Table Of Contents
Category
Real Estate
Retail and Ecommerce
Banking and Financial services
Decentralized Finance (DeFi)
Machine Learning (ML)
Natural Language Processing (NLP)
Artificial Intelligence (AI)
Decentralized Applications (DApps)
1. Introduction to AI Property Valuation
Artificial Intelligence (AI) is transforming various industries, and real estate is no exception. AI property valuation refers to the use of advanced algorithms and machine learning techniques to assess the value of real estate properties. This innovative approach enhances traditional valuation methods by providing more accurate, efficient, and data-driven insights. As the real estate market becomes increasingly competitive, AI property valuation is emerging as a vital tool for investors, appraisers, and real estate professionals.
- AI property valuation leverages vast amounts of data.
- It improves accuracy and reduces human error.
- The technology is reshaping how properties are bought, sold, and financed.
1.1. Evolution of Property Valuation
The process of property valuation has evolved significantly over the years. Traditionally, property valuation relied heavily on manual assessments conducted by appraisers. These assessments were based on comparable sales, property conditions, and local market trends. However, this method had its limitations, including subjectivity and time consumption. Early methods focused on physical inspections and manual calculations. The introduction of automated valuation models (AVMs) in the 1990s marked a significant shift, as AVMs utilized statistical models to estimate property values based on historical data.
With the advent of AI, property valuation has taken another leap forward. AI algorithms can analyze vast datasets, including market trends, economic indicators, and property features, to generate more precise valuations. This evolution has led to:
- Enhanced speed and efficiency in the valuation process.
- The ability to incorporate real-time data for more accurate assessments.
- A reduction in costs associated with traditional appraisal methods.
1.2. Role of AI in Real Estate
AI plays a crucial role in the real estate sector, particularly in property valuation. Its applications extend beyond mere valuation, impacting various aspects of the industry.
- Predictive Analytics: AI can forecast market trends and property values by analyzing historical data and current market conditions. This helps investors make informed decisions.
- Risk Assessment: AI algorithms can identify potential risks associated with property investments, such as market fluctuations or neighborhood changes.
- Personalized Recommendations: AI can analyze user preferences and behaviors to provide tailored property recommendations, enhancing the buying experience.
At Rapid Innovation, we harness the power of artificial intelligence real estate valuation to provide our clients with cutting-edge property valuation solutions. By integrating advanced algorithms and machine learning techniques, we help real estate professionals achieve greater accuracy and efficiency in their valuations, ultimately leading to improved ROI. The integration of AI in real estate is not just about valuation; it also streamlines processes, improves customer experiences, and enhances decision-making. As technology continues to advance, the role of AI in real estate will likely expand, offering even more innovative solutions for property valuation and beyond.
Refer to the image for a visual representation of the concepts discussed in the introduction to AI property valuation.
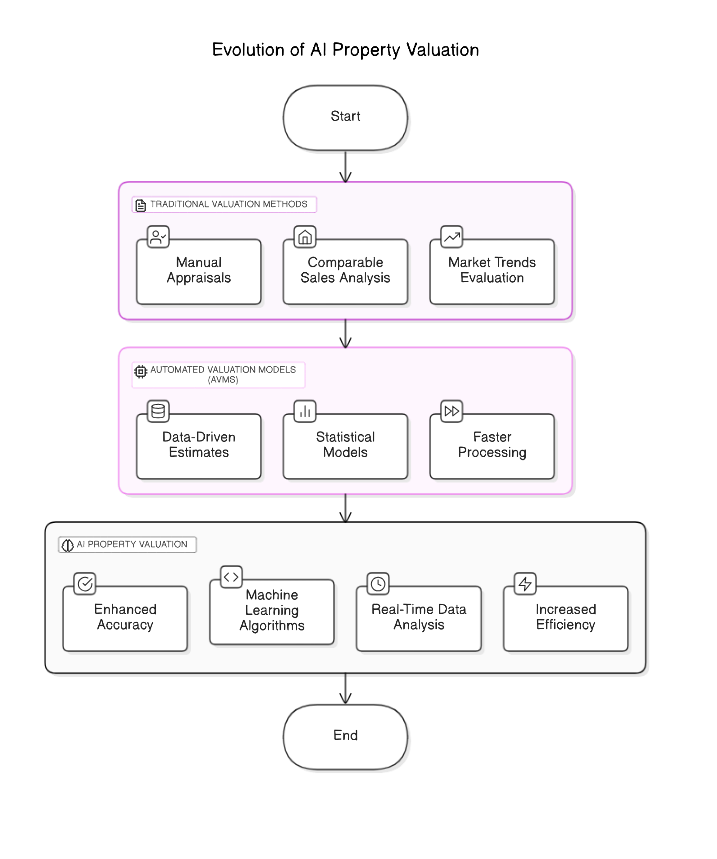
1.3. Benefits of Predictive Analysis
Predictive analysis is a powerful tool that leverages data, statistical algorithms, and machine learning techniques to identify the likelihood of future outcomes based on historical data. The benefits of predictive analysis are vast and can significantly impact various sectors.
- Enhanced Decision-Making: Predictive analysis provides actionable insights that help organizations make informed decisions. By understanding potential future trends, businesses can allocate resources more effectively, leading to improved operational efficiency. This is a key benefit of predictive analytics for business advantage.
- Improved Customer Experience: By analyzing customer behavior and preferences, companies can tailor their offerings to meet specific needs, resulting in increased customer satisfaction and loyalty. This personalized approach can drive repeat business and enhance brand reputation. Predictive analytics is beneficial to us to know how to better serve our customers.
- Cost Reduction: Predictive analysis can identify inefficiencies and areas for cost savings. For instance, businesses can optimize inventory levels, reducing holding costs and minimizing waste, which directly contributes to improved profit margins.
- Risk Management: Organizations can anticipate potential risks and develop strategies to mitigate them. This proactive approach can prevent losses and enhance overall stability, allowing businesses to navigate uncertainties with confidence.
- Increased Revenue: By predicting market trends and consumer behavior, businesses can capitalize on opportunities, leading to increased sales and revenue growth. This capability enables organizations to make timely investments and strategic decisions.
- Competitive Advantage: Companies that effectively utilize predictive analysis can stay ahead of competitors by quickly adapting to market changes and consumer demands. This agility can be a key differentiator in today's fast-paced business environment. The benefit of predictive analytics is evident in how it allows organizations to maintain a competitive edge. For more insights on this topic, check out the power of predictive analysis in retail.
1.4. Market Impact and Adoption
The adoption of predictive analysis is rapidly growing across various industries, driven by advancements in technology and the increasing availability of data. The market impact is significant, influencing how businesses operate and strategize.
- Industry Growth: The predictive analytics market is projected to grow substantially, with estimates suggesting it could reach over $10 billion by 2025. This growth is fueled by the need for data-driven decision-making.
- Diverse Applications: Predictive analysis is being adopted in various sectors, including healthcare, finance, retail, and manufacturing. Each industry utilizes predictive models to enhance operations, improve customer engagement, and drive innovation.
- Increased Investment: Organizations are investing heavily in predictive analytics tools and technologies. This investment is aimed at harnessing the power of big data and machine learning to gain insights that were previously unattainable.
- Integration with AI: The integration of predictive analysis with artificial intelligence (AI) is transforming how businesses operate. AI enhances predictive models, making them more accurate and efficient, which in turn maximizes return on investment (ROI) for organizations.
- Challenges in Adoption: Despite its benefits, some organizations face challenges in adopting predictive analysis, including data quality issues, lack of skilled personnel, and resistance to change. Overcoming these challenges is crucial for successful implementation.
2. Core Technology Components
The effectiveness of predictive analysis relies on several core technology components that work together to process data and generate insights.
- Data Collection: The first step in predictive analysis is gathering relevant data from various sources. This can include structured data from databases and unstructured data from social media, customer feedback, and more.
- Data Processing: Once collected, data must be cleaned and processed to ensure accuracy. This involves removing duplicates, handling missing values, and transforming data into a usable format.
- Statistical Algorithms: Predictive analysis employs various statistical algorithms to analyze data patterns. Common techniques include regression analysis, decision trees, and neural networks, each suited for different types of data and predictions.
- Machine Learning: Machine learning plays a crucial role in predictive analysis by enabling systems to learn from data and improve over time. Algorithms can adapt to new data, enhancing the accuracy of predictions.
- Visualization Tools: Effective visualization tools are essential for presenting predictive analysis results. These tools help stakeholders understand complex data insights through charts, graphs, and dashboards.
- Deployment and Monitoring: After developing predictive models, organizations must deploy them into their operational systems. Continuous monitoring is necessary to ensure models remain accurate and relevant as new data becomes available. Predictive analysis benefits organizations by providing insights that inform these processes.
Refer to the image below for a visual representation of the benefits of predictive analysis.

2.1. Machine Learning Models
Machine learning models are algorithms that enable computers to learn from and make predictions based on data. These models are essential in various applications, from finance to healthcare, and they can be categorized into several types:
- Supervised Learning: This involves training a model on labeled data, where the outcome is known. Common algorithms include linear regression, decision trees, and support vector machines. For instance, linear regression with scikit learn is a popular method for predicting continuous outcomes.
- Unsupervised Learning: In this approach, the model works with unlabeled data to identify patterns or groupings. Clustering algorithms like K-means and hierarchical clustering fall under this category. Restricted Boltzmann machines are also used in unsupervised learning tasks.
- Reinforcement Learning: This type of learning involves training models to make sequences of decisions by rewarding desired outcomes. It is widely used in robotics and game playing.
At Rapid Innovation, we specialize in developing tailored machine learning models that align with your business objectives. By employing techniques such as feature engineering, hyperparameter tuning, and ensemble methods, we enhance model performance to ensure you achieve greater ROI.
- Feature Engineering: Selecting and transforming variables to improve model performance, which is crucial in building effective machine learning models.
- Hyperparameter Tuning: Adjusting model parameters to optimize performance, particularly important in complex models like neural networks and random forests.
- Ensemble Methods: Combining multiple models, such as classification random forest and decision tree machine learning, to improve accuracy and robustness.
The effectiveness of machine learning models is often measured using metrics like accuracy, precision, recall, and F1 score. These metrics help in evaluating how well the model performs on unseen data, ensuring that your investment translates into actionable insights. For more information on how we can assist you with MLOps consulting services, visit our MLOps consulting services and learn about the power of data annotation services in enhancing AI and machine learning models.
2.2. Data Analytics Engine
A data analytics engine is a system designed to process and analyze large volumes of data efficiently. It plays a crucial role in transforming raw data into actionable insights. Key components of a data analytics engine include:
- Data Ingestion: The process of collecting and importing data from various sources, such as databases, APIs, and real-time streams.
- Data Processing: This involves cleaning, transforming, and aggregating data to prepare it for analysis. Techniques like ETL (Extract, Transform, Load) are commonly used.
- Data Storage: Storing processed data in databases or data warehouses for easy access and retrieval. Options include SQL databases, NoSQL databases, and cloud storage solutions.
- Data Visualization: Presenting data in graphical formats to help stakeholders understand trends and patterns. Tools like Tableau and Power BI are popular for this purpose.
- Analytics and Reporting: Generating reports and dashboards that provide insights into business performance and trends. This can include predictive analytics, which uses historical data to forecast future outcomes.
At Rapid Innovation, we build robust data analytics engines that significantly enhance decision-making processes by providing timely and relevant insights, ultimately driving business growth and maximizing your return on investment.
2.3. Market Intelligence Integration
Market intelligence integration refers to the process of combining various data sources and analytical tools to gain a comprehensive understanding of market trends, customer behavior, and competitive dynamics. This integration is vital for businesses looking to stay ahead in a rapidly changing environment. Key aspects include:
- Data Sources: Integrating data from multiple sources, such as social media, customer feedback, sales data, and market research reports, to create a holistic view of the market.
- Competitive Analysis: Monitoring competitors' activities, pricing strategies, and product offerings to identify opportunities and threats. This can involve tools like SWOT analysis and Porter’s Five Forces.
- Customer Insights: Analyzing customer data to understand preferences, buying behavior, and demographics. This helps in tailoring marketing strategies and product development.
- Trend Analysis: Identifying emerging trends and shifts in consumer behavior through data analysis. This can involve using machine learning models, such as neural networks and deep learning neural networks, to predict future market movements.
- Reporting and Dashboards: Creating visual representations of market intelligence data to facilitate quick decision-making. Dashboards can provide real-time insights into market conditions.
Integrating market intelligence into business strategies allows organizations to make informed decisions, optimize marketing efforts, and enhance customer satisfaction. By leveraging data analytics and machine learning, including tools like scikit learn random forest and decision tree scikit learn, Rapid Innovation empowers companies to gain a competitive edge in their respective industries, ensuring that your business goals are met efficiently and effectively.
Refer to the image for a visual representation of the concepts discussed in section 2.1 on Machine Learning Models.

2.4. Prediction Algorithms
Prediction algorithms are essential tools in data analysis and machine learning, enabling organizations to forecast future trends and behaviors based on historical data. These algorithms utilize statistical techniques and machine learning models, such as xgboost predict, logistic regression for prediction, and neural network market prediction, to identify patterns and make predictions, ultimately helping businesses achieve greater ROI.
- Types of prediction algorithms include: Â
- Regression Analysis: Used for predicting continuous outcomes. For example, linear regression can forecast sales based on historical data, allowing businesses to optimize inventory and marketing strategies. Logistic regression in r predict is a common method used in this category.
- Classification Algorithms: These algorithms categorize data into predefined classes. Examples include decision trees and support vector machines (svm prediction), which can be employed for customer segmentation and targeted marketing campaigns.
- Time Series Analysis: This method analyzes data points collected or recorded at specific time intervals, useful for stock market predictions or weather forecasting, enabling organizations to make proactive decisions. Algorithms for stock price prediction, such as xgboost for time series forecasting, are particularly valuable in this context.
- Key components of prediction algorithms: Â
- Data Preparation: Cleaning and organizing data to ensure accuracy, which is crucial for reliable predictions. Techniques like kmeans predict can be used for clustering data before applying prediction algorithms.
- Model Selection: Choosing the right algorithm based on the problem type and data characteristics, ensuring the model aligns with business objectives. For instance, machine learning algorithms for prediction can include random forest prediction and knn predict.
- Training and Testing: Splitting data into training and testing sets to evaluate model performance, allowing for continuous improvement and refinement. Superlearner r is a technique that can enhance model performance by combining multiple algorithms.
- Applications of prediction algorithms: Â
- Finance: Credit scoring and risk assessment, helping financial institutions minimize losses and enhance customer trust. For example, heart disease prediction using machine learning can also be applied in healthcare finance.
- Healthcare: Predicting patient outcomes and disease outbreaks, which can lead to better resource allocation and improved patient care. Bank churn prediction neural network is an example of a specific application in this field.
- Retail: Inventory management and customer behavior forecasting, enabling retailers to optimize stock levels and enhance customer satisfaction. Prediction algorithms in machine learning can significantly improve these processes.
Understanding and implementing prediction algorithms, such as those for market prediction and stock prediction algorithms, can significantly enhance decision-making processes across various industries, ultimately driving efficiency and effectiveness in achieving business goals. For more insights on how AI agents can be utilized in marketing applications.
2.5. Real-time Processing Systems
Real-time processing systems are designed to process data as it is generated, allowing organizations to respond to events and changes instantaneously. These systems are crucial in environments where timely information is essential, providing a competitive edge.
- Characteristics of real-time processing systems: Â
- Low Latency: The ability to process data with minimal delay, ensuring that organizations can act on insights without hesitation.
- Continuous Input: Handling a constant stream of data from various sources, which is vital for maintaining operational agility.
- Immediate Output: Providing results or actions in real-time, facilitating prompt decision-making.
- Types of real-time processing: Â
- Stream Processing: Analyzing data in motion, such as social media feeds or sensor data, which can inform marketing strategies and operational adjustments.
- Batch Processing: Although not real-time, it can be used in conjunction with real-time systems for historical analysis, providing context for immediate decisions.
- Benefits of real-time processing systems: Â
- Enhanced Decision Making: Organizations can make informed decisions quickly, reducing the risk of missed opportunities.
- Improved Customer Experience: Real-time data allows for personalized services and immediate responses, fostering customer loyalty.
- Operational Efficiency: Streamlined processes lead to cost savings and resource optimization, contributing to overall business performance.
Real-time processing systems are increasingly vital in sectors like finance, telecommunications, and e-commerce, where timely data can lead to competitive advantages.
3. Data Sources and Integration
Data sources and integration are critical components of any data-driven strategy. Organizations rely on various data sources to gather insights and make informed decisions. Integrating these data sources effectively is essential for comprehensive analysis, enabling businesses to leverage their data for maximum impact.
- Types of data sources: Â
- Structured Data: Organized data, such as databases and spreadsheets, that can be easily analyzed, providing a solid foundation for decision-making.
- Unstructured Data: Data that lacks a predefined format, including text, images, and videos, which can offer valuable insights when analyzed correctly.
- Semi-structured Data: Data that does not fit neatly into tables but contains tags or markers, such as JSON or XML files, allowing for flexible data integration.
- Data integration methods: Â
- ETL (Extract, Transform, Load): A traditional method for integrating data from multiple sources into a single repository, ensuring a comprehensive view of organizational data.
- Data Warehousing: Centralized storage that allows for efficient querying and analysis of integrated data, facilitating better insights and reporting.
- API Integration: Using application programming interfaces to connect different systems and share data in real-time, enhancing operational efficiency.
- Challenges in data integration: Â
- Data Quality: Ensuring accuracy and consistency across different sources, which is critical for reliable analysis.
- Data Silos: Isolated data that can hinder comprehensive analysis, limiting the potential for insights.
- Scalability: The ability to handle increasing volumes of data from diverse sources, ensuring that organizations can grow without compromising performance.
Effective data sources and integration strategies enable organizations to harness the full potential of their data, leading to better insights and improved decision-making, ultimately driving business success. Rapid Innovation is committed to helping clients navigate these complexities, ensuring they achieve their business goals efficiently and effectively.
Refer to the image for a visual representation of prediction algorithms and their components:
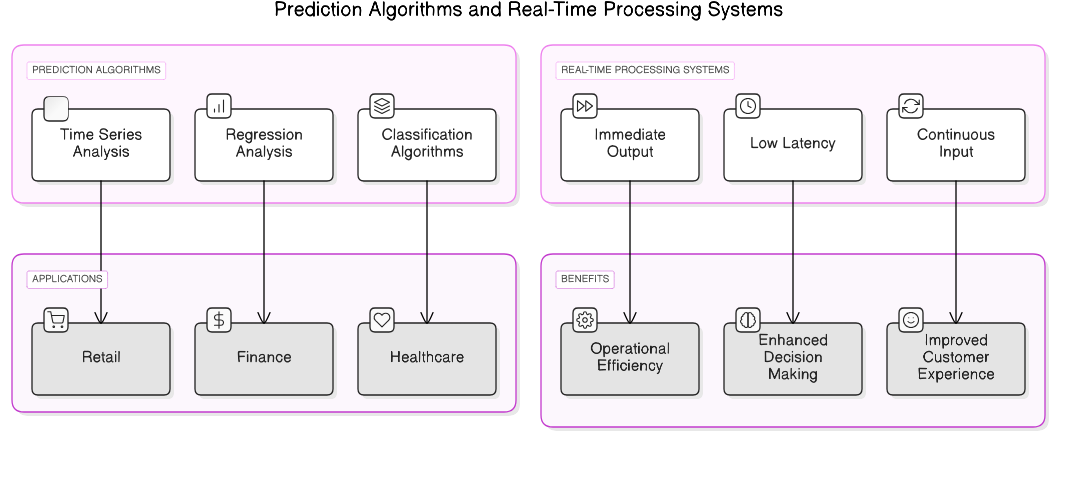
3.1. Property Historical Data
Understanding property historical data is crucial for making informed real estate decisions. This data provides insights into past property values, sales trends, and market performance over time.
- Historical property data includes: Â
- Previous sale prices of properties in a specific area.
- Time on the market for various properties.
- Changes in property values over different periods.
- Analyzing historical data helps in: Â
- Identifying patterns in property appreciation or depreciation.
- Understanding seasonal trends in real estate sales.
- Evaluating the impact of economic events on property values.
- Sources for historical property data include: Â
- Local property tax records.
- Real estate websites that track sales history.
- County assessor's offices that maintain property records.
At Rapid Innovation, we leverage advanced AI algorithms to analyze historical property data, enabling our clients to make data-driven decisions that enhance their investment strategies and maximize ROI. This analysis is essential for understanding housing market predictions and residential real estate market predictions. For more information on our services, visit our AI Real Estate Solutions.
3.2. Market Trends
Market trends refer to the general direction in which the real estate market is moving. These trends can indicate whether it is a buyer's or seller's market and can significantly influence investment decisions.
- Key market trends to monitor include: Â
- Changes in property prices: Are they rising or falling?
- Inventory levels: Is there a surplus or shortage of homes for sale?
- Buyer demand: Are more people looking to purchase homes?
- Understanding market trends can help investors: Â
- Time their purchases or sales effectively.
- Identify emerging neighborhoods with growth potential.
- Adjust their investment strategies based on market conditions.
- Tools for tracking market trends include: Â
- Real estate market reports from local agencies.
- Online platforms that provide market analytics.
- News articles and publications focused on real estate.
Rapid Innovation employs machine learning models to predict market trends, allowing our clients to stay ahead of the curve and make timely investment decisions that yield higher returns. This includes insights into real estate market forecast, real estate market trends, and trends in the real estate industry.
3.3. Economic Indicators
Economic indicators are statistics that provide insight into the overall health of the economy and can significantly impact the real estate market.
- Important economic indicators to consider include: Â
- Gross Domestic Product (GDP): A growing GDP often correlates with a strong real estate market.
- Unemployment rates: Lower unemployment typically leads to higher consumer confidence and increased home buying.
- Interest rates: Lower interest rates can make borrowing cheaper, encouraging more people to buy homes.
- Monitoring these indicators helps investors: Â
- Anticipate market shifts based on economic conditions.
- Make data-driven decisions regarding property investments.
- Understand the broader economic context that affects real estate.
- Resources for tracking economic indicators include: Â
- Government economic reports and statistics.
- Financial news websites that analyze economic trends.
- Economic research organizations that publish regular updates.
By integrating AI-driven analytics with economic indicators, Rapid Innovation empowers clients to make informed investment choices that align with market dynamics, ultimately driving greater ROI. This approach also considers real estate predictions 2025 and house price trends to ensure comprehensive market understanding.
3.4. Location Analytics
Location analytics refers to the process of analyzing spatial data to gain insights into various business operations and customer behaviors. This technique leverages geographic information systems (GIS) and data visualization tools to interpret data in a geographical context, making it essential for location analytics for businesses.
- Enhances decision-making: Businesses can make informed decisions by understanding where their customers are located and how they interact with products or services. Rapid Innovation employs advanced AI algorithms to analyze location data, enabling clients to optimize their strategies based on real-time insights.
- Improves marketing strategies: By analyzing location data, companies can tailor their marketing efforts to specific regions, increasing the effectiveness of campaigns. Our AI-driven solutions help clients identify high-potential markets, ensuring that marketing resources are allocated efficiently for maximum ROI.
- Optimizes resource allocation: Location analytics helps businesses identify the best locations for new stores or service centers, ensuring optimal resource distribution. Rapid Innovation's expertise in predictive analytics allows clients to forecast demand and strategically position their assets.
- Supports risk management: Understanding geographic risks, such as natural disasters or crime rates, allows businesses to develop contingency plans and mitigate potential losses. Our AI models can simulate various scenarios, helping clients prepare for unforeseen challenges.
- Facilitates competitive analysis: Companies can assess their competitors' locations and market presence, enabling them to strategize effectively. Rapid Innovation provides tools that analyze competitor data, giving clients a competitive edge in their market.
3.5. Demographic Data
Demographic data encompasses statistical information about the characteristics of a population. This data is crucial for businesses and organizations to understand their target audience and tailor their offerings accordingly.
- Age distribution: Knowing the age range of customers helps businesses design products and marketing strategies that appeal to specific age groups. Our AI solutions can segment customer demographics, allowing for targeted outreach.
- Gender breakdown: Understanding the gender composition of a target market can influence product development and advertising approaches. Rapid Innovation assists clients in analyzing gender-specific trends to enhance product relevance.
- Income levels: Analyzing income data allows businesses to price their products appropriately and identify potential market segments. Our AI tools provide insights into income distribution, helping clients optimize pricing strategies.
- Education levels: This information can guide companies in creating content and messaging that resonates with their audience's educational background. Rapid Innovation's analytics can tailor communication strategies based on educational demographics.
- Ethnic diversity: Recognizing the ethnic composition of a market can help businesses develop culturally relevant products and marketing campaigns. Our AI-driven insights enable clients to create inclusive strategies that resonate with diverse audiences.
3.6. Environmental Factors
Environmental factors refer to the external conditions that can impact a business's operations and decision-making processes. These factors can be natural, social, or economic and play a significant role in shaping business strategies.
- Climate conditions: Businesses must consider local climate when planning operations, as weather patterns can affect supply chains and customer behavior. Rapid Innovation leverages AI to analyze climate data, helping clients adapt their operations accordingly.
- Regulatory environment: Understanding local laws and regulations is essential for compliance and can influence business practices and strategies. Our consulting services ensure that clients remain compliant while optimizing their operations.
- Economic conditions: Economic indicators, such as unemployment rates and consumer spending, provide insights into market health and potential growth opportunities. Rapid Innovation's AI models can predict economic trends, allowing clients to make proactive decisions.
- Social trends: Keeping an eye on social movements and cultural shifts can help businesses adapt their strategies to align with changing consumer values. Our analytics tools track social trends, enabling clients to stay ahead of the curve.
- Technological advancements: Staying updated on technological trends can provide businesses with opportunities for innovation and improved efficiency. Rapid Innovation helps clients integrate cutting-edge technologies to enhance their operational capabilities.
4. Key Features and Capabilities
In the realm of real estate and property management, understanding the key features and capabilities of various tools and technologies, including smart home realty and proptech, is essential. These features not only enhance decision-making but also streamline processes, making them invaluable for investors, agents, and property managers alike.
4.1. Automated Valuation Models

‍
Automated Valuation Models (AVMs) are sophisticated algorithms that provide property valuations based on various data inputs. They leverage technology to deliver quick and accurate property assessments, which can be crucial for buyers, sellers, and investors in the context of artificial intelligence in real estate.
- Data-Driven Insights: AVMs utilize vast amounts of data, including recent sales, property characteristics, and market trends, to generate valuations. This data-driven approach ensures that the valuations are reflective of current market conditions.
- Speed and Efficiency: Traditional property appraisals can take days or even weeks. In contrast, AVMs can produce valuations in a matter of minutes, allowing stakeholders to make timely decisions.
- Cost-Effectiveness: By reducing the need for physical appraisals, AVMs can significantly lower costs associated with property valuation. This is particularly beneficial for large portfolios or frequent transactions in the realm of real estate technology.
- Consistency and Objectivity: AVMs eliminate human bias from the valuation process. They provide a consistent methodology for assessing property values, which can enhance trust among buyers and sellers.
- Integration with Other Tools: Many AVMs can be integrated with other real estate tools and platforms, allowing for seamless data sharing and enhanced functionality. This integration can improve overall workflow efficiency, especially when combined with real estate tech.
4.2. Trend Analysis
Trend analysis is a critical capability that helps stakeholders understand market dynamics and make informed decisions based on historical and current data. It involves examining patterns over time to predict future movements in the real estate market, including the impact of artificial intelligence for real estate.
- Market Insights: By analyzing trends, stakeholders can gain insights into property values, rental rates, and demand fluctuations. This information is vital for making strategic investment decisions.
- Identifying Opportunities: Trend analysis can help identify emerging markets or neighborhoods that are poised for growth. Investors can capitalize on these opportunities before they become mainstream, particularly in the context of real estate and technology.
- Risk Management: Understanding market trends allows stakeholders to assess potential risks. For instance, if a trend indicates a decline in property values, investors can adjust their strategies accordingly to mitigate losses.
- Performance Tracking: Trend analysis enables property managers and investors to track the performance of their assets over time. This can inform decisions about renovations, pricing strategies, and marketing efforts.
- Data Visualization: Many trend analysis tools offer data visualization features, making it easier to interpret complex data sets. Graphs and charts can help stakeholders quickly grasp market conditions and trends, including those influenced by virtual reality estate technologies.
In conclusion, both Automated Valuation Models and trend analysis are essential features in the real estate sector. They provide valuable insights, enhance efficiency, and support informed decision-making, making them indispensable tools for anyone involved in property management or investment. At Rapid Innovation, we harness these advanced technologies, including artificial intelligence and virtual reality estate agents, to empower our clients, ensuring they achieve greater ROI through informed strategies and streamlined operations. For more insights on the influence of AI in real estate market dynamics.
4.3. Risk Assessment
Risk assessment is a critical process that helps organizations identify, evaluate, and prioritize risks that could potentially impact their operations and objectives. This systematic approach allows businesses to develop strategies for risk assessment and mitigation strategies to manage these risks effectively.
- Identify Risks: Â
- Analyze internal and external factors that could pose threats.
- Consider financial, operational, strategic, and compliance risks, utilizing hazard identification strategies.
- Evaluate Risks: Â
- Assess the likelihood of each risk occurring.
- Determine the potential impact on the organization through risk analysis strategies.
- Prioritize Risks: Â
- Rank risks based on their severity and likelihood.
- Focus on high-priority risks that require immediate attention, employing business strategy risk assessment techniques.
- Develop Mitigation Strategies: Â
- Create action plans to minimize or eliminate risks, referencing risk assessment strategy examples.
- Implement controls and monitoring systems to track risk exposure, including risk analysis and mitigation strategies.
- Continuous Monitoring: Â
- Regularly review and update risk assessments.
- Adapt strategies as new risks emerge or existing risks evolve, ensuring the evaluation of risk management strategies is ongoing.
Effective risk assessment not only protects an organization but also enhances decision-making and strategic planning. By understanding potential risks, businesses can allocate resources more efficiently and maintain a competitive edge. At Rapid Innovation, we leverage advanced AI algorithms to automate risk identification and evaluation, ensuring that our clients can respond swiftly to emerging threats and optimize their risk management processes, particularly during new product launch risk analysis. For more information on how we can assist with this, check out our AI business automation solutions and learn about predictive analytics in finance.
4.4. Scenario Planning
Scenario planning is a strategic method used by organizations to envision and prepare for various future possibilities. This approach helps businesses anticipate changes in the market, technology, and other external factors that could impact their operations.
- Define Key Drivers: Â
- Identify the critical factors that could influence future scenarios.
- Consider economic, social, technological, and environmental trends.
- Develop Scenarios: Â
- Create a range of plausible future scenarios based on the identified drivers.
- Ensure scenarios are diverse and cover both optimistic and pessimistic outcomes.
- Analyze Implications: Â
- Evaluate how each scenario could affect the organization.
- Consider potential challenges and opportunities presented by each scenario.
- Develop Action Plans: Â
- Formulate strategies to address each scenario.
- Prepare contingency plans to respond to unexpected changes.
- Engage Stakeholders: Â
- Involve key stakeholders in the scenario planning process.
- Foster collaboration and gather diverse perspectives to enrich the analysis.
Scenario planning enables organizations to be proactive rather than reactive. By preparing for multiple futures, businesses can enhance their resilience and adaptability in an ever-changing environment. Rapid Innovation employs AI-driven analytics to simulate various scenarios, allowing our clients to visualize potential outcomes and make informed strategic decisions.
4.5. Comparative Market Analysis
Comparative market analysis (CMA) is a method used to evaluate a company's position in the market relative to its competitors. This analysis provides valuable insights that can inform strategic decisions and improve market positioning.
- Identify Competitors: Â
- Determine direct and indirect competitors in the market.
- Analyze their strengths, weaknesses, market share, and strategies.
- Gather Data: Â
- Collect relevant data on competitors, including pricing, product offerings, and customer reviews.
- Utilize market research reports and industry publications for comprehensive insights.
- Analyze Market Trends: Â
- Examine current market trends and consumer behavior.
- Identify emerging opportunities and potential threats in the market.
- Compare Performance Metrics: Â
- Evaluate key performance indicators (KPIs) such as sales growth, profitability, and customer satisfaction.
- Benchmark against competitors to identify areas for improvement.
- Develop Strategic Recommendations: Â
- Use insights from the analysis to inform marketing, product development, and pricing strategies.
- Adjust business strategies to capitalize on competitive advantages and address weaknesses.
Comparative market analysis is essential for businesses looking to enhance their competitive edge. By understanding the market landscape, organizations can make informed decisions that drive growth and success. Rapid Innovation utilizes AI tools to conduct in-depth market analysis, enabling our clients to identify trends and optimize their strategies for maximum ROI.
5. Property Analysis Parameters
When evaluating a property for investment or purchase, several key parameters come into play. Understanding these parameters can help investors make informed decisions and maximize their returns. Two critical aspects of property analysis are physical characteristics and location factors.
5.1. Physical Characteristics

‍
Physical characteristics refer to the tangible attributes of a property that can influence its value and usability. These characteristics can significantly impact the desirability and marketability of a property. Key physical characteristics include:
- Size and Layout: The total square footage and the layout of the property are crucial. A well-designed layout can enhance functionality, while an awkward layout may deter potential buyers or renters.
- Condition of the Property: The age and condition of the property play a vital role. Properties that require extensive repairs or renovations may not be as appealing, affecting their market value. Regular maintenance can help preserve property value.
- Building Materials: The quality of materials used in construction can affect durability and maintenance costs. Properties built with high-quality materials often have a longer lifespan and lower upkeep costs.
- Amenities: Features such as swimming pools, garages, and landscaping can add value. Properties with desirable amenities often attract higher offers and can command premium rents.
- Zoning Regulations: Understanding local zoning laws is essential. Zoning can dictate what can be built on the property and how it can be used, impacting its potential value and investment opportunities.
- Environmental Factors: Consideration of environmental aspects, such as flood zones or proximity to hazardous materials, is crucial. Properties in environmentally sensitive areas may face additional risks and insurance costs.
5.2. Location Factors
Location is often cited as the most critical factor in real estate. The right location can enhance property value and ensure a steady demand. Various location factors should be analyzed when assessing a property, including:
- Proximity to Amenities: Properties near schools, shopping centers, parks, and public transportation tend to be more desirable. Accessibility to essential services can significantly influence property values.
- Neighborhood Quality: The overall quality of the neighborhood, including safety, cleanliness, and community engagement, can impact property desirability. Areas with low crime rates and strong community ties often see higher property values.
- Economic Indicators: Local economic conditions, such as employment rates and income levels, can affect property demand. Areas with growing job markets typically experience increased interest in real estate.
- Future Development Plans: Researching upcoming developments in the area can provide insights into future property value. New infrastructure, commercial projects, or residential developments can enhance the attractiveness of a location.
- School Districts: Properties located in highly-rated school districts often command higher prices. Families prioritize access to quality education, making these areas more competitive in the real estate market.
- Market Trends: Understanding current market trends in the area can help predict future property values. Analyzing historical data and market forecasts can provide valuable insights for potential investors.
By carefully considering both physical characteristics and location factors, investors can make more informed decisions and identify properties with the highest potential for appreciation and rental income. At Rapid Innovation, we leverage advanced AI analytics to provide clients with data-driven insights into property investment analysis, real estate investment analysis, rental property analysis, and commercial real estate investment analysis, enabling them to optimize their investment strategies and achieve greater ROI. Additionally, our tools include real estate investment analysis software and rental property analysis calculators to assist in cash flow analysis real estate and investment property analysis.
5.3. Market Conditions
Understanding market conditions is crucial for anyone involved in real estate, whether buying, selling, or investing. Market conditions refer to the current state of the real estate market, influenced by various factors such as supply and demand, economic indicators, and interest rates.
- Supply and Demand: A high demand for properties with low inventory typically leads to increased prices. Conversely, an oversupply of homes can drive prices down. Rapid Innovation can leverage AI algorithms to analyze real-time data on supply and demand, helping clients make informed decisions that maximize their investment potential. This is where a comparative market analysis real estate (CMA) can be particularly useful.
- Economic Indicators: Factors like employment rates, GDP growth, and consumer confidence can significantly impact market conditions. A strong economy usually correlates with a robust real estate market. By utilizing predictive analytics, Rapid Innovation can assist clients in forecasting economic trends, enabling them to align their strategies with market movements for greater ROI. A real estate market analysis can provide insights into these economic indicators.
- Interest Rates: Lower interest rates make borrowing cheaper, encouraging more buyers to enter the market. This can lead to increased demand and higher property prices. Our AI-driven tools can help clients assess the impact of interest rate fluctuations on their investments, allowing for timely adjustments to their financial strategies. Understanding how these rates affect a comparative market assessment is essential for making informed decisions.
- Seasonal Trends: Real estate markets often experience seasonal fluctuations. For instance, spring and summer are typically more active seasons for home sales. Rapid Innovation can provide insights into seasonal patterns through data analysis, helping clients optimize their buying and selling strategies throughout the year. A cma for houses can help identify the best times to enter the market.
- Local Market Variations: Real estate is highly localized. Conditions can vary significantly from one city or neighborhood to another, influenced by local economic conditions and demographics. Our AI solutions can analyze local market data, enabling clients to identify lucrative opportunities tailored to specific regions. A realtor cma can provide localized insights that are crucial for understanding these variations.
5.4. Property History
The history of a property can provide valuable insights into its current value and potential future performance. Understanding a property's past can help buyers make informed decisions and identify any potential red flags.
- Previous Ownership: Knowing how many times a property has changed hands can indicate its desirability. Frequent sales may suggest underlying issues. Rapid Innovation can develop AI models that assess ownership history, providing clients with a clearer picture of property desirability. This is often a key component of a comparative market analysis.
- Renovations and Repairs: A history of renovations can enhance a property's value, but poorly executed repairs may raise concerns. It's essential to review permits and contractor details. Our AI tools can analyze renovation data to help clients understand the impact of past improvements on current property value, which is critical in a real estate market analysis.
- Market Performance: Analyzing how the property has appreciated or depreciated over time can provide insights into market trends and the property's long-term value. Rapid Innovation can utilize machine learning algorithms to predict future market performance based on historical data, aiding clients in making strategic investment decisions. This analysis is often part of a comprehensive cma for property.
- Legal Issues: Investigating any past legal disputes, liens, or zoning issues can help avoid future complications. A clear title is crucial for a smooth transaction. Our AI solutions can streamline the legal research process, ensuring clients are well-informed before proceeding with a purchase.
- Inspection Reports: Previous inspection reports can reveal recurring problems, such as structural issues or pest infestations, which may affect the property's current condition. Rapid Innovation can automate the analysis of inspection reports, providing clients with actionable insights to address potential issues proactively.
5.5. Neighborhood Analysis
A thorough neighborhood analysis is essential for understanding the broader context of a property. The neighborhood can significantly influence property values, quality of life, and investment potential.
- Demographics: Analyzing the population's age, income levels, and family structures can provide insights into the neighborhood's character and appeal. Rapid Innovation can employ AI to analyze demographic data, helping clients identify neighborhoods that align with their target market.
- Amenities: Proximity to schools, parks, shopping centers, and public transportation can enhance a neighborhood's desirability. Access to amenities often drives demand and property values. Our AI-driven tools can map out amenities in relation to properties, allowing clients to assess the attractiveness of various locations.
- Crime Rates: Safety is a top priority for many homebuyers. Researching local crime statistics can help assess the neighborhood's safety and overall appeal. Rapid Innovation can integrate crime data analysis into our solutions, providing clients with a comprehensive view of neighborhood safety.
- Future Development: Investigating planned developments or zoning changes can provide insights into the neighborhood's future growth and potential property value increases. Our AI models can predict the impact of future developments on property values, enabling clients to make informed investment choices.
- Community Engagement: Active community organizations and events can indicate a vibrant neighborhood. Engaged residents often contribute to a higher quality of life and property values. Rapid Innovation can analyze community engagement metrics, helping clients identify neighborhoods with strong social dynamics that enhance property value.
6. Prediction Models and Algorithms
Prediction models and algorithms are essential tools in various fields, including finance, healthcare, marketing, and more. They help organizations make informed decisions by forecasting future outcomes based on historical data. Understanding the different types of prediction models and algorithms can significantly enhance the accuracy of predictions and improve overall decision-making processes.
6.1. Statistical Analysis Methods

‍
Statistical analysis methods are foundational techniques used to analyze data and make predictions. These methods rely on mathematical theories and formulas to interpret data patterns and relationships. Key statistical analysis methods include:
- Regression Analysis: This method examines the relationship between dependent and independent variables. It helps in predicting the value of a dependent variable based on the known values of independent variables. Common types include linear regression and logistic regression, such as logistic regression for prediction.
- Time Series Analysis: This technique analyzes data points collected or recorded at specific time intervals. It is particularly useful for forecasting future values based on previously observed values. Time series analysis is widely used in stock market predictions and economic forecasting, including neural network time series forecasting and xgboost for time series forecasting.
- Hypothesis Testing: This method involves making assumptions about a population parameter and testing these assumptions using sample data. It helps determine if there is enough evidence to support a specific claim or hypothesis.
- Descriptive Statistics: This includes summarizing and describing the main features of a dataset. Measures such as mean, median, mode, and standard deviation provide insights into the data's central tendency and variability.
Statistical analysis methods are crucial for understanding data distributions and relationships, making them indispensable in prediction modeling. They provide a solid foundation for more complex algorithms and machine learning techniques.
6.2. Machine Learning Techniques
Machine learning techniques have revolutionized the field of prediction modeling by enabling computers to learn from data and improve their performance over time without being explicitly programmed. These techniques can handle large datasets and uncover complex patterns that traditional statistical methods may miss. Key machine learning techniques include:
- Supervised Learning: In this approach, the model is trained on labeled data, meaning that the input data is paired with the correct output. Common algorithms include decision trees, support vector machines, and neural networks, such as xgboost predict and random forest prediction. Supervised learning is widely used for classification and regression tasks.
- Unsupervised Learning: Unlike supervised learning, unsupervised learning deals with unlabeled data. The model tries to identify patterns and groupings within the data. Techniques such as clustering (e.g., K-means) and dimensionality reduction (e.g., PCA) fall under this category. Unsupervised learning is useful for exploratory data analysis and anomaly detection, including link prediction based on graph neural networks.
- Reinforcement Learning: This technique involves training models to make decisions by rewarding them for correct actions and penalizing them for incorrect ones. It is commonly used in robotics, gaming, and autonomous systems. Reinforcement learning helps in developing strategies that maximize cumulative rewards over time.
- Ensemble Methods: These methods combine multiple models to improve prediction accuracy. Techniques like bagging (e.g., Random Forest) and boosting (e.g., AdaBoost) leverage the strengths of various models to produce a more robust prediction, including random forest predict and xgboost forecasting.
- Deep Learning: A subset of machine learning, deep learning uses neural networks with many layers to analyze complex data patterns. It is particularly effective in image and speech recognition tasks. Deep learning has gained popularity due to its ability to process unstructured data and achieve high accuracy in predictions, such as recurrent neural network for time series forecasting and recurrent neural network time series forecasting.
At Rapid Innovation, we leverage these advanced prediction models and algorithms to help our clients achieve greater ROI. By implementing tailored statistical analysis and machine learning techniques, we enable organizations to make data-driven decisions that enhance operational efficiency and drive business growth. Our expertise in these areas ensures that clients can harness the power of predictive analytics to stay ahead in their respective industries. Additionally, our adaptive AI development services can further enhance your predictive capabilities. For more information on specific techniques like outlier detectio.
6.3. Neural Networks
Neural networks are a subset of machine learning models inspired by the human brain's structure and function. They consist of interconnected nodes or "neurons" that process data in layers. Neural networks are particularly effective for complex tasks such as image recognition, natural language processing, and more, including convolutional neural networks application and neural network imaging.
- Structure: Neural networks typically have an input layer, one or more hidden layers, and an output layer. Each layer consists of multiple neurons that perform calculations based on input data.
- Activation Functions: Neurons use activation functions to determine whether to pass their output to the next layer. Common activation functions include ReLU (Rectified Linear Unit), sigmoid, and tanh.
- Training Process: Neural networks learn through a process called backpropagation, where the model adjusts its weights based on the error of its predictions. This iterative process continues until the model achieves an acceptable level of accuracy.
- Applications: Neural networks are widely used in various fields, including image and speech recognition, autonomous vehicles, financial forecasting, and medical diagnosis. At Rapid Innovation, we leverage neural networks to help clients enhance their product offerings, improve customer experiences, and drive operational efficiencies, ultimately leading to greater ROI. This includes applications such as convolutional neural networks for image classification and neural network for image recognition.
- Deep Learning: A specialized form of neural networks, deep learning involves networks with many hidden layers, allowing for the modeling of highly complex patterns in large datasets. Our expertise in deep learning enables us to tackle intricate problems, providing clients with innovative solutions that can significantly boost their competitive edge. This includes the use of graph neural network applications and mobilenets efficient convolutional neural networks for mobile vision applications. For advanced projects, we also offer transformer model development to enhance performance and capabilities. Additionally, you can explore various types of artificial neural networks for a deeper understanding of their applications and structures.
6.4. Time Series Analysis
Time series analysis is a statistical technique used to analyze time-ordered data points. It helps in understanding underlying patterns, trends, and seasonal variations over time. This method is crucial for forecasting future values based on historical data.
- Components of Time Series: Time series data typically consists of: Â
- Trend: The long-term movement in the data.
- Seasonality: Regular patterns that repeat over a specific period.
- Cyclic Patterns: Fluctuations that occur at irregular intervals, often influenced by economic or environmental factors.
- Irregular Variations: Random noise or anomalies that do not follow a pattern.
- Methods of Analysis: Common techniques for time series analysis include: Â
- Moving Averages: Smoothing data to identify trends.
- Exponential Smoothing: Giving more weight to recent observations.
- ARIMA (AutoRegressive Integrated Moving Average): A popular statistical method for forecasting.
- Applications: Time series analysis is widely used in various domains, such as stock market analysis, economic forecasting, weather prediction, and resource consumption forecasting. Rapid Innovation employs time series analysis to help clients make data-driven decisions, optimize inventory management, and enhance financial planning, leading to improved profitability.
- Challenges: Time series data can be affected by outliers, missing values, and non-stationarity, which can complicate analysis and forecasting.
6.5. Regression Models
Regression models are statistical techniques used to understand the relationship between a dependent variable and one or more independent variables. They are essential for predicting outcomes and identifying trends in data.
- Types of Regression: Â
- Linear Regression: Assumes a linear relationship between the dependent and independent variables. It is the simplest form of regression.
- Multiple Regression: Involves two or more independent variables to predict the dependent variable.
- Logistic Regression: Used for binary outcomes, predicting the probability of a certain class or event.
- Polynomial Regression: Models the relationship as an nth degree polynomial, allowing for more complex relationships.
- Key Concepts: Â
- Coefficients: Indicate the strength and direction of the relationship between variables.
- R-squared: A statistical measure that represents the proportion of variance for the dependent variable explained by the independent variables.
- P-values: Help determine the significance of each predictor in the model.
- Applications: Regression models are widely used in various fields, including economics for demand forecasting, healthcare for predicting patient outcomes, marketing for analyzing consumer behavior, and real estate for property value estimation. At Rapid Innovation, we utilize regression models to provide clients with actionable insights that drive strategic initiatives and enhance overall performance.
- Limitations: Regression models can be sensitive to outliers and may not capture complex relationships unless appropriately specified. Additionally, multicollinearity among independent variables can lead to unreliable estimates. Our team at Rapid Innovation is adept at addressing these challenges, ensuring that our clients receive robust and reliable analytical solutions.
7. User Interface and Experience
User interface (UI) and user experience (UX) are critical components in the design of any software or application. A well-designed UI enhances usability, while a positive UX ensures that users find the application enjoyable and efficient to use. Focusing on these elements can significantly impact user satisfaction and retention.
7.1. Dashboard Design
The dashboard serves as the central hub for users, providing them with essential information at a glance. Effective dashboard design is crucial for facilitating quick decision-making and enhancing user engagement. Key considerations for dashboard design include:
- Clarity and Simplicity: A clean and straightforward layout helps users navigate the dashboard easily. Avoid clutter by limiting the number of elements displayed at once.
- Visual Hierarchy: Use size, color, and placement to emphasize the most important information. This helps users quickly identify key metrics and insights.
- Customization Options: Allow users to personalize their dashboards according to their preferences, which can include rearranging widgets, selecting data sources, or choosing color themes.
- Responsive Design: Ensure that the dashboard is accessible on various devices, including desktops, tablets, and smartphones. A responsive design enhances usability across different screen sizes.
- Real-Time Data: Incorporate real-time data updates to keep users informed of the latest information. This is particularly important for applications that rely on timely data, such as financial or operational dashboards.
- Intuitive Navigation: Use clear labels and icons to guide users through the dashboard. A well-structured navigation system reduces the learning curve and improves overall user experience.
7.2. Reporting Tools
Reporting tools are essential for analyzing data and generating insights. A well-designed reporting tool can empower users to make informed decisions based on accurate and relevant information. Important features of reporting tools include:
- User-Friendly Interface: The reporting tool should have an intuitive interface that allows users to create reports without extensive training. Drag-and-drop functionality can simplify the report-building process.
- Customizable Reports: Users should have the ability to customize reports to meet their specific needs, including selecting data fields, applying filters, and choosing visualization types.
- Data Visualization: Incorporate various visualization options, such as charts, graphs, and tables, to present data in an easily digestible format. Effective data visualization can enhance understanding and retention of information.
- Export Options: Provide users with the ability to export reports in multiple formats, such as PDF, Excel, or CSV. This flexibility allows users to share insights with stakeholders easily.
- Scheduled Reporting: Implement features that allow users to schedule reports to be generated and sent automatically. This saves time and ensures that stakeholders receive timely updates.
- Integration Capabilities: Ensure that the reporting tool can integrate with other systems and data sources, allowing users to pull in data from various platforms and enhancing the comprehensiveness of reports.
By focusing on user interface and experience, particularly in dashboard design and reporting tools, organizations can create applications that not only meet user needs but also foster engagement and satisfaction. At Rapid Innovation, we leverage our expertise in AI to enhance user interface design and user experience design, ensuring that our clients achieve greater ROI through improved user engagement and streamlined decision-making processes. Our approach includes user interface ux strategies, ui and ux design principles, and collaboration with ux design agencies to deliver exceptional results. We also emphasize the importance of a user-friendly interface in our ui design and reporting tools, ensuring that our clients benefit from effective ux design ui design practices.
7.3. Visualization Features
Visualization features are essential in data analysis and reporting, allowing users to interpret complex data sets easily. Effective data visualization transforms raw data into graphical representations, making it more accessible and understandable. Common types of visualizations include bar charts, line graphs, pie charts, heat maps, and scatter plots. Each type serves a different purpose and can highlight various aspects of the data. Many platforms offer interactive dashboards that allow users to manipulate data views in real-time, enhancing user engagement and facilitating deeper insights. Additionally, visualization features can help tell a story with data; by combining visuals with narrative elements, users can convey insights more effectively to stakeholders. Users often have the ability to customize visual elements, such as colors, labels, and scales, to align with branding or personal preferences. Furthermore, data visualization tools can often integrate with other software, such as Excel or CRM systems, to pull in data seamlessly and create comprehensive reports.
- Types of Visualizations: Common types include bar charts, line graphs, pie charts, heat maps, and scatter plots. Each type serves a different purpose and can highlight various aspects of the data, including data visualization examples and data visualization charts.
- Interactive Dashboards: Many platforms offer interactive dashboards that allow users to manipulate data views in real-time. This interactivity enhances user engagement and facilitates deeper insights, often seen in business intelligence dashboards.
- Data Storytelling: Visualization features can help tell a story with data. By combining visuals with narrative elements, users can convey insights more effectively to stakeholders, a practice often referred to as data storytelling.
- Customization Options: Users often have the ability to customize visual elements, such as colors, labels, and scales, to align with branding or personal preferences, which is a key feature of data visualization software.
- Integration with Other Tools: Visualization tools can often integrate with other software, such as Excel or CRM systems, to pull in data seamlessly and create comprehensive reports, enhancing the functionality of database visualization tools. For example, an AI agent for customer energy consumption can utilize these visualization features to provide insights.
7.4. Custom Alerts
Custom alerts are a powerful feature that allows users to stay informed about specific changes or events in their data. These alerts can be tailored to meet individual needs, ensuring that users receive timely notifications. Users can set thresholds for various metrics, such as sales figures or website traffic, and when these thresholds are crossed, alerts are triggered. Custom alerts can be sent through various channels, including email, SMS, or in-app notifications, ensuring that users receive updates in their preferred format. With custom alerts, users can monitor data in real-time, allowing for quick responses to critical changes or anomalies. Common use cases for custom alerts include monitoring stock levels, tracking website performance, and overseeing financial metrics. Many platforms provide an intuitive interface for setting up and managing alerts, making it easy for users to customize their notification preferences.
- Threshold Settings: Users can set thresholds for various metrics, such as sales figures or website traffic. When these thresholds are crossed, alerts are triggered.
- Multiple Notification Channels: Custom alerts can be sent through various channels, including email, SMS, or in-app notifications, ensuring that users receive updates in their preferred format.
- Real-Time Monitoring: With custom alerts, users can monitor data in real-time, allowing for quick responses to critical changes or anomalies.
- Use Cases: Common use cases for custom alerts include monitoring stock levels, tracking website performance, and overseeing financial metrics, which can be enhanced by data visualization tools.
- User-Friendly Interface: Many platforms provide an intuitive interface for setting up and managing alerts, making it easy for users to customize their notification preferences.
7.5. Mobile Integration
Mobile integration is increasingly important in today’s fast-paced world, allowing users to access data and insights on the go. This feature enhances flexibility and ensures that critical information is always at users' fingertips. Many data platforms offer dedicated mobile applications that provide full access to visualization tools, reports, and alerts, ensuring users can work from anywhere. Mobile integration often includes responsive design, which optimizes the user experience on various devices, including smartphones and tablets. Users can receive push notifications for custom alerts directly on their mobile devices, ensuring they never miss important updates. Some platforms allow users to download reports or data for offline access, which is particularly useful in areas with limited connectivity. Additionally, mobile integration often includes collaboration tools, enabling users to share insights and reports with team members in real-time, regardless of location.
- Mobile Apps: Many data platforms offer dedicated mobile applications that provide full access to visualization tools, reports, and alerts, ensuring users can work from anywhere, including access to data visualization software.
- Responsive Design: Mobile integration often includes responsive design, which optimizes the user experience on various devices, including smartphones and tablets.
- Push Notifications: Users can receive push notifications for custom alerts directly on their mobile devices, ensuring they never miss important updates.
- Offline Access: Some platforms allow users to download reports or data for offline access, which is particularly useful in areas with limited connectivity.
- Collaboration Features: Mobile integration often includes collaboration tools, enabling users to share insights and reports with team members in real-time, regardless of location, enhancing the overall data visualization experience.
8. Implementation Process
The implementation process is a critical phase in any project, particularly in technology and software development. It involves translating plans into action, ensuring that all components work together seamlessly. A well-structured implementation process can significantly enhance the chances of project success. Key steps in this process include defining clear objectives and goals, establishing a timeline for each phase of implementation, assigning roles and responsibilities to team members, and monitoring progress regularly to ensure adherence to the plan.
8.1. System Requirements
Understanding system requirements is essential for a successful implementation. This involves identifying the necessary hardware, software, and network configurations needed to support the new system. The key components include:
- Hardware Requirements: Determine the physical components needed, such as servers, workstations, and networking equipment. Consider factors like processing power, memory, and storage capacity.
- Software Requirements: Identify the software applications and tools required for the system to function effectively. This includes operating systems, databases, and any specialized software.
- Network Requirements: Assess the network infrastructure needed to support the system. This includes bandwidth, security protocols, and connectivity options.
- User Requirements: Gather input from end-users to understand their needs and expectations. This can help in tailoring the system to enhance user experience.
- Compliance and Security: Ensure that the system meets industry standards and regulations, including data protection laws and cybersecurity measures.
8.2. Data Setup
Data setup is a crucial step in the implementation process, as it involves preparing and organizing data for the new system. Proper data setup ensures that the system operates efficiently and effectively. The main aspects of data setup include:
- Data Migration: Plan for the transfer of existing data to the new system. This may involve cleaning, transforming, and validating data to ensure accuracy and consistency.
- Data Structure: Define the structure of the data within the new system. This includes creating databases, tables, and relationships that reflect the organization’s needs.
- Data Entry: Establish protocols for data entry to maintain data integrity. This may involve training staff on how to input data correctly and consistently.
- Data Backup: Implement a robust data backup strategy to prevent data loss. Regular backups can safeguard against hardware failures or data corruption.
- Testing Data: Conduct thorough testing of the data setup to identify any issues before going live. This includes checking for errors, inconsistencies, and performance bottlenecks.
- User Training: Provide training for users on how to access and utilize the data effectively. This ensures that they can leverage the system to its full potential.
At Rapid Innovation, we understand that a meticulous implementation process, including ERP implementation and project management implementation plan, is vital for achieving greater ROI. By ensuring that system and data requirements are thoroughly addressed, we help our clients minimize disruptions and maximize the efficiency of their operations. Our expertise in AI and technology allows us to tailor solutions that align with your business goals, ultimately driving success and profitability. We also focus on planning and implementation strategies to ensure a smooth transition during the implementation phases. For more insights on the importance of data quality in AI implementations.
8.3. Model Training
Model training is a critical phase in the machine learning lifecycle where algorithms learn from data. This process involves feeding a model a dataset, allowing it to identify patterns and make predictions.
- Data Preparation: Before training, data must be cleaned and preprocessed. This includes handling missing values, normalizing data, and encoding categorical variables. At Rapid Innovation, we emphasize the importance of high-quality data preparation to ensure that our clients' models are built on a solid foundation, leading to more accurate predictions and better business outcomes.
- Choosing the Right Algorithm: Selecting an appropriate algorithm is essential. Common algorithms include linear regression, decision trees, and neural networks, each suited for different types of problems. Our team at Rapid Innovation works closely with clients to understand their specific needs and choose the most effective algorithms that align with their business goals.
- Hyperparameter Tuning: Adjusting hyperparameters can significantly impact model performance. Techniques like grid search or random search help find the optimal settings. By leveraging our expertise in hyperparameter tuning, we help clients maximize the performance of their models, ultimately leading to greater ROI.
- Training Process: The model is trained using a training dataset, where it learns to minimize the error between predicted and actual outcomes. This is often done using techniques like gradient descent. Rapid Innovation employs advanced training techniques, including training in machine learning and training neural networks, to ensure that our clients' models are not only accurate but also efficient in their learning processes.
- Monitoring Performance: During training, it’s crucial to monitor metrics such as accuracy, precision, and recall to ensure the model is learning effectively. Our continuous monitoring approach allows us to make real-time adjustments, ensuring that the models we develop for our clients are consistently performing at their best. This includes monitoring during machine learning model training and training deep learning models.
8.4. Testing and Validation
Testing and validation are essential steps to ensure that the model performs well on unseen data. This phase helps assess the model's generalization capabilities.
- Validation Set: A portion of the dataset is set aside as a validation set. This data is not used during training and helps evaluate the model's performance. At Rapid Innovation, we ensure that our clients have a robust validation strategy in place to accurately assess model performance.
- Cross-Validation: Techniques like k-fold cross-validation are employed to ensure that the model is robust. This involves splitting the dataset into k subsets and training the model k times, each time using a different subset for validation. Our expertise in cross-validation techniques helps clients build models that are resilient and reliable.
- Performance Metrics: Various metrics are used to evaluate model performance, including: Â
- Accuracy: The ratio of correctly predicted instances to total instances.
- F1 Score: A balance between precision and recall, useful for imbalanced datasets.
- ROC-AUC: Measures the model's ability to distinguish between classes. By providing comprehensive performance evaluations, Rapid Innovation ensures that our clients can make informed decisions based on their model's effectiveness.
- Error Analysis: Analyzing errors helps identify patterns in mispredictions, guiding further improvements in the model. Our team conducts thorough error analysis to refine models, ensuring that our clients achieve optimal results. This includes training and testing in machine learning and training and testing data in machine learning.
8.5. Deployment Strategy
Deployment strategy refers to the process of integrating a trained model into a production environment where it can make predictions on new data.
- Deployment Environment: Choose the right environment for deployment, which could be cloud-based, on-premises, or edge devices, depending on the application requirements. Rapid Innovation assists clients in selecting the most suitable deployment environment to meet their operational needs.
- Model Serving: Implementing a model serving solution is crucial. This can be done using REST APIs or gRPC, allowing applications to interact with the model seamlessly. Our expertise in model serving ensures that clients can easily integrate AI capabilities into their existing systems.
- Monitoring and Maintenance: Post-deployment, continuous monitoring is necessary to ensure the model performs as expected. This includes tracking performance metrics and user feedback. Rapid Innovation provides ongoing support to ensure that our clients' models remain effective and relevant over time.
- Version Control: Maintaining different versions of the model is essential for managing updates and ensuring that the best-performing model is in use. We implement robust version control practices to help clients manage their models efficiently.
- Scalability: The deployment strategy should account for scalability, allowing the model to handle increased loads as usage grows. Techniques like load balancing and containerization can facilitate this. Rapid Innovation designs scalable solutions that grow with our clients' needs, ensuring long-term success.
By focusing on these aspects of model training, including training machine learning models, online machine learning, and transfer learning deep learning, testing and validation, and deployment strategy, organizations can ensure that their machine learning models are effective, reliable, and ready for real-world applications. At Rapid Innovation, we are committed to helping our clients achieve their business goals efficiently and effectively through our AI development and consulting solutions.
9. Accuracy and Validation
Accuracy and validation are critical components in any data-driven process, ensuring that the results produced are reliable and trustworthy. In various fields, from scientific research to business analytics, the integrity of data can significantly impact decision-making and outcomes. Accuracy refers to how close a measured value is to the actual (true) value, while validation is the process of confirming that a system, process, or product meets the required specifications and fulfills its intended purpose. To achieve high data accuracy and effective validation, organizations must implement robust quality control measures and conduct thorough error analysis.
9.1. Quality Control Measures
Quality control measures are systematic processes designed to ensure that the quality of data meets predefined standards. These measures help in identifying and rectifying errors before they affect the final output.
- Standard Operating Procedures (SOPs): Establishing clear SOPs helps maintain consistency in data collection and processing. This includes guidelines on how to handle data, perform analyses, and report results.
- Regular Audits: Conducting regular audits of data processes can help identify discrepancies and areas for improvement. Audits can be internal or external, depending on the organization's needs.
- Training and Development: Continuous training for staff involved in data handling ensures they are up-to-date with the latest techniques and technologies, reducing the likelihood of human error.
- Automated Data Validation Tools: Utilizing software tools that automatically check for data integrity can significantly enhance data accuracy. These tools can flag anomalies, missing values, or inconsistencies in real-time.
- Feedback Mechanisms: Implementing feedback loops allows for continuous improvement. Stakeholders can provide insights on data quality, leading to better practices and methodologies.
- Benchmarking: Comparing data against industry standards or historical data can help identify outliers and ensure that the data is within acceptable limits.
9.2. Error Analysis
Error analysis is the process of identifying, categorizing, and understanding errors that occur during data collection, processing, or analysis. This process is essential for improving accuracy and ensuring that future data handling is more reliable.
- Types of Errors: Errors can be classified into several categories, including: Â
- Systematic Errors: These are consistent and repeatable errors that occur due to a flaw in the measurement system.
- Random Errors: These errors occur unpredictably and can be caused by various factors, including environmental conditions or human mistakes.
- Blunders: These are gross errors that result from carelessness or misinterpretation of data.
- Root Cause Analysis: Identifying the root cause of errors is crucial for effective resolution. Techniques such as the "5 Whys" or fishbone diagrams can help trace back to the source of the problem.
- Statistical Methods: Employing statistical techniques to analyze errors can provide insights into their frequency and impact. This can include calculating error rates, confidence intervals, and conducting hypothesis testing.
- Documentation: Keeping detailed records of errors and the steps taken to resolve them is vital. This documentation can serve as a reference for future projects and help in training new staff.
- Continuous Improvement: Error analysis should lead to actionable insights that drive improvements in data processes. Organizations should regularly review their error analysis findings to refine their quality control measures.
- Collaboration: Engaging cross-functional teams in error analysis can provide diverse perspectives and solutions. Collaboration fosters a culture of accountability and shared responsibility for data quality.
By focusing on data accuracy and validation through quality control measures and error analysis, organizations can enhance their data integrity, leading to more informed decision-making and better outcomes. At Rapid Innovation, we leverage these principles to help our clients optimize their data processes, ensuring that they achieve greater ROI through reliable and actionable insights.
9.3. Confidence Scoring
Confidence scoring is a critical component in various fields, particularly in machine learning and data analysis. It quantifies the certainty of a model's predictions, helping users understand the reliability of the results.
- Confidence scores are typically expressed as probabilities ranging from 0 to 1.
- A higher score indicates greater confidence in the prediction, while a lower score suggests uncertainty.
- These scores can guide decision-making processes, especially in high-stakes environments like healthcare or finance. For instance, in a healthcare application, a model predicting patient outcomes with high confidence can lead to timely interventions, ultimately improving patient care and reducing costs.
- Confidence scoring can also help in identifying outliers or anomalies in data, allowing for more focused analysis. This is particularly useful in fraud detection, where identifying unusual patterns can lead to significant cost savings.
- It is essential to calibrate confidence scores to ensure they accurately reflect the true likelihood of outcomes.
In practice, confidence scoring can be implemented using various algorithms, such as logistic regression or support vector machines. These methods can provide insights into how well a model is performing and where improvements may be needed, enabling Rapid Innovation to enhance client solutions and drive greater ROI through generative AI development and AI distillation.
9.4. Validation Methods
Validation methods are essential for assessing the performance and reliability of predictive models. They help ensure that a model generalizes well to unseen data, which is crucial for its practical application.
- Cross-validation is a widely used technique that involves partitioning the dataset into subsets. The model is trained on some subsets and validated on others, providing a robust estimate of its performance. This method can help Rapid Innovation ensure that the models we develop for clients are both accurate and reliable.
- K-fold cross-validation is a specific type where the data is divided into 'k' subsets, and the model is trained and validated 'k' times, each time using a different subset for validation. This approach allows for a comprehensive evaluation of model performance.
- Holdout validation involves splitting the dataset into a training set and a test set. The model is trained on the training set and evaluated on the test set, providing a straightforward assessment of its performance. This method is often used in initial model assessments.
- Bootstrap validation is another method that involves repeatedly sampling from the dataset with replacement to create multiple training and validation sets. This technique can provide insights into the stability and reliability of the model.
- Each validation method has its strengths and weaknesses, and the choice often depends on the size of the dataset and the specific requirements of the analysis.
Using appropriate validation methods is crucial for avoiding overfitting, ensuring that the model performs well not just on the training data but also on new, unseen data. Rapid Innovation employs these methods to deliver robust AI solutions that meet client expectations.
9.5. Performance Metrics
Performance metrics are vital for evaluating the effectiveness of predictive models. They provide quantitative measures that help in comparing different models and understanding their strengths and weaknesses.
- Accuracy is one of the most straightforward metrics, representing the proportion of correct predictions made by the model.
- Precision measures the number of true positive predictions divided by the total number of positive predictions, indicating how many of the predicted positives were actually correct. This metric is crucial in applications where false positives can lead to significant costs.
- Recall, or sensitivity, assesses the model's ability to identify all relevant instances, calculated as the number of true positives divided by the total actual positives. High recall is essential in scenarios like disease detection, where missing a positive case can have serious consequences.
- F1 score is the harmonic mean of precision and recall, providing a balance between the two metrics, especially useful in cases of imbalanced datasets. This metric helps Rapid Innovation ensure that our models are not only accurate but also reliable in real-world applications.
- Area Under the Receiver Operating Characteristic Curve (AUC-ROC) evaluates the model's ability to distinguish between classes, with a higher score indicating better performance. This metric is particularly useful in binary classification problems.
Choosing the right performance metrics is essential for accurately assessing a model's capabilities and ensuring it meets the specific needs of the application. At Rapid Innovation, we leverage these metrics to optimize our AI solutions, ultimately driving greater ROI for our clients.
10. Market Applications
Market applications refer to the various sectors where real estate is utilized, each with its unique characteristics and demands. Understanding these applications is crucial for investors, developers, and stakeholders in the real estate industry.
10.1. Residential Properties
Residential properties encompass a wide range of housing options designed for individuals and families. This sector is vital for providing shelter and is often the first step for many in the real estate market.
- Types of Residential Properties: Â
- Single-family homes: Standalone houses typically occupied by one family.
- Multi-family units: Buildings that house multiple families, such as duplexes, triplexes, and apartment complexes.
- Condominiums: Individually owned units within a larger building or complex, often with shared amenities.
- Townhouses: Attached homes that share walls with neighboring units, offering a balance between single-family homes and apartments.
- Market Trends: Â
- Urbanization: Increasing migration to urban areas has led to a surge in demand for residential properties in cities.
- Affordability: Rising home prices have made affordable housing a critical issue, prompting government initiatives and private sector solutions.
- Sustainability: Eco-friendly homes and energy-efficient designs are gaining popularity among buyers, reflecting a shift towards sustainable living.
- Investment Opportunities: Â
- Rental properties: Investors can purchase residential properties to rent out, generating a steady income stream.
- Fix-and-flip: Buying distressed properties, renovating them, and selling for a profit is a common strategy in the residential market.
- Real Estate Investment Trusts (REITs): Investing in residential REITs that focus on residential properties allows individuals to gain exposure to the market without direct ownership. This includes options like residential REIT ETFs and the best residential REITs available.
10.2. Commercial Real Estate
Commercial real estate (CRE) includes properties used for business purposes. This sector is essential for economic growth and job creation, as it provides spaces for various industries.
- Types of Commercial Real Estate: Â
- Office buildings: Spaces designed for businesses, ranging from small offices to large corporate headquarters.
- Retail properties: Locations where goods and services are sold, including shopping centers, malls, and standalone stores.
- Industrial properties: Facilities used for manufacturing, warehousing, and distribution, often located near transportation hubs.
- Hospitality: Hotels, motels, and resorts that cater to travelers and tourists.
- Market Trends: Â
- E-commerce growth: The rise of online shopping has transformed retail spaces, leading to increased demand for warehouses and distribution centers.
- Flexible workspaces: The shift towards remote work has spurred interest in co-working spaces and flexible office solutions.
- Mixed-use developments: Combining residential, commercial, and recreational spaces in one project is becoming increasingly popular, promoting community living.
- Investment Opportunities: Â
- Long-term leases: Commercial properties often come with longer lease terms, providing stable cash flow for investors.
- Value-add investments: Purchasing underperforming properties and improving them can lead to significant returns.
- Diversification: Investing in different types of commercial properties can help mitigate risks and enhance portfolio performance.
Leveraging AI for Enhanced Market Insights
At Rapid Innovation, we understand the complexities of the real estate market and the importance of data-driven decision-making. By utilizing advanced AI algorithms, we can help clients analyze market trends, predict property values, and identify lucrative investment opportunities. For instance, our AI-powered analytics tools can assess urbanization patterns and affordability metrics, enabling investors to make informed decisions that align with current market demands.
By integrating AI solutions into your real estate strategy, you can achieve greater ROI through optimized investment choices and enhanced operational efficiencies. Let us partner with you to navigate the evolving landscape of real estate and unlock new avenues for growth, including insights into residential income property for sale and residential investment property for sale. Additionally, we can provide a list of apartment REITs and a comprehensive list of residential REITs, including the largest apartment REITs and information on equity residential REITs and equity residential properties. For more information on how we can assist you, check out our AI in Real Estate.
10.3. Investment Analysis
Investment analysis is a critical process that involves evaluating various investment opportunities to determine their potential for profitability and risk. This analysis helps investors make informed decisions about where to allocate their resources, ultimately leading to greater returns on investment.
- Types of Investment Analysis: Â
- Fundamental Analysis: Focuses on evaluating a company's financial health, including its earnings, revenue, and overall market position. Rapid Innovation leverages AI algorithms to analyze vast datasets, providing clients with deeper insights into market trends and company performance, including stock fundamental analysis.
- Technical Analysis: Involves analyzing statistical trends from trading activity, such as price movement and volume, to predict future price movements. Our AI-driven tools can automate this analysis, allowing for quicker and more accurate predictions, similar to stock analysis technical methods.
- Quantitative Analysis: Uses mathematical and statistical models to assess investment opportunities, often relying on historical data. Rapid Innovation employs machine learning techniques to enhance the accuracy of these models, helping clients make data-driven investment decisions, including stock trade analyzer tools.
- Key Metrics in Investment Analysis: Â
- Return on Investment (ROI): Measures the gain or loss generated relative to the investment cost. Our AI solutions can optimize investment strategies to maximize ROI.
- Net Present Value (NPV): Calculates the difference between the present value of cash inflows and outflows over a period. Rapid Innovation's predictive analytics can improve NPV calculations by incorporating real-time data.
- Internal Rate of Return (IRR): Represents the discount rate that makes the NPV of all cash flows from a particular project equal to zero. Our advanced modeling techniques can help clients identify projects with the highest IRR potential.
- Tools and Techniques: Â
- Financial Ratios: Such as price-to-earnings (P/E) ratio, debt-to-equity ratio, and current ratio, which provide insights into a company's performance. Rapid Innovation can automate the calculation and analysis of these ratios, saving time and reducing errors.
- Discounted Cash Flow (DCF) Analysis: Estimates the value of an investment based on its expected future cash flows, adjusted for time value. Our AI tools can enhance DCF analysis by integrating market conditions and trends.
- Scenario Analysis: Evaluates the potential outcomes of different scenarios to understand the risks and rewards associated with an investment. Rapid Innovation's simulation models allow clients to visualize various scenarios and make informed decisions, including using an AI agent personalized investment portfolio advisor.
10.4. Portfolio Management
Portfolio management is the art and science of making decisions about investment mix and policy, aligning investments to objectives, and balancing risk against performance. Effective portfolio management is essential for achieving long-term financial goals, and Rapid Innovation can enhance this process through AI-driven insights.
- Types of Portfolio Management: Â
- Active Management: Involves ongoing buying and selling of assets to outperform the market index. Our AI tools can analyze market conditions in real-time, enabling clients to make timely investment decisions.
- Passive Management: Focuses on long-term investment strategies, typically through index funds, with minimal trading. Rapid Innovation can help clients optimize their passive strategies through data analysis.
- Discretionary Management: The portfolio manager makes investment decisions on behalf of the client, based on their risk tolerance and investment goals. Our AI solutions can provide portfolio managers with actionable insights to enhance decision-making.
- Key Strategies in Portfolio Management: Â
- Asset Allocation: Diversifying investments across various asset classes (stocks, bonds, real estate) to reduce risk. Rapid Innovation's AI algorithms can recommend optimal asset allocation based on market conditions, including insights from stock market analysis.
- Rebalancing: Periodically adjusting the portfolio to maintain the desired level of asset allocation. Our tools can automate this process, ensuring portfolios remain aligned with client goals.
- Risk Management: Identifying, assessing, and prioritizing risks to minimize potential losses. Rapid Innovation employs advanced analytics to enhance risk assessment and management strategies.
- Performance Measurement: Â
- Benchmarking: Comparing portfolio performance against a relevant market index to gauge success. Our AI tools can provide real-time benchmarking insights.
- Sharpe Ratio: Measures risk-adjusted return, helping investors understand the return per unit of risk taken. Rapid Innovation can automate the calculation of this ratio, providing clients with quick insights.
- Alpha: Indicates how much an investment outperforms or underperforms a benchmark index. Our analytics can help clients identify sources of alpha in their portfolios, utilizing stock ratings free resources.
10.5. Development Projects
Development projects encompass a wide range of initiatives aimed at creating new assets or improving existing ones. These projects can be in various sectors, including real estate, infrastructure, and technology. Rapid Innovation can assist clients in optimizing their development projects through AI-driven insights and tools.
- Phases of Development Projects: Â
- Planning: Involves feasibility studies, market research, and project design to outline objectives and strategies. Our AI solutions can streamline the planning phase by providing data-driven insights.
- Execution: The actual implementation of the project, including construction, resource allocation, and timeline management. Rapid Innovation's project management tools can enhance execution efficiency.
- Monitoring and Evaluation: Ongoing assessment of project progress against goals, ensuring that it stays on track and within budget. Our AI analytics can provide real-time monitoring and evaluation capabilities.
- Key Considerations in Development Projects: Â
- Budgeting: Establishing a clear financial plan that outlines costs, funding sources, and expected returns. Rapid Innovation can assist in creating accurate budgeting models using AI.
- Stakeholder Engagement: Involving all relevant parties, including investors, community members, and regulatory bodies, to ensure project success. Our tools can facilitate effective communication and engagement strategies.
- Risk Assessment: Identifying potential risks, such as regulatory changes or market fluctuations, and developing mitigation strategies. Rapid Innovation employs advanced analytics to enhance risk assessment processes.
- Tools and Techniques: Â
- Project Management Software: Tools like Microsoft Project or Trello help in planning, tracking, and managing project tasks. Rapid Innovation can integrate AI capabilities into these tools for enhanced functionality.
- SWOT Analysis: Evaluates the project's strengths, weaknesses, opportunities, and threats to inform decision-making. Our AI tools can automate SWOT analysis, providing deeper insights.
- Gantt Charts: Visual representations of project timelines, helping teams understand task dependencies and deadlines. Rapid Innovation can enhance Gantt chart capabilities with predictive analytics for better project management, similar to stock charts free resources.
11. Integration Capabilities
Integration capabilities are essential for any software solution, particularly in industries like real estate, where seamless data flow can significantly enhance operational efficiency. Effective integration allows different systems to communicate, share data, and work together harmoniously. This is crucial for real estate professionals who rely on various tools to manage their operations.
11.1. CRM Systems
Customer Relationship Management (CRM) systems are vital for managing interactions with clients and prospects. Integration with CRM systems can provide numerous benefits:
- Centralized Data Management: Integrating with a CRM allows real estate agents to centralize client information, making it easier to track leads, manage follow-ups, and maintain relationships.
- Enhanced Communication: Automated updates from the CRM can keep agents informed about client interactions, ensuring timely responses and personalized service.
- Improved Marketing Efforts: Integration enables targeted marketing campaigns based on client data, helping agents reach the right audience with the right message.
- Streamlined Workflows: By connecting CRM systems with other tools, such as email marketing platforms or transaction management software, agents can streamline their workflows and reduce manual data entry.
- Analytics and Reporting: Integration allows for better data analysis, enabling agents to track performance metrics and make informed decisions based on real-time data.
Popular CRM systems in the real estate industry include Salesforce, HubSpot, and Zoho CRM. HubSpot real estate CRM is particularly noted for its user-friendly interface and robust features. These platforms often offer integration capabilities with various real estate tools, enhancing their functionality and providing a comprehensive solution for agents.
11.2. MLS Integration
Multiple Listing Service (MLS) integration is another critical aspect of real estate software. MLS databases are essential for real estate professionals, as they provide access to property listings and market data. The benefits of MLS integration include:
- Real-Time Data Access: MLS integration allows agents to access up-to-date property listings, ensuring they have the latest information to share with clients.
- Enhanced Property Search: By integrating MLS data into their websites or applications, agents can offer clients advanced search features, making it easier for them to find properties that meet their criteria.
- Automated Listing Updates: Integration can automate the process of updating property listings, reducing the risk of errors and ensuring that clients always see the most current information.
- Increased Visibility: Agents can showcase their listings on various platforms through MLS integration, increasing exposure and attracting more potential buyers.
- Compliance and Accuracy: MLS integration helps ensure that listings comply with local regulations and standards, maintaining the integrity of the data shared with clients.
At Rapid Innovation, we understand the importance of these integration capabilities. Our AI-driven solutions can enhance the functionality of CRM and MLS systems, enabling real estate professionals to achieve greater efficiency and effectiveness in their operations. By leveraging our expertise, clients can streamline their processes, improve client engagement, and ultimately drive higher ROI in their business endeavors. Many real estate software solutions offer MLS integration, including real estate CRM with MLS integration and real estate CRM IDX integration, allowing agents to leverage the power of MLS data while enhancing their service offerings. This integration is crucial for staying competitive in the fast-paced real estate market. Additionally, solutions like dotloop CRM integration further enhance the capabilities of real estate professionals, ensuring they have the tools necessary to succeed. If you're looking to enhance your integration capabilities, consider partnering with our team of property matching process to drive your success.
11.3. Property Management Software
Property management software is essential for landlords and property managers to streamline operations and enhance efficiency. This type of software automates various tasks, making it easier to manage properties effectively. Key features include:
- Tenant Management: Track tenant information, lease agreements, and communication history.
- Maintenance Tracking: Log maintenance requests and schedule repairs, ensuring timely responses.
- Rent Collection: Facilitate online rent payments, reducing late payments and improving cash flow.
- Accounting Features: Manage financial records, including income and expenses, with integrated accounting tools.
- Reporting Capabilities: Generate reports on occupancy rates, rental income, and maintenance costs for better decision-making.
At Rapid Innovation, we leverage AI technologies to enhance property management software, enabling predictive maintenance and automated tenant communication. By integrating machine learning algorithms, we help clients reduce operational costs and improve tenant satisfaction, ultimately leading to a greater return on investment (ROI). For more information on how AI can be utilized in lease management, check out our article on AI agents for lease management.
Popular property management software options include Buildium, AppFolio, and Rentec Direct. Other notable options include AppFolio company and Zillow Rent Manager, which offer various features tailored to different property management needs, from single-family homes to large apartment complexes. Additionally, property management software for small landlords is increasingly popular, with options like best free property management software for small landlords and best property management software for landlords.
11.4. Financial Tools
Financial tools are crucial for property managers and landlords to maintain a healthy financial status. These tools assist in budgeting, forecasting, and analyzing financial performance. Important tools include:
- Budgeting Software: Create and manage budgets to control expenses and maximize profits.
- Expense Tracking: Monitor all property-related expenses, ensuring accurate financial records.
- Cash Flow Analysis: Evaluate cash inflows and outflows to maintain liquidity and plan for future investments.
- Investment Analysis: Assess potential property investments using metrics like ROI and cap rate to make informed decisions.
- Tax Preparation Tools: Simplify tax filing by organizing financial data and generating necessary reports.
Using financial tools can lead to better financial management, allowing property owners to focus on growth and profitability. At Rapid Innovation, we implement AI-driven financial analytics that provide real-time insights, enabling property owners to make informed decisions that enhance profitability. Tools like QuickBooks and FreshBooks are popular choices for managing property finances.
11.5. Reporting Systems
Reporting systems are vital for property management as they provide insights into various aspects of property performance. These systems help in making data-driven decisions and improving overall management strategies. Key components include:
- Performance Metrics: Track key performance indicators (KPIs) such as occupancy rates, tenant turnover, and rental income.
- Customizable Reports: Generate tailored reports to meet specific management needs, allowing for deeper analysis.
- Data Visualization: Use graphs and charts to present data clearly, making it easier to understand trends and patterns.
- Compliance Reporting: Ensure adherence to local regulations by generating reports that meet legal requirements.
- Historical Data Analysis: Review past performance to identify trends and make informed predictions for future performance.
Effective reporting systems can significantly enhance decision-making processes, leading to improved property management outcomes. Rapid Innovation employs advanced data analytics and visualization tools to create comprehensive reporting systems that empower property managers to optimize their strategies. Tools like Tableau and Microsoft Power BI are commonly used for creating these systems, but our tailored solutions can provide even greater insights and ROI. Additionally, facility maintenance work order software and maintenance PM software are important components of a comprehensive property management system.
12. Regulatory Compliance
Regulatory compliance refers to the adherence to laws, regulations, guidelines, and specifications relevant to an organization’s business processes. It is crucial for maintaining operational integrity and avoiding legal penalties. Companies must navigate a complex landscape of regulations that vary by industry and region, including compliance regulations by industry and specific regulatory requirements.
- Ensures legal operation of the business Â
- Protects the organization from fines and penalties Â
- Enhances reputation and trust with customers Â
- Promotes ethical business practices Â
- Facilitates smoother operations and risk management Â
12.1. Industry Standards
Industry standards are established norms and guidelines that dictate best practices within a specific sector. Compliance with these standards is essential for ensuring quality, safety, and efficiency in products and services.
These standards provide a framework for quality assurance, enhance customer confidence in products and services, facilitate international trade by standardizing practices, encourage innovation and continuous improvement, and help organizations stay competitive in the market.
Organizations often align with standards set by recognized bodies such as ISO (International Organization for Standardization) or ANSI (American National Standards Institute). For example, ISO 9001 focuses on quality management systems, while ISO 27001 addresses information security management. Adhering to these standards can lead to certifications that enhance credibility and marketability.
At Rapid Innovation, we assist clients in navigating these industry standards by integrating AI-driven solutions that streamline compliance processes, ensuring that your organization not only meets but exceeds these benchmarks, ultimately leading to greater operational efficiency and ROI.
12.2. Data Privacy
Data privacy is a critical aspect of regulatory compliance, especially in an era where data breaches and cyber threats are prevalent. Organizations must protect personal and sensitive information from unauthorized access and misuse.
Compliance with data privacy laws ensures adherence to regulations like GDPR (General Data Protection Regulation) and CCPA (California Consumer Privacy Act), builds customer trust by safeguarding personal information, reduces the risk of data breaches and associated costs, promotes responsible data handling practices, and enhances organizational reputation and customer loyalty.
Data privacy regulations require organizations to implement robust data protection measures, including encryption, access controls, and regular audits. Companies must also provide transparency regarding data collection, usage, and sharing practices. Failure to comply with data privacy laws can result in significant fines and damage to reputation.
Rapid Innovation offers consulting services that leverage AI technologies to enhance data privacy measures, ensuring compliance with evolving regulations while minimizing risks. Our solutions not only protect sensitive information but also foster customer loyalty and trust, contributing to a stronger bottom line.
In conclusion, regulatory compliance, including legal compliance and compliance with the regulations, industry standards, and data privacy are interconnected elements that play a vital role in the success and sustainability of any organization. By prioritizing these areas, businesses can not only avoid legal repercussions but also foster a culture of trust and integrity. At Rapid Innovation, we are committed to helping our clients achieve these goals efficiently and effectively, driving greater ROI through our tailored AI solutions and best practices for navigating legal challenges.
12.3. Legal Requirements
Understanding legal requirements is crucial for any organization, as it ensures compliance with laws and regulations that govern operations. Legal requirements can vary significantly based on industry, location, and the nature of the business. Organizations must be aware of local, state, and federal laws that apply to their operations. Compliance with labor laws, including minimum wage, overtime, and workplace safety regulations, is essential. Data protection laws, such as the General Data Protection Regulation (GDPR) in Europe or the California Consumer Privacy Act (CCPA) in the U.S., dictate how personal information should be handled. Intellectual property laws protect the rights of creators and inventors, making it important for businesses to understand copyright, trademark, and patent regulations. Environmental regulations may require businesses to adhere to specific standards to minimize their ecological impact. Failure to comply with legal requirements can result in significant penalties, including fines, lawsuits, and damage to reputation.
- Organizations must be aware of local, state, and federal laws that apply to their operations.
- Compliance with labor laws, including minimum wage, overtime, and workplace safety regulations, is essential.
- Data protection laws, such as the General Data Protection Regulation (GDPR) in Europe or the California Consumer Privacy Act (CCPA) in the U.S., dictate how personal information should be handled.
- Intellectual property laws protect the rights of creators and inventors, making it important for businesses to understand copyright, trademark, and patent regulations.
- Environmental regulations may require businesses to adhere to specific standards to minimize their ecological impact.
- Failure to comply with legal requirements can result in significant penalties, including fines, lawsuits, and damage to reputation.
In addition to these general legal requirements, organizations should consider whether WCAG (Web Content Accessibility Guidelines) is a legal requirement. Many jurisdictions are increasingly recognizing WCAG as a standard for accessibility, leading to questions such as "is WCAG a legal requirement?" and "is WCAG 2.1 a legal requirement?" Understanding the implications of WCAG 2.0 and its updates is essential for compliance. Organizations must also be aware of the legal compliance guidelines that pertain to their specific industry and location. For more insights on how AI agents can assist with legal applications, you can refer to this use cases framework.
12.4. Ethics Guidelines
Ethics guidelines serve as a framework for decision-making and behavior within an organization. They help establish a culture of integrity and accountability, guiding employees in their daily actions. Organizations should develop a clear code of ethics that outlines expected behaviors and values. Ethical guidelines should address issues such as conflicts of interest, discrimination, harassment, and bribery. Training programs can help employees understand and apply ethical principles in their work. Transparency in decision-making processes fosters trust among employees and stakeholders. Encouraging open communication allows employees to voice concerns about unethical behavior without fear of retaliation. Regularly reviewing and updating ethics guidelines ensures they remain relevant and effective in addressing new challenges.
- Organizations should develop a clear code of ethics that outlines expected behaviors and values.
- Ethical guidelines should address issues such as conflicts of interest, discrimination, harassment, and bribery.
- Training programs can help employees understand and apply ethical principles in their work.
- Transparency in decision-making processes fosters trust among employees and stakeholders.
- Encouraging open communication allows employees to voice concerns about unethical behavior without fear of retaliation.
- Regularly reviewing and updating ethics guidelines ensures they remain relevant and effective in addressing new challenges.
12.5. Documentation
Proper documentation is vital for maintaining organizational integrity and ensuring compliance with legal and ethical standards. It serves as a record of actions, decisions, and policies, providing a reference for future activities. Documentation should include policies, procedures, and guidelines that govern operations. Keeping accurate records of financial transactions is essential for accountability and compliance with tax regulations. Employee records, including contracts, performance evaluations, and training documentation, help manage human resources effectively. Incident reports and compliance audits should be documented to track issues and ensure corrective actions are taken. Digital documentation systems can enhance accessibility and security, making it easier to retrieve and manage information. Regular audits of documentation practices can identify gaps and improve overall organizational efficiency.
- Documentation should include policies, procedures, and guidelines that govern operations.
- Keeping accurate records of financial transactions is essential for accountability and compliance with tax regulations.
- Employee records, including contracts, performance evaluations, and training documentation, help manage human resources effectively.
- Incident reports and compliance audits should be documented to track issues and ensure corrective actions are taken.
- Digital documentation systems can enhance accessibility and security, making it easier to retrieve and manage information.
- Regular audits of documentation practices can identify gaps and improve overall organizational efficiency.
At Rapid Innovation, we understand that navigating legal requirements, ethics guidelines, and documentation practices can be complex. Our AI-driven solutions can streamline compliance processes, ensuring that your organization adheres to all necessary regulations while enhancing operational efficiency. By leveraging our expertise, clients can achieve greater ROI through reduced legal risks and improved organizational integrity.
13. Best Practices
In the realm of data science and machine learning, adhering to best practices is crucial for ensuring the effectiveness and reliability of models. This section delves into two key areas: Data Management and Model Maintenance.
13.1. Data Management
Effective data management is foundational to successful data science projects. It encompasses the processes of collecting, storing, organizing, and maintaining data throughout its lifecycle. Here are some best practices for data management:
- Data Quality Assurance: Ensure that the data collected is accurate, complete, and relevant. Regularly validate and clean data to eliminate errors and inconsistencies. This aligns with data quality management best practices.
- Data Governance: Establish clear policies and procedures for data usage, access, and security. This includes defining roles and responsibilities for data stewardship, which is a key aspect of data governance best practices.
- Data Documentation: Maintain comprehensive documentation of data sources, data dictionaries, and data lineage. This helps in understanding the context and origin of the data, supporting good data management practices.
- Version Control: Implement version control for datasets, similar to code repositories. This allows tracking changes over time and facilitates collaboration among team members, reflecting master data management best practices.
- Data Storage Solutions: Choose appropriate storage solutions based on data volume and access needs. Options include cloud storage, databases, and data lakes, which are essential for effective data management practices.
- Data Privacy and Compliance: Adhere to regulations such as GDPR and HIPAA. Implement measures to protect sensitive data and ensure compliance with legal requirements, which is a critical aspect of data handling best practices.
- Data Integration: Utilize tools and techniques for integrating data from various sources. This can enhance the richness of the dataset and provide a more comprehensive view, in line with customer data management best practices.
- Regular Backups: Schedule regular backups of data to prevent loss due to system failures or accidental deletions, a fundamental principle in data management best practices.
- Scalability: Design data management systems that can scale with growing data needs. This ensures that performance remains optimal as data volume increases, reflecting the importance of master data governance best practices.
13.2. Model Maintenance
Model maintenance is essential for ensuring that machine learning models continue to perform well over time. As data and environments change, models may require updates and adjustments. Here are some best practices for model maintenance:
- Continuous Monitoring: Regularly monitor model performance using key metrics. This helps in identifying any degradation in accuracy or relevance, which is crucial for maintaining data quality.
- Retraining Models: Schedule periodic retraining of models with new data. This is crucial for adapting to changes in underlying patterns and trends, aligning with best data management practices.
- Versioning Models: Keep track of different versions of models. This allows for easy rollback to previous versions if a new model underperforms, similar to version control in data management.
- Documentation of Changes: Document any changes made to the model, including updates to algorithms, parameters, or data sources. This aids in understanding the evolution of the model, reflecting the importance of data documentation.
- Feedback Loops: Implement feedback mechanisms to gather insights from end-users. This can inform necessary adjustments and improvements to the model, enhancing the overall data governance.
- A/B Testing: Use A/B testing to compare the performance of different model versions. This helps in making data-driven decisions about which model to deploy, a practice often seen in business intelligence project management best practices.
- Performance Benchmarks: Establish benchmarks for model performance. Regularly compare current performance against these benchmarks to assess effectiveness, ensuring alignment with data management principles and best practices.
- Collaboration: Foster collaboration between data scientists, engineers, and domain experts. This ensures that models are aligned with business objectives and user needs, which is essential for effective customer master data management.
- Decommissioning Models: Have a clear process for retiring outdated or underperforming models. This helps in maintaining a streamlined and effective model portfolio, reflecting the principles of master data best practices.
By implementing these best practices in data management and model maintenance, organizations can enhance the reliability and effectiveness of their data-driven initiatives. At Rapid Innovation, we specialize in guiding clients through these best practices, ensuring that their AI projects yield greater ROI and align with their business goals. For more information on how we can assist with your AI projects, check out our the potential of business AI engineering best practices.
13.3. User Training
User training is a critical component in the successful implementation of any system or software, particularly in the realm of AI solutions. It ensures that users are equipped with the necessary skills and knowledge to effectively utilize the advanced tools at their disposal. Comprehensive training programs should be developed to cover all aspects of the system, including AI functionalities and best practices, such as user training for AI. Training can be delivered through various methods, including:
- In-person workshops
- Online courses
- Video tutorials
- User manuals and documentation
Regular training sessions should be scheduled to accommodate new users and updates to the system, especially as AI technologies evolve. Feedback mechanisms should be established to assess the effectiveness of the training and make necessary adjustments. Ongoing support should be available to assist users as they navigate the system post-training.
Investing in user training not only enhances productivity but also reduces the likelihood of errors and increases user satisfaction, ultimately leading to a greater return on investment (ROI) for businesses leveraging AI solutions.
13.4. Quality Assurance
Quality assurance (QA) is essential for maintaining high standards in products and services, particularly in AI development where precision and reliability are paramount. It involves systematic processes to ensure that the final output meets the required specifications and quality benchmarks. QA processes should include:
- Regular testing and evaluation of products or services
- Implementation of standardized procedures to minimize variability
- Continuous monitoring and feedback loops to identify areas for improvement
Key components of a robust QA program include:
- Defining quality metrics and benchmarks
- Conducting audits and inspections
- Engaging in root cause analysis for any defects or issues
Collaboration between teams is vital to ensure that quality is maintained throughout the development lifecycle. Training for QA personnel is crucial to keep them updated on best practices and emerging trends in quality management, especially in the fast-paced AI landscape.
A strong QA framework not only enhances customer satisfaction but also builds trust and credibility in the brand, leading to increased client retention and business growth.
13.5. Security Protocols
Security protocols are essential for protecting sensitive data and ensuring the integrity of systems, particularly in AI applications where data privacy is critical. They establish guidelines and measures to safeguard information from unauthorized access and breaches. Key elements of effective security protocols include:
- Data encryption to protect information during transmission and storage
- User authentication mechanisms, such as multi-factor authentication
- Regular security audits to identify vulnerabilities
Organizations should implement a comprehensive security policy that outlines:
- Roles and responsibilities for data protection
- Procedures for reporting and responding to security incidents
- Training programs for employees on security best practices
Compliance with industry standards and regulations, such as GDPR or HIPAA, is crucial for maintaining legal and ethical standards. Continuous monitoring of systems and networks helps in detecting and responding to threats in real-time.
By prioritizing security protocols, organizations can mitigate risks and protect their assets, ensuring a safe environment for both users and data, which is essential for fostering trust in AI solutions.
14. Case Studies
Case studies provide valuable insights into real-world applications of strategies and practices within the real estate market. They illustrate successes and challenges faced by various stakeholders, offering lessons that can be applied to future endeavors.
14.1. Residential Market Success
The residential market has seen numerous success stories that highlight effective strategies in property development, marketing, and sales. These case studies often focus on innovative approaches that have led to increased property values and satisfied homeowners.
- Innovative Design: Many successful residential projects incorporate modern design elements that appeal to buyers. For instance, open floor plans and energy-efficient features have become increasingly popular, attracting a demographic that values sustainability and aesthetics. Rapid Innovation can assist developers in utilizing AI-driven design tools to optimize layouts and enhance energy efficiency, ultimately increasing property appeal and value.
- Community Engagement: Successful residential developments often engage with the community during the planning phase. This can include public forums or surveys to gather input from potential residents, ensuring that the project meets local needs and preferences. By leveraging AI analytics, Rapid Innovation can help clients analyze community feedback more effectively, leading to better-informed development decisions.
- Marketing Strategies: Effective marketing plays a crucial role in residential success. Utilizing digital marketing, social media campaigns, and virtual tours can significantly enhance visibility and attract potential buyers. For example, a case study in a suburban area showed that properties marketed through social media platforms sold 30% faster than those using traditional methods. Rapid Innovation can enhance these strategies through AI-driven targeting and personalized marketing campaigns, maximizing reach and engagement.
- Location Analysis: The importance of location cannot be overstated. Successful residential projects are often situated in areas with good schools, amenities, and transportation links. A case study in a metropolitan area revealed that homes located near public transport hubs saw a 15% increase in value over five years. Rapid Innovation can provide advanced location analysis tools that utilize AI to predict future property values based on emerging trends and demographic shifts.
- Customer-Centric Approach: Developers who prioritize customer satisfaction tend to see better results. This includes offering flexible financing options, personalized services, and post-sale support, which can lead to positive word-of-mouth and repeat business. Rapid Innovation can implement AI-driven customer relationship management systems that enhance client interactions and streamline service delivery.
14.2. Commercial Property Analysis
The commercial property sector presents unique challenges and opportunities. Case studies in this area often focus on market trends, investment strategies, and the impact of economic factors on property values.
- Market Trends: Understanding market trends is essential for success in commercial real estate. For instance, a case study analyzing the rise of remote work showed that demand for office space in urban centers decreased, while suburban office spaces gained popularity. This shift prompted investors to reevaluate their portfolios and consider alternative locations. Rapid Innovation can provide AI tools that analyze market data in real-time, helping clients make informed investment decisions.
- Investment Strategies: Successful commercial property investments often involve thorough market analysis and risk assessment. A notable case study highlighted a firm that diversified its portfolio by investing in mixed-use developments, which provided stable cash flow and reduced risk during economic downturns. Rapid Innovation can assist in developing predictive models that assess investment risks and returns, enhancing decision-making processes.
- Sustainability Practices: Increasingly, commercial properties are incorporating sustainable practices to attract tenants and investors. A case study of a green office building demonstrated that energy-efficient features not only reduced operating costs but also increased tenant retention rates by 20%. Rapid Innovation can support clients in implementing AI solutions that optimize energy management and sustainability practices, leading to cost savings and improved tenant satisfaction.
- Tenant Relations: Building strong relationships with tenants is crucial in the commercial sector. A case study showed that a property management company that implemented regular communication and feedback mechanisms saw a significant decrease in tenant turnover, leading to higher occupancy rates. Rapid Innovation can enhance tenant engagement through AI-driven communication platforms that facilitate feedback and improve service responsiveness.
- Economic Impact: The economic environment plays a significant role in commercial property performance. A case study examining the effects of a recession on retail spaces revealed that properties in high-traffic areas fared better than those in less desirable locations, emphasizing the importance of location and market positioning. Rapid Innovation can provide clients with AI analytics that forecast economic trends and their potential impact on property values, enabling proactive strategy adjustments.
These case studies, including those on real estate private equity case study and commercial real estate case studies, illustrate the dynamic nature of both the residential and commercial real estate markets. By analyzing successes and challenges, stakeholders can develop strategies that enhance their chances of success in future projects. Rapid Innovation stands ready to support clients in leveraging AI technologies to achieve greater ROI and operational efficiency in their real estate endeavors, as demonstrated in various real estate case study examples and AI Copilot Development case study. Additionally, for more insights on the application of AI in real estate, you can explore how AI is transforming the industry.
14.3. Investment Portfolio Optimization
Investment portfolio optimization is a crucial process for investors aiming to maximize returns while minimizing risk. This involves selecting the right mix of assets to achieve the desired financial goals, including optimal portfolio allocation and investment optimization.
- Diversification is key: By spreading investments across various asset classes, such as stocks, bonds, and real estate, investors can reduce the impact of poor performance in any single investment. This is particularly important in creating tax efficient portfolios.
- Risk assessment: Understanding the risk tolerance of an investor is essential. This includes evaluating factors like age, income, and investment goals, which are critical for constructing an optimal investment portfolio.
- Asset allocation: Determining the right percentage of each asset class in a portfolio is vital. This can be adjusted based on market conditions and personal circumstances, ensuring efficient portfolio management.
- Use of technology: Advanced algorithms and software can assist in optimizing portfolios by analyzing vast amounts of data to identify the best investment opportunities. At Rapid Innovation, we leverage AI-driven analytics to provide tailored financial portfolio optimization solutions that enhance decision-making and improve overall investment performance, including stock portfolio optimization. Additionally, we explore the use of AI agents for investment strategy to further enhance portfolio management.
- Regular rebalancing: Periodically reviewing and adjusting the portfolio ensures that it remains aligned with the investor's goals and risk tolerance, which is essential for maintaining an ideal portfolio of stocks.
14.4. Market Prediction Accuracy
Market prediction accuracy refers to the ability to forecast future market movements and trends. Accurate predictions can significantly enhance investment strategies and decision-making.
- Historical data analysis: Investors often rely on historical market data to identify patterns and trends that may repeat in the future.
- Technical analysis: This involves using charts and indicators to predict future price movements based on past performance.
- Fundamental analysis: Evaluating a company's financial health, industry position, and economic factors can provide insights into its future performance.
- Machine learning and AI: These technologies are increasingly used to improve prediction accuracy by analyzing large datasets and identifying complex patterns. Rapid Innovation employs state-of-the-art machine learning models to enhance market prediction capabilities, enabling clients to make informed investment decisions.
- Limitations: Despite advancements, predicting market movements remains challenging due to the influence of unforeseen events and market sentiment.
14.5. ROI Analysis
Return on Investment (ROI) analysis is a critical metric for evaluating the efficiency of an investment. It measures the gain or loss generated relative to the amount invested.
- Calculation: ROI is typically calculated using the formula:
(Net Profit / Cost of Investment) x 100
. This provides a percentage that indicates the profitability of an investment. - Importance of ROI: A higher ROI indicates a more profitable investment, making it easier for investors to compare different opportunities.
- Time factor: ROI should be considered over a specific time frame to provide context. Short-term investments may yield different results compared to long-term holdings.
- Adjusting for risk: It's essential to consider the risk associated with an investment when analyzing ROI. A high ROI may not be attractive if it comes with significant risk.
- Benchmarking: Comparing ROI against industry standards or similar investments can help investors gauge performance and make informed decisions. At Rapid Innovation, we assist clients in conducting comprehensive ROI analyses, ensuring they can effectively measure the success of their investments and optimize their strategies for greater returns.
15. Future Developments
The future of technology is constantly evolving, and several key areas are poised for significant advancements. Understanding these developments can help businesses and individuals prepare for the changes ahead.
15.1. AI Advancements
Artificial Intelligence (AI) is at the forefront of technological innovation, with numerous advancements expected in the coming years. Key areas of focus include:
- Enhanced Machine Learning: Algorithms will become more sophisticated, allowing machines to learn from smaller datasets and improve their predictive capabilities. Rapid Innovation can assist businesses in implementing these advanced algorithms to optimize operations and enhance decision-making processes.
- Natural Language Processing (NLP): AI will continue to improve in understanding and generating human language, leading to more intuitive virtual assistants and chatbots. Our expertise in NLP can help clients develop customer service solutions that enhance user engagement and satisfaction.
- AI Ethics and Regulation: As AI becomes more integrated into daily life, discussions around ethical use and regulatory frameworks will intensify, ensuring responsible AI deployment. Rapid Innovation can guide organizations in navigating these complexities, ensuring compliance while maximizing the benefits of AI.
- AI in Healthcare: Expect breakthroughs in diagnostics, personalized medicine, and patient care through AI-driven tools that analyze vast amounts of medical data. We can help healthcare providers leverage AI to improve patient outcomes and streamline operations.
- Autonomous Systems: The development of self-driving vehicles and drones will advance, with improved safety features and regulatory acceptance. Rapid Innovation is positioned to support businesses in this sector by providing the necessary AI frameworks and technologies to enhance safety and efficiency.
- Most Advanced AI: The pursuit of the most advanced artificial intelligence will drive research and development, leading to innovations that can transform industries and improve everyday life.
15.2. Market Trends
The technology market is influenced by various trends that shape consumer behavior and business strategies. Notable trends include:
- Remote Work Solutions: The shift to remote work is likely to continue, driving demand for collaboration tools and cybersecurity solutions. Rapid Innovation can help organizations implement robust remote work strategies that enhance productivity and security.
- Sustainability Tech: Companies are increasingly focusing on sustainable practices, leading to innovations in green technology and renewable energy solutions. We can assist businesses in integrating AI to optimize resource management and reduce their carbon footprint.
- E-commerce Growth: Online shopping will keep expanding, with advancements in logistics, payment systems, and personalized shopping experiences. Our AI solutions can help e-commerce businesses enhance customer experiences and streamline operations, including our specialized AI retail and e-commerce solutions.
- Subscription Models: Businesses are moving towards subscription-based services, providing consumers with flexibility and continuous access to products and services. Rapid Innovation can support companies in developing AI-driven models that enhance customer retention and satisfaction.
- 5G Technology: The rollout of 5G networks will enhance connectivity, enabling faster data transfer and the proliferation of IoT devices. We can help businesses harness the power of 5G to develop innovative solutions that drive efficiency and growth.
These advancements in AI, including the latest developments in AI and recent developments in artificial intelligence, and market trends will significantly impact various industries, shaping the future landscape of technology and business. Rapid Innovation is committed to helping clients navigate these changes, ensuring they achieve greater ROI through effective and efficient AI solutions.
15.3. Technology Integration
Technology integration refers to the seamless incorporation of advanced technologies into existing systems and processes. This is crucial for businesses aiming to enhance efficiency, improve customer experience, and stay competitive in a rapidly evolving market. In the context of education, technology integration plays a vital role in enhancing learning experiences and outcomes.
- Enhanced productivity: Integrating technologies like automation and artificial intelligence can streamline operations, reducing manual tasks and increasing output. For instance, Rapid Innovation has helped clients automate routine processes, resulting in a significant increase in productivity and a reduction in operational bottlenecks. In educational settings, integrating technology in the classroom can lead to more efficient teaching methods and improved student engagement.
- Improved data management: Utilizing cloud computing and big data analytics allows organizations to manage vast amounts of information effectively, leading to better decision-making. Our solutions enable clients to harness their data, providing insights that drive strategic initiatives and enhance overall performance. The integration of technology into education also facilitates better data management for tracking student progress and outcomes.
- Customer engagement: Technologies such as chatbots and CRM systems enable businesses to interact with customers in real-time, providing personalized experiences that foster loyalty. Rapid Innovation has implemented AI-driven chatbots for clients, improving response times and customer satisfaction. Similarly, tech integration in the classroom can enhance student engagement through interactive learning tools.
- Cost reduction: By automating processes and optimizing resource allocation, companies can significantly lower operational costs. Our clients have experienced substantial cost savings through tailored automation strategies that minimize waste and enhance efficiency. In education, the integration of technology can also lead to cost savings by reducing the need for physical materials and streamlining administrative tasks.
- Scalability: Integrated technology solutions can easily adapt to growing business needs, allowing for expansion without major overhauls. Rapid Innovation designs scalable systems that grow with our clients, ensuring they remain agile in a dynamic market. The integration of technology in education allows for scalable solutions that can be adapted to different learning environments and student needs. For more information on how we can assist with AI-driven solutions in education, visit our AI EdTech Solutions and learn about the advantages and methods of application in this field.
15.4. Feature Expansion
Feature expansion involves the addition of new functionalities to existing products or services, enhancing their value and appeal to customers. This strategy is essential for businesses looking to maintain relevance and competitiveness in their respective markets, including the realm of technology integration in education.
- Customer feedback: Regularly gathering and analyzing customer feedback helps identify desired features, ensuring that expansions align with user needs. Rapid Innovation assists clients in implementing feedback loops that inform product development. In educational technology, feedback from teachers and students is crucial for effective tech integration.
- Competitive advantage: By continuously adding innovative features, companies can differentiate themselves from competitors and attract new customers. Our expertise in AI allows clients to introduce cutting-edge functionalities that set them apart in the marketplace. In the context of tech integration in the classroom, innovative features can enhance learning experiences and provide a competitive edge for educational institutions.
- Cross-selling opportunities: Expanding features can create opportunities for cross-selling, as customers may be more inclined to purchase complementary products or services. We help clients identify and develop these opportunities through strategic feature enhancements. In education, integrating digital technologies can lead to cross-selling opportunities for additional learning tools and resources.
- Increased user engagement: New features can enhance user experience, leading to higher engagement rates and customer retention. Rapid Innovation has successfully guided clients in launching features that resonate with users, resulting in improved retention metrics. The integration of technology in the classroom can significantly boost student engagement through interactive and personalized learning experiences.
- Market adaptability: Feature expansion allows businesses to respond to changing market trends and consumer preferences, ensuring they remain relevant. Our team works closely with clients to monitor trends and adapt their offerings accordingly, including the evolving landscape of technology integration in education.
15.5. Industry Evolution
Industry evolution refers to the gradual transformation of an industry over time, driven by technological advancements, changing consumer behaviors, and market dynamics. Understanding this evolution is vital for businesses to adapt and thrive, particularly in the context of technology integration in education.
- Technological advancements: Innovations such as artificial intelligence, blockchain, and the Internet of Things (IoT) are reshaping industries, creating new opportunities and challenges. Rapid Innovation stays at the forefront of these advancements, helping clients leverage new technologies for competitive advantage. The integration of technology into education is a prime example of how these advancements can transform learning environments.
- Consumer behavior shifts: As consumers become more tech-savvy, their expectations evolve, pushing businesses to adapt their offerings and marketing strategies. We assist clients in understanding these shifts and aligning their strategies to meet evolving consumer demands. In education, the shift towards integrating technology in the classroom reflects changing expectations from students and parents.
- Regulatory changes: Industries often face new regulations that can impact operations, requiring companies to stay informed and compliant to avoid penalties. Our consulting services ensure that clients navigate regulatory landscapes effectively. In the education sector, regulations surrounding technology integration must be carefully considered to ensure compliance and effectiveness.
- Globalization: The interconnectedness of markets means that businesses must consider global trends and competition, influencing their strategies and operations. Rapid Innovation provides insights into global market dynamics, helping clients position themselves strategically. The integration of technology in education is also influenced by global trends in teaching and learning methodologies.
- Sustainability focus: Increasing awareness of environmental issues is driving industries to adopt sustainable practices, impacting product development and supply chain management. We guide clients in integrating sustainable practices into their operations, enhancing their brand reputation and compliance. In education, integrating technology can also support sustainability efforts by reducing paper usage and promoting digital resources.
16. Resources and Support
In any organization or project, having access to the right resources and support is crucial for success. This section focuses on the essential components that provide guidance and assistance to users, ensuring they can effectively utilize tools and systems.
16.1. Training Materials
Training materials are vital for onboarding new users and enhancing the skills of existing team members. They serve as a foundation for understanding processes, tools, and best practices. Effective training materials can include:
- User Manuals: Comprehensive guides that outline how to use specific software or tools, often including step-by-step instructions, screenshots, and troubleshooting tips.
- Video Tutorials: Engaging visual content that demonstrates how to perform tasks or navigate systems. These can be particularly helpful for visual learners and can be accessed at any time.
- Webinars and Workshops: Live or recorded sessions that provide in-depth training on specific topics. These interactive formats allow users to ask questions and engage with trainers.
- FAQs and Quick Reference Guides: Concise documents that address common questions and provide quick tips. These resources are handy for users who need immediate assistance without sifting through extensive documentation.
- E-Learning Modules: Online courses that allow users to learn at their own pace, often including quizzes and assessments to reinforce learning.
- Community Forums: Platforms where users can share experiences, ask questions, and provide support to one another. These forums foster a sense of community and can be a valuable resource for problem-solving.
- SAP MM Purchasing Technical Reference and Learning Guide PDF: A specialized resource that provides in-depth knowledge and guidance on SAP MM purchasing processes, enhancing the training experience.
Investing in high-quality training materials not only enhances user proficiency but also boosts overall productivity. Organizations that prioritize training often see improved employee satisfaction and retention.
16.2. Technical Documentation
Technical documentation is essential for ensuring that users can effectively understand and utilize complex systems and technologies. It provides detailed information about the functionality, architecture, and maintenance of software or hardware. Key components of technical documentation include:
- System Architecture Diagrams: Visual representations of the system's components and their interactions, helping users grasp the overall structure and flow of information.
- API Documentation: Detailed descriptions of application programming interfaces (APIs), including endpoints, request/response formats, and authentication methods. This documentation is crucial for developers integrating different systems.
- Installation Guides: Step-by-step instructions for setting up software or hardware. Clear installation guides reduce the likelihood of errors during the setup process.
- Troubleshooting Guides: Resources that help users diagnose and resolve common issues, often including error codes, potential causes, and recommended solutions.
- Release Notes: Documentation that outlines new features, bug fixes, and changes in each software version. Keeping users informed about updates ensures they can take advantage of new functionalities.
- Maintenance and Support Procedures: Guidelines for ongoing maintenance and support, including contact information for technical support teams. This ensures users know where to turn for help when needed.
- Training Materials and Technical Documentation: Comprehensive resources that ensure users have access to the necessary information and support to effectively utilize the systems in place.
Having comprehensive technical documentation is crucial for minimizing downtime and ensuring that users can quickly resolve issues. It also serves as a reference point for future development and updates, making it easier to maintain systems over time.
By providing robust resources and support, Rapid Innovation empowers organizations to leverage AI agents for technical troubleshooting effectively, enhancing productivity and fostering a culture of continuous learning and improvement. This commitment to user support not only drives greater ROI but also positions clients for long-term success in an increasingly competitive landscape.
16.3. User Guides
User guides are essential resources that provide detailed instructions on how to use a product or service effectively. They serve as a roadmap for users, helping them navigate features and functionalities.
- Comprehensive instructions: User guides typically include step-by-step instructions, making it easier for users to understand how to operate a product. This is particularly important in AI applications, where complex algorithms and functionalities can be daunting for users. For instance, the midjourney user manual offers specific guidance on utilizing the platform effectively.
- Visual aids: Many user guides incorporate diagrams, screenshots, and illustrations to enhance understanding and provide visual context. In the realm of AI, visual representations of data flows or model architectures can significantly aid comprehension. User guides for AI often include these visual elements to clarify complex concepts.
- Troubleshooting tips: Effective user guides often include a section dedicated to troubleshooting common issues, helping users resolve problems independently. This is crucial in AI systems, where users may encounter unique challenges related to data input or model performance.
- Accessibility: User guides should be easily accessible, whether in print or digital format, allowing users to refer to them whenever needed. For AI solutions, having digital guides that can be integrated into the software interface can enhance user experience.
- Regular updates: As products evolve, user guides should be updated to reflect new features or changes, ensuring users have the most current information. In the fast-paced AI landscape, timely updates are essential to keep users informed about the latest advancements and functionalities.
16.4. FAQs
Frequently Asked Questions (FAQs) are a valuable tool for addressing common inquiries and concerns that users may have. They streamline communication and provide quick answers to users.
- Quick reference: FAQs allow users to find answers to their questions without needing to contact support, saving time for both users and support teams. This is especially beneficial in AI applications, where users may have specific queries about model training or data handling.
- Common issues: FAQs typically cover a range of topics, including product features, pricing, and troubleshooting, addressing the most common user concerns. In AI, this could include questions about data privacy, model accuracy, and integration with existing systems.
- Clarity and simplicity: Effective FAQs use clear and concise language, making it easy for users to understand the information provided. This is vital in AI, where technical jargon can often confuse users.
- Searchability: Organizing FAQs by categories or using a search function can enhance user experience, allowing users to find relevant information quickly. For AI solutions, categorizing FAQs by use cases or application areas can be particularly helpful.
- Continuous improvement: Regularly updating FAQs based on user feedback and emerging trends can help ensure that the information remains relevant and useful. In the AI domain, staying current with industry developments is crucial for maintaining user trust and satisfaction.
16.5. Support Services
Support services are crucial for providing assistance to users who encounter issues or have questions about a product or service. These services can take various forms, ensuring users receive the help they need.
- Multiple channels: Support services should be available through various channels, including phone, email, live chat, and social media, catering to different user preferences. In the context of AI, offering support through chatbots can provide immediate assistance to users.
- Knowledgeable staff: Support teams should be well-trained and knowledgeable about the product, enabling them to provide accurate and helpful assistance. In AI, having staff who understand both the technical and practical applications of the technology is essential for effective support.
- Response time: Timely responses to user inquiries are essential for maintaining customer satisfaction. Support services should aim for quick turnaround times, especially in AI, where users may be relying on the technology for critical business operations.
- Self-service options: Providing self-service resources, such as knowledge bases or community forums, can empower users to find solutions independently. In AI, these resources can include tutorials on model training or best practices for data management.
- Feedback mechanisms: Implementing feedback systems allows users to share their experiences with support services, helping organizations improve their offerings. In the AI sector, user feedback can be invaluable for refining algorithms and enhancing user experience.
‍