Table Of Contents
Category
1. Introduction to Behavioral Pattern Analysis
Behavioral pattern analysis is a crucial aspect of understanding human actions and interactions. It involves examining data to identify trends, habits, and anomalies in behavior. This analysis is increasingly important in various fields, including marketing, healthcare, finance, and cybersecurity.
- Behavioral pattern analysis helps organizations make informed decisions based on data-driven insights.
- It can reveal underlying motivations and preferences, allowing for more personalized experiences.
- The rise of artificial intelligence (AI) has significantly enhanced the capabilities of behavioral pattern analysis, enabling more sophisticated and accurate assessments.
At Rapid Innovation, we leverage advanced AI technologies to empower our clients in their behavioral pattern analysis efforts. Our AI agents are software programs that utilize machine learning algorithms to analyze vast amounts of data. They can identify patterns that may not be immediately apparent to human analysts. By integrating our AI solutions, organizations can gain deeper insights into consumer behavior, employee performance, and even social dynamics, including buying pattern analysis and buying pattern behaviour analysis.
- AI agents can process data at an unprecedented scale and speed.
- They can adapt to new information, continuously improving their analysis over time.
- The integration of AI in behavioral pattern analysis allows for real-time monitoring and predictive analytics.
In summary, behavioral pattern analysis is essential for understanding and predicting human behavior. The incorporation of AI agents into this field enhances the accuracy and efficiency of the analysis, providing organizations with valuable insights that can drive strategic decisions. At Rapid Innovation, we are committed to helping our clients achieve greater ROI through effective and efficient AI-driven solutions.
1.1. Understanding Behavioral Analytics
Behavioral analytics is the process of collecting and analyzing data about user behavior to gain insights into their actions, preferences, and motivations. This field has gained significant traction in recent years due to the increasing availability of data and advancements in technology.
- Behavioral analytics focuses on understanding how users interact with products, services, or platforms.
- It involves tracking user actions, such as clicks, page views, and time spent on specific content.
- The insights derived from behavioral analytics can help businesses improve user experience, optimize marketing strategies, and enhance product development.
- Key metrics often analyzed include conversion rates, user engagement, and retention rates.
- By understanding user behavior, companies can tailor their offerings to meet customer needs more effectively.
At Rapid Innovation, we leverage behavioral analytics, including user behavior analytics and customer behavior analytics, to help our clients identify key user trends, enabling them to refine their products and services for maximum impact. By implementing tailored analytics solutions, we empower businesses to achieve greater ROI through enhanced customer satisfaction and loyalty.
1.2. Evolution of Pattern Recognition
Pattern recognition is a critical component of behavioral analytics, allowing systems to identify trends and anomalies in user behavior. The evolution of pattern recognition has been influenced by advancements in technology and the growing complexity of data.
- Early pattern recognition systems relied on simple algorithms and manual data analysis.
- With the advent of machine learning and artificial intelligence, pattern recognition has become more sophisticated.
- Modern systems can analyze vast amounts of data in real-time, identifying patterns that would be impossible for humans to detect.
- Techniques such as neural networks and deep learning have revolutionized the field, enabling more accurate predictions and insights.
- The evolution of pattern recognition has led to applications in various industries, including finance, healthcare, and marketing.
At Rapid Innovation, we harness advanced pattern recognition techniques to provide our clients with actionable insights. By utilizing AI-driven models, we help businesses detect anomalies and trends that can inform strategic decisions, ultimately leading to improved operational efficiency and profitability.
1.3. Role of AI in Behavioral Analysis
Artificial intelligence (AI) plays a pivotal role in enhancing behavioral analysis by automating data processing and providing deeper insights into user behavior. AI technologies enable businesses to make data-driven decisions more efficiently.
- AI algorithms can analyze large datasets quickly, identifying trends and patterns that inform strategic decisions.
- Machine learning models can predict user behavior based on historical data, allowing for personalized marketing and improved customer experiences.
- Natural language processing (NLP) helps in understanding user sentiment and feedback, providing valuable insights into customer satisfaction.
- AI-driven tools can automate the segmentation of users based on behavior, enabling targeted marketing campaigns.
- The integration of AI in behavioral analysis leads to more accurate forecasting and better resource allocation for businesses.
At Rapid Innovation, we specialize in integrating AI into behavioral analysis frameworks, including user and entity behavior analytics and user behavior analysis, allowing our clients to unlock the full potential of their data. By employing machine learning and NLP, we enable businesses to enhance customer engagement and drive higher returns on investment through targeted strategies and personalized experiences.
1.4. Ethical Considerations and Privacy Framework
In the realm of AI-driven behavioral analysis, ethical considerations and privacy frameworks are paramount. As organizations increasingly rely on data to inform decisions, the need to protect individual privacy and ensure ethical use of data becomes critical.
- Informed Consent: Individuals should be made aware of how their data will be used. This includes clear communication about data collection methods and purposes.
- Data Minimization: Collect only the data necessary for the intended purpose. This reduces the risk of misuse and enhances privacy.
- Transparency: Organizations must be transparent about their data practices. This includes sharing information on data storage, processing, and sharing with third parties.
- Accountability: Establishing accountability mechanisms ensures that organizations adhere to ethical standards. This can involve regular audits and compliance checks.
- Bias Mitigation: AI systems can inadvertently perpetuate biases present in training data. It is essential to implement strategies to identify and mitigate these biases to ensure fair outcomes.
- User Rights: Individuals should have rights over their data, including access, correction, and deletion. This empowers users and fosters trust in AI systems.
- Regulatory Compliance: Adhering to regulations such as GDPR or CCPA is crucial. These laws set standards for data protection and privacy, ensuring that organizations operate within legal frameworks.
The ethical landscape surrounding AI is complex and requires ongoing dialogue among stakeholders, including technologists, ethicists, and policymakers. For more insights on best practices regarding data privacy in AI.
2. Core Components of AI-Driven Behavioral Analysis

‍
AI-driven behavioral analysis involves several core components that work together to derive insights from data. Understanding these components is essential for organizations looking to leverage AI effectively.
- Data Collection: Gathering relevant data from various sources is the first step. This can include user interactions, social media activity, and transaction histories.
- Data Processing: Once collected, data must be cleaned and processed. This involves removing duplicates, handling missing values, and transforming data into a usable format.
- Machine Learning Algorithms: These algorithms analyze the processed data to identify patterns and trends. Techniques such as supervised learning, unsupervised learning, and reinforcement learning are commonly used.
- Behavioral Insights: The ultimate goal is to extract actionable insights that can inform decision-making. This can include predicting user behavior, identifying customer segments, or optimizing marketing strategies.
- Visualization Tools: Presenting data in an understandable format is crucial. Visualization tools help stakeholders interpret complex data and make informed decisions.
- Feedback Loops: Continuous learning is essential for AI systems. Feedback loops allow models to improve over time based on new data and outcomes.
By understanding these core components, organizations can better implement AI-driven behavioral analysis to enhance their operations and customer engagement.
2.1. Data Collection Systems
Data collection systems are the backbone of AI-driven behavioral analysis. These systems enable organizations to gather, store, and manage data effectively.
- Types of Data: Organizations can collect various types of data, including structured data (e.g., databases, spreadsheets), unstructured data (e.g., social media posts, emails), and semi-structured data (e.g., XML, JSON).
- Data Sources: Common sources for data collection include web analytics tools (e.g., Google Analytics), customer relationship management (CRM) systems, social media platforms, and IoT devices.
- Collection Methods: Various methods can be employed to collect data, such as surveys and questionnaires, tracking user interactions on websites and apps, and API integrations with third-party services.
- Data Storage: Once collected, data must be stored securely. Options include cloud storage solutions (e.g., AWS, Azure), on-premises databases, and data lakes for unstructured data.
- Data Quality Assurance: Ensuring data quality is critical. This involves regular audits to identify inaccuracies, implementing validation checks during data entry, and establishing protocols for data cleaning and maintenance.
- Compliance and Security: Organizations must ensure that their data collection practices comply with relevant regulations. This includes implementing encryption for sensitive data, regularly updating security protocols, and training staff on data privacy best practices.
Effective data collection systems are essential for successful AI-driven behavioral analysis, as they provide the foundation for accurate insights and informed decision-making. Additionally, the integration of AI ABA (Applied Behavior Analysis) techniques can enhance the effectiveness of these systems by providing deeper insights into user behavior and improving the overall ethical framework surrounding data usage.
2.1.1. Sensor Networks
Sensor networks consist of interconnected devices that collect and transmit data about their environment. These networks are crucial in various applications, including smart cities, environmental monitoring, and industrial automation.
- Components of Sensor Networks: Â
- Sensors: Devices that detect changes in the environment, such as temperature, humidity, or motion. Examples include body sensor networks and low power wireless sensor networks.
- Communication Protocols: Methods that allow sensors to transmit data to a central system, such as Wi-Fi, Zigbee, or LoRaWAN. Wireless sensor networks often utilize these protocols for effective communication.
- Data Processing Units: Systems that analyze the data collected from sensors to derive meaningful insights.
- Applications: Â
- Smart Cities: Sensor networks help monitor traffic patterns, air quality, and energy consumption, leading to improved urban planning. Rapid Innovation can assist municipalities in implementing these networks, ensuring efficient data collection and analysis to enhance city management and resource allocation. Wireless sensor networks in IoT play a significant role in this area.
- Environmental Monitoring: Sensors can track changes in ecosystems, providing data for climate research and disaster management. Our expertise in AI can enhance predictive analytics, allowing organizations to respond proactively to environmental changes. Distributed sensor networks are particularly useful in this context.
- Industrial Automation: In manufacturing, sensor networks optimize processes by monitoring equipment performance and predicting maintenance needs. By integrating blockchain technology, we can ensure data integrity and traceability, leading to improved operational efficiency and reduced downtime. Industrial wireless sensor networks are key players in this sector.
- Challenges: Â
- Data Security: Protecting sensitive information collected by sensors is critical to prevent unauthorized access. Rapid Innovation employs advanced encryption and security protocols to safeguard data, addressing security in wireless sensor networks.
- Scalability: As the number of sensors increases, managing and processing the data becomes more complex. Our scalable solutions are designed to handle large volumes of data seamlessly, which is essential for applications of wireless sensor networks.
- Energy Consumption: Many sensors rely on battery power, making energy efficiency a priority in network design. We focus on developing energy-efficient solutions that extend the lifespan of sensor networks, including low power sensor networks.
2.1.2. Digital Footprint Tracking
Digital footprint tracking refers to the process of collecting and analyzing data generated by users' online activities. This information can be used for various purposes, including targeted advertising, user experience enhancement, and security monitoring.
- Types of Digital Footprints: Â
- Active Footprints: Data users intentionally share, such as social media posts, comments, and online purchases.
- Passive Footprints: Data collected without user consent, such as browsing history, location data, and cookies.
- Benefits: Â
- Personalized Experiences: Businesses can tailor their services and advertisements based on user behavior, leading to higher engagement rates. Our AI-driven analytics can help companies create highly personalized marketing strategies that resonate with their target audience.
- Enhanced Security: Tracking digital footprints can help identify suspicious activities and protect against fraud. We implement robust security measures to ensure that user data is protected while still providing valuable insights.
- Market Insights: Companies can analyze user behavior trends to inform product development and marketing strategies. Our expertise in data analytics allows clients to derive actionable insights from their digital footprint data.
- Concerns: Â
- Privacy Issues: Users may not be aware of the extent of data collection, raising concerns about consent and data ownership. Rapid Innovation emphasizes transparency and user consent in all data collection practices.
- Data Misuse: There is a risk that collected data could be used for malicious purposes, such as identity theft or unauthorized surveillance. We advocate for ethical data usage and implement strict compliance measures.
- Regulatory Compliance: Organizations must navigate complex regulations regarding data protection, such as GDPR and CCPA. Our consulting services help clients ensure compliance with these regulations, minimizing legal risks.
2.1.3. User Interaction Logging
User interaction logging involves recording and analyzing how users interact with digital platforms, such as websites and applications. This practice is essential for improving user experience and optimizing digital products.
- Key Metrics: Â
- Click Tracking: Monitoring where users click on a page to understand their interests and navigation patterns.
- Session Duration: Analyzing how long users spend on a site or app, which can indicate engagement levels.
- Conversion Rates: Measuring the percentage of users who complete desired actions, such as making a purchase or signing up for a newsletter.
- Applications: Â
- User Experience Design: Insights from interaction logs can inform design decisions, leading to more intuitive interfaces. Our team leverages AI to analyze user behavior and enhance the overall user experience.
- A/B Testing: Organizations can test different versions of a webpage or app to determine which performs better based on user interactions. We provide comprehensive A/B testing solutions that yield data-driven results.
- Customer Support: Logging interactions can help identify common user issues, allowing for improved support resources and FAQs. Our analytics tools can streamline customer support processes, leading to higher satisfaction rates.
- Challenges: Â
- Data Overload: Collecting extensive interaction data can lead to analysis paralysis if not managed effectively. Rapid Innovation offers data management solutions that simplify the analysis process.
- User Privacy: Organizations must balance the need for data with respect for user privacy, ensuring compliance with regulations. We prioritize user privacy in all our solutions, fostering trust and transparency.
- Interpretation of Data: Accurately interpreting user interaction data requires expertise and can be subjective, leading to potential biases in decision-making. Our team of experts ensures that data interpretation is objective and aligned with business goals. 2.1.4. Real-time Monitoring Systems
Real-time monitoring systems are essential for organizations that require immediate insights into their operations, performance, and security. These systems enable businesses to track various metrics and events as they happen, allowing for quick decision-making and response.
- Continuous data collection: Real-time monitoring systems gather data from various sources, including sensors, applications, and user interactions, ensuring that information is always up-to-date. This capability is crucial for organizations looking to leverage AI-driven insights for operational efficiency, such as real time network monitoring and real time monitoring systems.
- Instant alerts: These systems can trigger alerts based on predefined thresholds or anomalies, enabling teams to respond promptly to issues before they escalate. By integrating AI algorithms, Rapid Innovation can enhance the accuracy of these alerts, reducing false positives and ensuring that critical issues are prioritized, particularly in real time security monitoring.
- Enhanced visibility: By providing a comprehensive view of operations, real-time monitoring helps organizations identify trends, bottlenecks, and areas for improvement. This visibility is vital for businesses aiming to optimize processes and improve ROI through data-driven strategies, including real time network performance monitoring.
- Improved customer experience: Businesses can monitor user interactions in real-time, allowing them to address customer concerns immediately and enhance overall satisfaction. Rapid Innovation's expertise in AI can help tailor these interactions, leading to personalized experiences that drive customer loyalty, as seen in real time active directory monitoring.
- Integration with other systems: Real-time monitoring can be integrated with analytics and reporting tools, providing a holistic view of performance and facilitating data-driven decision-making. This integration is essential for organizations looking to harness the full potential of their data assets, including real time monitoring solutions and mysql real time monitoring. For more insights on how to revolutionize data processing.
2.2. Data Processing Infrastructure
Data processing infrastructure refers to the underlying framework that supports the collection, storage, and analysis of data. A robust data processing infrastructure is crucial for organizations looking to leverage big data and analytics for strategic advantage.
- Scalability: A well-designed infrastructure can scale to accommodate growing data volumes, ensuring that organizations can handle increased workloads without compromising performance. Rapid Innovation can help design scalable solutions that adapt to evolving business needs.
- Data storage solutions: Various storage options, such as cloud storage, on-premises databases, and data lakes, can be utilized to store structured and unstructured data efficiently. Our team can recommend the best storage solutions tailored to your specific requirements.
- Data integration: Effective data processing infrastructure allows for seamless integration of data from multiple sources, ensuring that organizations have a unified view of their information. Rapid Innovation specializes in creating integrated systems that enhance data accessibility and usability.
- Processing capabilities: The infrastructure should support various processing methods, including batch processing and real-time processing, to cater to different analytical needs. Our expertise in AI and blockchain can optimize these processes for better performance and reliability.
- Security and compliance: A strong data processing infrastructure must prioritize data security and adhere to regulatory requirements, protecting sensitive information from breaches and ensuring compliance with laws. Rapid Innovation emphasizes building secure infrastructures that safeguard your data assets.
2.2.1. Stream Processing
Stream processing is a method of handling continuous data streams in real-time, allowing organizations to analyze and act on data as it flows in. This approach is particularly valuable for applications that require immediate insights and responses.
- Low latency: Stream processing enables organizations to process data with minimal delay, making it ideal for time-sensitive applications such as fraud detection and real-time analytics. Rapid Innovation can implement low-latency solutions that enhance operational responsiveness.
- Event-driven architecture: Stream processing systems are often built on event-driven architectures, allowing them to react to incoming data events dynamically. Our expertise in AI can enhance these architectures, enabling smarter event handling and decision-making.
- Scalability: Stream processing frameworks can scale horizontally, accommodating increasing data volumes and ensuring consistent performance. Rapid Innovation can design scalable stream processing solutions that grow with your business.
- Complex event processing: Advanced stream processing systems can identify patterns and correlations within data streams, enabling organizations to derive actionable insights from complex datasets. Our team can leverage AI techniques to enhance these capabilities, driving better business outcomes.
- Integration with other technologies: Stream processing can be integrated with machine learning models and data storage solutions, enhancing the overall data processing capabilities of an organization. Rapid Innovation excels in creating integrated ecosystems that maximize the value of your data, including linux real time network monitor and real time network speed monitor.
2.2.2. Batch Processing
Batch processing is a method of executing a series of jobs or tasks on a computer without manual intervention. This approach is particularly useful in scenarios where large volumes of data need to be processed efficiently, such as in batch processes and batch productions.
- Characteristics of Batch Processing: Â
- Jobs are collected and processed in groups or batches, often referred to as a batch system.
- Processing occurs at scheduled intervals, rather than in real-time, which is common in micro batching.
- It is often used for tasks that do not require immediate results, such as payroll systems or data analysis.
- Advantages: Â
- High efficiency in resource utilization, as multiple jobs can be processed simultaneously, making it ideal for batch processing systems.
- Reduced operational costs due to less need for human intervention, which is a key feature of batching software.
- Ideal for processing large datasets, making it suitable for data mining and analytics, including iot batch job scenarios.
- Disadvantages: Â
- Lack of real-time processing can lead to delays in data availability.
- Errors in one job can affect the entire batch, complicating troubleshooting.
- Requires careful planning and scheduling to optimize performance, particularly in batch management.
Batch processing is widely used in various industries, including finance, healthcare, and telecommunications, where large-scale data processing is essential. Rapid Innovation can assist clients in implementing efficient batch processing systems that enhance productivity and reduce costs, ultimately leading to greater ROI. For example, clients can learn how to execute batch job raspberry pi for specific applications or utilize iot execute batch job strategies. Additionally, understanding the principles of pattern recognition in machine learning can further enhance the effectiveness of batch processing systems.
2.2.3. Hybrid Approaches
Hybrid approaches combine different methodologies to leverage the strengths of each while mitigating their weaknesses. In the context of data processing and machine learning, hybrid approaches often integrate batch processing and real-time processing.
- Key Features: Â
- Utilizes both batch and real-time data processing techniques.
- Allows for flexibility in handling various types of data workloads.
- Can adapt to changing requirements and data availability.
- Benefits: Â
- Enhanced performance by processing data in real-time while also handling large batches.
- Improved decision-making capabilities due to timely insights from real-time data.
- Scalability, as hybrid systems can grow and adapt to increasing data volumes.
- Applications: Â
- Used in industries like e-commerce for real-time inventory management and batch sales reporting.
- Financial services utilize hybrid approaches for fraud detection, combining real-time transaction monitoring with historical data analysis.
Hybrid approaches are becoming increasingly popular as organizations seek to balance the need for immediate insights with the ability to analyze large datasets. Rapid Innovation can guide clients in developing hybrid systems that optimize data processing, leading to improved operational efficiency and higher returns on investment.
2.3. Pattern Recognition Engines
Pattern recognition engines are systems designed to identify patterns and regularities in data. These engines are crucial in various applications, including image recognition, speech recognition, and data classification.
- Core Components: Â
- Feature extraction: Identifying relevant features from raw data to facilitate pattern recognition.
- Classification: Assigning labels to data based on identified patterns.
- Learning algorithms: Utilizing machine learning techniques to improve accuracy over time.
- Types of Pattern Recognition: Â
- Supervised learning: The model is trained on labeled data, learning to classify new data based on this training.
- Unsupervised learning: The model identifies patterns in data without prior labeling, useful for clustering and anomaly detection.
- Semi-supervised learning: Combines both labeled and unlabeled data to improve learning efficiency.
- Applications: Â
- Image and video analysis for facial recognition and object detection.
- Natural language processing for sentiment analysis and language translation.
- Medical diagnosis through the analysis of patient data and imaging.
Pattern recognition engines are integral to the development of artificial intelligence and machine learning applications. They enable systems to learn from data and make predictions or decisions based on identified patterns. Rapid Innovation specializes in creating advanced pattern recognition solutions that empower businesses to harness their data effectively, driving innovation and maximizing ROI.
2.3.1. Machine Learning Models
Machine learning models are algorithms that enable computers to learn from data and make predictions or decisions without being explicitly programmed. These models are essential in various applications, from finance to healthcare, and they can be categorized into several types:
- Supervised Learning: This involves training a model on labeled data, where the outcome is known. Common algorithms include: Â
- Linear Regression
- Decision Trees
- Support Vector Machines (SVM)
- Scikit learn linear regression
- Logistic regression model machine learning
- Unsupervised Learning: In this approach, the model works with unlabeled data to find patterns or groupings. Key techniques include: Â
- Clustering (e.g., K-Means, Hierarchical Clustering)
- Dimensionality Reduction (e.g., PCA)
- Restricted Boltzmann machine
- Reinforcement Learning: This type of learning involves training models to make sequences of decisions by rewarding desired actions. It is widely used in robotics and game playing.
At Rapid Innovation, we leverage these machine learning models, including classification random forest and random forest scikit learn, to help clients optimize their operations and enhance decision-making processes. For instance, in the finance sector, we have implemented supervised learning algorithms to predict stock prices, enabling clients to make informed investment decisions that significantly improve their ROI.
Machine learning models are evaluated based on their performance metrics, such as accuracy, precision, recall, and F1 score. The choice of model depends on the specific problem, the nature of the data, and the desired outcome. Techniques like ensemble learning in machine learning and ensemble machine learning are also utilized to improve model performance. For more information on the types of artificial neural networks.
2.3.2. Deep Learning Networks
Deep learning networks are a subset of machine learning that utilize neural networks with many layers (hence "deep"). These networks are particularly effective for complex tasks such as image and speech recognition. Key components include:
- Neural Networks: Composed of interconnected nodes (neurons) that process input data. Each layer transforms the data, allowing the model to learn hierarchical features.
- Convolutional Neural Networks (CNNs): Primarily used for image processing, CNNs excel at recognizing patterns and features in visual data. They use convolutional layers to automatically detect features like edges and textures. Convolutional neural networks are a key part of deep learning neural networks.
- Recurrent Neural Networks (RNNs): Designed for sequential data, RNNs are effective in tasks like natural language processing and time series analysis. They maintain a memory of previous inputs, allowing them to understand context.
- Training and Optimization: Deep learning models require large datasets and significant computational power. Techniques like dropout, batch normalization, and various optimization algorithms (e.g., Adam, SGD) are used to improve performance and prevent overfitting.
At Rapid Innovation, we harness deep learning networks, including neural network machine learning and deep learning models, to drive innovation in sectors such as healthcare, where we have developed models that analyze medical images to assist in early disease detection. This not only enhances patient outcomes but also reduces costs, thereby delivering greater ROI for healthcare providers.
Deep learning has revolutionized fields such as computer vision, natural language processing, and autonomous systems, making it a critical area of research and application.
2.3.3. Statistical Analysis Tools
Statistical analysis tools are essential for interpreting data and making informed decisions based on statistical methods. These tools help in understanding data distributions, relationships, and trends. Key tools and techniques include:
- Descriptive Statistics: Summarizes data through measures such as mean, median, mode, variance, and standard deviation. These metrics provide a quick overview of the data's characteristics.
- Inferential Statistics: Allows for making predictions or inferences about a population based on a sample. Techniques include hypothesis testing, confidence intervals, and regression analysis, including elasticnet regression and xgboost regression.
- Data Visualization Tools: Tools like Tableau, R, and Python libraries (e.g., Matplotlib, Seaborn) help in visualizing data through graphs and charts, making it easier to identify patterns and insights.
- Statistical Software: Programs like SPSS, SAS, and R provide comprehensive environments for performing complex statistical analyses. They offer a range of functions for data manipulation, analysis, and visualization.
At Rapid Innovation, we utilize statistical analysis tools to empower our clients with actionable insights derived from their data. For example, in the retail sector, we analyze customer behavior patterns to optimize inventory management, leading to increased sales and improved ROI.
Statistical analysis tools are crucial in various fields, including social sciences, healthcare, and business, enabling professionals to derive actionable insights from data.
2.4. Decision Support Systems
Decision Support Systems (DSS), including clinical decision support systems, are computer-based information systems that support business or organizational decision-making activities. They help in analyzing data and presenting actionable information to assist in making informed decisions. DSS can be used in various fields, including healthcare, finance, and supply chain management. They integrate data from multiple sources, providing a comprehensive view of the situation. DSS can utilize various analytical models to evaluate different scenarios, enhancing the decision-making process by providing timely and relevant information. Users can interact with the system to explore different options and outcomes.
At Rapid Innovation, we leverage advanced AI algorithms to enhance the capabilities of DSS, enabling our clients to achieve greater ROI through data-driven insights. For instance, in the finance sector, our AI-enhanced DSS can analyze market trends and predict future movements, allowing organizations to make informed investment decisions that maximize returns.
2.4.1. Alert Mechanisms
Alert mechanisms are critical components of Decision Support Systems. They notify users of significant changes or events that require immediate attention. These alerts can be automated and tailored to specific criteria, ensuring that decision-makers are informed promptly.
- Alerts can be triggered by predefined thresholds, such as sales dropping below a certain level or inventory reaching a critical low.
- They can be delivered through various channels, including email, SMS, or in-app notifications.
- Customizable settings allow users to define what constitutes an alert-worthy event.
- Alert mechanisms help in proactive decision-making, allowing organizations to respond quickly to potential issues.
Rapid Innovation can implement blockchain technology to enhance the security and integrity of alert mechanisms, ensuring that critical notifications are tamper-proof and reliable.
2.4.2. Reporting Frameworks
Reporting frameworks within Decision Support Systems provide structured ways to present data and insights. These frameworks help in generating reports that are easy to understand and actionable. They play a vital role in tracking performance, compliance, and strategic goals.
- Reporting frameworks can include dashboards, scorecards, and detailed reports.
- They often feature visualizations like charts and graphs to make data interpretation easier.
- Users can customize reports to focus on specific metrics or time periods.
- Automated reporting can save time and ensure consistency in data presentation.
By integrating AI-driven analytics into reporting frameworks, Rapid Innovation enables clients to uncover hidden patterns and trends, leading to more informed strategic decisions and improved business outcomes.
Incorporating effective alert mechanisms and reporting frameworks into Decision Support Systems, including clinical support systems and clinical decision systems, enhances their utility, making them indispensable tools for modern organizations. Rapid Innovation is committed to helping clients harness the power of AI and blockchain to achieve their business goals efficiently and effectively. If you're looking to enhance your DSS capabilities, consider hiring Action Transformer Developers from Rapid Innovation.
2.4.3. Visualization Tools
Visualization tools are essential in data analysis and interpretation, allowing users to transform complex data sets into understandable visual formats. These tools help in identifying patterns, trends, and outliers, making it easier for stakeholders to make informed decisions.
- Types of Visualization Tools: Â
- Business Intelligence Tools: Tools like Tableau and Power BI enable users to create interactive dashboards and reports, facilitating real-time insights that can drive strategic decisions. Tableau visualization and heat map in Tableau are popular features that enhance data representation.
- Statistical Visualization: Software such as R and Python libraries (like Matplotlib and Seaborn) are used for statistical data visualization, allowing for in-depth analysis of data distributions and relationships. Data visualization charts and data visualization graphs are commonly utilized in this category.
- Geospatial Visualization: Tools like ArcGIS and Google Maps help visualize data in a geographical context, which is particularly useful for location-based analytics.
- Benefits of Visualization Tools: Â
- Enhanced Understanding: Visual representations make it easier to grasp complex data, enabling stakeholders to quickly comprehend key insights.
- Improved Communication: Visuals can convey information more effectively than text or numbers alone, ensuring that all team members are aligned on data-driven decisions.
- Faster Decision-Making: Quick insights from visual data can lead to timely decisions, ultimately improving operational efficiency and responsiveness to market changes.
- Popular Visualization Tools: Â
- Tableau: Known for its user-friendly interface and powerful analytics capabilities, Tableau allows organizations to create compelling visual narratives from their data. Tableau visualisations and tableau viz are key features that enhance user experience.
- Power BI: Integrates well with Microsoft products and offers robust data modeling features, making it a preferred choice for businesses already using the Microsoft ecosystem. Heat map power bi is a notable visualization option.
- D3.js: A JavaScript library for producing dynamic, interactive data visualizations in web browsers, enabling custom visual solutions tailored to specific business needs.
- Considerations When Choosing a Visualization Tool: Â
- User Skill Level: Some tools require advanced technical skills, while others are more accessible, which can impact the speed of adoption within an organization. Data viz software and data visualization software vary in complexity.
- Integration Capabilities: Ensure the tool can integrate with existing data sources, as seamless data flow is critical for accurate and timely analysis.
- Cost: Evaluate the pricing model, as some tools may have hidden costs, which can affect the overall return on investment. Free data visualization tools and best data visualization tools are worth considering for budget-conscious organizations.
At Rapid Innovation, we leverage these visualization tools, including data visualization tools, database visualizer, and data visualization examples, to help our clients achieve their business goals efficiently and effectively. By integrating advanced analytics into their operations, we enable organizations to unlock greater ROI and drive sustainable growth. Additionally, our expertise in AI as a Service allows us to enhance these visualization capabilities further.
3.1.1. Supervised Learning
Supervised learning is a type of machine learning where the model is trained on a labeled dataset. This means that each training example is paired with an output label, allowing the algorithm to learn the relationship between the input data and the corresponding output. The process involves feeding the model a set of input-output pairs, where the model makes predictions based on the input data and is then corrected by comparing its predictions to the actual output. Common algorithms used in supervised learning include linear regression, logistic regression, decision trees, and support vector machines.
At Rapid Innovation, we leverage supervised learning to help our clients achieve significant business outcomes. For instance, in the healthcare sector, we have developed predictive models that assist in medical diagnosis, enabling healthcare providers to make informed decisions quickly, thereby improving patient outcomes and reducing costs. Our work in machine learning applications has led to innovative solutions in various fields, including machine learning for drug discovery.
Applications of supervised learning are vast and include:
- Image classification: Identifying objects within images.
- Spam detection: Classifying emails as spam or not spam.
- Medical diagnosis: Predicting diseases based on patient data.
Supervised learning is particularly effective when there is a large amount of labeled data available, as it allows the model to learn from examples and improve its accuracy over time. This is especially relevant in designing machine learning systems that can adapt to specific tasks.
3.1.2. Unsupervised Learning
Unsupervised learning, in contrast to supervised learning, deals with unlabeled data. The model is tasked with identifying patterns and structures within the data without any explicit guidance on what to look for. The primary goal is to explore the underlying structure of the data. Common techniques include clustering, dimensionality reduction, and association rule learning. Algorithms such as k-means clustering, hierarchical clustering, and principal component analysis (PCA) are frequently used.
At Rapid Innovation, we utilize unsupervised learning to help businesses uncover hidden insights from their data. For example, we have implemented customer segmentation solutions that allow retailers to tailor their marketing strategies based on purchasing behavior, leading to increased customer engagement and higher sales. Our expertise in manifold learning has also contributed to advancements in this area.
Key applications of unsupervised learning include:
- Customer segmentation: Grouping customers based on purchasing behavior.
- Anomaly detection: Identifying unusual patterns that do not conform to expected behavior.
- Market basket analysis: Discovering associations between products purchased together.
Unsupervised learning is particularly useful in scenarios where labeled data is scarce or expensive to obtain, allowing businesses to gain insights from their data without the need for extensive labeling. This is crucial for developing machine learning systems that can operate effectively in real-world environments.
3.1.3. Reinforcement Learning
Reinforcement learning (RL) is a type of machine learning where an agent learns to make decisions by interacting with an environment. The agent receives feedback in the form of rewards or penalties based on its actions, allowing it to learn optimal strategies over time. The learning process involves exploration (trying new actions) and exploitation (choosing known actions that yield high rewards). Key components of reinforcement learning include the agent, environment, actions, rewards, and states. Popular algorithms in reinforcement learning include Q-learning, deep Q-networks (DQN), and policy gradients.
At Rapid Innovation, we harness the power of reinforcement learning to develop advanced solutions for various industries. For instance, in the field of autonomous vehicles, we have created systems that enable self-driving cars to navigate complex environments safely and efficiently, significantly enhancing transportation safety and reducing operational costs. Our work in deep learning applications has been instrumental in achieving these advancements.
Applications of reinforcement learning are diverse and include:
- Game playing: Training agents to play games like chess or Go at superhuman levels.
- Robotics: Teaching robots to perform tasks through trial and error.
- Autonomous vehicles: Enabling self-driving cars to navigate complex environments.
Reinforcement learning is particularly powerful in situations where the optimal solution is not known in advance, allowing the agent to learn from its experiences and adapt to changing conditions. By partnering with Rapid Innovation, businesses can leverage these advanced machine learning techniques, including deep learning computer vision, to drive innovation and achieve greater ROI.
3.2. Natural Language Processing
Natural Language Processing (NLP) is a branch of artificial intelligence that focuses on the interaction between computers and humans through natural language. The goal of NLP is to enable machines to understand, interpret, and respond to human language in a valuable way.
- Key components of NLP include: Â
- Tokenization: Breaking down text into smaller units, such as words or phrases.
- Part-of-Speech Tagging: Identifying the grammatical parts of speech in a sentence.
- Named Entity Recognition: Detecting and classifying key entities in text, such as names, dates, and locations.
- Sentiment Analysis: Determining the emotional tone behind a series of words, often used in social media monitoring.
- Applications of NLP are vast and include: Â
- Chatbots and Virtual Assistants: Tools like Siri and Alexa use NLP to understand user queries and provide relevant responses. Rapid Innovation can develop customized chatbots that enhance customer engagement and streamline support processes, leading to improved customer satisfaction and retention.
- Text Analytics: Businesses analyze customer feedback and reviews to gauge sentiment and improve services. By leveraging NLP, Rapid Innovation helps clients extract actionable insights from large volumes of text data, enabling data-driven decision-making.
- Machine Translation: Services like Google Translate utilize NLP to convert text from one language to another. Rapid Innovation can implement advanced translation solutions that facilitate global communication and expand market reach.
NLP is continually evolving, with advancements in deep learning and neural networks enhancing its capabilities. For instance, models like BERT and GPT-3 have significantly improved the understanding of context and nuance in language. By integrating these cutting-edge technologies, Rapid Innovation empowers clients to harness the full potential of NLP for their business objectives.
Natural language processing techniques, such as natural language understanding and natural language generation, are also critical in developing sophisticated NLP applications. Furthermore, the integration of natural language programming and language processing AI is paving the way for more intuitive human-computer interactions. As the field of NLP continues to grow, the importance of defining NLP and understanding its various models becomes increasingly relevant for practitioners and researchers alike. Additionally, for those interested in enhancing their NLP capabilities, Rapid Innovation offers comprehensive computer vision software development services that can be integrated with NLP solutions.
3.3. Computer Vision
Computer Vision is a field of artificial intelligence that enables machines to interpret and make decisions based on visual data. It involves the extraction, analysis, and understanding of information from images and videos.
- Core techniques in computer vision include: Â
- Image Classification: Assigning a label to an image based on its content.
- Object Detection: Identifying and locating objects within an image.
- Image Segmentation: Dividing an image into segments to simplify its analysis.
- Facial Recognition: Identifying or verifying a person from a digital image or video frame.
- Real-world applications of computer vision are extensive: Â
- Autonomous Vehicles: Self-driving cars use computer vision to navigate and understand their surroundings. Rapid Innovation can assist automotive companies in developing robust computer vision systems that enhance safety and efficiency.
- Healthcare: Medical imaging analysis helps in diagnosing diseases by interpreting X-rays, MRIs, and CT scans. By implementing advanced computer vision solutions, Rapid Innovation aids healthcare providers in improving diagnostic accuracy and patient outcomes.
- Retail: Stores use computer vision for inventory management and customer behavior analysis. Rapid Innovation can create tailored solutions that optimize inventory processes and enhance the shopping experience.
The integration of computer vision with other technologies, such as augmented reality (AR) and machine learning, is driving innovation across various sectors. As the technology matures, its accuracy and efficiency continue to improve, making it an essential tool in modern applications.
3.4. Time Series Analysis
Time Series Analysis is a statistical technique used to analyze time-ordered data points. It helps in understanding underlying patterns, trends, and seasonal variations over time, making it crucial for forecasting and decision-making.
- Fundamental concepts in time series analysis include: Â
- Trend Analysis: Identifying long-term movements in data.
- Seasonality: Recognizing periodic fluctuations that occur at regular intervals.
- Cyclical Patterns: Understanding longer-term fluctuations that are not fixed in frequency.
- Autocorrelation: Measuring how current values in a series relate to past values.
- Common applications of time series analysis are: Â
- Financial Markets: Analyzing stock prices and economic indicators to predict future movements. Rapid Innovation can develop predictive models that help clients make informed investment decisions and manage risks effectively.
- Sales Forecasting: Businesses use historical sales data to project future sales and manage inventory. By employing time series analysis, Rapid Innovation enables clients to optimize their supply chain and improve operational efficiency.
- Weather Prediction: Meteorologists analyze historical weather data to forecast future conditions. Rapid Innovation can assist organizations in leveraging time series data for better resource planning and risk management.
Time series analysis employs various models, such as ARIMA (AutoRegressive Integrated Moving Average) and Exponential Smoothing, to make accurate predictions. The rise of big data and advanced analytics tools has further enhanced the ability to analyze complex time series data, leading to more informed decision-making across industries. Rapid Innovation is committed to helping clients unlock the value of their data through sophisticated time series analysis techniques.
3.5. Anomaly Detection Systems
Anomaly detection systems are crucial in identifying unusual patterns or behaviors in data that deviate from the norm. These systems are widely used across various industries, including finance, healthcare, and cybersecurity. The primary goal is to detect anomalies that could indicate fraud, network intrusions, or system failures.
- Definition: Anomaly detection refers to the process of identifying rare items, events, or observations that raise suspicions by differing significantly from the majority of the data. This includes techniques such as anomaly based detection and statistical anomaly detection in network security.
- Techniques: Common techniques include statistical methods, machine learning algorithms, and deep learning approaches. Each method has its strengths and weaknesses, depending on the context and data type. For instance, network anomaly detection using machine learning can be particularly effective in cybersecurity.
- Applications: Â
- Fraud detection in banking and finance
- Intrusion detection in cybersecurity, including anomaly based intrusion detection systems
- Fault detection in manufacturing processes
- Monitoring patient health in healthcare systems, where anomaly based detection can be critical.
- Challenges: Anomaly detection systems face challenges such as high false positive rates, the need for large datasets, and the difficulty of defining what constitutes an anomaly. For example, network anomalies meaning can vary significantly across different contexts.
- Importance: These systems help organizations mitigate risks, enhance security, and improve operational efficiency by providing timely alerts about potential issues, such as anomalous activity detected on the internal network.
4. Benefits and Advantages
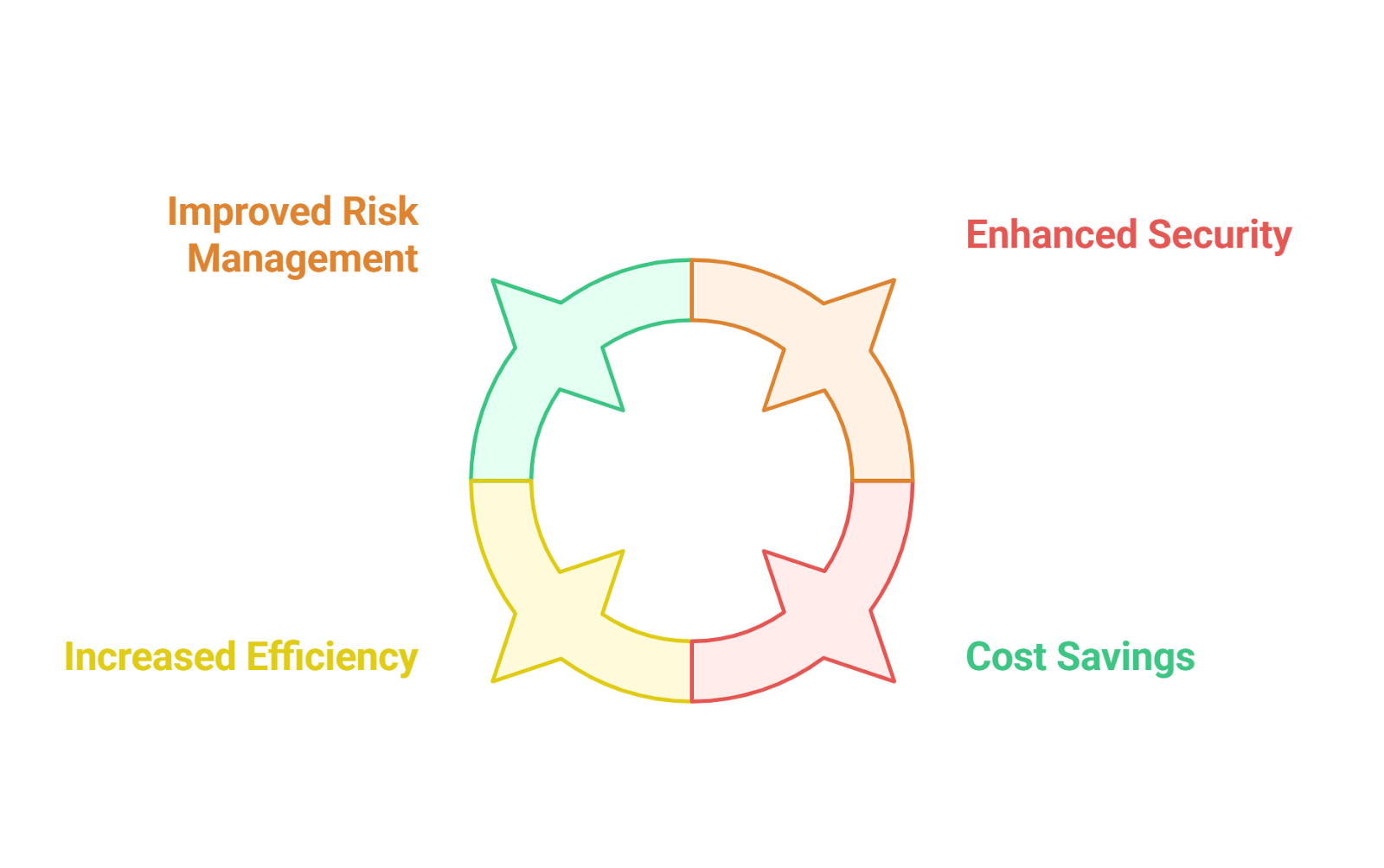
‍
The implementation of advanced technologies, including anomaly detection systems, offers numerous benefits and advantages to organizations. These benefits can significantly impact decision-making processes, operational efficiency, and overall business performance.
- Improved Risk Management: By identifying anomalies early, organizations can take proactive measures to mitigate risks before they escalate, particularly in areas like cyber security anomaly detection.
- Cost Savings: Early detection of issues can lead to significant cost savings by preventing losses associated with fraud, downtime, or system failures, which can be addressed through anomaly detection solutions.
- Enhanced Security: Anomaly detection systems bolster security measures by identifying potential threats in real-time, allowing for immediate response, especially in network behaviour anomaly detection.
- Increased Efficiency: Automating the detection of anomalies reduces the need for manual monitoring, freeing up resources for other critical tasks, such as managing active directory anomaly detection.
4.1. Enhanced Decision Making
Enhanced decision-making is one of the most significant advantages of implementing anomaly detection systems. By providing accurate
4.2. Predictive Capabilities
Predictive capabilities refer to the ability of a system or model to forecast future events based on historical data and trends. This is particularly important in various fields such as finance, healthcare, and supply chain management.
- Utilizes advanced analytics and machine learning algorithms to analyze large datasets. Â
- Identifies patterns and correlations that may not be immediately apparent. Â
- Enables organizations to make informed decisions by anticipating future outcomes. Â
- Enhances customer experience by predicting needs and preferences. Â
- Supports proactive strategies rather than reactive measures. Â
For example, in retail, predictive analytics can forecast inventory needs, helping businesses avoid stockouts or overstock situations. In healthcare, predictive modeling can identify patients at risk of developing certain conditions, allowing for early intervention. According to a report by McKinsey, companies that leverage predictive data analytics can improve their decision-making processes significantly. At Rapid Innovation, we harness these predictive capabilities, including prescriptive analytics, to empower our clients, enabling them to achieve greater ROI through data-driven insights and strategic foresight. Our expertise in power bi predictive analytics allows us to provide tailored solutions, such as predictive analytics in power bi and predictive analytics with power bi, ensuring our clients can effectively utilize their data. Additionally, our services include AI project estimation to help organizations accurately assess the resources and time required for their predictive analytics initiatives. Furthermore, we explore predictive analytics in finance to anticipate market trends and enhance financial decision-making.
4.3. Risk Mitigation
Risk mitigation involves strategies and actions taken to reduce the potential impact of risks on an organization. Effective risk management is crucial for maintaining stability and ensuring long-term success.
- Identifies potential risks through comprehensive assessments and analyses. Â
- Implements controls and measures to minimize the likelihood of risks occurring. Â
- Develops contingency plans to address risks if they materialize. Â
- Enhances compliance with regulations and standards, reducing legal risks. Â
- Fosters a culture of risk awareness among employees. Â
For instance, in finance, risk mitigation strategies may include diversifying investments to spread risk. In project management, conducting regular risk assessments can help identify potential issues early, allowing teams to address them proactively. According to the Project Management Institute, organizations that prioritize risk management can achieve better project outcomes. Rapid Innovation assists clients in developing robust risk mitigation frameworks, ensuring they can navigate uncertainties effectively and maintain operational integrity.
4.4. Resource Optimization
Resource optimization refers to the efficient and effective use of an organization’s resources, including time, money, and personnel. This is essential for maximizing productivity and minimizing waste.
- Analyzes resource allocation to identify areas for improvement. Â
- Implements technology solutions to streamline processes and reduce costs. Â
- Encourages collaboration and communication among teams to enhance efficiency. Â
- Monitors performance metrics to ensure resources are being utilized effectively. Â
- Adapts strategies based on changing market conditions and organizational needs. Â
For example, in manufacturing, resource optimization can lead to reduced production costs and improved product quality. In IT, optimizing server usage can enhance performance while lowering energy consumption. According to a study by Deloitte, organizations that focus on resource optimization can achieve significant cost savings and improved operational efficiency. At Rapid Innovation, we leverage our expertise in AI and blockchain to help clients optimize their resources, driving efficiency and maximizing their return on investment.
4.5. Improved Customer Experience
Enhancing customer experience is crucial for businesses aiming to build loyalty and drive sales. A positive customer experience can lead to repeat business and referrals, which are vital for growth. Here are some key aspects of how improved customer experience can be achieved:
- Personalization: Tailoring services and products to meet individual customer preferences can significantly enhance satisfaction. Utilizing advanced data analytics and AI algorithms allows businesses to understand customer behavior and preferences better, enabling them to deliver personalized experiences that resonate with their audience. This is particularly important in how to improve customer experience and how to increase customer experience.
- Omnichannel Support: Providing seamless support across various channels—such as social media, email, and live chat—ensures that customers can reach out in their preferred way. This flexibility can lead to quicker resolutions and higher satisfaction rates, as AI-driven chatbots can assist in managing inquiries efficiently across platforms. This approach is essential for improving customer service and how can we improve customer service.
- Feedback Mechanisms: Actively seeking customer feedback through surveys or reviews helps businesses identify areas for improvement. Implementing changes based on this feedback demonstrates that a company values its customers' opinions, fostering a sense of community and loyalty. This is a key aspect of customer experience improvement and how to improve customer satisfaction.
- Speed and Efficiency: Streamlining processes to reduce wait times and improve service delivery can greatly enhance the customer experience. Quick response times and efficient service, often powered by AI-driven solutions, can lead to higher customer satisfaction and retention. This is crucial for how to improve customer care and how to improve client experience.
- User-Friendly Interfaces: Ensuring that websites and applications are easy to navigate can significantly improve the customer experience. A well-designed interface, enhanced by AI insights, can reduce frustration and encourage customers to engage more with the brand. This is part of enhancing consumer experience and better customer experience.
4.6. Operational Efficiency
Operational efficiency refers to the ability of a business to deliver products or services in the most cost-effective manner without compromising quality. Improving operational efficiency can lead to significant cost savings and better resource management. Here are some strategies to enhance operational efficiency:
- Process Automation: Implementing automation tools can streamline repetitive tasks, reducing the time and effort required for manual processes. This can lead to increased productivity and lower operational costs, allowing teams to focus on higher-value activities.
- Lean Management: Adopting lean principles helps eliminate waste and optimize processes. By focusing on value-added activities, businesses can improve efficiency and reduce costs, ultimately enhancing their bottom line.
- Employee Training: Investing in employee training ensures that staff are well-equipped to perform their tasks efficiently. Skilled employees can work faster and make fewer mistakes, contributing to overall operational efficiency.
- Performance Metrics: Establishing key performance indicators (KPIs) allows businesses to measure efficiency and identify areas for improvement. Regularly reviewing these metrics can help in making informed decisions that drive operational excellence.
- Supply Chain Optimization: Streamlining the supply chain can reduce delays and lower costs. Collaborating with suppliers and using technology to track inventory can enhance overall efficiency, ensuring that resources are utilized effectively.
4.7. Compliance and Security
In today's digital landscape, compliance and security are paramount for businesses. Ensuring that operations adhere to regulations and protecting sensitive data are critical for maintaining trust and avoiding legal issues. Here are some essential aspects of compliance and security:
- Regulatory Compliance: Staying updated with industry regulations is crucial. Non-compliance can lead to hefty fines and damage to reputation. Regular audits and compliance training can help ensure adherence to laws, safeguarding the organization against potential risks.
- Data Protection: Implementing robust data protection measures, such as encryption and secure access controls, is essential for safeguarding sensitive information. This helps prevent data breaches and builds customer trust, which is vital for long-term success.
- Cybersecurity Measures: Investing in cybersecurity solutions, such as firewalls and intrusion detection systems, can protect against cyber threats. Regularly updating software and conducting security assessments are also vital to maintaining a secure operational environment.
- Employee Awareness: Training employees on compliance and security best practices can significantly reduce risks. Educated staff are less likely to fall victim to phishing attacks or inadvertently compromise data, creating a more secure workplace.
- Incident Response Plans: Having a well-defined incident response plan ensures that businesses can quickly address security breaches or compliance issues. This preparedness can minimize damage and restore operations swiftly, reinforcing the organization's commitment to security and compliance.
At Rapid Innovation, we leverage our expertise in AI and Blockchain to help businesses implement these strategies effectively, ensuring that they achieve their goals while maximizing ROI. By integrating advanced technologies, we empower our clients to enhance customer experiences, improve operational efficiency, and maintain robust compliance and security measures, including how to improve client retention and enhance client experience. For more insights, check out learning from real-world AI implementations.
5. Industry-Specific Use Cases
5.1. Financial Services
The financial services industry is rapidly evolving, driven by technological advancements and changing consumer expectations. Financial institutions are increasingly leveraging innovative solutions to enhance their operations, improve customer experiences, and mitigate risks. One of the most significant areas of focus is fraud detection, which has become a critical component in safeguarding assets and maintaining trust.
5.1.1. Fraud Detection
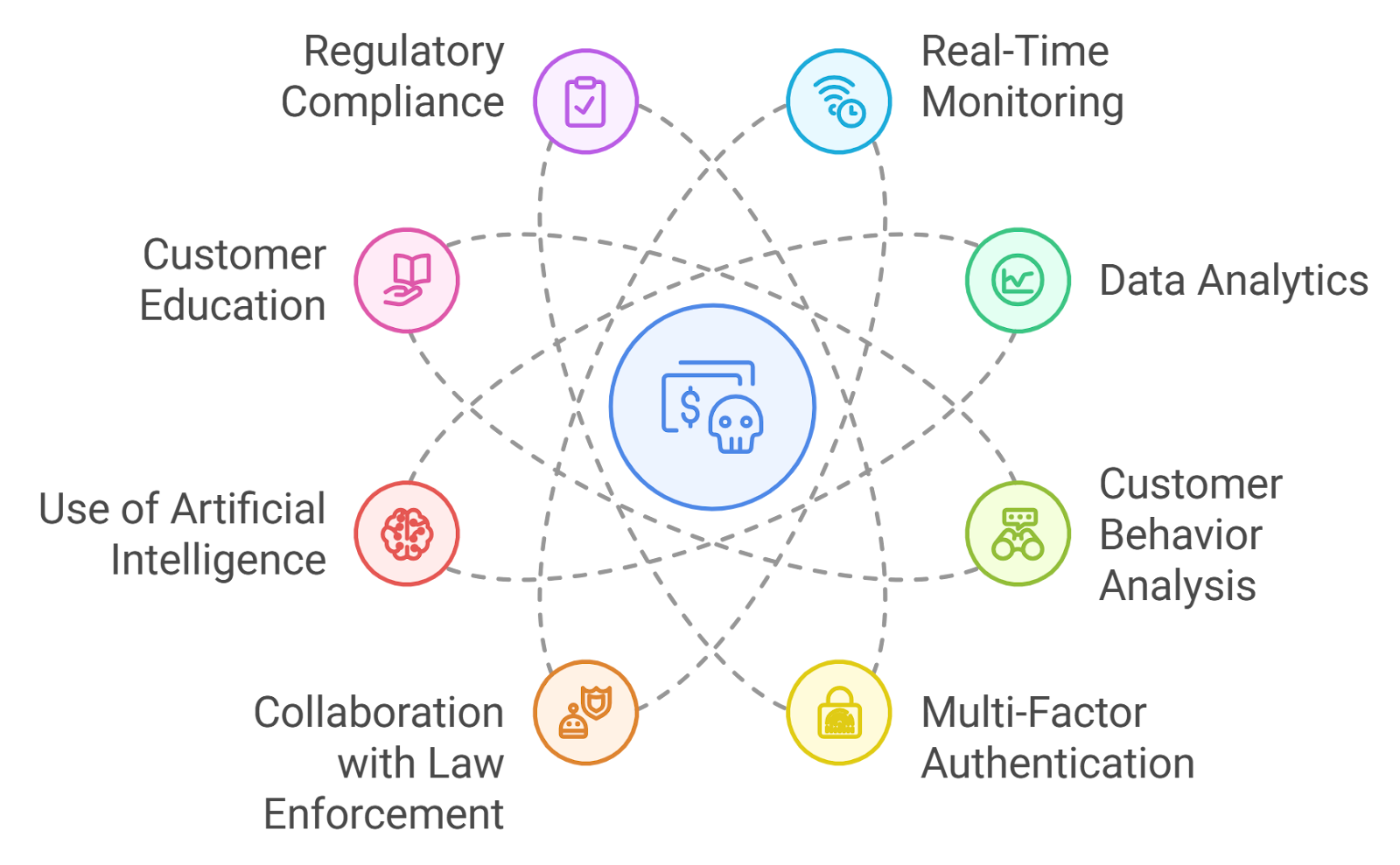
‍
Fraud detection in financial services involves identifying and preventing unauthorized transactions and activities that could lead to financial loss. With the rise of digital banking and online transactions, the need for robust fraud detection mechanisms has never been more crucial. Here are some key aspects of fraud detection in the financial sector:
- Real-Time Monitoring: Financial institutions utilize advanced algorithms and machine learning models to monitor transactions in real-time. This allows for immediate detection of suspicious activities, enabling quick responses to potential fraud. Rapid Innovation can assist in developing tailored AI solutions that enhance real-time monitoring capabilities, ensuring that clients can respond swiftly to threats.
- Data Analytics: By analyzing vast amounts of transaction data, banks can identify patterns and anomalies that may indicate fraudulent behavior. Predictive analytics helps in forecasting potential fraud risks based on historical data. Our expertise in data analytics can empower financial institutions to harness their data effectively, leading to improved decision-making and risk management.
- Customer Behavior Analysis: Understanding typical customer behavior is essential for effective fraud detection. Institutions can establish baseline behaviors for individual customers and flag transactions that deviate significantly from these norms. Rapid Innovation can implement AI-driven customer behavior analysis tools that adapt and learn over time, enhancing the accuracy of fraud detection.
- Multi-Factor Authentication (MFA): Implementing MFA adds an extra layer of security by requiring users to provide multiple forms of verification before completing a transaction. This significantly reduces the risk of unauthorized access. We can help integrate advanced MFA solutions that align with the latest security standards, ensuring robust protection for our clients.
- Collaboration with Law Enforcement: Financial institutions often collaborate with law enforcement agencies to share information about fraud trends and tactics. This partnership enhances the overall effectiveness of fraud prevention efforts. Rapid Innovation can facilitate the development of secure communication platforms that streamline information sharing between institutions and law enforcement.
- Use of Artificial Intelligence (AI): AI technologies are increasingly being integrated into fraud detection systems. Machine learning algorithms can learn from past fraud cases and improve their accuracy over time, reducing false positives and enhancing detection rates. Our team at Rapid Innovation specializes in creating AI models that evolve with emerging fraud patterns, ensuring that clients stay ahead of potential threats.
- Customer Education: Educating customers about common fraud schemes and safe banking practices is vital. Financial institutions often provide resources and training to help customers recognize and report suspicious activities. We can assist in developing engaging educational platforms that empower customers with the knowledge to protect themselves.
- Regulatory Compliance: Financial institutions must adhere to various regulations regarding fraud detection and prevention. Compliance with standards such as the Payment Card Industry Data Security Standard (PCI DSS) is essential to protect customer data and maintain trust. Rapid Innovation offers consulting services to ensure that our clients meet all regulatory requirements effectively.
- Case Studies: Numerous financial institutions have successfully implemented fraud detection systems that have significantly reduced losses. For example, a major bank reported a 30% decrease in fraud-related losses after adopting advanced machine learning techniques for transaction monitoring. Rapid Innovation has a proven track record of delivering similar results for our clients, enhancing their ROI through innovative technology solutions. Additionally, predicting fraud in financial payment services has become a key focus area, allowing institutions to proactively address potential threats before they materialize.
In conclusion, fraud detection is a critical aspect of the financial services industry, requiring a multi-faceted approach that combines technology, data analysis, and customer engagement. As fraud tactics continue to evolve, financial institutions must remain vigilant and proactive in their efforts to protect their assets and customers. Rapid Innovation is committed to providing the expertise and solutions necessary to help our clients achieve their business goals efficiently and effectively.
5.1.2. Trading Pattern Analysis
Trading pattern analysis is a crucial aspect of financial trading that involves examining historical price movements to identify trends and potential future price actions. This analysis helps traders make informed decisions based on patterns that have previously led to profitable trades, including candlestick formation patterns and chart patterns.
- Types of Patterns: Â
- Bullish Patterns: Indicate potential upward price movement, such as the "cup and handle" or "double bottom," which are common in stock chart patterns.
- Bearish Patterns: Suggest potential downward price movement, like the "head and shoulders" or "double top," often seen in share chart patterns.
- Continuation Patterns: Indicate that the current trend will continue, such as flags and pennants, which can be identified through technical analysis chart patterns.
- Technical Indicators: Â
- Moving Averages: Help smooth out price data to identify trends over specific periods.
- Relative Strength Index (RSI): Measures the speed and change of price movements to identify overbought or oversold conditions.
- Bollinger Bands: Provide a range within which the price is expected to move, helping traders identify volatility.
- Time Frames: Â
- Short-term: Day trading or swing trading often relies on minute or hourly charts, including intraday chart patterns.
- Long-term: Investors may analyze daily, weekly, or monthly charts to identify broader trends, utilizing stock market chart patterns.
- Volume Analysis: Analyzing trading volume alongside price movements can provide insights into the strength of a pattern. High volume during a breakout often confirms the validity of the pattern, which is crucial in understanding candlestick chart patterns.
- Psychological Factors: Market sentiment plays a significant role in trading patterns. Understanding trader psychology can help predict how patterns will unfold, including the impact of candle chart patterns.
At Rapid Innovation, we leverage advanced AI algorithms to enhance trading pattern analysis. By utilizing machine learning models, we can analyze vast datasets to identify patterns that may not be immediately visible to human traders, including candlestick analysis for intraday trading. This capability allows our clients to make data-driven decisions, ultimately leading to greater ROI. For more insights on how we integrate AI and ML in finance, check out our article on algorithmic trading leveraging AI and ML in finance.
5.1.3. Risk Assessment
Risk assessment in trading is the process of identifying, analyzing, and mitigating potential risks that could impact trading performance. Effective risk assessment is essential for protecting capital and ensuring long-term success in trading.
- Types of Risks: Â
- Market Risk: The risk of losses due to market fluctuations.
- Credit Risk: The risk that a counterparty will not fulfill their financial obligations.
- Liquidity Risk: The risk of not being able to buy or sell assets quickly without affecting their price.
- Risk Management Strategies: Â
- Position Sizing: Determining the appropriate amount of capital to risk on a single trade based on overall portfolio size.
- Stop-Loss Orders: Setting predetermined exit points to limit potential losses.
- Diversification: Spreading investments across various assets to reduce exposure to any single asset.
- Risk-Reward Ratio: Evaluating the potential reward of a trade relative to the risk taken. A common ratio is 1:2, meaning for every dollar risked, the potential reward is two dollars.
- Stress Testing: Simulating various market conditions to assess how a trading strategy would perform under different scenarios.
- Continuous Monitoring: Regularly reviewing and adjusting risk management strategies based on market conditions and personal trading performance.
Rapid Innovation employs blockchain technology to enhance transparency and security in risk assessment processes. By utilizing smart contracts, we can automate risk management strategies, ensuring that trades are executed according to predefined criteria without the risk of human error. This not only protects capital but also enhances overall trading efficiency.
5.2. Healthcare
The healthcare sector is a vital component of the economy, encompassing a wide range of services, products, and technologies aimed at improving health outcomes. Understanding the dynamics of the healthcare industry is essential for investors and stakeholders.
- Market Segments: Â
- Pharmaceuticals: Development and distribution of medications.
- Medical Devices: Manufacturing of equipment used in diagnosis and treatment.
- Healthcare Services: Hospitals, clinics, and telemedicine services.
- Trends Influencing Healthcare: Â
- Aging Population: An increasing number of elderly individuals drives demand for healthcare services.
- Technological Advancements: Innovations such as telehealth and wearable devices are transforming patient care.
- Regulatory Changes: Policies and regulations can significantly impact the healthcare landscape, affecting everything from drug pricing to insurance coverage.
- Investment Opportunities: Â
- Biotechnology: Investing in companies developing groundbreaking therapies and treatments.
- Health Tech: Companies focused on digital health solutions, including apps and platforms for patient management.
- Healthcare REITs: Real Estate Investment Trusts that invest in healthcare facilities, providing a way to gain exposure to the sector.
- Challenges in Healthcare: Â
- Rising Costs: Increasing healthcare costs can strain budgets for both consumers and providers.
- Regulatory Hurdles: Navigating complex regulations can be challenging for companies in the sector.
- Market Competition: The healthcare industry is highly competitive, with constant innovation and new entrants.
- Impact of Global Events: Events like the COVID-19 pandemic have highlighted the importance of healthcare infrastructure and the need for rapid response capabilities. This has led to increased investment in public health and emergency preparedness.
Understanding trading pattern analysis, risk assessment, and the healthcare sector is essential for making informed decisions in the financial markets. Each of these areas requires careful consideration and ongoing education to navigate successfully. Rapid Innovation is committed to providing cutting-edge AI and blockchain solutions that empower our clients to achieve their business goals efficiently and effectively.
5.2.1. Patient Behavior Monitoring
Patient behavior monitoring is a crucial aspect of healthcare that involves tracking and analyzing patients' actions and habits to improve health outcomes. This process can be facilitated through various technologies and methodologies, including:
- Wearable devices: Smartwatches and fitness trackers can monitor physical activity, heart rate, and sleep patterns, providing valuable data for healthcare providers.
- Mobile health applications: These apps can help patients log their symptoms, medication intake, and lifestyle choices, allowing for real-time feedback and adjustments to treatment plans.
- Telehealth platforms: Virtual consultations enable healthcare professionals to observe and interact with patients, offering insights into their behavior and adherence to treatment protocols.
The benefits of patient behavior monitoring include:
- Enhanced patient engagement: Patients who actively track their health are more likely to take ownership of their treatment.
- Improved health outcomes: Continuous monitoring can lead to timely interventions, reducing the risk of complications.
- Data-driven insights: Healthcare providers can analyze trends in patient behavior to tailor interventions and improve care strategies.
At Rapid Innovation, we leverage AI technologies to enhance patient behavior monitoring. By integrating machine learning algorithms, we can analyze vast amounts of data from wearable devices and mobile health applications, providing healthcare providers with actionable insights that lead to improved patient outcomes and greater ROI. For more information on AI agents for patient care.
5.2.2. Treatment Adherence
Treatment adherence refers to the extent to which patients follow prescribed medical advice, including medication regimens, lifestyle changes, and follow-up appointments. Ensuring high levels of treatment adherence is essential for effective healthcare delivery. Key factors influencing treatment adherence include:
- Patient education: Providing clear information about the importance of following treatment plans can empower patients to adhere to their regimens.
- Simplified medication regimens: Reducing the complexity of treatment plans can help patients remember to take their medications as prescribed.
- Support systems: Encouraging family involvement and peer support can motivate patients to stay committed to their treatment.
Strategies to improve treatment adherence include:
- Reminder systems: Automated text messages or app notifications can remind patients to take their medications or attend appointments.
- Regular follow-ups: Scheduled check-ins with healthcare providers can help address any concerns or barriers to adherence.
- Personalized care plans: Tailoring treatment plans to fit individual lifestyles and preferences can enhance patient commitment.
Rapid Innovation employs AI-driven solutions to create personalized care plans and reminder systems that significantly improve treatment adherence. By utilizing predictive analytics, we can identify patients at risk of non-adherence and implement targeted interventions, ultimately leading to better health outcomes and increased ROI for healthcare providers.
5.2.3. Early Warning Systems
Early warning systems (EWS) are tools designed to identify patients at risk of deterioration in their health status. These systems utilize data from various sources to provide timely alerts to healthcare providers. Key components of early warning systems include:
- Vital sign monitoring: Continuous tracking of vital signs such as heart rate, blood pressure, and oxygen saturation can help detect early signs of distress.
- Predictive analytics: Advanced algorithms analyze patient data to identify patterns that may indicate potential health crises.
- Interdisciplinary collaboration: EWS often involve a team of healthcare professionals who work together to interpret data and respond to alerts.
The advantages of implementing early warning systems are:
- Timely interventions: Early detection of health issues allows for prompt medical responses, potentially saving lives.
- Resource optimization: EWS can help prioritize care for patients who need immediate attention, improving overall efficiency in healthcare settings.
- Enhanced patient safety: By identifying at-risk patients early, healthcare providers can implement preventive measures to reduce complications.
At Rapid Innovation, we harness the power of blockchain technology to ensure the integrity and security of data used in early warning systems. By creating a decentralized and tamper-proof record of patient data, we enhance the reliability of predictive analytics, leading to timely interventions and improved patient safety. This not only optimizes resource allocation but also contributes to a higher return on investment for healthcare organizations.
5.3. Retail and E-commerce
The retail and e-commerce sectors have undergone significant transformations in recent years, driven by technological advancements and changing consumer behaviors. Businesses are increasingly leveraging data analytics, artificial intelligence, and machine learning to enhance customer experiences and optimize operations. Retailers are focusing on omnichannel strategies to provide seamless shopping experiences across physical and digital platforms. E-commerce platforms are expanding their offerings, including personalized recommendations and targeted marketing campaigns. The rise of mobile shopping has led to the development of user-friendly apps and mobile-optimized websites.
5.3.1. Customer Journey Analysis
Customer journey analysis involves mapping out the various stages a customer goes through when interacting with a brand, from awareness to post-purchase evaluation. Understanding this journey is crucial for retailers and e-commerce businesses to enhance customer satisfaction and drive sales. The key stages of the customer journey include:
- Awareness: Customers become aware of a product or brand through advertising, social media, or word-of-mouth.
- Consideration: Customers research and compare options, looking for reviews and recommendations, including insights from retail SEO strategies.
- Purchase: Customers make the final decision and complete the transaction.
- Post-Purchase: Customers evaluate their experience and may share feedback or engage with customer service.
The benefits of customer journey analysis are significant:
- Identifies pain points and areas for improvement in the shopping experience.
- Helps tailor marketing strategies to target customers at different stages.
- Enhances customer retention by fostering loyalty through personalized experiences.
Tools and techniques for customer journey analysis include:
- Customer feedback surveys to gather insights on experiences.
- Heatmaps and session recordings to analyze user behavior on websites.
- Data analytics platforms to track customer interactions across channels.
At Rapid Innovation, we utilize advanced AI algorithms to analyze customer journeys, enabling retailers to gain actionable insights that lead to improved customer engagement and increased sales. This is particularly important for retail SEO, as optimizing the customer journey can significantly impact search engine visibility and traffic.
5.3.2. Purchase Pattern Prediction
Purchase pattern prediction involves using data analytics and machine learning algorithms to forecast future buying behaviors based on historical data. This predictive analysis helps retailers and e-commerce businesses make informed decisions regarding inventory management, marketing strategies, and customer engagement. The key components of purchase pattern prediction are:
- Data Collection: Gathering data from various sources, including sales transactions, customer demographics, and online behavior.
- Data Analysis: Utilizing statistical methods and machine learning models to identify trends and patterns in purchasing behavior.
- Forecasting: Predicting future sales and customer preferences based on identified patterns.
The benefits of purchase pattern prediction include:
- Optimizes inventory management by anticipating demand for products.
- Enhances targeted marketing efforts by understanding customer preferences.
- Improves customer experience by offering personalized recommendations.
Techniques used in purchase pattern prediction consist of:
- Collaborative filtering to recommend products based on similar customer behaviors.
- Time series analysis to identify seasonal trends and fluctuations in sales.
- Regression analysis to understand the impact of various factors on purchasing decisions.
By leveraging Rapid Innovation's expertise in AI and machine learning, retailers can implement robust purchase pattern prediction models that not only enhance operational efficiency but also drive significant ROI through improved customer satisfaction and loyalty. This is especially relevant for retail ecommerce SEO, as understanding purchase patterns can inform more effective online marketing strategies.
By focusing on customer journey analysis and purchase pattern prediction, retailers and e-commerce businesses can create more effective strategies that enhance customer satisfaction and drive sales growth, ultimately benefiting from comprehensive retail ecommerce strategies.
5.3.3. Inventory Optimization
Inventory optimization is a critical component of supply chain management that focuses on maintaining the right balance of stock to meet customer demand while minimizing costs. Effective inventory optimization can lead to improved cash flow, reduced holding costs, and enhanced customer satisfaction.
- Key strategies for inventory optimization include: Â
- Demand Forecasting: Utilizing historical data and market trends to predict future demand accurately. Rapid Innovation leverages advanced AI algorithms to enhance demand forecasting accuracy, enabling businesses to align their inventory levels with actual market needs.
- Just-in-Time (JIT) Inventory: Reducing excess stock by receiving goods only as they are needed in the production process. Our consulting services help clients implement JIT strategies that minimize waste and optimize resource allocation.
- ABC Analysis: Categorizing inventory into three classes (A, B, and C) based on their importance and value, allowing businesses to prioritize management efforts. Rapid Innovation assists clients in developing tailored ABC analysis frameworks that drive focused inventory management.
- Safety Stock: Maintaining a buffer of inventory to protect against unexpected demand spikes or supply chain disruptions. We provide insights on optimal safety stock levels using predictive analytics, ensuring businesses are prepared for fluctuations.
- Automated Inventory Management Systems: Implementing software solutions that provide real-time inventory tracking and analytics. Our expertise in AI-driven automation allows clients to streamline their inventory processes, reducing manual errors and enhancing efficiency.
- Benefits of inventory optimization include: Â
- Improved cash flow management
- Reduced storage and holding costs
- Enhanced customer service levels
- Increased operational efficiency
By focusing on these inventory optimization strategies, businesses can achieve a more streamlined inventory process, ultimately leading to better financial performance and customer satisfaction. For advanced solutions in inventory management, consider our AI and blockchain powering digital security efficiency in 2024 that can enhance your operational efficiency.
5.4. Cybersecurity
Cybersecurity is an essential aspect of modern business operations, particularly as organizations increasingly rely on digital technologies. It involves protecting systems, networks, and data from cyber threats, ensuring the confidentiality, integrity, and availability of information.
- Key components of cybersecurity include: Â
- Risk Assessment: Identifying and evaluating potential risks to information systems and data. Rapid Innovation employs advanced risk assessment methodologies to help clients understand their vulnerabilities and develop robust security frameworks.
- Access Control: Implementing measures to restrict access to sensitive information based on user roles and responsibilities. Our blockchain solutions enhance access control by providing secure, decentralized identity management.
- Data Encryption: Protecting data by converting it into a secure format that can only be read by authorized users. We offer cutting-edge encryption technologies to safeguard sensitive information against unauthorized access.
- Regular Software Updates: Keeping systems and applications up to date to protect against vulnerabilities. Our proactive maintenance services ensure that clients' systems are always equipped with the latest security patches.
- Employee Training: Educating staff about cybersecurity best practices and potential threats, such as phishing attacks. Rapid Innovation provides tailored training programs to empower employees in recognizing and mitigating cyber threats.
- The importance of cybersecurity cannot be overstated: Â
- Cyberattacks can lead to significant financial losses, with the average cost of a data breach estimated at $4.24 million.
- Organizations face legal and regulatory consequences for failing to protect sensitive data.
- A strong cybersecurity posture enhances customer trust and brand reputation.
Investing in robust cybersecurity measures is crucial for safeguarding business assets and maintaining operational continuity.
5.4.1. Threat Detection
Threat detection is a vital aspect of cybersecurity that involves identifying potential security incidents before they can cause harm. Effective threat detection mechanisms enable organizations to respond swiftly to mitigate risks and protect sensitive information.
- Key methods of threat detection include: Â
- Intrusion Detection Systems (IDS): Monitoring network traffic for suspicious activity and potential threats. Rapid Innovation integrates advanced IDS solutions that utilize AI to enhance threat detection capabilities.
- Security Information and Event Management (SIEM): Aggregating and analyzing security data from various sources to identify anomalies and potential threats. Our SIEM solutions provide real-time insights, enabling organizations to respond proactively to security incidents.
- Behavioral Analytics: Using machine learning algorithms to establish a baseline of normal user behavior and detect deviations that may indicate a security threat. We implement behavioral analytics tools that enhance the accuracy of threat detection.
- Threat Intelligence: Gathering and analyzing information about emerging threats to stay ahead of potential attacks. Our threat intelligence services equip clients with actionable insights to fortify their defenses.
- Benefits of effective threat detection include: Â
- Early identification of potential security breaches
- Reduced response time to incidents
- Enhanced overall security posture
- Improved compliance with regulatory requirements
By implementing robust threat detection strategies, organizations can significantly reduce their vulnerability to cyber threats and protect their critical assets. Rapid Innovation is committed to helping clients achieve a secure and resilient operational environment through our comprehensive cybersecurity solutions.
5.4.2. User Behavior Analytics
User Behavior Analytics (UBA) is a crucial component in understanding how users interact with systems and applications. By analyzing user behavior, organizations can identify anomalies, detect potential security threats, and enhance user experience. UBA utilizes machine learning algorithms to establish a baseline of normal user behavior, monitoring various activities such as login times, frequency of access, and data usage patterns. Anomalies are flagged for further investigation, helping to identify potential insider threats or compromised accounts. Additionally, UBA can provide insights into user engagement, allowing organizations to tailor their services to better meet user needs. By leveraging UBA, companies can also improve compliance with regulations by ensuring that user activities align with established policies.
- UBA utilizes machine learning algorithms to establish a baseline of normal user behavior.
- It monitors various activities, such as login times, frequency of access, and data usage patterns.
- Anomalies are flagged for further investigation, helping to identify potential insider threats or compromised accounts.
- UBA can also provide insights into user engagement, allowing organizations to tailor their services to meet user needs better.
- By leveraging UBA, companies can improve compliance with regulations by ensuring that user activities align with established policies.
At Rapid Innovation, we harness the power of UBA to help our clients achieve greater ROI by enhancing security measures and improving user engagement. For instance, by implementing user behavior analytics software, a financial services client was able to detect unusual login patterns, which led to the identification of a compromised account. This proactive approach not only safeguarded sensitive data but also reinforced customer trust, ultimately resulting in increased customer retention and satisfaction. Our expertise in enterprise AI development allows us to deliver tailored solutions that meet the unique needs of each organization. Additionally, we explore the potential of proactive AI agents to further enhance user behavior analytics and security measures.
5.4.3. Access Pattern Monitoring
Access Pattern Monitoring (APM) focuses on tracking and analyzing how users access data and resources within an organization. This practice is essential for maintaining security and optimizing resource allocation. APM helps in identifying trends in user access, such as peak usage times and frequently accessed resources. It can reveal unauthorized access attempts, allowing for immediate action to mitigate risks. By understanding access patterns, organizations can implement more effective access controls and permissions. APM can also assist in capacity planning by identifying which resources are over or under-utilized. Regular monitoring of access patterns can enhance overall security posture and ensure compliance with data protection regulations.
- APM helps in identifying trends in user access, such as peak usage times and frequently accessed resources.
- It can reveal unauthorized access attempts, allowing for immediate action to mitigate risks.
- By understanding access patterns, organizations can implement more effective access controls and permissions.
- APM can also assist in capacity planning by identifying which resources are over or under-utilized.
- Regular monitoring of access patterns can enhance overall security posture and ensure compliance with data protection regulations.
At Rapid Innovation, we implement APM solutions that empower organizations to optimize their resource allocation and enhance security. For example, a healthcare client utilized APM to identify peak access times for sensitive patient data, allowing them to allocate resources more effectively and ensure compliance with HIPAA regulations. This not only improved operational efficiency but also reduced the risk of unauthorized access, leading to a more secure environment for patient information.
6. Implementation Framework
An effective implementation framework is vital for integrating User Behavior Analytics and Access Pattern Monitoring into an organization’s security strategy. This framework outlines the steps necessary to deploy these technologies successfully.
- Define objectives: Clearly outline the goals of implementing UBA and APM, such as improving security or enhancing user experience.
- Assess current infrastructure: Evaluate existing systems and processes to identify gaps and areas for improvement.
- Select appropriate tools: Choose analytics and monitoring tools that align with organizational needs and can integrate seamlessly with current systems.
- Develop a data governance strategy: Establish policies for data collection, storage, and usage to ensure compliance with regulations.
- Train staff: Provide training for employees on the importance of UBA and APM, as well as how to use the tools effectively.
- Monitor and adjust: Continuously assess the effectiveness of the implementation, making adjustments as necessary to optimize performance and security.
By following this implementation framework, organizations can effectively leverage User Behavior Analytics and Access Pattern Monitoring to enhance their security measures and improve overall operational efficiency. At Rapid Innovation, we guide our clients through each step of this framework, ensuring that they achieve their business goals efficiently and effectively.
6.1. Assessment and Planning
Assessment and planning are critical components in any project, especially in fields like education, healthcare, and technology. This phase involves evaluating the current situation, identifying needs, and developing a strategic plan to address those needs effectively. A thorough assessment ensures that resources are allocated efficiently and that the project aligns with the overall goals of the organization.
- Establish clear objectives for the assessment.
- Gather data through surveys, interviews, and observations.
- Analyze the data to identify gaps and opportunities.
- Involve stakeholders in the planning process to ensure buy-in.
- Develop a timeline and budget for the project.
6.1.1. Needs Analysis
Needs analysis is the process of identifying and evaluating the needs of a target group or organization. This step is essential for ensuring that the project addresses the right issues and provides value to its intended audience. A comprehensive needs analysis can lead to more effective solutions and better outcomes.
- Define the target audience and their characteristics.
- Use qualitative and quantitative methods to gather data.
- Identify existing resources and gaps in services.
- Prioritize needs based on urgency and impact.
- Document findings to guide the planning process.
6.1.2. Technical Requirements
Technical requirements outline the specific technological needs necessary to implement a project successfully. This includes hardware, software, and any other tools or systems required to achieve the project goals. Clearly defined technical requirements help ensure that the project is feasible and that all stakeholders understand what is needed for success.
- Identify the necessary hardware and software components.
- Assess compatibility with existing systems and infrastructure.
- Determine user requirements and accessibility needs.
- Establish security protocols and data management practices.
- Create a maintenance and support plan for ongoing operations.
At Rapid Innovation, we leverage our expertise in AI and Blockchain to enhance the assessment and planning process. For instance, in a healthcare project, we can utilize AI-driven analytics to gather and analyze data more efficiently, identifying critical gaps in patient care. In a blockchain initiative, we can ensure that the technical requirements include robust security measures and interoperability with existing systems, ultimately leading to a more streamlined implementation and greater ROI for our clients. By integrating advanced technologies into the assessment and planning phases, we help organizations achieve their business goals more effectively and efficiently.
Additionally, we utilize examples of monitoring and evaluation plans to ensure that our projects are on track and meeting their objectives. Our project assessment plan is designed to provide a clear framework for evaluating progress and making necessary adjustments. Furthermore, our project planning management and evaluation processes are tailored to fit the unique needs of each initiative, ensuring comprehensive oversight and successful outcomes. Finally, our project assessment and planning strategies are continuously refined based on feedback and results, allowing us to adapt and improve our approach over time.
6.1.3. Resource Planning
Resource planning is a critical component of project management that ensures the efficient allocation and utilization of resources. It involves identifying, scheduling, and managing resources such as personnel, equipment, and materials necessary for project completion. Effective resource planning can significantly impact project success by minimizing costs and maximizing productivity.
- Assessing resource availability: Understanding what resources are available and when they can be utilized is essential. This includes human resources, technology, and financial assets.
- Estimating resource requirements: Accurately estimating the amount and type of resources needed for each phase of the project helps in avoiding shortages or excesses. This may involve utilizing enterprise resource planning (ERP) systems to streamline the estimation process.
- Scheduling resources: Creating a timeline for when resources will be needed ensures that they are available at the right time, preventing delays. Implementing enterprise resource management systems can aid in effective scheduling.
- Monitoring resource usage: Regularly tracking resource consumption helps in identifying any discrepancies and allows for timely adjustments. Tools such as enterprise resource management software can facilitate this monitoring.
- Adjusting plans as necessary: Flexibility in resource planning is crucial. If unexpected challenges arise, being able to reallocate resources can keep the project on track. This is where ERP in IT can play a significant role.
Effective resource planning not only enhances project efficiency but also contributes to better risk management and stakeholder satisfaction. At Rapid Innovation, we leverage AI-driven analytics to optimize resource allocation, ensuring that our clients achieve greater ROI by utilizing their resources more effectively, including through the use of enterprise resource planning software.
6.2. Development and Integration

‍
Development and integration are vital stages in the project lifecycle, particularly in technology and software projects. This phase focuses on creating the product or service and ensuring that all components work together seamlessly.
- Defining development processes: Establishing clear methodologies, such as Agile or Waterfall, helps in structuring the development phase.
- Collaborating with stakeholders: Engaging with stakeholders throughout the development process ensures that their needs and expectations are met.
- Integrating systems and components: Ensuring that different systems and components work together is crucial for functionality. This may involve API integrations, data migration, and compatibility testing.
- Conducting testing and quality assurance: Rigorous testing is essential to identify and rectify issues before the final product launch. This includes unit testing, integration testing, and user acceptance testing.
- Documenting the development process: Keeping detailed records of the development process aids in future reference and helps in onboarding new team members.
Successful development and integration lead to a robust final product that meets user requirements and performs reliably. Rapid Innovation employs advanced blockchain technology to ensure secure and efficient integration, enhancing the overall reliability of the solutions we deliver.
6.2.1. Model Development
Model development is a specific aspect of the development phase, particularly relevant in fields such as data science, machine learning, and software engineering. It involves creating a model that accurately represents the system or process being studied or automated.
- Defining objectives: Clearly outlining the goals of the model helps in guiding the development process and ensuring that the final product meets its intended purpose.
- Selecting appropriate methodologies: Choosing the right modeling techniques, such as regression analysis, neural networks, or simulation models, is crucial for achieving accurate results.
- Data collection and preprocessing: Gathering relevant data and preparing it for analysis is a foundational step in model development. This may involve cleaning, normalizing, and transforming data.
- Training the model: Using training data to teach the model how to make predictions or decisions is a critical step. This often involves adjusting parameters to improve accuracy.
- Validating and testing the model: Ensuring that the model performs well on unseen data is essential. This includes using validation datasets and performance metrics to assess its effectiveness.
- Iterating and refining: Model development is often an iterative process. Based on testing results, adjustments may be necessary to enhance performance.
Effective model development leads to reliable predictions and insights, which can significantly impact decision-making processes in various industries. At Rapid Innovation, we harness the power of AI to create predictive models that drive strategic decisions, ultimately enhancing our clients' operational efficiency and profitability, including through the use of manufacturing resource planning and material requirement planning methodologies.
6.2.2. System Integration
System integration is a critical phase in the development of complex systems, ensuring that various components work together seamlessly. This process involves combining different subsystems and ensuring they function as a cohesive unit.
- Purpose of System Integration: Â
- To enhance functionality by allowing different systems to communicate.
- To improve efficiency by automating workflows across various platforms, such as through integrated computer systems and totally integrated automation.
- To reduce redundancy by consolidating data and processes.
- Types of Integration: Â
- Horizontal Integration: Connecting different systems at the same level, often through middleware.
- Vertical Integration: Linking systems at different levels, such as connecting operational systems with management systems, including human system integration.
- Star Integration: A central hub connects multiple systems, simplifying communication.
- Challenges in System Integration: Â
- Compatibility issues between different technologies.
- Data silos that prevent seamless information flow.
- Complexity in managing multiple integrations, especially with integrated data systems.
- Best Practices: Â
- Conduct thorough requirements analysis to understand integration needs.
- Use standardized protocols and APIs to facilitate communication, particularly in systems like sales force integration and integrated voice response systems.
- Implement robust monitoring tools to track integration performance.
At Rapid Innovation, we leverage our expertise in AI and Blockchain to streamline system integration processes. For instance, by utilizing AI-driven analytics, we can identify integration bottlenecks and optimize workflows, leading to improved operational efficiency and a higher return on investment (ROI) for our clients. Our solutions include interactive voice response systems and CRM system integrations like Salesforce CRM and HubSpot. Additionally, we explore the role of AI agents in software testing to enhance our integration strategies.
6.2.3. Testing and Validation
Testing and validation are essential steps in the software development lifecycle, ensuring that the system meets specified requirements and functions correctly. This phase helps identify defects and ensures the system is reliable and user-friendly.
- Importance of Testing: Â
- Ensures the system meets user expectations and requirements.
- Identifies bugs and issues before deployment, reducing costs associated with post-launch fixes.
- Enhances system security by identifying vulnerabilities.
- Types of Testing: Â
- Unit Testing: Testing individual components for functionality.
- Integration Testing: Ensuring that combined components work together as intended.
- System Testing: Validating the complete and integrated software product.
- User Acceptance Testing (UAT): Conducted by end-users to validate the system against their requirements.
- Validation Process: Â
- Involves checking if the system meets the business needs and requirements.
- Ensures that the system is built correctly and functions as intended.
- Often includes formal reviews and audits.
- Best Practices: Â
- Develop a comprehensive test plan that outlines testing strategies and objectives.
- Automate testing where possible to increase efficiency and coverage.
- Involve stakeholders in the testing process to gather feedback and ensure alignment with user needs.
At Rapid Innovation, we implement rigorous testing protocols, including automated testing solutions powered by AI, to enhance the reliability and security of our systems. This proactive approach not only minimizes post-launch issues but also significantly reduces costs, thereby maximizing ROI for our clients.
6.3. Deployment Strategies
Deployment strategies are crucial for the successful launch of a system, determining how software is delivered to users. A well-planned deployment strategy minimizes disruptions and ensures a smooth transition.
- Types of Deployment Strategies: Â
- Big Bang Deployment: All components are deployed at once. This approach can be risky but is straightforward.
- Phased Deployment: Gradually rolling out the system in stages, allowing for adjustments based on feedback.
- Parallel Deployment: Running the new system alongside the old one until the new system is fully operational.
- Canary Deployment: Releasing the system to a small subset of users first to monitor performance before a full rollout.
- Factors to Consider: Â
- User Readiness: Assessing whether users are prepared for the new system.
- Training Needs: Providing adequate training to ensure users can effectively use the new system, especially in systems like Freshdesk ticketing system.
- Support Mechanisms: Establishing support channels to assist users during the transition.
- Best Practices: Â
- Create a detailed deployment plan that outlines timelines, responsibilities, and resources.
- Conduct a pilot test to identify potential issues before full deployment.
- Monitor system performance post-deployment to address any issues quickly.
By focusing on system integration, testing and validation, and deployment strategies, organizations can ensure a successful software development lifecycle, leading to enhanced user satisfaction and operational efficiency. At Rapid Innovation, our commitment to leveraging AI and Blockchain technologies ensures that our clients achieve their business goals efficiently and effectively, ultimately driving greater ROI.
6.3.1. Phased Implementation
Phased implementation is a strategic approach to deploying new systems, processes, or technologies in manageable segments. This method allows organizations to minimize risks and ensure smoother transitions. By breaking down the implementation into phases, organizations can focus on one aspect at a time, making it easier to manage resources and timelines. Each phase provides an opportunity to gather feedback, assess performance, and make necessary adjustments before moving on to the next stage. Additionally, phased implementation allows for better allocation of resources, ensuring that teams are not overwhelmed and that budgets are adhered to. By implementing changes gradually, organizations can identify potential issues early and address them before they escalate. Each phase can also include training sessions for staff, ensuring that everyone is prepared and knowledgeable about the new systems or processes.
- Step-by-step approach: By breaking down the implementation into phases, organizations can focus on one aspect at a time, making it easier to manage resources and timelines.
- Feedback loops: Each phase provides an opportunity to gather feedback, assess performance, and make necessary adjustments before moving on to the next stage.
- Resource allocation: Phased implementation allows for better allocation of resources, ensuring that teams are not overwhelmed and that budgets are adhered to.
- Risk management: By implementing changes gradually, organizations can identify potential issues early and address them before they escalate.
- Training opportunities: Each phase can include training sessions for staff, ensuring that everyone is prepared and knowledgeable about the new systems or processes.
- Phased implementation approach: This structured method enhances the overall effectiveness of the deployment process.
- Phased implementation strategy: A well-defined strategy is crucial for successful execution and achieving desired outcomes.
- Advantages of phased implementation: Organizations can leverage the benefits of this approach to improve their transition to new systems.
6.3.2. Pilot Programs
Pilot programs are small-scale tests of new initiatives or technologies before a full-scale rollout. They serve as a proving ground to evaluate effectiveness and gather insights. Pilot programs allow organizations to test new ideas in a controlled setting, minimizing the impact of potential failures. These programs provide valuable data on user experience, operational efficiency, and overall effectiveness, which can inform future decisions. Involving stakeholders in pilot programs fosters buy-in and support for the initiative, as they can see firsthand the benefits and challenges. Feedback from pilot programs can lead to iterative improvements, ensuring that the final implementation is more refined and effective. By identifying issues early in a pilot program, organizations can avoid costly mistakes during a full rollout.
- Controlled environment: Pilot programs allow organizations to test new ideas in a controlled setting, minimizing the impact of potential failures.
- Data collection: These programs provide valuable data on user experience, operational efficiency, and overall effectiveness, which can inform future decisions.
- Stakeholder engagement: Involving stakeholders in pilot programs fosters buy-in and support for the initiative, as they can see firsthand the benefits and challenges.
- Iterative improvements: Feedback from pilot programs can lead to iterative improvements, ensuring that the final implementation is more refined and effective.
- Cost-effectiveness: By identifying issues early in a pilot program, organizations can avoid costly mistakes during a full rollout.
6.3.3. Scaling Considerations
Scaling considerations are crucial for organizations looking to expand successful initiatives or technologies beyond their initial implementation. Proper planning ensures that growth is sustainable and effective. Assessing whether existing infrastructure can support scaling is essential, including technology, human resources, and operational processes. Understanding market demand is vital for scaling; organizations should analyze customer needs and trends to ensure that scaling aligns with market opportunities. As initiatives scale, organizations must manage resources effectively, including finances, personnel, and technology, to avoid overextension. Developing standardized processes and protocols can facilitate smoother scaling, ensuring consistency across different locations or teams. Continuous monitoring and evaluation are necessary to assess the effectiveness of scaled initiatives, allowing for timely adjustments and improvements.
- Infrastructure readiness: Assessing whether existing infrastructure can support scaling is essential. This includes technology, human resources, and operational processes.
- Market demand: Understanding market demand is vital for scaling. Organizations should analyze customer needs and trends to ensure that scaling aligns with market opportunities.
- Resource management: As initiatives scale, organizations must manage resources effectively, including finances, personnel, and technology, to avoid overextension.
- Standardization: Developing standardized processes and protocols can facilitate smoother scaling, ensuring consistency across different locations or teams.
- Monitoring and evaluation: Continuous monitoring and evaluation are necessary to assess the effectiveness of scaled initiatives, allowing for timely adjustments and improvements.
At Rapid Innovation, we leverage our expertise in AI and Blockchain to guide organizations through these processes, ensuring that each phase of implementation, pilot program, and scaling consideration is optimized for maximum ROI. By utilizing advanced analytics and blockchain's transparency, we help clients make informed decisions that drive efficiency and effectiveness in achieving their business goals.
7. Challenges and Solutions
Data-driven decision-making is essential for businesses today, but it comes with its own set of challenges, including data management challenges and database management challenges. Understanding these challenges and their potential solutions can help organizations navigate the complexities of data management effectively.
7.1 Data Quality and Availability
Data quality and availability are critical components of effective data management. Poor data quality can lead to inaccurate insights, while data unavailability can hinder timely decision-making.
Inaccurate Data: Data can be flawed due to human error, outdated information, or incorrect data entry, resulting in misleading analytics and poor business decisions.
Inconsistent Data Sources: Organizations often gather data from multiple sources, leading to inconsistencies. Different formats, terminologies, and structures can complicate data integration.
Data Silos: Departments may hoard data, creating silos that prevent a holistic view of the organization, leading to missed opportunities and inefficiencies.
Solutions:
- Data Governance Framework: Implementing a robust data governance framework can help ensure data quality. This includes establishing data standards, ownership, and accountability, which Rapid Innovation can assist in developing tailored frameworks that align with your business objectives.
- Regular Data Audits: Conducting regular audits can help identify and rectify data quality issues. This process should include checks for accuracy, completeness, and consistency. Rapid Innovation offers advanced AI-driven tools that automate these audits, ensuring timely and accurate assessments.
- Centralized Data Management: Utilizing centralized data management systems can break down silos, allowing for better data integration and accessibility across departments. Our blockchain solutions can facilitate secure and transparent data sharing, enhancing collaboration and efficiency.
7.2 Privacy and Compliance
As data collection increases, so do concerns about privacy and compliance with regulations. Organizations must navigate a complex landscape of laws and ethical considerations, including issues in managing data and data governance in healthcare challenges.
Regulatory Compliance: Laws such as GDPR and CCPA impose strict requirements on how organizations collect, store, and use personal data. Non-compliance can result in hefty fines and legal repercussions.
Data Breaches: The risk of data breaches is ever-present. A breach can compromise sensitive information, leading to loss of customer trust and potential legal action.
Consumer Trust: With growing awareness of data privacy issues, consumers are increasingly concerned about how their data is used. Organizations must prioritize transparency to maintain trust.
Solutions:
- Privacy Policies and Training: Developing clear privacy policies and providing training for employees can help ensure compliance. Rapid Innovation can assist in crafting comprehensive policies tailored to your organization’s needs, ensuring that employees understand the importance of data privacy and the specific regulations that apply to their roles.
- Data Encryption and Security Measures: Implementing strong data encryption and security protocols can protect sensitive information from unauthorized access. Regular security assessments can help identify vulnerabilities. Our blockchain technology offers enhanced security features that can safeguard your data against breaches.
- Transparency and Communication: Organizations should communicate openly with customers about data usage. Providing clear information about data collection practices can enhance consumer trust and loyalty. Rapid Innovation can help design user-friendly communication strategies that foster transparency and build customer confidence.
By addressing these data management problems and solutions with effective strategies, organizations can improve their data management practices, ensuring better decision-making and compliance with regulations. Rapid Innovation is committed to helping clients navigate these complexities, leveraging our expertise in AI and blockchain to drive greater ROI and operational efficiency, particularly in managing data in cloud computing and tackling master data management challenges.
7.3. Technical Infrastructure
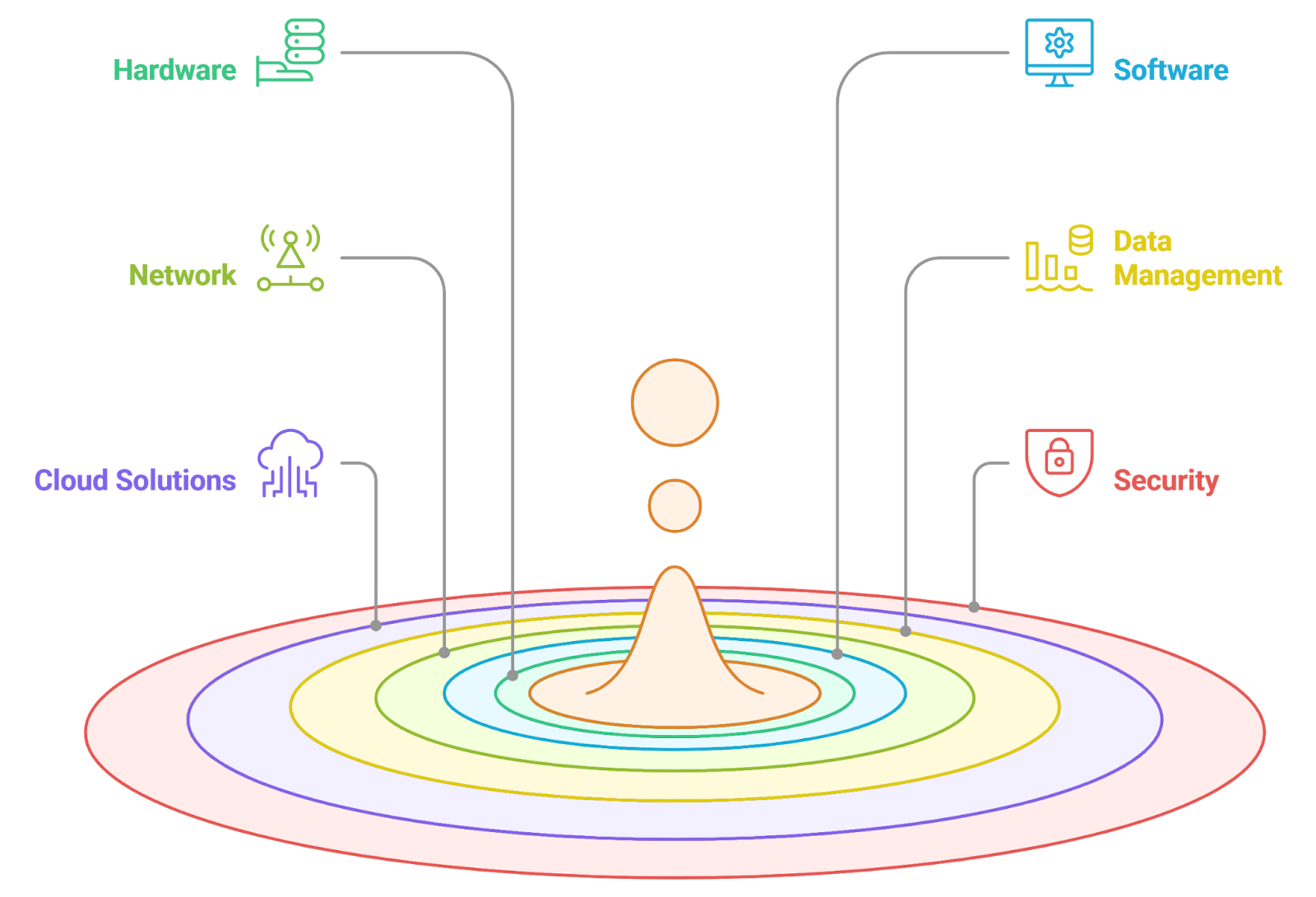
‍
A robust technical infrastructure is essential for any organization aiming to leverage technology effectively. This infrastructure encompasses hardware, software, networks, and data management systems that support business operations.
- Hardware: This includes servers, computers, and networking equipment. Organizations must invest in reliable hardware to ensure smooth operations and minimize downtime. Rapid Innovation can assist in selecting and implementing the right technical infrastructure solutions tailored to your specific operational needs.
- Software: The choice of software solutions, including operating systems, applications, and databases, is crucial. Organizations should select software that aligns with their business needs and can scale as they grow. Our expertise in AI and Blockchain allows us to recommend and develop software solutions that enhance efficiency and drive innovation.
- Network: A strong network infrastructure is vital for connectivity. This includes both local area networks (LAN) and wide area networks (WAN), ensuring that all devices can communicate efficiently. Rapid Innovation can help design and implement a network architecture that supports your business goals while ensuring optimal performance.
- Data Management: Effective data management systems are necessary for storing, retrieving, and analyzing data. This includes databases and data warehouses that support business intelligence initiatives. Our team can develop advanced data management solutions that leverage AI for predictive analytics, enabling better decision-making and greater ROI.
- Cloud Solutions: Many organizations are moving towards cloud-based solutions for flexibility and scalability. Cloud infrastructure allows for remote access and can reduce the need for extensive on-premises hardware. Rapid Innovation specializes in cloud migration strategies that ensure a seamless transition while maximizing cost savings.
- Security: A secure technical infrastructure is paramount. This includes firewalls, encryption, and regular security audits to protect sensitive data from breaches. Our blockchain solutions can enhance security protocols, providing an immutable record of transactions and data integrity. Additionally, our expertise as an AI technology consulting company can help you implement advanced security measures tailored to your needs.
7.4. Skills and Training
Investing in skills and training is critical for maximizing the potential of any technical infrastructure. Employees must be equipped with the necessary skills to utilize technology effectively.
- Continuous Learning: Technology is constantly evolving, and ongoing training is essential. Organizations should encourage employees to participate in workshops, webinars, and online courses to stay updated. Rapid Innovation offers tailored training programs that focus on the latest advancements in AI and Blockchain.
- Technical Skills: Employees should possess relevant technical skills, such as programming, data analysis, and cybersecurity. These skills enable them to leverage technology for improved productivity. Our training modules are designed to enhance these skills, ensuring your team is well-equipped to handle emerging technologies.
- Soft Skills: In addition to technical skills, soft skills like problem-solving, communication, and teamwork are vital. These skills help employees collaborate effectively and adapt to changes in technology.
- Certification Programs: Organizations can benefit from offering certification programs in relevant technologies. Certifications can enhance employee credibility and ensure they are knowledgeable about industry standards. Rapid Innovation can assist in developing certification pathways that align with industry best practices.
- Mentorship: Establishing mentorship programs can facilitate knowledge transfer between experienced employees and newcomers. This fosters a culture of learning and helps build a skilled workforce.
- Performance Evaluation: Regular performance evaluations can identify skill gaps and training needs. Organizations should use these evaluations to tailor training programs to meet specific employee needs.
7.5. Integration with Legacy Systems
Integrating new technologies with legacy systems is a significant challenge for many organizations. Legacy systems, while often outdated, may still play a crucial role in daily operations.
- Assessment: Before integration, organizations should assess their existing legacy systems to understand their capabilities and limitations. This assessment helps identify which systems can be integrated and which may need replacement.
- Middleware Solutions: Middleware can facilitate communication between new applications and legacy systems. This software acts as a bridge, allowing different systems to work together seamlessly. Rapid Innovation can develop custom middleware solutions that ensure smooth interoperability.
- Data Migration: Migrating data from legacy systems to new platforms is often necessary. Organizations must ensure data integrity and security during this process to avoid data loss or corruption. Our team specializes in secure data migration strategies that minimize risk and ensure compliance.
- Phased Approach: A phased approach to integration can minimize disruption. Organizations can gradually implement new systems while maintaining legacy systems until the transition is complete.
- Training for Users: Employees must be trained on both legacy and new systems. This ensures they can navigate the transition smoothly and continue to perform their tasks effectively.
- Vendor Support: Engaging with vendors who specialize in integration can provide valuable expertise. These vendors can offer solutions tailored to the organization’s specific needs and help troubleshoot any issues that arise during integration. Rapid Innovation can serve as your trusted partner in navigating these complexities, ensuring a successful integration process.
7.6. Cost Management
Cost management is a critical aspect of project management that involves planning, estimating, budgeting, and controlling costs to ensure that a project is completed within the approved budget. Effective cost management helps organizations maximize their resources and achieve their financial goals.
- Planning: This involves defining how project costs will be managed throughout the project lifecycle. A cost management plan outlines the processes for estimating, budgeting, and controlling costs, ensuring that all financial aspects are aligned with the project's objectives.
- Estimating: Accurate cost estimation is essential for project success. Techniques such as analogous estimating, parametric estimating, and bottom-up estimating can be used to forecast project costs based on historical data and expert judgment. Rapid Innovation leverages AI algorithms to enhance the accuracy of these estimations, allowing clients to make informed financial decisions. Tools like construction cost management software and multi cloud cost management tools can also aid in this process. For detailed insights on estimating blockchain projects, visit blockchain project estimation.
- Budgeting: Once costs are estimated, the next step is to create a budget. This budget serves as a baseline for measuring project performance and includes all costs associated with the project, such as labor, materials, and overhead. By utilizing blockchain technology, Rapid Innovation ensures transparency and traceability in budget allocation, fostering trust among stakeholders. Software solutions like jira software pricing and trello premium cost can help in effective budgeting.
- Controlling: Cost control involves monitoring project expenditures and performance against the budget. This includes tracking actual costs, analyzing variances, and implementing corrective actions when necessary to keep the project on track. Our AI-driven analytics tools provide real-time insights, enabling proactive adjustments to maintain budget adherence. Tools such as datadog cloud cost management and finops tools can enhance this process.
- Tools and Techniques: Various tools can assist in cost management, including: Â
- Earned Value Management (EVM) for tracking project performance.
- Cost-benefit analysis to evaluate the financial viability of project decisions.
- Software solutions for budgeting and forecasting, which can be integrated with AI and blockchain for enhanced functionality. Options like hootsuite pricing and sprout social pricing can be considered for project management needs.
- Importance: Effective cost management leads to: Â
- Improved financial performance.
- Enhanced decision-making capabilities.
- Increased stakeholder satisfaction.
8. Performance Measurement
Performance measurement is the process of evaluating the efficiency and effectiveness of a project or organization. It involves collecting data, analyzing performance metrics, and making informed decisions to improve outcomes.
- Purpose: The primary goal of performance measurement is to assess progress toward achieving project objectives. It helps identify areas for improvement and ensures that resources are being used effectively.
- Components: Key components of performance measurement include: Â
- Setting clear objectives and goals.
- Defining performance metrics and indicators.
- Collecting and analyzing data.
- Reporting findings to stakeholders.
- Benefits: Implementing a robust performance measurement system can lead to: Â
- Enhanced accountability among team members.
- Better alignment of projects with organizational goals.
- Increased transparency and communication with stakeholders.
8.1. Key Performance Indicators
Key Performance Indicators (KPIs) are quantifiable measures used to evaluate the success of an organization, project, or individual in meeting objectives. KPIs provide a clear framework for assessing performance and making data-driven decisions.
- Types of KPIs: KPIs can be categorized into several types, including: Â
- Financial KPIs: Measure financial performance, such as revenue growth, profit margins, and return on investment (ROI).
- Operational KPIs: Focus on the efficiency of processes, such as cycle time, throughput, and resource utilization.
- Customer KPIs: Assess customer satisfaction and engagement, including Net Promoter Score (NPS) and customer retention rates.
- Project KPIs: Evaluate project-specific metrics, such as schedule variance, cost variance, and project completion rates.
- Selecting KPIs: When choosing KPIs, consider the following: Â
- Relevance to organizational goals.
- Measurability and availability of data.
- Actionability to drive improvements.
- Implementation: To effectively implement KPIs: Â
- Establish a baseline for comparison.
- Set targets for each KPI.
- Regularly review and adjust KPIs as needed to reflect changing priorities.
- Benefits of KPIs: Utilizing KPIs can lead to: Â
- Improved decision-making based on data.
- Enhanced performance tracking and accountability.
- Greater alignment of team efforts with strategic objectives.
In conclusion, effective cost management and performance measurement, including the use of KPIs, are essential for the success of any project or organization. By focusing on these areas, organizations can ensure they are operating efficiently and effectively, ultimately leading to better outcomes and increased stakeholder satisfaction. Rapid Innovation is committed to providing the tools and expertise necessary to help clients achieve these goals through innovative AI and blockchain solutions.
8.2. ROI Analysis
Return on Investment (ROI) analysis is a critical component in evaluating the effectiveness of any project or initiative. It helps organizations determine the financial return generated from their investments relative to the costs incurred.
- ROI is calculated using the formula:
language="language-plaintext"``` -a1b2c3- Â ROI = (Net Profit / Cost of Investment) x 100
- A positive ROI indicates that the investment has generated more value than it cost, while a negative ROI suggests a loss.
- Key factors to consider in ROI analysis include: Â
- Initial costs: All expenses related to the project, including labor, materials, and overhead.
- Ongoing costs: Recurring expenses that may arise during the project's lifecycle.
- Revenue generation: The income produced as a result of the investment.
- Timeframe: The period over which the ROI is calculated, as longer timeframes can affect the overall return.
At Rapid Innovation, we leverage advanced AI and Blockchain technologies to enhance ROI for our clients. For instance, by implementing AI-driven analytics, we help businesses identify inefficiencies and optimize operations, leading to significant cost savings. Additionally, our Blockchain solutions ensure transparency and security in transactions, which can reduce fraud and increase trust, ultimately driving higher revenue generation.
Conducting a thorough ROI analysis allows organizations to make informed decisions about future investments, prioritize projects, and allocate resources effectively. It also provides a clear picture of the financial health of a project, enabling stakeholders to assess its viability. This includes understanding the analysis of return on investment and utilizing tools like the Dupont formula ROI and financial ROI analysis to gain deeper insights. Furthermore, ROI analysis marketing strategies can be developed to maximize returns on marketing campaigns, while social return on investment analysis can help evaluate the broader impacts of investments.
8.3. Quality Metrics
Quality metrics are essential tools for measuring the performance and effectiveness of processes, products, or services. They help organizations ensure that they meet customer expectations and maintain high standards.
- Common quality metrics include: Â
- Defect rates: The number of defects per unit produced, indicating the quality of manufacturing processes.
- Customer satisfaction scores: Surveys and feedback that gauge how well a product or service meets customer needs.
- First-pass yield: The percentage of products that meet quality standards without rework, reflecting process efficiency.
- Quality metrics can be categorized into: Â
- Process metrics: Focus on the efficiency and effectiveness of processes.
- Product metrics: Assess the quality of the final product.
- Service metrics: Evaluate the quality of service delivery.
Implementing quality metrics allows organizations to identify areas for improvement, enhance customer satisfaction, and reduce costs associated with poor quality. Regular monitoring of these metrics can lead to continuous improvement and a stronger competitive position in the market. At Rapid Innovation, we utilize AI to automate quality assessments, ensuring that our clients consistently meet high standards and improve their overall product quality.
8.4. Impact Assessment
Impact assessment is a systematic process used to evaluate the potential effects of a project or initiative on various stakeholders and the environment. It helps organizations understand the broader implications of their actions and make informed decisions.
- Key components of impact assessment include: Â
- Social impact: Evaluating how a project affects communities, including job creation, health, and education.
- Economic impact: Analyzing the financial implications, such as changes in local economies and business growth.
- Environmental impact: Assessing the potential effects on natural resources, ecosystems, and biodiversity.
- The impact assessment process typically involves: Â
- Scoping: Identifying the key issues and stakeholders to be considered.
- Data collection: Gathering relevant information through surveys, interviews, and research.
- Analysis: Evaluating the data to determine potential impacts and risks.
- Reporting: Documenting findings and recommendations for stakeholders.
Conducting a thorough impact assessment enables organizations to mitigate negative effects, enhance positive outcomes, and ensure compliance with regulations. It also fosters transparency and accountability, building trust with stakeholders and the community. Rapid Innovation employs Blockchain technology to enhance the integrity of impact assessments, ensuring that data is secure and verifiable, which is crucial for maintaining stakeholder trust.
8.5. Continuous Improvement
Continuous improvement is a systematic, ongoing effort to enhance products, services, or processes. It is a fundamental aspect of quality management and operational excellence. Organizations that embrace continuous improvement foster a culture of innovation and adaptability, which is essential in today’s fast-paced business environment.
- Focus on incremental changes rather than radical transformations. Â
- Utilize methodologies such as Lean, Six Sigma, and Kaizen strategies to streamline processes. Â
- Encourage employee involvement and feedback to identify areas for improvement. Â
- Implement regular training and development programs to enhance skills and knowledge. Â
- Use data-driven decision-making to assess performance and identify improvement opportunities. Â
- Establish key performance indicators (KPIs) to measure progress and success. Â
- Foster a culture of accountability where employees take ownership of their roles in the improvement process. Â
- Regularly review and adjust continuous improvement strategies based on feedback and performance metrics. Â
Organizations that prioritize continuous improvement can achieve significant benefits, including increased efficiency, reduced waste, and enhanced customer satisfaction. By creating a culture that values ongoing development, businesses can remain competitive and responsive to market changes. At Rapid Innovation, we leverage AI and Blockchain technologies to facilitate this continuous improvement process, ensuring that our clients can adapt swiftly to evolving market demands and optimize their operational workflows through continuous improvement strategic plans and process improvement strategies.
9. Future Trends and Evolution
The future of business and technology is rapidly evolving, driven by advancements in various fields. Understanding these trends is crucial for organizations looking to stay ahead of the curve.
- Increased integration of technology in everyday operations. Â
- Greater emphasis on sustainability and corporate social responsibility. Â
- The rise of remote work and digital collaboration tools. Â
- Enhanced focus on customer experience and personalization. Â
- The growing importance of data analytics and business intelligence. Â
- Expansion of e-commerce and digital marketing strategies. Â
- Adoption of agile methodologies to improve project management and responsiveness. Â
As these trends continue to evolve, organizations must adapt their strategies to leverage new opportunities and address emerging challenges.
9.1. Advanced AI Capabilities
Advanced AI capabilities are transforming industries by enabling organizations to automate processes, enhance decision-making, and improve customer interactions. The integration of AI technologies is reshaping the business landscape, offering numerous advantages.
- Machine learning algorithms can analyze vast amounts of data to identify patterns and trends. Â
- Natural language processing (NLP) allows for improved communication between humans and machines. Â
- AI-driven chatbots enhance customer service by providing instant responses and support. Â
- Predictive analytics helps businesses forecast demand and optimize inventory management. Â
- Robotic process automation (RPA) streamlines repetitive tasks, freeing up human resources for more strategic work. Â
- AI can enhance cybersecurity measures by detecting anomalies and potential threats in real-time. Â
- Personalization engines powered by AI can tailor marketing efforts to individual customer preferences. Â
As organizations continue to harness advanced AI capabilities, they can drive innovation, improve operational efficiency, and create more personalized experiences for their customers. Embracing these technologies will be essential for staying competitive in the future. Rapid Innovation is committed to guiding clients through this transformative journey, ensuring they maximize their ROI by effectively integrating AI and Blockchain solutions into their business strategies, including continuous quality improvement (CQI) strategies to optimize their practice.
9.2. Edge Computing Integration
Edge computing is a distributed computing paradigm that brings computation and data storage closer to the location where it is needed. This integration is crucial for enhancing the performance of applications, especially those requiring real-time data processing, such as mobile edge computing.
- Reduces latency: By processing data near the source, edge computing minimizes the time it takes for data to travel to a centralized cloud server. This is particularly beneficial for applications like autonomous vehicles and smart manufacturing, where Rapid Innovation can implement tailored edge solutions to optimize performance and responsiveness. For instance, mobile edge computing 5G can significantly enhance the responsiveness of these applications.
- Bandwidth efficiency: Edge computing reduces the amount of data that needs to be sent to the cloud, conserving bandwidth and lowering costs. This is essential for IoT devices that generate massive amounts of data. Rapid Innovation can help clients design and deploy edge architectures that maximize bandwidth efficiency, leading to significant cost savings. Examples of edge computing in IoT include smart sensors and connected devices that process data locally.
- Enhanced security: By keeping sensitive data closer to its source, edge computing can reduce the risk of data breaches during transmission. Local processing can also implement security measures tailored to specific environments. Rapid Innovation's expertise in security protocols ensures that edge solutions are robust and compliant with industry standards, particularly in industrial edge computing scenarios.
- Scalability: Edge computing allows organizations to scale their operations more efficiently. As the number of connected devices grows, edge solutions can be deployed incrementally without overhauling existing infrastructure. Rapid Innovation assists clients in developing scalable edge strategies that align with their growth objectives, including the integration of fog and edge computing.
- Real-time analytics: Businesses can leverage edge computing for real-time data analysis, enabling quicker decision-making and accelerating innovation.
9.5. Technology Convergence
Technology convergence refers to the merging of distinct technologies into a unified system that enhances functionality and user experience. This phenomenon is increasingly prevalent in various sectors, including telecommunications, computing, and media. The integration of different technologies leads to innovative solutions that can improve efficiency and productivity. Examples include smartphones, which combine telecommunication, computing, and multimedia capabilities into a single device. The Internet of Things (IoT) is another prime example, where everyday objects are connected to the internet, allowing for data exchange and automation. Convergence can lead to cost savings by reducing the need for multiple devices and systems. It fosters collaboration across industries, enabling companies to leverage each other's strengths and create new business models. The rise of cloud computing has also facilitated technology convergence, allowing for seamless access to data and applications from various devices.
At Rapid Innovation, we harness the power of technology convergence to deliver tailored AI and Blockchain solutions that drive efficiency and enhance user experiences. For instance, by integrating AI algorithms with Blockchain technology, we help clients create secure and transparent systems that streamline operations and improve decision-making processes. Our partnerships with companies like converge technology solutions and nutanix hyperconverged allow us to provide cutting-edge solutions in hyperconverged infrastructure. As technology continues to evolve, the trend of convergence is expected to accelerate, leading to even more integrated solutions that enhance user experiences and drive innovation. We also keep an eye on industry standards, such as the gartner hyperconverged magic quadrant 2021, to ensure we are at the forefront of technology convergence. Additionally, our expertise in adaptive AI development allows us to create solutions that are not only innovative but also adaptable to the changing technological landscape.
10. Best Practices and Guidelines
Implementing best practices and guidelines is essential for organizations to ensure effective operations, compliance, and risk management. These practices provide a framework for decision-making and help maintain consistency across various processes.
- Establish clear objectives and goals to guide the implementation of best practices. Â
- Involve stakeholders in the development of guidelines to ensure buy-in and relevance. Â
- Regularly review and update practices to adapt to changing regulations and market conditions. Â
- Provide training and resources to employees to ensure they understand and can effectively implement the guidelines. Â
- Monitor and measure the effectiveness of practices to identify areas for improvement. Â
- Foster a culture of continuous improvement where feedback is encouraged and acted upon. Â
By adhering to best practices and guidelines, organizations can enhance their operational efficiency, reduce risks, and improve overall performance.
10.1. Data Governance
Data governance is a critical aspect of managing an organization's data assets. It involves the establishment of policies, procedures, and standards to ensure data quality, security, and compliance. Effective data governance helps organizations maintain the integrity and accuracy of their data. It ensures that data is accessible to authorized users while protecting sensitive information from unauthorized access. A well-defined data governance framework includes roles and responsibilities for data stewardship, data ownership, and data management. Organizations should implement data quality measures to regularly assess and improve the reliability of their data. Compliance with regulations such as GDPR and HIPAA is a key component of data governance, ensuring that organizations meet legal requirements for data handling. Regular audits and assessments can help identify gaps in data governance practices and provide opportunities for improvement.
By prioritizing data governance, organizations can enhance their decision-making capabilities, mitigate risks, and build trust with stakeholders. At Rapid Innovation, we assist clients in establishing robust data governance frameworks that align with their business objectives, ensuring they can leverage their data assets effectively while maintaining compliance and security. Our expertise in converge technology group and converge it solution further strengthens our ability to support clients in this area.
10.2. Model Management
Model management is a critical aspect of machine learning and artificial intelligence development. It involves the systematic organization, versioning, and deployment of models to ensure they perform optimally in production environments. Effective model management, particularly in ai model management, can significantly enhance the efficiency and reliability of AI systems, ultimately leading to greater ROI for businesses.
- Version Control: Keeping track of different versions of models is essential. This allows teams to revert to previous versions if a new model underperforms or introduces errors, minimizing downtime and ensuring consistent performance.
- Documentation: Comprehensive documentation of model parameters, training data, and performance metrics is vital. This ensures transparency and facilitates collaboration among team members, enabling quicker decision-making and reducing the time to market for new features.
- Monitoring and Maintenance: Continuous monitoring of model performance is necessary to detect any degradation over time. Regular updates and retraining may be required to maintain accuracy, ensuring that the AI systems remain aligned with evolving business needs.
- Deployment Strategies: Different deployment strategies, such as A/B testing or canary releases, can help in assessing model performance in real-world scenarios before full-scale deployment. This approach minimizes risks and allows for data-driven adjustments.
- Collaboration Tools: Utilizing tools like MLflow or DVC can streamline the model management process, making it easier to track experiments and share results across teams. This enhances productivity and fosters innovation, ultimately contributing to a higher return on investment. For more insights on responsible practices, refer to the ethical AI development guide.
10.3. Security Protocols
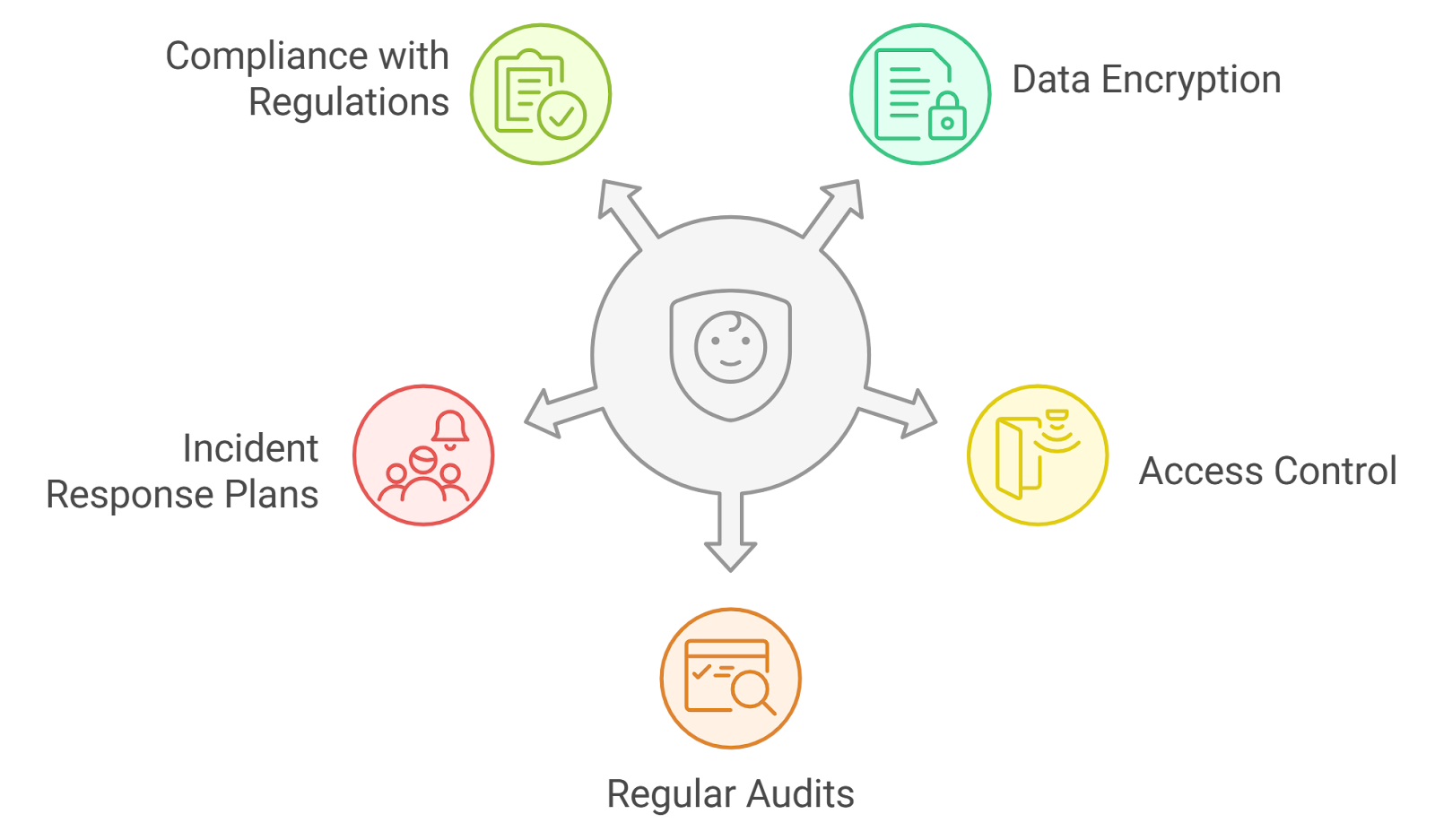
‍
Security protocols are essential in safeguarding AI systems and the data they utilize. As AI becomes more integrated into various sectors, the need for robust security measures has never been more critical.
- Data Encryption: Encrypting sensitive data both at rest and in transit protects it from unauthorized access. This is particularly important for personal data and proprietary information, ensuring compliance and building trust with clients.
- Access Control: Implementing strict access controls ensures that only authorized personnel can interact with AI systems. Role-based access control (RBAC) can help manage permissions effectively, reducing the risk of data breaches.
- Regular Audits: Conducting regular security audits can identify vulnerabilities within AI systems. This proactive approach helps in mitigating risks before they can be exploited, safeguarding both the organization and its clients.
- Incident Response Plans: Having a well-defined incident response plan is crucial for addressing security breaches. This plan should outline steps for containment, eradication, and recovery, ensuring business continuity and minimizing financial losses.
- Compliance with Regulations: Adhering to regulations such as GDPR or HIPAA is essential for organizations handling sensitive data. Compliance not only protects data but also builds trust with users, enhancing the organization's reputation and market position.
10.4. Ethical Framework
An ethical framework is vital for guiding the development and deployment of AI technologies. It ensures that AI systems are designed and used responsibly, considering their impact on society.
- Fairness and Bias Mitigation: AI systems should be designed to minimize bias and ensure fairness. This involves using diverse training datasets and regularly testing models for biased outcomes, which can enhance user trust and satisfaction.
- Transparency: Providing transparency in AI decision-making processes helps users understand how decisions are made. This can be achieved through explainable AI techniques, fostering user confidence and promoting ethical practices.
- Accountability: Establishing clear accountability for AI systems is crucial. Organizations should define who is responsible for the outcomes of AI decisions and ensure that there are mechanisms for redress, reinforcing ethical standards and corporate responsibility.
- User Privacy: Respecting user privacy is a fundamental ethical consideration. Organizations should implement data minimization practices and obtain informed consent for data usage, which can enhance customer loyalty and brand integrity.
- Social Impact Assessment: Conducting assessments to evaluate the potential social impact of AI technologies can help identify risks and benefits. This proactive approach fosters responsible innovation, aligning business goals with societal values and enhancing overall ROI, particularly in model management in ai.
10.5. Documentation Standards
Documentation standards are essential for ensuring consistency, clarity, and quality in the creation and management of documents across various fields. These standards help organizations maintain a uniform approach to documentation, which is crucial for effective communication, compliance, and operational efficiency. Here are some key aspects of documentation standards:
- Purpose and Importance Â
- Establishes a clear framework for creating documents.
- Enhances readability and comprehension for users.
- Facilitates compliance with legal and regulatory requirements.
- Promotes consistency across different departments and teams.
- Types of Documentation Â
- Technical Documentation: Includes user manuals, installation guides, and API documentation, particularly relevant in AI and Blockchain projects where precise technical details are crucial for implementation.
- Policy and Procedure Documents: Outlines organizational policies, procedures, and guidelines, ensuring that all stakeholders are aligned with the operational framework.
- Training Materials: Encompasses training manuals, e-learning content, and onboarding documents, essential for equipping teams with the knowledge to leverage AI and Blockchain technologies effectively.
- Reports and Proposals: Involves business reports, project proposals, and research findings, which are vital for securing stakeholder buy-in and demonstrating ROI.
- Key Elements of Documentation Standards Â
- Structure: Defines the layout and organization of documents, including headings, subheadings, and sections, which aids in navigating complex AI and Blockchain documentation.
- Style: Specifies the tone, language, and formatting to be used, ensuring a professional appearance that reflects the innovative nature of Rapid Innovation.
- Terminology: Establishes a consistent vocabulary to avoid confusion and misinterpretation, particularly important in technical fields like AI and Blockchain.
- Version Control: Implements a system for tracking changes and updates to documents, ensuring users access the most current information, which is critical in fast-evolving tech environments.
- Best Practices for Implementing Documentation Standards Â
- Develop a Style Guide: Create a comprehensive style guide that outlines formatting, language, and design principles tailored to the specific needs of AI and Blockchain documentation.
- Train Staff: Provide training sessions for employees on documentation standards and best practices, ensuring that all team members are equipped to produce high-quality documentation, including the use of an iso 9001 internal audit checklist.
- Use Templates: Develop standardized templates for different types of documents, such as a standard invoice template or an iso audit checklist, to streamline the creation process, enhancing efficiency and consistency.
- Regular Reviews: Schedule periodic reviews of documentation to ensure compliance with established standards and to update outdated information, maintaining relevance in a rapidly changing landscape.
- Tools and Technologies Â
- Document Management Systems (DMS): Utilize DMS to store, organize, and manage documents efficiently, facilitating easy access to critical information for AI and Blockchain projects.
- Collaboration Tools: Implement tools that facilitate collaboration among team members during the documentation process, fostering innovation and teamwork.
- Version Control Software: Use version control systems to track changes and maintain document integrity, ensuring that all stakeholders are working with the latest information.
- Compliance and Regulatory Considerations Â
- Adhere to industry-specific regulations and standards, such as ISO, FDA, or HIPAA, which may dictate specific documentation requirements, particularly in sectors where AI and Blockchain are applied, including the use of auditing guidelines and gaap disclosure checklist.
- Ensure that documentation is accessible to all relevant stakeholders, including employees, clients, and regulatory bodies, promoting transparency and trust.
- Continuous Improvement Â
- Encourage feedback from users to identify areas for improvement in documentation practices, fostering a culture of continuous enhancement.
- Stay updated on industry trends and advancements in documentation technology to enhance efficiency and effectiveness, ensuring that Rapid Innovation remains at the forefront of the industry.
By adhering to established documentation standards, organizations can improve communication, enhance operational efficiency, and ensure compliance with regulatory requirements. This structured approach to documentation not only benefits internal processes but also enhances the overall user experience, ultimately contributing to greater ROI for clients leveraging AI and Blockchain solutions, while also considering standards for comparisons in financial statement analysis and the use of an audit data standards order to cash.