Table Of Contents
Category
Artificial Intelligence (AI)
Machine Learning (ML)
Natural Language Processing (NLP)
Decentralized Finance (DeFi)
Blockchain-as-a-Service (BaaS)
Automation
Blockchain
Decentralized Applications (DApps)
1. Introduction to User Behavior Analysis
User behavior analysis is a critical aspect of understanding how individuals interact with digital platforms. By examining user behavior, businesses can gain insights into preferences, habits, and trends that inform marketing strategies, product development, and customer service improvements. User behavior analysis involves collecting and interpreting data from various sources, including website interactions, social media engagement, and mobile app usage. The goal is to create a comprehensive profile of user behavior that can be used to enhance user experience and drive engagement. Understanding user behavior helps in identifying patterns that can predict future actions, allowing businesses to tailor their offerings accordingly.
The rise of artificial intelligence (AI) has significantly transformed user behavior analysis. AI agents can process vast amounts of data quickly and accurately, uncovering insights that would be difficult to detect manually.
- AI algorithms can analyze user interactions in real-time, providing immediate feedback and recommendations. Â
- Machine learning models can adapt to changing user behaviors, ensuring that businesses remain relevant and responsive to their audience. Â
- Predictive analytics powered by AI can forecast trends, enabling proactive decision-making. Â
At Rapid Innovation, we harness the power of AI to enhance user behavior analysis for our clients. By implementing tailored AI solutions, we help businesses not only understand their users better but also optimize their strategies for greater ROI. For instance, a retail client utilized our AI-driven insights to refine their marketing campaigns, resulting in a 30% increase in conversion rates.
In today's digital landscape, understanding user behavior is not just beneficial; it is essential for maintaining a competitive edge. By leveraging AI-driven insights, businesses can create personalized experiences that resonate with users, ultimately leading to increased loyalty and conversion rates. This includes utilizing user behavior analytics tools, user and entity behavior analytics, and user behavior analytics solutions to gain deeper insights. Additionally, incorporating machine learning user behavior analysis and web user behavior analytics can further enhance the understanding of user interactions. The integration of behavioral analytics in cyber security also plays a crucial role in safeguarding user data while analyzing user behavior. For more information on how AI agents can assist in user acquisition.
1.1. Fundamentals of Behavioral Analytics
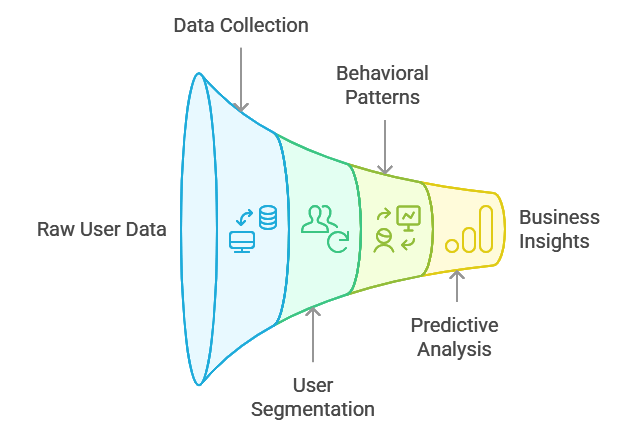
‍
Behavioral analytics is the process of collecting and analyzing data on user behavior to understand patterns and trends. This field combines data science, psychology, and business intelligence to derive insights that can drive decision-making. Key components of behavioral analytics include:
- Data Collection: Gathering data from various sources such as websites, mobile apps, and social media platforms.
- User Segmentation: Dividing users into groups based on similar behaviors or characteristics to tailor marketing strategies.
- Behavioral Patterns: Identifying trends in user actions, such as purchase history, browsing habits, and engagement levels.
- Predictive Analysis: Using historical data to forecast future behaviors, enabling businesses to anticipate customer needs.
Behavioral analytics is crucial for businesses aiming to enhance customer experience and optimize marketing efforts. By understanding how users interact with products or services, companies can make informed decisions that lead to increased engagement and conversion rates. At Rapid Innovation, we leverage behavioral analytics, including user behavior analytics and customer behavior analytics, to help our clients identify key user segments and optimize their marketing strategies, ultimately driving greater ROI. Additionally, we offer custom AI model development to further enhance these analytics capabilities.
1.2. Role of AI in Pattern Recognition
Artificial Intelligence (AI) plays a significant role in pattern recognition within behavioral analytics. AI algorithms can process vast amounts of data quickly and accurately, identifying complex patterns that may not be apparent to human analysts. Key functions of AI in pattern recognition include:
- Machine Learning: Algorithms learn from data, improving their accuracy over time as they are exposed to more information.
- Natural Language Processing (NLP): AI can analyze text data from customer feedback, social media, and reviews to identify sentiment and trends.
- Anomaly Detection: AI can spot unusual patterns or behaviors that may indicate fraud or other issues, allowing for timely intervention.
- Real-time Analysis: AI enables businesses to analyze user behavior in real-time, allowing for immediate adjustments to marketing strategies or user experiences.
The integration of AI in behavioral analytics not only enhances the accuracy of insights but also allows for more personalized customer interactions, ultimately driving better business outcomes. Rapid Innovation utilizes advanced AI techniques, including user and entity behavior analytics, to empower our clients with actionable insights, ensuring they stay ahead of market trends and maximize their investment.
1.3. Ethical Considerations and Privacy
As behavioral analytics and AI continue to evolve, ethical considerations and privacy concerns become increasingly important. The collection and analysis of user data raise questions about consent, data security, and the potential for misuse. Key ethical considerations include:
- Informed Consent: Users should be aware of what data is being collected and how it will be used. Transparency is crucial in building trust.
- Data Security: Protecting user data from breaches and unauthorized access is essential. Companies must implement robust security measures.
- Bias in Algorithms: AI systems can inadvertently perpetuate biases present in the training data, leading to unfair treatment of certain user groups.
- User Autonomy: Users should have the right to control their data, including the ability to opt-out of data collection practices.
Addressing these ethical considerations is vital for businesses to maintain customer trust and comply with regulations such as the General Data Protection Regulation (GDPR). By prioritizing ethical practices, companies can leverage behavioral analytics responsibly while respecting user privacy. At Rapid Innovation, we are committed to upholding the highest ethical standards in our AI solutions, ensuring that our clients can confidently navigate the complexities of data privacy while achieving their business objectives. This includes being mindful of user behavior tracking and ensuring that practices align with user expectations and legal requirements.
1.4. Key Applications and Use Cases
The applications and use cases of technology are vast and varied, impacting numerous sectors. Understanding these applications can help organizations leverage technology effectively.
- Healthcare: Telemedicine platforms enable remote consultations, improving access to healthcare. Wearable devices monitor patient vitals, providing real-time health data. AI-driven diagnostics assist in identifying diseases more accurately, leading to better patient outcomes and reduced costs. Additionally, medical photonics plays a crucial role in advanced imaging techniques.
- Finance: Blockchain technology enhances security and transparency in transactions, fostering trust among users. Robo-advisors provide automated investment advice based on algorithms, optimizing portfolio management. Fraud detection systems utilize machine learning to identify suspicious activities, significantly reducing financial losses.
- Retail: E-commerce platforms leverage personalized recommendations to enhance customer experience, driving sales and customer loyalty. Inventory management systems optimize stock levels and reduce waste, improving operational efficiency. Augmented reality (AR) applications allow customers to visualize products before purchase, enhancing engagement and satisfaction.
- Manufacturing: IoT devices monitor equipment performance, reducing downtime and maintenance costs. Predictive maintenance employs data analytics to foresee equipment failures, allowing for timely interventions. Automation and robotics streamline production processes, increasing efficiency and output. The integration of enterprise systems further enhances operational capabilities.
- Education: E-learning platforms offer flexible learning opportunities for students, catering to diverse learning styles. Learning management systems (LMS) track student progress and engagement, enabling personalized learning experiences. Virtual reality (VR) provides immersive learning experiences in various subjects, enhancing knowledge retention. Tools like Adobe Creative Cloud and multimedia technology are also utilized to create engaging educational content.
- Transportation: Autonomous vehicles are being developed to enhance road safety and efficiency, potentially reducing accidents. Ride-sharing apps optimize transportation options for users, improving convenience and reducing costs. Fleet management systems enhance logistics and reduce operational costs, leading to greater profitability.
These applications demonstrate the transformative power of technology across different industries, driving innovation and improving efficiency. At Rapid Innovation, we specialize in harnessing these technologies to help our clients achieve their business goals efficiently and effectively, ultimately leading to greater ROI. Our expertise in adaptive AI development allows us to tailor solutions that meet the unique needs of each sector. Additionally, understanding the importance of data quality is crucial for successful AI implementations.
2. Technical Foundation
The technical foundation of any technology is crucial for its functionality and effectiveness. Understanding the underlying components helps in grasping how technology operates and can be improved.
- Software Architecture: Defines the structure of software systems, including components and their interactions. Microservices architecture allows for modular development, enhancing scalability. Cloud-native applications leverage cloud computing for flexibility and resilience. Applications like Adobe Acrobat Creative Cloud and other computer software and media applications are examples of software that benefit from robust architecture.
- Hardware Infrastructure: Servers, storage, and networking equipment form the backbone of technology systems. Edge computing reduces latency by processing data closer to the source. High-performance computing (HPC) enables complex calculations and data analysis.
- Data Management: Databases store and manage data efficiently, supporting various applications. Data lakes allow for the storage of large volumes of unstructured data. Data governance ensures data quality, security, and compliance with regulations.
- Networking: Reliable network infrastructure is essential for data transmission and communication. 5G technology enhances connectivity, enabling faster data transfer rates. Virtual private networks (VPNs) provide secure remote access to organizational resources.
- Security Protocols: Cybersecurity measures protect systems from unauthorized access and attacks. Encryption ensures data confidentiality during transmission and storage. Regular security audits help identify vulnerabilities and mitigate risks.
Understanding the technical foundation is vital for organizations to implement and maintain effective technology solutions.
2.1. Data Collection Architecture
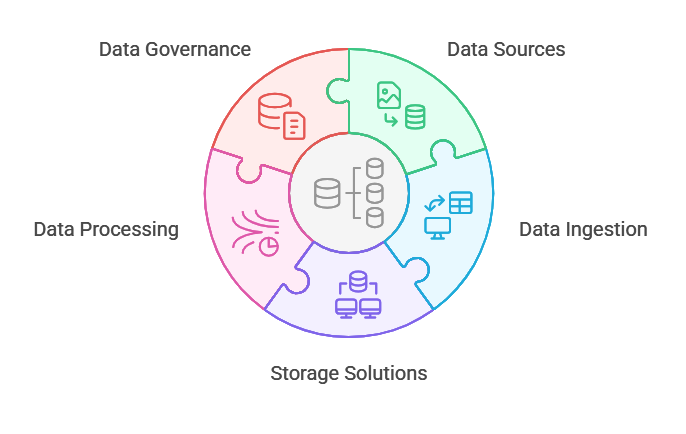
‍
Data collection architecture is a critical component of any data-driven organization. It outlines how data is gathered, stored, and processed, ensuring that valuable insights can be derived.
- Data Sources: Structured data comes from databases and spreadsheets, while unstructured data includes text, images, and videos. IoT devices generate real-time data from various sensors and applications. Social media platforms provide user-generated content that can be analyzed for trends.
- Data Ingestion: Batch processing collects data at scheduled intervals, suitable for large volumes of data. Real-time processing captures data as it is generated, enabling immediate analysis. Data pipelines automate the flow of data from sources to storage and processing systems.
- Storage Solutions: Data warehouses store structured data for analysis and reporting. Data lakes accommodate both structured and unstructured data, allowing for flexible querying. Cloud storage solutions provide scalable options for data storage and access.
- Data Processing: ETL (Extract, Transform, Load) processes prepare data for analysis by cleaning and transforming it. Data analytics tools enable organizations to derive insights from collected data. Machine learning algorithms can be applied to identify patterns and make predictions.
- Data Governance: Policies and procedures ensure data quality, security, and compliance with regulations. Metadata management helps in understanding the context and lineage of data. Data stewardship assigns responsibilities for data management within the organization.
A well-designed data collection architecture is essential for organizations to harness the power of data, enabling informed decision-making and strategic planning. At Rapid Innovation, we assist clients in developing robust data collection architectures that drive actionable insights and enhance overall business performance.
2.1.1. User Interaction Tracking
User interaction tracking is a crucial aspect of understanding how users engage with a website or application. This process involves collecting data on user behaviors, preferences, and interactions to enhance user experience and optimize performance. It helps identify popular features and content, enables personalized user experiences based on behavior, assists in identifying pain points in user journeys, provides insights for A/B testing and feature improvements, and facilitates targeted marketing strategies.
At Rapid Innovation, we leverage user interaction tracking to help our clients achieve greater ROI by tailoring their digital experiences to meet user needs effectively. For instance, by implementing click tracking and heatmaps, we can provide actionable insights that allow businesses to refine their user interfaces, leading to increased engagement and conversion rates. This is particularly important for businesses that track user interaction on their website.
Common methods for user interaction tracking include:
- Click tracking: Monitoring where users click on a page.
- Scroll tracking: Analyzing how far down a page users scroll.
- Session recording: Capturing user sessions to observe navigation patterns.
- Heatmaps: Visual representations of user interactions on a page.
Tools like Google Analytics, Hotjar, and Mixpanel are widely used for user interaction tracking. These tools provide valuable insights that can lead to improved user engagement and higher conversion rates. Additionally, our expertise extends to providing tailored solutions for industries such as real estate, where we offer AI real estate solutions to enhance user interaction and engagement. Furthermore, we explore the rise of AI-enhanced wearables to further improve user experiences.
2.1.2. Event Logging Systems
Event logging systems are essential for capturing and storing events that occur within an application or system. These systems help developers and analysts understand application performance, user behavior, and system health. They record user actions, system events, and errors, provide a historical record for troubleshooting and analysis, enable real-time monitoring of application performance, and support compliance and auditing requirements.
At Rapid Innovation, we implement robust event logging systems that empower our clients to gain deep insights into their applications. By utilizing tools like the ELK Stack or Splunk, we enable organizations to analyze user interactions and system performance, leading to better decision-making and improved application reliability.
Key components of event logging systems include:
- Event sources: The origin of the events, such as user actions or system processes.
- Event storage: Databases or log files where events are stored for analysis.
- Event processing: Tools that analyze and visualize logged events.
Popular event logging systems include ELK Stack (Elasticsearch, Logstash, Kibana), Splunk, and Fluentd. These systems allow organizations to gain insights into user interactions and system performance, leading to better decision-making and improved application reliability.
2.1.3. Real-time Data Streams
Real-time data streams refer to the continuous flow of data that is processed and analyzed as it is generated. This technology is vital for applications that require immediate insights and responses, such as financial trading platforms, social media monitoring, and IoT applications. Real-time data streams enable instant data processing and analysis, support timely decision-making and actions, enhance user experiences with real-time updates, and facilitate monitoring of system performance and user interactions.
At Rapid Innovation, we harness the power of real-time data streams to help our clients stay ahead of the competition. By implementing technologies like Apache Kafka and Amazon Kinesis, we enable businesses to respond swiftly to changing conditions and user needs, ultimately improving operational efficiency and enhancing customer satisfaction.
Key technologies and frameworks for real-time data streaming include:
- Apache Kafka: A distributed streaming platform that handles real-time data feeds.
- Apache Flink: A stream processing framework for real-time analytics.
- Amazon Kinesis: A cloud-based service for real-time data processing.
Real-time data streams are increasingly important in today’s fast-paced digital landscape, allowing businesses to respond quickly to changing conditions and user needs. By leveraging real-time data, organizations can improve operational efficiency and enhance customer satisfaction.
2.2. Data Processing Pipeline
A data processing pipeline is a series of data processing steps that transform raw data into a usable format for analysis or machine learning. This pipeline is crucial for ensuring data quality and consistency, which directly impacts the performance of machine learning models.
- Data Collection: The first step involves gathering data from various sources, such as databases, APIs, or web scraping. This data can be structured or unstructured.
- Data Cleaning: Raw data often contains errors, duplicates, or missing values. Data cleaning involves identifying and correcting these issues to ensure accuracy. Techniques include: Â
- Removing duplicates
- Filling in missing values
- Correcting inconsistencies
- Data Transformation: This step involves converting data into a suitable format for analysis. Common transformations include: Â
- Normalization or standardization of numerical values
- Encoding categorical variables
- Aggregating data for summarization
- Data Integration: This step combines data from different sources to create a unified dataset. This may involve merging tables or joining datasets based on common keys.
- Data Storage: Once processed, data needs to be stored efficiently. Options include: Â
- Relational databases (e.g., MySQL, PostgreSQL)
- NoSQL databases (e.g., MongoDB, Cassandra)
- Data lakes for large volumes of unstructured data
- Data Analysis: The final step involves analyzing the processed data to extract insights. This can be done using statistical methods or machine learning algorithms.
A well-structured data processing pipeline, such as an etl pipeline or an aws etl pipeline, is essential for effective data analysis and machine learning, as it ensures that the data is clean, consistent, and ready for modeling. At Rapid Innovation, we specialize in designing and implementing robust data processing pipelines tailored to your specific business needs, ensuring that you can derive actionable insights efficiently and effectively. Our expertise includes creating data ingestion pipelines and developing automated etl pipelines, as well as utilizing tools like databricks etl pipeline for enhanced performance. For more information on enhancing AI and machine learning models, check out our article on data annotation services.
2.3. Machine Learning Models
Machine learning models are algorithms that learn from data to make predictions or decisions without being explicitly programmed. These models can be categorized into various types based on their learning approach, complexity, and application.
- Types of Machine Learning Models: Â
- Supervised Learning: Models learn from labeled data, where the input-output pairs are known.
- Unsupervised Learning: Models identify patterns in data without labeled outputs.
- Reinforcement Learning: Models learn by interacting with an environment and receiving feedback in the form of rewards or penalties.
- Model Selection: Choosing the right model depends on the problem at hand, the nature of the data, and the desired outcome. Common models include: Â
- Linear regression for continuous outcomes
- Decision trees for classification tasks
- Neural networks for complex patterns
- Model Evaluation: After training, models must be evaluated using metrics such as accuracy, precision, recall, and F1 score to ensure they perform well on unseen data.
- Model Deployment: Once validated, models can be deployed into production environments for real-time predictions or batch processing.
Understanding the different types of machine learning models and their applications is crucial for selecting the right approach to solve specific problems. Rapid Innovation provides expert guidance in model selection and deployment, ensuring that your machine learning initiatives yield maximum ROI.
2.3.1. Supervised Learning Approaches
Supervised learning is a type of machine learning where models are trained on labeled datasets. This approach is widely used for tasks such as classification and regression.
- Key Characteristics: Â
- Requires labeled data: Each training example includes input features and the corresponding output label.
- Goal: The model learns to map inputs to outputs, enabling it to make predictions on new, unseen data.
- Common Algorithms: Â
- Linear Regression: Used for predicting continuous outcomes. It establishes a linear relationship between input features and the target variable.
- Logistic Regression: A classification algorithm that predicts binary outcomes by estimating probabilities using a logistic function.
- Support Vector Machines (SVM): Effective for high-dimensional spaces, SVMs find the optimal hyperplane that separates different classes.
- Decision Trees: These models split data into subsets based on feature values, making them easy to interpret.
- Random Forests: An ensemble method that combines multiple decision trees to improve accuracy and reduce overfitting.
- Applications: Â
- Spam Detection: Classifying emails as spam or not spam based on features like keywords and sender information.
- Image Recognition: Identifying objects in images by training on labeled datasets of images and their corresponding labels.
- Medical Diagnosis: Predicting diseases based on patient data, such as symptoms and medical history.
- Challenges: Â
- Overfitting: When a model learns noise in the training data, it performs poorly on new data. Techniques like cross-validation and regularization can help mitigate this.
- Data Imbalance: When one class is significantly more frequent than others, it can lead to biased models. Techniques like resampling or using different evaluation metrics can address this issue.
Supervised learning approaches are powerful tools for predictive modeling, enabling businesses and researchers to derive insights and make informed decisions based on data. At Rapid Innovation, we leverage these techniques to help our clients achieve their business objectives efficiently and effectively, including the development of data pipelines for machine learning and the design of data integration pipelines.
2.3.2. Unsupervised Pattern Discovery
Unsupervised pattern discovery is a crucial aspect of data analysis that involves identifying patterns and structures in data without prior labels or categories. This method is particularly useful in scenarios where labeled data is scarce or unavailable.
- Key Techniques: Â
- Clustering: Groups similar data points together based on their features. Common algorithms include K-means, hierarchical clustering, and DBSCAN.
- Dimensionality Reduction: Techniques like Principal Component Analysis (PCA) and t-Distributed Stochastic Neighbor Embedding (t-SNE) help reduce the number of variables under consideration, making it easier to visualize and analyze data.
- Anomaly Detection: Identifies outliers or unusual data points that do not conform to expected patterns, which can be critical in fraud detection and network security.
- Applications: Â
- Market Basket Analysis: Understanding customer purchasing behavior by discovering associations between products.
- Image Segmentation: Separating different objects within an image for better analysis in computer vision tasks.
- Gene Expression Analysis: Identifying patterns in biological data to understand disease mechanisms and treatment responses.
Unsupervised pattern discovery techniques can reveal hidden structures in data, leading to insights that can drive decision-making and strategy. At Rapid Innovation, we leverage these techniques to help clients uncover valuable insights from their data, ultimately enhancing their decision-making processes and driving greater ROI.
2.3.3. Reinforcement Learning Applications
Reinforcement learning (RL) is a type of machine learning where an agent learns to make decisions by taking actions in an environment to maximize cumulative rewards. This approach is inspired by behavioral psychology and is particularly effective in dynamic and complex environments.
- Key Concepts: Â
- Agent: The learner or decision-maker that interacts with the environment.
- Environment: The context in which the agent operates, including all possible states and actions.
- Reward Signal: Feedback from the environment that indicates the success of an action taken by the agent.
- Applications: Â
- Robotics: RL is used to train robots to perform tasks such as walking, grasping objects, and navigating environments autonomously.
- Game Playing: RL has achieved remarkable success in games like Go and chess, where agents learn strategies that surpass human capabilities.
- Autonomous Vehicles: RL helps in decision-making processes for navigation, obstacle avoidance, and route optimization.
Reinforcement learning is transforming industries by enabling systems to learn from their experiences and improve over time. Rapid Innovation applies RL techniques to optimize processes and enhance operational efficiency for our clients, leading to significant improvements in performance and ROI.
3. Behavioral Pattern Recognition
Behavioral pattern recognition involves analyzing and interpreting patterns in human behavior through various data sources. This field combines elements of psychology, data science, and machine learning to understand and predict actions based on historical data.
- Key Techniques: Â
- Feature Extraction: Identifying relevant features from raw data that can help in recognizing behavioral patterns.
- Classification Algorithms: Using supervised learning techniques like decision trees, support vector machines, and neural networks to categorize behaviors.
- Time-Series Analysis: Analyzing data points collected or recorded at specific time intervals to identify trends and patterns over time.
- Applications: Â
- Fraud Detection: Monitoring transactions to identify unusual patterns that may indicate fraudulent activity.
- User Experience Optimization: Analyzing user interactions on websites or applications to improve design and functionality.
- Health Monitoring: Tracking patient behaviors and symptoms to predict health outcomes and tailor interventions.
Behavioral pattern recognition is essential for businesses and organizations aiming to enhance customer experiences and improve operational efficiency. At Rapid Innovation, we utilize these techniques to help our clients better understand their customers and optimize their services, ultimately driving greater business success.
3.1. Click Pattern Analysis

‍
Click pattern analysis is a crucial aspect of understanding user behavior on websites. It involves examining how users interact with various elements on a webpage, such as buttons, links, and images. By analyzing these click patterns, businesses can gain insights into user preferences and optimize their website for better engagement, which is a key component of user behavior analytics.
- Identifying popular areas: Click pattern analysis helps identify which parts of a webpage attract the most attention. This can inform design decisions, ensuring that important information is placed where users are most likely to see it.
- Understanding user intent: By analyzing where users click, businesses can infer their intentions. For example, if many users click on a specific product image, it may indicate a strong interest in that product, which is essential for user behavior analysis.
- Improving conversion rates: By optimizing the layout based on click patterns, businesses can enhance user experience and increase conversion rates. For instance, placing call-to-action buttons in high-click areas can lead to more sign-ups or purchases.
- Tools for analysis: Various tools, such as heatmaps and click-tracking software, can provide visual representations of click patterns, making it easier to interpret data and make informed decisions. These tools are integral to user behavior analytics tools.
At Rapid Innovation, we leverage advanced AI algorithms to conduct click pattern analysis, enabling our clients to make data-driven design choices that significantly enhance user engagement and drive higher ROI through user and entity behavior analytics. Additionally, our expertise extends to metaverse avatar development services that can further enhance user interaction in digital environments. For more insights on how AI agents can improve content discovery workflows, check out our article on AI agents and content discovery workflow.
3.2. Navigation Flow Mapping
Navigation flow mapping is the process of visualizing how users navigate through a website. This analysis helps identify the paths users take, the pages they visit, and where they drop off. Understanding navigation flow is essential for enhancing user experience and ensuring that visitors can easily find the information they need.
- Visual representation: Navigation flow maps provide a clear visual representation of user journeys, highlighting the most common paths taken by visitors. This can help identify bottlenecks or confusing areas in the navigation structure.
- Identifying drop-off points: By analyzing where users leave the site, businesses can pinpoint pages that may need improvement. High drop-off rates on specific pages can indicate issues with content, design, or usability.
- Enhancing user experience: By optimizing navigation based on flow mapping, businesses can create a more intuitive experience. This may involve simplifying menus, improving internal linking, or reorganizing content to align with user expectations.
- Tools for mapping: Tools like Google Analytics and user flow visualization software can assist in creating navigation flow maps, providing valuable insights into user behavior.
Rapid Innovation employs sophisticated AI-driven navigation flow mapping techniques to help clients streamline their website structures, ultimately leading to improved user satisfaction and increased conversion rates, which are critical aspects of user behavior analytics.
3.3. Time-based Behavior Analysis
Time-based behavior analysis focuses on how long users spend on a website and individual pages. This analysis can reveal important trends about user engagement and content effectiveness. Understanding time-based behavior is vital for optimizing content and improving overall user experience.
- Measuring engagement: The amount of time users spend on a page can indicate how engaging the content is. Longer time spent may suggest that users find the content valuable, while shorter times may indicate a lack of interest.
- Identifying content effectiveness: By analyzing time spent on different pages, businesses can determine which content resonates with their audience. This can inform future content strategies and help prioritize topics that generate interest.
- Bounce rates: Time-based analysis can also help identify bounce rates, which indicate how quickly users leave a page. High bounce rates may suggest that the content is not meeting user expectations or that the page is not user-friendly.
- Tools for analysis: Google Analytics and other web analytics tools can provide insights into time-based behavior, allowing businesses to track user engagement and make data-driven decisions.
At Rapid Innovation, we utilize AI technologies to conduct comprehensive time-based behavior analysis, enabling our clients to refine their content strategies and enhance user engagement, ultimately leading to greater ROI through user behavior analytics cyber security and user behavior analysis.
3.4. Device Usage Patterns
Understanding device usage patterns is crucial for businesses aiming to optimize their digital strategies. These patterns reveal how users interact with various devices, which can significantly influence marketing and product development.
- Mobile Dominance: A significant portion of internet traffic now comes from mobile devices. According to recent statistics, mobile devices account for over 50% of global web traffic. This trend emphasizes the need for mobile-friendly websites and applications, which Rapid Innovation can help develop to ensure optimal user engagement and conversion rates.
- Multi-device Usage: Users often switch between devices throughout the day. For instance, they may start browsing on a smartphone, continue on a tablet, and finalize purchases on a desktop. This behavior highlights the importance of a seamless user experience across platforms. Rapid Innovation can assist in creating integrated solutions that provide a consistent experience, enhancing customer satisfaction and loyalty.
- Time of Day Variations: Device usage can vary significantly based on the time of day. For example, mobile usage tends to peak during commuting hours, while desktop usage is more common during work hours. Understanding these patterns can help businesses time their marketing efforts effectively. Rapid Innovation can leverage this data to optimize marketing strategies, ensuring that clients reach their audience at the right moment.
- Demographic Differences: Different demographics exhibit distinct device preferences. Younger users may prefer mobile devices, while older generations might lean towards desktops. Tailoring content and marketing strategies to these preferences can enhance engagement. Rapid Innovation can provide insights and analytics to help clients customize their approaches based on demographic data.
- App vs. Browser Usage: Many users prefer apps for specific tasks, such as shopping or social media, while using browsers for research. Recognizing this can guide businesses in developing both app and web strategies to cater to user preferences. Rapid Innovation can assist in creating both mobile applications and responsive websites that meet user needs effectively. For more insights on how AI agents can enhance marketing applications.
3.5. Cross-platform Behavior Correlation
Cross-platform behavior correlation refers to the analysis of user interactions across multiple platforms and devices. This analysis helps businesses understand how users engage with their brand in different environments.
- User Journey Mapping: By tracking user behavior across platforms, businesses can create detailed user journey maps. These maps illustrate how users transition from one device to another, providing insights into their decision-making processes. Rapid Innovation can implement advanced analytics tools to help clients visualize and optimize these journeys.
- Consistent Messaging: Maintaining consistent messaging across platforms is vital. Users expect a cohesive experience, whether they are interacting with a brand on social media, a website, or a mobile app. Inconsistent messaging can lead to confusion and disengagement. Rapid Innovation can help ensure that messaging is aligned across all channels, enhancing brand integrity.
- Data Integration: Integrating data from various platforms allows businesses to gain a holistic view of user behavior. This integration can reveal patterns that may not be apparent when analyzing data from a single platform. Rapid Innovation specializes in data integration solutions that provide clients with comprehensive insights for better decision-making.
- Targeted Marketing: Understanding cross-platform behavior enables businesses to create targeted marketing campaigns. For instance, if a user frequently browses products on a mobile app but makes purchases on a desktop, targeted ads can be tailored to encourage mobile app purchases. Rapid Innovation can develop sophisticated marketing strategies that leverage user data for maximum impact.
- Enhanced Customer Experience: By analyzing cross-platform behavior, businesses can identify pain points in the user experience. Addressing these issues can lead to improved customer satisfaction and loyalty. Rapid Innovation can provide actionable insights and solutions to enhance the overall customer experience.
4. Advanced Analytics Components
Advanced analytics components are essential tools and techniques that enable businesses to derive actionable insights from data. These components enhance decision-making and drive strategic initiatives.
- Predictive Analytics: This component uses historical data to forecast future trends. By analyzing past user behavior, businesses can predict future actions, such as purchasing patterns or churn rates. Predictive analytics helps in proactive decision-making. Rapid Innovation can implement predictive models that empower clients to anticipate market changes and customer needs.
- Machine Learning Algorithms: Machine learning algorithms analyze vast amounts of data to identify patterns and trends. These algorithms can automate processes, improve personalization, and enhance customer targeting. They are crucial for businesses looking to leverage big data effectively. Rapid Innovation can develop tailored machine learning solutions that drive efficiency and innovation.
- Data Visualization Tools: Effective data visualization tools transform complex data sets into easily understandable visual formats. These tools help stakeholders quickly grasp insights and make informed decisions. Rapid Innovation can integrate advanced visualization tools to present data in a way that supports strategic initiatives.
- Real-time Analytics: Real-time analytics allows businesses to monitor user behavior as it happens. This capability is essential for responding to trends and making immediate adjustments to marketing strategies or product offerings. Rapid Innovation can implement real-time analytics solutions that enable clients to stay agile in a fast-paced market.
- Sentiment Analysis: This component analyzes user-generated content, such as reviews and social media posts, to gauge public sentiment about a brand or product. Understanding sentiment can guide marketing strategies and product development. Rapid Innovation can leverage sentiment analysis to help clients refine their offerings based on customer feedback.
- A/B Testing: A/B testing involves comparing two versions of a webpage or app to determine which performs better. This method helps businesses optimize user experience and increase conversion rates by making data-driven decisions. Rapid Innovation can facilitate A/B testing processes to ensure that clients achieve the best possible outcomes.
- Customer Segmentation: Advanced analytics enables businesses to segment their customer base into distinct groups based on behavior, preferences, and demographics. This segmentation allows for more targeted marketing efforts and personalized experiences. Rapid Innovation can assist clients in developing effective segmentation strategies that enhance engagement and ROI.
- Attribution Modeling: Attribution modeling helps businesses understand which marketing channels contribute most to conversions. By analyzing the customer journey, businesses can allocate resources more effectively and optimize their marketing strategies. Rapid Innovation can provide clients with robust attribution models that enhance their marketing effectiveness and return on investment.
4.1. Sentiment Analysis
Sentiment analysis is a natural language processing (NLP) technique used to determine the emotional tone behind a series of words. This process is crucial for businesses and organizations to understand public opinion, customer feedback, and social media interactions. It involves classifying text as positive, negative, or neutral.
Sentiment analysis can be applied to various data sources, including social media posts, customer reviews, and survey responses. Techniques used in sentiment analysis include machine learning algorithms and lexicon-based approaches. Businesses leverage sentiment analysis to gauge customer satisfaction, monitor brand reputation, and inform marketing strategies.
At Rapid Innovation, we help clients implement sentiment analysis solutions, including google cloud platform sentiment analysis and microsoft dynamics sentiment analysis, tailored to their specific needs, enabling them to derive actionable insights from customer interactions. By utilizing advanced NLP techniques, we empower organizations to enhance their customer engagement strategies and improve overall satisfaction. Additionally, we offer sentiment monitoring tools and sentiment analytics solutions to further support our clients.
Our expertise also extends to specific applications, such as twitter sentiment analysis kaggle solution and sentiment analysis on movie reviews kaggle solution, providing comprehensive insights into various domains. For more information on related techniques, you can read about anomaly detection.
4.2. Anomaly Detection
Anomaly detection refers to the identification of unusual patterns or outliers in data that do not conform to expected behavior. This technique is essential in various fields, including finance, healthcare, and cybersecurity. Anomaly detection helps in fraud detection, network security, and quality control in manufacturing.
Common methods for anomaly detection include statistical tests, machine learning algorithms (e.g., clustering, classification), and time-series analysis. The benefits of anomaly detection include early identification of potential issues, reduction of false positives in alerts, and enhanced decision-making processes. Industries utilizing anomaly detection include banking and finance for transaction monitoring, healthcare for patient monitoring systems, and IT for system performance monitoring.
At Rapid Innovation, we assist clients in deploying robust anomaly detection systems that significantly reduce fraud losses and enhance operational efficiency. Research indicates that implementing anomaly detection can reduce fraud losses by up to 50%.
4.3. Predictive Modeling
Predictive modeling is a statistical technique that uses historical data to forecast future outcomes. This approach is widely used across various industries to make informed decisions based on data-driven insights. Key components of predictive modeling include data collection and preprocessing, feature selection and engineering, and model selection and validation.
Common applications of predictive modeling include customer behavior prediction, sales forecasting, and risk assessment in finance. Techniques used in predictive modeling include regression analysis, decision trees, and neural networks. The benefits of predictive modeling are improved accuracy in forecasting, enhanced operational efficiency, and better resource allocation.
At Rapid Innovation, we provide comprehensive predictive modeling solutions that help organizations optimize their strategies and improve profitability. Tools for predictive modeling include R and Python programming languages, SAS and SPSS software, and Microsoft Azure Machine Learning. According to a study, organizations that utilize predictive analytics can increase their profitability by 8-10%.
4.4. Session Analysis
Session analysis is a critical component of understanding user behavior on digital platforms. It involves examining the interactions users have during a single visit to a website or application. This analysis helps identify patterns, preferences, and potential areas for improvement.
- Definition of a Session: A session typically begins when a user enters a site and ends when they leave or become inactive for a specified period.
- Key Metrics: Important metrics in session analysis include: Â
- Session duration: The total time a user spends on the site.
- Pages per session: The average number of pages viewed during a session.
- Bounce rate: The percentage of sessions where users leave after viewing only one page.
- Tools for Session Analysis: Various analytics tools can facilitate session analysis, such as Google Analytics, Mixpanel, and Hotjar. These tools provide insights into user behavior and engagement, including user behavior analytics tools and user behavior analytics solutions.
- User Journey Mapping: By analyzing sessions, businesses can create user journey maps that visualize the steps users take, helping to identify drop-off points and optimize the user experience. This process is enhanced by user and entity behavior analytics.
- Behavioral Insights: Session analysis can reveal: Â
- Popular content: Understanding which pages attract the most visits.
- User engagement: Identifying which features or content keep users engaged, which can be further analyzed through user behavior analysis.
- Conversion paths: Analyzing the steps leading to conversions can help refine marketing strategies, supported by user behavior analytics cyber security insights.
4.5. Feature Engineering for Behavior Analysis
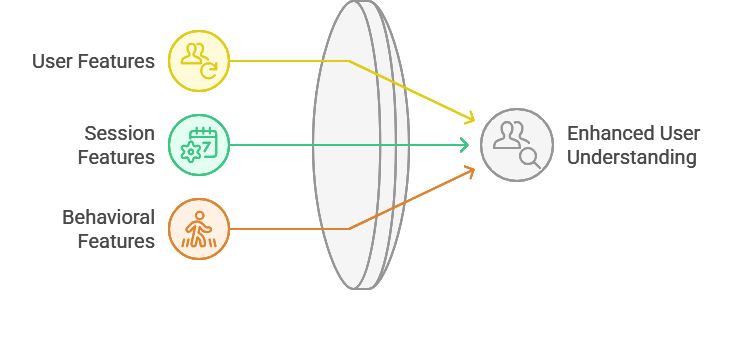
‍
Feature engineering is the process of selecting, modifying, or creating new features from raw data to improve the performance of machine learning models. In the context of behavior analysis, feature engineering plays a vital role in understanding user interactions and predicting future behavior.
- Importance of Feature Engineering: Well-engineered features can significantly enhance the accuracy of predictive models, leading to better insights into user behavior, particularly through user behavior analytics.
- Types of Features: Â
- User Features: Demographic information, account age, and previous interactions.
- Session Features: Session duration, number of pages visited, and time spent on each page.
- Behavioral Features: Click patterns, scroll depth, and engagement with specific content, which can be analyzed using behavior analytics tools.
- Techniques for Feature Engineering: Â
- Aggregation: Summarizing data over time, such as average session duration or total clicks.
- Transformation: Normalizing or scaling features to ensure they contribute equally to model performance.
- Encoding: Converting categorical variables into numerical formats, such as one-hot encoding for user demographics.
- Tools and Libraries: Popular tools for feature engineering include Python libraries like Pandas and Scikit-learn, which provide functionalities for data manipulation and model building, essential for user behavior analytics tools.
- Impact on Behavior Analysis: Effective feature engineering can lead to: Â
- Improved user segmentation: More accurate identification of user groups based on behavior, facilitated by user behavior analytics.
- Enhanced predictive modeling: Better forecasting of user actions and preferences.
- Tailored user experiences: Personalized content and recommendations based on user behavior, supported by user behavior analytics solutions.
5. User Segmentation and Profiling
User segmentation and profiling are essential strategies for understanding diverse user groups and tailoring marketing efforts accordingly. By categorizing users based on shared characteristics, businesses can create more targeted and effective campaigns.
- Definition of User Segmentation: User segmentation involves dividing a user base into distinct groups based on specific criteria, such as demographics, behavior, or preferences.
- Benefits of User Segmentation: Â
- Improved targeting: Tailoring marketing messages to specific user groups increases engagement and conversion rates.
- Enhanced user experience: Personalized experiences lead to higher satisfaction and loyalty.
- Efficient resource allocation: Focused marketing efforts can reduce costs and improve ROI.
- Common Segmentation Criteria: Â
- Demographic Segmentation: Age, gender, income level, and education.
- Behavioral Segmentation: Purchase history, website interactions, and engagement levels, which can be analyzed through user behavior analytics.
- Geographic Segmentation: Location-based targeting to cater to regional preferences.
- Tools for User Segmentation: Various analytics platforms, such as Google Analytics, HubSpot, and Segment, offer features for user segmentation and profiling, including user and entity behavior analytics.
- Creating User Profiles: User profiling involves developing detailed descriptions of user segments, including: Â
- Interests and preferences: Understanding what content or products resonate with each segment.
- Pain points: Identifying challenges faced by different user groups.
- Buying behavior: Analyzing how different segments make purchasing decisions.
- Dynamic Segmentation: Implementing dynamic segmentation allows businesses to adjust user groups in real-time based on changing behaviors or preferences, ensuring that marketing efforts remain relevant.
- Impact on Marketing Strategies: Effective user segmentation and profiling can lead to: Â
- Higher engagement rates: Personalized content resonates more with users.
- Increased conversion rates: Targeted campaigns are more likely to convert.
- Better customer retention: Understanding user needs fosters loyalty and repeat business.
At Rapid Innovation, we leverage session analysis and feature engineering to help our clients gain deeper insights into user behavior, ultimately driving greater ROI through targeted marketing strategies and enhanced user experiences. By employing advanced analytics and machine learning techniques, including user behavior analytics and user behavior analysis, we empower businesses to make data-driven decisions that align with their goals.
5.1. Behavioral Clustering
Behavioral clustering is a technique used to group users based on their actions and interactions with a product or service. This method helps businesses understand user behavior patterns, enabling them to tailor their marketing strategies effectively.
- Identifies distinct user segments based on behavior. Â
- Utilizes data analytics to analyze user interactions, including user behavior analytics and user and entity behavior analytics. Â
- Helps in personalizing user experiences and improving engagement through user behavior analysis. Â
- Can lead to increased conversion rates by targeting specific user needs. Â
- Commonly used in e-commerce, social media, and content platforms. Â
By employing behavioral clustering, companies can create targeted campaigns that resonate with specific user groups. For instance, an e-commerce platform might cluster users based on their purchasing habits, allowing for personalized recommendations and promotions. This approach not only enhances user satisfaction but also drives sales, ultimately contributing to a greater return on investment (ROI) for businesses. Additionally, integrating advanced technologies such as ChatGPT can further enhance these efforts by providing intelligent insights and automation. Furthermore, businesses can leverage product recommendations in e-commerce to optimize their marketing strategies and improve user engagement.
5.2. User Persona Development
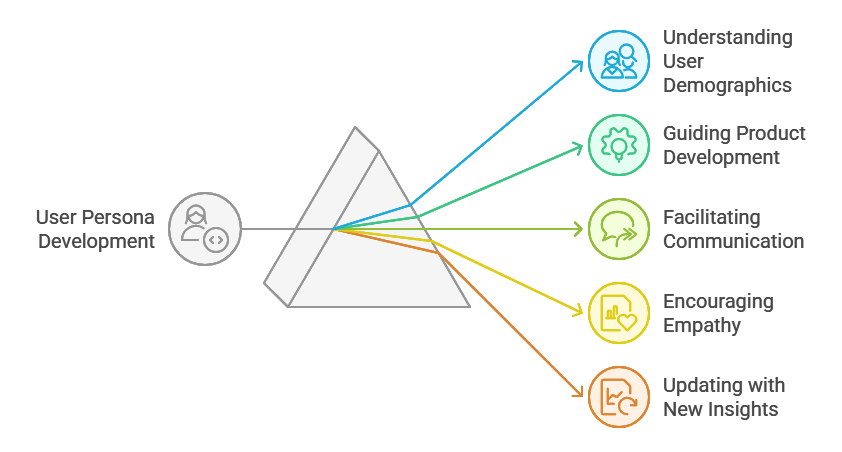
‍
User persona development involves creating detailed profiles that represent different segments of a target audience. These personas are based on research and data, capturing the characteristics, needs, and motivations of users.
- Helps in understanding user demographics, preferences, and pain points through user behavior analytics tools. Â
- Guides product development and marketing strategies. Â
- Facilitates better communication and engagement with users. Â
- Encourages empathy by putting a human face to data. Â
- Can be updated as new insights are gained, including insights from user behaviour analytics. Â
Creating user personas allows businesses to visualize their audience, making it easier to design products and services that meet user needs. For example, a software company might develop personas for different types of users, such as tech-savvy millennials and older, less tech-oriented individuals. This differentiation helps in crafting tailored marketing messages and user experiences, leading to improved customer engagement and loyalty.
5.3. Dynamic Profile Updates
Dynamic profile updates refer to the continuous process of refreshing user profiles based on real-time data and interactions. This approach ensures that user information remains current and relevant, enhancing personalization efforts.
- Utilizes machine learning and AI to analyze user behavior, including user behavior analytics cyber security. Â
- Allows for real-time adjustments to user profiles, supported by uba user behavior analytics. Â
- Improves targeting accuracy for marketing campaigns. Â
- Enhances user experience by providing timely and relevant content. Â
- Supports adaptive strategies in response to changing user needs. Â
By implementing dynamic profile updates, businesses can respond quickly to shifts in user behavior, ensuring that their marketing efforts remain effective. For instance, a streaming service might update user profiles based on viewing habits, allowing for personalized recommendations that reflect current interests. This adaptability not only improves user satisfaction but also fosters long-term loyalty, ultimately driving higher ROI for the organization.
At Rapid Innovation, we leverage these advanced techniques, including user behavior analytics tools and behavior analytics tools, to help our clients achieve their business goals efficiently and effectively, ensuring that they stay ahead in a competitive landscape.
5.4. Cohort Analysis
Cohort analysis is a powerful analytical tool used to understand the behavior and performance of specific groups of users over time. By segmenting users into cohorts based on shared characteristics or experiences, businesses can gain insights into trends, retention rates, and overall engagement.
- Definition: A cohort is a group of users who share a common characteristic, such as the month they signed up or the marketing campaign that brought them in. This concept is central to cohort analysis definition and is often illustrated through a cohort analysis example.
- Purpose: The primary goal of cohort analysis is to track how these groups behave over time, allowing businesses to identify patterns and make data-driven decisions. This is particularly useful in customer cohort analysis and cohort retention analysis.
- Benefits: Â
- Improved retention strategies: By understanding which cohorts are more likely to churn, businesses can tailor their retention efforts effectively, leading to increased customer loyalty and reduced acquisition costs. This is a key aspect of cohort retention.
- Enhanced marketing effectiveness: Analyzing cohorts can reveal which marketing channels are most effective for different user segments, enabling businesses to allocate resources more efficiently and maximize ROI. This can be achieved through cohort analysis in tools like tableau cohort analysis and cohort analysis excel.
- Product development insights: Understanding how different cohorts interact with a product can inform future development and feature prioritization, ensuring that offerings align with user needs. The meaning of cohort analysis extends to how it can guide product enhancements.
Cohort analysis can be applied across various industries, from e-commerce to SaaS, providing valuable insights that drive growth and customer satisfaction. The importance of cohort analysis cannot be overstated, as it helps businesses define cohort analysis and understand their customer base better. Additionally, implementing an AI customer service agent can enhance the effectiveness of cohort analysis by providing real-time insights and support. Furthermore, exploring how AI is transforming the field of neuromarketing can provide additional context for understanding user behavior and preferences.
5.5. Demographic Integration
Demographic integration involves combining demographic data with other types of data to create a comprehensive view of users. This approach allows businesses to tailor their strategies based on the characteristics of their target audience.
- Importance: Understanding demographics is crucial for effective marketing and product development. It helps businesses identify who their customers are and what they need.
- Key demographic factors: Â
- Age: Different age groups may have varying preferences and behaviors, influencing product design and marketing strategies.
- Gender: Marketing messages can be tailored based on gender-specific interests, enhancing engagement and conversion rates.
- Location: Geographic data can influence product offerings and marketing strategies, allowing for localized approaches that resonate with specific audiences.
- Benefits of demographic integration: Â
- Targeted marketing: Businesses can create personalized campaigns that resonate with specific demographic groups, leading to higher engagement and conversion rates.
- Enhanced user experience: By understanding user demographics, companies can design products and services that meet the needs of their audience, fostering customer satisfaction and loyalty.
- Improved customer segmentation: Demographic data allows for more precise segmentation, leading to better engagement and conversion rates.
Integrating demographic data with other analytics can lead to more informed decision-making and ultimately drive business success.
6. Real-time Analysis Capabilities
Real-time analysis capabilities refer to the ability to process and analyze data as it is generated, allowing businesses to make immediate decisions based on current information. This capability is increasingly important in today’s fast-paced digital landscape.
- Definition: Real-time analysis involves the continuous monitoring of data streams and the immediate processing of that data to provide insights.
- Importance: In a world where consumer preferences and market conditions can change rapidly, real-time analysis enables businesses to stay agile and responsive.
- Key features of real-time analysis: Â
- Instant data processing: Data is analyzed as it comes in, providing up-to-the-minute insights that can inform strategic decisions.
- Dashboards and visualizations: Real-time dashboards allow stakeholders to visualize data trends and metrics instantly, facilitating quick assessments of performance.
- Alerts and notifications: Businesses can set up alerts for specific metrics, enabling quick responses to changes in performance and ensuring that opportunities are not missed.
- Benefits of real-time analysis: Â
- Enhanced decision-making: Immediate access to data allows for quicker, more informed decisions, leading to improved operational efficiency.
- Improved customer engagement: Businesses can respond to customer interactions in real-time, enhancing the overall experience and fostering loyalty.
- Competitive advantage: Companies that leverage real-time data can adapt faster than their competitors, leading to better market positioning and increased profitability.
Incorporating real-time analysis capabilities into business operations can significantly enhance responsiveness and drive better outcomes. Rapid Innovation specializes in implementing these advanced analytical tools, helping clients achieve greater ROI through data-driven strategies.
6.1. Stream Processing
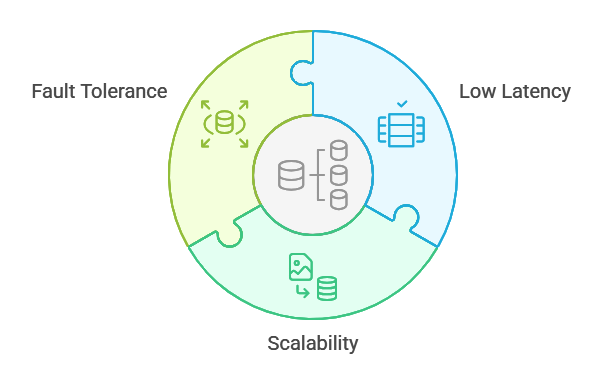
‍
Stream processing is a method of handling continuous data streams in real-time. It allows organizations to analyze and act on data as it flows in, rather than waiting for batch processing. This approach is crucial for applications that require immediate insights and actions, such as realtime data processing and real time data integration.
- Key characteristics of stream processing include:
- Low Latency: Data is processed as it arrives, ensuring minimal delay.
- Scalability: Systems can handle increasing volumes of data without significant performance degradation.
- Fault Tolerance: Stream processing frameworks often include mechanisms to recover from failures, ensuring data integrity.
Popular stream processing frameworks include Apache Kafka, Apache Flink, and Apache Spark Streaming. These tools enable businesses to build applications that can monitor, analyze, and respond to data in real-time, including kafka real time streaming and real time stream processing.
- Use cases for stream processing:
- Fraud Detection: Financial institutions can monitor transactions in real-time to identify and prevent fraudulent activities, significantly reducing potential losses.
- IoT Data Processing: Devices generate continuous data streams that can be analyzed for insights, such as monitoring environmental conditions or equipment performance, leading to proactive maintenance and operational efficiency.
- Social Media Analytics: Companies can track social media interactions in real-time to gauge public sentiment and respond accordingly, enhancing brand reputation and customer engagement.
6.2. Event-driven Architecture
Event-driven architecture (EDA) is a software design paradigm that focuses on the production, detection, consumption, and reaction to events. In this architecture, components communicate through events, allowing for a more decoupled and flexible system.
- Key components of event-driven architecture include: Â
- Event Producers: These are systems or applications that generate events based on specific actions or changes in state.
- Event Channels: These are the pathways through which events are transmitted, often using message brokers like RabbitMQ or Apache Kafka.
- Event Consumers: These are applications or services that listen for and respond to events.
- Benefits of adopting an event-driven architecture: Â
- Scalability: Systems can scale independently, allowing for better resource management and cost efficiency.
- Responsiveness: Applications can react to events in real-time, improving user experience and operational agility.
- Flexibility: New components can be added or modified without disrupting the entire system, facilitating innovation and faster time-to-market.
- Common use cases for event-driven architecture include: Â
- Microservices Communication: Microservices can communicate through events, allowing for loose coupling and easier maintenance, which enhances overall system resilience.
- Real-time Analytics: Businesses can analyze data as it comes in, enabling faster decision-making and improved strategic planning, including real time analytics processing.
- User Interaction Tracking: Websites and applications can track user interactions in real-time to personalize experiences, leading to increased customer loyalty and satisfaction.
6.3. Real-time Decision Making
Real-time decision making refers to the ability to analyze data and make decisions instantly as events occur. This capability is essential for organizations that operate in fast-paced environments where timely responses can significantly impact outcomes.
- Key aspects of real-time decision making include: Â
- Data Integration: Combining data from various sources to provide a comprehensive view for decision-making, ensuring that insights are based on the most relevant information, such as real time data integration.
- Advanced Analytics: Utilizing machine learning and AI algorithms to analyze data and predict outcomes, enabling organizations to make informed decisions quickly.
- Automated Responses: Implementing systems that can automatically act on insights derived from data analysis, reducing the need for manual intervention and speeding up response times.
- Benefits of real-time decision making: Â
- Improved Agility: Organizations can quickly adapt to changing conditions, such as market trends or customer preferences, enhancing their competitive edge.
- Enhanced Customer Experience: Businesses can provide personalized services and responses, leading to higher customer satisfaction and retention rates.
- Competitive Advantage: Companies that leverage real-time insights can outperform competitors who rely on traditional decision-making processes, driving greater ROI.
- Examples of real-time decision making applications: Â
- Dynamic Pricing: E-commerce platforms can adjust prices based on demand, competitor pricing, and inventory levels, maximizing revenue opportunities.
- Supply Chain Management: Companies can optimize inventory levels and logistics in real-time based on current demand and supply conditions, reducing costs and improving service levels.
- Healthcare Monitoring: Real-time data from patient monitoring systems can alert healthcare providers to critical changes in a patient's condition, enabling timely interventions that can save lives.
At Rapid Innovation, we specialize in implementing these advanced technologies to help our clients achieve their business goals efficiently and effectively. By leveraging stream processing, event-driven architecture, and real-time decision-making capabilities, including real time etl and real time data ingestion, we empower organizations to enhance their operational efficiency, improve customer experiences, and ultimately drive greater ROI.
6.4. Adaptive Response Systems
Adaptive Response Systems are crucial in today's fast-paced technological landscape. These systems are designed to adjust and respond to changing conditions in real-time, ensuring optimal performance and efficiency.
- Key features of Adaptive Response Systems include:
- Real-time data analysis: These systems continuously monitor data inputs to make informed decisions.
- Dynamic adjustments: They can modify their operations based on environmental changes or user interactions.
- User-centric design: Focus on enhancing user experience by adapting to individual preferences and behaviors.
At Rapid Innovation, we leverage Adaptive Response Systems to help our clients across various sectors, including healthcare, finance, and manufacturing. For instance, in healthcare, these systems can adjust treatment plans based on patient responses, leading to better outcomes and higher patient satisfaction. In finance, they can adapt trading strategies based on market fluctuations, optimizing investment returns and minimizing risks.
The implementation of Adaptive Response Systems can lead to significant benefits, such as increased efficiency and productivity, enhanced customer satisfaction, and improved decision-making processes, ultimately driving greater ROI for our clients. This is similar to how the adaptive immune system functions, adjusting to specific threats and ensuring optimal responses. For more information on how we can assist you, check out our AI consulting services.
6.5. Performance Optimization
Performance Optimization refers to the process of enhancing the efficiency and effectiveness of systems, applications, or processes. This is essential for ensuring that resources are utilized to their fullest potential, leading to better outcomes.
- Key aspects of Performance Optimization include:
- Resource management: Efficient allocation of resources such as CPU, memory, and bandwidth.
- Load balancing: Distributing workloads evenly across servers to prevent bottlenecks.
- Code optimization: Streamlining code to reduce execution time and improve responsiveness.
At Rapid Innovation, we understand that Performance Optimization is critical in various fields, including software development, web hosting, and cloud computing. For example, in software development, optimizing code can lead to faster application load times, enhancing user experience and retention. In web hosting, performance optimization can improve website speed, which is vital for retaining visitors and increasing conversion rates.
Benefits of Performance Optimization include reduced operational costs, enhanced user experience, and increased system reliability and uptime, all contributing to a higher return on investment for our clients. This mirrors the principles of adaptive immunity, where systems are optimized to respond effectively to various challenges.
7. Integration and Implementation
Integration and Implementation are vital steps in deploying new systems or technologies within an organization. This process ensures that new solutions work seamlessly with existing systems and processes.
- Key components of Integration and Implementation include:
- System compatibility: Ensuring that new technologies can communicate and function with current systems.
- Data migration: Transferring data from old systems to new ones without loss or corruption.
- User training: Providing adequate training to users to ensure they can effectively utilize the new systems.
Successful integration and implementation can lead to numerous advantages, such as streamlined operations with improved workflows and reduced redundancies, enhanced data accuracy with better data management and reporting capabilities, and increased employee productivity, allowing users to focus on their tasks without being hindered by outdated systems.
Challenges in Integration and Implementation may include resistance to change from employees, technical difficulties during data migration, and ensuring ongoing support and maintenance for new systems. This is akin to the challenges faced in establishing an effective adaptive immune system, where integration of new immune responses is crucial.
By addressing these challenges and focusing on effective strategies, organizations can successfully integrate and implement new technologies, leading to improved performance and competitiveness in their respective markets. At Rapid Innovation, we are committed to guiding our clients through this process, ensuring that they achieve their business goals efficiently and effectively, much like the adaptive immune system's role in maintaining health and resilience.
7.1. System Architecture
System architecture is the conceptual model that defines the structure, behavior, and various views of a system. It serves as a blueprint for both the system and the project developing it. A well-defined system architecture is crucial for ensuring scalability, maintainability, and performance, which are essential for achieving greater ROI in your business operations.
- Components of System Architecture: Â
- Client-Server Model: This model separates the client (user interface) from the server (data processing), allowing for better resource management and scalability. Rapid Innovation leverages this model to ensure that client applications can scale independently of backend services, optimizing resource allocation and enhancing user experience.
- Microservices Architecture: This approach breaks down applications into smaller, independent services that can be developed, deployed, and scaled independently. By adopting microservices, Rapid Innovation enables clients to innovate rapidly and deploy updates without affecting the entire system, leading to increased agility and reduced time-to-market. This is particularly relevant in microservices system design.
- Layered Architecture: This divides the system into layers, such as presentation, business logic, and data access, promoting separation of concerns. This structure allows for easier maintenance and updates, ensuring that clients can adapt to changing business needs efficiently.
- Key Considerations: Â
- Scalability: The architecture should support growth in users and data without significant rework. Rapid Innovation designs systems that can seamlessly scale, ensuring that clients can handle increased demand without compromising performance. This is a critical aspect of system architecture design.
- Performance: It should ensure that the system responds quickly to user requests. Our expertise in optimizing system performance translates into faster response times and improved user satisfaction for our clients.
- Security: Incorporating security measures at every layer is essential to protect data and user privacy. Rapid Innovation prioritizes security in system architecture, helping clients mitigate risks and comply with regulatory requirements. This aligns with secure by design architecture principles.
- Tools and Technologies: Â
- Cloud Services: Platforms like AWS, Azure, and Google Cloud provide scalable infrastructure. Rapid Innovation utilizes these services to offer clients flexible and cost-effective solutions that grow with their business, particularly in cloud computing architecture design.
- Containerization: Tools like Docker and Kubernetes help in managing microservices efficiently. By implementing containerization, we enable clients to achieve greater operational efficiency and resource utilization.
- Frameworks: Using frameworks like Spring Boot or Django can streamline development. Rapid Innovation employs these frameworks to accelerate development cycles, allowing clients to bring their products to market faster.
7.2. API Design and Documentation
API design is a critical aspect of software development, as it defines how different software components interact. A well-designed API can enhance usability and facilitate integration with other systems, ultimately driving greater ROI for clients.
- Principles of Good API Design: Â
- Simplicity: APIs should be easy to understand and use. Clear naming conventions and straightforward endpoints are essential. Rapid Innovation focuses on creating intuitive APIs that reduce the learning curve for developers, enabling faster integration and adoption.
- Consistency: Consistent naming and structure across endpoints help developers predict behavior and reduce learning curves. Our commitment to consistency ensures that clients can maintain and extend their APIs with ease.
- Versioning: Implementing versioning allows for backward compatibility and smooth transitions when updates are made. This practice minimizes disruptions for clients and their users, ensuring a seamless experience.
- Documentation Best Practices: Â
- Comprehensive Guides: Include detailed descriptions of endpoints, request/response formats, and error codes. Rapid Innovation emphasizes thorough documentation to empower developers and reduce support requests.
- Interactive Documentation: Tools like Swagger or Postman can provide interactive documentation, allowing developers to test APIs directly. This hands-on approach enhances understanding and accelerates development.
- Examples and Use Cases: Providing real-world examples helps users understand how to implement the API effectively. Our focus on practical examples ensures that clients can leverage APIs to their fullest potential.
- Testing and Feedback: Â
- Automated Testing: Implement automated tests to ensure API functionality remains intact during updates. Rapid Innovation integrates testing into the development process, ensuring high-quality APIs that meet client expectations.
- User Feedback: Regularly gather feedback from developers using the API to identify pain points and areas for improvement. This iterative approach allows us to refine APIs continuously, enhancing client satisfaction.
7.3. Database Selection and Setup
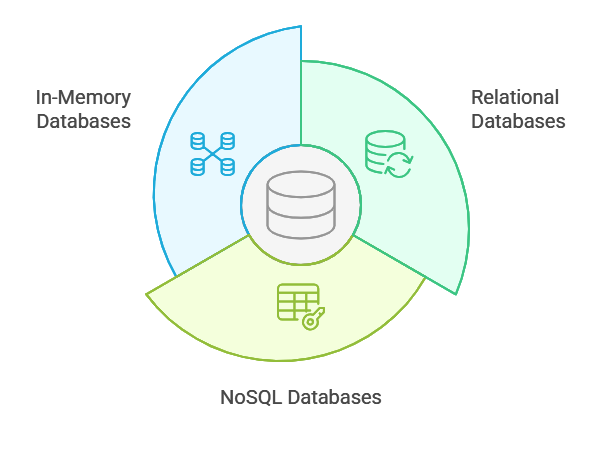
‍
Choosing the right database is crucial for the performance and scalability of an application. The database should align with the application’s requirements, including data structure, access patterns, and scalability needs, which are vital for maximizing ROI.
- Types of Databases: Â
- Relational Databases: Such as MySQL and PostgreSQL, are ideal for structured data and complex queries. Rapid Innovation helps clients select the right relational database to ensure data integrity and efficient querying.
- NoSQL Databases: Options like MongoDB and Cassandra are better suited for unstructured data and horizontal scaling. We guide clients in choosing NoSQL solutions that support their unique data needs and growth trajectories.
- In-Memory Databases: Redis and Memcached provide fast data access for applications requiring high performance. Our expertise in in-memory databases enables clients to achieve low-latency data access, enhancing application responsiveness.
- Factors to Consider: Â
- Data Structure: Understand the nature of the data (structured vs. unstructured) to choose the appropriate database type. Rapid Innovation conducts thorough assessments to ensure optimal database selection.
- Scalability: Consider how the database will handle increased loads and data growth. We design database architectures that can scale seamlessly with client needs, which is a key aspect of data warehouse architecture design.
- Consistency vs. Availability: Determine the need for strong consistency versus high availability based on application requirements. Our team helps clients strike the right balance to meet their operational goals.
- Setup and Configuration: Â
- Schema Design: For relational databases, design a normalized schema to reduce redundancy and improve data integrity. Rapid Innovation employs best practices in schema design to enhance database performance.
- Indexing: Implement indexing strategies to enhance query performance. Our expertise in indexing ensures that clients can retrieve data quickly and efficiently.
- Backup and Recovery: Establish a robust backup and recovery plan to protect data against loss. We prioritize data protection strategies to safeguard client information.
- Monitoring and Maintenance: Â
- Performance Monitoring: Use tools to monitor database performance and identify bottlenecks. Rapid Innovation implements monitoring solutions that provide clients with insights into database health and performance.
- Regular Maintenance: Schedule regular maintenance tasks, such as updates and optimizations, to ensure the database runs efficiently. Our proactive maintenance approach minimizes downtime and maximizes system reliability.
7.4. Scaling Considerations
Scaling is a critical aspect of any system, especially when dealing with large datasets or increasing user demands. Proper scaling ensures that applications can handle growth without compromising performance. Here are key considerations for scaling:
- Vertical Scaling: This involves adding more resources (CPU, RAM) to existing machines. It’s often simpler but has limits based on hardware capabilities.
- Horizontal Scaling: This approach adds more machines to the pool, distributing the load. It’s more complex but allows for greater flexibility and redundancy.
- Load Balancing: Implementing load balancers helps distribute incoming traffic evenly across servers, preventing any single server from becoming a bottleneck.
- Database Sharding: This technique involves splitting a database into smaller, more manageable pieces, allowing for faster queries and improved performance.
- Caching Strategies: Utilizing caching mechanisms (like Redis or Memcached) can significantly reduce database load and improve response times.
- Microservices Architecture: Breaking down applications into smaller, independent services can enhance scalability, as each service can be scaled independently based on demand.
- Monitoring and Analytics: Regularly monitoring system performance and user behavior helps identify scaling needs before they become critical issues.
- Cloud Solutions: Leveraging cloud services (like AWS, Azure, or Google Cloud) can provide on-demand resources that scale automatically based on traffic.
At Rapid Innovation, we understand that effective scaling is essential for maximizing your return on investment (ROI). By implementing these scaling strategies, we help our clients ensure that their systems can grow seamlessly, allowing them to focus on their core business objectives without the worry of performance bottlenecks. Our scalable growth strategy is designed to adapt to changing demands, ensuring that our clients can implement a strategy to scale effectively. For more information on how we can assist you, check out our AI as a Service.
7.5. Security Implementation
Security is paramount in any system, especially when handling sensitive data. A robust security implementation protects against unauthorized access, data breaches, and other cyber threats. Key aspects include:
- Data Encryption: Encrypting data both at rest and in transit ensures that sensitive information is protected from unauthorized access.
- Access Control: Implementing strict access controls ensures that only authorized users can access specific data or functionalities. Role-based access control (RBAC) is a common method.
- Regular Security Audits: Conducting regular security assessments helps identify vulnerabilities and ensures compliance with security standards.
- Firewalls and Intrusion Detection Systems: Utilizing firewalls and intrusion detection systems (IDS) can help monitor and protect against malicious activities.
- Secure APIs: Ensuring that APIs are secure through authentication and authorization mechanisms is crucial, as they are often a target for attacks.
- User Education: Training users on security best practices, such as recognizing phishing attempts, can significantly reduce the risk of breaches.
- Incident Response Plan: Having a well-defined incident response plan ensures that organizations can quickly respond to and mitigate security incidents.
- Compliance with Regulations: Adhering to regulations like GDPR, HIPAA, or PCI-DSS is essential for protecting user data and maintaining trust.
At Rapid Innovation, we prioritize security in our development processes, ensuring that our clients can operate confidently in a digital landscape. Our comprehensive security strategies not only protect sensitive data but also enhance overall system integrity, contributing to a greater ROI.
8. Visualization and Reporting
Effective visualization and reporting are vital for interpreting data and making informed decisions. They transform complex data into understandable formats. Key components include:
- Data Visualization Tools: Utilizing tools like Tableau, Power BI, or Google Data Studio can help create interactive and insightful visualizations.
- Dashboards: Custom dashboards provide real-time insights into key performance indicators (KPIs), allowing stakeholders to monitor progress at a glance.
- Charts and Graphs: Using various types of charts (bar, line, pie) helps convey information clearly and effectively.
- Storytelling with Data: Combining data with narrative elements can enhance understanding and engagement, making reports more impactful.
- Automated Reporting: Automating report generation saves time and ensures that stakeholders receive timely updates without manual intervention.
- User-Friendly Interfaces: Ensuring that visualization tools are intuitive and easy to navigate encourages broader usage across teams.
- Data Integration: Integrating data from multiple sources provides a comprehensive view, enhancing the quality of insights derived from visualizations.
- Feedback Mechanisms: Implementing feedback loops allows users to suggest improvements, ensuring that reporting tools evolve to meet their needs.
At Rapid Innovation, we leverage advanced visualization techniques to empower our clients with actionable insights. By transforming data into clear, compelling narratives, we help organizations make informed decisions that drive business success and maximize ROI.
8.1. Interactive Dashboards
Interactive dashboards are powerful tools that allow users to visualize and analyze data in real-time. They provide a dynamic interface where users can interact with data through various visual elements such as charts, graphs, and maps. Key features of interactive dashboards include:
- Real-time data updates: Users can see the most current data without needing to refresh or reload.
- Customizable views: Users can tailor the dashboard to display the metrics that matter most to them.
- Drill-down capabilities: Users can click on specific data points to explore more detailed information.
- User-friendly interfaces: Intuitive designs make it easy for non-technical users to navigate and understand the data.
At Rapid Innovation, we leverage interactive dashboards, such as tableau interactive dashboards and power bi interactive dashboards, to empower our clients to track performance metrics, monitor KPIs, and make data-driven decisions efficiently. For instance, a healthcare client utilized our interactive dashboard solution to visualize patient data in real-time, leading to improved patient outcomes and operational efficiency. By enhancing collaboration, teams can share insights and findings easily, ultimately driving greater ROI. We also provide interactive dashboard examples and create interactive dashboards tailored to specific needs, including interactive dashboards in Google Sheets and interactive dashboards in Power BI. If you're looking to enhance your dashboard capabilities, consider hiring our Action Transformer Developers to help you achieve your goals.
8.2. Pattern Visualization Tools
Pattern visualization tools are essential for identifying trends and patterns within large datasets. These tools help users make sense of complex data by presenting it in a visually appealing and understandable format. Benefits of using pattern visualization tools include:
- Enhanced data interpretation: Visual representations make it easier to spot trends, correlations, and anomalies.
- Improved decision-making: By understanding patterns, organizations can make informed decisions based on data insights.
- Support for predictive analytics: These tools can help forecast future trends based on historical data patterns.
- Diverse visualization options: Users can choose from various formats, such as heat maps, scatter plots, and line graphs, to best represent their data.
Rapid Innovation employs pattern visualization tools to assist clients in fields like data science and marketing analytics. For example, a retail client used our pattern visualization capabilities to analyze customer purchasing behavior, enabling them to tailor marketing strategies effectively. This resulted in a significant increase in sales and customer engagement, showcasing how understanding data trends can lead to substantial business growth.
8.3. Automated Report Generation
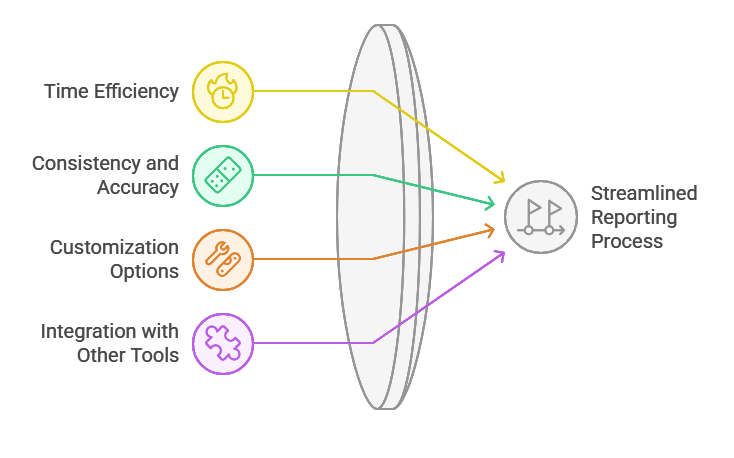
‍
Automated report generation streamlines the process of creating reports by using software to compile data and generate insights without manual intervention. This technology saves time and reduces the likelihood of human error. Advantages of automated report generation include:
- Time efficiency: Reports can be generated quickly, allowing teams to focus on analysis rather than data collection.
- Consistency and accuracy: Automated processes ensure that reports are generated using the same criteria, leading to uniformity in data presentation.
- Customization options: Users can set parameters for the reports, ensuring that the generated content meets specific needs.
- Integration with other tools: Many automated reporting tools can connect with data sources and visualization platforms, creating a seamless workflow.
At Rapid Innovation, we recognize the importance of timely insights for businesses that require regular reporting, such as financial institutions and marketing agencies. By implementing automated report generation, we have helped clients reduce reporting time by up to 70%, allowing them to focus on strategic initiatives rather than manual data compilation. This efficiency not only enhances productivity but also contributes to a stronger competitive edge in their respective markets.
8.4. Custom Alert Systems
Custom alert systems are essential tools for organizations looking to enhance their operational efficiency and responsiveness. These custom alert systems allow users to set personalized notifications based on specific criteria, ensuring that critical information is communicated promptly.
- Tailored Notifications: Users can customize alerts based on their unique needs, such as project deadlines, budget thresholds, or compliance requirements. This personalization ensures that stakeholders receive the most relevant information, leading to better resource allocation and project management.
- Real-time Updates: Custom alert systems provide real-time notifications, enabling stakeholders to react swiftly to changes or issues as they arise. This immediacy can significantly reduce downtime and enhance productivity, ultimately contributing to a higher return on investment (ROI).
- Integration Capabilities: These custom alert systems can often integrate with existing software and platforms, allowing for seamless communication across various departments. By streamlining workflows, organizations can reduce operational silos and improve overall efficiency.
- User-friendly Interfaces: Many custom alert systems feature intuitive dashboards that make it easy for users to manage their alerts and preferences. This ease of use encourages adoption across the organization, maximizing the benefits of the system.
- Enhanced Decision-Making: By receiving timely alerts, stakeholders can make informed decisions quickly, improving overall organizational agility. This capability allows businesses to pivot and adapt to market changes, driving better financial outcomes.
8.5. Stakeholder-specific Views
Stakeholder-specific views are crucial for ensuring that different users within an organization have access to the information that is most relevant to them. This tailored approach enhances communication and collaboration among various teams.
- Role-based Access: Different stakeholders can be granted access to specific data sets based on their roles, ensuring they only see what is pertinent to their responsibilities. This targeted access not only improves security but also enhances productivity by reducing information overload.
- Custom Dashboards: Users can create personalized dashboards that highlight key performance indicators (KPIs) and metrics relevant to their functions. This customization allows teams to focus on what matters most, driving better performance and accountability.
- Improved Collaboration: By providing stakeholders with the information they need, organizations can foster better collaboration and reduce misunderstandings. Enhanced communication leads to more effective teamwork and project outcomes.
- Enhanced Reporting: Stakeholder-specific views allow for more targeted reporting, making it easier to analyze data and derive insights that are relevant to specific teams. This capability supports data-driven decision-making, which is essential for achieving strategic business goals.
- Increased Engagement: When stakeholders have access to tailored information, they are more likely to engage with the data and contribute to discussions and decision-making processes. This engagement fosters a culture of accountability and innovation within the organization.
9. Privacy and Compliance
Privacy and compliance are critical considerations for organizations in today's data-driven landscape. Ensuring that sensitive information is protected and that regulatory requirements are met is essential for maintaining trust and avoiding legal repercussions.
- Data Protection Regulations: Organizations must comply with various data protection laws, such as GDPR, HIPAA, and CCPA, which dictate how personal information should be handled. Adhering to these regulations not only protects the organization but also builds trust with clients and stakeholders.
- Risk Management: Implementing robust privacy measures helps mitigate risks associated with data breaches and unauthorized access, protecting both the organization and its clients. This proactive approach can save organizations from costly legal issues and reputational damage.
- Transparency: Organizations should maintain transparency about their data collection and usage practices, informing stakeholders about how their information is being used. This transparency is vital for fostering trust and ensuring compliance with regulations.
- Regular Audits: Conducting regular audits of data handling practices ensures compliance with regulations and helps identify areas for improvement. These audits can also uncover opportunities for operational efficiencies, further enhancing ROI.
- Employee Training: Providing training for employees on privacy policies and compliance requirements is essential for fostering a culture of data protection within the organization. Well-informed employees are key to maintaining compliance and safeguarding sensitive information.
At Rapid Innovation, we leverage our expertise in AI to implement these custom alert systems and practices, helping organizations achieve their business goals efficiently and effectively while maximizing their return on investment. Additionally, our Natural Language Processing solutions can further enhance the capabilities of these systems, providing deeper insights and more effective communication. For more insights on AI in knowledge management, check out our article on AI Knowledge Management in 2024.
9.1. Data Protection Frameworks
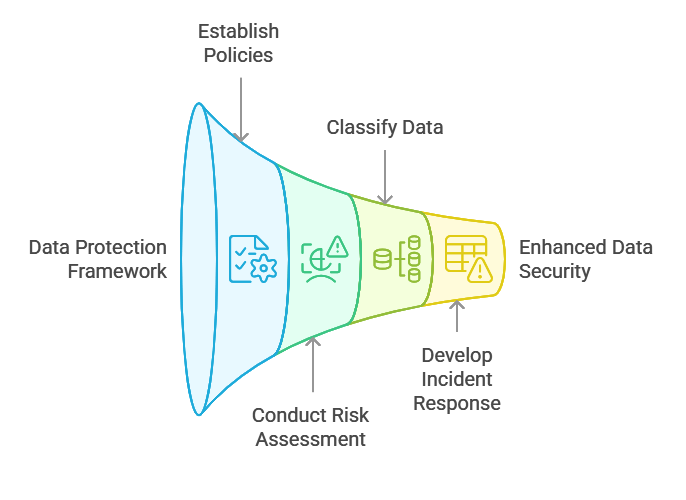
‍
Data protection frameworks, such as the GDPR framework, NIST data privacy framework, and APEC data privacy framework, are essential structures that organizations implement to safeguard personal data. These frameworks provide guidelines and best practices for managing data privacy and security. Key components of data protection frameworks include:
- Policies and Procedures: Establishing clear policies for data handling, storage, and processing.
- Risk Assessment: Regularly evaluating potential risks to data security and privacy.
- Data Classification: Categorizing data based on sensitivity to apply appropriate protection measures.
- Incident Response Plans: Developing strategies to respond to data breaches or security incidents effectively.
Organizations often adopt various frameworks, such as ISO 27001, NIST Cybersecurity Framework, and others, to ensure compliance with legal requirements and industry standards. These frameworks help in building trust with customers and stakeholders by demonstrating a commitment to data protection. At Rapid Innovation, we assist clients in implementing these frameworks effectively, including the data protection program framework and data privacy compliance framework, ensuring that their data protection strategies align with their business goals, ultimately leading to greater ROI through enhanced security and trust. Our expertise also extends to MLOps consulting services that can further enhance your data management capabilities. For more insights on managing data privacy, check out our article on best practices for AI and data privacy.
9.2. GDPR Compliance
The General Data Protection Regulation (GDPR) is a comprehensive data protection law in the European Union that came into effect in May 2018. GDPR compliance is crucial for any organization that processes personal data of EU citizens, regardless of where the organization is based. Key principles of GDPR compliance include:
- Lawfulness, Fairness, and Transparency: Organizations must process personal data lawfully and transparently.
- Purpose Limitation: Data should only be collected for specified, legitimate purposes and not further processed in a manner incompatible with those purposes.
- Data Minimization: Only the necessary amount of personal data should be collected and processed.
- Accuracy: Organizations must ensure that personal data is accurate and kept up to date.
- Storage Limitation: Personal data should not be kept longer than necessary for the purposes for which it was processed.
- Integrity and Confidentiality: Organizations must implement appropriate security measures to protect personal data.
Failure to comply with GDPR can result in significant fines, reaching up to €20 million or 4% of annual global turnover, whichever is higher. Therefore, organizations must prioritize GDPR compliance to avoid legal repercussions and maintain customer trust. Rapid Innovation offers consulting services to help clients navigate the complexities of GDPR compliance, including the GDPR compliance framework and GDPR controls framework, ensuring that they not only meet legal requirements but also enhance their operational efficiency and customer relationships.
9.3. User Consent Management
User consent management is a critical aspect of data protection, particularly under regulations like GDPR. It involves obtaining, managing, and documenting user consent for data processing activities. Important elements of user consent management include:
- Clear Consent Requests: Organizations must provide clear and concise information about what users are consenting to, including the purpose of data collection.
- Granular Consent Options: Users should have the ability to provide consent for specific data processing activities rather than a blanket consent for all.
- Easy Withdrawal of Consent: Users must be able to withdraw their consent easily at any time, and organizations should have processes in place to honor these requests.
- Documentation and Record-Keeping: Organizations should maintain records of consent to demonstrate compliance with data protection laws.
Effective user consent management not only ensures compliance with legal requirements but also enhances user trust and engagement. By being transparent and respectful of user privacy, organizations can foster stronger relationships with their customers. Rapid Innovation provides tailored solutions for user consent management, enabling clients to implement robust systems that not only comply with regulations but also drive customer loyalty and satisfaction, ultimately contributing to a higher return on investment.
9.4. Data Anonymization
Data anonymization is a crucial process in data management that involves removing or altering personally identifiable information (PII) from datasets. This practice ensures that individuals cannot be easily identified from the data, thereby enhancing privacy and compliance with regulations such as GDPR and HIPAA.
- Protects sensitive information: By anonymizing data, organizations can share datasets without risking exposure of personal information. This is particularly beneficial for companies looking to collaborate on research or analytics without compromising client confidentiality.
- Facilitates data analysis: Anonymized data can still be used for analytics, allowing businesses to derive insights without compromising individual privacy. For instance, Rapid Innovation can assist clients in developing AI models that leverage anonymized datasets to uncover trends and patterns, ultimately driving better business decisions.
- Reduces risk of data breaches: Anonymization minimizes the impact of potential data breaches, as the exposed data cannot be traced back to individuals. This is essential for organizations that handle sensitive information, as it helps maintain trust with customers and stakeholders.
- Supports compliance: Many regulations require data anonymization to protect user privacy, making it essential for organizations to implement these practices. Rapid Innovation can guide clients through the complexities of compliance, ensuring that their data management practices align with legal requirements.
- Techniques include: Â
- Masking: Replacing sensitive data with fictional but realistic values.
- Aggregation: Summarizing data to present it in a way that individual identities are not discernible.
- Perturbation: Adding noise to the data to obscure individual values while maintaining overall trends.
- K anonymization: Ensuring that each individual cannot be distinguished from at least k-1 others in the dataset.
- Pseudo anonymization: Replacing identifiable data with pseudonyms to protect individual identities while allowing data processing.
- Types of data anonymization: Various methods exist, including data masking and anonymization, which can be tailored to specific organizational needs.
- Data anonymization best practices: Organizations should adopt best practices to ensure effective anonymization, including regular audits and updates to anonymization techniques.
9.5. Audit Trails
Audit trails are systematic records that document the sequence of activities or transactions within a system. They are essential for maintaining accountability, security, and compliance in various industries.
- Enhances security: Audit trails help organizations track unauthorized access or changes to sensitive data, providing a clear record of who accessed what and when. This is vital for organizations that prioritize data integrity and security.
- Supports compliance: Many regulatory frameworks require organizations to maintain audit trails to demonstrate adherence to data protection laws. Rapid Innovation can assist clients in establishing robust audit trail systems that meet regulatory standards.
- Facilitates troubleshooting: In the event of a system failure or data breach, audit trails can help identify the root cause and prevent future occurrences. This capability is crucial for organizations aiming to enhance their operational resilience.
- Key components include: Â
- User identification: Recording who performed actions within the system.
- Timestamping: Documenting when each action occurred.
- Action details: Describing what actions were taken, such as data modifications or access attempts.
- Best practices for maintaining audit trails: Â
- Regularly review and analyze audit logs to identify anomalies.
- Ensure logs are tamper-proof and securely stored.
- Implement automated tools for real-time monitoring and alerts.
10. Use Case Implementations
Use case implementations refer to the practical applications of a system or technology in real-world scenarios. They demonstrate how specific features or functionalities can solve problems or enhance processes within an organization.
- Identifying business needs: Use cases help organizations pinpoint specific requirements and challenges that need addressing. Rapid Innovation can work with clients to develop tailored use cases that align with their strategic objectives.
- Enhancing user experience: By focusing on user interactions, use cases can guide the design of systems that are intuitive and user-friendly. This is particularly important in AI applications, where user engagement can significantly impact adoption rates.
- Supporting development: Use cases provide a clear framework for developers, ensuring that all necessary features are included in the final product. Rapid Innovation's expertise in AI and technology development ensures that these frameworks are effectively translated into functional solutions.
- Examples of use case implementations: Â
- Healthcare: Implementing electronic health records (EHR) systems to streamline patient data management and improve care coordination.
- Finance: Utilizing blockchain technology for secure and transparent transactions, reducing fraud and enhancing trust.
- Retail: Leveraging customer data analytics to personalize marketing strategies and improve customer engagement.
- Benefits of use case implementations: Â
- Improved efficiency: Streamlining processes can lead to significant time and cost savings.
- Increased innovation: Exploring various use cases can inspire new ideas and solutions.
- Better decision-making: Data-driven insights from use case implementations can inform strategic planning and operational improvements.
At Rapid Innovation, we are committed to helping our clients achieve greater ROI through effective data management, compliance, and innovative technology solutions. Our expertise in AI and data practices positions us as a valuable partner in navigating the complexities of modern business challenges. For more information on how we can assist you, consider hiring generative AI engineers to enhance your data anonymization efforts.
10.1. E-commerce Behavior Analysis
E-commerce behavior analysis involves studying how consumers interact with online shopping platforms. Understanding these behaviors is crucial for businesses aiming to enhance user experience and increase sales.
- Key metrics to analyze include: Â
- Conversion rates: The percentage of visitors who make a purchase.
- Cart abandonment rates: The percentage of users who add items to their cart but do not complete the purchase.
- Average order value (AOV): The average amount spent per transaction.
- Tools and techniques for behavior analysis: Â
- Web analytics: Platforms like Google Analytics provide insights into user behavior, traffic sources, and demographics.
- Heatmaps: Tools such as Hotjar or Crazy Egg visualize where users click, scroll, and spend time on a webpage.
- A/B testing: Experimenting with different website layouts or product placements to determine which version performs better.
- Benefits of e-commerce behavior analysis: Â
- Improved user experience through personalized recommendations, which can be powered by AI algorithms that analyze user data to suggest products tailored to individual preferences.
- Enhanced marketing strategies based on consumer preferences, allowing businesses to target their campaigns more effectively and increase ROI.
- Increased customer retention by understanding pain points and addressing them, leading to a more loyal customer base. For more insights on how AI can be integrated into financial planning, check out this article on AI in Financial Planning.
10.2. Content Platform Engagement
Content platform engagement refers to how users interact with content across various digital platforms. High engagement levels indicate that users find the content valuable and relevant.
- Factors influencing engagement: Â
- Content quality: Well-researched, informative, and entertaining content tends to attract more users.
- Visual appeal: Use of images, videos, and infographics can significantly enhance user engagement.
- Interactivity: Quizzes, polls, and comment sections encourage users to participate actively.
- Metrics to measure engagement: Â
- Time on page: The average duration users spend on a specific piece of content.
- Social shares: The number of times content is shared on social media platforms.
- Comments and feedback: User interactions in the form of comments can provide insights into audience sentiment.
- Strategies to boost engagement: Â
- Create compelling headlines that grab attention.
- Use storytelling techniques to make content relatable.
- Optimize content for mobile devices to reach a broader audience.
10.3. Financial Services Applications
Financial services applications encompass a wide range of digital tools designed to facilitate financial transactions, management, and planning. These applications have transformed how consumers and businesses manage their finances.
- Types of financial services applications: Â
- Mobile banking apps: Allow users to manage their bank accounts, transfer money, and pay bills from their smartphones.
- Investment platforms: Enable users to buy and sell stocks, bonds, and other securities easily.
- Budgeting tools: Help users track their spending, set financial goals, and manage their budgets effectively.
- Key features of successful financial applications: Â
- User-friendly interface: A clean, intuitive design enhances user experience.
- Security measures: Robust encryption and two-factor authentication protect sensitive financial data.
- Real-time updates: Providing users with up-to-date information on their accounts and transactions.
- Benefits of financial services applications: Â
- Increased accessibility to financial services for users worldwide, facilitated by AI-driven solutions that streamline processes and enhance user experience.
- Enhanced financial literacy through educational resources and tools, empowering users to make informed financial decisions.
- Streamlined processes that save time and reduce the need for in-person visits to banks or financial institutions, ultimately leading to greater efficiency and cost savings for both users and providers.
At Rapid Innovation, we leverage our expertise in AI to help clients implement these strategies effectively, ensuring they achieve greater ROI and meet their business goals efficiently. Additionally, our services include DeFi wallet development to enhance your financial applications.
10.4. Healthcare User Patterns

‍
Understanding healthcare user patterns is crucial for improving patient care and enhancing the overall healthcare experience. These patterns reveal how patients interact with healthcare services, which can inform better service delivery and resource allocation.
- Patient Engagement: Patients are increasingly using digital platforms to engage with healthcare providers, including scheduling appointments, accessing medical records, and communicating with healthcare professionals. Rapid Innovation can assist healthcare organizations in developing user-friendly digital solutions that enhance patient engagement and streamline communication.
- Telehealth Usage: The rise of telehealth has transformed how patients seek care. Many prefer virtual consultations for convenience and accessibility, especially for non-emergency issues. Our AI-driven telehealth solutions can optimize appointment scheduling and improve patient-provider interactions, leading to higher satisfaction rates.
- Mobile Health Applications: The use of mobile health apps is on the rise, with patients utilizing these apps for medication reminders, tracking health metrics, and accessing educational resources. Rapid Innovation can help design and implement mobile applications that cater to specific patient needs, ultimately improving adherence to treatment plans.
- Health Information Seeking: Patients often turn to online resources for health information before consulting a healthcare provider. This trend emphasizes the need for reliable and accurate online health content. Our expertise in AI can help healthcare providers create intelligent content delivery systems that ensure patients receive trustworthy information.
- Demographic Variations: User patterns can vary significantly across different demographics. Younger patients may prefer digital interactions, while older patients might favor traditional in-person visits. By leveraging data analytics, Rapid Innovation can help healthcare organizations tailor their services to meet the diverse needs of their patient populations. Additionally, our Metaverse Healthcare Solutions can provide innovative approaches to engage patients across various demographics. For more insights on enhancing patient interactions, check out our healthcare chatbot development.
By analyzing these patterns, healthcare providers can tailor their services to meet the needs of their patients more effectively.
10.5. Educational Platform Analytics
Educational platform analytics provide insights into how users interact with online learning environments. These analytics are essential for improving educational outcomes and enhancing user experience.
- User Engagement Metrics: Tracking metrics such as time spent on the platform, course completion rates, and participation in discussions can help educators understand user engagement levels. Rapid Innovation can implement AI analytics tools that provide real-time insights into user behavior, enabling educators to make data-driven decisions.
- Content Effectiveness: Analyzing which courses or modules are most popular can inform curriculum development. This data helps educators identify what content resonates with learners. Our solutions can facilitate adaptive learning experiences that cater to individual learning preferences.
- Learning Pathways: Understanding how users navigate through courses can reveal common learning pathways. This information can be used to optimize course structures and improve user flow. Rapid Innovation can develop AI algorithms that personalize learning pathways based on user interactions.
- Feedback Mechanisms: Collecting user feedback through surveys and assessments can provide qualitative insights into the learning experience. This feedback is vital for continuous improvement. Our AI-driven feedback analysis tools can help institutions quickly identify areas for enhancement.
- Demographic Insights: Analyzing user demographics can help tailor educational content to meet the needs of diverse learner groups, ensuring inclusivity and accessibility. Rapid Innovation can assist in creating targeted educational strategies that address the unique needs of various demographic segments.
By leveraging educational platform analytics, institutions can create more effective and engaging learning experiences.
11. Performance Optimization
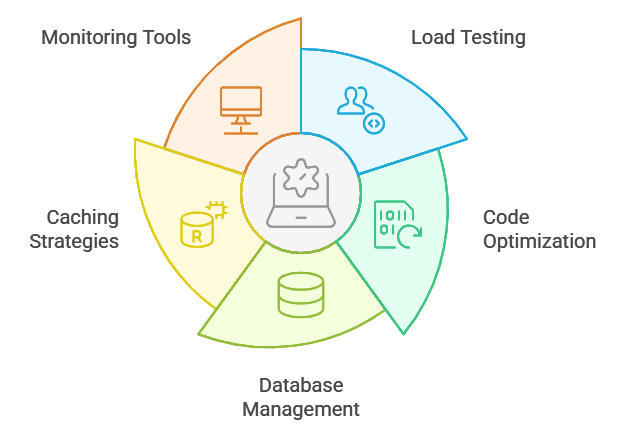
‍
Performance optimization is essential for ensuring that systems, applications, and services run efficiently and effectively. This process involves identifying bottlenecks and implementing strategies to enhance performance.
- Load Testing: Conducting load tests helps determine how a system performs under various conditions. This testing is crucial for identifying potential issues before they affect users. Rapid Innovation can implement advanced load testing solutions that simulate real-world usage scenarios.
- Code Optimization: Reviewing and refining code can lead to significant performance improvements, including eliminating redundancies and optimizing algorithms for faster execution. Our team of experts can provide code review services that enhance application performance.
- Database Management: Efficient database management practices, such as indexing and query optimization, can drastically improve data retrieval times and overall system performance. Rapid Innovation can design database architectures that maximize efficiency and scalability.
- Caching Strategies: Implementing caching can reduce load times by storing frequently accessed data in memory, minimizing the need for repeated data retrieval from slower storage systems. Our solutions can help organizations implement effective caching strategies tailored to their specific needs.
- Monitoring Tools: Utilizing performance monitoring tools allows for real-time tracking of system performance. These tools can alert administrators to issues before they escalate, ensuring a smooth user experience. Rapid Innovation can integrate AI-powered monitoring solutions that provide actionable insights for continuous improvement.
By focusing on performance optimization, organizations can enhance user satisfaction, reduce operational costs, and improve overall system reliability.
11.1. Model Optimization
Model optimization is a crucial process in machine learning and artificial intelligence that aims to enhance the performance of predictive models. It involves fine-tuning various parameters and algorithms to achieve the best possible accuracy and efficiency. At Rapid Innovation, we leverage our expertise in model optimization techniques to help clients achieve greater ROI by ensuring their AI models perform at peak efficiency.
- Key techniques for model optimization include: Â
- Hyperparameter tuning techniques: Adjusting parameters that govern the training process, such as learning rate, batch size, and number of epochs, to maximize model performance.
- Feature selection: Identifying and using only the most relevant features to improve model performance and reduce overfitting, which can lead to more accurate predictions.
- Regularization: Applying techniques like L1 or L2 regularization to prevent overfitting by penalizing large coefficients in the model, thus enhancing generalization.
- Ensemble methods: Combining multiple models to improve overall performance, such as bagging, boosting, and stacking, which can lead to more robust predictions.
- Tools and frameworks for model optimization: Â
- Grid Search: A method for systematically working through multiple combinations of parameter tunes, cross-validating as it goes to determine which tune gives the best performance.
- Random Search: A more efficient alternative to grid search that randomly samples parameter combinations, saving time while still achieving optimal results.
- Bayesian hyperparameter optimization: A probabilistic model that helps in finding the minimum of a function, often used for hyperparameter tuning, allowing for more informed decision-making.
Effective model optimization can lead to significant improvements in model accuracy, making it a vital step in the machine learning pipeline. By partnering with Rapid Innovation, clients can ensure their models are not only optimized for performance but also aligned with their business objectives, ultimately driving greater ROI. For specialized needs, we also offer transformer model development services to enhance your AI capabilities.
11.2. Resource Management
Resource management in computing refers to the efficient and effective allocation of resources such as CPU, memory, storage, and network bandwidth. Proper resource management is essential for optimizing performance and ensuring that applications run smoothly. At Rapid Innovation, we assist clients in implementing resource management strategies that enhance operational efficiency and reduce costs.
- Key aspects of resource management include: Â
- Load balancing: Distributing workloads evenly across servers to prevent any single server from becoming a bottleneck, ensuring optimal performance.
- Resource allocation: Assigning the right amount of resources to different applications based on their needs and priority, which can lead to improved application responsiveness.
- Monitoring and scaling: Continuously tracking resource usage and scaling resources up or down based on demand, often using cloud services for flexibility and cost-effectiveness.
- Benefits of effective resource management: Â
- Improved performance: Ensures that applications run efficiently without unnecessary delays, enhancing user satisfaction.
- Cost savings: Reduces waste by optimizing resource usage, which can lead to lower operational costs and increased profitability.
- Enhanced reliability: Minimizes downtime and improves the overall stability of applications, fostering trust and dependability.
Implementing robust resource management strategies is essential for organizations to maximize their IT infrastructure's potential and ensure seamless operations. Rapid Innovation's expertise in this area enables clients to achieve their business goals more effectively.
11.3. Caching Strategies
Caching strategies are techniques used to store frequently accessed data in a temporary storage area, known as a cache, to improve data retrieval speed and reduce latency. Effective caching can significantly enhance application performance and user experience. Rapid Innovation helps clients design and implement caching strategies that optimize their applications for speed and efficiency.
- Common caching strategies include: Â
- In-memory caching: Storing data in RAM for quick access, often using tools like Redis or Memcached, which can drastically reduce response times.
- Disk caching: Storing data on disk drives for larger datasets that do not fit in memory, though with slower access times compared to in-memory caching.
- Content Delivery Networks (CDNs): Distributing cached content across multiple geographical locations to reduce latency for users accessing the data from different regions.
- Factors to consider when implementing caching strategies: Â
- Cache expiration: Setting time limits for how long data should remain in the cache to ensure that users receive up-to-date information.
- Cache invalidation: Establishing rules for when cached data should be refreshed or removed to prevent stale data from being served.
- Cache size: Determining the optimal size of the cache to balance between performance and resource usage.
By employing effective caching strategies, organizations can significantly improve application performance, reduce server load, and enhance the overall user experience. Rapid Innovation's tailored caching solutions ensure that clients can meet their performance goals while maximizing resource efficiency.
11.4. Query Optimization
Query optimization is a critical process in database management that enhances the performance of database queries. It involves analyzing and improving the execution of SQL queries to ensure they run efficiently. Effective query optimization can significantly reduce response times and resource consumption, ultimately leading to greater ROI for businesses.
- Understanding Query Execution Plans: Database systems generate execution plans that outline how queries will be executed. Analyzing these plans helps identify bottlenecks and inefficient operations, allowing Rapid Innovation to fine-tune queries for optimal performance, including the use of sql query optimizer techniques.
- Indexing: Proper indexing can drastically improve query performance. Indexes allow the database to find data without scanning entire tables, reducing the time taken for data retrieval. Rapid Innovation employs advanced indexing strategies to ensure clients experience faster data access, which is a key aspect of performance tuning in sql.
- Query Rewriting: Sometimes, rewriting a query can lead to better performance. Simplifying complex queries or breaking them into smaller parts can enhance execution speed. Our team at Rapid Innovation specializes in query rewriting techniques that maximize efficiency, including optimising sql queries for better results.
- Statistics and Histograms: Maintaining up-to-date statistics about data distribution helps the query optimizer make informed decisions. Histograms provide a more detailed view of data distribution, aiding in better execution plan selection. Rapid Innovation ensures that clients' databases are equipped with accurate statistics for optimal query performance, leveraging sql optimization techniques.
- Avoiding Unnecessary Columns: Selecting only the necessary columns in a query reduces the amount of data processed. This practice minimizes memory usage and speeds up data retrieval, which is a key focus area for our optimization strategies, including sql code optimizer practices.
- Using Joins Wisely: Understanding the types of joins (inner, outer, etc.) and their performance implications is crucial. Optimizing join conditions can lead to significant performance improvements. Rapid Innovation helps clients implement effective join strategies to enhance query execution, which is part of our sql tuning techniques.
- Limiting Result Sets: Using clauses like
LIMIT
orTOP
can reduce the number of rows returned, speeding up query execution. This is particularly useful in applications where only a subset of data is needed, and our solutions are designed to leverage this technique effectively, aligning with performance optimization in sql. For more insights on optimizing resources, check out AI agents for IT resource optimization.
11.5. Load Balancing
Load balancing is a technique used to distribute workloads across multiple computing resources, ensuring no single resource is overwhelmed. In database management, load balancing enhances performance, reliability, and availability, which are essential for achieving business goals.
- Types of Load Balancing: Â
- Horizontal Scaling: Adding more servers to distribute the load.
- Vertical Scaling: Upgrading existing servers to handle more load.
- Database Replication: Replicating databases across multiple servers allows read requests to be distributed, reducing the load on a single database server and improving response times. Rapid Innovation implements robust replication strategies to enhance system performance.
- Connection Pooling: Connection pooling allows multiple clients to share a limited number of database connections, reducing the overhead of establishing connections and improving resource utilization. Our solutions optimize connection pooling to ensure efficient resource management.
- Traffic Distribution: Load balancers can intelligently route traffic based on current server loads, ensuring that no single server becomes a bottleneck. Rapid Innovation utilizes advanced traffic distribution techniques to maintain high availability.
- Failover Mechanisms: Load balancing can include failover strategies to maintain availability. If one server fails, traffic can be redirected to another server without downtime, ensuring business continuity.
- Monitoring and Analytics: Continuous monitoring of server performance helps in making informed load balancing decisions. Analytics can identify trends and predict when to scale resources, allowing Rapid Innovation to proactively manage client systems.
- Cloud-Based Load Balancing: Many cloud providers offer built-in load balancing solutions. These services automatically adjust resources based on demand, providing flexibility and scalability that Rapid Innovation leverages for client solutions.
12. Future Developments
The future of database management is poised for significant advancements driven by emerging technologies and evolving user needs. As data continues to grow exponentially, innovative solutions will be necessary to manage and utilize this data effectively.
- Artificial Intelligence and Machine Learning: AI and ML will play a crucial role in automating database management tasks. Predictive analytics can optimize performance and enhance decision-making processes, which Rapid Innovation integrates into its solutions to drive efficiency.
- Serverless Databases: The rise of serverless architectures will change how databases are deployed and managed. This model allows developers to focus on application logic without worrying about infrastructure management.
- Multi-Model Databases: Future databases will increasingly support multiple data models (e.g., relational, document, graph). This flexibility will allow organizations to choose the best model for their specific use cases.
- Increased Focus on Security: As data breaches become more common, future developments will prioritize security features. Enhanced encryption, access controls, and auditing capabilities will be essential.
- Real-Time Data Processing: The demand for real-time analytics will drive the development of databases that can handle streaming data. Technologies like Apache Kafka and NoSQL databases will become more prevalent.
- Edge Computing: With the growth of IoT devices, databases will need to support edge computing. This will enable data processing closer to the source, reducing latency and bandwidth usage.
- Quantum Computing: Although still in its infancy, quantum computing has the potential to revolutionize database management. It could enable faster data processing and complex problem-solving capabilities.
- Enhanced User Interfaces: Future database management systems will focus on user-friendly interfaces. Improved visualization tools will help users interact with data more intuitively.
These developments will shape the future landscape of database management, making it more efficient, secure, and adaptable to changing technological environments. Rapid Innovation is committed to staying at the forefront of these advancements, ensuring our clients achieve their business goals effectively and efficiently.
12.1. Emerging Technologies
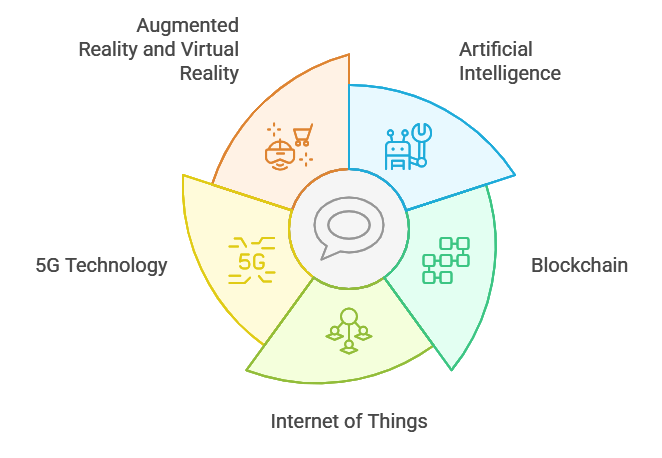
‍
Emerging technologies are reshaping industries and driving innovation across various sectors. These technologies are characterized by their potential to disrupt existing markets and create new opportunities. Key emerging technologies include:
- Artificial Intelligence (AI): AI is revolutionizing how businesses operate, enabling automation, predictive analytics, and enhanced decision-making. At Rapid Innovation, we harness AI to help clients streamline operations, reduce costs, and improve customer engagement, ultimately leading to greater ROI. New AI technology and advancements in artificial intelligence technology are at the forefront of this transformation.
- Blockchain: This decentralized ledger technology is transforming finance, supply chain management, and data security by providing transparency and traceability. Our expertise in blockchain allows clients to implement secure and efficient systems that enhance trust and accountability in their transactions.
- Internet of Things (IoT): IoT connects devices and systems, allowing for real-time data collection and analysis, which enhances operational efficiency and customer experiences. Rapid Innovation assists clients in leveraging IoT to gather actionable insights, optimize processes, and create smarter products. Emerging technologies in healthcare are also utilizing IoT for better patient monitoring and care.
- 5G Technology: The rollout of 5G networks is set to enhance connectivity, enabling faster data transfer and supporting the growth of smart cities and autonomous vehicles. We guide clients in adopting 5G solutions that improve communication and operational capabilities.
- Augmented Reality (AR) and Virtual Reality (VR): These technologies are changing how consumers interact with products and services, offering immersive experiences in gaming, education, and retail. Rapid Innovation helps businesses integrate AR and VR to create engaging customer experiences that drive sales and brand loyalty.
The impact of these technologies is profound, as they not only improve efficiency but also create new business models and revenue streams. Companies that adopt these emerging technologies can gain a competitive edge in their respective markets. New technologies in health and emerging technologies in healthcare industry are examples of sectors benefiting from these advancements.
12.2. AI Model Evolution
The evolution of AI models has been a significant driver of advancements in technology. Over the years, AI has transitioned from simple rule-based systems to complex machine learning algorithms. Key aspects of AI model evolution include:
- Deep Learning: This subset of machine learning uses neural networks with many layers to analyze data patterns, leading to breakthroughs in image and speech recognition. Rapid Innovation employs deep learning techniques to develop solutions that enhance accuracy and performance for our clients.
- Natural Language Processing (NLP): NLP has evolved to enable machines to understand and generate human language, facilitating applications like chatbots and virtual assistants. We leverage NLP to create intelligent systems that improve customer interactions and streamline communication.
- Reinforcement Learning: This approach allows AI systems to learn through trial and error, optimizing their performance in dynamic environments, such as gaming and robotics. Our expertise in reinforcement learning enables clients to develop adaptive systems that improve over time.
- Transfer Learning: This technique enables models trained on one task to be adapted for another, significantly reducing the time and data required for training. Rapid Innovation utilizes transfer learning to accelerate project timelines and reduce costs for our clients.
- Explainable AI (XAI): As AI systems become more complex, the need for transparency and interpretability has led to the development of XAI, which helps users understand AI decision-making processes. We prioritize XAI in our solutions to ensure clients can trust and validate their AI systems.
The evolution of AI models is crucial for enhancing their accuracy, efficiency, and applicability across various domains, including healthcare, finance, and transportation. The latest technological advancements in AI are paving the way for new applications and solutions.
12.3. Integration Possibilities
The integration of emerging technologies and AI models presents numerous possibilities for innovation and efficiency. Organizations can leverage these technologies to create synergies that enhance their operations. Key integration possibilities include:
- Smart Automation: Combining AI with IoT can lead to smart automation solutions that optimize processes in manufacturing, logistics, and supply chain management. Rapid Innovation helps clients implement these solutions to reduce operational costs and improve productivity.
- Data-Driven Decision Making: Integrating AI with big data analytics allows organizations to derive actionable insights from vast amounts of data, improving strategic planning and operational efficiency. We assist clients in building data-driven cultures that enhance decision-making capabilities.
- Enhanced Customer Experiences: By integrating AI with AR and VR, businesses can create personalized and immersive customer experiences, leading to increased engagement and satisfaction. Our team at Rapid Innovation designs tailored solutions that elevate customer interactions and drive loyalty.
- Predictive Maintenance: The combination of AI and IoT enables predictive maintenance in industries like manufacturing and transportation, reducing downtime and maintenance costs. We work with clients to implement predictive maintenance strategies that enhance asset reliability.
- Blockchain and AI: Integrating AI with blockchain can enhance security and efficiency in transactions, enabling smarter contracts and fraud detection. Rapid Innovation guides clients in leveraging this integration to create secure and efficient business processes.
These integration possibilities not only streamline operations but also foster innovation, allowing businesses to stay ahead in a rapidly changing technological landscape. At Rapid Innovation, we are committed to helping our clients navigate these emerging technologies to achieve their business goals efficiently and effectively. New technology trends and best new technologies are essential for staying competitive in this environment.
12.4. Scalability Improvements
Scalability is a critical aspect of any system, especially in the context of technology and software development. It refers to the ability of a system to handle an increasing amount of work or its potential to accommodate growth. Recent advancements in scalability improvements have focused on several key areas:
- Cloud Computing: The rise of cloud services has enabled businesses to scale their operations without the need for significant upfront investment in hardware. This allows for flexible resource allocation based on demand, which Rapid Innovation leverages to help clients optimize their infrastructure costs and improve operational efficiency.
- Microservices Architecture: By breaking down applications into smaller, independent services, organizations can scale individual components without affecting the entire system. This approach enhances deployment speed and system resilience, enabling Rapid Innovation to assist clients in adopting agile methodologies that foster innovation and faster time-to-market.
- Load Balancing: Implementing load balancers helps distribute incoming traffic across multiple servers, ensuring no single server becomes a bottleneck. This leads to improved performance and reliability, which Rapid Innovation can integrate into client systems to enhance user experience and satisfaction.
- Database Sharding: This technique involves splitting a database into smaller, more manageable pieces, allowing for parallel processing and improved query performance. It is particularly useful for handling large datasets, and Rapid Innovation employs this strategy to help clients manage their data more effectively, leading to better insights and decision-making.
- Content Delivery Networks (CDNs): CDNs cache content closer to users, reducing latency and improving load times. This is essential for applications with a global user base, and Rapid Innovation can implement CDN solutions to ensure that clients' applications perform optimally across different regions.
- Horizontal Scaling: This method involves adding more machines or instances to handle increased load rather than upgrading existing hardware. It is often more cost-effective and provides better redundancy, allowing Rapid Innovation to guide clients in scaling their operations efficiently while maximizing ROI. For those interested in expanding their capabilities, security token development services can be a valuable addition. Additionally, exploring decentralized identity systems can further enhance scalability and security.
Recent scalability improvements have also emphasized the importance of optimizing existing systems to ensure they can grow alongside business needs, thereby enhancing overall performance and user satisfaction.
13.1. Common Issues
In software development and IT operations, various common issues can arise that hinder performance and functionality. Understanding these issues is crucial for effective troubleshooting and maintenance.
- Software Bugs: These are errors in the code that can lead to unexpected behavior. Bugs can arise from syntax errors, logical errors, or incorrect assumptions made during development.
- Configuration Errors: Misconfigurations in software settings can lead to system failures or degraded performance. This often occurs when default settings are not adjusted to fit specific use cases.
- Network Problems: Connectivity issues can disrupt communication between systems. This includes problems like high latency, packet loss, or complete outages.
- Resource Limitations: Insufficient memory, CPU, or disk space can lead to application crashes or slow performance. Monitoring resource usage is essential to prevent these issues.
- Compatibility Issues: Software may not function correctly across different platforms or versions. This is particularly common when integrating third-party libraries or services.
- Security Vulnerabilities: Flaws in software can expose systems to attacks. Regular updates and patches are necessary to mitigate these risks.
- User Errors: Sometimes, issues arise from user actions, such as incorrect data entry or improper use of software features.
At Rapid Innovation, we understand these challenges and leverage our AI-driven solutions to help clients identify and resolve these common issues efficiently. By implementing advanced application performance monitoring tools and debugging tools, we enable businesses to minimize downtime and enhance overall system performance, ultimately leading to greater ROI. Additionally, we explore the role of AI agents in software testing to further enhance our troubleshooting capabilities.
13.2. Debugging Strategies
Debugging is a critical process in software development that involves identifying and resolving issues. Employing effective debugging strategies can significantly reduce the time spent on troubleshooting.
- Reproduce the Issue: Start by replicating the problem consistently. This helps in understanding the conditions under which the issue occurs.
- Check Logs: Review application and system logs for error messages or warnings. Logs can provide valuable insights into what went wrong.
- Use Debugging Tools: Utilize integrated development environment (IDE) debugging tools to step through code, inspect variables, and monitor execution flow.
- Isolate Components: Break down the application into smaller parts to identify where the issue lies. This can involve disabling certain features or modules temporarily.
- Consult Documentation: Refer to official documentation for libraries or frameworks being used. This can help clarify expected behavior and identify potential misuses.
- Peer Review: Collaborate with colleagues to review the code. A fresh set of eyes can often spot issues that the original developer may have overlooked.
- Test Cases: Implement unit tests and integration tests to catch issues early in the development process. Automated testing can help ensure that new changes do not introduce new bugs.
At Rapid Innovation, we emphasize the importance of a structured debugging approach. Our team employs AI-enhanced debugging tools that can analyze code patterns and predict potential issues, allowing for quicker resolutions and improved software quality.
13.3. Performance Monitoring
Performance monitoring is essential for maintaining the health of applications and systems. It involves tracking various metrics to ensure optimal performance and quick identification of issues.
- Resource Utilization: Monitor CPU, memory, disk, and network usage to identify bottlenecks. Tools like
Prometheus
orGrafana
can provide real-time insights. - Response Times: Track the time it takes for applications to respond to user requests. High response times can indicate performance issues that need addressing.
- Error Rates: Keep an eye on the frequency of errors occurring in the application. A sudden spike in error rates can signal underlying problems.
- User Experience Metrics: Measure user engagement and satisfaction through metrics like page load times and transaction completion rates. Tools like
Google Analytics
can help gather this data. - Application Performance Management (APM): Implement application performance management tools to gain deeper insights into application performance. These tools can help identify slow transactions and pinpoint the root cause of performance issues.
- Alerts and Notifications: Set up alerts for critical performance thresholds. This ensures that teams are notified immediately when performance dips below acceptable levels.
- Regular Reviews: Conduct periodic performance reviews to assess system health and make necessary adjustments. This proactive approach can prevent issues before they impact users.
At Rapid Innovation, we utilize AI-driven application performance monitoring solutions that provide predictive analytics, enabling businesses to anticipate issues before they arise. This proactive stance not only enhances system reliability but also contributes to a significant increase in ROI by ensuring optimal performance and user satisfaction.
13.4. System Updates
System updates are crucial for maintaining the security, performance, and functionality of any software or hardware system. Regular updates ensure that systems are equipped with the latest features and security patches, which can protect against vulnerabilities and improve user experience.
- Security Enhancements: Updates often include patches for known vulnerabilities, reducing the risk of cyberattacks. Keeping systems updated is essential for safeguarding sensitive data, particularly in AI applications where data integrity is paramount.
- Performance Improvements: Updates can optimize system performance, making applications run smoother and faster. This can lead to increased productivity and a better user experience, allowing businesses to leverage AI solutions more effectively.
- New Features: Many updates introduce new functionalities that can enhance usability and provide users with additional tools to work more efficiently. For instance, AI-driven analytics tools can be enhanced through regular updates, providing clients with deeper insights.
- Compatibility: Regular updates ensure that software remains compatible with other applications and systems, preventing issues that can arise from outdated software. This is especially important in AI environments where integration with various data sources is critical.
- Compliance: For businesses, staying updated is often necessary to comply with industry regulations and standards, which can help avoid legal issues. Rapid Innovation assists clients in navigating these compliance requirements through timely updates.
To ensure effective system updates, organizations should establish a routine schedule for checking and applying updates. This can include:
- Automatic updates where feasible, to minimize manual intervention.
- Regular audits to ensure all systems are up to date.
- User training to inform staff about the importance of updates and how to apply them.
13.5. Backup and Recovery
Backup and recovery processes are essential components of data management strategies. They ensure that critical data is preserved and can be restored in the event of data loss due to hardware failure, cyberattacks, or natural disasters.
- Data Protection: Regular backups protect against data loss, ensuring that important information is not permanently lost. This is vital for both personal and business data, particularly in AI projects where data is a key asset.
- Disaster Recovery: A robust recovery plan allows organizations to quickly restore operations after a data loss incident, minimizing downtime and financial impact. Rapid Innovation helps clients develop tailored disaster recovery plans that align with their specific needs.
- Version Control: Backups can provide access to previous versions of files, allowing users to recover from accidental deletions or unwanted changes. This is particularly useful in AI model development, where iterative improvements are common.
- Compliance and Legal Requirements: Many industries have regulations that require data to be backed up and retrievable, making a solid backup strategy essential for compliance. Rapid Innovation ensures that clients meet these requirements through comprehensive backup solutions.
- Peace of Mind: Knowing that data is backed up can reduce anxiety for users and organizations, allowing them to focus on their core activities without fear of data loss.
To implement an effective backup and recovery strategy, consider the following best practices:
- Regular Backup Schedule: Establish a routine for backups, whether daily, weekly, or monthly, depending on the volume and importance of the data.
- Multiple Backup Locations: Use a combination of on-site and off-site backups to ensure data is safe from local disasters.
- Test Recovery Procedures: Regularly test the recovery process to ensure that backups can be restored quickly and effectively when needed.
- Use Reliable Backup Solutions: Invest in reputable backup software and hardware to ensure data integrity and security.
- Educate Users: Train staff on the importance of backups and how to access recovery options, ensuring everyone understands their role in data protection. Rapid Innovation provides training and support to empower organizations in their data management efforts, including system updates and what's new in OpenAI's fine-tuning API.
‍