Table Of Contents
Category
legal
Artificial Intelligence
IoT
Blockchain
1. Introduction to AI-Driven Academic Performance Prediction
Artificial Intelligence (AI) is transforming various sectors, and education is no exception. AI-driven academic performance prediction utilizes advanced algorithms and data analytics to forecast students' academic outcomes. This innovative approach is revolutionizing educational intelligence by providing insights that can enhance teaching methods, improve student engagement, and ultimately boost academic success.
- AI systems analyze vast amounts of data, including:
- Student demographics
- Historical academic performance
- Attendance records
- Engagement metrics from online learning platforms
- The predictive models can identify at-risk students early, allowing educators to intervene proactively.
- By leveraging machine learning techniques, these systems continuously improve their accuracy over time, adapting to new data and trends.
- The integration of AI in education not only supports personalized learning experiences but also helps institutions allocate resources more effectively.
- Educational institutions can use these predictions to tailor interventions, such as:
- Targeted tutoring programs
- Customized learning plans
- Enhanced support services
At Rapid Innovation, we specialize in implementing AI-driven academic performance prediction solutions that empower educational institutions to harness the full potential of their data. By partnering with us, clients can expect to achieve greater ROI through improved student outcomes and optimized resource allocation. AI-driven academic performance prediction is not just about numbers; it’s about understanding the unique needs of each student and fostering an environment where they can thrive. As educational institutions increasingly adopt these technologies, the potential for improved academic outcomes becomes more significant.
Refer to the image for a visual representation of AI-driven academic performance prediction and its components:
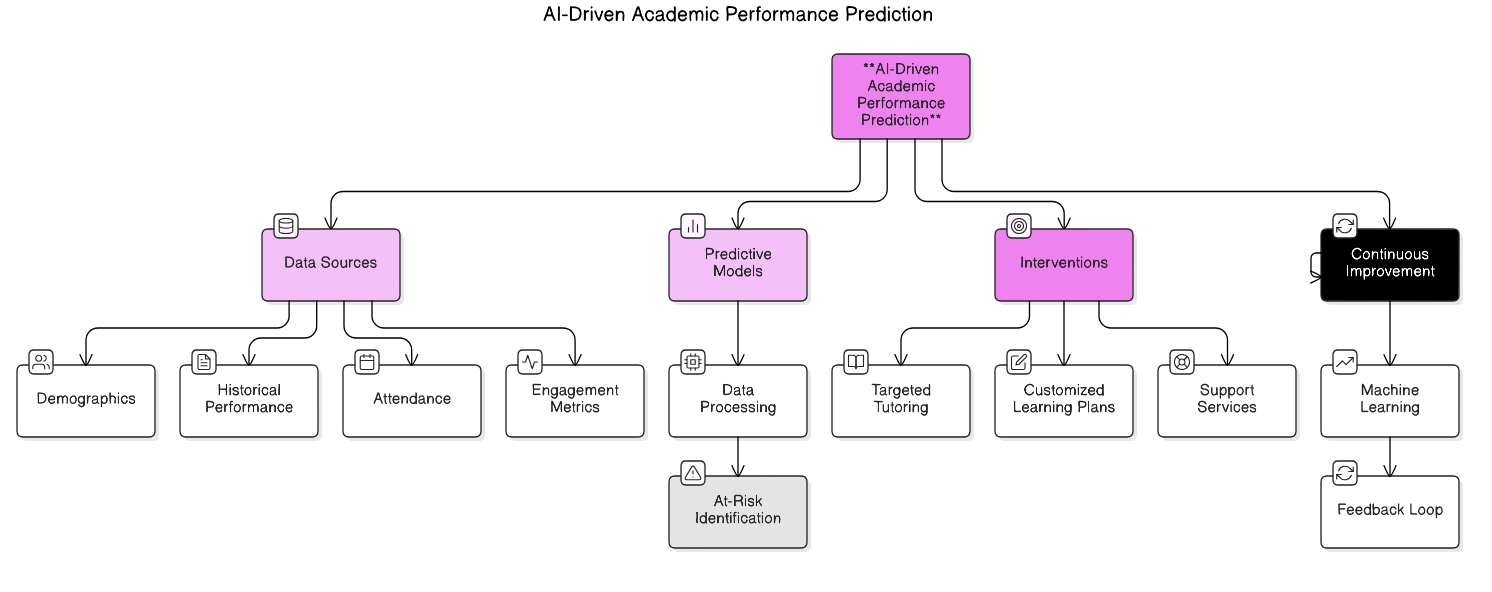
1.1. Defining Academic Performance Prediction
Academic performance prediction refers to the process of forecasting a student's future academic success based on various indicators and data points. This predictive analysis can help educators, administrators, and policymakers make informed decisions to enhance student outcomes.
- Key components of academic performance prediction include:
- Historical academic data: Grades, test scores, and attendance records.
- Behavioral indicators: Participation in class, engagement in extracurricular activities, and social interactions.
- Demographic factors: Socioeconomic status, parental education levels, and geographic location.
The goal of academic performance prediction is to identify students who may be at risk of underperforming and to implement timely interventions. Predictive models often utilize machine learning algorithms to analyze vast amounts of data, allowing for more accurate predictions and personalized learning experiences. This includes predicting student academic performance and predicting students' academic performance using artificial neural networks. At Rapid Innovation, we leverage our expertise in AI to develop tailored predictive models that empower educational institutions to enhance student success and achieve greater ROI.
1.2. Evolution of Predictive Analytics in Education
Predictive analytics in education has evolved significantly over the past few decades, driven by advancements in technology and data science.
- Early stages:
- Initially, educational institutions relied on simple statistical methods to analyze student performance.
- Data collection was limited, often focusing on standardized test scores and grades.
- Technological advancements:
- The rise of big data and cloud computing has enabled schools to collect and analyze larger datasets.
- Machine learning and artificial intelligence have transformed predictive analytics, allowing for more sophisticated models that can identify patterns and trends.
- Current trends:
- Many educational institutions now use predictive analytics to inform curriculum development, resource allocation, and student support services.
- The integration of learning management systems (LMS) and student information systems (SIS) has streamlined data collection and analysis processes.
- Future directions:
- As predictive analytics continues to evolve, there is a growing emphasis on ethical considerations, data privacy, and the need for transparency in how data is used.
At Rapid Innovation, we stay at the forefront of these trends, ensuring our clients benefit from the latest advancements in predictive analytics to optimize their educational strategies and improve overall performance. This includes academic performance prediction and predicting student performance by using data mining methods for classification.
1.3. The Role of AI in Educational Insights
Artificial intelligence (AI) plays a crucial role in generating insights that can enhance educational practices and improve student outcomes.
- Data analysis:
- AI algorithms can process vast amounts of data quickly, identifying trends and correlations that may not be apparent through traditional analysis. This capability allows educators to gain insights into student learning patterns, helping to tailor instruction to meet individual needs.
- Personalized learning:
- AI can facilitate personalized learning experiences by adapting content and assessments based on a student's performance and learning style. This approach can lead to increased engagement and motivation among students.
- Predictive modeling:
- AI-driven predictive models can forecast student performance, enabling early intervention for those at risk of falling behind. These models can also help identify effective teaching strategies and resources that contribute to student success.
- Continuous improvement:
- AI can support ongoing evaluation and improvement of educational programs by analyzing outcomes and providing actionable insights. This data-driven approach fosters a culture of continuous improvement within educational institutions.
By integrating AI solutions into educational frameworks, Rapid Innovation helps institutions not only to enhance their teaching methodologies but also to achieve significant returns on investment through improved student outcomes and operational efficiencies.
Refer to the image for a visual representation of the key concepts discussed in academic performance prediction.

1.4. Ethical Considerations and Challenges
Ethical considerations in education, particularly in assessment, are crucial for ensuring fairness, transparency, and integrity. As educational systems evolve, several ethical challenges arise that educators and policymakers must address.
- Data Privacy: With the increasing use of technology in assessments, student data privacy is a significant concern. Schools must ensure that personal information is protected and used responsibly, adhering to regulations like FERPA in the U.S. and GDPR in Europe. Rapid Innovation emphasizes the importance of implementing robust data protection measures in AI and blockchain solutions to safeguard sensitive information.
- Equity and Access: There is a risk that technology-driven assessments, including educational assessment technology, may exacerbate existing inequalities. Students from disadvantaged backgrounds may lack access to necessary resources, such as reliable internet or devices, leading to disparities in performance. Rapid Innovation can assist educational institutions in developing inclusive technologies that ensure equitable access to assessment tools for all students.
- Bias in Algorithms: Many assessment tools utilize algorithms that can inadvertently perpetuate biases. If the data used to train these algorithms is biased, the outcomes can be unfair. Continuous monitoring and adjustment of these systems are essential to mitigate bias. Rapid Innovation offers AI solutions that include bias detection and correction mechanisms, ensuring fair assessment outcomes.
- Informed Consent: Students and parents should be fully informed about how assessment data will be used, including understanding the implications of their data being collected and analyzed. Rapid Innovation advocates for transparent data usage policies and consent management systems that empower users to make informed decisions.
- Accountability: As assessments become more automated, questions arise about accountability. It is essential to determine who is responsible for the outcomes of an assessment, as educators, institutions, and technology providers must share this responsibility. Rapid Innovation promotes accountability frameworks within its AI and blockchain solutions, ensuring that all stakeholders are aware of their roles and responsibilities.
1.5. Paradigm Shift in Educational Assessment

The landscape of educational assessment is undergoing a significant transformation, driven by technological advancements and changing educational philosophies. This paradigm shift is characterized by several key trends.
- From Standardized Testing to Personalized Assessment: Traditional standardized tests are being replaced by more personalized assessment methods that focus on individual learning paths and competencies rather than a one-size-fits-all approach.
- Integration of Formative Assessments: Continuous assessment through formative methods is gaining traction, allowing educators to monitor student progress in real-time and adjust instruction accordingly, fostering a more supportive learning environment.
- Use of Technology: Digital tools and platforms, including ict and assessment in learning, are revolutionizing how assessments are conducted. Online assessments can provide immediate feedback, making it easier for students to understand their strengths and weaknesses.
- Competency-Based Assessment: There is a growing emphasis on assessing students based on their competencies rather than time spent in class. This shift encourages mastery of skills and knowledge, allowing students to progress at their own pace.
- Collaboration and Peer Assessment: Collaborative assessments, where students evaluate each other's work, are becoming more common. This method enhances critical thinking and fosters a sense of community among learners.
2. Foundational Technologies
Foundational technologies play a pivotal role in shaping the future of education and assessment. These technologies provide the infrastructure and tools necessary for innovative teaching and learning practices.
- Learning Management Systems (LMS): LMS platforms like Moodle and Canvas facilitate the organization and delivery of educational content, enabling educators to create, manage, and assess courses efficiently.
- Artificial Intelligence (AI): AI is transforming educational assessment by providing personalized learning experiences. AI-driven tools can analyze student performance data to offer tailored recommendations and support.
- Big Data Analytics: The use of big data in education allows for deeper insights into student learning patterns. By analyzing large datasets, educators can identify trends and make informed decisions to improve teaching strategies.
- Cloud Computing: Cloud technology enables easy access to educational resources and tools from anywhere, supporting remote learning and collaboration among students and educators.
- Gamification: Incorporating game elements into assessments can enhance student engagement. Gamification encourages active participation and can lead to improved learning outcomes.
- Virtual and Augmented Reality (VR/AR): VR and AR technologies provide immersive learning experiences, simulating real-world scenarios that allow students to apply their knowledge in practical contexts.
- Blockchain: Blockchain technology offers a secure way to store and verify educational credentials, helping to combat credential fraud and streamline the verification process for employers and institutions. Rapid Innovation leverages blockchain to enhance the security and integrity of educational records, ensuring that credentials are verifiable and tamper-proof.
By leveraging these foundational technologies, including technology and assessment in education and technology and student assessment, educational institutions can create more effective, engaging, and equitable assessment practices that meet the needs of diverse learners. Rapid Innovation is committed to helping clients navigate this transformative landscape, ensuring they achieve greater ROI through innovative solutions tailored to their specific needs.
Refer to the image for a visual representation of the ethical considerations and challenges in educational assessment.
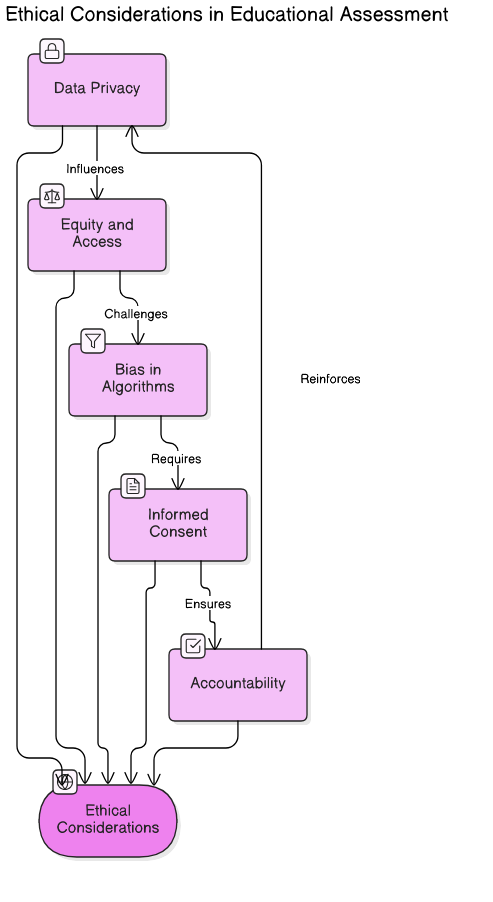
2.1. Machine Learning Fundamentals
Machine learning (ML) is a subset of artificial intelligence (AI) that focuses on the development of algorithms that allow computers to learn from and make predictions based on data. Understanding the fundamentals of machine learning is crucial for anyone looking to leverage this technology effectively, especially in a business context where Rapid Innovation can assist in achieving greater ROI.
- Types of Machine Learning:
- Supervised Learning: Involves training a model on a labeled dataset, where the outcome is known. Common algorithms include linear regression, decision trees, and support vector machines. Rapid Innovation utilizes supervised learning and supervised machine learning algorithms to enhance customer segmentation and targeting, leading to more effective marketing strategies.
- Unsupervised Learning: Deals with unlabeled data, aiming to find hidden patterns or intrinsic structures. Techniques include clustering (e.g., K-means) and dimensionality reduction (e.g., PCA). Our expertise in unsupervised learning and methods of machine learning helps clients uncover valuable insights from their data, optimizing product offerings and improving customer experiences.
- Reinforcement Learning: Focuses on training models to make sequences of decisions by rewarding desired behaviors and punishing undesired ones. This is often used in robotics and game playing. Rapid Innovation applies reinforcement learning in dynamic pricing models, allowing businesses to adapt to market changes in real-time.
- Key Concepts:
- Features and Labels: Features are the input variables used for prediction, while labels are the output variables. Understanding these concepts is vital for effective model training and deployment.
- Training and Testing: The dataset is typically split into training and testing sets to evaluate the model's performance. Rapid Innovation emphasizes rigorous testing to ensure models perform reliably in production environments.
- Overfitting and Underfitting: Overfitting occurs when a model learns noise in the training data, while underfitting happens when it fails to capture the underlying trend. Our team at Rapid Innovation employs techniques to balance model complexity, ensuring optimal performance.
- Applications:
- Image recognition
- Fraud detection
- Recommendation systems
By leveraging these machine learning fundamentals, including machine learning techniques and methods in machine learning, Rapid Innovation empowers clients to harness data-driven insights, ultimately leading to improved decision-making and increased ROI.
2.2. Predictive Analytics Techniques
Predictive analytics involves using statistical algorithms and machine learning techniques to identify the likelihood of future outcomes based on historical data. This field is essential for businesses looking to make data-driven decisions, and Rapid Innovation specializes in implementing these techniques to drive success.
- Common Techniques:
- Regression Analysis: Used to predict a continuous outcome variable based on one or more predictor variables. Linear regression is a popular method. Our team utilizes regression analysis to forecast sales trends, enabling clients to optimize inventory management.
- Time Series Analysis: Involves analyzing time-ordered data points to forecast future values. Techniques include ARIMA and exponential smoothing. Rapid Innovation employs time series analysis to help clients anticipate market fluctuations and adjust strategies accordingly.
- Classification: A method used to predict categorical outcomes. Algorithms like logistic regression, decision trees, and random forests are commonly employed. We leverage classification techniques, including machine learning classification and ml classification, to enhance customer targeting and improve conversion rates.
- Data Sources:
- Historical sales data
- Customer behavior data
- Market trends
- Benefits:
- Improved decision-making
- Enhanced customer insights
- Increased operational efficiency
Through our expertise in predictive analytics, including machine learning classification algorithms, Rapid Innovation enables clients to make informed decisions that drive growth and maximize ROI.
2.3. Natural Language Processing
Natural Language Processing (NLP) is a branch of artificial intelligence that focuses on the interaction between computers and humans through natural language. It enables machines to understand, interpret, and respond to human language in a valuable way, and Rapid Innovation harnesses NLP to enhance client engagement.
- Core Components:
- Tokenization: The process of breaking down text into individual words or phrases. This foundational step is crucial for effective text analysis.
- Sentiment Analysis: Determines the emotional tone behind a series of words, often used in social media monitoring. Rapid Innovation utilizes sentiment analysis to gauge customer feedback and improve brand perception.
- Named Entity Recognition (NER): Identifies and classifies key entities in text, such as names, organizations, and locations. Our NER capabilities help clients extract actionable insights from unstructured data.
- Techniques:
- Text Classification: Assigns predefined categories to text data, useful for spam detection and topic categorization. We implement text classification to streamline content moderation processes for our clients.
- Machine Translation: Automatically translates text from one language to another, as seen in Google Translate. Rapid Innovation offers machine translation solutions to help businesses expand their global reach.
- Chatbots and Virtual Assistants: Use NLP to understand user queries and provide relevant responses. Our chatbot solutions enhance customer service efficiency, leading to improved customer satisfaction.
- Applications:
- Customer service automation
- Content recommendation
- Voice-activated assistants
Understanding these fundamental concepts in machine learning, including ensemble learning and ensemble techniques machine learning, predictive analytics, and natural language processing is essential for harnessing the power of data in today's technology-driven world. Rapid Innovation is committed to helping clients navigate these technologies to achieve their business goals efficiently and effectively.
Refer to the image for a visual representation of the concepts discussed in the Machine Learning Fundamentals section.
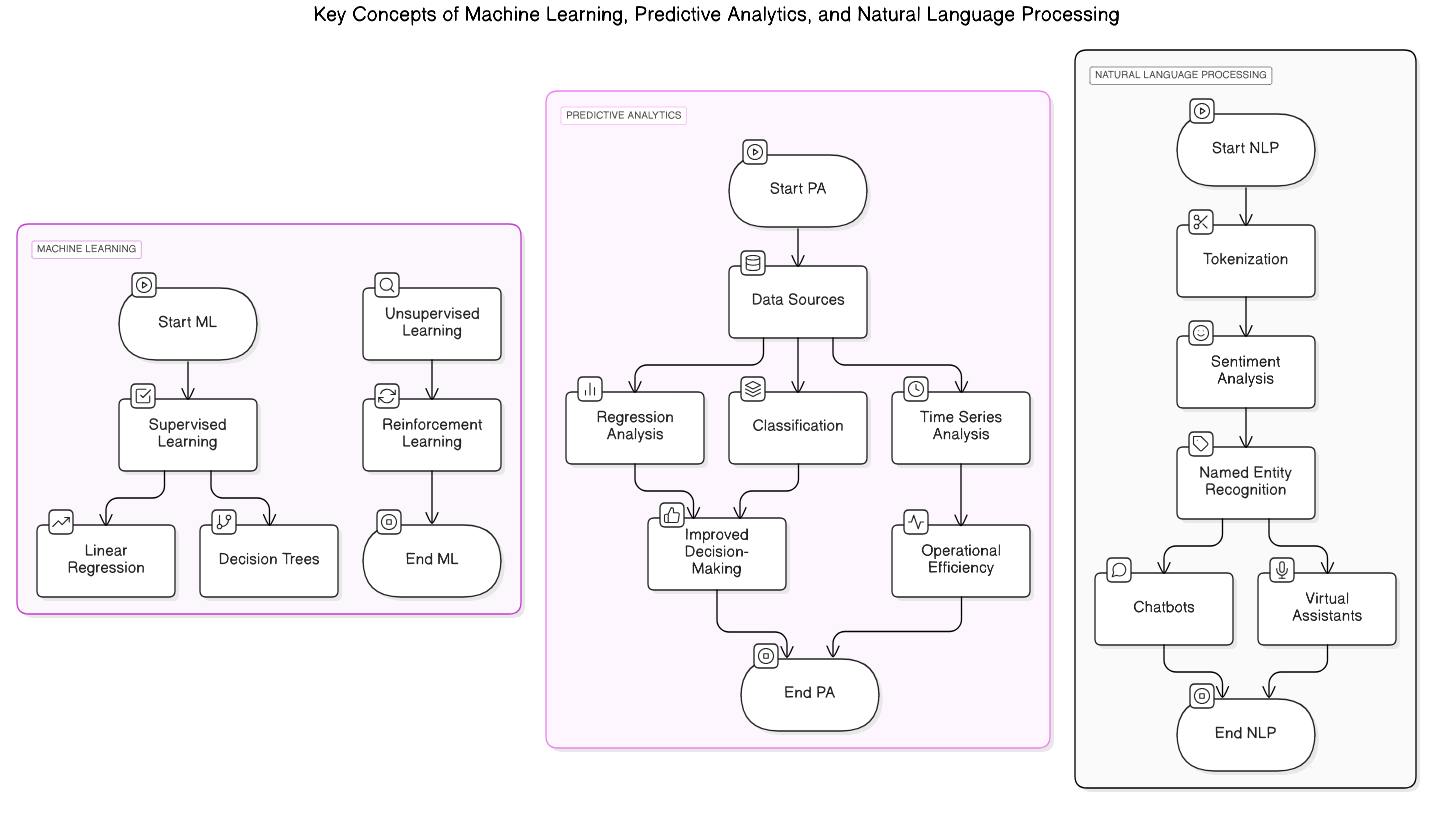
2.4. Data Mining and Pattern Recognition
Data mining is the process of discovering patterns and knowledge from large amounts of data. It involves using algorithms and statistical techniques to analyze data sets and extract valuable insights. Pattern recognition, a subset of data mining, focuses on identifying regularities and trends within data.
- Key Techniques:
- Classification: Assigning items in a dataset to target categories or classes.
- Clustering: Grouping a set of objects in such a way that objects in the same group are more similar than those in other groups.
- Association Rule Learning: Discovering interesting relations between variables in large databases, such as association rule mining in data mining.
- Applications:
- Market Basket Analysis: Understanding consumer purchasing behavior by analyzing transaction data, a common application of data mining techniques.
- Fraud Detection: Identifying unusual patterns that may indicate fraudulent activity.
- Image and Speech Recognition: Enhancing user experience in applications like virtual assistants and security systems.
- Tools and Technologies:
- Software: Tools like RapidMiner, KNIME, and Weka are popular for data mining tasks, including crisp DM data mining.
- Programming Languages: Languages such as Python and R offer libraries like Scikit-learn and caret for implementing data mining techniques.
At Rapid Innovation, we leverage data mining and pattern recognition to help clients uncover actionable insights from their data, leading to improved decision-making and greater ROI. For instance, our expertise in market basket analysis has enabled retail clients to optimize their inventory and marketing strategies, resulting in increased sales and customer satisfaction.
Data mining and pattern recognition are crucial in various fields, including finance, healthcare, and marketing, as they help organizations make data-driven decisions. The cross-industry standard process for data mining (CRISP-DM) is a widely used framework that guides the data mining process.
2.5. Neural Network Architectures
Neural networks are computational models inspired by the human brain, designed to recognize patterns and solve complex problems. They consist of interconnected nodes (neurons) organized in layers, which process input data and produce output.
- Types of Neural Networks:
- Feedforward Neural Networks: The simplest type, where connections between nodes do not form cycles.
- Convolutional Neural Networks (CNNs): Primarily used for image processing, they utilize convolutional layers to detect features.
- Recurrent Neural Networks (RNNs): Designed for sequential data, they have connections that loop back, making them suitable for tasks like language modeling.
- Key Features:
- Activation Functions: Functions like ReLU, sigmoid, and tanh determine the output of neurons.
- Backpropagation: A method for training neural networks by minimizing the error between predicted and actual outputs.
- Applications:
- Natural Language Processing: Used in chatbots and translation services.
- Autonomous Vehicles: Enabling real-time decision-making based on sensor data.
- Healthcare: Assisting in disease diagnosis and personalized medicine.
At Rapid Innovation, we harness the power of neural network architectures to develop advanced AI solutions tailored to our clients' needs. For example, our work in natural language processing has helped businesses enhance customer engagement through intelligent chatbots, leading to improved customer service and retention.
Neural network architectures have revolutionized machine learning, enabling advancements in artificial intelligence and deep learning.
2.6. Statistical Modeling Approaches
Statistical modeling involves creating mathematical representations of real-world processes to analyze and predict outcomes. It uses statistical methods to estimate relationships among variables and make inferences about populations based on sample data.
- Common Statistical Models:
- Linear Regression: Models the relationship between a dependent variable and one or more independent variables.
- Logistic Regression: Used for binary classification problems, predicting the probability of a categorical outcome.
- Time Series Analysis: Analyzes data points collected or recorded at specific time intervals to identify trends and seasonal patterns.
- Key Concepts:
- Hypothesis Testing: A method for testing assumptions about a population parameter.
- Confidence Intervals: A range of values that is likely to contain the population parameter with a certain level of confidence.
- ANOVA (Analysis of Variance): A statistical method used to compare means among three or more groups.
- Applications:
- Economics: Modeling economic indicators to forecast trends.
- Social Sciences: Analyzing survey data to understand public opinion.
- Environmental Science: Assessing the impact of variables on climate change.
At Rapid Innovation, we apply statistical modeling approaches to provide our clients with robust analytical frameworks that inform strategic decisions. For instance, our expertise in time series analysis has enabled clients in finance to accurately forecast market trends, enhancing their investment strategies and maximizing returns.
Statistical modeling approaches are essential for data analysis, providing a framework for making informed decisions based on empirical evidence. Data mining techniques play a significant role in enhancing these statistical models, allowing for more accurate predictions and insights.
3. Data Ecosystem for Performance Prediction
The data ecosystem for performance prediction is a complex network of various data sources and methodologies that work together to analyze and forecast outcomes. This ecosystem is crucial for educational institutions, businesses, and organizations aiming to enhance performance metrics and make informed decisions. At Rapid Innovation, we leverage advanced AI and Blockchain technologies to optimize this ecosystem, ensuring that our clients achieve their business goals efficiently and effectively.
3.1 Data Sources and Collection

Data sources play a pivotal role in performance prediction. The quality and variety of data collected can significantly influence the accuracy of predictions. Here are some key aspects of data sources and collection methods:
- Diversity of Data: Performance prediction relies on a mix of quantitative and qualitative data, including numerical data such as test scores and qualitative data such as student feedback. Our AI-driven analytics tools can process and analyze this diverse data to provide actionable insights, particularly in predicting student performance.
- Real-time Data: Collecting data in real-time allows for more accurate predictions, which can include attendance records, engagement metrics, and other dynamic indicators. Rapid Innovation employs real-time data processing capabilities to ensure that our clients can make timely decisions based on the latest information.
- Data Integration: Combining data from various sources enhances the predictive model. For instance, integrating academic records with behavioral data can provide a more comprehensive view of performance. Our Blockchain solutions ensure secure and seamless data integration, enhancing data integrity and trustworthiness.
3.1.1 Academic Records
Academic records are one of the most critical data sources in the performance prediction ecosystem, providing a wealth of information that can be analyzed to forecast future performance. Key components of academic records include:
- Grades and Test Scores: These are the most direct indicators of a student's academic performance. Analyzing trends in grades over time can help predict future success or challenges. Our AI algorithms can identify patterns and correlations that may not be immediately apparent, especially when predicting student performance with deep neural networks.
- Course Enrollment History: Understanding which courses a student has taken can provide insights into their strengths and weaknesses. For example, a student excelling in mathematics may be more likely to succeed in advanced STEM courses. Rapid Innovation can help institutions develop predictive models that leverage this data for personalized learning pathways.
- Attendance Records: Regular attendance is often correlated with better academic performance. Tracking attendance can help identify students at risk of underperforming. Our solutions can automate attendance tracking and provide real-time alerts for at-risk students.
- Extracurricular Activities: Participation in clubs, sports, and other activities can influence academic performance. Students engaged in extracurriculars often develop skills that contribute to their overall success. By analyzing this data, we can help institutions create holistic support systems for students.
- Demographic Information: Factors such as age, gender, and socioeconomic status can impact academic performance. Analyzing this data can help identify trends and tailor interventions. Our AI tools can segment this data to provide targeted insights for different demographic groups.
The effective use of academic records in performance prediction requires robust data collection methods. Institutions must ensure that data is collected consistently and accurately. This can involve:
- Automated Data Entry: Utilizing technology to automate the collection of academic records can reduce errors and save time. Rapid Innovation offers solutions that streamline data entry processes, enhancing efficiency.
- Data Privacy Compliance: Institutions must adhere to regulations regarding student data privacy, ensuring that sensitive information is protected. Our Blockchain technology provides a secure framework for data management, ensuring compliance with privacy regulations.
- Regular Updates: Keeping academic records up-to-date is essential for accurate predictions. Institutions should establish protocols for regularly reviewing and updating data. Our systems can facilitate automated updates, ensuring that data remains current and relevant.
In conclusion, the data ecosystem for performance prediction is multifaceted, with academic records serving as a foundational element. By leveraging diverse data sources and employing effective collection methods, organizations can enhance their predictive capabilities and ultimately improve performance outcomes. This includes understanding the performance of LSTM and BiLSTM in forecasting time series data. At Rapid Innovation, we are committed to helping our clients harness the power of AI and Blockchain to achieve greater ROI and drive success through our AI-driven digital twins.
3.1.2. Learning Management Systems
Learning Management Systems (LMS) are software applications designed to facilitate the administration, documentation, tracking, reporting, and delivery of educational courses or training programs. They play a crucial role in modern education, especially in online and blended learning environments.
- Centralized Learning: LMS provides a centralized platform where students can access course materials, submit assignments, and participate in discussions. This streamlines the learning process and enhances accessibility.
- Tracking Progress: Educators can monitor student progress through built-in analytics tools. This allows for timely interventions if a student is struggling, ensuring that no one falls behind.
- Customization: Many LMS platforms, such as learning management solutions and learning management systems, offer customizable features that cater to different learning styles. This personalization can improve student engagement and retention.
- Collaboration Tools: LMS often includes forums, chat rooms, and group project features, fostering collaboration among students. This interaction can enhance the learning experience and build a sense of community.
- Integration with Other Tools: Many LMS can integrate with other educational technologies, such as video conferencing tools and assessment software, creating a more cohesive learning environment.
At Rapid Innovation, we leverage AI to enhance LMS capabilities, enabling predictive analytics that can identify at-risk students and suggest personalized learning paths. Our blockchain solutions can ensure secure and transparent tracking of student progress and achievements, providing a trustworthy record for both educators and learners. For more information on our services, visit our AI EdTech Solutions and learn about the advantages and methods of application.
3.1.3. Student Interaction Metrics
Student interaction metrics are essential for understanding how students engage with course content and with each other. These metrics provide valuable insights into student behavior and can inform instructional strategies.
- Engagement Levels: Metrics such as time spent on tasks, participation in discussions, and completion rates can indicate how engaged students are with the material. High engagement often correlates with better learning outcomes.
- Interaction Frequency: Tracking how often students interact with peers and instructors can reveal patterns in communication. Frequent interactions can enhance understanding and retention of information.
- Content Access Patterns: Analyzing which resources students access most frequently can help educators identify which materials are most effective and which may need improvement.
- Feedback Mechanisms: Regular feedback from students about their learning experiences can be collected through surveys and polls. This data can guide course adjustments and improve overall satisfaction.
- Predictive Analytics: By analyzing interaction metrics, educators can predict which students may need additional support, allowing for proactive measures to enhance student success.
Rapid Innovation employs advanced AI algorithms to analyze these interaction metrics, providing educators with actionable insights that can lead to improved teaching strategies and enhanced student outcomes.
3.1.4. Psychological and Behavioral Data
Psychological and behavioral data encompasses a range of information that can provide insights into students' mental states, motivations, and learning behaviors. Understanding these factors is crucial for creating effective educational experiences.
- Motivation Levels: Assessing students' intrinsic and extrinsic motivations can help educators tailor their approaches. Motivated students are more likely to engage deeply with the material and persist through challenges.
- Learning Styles: Data on how students prefer to learn—whether through visual aids, hands-on activities, or auditory methods—can inform instructional design. Catering to diverse learning styles can enhance engagement and comprehension.
- Stress and Anxiety Indicators: Monitoring signs of stress or anxiety, such as changes in participation or performance, can help educators provide timely support. Creating a supportive environment can mitigate these issues.
- Behavioral Patterns: Analyzing attendance, participation, and submission rates can reveal behavioral trends. Identifying at-risk students early allows for targeted interventions.
- Emotional Well-being: Surveys and assessments can gauge students' emotional states, providing insights into their overall well-being. A positive emotional climate can significantly impact learning outcomes.
By integrating psychological and behavioral data with our AI-driven analytics, Rapid Innovation empowers educators to create tailored learning experiences that address individual student needs, ultimately leading to improved educational outcomes.
By leveraging Learning Management Systems, such as learning content management systems and enterprise learning management, student interaction metrics, and psychological and behavioral data, educators can create a more effective and supportive learning environment. These tools and insights enable a deeper understanding of student needs, ultimately leading to improved educational experiences and outcomes. Rapid Innovation stands ready to assist educational institutions in harnessing these technologies for greater efficiency and effectiveness in achieving their business goals.
3.2. Data Quality and Preprocessing
Data quality is crucial for any data-driven project, as it directly impacts the accuracy and reliability of the results. Poor quality data can lead to misleading insights and ineffective decision-making.
- Importance of Data Quality: High-quality data ensures that the analysis reflects true patterns and trends. It is essential for building robust models and achieving reliable outcomes, ultimately leading to greater ROI for businesses.
- Common Data Quality Issues:
- Incomplete data: Missing values can skew results.
- Inconsistent data: Variations in data formats can lead to confusion.
- Duplicate data: Redundant entries can inflate the dataset and distort analysis.
- Preprocessing Techniques:
- Data cleaning: Removing or correcting erroneous data entries.
- Data transformation: Normalizing or standardizing data to ensure consistency.
- Data integration: Combining data from different sources to create a unified dataset.
- Tools for Data Quality Assessment:
- Data profiling tools: Help identify data quality issues.
- ETL (Extract, Transform, Load) tools: Facilitate data cleaning and integration.
- Data quality assessment tools: Such as machine learning data quality assessment and data quality assessment tools excel can enhance the evaluation process.
- Routine data quality assessment tool: Ensures ongoing monitoring of data integrity.
Investing time in data quality and preprocessing can significantly enhance the effectiveness of subsequent analyses and models, allowing Rapid Innovation to help clients achieve their business goals efficiently. Techniques such as data quality evaluation and data integrity risk assessment are essential components of this process. Additionally, utilizing data quality assessment using python can streamline the assessment process and improve overall data quality.
3.3. Feature Engineering
Feature engineering is the process of selecting, modifying, or creating new features from raw data to improve the performance of machine learning models. It plays a vital role in determining the success of predictive analytics.
- Definition of Features: Features are individual measurable properties or characteristics used by algorithms to make predictions.
- Importance of Feature Engineering:
- Enhances model accuracy: Well-engineered features can lead to better predictions.
- Reduces overfitting: By selecting relevant features, models can generalize better to unseen data.
- Common Techniques:
- Feature selection: Identifying and retaining the most relevant features while discarding irrelevant ones.
- Feature extraction: Creating new features from existing data, such as aggregating or transforming variables.
- Encoding categorical variables: Converting categorical data into numerical format for model compatibility.
- Tools and Libraries:
- Scikit-learn: Offers various methods for feature selection and transformation.
- Pandas: Useful for data manipulation and feature creation.
Effective feature engineering can significantly improve the performance of machine learning models, making it a critical step in the data science workflow. Rapid Innovation leverages these techniques to ensure clients achieve optimal results and maximize their return on investment.
3.4. Privacy and Consent Frameworks
In today's data-driven world, privacy and consent frameworks are essential for protecting individuals' personal information. Organizations must navigate complex regulations and ethical considerations when handling data.
- Importance of Privacy: Protecting user data builds trust and ensures compliance with legal standards. Breaches can lead to severe reputational damage and financial penalties.
- Key Regulations:
- General Data Protection Regulation (GDPR): A comprehensive regulation in the EU that governs data protection and privacy.
- California Consumer Privacy Act (CCPA): A state law that enhances privacy rights for residents of California.
- Consent Mechanisms:
- Explicit consent: Users must actively agree to data collection and processing.
- Opt-in and opt-out options: Providing users with choices regarding their data usage.
- Best Practices for Compliance:
- Conduct regular audits: To ensure adherence to privacy regulations.
- Implement data minimization principles: Collect only the data necessary for specific purposes.
- Educate employees: On data privacy policies and practices.
Establishing robust privacy and consent frameworks is not only a legal requirement but also a fundamental aspect of ethical data management. Rapid Innovation assists clients in navigating these complexities, ensuring compliance while fostering trust with their users.
3.5. Ethical Data Management
Ethical data management is crucial in today's data-driven world. It involves the responsible collection, storage, and use of data while ensuring privacy and compliance with regulations. Key aspects include:
- Data Privacy: Organizations must prioritize the privacy of individuals by implementing robust data protection measures. This includes anonymizing personal data and obtaining consent before data collection.
- Transparency: Companies should be transparent about how they collect and use data. Clear communication about data practices builds trust with users and stakeholders.
- Data Minimization: Collect only the data necessary for specific purposes. This reduces the risk of data breaches and ensures compliance with regulations like GDPR.
- Accountability: Organizations must take responsibility for their data practices. This includes regular audits and assessments to ensure compliance with ethical standards and addressing any ethical issues in data management.
- Bias Mitigation: Addressing bias in data collection and analysis is essential. This involves using diverse datasets and regularly reviewing algorithms to prevent discrimination.
- Data Security: Implement strong security measures to protect data from unauthorized access. This includes encryption, access controls, and regular security audits.
- Regulatory Compliance: Stay updated with data protection laws and regulations. Non-compliance can lead to severe penalties and damage to reputation, highlighting the importance of understanding legal and ethical issues in data management.
By adhering to ethical data management practices, organizations can foster trust, enhance their reputation, and ensure long-term success in a data-centric environment. At Rapid Innovation, we emphasize ethical data management as a cornerstone of our AI and Blockchain solutions, ensuring that our clients not only meet regulatory requirements but also build lasting relationships with their customers. For more insights on ethical data management, you can refer to our article on best practices in AI and data privacy.
4. AI Agent Architectural Design
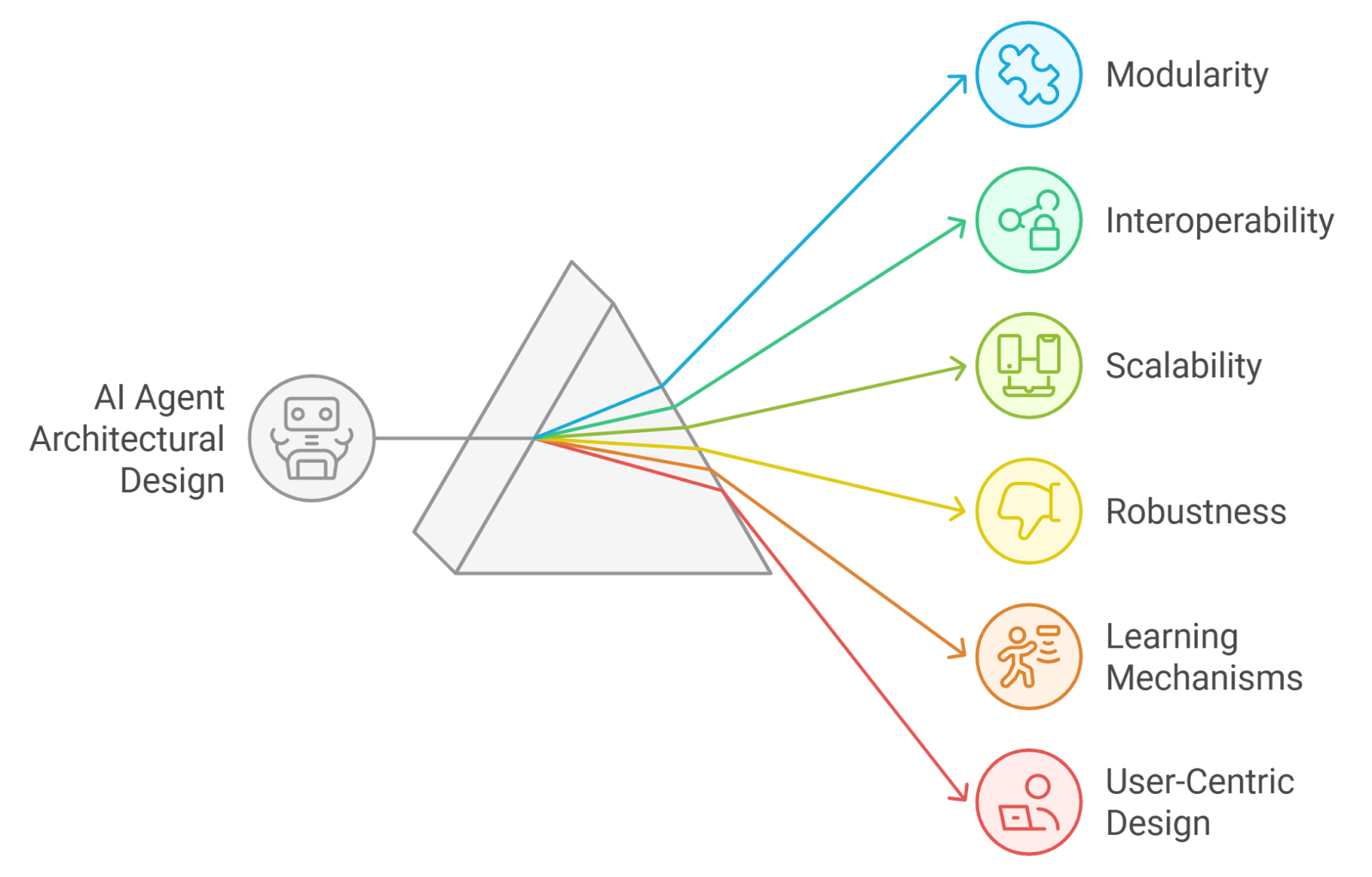
AI agent architectural design refers to the framework and structure that define how AI agents operate, interact, and learn. A well-designed architecture is essential for the effectiveness and efficiency of AI systems. Key components include:
- Modularity: Design AI agents in a modular fashion, allowing for easy updates and maintenance. This promotes flexibility and scalability.
- Interoperability: Ensure that AI agents can communicate and work with other systems seamlessly. This enhances collaboration and data sharing across platforms.
- Scalability: The architecture should support scaling up or down based on demand. This is crucial for handling varying workloads and ensuring optimal performance.
- Robustness: AI agents must be designed to handle errors and unexpected situations gracefully. This includes implementing fail-safes and recovery mechanisms.
- Learning Mechanisms: Incorporate various learning techniques, such as supervised, unsupervised, and reinforcement learning, to enhance the agent's ability to adapt and improve over time.
- User-Centric Design: Focus on the end-user experience. The architecture should facilitate easy interaction and provide meaningful feedback to users.
- Ethical Considerations: Integrate ethical guidelines into the design process to ensure that AI agents operate fairly and responsibly, addressing professional legal and ethical issues in data management.
A well-thought-out AI agent architectural design can significantly enhance the performance and reliability of AI systems, leading to better outcomes for users and organizations alike. Rapid Innovation specializes in creating tailored AI architectures that align with our clients' specific business needs, ensuring they achieve greater ROI through efficient and effective solutions.
4.1. Predictive Model Architectures
Predictive model architectures are frameworks that enable the development of models capable of forecasting future outcomes based on historical data. These architectures are essential in various fields, including finance, healthcare, and marketing. Key elements include:
- Linear Models: Simple yet effective, linear models assume a linear relationship between input variables and the target variable. They are easy to interpret and implement.
- Tree-Based Models: Decision trees and ensemble methods like Random Forest and Gradient Boosting are popular for their ability to handle non-linear relationships and interactions between variables.
- Neural Networks: Deep learning architectures, such as Convolutional Neural Networks (CNNs) and Recurrent Neural Networks (RNNs), are powerful for complex data types like images and sequences. They excel in capturing intricate patterns.
- Time Series Models: For data that varies over time, models like ARIMA and Seasonal Decomposition of Time Series (STL) are used to capture trends and seasonality.
- Feature Engineering: The architecture should support effective feature extraction and selection, which are critical for improving model performance.
- Model Evaluation: Implement robust evaluation metrics to assess model accuracy and reliability. Common metrics include Mean Absolute Error (MAE) and Root Mean Squared Error (RMSE).
- Deployment and Scalability: Ensure that the predictive model can be easily deployed and scaled to handle real-time data and user demands.
By leveraging various predictive model architectures, organizations can enhance their decision-making processes and gain valuable insights from their data. Rapid Innovation's expertise in predictive modeling empowers clients to harness their data effectively, driving informed decisions and maximizing their return on investment.
4.2. Multi-Dimensional Analysis Frameworks
Multi-dimensional analysis frameworks are essential tools in data analytics, allowing organizations to examine data from various perspectives. These frameworks enable businesses to gain deeper insights and make informed decisions based on comprehensive data analysis.
- Definition: Multi-dimensional analysis involves evaluating data across multiple dimensions, such as time, geography, and product categories. This approach helps in identifying trends, patterns, and anomalies that may not be visible through traditional analysis methods.
- Benefits:
- Enhanced decision-making: By analyzing data from different angles, organizations can make more informed strategic decisions.
- Improved data visualization: Multi-dimensional frameworks often include visualization tools that help stakeholders understand complex data sets easily.
- Better performance tracking: Organizations can monitor key performance indicators (KPIs) across various dimensions, leading to more effective performance management.
- Applications:
- Business intelligence: Companies use multi-dimensional analysis frameworks to assess sales performance, customer behavior, and market trends. Rapid Innovation can assist in implementing these frameworks to enhance your business intelligence capabilities, ultimately driving greater ROI.
- Financial analysis: Financial institutions leverage multi-dimensional analysis frameworks to analyze risk, investment performance, and regulatory compliance. Our expertise in AI can help automate and optimize these analyses, ensuring timely and accurate insights.
- Healthcare: Multi-dimensional analysis frameworks help healthcare providers track patient outcomes, resource utilization, and treatment effectiveness. Rapid Innovation can develop tailored solutions that integrate these frameworks into healthcare systems, improving patient care and operational efficiency.
4.3. Real-Time Monitoring Systems
Real-time monitoring systems are critical for organizations that require immediate insights into their operations. These systems provide continuous data collection and analysis, enabling businesses to respond swiftly to changes and challenges.
- Definition: Real-time monitoring systems collect and analyze data as it is generated, allowing organizations to track performance and detect issues instantly.
- Benefits:
- Immediate insights: Organizations can access up-to-the-minute data, facilitating quick decision-making.
- Proactive issue resolution: By identifying problems in real-time, businesses can address issues before they escalate.
- Enhanced operational efficiency: Continuous monitoring helps optimize processes and resource allocation.
- Applications:
- Manufacturing: Real-time monitoring systems track production lines, ensuring efficiency and quality control. Rapid Innovation can implement AI-driven solutions that enhance monitoring capabilities, leading to reduced downtime and increased productivity.
- IT infrastructure: Organizations use these systems to monitor network performance, security threats, and system health. Our blockchain expertise can enhance security and data integrity in these monitoring systems.
- Customer service: Real-time monitoring of customer interactions helps businesses improve service delivery and customer satisfaction. We can develop AI solutions that analyze customer interactions in real-time, enabling personalized service and improved customer experiences.
4.4. Adaptive Learning Mechanisms
Adaptive learning mechanisms are innovative approaches that enable systems to learn from data and improve over time. These mechanisms are particularly valuable in environments where conditions change frequently, requiring continuous adaptation.
- Definition: Adaptive learning refers to the ability of a system to modify its behavior based on new data and experiences, enhancing its performance and accuracy.
- Benefits:
- Continuous improvement: Systems can evolve and improve their predictions or recommendations based on new information.
- Personalization: Adaptive learning allows for tailored experiences, enhancing user engagement and satisfaction.
- Increased efficiency: By learning from past data, systems can optimize processes and reduce errors.
- Applications:
- E-commerce: Adaptive learning mechanisms help personalize product recommendations based on user behavior and preferences. Rapid Innovation can develop AI algorithms that enhance recommendation systems, driving higher conversion rates and customer loyalty.
- Education: Adaptive learning platforms adjust content delivery based on individual student performance, promoting better learning outcomes. Our solutions can integrate AI to create personalized learning experiences that adapt to each student's needs.
- Marketing: Businesses use adaptive learning to refine targeting strategies and improve campaign effectiveness based on real-time feedback. Rapid Innovation can leverage AI to analyze campaign data, optimizing marketing efforts for maximum ROI.
4.5. Personalization Strategies

Personalization strategies are essential for enhancing user experience and engagement across various platforms. By tailoring content and services to individual preferences, businesses can significantly improve customer satisfaction and loyalty. Here are some effective personalization strategies:
- Data Collection: Gather data from user interactions, preferences, and behaviors, including browsing history, purchase history, and demographic information. The more data you collect, the better you can understand your audience.
- Segmentation: Divide your audience into distinct segments based on shared characteristics. This allows for targeted marketing efforts and personalized content delivery. For example, you might segment users by age, location, or purchasing habits, which is a key aspect of email audience segmentation.
- Dynamic Content: Use dynamic content that changes based on user behavior or preferences. This can include personalized product recommendations, tailored email campaigns, or customized landing pages that reflect user interests, aligning with personalized video marketing and website personalization strategies.
- Behavioral Targeting: Implement behavioral targeting techniques to deliver relevant ads and content based on user actions. For instance, if a user frequently browses a specific category, show them related products or articles, which is a fundamental part of marketing personalization strategies.
- Feedback Loops: Create mechanisms for users to provide feedback on their experiences. This can help refine personalization efforts and ensure that the content remains relevant and engaging.
- Machine Learning Algorithms: Utilize machine learning algorithms to analyze user data and predict preferences. These algorithms can help automate the personalization process, making it more efficient and effective. At Rapid Innovation, we leverage advanced machine learning techniques to enhance personalization, ensuring that our clients can deliver tailored experiences that drive engagement and conversion, including ecommerce personalization strategies. For more insights on implementing explainable AI for transparent agent decisions.
- A/B Testing: Conduct A/B testing to evaluate the effectiveness of different personalization strategies. This allows you to identify what resonates best with your audience and optimize your approach accordingly, which is crucial for developing a successful personalization marketing strategy.
4.6. Explainable AI Techniques
Explainable AI (XAI) techniques are crucial for building trust and transparency in artificial intelligence systems. As AI becomes more integrated into decision-making processes, stakeholders need to understand how these systems arrive at their conclusions. Here are some key explainable AI techniques:
- Model Transparency: Use models that are inherently interpretable, such as decision trees or linear regression. These models allow users to easily understand how input features influence predictions.
- Feature Importance: Implement techniques that highlight the importance of different features in the decision-making process. For example, SHAP (SHapley Additive exPlanations) values can provide insights into how each feature contributes to a model's output.
- Local Explanations: Generate local explanations for individual predictions. This can help users understand why a specific decision was made for a particular instance, enhancing trust in the AI system.
- Visualization Tools: Utilize visualization tools to present complex data and model behavior in an understandable format. Graphical representations can make it easier for users to grasp how AI systems function.
- User-Centric Design: Design AI systems with the end-user in mind. Ensure that explanations are tailored to the user's level of expertise and understanding, making them accessible to a broader audience.
- Documentation and Communication: Provide clear documentation and communication about how the AI system works. This includes explaining the data sources, algorithms used, and the rationale behind specific decisions.
- Regulatory Compliance: Stay informed about regulations regarding AI transparency and accountability. Compliance with these regulations can enhance the credibility of your AI systems and foster user trust.
5. Prediction Methodologies

Prediction methodologies are essential for making informed decisions based on data analysis. These methodologies leverage statistical techniques and machine learning algorithms to forecast future outcomes. Here are some widely used prediction methodologies:
- Regression Analysis: This statistical method estimates the relationships among variables and is commonly used for predicting continuous outcomes, such as sales revenue or temperature.
- Time Series Analysis: This technique analyzes data points collected or recorded at specific time intervals. It is particularly useful for forecasting trends over time, such as stock prices or economic indicators.
- Classification Algorithms: These algorithms categorize data into predefined classes. Common classification techniques include logistic regression, decision trees, and support vector machines, often used for tasks like spam detection or customer segmentation.
- Ensemble Methods: These methods combine multiple models to improve prediction accuracy. Techniques like bagging and boosting can enhance the performance of individual models by reducing variance and bias.
- Neural Networks: Inspired by the human brain, neural networks are powerful tools for complex prediction tasks. They excel in recognizing patterns in large datasets, making them suitable for applications like image recognition and natural language processing.
- Bayesian Methods: These methods incorporate prior knowledge and update predictions as new data becomes available. Bayesian approaches are particularly useful in situations with uncertainty and can provide probabilistic predictions.
- Cross-Validation: This technique assesses the performance of predictive models by partitioning the data into training and testing sets, helping ensure that models generalize well to unseen data.
- Hyperparameter Tuning: Optimize model performance by adjusting hyperparameters, which are settings that govern the learning process. Techniques like grid search and random search can help identify the best hyperparameter values.
By employing these prediction methodologies, organizations can enhance their decision-making processes and gain valuable insights from their data. At Rapid Innovation, we specialize in implementing these methodologies to help our clients achieve greater ROI and drive business success, including the use of personalized marketing products and customized marketing strategies.
5.1. Supervised Learning Approaches
Supervised learning is a type of machine learning where the model is trained on labeled data. This means that the input data is paired with the correct output, allowing the algorithm to learn the relationship between the two. Supervised learning is widely used in various applications, including classification and regression tasks, enabling businesses to make data-driven decisions that enhance operational efficiency and drive ROI.
- Classification: This involves predicting a categorical label, such as identifying whether an email is spam or not. Common algorithms include:
- Logistic Regression
- Decision Trees
- Support Vector Machines (SVM)
- Neural Networks
For instance, Rapid Innovation can implement supervised classification machine learning algorithms to help clients automate customer support by accurately categorizing inquiries, leading to faster response times and improved customer satisfaction.
- Regression: This involves predicting a continuous value, for instance, predicting house prices based on features like size and location. Common algorithms include:
- Linear Regression
- Polynomial Regression
- Random Forest Regression
- Gradient Boosting Machines
By utilizing supervised machine learning regression techniques, Rapid Innovation can assist clients in forecasting sales or optimizing pricing strategies, ultimately enhancing profitability.
- Key Characteristics:
- Requires a labeled dataset for training.
- Performance can be evaluated using metrics like accuracy, precision, recall, and F1 score.
- Overfitting is a common challenge, where the model performs well on training data but poorly on unseen data.
5.2. Unsupervised Learning Techniques
Unsupervised learning is a type of machine learning that deals with unlabeled data. The model tries to learn the underlying structure of the data without any explicit guidance on what the output should be. This approach is particularly useful for exploratory data analysis and pattern recognition, allowing businesses to uncover insights that can inform strategic decisions.
- Clustering: This technique groups similar data points together. Common algorithms include:
- K-Means Clustering
- Hierarchical Clustering
- DBSCAN (Density-Based Spatial Clustering of Applications with Noise)
Rapid Innovation can leverage clustering techniques to segment customers based on purchasing behavior, enabling targeted marketing campaigns that increase conversion rates.
- Dimensionality Reduction: This technique reduces the number of features in a dataset while retaining essential information. Common algorithms include:
- Principal Component Analysis (PCA)
- t-Distributed Stochastic Neighbor Embedding (t-SNE)
- Autoencoders
By applying dimensionality reduction, Rapid Innovation can help clients visualize complex datasets, making it easier to identify trends and patterns that drive business growth.
- Key Characteristics:
- Does not require labeled data, making it suitable for large datasets where labeling is impractical.
- Useful for discovering hidden patterns and structures in data.
- Evaluation can be challenging, as there are no clear metrics for success.
5.3. Ensemble Prediction Models
Ensemble prediction models combine multiple individual models to improve overall performance. The idea is that by aggregating the predictions of several models, the ensemble can achieve better accuracy and robustness than any single model. This approach is particularly effective in reducing overfitting and improving generalization, which is crucial for businesses aiming to make reliable predictions.
- Bagging: This technique involves training multiple models on different subsets of the data and averaging their predictions. Common algorithms include:
- Random Forest: An ensemble of decision trees that improves accuracy and reduces overfitting.
- Bootstrap Aggregating: A method that uses bootstrapped samples to create diverse models.
Rapid Innovation can implement bagging techniques to enhance the accuracy of predictive analytics, leading to more informed decision-making.
- Boosting: This technique focuses on training models sequentially, where each new model attempts to correct the errors made by the previous ones. Common algorithms include:
- AdaBoost: Adjusts the weights of misclassified instances to focus on difficult cases.
- Gradient Boosting: Builds models in a stage-wise fashion, optimizing for a loss function.
By utilizing boosting methods, Rapid Innovation can help clients improve their predictive models, resulting in better risk assessment and management.
- Stacking: This technique involves training multiple models and then using another model to combine their predictions. This meta-model learns how to best combine the outputs of the base models.
- Key Characteristics:
- Often leads to improved predictive performance.
- Can handle a variety of data types and structures.
- Requires careful tuning of hyperparameters to achieve optimal results.
Through these advanced supervised machine learning techniques, Rapid Innovation empowers clients to harness the full potential of their data, driving efficiency and maximizing ROI in their operations. Additionally, for more information on outlier detection, visit Outlier Detection: What You Need to Know and for how to implement adaptive AI solutions, visit Adaptive AI Development.
5.4. Time Series Forecasting
Time series forecasting is a statistical technique used to predict future values based on previously observed values. This method is particularly useful in various fields such as finance, economics, and environmental science, and can significantly enhance decision-making processes for businesses.
- Key components of time series data include:
- Trend: The long-term movement in the data.
- Seasonality: Regular patterns that repeat over a specific period.
- Cyclic patterns: Fluctuations that occur at irregular intervals.
- Irregular variations: Random noise or outliers in the data.
- Common methods for time series forecasting include:
- ARIMA (AutoRegressive Integrated Moving Average): A popular model that combines autoregression and moving averages, allowing businesses to make informed predictions about future performance.
- Exponential Smoothing: A technique that applies decreasing weights to past observations, which can be particularly useful for organizations looking to adapt quickly to changing market conditions.
- Seasonal Decomposition of Time Series (STL): A method that separates seasonal effects from the trend and noise, enabling companies to better understand underlying patterns in their data.
- Applications of time series forecasting:
- Stock market predictions: Analyzing historical stock prices to forecast future movements, helping investors make strategic decisions.
- Sales forecasting: Estimating future sales based on past sales data, which can optimize inventory management and resource allocation.
- Weather forecasting: Predicting future weather conditions using historical weather data, crucial for industries like agriculture and logistics.
- Time series forecasting in R: Utilizing R programming for effective time series analysis and forecasting.
- Time series analysis in Python: Implementing Python libraries for comprehensive time series forecasting.
- Time series machine learning: Applying machine learning techniques to enhance forecasting accuracy.
5.5. Probabilistic Prediction Frameworks
Probabilistic prediction frameworks provide a way to quantify uncertainty in predictions. Unlike deterministic models that offer a single outcome, probabilistic models generate a range of possible outcomes, each with an associated probability, thus enhancing strategic planning.
- Key features of probabilistic prediction frameworks include:
- Uncertainty quantification: Assessing the likelihood of various outcomes, which is essential for risk management.
- Bayesian inference: A statistical method that updates the probability of a hypothesis as more evidence becomes available, allowing businesses to refine their strategies based on new data.
- Monte Carlo simulations: A computational technique that uses random sampling to estimate the probability of different outcomes, providing insights into potential risks and rewards.
- Benefits of using probabilistic prediction frameworks:
- Better decision-making: By understanding the range of possible outcomes, stakeholders can make more informed decisions that align with their business objectives.
- Risk assessment: Identifying and quantifying risks associated with different scenarios, which is vital for strategic planning.
- Flexibility: Adapting to new information and changing conditions, ensuring that organizations remain agile in a dynamic market.
- Applications of probabilistic prediction frameworks:
- Finance: Estimating the risk of investment portfolios, enabling better asset management.
- Healthcare: Predicting patient outcomes based on various treatment options, which can improve patient care and resource allocation.
- Supply chain management: Forecasting demand and managing inventory levels, leading to cost savings and improved efficiency.
- Regression analysis of time series: Utilizing regression techniques to analyze time series data for better forecasting.
5.6. Early Warning Systems
Early warning systems (EWS) are tools designed to detect and alert stakeholders about potential risks or hazards before they occur. These systems are crucial in disaster management, public health, and environmental monitoring, and can significantly enhance organizational resilience.
- Key components of early warning systems include:
- Monitoring: Continuous observation of relevant indicators to detect anomalies, ensuring timely responses to emerging threats.
- Data analysis: Using statistical and computational methods to interpret data and identify trends, which is essential for proactive risk management.
- Communication: Disseminating alerts and information to stakeholders in a timely manner, fostering a culture of preparedness.
- Benefits of early warning systems:
- Risk reduction: Providing advance notice of potential hazards allows for proactive measures to mitigate risks, safeguarding assets and lives.
- Improved response: Enhancing the ability of organizations to respond quickly and effectively to emerging threats, minimizing potential damage.
- Public awareness: Educating communities about potential risks and encouraging preparedness, which is vital for community resilience.
- Applications of early warning systems:
- Natural disasters: Monitoring seismic activity to predict earthquakes or tracking weather patterns for hurricanes, enabling timely evacuations and resource allocation.
- Public health: Detecting outbreaks of infectious diseases through surveillance systems, which can lead to quicker interventions and better health outcomes.
- Environmental monitoring: Assessing air and water quality to identify pollution events, ensuring compliance with regulations and protecting public health.
At Rapid Innovation, we leverage advanced AI and blockchain technologies to implement these methodologies, helping our clients achieve greater ROI through data-driven insights and proactive risk management. Our expertise in these domains ensures that businesses can navigate uncertainties with confidence and agility, utilizing models for time series analysis and regression and time series techniques effectively.
6. Comprehensive Performance Indicators
Comprehensive performance indicators are essential tools for evaluating the effectiveness of educational programs and student outcomes. These indicators provide a holistic view of student performance, helping educators, administrators, and policymakers make informed decisions. Two critical components of comprehensive performance indicators are academic achievement metrics and learning style analysis.
6.1. Academic Achievement Metrics

Academic achievement metrics are quantitative measures that assess students' performance in various subjects and skills. These metrics are crucial for understanding how well students are meeting educational standards and achieving learning objectives, including educational performance indicators and academic performance indicators for students.
- Standardized Test Scores: These scores provide a benchmark for comparing student performance across different schools and districts. They help identify areas where students excel or need improvement, serving as key performance indicators for schools.
- Grade Point Average (GPA): GPA is a widely recognized metric that reflects a student's overall academic performance. It is often used for college admissions and scholarship eligibility, making it one of the key performance indicators in teaching and learning.
- Course Completion Rates: Tracking the percentage of students who complete their courses can indicate the effectiveness of instructional methods and student engagement, which are important indicators of students' academic performance.
- Attendance Rates: Regular attendance is linked to academic success. Monitoring attendance can help identify students at risk of falling behind, serving as an indicator of academic achievement.
- Graduation Rates: This metric reflects the percentage of students who successfully complete their education within a specified timeframe. High graduation rates are often indicative of a supportive learning environment and are considered key performance indicators for secondary schools.
These metrics can be analyzed to identify trends and patterns, allowing educators to tailor their teaching strategies to meet the needs of their students. For instance, if standardized test scores reveal a consistent decline in math performance, targeted interventions can be implemented to address this issue, aligning with examples of performance indicators in education.
6.2. Learning Style Analysis
Learning style analysis involves understanding the different ways in which students absorb, process, and retain information. Recognizing these diverse learning styles is essential for creating an inclusive and effective educational environment.
- Visual Learners: These students learn best through visual aids such as charts, graphs, and images. Incorporating multimedia presentations can enhance their understanding.
- Auditory Learners: Auditory learners benefit from listening to lectures, discussions, and audio materials. Engaging them through group discussions and oral presentations can be effective.
- Kinesthetic Learners: These learners thrive on hands-on experiences and physical activities. Incorporating experiments, role-playing, and interactive activities can help them grasp concepts better.
- Reading/Writing Learners: These students prefer reading texts and writing notes. Providing them with written materials and encouraging note-taking can enhance their learning experience.
By analyzing the predominant learning styles within a classroom, educators can adapt their teaching methods to cater to the diverse needs of their students. This personalized approach can lead to improved engagement and academic performance, which are critical indicators of academic achievement.
In conclusion, comprehensive performance indicators, including academic achievement metrics and learning style analysis, play a vital role in enhancing educational outcomes. By leveraging these tools, educators can create a more effective and inclusive learning environment that supports all students in reaching their full potential.
At Rapid Innovation, we understand the importance of data-driven insights in educational settings. Our AI and Blockchain solutions can help institutions collect, analyze, and visualize these performance indicators efficiently, enabling educators to make informed decisions that drive student success and improve overall educational quality. By integrating advanced analytics and secure data management, we empower educational organizations to achieve greater ROI and foster an environment conducive to learning and growth, including the use of key performance indicators for teachers and key performance indicators in primary schools. Explore our AI business automation solutions to learn more about how we can assist you.
6.3. Psychological and Emotional Factors
Psychological and emotional factors play a significant role in shaping an individual's behavior, decision-making, and overall well-being. These factors can influence various aspects of life, including academic performance, relationships, and career choices. Understanding these influences is crucial for personal development and mental health.
- Self-esteem: A person's self-worth can greatly affect their motivation and performance. High self-esteem often leads to greater psychological resilience and a willingness to take on challenges, while low self-esteem can result in anxiety and avoidance behaviors.
- Stress and Anxiety: Chronic stress and anxiety can impair cognitive functions, leading to difficulties in concentration and decision-making. Managing stress through techniques such as mindfulness and relaxation can improve emotional well-being and performance.
- Emotional Intelligence: The ability to recognize and manage one's emotions, as well as the emotions of others, is vital for effective communication and relationship-building. High emotional intelligence can enhance teamwork and leadership skills, which are essential in collaborative environments, including those driven by AI and Blockchain technologies.
- Coping Mechanisms: Individuals develop various coping strategies to deal with emotional challenges. Healthy coping mechanisms, such as problem-solving and seeking support, can lead to better outcomes compared to maladaptive strategies like avoidance or substance abuse.
- Motivation: Intrinsic and extrinsic motivation can drive behavior. Understanding what motivates an individual can help in setting achievable goals and maintaining engagement in tasks, which is particularly relevant in the fast-paced fields of AI and Blockchain.
- Psychological Resilience: The ability to bounce back from setbacks and adapt to challenging situations is a key component of psychological resilience. This trait can be influenced by various psychological factors, including self-esteem and coping mechanisms.
- Psychological Behavior Examples: Observing psychological behavior examples can provide insights into how individuals respond to different situations, which can be beneficial for understanding consumer behavior and psychology. For instance, initiatives like Cardano for Social Impact illustrate how psychological factors can be leveraged for community development and engagement.
6.4. Socio-Economic Influences
Socio-economic factors significantly impact an individual's opportunities, lifestyle, and overall quality of life. These influences can shape educational attainment, career prospects, and health outcomes.
- Income Level: Higher income levels often correlate with better access to resources, including education, healthcare, and extracurricular activities. Conversely, lower income can limit opportunities and create barriers to success.
- Education: The educational background of an individual and their family can influence career choices and earning potential. Access to quality education is often determined by socio-economic status, affecting long-term outcomes.
- Social Networks: Socio-economic status can affect the quality and extent of social networks. Strong networks can provide support, mentorship, and job opportunities, while weaker networks may limit access to these resources.
- Cultural Capital: Individuals from higher socio-economic backgrounds may have greater exposure to cultural experiences, which can enhance social skills and confidence. This cultural capital can influence academic and professional success.
- Health Disparities: Socio-economic status is linked to health outcomes. Individuals from lower socio-economic backgrounds may face higher rates of chronic illnesses, which can affect their ability to work and participate in society.
6.5. Extracurricular Performance
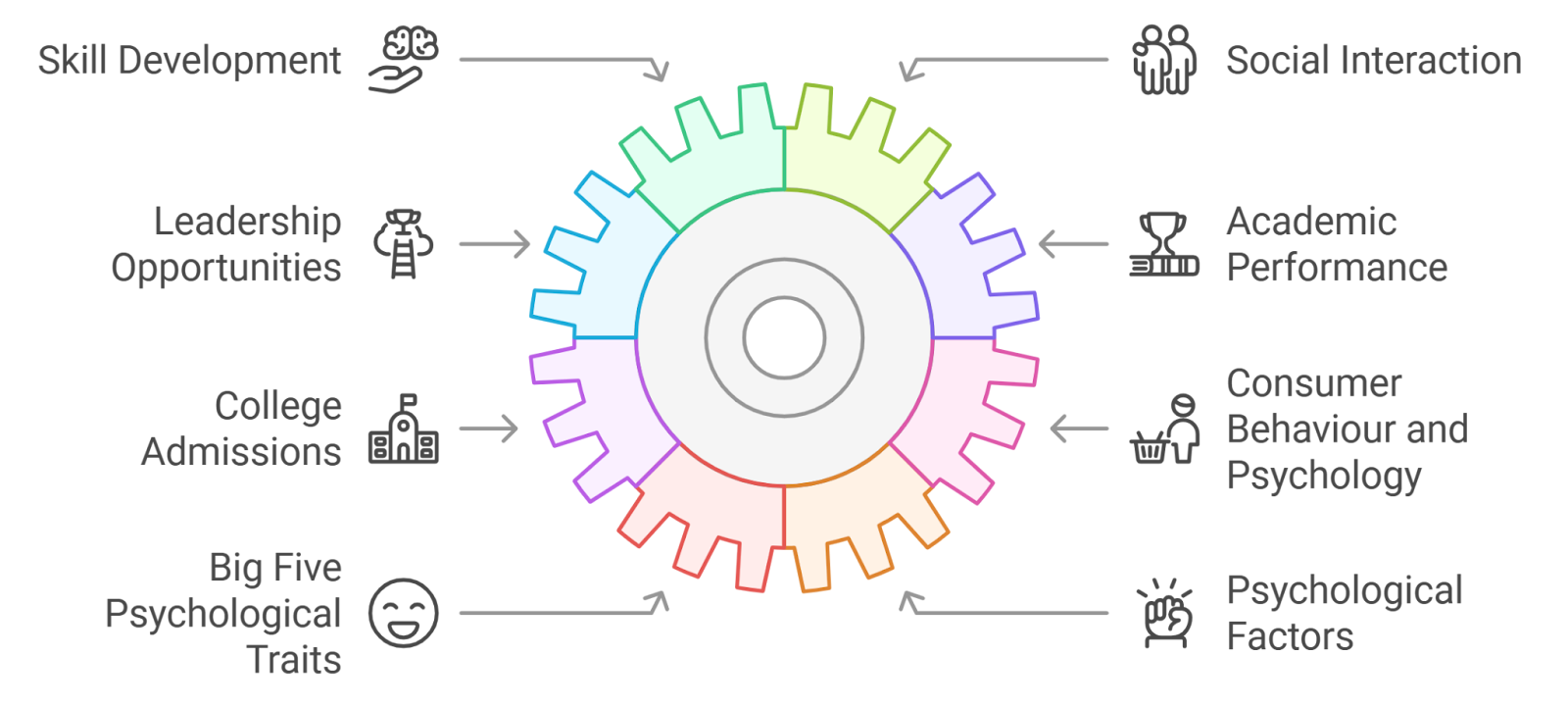
Extracurricular activities play a vital role in personal development, providing opportunities for skill enhancement, social interaction, and leadership experience. Participation in these activities can have a lasting impact on an individual's life.
- Skill Development: Engaging in extracurricular activities helps individuals develop various skills, including teamwork, communication, and time management. These skills are transferable to academic and professional settings, particularly in innovative fields like AI and Blockchain.
- Social Interaction: Extracurriculars provide a platform for meeting new people and building friendships. This social interaction can enhance emotional well-being and create a sense of belonging.
- Leadership Opportunities: Many extracurricular activities offer leadership roles, allowing individuals to develop their leadership skills. These experiences can be beneficial for future career advancement, especially in technology-driven industries.
- Academic Performance: Research indicates that students involved in extracurricular activities often perform better academically. The discipline and time management skills gained through these activities can translate into improved study habits.
- College Admissions: Participation in extracurricular activities can enhance college applications. Admissions committees often look for well-rounded candidates who demonstrate commitment and passion outside of academics, which can be further supported by skills gained in AI and Blockchain projects.
- Consumer Behaviour and Psychology: Understanding consumer behaviour and psychology can also be enhanced through participation in extracurricular activities, as these experiences can shape an individual's interests and preferences.
- Big Five Psychological Traits: The big five psychological traits can influence how individuals engage in extracurricular activities and interact with peers, impacting their overall development and success.
- Psychological Factors: Various psychological factors, including motivation and emotional intelligence, play a role in how individuals perform in extracurricular settings and their subsequent academic and career outcomes.
6.6. Holistic Student Profiling
Holistic student profiling is an approach that considers the complete spectrum of a student's abilities, interests, and experiences. This method goes beyond traditional academic metrics to create a more comprehensive understanding of each student.
- Focus on multiple dimensions:
- Academic performance
- Social-emotional skills
- Extracurricular activities
- Personal interests and aspirations
- Benefits of holistic profiling:
- Tailored educational experiences that cater to individual needs
- Enhanced student engagement and motivation
- Improved identification of strengths and areas for growth
- Data collection methods:
- Surveys and questionnaires to gather student interests and self-assessments
- Portfolios showcasing student work and achievements
- Teacher observations and feedback
- Implementation of technology:
- Use of learning management systems to track student progress
- Data analytics tools to analyze student profiles and outcomes
- Integration of artificial intelligence to personalize learning experiences, enabling educators to adapt teaching methods based on individual student data.
- Importance of collaboration:
- Involvement of teachers, counselors, and parents in the profiling process
- Regular updates and reviews of student profiles to reflect changes over time
- Creating a supportive environment that encourages student input
7. Implementation Strategies
Effective implementation strategies are crucial for the success of educational initiatives. These strategies ensure that the proposed changes are executed smoothly and achieve the desired outcomes.
- Establish clear goals:
- Define specific objectives for the initiative
- Align goals with institutional mission and vision
- Ensure that all stakeholders understand the purpose of the changes
- Develop a detailed action plan:
- Outline steps required for implementation
- Assign responsibilities to team members
- Set timelines for each phase of the project
- Engage stakeholders:
- Involve faculty, staff, students, and parents in the planning process
- Conduct workshops and meetings to gather input and feedback
- Foster a sense of ownership among stakeholders to encourage buy-in
- Monitor progress:
- Regularly assess the implementation process against established benchmarks
- Use data to identify areas for improvement
- Adjust strategies as needed based on feedback and outcomes
- Provide professional development:
- Offer training sessions for educators to equip them with necessary skills
- Share best practices and resources to support implementation
- Encourage collaboration among staff to share experiences and insights
7.1. Institutional Readiness Assessment
An institutional readiness assessment is a critical step in determining whether an organization is prepared to implement new initiatives. This assessment evaluates various factors that influence the success of the proposed changes.
- Key components of readiness assessment:
- Current infrastructure and resources
- Staff expertise and willingness to adapt
- Existing policies and procedures that may impact implementation
- Steps to conduct an assessment:
- Gather data through surveys, interviews, and focus groups
- Analyze institutional strengths and weaknesses
- Identify potential barriers to implementation
- Importance of stakeholder involvement:
- Engage faculty and staff in the assessment process to gain diverse perspectives
- Encourage open dialogue about concerns and suggestions
- Use feedback to inform the planning and implementation phases
- Evaluate organizational culture:
- Assess the institution's openness to change and innovation
- Identify existing support systems that can facilitate the transition
- Determine the level of collaboration among departments
- Develop a readiness report:
- Summarize findings from the assessment
- Highlight areas of strength and opportunities for growth
- Provide recommendations for addressing identified gaps
- Continuous improvement:
- Use the assessment as a baseline for future evaluations
- Regularly revisit readiness factors as the institution evolves
- Foster a culture of ongoing assessment and adaptation to ensure long-term success.
At Rapid Innovation, we leverage our expertise in AI and Blockchain to enhance the effectiveness of holistic student profiling strategies, ensuring that educational institutions can achieve their goals efficiently and effectively. By integrating advanced data analytics and secure blockchain solutions, we help organizations optimize their processes, leading to greater ROI and improved educational outcomes. For more information, visit our article on AI in Education.
7.2. Data Integration Approaches

Data integration is a crucial step in the data processing pipeline, especially when dealing with large datasets from various sources. Effective data integration ensures that data is unified, consistent, and ready for analysis or model training. Here are some common data integration approaches:
- ETL (Extract, Transform, Load): This traditional method involves extracting data from different sources, transforming it into a suitable format, and loading it into a target database. ETL is widely used in data warehousing and can significantly enhance the efficiency of data management processes, leading to improved decision-making.
- ELT (Extract, Load, Transform): In contrast to ETL, ELT loads raw data into the target system first and then transforms it. This approach is beneficial for big data environments where storage is cheaper than processing power. Rapid Innovation leverages ELT to help clients manage vast amounts of data efficiently, ensuring they can derive insights quickly.
- Data Virtualization: This technique allows users to access and manipulate data without needing to know its physical location. It creates a virtual layer that integrates data from multiple sources in real-time, enabling organizations to make informed decisions based on the most current data available.
- API Integration: Application Programming Interfaces (APIs) enable different software systems to communicate. By using APIs, organizations can integrate data from various applications seamlessly. Rapid Innovation assists clients in developing robust API integrations that facilitate smooth data flow across platforms, enhancing operational efficiency.
- Data Federation: This approach creates a unified view of data from disparate sources without physically moving it. It allows for real-time access and querying of data across different systems, which is essential for organizations that require immediate insights for strategic planning.
- Batch vs. Real-time Integration: Depending on the use case, data can be integrated in batches (periodically) or in real-time (as it is generated). Real-time integration is essential for applications requiring immediate insights, and Rapid Innovation helps clients implement solutions that cater to their specific data integration needs.
Choosing the right data integration approach depends on factors such as data volume, velocity, variety, and the specific needs of the organization. By considering various data integration methodologies, clients can ensure they select the most effective data integration strategy to achieve their business goals efficiently. Additionally, understanding the types of data integration approaches available can further guide organizations in their decision-making process. By partnering with Rapid Innovation, clients can ensure they select the most effective data integration strategy to achieve their business goals efficiently, including large language model development and MLOps vs. DevOps.
7.3. Model Training and Validation
Model training and validation are critical components of the machine learning lifecycle. They ensure that the model performs well on unseen data and generalizes effectively. Here are key aspects of this process:
- Training Data: The model learns from a training dataset, which should be representative of the problem domain. A well-curated dataset improves the model's ability to learn patterns.
- Validation Data: This dataset is used to tune model parameters and prevent overfitting. It helps in assessing how well the model performs on data it hasn't seen during training.
- Cross-Validation: This technique involves partitioning the training data into subsets, training the model on some subsets, and validating it on others. K-fold cross-validation is a popular method that enhances model reliability.
- Hyperparameter Tuning: Adjusting hyperparameters (settings that govern the training process) is essential for optimizing model performance. Techniques like grid search and random search are commonly used.
- Performance Metrics: Evaluating model performance requires metrics such as accuracy, precision, recall, F1 score, and AUC-ROC. These metrics help in understanding how well the model is performing.
- Test Data: After training and validation, the model is evaluated on a separate test dataset to assess its performance in real-world scenarios. This step is crucial for ensuring the model's robustness.
Effective model training and validation lead to better predictive performance and increased trust in the model's outputs. Rapid Innovation employs best practices in model training and validation to ensure that clients achieve optimal results from their AI initiatives.
7.4. Continuous Learning Mechanisms
Continuous learning mechanisms are essential for keeping machine learning models relevant and effective over time. As data evolves, models must adapt to maintain their accuracy. Here are some key aspects of continuous learning:
- Incremental Learning: This approach allows models to learn from new data without retraining from scratch. It is particularly useful in dynamic environments where data is constantly changing.
- Online Learning: In online learning, models are updated continuously as new data arrives. This method is beneficial for applications requiring real-time predictions and adjustments.
- Feedback Loops: Implementing feedback loops enables models to learn from their predictions. By analyzing the outcomes of their predictions, models can adjust and improve over time.
- Model Monitoring: Continuous monitoring of model performance is crucial. Metrics should be tracked to identify when a model's performance degrades, indicating the need for retraining or adjustment.
- Data Drift Detection: This involves monitoring changes in data distribution over time. Detecting data drift helps in understanding when a model may no longer be valid and requires updates.
- Automated Retraining: Setting up automated processes for retraining models based on performance metrics or data drift can streamline the continuous learning process.
By implementing continuous learning mechanisms, organizations can ensure that their machine learning models remain effective and relevant in a rapidly changing data landscape. Rapid Innovation supports clients in establishing these mechanisms, ensuring their AI solutions continue to deliver value over time.
7.5. Performance Monitoring
Performance monitoring is a critical aspect of any project or initiative, ensuring that objectives are met and resources are utilized effectively. It involves the systematic collection and analysis of data to assess the progress and impact of a program.
- Establishing Key Performance Indicators (KPIs) is essential for measuring success. These indicators should be specific, measurable, achievable, relevant, and time-bound (SMART). At Rapid Innovation, we assist clients in defining KPIs that align with their strategic goals, ensuring that they can effectively measure their progress.
- Regular data collection helps in tracking performance over time. This can include surveys, interviews, and observational studies. Our AI-driven tools automate data collection processes, allowing for real-time insights and reducing manual effort. Utilizing application performance monitoring tools can enhance this process.
- Analyzing data allows for identifying trends, strengths, and weaknesses in the program. This analysis can inform decision-making and strategy adjustments. By leveraging advanced analytics and machine learning algorithms, Rapid Innovation helps clients uncover actionable insights from their data, including insights from application performance monitoring solutions.
- Feedback loops are crucial. Engaging stakeholders in the monitoring process can provide valuable insights and foster a sense of ownership. We facilitate stakeholder engagement through interactive dashboards and reporting tools that keep everyone informed and involved, including the use of APM tools.
- Utilizing technology can enhance performance monitoring. Tools like dashboards and analytics software can streamline data collection and visualization. Rapid Innovation develops customized solutions that integrate seamlessly with existing systems, providing clients with a comprehensive view of their performance metrics, including server monitor tools and application performance management tools.
- Reporting findings to stakeholders ensures transparency and accountability. Regular updates can keep everyone informed and engaged. Our solutions include automated reporting features that deliver insights to stakeholders in a timely manner, enhancing communication and trust, particularly through application performance monitoring.
7.6. Intervention Design
Intervention design refers to the process of creating strategies and actions aimed at addressing specific issues or achieving desired outcomes. A well-structured intervention can significantly enhance the effectiveness of a program.
- Identifying the target population is the first step. Understanding the demographics, needs, and challenges of the group is essential for tailoring interventions. Rapid Innovation employs data analytics to segment populations effectively, ensuring targeted interventions.
- Conducting a needs assessment helps in pinpointing the specific issues that require intervention. This can involve surveys, focus groups, or community consultations. Our AI tools can analyze large datasets to identify pressing needs quickly and accurately.
- Setting clear objectives is vital. These should align with the overall goals of the program and be achievable within the given timeframe. We work with clients to establish SMART objectives that guide their intervention strategies.
- Developing a theory of change can guide the intervention design. This framework outlines how the intervention will lead to the desired outcomes. Rapid Innovation assists clients in mapping out their theories of change, ensuring clarity and focus in their initiatives.
- Engaging stakeholders in the design process ensures that the intervention is relevant and culturally appropriate. Collaboration with community members can enhance buy-in and support. We facilitate stakeholder workshops to co-create interventions that resonate with the target audience.
- Pilot testing the intervention allows for identifying potential issues before full-scale implementation. Feedback from the pilot can inform necessary adjustments. Our iterative approach to intervention design ensures that clients can refine their strategies based on real-world feedback.
8. Use Cases and Applications

Use cases and applications illustrate how concepts and strategies can be implemented in real-world scenarios. They provide practical examples that can guide organizations in their efforts.
- Education: Performance monitoring in educational settings can help track student progress and identify areas needing improvement. Interventions such as tutoring programs can be designed based on performance data, supported by our AI analytics.
- Healthcare: In healthcare, monitoring patient outcomes can inform treatment plans. Interventions like wellness programs can be tailored to address specific health issues identified through data analysis, leveraging our blockchain solutions for secure data sharing.
- Business: Companies can use performance monitoring to assess employee productivity and engagement. Interventions such as training programs can be designed to enhance skills based on performance metrics, with our AI tools providing insights into employee performance trends.
- Environmental Management: Monitoring environmental indicators can help organizations assess the impact of their initiatives. Interventions like community clean-up events can be designed to address specific environmental concerns, supported by our data-driven insights.
- Social Services: Performance monitoring in social services can help evaluate program effectiveness. Interventions such as counseling services can be tailored based on the needs identified through data collection, with our blockchain solutions ensuring data integrity and security.
By understanding and applying performance monitoring and intervention design, organizations can enhance their effectiveness and achieve their goals more efficiently, with Rapid Innovation as a trusted partner in leveraging AI and blockchain technologies for greater ROI, including the use of solutions like Datadog APM and Google application performance monitoring.
8.1. Early Intervention Programs
Early intervention programs, such as early childhood intervention programs and early intervention projects, are designed to identify and support children who may be at risk of developmental delays or learning difficulties. These programs are crucial in providing timely assistance, which can significantly improve long-term outcomes for children.
- Focus on children from birth to age five, as this is a critical period for brain development.
- Utilize assessments to identify children who may need additional support, including those identified through early steps and early on intervention strategies.
- Offer a range of services, including speech therapy, occupational therapy, and behavioral interventions, as part of early intervention for speech and language.
- Engage families in the process, providing them with resources and training to support their child's development at home, particularly through early childhood early intervention initiatives.
- Research indicates that early intervention can lead to improved academic performance and social skills.
- Programs often collaborate with schools, healthcare providers, and community organizations to create a comprehensive support system, including partnerships with early intervention specialists.
8.2. Personalized Learning Paths
Personalized learning paths are tailored educational experiences designed to meet the unique needs and interests of each student. This approach recognizes that every learner is different and requires a customized strategy to thrive.
- Incorporate technology to facilitate individualized learning experiences, such as adaptive learning software.
- Allow students to progress at their own pace, ensuring mastery of concepts before moving on.
- Use data-driven assessments to inform instruction and adjust learning paths as needed.
- Encourage student choice in learning activities, fostering engagement and motivation.
- Research shows that personalized learning can lead to higher student achievement and satisfaction.
- Promote collaboration among educators, students, and parents to create a supportive learning environment.
8.3. Resource Allocation
Effective resource allocation is essential for maximizing the impact of educational programs and ensuring that all students have access to the tools they need to succeed. This involves strategically distributing financial, human, and material resources.
- Assess the needs of students and schools to determine where resources are most needed.
- Prioritize funding for programs that have demonstrated effectiveness in improving student outcomes, including those related to early childhood intervention and early intervention speech pathology.
- Invest in professional development for teachers to enhance their skills and instructional strategies.
- Ensure equitable distribution of resources, particularly in underserved communities.
- Monitor and evaluate the impact of resource allocation to make informed decisions for future investments.
- Studies indicate that schools with adequate resources see improved student performance and engagement.
- Foster partnerships with local businesses and organizations to supplement resources and support educational initiatives.
How Rapid Innovation Can Help
At Rapid Innovation, we leverage AI and Blockchain technologies to enhance the effectiveness of educational programs, including early intervention, personalized learning, and resource allocation.
- AI-Driven Insights: Our AI solutions can analyze data from assessments to identify children in need of early intervention, ensuring timely support and better outcomes.
- Personalized Learning Solutions: We develop adaptive learning platforms that create personalized learning paths for students, allowing them to progress at their own pace and enhancing engagement.
- Blockchain for Resource Management: By utilizing Blockchain technology, we can ensure transparent and equitable distribution of resources, tracking funding and resource allocation in real-time.
By integrating these advanced technologies, Rapid Innovation helps educational institutions achieve greater ROI, improve student outcomes, and foster a more effective learning environment.
8.4. Curriculum Design
Curriculum design is a critical aspect of educational institutions, focusing on creating a structured framework for teaching and learning. A well-designed curriculum aligns educational goals with student needs, ensuring that learners acquire the necessary knowledge and skills. This includes elements such as curriculum vitae graphic design and purposeful design curriculum.
- Alignment with Standards: The curriculum should meet national and state educational standards, ensuring that students are prepared for future academic and career opportunities.
- Inclusivity: A diverse curriculum accommodates various learning styles and backgrounds, promoting equity in education.
- Interdisciplinary Approach: Integrating subjects encourages critical thinking and helps students make connections between different fields of study, such as curriculum and design.
- Continuous Improvement: Regularly reviewing and updating the curriculum based on feedback from students, faculty, and industry trends is essential for maintaining relevance. This can involve purposeful designs that enhance the learning experience.
- Technology Integration: Incorporating technology into the curriculum enhances learning experiences and prepares students for a digital world. Rapid Innovation can assist educational institutions in implementing AI-driven tools that personalize learning experiences, thereby increasing student engagement and retention. This includes tools for curriculum vitae design and curriculum vitae indesign.
- Assessment Strategies: Effective assessment methods should be embedded within the curriculum to evaluate student progress and inform instructional practices. Utilizing AI analytics can provide real-time insights into student performance, allowing for timely interventions. Instructional designer cv can be a useful resource for developing these strategies. Additionally, exploring how NFTs can revolutionize academic credential verification can enhance the credibility of the curriculum.
8.5. Student Support Services
Student support services play a vital role in enhancing the overall educational experience. These services are designed to assist students in overcoming academic, personal, and social challenges.
- Academic Advising: Providing guidance on course selection, degree requirements, and career planning helps students navigate their educational journey.
- Tutoring and Learning Centers: Offering additional academic support through tutoring services can improve student performance and retention rates.
- Mental Health Services: Access to counseling and mental health resources is crucial for supporting students' emotional well-being.
- Financial Aid Assistance: Helping students understand and access financial aid options can alleviate financial burdens and promote enrollment.
- Career Services: Providing resources for internships, job placements, and resume building prepares students for the workforce.
- Extracurricular Activities: Encouraging participation in clubs and organizations fosters a sense of community and enhances personal development.
8.6. Institutional Strategic Planning

Institutional strategic planning is a systematic process that outlines an educational institution's long-term goals and objectives. This planning is essential for ensuring sustainability and growth.
- Vision and Mission Statements: Clearly defined vision and mission statements guide the institution's direction and decision-making processes.
- Stakeholder Engagement: Involving faculty, staff, students, and community members in the planning process ensures diverse perspectives and buy-in.
- Data-Driven Decision Making: Utilizing data analytics to assess institutional performance and identify areas for improvement is crucial for effective planning. Rapid Innovation can provide advanced AI solutions that analyze institutional data, enabling informed decision-making.
- Resource Allocation: Strategic planning helps prioritize resource allocation, ensuring that funds and personnel are directed toward key initiatives.
- Performance Metrics: Establishing measurable goals allows institutions to track progress and make necessary adjustments to strategies.
- Adaptability: A successful strategic plan should be flexible, allowing institutions to respond to changing educational landscapes and emerging challenges. Blockchain technology can enhance adaptability by providing secure and transparent data management, facilitating quick adjustments to strategies as needed. This adaptability is essential in areas such as the design of the curriculum and create curriculum initiatives, including specialized programs like berean builders earth science.
9. Advanced Predictive Capabilities
Advanced predictive capabilities, such as predictive analytics and predictive modeling, are transforming various industries by leveraging data to forecast future trends, behaviors, and outcomes. These capabilities are particularly significant in fields such as healthcare, finance, marketing, and technology. By utilizing sophisticated algorithms and machine learning techniques, organizations can make informed decisions and enhance their strategic planning.
9.1 Multimodal Learning Analysis
Multimodal learning analysis refers to the integration of multiple data types and sources to improve predictive accuracy. This approach combines various modalities, such as text, images, audio, and video, to create a more comprehensive understanding of the data.
- Enhanced data interpretation: By analyzing different types of data together, organizations can uncover insights that may not be visible when examining a single modality.
- Improved model performance: Multimodal learning can lead to better predictive models, as it captures the complexity of real-world scenarios.
- Applications across industries: This technique is used in various fields, including:
- Healthcare: Combining patient records, imaging data, and genetic information to predict disease outcomes, utilizing predictive analytics in healthcare.
- Marketing: Analyzing customer reviews, social media interactions, and purchase history to forecast consumer behavior through predictive data analytics.
- Autonomous vehicles: Integrating sensor data, camera feeds, and GPS information to enhance navigation and safety.
At Rapid Innovation, we harness the power of multimodal learning analysis to help our clients achieve greater ROI by developing tailored predictive models that drive strategic decision-making and operational efficiency, including power bi predictive analytics.
9.2 Emotional Intelligence Prediction
Emotional intelligence prediction involves using data analytics and machine learning to assess and predict emotional responses and behaviors. This capability is increasingly important in areas such as human resources, customer service, and mental health.
- Understanding emotional dynamics: By analyzing verbal and non-verbal cues, organizations can gain insights into individuals' emotional states, which can inform decision-making processes.
- Enhancing interpersonal interactions: Predicting emotional responses can help improve communication and relationships within teams and with customers.
- Applications in various sectors:
- Human resources: Assessing candidates' emotional intelligence during the hiring process to ensure better team fit and performance.
- Customer service: Analyzing customer interactions to predict satisfaction levels and tailor responses accordingly.
- Mental health: Utilizing data from wearable devices and social media to identify individuals at risk of emotional distress, including predictive healthcare approaches.
At Rapid Innovation, we implement emotional intelligence prediction models that empower organizations to enhance employee engagement and customer satisfaction, ultimately leading to improved retention rates and business performance.
By integrating advanced predictive capabilities like multimodal learning analysis and emotional intelligence prediction, organizations can enhance their decision-making processes, improve customer experiences, and foster a more productive work environment. Rapid Innovation is committed to guiding clients through this transformative journey, ensuring they leverage these technologies, such as prescriptive analytics and azure predictive analytics, for maximum impact and ROI. Additionally, we offer custom AI model development to meet specific business needs.
9.3. Career Trajectory Forecasting
Career trajectory forecasting involves predicting the potential career paths individuals may take based on various factors such as skills, education, experience, and market trends. This process is essential for both employees and employers to understand future opportunities and prepare accordingly.
- Utilizes data analytics to assess career progression patterns.
- Incorporates labor market trends to identify high-demand roles.
- Helps individuals make informed decisions about further education or skill development.
- Assists organizations in workforce planning and talent management.
- Can be enhanced with machine learning algorithms to improve accuracy over time.
At Rapid Innovation, we leverage advanced AI techniques to provide precise career trajectory forecasting, enabling organizations to align their training programs with future needs. This proactive approach not only benefits the workforce but also enhances organizational performance, ultimately leading to greater ROI. If you're looking to enhance your capabilities, consider our services to hire generative AI engineers.
9.4. Skill Gap Identification
Skill gap identification is the process of recognizing the discrepancies between the skills that employees currently possess and the skills required to meet organizational goals. This analysis is crucial for maintaining a competitive edge in today's fast-paced business environment.
- Involves assessing current employee skills through surveys and performance reviews.
- Compares existing skills against industry benchmarks and future job requirements.
- Identifies specific areas where training and development are needed.
- Supports strategic workforce planning by highlighting potential hiring needs.
- Encourages a culture of continuous learning and professional development.
By identifying skill gaps, organizations can implement targeted training programs, ensuring that their workforce remains relevant and capable of meeting evolving demands. Rapid Innovation employs AI-driven analytics to facilitate this process, enhancing employee satisfaction and driving overall business success.
9.5. Adaptive Recommendation Systems
Adaptive recommendation systems are advanced tools that provide personalized suggestions based on user behavior, preferences, and needs. In the context of career development and skill enhancement, these systems play a vital role in guiding individuals toward suitable learning opportunities and career paths.
- Leverages algorithms to analyze user data and predict preferences.
- Offers tailored recommendations for courses, certifications, and job opportunities.
- Adapts to changes in user behavior, ensuring ongoing relevance of suggestions.
- Enhances user engagement by providing a personalized experience.
- Can integrate with existing learning management systems for seamless access.
By utilizing adaptive recommendation systems, organizations can foster a more engaged and skilled workforce. Rapid Innovation's expertise in AI allows us to create customized solutions that empower employees to take charge of their career development while helping organizations retain top talent by providing meaningful growth opportunities.
9.6. Predictive Mental Health Support
Predictive mental health support leverages advanced analytics and machine learning to anticipate mental health issues before they escalate. This proactive approach can significantly enhance the quality of care provided to individuals.
- Early identification of mental health conditions can lead to timely interventions, reducing the severity of symptoms.
- Predictive models analyze various data sources, including social media activity, wearable device data, and electronic health records, to identify patterns indicative of mental health decline.
- These models can help healthcare providers tailor interventions based on individual risk factors, improving treatment outcomes.
- By utilizing algorithms, predictive mental health support can also assist in resource allocation, ensuring that those at higher risk receive the necessary attention.
- The integration of AI in mental health care can facilitate continuous monitoring, allowing for real-time adjustments to treatment plans.
The potential of predictive mental health support is vast, but it requires careful implementation to ensure ethical considerations are met. For more information on the role of AI in this field, check out our article on AI for Mental Health Care.
10. Challenges and Limitations
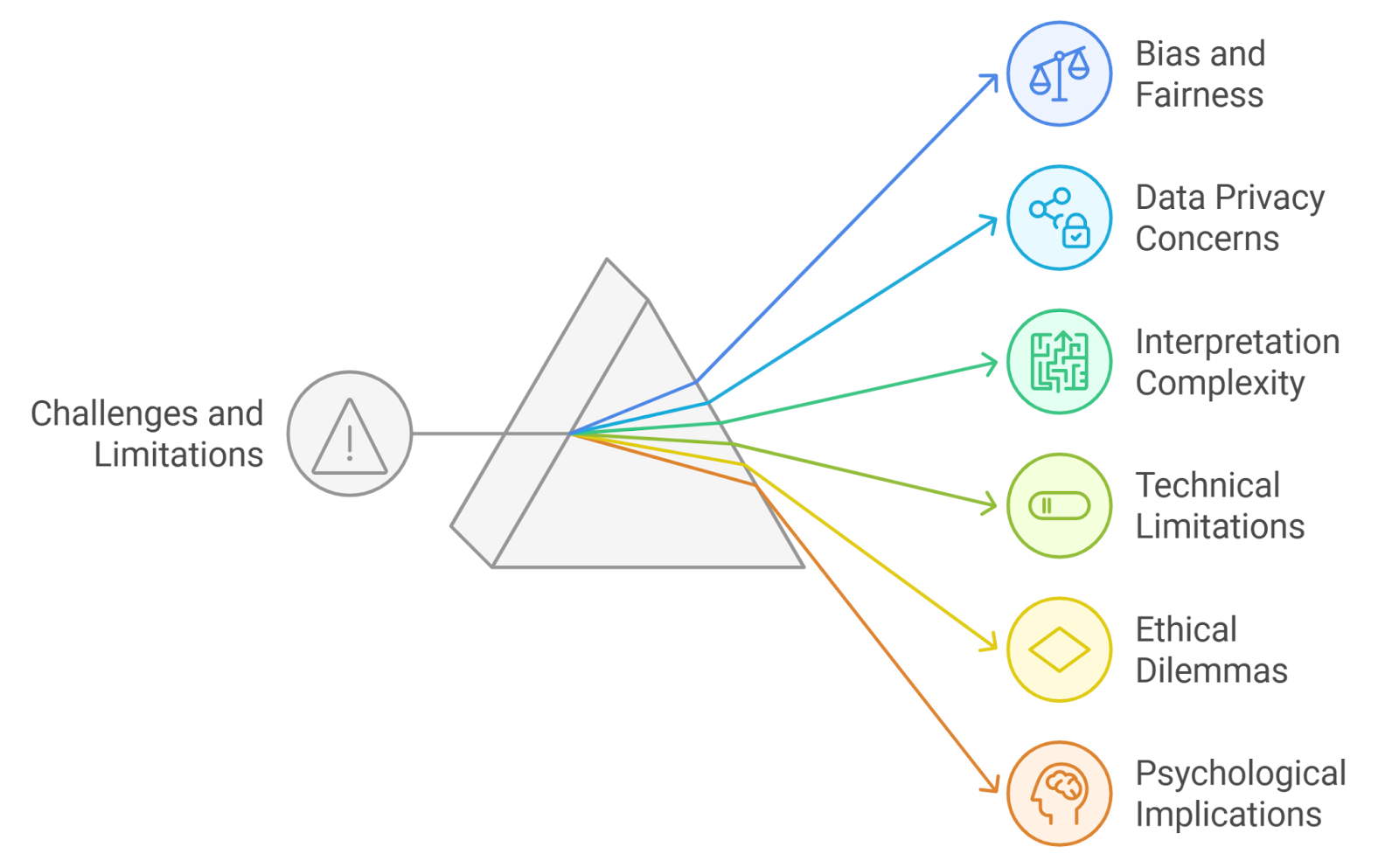
While predictive mental health support offers numerous benefits, it also faces several challenges and limitations that must be addressed to ensure its effectiveness and ethical use.
- Data Privacy: The collection and analysis of personal data raise significant privacy concerns. Individuals may be hesitant to share sensitive information, fearing misuse or breaches.
- Accuracy of Predictions: Predictive models are only as good as the data they are trained on. Inaccurate predictions can lead to misdiagnosis or unnecessary anxiety for individuals.
- Accessibility: Not all individuals have equal access to technology or mental health resources, which can exacerbate existing disparities in mental health care.
Addressing these challenges is crucial for the successful implementation of predictive mental health support systems.
10.1. Bias and Fairness
Bias and fairness are critical issues in the development and deployment of predictive mental health support systems.
- Data Bias: Predictive models can inherit biases present in the training data. If the data is not representative of diverse populations, the model may perform poorly for underrepresented groups.
- Algorithmic Fairness: Ensuring that algorithms do not perpetuate existing inequalities is essential. This includes evaluating how different demographic factors, such as race, gender, and socioeconomic status, influence predictions.
- Transparency: The lack of transparency in how predictive models operate can lead to mistrust among users. Clear communication about how data is used and how predictions are made is vital for fostering trust.
To mitigate bias and ensure fairness, it is essential to implement rigorous testing and validation processes, engage diverse stakeholders in the development process, and continuously monitor outcomes to identify and address disparities.
At Rapid Innovation, we understand the complexities of implementing predictive mental health support systems. Our expertise in AI and advanced analytics allows us to assist clients in developing tailored solutions that not only enhance patient care but also address ethical considerations, ensuring compliance with data privacy regulations. By leveraging our capabilities, clients can achieve greater ROI through improved treatment outcomes and optimized resource allocation, ultimately leading to a more effective mental health care system. For more information on our services, visit our AI Healthcare Management page.
10.2. Data Privacy Concerns
Data privacy concerns have become increasingly prominent in today's digital landscape. As organizations collect vast amounts of personal data, the risk of breaches and misuse escalates. Key aspects of data privacy concerns include:
- Personal Data Exposure: With the rise of data breaches, sensitive information such as names, addresses, and financial details can be exposed. According to a report by the Identity Theft Resource Center, there were over 1,100 data breaches in 2020 alone, affecting millions of individuals. This is particularly concerning in the context of AI and privacy, where the misuse of personal data can lead to significant privacy issues.
- Regulatory Compliance: Organizations must navigate complex regulations like GDPR and CCPA, which impose strict guidelines on data collection and usage. Non-compliance can lead to hefty fines and reputational damage. Rapid Innovation assists clients in implementing robust data governance frameworks that ensure compliance with these regulations, thereby minimizing legal risks and enhancing trust. This is especially relevant for AI privacy concerns, as organizations must ensure their AI systems adhere to these regulations.
- User Trust: Consumers are becoming more aware of their data rights and are increasingly concerned about how their information is used. A survey by Pew Research Center found that 79% of Americans are concerned about how companies use their data. By leveraging blockchain technology, Rapid Innovation can help organizations create transparent data usage policies that foster user trust and engagement. This is crucial in addressing AI privacy issues and building confidence in AI-driven solutions.
- Data Ownership: Questions about who owns the data and how it can be used are becoming more prevalent. Users often feel they have little control over their personal information once it is shared online. Rapid Innovation can implement decentralized solutions that empower users with greater control over their data, enhancing their sense of ownership. This is particularly important in discussions around big data and privacy, where ownership and control are often contested.
- Anonymization Challenges: While anonymizing data can protect privacy, it is not foolproof. Techniques can sometimes be reversed, leading to the re-identification of individuals. Our AI-driven solutions can enhance anonymization techniques, making it more difficult to re-identify individuals while still allowing for valuable data insights. This is a significant concern in the realm of biometric privacy concerns, where re-identification can have serious implications.
10.3. Interpretation Complexity
Interpretation complexity refers to the challenges associated with understanding and analyzing data accurately. This complexity can arise from various factors:
- Data Volume: The sheer volume of data generated can overwhelm analysts. Big data analytics tools are often required to sift through and make sense of large datasets. Rapid Innovation provides advanced AI analytics solutions that can efficiently process and analyze large volumes of data, enabling organizations to derive actionable insights quickly. This is particularly relevant in the context of big data privacy issues, where managing large datasets responsibly is crucial.
- Data Variety: Data comes in various formats, including structured, semi-structured, and unstructured. Each type requires different analytical approaches, complicating interpretation. Our expertise in AI allows us to develop tailored solutions that can handle diverse data types, ensuring comprehensive analysis. This is essential for addressing privacy issues with AI, as different data types may have varying implications for privacy.
- Contextual Understanding: Data must be interpreted within the right context. Misinterpretation can lead to incorrect conclusions, impacting decision-making processes. Rapid Innovation employs AI algorithms that enhance contextual understanding, allowing organizations to make informed decisions based on accurate data interpretations. This is particularly important in the context of data mining and privacy, where context can significantly affect the interpretation of data.
- Bias in Data: Data can be biased due to how it is collected or the demographics of the sample. This bias can skew results and lead to misleading interpretations. Our AI solutions include bias detection mechanisms that help organizations identify and mitigate bias in their data, leading to more reliable outcomes. Addressing bias is crucial in discussions around AI privacy issues, as biased data can lead to unfair treatment of individuals.
- Evolving Data: Data is not static; it changes over time. Keeping up with these changes and understanding their implications can be challenging for analysts. Rapid Innovation's AI systems are designed to adapt to evolving data landscapes, ensuring that organizations remain agile and responsive to new information. This adaptability is vital in the context of big data and privacy, where the landscape is constantly shifting.
10.4. Technical Limitations
Technical limitations can hinder the effective use of data and analytics. These limitations can manifest in several ways:
- Infrastructure Constraints: Organizations may lack the necessary infrastructure to support advanced data analytics. This includes hardware, software, and network capabilities. Rapid Innovation offers cloud-based solutions that provide scalable infrastructure, enabling organizations to leverage advanced analytics without significant upfront investments. This is particularly relevant for organizations dealing with AI and privacy, as robust infrastructure is essential for secure data handling.
- Skill Gaps: There is often a shortage of skilled professionals who can effectively analyze and interpret data. This skills gap can limit an organization's ability to leverage data for strategic advantage. Our consulting services include training and upskilling programs that empower teams to harness the full potential of data analytics. Addressing this gap is crucial for organizations looking to navigate the complexities of data privacy concerns.
- Integration Issues: Combining data from different sources can be technically challenging. Incompatibilities between systems can lead to data silos, making it difficult to obtain a holistic view. Rapid Innovation specializes in developing integration solutions that unify disparate data sources, providing organizations with a comprehensive view of their data landscape. This is particularly important in the context of big data privacy issues, where a unified view can enhance data governance.
- Data Quality: Poor data quality can significantly impact analysis. Inaccurate, incomplete, or outdated data can lead to erroneous conclusions and decisions. Our AI-driven data cleansing solutions ensure high data quality, enabling organizations to make decisions based on reliable information. Ensuring data quality is essential for addressing AI privacy issues, as poor data can lead to privacy violations.
- Scalability Challenges: As data volumes grow, systems must be able to scale accordingly. Many organizations struggle to scale their analytics capabilities to keep pace with increasing data demands. Rapid Innovation's scalable solutions are designed to grow with your organization, ensuring that you can continue to derive value from your data as it expands. This scalability is crucial for organizations dealing with big data and privacy, as they must be able to adapt to growing data needs while maintaining privacy standards.
10.5. Ethical Dilemmas
Ethical dilemmas arise in various contexts, particularly in fields such as medicine, business, and technology. These dilemmas often involve situations where moral principles conflict, making it challenging to determine the right course of action.
- Conflicting Values: Individuals may face situations where their personal values clash with professional responsibilities. For example, a healthcare provider may struggle between patient confidentiality and the need to report a communicable disease.
- Decision-Making Challenges: Ethical dilemmas often require individuals to make tough choices that can have significant consequences. This can lead to stress and anxiety, as the decision-maker weighs the potential outcomes of their actions. Examples of ethical problems in business can illustrate these challenges.
- Impact on Stakeholders: Ethical decisions can affect various stakeholders, including employees, customers, and the community. For instance, a company may face a dilemma between maximizing profits and ensuring fair labor practices, which is a common ethical dilemma in the workplace.
- Regulatory Compliance: Organizations must navigate complex legal frameworks that govern ethical behavior. Failure to comply can result in legal repercussions and damage to reputation. Current ethical issues in business often highlight the importance of regulatory compliance.
- Cultural Differences: Ethical standards can vary significantly across cultures, leading to misunderstandings and conflicts in international business or healthcare settings. Companies with ethical dilemmas may struggle to reconcile these differences.
10.6. Psychological Implications
The psychological implications of ethical dilemmas can be profound, affecting both individuals and organizations. Understanding these implications is crucial for fostering a healthy work environment and promoting ethical behavior.
- Stress and Anxiety: Individuals faced with ethical dilemmas often experience heightened levels of stress and anxiety. The pressure to make the right decision can lead to mental fatigue and burnout, especially in situations involving ethical problems in accounting.
- Moral Distress: Professionals, particularly in healthcare, may experience moral distress when they are unable to act according to their ethical beliefs. This can lead to feelings of guilt and frustration, as seen in many ethical dilemma examples in business.
- Decision Fatigue: Constantly facing ethical dilemmas can lead to decision fatigue, where individuals become overwhelmed by the need to make choices. This can result in poor decision-making or avoidance of difficult situations, which is a common issue in ethical dilemmas in business today.
- Impact on Mental Health: Prolonged exposure to ethical dilemmas can contribute to mental health issues, including depression and anxiety disorders. It is essential for organizations to provide support systems for employees facing these challenges, particularly in the context of ethical issues in the workplace.
- Organizational Culture: The way an organization addresses ethical dilemmas can shape its culture. A supportive environment encourages open discussions about ethics, while a punitive approach may lead to fear and silence. Real-life examples of ethical dilemmas in business can serve as valuable lessons for fostering a positive organizational culture.
11. Technological Integration
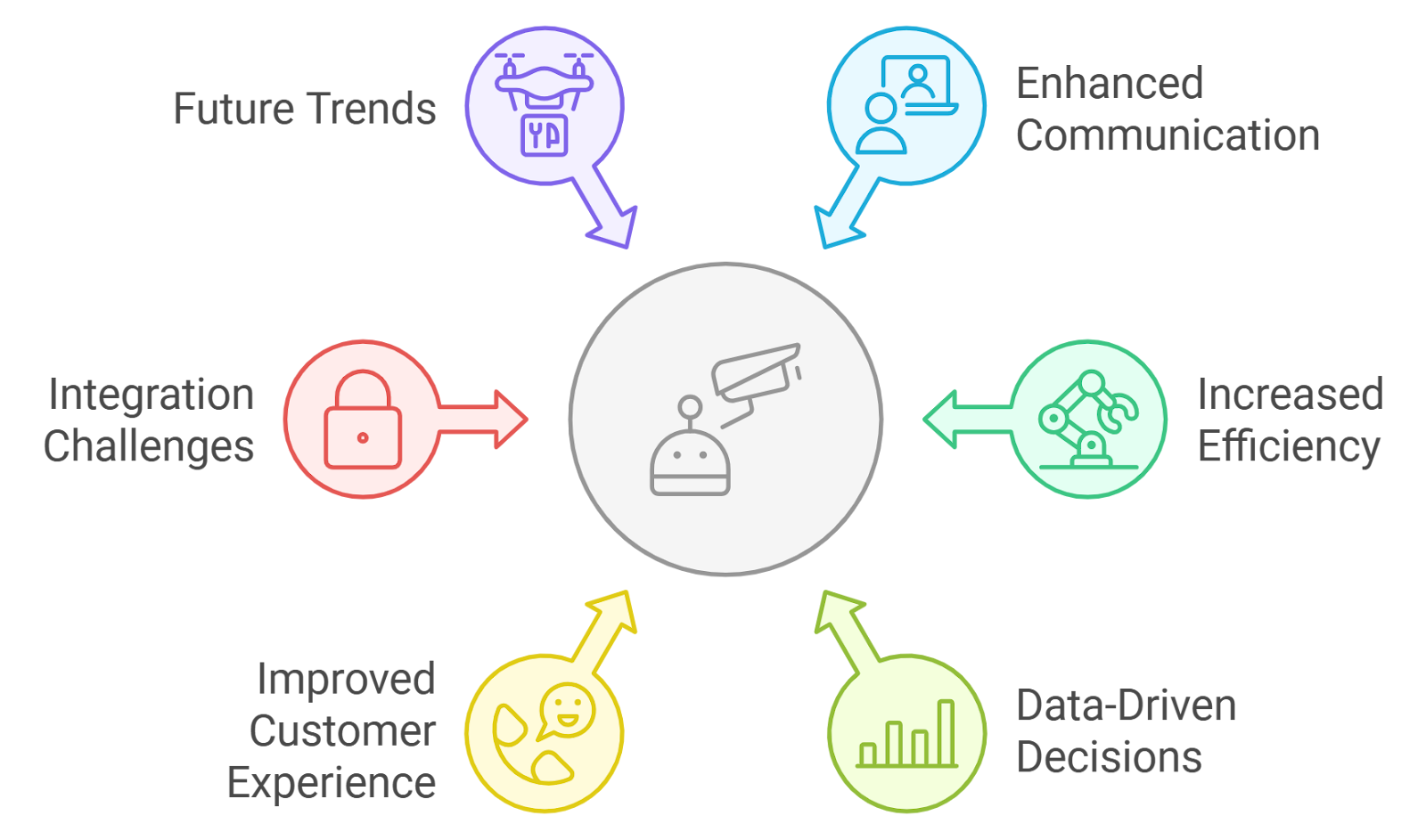
Technological integration refers to the incorporation of technology into various aspects of business and daily life. This process can enhance efficiency, improve communication, and drive innovation.
- Enhanced Communication: Technology facilitates seamless communication within organizations. Tools like instant messaging, video conferencing, and collaborative platforms enable teams to work together effectively, regardless of location.
- Increased Efficiency: Automation and advanced software solutions streamline processes, reducing the time and effort required for tasks. This can lead to increased productivity and cost savings for businesses.
- Data-Driven Decision Making: The integration of technology allows organizations to collect and analyze vast amounts of data. This data-driven approach enables informed decision-making and helps identify trends and opportunities.
- Improved Customer Experience: Technology enhances customer interactions through personalized services and support. Businesses can leverage customer data to tailor their offerings, leading to higher satisfaction and loyalty.
- Challenges of Integration: While technological integration offers numerous benefits, it also presents challenges. Organizations must address issues such as cybersecurity, employee training, and resistance to change to ensure successful implementation.
- Future Trends: As technology continues to evolve, organizations must stay ahead of trends such as artificial intelligence, machine learning, and the Internet of Things (IoT). Embracing these advancements can provide a competitive edge in the marketplace.
At Rapid Innovation, we understand the complexities of ethical dilemmas and the psychological implications they carry. Our expertise in AI and Blockchain technology can help organizations navigate these challenges effectively. By implementing AI-driven decision-making tools, we enable businesses to analyze ethical scenarios and their potential impacts on stakeholders, leading to more informed and responsible choices. Additionally, our Blockchain solutions ensure transparency and accountability, fostering trust among stakeholders and enhancing compliance with regulatory frameworks. Through our tailored consulting services, we empower organizations to create a supportive culture that prioritizes ethical behavior while leveraging technology for greater efficiency and innovation.
11.1. Learning Management System Integration
Learning Management System (LMS) integration is crucial for educational institutions aiming to streamline their teaching and learning processes. An LMS serves as a centralized platform where educators can manage course materials, track student progress, and facilitate communication.
- Seamless Data Flow: Integrating an LMS with other systems, such as Student Information Systems (SIS) and content management systems, ensures a smooth flow of data. This reduces redundancy and minimizes errors in student records, ultimately enhancing operational efficiency. Integration LMS with various platforms can significantly improve this process.
- Enhanced User Experience: A well-integrated LMS provides a user-friendly interface for both students and educators, encouraging engagement and improving the overall learning experience. Rapid Innovation can assist in customizing the LMS to align with specific institutional needs, including API LMS solutions.
- Customization and Scalability: Institutions can customize their LMS to meet specific needs, such as adding plugins or tools that enhance functionality. As the institution grows, the LMS can scale to accommodate more users and courses, ensuring long-term viability. This is particularly important for LMS integration standards.
- Analytics and Reporting: Integration allows for better data analytics, enabling educators to track student performance and engagement. This data can inform instructional strategies and improve learning outcomes, leading to a more effective educational environment. Learning management system integrations can provide valuable insights.
- Cost Efficiency: By integrating various systems, institutions can reduce operational costs and improve resource allocation, leading to better financial management and sustainability. Rapid Innovation's expertise in AI can further optimize these processes, enhancing ROI. Docebo integrations can also contribute to cost efficiency.
11.2. Student Information Systems
Student Information Systems (SIS) are essential for managing student data and administrative processes in educational institutions. An effective SIS helps streamline operations and improve communication among stakeholders.
- Centralized Data Management: An SIS centralizes student records, including enrollment, grades, attendance, and financial information, ensuring that all data is easily accessible and up-to-date. This centralization is vital for informed decision-making.
- Improved Communication: SIS platforms often include communication tools that facilitate interaction between students, parents, and educators. This enhances transparency and keeps everyone informed about academic progress and important announcements.
- Compliance and Reporting: Educational institutions must comply with various regulations and reporting requirements. An SIS simplifies this process by automating data collection and generating reports, ensuring compliance with local and federal guidelines.
- Enhanced Student Services: With an SIS, institutions can offer better services to students, such as online registration, course selection, and access to academic resources, improving the overall student experience and satisfaction.
- Data Security: Protecting sensitive student information is paramount. A robust SIS includes security measures to safeguard data against breaches and unauthorized access, ensuring trust and reliability.
11.3. Cloud Computing Strategies
Cloud computing strategies are transforming how educational institutions manage their IT resources and deliver services. By leveraging cloud technology, schools and universities can enhance their operational efficiency and improve accessibility.
- Cost Savings: Cloud computing reduces the need for expensive on-premises hardware and maintenance. Institutions can pay for only the resources they use, leading to significant cost savings.
- Scalability: Cloud solutions allow institutions to scale their IT resources up or down based on demand. This flexibility is particularly beneficial during peak enrollment periods or when launching new programs.
- Enhanced Collaboration: Cloud-based tools facilitate collaboration among students and educators, enabling real-time sharing of resources and information. This fosters a more interactive and engaging learning environment.
- Disaster Recovery: Cloud computing offers robust disaster recovery solutions, ensuring that critical data is backed up and can be restored quickly in case of an emergency. This minimizes downtime and protects institutional data.
- Accessibility: Cloud services enable students and educators to access resources from anywhere, at any time, using any device. This promotes a more flexible learning environment and supports remote education initiatives.
By leveraging Rapid Innovation's expertise in AI and Blockchain, educational institutions can not only integrate these systems effectively but also enhance their overall operational efficiency, leading to greater ROI and improved educational outcomes. The integration of tools like Microsoft Teams LMS and Moodle Salesforce integration can further enhance the learning experience.
11.4. Edge Computing Applications
Edge computing refers to the practice of processing data closer to the source of data generation rather than relying solely on centralized data centers. This approach significantly reduces latency and bandwidth usage, making it ideal for various applications.
- Real-Time Data Processing: Edge computing enables real-time analytics, which is crucial for applications like autonomous vehicles and industrial automation. By processing data on-site, these systems can make immediate decisions without the delay of sending data to a remote server. Rapid Innovation can assist clients in implementing edge computing solutions that enhance operational efficiency and drive faster decision-making. This is particularly relevant in the context of mobile edge computing and its applications in 5G networks.
- Smart Cities: In smart city initiatives, edge computing supports traffic management systems, waste management, and public safety applications. Sensors deployed throughout the city can analyze data locally to optimize traffic flow or monitor environmental conditions. Our expertise in AI and blockchain can help municipalities leverage these technologies for improved urban management and citizen engagement, showcasing examples of edge computing in action.
- Healthcare: In healthcare, edge computing can facilitate remote patient monitoring and telemedicine. Wearable devices can process health data in real-time, alerting healthcare providers to any anomalies without the need for constant data transmission to a central server. Rapid Innovation can develop tailored solutions that enhance patient care and streamline healthcare operations, including applications of edge computing in healthcare settings.
- Retail: Retailers use edge computing to enhance customer experiences through personalized marketing and inventory management. By analyzing customer behavior in-store, businesses can adjust their strategies on the fly. Our team can help retailers implement edge solutions that drive customer engagement and optimize inventory levels, resulting in greater ROI. This is part of the broader trend of edge computing applications across various industries.
- Manufacturing: In manufacturing, edge computing can monitor machinery and equipment in real-time, predicting maintenance needs and reducing downtime. This leads to increased efficiency and cost savings. Rapid Innovation can provide clients with advanced edge computing solutions that enhance productivity and minimize operational disruptions, including industrial edge computing applications.
11.5. IoT and Wearable Technologies
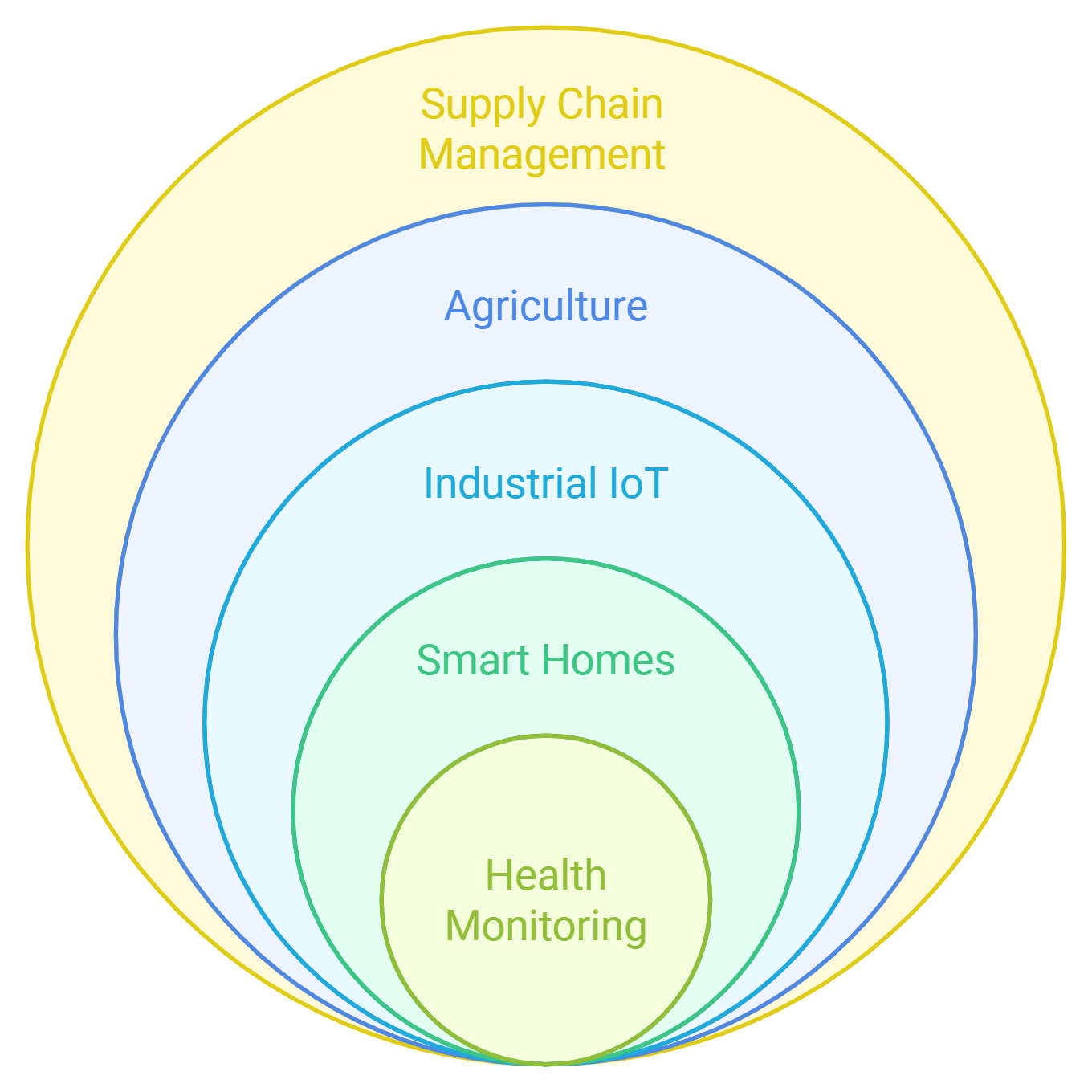
The Internet of Things (IoT) and wearable technologies are transforming how we interact with the world around us. These technologies enable seamless connectivity and data exchange between devices, enhancing user experiences and operational efficiencies.
- Health Monitoring: Wearable devices like smartwatches and fitness trackers monitor vital signs, activity levels, and sleep patterns. This data can be shared with healthcare providers for better health management. Rapid Innovation can develop IoT solutions that empower healthcare providers with actionable insights for improved patient outcomes, leveraging mobile edge computing for real-time data processing.
- Smart Homes: IoT devices in smart homes allow users to control lighting, heating, and security systems remotely. This not only enhances convenience but also improves energy efficiency. Our expertise can help clients create smart home solutions that enhance user experience and drive energy savings, integrating edge computing for enhanced performance.
- Industrial IoT (IIoT): In industrial settings, IoT devices monitor equipment performance and environmental conditions. This data helps in predictive maintenance and optimizing production processes. Rapid Innovation can assist manufacturers in deploying IIoT solutions that enhance operational efficiency and reduce costs, utilizing edge computing infrastructure for better data management.
- Agriculture: IoT technologies are used in precision agriculture to monitor soil conditions, crop health, and weather patterns. This data-driven approach helps farmers make informed decisions, leading to increased yields. Our team can develop IoT applications that support sustainable agricultural practices and improve productivity, showcasing the role of edge computing in agriculture.
- Supply Chain Management: Wearable technologies and IoT devices improve supply chain visibility. RFID tags and GPS trackers provide real-time data on inventory levels and shipment locations, enhancing logistics efficiency. Rapid Innovation can help businesses implement IoT solutions that streamline supply chain operations and enhance transparency, integrating edge computing for real-time insights.
11.6. Cross-Platform Compatibility
Cross-platform compatibility refers to the ability of software applications to function across different operating systems and devices. This is increasingly important in a world where users expect seamless experiences regardless of the platform they are using.
- User Experience: Ensuring cross-platform compatibility enhances user experience by providing consistent functionality and design across devices. This is crucial for applications that users access on both mobile and desktop platforms. Rapid Innovation can help clients design applications that deliver a unified experience across all platforms, including those leveraging edge computing technologies.
- Development Efficiency: Cross-platform development frameworks, such as
React Native
andFlutter
, allow developers to write code once and deploy it across multiple platforms. This reduces development time and costs. Our expertise in these frameworks enables us to deliver high-quality applications efficiently, including those that utilize edge computing capabilities. - Wider Audience Reach: Applications that are compatible with various platforms can reach a broader audience. This is particularly important for businesses looking to maximize their market presence. Rapid Innovation can assist clients in expanding their reach through cross-platform solutions that cater to diverse user bases, including those in the edge computing space.
- Maintenance and Updates: Cross-platform applications simplify maintenance and updates. Developers can implement changes in one codebase, ensuring that all users receive the latest features and security updates simultaneously. Our team can provide ongoing support to ensure that applications remain up-to-date and secure, including those that integrate edge computing functionalities.
- Integration with Other Technologies: Cross-platform compatibility facilitates integration with other technologies, such as IoT devices and cloud services. This interoperability is essential for creating comprehensive solutions that leverage multiple technologies. Rapid Innovation can help clients develop integrated solutions that harness the power of AI, blockchain, and IoT for enhanced business outcomes, including those that utilize mobile edge computing and fog computing architectures.
12. Economic and Strategic Implications
Understanding the economic and strategic implications of a project or investment is crucial for decision-making. This involves analyzing both the costs and benefits associated with the initiative, as well as evaluating the potential return on investment (ROI). At Rapid Innovation, we leverage our expertise in AI and Blockchain to provide clients with insights that enhance their decision-making processes, ultimately leading to greater efficiency and effectiveness in achieving business goals.
12.1 Cost-Benefit Analysis
A cost-benefit analysis (CBA) is a systematic approach to estimating the strengths and weaknesses of alternatives. It helps in determining the best approach to achieve benefits while minimizing costs.
- Definition: CBA involves comparing the total expected costs of a project against the total expected benefits, expressed in monetary terms. This is often referred to as a cost and benefit analysis.
- Components:
- Direct Costs: These include expenses directly tied to the project, such as materials, labor, and overhead.
- Indirect Costs: These are costs that are not directly accountable to a specific project, like administrative expenses.
- Tangible Benefits: These are measurable benefits, such as increased revenue or reduced operational costs.
- Intangible Benefits: These include non-monetary advantages, such as improved customer satisfaction or brand reputation.
- Steps in Conducting CBA:
- Identify the project scope and objectives.
- List all costs and benefits associated with the project.
- Assign monetary values to each cost and benefit.
- Calculate the net present value (NPV) to determine the project's viability.
- Importance:
- Helps stakeholders make informed decisions.
- Provides a clear framework for evaluating the feasibility of projects.
- Aids in prioritizing projects based on their economic impact.
A well-executed CBA can reveal whether a project is worth pursuing or if resources should be allocated elsewhere. For instance, when a client approached us to implement a blockchain solution for supply chain transparency, our cost benefit assessment highlighted not only the cost savings from reduced fraud but also the intangible benefits of enhanced brand trust.
12.2 Return on Investment
Return on Investment (ROI) is a key performance indicator used to evaluate the efficiency of an investment. It measures the gain or loss generated relative to the amount of money invested.
- Definition: ROI is calculated by dividing the net profit from an investment by the initial cost of the investment, often expressed as a percentage.
- Formula:
- -
language="language-roi"```ROI = (Net Profit / Cost of Investment) x 100
- Factors Influencing ROI:
- Time Frame: The duration over which the investment is held can significantly impact ROI. Short-term investments may yield quick returns, while long-term investments may require patience.
- Market Conditions: Economic factors, such as inflation rates and market demand, can affect the profitability of an investment.
- Operational Efficiency: The effectiveness of management and operational processes can enhance or diminish ROI.
- Benefits of Calculating ROI:
- Provides a clear metric for comparing different investment opportunities.
- Helps in assessing the financial viability of projects.
- Aids in strategic planning and resource allocation.
- Limitations:
- ROI does not account for the time value of money unless adjusted for NPV.
- It may overlook qualitative factors that could influence long-term success.
In conclusion, both cost-benefit analysis and return on investment are essential tools for evaluating the economic and strategic implications of projects. They provide a framework for making informed decisions that align with organizational goals and financial objectives. At Rapid Innovation, we utilize these methodologies, including cost benefit analysis examples and cost benefit analysis with examples, to ensure that our clients not only achieve their desired outcomes but also maximize their ROI through innovative AI and Blockchain solutions.
12.3. Competitive Advantages
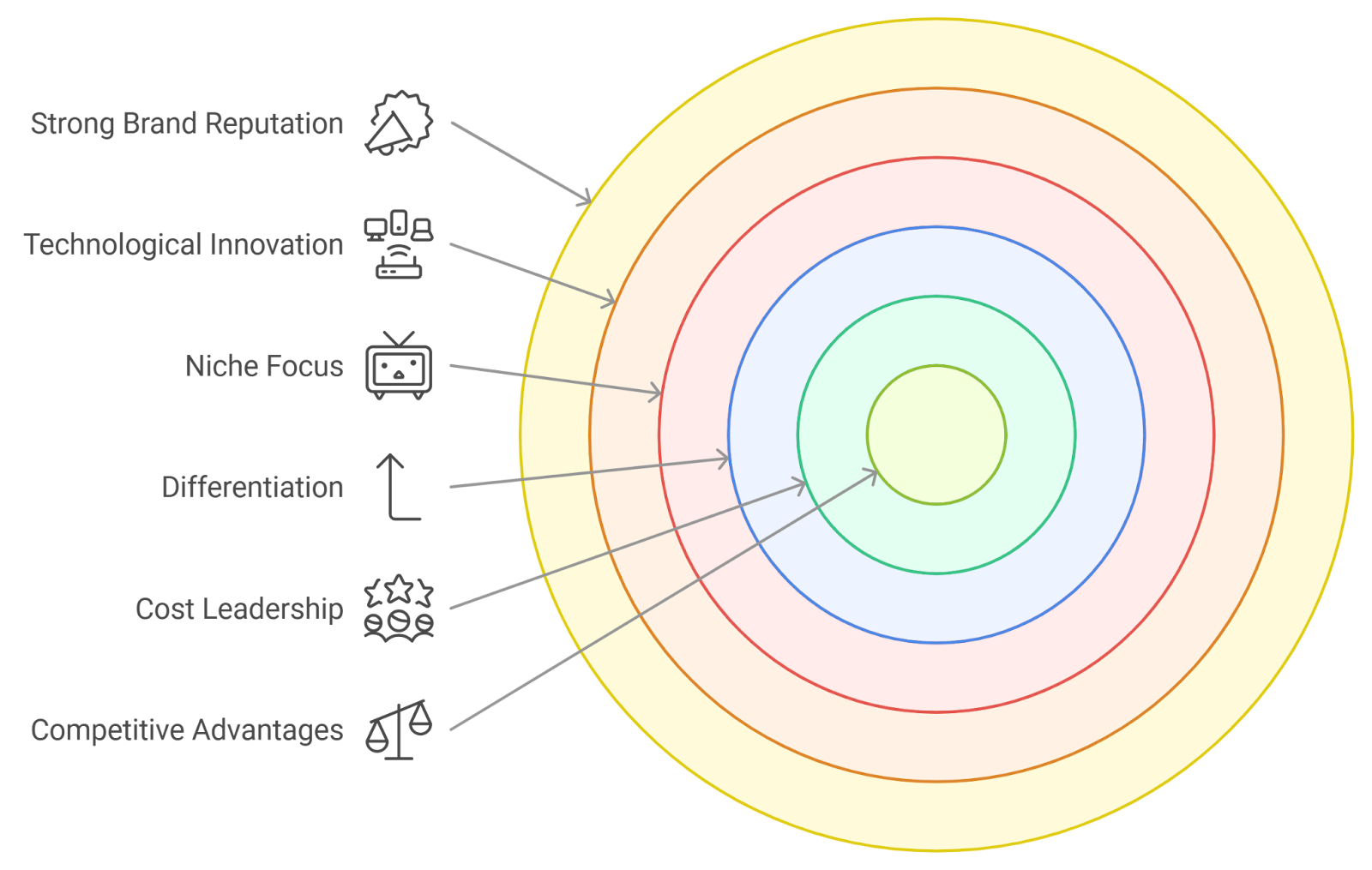
Competitive advantages are the unique attributes or benefits that allow a company to outperform its rivals. These advantages can stem from various factors, including:
- Cost Leadership: Companies that can produce goods or services at a lower cost than competitors can offer lower prices, attracting more customers. This strategy often involves economies of scale, efficient production processes, and cost-effective supply chain management. At Rapid Innovation, we utilize AI-driven analytics to optimize resource allocation and reduce operational costs, enabling our clients to achieve cost leadership in their respective markets.
- Differentiation: Businesses that offer unique products or services can create a strong brand identity. This can be achieved through superior quality, innovative features, or exceptional customer service. Rapid Innovation helps clients differentiate themselves by integrating advanced AI solutions and blockchain technology, enhancing product offerings and customer experiences, which allows them to charge premium prices and build customer loyalty. This is a key aspect of the concept of sustainable competitive advantage.
- Niche Focus: Some companies thrive by targeting specific market segments. By catering to the unique needs of a niche audience, these businesses can establish themselves as experts in their field, leading to customer trust and loyalty. Rapid Innovation assists clients in identifying and targeting niche markets through data-driven insights, ensuring they meet the specific demands of their audience effectively. This approach can be seen as a strategic competitive advantage.
- Technological Innovation: Companies that leverage cutting-edge technology can streamline operations, enhance product offerings, and improve customer experiences. Rapid Innovation specializes in AI and blockchain development, enabling our clients to adopt the latest technological advancements that lead to increased efficiency and a stronger market position. This aligns with Michael Porter’s views on competitive advantage.
- Strong Brand Reputation: A well-established brand can serve as a competitive advantage. Companies with a positive reputation often enjoy customer loyalty and can attract new customers more easily. By implementing robust blockchain solutions for transparency and security, Rapid Innovation helps clients build trust and credibility in their brands. This is crucial for maintaining a competitive advantage in business.
- Strategic Partnerships: Collaborating with other businesses can provide access to new markets, resources, and technologies. Strategic alliances can enhance a company's competitive position and foster innovation. Rapid Innovation actively seeks partnerships with industry leaders to provide our clients with comprehensive solutions that enhance their competitive edge. Companies with competitive advantage examples often leverage such partnerships.
12.4. Future Investment Strategies
Future investment strategies are essential for businesses looking to grow and adapt to changing market conditions. Key strategies include:
- Diversification: Investing in a variety of assets or markets can reduce risk. Companies may explore new product lines, geographic markets, or industries to spread their investment risk.
- Sustainable Investments: With increasing awareness of environmental issues, many companies are focusing on sustainable practices. Investing in green technologies and sustainable resources can not only improve a company's image but also lead to long-term cost savings.
- Digital Transformation: Embracing digital technologies is crucial for staying competitive. Companies should invest in digital tools, e-commerce platforms, and data analytics to enhance operational efficiency and customer engagement.
- Research and Development (R&D): Investing in R&D can lead to innovation and new product development. Companies that prioritize R&D are better positioned to adapt to market changes and meet evolving customer needs.
- Talent Development: Investing in employee training and development can enhance productivity and innovation. A skilled workforce is essential for executing business strategies effectively.
- Global Expansion: Exploring international markets can provide new growth opportunities. Companies should assess potential markets for expansion and consider factors such as local regulations, cultural differences, and market demand.
12.5. Market Trends
Understanding market trends is vital for businesses to remain competitive and relevant. Key market trends include:
- Digitalization: The shift towards digital platforms continues to grow. Businesses are increasingly adopting e-commerce, social media marketing, and online customer engagement strategies.
- Sustainability: Consumers are becoming more environmentally conscious, leading to a demand for sustainable products and practices. Companies that prioritize sustainability can attract eco-minded customers and enhance their brand image.
- Health and Wellness: The focus on health and wellness is rising, with consumers seeking products that promote well-being. This trend is influencing various industries, from food and beverage to fitness and personal care.
- Remote Work: The COVID-19 pandemic accelerated the trend of remote work. Companies are adapting to flexible work arrangements, which can impact office space needs and employee engagement strategies.
- Personalization: Customers increasingly expect personalized experiences. Businesses are leveraging data analytics to tailor products, services, and marketing efforts to individual preferences.
- E-commerce Growth: Online shopping continues to expand, driven by convenience and changing consumer behaviors. Companies must invest in robust e-commerce platforms to meet this demand.
- Technological Advancements: Innovations such as artificial intelligence, machine learning, and blockchain are reshaping industries. Companies that adopt these technologies can improve efficiency and gain a competitive edge. Rapid Innovation is at the forefront of these advancements, providing clients with the tools they need to thrive in an ever-evolving marketplace. Understanding the competitive advantage in strategic management is essential for navigating these trends effectively.
12.6. Talent Development
Talent development is a crucial aspect of organizational growth and sustainability. It involves enhancing the skills, knowledge, and abilities of employees to meet current and future business needs. Effective talent development strategies can lead to increased employee engagement, improved performance, and higher retention rates.
- Importance of Talent Development
- Aligns employee skills with organizational goals.
- Fosters a culture of continuous learning.
- Enhances employee satisfaction and loyalty.
- Key Components of Talent Development
- Training Programs: Structured learning opportunities that focus on specific skills or knowledge areas, including AI and blockchain technologies, which are essential for modern business operations.
- Mentorship: Pairing less experienced employees with seasoned professionals to facilitate knowledge transfer, particularly in specialized fields like AI and blockchain.
- Career Pathing: Providing clear pathways for advancement within the organization, ensuring employees are equipped to take on roles that leverage emerging technologies.
- Strategies for Effective Talent Development
- Assess Skills Gaps: Regularly evaluate the skills of employees to identify areas for improvement, especially in relation to AI and blockchain competencies.
- Offer Diverse Learning Opportunities: Incorporate various learning methods, such as online courses, workshops, and on-the-job training, focusing on the latest advancements in technology.
- Encourage Feedback: Create a feedback-rich environment where employees can learn from their experiences, fostering a culture of innovation and adaptability.
- Developing a talent management strategy: Establish a comprehensive approach to managing and developing talent within the organization.
- Talent management and development strategy: Integrate talent management with development initiatives to ensure alignment with business objectives.
- Learning and talent development strategy: Focus on continuous learning as a key component of talent development.
- Measuring Success
- Track Employee Progress: Use performance metrics to assess the effectiveness of development programs, particularly in technology-related training.
- Employee Surveys: Gather feedback on training initiatives to understand their impact on employee performance and satisfaction.
- Retention Rates: Monitor turnover rates to gauge the success of talent development efforts, ensuring that employees feel valued and engaged.
- Talent development policy: Implement policies that support and promote talent development initiatives across the organization.
Investing in talent development not only enhances individual capabilities but also drives organizational success. Companies that prioritize employee growth, particularly in AI and blockchain, are better positioned to adapt to changing market conditions and technological advancements. This includes developing and retaining talent through effective talent acquisition strategies and ensuring a robust talent management development plan.
13. Emerging Trends and Future Outlook
The business landscape is constantly evolving, influenced by technological advancements, changing consumer behaviors, and global economic shifts. Understanding emerging trends is essential for organizations to remain competitive and innovative.
- Key Emerging Trends
- Digital Transformation: Organizations are increasingly adopting digital technologies to streamline operations and enhance customer experiences.
- Remote Work: The rise of remote work has changed how companies operate, leading to a more flexible workforce.
- Sustainability: Businesses are focusing on sustainable practices to meet consumer demand for environmentally friendly products and services.
- Future Outlook
- Increased Automation: Automation will continue to reshape industries, leading to greater efficiency and productivity.
- Enhanced Customer Experience: Companies will leverage data analytics to personalize customer interactions and improve satisfaction.
- Workforce Diversity: Organizations will prioritize diversity and inclusion, recognizing the value of varied perspectives in driving innovation.
Staying ahead of these trends requires organizations to be agile and adaptable, continuously reassessing their strategies to align with the evolving market landscape.
13.1. AI and Quantum Computing
Artificial Intelligence (AI) and quantum computing are two of the most transformative technologies shaping the future of various industries. Their integration into business processes is expected to revolutionize operations, decision-making, and problem-solving.
- AI in Business
- Automation of Routine Tasks: AI can handle repetitive tasks, freeing up human resources for more strategic activities.
- Data Analysis: AI algorithms can analyze vast amounts of data quickly, providing insights that inform business decisions.
- Enhanced Customer Interactions: AI-powered chatbots and virtual assistants improve customer service by providing instant responses.
- Quantum Computing Potential
- Speed and Efficiency: Quantum computers can process complex calculations at unprecedented speeds, solving problems that are currently infeasible for classical computers.
- Advanced Simulations: Industries such as pharmaceuticals and materials science can benefit from quantum simulations to develop new products and solutions.
- Cryptography: Quantum computing has the potential to revolutionize data security, making encryption methods more robust.
- Challenges and Considerations
- Ethical Implications: The rise of AI raises concerns about privacy, bias, and job displacement that organizations must address.
- Investment and Resources: Implementing AI and quantum computing requires significant investment in technology and talent.
- Skill Development: Organizations need to invest in training employees to work effectively with these advanced technologies.
The convergence of AI and quantum computing presents immense opportunities for innovation and efficiency. Organizations that embrace these technologies will be better equipped to navigate the complexities of the future business landscape.
13.2. Neuroplasticity Insights
Neuroplasticity refers to the brain's ability to reorganize itself by forming new neural connections throughout life. This remarkable capacity allows individuals to adapt to new experiences, learn new information, and recover from injuries. Understanding neuroplasticity has profound implications for education, therapy, and personal development.
- Neuroplasticity occurs at various levels, from cellular changes to large-scale brain reorganization.
- It is influenced by factors such as age, environment, and experiences.
- Engaging in challenging tasks can stimulate neuroplastic changes, enhancing cognitive functions.
- Techniques like mindfulness and meditation have been shown to promote neuroplasticity, improving focus and emotional regulation.
- Research indicates that learning a new skill, such as playing a musical instrument, can lead to structural changes in the brain.
The insights gained from neuroplasticity research, including neuroplasticity education and neuroplasticity and education, can inform teaching methods, therapeutic approaches, and personal growth strategies. By leveraging these insights, educators and therapists can create environments that foster learning and recovery, ultimately enhancing cognitive abilities and emotional well-being. For more on this topic, check out AI-Powered Multimodal Learning: Revolutionizing Cross-Disciplinary Innovation.
13.3. Cognitive Science Integration
Cognitive science is an interdisciplinary field that studies the mind and its processes, including how people think, learn, and remember. Integrating cognitive science into various domains, such as education and artificial intelligence, can lead to more effective strategies for learning and problem-solving.
- Cognitive science combines psychology, neuroscience, linguistics, philosophy, and computer science.
- Understanding cognitive processes can help educators design curricula that align with how the brain learns best.
- Techniques such as spaced repetition and retrieval practice are grounded in cognitive science and have been shown to improve retention and understanding.
- The use of technology in education can be enhanced by cognitive science principles, leading to more engaging and effective learning experiences.
- Cognitive load theory emphasizes the importance of managing the amount of information presented to learners to optimize understanding and retention.
By integrating cognitive science into educational practices and technology development, we can create more effective learning environments that cater to diverse learning styles and needs.
13.4. Personalized Learning Ecosystems
Personalized learning ecosystems are tailored educational environments that adapt to the individual needs, preferences, and interests of learners. This approach recognizes that each student has unique strengths and challenges, and it aims to provide customized pathways for learning.
- Personalized learning can be facilitated through technology, such as adaptive learning platforms that adjust content based on student performance.
- Key components of personalized learning include student agency, differentiated instruction, and ongoing assessment.
- By incorporating student interests and real-world applications, personalized learning can increase engagement and motivation.
- Collaboration among educators, parents, and students is essential for creating effective personalized learning plans.
- Data-driven insights can help educators identify areas where students may need additional support or enrichment.
The development of personalized learning ecosystems can lead to improved educational outcomes, as students are more likely to thrive in environments that cater to their individual learning journeys.
13.5. Rapid Innovation's Role
At Rapid Innovation, we harness the principles of neuroplasticity and cognitive science to develop AI-driven educational solutions that enhance learning experiences. By leveraging advanced algorithms, we create adaptive learning platforms that respond to individual student needs, promoting personalized learning ecosystems. Our expertise in AI allows us to analyze data-driven insights, enabling educators to tailor their approaches effectively, ultimately leading to greater ROI for educational institutions. Through our blockchain solutions, we ensure secure and transparent data management, fostering trust and collaboration among all stakeholders in the learning process. Additionally, we explore the intersection of neuroplasticity language learning and its impact on educational strategies, including insights from experts like Laura Boyd PhD on neuroplasticity.
13.5. Global Educational Transformation
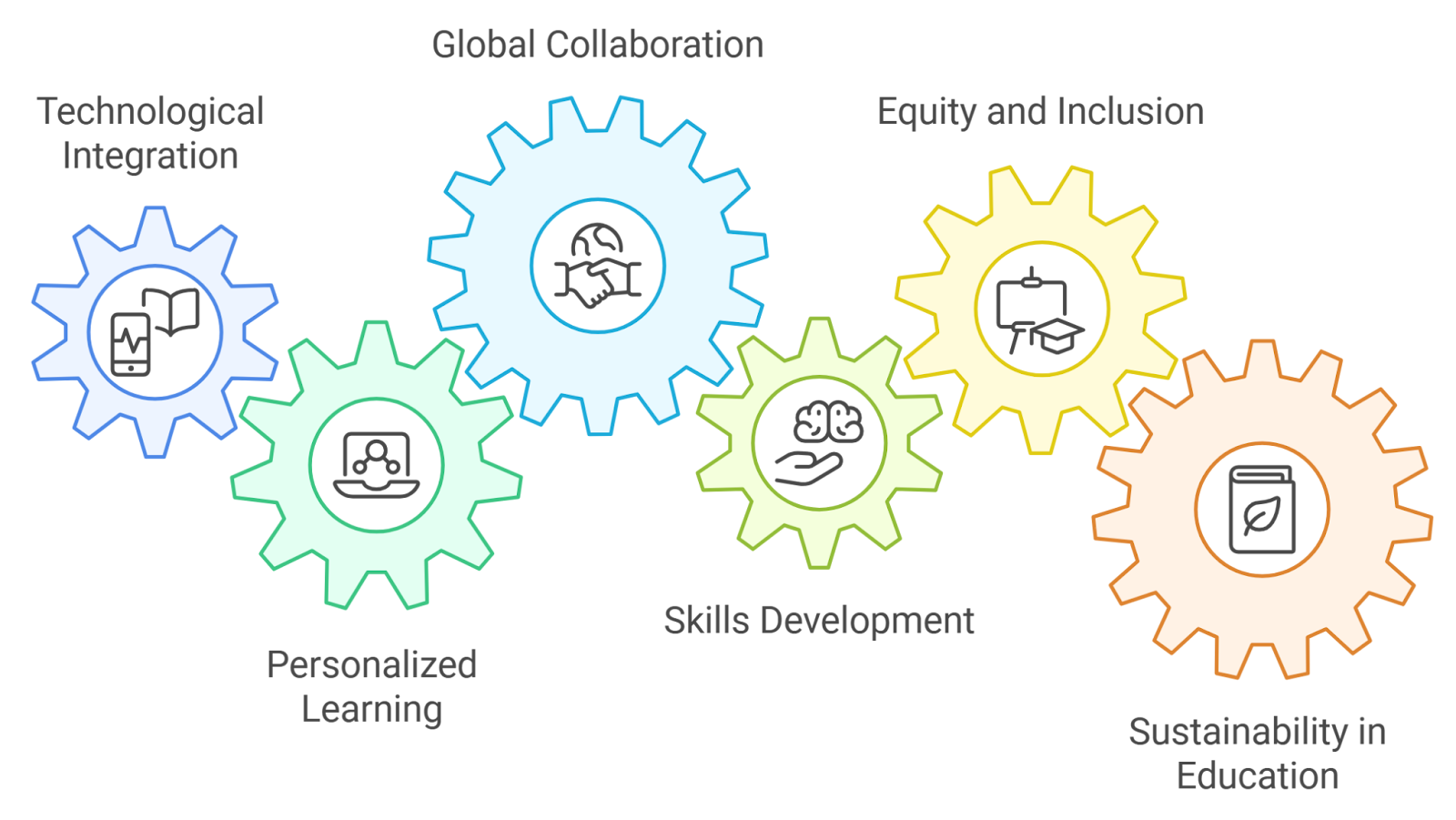
The landscape of education is undergoing a significant transformation on a global scale. This shift is driven by various factors, including technological advancements, changing societal needs, and the demand for more personalized learning experiences.
- Technological Integration: The rise of digital tools and platforms has revolutionized how education is delivered. Online learning, virtual classrooms, and educational apps are becoming commonplace, making education more accessible to diverse populations. Rapid Innovation leverages AI and blockchain technologies to create secure, scalable, and efficient educational platforms that enhance the learning experience. This is particularly evident in the context of digital transformation in higher education and digital transformation for education.
- Personalized Learning: There is a growing emphasis on tailoring education to meet individual student needs. Adaptive learning technologies analyze student performance and adjust content accordingly, fostering a more engaging and effective learning environment. Our AI-driven solutions enable institutions to implement personalized learning pathways, resulting in improved student engagement and retention rates. This aligns with the principles of digital education transformation and teaching for transformation.
- Global Collaboration: Educational institutions are increasingly collaborating across borders. This global approach allows for the sharing of resources, knowledge, and best practices, enhancing the quality of education worldwide. Rapid Innovation facilitates these collaborations through blockchain technology, ensuring secure and transparent sharing of educational resources and credentials. The concept of creative schools by Ken Robinson emphasizes the importance of such collaboration in fostering innovative educational practices.
- Focus on Skills Development: The shift from rote learning to skills-based education is gaining momentum. Emphasis is placed on critical thinking, creativity, and problem-solving skills, preparing students for the demands of the modern workforce. Our AI solutions help institutions design curricula that align with industry needs, ultimately enhancing students' employability. This is a key aspect of educational transformation and higher education transformation.
- Equity and Inclusion: Efforts are being made to ensure that education is equitable and inclusive. This includes addressing barriers faced by marginalized groups and providing resources to support diverse learning needs. Rapid Innovation's AI tools can identify gaps in access and tailor interventions to support underrepresented students, promoting a more inclusive educational environment. The focus on education and social transformation is crucial in this context.
- Sustainability in Education: There is a growing recognition of the importance of sustainability in education. Institutions are incorporating environmental education and promoting sustainable practices within their curricula. Our consulting services guide educational institutions in integrating sustainability into their operations and curricula, ensuring long-term viability and relevance. This aligns with the broader goals of transformation in schools and the need for educational transformation. For instance, the integration of the metaverse in education offers an immersive learning experience that can further enhance these efforts.
13.6. Predictive Ethical Frameworks
As technology continues to evolve, the need for predictive ethical frameworks becomes increasingly important. These frameworks help guide decision-making processes in various fields, including education, healthcare, and technology.
- Understanding Predictive Analytics: Predictive analytics involves using data to forecast future outcomes. In education, this can mean predicting student performance, identifying at-risk students, and tailoring interventions accordingly. Rapid Innovation employs advanced AI algorithms to provide actionable insights that empower educators to make data-driven decisions. This is particularly relevant in the context of digital transformation in schools.
- Ethical Considerations: The use of predictive analytics raises ethical questions, such as data privacy, consent, and potential biases in algorithms. Establishing ethical guidelines is crucial to ensure that data is used responsibly and equitably. Our team at Rapid Innovation works with clients to develop ethical frameworks that prioritize transparency and accountability in data usage. This is essential for ensuring that digital transformation executive education is conducted ethically.
- Framework Development: Developing predictive ethical frameworks involves collaboration among stakeholders, including educators, policymakers, and technologists. These frameworks should address:
- Transparency in data usage
- Accountability for outcomes
- Inclusivity in data representation
- Real-World Applications: Predictive ethical frameworks can be applied in various scenarios, such as:
- Enhancing student support services by identifying those who may need additional help
- Improving curriculum design based on predicted student engagement and success rates
- Ensuring fair admissions processes by analyzing applicant data without bias
- Continuous Evaluation: It is essential to continuously evaluate and update predictive ethical frameworks to adapt to new technologies and societal changes. This ensures that ethical considerations remain relevant and effective.
14. Implementation Roadmap
An effective implementation roadmap is crucial for successfully executing educational transformation initiatives and predictive ethical frameworks. This roadmap outlines the steps necessary to achieve desired outcomes while ensuring stakeholder engagement and resource allocation.
- Define Objectives: Clearly outline the goals of the educational transformation and the ethical frameworks. This includes identifying key performance indicators (KPIs) to measure success.
- Stakeholder Engagement: Involve all relevant stakeholders, including educators, students, parents, and community members. Their input is vital for understanding needs and gaining support for initiatives.
- Resource Allocation: Assess the resources required for implementation, including funding, technology, and personnel. Ensure that resources are allocated efficiently to support the initiatives.
- Pilot Programs: Start with pilot programs to test new approaches on a smaller scale. This allows for adjustments based on feedback and results before a full-scale rollout.
- Training and Support: Provide training for educators and staff to ensure they are equipped to implement new technologies and methodologies. Ongoing support is essential for addressing challenges that may arise.
- Monitoring and Evaluation: Establish a system for monitoring progress and evaluating the effectiveness of the initiatives. Regular assessments will help identify areas for improvement and ensure accountability.
- Feedback Loops: Create mechanisms for continuous feedback from stakeholders. This will help refine strategies and ensure that the initiatives remain aligned with the needs of the community.
- Scalability: Plan for scalability from the outset. Consider how successful initiatives can be expanded to reach a broader audience or adapted for different contexts.
- Sustainability: Ensure that the initiatives are sustainable in the long term. This includes securing ongoing funding, maintaining stakeholder engagement, and adapting to changing educational landscapes.
By following this implementation roadmap, educational institutions can effectively navigate the complexities of transformation and ethical considerations, ultimately leading to improved educational outcomes for all students. Rapid Innovation is committed to partnering with educational institutions to drive these initiatives forward, ensuring they achieve greater ROI through innovative technology solutions.
14.1. Preliminary Assessment

A preliminary environmental site assessment is a critical first step in any project or initiative. It involves evaluating the feasibility, potential impact, and resources required for a proposed program. This assessment helps stakeholders make informed decisions before committing significant time and resources.
- Identify objectives: Clearly define what the project aims to achieve. This could include specific goals such as improving efficiency, increasing revenue, or enhancing customer satisfaction.
- Analyze current state: Review existing processes, systems, and resources to understand the baseline. This includes gathering data on performance metrics and stakeholder feedback.
- Stakeholder engagement: Involve key stakeholders early in the assessment process. Their insights can provide valuable context and help identify potential challenges.
- Risk assessment: Evaluate potential risks associated with the project. This includes financial, operational, and reputational risks that could impact the initiative's success.
- Resource evaluation: Determine the resources required for the project, including personnel, technology, and budget. This helps in understanding the investment needed and potential return on investment (ROI).
- Benchmarking: Compare similar projects or initiatives to identify best practices and lessons learned. This can provide insights into what works and what doesn’t.
At Rapid Innovation, we leverage our expertise in AI and Blockchain to conduct thorough preliminary assessments that align with your business goals. For instance, when working with a retail client, we identified inefficiencies in their supply chain through data analysis, leading to a targeted AI solution that improved operational efficiency by 30%, ultimately enhancing their ROI.
14.2. Pilot Program Design
Designing a pilot program is essential for testing the feasibility of a new initiative on a smaller scale before full implementation. A well-structured pilot can provide valuable insights and help refine the approach.
- Define scope: Clearly outline the objectives, target audience, and key performance indicators (KPIs) for the pilot program. This ensures that everyone involved understands the goals and expectations.
- Select participants: Choose a representative sample of participants for the pilot. This could include a mix of users, stakeholders, and team members to gather diverse feedback.
- Develop a timeline: Create a detailed timeline for the pilot program, including key milestones and deadlines. This helps keep the project on track and ensures timely evaluations.
- Design the intervention: Outline the specific actions, tools, or processes that will be tested during the pilot. This could involve new technologies, workflows, or training programs.
- Monitor and evaluate: Establish a framework for monitoring the pilot's progress and evaluating its success. This includes collecting data on KPIs and gathering qualitative feedback from participants.
- Adjustments: Be prepared to make adjustments based on feedback and data collected during the pilot. Flexibility is key to refining the program for broader implementation.
At Rapid Innovation, we have successfully implemented pilot programs for clients in various sectors. For example, a financial services client tested a blockchain-based solution for secure transactions, which resulted in a 25% reduction in processing time and increased customer trust.
14.3. Scalability Considerations
Scalability is a crucial factor in determining whether a project can grow and adapt to meet increasing demands. Understanding scalability considerations helps ensure that a program can be expanded effectively without compromising quality or performance.
- Infrastructure: Assess whether the current infrastructure can support growth. This includes evaluating technology, processes, and human resources to determine if they can handle increased demand.
- Cost implications: Analyze the financial aspects of scaling the program. Consider whether the costs will increase proportionally with growth or if there are opportunities for cost savings through economies of scale.
- Process efficiency: Identify areas where processes can be streamlined to accommodate growth. This may involve automating tasks, improving workflows, or eliminating redundancies.
- Training and support: Ensure that adequate training and support are available for new users or participants as the program scales. This helps maintain quality and consistency in service delivery.
- Feedback loops: Establish mechanisms for ongoing feedback as the program expands. This allows for continuous improvement and adaptation to changing needs.
- Market demand: Evaluate the market demand for the program. Understanding the target audience and their needs is essential for determining whether scaling is viable and sustainable.
Rapid Innovation emphasizes scalability in all our projects. For instance, when developing an AI-driven customer service chatbot for a client, we ensured that the architecture could handle increased user interactions, resulting in a solution that not only met current demands but also positioned the client for future growth.
14.4. Change Management
Change management is a structured approach to transitioning individuals, teams, and organizations from a current state to a desired future state. It is essential for ensuring that changes are implemented smoothly and successfully, minimizing resistance and maximizing engagement.
- Key components of change management include:
- Communication: Clear and consistent messaging about the change helps to alleviate fears and uncertainties. A change management communication plan is vital to ensure that all stakeholders are informed throughout the process.
- Training: Providing adequate training ensures that all stakeholders are equipped with the necessary skills and knowledge to adapt to the change. This is particularly important in organizational change management strategies where training can mitigate resistance.
- Support: Offering support systems, such as coaching or mentoring, can help individuals navigate the transition more effectively. Coping with change in the workplace is easier when support is readily available.
- Feedback Mechanisms: Establishing channels for feedback allows for adjustments to be made in response to concerns or challenges that arise during the change process. Change management techniques can be employed to gather and analyze this feedback.
At Rapid Innovation, we leverage our expertise in AI and Blockchain to facilitate effective change management. For instance, by utilizing AI-driven analytics, we can predict potential resistance points and tailor communication strategies accordingly. Our Blockchain solutions can enhance transparency in the change process, fostering trust among stakeholders. Effective change management can lead to increased employee engagement and morale, higher rates of project success, and improved organizational performance. Organizations that implement robust change management strategies, such as change management strategies for successful ERP implementation, are more likely to achieve their desired outcomes and sustain improvements over time. A well-defined change management strategy example can serve as a guide for organizations looking to navigate their own transitions. Additionally, our services include AI project estimation to help organizations accurately assess the resources and time required for successful change initiatives.
14.5. Continuous Improvement Framework
A continuous improvement framework is a systematic approach to enhancing processes, products, or services over time. It focuses on incremental improvements rather than large-scale changes, fostering a culture of ongoing enhancement.
- Key elements of a continuous improvement framework include:
- Plan-Do-Check-Act (PDCA): This iterative process encourages organizations to plan changes, implement them, check the results, and act on what is learned.
- Data-Driven Decision Making: Utilizing data analytics to identify areas for improvement ensures that decisions are based on evidence rather than assumptions.
- Employee Involvement: Engaging employees at all levels in the improvement process fosters a sense of ownership and accountability.
- Standardization: Establishing standard operating procedures helps to maintain consistency and quality across processes.
At Rapid Innovation, we integrate AI technologies to enhance data-driven decision-making, allowing organizations to identify improvement opportunities with precision. Our continuous improvement framework not only enhances efficiency and productivity but also reduces waste and costs, ultimately leading to increased customer satisfaction through better quality products and services. Organizations that embrace continuous improvement are better positioned to adapt to changing market conditions and customer needs.
14.6. Stakeholder Engagement
Stakeholder engagement is the process of involving individuals or groups who have an interest in or are affected by a project or organization. Effective stakeholder engagement is crucial for building trust, fostering collaboration, and ensuring project success.
- Key strategies for effective stakeholder engagement include:
- Identifying Stakeholders: Recognizing all relevant stakeholders, including employees, customers, suppliers, and community members, is the first step in engagement.
- Understanding Needs and Expectations: Conducting surveys, interviews, or focus groups can help gather insights into stakeholder concerns and priorities.
- Regular Communication: Keeping stakeholders informed through updates, newsletters, or meetings helps to build transparency and trust.
- Involving Stakeholders in Decision-Making: Engaging stakeholders in the decision-making process can lead to better outcomes and increased buy-in.
At Rapid Innovation, we emphasize the importance of stakeholder engagement in our projects. By utilizing AI tools to analyze stakeholder feedback and preferences, we can tailor our strategies to meet their needs effectively. The benefits of strong stakeholder engagement include improved project outcomes through diverse perspectives, enhanced reputation and credibility of the organization, and increased loyalty and support from stakeholders. Organizations that prioritize stakeholder engagement are more likely to achieve their goals and foster long-term relationships with their stakeholders.