Table Of Contents
Category
Machine Learning (ML)
Artificial Intelligence (AI)
IoT
Blockchain
Manufacturing
Logistics
Supplychain
Cryptocurrency Solutions
Decentralized Finance (DeFi)
Cloud Computing
1. Introduction to Predictive Device Failure Detection
Predictive device failure detection is a crucial aspect of modern industrial operations, leveraging advanced technologies to foresee potential equipment failures before they occur. This proactive approach minimizes downtime, reduces maintenance costs, and enhances overall operational efficiency. Predictive maintenance utilizes data analytics, machine learning, and artificial intelligence (AI) to monitor equipment health by analyzing historical data and real-time sensor inputs. Predictive models can identify patterns that indicate impending failures, transitioning from reactive maintenance—which addresses issues after they arise—to a more strategic, predictive approach.
The rise of the Internet of Things (IoT) has significantly contributed to the effectiveness of predictive device failure detection. With numerous sensors embedded in machinery, organizations can collect vast amounts of data, which can be analyzed to predict when a device is likely to fail. This technology is applicable across various industries, including manufacturing, energy, transportation, and healthcare. Companies can save substantial costs by preventing unexpected breakdowns and optimizing maintenance schedules through IoT predictive maintenance.
At Rapid Innovation, we specialize in integrating AI-driven predictive maintenance solutions tailored to your specific operational needs. By leveraging our expertise in predictive maintenance solutions, clients can enhance their predictive capabilities, leading to improved ROI through reduced operational disruptions and optimized resource allocation.
Incorporating AI agents into predictive maintenance systems enhances their capabilities. These AI agents can process large datasets quickly, identify anomalies, and provide actionable insights. AI algorithms can learn from new data, continuously improving their predictive accuracy. This adaptability is essential in dynamic environments where equipment conditions can change rapidly, making IoT and predictive maintenance a powerful combination. For more information on how AI agents can be utilized in maintenance tracking.
Overall, predictive device failure detection represents a significant advancement in maintenance strategies, enabling organizations to operate more efficiently and effectively. With Rapid Innovation's consulting and development services, including partnerships with predictive maintenance companies like IBM, SAP, and AWS, businesses can harness the power of AI to transform their maintenance practices and achieve their strategic goals.
1.1. Overview and Importance
Failure detection systems are critical components in various industries, including manufacturing, telecommunications, and IT. These systems are designed to identify and diagnose failures in equipment or processes, ensuring that operations run smoothly and efficiently. They help minimize downtime, which can lead to significant financial losses. Early detection of failures can prevent catastrophic events, enhancing safety. Additionally, failure detection systems contribute to improved maintenance strategies, allowing for predictive maintenance rather than reactive approaches.
The importance of failure detection systems cannot be overstated. They play a vital role in enhancing operational efficiency by ensuring that equipment is functioning optimally, reducing maintenance costs through timely interventions, and increasing customer satisfaction by ensuring consistent service delivery. At Rapid Innovation, we leverage our expertise in AI to develop tailored failure detection solutions that align with your business goals, ultimately driving greater ROI. For more insights on how AI can enhance procurement intelligence, check out our article on AI Agent Procurement Intelligence Engine.
1.2. Evolution of Failure Detection Systems
The evolution of failure detection systems has been marked by significant technological advancements. Initially, these systems relied on basic monitoring techniques, such as manual inspections and simple alarms. Over time, the following developments have occurred:
- Introduction of automated monitoring systems that utilize sensors to collect data in real-time.
- Development of sophisticated algorithms that analyze data to predict potential failures before they occur.
- Integration of Internet of Things (IoT) technology, allowing for remote monitoring and control of devices.
The shift from reactive to proactive maintenance strategies has been a game-changer. Modern failure detection systems can now:
- Utilize machine learning to improve accuracy in failure predictions.
- Leverage big data analytics to process vast amounts of information for better decision-making.
- Incorporate cloud computing for enhanced data storage and accessibility.
At Rapid Innovation, we harness these advancements to create customized solutions that not only detect failures but also optimize maintenance schedules, ensuring that your operations remain efficient and cost-effective.
1.3. Role of AI in Modern Device Monitoring
Artificial Intelligence (AI) has revolutionized the landscape of device monitoring and failure detection systems. By harnessing the power of AI, organizations can achieve unprecedented levels of efficiency and accuracy in their monitoring systems. AI algorithms can analyze historical data to identify patterns and anomalies that may indicate potential failures. Machine learning models can continuously improve their predictions based on new data, leading to more reliable outcomes. Furthermore, AI-driven failure detection systems can automate responses to detected failures, reducing the need for human intervention.
The integration of AI in failure detection systems offers several advantages:
- Enhanced predictive maintenance capabilities, allowing organizations to schedule maintenance activities based on actual equipment conditions rather than fixed schedules.
- Improved resource allocation, as AI can help prioritize which devices require immediate attention.
- Increased operational resilience, as AI systems can adapt to changing conditions and provide real-time insights.
At Rapid Innovation, we specialize in developing AI-driven failure detection systems that empower organizations to not only monitor their devices effectively but also to make informed decisions that enhance operational efficiency and reduce costs.
In conclusion, the combination of advanced failure detection systems and AI technology is transforming how organizations monitor and maintain their devices, leading to greater efficiency, safety, and cost savings. By partnering with Rapid Innovation, you can leverage these advancements to achieve your business goals efficiently and effectively.
1.4. Business Impact and ROI
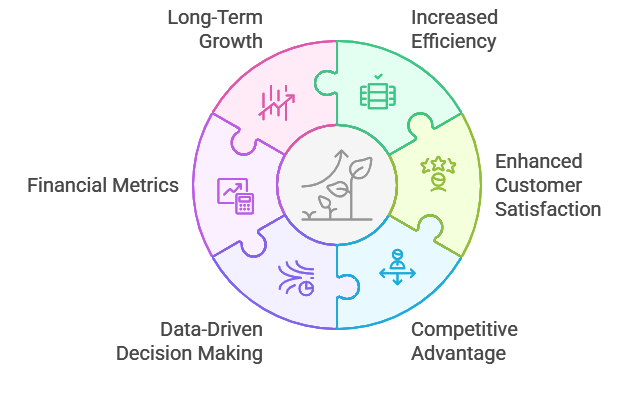
‍
Understanding the business impact and return on investment (ROI) of a project or initiative is crucial for organizations aiming to maximize their resources. The business impact refers to the tangible and intangible effects that a project has on an organization, while ROI measures the financial return relative to the investment made.
- Increased Efficiency: Implementing new systems or processes can streamline operations, reducing time and costs. For example, Rapid Innovation has helped clients automate their workflows, leading to faster processing times and fewer errors, ultimately enhancing productivity.
- Enhanced Customer Satisfaction: Improved services or products can lead to higher customer satisfaction, resulting in increased loyalty and repeat business. Rapid Innovation's AI-driven solutions have enabled clients to personalize their offerings, making satisfied customers more likely to recommend their business to others.
- Competitive Advantage: Organizations that leverage innovative technologies or processes can gain a competitive edge in the market. Rapid Innovation assists clients in adopting cutting-edge AI solutions, which can lead to increased market share and profitability.
- Data-Driven Decision Making: Investments in analytics and reporting tools can provide insights that drive better business decisions. Rapid Innovation empowers clients with advanced analytics capabilities, leading to more effective strategies and improved performance.
- Financial Metrics: Calculating ROI involves comparing the net profit from an investment to its cost. A positive ROI indicates that the benefits outweigh the costs, making the investment worthwhile. According to a study, companies that invest in technology can see an ROI of up to 300% over three years.
- Long-Term Growth: Investments that may not show immediate returns can lead to long-term growth opportunities, including developing new markets or enhancing product offerings.
2. System Architecture
System architecture refers to the conceptual model that defines the structure, behavior, and various views of a system. It serves as a blueprint for both the system and the project developing it. A well-defined system architecture is essential for ensuring that all components work together effectively.
- Scalability: A good system architecture allows for easy scaling of resources to meet growing demands, which is crucial for businesses that anticipate growth or fluctuating workloads.
- Integration: Effective architecture facilitates the integration of various components, ensuring that they communicate seamlessly. This is vital for maintaining data consistency and operational efficiency.
- Security: A robust architecture incorporates security measures to protect sensitive data and systems from threats. This includes implementing firewalls, encryption, and access controls.
- Flexibility: The architecture should be adaptable to changes in technology or business needs, allowing organizations to pivot quickly in response to market demands.
- Performance: System architecture impacts the overall performance of applications. Optimized architecture can lead to faster response times and improved user experiences.
2.1. Core Components
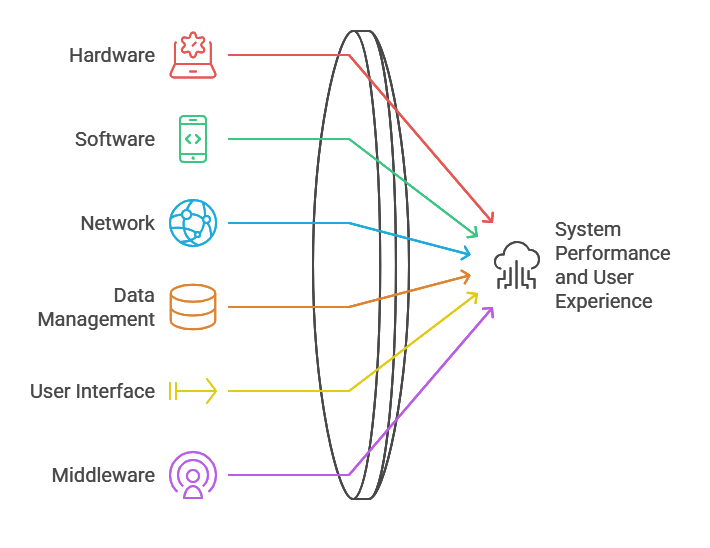
‍
Core components of system architecture are the fundamental building blocks that define how a system operates. Understanding these components is essential for designing effective systems.
- Hardware: This includes the physical devices such as servers, computers, and networking equipment that support the system. The choice of hardware can significantly affect performance and scalability.
- Software: The applications and operating systems that run on the hardware are critical. This includes both system software (like operating systems) and application software (like databases and user interfaces).
- Network: The network infrastructure connects different components of the system, enabling communication and data transfer. A reliable network is essential for system performance and user accessibility.
- Data Management: Effective data management practices ensure that data is stored, retrieved, and processed efficiently. This includes databases, data warehouses, and data lakes.
- User Interface: The user interface (UI) is how users interact with the system. A well-designed UI enhances user experience and can lead to higher productivity.
- Middleware: Middleware acts as a bridge between different software applications, allowing them to communicate and share data. It is essential for integrating disparate systems and ensuring smooth operations.
2.1.1. Data Collection Layer
The Data Collection Layer is the foundational component of any data-driven system. It is responsible for gathering raw data from various sources, ensuring that the data is accurate, relevant, and timely. This layer plays a crucial role in the overall data pipeline, as the quality of data collected directly impacts the effectiveness of subsequent processing and analysis.
- Sources of Data: Â
- IoT devices
- Social media platforms
- Transactional databases
- Web scraping
- APIs from third-party services
- Key Functions: Â
- Data ingestion: Collecting data in real-time or batch mode.
- Data validation: Ensuring the accuracy and integrity of the data collected.
- Data storage: Temporarily holding data before it is processed.
- Technologies Used: Â
- Apache Kafka for real-time data streaming.
- Apache NiFi for data flow automation.
- ETL (Extract, Transform, Load) tools for batch processing.
The effectiveness of the Data Collection Layer is critical for businesses aiming to leverage big data analytics. A robust data collection strategy, including techniques of data collection and data gathering techniques in research, can lead to better insights and informed decision-making. At Rapid Innovation, we assist clients in implementing efficient data collection frameworks that enhance data quality and accessibility, ultimately driving greater ROI. This includes data collection procedures for qualitative research and automating data capture to streamline the process. For more on the importance of data quality.
2.1.2. Processing Engine
The Processing Engine is the core component that transforms raw data into a structured format suitable for analysis. This layer is responsible for executing various data processing tasks, including data cleaning, transformation, and aggregation. The efficiency of the Processing Engine can significantly affect the speed and accuracy of data analysis.
- Key Functions: Â
- Data cleaning: Removing duplicates, correcting errors, and handling missing values.
- Data transformation: Converting data into a usable format, such as normalizing or encoding.
- Data aggregation: Summarizing data to provide insights at different levels.
- Processing Techniques: Â
- Batch processing: Handling large volumes of data at once, often used for historical data analysis.
- Stream processing: Analyzing data in real-time as it is collected, ideal for time-sensitive applications.
- Technologies Used: Â
- Apache Spark for distributed data processing.
- Apache Flink for real-time stream processing.
- Hadoop MapReduce for batch processing.
The Processing Engine is essential for ensuring that data is not only processed efficiently but also accurately. A well-optimized processing engine can lead to faster insights and improved operational efficiency. Rapid Innovation helps clients streamline their processing capabilities, enabling them to make timely and informed decisions that enhance their competitive edge. This includes collecting and analyzing data to ensure comprehensive insights.
2.1.3. AI Analysis Module
The AI Analysis Module is the advanced layer that applies artificial intelligence and machine learning algorithms to the processed data. This module is designed to extract meaningful insights, identify patterns, and make predictions based on historical data. The integration of AI into data analysis enhances the capability to derive actionable insights from complex datasets.
- Key Functions: Â
- Predictive analytics: Using historical data to forecast future trends.
- Pattern recognition: Identifying trends and anomalies in data.
- Natural language processing: Analyzing text data for sentiment analysis and topic modeling.
- Techniques Used: Â
- Supervised learning: Training models on labeled data to make predictions.
- Unsupervised learning: Discovering hidden patterns in unlabeled data.
- Reinforcement learning: Training models through trial and error to optimize decision-making.
- Technologies Used: Â
- TensorFlow and PyTorch for building machine learning models.
- Scikit-learn for traditional machine learning algorithms.
- Apache Mahout for scalable machine learning.
The AI Analysis Module is pivotal for organizations looking to harness the power of data-driven decision-making. By leveraging AI, businesses can gain deeper insights, improve customer experiences, and drive innovation. At Rapid Innovation, we empower our clients to utilize advanced AI techniques, ensuring they stay ahead in their industries and achieve substantial returns on their investments. This includes understanding the meaning of collection of data and the importance of data gathering and analysis in driving business success.
2.1.4. Alert and Reporting System
An effective alert and reporting system is crucial for monitoring and managing operations within any organization. This system serves as a proactive measure to identify issues before they escalate, ensuring that stakeholders are informed and can take timely action.
- Real-time Alerts: The alert and reporting system should provide real-time notifications for critical events, such as system failures, security breaches, or performance degradation. This allows teams to respond quickly to mitigate potential damage, ultimately enhancing operational efficiency and reducing downtime.
- Customizable Reporting: Users should have the ability to customize reports based on specific metrics and KPIs relevant to their roles. This ensures that the information presented is actionable and tailored to the needs of different departments, leading to more informed decision-making and improved performance.
- Historical Data Analysis: The alert and reporting system should maintain historical data to analyze trends over time. This can help in identifying recurring issues and understanding the root causes of problems, enabling organizations to implement preventive measures and optimize processes.
- User-Friendly Interface: A simple and intuitive interface is essential for users to navigate the alert and reporting system effectively. This reduces the learning curve and increases adoption rates among team members, fostering a culture of data-driven decision-making.
- Integration with Other Tools: The alert and reporting system should seamlessly integrate with other tools and platforms used within the organization, such as project management software, CRM systems, and communication tools. This enhances collaboration and ensures that all relevant data is accessible in one place, streamlining workflows and improving overall productivity.
At Rapid Innovation, we specialize in developing and implementing alert and reporting systems to help our clients achieve their business goals efficiently and effectively, ultimately driving greater ROI through enhanced operational capabilities. If you're looking to enhance your alert and reporting system, consider hiring our Action Transformer Developers to assist you in this process. Additionally, you can explore the benefits of generative AI in automated financial reporting for further insights.
2.2. Integration Points
Integration points are critical for ensuring that various systems and applications within an organization work together efficiently. Identifying and implementing these integration points can lead to improved workflows and data consistency.
- API Connectivity: Application Programming Interfaces (APIs) are essential for enabling different software applications to communicate with each other. Organizations should leverage APIs to connect disparate systems, allowing for data exchange and functionality sharing, which can significantly enhance operational efficiency.
- Data Synchronization: Ensuring that data is synchronized across systems is vital for maintaining accuracy and consistency. Integration points should facilitate real-time data updates, reducing the risk of discrepancies and errors, thereby improving data integrity.
- Third-Party Integrations: Many organizations rely on third-party applications for specific functions, such as marketing automation or customer support. Identifying integration points with these tools can enhance overall efficiency and provide a more comprehensive view of operations, leading to better strategic planning.
- Workflow Automation: Integration points can enable workflow automation, reducing manual tasks and streamlining processes. This can lead to increased productivity and reduced operational costs, ultimately contributing to a higher return on investment.
- Security Considerations: When establishing integration points, it is essential to consider security implications. Data should be encrypted during transmission, and access controls should be implemented to protect sensitive information, ensuring compliance with industry standards.
2.3. Scalability Considerations
Scalability is a critical factor for any organization looking to grow and adapt to changing market conditions. A scalable system can accommodate increased workloads without compromising performance or requiring significant reconfiguration.
- Infrastructure Flexibility: Organizations should invest in flexible infrastructure that can easily scale up or down based on demand. This includes cloud-based solutions that allow for on-demand resource allocation, ensuring that businesses can respond swiftly to market changes.
- Load Balancing: Implementing load balancing techniques can help distribute workloads evenly across servers, ensuring that no single server becomes a bottleneck. This enhances performance and reliability as user demand increases, supporting sustained growth.
- Modular Architecture: A modular system architecture allows organizations to add or remove components as needed. This flexibility enables businesses to adapt to new requirements without overhauling the entire system, facilitating innovation and agility.
- Performance Monitoring: Continuous performance monitoring is essential for identifying potential scalability issues before they impact operations. Organizations should implement monitoring tools to track system performance and resource utilization, ensuring optimal performance at all times.
- Cost Management: As organizations scale, it is crucial to manage costs effectively. This includes evaluating the cost implications of scaling up infrastructure and ensuring that the return on investment justifies the expenses incurred, allowing for sustainable growth and profitability.
2.4. Security Architecture
Security architecture is a critical component in the design and implementation of any system, particularly in the context of information technology and data management. It encompasses the framework and strategies that protect data integrity, confidentiality, and availability.
- Components of Security Architecture: Â
- Policies and Standards: Establishing clear security policies and standards is essential for guiding the behavior of users and systems.
- Access Control: Implementing robust access control mechanisms ensures that only authorized users can access sensitive data.
- Network Security: Utilizing firewalls, intrusion detection systems, and secure communication protocols helps protect data in transit.
- Data Encryption: Encrypting data both at rest and in transit safeguards it from unauthorized access and breaches.
- Monitoring and Auditing: Continuous monitoring and regular audits help identify vulnerabilities and ensure compliance with security policies.
- Frameworks and Models: Â
- Zero Trust Architecture: This model assumes that threats could be internal or external, requiring strict verification for every user and device.
- Defense in Depth: This strategy involves multiple layers of security controls to protect data, making it harder for attackers to penetrate the system.
- SASE Architecture: This framework combines network security functions with WAN capabilities to support the secure access needs of users and devices.
- Compliance and Regulations: Adhering to regulations such as GDPR, HIPAA, and PCI-DSS is crucial for maintaining security and protecting user data.
- Emerging Technologies: The integration of AI and machine learning in security architecture can enhance threat detection and response capabilities, allowing organizations to proactively address potential security risks. This is particularly relevant in the context of cloud security architecture, where organizations must secure their cloud environments against evolving threats. For more information on how we can assist with this, our insights on AI agents for IoT sensor integration.
3. Data Collection and Preprocessing
Data collection and preprocessing are vital steps in the data analysis pipeline, ensuring that the data used for analysis is accurate, relevant, and ready for processing. This phase involves gathering data from various sources and preparing it for further analysis.
- Importance of Data Collection: Â
- Accurate data collection is essential for making informed decisions and helps in identifying trends, patterns, and anomalies in the data.
- Methods of Data Collection: Â
- Surveys and Questionnaires: Collecting data directly from users or stakeholders.
- Web Scraping: Extracting data from websites for analysis.
- APIs: Utilizing application programming interfaces to gather data from other software applications.
- Data Preprocessing Steps: Â
- Data Cleaning: Removing duplicates, correcting errors, and handling missing values to ensure data quality.
- Data Transformation: Converting data into a suitable format or structure for analysis, such as normalization or standardization.
- Data Integration: Combining data from different sources to create a unified dataset.
3.1. Sensor Types and Deployment
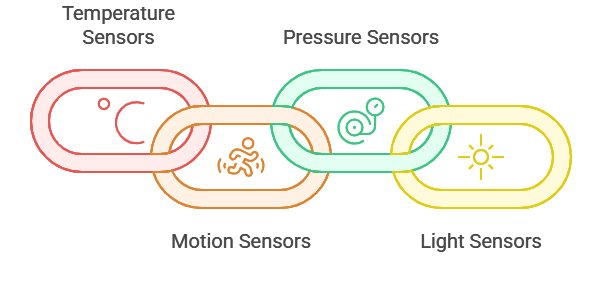
‍
Sensors play a crucial role in data collection, especially in the context of the Internet of Things (IoT) and smart systems. Understanding the types of sensors and their deployment strategies is essential for effective data gathering.
- Types of Sensors: Â
- Temperature Sensors: Measure ambient temperature and are widely used in climate control systems.
- Motion Sensors: Detect movement and are commonly used in security systems and smart homes.
- Pressure Sensors: Monitor pressure levels in various applications, including industrial processes and weather stations.
- Light Sensors: Measure light intensity and are used in smart lighting systems.
- Deployment Strategies: Â
- Fixed Deployment: Sensors are installed in a specific location for continuous monitoring, such as in smart buildings.
- Mobile Deployment: Sensors are placed on mobile devices or vehicles to collect data on the go, useful in logistics and transportation.
- Distributed Networks: A network of sensors is deployed across a wide area to gather comprehensive data, often used in environmental monitoring.
- Challenges in Sensor Deployment: Â
- Connectivity Issues: Ensuring reliable communication between sensors and data collection systems can be challenging.
- Power Management: Many sensors operate on battery power, necessitating efficient energy management strategies.
- Data Security: Protecting the data collected by sensors from unauthorized access and breaches is crucial, particularly in the context of computer security architecture.
- Applications of Sensors: Â
- Smart Cities: Sensors are used for traffic management, waste management, and environmental monitoring.
- Healthcare: Wearable sensors monitor patient health metrics in real-time.
- Agriculture: Soil moisture sensors help optimize irrigation and improve crop yields.
At Rapid Innovation, we leverage our expertise in AI and data management to enhance security architecture and data collection processes for our clients. By implementing advanced security measures and efficient data handling strategies, we help organizations achieve greater ROI and ensure their systems are robust against emerging threats, including those related to cyber security architecture and cloud computing security architecture.
3.2. Data Sources
Data sources are critical for gathering insights and making informed decisions in various fields, including technology, environmental science, and business analytics. Understanding the types of data sources available can enhance the quality of analysis and improve outcomes. Two significant categories of data sources are device telemetry and environmental metrics.
3.2.1. Device Telemetry
Device telemetry refers to the automated process of collecting and transmitting data from devices to a central system for monitoring and analysis. This data can come from various devices, including sensors, IoT devices, and machinery.
Key aspects of device telemetry include:
- Real-time data collection: Telemetry systems can provide immediate insights, allowing for quick decision-making.
- Remote monitoring: Devices can be monitored from anywhere, reducing the need for physical presence.
- Data accuracy: Automated data collection minimizes human error, ensuring more reliable data.
- Scalability: Telemetry systems can easily scale to accommodate more devices as needed.
Applications of device telemetry are vast and include:
- Healthcare: Wearable devices collect health metrics like heart rate and activity levels, providing valuable data for patient monitoring. Rapid Innovation can help healthcare providers implement AI-driven analytics to interpret this data, leading to improved patient outcomes and operational efficiencies.
- Manufacturing: Machinery telemetry helps in predictive maintenance, reducing downtime and improving efficiency. By integrating AI algorithms, Rapid Innovation enables manufacturers to predict equipment failures before they occur, thus maximizing ROI through reduced maintenance costs.
- Transportation: Fleet management systems use telemetry to track vehicle performance and optimize routes. Our AI solutions can analyze this data to enhance route planning, reduce fuel consumption, and improve overall fleet efficiency.
3.2.2. Environmental Metrics
Environmental metrics involve the collection and analysis of data related to environmental conditions. This data is crucial for understanding the impact of human activities on the environment and for developing strategies for sustainability.
Important components of environmental metrics include:
- Air quality: Measurements of pollutants and particulate matter help assess the health of the atmosphere.
- Water quality: Data on chemical composition and biological indicators of water bodies inform conservation efforts.
- Climate data: Temperature, humidity, and precipitation metrics are essential for climate modeling and forecasting.
The significance of environmental metrics can be seen in various sectors:
- Urban planning: Data on air and noise pollution can guide the development of greener cities. Rapid Innovation can assist urban planners by providing AI tools that analyze environmental data to create sustainable urban designs.
- Agriculture: Farmers use environmental metrics to optimize irrigation and crop management, enhancing yield while conserving resources. Our AI solutions can analyze weather patterns and soil conditions to provide actionable insights, leading to increased agricultural productivity and sustainability.
- Policy-making: Governments rely on environmental data to create regulations aimed at reducing pollution and protecting natural resources. Rapid Innovation can support policymakers by developing AI models that simulate the impact of various regulations, helping to create more effective environmental policies.
In conclusion, both device telemetry and environmental metrics serve as vital data sources that contribute to informed decision-making across multiple domains. By leveraging these data sources, organizations can enhance operational efficiency, promote sustainability, and improve overall outcomes. Rapid Innovation is committed to helping clients harness the power of AI to achieve these goals effectively and efficiently, ultimately driving greater ROI.
Additionally, various projects can enhance data analysis capabilities, such as sql projects for data analysis, data analysis projects in python, and data analytics projects for beginners. For those looking to delve deeper, big data analytics projects and procurement data analytics can provide valuable insights. Open source data analytics and open source data analysis tools can also be beneficial for organizations seeking cost-effective solutions. Furthermore, integrating tools like azure synapse data warehouse and adobe analytics data feed can streamline data management and analysis processes.
3.2.3. Historical Failure Data
Historical failure data is crucial for understanding the reliability and performance of systems and components over time. This data provides insights into past failures, enabling organizations to identify patterns and trends that can inform future maintenance and operational strategies.
- Helps in predictive maintenance: By analyzing historical failure data, organizations can predict when a component is likely to fail, allowing for timely interventions. Rapid Innovation leverages advanced AI algorithms to analyze this data, enabling clients to implement predictive maintenance strategies that significantly reduce downtime and maintenance costs.
- Identifies root causes: Understanding the reasons behind past failures can help in addressing underlying issues, reducing the likelihood of recurrence. Our AI-driven analytics tools assist clients in pinpointing root causes, leading to more effective solutions and improved system reliability.
- Supports risk assessment: Historical failure data can be used to evaluate the risk associated with specific components or systems, aiding in prioritization of maintenance efforts. Rapid Innovation's data analytics capabilities empower organizations to assess risks accurately, ensuring that resources are allocated where they are needed most.
- Enhances decision-making: Access to comprehensive failure data allows for informed decision-making regarding resource allocation and operational changes. Our AI solutions provide actionable insights that help clients make strategic decisions, ultimately driving greater ROI.
- Facilitates compliance: Many industries require documentation of historical failure data for regulatory compliance, making it essential for organizations to maintain accurate records. Rapid Innovation assists clients in automating compliance processes, ensuring that they meet regulatory requirements efficiently. For more information on how we can help, check out our Enterprise AI Development services.
3.2.4. Maintenance Records
Maintenance records are essential for tracking the upkeep and servicing of equipment and systems. These records provide a detailed history of maintenance activities, which is vital for ensuring operational efficiency and safety.
- Provides a maintenance history: Detailed records help in understanding the frequency and type of maintenance performed on equipment, which can inform future maintenance schedules. Our solutions enable clients to maintain comprehensive maintenance histories, optimizing their maintenance planning.
- Aids in warranty claims: Accurate maintenance records are often necessary for validating warranty claims, ensuring that organizations can recover costs for repairs or replacements. Rapid Innovation's systems streamline record-keeping, making it easier for clients to manage warranty claims effectively.
- Supports performance analysis: By reviewing maintenance records, organizations can assess the effectiveness of their maintenance strategies and make necessary adjustments. Our AI tools provide insights that help clients refine their maintenance approaches, leading to improved performance.
- Enhances accountability: Maintenance records create a clear trail of responsibility, ensuring that all maintenance activities are documented and can be reviewed if issues arise. Rapid Innovation's solutions promote accountability by providing transparent and accessible maintenance records.
- Improves resource management: Understanding maintenance needs through records allows organizations to allocate resources more effectively, optimizing both time and budget. Our data-driven insights help clients manage their resources efficiently, maximizing their operational effectiveness.
3.3. Data Quality Management
Data quality management (DQM) is a systematic approach to ensuring that data is accurate, consistent, and reliable. In the context of maintenance and operational data, DQM is essential for making informed decisions and optimizing processes.
- Ensures data accuracy: DQM processes help in identifying and correcting errors in data, ensuring that organizations are working with reliable information. Rapid Innovation employs AI techniques to enhance data accuracy, enabling clients to trust their data for critical decision-making.
- Promotes consistency: By standardizing data entry and management practices, DQM helps maintain consistency across datasets, which is crucial for analysis and reporting. Our solutions ensure that clients' data management practices are aligned, facilitating seamless analysis.
- Facilitates data integration: High-quality data can be easily integrated from various sources, enabling comprehensive analysis and insights. Rapid Innovation's expertise in data integration allows clients to harness the full potential of their data, driving better business outcomes.
- Supports compliance: Many industries have strict data quality standards; effective DQM ensures that organizations meet these requirements, avoiding potential penalties. Our DQM solutions help clients navigate compliance challenges with ease, safeguarding their operations.
- Enhances decision-making: Reliable data is the foundation of effective decision-making, allowing organizations to make strategic choices based on accurate information. Rapid Innovation empowers clients with high-quality data, enabling them to make informed decisions that drive growth and efficiency.
3.4. Real-time Data Processing
Real-time data processing refers to the immediate processing of data as it is generated or received. This capability is crucial for businesses that rely on timely information to make decisions.
- Enables instant insights: Organizations can analyze data on-the-fly, allowing for quick decision-making. For instance, a retail company can adjust its inventory in real-time based on customer purchasing patterns, leading to reduced stockouts and increased sales. This is a key aspect of real time data integration.
- Supports various applications: Industries such as finance, healthcare, and e-commerce benefit from real-time analytics to monitor transactions, patient data, and customer behavior. For example, financial institutions can detect fraudulent transactions as they occur, minimizing losses through real time data analysis.
- Enhances customer experience: Businesses can provide personalized services and recommendations based on real-time data, improving customer satisfaction. A streaming service, for example, can suggest content based on a user's current viewing habits, leveraging real time stream analytics.
- Utilizes technologies: Tools like Apache Kafka, Apache Flink, and Amazon Kinesis are popular for handling real-time data streams, enabling organizations to harness the power of their data effectively. Kafka real time streaming is particularly noted for its scalability and reliability in real time processing.
- Facilitates predictive analytics: By processing data in real-time, companies can predict trends and behaviors, leading to proactive strategies. For instance, a healthcare provider can anticipate patient needs and allocate resources accordingly, which is essential in real time etl processes. Rapid Innovation offers MLOps consulting services to help organizations optimize their real-time data processing capabilities. Additionally, the importance of data annotation services in enhancing AI and machine learning models cannot be overlooked.
3.5. Data Storage Solutions
Data storage solutions are essential for managing the vast amounts of data generated by organizations. Choosing the right storage solution can significantly impact data accessibility, security, and cost.
- Types of storage: Â
- On-premises storage: Physical servers located within the organization, offering control but requiring maintenance. This option is suitable for businesses with strict data compliance requirements.
- Cloud storage: Services like Amazon S3, Google Cloud Storage, and Microsoft Azure provide scalable and flexible storage options, allowing businesses to pay only for what they use.
- Hybrid storage: Combines on-premises and cloud solutions, allowing businesses to optimize costs and performance while maintaining control over sensitive data.
- Considerations for selection: Â
- Scalability: The ability to grow with the organization’s data needs is crucial for long-term success.
- Security: Ensuring data is protected from breaches and unauthorized access is paramount, especially in industries like finance and healthcare.
- Cost-effectiveness: Balancing performance with budget constraints helps organizations maximize their ROI.
- Accessibility: Ensuring data can be easily accessed by authorized users enhances operational efficiency.
- Emerging technologies: Â
- Object storage: Ideal for unstructured data, offering high scalability and durability, which is essential for big data applications.
- Data lakes: Centralized repositories that allow for the storage of structured and unstructured data at scale, enabling advanced analytics and machine learning initiatives.
4. AI and Machine Learning Implementation
AI and machine learning (ML) are transforming how businesses operate by enabling data-driven decision-making and automating processes. Implementing these technologies can lead to significant competitive advantages.
- Key components of implementation: Â
- Data collection: Gathering high-quality data is crucial for training AI models. Rapid Innovation assists clients in establishing robust data pipelines to ensure data integrity.
- Model selection: Choosing the right algorithms based on the specific use case, such as supervised or unsupervised learning, is essential for achieving desired outcomes.
- Training and validation: Training models on historical data and validating their performance to ensure accuracy is a critical step in the implementation process.
- Applications of AI and ML: Â
- Predictive analytics: Forecasting trends and behaviors to inform business strategies, such as predicting customer churn and optimizing marketing efforts.
- Natural language processing (NLP): Enhancing customer interactions through chatbots and sentiment analysis, leading to improved customer engagement and satisfaction.
- Image recognition: Automating quality control in manufacturing or enhancing security systems, which can significantly reduce operational costs.
- Challenges in implementation: Â
- Data quality: Ensuring the data used is clean and relevant for accurate model training is a challenge that Rapid Innovation helps clients overcome through data cleansing and preprocessing techniques.
- Integration: Seamlessly incorporating AI solutions into existing systems and workflows is vital for maximizing the benefits of AI technologies.
- Talent acquisition: Finding skilled professionals who can develop and manage AI initiatives is a common hurdle, and Rapid Innovation offers consulting services to bridge this gap.
- Future trends: Â
- Increased automation: More processes will be automated, reducing human intervention and increasing efficiency.
- Ethical AI: Growing focus on responsible AI practices to mitigate bias and ensure fairness will shape the future of AI development.
- Edge computing: Processing data closer to the source to improve response times and reduce latency will become increasingly important as IoT devices proliferate.
By leveraging Rapid Innovation's expertise in real-time data processing, including real time stream processing and real time data ingestion, data storage solutions, and AI implementation, organizations can achieve their business goals efficiently and effectively, ultimately leading to greater ROI.
4.1. Model Selection

‍
Model selection is a critical step in the machine learning process. It involves choosing the most appropriate algorithm or model to solve a specific problem based on the data available. The right model can significantly impact the performance and accuracy of predictions, ultimately leading to greater ROI for your business. Factors to consider during model selection include:
- Nature of the data: structured vs. unstructured
- Size of the dataset
- Complexity of the problem
- Required interpretability of the model
- Computational resources available
Choosing the right model often requires experimentation and validation to ensure that it generalizes well to unseen data. At Rapid Innovation, we leverage our expertise to guide clients through this process, ensuring that the selected model aligns with their business objectives and maximizes efficiency. This includes considerations for machine learning features selection and various machine learning model selection strategies.
4.1.1. Supervised Learning Approaches
Supervised learning is a type of machine learning where the model is trained on labeled data. This means that the input data is paired with the correct output, allowing the model to learn the relationship between the two. Common supervised learning approaches include:
- Regression: Used for predicting continuous values. Examples include linear regression and polynomial regression.
- Classification: Used for predicting categorical outcomes. Examples include logistic regression, decision trees, and support vector machines (SVM).
- Ensemble Methods: Techniques like random forests and gradient boosting combine multiple models to improve accuracy and robustness.
Key characteristics of supervised learning include:
- Requires a labeled dataset for training.
- Performance can be evaluated using metrics like accuracy, precision, recall, and F1 score.
- Suitable for problems where historical data is available and the relationship between input and output is known.
By employing supervised learning techniques, Rapid Innovation has helped clients in various industries enhance their predictive capabilities, leading to improved decision-making and increased profitability. This often involves choosing a machine learning model that best fits the data and problem context.
4.1.2. Unsupervised Learning Methods
Unsupervised learning, in contrast, deals with unlabeled data. The model attempts to learn the underlying structure or distribution of the data without any explicit guidance on what the output should be. Common unsupervised learning methods include:
- Clustering: Groups similar data points together. Examples include k-means clustering and hierarchical clustering.
- Dimensionality Reduction: Reduces the number of features while preserving essential information. Techniques include Principal Component Analysis (PCA) and t-distributed Stochastic Neighbor Embedding (t-SNE).
- Anomaly Detection: Identifies unusual data points that do not conform to expected patterns. This is useful in fraud detection and network security.
Key characteristics of unsupervised learning include:
- Does not require labeled data, making it useful for exploratory data analysis.
- Performance evaluation can be challenging since there are no predefined labels.
- Suitable for discovering hidden patterns or intrinsic structures in data.
Both supervised and unsupervised learning approaches have their unique advantages and applications. The choice between them depends on the specific problem, the nature of the data, and the desired outcomes. Rapid Innovation's tailored approach ensures that clients can effectively harness these methodologies to uncover insights and drive strategic initiatives, ultimately achieving their business goals efficiently and effectively. This includes model evaluation and selection in machine learning, as well as adaptive deep learning model selection on embedded systems, ensuring that the best practices in model selection and validation in machine learning are followed. For a comprehensive understanding of these concepts, you can refer to machine learning.
4.1.3. Deep Learning Models
Deep learning models are a subset of machine learning that utilize neural networks with multiple layers to analyze various forms of data. These models have gained immense popularity due to their ability to automatically learn representations from raw data, making them particularly effective for complex tasks.
- Neural Networks: Deep learning primarily relies on artificial neural networks, which are designed to mimic the way the human brain processes information. At Rapid Innovation, we leverage these networks, including deep neural networks and deep belief networks, to create tailored solutions that enhance decision-making processes for our clients.
- Convolutional Neural Networks (CNNs): These are particularly effective for image processing tasks, as they can capture spatial hierarchies in images. CNNs, also referred to as convolutional neural nets, are widely used in applications such as facial recognition and object detection. By implementing CNNs, we have helped clients in retail optimize their inventory management through visual recognition systems.
- Recurrent Neural Networks (RNNs): RNNs are designed for sequential data, making them ideal for tasks like natural language processing and time series analysis. They can remember previous inputs, which is crucial for understanding context in language. Our expertise in recurrent neural networks, including RNN recurrent neural networks, has enabled clients in the finance sector to enhance their predictive analytics for stock market trends.
- Transfer Learning: This technique allows models trained on one task to be adapted for another, significantly reducing the amount of data and time required for training. For instance, a model trained on a large dataset can be fine-tuned for a specific application, enhancing performance. Rapid Innovation has successfully utilized transfer learning to accelerate project timelines for clients in healthcare, improving diagnostic accuracy with limited data.
- Applications: Deep learning models, including convolutional networks and deep neural nets, are used in various fields, including healthcare for disease diagnosis, finance for fraud detection, and autonomous vehicles for navigation. Our tailored deep learning solutions have consistently delivered greater ROI for clients by streamlining operations and enhancing service delivery. For advanced solutions, consider our transformer model development and learn more about the types of artificial neural networks.
4.1.4. Hybrid Approaches
Hybrid approaches combine different machine learning techniques to leverage the strengths of each method. This can lead to improved performance and robustness in various applications.
- Ensemble Learning: This technique involves combining multiple models to produce a single output. Methods like bagging and boosting are common ensemble techniques that can enhance predictive accuracy. At Rapid Innovation, we implement ensemble learning to improve the reliability of our clients' predictive models.
- Combining Deep Learning with Traditional Methods: Hybrid models may integrate deep learning with traditional machine learning algorithms. For example, using deep learning for feature extraction followed by a traditional classifier can yield better results. Our clients have benefited from this approach, achieving higher accuracy in their data-driven decisions.
- Multi-Modal Learning: This approach involves integrating data from different sources or modalities, such as text, images, and audio. By combining these diverse data types, hybrid models can provide a more comprehensive understanding of the problem at hand. Rapid Innovation has successfully applied multi-modal learning in projects that require a holistic view of customer behavior, leading to improved marketing strategies.
- Applications: Hybrid approaches are particularly useful in fields like healthcare, where combining clinical data with imaging data can lead to better diagnostic models. They are also used in recommendation systems, where user behavior and item characteristics are analyzed together. Our expertise in hybrid models has enabled clients to achieve significant improvements in their operational efficiency and customer satisfaction.
4.2. Feature Engineering
Feature engineering is the process of selecting, modifying, or creating new features from raw data to improve the performance of machine learning models. It plays a crucial role in the success of any machine learning project.
- Importance of Features: The quality of features directly impacts the model's ability to learn and make accurate predictions. Well-engineered features can significantly enhance model performance. At Rapid Innovation, we prioritize feature engineering to ensure our clients' models are robust and effective.
- Techniques: Â
- Feature Selection: This involves identifying the most relevant features from the dataset, which can reduce overfitting and improve model interpretability. Our team employs advanced techniques to ensure that only the most impactful features are utilized in model training.
- Feature Transformation: Techniques like normalization, scaling, and encoding categorical variables help in preparing data for modeling. For instance, scaling numerical features ensures that they contribute equally to the model. We guide our clients through these transformations to optimize their data for analysis.
- Creating New Features: Sometimes, combining existing features or deriving new ones can provide additional insights. For example, creating interaction terms or aggregating data can reveal hidden patterns. Our innovative feature creation strategies have led to enhanced model performance for our clients.
- Tools: Various tools and libraries, such as Scikit-learn and Pandas, facilitate feature engineering by providing functions for data manipulation and transformation. Rapid Innovation utilizes these tools to streamline the feature engineering process, ensuring efficiency and effectiveness.
- Impact on Model Performance: Effective feature engineering can lead to significant improvements in model accuracy and efficiency, making it a critical step in the machine learning pipeline. Our commitment to excellence in feature engineering has consistently resulted in higher ROI for our clients, enabling them to achieve their business goals with greater efficiency.
4.3. Model Training Process
The model training process is a critical phase in machine learning where the algorithm learns from the training data. This process involves several key steps:
- Data Preparation: Before training, data must be cleaned and preprocessed. This includes handling missing values, normalizing data, and encoding categorical variables. Proper data preparation ensures that the model can learn effectively, which is a service Rapid Innovation excels in, helping clients streamline their data for optimal model performance. This is particularly important in training machine learning models and preparing machine learning train data.
- Choosing a Model: Selecting the right algorithm is essential. Different models, such as linear regression, decision trees, or neural networks, have unique strengths and weaknesses. The choice depends on the problem type, data characteristics, and desired outcomes. Rapid Innovation provides expert consulting to guide clients in selecting the most suitable model for their specific needs, including training in machine learning and training neural networks.
- Splitting the Data: The dataset is typically divided into training, validation, and test sets. The training set is used to train the model, while the validation set helps tune hyperparameters. The test set evaluates the model's performance on unseen data. Our team ensures that this process is executed correctly to maximize the model's effectiveness, especially in machine learning training and testing.
- Training the Model: During training, the model learns by adjusting its parameters to minimize the error between predicted and actual outcomes. This is often done using optimization algorithms like gradient descent. Rapid Innovation employs advanced techniques to enhance the training process, ensuring clients achieve better results in less time. This includes methods like xgboost training and online machine learning, as well as fine-tuning language models.
- Monitoring Performance: Throughout the training process, it’s crucial to monitor performance metrics such as accuracy, precision, recall, and loss. This helps in understanding how well the model is learning and if adjustments are needed. Our analytics tools provide real-time insights, allowing clients to make informed decisions and adjustments swiftly, particularly during training and testing in machine learning.
4.4. Validation Techniques
Validation techniques are essential for assessing the performance of a machine learning model. They help ensure that the model generalizes well to new, unseen data. Key validation techniques include:
- Cross-Validation: This technique involves dividing the dataset into multiple subsets or folds. The model is trained on some folds and validated on others. K-fold cross-validation is a popular method where the data is split into K subsets, and the model is trained K times, each time using a different fold for validation.
- Holdout Method: In this simpler approach, the dataset is split into two parts: a training set and a test set. The model is trained on the training set and evaluated on the test set. This method is straightforward but can lead to variability in results based on how the data is split.
- Leave-One-Out Cross-Validation (LOOCV): This is a special case of cross-validation where each training set is created by leaving out one data point. This method is computationally expensive but provides a thorough evaluation of the model.
- Stratified Sampling: When dealing with imbalanced datasets, stratified sampling ensures that each class is represented proportionally in both training and validation sets. This helps in achieving a more reliable evaluation of the model's performance.
4.5. Model Optimization
Model optimization is the process of improving a machine learning model's performance. It involves fine-tuning various aspects of the model to achieve better accuracy and efficiency. Key strategies for model optimization include:
- Hyperparameter Tuning: Hyperparameters are settings that govern the training process, such as learning rate, batch size, and the number of layers in a neural network. Techniques like grid search, random search, and Bayesian optimization can be used to find the best hyperparameter values.
- Feature Selection: Selecting the most relevant features can significantly enhance model performance. Techniques such as recursive feature elimination, LASSO regression, and tree-based feature importance can help identify and retain the most impactful features.
- Regularization: To prevent overfitting, regularization techniques like L1 (LASSO) and L2 (Ridge) can be applied. These methods add a penalty for larger coefficients, encouraging simpler models that generalize better to new data.
- Ensemble Methods: Combining multiple models can lead to improved performance. Techniques like bagging, boosting, and stacking leverage the strengths of different models to create a more robust final prediction.
- Early Stopping: During training, monitoring the model's performance on a validation set can help prevent overfitting. If the performance starts to degrade, training can be halted early to retain the best model.
- Data Augmentation: In scenarios with limited data, data augmentation techniques can artificially expand the training dataset. This is particularly useful in image processing, where transformations like rotation, scaling, and flipping can create new training examples.
By implementing these strategies, practitioners can enhance the performance of their machine learning models, ensuring they are both accurate and efficient. Rapid Innovation is committed to helping clients navigate these processes, ultimately leading to greater ROI and successful project outcomes, including training in ML and exploring advanced concepts like transfer learning deep learning and federated learning models.
5. Failure Detection Mechanisms
Failure detection mechanisms are essential in various industries, particularly in manufacturing, IT, and engineering. These failure detection mechanisms help identify potential failures before they escalate into significant issues, ensuring operational efficiency and safety. Two critical components of failure detection mechanisms are early warning indicators and pattern recognition.
5.1. Early Warning Indicators
Early warning indicators (EWIs) are metrics or signals that provide advance notice of potential failures. They are crucial for proactive maintenance and risk management. By monitoring these early warning indicators, organizations can take corrective actions before a failure occurs.
- Types of Early Warning Indicators: Â
- Performance Metrics: These include key performance indicators (KPIs) that track the efficiency and effectiveness of systems. For example, a sudden drop in production output may signal equipment malfunction.
- Environmental Factors: Changes in temperature, humidity, or vibration levels can indicate potential failures in machinery. Monitoring these factors can help in predicting equipment breakdowns.
- User Feedback: Regular feedback from users can serve as an early warning. If multiple users report issues with a system, it may indicate an underlying problem that needs attention.
- Benefits of Early Warning Indicators: Â
- Proactive Maintenance: By identifying issues early, organizations can schedule maintenance before a failure occurs, reducing downtime.
- Cost Savings: Early detection can lead to significant cost savings by preventing extensive repairs or replacements.
- Improved Safety: Identifying potential failures early can enhance workplace safety by preventing accidents related to equipment failure.
5.2. Pattern Recognition

‍
Pattern recognition involves analyzing data to identify trends or anomalies that may indicate potential failures. This technique is increasingly used in various fields, including machine learning, data analytics, and predictive maintenance.
- How Pattern Recognition Works: Â
- Data Collection: Organizations collect data from various sources, such as sensors, logs, and user interactions. This data serves as the foundation for pattern recognition.
- Algorithm Application: Advanced algorithms analyze the collected data to identify patterns or trends. Machine learning models can be trained to recognize normal operating conditions and flag deviations.
- Anomaly Detection: When the system detects a pattern that deviates from the norm, it raises an alert. This can indicate a potential failure that requires further investigation.
- Applications of Pattern Recognition: Â
- Predictive Maintenance: By recognizing patterns in equipment performance, organizations can predict when maintenance is needed, reducing unexpected breakdowns.
- Quality Control: In manufacturing, pattern recognition can help identify defects in products by analyzing production data, ensuring higher quality standards.
- Fraud Detection: In finance, pattern recognition is used to detect fraudulent activities by identifying unusual transaction patterns.
- Benefits of Pattern Recognition: Â
- Enhanced Decision-Making: By providing insights into potential failures, pattern recognition aids in informed decision-making.
- Increased Efficiency: Organizations can optimize operations by addressing issues before they escalate, leading to improved productivity.
- Data-Driven Insights: Pattern recognition leverages data analytics to provide actionable insights, enhancing overall operational effectiveness.
In conclusion, failure detection mechanisms, particularly early warning indicators and pattern recognition, play a vital role in maintaining operational efficiency and safety across various industries. By implementing these failure detection mechanisms, organizations can proactively address potential failures, leading to significant cost savings and improved performance. At Rapid Innovation, we leverage our expertise in AI to develop tailored solutions that enhance these mechanisms, ensuring our clients achieve greater ROI through improved operational resilience and efficiency. For more information on how we can assist you, check out our computer vision software development services.
5.3. Anomaly Detection
Anomaly detection is a critical process in various fields, including cybersecurity, finance, and healthcare. It involves identifying patterns in data that do not conform to expected behavior. This technique is essential for early detection of potential issues, fraud, or system failures, enabling organizations to respond proactively.
- Definition: Anomaly detection refers to the identification of rare items, events, or observations that raise suspicions by differing significantly from the majority of the data.
- Techniques: Common methods include statistical tests, machine learning algorithms, and clustering techniques, all of which can be tailored to specific industry needs. Anomaly detection methods can also include outlier detection algorithms and statistical anomaly detection.
- Applications: Â
- Fraud detection in banking transactions, where Rapid Innovation's AI solutions can analyze transaction patterns to flag suspicious activities in real-time.
- Intrusion detection in network security, utilizing machine learning to identify unusual access patterns that may indicate a breach, including network traffic anomaly detection.
- Monitoring patient health data for unusual patterns, allowing healthcare providers to intervene before critical issues arise, which can be enhanced through anomaly detection for data quality.
- Importance: Early detection of anomalies can prevent significant losses and enhance decision-making processes, ultimately leading to greater ROI for organizations. Techniques such as anomaly detection using Python and scikit learn outlier detection can streamline this process.
- Challenges: Â
- High false positive rates can lead to unnecessary investigations, which can be mitigated through advanced model training and refinement.
- The need for large datasets to train models effectively, a challenge that Rapid Innovation addresses by leveraging synthetic data generation techniques.
- Variability in data can complicate the detection process, necessitating continuous model updates and monitoring, particularly in outlier detection time series.
5.4. Failure Classification
Failure classification is the process of categorizing failures based on their characteristics and causes. This is crucial for understanding the nature of failures and implementing effective solutions.
- Definition: Failure classification involves analyzing failures to determine their type, severity, and potential impact on operations.
- Types of Failures: Â
- Hard failures: Complete system breakdowns requiring immediate attention.
- Soft failures: Partial failures that may not halt operations but can degrade performance.
- Methods: Â
- Root cause analysis (RCA) to identify underlying issues.
- Failure mode and effects analysis (FMEA) to assess potential failure modes.
- Benefits: Â
- Improved maintenance strategies by understanding failure patterns, which can be enhanced through AI-driven predictive analytics.
- Enhanced safety by identifying critical failure points, allowing organizations to prioritize interventions.
- Cost reduction through targeted interventions, ultimately leading to improved operational efficiency.
- Challenges: Â
- Data collection can be time-consuming and complex, a challenge that Rapid Innovation can help streamline through automated data gathering solutions.
- Classifying failures accurately requires expertise and experience, which our team of specialists provides.
- Continuous monitoring is necessary to keep classification systems updated, ensuring that organizations remain resilient against emerging threats.
5.5. Risk Assessment
Risk assessment is a systematic process of evaluating potential risks that could negatively impact an organization. It is essential for effective risk management and decision-making.
- Definition: Risk assessment involves identifying, analyzing, and evaluating risks to determine their potential impact and likelihood.
- Steps in Risk Assessment: Â
- Risk identification: Recognizing potential risks that could affect the organization.
- Risk analysis: Evaluating the likelihood and impact of identified risks.
- Risk evaluation: Comparing estimated risks against risk criteria to determine significance.
- Importance: Â
- Helps organizations prioritize risks based on their potential impact, enabling more strategic resource allocation.
- Facilitates informed decision-making and resource allocation, ultimately enhancing operational resilience.
- Enhances compliance with regulations and standards, reducing the risk of penalties and reputational damage.
- Tools and Techniques: Â
- Qualitative assessments for subjective evaluation.
- Quantitative assessments for numerical analysis.
- Risk matrices to visualize risk levels, which can be integrated into AI-driven dashboards for real-time monitoring.
- Challenges: Â
- Dynamic environments can lead to rapidly changing risk profiles, necessitating agile risk management strategies.
- Subjectivity in risk evaluation can result in inconsistencies, which can be minimized through standardized AI models.
- Requires ongoing monitoring and updating to remain effective, a service that Rapid Innovation can provide through continuous support and system enhancements.
5.6. Prediction Confidence Scoring
Prediction confidence scoring is a critical aspect of machine learning and data analytics that helps users understand the reliability of predictions made by models. This scoring system quantifies the certainty of a model's output, allowing stakeholders to make informed decisions based on the level of confidence associated with each prediction. Confidence scores are typically expressed as probabilities, ranging from 0 to 1. A higher score indicates greater confidence in the prediction, while a lower score suggests uncertainty. This scoring can be particularly useful in applications such as finance, healthcare, and marketing, where decisions based on predictions can have significant consequences.
Incorporating prediction confidence scoring into machine learning models can enhance their usability by:
- Providing transparency: Users can see how confident the model is in its predictions, which can help in assessing risk.
- Enabling better decision-making: Stakeholders can prioritize actions based on the confidence level of predictions.
- Improving model performance: By analyzing confidence scores, data scientists can identify areas where the model may need improvement or retraining.
For example, in a healthcare setting, a model predicting patient outcomes might provide a confidence score of 0.85 for a specific diagnosis. This high score indicates a strong likelihood of accuracy, allowing healthcare professionals to act with greater assurance. Conversely, a score of 0.45 would suggest caution, prompting further investigation before making treatment decisions.
6. Real-time Monitoring System
A real-time monitoring system is essential for organizations that rely on continuous data analysis and immediate feedback. This system allows businesses to track performance metrics, detect anomalies, and respond to changes in real-time, ensuring that they remain agile and competitive. Real-time monitoring systems can be applied across various industries, including finance, manufacturing, and e-commerce. They utilize advanced technologies such as IoT devices, cloud computing, and big data analytics to gather and process data instantaneously. The benefits of implementing a real-time monitoring system include enhanced operational efficiency, improved customer satisfaction, and proactive risk management.
Key components of a real-time monitoring system include:
- Data collection: Continuous data gathering from various sources, such as sensors, user interactions, and transaction logs.
- Data processing: Immediate analysis of incoming data to identify trends, patterns, and anomalies.
- Alerting mechanisms: Automated notifications to stakeholders when specific thresholds are met or exceeded, enabling quick responses to potential issues.
By leveraging a real-time monitoring system, organizations can make data-driven decisions that enhance their overall performance and responsiveness to market changes.
6.1. Dashboard Design
Dashboard design is a crucial element of any real-time monitoring system, as it serves as the primary interface for users to visualize and interact with data. An effective dashboard provides a clear, concise overview of key performance indicators (KPIs) and other relevant metrics, enabling users to quickly assess the state of their operations. A well-designed dashboard should prioritize usability and accessibility, ensuring that users can easily navigate and interpret the information presented. Key features of an effective dashboard include:
- Intuitive layout: Organizing information logically to facilitate quick understanding.
- Visualizations: Utilizing charts, graphs, and other visual elements to represent data clearly.
- Customization: Allowing users to tailor the dashboard to their specific needs and preferences.
When designing a dashboard, consider the following best practices:
- Identify the target audience: Understand who will be using the dashboard and what information is most relevant to them.
- Focus on key metrics: Highlight the most important KPIs that align with organizational goals.
- Ensure real-time updates: Provide users with the latest data to support timely decision-making.
By implementing these design principles, organizations can create dashboards that not only enhance data visibility but also empower users to make informed decisions based on real-time insights.
At Rapid Innovation, we specialize in integrating these advanced AI capabilities into your business processes, ensuring that you achieve greater ROI through informed decision-making and enhanced operational efficiency. Our expertise in machine learning and real-time data analytics positions us as a trusted partner in your journey towards digital transformation. For tailored solutions, explore our custom AI model development services and learn more about AI agent market trend predictor.
6.2. Alert Configuration
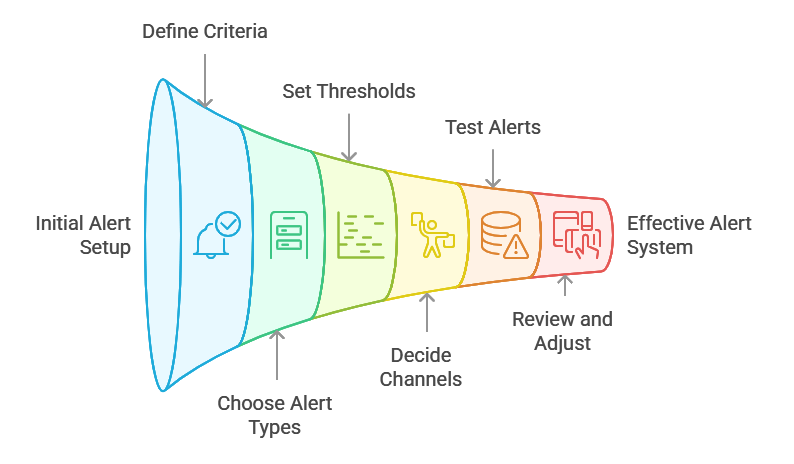
‍
Alert configuration is a critical aspect of system monitoring and management. It involves setting up notifications that inform administrators about specific events or thresholds that have been reached within a system, including system monitoring alerts. Proper alert configuration ensures that issues are identified and addressed promptly, minimizing downtime and maintaining system performance.
- Define alert criteria: Establish clear parameters for what constitutes an alert. This could include error rates, response times, or resource usage levels.
- Choose alert types: Determine the types of alerts needed, such as critical, warning, or informational alerts. Each type should have a different level of urgency and response protocol.
- Set thresholds: Configure thresholds for alerts based on historical data and expected performance. This helps in avoiding false positives and ensures that alerts are meaningful.
- Notification channels: Decide on the channels through which alerts will be sent, such as email, SMS, or integration with monitoring tools like Slack or PagerDuty.
- Test alerts: Regularly test the alert system to ensure that notifications are sent correctly and received by the intended recipients.
- Review and adjust: Continuously review alert configurations to adapt to changing system requirements and performance metrics.
6.3. Performance Metrics
Performance metrics are essential for evaluating the efficiency and effectiveness of a system. They provide quantitative data that helps in understanding how well a system is functioning and where improvements can be made.
- Key performance indicators (KPIs): Identify the most relevant KPIs for your system, such as uptime, response time, and transaction throughput.
- Data collection: Implement tools and processes for collecting performance data. This can include application performance monitoring (APM) tools, log analysis, and user feedback.
- Benchmarking: Compare performance metrics against industry standards or historical data to identify areas for improvement.
- Visualization: Use dashboards and visual reports to present performance metrics in an easily digestible format. This aids in quick decision-making and trend analysis.
- Regular reviews: Schedule regular reviews of performance metrics to ensure that the system is meeting its goals and to identify any potential issues early on.
6.4. Resource Utilization
Resource utilization refers to how effectively a system uses its available resources, such as CPU, memory, disk space, and network bandwidth. Monitoring resource utilization is vital for optimizing performance and ensuring that systems run efficiently.
- Monitor resource usage: Use monitoring tools to track resource utilization in real-time. This helps in identifying bottlenecks and underutilized resources.
- Analyze trends: Look for patterns in resource usage over time. Understanding peak usage times can help in capacity planning and resource allocation.
- Optimize configurations: Adjust system configurations based on resource utilization data. This may involve scaling resources up or down, depending on demand.
- Implement resource limits: Set limits on resource usage for applications to prevent any single application from monopolizing system resources.
- Plan for scalability: Ensure that the system can scale resources as needed to accommodate growth or increased demand. This may involve cloud solutions or additional hardware.
- Regular audits: Conduct regular audits of resource utilization to identify inefficiencies and areas for improvement. This can lead to cost savings and enhanced performance.
At Rapid Innovation, we leverage advanced AI technologies to enhance alert configuration, performance metrics, and resource utilization. By implementing AI agents for IT resource optimization, we help clients achieve greater ROI through proactive issue resolution, optimized resource management, and data-driven decision-making. Our expertise ensures that your systems not only perform efficiently but also adapt to evolving business needs, ultimately driving your success in a competitive landscape.
6.5. System Health Monitoring
System health monitoring is a critical aspect of IT infrastructure management. It involves continuously tracking the performance and status of various system components to ensure optimal operation. Effective system health monitoring can help identify potential issues before they escalate into significant problems. Key components of system health monitoring include:
- Performance Metrics: Monitoring CPU usage, memory consumption, disk I/O, and network traffic to assess system performance.
- Availability Checks: Ensuring that all system components are operational and accessible.
- Error Tracking: Identifying and logging errors or anomalies in system behavior.
- Resource Utilization: Analyzing how resources are being used to prevent bottlenecks.
The benefits of system health monitoring are significant:
- Proactive Issue Resolution: Early detection of issues allows for timely intervention, reducing downtime.
- Improved Performance: Continuous monitoring helps optimize resource allocation and system performance.
- Enhanced Security: Monitoring can detect unauthorized access or unusual activity, contributing to overall system security.
Several tools are available for system health monitoring:
- Nagios: An open-source tool that provides comprehensive monitoring capabilities.
- Zabbix: Offers real-time monitoring and alerting for various system metrics.
- Prometheus: A powerful monitoring system that collects and stores metrics as time series data.
At Rapid Innovation, we leverage advanced AI technologies to enhance system health monitoring for our clients. By integrating machine learning algorithms, we can predict potential system failures before they occur, allowing businesses to take proactive measures. This not only minimizes downtime but also significantly improves the return on investment (ROI) by ensuring that systems operate at peak efficiency. Additionally, we utilize remote patient monitoring systems and health monitoring systems to ensure the well-being of our clients' IT infrastructure. For more insights on how AI agents can contribute to proactive system monitoring, check out our article on AI agents for proactive system monitoring.
7. Response and Automation
Response and automation in IT management refer to the processes and tools used to react to system alerts and automate routine tasks. This approach enhances efficiency and reduces the likelihood of human error. The importance of response and automation includes:
- Speed: Automated responses can significantly reduce the time taken to address issues.
- Consistency: Automation ensures that responses are uniform and follow best practices.
- Resource Optimization: Frees up IT staff to focus on more strategic tasks rather than routine monitoring.
Key elements of response and automation are:
- Incident Response Plans: Clearly defined procedures for addressing various types of incidents.
- Automation Tools: Software that can execute predefined actions in response to specific alerts.
- Integration with Monitoring Systems: Ensuring that automation tools work seamlessly with monitoring solutions for real-time responses.
Examples of automation in IT include:
- Self-healing Systems: Automatically restarting services or reallocating resources when issues are detected.
- Scheduled Maintenance: Automating routine updates and patches to minimize downtime.
- Alerting Systems: Sending notifications to relevant personnel when specific thresholds are met.
7.1. Alert Prioritization
Alert prioritization is the process of categorizing alerts based on their severity and potential impact on the system. This practice is essential for effective incident management and ensures that critical issues are addressed promptly. Factors influencing alert prioritization include:
- Severity Level: Assessing the potential impact of an alert on system performance and availability.
- Frequency of Occurrence: Determining how often an alert is triggered can help prioritize recurring issues.
- Business Impact: Evaluating how an alert affects business operations and user experience.
The benefits of alert prioritization are:
- Efficient Resource Allocation: Ensures that IT teams focus on the most critical issues first.
- Reduced Alert Fatigue: Helps prevent overwhelming staff with low-priority alerts, allowing them to concentrate on significant problems.
- Improved Response Times: Prioritizing alerts leads to faster resolution of high-impact incidents.
Strategies for effective alert prioritization include:
- Use of Triage Systems: Implementing a triage process to categorize alerts based on predefined criteria.
- Machine Learning Algorithms: Leveraging AI to analyze historical data and predict the severity of alerts.
- Regular Review and Adjustment: Continuously assessing and refining alert thresholds and prioritization criteria based on evolving system dynamics.
By implementing robust system health monitoring, effective response and automation strategies, and a well-defined alert prioritization process, organizations can significantly enhance their IT operations, ensuring higher availability and performance of their systems. At Rapid Innovation, we are committed to helping our clients achieve these goals efficiently and effectively, ultimately driving greater ROI through our tailored solutions, including the integration of remote patient monitoring platforms and health monitoring systems.
7.2. Automated Response Protocols
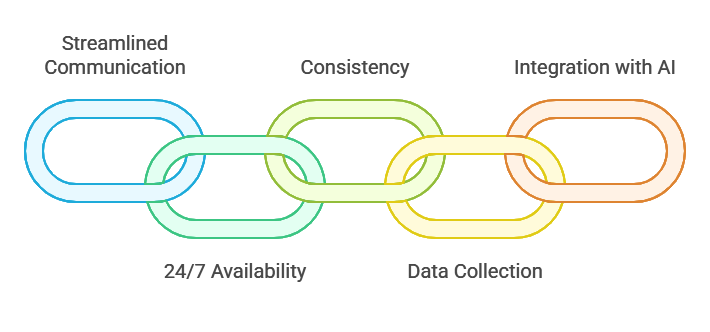
‍
Automated response protocols, such as automated IVR systems and automated voice response systems, are essential for enhancing efficiency and ensuring timely communication in various sectors, including customer service, IT support, and emergency management. These protocols utilize technology to automate responses to common inquiries or situations, reducing the need for human intervention.
- Streamlined Communication: Automated responses can handle frequently asked questions, allowing human agents to focus on more complex issues, thereby improving overall service quality. Automated IVR and automated voice response can significantly aid in this process.
- 24/7 Availability: Automated systems, including automated call response systems, can provide round-the-clock support, ensuring that users receive assistance at any time, which is crucial for maintaining customer satisfaction and loyalty.
- Consistency: Automated responses ensure that information is delivered uniformly, reducing the risk of human error and enhancing the reliability of communication. Automated voice response IVR systems contribute to this consistency.
- Data Collection: Automated systems can gather data on user interactions, helping organizations analyze trends and improve services, ultimately leading to better decision-making. For instance, osticket auto response can be utilized to streamline data collection.
- Integration with AI: Advanced automated response systems, such as automated IVR call solutions, can leverage artificial intelligence to provide personalized responses based on user behavior and preferences, enhancing user experience and engagement. For more advanced solutions, consider our natural language processing solutions and explore generative AI in customer service.
7.3. Maintenance Scheduling
Maintenance scheduling is a critical aspect of operational efficiency in various industries, including manufacturing, transportation, and facility management. Proper scheduling ensures that equipment and systems are maintained regularly, minimizing downtime and extending their lifespan.
- Predictive Maintenance: Utilizing data analytics to predict when maintenance should occur can prevent unexpected failures and reduce costs, leading to significant savings and improved operational efficiency.
- Resource Optimization: Effective scheduling allows for better allocation of resources, ensuring that maintenance tasks do not interfere with production schedules, thus maximizing productivity.
- Compliance: Regular maintenance helps organizations comply with safety regulations and industry standards, reducing the risk of penalties and enhancing organizational reputation.
- Improved Safety: Scheduled maintenance can identify potential hazards before they become serious issues, enhancing workplace safety and protecting employees.
- Documentation: Keeping detailed records of maintenance activities helps organizations track performance and plan future maintenance needs, facilitating continuous improvement.
7.4. Resource Allocation
Resource allocation is the process of distributing available resources—such as personnel, equipment, and finances—effectively to achieve organizational goals. Proper resource allocation is vital for maximizing productivity and minimizing waste.
- Prioritization: Identifying critical projects and allocating resources accordingly ensures that essential tasks receive the attention they need, driving project success.
- Flexibility: Effective resource allocation allows organizations to adapt to changing circumstances, such as unexpected demand or project delays, ensuring resilience in operations.
- Cost Efficiency: By optimizing resource use, organizations can reduce operational costs and improve their bottom line, contributing to greater financial health.
- Performance Monitoring: Regularly assessing resource allocation helps organizations identify areas for improvement and make informed decisions, fostering a culture of accountability and excellence.
- Collaboration: Encouraging collaboration among teams can lead to more effective resource sharing and utilization, enhancing overall productivity and innovation.
At Rapid Innovation, we leverage these principles to help our clients implement automated response protocols, such as automated IVR and automated voice response systems, optimize maintenance scheduling, and enhance resource allocation, ultimately driving greater ROI and achieving their business goals efficiently and effectively.
7.5. Incident Management Integration
Incident management integration is a crucial aspect of IT service management (ITSM) that ensures seamless coordination between various IT processes and tools. This integration helps organizations respond to incidents effectively, minimizing downtime and enhancing service quality.
- Streamlined Communication: Integrating incident management with other ITSM processes, such as problem management and change management, facilitates better communication among teams. This ensures that all stakeholders are informed about incidents and their resolutions, leading to quicker response times. Tools like jira incident management and incident management in jira can enhance this communication.
- Automation of Processes: Automation tools can be integrated to handle repetitive tasks, such as ticket creation and notifications. This reduces manual effort and allows IT teams to focus on more complex issues, ultimately leading to greater efficiency and cost savings. For instance, using slack incident management can automate notifications and updates.
- Enhanced Reporting and Analytics: Integration allows for comprehensive data collection across various systems, enabling better reporting and analytics. Organizations can track incident trends, response times, and resolution effectiveness, leading to continuous improvement and informed decision-making. Solutions like zendesk incident management and freshdesk incident management provide valuable insights through their reporting features.
- Improved User Experience: A well-integrated incident management system provides users with a single point of contact for reporting issues. This enhances user satisfaction as they receive timely updates and resolutions, fostering a positive relationship between IT and end-users. Utilizing tools like jira service desk incident management can streamline this process.
- Compliance and Risk Management: Integration helps ensure that incident management processes comply with industry standards and regulations. It also aids in identifying potential risks associated with incidents, allowing for proactive measures that protect the organization’s interests. For example, incident management in salesforce can help maintain compliance with data regulations. Additionally, organizations can explore supply chain innovation to enhance their operational efficiency.
8. Implementation Strategy
An effective implementation strategy is essential for the successful deployment of any ITSM solution, including incident management systems. A well-structured approach ensures that the integration aligns with organizational goals and meets user needs.
- Define Objectives: Clearly outline the goals of the incident management integration. Objectives may include reducing incident response times, improving service quality, or enhancing user satisfaction, all of which contribute to a higher return on investment (ROI). Consider using incident management integration with opsgenie incidents for better tracking.
- Stakeholder Engagement: Involve key stakeholders from various departments early in the process. Their input can provide valuable insights into the specific needs and challenges faced by different teams, ensuring that the solution is tailored to the organization. Engaging with teams using slack incident management bot can enhance collaboration.
- Develop a Roadmap: Create a detailed implementation roadmap that outlines the steps, timelines, and resources required. This roadmap should include milestones to track progress and ensure accountability, facilitating a smooth transition.
- Resource Allocation: Assess the resources needed for implementation, including personnel, technology, and budget. Ensure that the necessary tools and technologies are in place to support the integration, maximizing the potential for success.
- Training and Support: Provide training for staff on the new incident management processes and tools. Ongoing support should be available to address any issues that arise during and after implementation, ensuring that teams are well-equipped to utilize the new system effectively.
- Monitor and Evaluate: After implementation, continuously monitor the performance of the incident management system. Evaluate its effectiveness against the defined objectives and make adjustments as necessary to optimize performance and ROI.
8.1. Prerequisites Assessment

‍
Before implementing an incident management system, conducting a prerequisites assessment is vital. This assessment helps identify the necessary conditions and resources required for a successful integration.
- Current System Evaluation: Assess the existing ITSM tools and processes in place. Identify gaps and areas for improvement that the new system should address, ensuring that the integration is built on a solid foundation. Consider the integration of incident management servicenow docs for comprehensive evaluation.
- Infrastructure Readiness: Evaluate the current IT infrastructure to ensure it can support the new incident management system. Consider factors such as server capacity, network bandwidth, and software compatibility to avoid potential bottlenecks.
- User Requirements: Gather input from end-users to understand their needs and expectations. This can help tailor the incident management system to better serve the organization, enhancing user satisfaction and engagement.
- Compliance Considerations: Review any regulatory or compliance requirements that may impact the implementation. Ensure that the new system adheres to industry standards and best practices, mitigating risks associated with non-compliance.
- Change Management Readiness: Assess the organization’s readiness for change, including the culture and willingness to adopt new processes. Identify potential resistance and develop strategies to address it, fostering a supportive environment for the integration.
- Budget and Resource Availability: Determine the budget available for the implementation and ongoing maintenance of the incident management system. Ensure that sufficient resources, including personnel and technology, are allocated for a successful rollout, maximizing the potential for a strong ROI.
8.2. Pilot Program Design
A pilot program is a crucial step in testing new initiatives before full-scale implementation. The design of a pilot program should be methodical and strategic to ensure that it effectively evaluates the feasibility and impact of the proposed changes.
- Define objectives: Clearly outline what the pilot aims to achieve. This could include testing new technologies, processes, or methodologies that align with your business goals.
- Select a target group: Choose a representative sample of users or stakeholders who will participate in the pilot. This group should reflect the diversity of the larger population to ensure comprehensive feedback.
- Develop a timeline: Establish a clear timeline for the pilot program, including start and end dates, milestones, and evaluation points to keep the project on track.
- Create evaluation criteria: Determine how success will be measured. This could involve quantitative metrics, such as performance data, and qualitative feedback from participants, which Rapid Innovation can help analyze using AI-driven insights.
- Plan for scalability: Consider how the pilot can be expanded or adapted based on the results. This includes identifying potential challenges and solutions for broader implementation, ensuring that your investment yields a greater ROI.
- Gather resources: Ensure that all necessary resources, including personnel, technology, and budget, are allocated for the pilot program, leveraging Rapid Innovation's expertise in resource optimization.
8.3. Deployment Phases
Deployment phases are essential for the successful rollout of any new initiative. A structured approach helps manage risks and ensures that all stakeholders are prepared for the changes.
- Phase 1: Preparation Â
- Conduct a needs assessment to identify gaps and requirements.
- Develop a detailed project plan outlining tasks, responsibilities, and timelines.
- Communicate with stakeholders to ensure alignment and buy-in.
- Phase 2: Pilot Implementation Â
- Launch the pilot program with the selected group.
- Monitor progress closely, collecting data and feedback throughout the process.
- Make adjustments as necessary based on real-time insights, utilizing AI tools for data analysis.
- Phase 3: Evaluation Â
- Analyze the data collected during the pilot phase.
- Assess whether the objectives were met and identify areas for improvement.
- Gather feedback from participants to understand their experiences and suggestions.
- Phase 4: Full-Scale Deployment Â
- Based on the evaluation, finalize the implementation plan for a broader rollout.
- Communicate the changes to all stakeholders, ensuring they understand the benefits and processes involved.
- Provide ongoing support and resources to facilitate a smooth transition, enhancing user adoption and satisfaction.
8.4. Training Requirements
Training is a vital component of any new initiative, ensuring that all users are equipped with the necessary skills and knowledge to adapt to changes.
- Identify training needs: Assess the skills gap among users to determine what training is required. This can be done through surveys, interviews, or performance evaluations.
- Develop training materials: Create comprehensive training resources, including manuals, online courses, and hands-on workshops. Ensure that materials are user-friendly and accessible.
- Schedule training sessions: Plan training sessions that accommodate the schedules of all participants. Consider offering multiple sessions to cater to different learning paces.
- Implement training: Conduct the training sessions, ensuring that participants engage with the material and have opportunities to ask questions.
- Evaluate training effectiveness: After training, assess participants' understanding and retention of the material. This can be done through quizzes, practical assessments, or feedback forms.
- Provide ongoing support: Establish a support system for users post-training, including access to resources, FAQs, and a help desk for troubleshooting.
By focusing on these key areas—pilot program design, deployment phases, and training requirements—organizations can enhance their chances of successful implementation and adoption of new initiatives, ultimately driving greater ROI and achieving business goals efficiently and effectively with the support of Rapid Innovation.
8.5. Change Management
Change management is a structured approach to transitioning individuals, teams, and organizations from a current state to a desired future state. It is essential for ensuring that changes are implemented smoothly and effectively, minimizing resistance and maximizing engagement.
- Importance of Change Management: Â
- Facilitates smooth transitions during organizational changes.
- Reduces resistance from employees by involving them in the process.
- Enhances communication and transparency throughout the organization.
- Key Components of Change Management: Â
- Leadership Support: Strong leadership is crucial for guiding the change process and providing direction.
- Stakeholder Engagement: Involving stakeholders early helps in understanding their concerns and gaining their support.
- Training and Development: Providing training ensures that employees have the necessary skills to adapt to new systems or processes.
- Change Management Models: Â
- ADKAR Model: Focuses on Awareness, Desire, Knowledge, Ability, and Reinforcement.
- Kotter’s 8-Step Process: Emphasizes creating urgency, forming a coalition, and anchoring new approaches in the culture.
- Challenges in Change Management: Â
- Resistance to change can stem from fear of the unknown or perceived threats to job security.
- Lack of communication can lead to misunderstandings and decreased morale.
- Insufficient training can result in employees feeling unprepared for new roles or technologies.
At Rapid Innovation, we understand that effective change management is critical for the successful implementation of AI solutions. By leveraging our expertise in change management strategies, we help organizations navigate the complexities of change, ensuring that employees are engaged and equipped to embrace new technologies. For instance, when implementing AI-driven analytics, we provide comprehensive training and support to minimize resistance and enhance user adoption, ultimately leading to greater ROI. Additionally, our AI customer service agent solutions are designed to streamline communication and improve customer interactions, further supporting change management efforts. You can learn more about our approach to change management and its real-world applications.
8.6. Success Metrics
Success metrics are critical for evaluating the effectiveness of initiatives and ensuring that organizational goals are met. They provide a framework for measuring progress and identifying areas for improvement.
- Importance of Success Metrics: Â
- Helps organizations track performance and make data-driven decisions.
- Provides accountability by establishing clear expectations and outcomes.
- Facilitates continuous improvement by identifying strengths and weaknesses.
- Types of Success Metrics: Â
- Quantitative Metrics: These include numerical data such as sales figures, customer satisfaction scores, and employee turnover rates.
- Qualitative Metrics: These involve subjective assessments, such as employee feedback and customer testimonials.
- Leading vs. Lagging Indicators: Leading indicators predict future performance, while lagging indicators reflect past performance.
- Developing Success Metrics: Â
- Align metrics with organizational goals to ensure relevance.
- Involve stakeholders in the development process to gain buy-in and diverse perspectives.
- Regularly review and adjust metrics to reflect changing business environments and objectives.
- Common Success Metrics: Â
- Return on Investment (ROI): Measures the profitability of an investment relative to its cost.
- Net Promoter Score (NPS): Assesses customer loyalty and satisfaction.
- Employee Engagement Scores: Evaluates the level of employee commitment and motivation.
At Rapid Innovation, we emphasize the importance of success metrics in our AI projects. By establishing clear KPIs aligned with business objectives, we enable organizations to track the effectiveness of their AI initiatives. For example, by measuring ROI on AI-driven customer service solutions, clients can see tangible improvements in efficiency and customer satisfaction, leading to enhanced business outcomes.
9. Industry-Specific Applications
Industry-specific applications refer to tailored solutions and practices that address the unique challenges and requirements of different sectors. Understanding these applications is crucial for organizations to remain competitive and effective.
- Importance of Industry-Specific Applications: Â
- Provides targeted solutions that enhance operational efficiency.
- Addresses regulatory and compliance requirements specific to the industry.
- Facilitates innovation by leveraging industry best practices.
- Examples of Industry-Specific Applications: Â
- Healthcare: Implementation of electronic health records (EHR) systems to improve patient care and streamline operations.
- Manufacturing: Use of lean manufacturing techniques to reduce waste and improve productivity.
- Retail: Adoption of omnichannel strategies to enhance customer experience and increase sales.
- Benefits of Industry-Specific Applications: Â
- Increases adaptability to market changes and customer demands.
- Enhances collaboration among stakeholders within the industry.
- Drives growth by leveraging industry trends and technologies.
- Challenges in Implementing Industry-Specific Applications: Â
- Resistance to change from employees accustomed to traditional practices.
- High costs associated with technology adoption and training.
- Need for ongoing support and maintenance to ensure effectiveness.
By focusing on change management strategies, success metrics, and industry-specific applications, organizations can navigate the complexities of their environments and achieve sustainable growth. Rapid Innovation is committed to providing tailored AI solutions that not only address these challenges but also drive significant ROI for our clients.
9.1. Manufacturing Equipment
Manufacturing equipment is essential for the production of goods across various industries, including original equipment manufacturer (OEM) sectors. The right machinery can significantly enhance efficiency, reduce costs, and improve product quality.
- Types of Manufacturing Equipment: Â
- CNC Machines: Computer Numerical Control machines automate the manufacturing process, allowing for precision and repeatability. Rapid Innovation can assist in integrating AI algorithms to optimize CNC operations, leading to reduced waste and increased throughput.
- 3D Printers: These machines create three-dimensional objects from digital files, revolutionizing prototyping and production. By leveraging AI, we can enhance the design process, enabling faster iterations and improved material usage.
- Robotics: Automated robots are increasingly used for tasks such as assembly, welding, and painting, improving speed and safety. Our expertise in AI can help in developing smarter robotic systems that adapt to changing production needs.
- Importance of Maintenance: Â
- Regular maintenance of manufacturing equipment is crucial to prevent breakdowns and ensure optimal performance. Predictive maintenance techniques, using IoT sensors, can help identify potential issues before they lead to costly downtime. Rapid Innovation can implement AI-driven predictive analytics to enhance maintenance schedules, ultimately reducing operational costs.
- Industry Trends: Â
- The rise of smart manufacturing, integrating IoT and AI, is transforming traditional manufacturing processes. Sustainability is becoming a priority, with manufacturers investing in energy-efficient equipment and processes. This includes medical equipment manufacturers and construction equipment manufacturers who are adapting to these trends. Rapid Innovation can guide clients in adopting these technologies, ensuring they stay competitive in a rapidly evolving market. For expert guidance, consider partnering with an AI consulting company like Rapid Innovation. Additionally, our AI agent for equipment performance analysis can provide valuable insights into optimizing manufacturing processes.
9.2. Data Center Infrastructure
Data center infrastructure is the backbone of modern IT operations, supporting everything from cloud computing to big data analytics. A well-designed data center ensures reliability, security, and scalability.
- Key Components: Â
- Servers: High-performance servers are essential for processing and storing data. Our AI solutions can optimize server utilization, leading to significant cost savings and improved performance.
- Networking Equipment: Routers, switches, and firewalls facilitate data transfer and protect against cyber threats. Rapid Innovation can enhance network security through AI-driven threat detection and response systems.
- Cooling Systems: Effective cooling solutions are necessary to maintain optimal operating temperatures and prevent overheating. We can implement AI-based monitoring systems to optimize cooling efficiency, reducing energy consumption.
- Importance of Redundancy: Â
- Redundant power supplies and backup systems are critical to ensure continuous operation during outages. Data centers often implement disaster recovery plans to safeguard against data loss. Rapid Innovation can assist in developing robust disaster recovery strategies powered by AI analytics.
- Trends in Data Center Design: Â
- Modular data centers are gaining popularity for their flexibility and scalability. The shift towards edge computing is driving the need for smaller, decentralized data centers closer to end-users. Our expertise can help clients design and implement these modern infrastructures effectively.
9.3. Transportation Systems
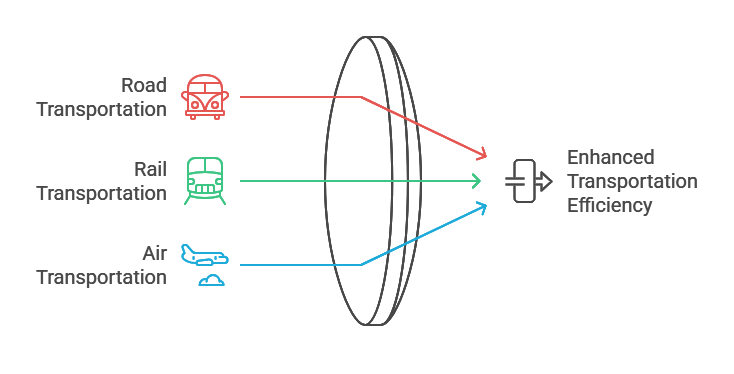
‍
Transportation systems are vital for the movement of goods and people, impacting economic growth and urban development. Efficient transportation networks enhance connectivity and reduce travel times.
- Types of Transportation Systems: Â
- Road Transportation: Includes vehicles such as trucks and buses, essential for local and regional distribution. Rapid Innovation can develop AI solutions for route optimization, reducing fuel consumption and improving delivery times.
- Rail Transportation: Trains are a cost-effective option for transporting large volumes of goods over long distances. Our AI-driven analytics can enhance scheduling and maintenance, leading to increased efficiency.
- Air Transportation: Air freight is the fastest method for shipping goods internationally, though it can be more expensive. We can help clients analyze and optimize their air freight operations using AI insights.
- Importance of Infrastructure Investment: Â
- Investing in transportation infrastructure can lead to improved safety, reduced congestion, and enhanced economic productivity. Public-private partnerships are increasingly being used to fund transportation projects. Rapid Innovation can provide strategic consulting to ensure these investments yield maximum returns.
- Emerging Trends: Â
- The rise of electric and autonomous vehicles is transforming the transportation landscape. Smart transportation systems, utilizing IoT and data analytics, are improving traffic management and reducing emissions. Rapid Innovation is at the forefront of these developments, helping clients implement cutting-edge solutions that drive efficiency and sustainability. This includes collaboration with heavy machinery manufacturers and healthcare equipment manufacturers to enhance logistics and supply chain operations.
9.4. Healthcare Devices
Healthcare devices are revolutionizing the medical field by integrating advanced technology to improve patient care and streamline operations. These devices range from wearable health monitors to sophisticated diagnostic equipment, including medical equipment store near me and medical equipment supplies.
- Wearable devices: Smartwatches and fitness trackers monitor vital signs such as heart rate, blood pressure, and oxygen levels. They provide real-time data to both patients and healthcare providers, enabling proactive health management.
- Telemedicine: Remote monitoring devices allow healthcare professionals to track patients' health from afar, which is particularly beneficial for chronic disease management where continuous monitoring is crucial. This includes access to medical equipment and supplies.
- Smart implants: Devices like pacemakers and insulin pumps are now equipped with IoT capabilities, allowing for remote adjustments and data collection. This enhances patient safety and treatment efficacy.
- Data analytics: Healthcare devices generate vast amounts of data that can be analyzed to identify trends, improve treatment protocols, and enhance patient outcomes. Rapid Innovation leverages AI-driven analytics to help healthcare providers make data-informed decisions, ultimately leading to better patient care and increased operational efficiency.
- Regulatory compliance: As healthcare devices become more advanced, they must adhere to strict regulations to ensure patient safety and data security. Rapid Innovation assists clients in navigating these regulatory landscapes, ensuring that their devices meet compliance standards while maximizing functionality.
The integration of IoT in healthcare devices is expected to grow significantly, with projections indicating that the global market for connected healthcare devices will reach $500 billion by 2025. This growth is supported by the availability of medical equipment supply store and durable medical equipment & medical supplies.
9.5. Industrial IoT
Industrial IoT (IIoT) refers to the use of IoT technology in manufacturing and industrial processes. It enhances operational efficiency, reduces costs, and improves safety across various sectors.
- Predictive maintenance: IIoT devices can monitor equipment performance in real-time, predicting failures before they occur. This minimizes downtime and extends the lifespan of machinery. Rapid Innovation employs AI algorithms to enhance predictive maintenance strategies, ensuring that clients can maintain optimal operational performance.
- Supply chain optimization: IoT sensors track inventory levels and shipments, providing real-time data that helps businesses manage their supply chains more effectively. Our consulting services help clients implement IIoT solutions that streamline their supply chain processes, leading to significant cost savings.
- Enhanced safety: Wearable IoT devices can monitor workers' health and safety conditions, alerting them to potential hazards in real-time. Rapid Innovation develops tailored solutions that prioritize worker safety while improving overall productivity.
- Energy management: IIoT solutions can optimize energy consumption in manufacturing processes, leading to significant cost savings and reduced environmental impact. Our expertise in AI-driven energy management systems allows clients to achieve greater sustainability and efficiency.
- Data-driven decision-making: The vast amount of data collected by IIoT devices allows companies to make informed decisions, improving overall productivity and efficiency. Rapid Innovation's data analytics capabilities empower clients to harness this data effectively, driving better business outcomes.
The IIoT market is projected to grow to $1.1 trillion by 2026, driven by advancements in technology and increasing demand for automation.
10. Advanced Features
Advanced features in technology are transforming how devices operate and interact with users. These features enhance functionality, improve user experience, and provide greater insights.
- Artificial Intelligence (AI): AI integration allows devices to learn from user behavior, making them more intuitive and responsive. This leads to personalized experiences and improved efficiency. Rapid Innovation specializes in developing AI solutions that enhance device capabilities, driving greater ROI for our clients.
- Machine Learning (ML): Devices equipped with ML algorithms can analyze data patterns, enabling predictive analytics and smarter decision-making. Our team at Rapid Innovation helps clients implement ML solutions that optimize their operations and improve service delivery.
- Enhanced connectivity: Advanced features often include improved connectivity options, such as 5G and Wi-Fi 6, which facilitate faster data transfer and more reliable connections.
- Voice recognition: Many devices now incorporate voice-activated controls, making them more user-friendly and accessible, especially for individuals with disabilities.
- Augmented Reality (AR) and Virtual Reality (VR): These technologies are being integrated into various applications, from training simulations to interactive user interfaces, enhancing engagement and learning.
The continuous evolution of advanced features is essential for staying competitive in the technology landscape, as they drive innovation and improve overall user satisfaction. Rapid Innovation is committed to helping clients leverage these advanced features to achieve their business goals efficiently and effectively, including access to medical device companies and healthcare device companies.
10.1. Multi-device Correlation
Multi-device correlation refers to the ability to analyze and interpret data from various devices and platforms to gain a comprehensive understanding of user behavior and system performance. This approach is crucial in today's interconnected world, where users engage with multiple devices, such as smartphones, tablets, and computers.
- Enhances user experience by providing a seamless transition between devices.
- Allows businesses to track user interactions across different platforms, leading to better-targeted marketing strategies.
- Facilitates the identification of patterns in user behavior, which can inform product development and service improvements.
- Supports the integration of data from IoT devices, enabling real-time monitoring and analytics.
- Helps in understanding the customer journey, leading to improved customer satisfaction and retention.
By leveraging multi-device correlation, organizations can create a more cohesive digital experience, ultimately driving engagement and conversion rates. At Rapid Innovation, we utilize advanced AI algorithms to implement multi-device correlation, enabling our clients to enhance their marketing strategies and improve user engagement, thereby achieving greater ROI. For more insights on how we can help, learn about predictive analytics in finance.
10.2. Predictive Maintenance Scheduling
Predictive maintenance scheduling is a proactive approach to maintenance that uses data analytics and machine learning to predict when equipment is likely to fail. This strategy helps organizations minimize downtime and reduce maintenance costs.
- Utilizes real-time data from sensors and IoT devices to monitor equipment health.
- Predicts potential failures before they occur, allowing for timely interventions.
- Reduces unplanned downtime, which can significantly impact productivity and revenue.
- Optimizes maintenance schedules, ensuring that resources are allocated efficiently.
- Enhances safety by identifying potential hazards before they lead to accidents.
Implementing predictive maintenance can lead to substantial cost savings and improved operational efficiency, making it a vital component of modern asset management strategies. Rapid Innovation's expertise in machine learning allows us to develop tailored predictive maintenance solutions that help our clients reduce costs and enhance operational performance.
10.3. Cost Impact Analysis
Cost impact analysis is a systematic approach to evaluating the financial implications of a decision or change within an organization. This analysis helps businesses understand the potential costs and benefits associated with various strategies.
- Assesses both direct and indirect costs, providing a comprehensive view of financial impact.
- Aids in decision-making by quantifying the potential return on investment (ROI) for different initiatives.
- Supports budget planning and resource allocation by identifying cost drivers.
- Helps in risk management by evaluating the financial consequences of potential risks.
- Facilitates communication with stakeholders by presenting clear financial data.
By conducting a thorough cost impact analysis, organizations can make informed decisions that align with their financial goals and strategic objectives. At Rapid Innovation, we empower our clients to make data-driven decisions through comprehensive cost impact analyses, ensuring they maximize their ROI while minimizing risks.
10.4. Lifecycle Management
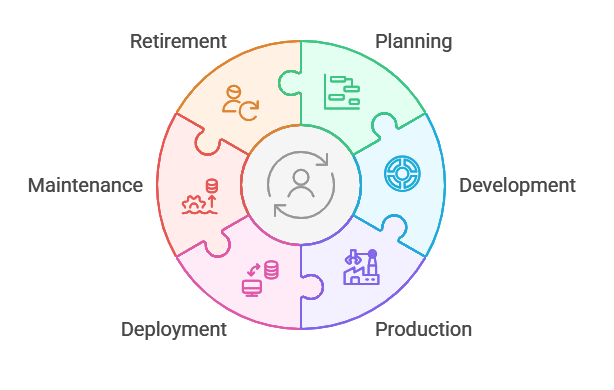
‍
Lifecycle management refers to the systematic approach to managing the entire lifecycle of a product or service from inception, through engineering design and manufacturing, to service and disposal. Effective lifecycle management ensures that products are developed, maintained, and retired in a way that maximizes their value and minimizes costs.
- Key stages in lifecycle management include: Â
- Planning: Identifying market needs and defining product specifications.
- Development: Designing and engineering the product, including prototyping and testing.
- Production: Manufacturing the product while ensuring quality control.
- Deployment: Distributing the product to customers and providing necessary training.
- Maintenance: Offering ongoing support and updates to enhance product performance.
- Retirement: Phasing out the product responsibly, including recycling or disposal.
- Benefits of effective lifecycle management: Â
- Reduces operational costs by streamlining processes.
- Enhances product quality through continuous improvement.
- Increases customer satisfaction by ensuring timely updates and support.
- Supports sustainability initiatives by managing resources efficiently.
At Rapid Innovation, we leverage advanced AI-driven lifecycle management tools and methodologies, such as Product Lifecycle Management (PLM) software and application lifecycle management software, to help organizations track and manage these stages effectively. By utilizing data analytics, we empower our clients to make informed decisions that enhance product performance and longevity, ultimately leading to greater ROI. Our expertise in AI retail and e-commerce solutions further supports our clients in achieving their objectives. Additionally, we focus on unleashing the power of AI in PLM to enhance lifecycle management processes.
10.5. Performance Optimization
Performance optimization involves enhancing the efficiency and effectiveness of a product or service to meet or exceed user expectations. This process is crucial for maintaining competitiveness in the market and ensuring customer satisfaction.
- Strategies for performance optimization include: Â
- Benchmarking: Comparing performance metrics against industry standards to identify areas for improvement.
- Data Analysis: Utilizing analytics tools to gather insights on product usage and performance.
- User Feedback: Collecting and analyzing customer feedback to understand pain points and areas for enhancement.
- Continuous Improvement: Implementing iterative changes based on performance data and user feedback.
- Key performance indicators (KPIs) to monitor: Â
- Speed: How quickly a product performs its intended functions.
- Reliability: The consistency of performance over time.
- Scalability: The ability to maintain performance levels as demand increases.
- User Experience: Overall satisfaction and ease of use from the customer's perspective.
By focusing on performance optimization, organizations can not only improve their products but also enhance brand loyalty and market share. At Rapid Innovation, we assist clients in conducting regular assessments and updates to ensure that their products remain relevant and competitive, thereby maximizing their return on investment.
11. Maintenance and Updates
Maintenance and updates are critical components of product management that ensure longevity and optimal performance. Regular maintenance helps prevent issues before they arise, while updates introduce new features and improvements.
- Types of maintenance include: Â
- Preventive Maintenance: Scheduled checks and updates to prevent potential failures.
- Corrective Maintenance: Addressing issues as they arise to restore functionality.
- Adaptive Maintenance: Modifying the product to accommodate changes in the environment or user needs.
- Importance of updates: Â
- Security: Regular updates help protect against vulnerabilities and cyber threats.
- Performance Enhancements: Updates can improve speed, efficiency, and user experience.
- New Features: Introducing new functionalities keeps the product competitive and engaging for users.
- Best practices for maintenance and updates: Â
- Establish a Schedule: Regularly planned maintenance and updates can prevent downtime and enhance reliability.
- Communicate with Users: Keeping customers informed about updates and maintenance schedules fosters trust and satisfaction.
- Monitor Performance: Continuously track product performance to identify when maintenance or updates are necessary.
By prioritizing maintenance and updates, organizations can ensure that their products remain functional, secure, and aligned with user expectations. This proactive approach not only extends the product's lifecycle but also enhances overall customer satisfaction. At Rapid Innovation, we provide tailored maintenance and update solutions that align with our clients' business goals, ensuring they achieve optimal performance and ROI. Our expertise in contract lifecycle management and information lifecycle management further supports our clients in achieving their objectives.
11.1. Model Retraining
Model retraining is a crucial process in machine learning and artificial intelligence that ensures the model remains accurate and relevant over time. As new data becomes available or as the environment changes, the original model may become less effective.
- Importance of retraining: Â
- Keeps the model updated with the latest data trends.
- Improves accuracy by incorporating new patterns and insights.
- Helps in adapting to changes in user behavior or market conditions.
- When to retrain: Â
- Significant changes in data distribution (concept drift).
- Performance metrics drop below acceptable thresholds.
- Introduction of new features or data sources.
- Retraining strategies: Â
- Periodic retraining based on a fixed schedule.
- Trigger-based retraining when specific performance metrics are met.
- Continuous learning where the model updates in real-time as new data arrives.
- Challenges: Â
- Computational costs associated with retraining large models.
- Ensuring data quality and relevance during the retraining process.
- Balancing between overfitting and underfitting during model updates.
At Rapid Innovation, we understand the importance of model retraining in maintaining the effectiveness of AI solutions. By implementing tailored retraining strategies, we help our clients ensure their models adapt to evolving data landscapes, ultimately leading to improved decision-making and greater ROI. For more information on our services, including Stable Diffusion Development, please visit our website. You can also learn about the latest advancements in model retraining techniques in our article on OpenAI's fine-tuning API.
11.2. System Updates
System updates are essential for maintaining the performance, security, and functionality of software systems, including machine learning models. Regular updates help in addressing bugs, improving features, and ensuring compatibility with new technologies.
- Types of updates: Â
- Security updates to protect against vulnerabilities.
- Feature updates that enhance system capabilities.
- Performance updates that optimize speed and efficiency.
- Importance of system updates: Â
- Protects against security threats and data breaches.
- Ensures compliance with industry standards and regulations.
- Improves user experience by providing new functionalities.
- Best practices for system updates: Â
- Schedule regular updates to minimize downtime.
- Test updates in a staging environment before deployment.
- Maintain a rollback plan in case of issues post-update.
- Challenges: Â
- Potential downtime during the update process.
- Compatibility issues with existing systems or software.
- User resistance to changes in familiar interfaces.
At Rapid Innovation, we prioritize system updates as a key component of our AI solutions. By ensuring that our clients' systems are regularly updated, we help them maintain security, enhance functionality, and improve user satisfaction, all of which contribute to a higher return on investment.
11.3. Performance Tuning
Performance tuning is the process of optimizing a system or model to achieve the best possible performance. This involves adjusting various parameters and configurations to enhance speed, efficiency, and accuracy.
- Key areas of performance tuning: Â
- Algorithm optimization to improve processing speed.
- Resource allocation to ensure efficient use of hardware.
- Hyperparameter tuning to find the best settings for machine learning models.
- Importance of performance tuning: Â
- Enhances user satisfaction by reducing wait times and improving responsiveness.
- Increases the efficiency of resource usage, leading to cost savings.
- Improves the overall accuracy and reliability of predictions.
- Techniques for performance tuning: Â
- Profiling to identify bottlenecks in the system.
- Load testing to understand how the system performs under stress.
- Continuous monitoring to track performance metrics over time.
- Challenges: Â
- Balancing performance improvements with system stability.
- The complexity of tuning parameters in large-scale systems.
- Keeping up with evolving technologies and methodologies in performance optimization.
At Rapid Innovation, we specialize in performance tuning to ensure that our clients' AI systems operate at peak efficiency. By employing advanced techniques and best practices, we help organizations achieve faster processing times and improved accuracy, leading to significant cost savings and enhanced ROI.
11.4. Configuration Management
Configuration management is a critical process in IT and software development that ensures systems and software are consistent, reliable, and meet specified requirements. It involves maintaining the integrity of systems over time, which is essential for operational efficiency and security.
- Definition: Configuration management refers to the systematic handling of changes in a system, ensuring that the system's performance, functionality, and security are maintained throughout its lifecycle.
- Key Components: Â
- Configuration Identification: Establishing and maintaining a list of all components in a system, including hardware, software, and documentation.
- Change Control: Implementing processes to manage changes to the system, ensuring that all modifications are documented, reviewed, and approved.
- Configuration Status Accounting: Keeping track of the status of configuration items and changes, providing visibility into the current state of the system.
- Configuration Audits: Regularly reviewing and verifying that the system's configuration aligns with the documented requirements and standards.
- Benefits: Â
- Improved system reliability and performance.
- Enhanced security posture by minimizing vulnerabilities.
- Streamlined troubleshooting and maintenance processes.
- Better compliance with regulatory requirements.
Effective configuration management tools, such as Ansible, Puppet, Chef, and SaltStack, can automate many of these processes, reducing the risk of human error and increasing efficiency. At Rapid Innovation, we leverage these tools to help our clients implement robust configuration management practices, including the use of configuration management database (CMDB) solutions, ultimately leading to greater operational efficiency and a higher return on investment (ROI). Additionally, our expertise extends to services like crypto token development to support innovative solutions in the digital landscape. For more insights on maintaining compliance, you can read about AI and machine learning for regulatory compliance.
11.5. Documentation

‍
Documentation is a vital aspect of any project or system, serving as a reference point for users, developers, and stakeholders. It encompasses all written materials that describe the system, its components, and its processes.
- Importance of Documentation: Â
- Provides clarity and understanding of system functionalities.
- Facilitates onboarding and training for new team members.
- Acts as a historical record of system changes and decisions.
- Supports compliance with industry standards and regulations.
- Types of Documentation: Â
- Technical Documentation: Detailed information about system architecture, APIs, and codebase.
- User Documentation: Guides and manuals for end-users, explaining how to use the system effectively.
- Process Documentation: Outlines workflows, procedures, and best practices for system management and operation.
- Compliance Documentation: Records that demonstrate adherence to regulatory requirements and standards.
- Best Practices: Â
- Keep documentation up-to-date to reflect the current state of the system.
- Use clear and concise language to enhance understanding.
- Organize documentation logically, making it easy to navigate.
- Utilize visual aids, such as diagrams and flowcharts, to clarify complex concepts.
Investing in comprehensive documentation not only improves communication among team members but also enhances the overall quality and maintainability of the system. Rapid Innovation assists clients in creating and maintaining effective documentation strategies, ensuring that knowledge is preserved and easily accessible, which in turn contributes to improved project outcomes and ROI.
12. Compliance and Security
Compliance and security are intertwined aspects of modern IT practices, focusing on adhering to regulations while protecting sensitive data and systems from threats.
- Compliance: Â
- Refers to the adherence to laws, regulations, and standards that govern data protection and privacy.
- Common frameworks include GDPR, HIPAA, and PCI-DSS, which set specific requirements for data handling and security.
- Organizations must regularly assess their compliance status and implement necessary changes to meet evolving regulations.
- Security: Â
- Involves protecting systems and data from unauthorized access, breaches, and other cyber threats.
- Key security measures include:
- Access Control: Implementing user authentication and authorization protocols to restrict access to sensitive information.
- Data Encryption: Using encryption techniques to protect data at rest and in transit, ensuring confidentiality.
- Regular Security Audits: Conducting assessments to identify vulnerabilities and ensure compliance with security policies.
- Integration of Compliance and Security: Â
- A robust compliance program enhances security by establishing clear guidelines for data protection.
- Security measures should be designed to meet compliance requirements, ensuring that organizations are not only secure but also legally compliant.
- Continuous monitoring and reporting are essential to maintain both compliance and security, allowing organizations to respond swiftly to any incidents or changes in regulations.
By prioritizing compliance and security, organizations can build trust with customers and stakeholders while safeguarding their assets against potential threats. Rapid Innovation provides expert consulting and development services to help clients navigate the complexities of compliance and security, ensuring that they not only meet regulatory requirements but also enhance their overall security posture, leading to improved ROI.
12.1. Data Privacy
Data privacy refers to the proper handling, processing, and storage of personal information. It is crucial for organizations to protect sensitive data to maintain customer trust and comply with legal requirements. Individuals have the right to know how their data is collected, used, and shared. Organizations must implement measures to safeguard personal information from unauthorized access. Data privacy laws, such as the General Data Protection Regulation (GDPR) in Europe, impose strict guidelines on data handling practices, including gdpr compliance and gdpr compliance regulations. Companies should conduct regular audits to ensure compliance with data privacy regulations, including gdpr requirements and compliance with the data protection act. Transparency is key; organizations should provide clear privacy policies to inform users about their data practices.
At Rapid Innovation, we understand the importance of data privacy in the AI landscape. Our solutions are designed to help organizations implement robust data privacy measures, ensuring compliance with regulations such as gdpr and compliance while maximizing the value of their data. By leveraging AI-driven analytics, we assist clients in identifying potential data vulnerabilities and enhancing their data governance frameworks, ultimately leading to greater ROI. For best practices in AI data privacy, visit our best practices for AI data privacy.
- Individuals have the right to know how their data is collected, used, and shared.
- Organizations must implement measures to safeguard personal information from unauthorized access.
- Data privacy laws, such as the General Data Protection Regulation (GDPR) in Europe, impose strict guidelines on data handling practices.
- Companies should conduct regular audits to ensure compliance with data privacy regulations.
- Transparency is key; organizations should provide clear privacy policies to inform users about their data practices.
12.2. Industry Regulations
Industry regulations are rules and standards that govern how businesses operate within specific sectors. These regulations are designed to protect consumers, ensure fair competition, and maintain industry integrity. Different industries have unique regulations, such as HIPAA for healthcare and PCI DSS for payment card transactions. Compliance with industry regulations, including ccpa compliance and gdpr ccpa compliance, is essential to avoid legal penalties and maintain a good reputation. Organizations should stay updated on changes in regulations to ensure ongoing compliance. Regular training for employees on industry regulations can help mitigate risks associated with non-compliance. Engaging with legal experts can provide valuable insights into navigating complex regulatory landscapes.
At Rapid Innovation, we provide consulting services that help organizations navigate the complex regulatory environment. Our expertise in AI allows us to develop tailored solutions that ensure compliance with industry regulations while optimizing operational efficiency. By automating compliance processes, we help clients reduce costs and enhance their competitive edge.
- Different industries have unique regulations, such as HIPAA for healthcare and PCI DSS for payment card transactions.
- Compliance with industry regulations is essential to avoid legal penalties and maintain a good reputation.
- Organizations should stay updated on changes in regulations to ensure ongoing compliance.
- Regular training for employees on industry regulations can help mitigate risks associated with non-compliance.
- Engaging with legal experts can provide valuable insights into navigating complex regulatory landscapes.
12.3. Security Protocols
Security protocols are essential measures that organizations implement to protect their data and systems from cyber threats. These protocols help ensure the confidentiality, integrity, and availability of information. Strong password policies should be enforced to prevent unauthorized access. Multi-factor authentication (MFA) adds an extra layer of security by requiring additional verification steps. Regular software updates and patch management are crucial to protect against vulnerabilities. Data encryption should be used to secure sensitive information both in transit and at rest. Organizations should conduct regular security assessments and penetration testing to identify and address potential weaknesses. Employee training on security best practices can significantly reduce the risk of human error leading to data breaches.
At Rapid Innovation, we prioritize security in our AI solutions. We implement advanced security protocols that not only protect sensitive data but also enhance the overall resilience of our clients' systems. By integrating AI-driven security measures, we help organizations proactively identify and mitigate potential threats, ensuring a secure environment that fosters innovation and growth.
- Strong password policies should be enforced to prevent unauthorized access.
- Multi-factor authentication (MFA) adds an extra layer of security by requiring additional verification steps.
- Regular software updates and patch management are crucial to protect against vulnerabilities.
- Data encryption should be used to secure sensitive information both in transit and at rest.
- Organizations should conduct regular security assessments and penetration testing to identify and address potential weaknesses.
- Employee training on security best practices can significantly reduce the risk of human error leading to data breaches.
12.4. Audit Requirements
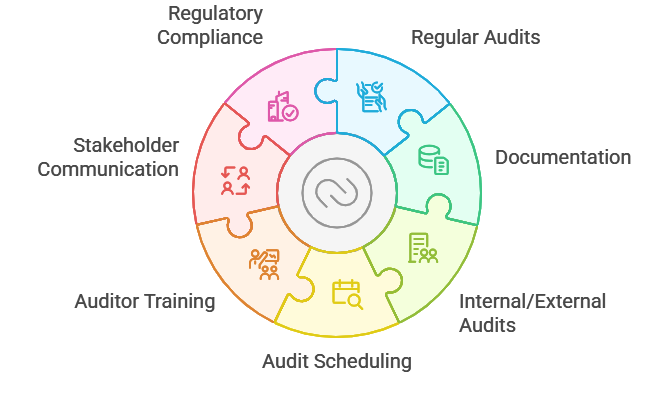
‍
Audit requirements are essential for ensuring compliance, accountability, and transparency within an organization. They help in identifying areas of improvement and ensuring that processes are followed correctly.
- Regular audits should be conducted to assess the effectiveness of internal controls. Rapid Innovation can assist in automating these audits using AI-driven tools, which can analyze vast amounts of data quickly and accurately, leading to more efficient audit cycles. This includes adhering to standards such as the IIA internal audit standards and GAAS audit standards.
- Documentation of audit findings is crucial for tracking progress and implementing corrective actions. Our solutions can streamline documentation processes, ensuring that findings are recorded and accessible for future reference, including those related to the certified internal auditor CIA certification.
- Audits can be internal or external, with external audits providing an unbiased perspective. Rapid Innovation can facilitate external audits by providing data analytics tools that enhance the auditor's ability to assess compliance effectively, including compliance with Sarbanes Oxley audit requirements.
- Organizations should establish a clear audit schedule, detailing the frequency and scope of audits. We can help clients develop tailored audit schedules that align with their specific business needs and regulatory requirements, including those dictated by the Sarbox audit.
- Auditors should be trained and knowledgeable about relevant regulations and standards, such as the IIA standards and generally accepted audit standards. Rapid Innovation offers training programs that leverage AI to keep auditors updated on the latest compliance standards and best practices, including the certified internal auditor certification.
- The results of audits should be communicated to stakeholders to maintain transparency. Our platforms can automate reporting processes, ensuring that stakeholders receive timely and accurate information regarding audit certifications and findings.
- Organizations must ensure that they are compliant with industry-specific regulations, which may dictate specific audit requirements. We provide consulting services to help organizations navigate complex regulatory landscapes, including those related to audit certifications and financial reporting audits. For more insights on how AI can enhance knowledge management in audits, check out AI Knowledge Management.
12.5. Access Control
Access control is a critical component of information security, ensuring that only authorized individuals can access sensitive data and systems. Effective access control measures help protect against data breaches and unauthorized access.
- Implement role-based access control (RBAC) to assign permissions based on user roles. Rapid Innovation can develop customized RBAC systems that align with your organizational structure.
- Use multi-factor authentication (MFA) to enhance security by requiring multiple forms of verification. Our solutions can integrate MFA seamlessly into existing systems, providing an additional layer of security.
- Regularly review and update access permissions to reflect changes in personnel or job functions. We can automate this process, ensuring that access rights are always up-to-date and compliant.
- Monitor access logs to detect any unauthorized access attempts or anomalies. Our AI-driven monitoring tools can analyze access patterns in real-time, alerting organizations to potential security threats.
- Educate employees about the importance of access control and secure password practices. Rapid Innovation offers training modules that focus on best practices for data security and access management.
- Establish a clear policy for granting and revoking access to sensitive information. We can assist in developing comprehensive access control policies tailored to your organization’s needs.
- Utilize encryption to protect data both at rest and in transit. Our encryption solutions ensure that sensitive data remains secure, even in the event of a breach.
13. Future Developments
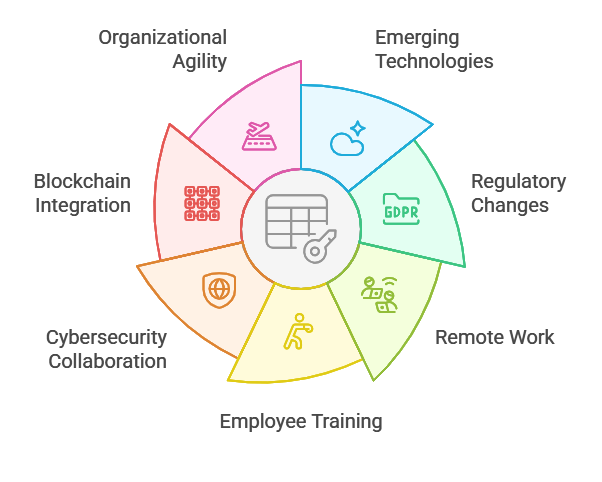
‍
Future developments in technology and regulations will significantly impact how organizations manage audits and access control. Staying ahead of these changes is crucial for maintaining security and compliance.
- Emerging technologies, such as artificial intelligence (AI) and machine learning, will enhance audit processes by automating data analysis and identifying patterns. Rapid Innovation specializes in developing AI solutions that can transform traditional audit methodologies, leading to greater efficiency and accuracy.
- Regulatory changes may require organizations to adapt their audit and access control practices to meet new compliance standards. Our consulting services can help organizations stay compliant with evolving regulations, including those related to audit certifications.
- The rise of remote work will necessitate more robust access control measures to secure data accessed from various locations. We can implement advanced access control solutions that cater to the needs of a remote workforce.
- Organizations should invest in continuous training for employees to keep them informed about the latest security practices and compliance requirements. Rapid Innovation provides ongoing training programs that leverage the latest in AI and cybersecurity, including training on IIA standards and internal auditor certification.
- Collaboration with cybersecurity experts will be essential to develop strategies that address evolving threats. Our team of experts can work alongside your organization to create tailored security strategies.
- The integration of blockchain technology may provide new ways to enhance audit trails and ensure data integrity. Rapid Innovation is at the forefront of blockchain solutions, offering innovative ways to secure and verify audit processes.
- Organizations must remain agile and ready to adapt to technological advancements and regulatory changes to maintain a secure environment. Our agile development approach ensures that we can quickly respond to your organization’s changing needs.
13.1. Emerging Technologies
Emerging technologies are reshaping industries and creating new opportunities for businesses. These innovations often leverage advancements in areas such as artificial intelligence, blockchain, and the Internet of Things (IoT).
- Artificial Intelligence (AI): AI is transforming how businesses operate by automating processes, enhancing decision-making, and improving customer experiences. At Rapid Innovation, we harness machine learning algorithms to analyze vast amounts of data, enabling our clients to identify trends and make informed predictions that drive greater ROI. This is particularly relevant in the context of new technologies in business. If you're looking to enhance your AI capabilities, consider our generative AI engineers and learn more about AI in business process automation.
- Blockchain: This technology offers secure and transparent transaction methods, making it ideal for industries like finance, supply chain, and healthcare. Rapid Innovation helps clients implement blockchain solutions that enhance trust and reduce fraud by providing a decentralized ledger of transactions, ultimately leading to more secure and efficient operations. The latest technologies in business are increasingly incorporating blockchain for enhanced security.
- Internet of Things (IoT): IoT connects devices and systems, allowing for real-time data collection and analysis. Our expertise in IoT enables businesses to optimize operations, improve efficiency, and enable predictive maintenance across various sectors, including manufacturing and logistics, resulting in significant cost savings and improved service delivery. Emerging technologies for businesses are heavily reliant on IoT capabilities.
- Augmented Reality (AR) and Virtual Reality (VR): These technologies are revolutionizing training, marketing, and customer engagement. Rapid Innovation assists businesses in creating immersive experiences that enhance learning and product demonstrations, leading to increased customer satisfaction and engagement. New technologies in entrepreneurship are leveraging AR and VR for innovative business models.
- 5G Technology: The rollout of 5G networks is set to enhance connectivity and speed, enabling more devices to connect seamlessly. Rapid Innovation is at the forefront of facilitating advancements in smart cities, autonomous vehicles, and telemedicine, helping clients leverage 5G for innovative solutions that drive growth. The demand for emerging technologies in business management is also being fueled by the capabilities of 5G.
13.4. Feature Roadmap
A feature roadmap is a strategic tool that outlines the planned features and enhancements for a product over a specific timeline. It serves as a visual representation of the product's development trajectory, helping teams align their efforts and communicate progress to stakeholders. Here are the key components and considerations for an effective feature roadmap:
- Vision and Goals: Clearly define the overarching vision for the product, set specific, measurable goals that the features aim to achieve, and ensure alignment with the company’s strategic objectives.
- Prioritization of Features: Use frameworks like MoSCoW (Must have, Should have, Could have, Won't have) to prioritize features. Consider factors such as customer feedback, market demand, and technical feasibility, and regularly reassess priorities based on changing business needs and user feedback.
- Timeline and Milestones: Establish a realistic timeline for feature development and release. Break down the timeline into key milestones to track progress and include buffer time for unforeseen challenges or delays.
- Stakeholder Involvement: Engage stakeholders, including customers, team members, and executives, in the roadmap creation process. Gather input to ensure the roadmap reflects the needs and expectations of all parties involved, and maintain transparency by sharing updates and soliciting feedback regularly.
- Flexibility and Adaptability: Recognize that the roadmap is a living document that may need adjustments. Be prepared to pivot based on new information, market changes, or shifts in user behavior, and implement a review process to evaluate the roadmap periodically.
- Communication and Collaboration: Use the roadmap as a communication tool to keep everyone informed about upcoming features and timelines. Foster collaboration among cross-functional teams, including development, marketing, and sales, and utilize project management tools like Trello for roadmap visualization and tracking progress.
- User-Centric Approach: Focus on features that enhance user experience and address pain points. Conduct user research and usability testing to validate feature ideas, and prioritize features that provide the most value to users.
- Metrics and KPIs: Define key performance indicators (KPIs) to measure the success of each feature post-launch. Use analytics tools to track user engagement and satisfaction, and adjust future roadmap items based on performance data and user feedback.
- Documentation and Version Control: Maintain thorough documentation of the roadmap, including changes and rationale. Use version control to track updates and ensure all team members are on the same page, and archive previous versions for reference and learning.
- Integration with Other Plans: Ensure the feature roadmap aligns with other strategic plans, such as marketing and sales strategies. Coordinate with other departments to maximize the impact of new features, and consider dependencies between features and other projects, including integration roadmap considerations.
- Long-Term Vision: While focusing on immediate features, keep an eye on the long-term vision for the product. Plan for future enhancements that align with evolving market trends and technologies, and encourage innovation by allowing room for experimental features in the roadmap.
- Feedback Loop: Establish a continuous feedback loop with users and stakeholders. Use surveys, interviews, and analytics to gather insights on feature performance, and incorporate feedback into future iterations of the roadmap.
- Tools and Resources: Utilize roadmap software and tools to create and manage the feature roadmap effectively. Explore options like Trello, Aha!, or ProductPlan for visualizing and sharing the roadmap, and leverage templates and best practices from industry leaders to enhance your roadmap. Consider integrating Aha with Jira for streamlined project management and tracking.
- Training and Support: Provide training for team members on how to use the roadmap effectively. Offer support resources to help teams understand the importance of the roadmap, and encourage a culture of collaboration and open communication regarding roadmap updates.
By focusing on these components, a feature roadmap can become a powerful tool for guiding product development, aligning teams, and ensuring that the product evolves in a way that meets user needs and business goals. At Rapid Innovation, we leverage our expertise in AI development to help clients create and implement effective feature roadmaps that drive greater ROI and align with their strategic objectives, including the development of a public roadmap and customer-facing roadmap strategies. For more information on how we can assist with your AI project estimation needs, visit our AI project estimation company.
‍