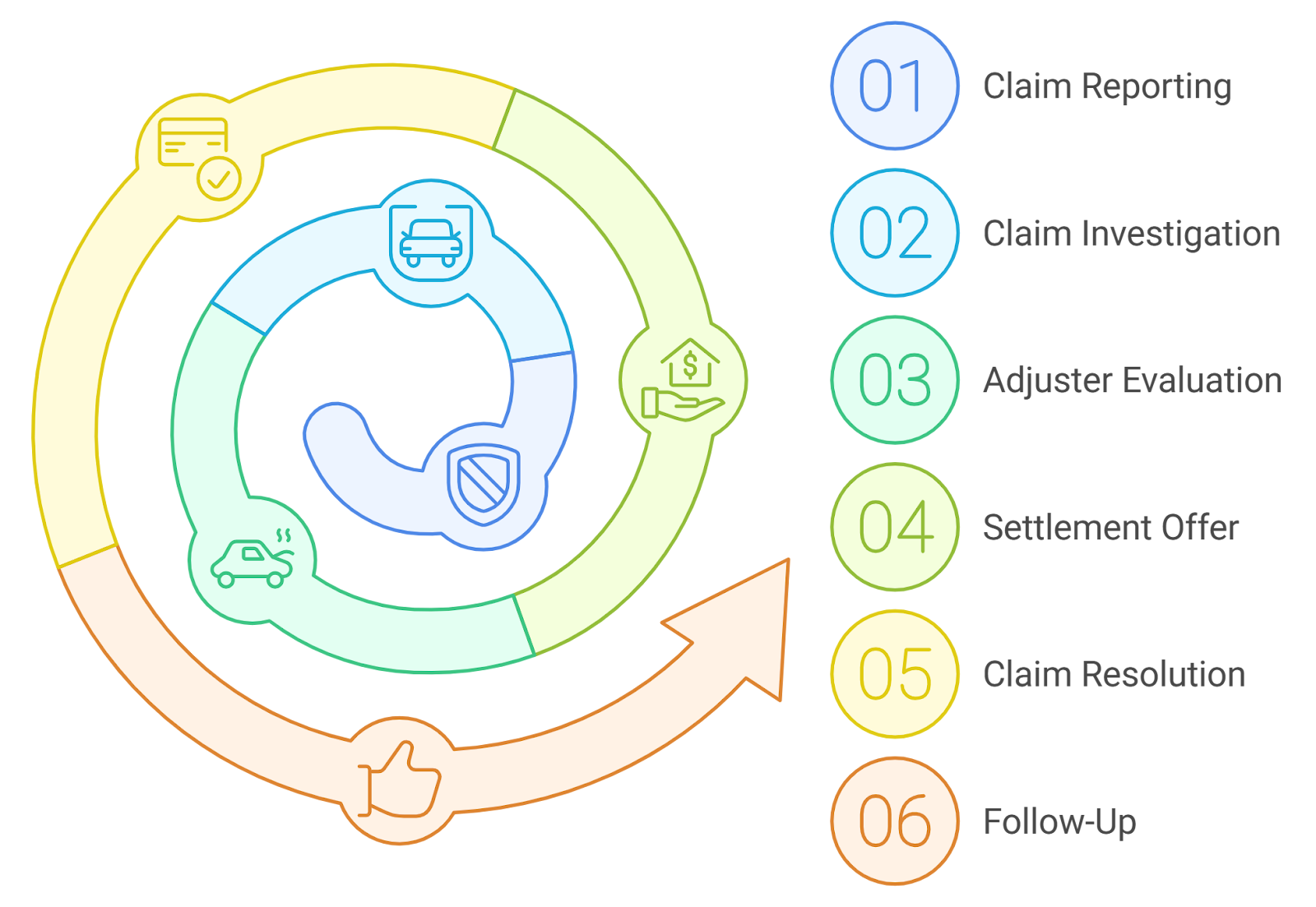
‍
Auto insurance claims processing is a critical aspect of the insurance industry, ensuring that policyholders receive timely and fair compensation for their losses. The process typically involves several key steps:
- Claim Reporting: The first step is for the policyholder to report the incident to their insurance company. This can often be done through a mobile app, online portal, or by calling a claims representative. Providing accurate and detailed information is crucial for a smooth claims process. This includes understanding the car insurance claim process and the necessary documentation required for filing.
- Claim Investigation: Once the claim is reported, the insurance company will investigate the circumstances surrounding the incident. This may involve reviewing police reports, interviewing witnesses, and assessing damages. The goal is to determine liability and the extent of the damages, which is essential in the motor insurance claim process.
- Adjuster Evaluation: An insurance adjuster is assigned to evaluate the claim. They will assess the damages to the vehicle and any other related costs, such as medical expenses or lost wages. The adjuster will also review the policyholder's coverage to ensure that the claim falls within the policy limits. This step is crucial in the automobile insurance claims process.
- Settlement Offer: After the investigation and evaluation, the insurance company will make a settlement offer to the policyholder. This offer is based on the adjuster's findings and the terms of the policy. Policyholders have the right to negotiate this offer if they believe it is insufficient, especially in cases like car accident insurance claims.
- Claim Resolution: Once both parties agree on the settlement, the claim is resolved. The insurance company will issue a payment to the policyholder or directly to the repair shop, depending on the situation. This is a key part of the car insurance claim procedure.
- Follow-Up: After the claim is settled, it’s important for policyholders to follow up to ensure that all aspects of the claim have been addressed and that they are satisfied with the outcome. This is particularly relevant in the context of the auto accident insurance claim process.
Efficient auto insurance claims processing is essential for customer satisfaction and retention. According to a study, 70% of customers expect their claims to be processed within a week. This expectation is reflected in the auto claims process and the overall car insurance process.
At Rapid Innovation, we leverage AI technologies to streamline the claims process, reducing the time taken for each step and enhancing accuracy. For instance, our AI-driven analytics can quickly assess damages through image recognition, expediting the adjuster evaluation phase. This not only improves customer satisfaction but also leads to a higher return on investment (ROI) for insurance companies by minimizing operational costs and enhancing efficiency.
6.4. Life Insurance Underwriting
Life insurance underwriting is the process through which insurers assess the risk of insuring an individual. This process is crucial for determining the premium rates and coverage options available to applicants. Key components of life insurance underwriting include:
- Application Review: The underwriting process begins with a thorough review of the applicant's life insurance application. This includes personal information, health history, lifestyle choices, and financial details.
- Medical Underwriting: Insurers often require medical examinations or health questionnaires to evaluate the applicant's health status. This may include blood tests, urine tests, and other assessments to identify any pre-existing conditions or health risks.
- Risk Assessment: Underwriters analyze the information gathered to assess the risk associated with insuring the applicant. Factors such as age, gender, occupation, and lifestyle habits (e.g., smoking, alcohol consumption) play a significant role in this assessment.
- Premium Determination: Based on the risk assessment, underwriters determine the appropriate premium for the policy. Higher risk applicants may face higher premiums or may be denied coverage altogether.
- Policy Issuance: Once underwriting is complete, the insurer will issue the policy if the applicant is approved. The policy will outline the coverage amount, premium, and any exclusions or limitations.
- Ongoing Review: Some insurers may conduct periodic reviews of existing policies, especially if there are significant changes in the insured's health or lifestyle. This can lead to adjustments in premiums or coverage.
Life insurance underwriting is essential for maintaining the financial stability of insurance companies. It ensures that premiums are set at levels that reflect the risk of loss. The underwriting process can take anywhere from a few days to several weeks, depending on the complexity of the case.
Rapid Innovation employs AI algorithms to enhance the underwriting process by automating data collection and risk assessment. This not only speeds up the application review but also improves accuracy in premium determination, ultimately leading to better customer experiences and increased profitability for insurers.
6.5. Specialized Claim Types
Specialized claim types refer to unique categories of insurance claims that require specific handling due to their complexity or the nature of the coverage. Understanding these specialized claims is vital for both insurers and policyholders. Some common specialized claim types include:
- Natural Disaster Claims: These claims arise from events such as hurricanes, earthquakes, or floods. They often involve extensive damage assessments and may require additional documentation, such as government declarations of disaster areas.
- Workers' Compensation Claims: These claims are filed by employees who suffer work-related injuries or illnesses. The process involves medical evaluations, employer reports, and sometimes legal proceedings to determine compensation for lost wages and medical expenses.
- Cyber Insurance Claims: With the rise of cyber threats, businesses are increasingly purchasing cyber insurance. Claims in this category may involve data breaches, ransomware attacks, and business interruption losses. The claims process often requires forensic investigations and legal consultations.
- Health Insurance Claims: These claims can be complex due to the variety of services covered, including hospital stays, surgeries, and outpatient care. The claims process often involves coordination between healthcare providers and insurers to ensure proper reimbursement.
- Liability Claims: These claims arise when a policyholder is held responsible for causing harm to another person or property. Liability claims can involve legal proceedings and negotiations to settle disputes.
Specialized claims require insurers to have knowledgeable staff and efficient processes in place to handle the unique challenges they present. The complexity of these claims can lead to longer processing times and increased scrutiny from both insurers and policyholders. Understanding the nuances of specialized claims is essential for effective risk management and customer service.
At Rapid Innovation, we utilize blockchain technology to enhance transparency and traceability in specialized claims processing. By creating immutable records of claims and their statuses, we help insurers reduce fraud and improve trust with policyholders, ultimately leading to a more efficient claims process and higher ROI.
6.6. Cross-Industry Applications
Cross-industry applications of technology, particularly artificial intelligence (AI) and machine learning (ML), are transforming various sectors by enhancing efficiency, productivity, and innovation. These applications are not confined to a single industry but span multiple fields, showcasing the versatility of AI solutions that Rapid Innovation can implement for clients seeking to achieve their business goals.
- Healthcare: AI applications in various industries are revolutionizing diagnostics, patient care, and drug discovery. Machine learning algorithms analyze medical images, predict patient outcomes, and personalize treatment plans. For instance, AI can identify patterns in patient data that may indicate the onset of diseases, leading to earlier interventions. Rapid Innovation can assist healthcare providers in integrating AI solutions to improve patient outcomes and operational efficiency.
- Finance: In the financial sector, AI is used for fraud detection, risk assessment, and algorithmic trading. Machine learning models analyze transaction patterns to flag suspicious activities, while predictive analytics help in making informed investment decisions. Rapid Innovation can develop tailored AI systems that enhance security and optimize financial operations, ultimately driving greater ROI for financial institutions.
- Retail: AI enhances customer experience through personalized recommendations, inventory management, and supply chain optimization. Retailers use AI-driven analytics to understand consumer behavior, forecast demand, and streamline operations. Rapid Innovation can help retailers implement AI solutions that not only improve customer satisfaction but also increase sales and reduce costs.
- Manufacturing: AI applications in manufacturing include predictive maintenance, quality control, and automation of production processes. By analyzing data from machinery, AI can predict failures before they occur, reducing downtime and maintenance costs. Rapid Innovation can support manufacturers in adopting AI technologies that lead to significant cost savings and improved production efficiency.
- Transportation: AI is integral to the development of autonomous vehicles and smart traffic management systems. Machine learning algorithms process real-time data to optimize routes, reduce congestion, and improve safety. Rapid Innovation can collaborate with transportation companies to develop AI solutions that enhance operational efficiency and safety.
- Agriculture: AI technologies are applied in precision farming, crop monitoring, and yield prediction. Drones equipped with AI analyze crop health, while machine learning models help farmers make data-driven decisions to enhance productivity. Rapid Innovation can assist agricultural businesses in leveraging AI to maximize yield and minimize resource usage.
These cross-industry applications demonstrate how AI and ML can drive innovation and efficiency across various sectors, ultimately leading to improved outcomes and competitive advantages for our clients. For more information on the types, benefits, and real-world uses of AI agents.
7. Advanced AI Agent Capabilities
Advanced AI agent capabilities refer to the sophisticated functionalities that AI systems can perform, enabling them to operate autonomously and make complex decisions. These capabilities are essential for developing intelligent systems that can adapt to dynamic environments and user needs, which is a core focus of Rapid Innovation's development efforts.
- Natural Language Processing (NLP): AI agents can understand and generate human language, allowing for seamless interaction with users. This capability is crucial for chatbots, virtual assistants, and customer service applications. Rapid Innovation can implement NLP solutions that enhance customer engagement and streamline communication.
- Machine Learning: Advanced AI agents utilize machine learning algorithms to learn from data and improve their performance over time. This enables them to recognize patterns, make predictions, and adapt to new information. Rapid Innovation can develop custom machine learning models tailored to specific business needs, driving efficiency and effectiveness.
- Computer Vision: AI agents equipped with computer vision can interpret and analyze visual data from the world around them. This capability is vital for applications such as facial recognition, object detection, and autonomous navigation. Rapid Innovation can create computer vision solutions that enhance security and operational capabilities for various industries.
- Multi-Agent Systems: Advanced AI agents can collaborate with other agents to solve complex problems. These systems can share information, negotiate, and coordinate actions to achieve common goals. Rapid Innovation can design multi-agent systems that optimize processes and improve decision-making across organizations.
- Emotional Intelligence: Some AI agents are designed to recognize and respond to human emotions, enhancing user interaction and engagement. This capability is particularly useful in customer service and mental health applications. Rapid Innovation can develop emotionally intelligent AI solutions that foster better relationships between businesses and their customers.
7.1. Autonomous Decision Making
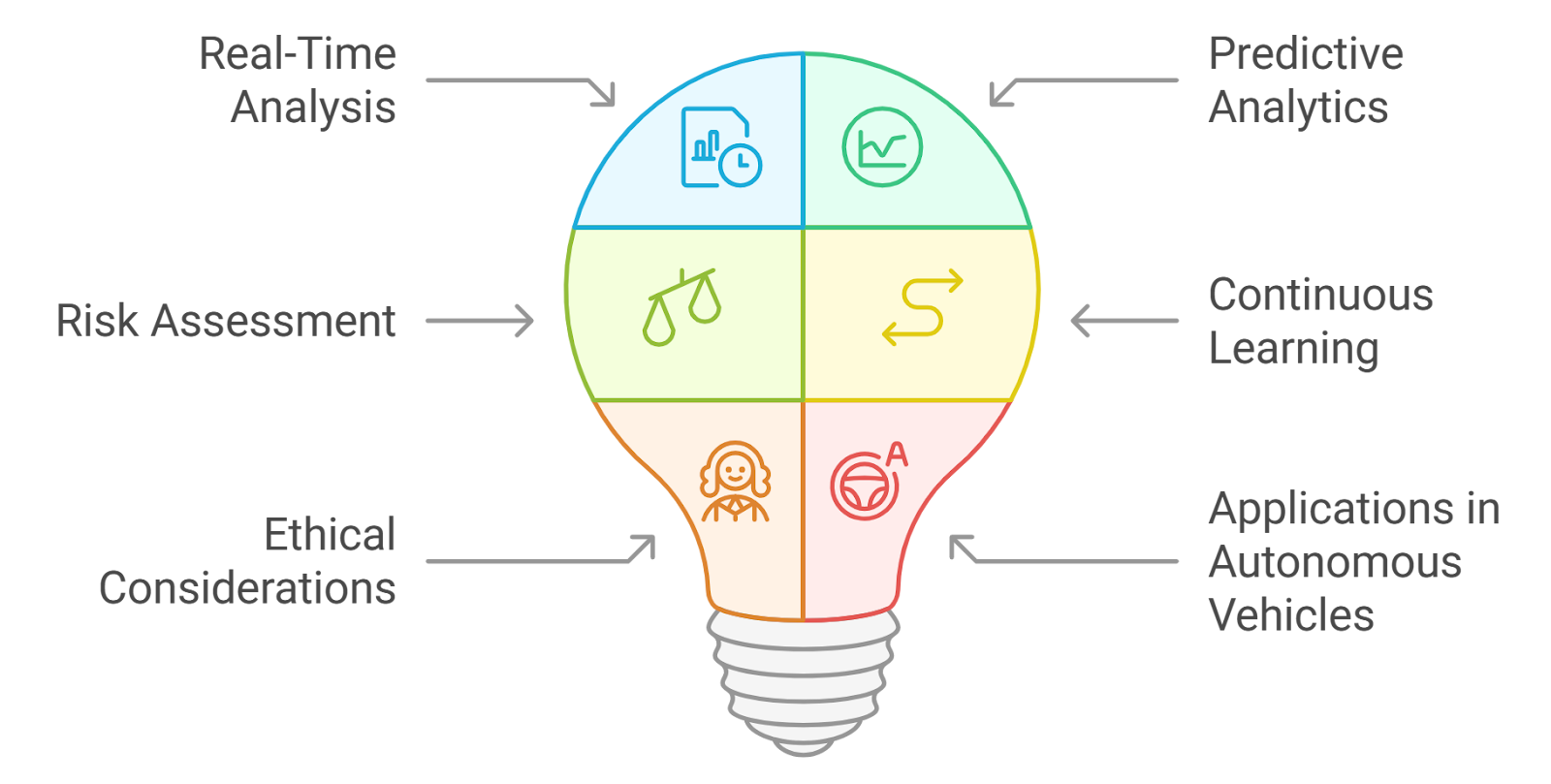
‍
Autonomous decision-making is a critical capability of advanced AI agents, allowing them to make choices without human intervention. This functionality is essential for applications that require real-time responses and adaptability, aligning with Rapid Innovation's mission to empower businesses through intelligent automation.
- Real-Time Analysis: Autonomous AI agents can analyze vast amounts of data in real-time, enabling them to make informed decisions quickly. This is particularly important in industries like finance, where market conditions can change rapidly. Rapid Innovation can implement real-time analytics solutions that enhance responsiveness and agility.
- Predictive Analytics: By leveraging historical data, AI agents can predict future trends and outcomes. This capability allows businesses to make proactive decisions, such as adjusting inventory levels or optimizing marketing strategies. Rapid Innovation can help organizations harness predictive analytics to drive strategic initiatives and improve ROI.
- Risk Assessment: Autonomous decision-making systems can evaluate risks associated with various options. For example, in healthcare, AI can assess the potential risks and benefits of different treatment plans, aiding doctors in their decision-making process. Rapid Innovation can develop risk assessment tools that enhance decision-making across sectors.
- Continuous Learning: Advanced AI agents can learn from their experiences and improve their decision-making capabilities over time. This continuous learning process enables them to adapt to changing environments and user preferences. Rapid Innovation can create systems that evolve with business needs, ensuring long-term value.
- Ethical Considerations: As AI systems become more autonomous, ethical considerations regarding their decision-making processes become increasingly important. Ensuring transparency, accountability, and fairness in AI decisions is crucial for building trust and acceptance among users. Rapid Innovation prioritizes ethical AI development to foster responsible innovation.
- Applications in Autonomous Vehicles: In the transportation sector, autonomous vehicles rely on AI agents to make real-time decisions based on sensor data. These vehicles must navigate complex environments, respond to unexpected obstacles, and ensure passenger safety. Rapid Innovation can support the development of AI solutions that enhance the safety and efficiency of autonomous transportation systems.
The ability of AI agents to make autonomous decisions is reshaping industries and creating new opportunities for innovation. As these technologies continue to evolve, their impact on society and the economy will be profound, and Rapid Innovation is committed to guiding clients through this transformative journey.
7.2. Predictive Cost Estimation
Predictive cost estimation is a crucial aspect of project management and financial planning, particularly in industries such as construction, manufacturing, and IT. This method leverages historical data, statistical algorithms, and machine learning techniques to forecast future costs accurately.
- Utilizes historical data to identify trends and patterns. Â
- Employs advanced analytics to predict potential cost overruns. Â
- Enhances budgeting accuracy by providing data-driven insights. Â
- Supports decision-making by offering a clearer financial outlook. Â
- Reduces the risk of unexpected expenses through proactive planning. Â
By implementing predictive cost estimation, organizations can improve their financial performance and resource allocation. This approach not only helps in setting realistic budgets but also in identifying areas where cost savings can be achieved. For instance, a study by McKinsey found that companies using predictive cost estimation can reduce project costs by up to 20%. At Rapid Innovation, we harness the power of AI to provide tailored predictive cost estimation solutions that empower our clients to make informed financial decisions, ultimately leading to greater ROI.
7.3. Self-Healing Claim Processes
Self-healing claim processes refer to automated systems that can identify, rectify, and optimize claims without human intervention. This innovation is particularly beneficial in the insurance and finance sectors, where claims processing can be time-consuming and prone to errors.
- Automates the claims review process, reducing manual workload. Â
- Uses AI to detect anomalies and rectify issues in real-time. Â
- Enhances customer satisfaction by speeding up claim resolutions. Â
- Minimizes fraud through advanced detection algorithms. Â
- Provides insights for continuous improvement in claims management. Â
The self-healing capability allows organizations to respond swiftly to claims, ensuring that customers receive timely resolutions. This not only improves operational efficiency but also fosters trust and loyalty among clients. According to a report by Accenture, companies that adopt self-healing processes can improve their claims processing speed by up to 50%. Rapid Innovation specializes in developing self-healing claim processes that streamline operations and enhance customer experiences, driving significant cost savings and efficiency for our clients.
7.4. Intelligent Customer Communication
Intelligent customer communication involves using advanced technologies like AI and machine learning to enhance interactions between businesses and their customers. This approach focuses on delivering personalized, timely, and relevant information to improve customer experience.
- Leverages chatbots and virtual assistants for 24/7 support. Â
- Analyzes customer data to tailor communication strategies. Â
- Automates responses to frequently asked questions, freeing up human agents. Â
- Enhances engagement through personalized marketing messages. Â
- Utilizes sentiment analysis to gauge customer satisfaction and adjust strategies accordingly. Â
By adopting intelligent customer communication, businesses can create a more engaging and responsive environment for their clients. This not only leads to higher customer satisfaction but also drives sales and retention. Research indicates that companies using AI-driven communication tools can see a 30% increase in customer engagement. At Rapid Innovation, we provide cutting-edge solutions that leverage AI to enhance customer interactions, ensuring our clients achieve their business goals effectively and efficiently.
7.5. Advanced Anomaly Detection
Advanced anomaly detection refers to sophisticated techniques used to identify unusual patterns or behaviors in data that deviate from the norm. This is crucial in various fields, including cybersecurity, finance, and healthcare, where detecting anomalies can prevent fraud, security breaches, or medical errors.
- Machine Learning Algorithms: Â
- Supervised learning models, such as decision trees and support vector machines, can be trained on labeled datasets to identify anomalies. Rapid Innovation leverages these models to help clients in finance detect fraudulent transactions, thereby enhancing their security measures and reducing potential losses.
- Unsupervised learning techniques, like clustering and autoencoders, can detect outliers without prior labeling. By implementing these techniques, we assist healthcare organizations in identifying unusual patient data patterns that may indicate medical errors or crises. Techniques such as anomaly detection using machine learning and statistical anomaly detection are also employed to enhance accuracy.
- Real-Time Monitoring: Â
- Advanced systems can analyze data streams in real-time, allowing for immediate detection of anomalies. This is particularly important in industries like finance, where rapid detection can mitigate losses. Rapid Innovation provides clients with real-time monitoring solutions that empower them to respond swiftly to potential threats, including network traffic anomaly detection.
- Multi-Dimensional Analysis: Â
- Anomaly detection can be enhanced by analyzing data across multiple dimensions, such as time, location, and user behavior. This holistic approach helps in identifying complex patterns that single-dimensional analysis might miss. Our expertise in multi-dimensional analysis enables clients to gain deeper insights into their operations, leading to more informed decision-making. Techniques like outlier detection in time series and cluster-based anomaly detection are utilized for this purpose.
- Applications: Â
- In cybersecurity, advanced anomaly detection can identify unusual login attempts or data access patterns. Rapid Innovation has successfully implemented such systems for clients, significantly improving their security posture. Applications include network anomaly detection using machine learning and traffic anomaly detection.
- In healthcare, it can help in monitoring patient vitals to detect potential health crises. Our solutions have been instrumental in enabling healthcare providers to enhance patient safety and care quality, including anomaly detection for data quality.
7.6. Contextual Understanding
Contextual understanding refers to the ability of systems to interpret data within its relevant context, enhancing the accuracy and relevance of insights derived from the data. This is particularly important in natural language processing, machine learning, and data analytics.
- Importance of Context: Â
- Context helps in disambiguating data, allowing systems to make more informed decisions. For example, the word "bank" can refer to a financial institution or the side of a river, depending on the context. Rapid Innovation emphasizes the importance of context in our AI solutions to ensure accurate data interpretation.
- Techniques for Contextual Understanding: Â
- Natural Language Processing (NLP): Â
- NLP techniques, such as word embeddings and transformers, enable machines to understand the nuances of human language. These techniques help in grasping the sentiment, intent, and meaning behind words in a given context. Our NLP solutions have empowered clients to enhance customer interactions and improve service delivery.
- Semantic Analysis: Â
- This involves analyzing the meaning of words and phrases in relation to their context, improving the accuracy of data interpretation. It can be applied in various fields, including customer service, where understanding customer sentiment is crucial. Rapid Innovation utilizes semantic analysis to help businesses tailor their marketing strategies effectively.
- Natural Language Processing (NLP): Â
- Applications: Â
- In customer support, contextual understanding can help chatbots provide more relevant responses based on previous interactions. Our AI-driven chatbots have significantly improved customer satisfaction for our clients.
- In marketing, it allows for personalized content delivery based on user behavior and preferences. By leveraging contextual understanding, we assist clients in maximizing their marketing ROI through targeted campaigns.
8. Challenges and Limitations

‍
Despite the advancements in anomaly detection and contextual understanding, several challenges and limitations persist that can hinder their effectiveness.
- Data Quality: Â
- Poor quality data can lead to inaccurate anomaly detection and misinterpretation of context. Incomplete, noisy, or biased data can skew results and lead to false positives or negatives. Rapid Innovation emphasizes the importance of data quality and offers consulting services to help clients improve their data management practices.
- Complexity of Algorithms: Â
- Advanced algorithms can be complex and require significant computational resources. This complexity can make them difficult to implement and maintain, especially for smaller organizations. We provide tailored solutions that simplify the implementation of these algorithms, ensuring that our clients can leverage advanced technologies without overwhelming their resources.
- Interpretability: Â
- Many machine learning models, particularly deep learning, operate as "black boxes," making it challenging to understand how decisions are made. Lack of interpretability can lead to trust issues among users and stakeholders. Rapid Innovation focuses on developing interpretable models that enhance transparency and build trust with end-users.
- Evolving Patterns: Â
- Anomalies can evolve over time, making it difficult for models to adapt to new patterns without retraining. Continuous monitoring and updating of models are necessary to maintain accuracy. Our ongoing support services ensure that clients' models remain effective in the face of changing data landscapes.
- Contextual Variability: Â
- Context can change rapidly, and systems may struggle to keep up with these changes. This variability can lead to misinterpretation of data, especially in dynamic environments. Rapid Innovation's adaptive solutions are designed to accommodate contextual changes, ensuring that our clients can maintain accurate insights.
- Ethical Considerations: Â
- The use of advanced anomaly detection and contextual understanding raises ethical concerns, particularly regarding privacy and data security. Organizations must navigate these issues carefully to avoid potential backlash from users and regulatory bodies. We guide our clients in implementing ethical AI practices, ensuring compliance with regulations while maximizing the benefits of their data-driven initiatives.
8.1. Data Quality and Reliability
Data quality and reliability are critical components in the realm of artificial intelligence (AI) and machine learning (ML). High-quality data ensures that AI systems function effectively and produce accurate results, ultimately leading to greater ROI for businesses.
- Accuracy: Data must be correct and free from errors. Inaccurate data can lead to flawed models and misguided decisions, which can be costly for organizations. Rapid Innovation employs advanced data validation techniques to ensure accuracy, helping clients avoid potential pitfalls.
- Completeness: Datasets should be comprehensive, containing all necessary information. Missing data can skew results and reduce the model's effectiveness. Our team assists clients in identifying gaps in their datasets and implementing strategies to fill those gaps, enhancing the overall quality of their AI initiatives.
- Consistency: Data should be uniform across different sources. Inconsistencies can arise from various formats or definitions, leading to confusion and misinterpretation. Rapid Innovation provides data integration solutions that ensure consistency across all data sources, enabling clients to make informed decisions.
- Timeliness: Data must be up-to-date. Outdated information can render models obsolete and irrelevant. We help organizations establish real-time data pipelines, ensuring that they always have access to the most current information for their AI applications.
- Reliability: Data sources should be trustworthy. Using data from unreliable sources can compromise the integrity of AI systems. Rapid Innovation emphasizes the importance of sourcing data from reputable providers and conducts thorough assessments to ensure reliability. The trustworthiness of data and data quality refers to the assurance that the data used in AI systems is both accurate and dependable.
Ensuring data quality involves regular audits, validation processes, and employing data cleaning techniques. Organizations should invest in robust data governance frameworks to maintain high standards of data quality and reliability, which is a service Rapid Innovation offers to enhance operational efficiency. Data accuracy and reliability are essential for organizations to achieve their AI objectives effectively.
8.2. Ethical AI Considerations
Ethical considerations in AI are paramount as these technologies increasingly influence various aspects of society. Addressing ethical issues helps build trust and ensures that AI systems are used responsibly, which is essential for long-term success.
- Bias and Fairness: AI systems can inadvertently perpetuate biases present in training data. It is essential to identify and mitigate these biases to ensure fairness in AI outcomes. Rapid Innovation employs bias detection algorithms to help clients create fairer AI models.
- Transparency: AI algorithms should be transparent, allowing users to understand how decisions are made. This transparency fosters accountability and trust. We assist organizations in developing explainable AI solutions that clarify decision-making processes.
- Privacy: Protecting user data is crucial. Organizations must implement measures to safeguard personal information and comply with privacy regulations. Rapid Innovation provides consulting on best practices for data privacy, ensuring compliance with regulations like GDPR.
- Accountability: There should be clear lines of accountability for AI decisions. Organizations must establish who is responsible for the outcomes produced by AI systems. We help clients define accountability frameworks that align with their organizational goals.
- Impact on Employment: The deployment of AI can disrupt job markets. Ethical considerations should include strategies for workforce transition and reskilling. Rapid Innovation offers training programs to help organizations reskill their workforce in light of AI advancements.
Organizations should adopt ethical frameworks and guidelines to navigate these considerations effectively. Engaging stakeholders in discussions about ethical AI can also promote a more inclusive approach, a service we facilitate through workshops and collaborative sessions.
8.3. Regulatory Compliance
Regulatory compliance is essential for organizations deploying AI technologies. Adhering to regulations helps mitigate risks and ensures that AI systems operate within legal boundaries, ultimately protecting the organization’s reputation and bottom line.
- Data Protection Laws: Compliance with laws such as the General Data Protection Regulation (GDPR) is crucial. These laws govern how personal data is collected, processed, and stored. Rapid Innovation assists clients in understanding and implementing necessary compliance measures.
- Industry-Specific Regulations: Different industries have specific regulations that must be followed. For example, healthcare AI applications must comply with HIPAA in the United States. Our team provides tailored compliance solutions that address the unique needs of various sectors.
- Accountability Standards: Organizations should establish accountability standards to ensure that AI systems are used responsibly and ethically. We help clients create comprehensive accountability frameworks that align with industry best practices.
- Regular Audits: Conducting regular audits helps organizations assess compliance with regulations and identify areas for improvement. Rapid Innovation offers audit services to ensure that clients remain compliant and can adapt to changing regulations.
- Documentation: Maintaining thorough documentation of AI processes and decisions is vital for demonstrating compliance and accountability. We assist organizations in developing robust documentation practices that support transparency and regulatory adherence.
Staying informed about evolving regulations is essential for organizations to remain compliant. Engaging with legal experts and industry bodies can provide valuable insights into regulatory requirements, a service that Rapid Innovation facilitates through our extensive network of industry contacts.
8.4. Integration Complexities
Integrating new technologies, especially in the realm of artificial intelligence and machine learning, can present significant challenges, including ai integration challenges. These complexities can arise from various factors, including:
- Legacy Systems: Many organizations rely on outdated systems that may not be compatible with modern technologies, leading to increased costs and time delays during integration. Rapid Innovation specializes in modernizing these legacy systems, ensuring seamless integration with cutting-edge AI solutions that enhance operational efficiency.
- Data Silos: Data often exists in isolated systems, making it difficult to access and utilize effectively. Breaking down these silos is crucial for successful integration. Our team at Rapid Innovation employs advanced data integration techniques to unify disparate data sources, enabling organizations to leverage their data for informed decision-making.
- Interoperability Issues: Different systems may use varying protocols and standards, complicating the integration process. Ensuring that all components can communicate effectively is essential. Rapid Innovation's expertise in developing interoperable solutions ensures that your systems work together harmoniously, maximizing the value of your technology investments.
- Scalability Concerns: As organizations grow, their systems must be able to scale accordingly. Integrating solutions that can adapt to increasing demands is vital for long-term success. We design scalable AI and blockchain solutions that evolve with your business, ensuring sustained performance and ROI.
- Change Management: Employees may resist new technologies due to fear of the unknown or lack of training. Effective change management strategies are necessary to facilitate a smooth transition. Rapid Innovation provides comprehensive training and support to empower your workforce, fostering a culture of innovation and adaptability.
Organizations must carefully plan their integration strategies to address these complexities, including ai integration challenges. This includes conducting thorough assessments of existing systems, investing in training, and ensuring that new technologies align with business goals.
8.5. Performance and Accuracy Challenges
Performance and accuracy are critical factors in the success of any technology implementation, particularly in AI and machine learning applications. Challenges in these areas can significantly impact outcomes:
- Data Quality: The accuracy of AI models heavily relies on the quality of the data used for training. Poor-quality data can lead to inaccurate predictions and unreliable results. Rapid Innovation emphasizes the importance of high-quality data collection and preprocessing to enhance model performance.
- Model Overfitting: When a model is too complex, it may perform well on training data but poorly on unseen data. Striking the right balance between complexity and generalization is essential. Our team employs advanced techniques to optimize model architectures, ensuring robust performance across diverse datasets.
- Latency Issues: In real-time applications, delays in processing can hinder performance. Optimizing algorithms for speed without sacrificing accuracy is a common challenge. Rapid Innovation focuses on developing efficient algorithms that deliver real-time insights, enhancing user experience and operational efficiency.
- Resource Limitations: Insufficient computational resources can lead to slower processing times and reduced model performance. Organizations must ensure they have the necessary infrastructure in place. We assist clients in assessing their infrastructure needs and implementing scalable solutions that meet their growing demands.
- Evaluation Metrics: Choosing the right metrics to evaluate model performance is crucial. Relying on inappropriate metrics can give a false sense of accuracy and lead to poor decision-making. Rapid Innovation guides organizations in selecting and implementing appropriate evaluation frameworks to ensure reliable performance assessments.
To overcome these challenges, organizations should focus on continuous monitoring and improvement of their models, invest in high-quality data collection, and utilize robust evaluation frameworks.
8.6. Bias Mitigation Strategies
Bias in AI and machine learning can lead to unfair outcomes and perpetuate existing inequalities. Addressing bias is essential for creating ethical and effective systems. Here are some strategies for mitigating bias:
- Diverse Data Sets: Ensuring that training data is representative of the population can help reduce bias. This includes considering various demographics, backgrounds, and experiences. Rapid Innovation advocates for the use of diverse datasets to create more inclusive AI models.
- Bias Audits: Regularly conducting audits of AI models can help identify and address biases. This involves analyzing model outputs for fairness and accuracy across different groups. Our team offers comprehensive bias auditing services to ensure your AI systems operate fairly and transparently.
- Algorithmic Transparency: Making algorithms more transparent allows stakeholders to understand how decisions are made. This can help identify potential biases in the decision-making process. Rapid Innovation promotes algorithmic transparency, enabling organizations to build trust with their users.
- Inclusive Design: Involving diverse teams in the design and development of AI systems can lead to more equitable outcomes. Different perspectives can help identify potential biases early in the process. We emphasize collaborative design practices that incorporate diverse viewpoints to enhance fairness in AI solutions.
- Continuous Learning: Implementing feedback loops that allow models to learn from new data can help reduce bias over time. This ensures that systems remain relevant and fair as societal norms evolve. Rapid Innovation integrates continuous learning mechanisms into AI systems, promoting adaptability and fairness.
By actively addressing bias, organizations can create more equitable AI systems that serve all users fairly and effectively.
9. Economic and Strategic Implications
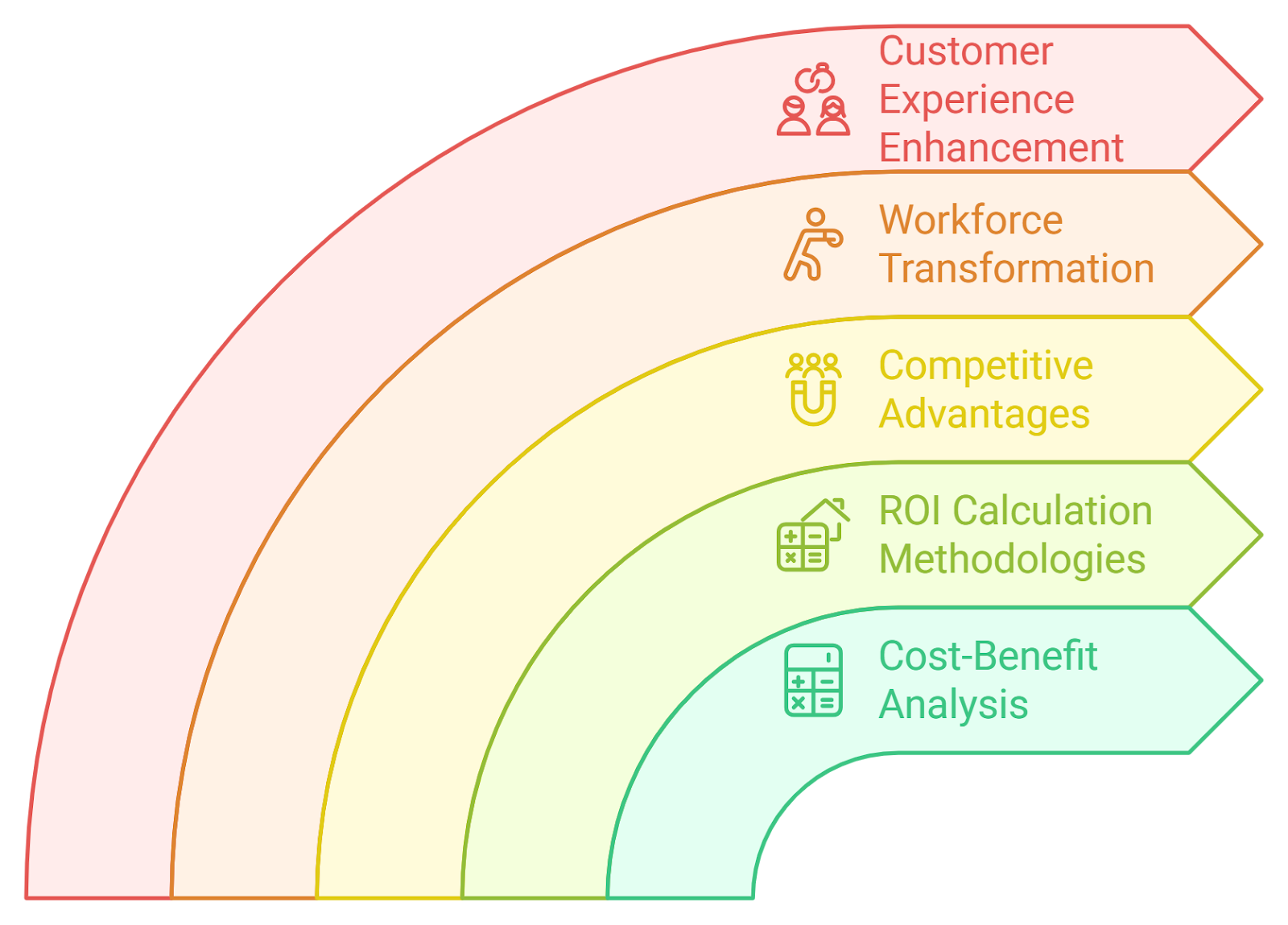
‍
Understanding the economic implications analysis and strategic implications of a project or investment is crucial for decision-making. This involves analyzing the potential costs and benefits, as well as calculating the return on investment (ROI) to ensure that resources are allocated effectively.
9.1 Cost-Benefit Analysis
Cost-benefit analysis (CBA) is a systematic approach to evaluating the economic advantages and disadvantages of a project. It helps stakeholders make informed decisions by quantifying the expected costs and benefits.
- Definition: CBA compares the total expected costs of a project against its total expected benefits, expressed in monetary terms.
- Purpose: The primary goal is to determine whether the benefits outweigh the costs, thus justifying the investment.
- Components: Â
- Direct Costs: These include expenses directly associated with the project, such as materials, labor, and overhead.
- Indirect Costs: These are secondary costs that may arise, such as environmental impacts or social implications.
- Tangible Benefits: These are measurable benefits, like increased revenue or reduced operational costs.
- Intangible Benefits: These include non-quantifiable advantages, such as improved customer satisfaction or brand reputation.
- Steps in CBA: Â
- Identify the project scope and objectives.
- List all potential costs and benefits.
- Assign monetary values to each cost and benefit.
- Calculate the net present value (NPV) to assess the project's viability.
- Limitations: Â
- Difficulty in quantifying intangible benefits.
- Potential biases in estimating costs and benefits.
- Changes in market conditions can affect the accuracy of predictions.
CBA is widely used in various sectors, including healthcare, infrastructure, and environmental projects, to ensure that investments yield positive economic outcomes. At Rapid Innovation, we leverage advanced AI algorithms to enhance the accuracy of CBA, enabling clients to make data-driven decisions that align with their strategic goals.
9.2 ROI Calculation Methodologies
Return on investment (ROI) is a key performance indicator that measures the profitability of an investment relative to its cost. Understanding different methodologies for calculating ROI is essential for evaluating the effectiveness of various projects.
- Definition: ROI is calculated by dividing the net profit from an investment by the initial cost of the investment, usually expressed as a percentage.
- Basic ROI Formula: Â
- ROI = (Net Profit / Cost of Investment) x 100
- Net Profit = Total Revenue - Total Costs
- Methodologies: Â
- Simple ROI: This straightforward method uses the basic formula to provide a quick assessment of profitability.
- Annualized ROI: This approach adjusts the ROI calculation to reflect annual performance, making it easier to compare investments of different durations.
- Risk-Adjusted ROI: This methodology incorporates the risk factor associated with an investment, providing a more nuanced view of potential returns.
- Factors Influencing ROI: Â
- Time Frame: The duration of the investment can significantly impact ROI calculations.
- Market Conditions: Economic fluctuations can affect both costs and revenues, altering the ROI.
- Operational Efficiency: Improvements in processes can lead to higher returns, making efficiency a critical factor in ROI calculations.
- Limitations: Â
- ROI does not account for the time value of money unless adjusted.
- It may oversimplify complex investments by focusing solely on financial returns.
- Different methodologies can yield varying results, leading to potential confusion.
ROI is a vital tool for businesses and investors, helping them assess the profitability of their investments and make strategic decisions based on quantitative data. At Rapid Innovation, we utilize cutting-edge blockchain technology to ensure transparency and traceability in ROI calculations, empowering clients to achieve greater returns on their investments while minimizing risks. Additionally, understanding the cost of building AI agents is essential for making informed economic decisions in this rapidly evolving field.
9.3. Competitive Advantages
In today's fast-paced business environment, organizations must leverage competitive advantages to stand out in the marketplace. Competitive advantages can stem from various sources, including unique products, superior customer service, and innovative technologies.
- Unique Selling Proposition (USP): A clear USP differentiates a company from its competitors. This could be a patented technology, exclusive partnerships, or a unique brand identity. At Rapid Innovation, we help clients identify and develop their USPs through tailored AI and Blockchain solutions that enhance their market positioning.
- Cost Leadership: Companies that can produce goods or services at a lower cost than their competitors can offer lower prices, attracting price-sensitive customers. Our expertise in AI-driven automation and Blockchain efficiency allows clients to reduce operational costs while maintaining quality. This approach is a key aspect of the cost leadership business strategy.
- Quality and Reliability: Consistently delivering high-quality products or services builds trust and loyalty among customers, leading to repeat business. Rapid Innovation employs advanced AI algorithms to ensure quality control and reliability in product development, which is essential for maintaining a competitive advantage of a firm.
- Innovation: Organizations that prioritize research and development can introduce groundbreaking products or services, keeping them ahead of the competition. We assist clients in harnessing the power of AI and Blockchain to foster innovation, enabling them to launch new offerings that meet evolving market demands. This innovation is a critical component of sustainable competitive advantage.
- Strong Brand Reputation: A well-established brand can command customer loyalty and trust, making it difficult for new entrants to compete. Our consulting services help clients build and maintain a strong brand reputation through effective use of technology and strategic marketing, which is vital for companies with competitive advantage.
- Customer Relationships: Building strong relationships with customers through personalized service and engagement can create a loyal customer base that is less likely to switch to competitors. Rapid Innovation leverages AI to analyze customer data, enabling clients to enhance their engagement strategies and foster long-term loyalty, which is a strategic competitive advantage.
9.4. Workforce Transformation
Workforce transformation is essential for organizations aiming to adapt to changing market demands and technological advancements. This process involves reshaping the workforce to enhance productivity, engagement, and overall performance.
- Upskilling and Reskilling: Investing in employee training programs ensures that the workforce remains competitive and capable of handling new technologies and processes. We provide tailored training solutions focused on AI and Blockchain technologies to equip employees with the necessary skills, which is crucial for maintaining competitiveness advantage.
- Embracing Remote Work: The shift to remote work has transformed how organizations operate. Companies that adapt to flexible work arrangements can attract top talent and improve employee satisfaction. Our solutions facilitate seamless collaboration and communication for remote teams, enhancing their competitive advantage.
- Diversity and Inclusion: A diverse workforce brings varied perspectives and ideas, fostering innovation and creativity. Organizations that prioritize inclusion can enhance their overall performance. Rapid Innovation promotes diversity through inclusive hiring practices and team-building initiatives, contributing to a sustainable competitive advantage.
- Employee Engagement: Engaged employees are more productive and committed to their work. Implementing feedback mechanisms and recognition programs can boost morale and retention. We help organizations develop engagement strategies that leverage AI insights to understand employee needs, which is essential for a company's competitive strategy.
- Agile Work Practices: Adopting agile methodologies allows teams to respond quickly to changes in the market, improving efficiency and collaboration. Our consulting services guide organizations in implementing agile frameworks tailored to their specific needs, enhancing their strategic management and competitive advantage.
- Technology Integration: Utilizing advanced tools and platforms can streamline operations and enhance communication within teams, leading to better outcomes. Rapid Innovation specializes in integrating AI and Blockchain technologies to optimize workflows and improve team dynamics, which is vital for maintaining a competitive advantage in strategic management.
9.5. Customer Experience Enhancement
Enhancing customer experience (CX) is crucial for businesses looking to retain customers and drive growth. A positive CX can lead to increased customer loyalty, higher sales, and improved brand reputation.
- Personalization: Tailoring products, services, and communications to individual customer preferences can significantly enhance their experience. This can be achieved through data analytics and customer feedback. Rapid Innovation employs AI-driven analytics to help clients create personalized experiences that resonate with their customers, contributing to their competitive advantage.
- Omnichannel Support: Providing seamless support across multiple channels (e.g., social media, email, phone) ensures that customers can reach out in their preferred way, improving satisfaction. Our solutions enable clients to implement effective omnichannel strategies that enhance customer interactions, which is essential for a business with competitive advantage.
- User-Friendly Interfaces: Simplifying website and app navigation enhances the customer journey, making it easier for users to find what they need and complete transactions. We design intuitive interfaces that improve usability and drive customer engagement, which is a key aspect of competitive advantage in business.
- Proactive Communication: Keeping customers informed about their orders, services, or any changes can build trust and reduce frustration. Our AI solutions enable clients to automate communication, ensuring timely updates and enhancing customer satisfaction, which is vital for maintaining a competitive advantage.
- Feedback Loops: Actively seeking customer feedback and implementing changes based on their suggestions can demonstrate that a company values its customers' opinions. We assist clients in establishing effective feedback mechanisms that drive continuous improvement, contributing to their competitive strategy.
- Loyalty Programs: Rewarding customers for their loyalty through discounts, exclusive offers, or points systems can encourage repeat business and foster long-term relationships. Rapid Innovation helps clients design and implement loyalty programs that leverage Blockchain for transparency and security, enhancing their sustainable competitive advantage.
10. Technology Integration
Technology integration in various industries has become essential for improving efficiency, enhancing customer experience, and streamlining operations. In the insurance sector, the adoption of advanced technologies like blockchain and the Internet of Things (IoT) is transforming traditional processes, particularly in claims processing and data management, including insurance technology integration.
10.1 Blockchain in Claims Processing
Blockchain technology is revolutionizing claims processing by providing a secure, transparent, and efficient way to handle transactions. This decentralized ledger system allows for real-time tracking of claims, reducing the potential for fraud and errors.
- Transparency and Trust: Blockchain creates an immutable record of all transactions, ensuring that all parties involved in the claims process can access the same information. This transparency builds trust between insurers and policyholders.
- Faster Claims Settlement: By automating claims processing through smart contracts, insurers can expedite the settlement process. Smart contracts automatically execute transactions when predefined conditions are met, significantly reducing the time taken to process claims.
- Fraud Prevention: The secure nature of blockchain makes it difficult for fraudulent claims to be submitted. Each claim can be verified against the blockchain, ensuring that only legitimate claims are processed.
- Cost Efficiency: Reducing the time and resources spent on claims processing can lead to significant cost savings for insurance companies. This efficiency can be passed on to customers in the form of lower premiums.
- Interoperability: Blockchain can facilitate better communication between different insurance providers and stakeholders, allowing for a more integrated approach to claims processing.
The potential of blockchain in claims processing is vast, and as more insurers adopt this technology, we can expect to see significant improvements in the overall efficiency of the insurance industry. At Rapid Innovation, we specialize in implementing blockchain solutions that enhance operational efficiency and drive greater ROI for our clients.
10.2 IoT and Sensor Data Integration
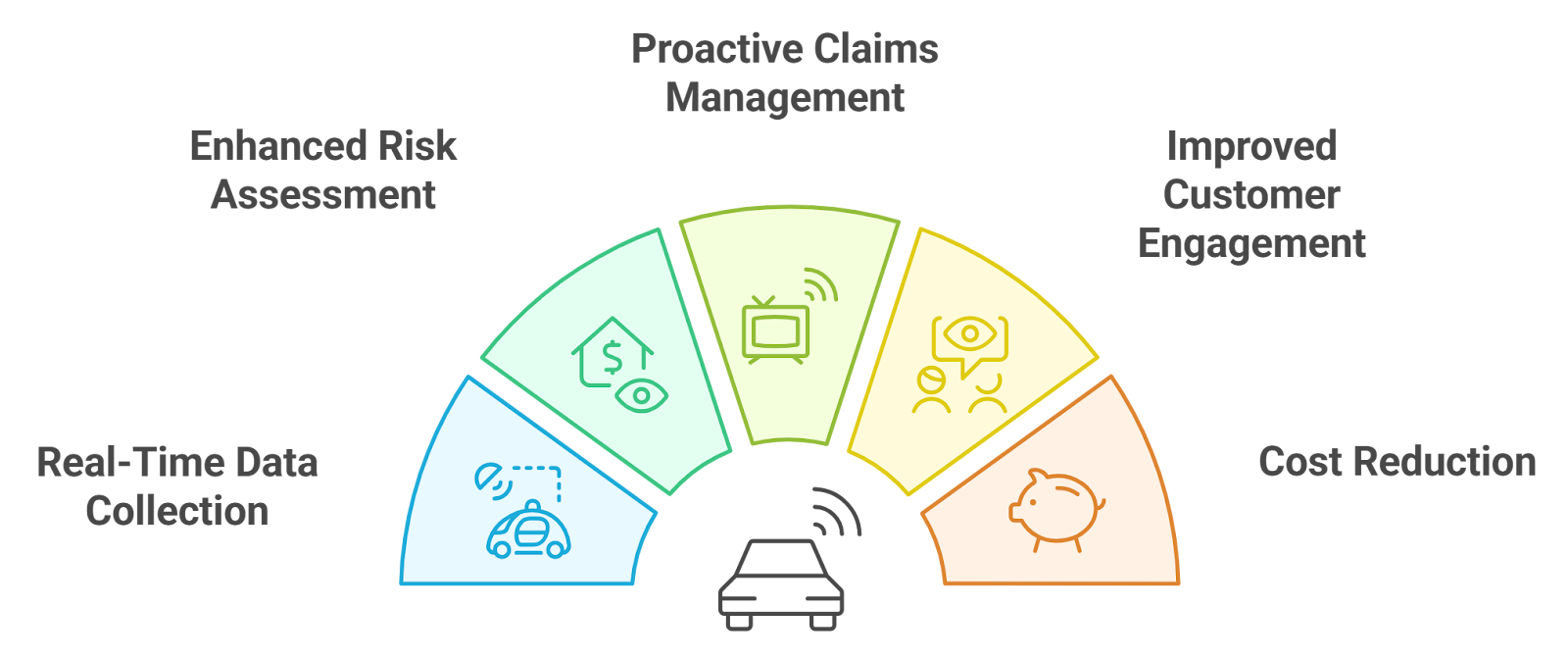
‍
The integration of IoT and sensor data into the insurance sector is transforming how insurers assess risk, manage claims, and engage with customers. IoT devices collect real-time data that can be used to inform underwriting processes and improve customer service, showcasing the importance of insurance technology integration.
- Real-Time Data Collection: IoT devices, such as telematics in vehicles or smart home sensors, provide insurers with continuous data streams. This data can be used to assess risk more accurately and tailor policies to individual customers.
- Enhanced Risk Assessment: By analyzing data from IoT devices, insurers can gain insights into customer behavior and risk factors. For example, a driver’s habits can be monitored through telematics, allowing insurers to offer personalized premiums based on driving behavior.
- Proactive Claims Management: IoT technology enables insurers to respond proactively to incidents. For instance, if a smart home sensor detects a water leak, the insurer can be alerted immediately, allowing for quicker response times and potentially reducing damage.
- Improved Customer Engagement: Insurers can use data from IoT devices to engage with customers more effectively. Personalized communication based on real-time data can enhance customer satisfaction and loyalty.
- Cost Reduction: By leveraging IoT data, insurers can reduce losses and improve operational efficiency. This can lead to lower costs for both the insurer and the insured.
The integration of IoT and sensor data is reshaping the insurance landscape, enabling companies to offer more personalized services and improve overall operational efficiency. As technology continues to evolve, the potential for IoT in the insurance industry will only grow. Rapid Innovation is at the forefront of this transformation, helping clients harness IoT capabilities to achieve their business goals effectively and efficiently through insurance technology integration.
10.3. Telematics and Real-time Monitoring
Telematics refers to the integration of telecommunications and monitoring systems, primarily used in vehicles and machinery. It enables the collection, transmission, and analysis of data in real-time, providing valuable insights for businesses and consumers alike.
- Enhanced Fleet Management: Telematics allows companies to track their vehicles' locations, speeds, and routes in real-time. This leads to improved route optimization, reduced fuel consumption, and enhanced driver safety, ultimately contributing to a higher return on investment (ROI).
- Predictive Maintenance: By monitoring vehicle performance and health, telematics can predict when maintenance is needed, reducing downtime and repair costs. This proactive approach can extend the lifespan of vehicles and equipment, ensuring that assets are utilized efficiently.
- Improved Customer Experience: Real-time monitoring enables businesses to provide accurate delivery times and updates to customers, enhancing satisfaction and trust. This can lead to increased customer loyalty and repeat business, further driving revenue growth.
- Data-Driven Decisions: The data collected through telematics can be analyzed to identify trends and patterns, helping businesses make informed decisions regarding operations and resource allocation. This analytical capability can lead to more strategic investments and improved operational efficiency.
- Regulatory Compliance: Many industries require compliance with safety and environmental regulations. Telematics can help ensure adherence to these regulations by providing necessary data and reports, thereby mitigating risks and potential fines.
At Rapid Innovation, we leverage our expertise in AI and Blockchain to help clients implement telematics and real-time monitoring technologies effectively, ensuring they achieve their business goals efficiently and effectively while maximizing ROI. Additionally, we explore how microservices support CIOs in realizing business-IT alignment.
10.4. API and Microservices Architecture
API (Application Programming Interface) and microservices architecture are essential components of modern software development, enabling flexibility, scalability, and integration.
- Modular Development: Microservices architecture breaks down applications into smaller, independent services that can be developed, deployed, and scaled individually. This modularity allows teams to work on different components simultaneously, speeding up development and reducing time to market.
- Enhanced Scalability: With microservices, businesses can scale specific services based on demand without affecting the entire application. This leads to more efficient resource utilization and cost savings, ultimately improving ROI.
- Improved Integration: APIs facilitate communication between different software systems, allowing for seamless integration of services. This is crucial for businesses that rely on multiple platforms and tools, enabling them to leverage existing investments effectively.
- Faster Time to Market: By leveraging APIs and microservices, organizations can quickly develop and deploy new features or services, responding to market demands more effectively. This agility can provide a competitive edge in rapidly changing markets.
- Technology Agnosticism: Microservices can be built using different programming languages and technologies, allowing businesses to choose the best tools for each service without being tied to a single technology stack. This flexibility can lead to better performance and innovation.
10.5. Legacy System Modernization
Legacy system modernization involves updating outdated software and hardware systems to improve efficiency, security, and functionality. Many organizations face challenges with legacy systems that hinder their ability to innovate and compete.
- Increased Efficiency: Modernizing legacy systems can streamline processes, reduce manual work, and improve overall operational efficiency. This can lead to significant cost savings and better resource allocation, enhancing overall business performance.
- Enhanced Security: Older systems often lack the security features necessary to protect against modern threats. Upgrading to newer technologies can help organizations safeguard sensitive data and comply with regulatory requirements, reducing the risk of data breaches.
- Improved User Experience: Legacy systems may not provide a user-friendly interface, leading to frustration among employees. Modernization can enhance usability, making it easier for staff to perform their tasks effectively, which can improve productivity.
- Integration Capabilities: Newer systems are designed to integrate seamlessly with other applications and services. This allows organizations to leverage existing data and tools, enhancing overall functionality and enabling better decision-making.
- Future-Proofing: By modernizing legacy systems, organizations can ensure they remain competitive in a rapidly changing technological landscape. This adaptability is crucial for long-term success and growth, allowing businesses to capitalize on new opportunities as they arise.
11. Emerging Trends and Future Outlook
The insurance industry is undergoing significant transformation due to technological advancements. Emerging trends such as quantum computing and explainable AI are set to reshape the landscape of claims processing and decision-making. Additionally, various insurance technology trends, including digital trends in insurance and emerging technologies in the insurance industry, are influencing this evolution.
11.1 Quantum Computing in Claims
Quantum computing represents a revolutionary leap in computational power, enabling the processing of complex data sets at unprecedented speeds. This technology has the potential to transform claims management in several ways:
- Enhanced Data Processing: Quantum computers can analyze vast amounts of data quickly, allowing insurers to process claims more efficiently. This can lead to faster claim resolutions and improved customer satisfaction, aligning with the latest trends in insurance technology.
- Risk Assessment: By utilizing quantum algorithms, insurers can better assess risks associated with claims. This can lead to more accurate underwriting and pricing models, ultimately benefiting both insurers and policyholders, particularly in the context of property and casualty insurance technology trends.
- Fraud Detection: Quantum computing can enhance fraud detection capabilities by analyzing patterns and anomalies in claims data. This can help insurers identify fraudulent claims more effectively, reducing losses and improving overall profitability, which is crucial in the current insurance tech trends.
- Predictive Analytics: With the ability to process complex variables, quantum computing can improve predictive analytics in claims management. Insurers can better forecast claim trends and adjust their strategies accordingly, reflecting the emerging technologies in the insurance industry.
As quantum computing continues to evolve, its integration into the insurance sector will likely lead to more innovative solutions and improved operational efficiencies. Rapid Innovation is at the forefront of this transformation, offering tailored quantum computing solutions that empower insurers to leverage this technology for enhanced operational performance and ROI.
11.2 Explainable AI Technologies
Explainable AI (XAI) refers to artificial intelligence systems designed to provide transparent and understandable insights into their decision-making processes. In the context of insurance claims, XAI is becoming increasingly important for several reasons:
- Transparency: XAI allows insurers to explain how decisions are made regarding claims. This transparency builds trust with policyholders, as they can understand the rationale behind claim approvals or denials, which is essential in the context of insurance technology trends.
- Regulatory Compliance: As regulations around AI and data usage become stricter, XAI helps insurers comply with legal requirements. By providing clear explanations of AI-driven decisions, insurers can demonstrate accountability and adherence to regulations, aligning with the trends in insurance technology.
- Improved Decision-Making: XAI can enhance the decision-making process by providing insights into the factors influencing claims outcomes. This can help claims adjusters make more informed decisions and reduce biases in the claims process, reflecting the latest trends in insurance technology.
- Customer Engagement: By leveraging XAI, insurers can improve customer engagement. When policyholders receive clear explanations for claims decisions, they are more likely to feel valued and understood, leading to better customer relationships, which is a key aspect of digital trends in insurance.
The adoption of explainable AI technologies in claims processing is expected to grow, as insurers seek to balance the benefits of AI with the need for transparency and accountability. Rapid Innovation specializes in developing XAI solutions that not only enhance decision-making but also foster trust and compliance in the insurance sector.
In conclusion, both quantum computing and explainable AI are poised to significantly impact the future of claims management in the insurance industry. By embracing these emerging trends, including insurance technology trends 2022 and 2023, insurers can enhance efficiency, improve customer satisfaction, and navigate the complexities of modern claims processing. Rapid Innovation is committed to guiding clients through this technological evolution, ensuring they achieve greater ROI and operational excellence.
11.3. Autonomous Insurance Ecosystems
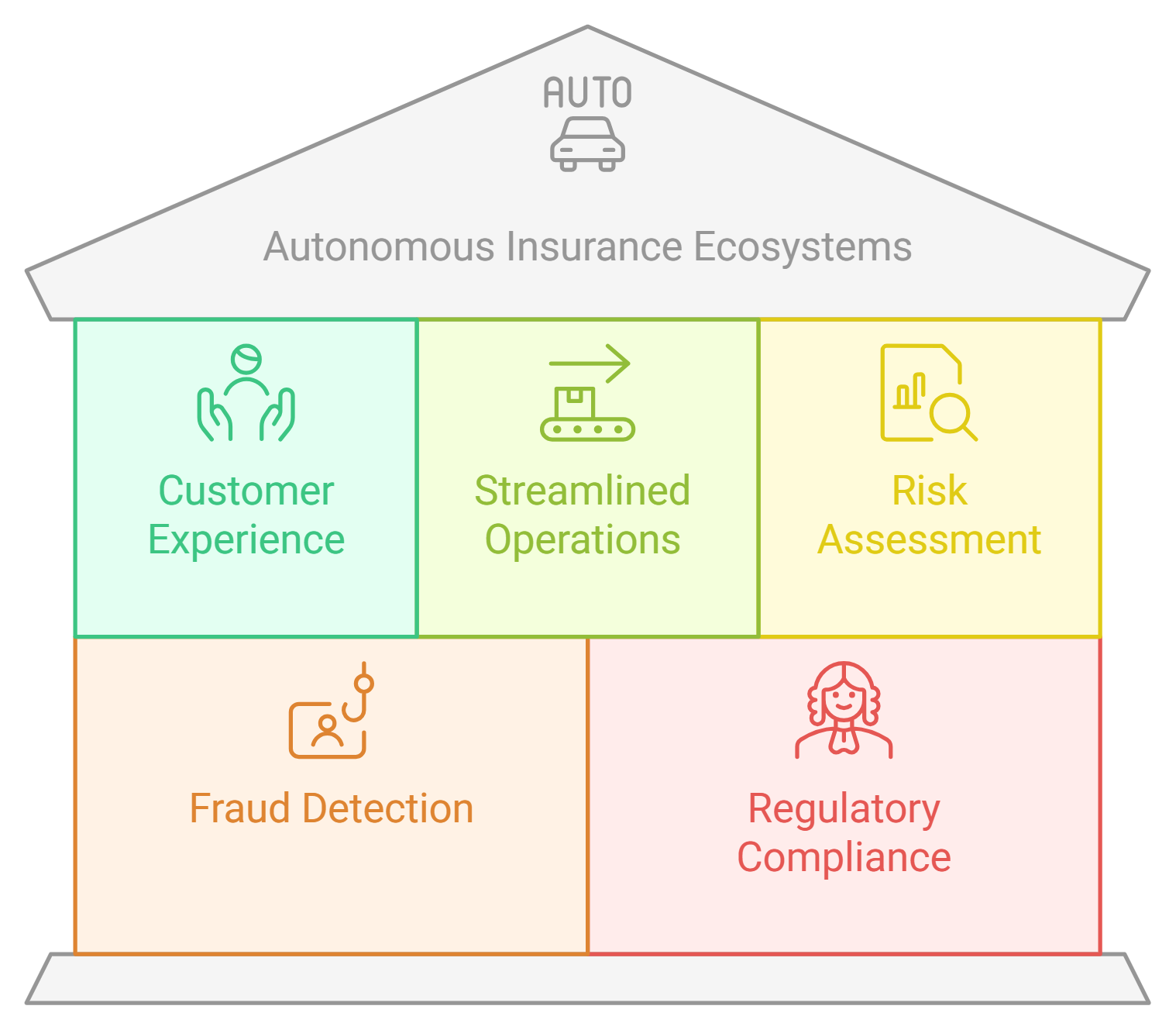
‍
Autonomous insurance ecosystems represent a transformative shift in the insurance industry, leveraging advanced technologies to create self-operating systems that enhance efficiency and customer experience. These ecosystems integrate various technologies, including artificial intelligence (AI), machine learning, and blockchain, to automate processes and improve decision-making.
- Enhanced customer experience: Autonomous insurance ecosystems can provide personalized insurance solutions, tailoring policies to individual needs based on real-time data analysis. Rapid Innovation can assist in developing these systems, ensuring that clients can offer customized experiences that meet the evolving demands of their customers.
- Streamlined operations: Automation reduces manual intervention, leading to faster claims processing and policy issuance, which can significantly lower operational costs. Our expertise in AI and blockchain allows us to implement solutions that optimize these processes, resulting in improved efficiency and reduced overhead.
- Risk assessment: AI algorithms can analyze vast amounts of data to identify potential risks more accurately, allowing insurers to adjust premiums and coverage accordingly. Rapid Innovation can help clients harness AI capabilities to enhance their risk assessment models, leading to better-informed decisions and increased profitability. Additionally, insights from AI in underwriting for insurance can further refine these processes.
- Fraud detection: Machine learning models can detect unusual patterns in claims, helping to identify fraudulent activities more effectively. By integrating advanced analytics, we empower our clients to mitigate fraud risks, ultimately protecting their bottom line.
- Regulatory compliance: Autonomous insurance ecosystems can ensure adherence to regulations by automatically updating policies and procedures in response to changing laws. Rapid Innovation's blockchain solutions can enhance transparency and traceability, ensuring compliance while minimizing the risk of regulatory penalties.
The shift towards autonomous insurance ecosystems is not just about technology; it also involves a cultural change within organizations, emphasizing agility and innovation.
11.4. Predictive Intelligence Evolution
Predictive intelligence is evolving rapidly, transforming how businesses, including insurance companies, operate. This evolution is driven by advancements in data analytics, machine learning, and AI, enabling organizations to anticipate customer needs and market trends more accurately.
- Data-driven decision-making: Predictive intelligence allows insurers to analyze historical data and identify patterns, leading to more informed decisions regarding underwriting, pricing, and risk management. Rapid Innovation can assist clients in implementing predictive analytics frameworks that enhance their decision-making processes.
- Customer insights: By leveraging predictive analytics, insurers can gain deeper insights into customer behavior, preferences, and potential churn, allowing for targeted marketing strategies. Our expertise enables clients to develop data-driven marketing campaigns that resonate with their target audience.
- Improved claims management: Predictive models can forecast claim volumes and types, enabling insurers to allocate resources more effectively and enhance customer service. Rapid Innovation can help clients optimize their claims management processes, leading to improved customer satisfaction and retention.
- Enhanced underwriting processes: Predictive intelligence can streamline underwriting by assessing risk factors more accurately, leading to faster approvals and better pricing strategies. Our solutions empower clients to refine their underwriting processes, resulting in increased efficiency and profitability.
- Competitive advantage: Companies that adopt predictive intelligence can stay ahead of competitors by quickly adapting to market changes and customer demands. Rapid Innovation's tailored solutions ensure that clients leverage predictive intelligence to maintain a competitive edge in the market.
As predictive intelligence continues to evolve, its integration into business processes will become increasingly essential for maintaining a competitive edge in the insurance industry.
11.5. Global Technological Convergence
Global technological convergence refers to the merging of various technologies and industries, leading to innovative solutions and new business models. This phenomenon is particularly relevant in the insurance sector, where advancements in technology are reshaping traditional practices.
- Cross-industry collaboration: Insurers are increasingly partnering with tech companies, fintechs, and insurtechs to leverage their expertise and develop innovative products and services. Rapid Innovation facilitates these collaborations, helping clients access cutting-edge technologies and expertise.
- Integration of IoT: The Internet of Things (IoT) is playing a crucial role in global technological convergence, providing insurers with real-time data from connected devices, which enhances risk assessment and policy customization. Our solutions enable clients to harness IoT data effectively, leading to improved risk management and customer engagement.
- Blockchain technology: The adoption of blockchain in insurance is revolutionizing data security and transparency, enabling secure transactions and reducing fraud. Rapid Innovation specializes in blockchain solutions that enhance data integrity and trust in insurance transactions.
- Artificial intelligence and machine learning: These technologies are converging with traditional insurance practices to automate processes, improve customer service, and enhance risk management. Our expertise in AI and machine learning allows us to develop innovative solutions that drive operational efficiency and customer satisfaction.
- Regulatory challenges: As technologies converge, regulators face the challenge of keeping up with rapid changes, necessitating new frameworks to ensure consumer protection and market stability. Rapid Innovation stays ahead of regulatory trends, helping clients navigate compliance challenges effectively.
The impact of global technological convergence on the insurance industry is profound, driving innovation and creating opportunities for growth and efficiency. Rapid Innovation is committed to guiding clients through this transformative landscape, ensuring they achieve their business goals efficiently and effectively.
12. Implementation Roadmap
An effective implementation roadmap, such as a safe implementation roadmap or a scaled agile implementation roadmap, is crucial for the successful execution of any project. It provides a structured approach to ensure that all necessary steps are taken to achieve the desired outcomes. This section outlines the key components of the implementation roadmap, focusing on the initial assessment and strategy, as well as the design of a pilot program.
12.1 Initial Assessment and Strategy
The initial assessment and strategy phase is vital for laying the groundwork for the project. This phase involves evaluating the current situation, identifying needs, and formulating a strategic plan to address those needs.
- Conduct a Needs Analysis: Identify the specific needs of the organization or target audience by gathering data through surveys, interviews, and focus groups to understand pain points and expectations. This analysis is essential for tailoring AI and Blockchain solutions that directly address client challenges.
- Set Clear Objectives: Define measurable goals that align with the overall vision of the project, ensuring that objectives are SMART (Specific, Measurable, Achievable, Relevant, Time-bound). For instance, if a client aims to enhance operational efficiency through AI, objectives could include reducing processing time by a specific percentage.
- Stakeholder Engagement: Involve key stakeholders early in the process to gain insights and foster buy-in. Create a communication plan to keep stakeholders informed and engaged throughout the project. Engaging stakeholders ensures that the AI and Blockchain solutions developed are aligned with their expectations and business needs.
- Resource Assessment: Evaluate the resources available, including budget, personnel, and technology. Identify any gaps in resources that may need to be addressed before implementation. This assessment helps in determining the feasibility of integrating advanced technologies like AI and Blockchain.
- Risk Analysis: Identify potential risks and challenges that could impact the project. Develop mitigation strategies to address these risks proactively. For example, in Blockchain projects, risks may include regulatory compliance, which can be addressed through thorough legal assessments.
- Develop a Strategic Plan: Create a comprehensive strategy that outlines the steps needed to achieve the project objectives, including timelines, responsibilities, and key performance indicators (KPIs) to measure success. This plan serves as a roadmap for deploying AI and Blockchain solutions effectively, similar to a scaled agile framework implementation roadmap.
12.2 Pilot Program Design
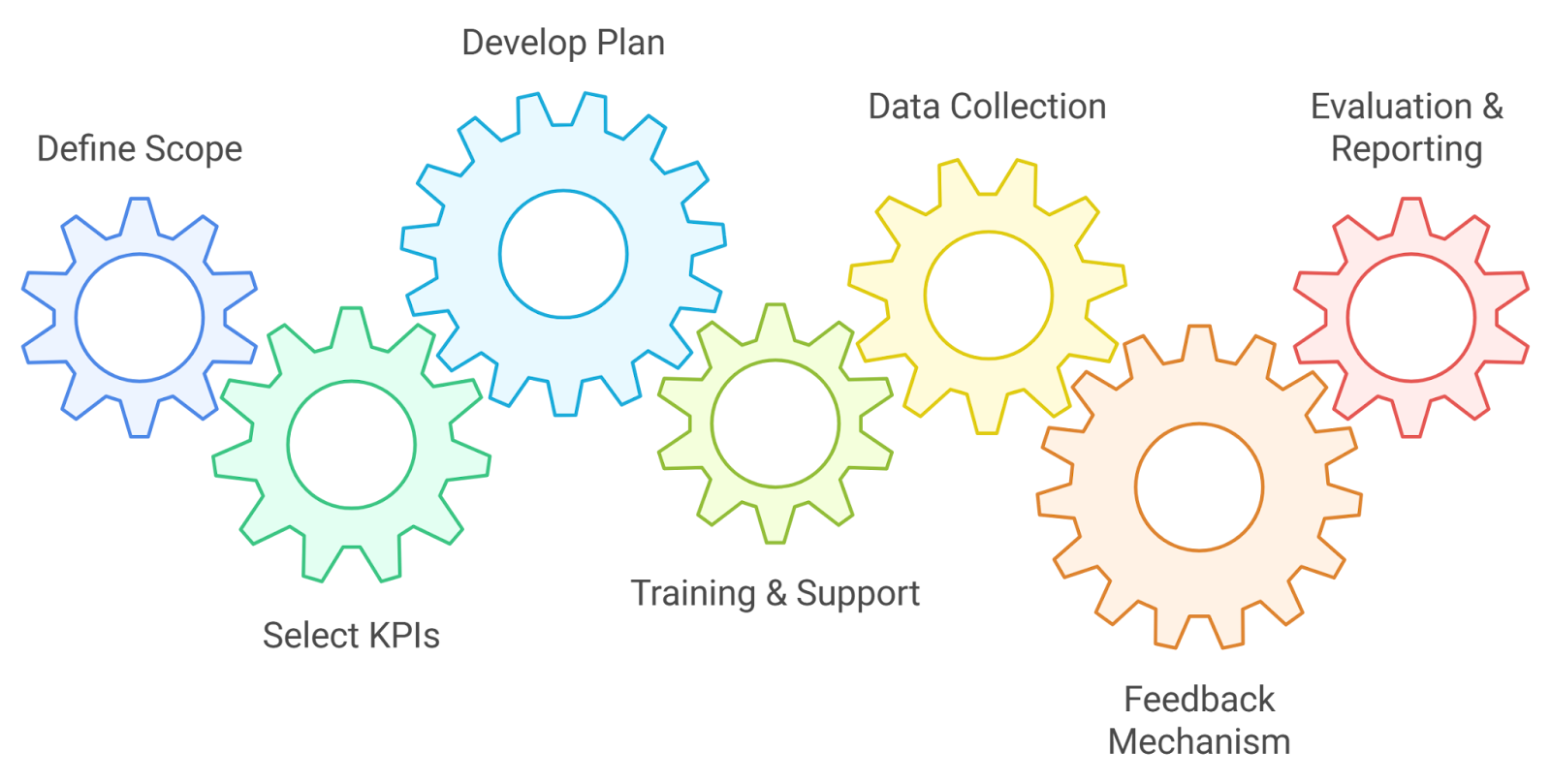
‍
Designing a pilot program is an essential step in the implementation roadmap. A pilot program allows for testing the proposed solutions on a smaller scale before full-scale implementation.
- Define the Scope of the Pilot: Determine the specific objectives and outcomes you want to achieve with the pilot program. Identify the target audience and the geographical area for the pilot. This focused approach allows for precise evaluation of AI and Blockchain applications.
- Select Key Performance Indicators (KPIs): Establish KPIs to measure the success of the pilot program, ensuring that KPIs are aligned with the overall project objectives. For example, in an AI-driven customer service pilot, KPIs could include response time and customer satisfaction scores.
- Develop a Detailed Plan: Create a step-by-step plan for executing the pilot program, including timelines and responsibilities. Outline the resources needed, such as personnel, technology, and budget. This detailed planning is crucial for the successful deployment of innovative technologies, akin to a power bi implementation roadmap or a salesforce implementation roadmap.
- Training and Support: Provide training for staff and participants involved in the pilot program. Ensure that support mechanisms are in place to address any issues that arise during the pilot. Training is particularly important in AI and Blockchain projects, where users may need to adapt to new systems.
- Data Collection and Analysis: Implement a system for collecting data throughout the pilot program. Analyze the data to assess the effectiveness of the program and identify areas for improvement. Data-driven insights are essential for refining AI algorithms and Blockchain functionalities.
- Feedback Mechanism: Establish a process for gathering feedback from participants and stakeholders. Use this feedback to make necessary adjustments before full-scale implementation. Continuous feedback loops enhance the adaptability of AI and Blockchain solutions.
- Evaluation and Reporting: Conduct a thorough evaluation of the pilot program to determine its success. Prepare a report summarizing the findings, lessons learned, and recommendations for the next steps. This evaluation is critical for demonstrating ROI and guiding future projects, similar to an implementation roadmap example or an ERP implementation roadmap.
By following these structured steps in the initial assessment and strategy phase, as well as in the pilot program design, organizations can create a solid foundation for successful project implementation. This roadmap not only helps in identifying potential challenges but also ensures that the project aligns with the overall goals and objectives of the organization. Rapid Innovation is committed to guiding clients through this process, leveraging our expertise in AI consulting to drive efficiency and maximize ROI, much like a safe agile implementation roadmap or a scaled agile framework roadmap.
12.3. Scalability Considerations
Scalability is a critical aspect of any system or process, particularly in technology and business environments. It refers to the ability of a system to handle a growing amount of work or its potential to accommodate growth. Here are some key considerations for scalability:
- Horizontal vs. Vertical Scaling: Horizontal scaling involves adding more machines or nodes to a system, while vertical scaling means upgrading the existing hardware. Choosing the right approach depends on the specific needs and architecture of the system. Rapid Innovation can assist clients in determining the most effective scaling strategy based on their unique requirements, ensuring optimal performance and cost-efficiency. This is particularly relevant in the context of scalability solutions for blockchain and crypto environments.
- Performance Metrics: Establish clear performance metrics to evaluate how well the system scales. Metrics may include response time, throughput, and resource utilization. By leveraging AI-driven analytics, Rapid Innovation helps clients monitor these metrics in real-time, enabling proactive adjustments to maintain performance as demand grows. This is crucial for assessing the scalability of blockchain and cryptocurrency systems.
- Load Balancing: Implement load balancing to distribute workloads evenly across servers. This ensures no single server becomes a bottleneck, enhancing overall system performance. Our expertise in blockchain technology can also facilitate decentralized load balancing solutions, improving resilience and efficiency, which is essential for scaling crypto applications.
- Database Scalability: Consider how the database will scale with increased data and user load. Techniques such as sharding, replication, and caching can help manage database scalability. Rapid Innovation provides tailored database solutions that ensure seamless scalability, allowing clients to handle increased transactions without compromising speed or reliability. This is particularly important for scalability in blockchain applications.
- Cloud Solutions: Leverage cloud services that offer scalable resources on-demand. This allows businesses to adjust their infrastructure based on current needs without significant upfront investment. Rapid Innovation partners with leading cloud providers to deliver customized cloud solutions that align with clients' growth trajectories, including those in the blockchain and crypto sectors.
- Testing for Scalability: Conduct stress testing and load testing to identify potential scalability issues before they impact users. This proactive approach helps in planning for future growth. Our team employs advanced testing methodologies to simulate various load scenarios, ensuring that systems are robust and ready for expansion, especially in the context of blockchain scalability solutions.
By focusing on scalability, including blockchain scalability solutions and scalability of cryptocurrency, change management, and continuous improvement, organizations can position themselves for long-term success and adaptability in a rapidly changing environment. Rapid Innovation is committed to guiding clients through these critical areas, ensuring they achieve their business goals efficiently and effectively while maximizing ROI.
13. Regulatory and Compliance Landscape
The regulatory and compliance landscape is a critical aspect of business operations, especially in today's interconnected world. Organizations must navigate a complex web of regulations that vary by region, industry, and technology. Understanding these regulations, including regulatory compliance, is essential for maintaining compliance, protecting data, and ensuring operational integrity.
13.1 Global Regulatory Perspectives
The global regulatory landscape is characterized by a diverse set of laws and guidelines that govern various aspects of business operations. Key considerations include:
- Varied Regulations Across Regions: Different countries have unique regulatory frameworks. For instance, the European Union has stringent regulations like the General Data Protection Regulation (GDPR), while the United States has a more fragmented approach with sector-specific regulations, including compliance regulations by industry.
- Harmonization Efforts: There are ongoing efforts to harmonize regulations across borders. Initiatives like the OECD Guidelines for Multinational Enterprises aim to create a more consistent regulatory environment, facilitating international trade and investment.
- Industry-Specific Regulations: Certain industries, such as finance and healthcare, face additional regulatory scrutiny. Compliance with regulations like the Health Insurance Portability and Accountability Act (HIPAA) in the U.S. or the Payment Card Industry Data Security Standard (PCI DSS) is crucial for organizations operating in these sectors. This includes banking compliance regulations and compliance with the regulations specific to financial services.
- Emerging Regulations: New regulations are continually emerging, particularly in response to technological advancements. For example, regulations surrounding artificial intelligence (AI) and blockchain technology are being developed to address ethical and security concerns. Rapid Innovation specializes in helping organizations understand and comply with these emerging regulations, ensuring that they can leverage AI and blockchain technologies while adhering to legal requirements, including regulatory compliance services.
- Impact of Non-Compliance: Failing to comply with regulations can result in severe penalties, including fines, legal action, and reputational damage. Organizations must stay informed about regulatory changes to mitigate these risks. Rapid Innovation offers consulting services to help clients navigate these complexities, reducing the likelihood of non-compliance and enhancing their operational integrity through compliance and regulatory management.
13.2 Data Privacy Considerations
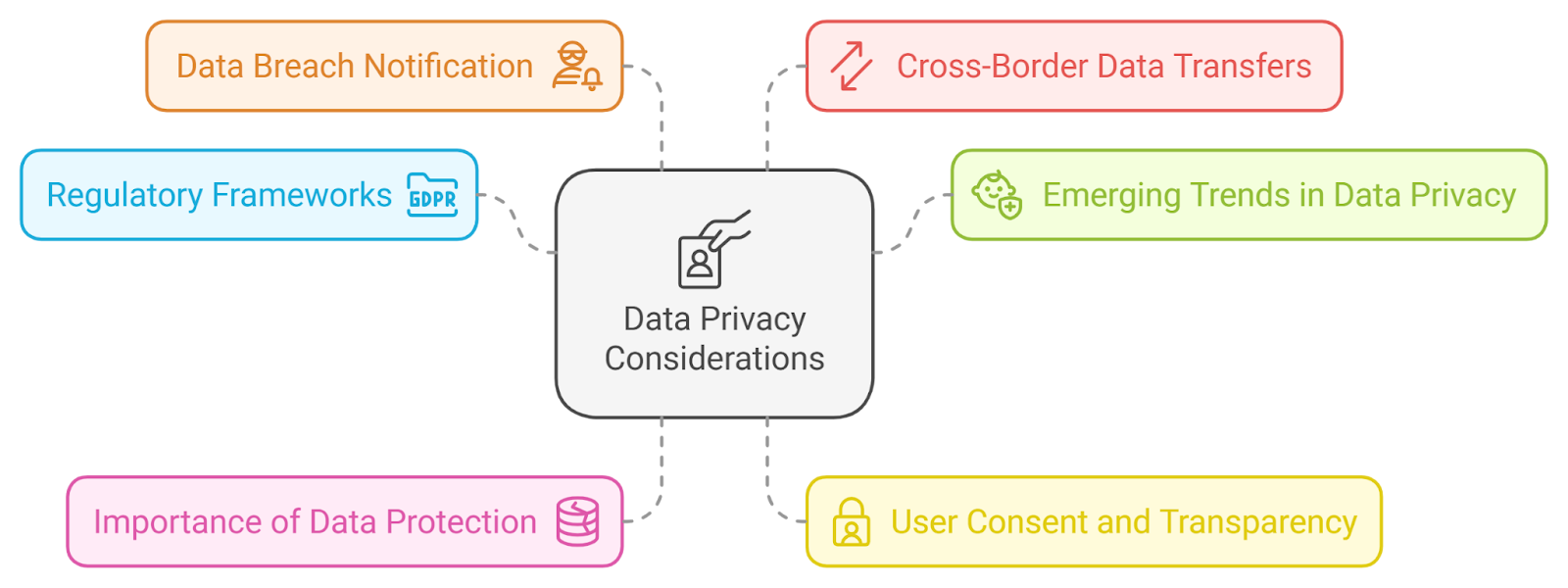
‍
Data privacy is a significant concern for organizations worldwide, driven by increasing public awareness and regulatory scrutiny. Key considerations include:
- Importance of Data Protection: Protecting personal data is not only a legal requirement but also essential for maintaining customer trust. Organizations must implement robust data protection measures to safeguard sensitive information, ensuring compliance with legal and regulatory requirements.
- Regulatory Frameworks: Various regulations govern data privacy, including GDPR in Europe, the California Consumer Privacy Act (CCPA) in the U.S., and the Personal Information Protection and Electronic Documents Act (PIPEDA) in Canada. Each regulation has specific requirements for data collection, storage, and processing. Rapid Innovation assists clients in developing compliant data management strategies that align with these frameworks, including compliance with the PCI DSS regulatory requirements.
- User Consent and Transparency: Many data privacy regulations emphasize the importance of obtaining user consent before collecting personal data. Organizations must be transparent about how they use data and provide users with options to control their information, aligning with compliance to regulation standards.
- Data Breach Notification: Regulations often require organizations to notify affected individuals and authorities in the event of a data breach. Timely communication is crucial to mitigate potential harm and comply with legal obligations. Rapid Innovation can help implement systems that ensure compliance with these notification requirements.
- Cross-Border Data Transfers: Transferring data across borders can complicate compliance efforts. Organizations must understand the regulations governing international data transfers, such as the EU-U.S. Privacy Shield framework, to ensure compliance.
- Emerging Trends in Data Privacy: As technology evolves, so do data privacy concerns. Trends such as increased use of biometric data, the rise of the Internet of Things (IoT), and the growing importance of data ethics are shaping the future of data privacy regulations. Rapid Innovation stays ahead of these trends, providing clients with insights and solutions that align with evolving regulatory landscapes, including regtech solutions.
In conclusion, navigating the regulatory and compliance landscape requires a proactive approach. Organizations must stay informed about global regulatory perspectives and data privacy considerations to ensure compliance and protect their operations. Rapid Innovation is committed to guiding clients through these complexities, enabling them to achieve their business goals efficiently and effectively while maximizing their return on investment through certified regulatory compliance and financial services regulatory and compliance strategies, including overview of regulations compliance best practice.
13.3. Ethical AI Guidelines
Ethical AI guidelines are essential for ensuring that artificial intelligence systems operate in a manner that is fair, responsible, and aligned with societal values. These guidelines help mitigate risks associated with AI technologies, such as bias, discrimination, and privacy violations. Various frameworks, including the 'ai ethical guidelines' and 'ethics guidelines for trustworthy ai', provide a foundation for these practices.
- Fairness: AI systems should be designed to treat all individuals equitably, avoiding biases based on race, gender, or socioeconomic status.
- Accountability: Developers and organizations must take responsibility for the outcomes of their AI systems, ensuring that there are mechanisms in place to address any negative impacts.
- Privacy: Ethical AI must prioritize user privacy, implementing robust data protection measures to safeguard personal information.
- Transparency: AI algorithms should be understandable and explainable, allowing users to comprehend how decisions are made.
- Collaboration: Stakeholders, including governments, businesses, and civil society, should work together to establish and uphold ethical standards in AI development, as outlined in the 'ethical guidelines for ai' and 'ethical ai guidelines'.
Organizations like the IEEE and the European Commission have published frameworks and guidelines, such as the 'eu ethics guidelines for trustworthy ai', to promote ethical AI practices. These resources serve as valuable references for companies looking to align their AI initiatives with ethical standards, including the 'ethics guidelines for trustworthy ai european commission' and 'ethics guidelines for trustworthy ai pdf'.
At Rapid Innovation, we understand the importance of these ethical considerations and compliance challenges. Our expertise in AI and Blockchain development allows us to guide organizations in implementing ethical AI practices while ensuring compliance with international regulations. By leveraging our consulting solutions, clients can achieve greater ROI through responsible AI deployment that aligns with their business goals and societal values. For a comprehensive overview of ethical AI practices, refer to our ethical AI development guide.
13.4. Transparency and Accountability
Transparency and accountability are critical components of responsible AI deployment. They ensure that AI systems are not only effective but also trustworthy and justifiable in their operations.
- Explainability: AI systems should provide clear explanations for their decisions, enabling users to understand the rationale behind outcomes. This is particularly important in high-stakes areas like healthcare and criminal justice.
- Auditability: Organizations should implement regular audits of their AI systems to assess performance, identify biases, and ensure compliance with ethical standards, as highlighted in 'the ethics of ai ethics an evaluation of guidelines'.
- Reporting: Companies must establish clear reporting mechanisms for stakeholders to voice concerns or report issues related to AI systems.
- Governance: Strong governance frameworks should be in place to oversee AI development and deployment, ensuring that ethical considerations are integrated into every stage of the process.
- Stakeholder Engagement: Engaging with diverse stakeholders, including affected communities, can help organizations understand the broader implications of their AI systems and foster trust.
By prioritizing transparency and accountability, organizations can build public confidence in AI technologies and mitigate potential risks associated with their use.
13.5. Cross-Border Compliance Challenges
As AI technologies continue to evolve, they often operate across international borders, leading to complex compliance challenges. Different countries have varying regulations and standards regarding data protection, privacy, and AI ethics, which can create obstacles for organizations.
- Regulatory Divergence: Countries may have different laws governing AI, making it difficult for companies to navigate compliance. For example, the European Union's General Data Protection Regulation (GDPR) imposes strict data protection requirements that may not align with regulations in other regions.
- Data Transfer: Cross-border data transfers can be complicated by differing privacy laws. Organizations must ensure that they comply with both local and international regulations when handling personal data.
- Enforcement Variability: The enforcement of AI regulations can vary significantly between jurisdictions, leading to uncertainty for companies operating globally. This inconsistency can create challenges in maintaining compliance and managing risks.
- Cultural Differences: Different cultural attitudes towards privacy and technology can influence how AI is perceived and regulated in various regions. Organizations must be sensitive to these differences when developing and deploying AI solutions.
- Collaboration: To address these challenges, international cooperation and dialogue among regulators, businesses, and civil society are essential. Establishing common standards and frameworks can help facilitate compliance and promote responsible AI use across borders.
Navigating cross-border compliance challenges requires a proactive approach, with organizations staying informed about regulatory developments and engaging with stakeholders to ensure alignment with diverse legal frameworks.