Table Of Contents
Category
Machine Learning (ML)
Natural Language Processing (NLP)
Artificial Intelligence (AI)
Decentralized Finance (DeFi)
Marketing and Media
1. Introduction to AI Market Trend Prediction
The landscape of market trend prediction has undergone a significant transformation with the advent of artificial intelligence (AI). Businesses and investors are increasingly relying on AI technologies to analyze vast amounts of data, identify patterns, and forecast future market movements. This shift is not merely a trend; it represents a fundamental change in how market analysis is conducted. AI market trend prediction leverages machine learning algorithms, natural language processing, and big data analytics to provide insights that were previously unattainable.
- AI enhances the accuracy of predictions.
- It allows for real-time data analysis.
- Businesses can make informed decisions based on predictive insights.
1.1. Evolution of Market Analysis
Market analysis has evolved from traditional methods to more sophisticated approaches over the years. Initially, market analysis relied heavily on manual data collection and human intuition. Analysts would study historical data, economic indicators, and market sentiment to make predictions. However, this approach had limitations, including time-consuming data collection processes, human biases affecting decision-making, and the inability to process large datasets efficiently.
With the rise of technology, particularly the internet and data analytics tools, market analysis began to incorporate quantitative methods. Statistical models and algorithms started to play a role, allowing analysts to process larger datasets and derive insights more quickly. The introduction of AI has further revolutionized this field by automating data collection and analysis, utilizing advanced algorithms to identify complex patterns, and providing AI market trend prediction that can adapt to changing market conditions.
1.2. Role of AI in Market Prediction
AI plays a crucial role in enhancing the accuracy and efficiency of market predictions. By employing machine learning techniques, AI systems can analyze historical data and recognize patterns that may not be immediately apparent to human analysts. Key aspects of AI's role in market prediction include:
- Predictive Analytics: AI algorithms can forecast future market trends based on historical data, allowing businesses to anticipate changes and adjust their strategies accordingly. For instance, Rapid Innovation has helped clients implement predictive models that have led to a 30% increase in forecasting accuracy, directly impacting their bottom line.
- Sentiment Analysis: Natural language processing enables AI to analyze social media, news articles, and other text sources to gauge public sentiment about a particular market or product. This insight can be invaluable for understanding market dynamics and has been utilized by our clients to tailor their marketing strategies effectively.
- Real-time Data Processing: AI can process and analyze data in real-time, providing businesses with up-to-the-minute insights that can inform decision-making. Rapid Innovation has developed solutions that allow clients to react swiftly to market changes, enhancing their competitive edge.
- Risk Management: AI can help identify potential risks in the market by analyzing various factors, including economic indicators and market sentiment, allowing businesses to mitigate risks effectively. Our consulting services have enabled clients to implement risk assessment frameworks that have reduced potential losses by up to 25%.
- Enhanced Decision-Making: By providing data-driven insights, AI empowers businesses to make informed decisions, reducing reliance on gut feelings or outdated information. Rapid Innovation's AI-driven dashboards have transformed how clients visualize data, leading to more strategic decision-making processes.
The integration of AI into market trend prediction is not just a passing trend; it is a fundamental shift that is reshaping how businesses operate in an increasingly complex and data-driven world. As AI technology continues to evolve, its impact on market analysis will likely grow, offering even more sophisticated tools for predicting market trends. Rapid Innovation is committed to helping clients harness these advancements to achieve greater ROI and drive their business success.
Refer to the image for a visual representation of the concepts discussed in the introduction to AI market trend prediction:

1.3. Core Benefits and Limitations
Benefits of AI Technology:
- Increased Efficiency: AI can automate repetitive tasks, allowing businesses to focus on more strategic activities. This leads to significant time savings and improved productivity, enabling organizations to allocate resources more effectively. The benefits of AI technology are evident in various sectors, including banking and healthcare.
- Enhanced Decision-Making: AI systems can analyze vast amounts of data quickly, providing insights that help organizations make informed decisions. This data-driven approach reduces human error and bias, leading to more accurate outcomes. The benefits of artificial intelligence in business are particularly notable in decision-making processes.
- Cost Reduction: By automating processes and improving efficiency, AI can lead to substantial cost savings. Companies can reduce labor costs and minimize operational expenses, ultimately increasing their return on investment (ROI). The advantages of artificial intelligence are clear when it comes to financial management.
- Personalization: AI enables businesses to offer personalized experiences to customers. By analyzing user behavior, AI can tailor recommendations and services, enhancing customer satisfaction and loyalty, which can drive revenue growth. The benefits of artificial intelligence in healthcare also include personalized treatment plans.
- Scalability: AI solutions can easily scale with business growth. As demand increases, AI systems can handle larger volumes of data and transactions without a significant increase in costs, allowing businesses to expand without compromising performance. The pros of artificial intelligence are evident in its ability to support growth.
Limitations of AI Technology:
- High Initial Investment: Implementing AI solutions can require significant upfront costs, including technology acquisition and training. This can be a barrier for some organizations, particularly smaller businesses. The benefits and risks of artificial intelligence must be carefully weighed before investment.
- Data Privacy Concerns: The use of AI often involves collecting and analyzing large amounts of personal data, raising concerns about privacy and security. Companies must navigate these challenges to maintain customer trust. The benefits of using artificial intelligence must be balanced with ethical considerations.
- Job Displacement: Automation through AI can lead to job losses in certain sectors, creating a need for workforce retraining and adaptation. Organizations must consider the social implications of their AI strategies, including the potential risks associated with job displacement.
- Dependence on Data Quality: AI systems rely heavily on the quality of data. Poor data can lead to inaccurate predictions and decisions, which can undermine the effectiveness of AI initiatives. The benefits of artificial intelligence in cyber security highlight the importance of data integrity.
- Ethical Considerations: The deployment of AI raises ethical questions, particularly regarding bias in algorithms and the potential for misuse. Companies must be vigilant in addressing these issues to ensure responsible AI use. The positive aspects of artificial intelligence must be promoted alongside a commitment to ethical practices.
1.4. Current Market Overview
The AI market is experiencing rapid growth and transformation. Key aspects of the current market include:
- Market Size and Growth: The global AI market is projected to reach significant valuations in the coming years, driven by advancements in machine learning, natural language processing, and computer vision. The benefits of artificial intelligence are becoming increasingly recognized across various industries.
- Industry Adoption: Various sectors, including healthcare, finance, retail, and manufacturing, are increasingly adopting AI technologies to enhance operations and customer experiences. The benefits of artificial intelligence in business are driving this trend.
- Investment Trends: Venture capital investment in AI startups has surged, indicating strong confidence in the technology's potential. Companies are investing in AI research and development to stay competitive, recognizing the advantages of AI technology.
- Geographical Insights: North America remains a leader in AI development, but Asia-Pacific is rapidly emerging as a significant player, with countries like China and India investing heavily in AI initiatives. The benefits of artificial intelligence to society are becoming a global conversation.
- Regulatory Landscape: As AI technology evolves, governments are beginning to establish regulations to ensure ethical use and data protection, impacting how companies implement AI solutions. The benefits and risks of artificial intelligence are prompting discussions on regulatory frameworks.
2. Understanding AI Market Trend Predictors
AI market trend predictors are essential for businesses looking to navigate the evolving landscape of artificial intelligence. Key predictors include:
- Technological Advancements: Continuous improvements in algorithms, computing power, and data storage capabilities are driving AI innovation. Companies must stay updated on these advancements to leverage new opportunities, including the benefits of AI technology.
- Consumer Behavior: Understanding shifts in consumer preferences and behaviors can help businesses tailor their AI strategies. For instance, the demand for personalized experiences is pushing companies to invest in AI-driven customer insights, reflecting the benefits of artificial intelligence in business.
- Economic Factors: Economic conditions, such as recession or growth, can influence AI adoption rates. During economic downturns, companies may prioritize cost-saving AI solutions, while growth periods may see increased investment in innovative AI applications, highlighting the pros of artificial intelligence.
- Competitive Landscape: Monitoring competitors' AI initiatives can provide insights into market trends. Companies that adopt AI early can gain a competitive edge, prompting others to follow suit, showcasing the benefits of artificial intelligence in various sectors.
- Regulatory Changes: As governments introduce new regulations regarding data privacy and AI ethics, businesses must adapt their strategies to comply with these changes. This can impact the pace of AI adoption and innovation, emphasizing the importance of understanding the benefits and risks of artificial intelligence.
By understanding these trend predictors, businesses can make informed decisions about their AI strategies, ensuring they remain competitive in a rapidly changing market. Rapid Innovation is here to guide you through these complexities, helping you harness the power of AI to achieve your business goals efficiently and effectively.
Refer to the image for a visual representation of the core benefits and limitations of AI technology.

2.1. Key Components and Architecture
Understanding the key components and architecture of a system is crucial for effective implementation and operation. The architecture typically consists of several layers that work together to process data and deliver insights.
- Data Layer: This is where all the raw data is stored. It can include databases, data lakes, and data warehouses. The data layer is essential for ensuring that data is accessible and organized for analysis, enabling Rapid Innovation to provide clients with a solid foundation for their data-driven strategies.
- Processing Layer: This layer is responsible for transforming raw data into a usable format. It includes ETL (Extract, Transform, Load) processes, data cleaning, and data integration. Tools like Apache Spark and Hadoop are often used in this layer to handle large datasets efficiently, allowing Rapid Innovation to streamline data processing for enhanced operational efficiency. Data integration platforms and data integration tools play a significant role in this process, ensuring seamless data flow.
- Analytics Layer: This layer focuses on analyzing the processed data. It includes statistical analysis, data mining, and machine learning algorithms. This is where insights are generated, and predictive models are built, empowering clients to make informed decisions that drive greater ROI.
- Presentation Layer: The final layer is where the results are visualized and presented to users. Dashboards, reports, and data visualization tools like Tableau or Power BI are commonly used to communicate findings effectively, ensuring that stakeholders can easily interpret data insights.
- User Interface: This component allows users to interact with the system. A well-designed user interface enhances user experience and facilitates easier access to data and insights, which is a key focus for Rapid Innovation in delivering user-friendly solutions.
- Security and Governance: Ensuring data security and compliance with regulations is critical. This includes implementing access controls, encryption, and data governance policies to protect sensitive information, thereby safeguarding client data and maintaining trust.
2.2. Data Sources and Integration
Data sources are the backbone of any analytical system, providing the raw material needed for analysis and decision-making. Integrating these diverse data sources is essential for creating a comprehensive view of the information landscape.
- Structured Data: This includes data that is organized in a predefined manner, such as databases and spreadsheets. Examples include customer records, sales data, and inventory lists, which Rapid Innovation can leverage to enhance client analytics.
- Unstructured Data: This type of data does not have a predefined format. It includes text documents, social media posts, images, and videos. Unstructured data can provide valuable insights but requires advanced processing techniques to analyze, a capability that Rapid Innovation excels in.
- Real-time Data: Data that is generated continuously and needs to be processed in real-time. Examples include sensor data from IoT devices, website clickstream data, and financial transactions. Rapid Innovation helps clients harness real-time data for timely decision-making.
- APIs and Web Services: These are essential for integrating data from various external sources. APIs allow different systems to communicate and share data seamlessly, which is crucial for Rapid Innovation's integration solutions.
- Data Warehousing: A data warehouse consolidates data from multiple sources into a single repository, enabling easier access and analysis of data from different origins, thus enhancing the analytical capabilities of Rapid Innovation's clients. Data integration solutions and data integration services are vital in this context.
- Data Quality and Cleansing: Ensuring the accuracy and consistency of data is vital. Data cleansing processes help identify and rectify errors, duplicates, and inconsistencies in the data, ensuring that clients can rely on high-quality data for their analyses. Tools like Talend Open Studio for data integration and EDI integration software can assist in maintaining data quality.
2.3. Machine Learning Models
Machine learning models are at the heart of predictive analytics and data-driven decision-making. These models learn from historical data to make predictions or identify patterns.
- Supervised Learning: This approach involves training a model on labeled data, where the outcome is known. Common algorithms include linear regression, decision trees, and support vector machines. Supervised learning is widely used for classification and regression tasks, enabling Rapid Innovation to deliver tailored solutions for client needs.
- Unsupervised Learning: In this approach, the model is trained on unlabeled data. It identifies patterns and groupings without prior knowledge of outcomes. Clustering algorithms like K-means and hierarchical clustering are examples of unsupervised learning, which Rapid Innovation utilizes to uncover hidden insights for clients.
- Reinforcement Learning: This type of learning involves training models through trial and error. The model learns to make decisions by receiving feedback from its actions. It is commonly used in robotics and game playing, showcasing Rapid Innovation's versatility in applying machine learning across various domains.
- Deep Learning: A subset of machine learning that uses neural networks with many layers. Deep learning is particularly effective for tasks like image and speech recognition, requiring large amounts of data and computational power, which Rapid Innovation can harness to solve complex client challenges.
- Model Evaluation: Evaluating the performance of machine learning models is crucial. Metrics such as accuracy, precision, recall, and F1 score are commonly used to assess model effectiveness, ensuring that Rapid Innovation delivers high-performing solutions.
- Deployment and Monitoring: Once a model is trained and evaluated, it needs to be deployed in a production environment. Continuous monitoring is essential to ensure the model performs well over time and adapts to new data, a service that Rapid Innovation provides to ensure sustained client success. Tools like Dell Boomi Master Data Management and cloud-based data integration solutions can facilitate this process. For more information on enhancing AI and machine learning models, you can refer to data annotation services.
Refer to the image for a visual representation of the key components and architecture of a system.

2.4. Prediction Mechanisms
Prediction mechanisms are essential components in various fields, including finance, healthcare, and technology. These mechanisms utilize algorithms and statistical models to forecast future events based on historical data.
- Machine Learning: Many prediction mechanisms leverage machine learning techniques to improve accuracy. Algorithms learn from past data, identifying patterns and trends that can be used to make informed predictions. At Rapid Innovation, we implement tailored machine learning models that help clients optimize their operations, leading to significant cost savings and increased efficiency.
- Time Series Analysis: This method involves analyzing data points collected or recorded at specific time intervals. It helps in understanding seasonal trends and cyclical patterns, which are crucial for making future predictions. Our expertise in time series analysis allows clients to anticipate market fluctuations, enabling proactive decision-making.
- Regression Analysis: This statistical method estimates the relationships among variables. It is widely used to predict outcomes based on the influence of one or more independent variables. By employing regression analysis, Rapid Innovation assists clients in identifying key drivers of performance, ultimately enhancing their strategic planning.
- Neural Networks: These are computational models inspired by the human brain. They are particularly effective in recognizing complex patterns and making predictions in large datasets. Our team at Rapid Innovation utilizes neural networks to develop sophisticated predictive models that drive innovation and improve customer experiences.
- Real-time Data Processing: Modern prediction mechanisms often incorporate real-time data, allowing for more dynamic and responsive forecasting. This is especially important in industries like finance, where market conditions can change rapidly. Rapid Innovation's solutions enable clients to harness real-time data, ensuring they remain competitive and responsive to market demands.
3. Core Features and Capabilities
Core features and capabilities of prediction mechanisms are what make them valuable tools across various industries. These features enhance their functionality and effectiveness in delivering accurate forecasts.
- Data Integration: The ability to integrate data from multiple sources is crucial. This includes structured and unstructured data, which can provide a more comprehensive view for predictions. Rapid Innovation excels in creating integrated data environments that empower clients to make data-driven decisions.
- Scalability: Effective prediction mechanisms can scale to handle increasing amounts of data without a loss in performance. This is vital for organizations that experience rapid growth. Our scalable solutions ensure that clients can adapt to changing data landscapes without compromising on performance.
- User-friendly Interfaces: Many prediction tools come with intuitive interfaces that allow users to easily input data and interpret results, making them accessible to non-technical users. Rapid Innovation prioritizes user experience, ensuring that our tools are designed for ease of use, facilitating broader adoption across organizations.
- Customization: The ability to customize models and algorithms to fit specific business needs is a significant advantage. This ensures that predictions are relevant and tailored to the unique context of the organization. At Rapid Innovation, we work closely with clients to develop bespoke solutions that align with their strategic objectives.
- Reporting and Visualization: Advanced reporting tools and visualization capabilities help users understand predictions better. Graphs, charts, and dashboards can make complex data more digestible. Our reporting solutions empower clients to visualize their data effectively, enhancing their ability to communicate insights and drive action.
3.1. Pattern Recognition

‍
Pattern recognition is a critical aspect of prediction mechanisms, enabling systems to identify trends and anomalies within data. This capability is foundational for making accurate forecasts.
- Image and Signal Processing: Pattern recognition is widely used in image and signal processing, where algorithms can identify objects, faces, or specific signals within large datasets. Rapid Innovation leverages these techniques to develop innovative solutions for clients in sectors such as security and healthcare.
- Natural Language Processing (NLP): In the realm of text data, pattern recognition helps in understanding sentiment, context, and meaning. This is particularly useful in applications like chatbots and customer service automation. Our NLP solutions enhance client interactions, leading to improved customer satisfaction and engagement.
- Anomaly Detection: Identifying outliers or unusual patterns in data is essential for fraud detection, network security, and quality control. Pattern recognition algorithms can flag these anomalies for further investigation. Rapid Innovation's anomaly detection capabilities help clients mitigate risks and enhance operational integrity.
- Classification: This involves categorizing data into predefined classes based on identified patterns. It is commonly used in spam detection, medical diagnosis, and credit scoring. Our classification solutions enable clients to streamline processes and improve accuracy in decision-making.
- Predictive Maintenance: In industrial settings, pattern recognition can predict equipment failures by analyzing historical performance data, allowing for timely maintenance and reducing downtime. Rapid Innovation's predictive maintenance solutions help clients optimize asset management, resulting in significant cost reductions.
By leveraging these prediction mechanisms, features, and capabilities, organizations can enhance their predictive analytics, leading to better decision-making and improved outcomes. Rapid Innovation is committed to helping clients achieve greater ROI through innovative AI solutions tailored to their unique business needs. For those looking to enhance their capabilities, consider our Action Transformer Developers to drive your projects forward. Additionally, you can explore more about pattern recognition in machine learning for a comprehensive overview.
Refer to the image for a visual representation of the prediction mechanisms discussed in section 2.4.
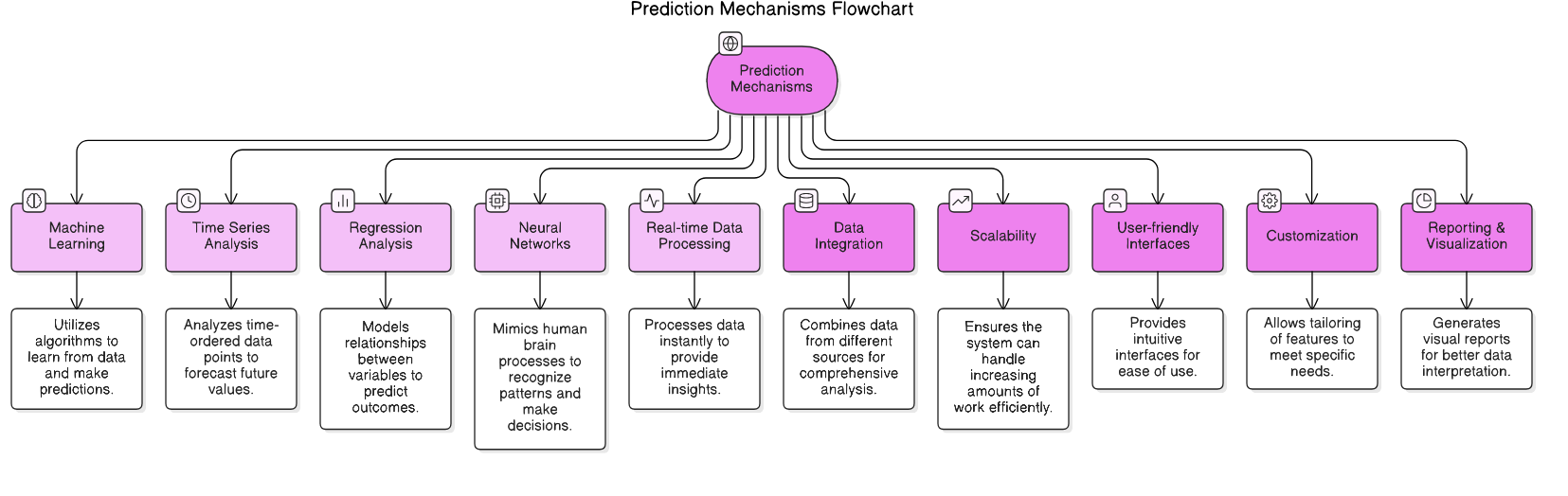
3.2. Real-time Analysis
Real-time analysis refers to the immediate processing and evaluation of data as it becomes available. This capability is crucial in various fields, including finance, marketing, and healthcare. The benefits of real-time analysis include:
- Immediate Decision-Making: Organizations can make informed decisions quickly, responding to market changes or customer needs without delay.
- Enhanced Customer Experience: Businesses can tailor their services or products based on real-time feedback, improving customer satisfaction and loyalty.
- Operational Efficiency: By analyzing data in real-time, companies can identify inefficiencies and optimize processes, leading to cost savings and increased productivity.
Technologies such as big data analytics, machine learning, and cloud computing facilitate real-time analysis. For instance, financial institutions use real-time data to monitor stock prices and execute trades instantly, which can significantly impact profitability. According to a report by Gartner, organizations that leverage real-time analytics can improve their operational efficiency by up to 30%. At Rapid Innovation, we empower our clients to harness these technologies, enabling them to make data-driven decisions that enhance their competitive edge and drive greater ROI. This includes utilizing real-time big data analytics and real-time predictive analytics to gain deeper insights into customer behavior and market trends. Additionally, our real-time analytics software allows businesses to perform realtime data analysis effectively, ensuring they stay ahead in a competitive landscape. For tailored solutions, consider our AI insurance solutions that leverage real-time analysis for enhanced decision-making. Furthermore, the future of personalized risk evaluation in insurance with AI agents.
3.3. Multi-market Correlation
Multi-market correlation involves analyzing the relationships and interactions between different markets or asset classes. Understanding these correlations is essential for investors and businesses as it helps in:
- Diversification Strategies: By recognizing how different markets move in relation to one another, investors can create diversified portfolios that minimize risk.
- Risk Management: Identifying correlations allows businesses to anticipate potential risks and adjust their strategies accordingly.
- Market Predictions: Analyzing correlations can provide insights into future market movements, enabling better forecasting and planning.
For example, the correlation between stock markets and commodities can indicate how economic changes affect various sectors. A study by the CFA Institute found that understanding multi-market correlations can enhance portfolio performance by up to 15%. At Rapid Innovation, we utilize advanced analytical tools to help our clients visualize these relationships, enabling them to make informed, data-driven decisions that optimize their investment strategies.
3.4. Risk Assessment
Risk assessment is the systematic process of identifying, analyzing, and evaluating potential risks that could negatively impact an organization or project. This process is vital for effective risk management and includes:
- Identifying Risks: Recognizing potential threats, whether they are financial, operational, or reputational.
- Analyzing Risks: Evaluating the likelihood and impact of identified risks to prioritize them effectively.
- Mitigating Risks: Developing strategies to minimize or eliminate risks, ensuring that the organization can continue to operate smoothly.
Effective risk assessment can lead to better decision-making and resource allocation. Organizations that implement robust risk assessment frameworks can reduce their exposure to losses significantly. According to a study by the Risk Management Society, companies that actively engage in risk assessment can lower their operational costs by up to 20%. At Rapid Innovation, we assist our clients in developing comprehensive risk assessment strategies using tools such as SWOT analysis, risk matrices, and scenario planning, ultimately enhancing their resilience and operational efficiency. Our approach also incorporates real-time customer analytics to better understand and mitigate risks associated with customer behavior.
3.5. Sentiment Analysis
Sentiment analysis is a crucial aspect of data analysis that focuses on understanding the emotional tone behind a series of words. It is widely used in various fields, including marketing, customer service, and social media monitoring. The primary goal is to determine whether the sentiment expressed in a piece of text is positive, negative, or neutral.
- Techniques used in sentiment analysis include: Â
- Natural Language Processing (NLP): This involves algorithms that can interpret and analyze human language, enabling businesses to understand customer emotions and feedback more effectively. This includes sentiment analysis natural language processing and sentiment analysis using natural language processing.
- Machine Learning: Models are trained on labeled datasets to classify sentiments based on patterns, allowing for continuous improvement in accuracy and relevance. This is often referred to as machine learning for sentiment analysis and sentiment analysis using machine learning.
- Lexicon-based approaches: These use predefined lists of words associated with positive or negative sentiments, providing a foundational method for sentiment classification. Various methods of sentiment analysis can be employed, including sentiment analysis techniques.
- Applications of sentiment analysis: Â
- Brand monitoring: Companies can track public perception of their brand through social media and reviews, enabling them to respond proactively to customer sentiments and enhance brand loyalty. This is particularly relevant in sentiment analysis twitter, where brands can gauge public opinion.
- Customer feedback: Businesses analyze customer reviews to improve products and services, leading to increased customer satisfaction and retention. This can involve sentiment analysis of twitter data to understand customer opinions.
- Political analysis: Sentiment analysis can gauge public opinion on political issues or candidates, providing valuable insights for campaign strategies. Opinion mining and sentiment analysis are often used in this context.
- Challenges in sentiment analysis: Â
- Sarcasm detection: Understanding sarcasm can be difficult for algorithms, which may lead to misinterpretation of sentiments.
- Contextual meaning: Words can have different meanings based on context, complicating analysis and requiring sophisticated models to accurately capture sentiment.
- Multilingual analysis: Analyzing sentiments in multiple languages requires extensive resources and expertise, which can be a barrier for global organizations.
4. Data Processing and Analysis
Data processing and analysis involve transforming raw data into meaningful insights. This process is essential for making informed decisions based on data-driven evidence. It encompasses various stages, including data cleaning, transformation, and analysis.
- Key stages in data processing: Â
- Data Cleaning: Removing inaccuracies, duplicates, and irrelevant information to ensure data quality, which is critical for reliable analysis.
- Data Transformation: Converting data into a suitable format for analysis, which may include normalization or aggregation, ensuring that the data is ready for insightful analysis.
- Data Analysis: Applying statistical methods and algorithms to extract insights and patterns from the data, enabling organizations to make informed decisions.
- Tools and techniques for data processing: Â
- Spreadsheet software: Tools like Microsoft Excel or Google Sheets for basic data manipulation, suitable for small-scale analysis.
- Programming languages: Python and R are popular for more complex data analysis tasks, providing flexibility and power for advanced analytics.
- Data visualization tools: Software like Tableau or Power BI helps in presenting data insights visually, making it easier for stakeholders to understand and act on the findings.
- Importance of data processing: Â
- Improved decision-making: Accurate data analysis leads to better business strategies, allowing organizations to align their goals with market demands.
- Enhanced efficiency: Streamlined data processing saves time and resources, enabling teams to focus on strategic initiatives rather than manual data handling.
- Competitive advantage: Organizations that effectively analyze data can outperform their competitors by leveraging insights to drive innovation and customer engagement.
4.1. Data Collection Methods
Data collection methods are essential for gathering the information needed for analysis. The choice of method can significantly impact the quality and relevance of the data collected. Various techniques can be employed, depending on the research objectives and available resources.
- Common data collection methods: Â
- Surveys and Questionnaires: These are widely used to gather quantitative data from a large audience, providing a broad understanding of customer preferences.
- Interviews: Conducting one-on-one interviews can provide in-depth qualitative insights, allowing for a deeper understanding of customer motivations and sentiments.
- Observations: Directly observing subjects in their natural environment can yield valuable data, offering real-world context to the analysis.
- Digital data collection methods: Â
- Web scraping: Automated tools can extract data from websites for analysis, enabling organizations to gather large datasets efficiently.
- Social media monitoring: Analyzing posts and comments on social platforms can provide real-time insights into customer sentiments and trends, including sentiment analysis twitter.
- APIs: Many platforms offer APIs that allow for the extraction of structured data, facilitating seamless integration into analysis workflows.
- Considerations for data collection: Â
- Sample size: A larger sample size can lead to more reliable results, enhancing the validity of the analysis.
- Data privacy: Ensuring compliance with regulations like GDPR is crucial when collecting personal data, protecting both the organization and its customers.
- Data validity: Using validated instruments and methods enhances the credibility of the collected data, ensuring that insights are based on accurate information.
By understanding sentiment analysis, data processing, and data collection methods, organizations can leverage data to drive strategic decisions and improve overall performance. Rapid Innovation is committed to helping clients navigate these complexities, ensuring they achieve greater ROI through effective data-driven strategies.
4.1.1. Market Data
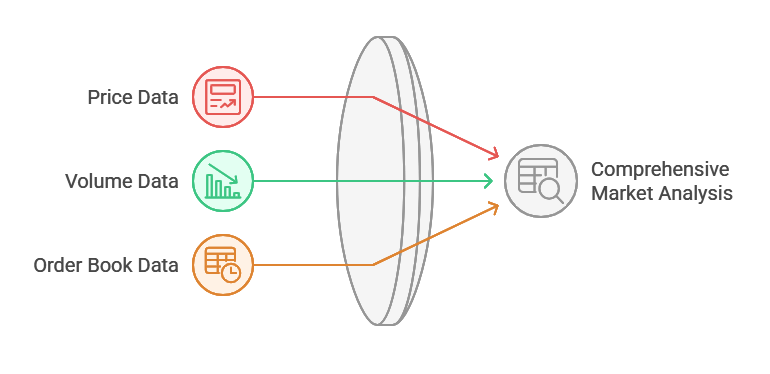
‍
Market data refers to the information that reflects the current state of financial markets. It includes a variety of metrics that help investors and analysts make informed decisions. Understanding market data is crucial for anyone involved in trading or investing.
- Types of Market Data: Â
- Price Data: This includes the current price of stocks, bonds, commodities, and currencies.
- Volume Data: Indicates the number of shares or contracts traded in a given period, which can signal market activity.
- Order Book Data: Shows the list of buy and sell orders for a particular asset, providing insight into market depth.
- Sources of Market Data: Â
- Financial news websites
- Stock exchanges
- Brokerage platforms
- Market research data
- Market research database
- Importance of Market Data: Â
- Helps in identifying trends and patterns.
- Aids in risk management by providing insights into market volatility.
- Essential for technical analysis, which relies on historical price movements.
- E commerce analytics can provide additional insights into consumer behavior and market trends.
At Rapid Innovation, we leverage advanced AI algorithms to analyze market data efficiently, enabling our clients to make data-driven decisions that enhance their investment strategies and ultimately lead to greater ROI. Our services include competitor pricing analysis and market survey analysis to further refine our clients' approaches. For more information on how AI can enhance market data analysis.
4.1.2. News and Social Media
News and social media play a significant role in shaping market sentiment and influencing investor behavior. The rapid dissemination of information can lead to immediate market reactions.
- Impact of News: Â
- Economic reports, earnings announcements, and geopolitical events can cause significant price fluctuations.
- Positive news can lead to bullish trends, while negative news can trigger sell-offs.
- Role of Social Media: Â
- Platforms like Twitter and Reddit have become influential in the financial world, with trends often driven by social media discussions.
- Retail investors increasingly rely on social media for investment tips and market insights.
- Monitoring News and Social Media: Â
- Investors should track financial news outlets and social media channels to stay updated.
- Tools like sentiment analysis can help gauge public opinion and predict market movements.
- Competitor web analytics can also provide insights into how market sentiment is shifting.
Rapid Innovation employs natural language processing (NLP) techniques to monitor news and social media sentiment, providing our clients with actionable insights that can inform their trading strategies and enhance their market positioning.
4.1.3. Economic Indicators
Economic indicators are statistics that provide insight into the overall health of an economy. They are essential for investors as they can influence market trends and investment strategies.
- Types of Economic Indicators: Â
- Leading Indicators: Predict future economic activity (e.g., stock market performance, new housing starts).
- Lagging Indicators: Reflect past economic performance (e.g., unemployment rates, GDP growth).
- Coincident Indicators: Occur simultaneously with economic changes (e.g., retail sales, industrial production).
- Key Economic Indicators: Â
- Gross Domestic Product (GDP): Measures the total economic output of a country.
- Consumer Price Index (CPI): Indicates inflation by measuring changes in the price level of a basket of consumer goods and services.
- Unemployment Rate: Reflects the percentage of the labor force that is unemployed and actively seeking employment.
- Importance of Economic Indicators: Â
- Help investors assess the economic environment and make informed decisions.
- Can signal potential market trends and shifts in investor sentiment.
- Essential for understanding macroeconomic conditions that affect various sectors.
- Healthcare analytics market trends can also influence investment strategies in the health sector.
At Rapid Innovation, we utilize predictive analytics to interpret economic indicators, allowing our clients to anticipate market movements and adjust their strategies accordingly, thereby maximizing their investment returns. Our market report research and market analysis report services provide comprehensive insights into various sectors.
4.2. Data Cleaning and Preprocessing
Data cleaning and preprocessing are critical steps in the data analysis pipeline. This phase ensures that the data is accurate, consistent, and usable for further analysis or modeling, ultimately leading to improved business outcomes for our clients at Rapid Innovation. This process often involves data cleaning and preprocessing in python to streamline operations.
- Handling Missing Values: Missing data can skew results and lead to inaccurate conclusions. Techniques include: Â
- Imputation: Filling in missing values using statistical methods like mean, median, or mode, which helps maintain the integrity of the dataset.
- Deletion: Removing records with missing values, though this can lead to loss of valuable information. Our team carefully evaluates the impact of deletion to ensure minimal disruption to analysis.
- Removing Duplicates: Duplicate entries can distort analysis. Identifying and removing duplicates ensures that each data point is unique, enhancing the reliability of insights derived from the data. This is part of the broader data cleaning and data preprocessing efforts.
- Data Type Conversion: Ensuring that data types are appropriate for analysis is essential. For example, converting date strings into date objects or categorical variables into numerical formats allows for more effective modeling and analysis.
- Outlier Detection: Outliers can significantly affect model performance. Techniques such as Z-scores or IQR (Interquartile Range) can help identify and manage outliers, ensuring that the model is trained on representative data.
- Normalization and Standardization: Scaling data to a common range or distribution helps improve model performance. Normalization adjusts values to a 0-1 range, while standardization transforms data to have a mean of 0 and a standard deviation of 1, facilitating better comparisons across features.
- Text Preprocessing: For text data, preprocessing steps include tokenization, stemming, and removing stop words to prepare the data for analysis. This is particularly beneficial for clients looking to leverage natural language processing (NLP) for insights. Data cleaning pada data preprocessing dilakukan to ensure that text data is ready for analysis.
4.3. Feature Engineering
Feature engineering is the process of using domain knowledge to create new features or modify existing ones to improve model performance. This step is crucial as the right features can significantly enhance the predictive power of a model, leading to greater ROI for our clients.
- Creating New Features: New features can be derived from existing data. For example: Â
- Combining date features into a single feature representing the day of the week can reveal patterns in customer behavior.
- Extracting text features such as word counts or sentiment scores from textual data can provide deeper insights into customer feedback.
- Feature Selection: Not all features contribute equally to model performance. Techniques for feature selection include: Â
- Correlation analysis to identify and remove redundant features, streamlining the model and improving interpretability.
- Recursive feature elimination to systematically remove less important features, ensuring that only the most impactful variables are included.
- Encoding Categorical Variables: Categorical data must be converted into numerical formats for modeling. Common methods include: Â
- One-hot encoding: Creating binary columns for each category, which is essential for algorithms that require numerical input.
- Label encoding: Assigning a unique integer to each category, simplifying the modeling process.
- Binning: Grouping continuous variables into discrete bins can help capture non-linear relationships. For instance, age can be binned into ranges like 0-18, 19-35, etc., allowing for more nuanced analysis.
- Polynomial Features: Creating polynomial combinations of features can help capture interactions between variables, enhancing model complexity and performance, which can lead to more accurate predictions.
4.4. Model Training and Validation
Model training and validation are essential steps in building a robust predictive model. This phase involves selecting the right algorithms, training the model on the data, and validating its performance, all of which are critical for delivering effective solutions to our clients.
- Choosing the Right Algorithm: The choice of algorithm depends on the problem type (classification, regression, etc.) and the nature of the data. Common algorithms include: Â
- Linear regression for continuous outcomes.
- Decision trees for both classification and regression tasks.
- Neural networks for complex patterns in large datasets, which can be particularly beneficial for clients with extensive data.
- Splitting the Data: Data should be divided into training and testing sets to evaluate model performance. A common split is 80% for training and 20% for testing, ensuring that the model is tested on unseen data.
- Training the Model: During training, the model learns from the training data. This involves adjusting parameters to minimize the error between predicted and actual outcomes, which is crucial for achieving high accuracy.
- Hyperparameter Tuning: Fine-tuning model parameters can significantly improve performance. Techniques include: Â
- Grid search: Testing a range of hyperparameter values to find the optimal settings.
- Random search: Randomly sampling hyperparameter combinations, which can be more efficient in certain scenarios.
- Validation Techniques: To ensure the model generalizes well to unseen data, various validation techniques can be employed: Â
- Cross-validation: Dividing the data into multiple subsets to train and validate the model multiple times, providing a more robust evaluation.
- Holdout validation: Using a separate validation set to assess model performance, ensuring that the model is not overfitting.
- Performance Metrics: Evaluating model performance is crucial. Common metrics include: Â
- Accuracy for classification tasks.
- Mean Absolute Error (MAE) for regression tasks.
- F1 score for balancing precision and recall in classification, which is essential for applications where false positives and negatives carry significant costs.
By following these steps in data cleaning and preprocessing, feature engineering, and model training and validation, Rapid Innovation empowers clients to build robust predictive models that deliver accurate and reliable results, ultimately driving greater ROI and achieving business goals efficiently and effectively.
5. Prediction Models and Algorithms
Prediction models and algorithms are essential tools in data science and analytics, enabling organizations to forecast outcomes based on historical data. These models leverage statistical techniques and computational power to identify patterns and make informed predictions. The two primary categories of prediction models are machine learning models and deep learning networks.
5.1. Machine Learning Models

‍
Machine learning (ML) models are algorithms that learn from data to make predictions or decisions without being explicitly programmed. They are widely used across various industries for tasks such as classification, regression, and clustering.
- Types of Machine Learning: Â
- Supervised Learning: Involves training a model on labeled data, where the outcome is known. Common algorithms include linear regression, decision trees, and support vector machines.
- Unsupervised Learning: Deals with unlabeled data, aiming to find hidden patterns. Examples include k-means clustering and hierarchical clustering.
- Reinforcement Learning: Focuses on training models through trial and error, using feedback from actions taken. This is often used in robotics and game playing.
- Key Features: Â
- Scalability: Machine learning models can handle large datasets, making them suitable for big data applications.
- Adaptability: These models can improve over time as they are exposed to more data, enhancing their predictive accuracy.
- Interpretability: Many machine learning algorithms provide insights into how predictions are made, which is crucial for decision-making.
- Applications: Â
- Fraud detection in finance: Rapid Innovation has successfully implemented ML models to identify fraudulent transactions, significantly reducing financial losses for clients.
- Predictive maintenance in manufacturing: By analyzing equipment data, we help clients predict failures before they occur, optimizing maintenance schedules and reducing downtime.
- Customer segmentation in marketing: Our ML solutions enable businesses to tailor marketing strategies based on customer behavior, leading to increased engagement and sales.
- Demand prediction models: These models help businesses forecast future demand for products, allowing for better inventory management and resource allocation.
- Churn prediction model: By analyzing customer behavior, we can identify those at risk of leaving, enabling proactive retention strategies.
- Sales forecasting models: These models utilize historical sales data to predict future sales trends, aiding in strategic planning.
5.2. Deep Learning Networks
Deep learning (DL) networks are a subset of machine learning that utilize neural networks with multiple layers to analyze various forms of data. They are particularly effective for complex tasks such as image and speech recognition.
- Structure of Deep Learning Networks: Â
- Neural Networks: Composed of interconnected nodes (neurons) that process input data. Each layer transforms the data, allowing the model to learn intricate patterns.
- Convolutional Neural Networks (CNNs): Specialized for processing grid-like data, such as images. They excel in tasks like image classification and object detection.
- Recurrent Neural Networks (RNNs): Designed for sequential data, making them ideal for tasks like natural language processing and time series analysis, including time series forecasting.
- Advantages: Â
- High Accuracy: Deep learning models often outperform traditional machine learning models in tasks involving large datasets and complex patterns.
- Feature Extraction: They automatically identify relevant features from raw data, reducing the need for manual feature engineering.
- Versatility: Deep learning can be applied to various domains, including healthcare, finance, and autonomous vehicles.
- Challenges: Â
- Data Requirements: Deep learning models typically require large amounts of labeled data for training, which can be a barrier in some applications.
- Computational Power: Training deep learning models demands significant computational resources, often necessitating specialized hardware like GPUs.
- Interpretability: These models can be seen as "black boxes," making it difficult to understand how they arrive at specific predictions.
In conclusion, both machine learning models and deep learning networks play crucial roles in prediction algorithms. While machine learning offers a range of techniques suitable for various applications, such as time series forecasting and forecasting using regression, deep learning excels in handling complex data types and achieving high accuracy. At Rapid Innovation, we leverage these advanced predictive models, including Bayesian forecast and forecasting ARIMA model, to help our clients achieve greater ROI by making data-driven decisions that enhance operational efficiency and drive business growth. Understanding the strengths and limitations of each approach is essential for selecting the right model for specific predictive tasks. For more insights on AI subfields and technologies.
5.3. Time Series Analysis
Time series analysis is a statistical technique used to analyze time-ordered data points. It is essential in various fields such as finance, economics, and environmental science. The primary goal is to identify trends, seasonal patterns, and cyclical behaviors in data over time.
- Key components of time series analysis include: Â
- Trend: The long-term movement in the data, indicating whether it is increasing, decreasing, or remaining constant.
- Seasonality: Regular patterns that repeat over specific intervals, such as monthly or quarterly.
- Cyclic patterns: Fluctuations that occur at irregular intervals, often influenced by economic or environmental factors.
- Common methods used in time series analysis: Â
- ARIMA (AutoRegressive Integrated Moving Average): A popular statistical method for forecasting future points in a series.
- Exponential Smoothing: A technique that applies decreasing weights to past observations, making it useful for short-term forecasting.
- Seasonal Decomposition: Breaking down a time series into its components to better understand underlying patterns.
- Applications of time series analysis: Â
- Stock market analysis: Predicting stock prices based on historical data.
- Sales forecasting: Estimating future sales based on past performance.
- Weather forecasting: Analyzing historical weather data to predict future conditions.
At Rapid Innovation, we leverage time series analysis to help clients optimize their operations and make data-driven decisions. For instance, by implementing ARIMA models, we have assisted a retail client in accurately forecasting sales, leading to a 20% reduction in inventory costs and a significant increase in ROI. We also utilize time series analysis in regression analysis of time series to enhance our forecasting capabilities.
5.4. Natural Language Processing
Natural Language Processing (NLP) is a branch of artificial intelligence that focuses on the interaction between computers and human language. It enables machines to understand, interpret, and generate human language in a valuable way.
- Key components of NLP include: Â
- Tokenization: The process of breaking down text into smaller units, such as words or phrases.
- Sentiment Analysis: Determining the emotional tone behind a series of words, often used in social media monitoring.
- Named Entity Recognition (NER): Identifying and classifying key entities in text, such as names, organizations, and locations.
- Techniques used in NLP: Â
- Machine Learning: Algorithms that learn from data to improve their performance over time.
- Deep Learning: A subset of machine learning that uses neural networks to analyze complex patterns in data.
- Transformers: Advanced models like BERT and GPT that have revolutionized NLP by enabling better context understanding.
- Applications of NLP: Â
- Chatbots: Automating customer service interactions through conversational agents.
- Text summarization: Condensing large volumes of text into concise summaries.
- Language translation: Converting text from one language to another with high accuracy.
At Rapid Innovation, we harness NLP to enhance customer engagement and streamline communication. For example, our implementation of advanced chatbots has improved response times for a client by 50%, resulting in higher customer satisfaction and retention rates.
5.5. Hybrid Approaches
Hybrid approaches combine multiple methodologies or techniques to leverage their strengths and mitigate weaknesses. In data science and machine learning, hybrid models can enhance predictive accuracy and provide more robust solutions.
- Characteristics of hybrid approaches: Â
- Integration of models: Combining different algorithms, such as blending machine learning with rule-based systems.
- Multi-modal data: Utilizing various data types, such as text, images, and numerical data, to improve analysis.
- Ensemble methods: Techniques like bagging and boosting that combine predictions from multiple models to enhance performance.
- Benefits of hybrid approaches: Â
- Improved accuracy: By integrating different models, hybrid approaches can achieve better predictive performance.
- Flexibility: They can adapt to various types of data and problems, making them versatile in application.
- Robustness: Combining models can reduce the risk of overfitting and improve generalization to unseen data.
- Examples of hybrid approaches: Â
- Stacked Generalization: A method where multiple models are trained and their predictions are used as input for a final model.
- Feature Engineering: Using domain knowledge to create new features that enhance model performance.
- Transfer Learning: Applying knowledge gained from one task to improve performance on a related task, particularly in deep learning contexts.
At Rapid Innovation, we employ hybrid approaches to deliver tailored solutions that meet our clients' unique needs. For instance, by integrating machine learning with rule-based systems, we have developed a predictive maintenance solution for a manufacturing client, resulting in a 30% reduction in downtime and substantial cost savings. Our expertise in time series and regression analysis further enhances our hybrid modeling capabilities. For more information on Natural Language Processing, visit this link.
6. Market Indicators and Metrics
Market indicators and metrics are essential tools for traders and investors to assess the performance of financial markets. They provide insights into market trends, potential price movements, and overall economic health. Understanding these indicators can help in making informed investment decisions, ultimately leading to greater returns on investment (ROI). This is similar to how lead generation KPIs and digital key performance indicators are used in marketing to measure effectiveness and success.
6.1. Technical Indicators
Technical indicators are mathematical calculations based on historical price and volume data. They help traders identify trends, reversals, and potential entry or exit points in the market. Some commonly used technical indicators include:
- Moving Averages: These smooth out price data to identify trends over a specific period. The two most popular types are the Simple Moving Average (SMA) and the Exponential Moving Average (EMA).
- Relative Strength Index (RSI): This momentum oscillator measures the speed and change of price movements. It ranges from 0 to 100 and is typically used to identify overbought or oversold conditions.
- Bollinger Bands: These consist of a middle band (SMA) and two outer bands that represent standard deviations from the SMA. They help traders assess volatility and potential price reversals.
- MACD (Moving Average Convergence Divergence): This indicator shows the relationship between two moving averages of a security’s price. It helps identify potential buy and sell signals.
- Volume: Analyzing trading volume can provide insights into the strength of a price movement. High volume during a price increase suggests strong buying interest, while high volume during a price decrease indicates strong selling pressure.
Technical indicators are widely used in various trading strategies, including day trading, swing trading, and long-term investing. They can be combined to create a comprehensive trading system that enhances decision-making. At Rapid Innovation, we leverage AI algorithms to analyze these technical indicators, providing clients with actionable insights that can lead to improved trading outcomes and higher ROI, much like how campaign KPIs and demand generation KPIs are utilized to optimize marketing efforts.
6.2. Fundamental Analysis
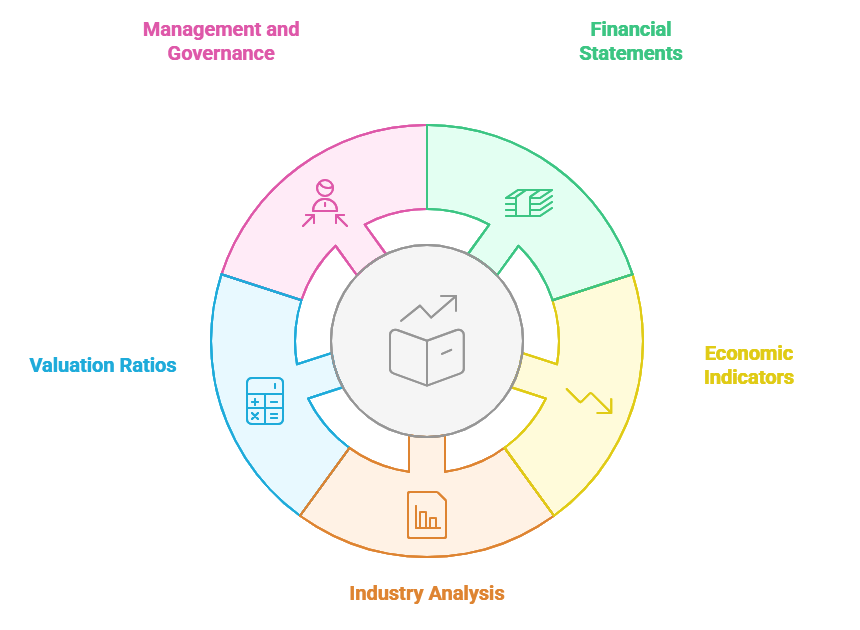
‍
Fundamental analysis involves evaluating a security's intrinsic value by examining related economic, financial, and other qualitative and quantitative factors. This approach is essential for long-term investors who seek to understand the underlying value of an asset. Key components of fundamental analysis include:
- Financial Statements: Analyzing a company's income statement, balance sheet, and cash flow statement provides insights into its financial health. Key metrics include revenue, profit margins, debt levels, and cash flow.
- Economic Indicators: Macroeconomic factors such as GDP growth, unemployment rates, inflation, and interest rates can significantly impact market performance. Understanding these indicators helps investors gauge the overall economic environment.
- Industry Analysis: Evaluating the competitive landscape and market trends within a specific industry can provide context for a company's performance. Factors such as market share, growth potential, and regulatory environment are crucial.
- Valuation Ratios: Common ratios used in fundamental analysis include Price-to-Earnings (P/E), Price-to-Book (P/B), and Dividend Yield. These ratios help investors assess whether a stock is overvalued or undervalued compared to its peers.
- Management and Governance: The quality of a company's management team and its governance practices can influence its long-term success. Investors often consider the track record of executives and board members when making investment decisions.
Fundamental analysis is particularly useful for value investors who seek to identify undervalued stocks with strong growth potential. By focusing on the underlying factors that drive a company's performance, investors can make more informed decisions that align with their investment goals. Rapid Innovation can assist clients in integrating AI-driven analytics into their fundamental analysis processes, enhancing their ability to identify investment opportunities and optimize their portfolios for greater ROI, similar to how B2B KPIs and ABM KPIs are used to measure success in business strategies. For more insights on automated trading solutions.
6.3. Market Sentiment
Market sentiment refers to the overall attitude of investors toward a particular security or financial market. It is a crucial factor that can influence price movements and trading decisions. Understanding market sentiment, including fx market sentiment and stock sentiment analysis, can help traders and investors gauge the mood of the market, which can lead to more informed investment choices.
- Bullish vs. Bearish Sentiment: Â
- Bullish sentiment indicates optimism, where investors expect prices to rise.
- Bearish sentiment reflects pessimism, with expectations of falling prices.
- Indicators of Market Sentiment: Â
- Surveys and polls, such as the American Association of Individual Investors (AAII) sentiment survey, provide insights into investor attitudes.
- Social media and news sentiment analysis, including sentiment analysis for stocks and sentiment analysis trading, can also reveal public perception and mood.
- Impact on Trading: Â
- Positive sentiment can lead to increased buying activity, driving prices higher.
- Negative sentiment may result in selling pressure, causing prices to decline.
- Contrarian Indicators: Â
- Some investors use market sentiment as a contrarian indicator, buying when sentiment is overly negative and selling when it is overly positive. Tools like stock sentiment tracker can assist in this analysis.
6.4. Volume Analysis
Volume analysis involves examining the number of shares or contracts traded in a security or market during a specific period. It is a vital tool for traders and investors, as it provides insights into the strength or weakness of price movements.
- High Volume vs. Low Volume: Â
- High trading volume often indicates strong investor interest and can confirm price trends.
- Low volume may suggest a lack of interest, making price movements less reliable.
- Volume Trends: Â
- An increase in volume during a price rise can signal a strong uptrend.
- Conversely, rising prices with declining volume may indicate a potential reversal.
- Volume Indicators: Â
- On-Balance Volume (OBV) and Volume Moving Averages are popular tools used to analyze volume trends.
- Volume spikes can indicate significant news or events impacting the security.
- Volume and Market Sentiment: Â
- Volume can also reflect market sentiment; for instance, high volume during a market rally may suggest bullish sentiment, which can be analyzed through sentiment analysis for trading.
6.5. Volatility Measures
Volatility measures the degree of variation in a trading price series over time. It is a critical concept in finance, as it helps investors understand the risk associated with a particular security or market.
- Types of Volatility: Â
- Historical Volatility: Based on past price movements, calculated using standard deviation.
- Implied Volatility: Derived from options pricing, reflecting market expectations of future volatility.
- Volatility Indicators: Â
- The VIX (Volatility Index) is a popular measure of market volatility, often referred to as the "fear index."
- Bollinger Bands are another tool that uses volatility to indicate potential price movements.
- Impact on Trading Strategies: Â
- High volatility can present opportunities for traders to capitalize on price swings.
- Conversely, it can also increase risk, leading to potential losses.
- Volatility and Market Sentiment: Â
- Increased volatility often correlates with heightened market uncertainty and can reflect bearish sentiment, which can be assessed through sentiment analysis forex.
- Low volatility may indicate a stable market environment, often associated with bullish sentiment, as seen in daily market sentiment reports.
At Rapid Innovation, we leverage advanced AI algorithms to analyze market sentiment, including market sentiment analysis and sentiment analysis of stock market trends, volume, and volatility, providing our clients with actionable insights that enhance their trading strategies. By integrating AI-driven analytics, we help businesses achieve greater ROI through informed decision-making and optimized trading performance. Additionally, our expertise extends to crypto market making services and crypto arbitrage bot development, ensuring that our clients can navigate the complexities of the cryptocurrency market effectively.
7. Implementation Strategy
An effective implementation strategy is crucial for the successful deployment of any system or project. It outlines the steps necessary to ensure that the project meets its objectives and is completed on time and within budget. A well-defined implementation strategy includes planning, execution, monitoring, and evaluation phases.
- Clearly define project goals and objectives.
- Identify key stakeholders and their roles.
- Develop a detailed project timeline with milestones.
- Allocate resources effectively, including budget and personnel.
- Establish communication channels for updates and feedback.
7.1. System Requirements
Understanding system requirements is essential for the successful implementation of any project. System requirements outline the necessary specifications and functionalities that the system must meet to fulfill its intended purpose. These requirements can be categorized as follows:
- Functional Requirements: These describe what the system should do, including specific features and capabilities.
- Non-Functional Requirements: These include performance metrics, security standards, and usability criteria.
- Technical Requirements: These specify the hardware and software needed to support the system, including operating systems, databases, and network infrastructure.
- Compliance Requirements: Ensure that the system adheres to relevant regulations and standards, such as GDPR or HIPAA.
Gathering system requirements typically involves engaging with stakeholders through interviews and surveys, conducting workshops to brainstorm and refine requirements, and reviewing existing documentation and systems for insights.
7.2. Integration Process
The integration process is a critical phase in the implementation strategy, as it involves combining various components of the system to work together seamlessly. A successful integration process ensures that all parts of the system communicate effectively and function as intended. The key steps in the integration process include:
- Assess Compatibility: Evaluate the compatibility of existing systems and new components to identify potential integration challenges.
- Develop an Integration Plan: Create a detailed plan that outlines the steps for integrating different system components, including timelines and responsibilities.
- Use Middleware Solutions: Consider using middleware to facilitate communication between disparate systems, ensuring data flows smoothly.
- Conduct Testing: Perform rigorous testing to identify and resolve any issues before full deployment. This includes unit testing, integration testing, and user acceptance testing.
- Monitor and Optimize: After integration, continuously monitor system performance and make necessary adjustments to optimize functionality.
By following a structured implementation strategy, organizations can enhance the likelihood of project success, ensuring that the system meets its intended goals and delivers value to stakeholders. At Rapid Innovation, we leverage our expertise in AI to streamline these processes, ensuring that our clients achieve greater ROI through efficient project execution and integration. This includes developing an implementation strategy example and utilizing a strategy implementation plan to guide our efforts. We also focus on strategic planning and implementation to align our projects with broader organizational goals, ensuring that our approach is comprehensive and effective. For more insights on AI development and integration, you can refer to our AI Development and Integration Guide for Individuals.
7.3. Testing and Validation
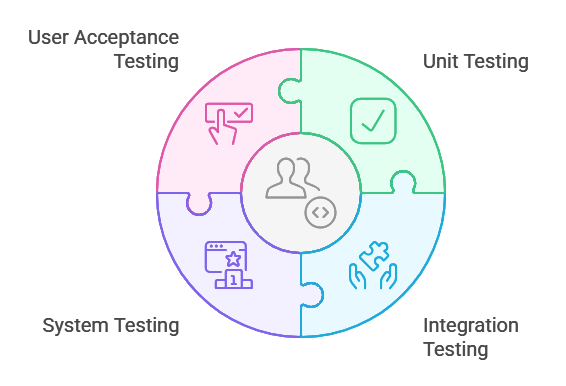
‍
Testing and validation are critical steps in the development process, ensuring that a product meets its intended requirements and functions correctly. This phase involves various methodologies to assess the quality and reliability of the product, which is essential for achieving greater ROI for our clients at Rapid Innovation.
- Types of Testing: Â
- Unit Testing: Focuses on individual components to verify their correctness, allowing for early detection of issues that could lead to costly fixes later.
- Integration Testing: Ensures that different modules work together as expected, minimizing integration problems that can delay project timelines.
- System Testing: Validates the complete and integrated software product, ensuring it meets all specified requirements before deployment.
- User Acceptance Testing (UAT): Confirms that the product meets user needs and requirements, which is crucial for user adoption and satisfaction.
- Validation Techniques: Â
- Manual Testing: Involves human testers to execute test cases and report issues, providing a thorough examination of user experience.
- Automated Testing: Uses scripts and tools to perform tests, increasing efficiency and coverage, which can significantly reduce time-to-market.
- Performance Testing: Assesses the responsiveness, speed, and stability under load, ensuring the product can handle real-world usage scenarios.
- Importance of Testing: Â
- Identifies defects early in the development cycle, reducing costs and enhancing overall project efficiency.
- Enhances user satisfaction by ensuring a reliable product that meets expectations.
- Complies with industry standards and regulations, which is vital for maintaining credibility and trust with clients.
- Verification and Validation: The processes of verification and validation in software testing are essential to ensure that the product meets the specified requirements and is fit for use. This includes software validation testing and the verification and validation in software engineering.
- Validation Testing in Software Testing: This involves specific techniques to ensure that the software meets the needs of the users and stakeholders, often illustrated through examples of validation in software testing.
- Verification Testing and Validation Testing: These methodologies work together to ensure that the software is both built correctly (verification) and meets the intended use (validation).
- Requirements Traceability Verification Matrix: This tool is crucial for tracking requirements throughout the testing process, ensuring that all requirements are verified and validated.
- VV Model in Software Testing: The verification and validation model in software testing provides a structured approach to ensure quality throughout the development lifecycle.
At Rapid Innovation, we also specialize in Stable Diffusion Development to enhance our testing and validation processes. Additionally, we explore the role of AI agents in software testing to further improve our methodologies.
7.4. Performance Optimization
Performance optimization is essential for enhancing the efficiency and speed of a product. It involves analyzing and improving various aspects of the system to ensure it operates at its best, ultimately leading to a higher return on investment for our clients.
- Key Areas of Focus: Â
- Code Optimization: Refactoring code to improve execution speed and reduce resource consumption, which can lead to lower operational costs.
- Database Optimization: Enhancing database queries and indexing to speed up data retrieval, improving overall application performance.
- Network Optimization: Reducing latency and improving bandwidth usage for better data transfer, ensuring a seamless user experience.
- Techniques for Optimization: Â
- Caching: Storing frequently accessed data in memory to reduce load times, which can significantly enhance user satisfaction.
- Load Balancing: Distributing workloads across multiple servers to ensure no single server is overwhelmed, improving reliability and uptime.
- Asynchronous Processing: Allowing tasks to run in the background, improving user experience by making applications more responsive.
- Benefits of Performance Optimization: Â
- Improved user experience through faster load times and responsiveness, leading to higher user retention rates.
- Increased scalability, allowing the system to handle more users and data without performance degradation.
- Reduced operational costs by minimizing resource usage, which directly contributes to a better ROI.
7.5. Maintenance Protocols
Maintenance protocols are essential for ensuring the long-term functionality and reliability of a product. These protocols outline the procedures for regular updates, bug fixes, and system enhancements, which are crucial for sustaining client satisfaction and operational efficiency.
- Types of Maintenance: Â
- Corrective Maintenance: Fixing defects and issues as they arise, ensuring the product remains functional and reliable.
- Adaptive Maintenance: Updating the system to accommodate changes in the environment or technology, keeping the product relevant and competitive.
- Perfective Maintenance: Enhancing system performance and features based on user feedback, which is vital for continuous improvement.
- Maintenance Best Practices: Â
- Regular Updates: Implementing software updates to address security vulnerabilities and improve functionality, protecting client investments.
- Documentation: Keeping detailed records of changes, issues, and resolutions to facilitate future maintenance, ensuring a smoother process.
- Monitoring: Continuously tracking system performance and user feedback to identify areas for improvement, allowing for proactive adjustments.
- Importance of Maintenance Protocols: Â
- Ensures system reliability and availability over time, which is essential for maintaining client trust.
- Reduces the risk of system failures and downtime, protecting revenue streams and user satisfaction.
- Enhances user satisfaction by providing a consistently high-quality experience, leading to increased loyalty and referrals.
At Rapid Innovation, we leverage these methodologies to help our clients achieve their business goals efficiently and effectively, ensuring a greater return on their investments.
8. Risk Management
Risk management is a crucial aspect of any investment strategy, particularly in volatile markets. It involves identifying, assessing, and prioritizing risks followed by coordinated efforts to minimize, monitor, and control the probability or impact of unfortunate events. Effective risk management can protect investments and enhance returns.
- Establishing a risk management framework is essential for any investor.
- It helps in making informed decisions and maintaining a balanced portfolio.
- Regularly reviewing and adjusting risk management strategies, including risk mitigation strategies, is vital to adapt to changing market conditions.
8.1. Prediction Accuracy
Prediction accuracy refers to the ability to forecast market movements and trends accurately. In the context of risk management, it plays a significant role in making informed investment decisions. Accurate predictions can lead to better investment choices and reduced risk exposure. Utilizing advanced analytical tools and techniques can enhance prediction accuracy, while historical data analysis, market trends, and economic indicators are essential for improving forecasting.
At Rapid Innovation, we leverage AI-driven analytics to improve prediction accuracy for our clients. By employing sophisticated statistical models and machine learning algorithms, we help investors analyze vast datasets, enabling them to make data-driven decisions.
Investors should consider the following to improve prediction accuracy:
- Employing statistical models and algorithms to analyze data.
- Keeping abreast of market news and economic reports.
- Engaging in scenario analysis to understand potential outcomes.
However, it is important to note that no prediction method is foolproof. Market conditions can change rapidly, and unforeseen events can impact accuracy. Therefore, maintaining a flexible approach to risk management, including risk response strategies, is crucial.
8.2. Market Volatility
Market volatility refers to the degree of variation in trading prices over time. High volatility indicates a significant price fluctuation, while low volatility suggests stability. Understanding market volatility is essential for effective risk management. Volatile markets can present both opportunities and risks for investors. High volatility can lead to increased uncertainty, making it challenging to predict market movements. Investors must be prepared for sudden price changes and adjust their strategies accordingly.
To manage risks associated with market volatility, consider the following strategies:
- Diversifying investments across various asset classes to spread risk.
- Utilizing stop-loss orders to limit potential losses.
- Monitoring market trends and adjusting portfolios in response to volatility.
At Rapid Innovation, we assist clients in developing robust risk management frameworks that incorporate real-time data analysis and predictive modeling. This enables investors to respond proactively to market fluctuations.
Investors should also be aware of the factors that contribute to market volatility, such as:
- Economic indicators (e.g., unemployment rates, inflation).
- Geopolitical events (e.g., elections, trade disputes).
- Market sentiment and investor behavior.
By understanding and managing market volatility, including implementing risk handling strategies, investors can better protect their portfolios and capitalize on potential opportunities. Rapid Innovation is committed to providing the tools and insights necessary for our clients to navigate these challenges effectively, including strategic risk management strategies and best risk management strategy trading. For those interested in expanding their investment opportunities, our AI agents for investment strategy can provide innovative solutions tailored to your needs.
8.3. System Reliability
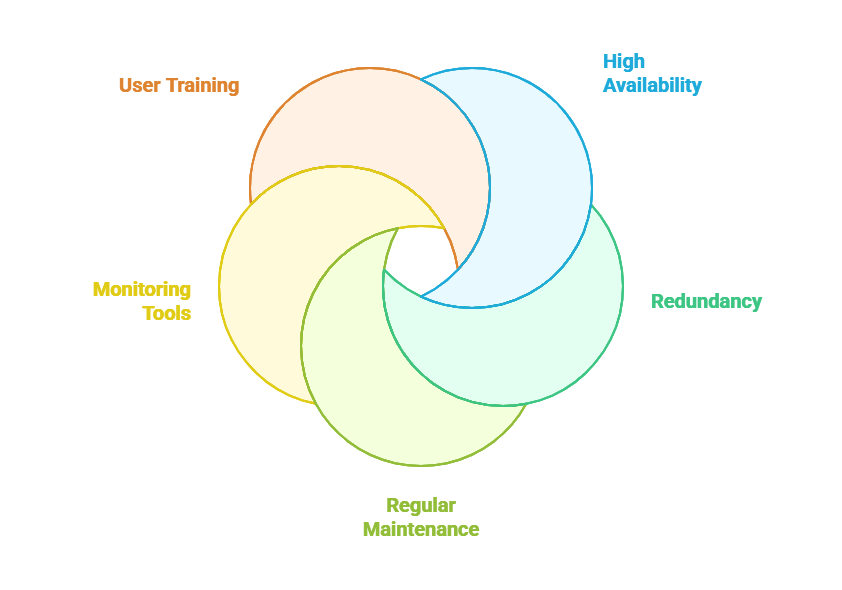
‍
System reliability refers to the ability of a system to consistently perform its intended functions without failure. In the context of technology and data management, system reliability is crucial for ensuring that operations run smoothly and that users can depend on the system for accurate information. At Rapid Innovation, we leverage advanced AI solutions to enhance system reliability, ensuring that our clients can achieve their business goals efficiently.
- High availability: Our AI-driven systems are designed to minimize downtime, ensuring that users can access services whenever needed. For instance, we implement predictive analytics to foresee potential system failures and proactively address them, similar to practices in site reliability engineering at Google.
- Redundancy: We advocate for implementing backup systems and components, which can help maintain functionality in case of a failure. Our solutions include automated failover systems that ensure seamless transitions during outages, akin to the strategies employed by site reliability engineers at Google.
- Regular maintenance: Routine checks and updates are integral to our approach, preventing unexpected breakdowns and enhancing overall system performance. Our AI tools can automate maintenance schedules, ensuring that systems are always up-to-date, much like the principles of reliability engineering and system safety.
- Monitoring tools: Utilizing sophisticated software to track system performance, we help identify potential issues before they escalate. Our real-time monitoring solutions provide actionable insights, allowing for timely interventions, which is a key aspect of system reliability engineering.
- User training: We emphasize the importance of user training to reduce the likelihood of human error, which can compromise system reliability. Our tailored training programs equip users with the knowledge to effectively utilize AI systems, similar to the training provided for hardware reliability engineers.
8.4. Data Quality
Data quality is a critical aspect of data management that refers to the accuracy, completeness, consistency, and reliability of data. High-quality data is essential for making informed decisions and driving business success. Rapid Innovation employs AI methodologies to enhance data quality, ensuring our clients can maximize their ROI.
To ensure data quality, organizations must focus on several key areas:
- Accuracy: Our AI algorithms are designed to ensure that data is correct and free from errors, facilitating reliable analysis and reporting.
- Completeness: We assist organizations in collecting and storing all necessary data to provide a comprehensive view of the subject matter, enhancing decision-making capabilities.
- Consistency: Our solutions ensure that data remains uniform across different systems and platforms, avoiding discrepancies that can hinder business operations.
- Timeliness: We implement systems that ensure data is up-to-date, reflecting the current state of affairs and enabling timely decision-making.
- Validation processes: Our AI-driven validation processes help maintain data quality by identifying and correcting errors, thus supporting informed business strategies.
8.5. Compliance Issues
Compliance issues refer to the challenges organizations face in adhering to laws, regulations, and standards that govern their operations. Non-compliance can lead to legal penalties, financial losses, and reputational damage. Rapid Innovation provides consulting solutions to help organizations navigate compliance challenges effectively.
To address compliance issues, organizations should consider the following:
- Regulatory frameworks: We help organizations stay informed about relevant regulations, such as GDPR, HIPAA, or PCI-DSS, ensuring compliance through tailored solutions.
- Data protection: Our robust data security measures are essential to protect sensitive information and comply with privacy laws, utilizing AI to enhance security protocols.
- Audits and assessments: We conduct regular audits to identify compliance gaps and ensure that policies and procedures are being followed, providing clients with peace of mind.
- Employee training: Our training programs educate staff about compliance requirements, fostering a culture of accountability and reducing the risk of violations.
- Documentation: We assist in maintaining thorough records of compliance efforts, providing evidence of adherence to regulations and facilitating audits, ultimately supporting our clients' business objectives.
9. User Interface and Experience
User interface (UI) and user experience (UX) are critical components in the design of any application or website. A well-designed UI enhances usability, while a positive UX ensures that users find value in the product. Together, they contribute to user satisfaction and retention.
9.1. Dashboard Design
Dashboard design is a vital aspect of UI/UX, especially for applications that require data monitoring and analysis. A well-structured dashboard provides users with a clear overview of key metrics and insights.
- Clarity and Simplicity: The dashboard should present information in a straightforward manner. Avoid clutter by limiting the number of elements displayed at once and use whitespace effectively to separate different sections.
- Customization: Allow users to customize their dashboards according to their preferences. This can include rearranging widgets, selecting which metrics to display, and choosing color themes. Customization enhances user engagement and satisfaction, which is a key aspect of user interface design.
- Responsiveness: Ensure that the dashboard is responsive across various devices, including desktops, tablets, and smartphones. A responsive design adapts to different screen sizes, providing a seamless experience for users, which is essential in ui and ux design.
- Real-time Data: Incorporate real-time data updates to keep users informed. This is particularly important for applications that track performance metrics, sales figures, or social media engagement, aligning with the principles of ux design ui design.
- Intuitive Navigation: Design the dashboard with intuitive navigation in mind. Users should easily find the information they need without extensive searching. Use clear labels and logical groupings for different sections, which is a fundamental aspect of user interface ux.
- Feedback Mechanisms: Implement feedback mechanisms, such as notifications or alerts, to inform users of important changes or updates. This keeps users engaged and aware of critical information, enhancing the overall user interface design.
9.2. Visualization Tools
Visualization tools play a crucial role in transforming complex data into understandable formats. Effective data visualization enhances user comprehension and decision-making.
- Variety of Charts and Graphs: Offer a range of visualization options, including bar charts, line graphs, pie charts, and heat maps. Different types of data may be better represented by specific visual formats, which is important in design ux ui.
- Interactive Elements: Incorporate interactive elements that allow users to explore data further. Features like tooltips, zooming, and filtering can help users gain deeper insights into the data presented, a key feature in ui interface design.
- Color Schemes: Use color schemes strategically to convey information. Colors can represent different categories or highlight trends. Ensure that the color choices are accessible to all users, including those with color blindness, which is a consideration in ux design agencies.
- Data Context: Provide context for the data being visualized. This can include annotations, legends, and descriptions that help users understand what the data represents and its significance, enhancing the user experience in ui ux designer roles.
- Performance Optimization: Ensure that visualization tools are optimized for performance. Slow-loading visualizations can frustrate users and lead to disengagement. Use efficient coding practices and consider data caching techniques, which are essential in ux designer ui designer collaborations.
- User Education: Offer tutorials or guides on how to use the visualization tools effectively. Educating users on how to interpret the data can enhance their overall experience and increase the value they derive from the application, a critical aspect of user interface design online.
By focusing on user interface and experience, particularly in dashboard design and visualization tools, Rapid Innovation can help clients create applications that significantly improve user engagement and satisfaction, ultimately leading to greater ROI. Our expertise in AI-driven design solutions ensures that your applications not only meet user needs but also adapt to evolving market demands, providing a competitive edge in your industry, especially in the realm of AI for product design optimization ux and ui designers.
9.3. Alert Systems

‍
Alert systems are crucial components of modern software applications, particularly in fields like cybersecurity, finance, and healthcare. These systems help users stay informed about critical events or changes in their environment, ultimately enhancing operational efficiency and decision-making.
- Real-time notifications: Alert systems provide immediate updates on significant occurrences, ensuring users can respond promptly. For instance, in a financial trading platform, real-time alerts can notify traders of market fluctuations, enabling them to make timely investment decisions.
- Customizable alerts: Users can often tailor alerts based on their preferences, such as setting thresholds for specific metrics or choosing preferred communication channels (email, SMS, etc.). This flexibility allows organizations to prioritize alerts that matter most to their operations, such as alerts from medical alert systems or life alert companies.
- Integration capabilities: Many alert systems can integrate with other tools and platforms, allowing for a seamless flow of information and enhancing overall efficiency. For example, integrating an alert system with a project management tool can streamline communication and task management, similar to how a first alert carbon monoxide alarm integrates with home safety systems.
- Escalation procedures: In case of critical alerts, systems can escalate notifications to higher management or specialized teams, ensuring that urgent issues receive the necessary attention. This feature is particularly beneficial in healthcare settings, where timely responses can be life-saving, such as with medical alert devices for seniors.
- Historical data tracking: Alert systems often maintain logs of past alerts, enabling users to analyze trends and improve future responses. By leveraging historical data, organizations can refine their alert criteria and enhance their overall risk management strategies, which is essential for systems like driveway security alarms or motion detector alarms.
At Rapid Innovation, we leverage these advanced features in our AI development and consulting solutions to help clients achieve greater ROI. By implementing robust alert systems, such as personal alert safety systems and medical alarm systems, comprehensive reporting features, and extensive customization options, we empower organizations to optimize their operations, make informed decisions, and ultimately drive business success through our AI business automation solutions.
9.4. Reporting Features
Reporting features are essential for analyzing data and making informed decisions. They provide users with insights into performance, trends, and areas for improvement, ultimately driving better business outcomes.
- Custom report generation: Users can create tailored reports that focus on specific metrics or timeframes, allowing for a more in-depth analysis of relevant data. This capability enables organizations to track KPIs effectively and adjust strategies accordingly, such as monitoring the effectiveness of life alert pricing.
- Visual data representation: Many reporting tools offer charts, graphs, and dashboards that make complex data easier to understand at a glance. Visual representations can help stakeholders quickly grasp performance trends and make data-driven decisions, including those related to first alert fire and carbon monoxide alarms.
- Scheduled reporting: Users can automate the generation and distribution of reports, ensuring that stakeholders receive timely updates without manual intervention. This automation saves time and ensures that decision-makers have access to the latest information.
- Data export options: Reporting features often allow users to export data in various formats (CSV, PDF, etc.), facilitating sharing and further analysis. This flexibility supports collaboration across teams and departments.
- Interactive reporting: Some advanced reporting tools enable users to interact with data, drilling down into specific areas for a more detailed examination. This interactivity empowers users to explore data insights and uncover hidden opportunities.
9.5. Customization Options
Customization options are vital for ensuring that software applications meet the unique needs of different users and organizations. They allow for a more personalized experience and can significantly enhance user satisfaction, leading to improved productivity.
- User interface customization: Users can often modify the layout, colors, and themes of the application to align with their preferences or corporate branding. A tailored interface can improve user engagement and satisfaction, especially for applications related to medical alert systems or life alert necklaces.
- Feature toggles: Many applications allow users to enable or disable specific features based on their requirements, ensuring that they only see what is relevant to them. This adaptability can streamline workflows and enhance user experience.
- Workflow customization: Users can often tailor workflows to match their processes, improving efficiency and reducing the learning curve for new users. Custom workflows can lead to faster onboarding and increased productivity.
- Role-based access: Customization options frequently include setting permissions based on user roles, ensuring that sensitive information is only accessible to authorized personnel. This security measure is crucial for maintaining data integrity and compliance.
- API access: For organizations with specific needs, many applications offer API access, allowing for deeper integration with other systems and enabling custom functionality. This capability can enhance operational efficiency and support unique business processes.
At Rapid Innovation, we leverage these advanced features in our AI development and consulting solutions to help clients achieve greater ROI. By implementing robust alert systems, such as personal alert safety systems and medical alarm systems, comprehensive reporting features, and extensive customization options, we empower organizations to optimize their operations, make informed decisions, and ultimately drive business success.
10. Performance Metrics
Performance metrics are essential for evaluating the effectiveness of predictive models and algorithms. They provide insights into how well a model performs in terms of accuracy, reliability, and overall effectiveness. Understanding these performance metrics is crucial for data scientists and analysts to refine their models and improve decision-making processes. Performance metrics help in comparing different models, guide the selection of the best model for a specific task, and indicate areas for improvement in model training.
10.1. Accuracy Measures

‍
Accuracy measures are fundamental in assessing the performance of a predictive model. They quantify how often the model makes correct predictions compared to the total number of predictions made. Common accuracy measures include:
- Overall Accuracy: This is the ratio of correctly predicted instances to the total instances. It is a straightforward metric but can be misleading in imbalanced datasets.
- Precision: This metric indicates the proportion of true positive predictions among all positive predictions made by the model. High precision means that when the model predicts a positive outcome, it is likely to be correct.
- Recall (Sensitivity): Recall measures the proportion of actual positive instances that were correctly identified by the model. High recall indicates that the model successfully captures most of the positive cases.
- F1 Score: The F1 score is the harmonic mean of precision and recall. It provides a balance between the two metrics, making it useful when dealing with imbalanced datasets.
- Area Under the ROC Curve (AUC-ROC): This metric evaluates the model's ability to distinguish between classes. A higher AUC indicates better model performance.
Understanding these accuracy measures allows data scientists to choose the right metric based on the specific context of their problem. For instance, in medical diagnosis, high recall may be prioritized to ensure that most positive cases are identified, even if it means sacrificing some precision.
10.2. Prediction Success Rates
Prediction success rates provide a clear picture of how well a model performs in making accurate predictions. This metric is particularly useful in evaluating the effectiveness of classification models. Key aspects of prediction success rates include:
- Success Rate Calculation: The prediction success rate is calculated by dividing the number of correct predictions by the total number of predictions made. This gives a percentage that reflects the model's overall effectiveness.
- Contextual Relevance: The importance of prediction success rates can vary depending on the application. For example, in fraud detection, a high success rate is crucial to minimize losses, while in customer segmentation, the focus may be on understanding patterns rather than achieving a high success rate.
- Benchmarking: Comparing prediction success rates against industry standards or previous models can help in assessing improvements. This benchmarking can guide further refinements and adjustments to the model.
- Impact of Class Imbalance: In cases where one class significantly outnumbers another, the prediction success rate can be misleading. Therefore, it is essential to consider additional metrics, such as precision and recall, to get a comprehensive view of model performance.
- Real-World Implications: High prediction success rates can lead to better decision-making and resource allocation. For instance, in marketing, accurately predicting customer behavior can enhance targeting strategies and improve return on investment.
By focusing on both accuracy measures and prediction success rates, organizations can develop robust models that not only perform well statistically but also deliver real-world value. At Rapid Innovation, we leverage these performance metrics for predictive models to ensure that our AI solutions are tailored to meet your specific business goals, ultimately driving greater ROI and operational efficiency. Additionally, our expertise in fine-tuning language models enhances the performance of AI systems, ensuring they align with your unique requirements. For more insights on model development, check out our best practices for transformer model development.
10.3. Response Time
Response time is a critical metric in evaluating the performance of any system, particularly in technology and customer service sectors. It refers to the duration it takes for a system to react to a request or an action. A shorter response time is often associated with higher user satisfaction and improved operational efficiency.
- Importance of Response Time: Â
- Enhances user experience by providing quick feedback.
- Reduces frustration and increases engagement.
- Critical for real-time applications, such as online gaming or financial trading.
- Factors Affecting Response Time: Â
- Network latency: Delays caused by data transmission over the internet.
- Server performance: The capability of the server to process requests efficiently.
- Application design: Well-optimized applications can handle requests faster.
- Measuring Response Time: Â
- Use tools like Google PageSpeed Insights or GTmetrix to analyze web application response times.
- Monitor server logs to identify bottlenecks in processing requests.
- Conduct response time analysis using tools like Wireshark to gain deeper insights into network performance.
- Best Practices to Improve Response Time: Â
- Optimize code and database queries to reduce processing time.
- Implement caching strategies to store frequently accessed data.
- Use Content Delivery Networks (CDNs) to decrease latency for users in different geographical locations.
10.4. System Reliability
System reliability refers to the ability of a system to consistently perform its intended functions without failure over a specified period. High reliability is essential for maintaining user trust and ensuring business continuity.
- Key Aspects of System Reliability: Â
- Availability: The system should be operational and accessible when needed.
- Fault tolerance: The system should continue to operate even in the event of a failure.
- Maintainability: The ease with which a system can be repaired or updated.
- Factors Influencing System Reliability: Â
- Hardware quality: High-quality components reduce the likelihood of failure.
- Software robustness: Well-tested software is less prone to bugs and crashes.
- Regular maintenance: Scheduled updates and checks can prevent unexpected downtimes.
- Measuring System Reliability: Â
- Use metrics like Mean Time Between Failures (MTBF) and Mean Time To Repair (MTTR) to assess reliability.
- Conduct regular stress tests to evaluate how the system performs under heavy loads.
- Strategies to Enhance System Reliability: Â
- Implement redundancy: Use backup systems to take over in case of a failure.
- Monitor system performance continuously to identify potential issues before they escalate.
- Invest in training for staff to ensure they can effectively manage and troubleshoot the system.
10.5. ROI Analysis
Return on Investment (ROI) analysis is a financial metric used to evaluate the profitability of an investment relative to its cost. It helps businesses determine whether a project or investment is worth pursuing based on its expected returns.
- Importance of ROI Analysis: Â
- Provides a clear picture of the financial benefits of an investment.
- Aids in decision-making by comparing different investment opportunities.
- Helps in justifying expenditures to stakeholders.
- Key Components of ROI Calculation: Â
- Initial investment: The total cost incurred to implement the project.
- Net profit: The total revenue generated minus the total costs associated with the investment.
- Time frame: The period over which the returns are measured.
- Formula for ROI: Â
- - Â
language="language-roi"```ROI = (Net Profit / Initial Investment) x 100
- This formula provides a percentage that indicates the efficiency of the investment. Â
- Factors to Consider in ROI Analysis:
- Tangible benefits: Direct financial gains from the investment.
- Intangible benefits: Improvements in customer satisfaction, brand reputation, or employee morale.
- Risk assessment: Evaluating potential risks that could impact the expected returns. Â
- Best Practices for Conducting ROI Analysis:
- Use historical data to inform projections and assumptions.
- Consider both short-term and long-term impacts of the investment.
- Regularly review and update the analysis as new data becomes available.
By focusing on response time, system reliability, and ROI analysis, organizations can enhance their operational efficiency, improve user satisfaction, and make informed financial decisions. At Rapid Innovation, we leverage our expertise in AI development to help clients optimize these critical areas, ultimately driving greater ROI and achieving their business goals efficiently and effectively. For more information on our services, check out our AI agent procurement intelligence engine.
11. Case Studies
Case studies provide valuable insights into real-world applications of theories and methodologies. They allow researchers and practitioners to analyze specific instances, drawing lessons that can be applied to similar situations. In the financial sector, case studies on stock market prediction and cryptocurrency analysis are particularly relevant due to the rapid changes and complexities involved.
11.1. Stock Market Prediction
Stock market prediction involves forecasting the future price movements of stocks based on historical data, market trends, and various analytical techniques. This area has garnered significant attention due to its potential for high returns.
- Techniques Used: Â
- Technical analysis: Involves analyzing price charts and patterns to predict future movements.
- Fundamental analysis: Focuses on a company's financial health, including earnings, revenue, and market conditions.
- Machine learning: Algorithms are trained on historical data to identify patterns and make predictions.
- Case Study Example:
A notable case study is the use of machine learning algorithms by hedge funds to predict stock prices. For instance, a hedge fund implemented a deep learning model that analyzed vast amounts of historical stock data, news articles, and social media sentiment. The model achieved a prediction accuracy of over 70%, significantly outperforming traditional methods. This approach is similar to the methodologies used in apple stock price prediction tomorrow and amazon stock price prediction. - Challenges: Â
- Market volatility: Sudden changes can disrupt predictions, as seen in the context of next stock market crash prediction and next market crash prediction.
- Data quality: Inaccurate or incomplete data can lead to poor predictions.
- Overfitting: Models may perform well on historical data but fail in real-time scenarios.
- Impact:
Successful stock market prediction can lead to substantial financial gains for investors and traders. It also contributes to market efficiency by reflecting available information in stock prices. At Rapid Innovation, we leverage advanced machine learning techniques to help clients enhance their predictive capabilities, ultimately driving greater ROI. This includes services like stock market forecast tomorrow and tomorrow share market prediction.
11.2. Cryptocurrency Analysis
Cryptocurrency analysis focuses on evaluating digital currencies to understand their market behavior, trends, and potential for investment. Given the volatility and rapid evolution of cryptocurrencies, this analysis is crucial for investors.
- Techniques Used: Â
- Sentiment analysis: Evaluating social media and news sentiment to gauge public perception.
- Technical analysis: Similar to stock analysis, it involves charting price movements and identifying patterns.
- On-chain analysis: Examining blockchain data to understand transaction volumes, wallet activity, and other metrics.
- Case Study Example:
A prominent case study involved the analysis of Bitcoin's price movements during the 2017 bull run. Researchers utilized sentiment analysis tools to track Twitter activity and news articles. They found a strong correlation between positive sentiment and price increases, suggesting that public perception significantly influenced Bitcoin's value during that period. This is akin to the methodologies used in stock predictor and forecasting stock. - Challenges: Â
- Market manipulation: The cryptocurrency market is susceptible to pump-and-dump schemes, making analysis difficult.
- Regulatory changes: New regulations can impact prices and market behavior unpredictably.
- Data volatility: Cryptocurrency prices can fluctuate dramatically within short time frames, complicating analysis.
- Impact:
Effective cryptocurrency analysis can help investors make informed decisions, manage risks, and capitalize on market opportunities. It also aids in understanding the broader implications of blockchain technology and its potential to disrupt traditional financial systems. At Rapid Innovation, we provide tailored consulting solutions that empower clients to navigate the complexities of cryptocurrency markets, enhancing their investment strategies and maximizing returns. This includes insights into share market predictions and stock exchange prediction.
11.3. Commodity Markets
Commodity markets are platforms where raw materials and primary products are traded. These markets play a crucial role in the global economy, influencing prices and availability of essential goods.
- Types of commodities: Â
- Hard commodities: Natural resources that are mined or extracted, such as oil, gold, and metals.
- Soft commodities: Agricultural products or livestock, including wheat, coffee, and cattle.
- Importance of commodity markets: Â
- Price discovery: Commodity markets help in determining the fair market value of goods based on supply and demand.
- Risk management: Producers and consumers use futures contracts, such as crude oil futures contracts and futures trading, to hedge against price fluctuations.
- Economic indicators: Commodity prices, including wti crude oil price and brent crude oil price, often reflect economic health, as rising prices can indicate increased demand or inflation.
- Key players in commodity markets: Â
- Producers: Farmers, miners, and manufacturers who supply raw materials.
- Consumers: Industries and businesses that require commodities for production.
- Speculators: Investors who trade commodities to profit from price changes.
- Trends in commodity markets: Â
- Increased volatility due to geopolitical tensions and climate change.
- Growing interest in sustainable and ethical sourcing of commodities.
- Technological advancements improving trading efficiency and transparency.
At Rapid Innovation, we leverage AI technologies to analyze market trends and provide predictive insights, enabling our clients to make informed decisions in commodity trading. By utilizing machine learning algorithms, we can help clients optimize their trading strategies, ultimately leading to greater ROI in commodity market trading. Additionally, our expertise extends to security token development services that can enhance the trading experience in commodity markets. For insights on how AI is transforming trading strategies, check out our article on AI in stock trading.
11.4. Forex Trading
Forex trading, or foreign exchange trading, involves the buying and selling of currencies in the global market. It is one of the largest financial markets, with a daily trading volume exceeding $6 trillion.
- Key features of forex trading: Â
- Currency pairs: Currencies are traded in pairs, such as EUR/USD or GBP/JPY, indicating the value of one currency against another.
- Leverage: Traders can control large positions with a relatively small amount of capital, increasing potential profits and risks.
- 24-hour market: Forex trading occurs around the clock, allowing traders to react to global events in real-time.
- Benefits of forex trading: Â
- High liquidity: The forex market is highly liquid, enabling quick transactions and minimal price fluctuations.
- Accessibility: Retail traders can easily access the market through online platforms and brokers.
- Diverse strategies: Traders can employ various strategies, including day trading, swing trading, and scalping.
- Risks associated with forex trading: Â
- Market volatility: Currency values can fluctuate rapidly, leading to potential losses.
- Leverage risks: While leverage can amplify profits, it can also magnify losses.
- Lack of regulation: The forex market is less regulated than other financial markets, increasing the risk of fraud.
At Rapid Innovation, we provide AI-driven analytics and trading algorithms that help clients navigate the complexities of forex trading. Our solutions enhance decision-making processes, allowing traders to capitalize on market opportunities and mitigate risks effectively.
11.5. Real Estate Trends
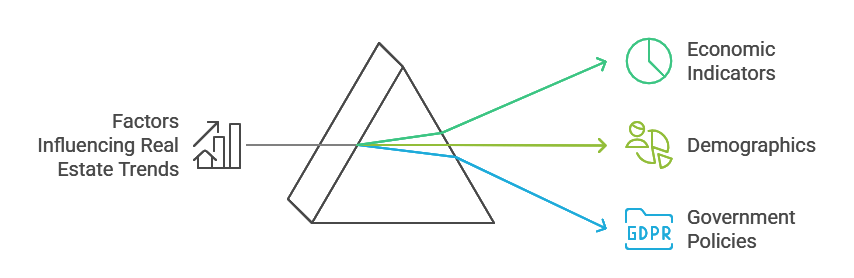
‍
Real estate trends reflect the dynamics of property markets, influenced by economic conditions, demographic shifts, and technological advancements. Understanding these trends is essential for investors, buyers, and sellers.
- Current trends in real estate: Â
- Remote work impact: The rise of remote work has shifted demand from urban to suburban and rural areas, affecting property values.
- Sustainability: Eco-friendly buildings and sustainable practices are becoming increasingly important to buyers and investors.
- Technology integration: Smart home technology and virtual tours are enhancing the buying and selling experience.
- Factors influencing real estate trends: Â
- Economic indicators: Interest rates, employment rates, and inflation can significantly impact property demand and prices.
- Demographics: Changes in population, such as aging populations or millennials entering the market, shape housing needs.
- Government policies: Tax incentives, zoning laws, and housing regulations can influence real estate development and investment.
- Future outlook for real estate: Â
- Continued growth in suburban and rural markets as remote work persists.
- Increased focus on affordable housing solutions to address housing shortages.
- Adoption of technology in real estate transactions, making processes more efficient and transparent.
Rapid Innovation is at the forefront of integrating AI technologies into the real estate sector. Our solutions provide predictive analytics for market trends, helping clients make data-driven investment decisions that enhance their portfolio performance and maximize returns.
12. Best Practices
In the realm of data science and machine learning, adhering to best practices is crucial for achieving optimal results. These practices not only enhance the quality of your models but also streamline the workflow, making it easier to manage and interpret data. At Rapid Innovation, we leverage these best practices to help our clients achieve their business goals efficiently and effectively. Below, we delve into two key areas of best practices: Data Management and Model Selection.
12.1. Data Management
Effective data management is the backbone of any successful data science project. It involves the systematic organization, storage, and utilization of data to ensure accuracy and accessibility. Here are some best practices for data management that we implement for our clients:
- Data Quality Assurance: Regularly check for data accuracy, completeness, and consistency. Implement validation rules to catch errors early, ensuring that your data is reliable for decision-making. This aligns with data quality management best practices.
- Data Cleaning: Remove duplicates, handle missing values, and correct inconsistencies. This step is vital for ensuring that your model is trained on high-quality data, which in turn leads to better performance and ROI. Good data management practices are essential here.
- Data Documentation: Maintain clear documentation of data sources, transformations, and any preprocessing steps. This helps in understanding the data lineage and facilitates collaboration among teams, enhancing project efficiency. Following data management principles and best practices can aid in this process.
- Version Control: Use version control systems for datasets, similar to how code is managed. This allows you to track changes and revert to previous versions if necessary, minimizing risks associated with data alterations. This is a key aspect of database management best practices.
- Data Security: Implement robust security measures to protect sensitive data. This includes encryption, access controls, and regular audits, ensuring compliance with regulations and safeguarding your business interests. Adhering to data governance best practices is crucial here.
- Data Storage Solutions: Choose appropriate storage solutions based on data size and access needs. Options include cloud storage, databases, and data lakes, tailored to optimize performance and cost-effectiveness. This is part of effective data management practices.
- Data Governance: Establish policies and procedures for data management to ensure compliance with regulations and ethical standards, fostering trust and accountability in your data practices. This includes master data governance best practices.
12.2. Model Selection
Choosing the right model is critical for the success of any machine learning project. The model selection process should be systematic and based on the specific requirements of the task at hand. Here are some best practices for model selection that we advocate for our clients:
- Understand the Problem: Clearly define the problem you are trying to solve. Is it a classification, regression, or clustering task? Understanding the problem helps in narrowing down the model options, leading to more targeted solutions.
- Evaluate Multiple Models: Don’t settle for the first model you try. Experiment with various algorithms to find the one that performs best for your data, maximizing the potential for a higher ROI.
- Cross-Validation: Use cross-validation techniques to assess model performance. This helps in understanding how the model will generalize to unseen data, ensuring robustness in real-world applications.
- Feature Selection: Identify and select the most relevant features for your model. This can improve performance and reduce overfitting, ultimately leading to more accurate predictions.
- Hyperparameter Tuning: Optimize model parameters through techniques like grid search or random search. Fine-tuning can significantly enhance model accuracy, providing a competitive edge in your market.
- Performance Metrics: Choose appropriate metrics to evaluate model performance based on the problem type. Common metrics include accuracy, precision, recall, and F1 score, which help in quantifying success.
- Model Interpretability: Consider the interpretability of the model, especially in fields like healthcare or finance where understanding the decision-making process is crucial. This transparency can build trust with stakeholders.
- Scalability: Ensure that the selected model can handle the scale of data you expect in production. Some models may perform well on small datasets but struggle with larger ones, impacting long-term viability.
By following these best practices in data management and model selection, including customer data management best practices and master data management best practices, Rapid Innovation empowers clients to enhance the effectiveness of their data science projects and achieve better outcomes, ultimately driving greater ROI.
12.3. Risk Assessment
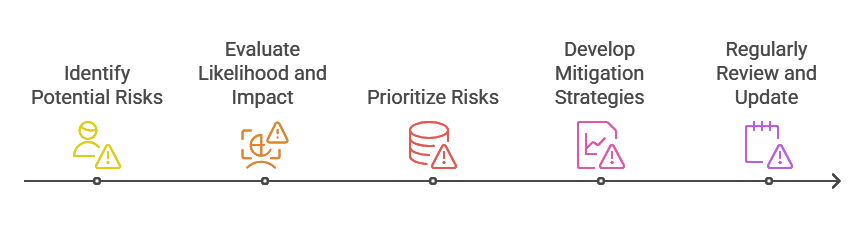
‍
Risk assessment is a critical process in identifying, evaluating, and prioritizing risks associated with a system or project. It helps organizations understand potential vulnerabilities and the impact of various threats.
- Identify potential risks: This includes both internal and external threats, such as cyberattacks, data breaches, and system failures. Hazard identification is a key component of this step.
- Evaluate the likelihood and impact: Assess how likely each risk is to occur and the potential consequences if it does. This can be done using qualitative and quantitative methods, including a job risk analysis to better understand specific roles.
- Prioritize risks: Rank the risks based on their severity and likelihood, allowing organizations to focus on the most critical issues first. Utilizing a risk assessment risk matrix can aid in this prioritization.
- Develop mitigation strategies: Create plans to reduce or eliminate risks, which may include implementing security measures, training staff, or developing contingency plans. This is often part of a comprehensive risk assessment plan example.
- Regularly review and update: Risk assessments should not be a one-time activity. Regular reviews ensure that new risks are identified and existing risks are managed effectively. This includes an assessment of risk example to guide future evaluations.
Effective risk assessment can lead to better decision-making and resource allocation, ultimately enhancing the overall security posture of an organization. At Rapid Innovation, we leverage advanced AI algorithms to automate risk identification and evaluation, enabling our clients to achieve greater ROI by proactively addressing vulnerabilities before they escalate into significant issues. Our approach includes cyber risk assessment and security risk assessment to cover all bases, ensuring a thorough assessment of the risk landscape. For organizations looking to enhance their risk management strategies, our AI agents for risk assessment can provide valuable insights and solutions.
12.4. Performance Monitoring
Performance monitoring is essential for ensuring that systems and processes operate efficiently and effectively. It involves the continuous assessment of various metrics to gauge performance against established benchmarks.
- Define key performance indicators (KPIs): Identify specific metrics that align with organizational goals, such as system uptime, response times, and user satisfaction.
- Collect data: Use tools and software to gather data on the defined KPIs. This can include automated monitoring systems that track performance in real-time.
- Analyze performance: Regularly review the collected data to identify trends, anomalies, and areas for improvement. This analysis can help in understanding how well the system is performing.
- Implement corrective actions: If performance falls below acceptable levels, develop and implement strategies to address the issues. This may involve optimizing processes, upgrading technology, or providing additional training to staff.
- Report findings: Share performance results with stakeholders to maintain transparency and facilitate informed decision-making.
By maintaining a robust performance monitoring system, organizations can ensure that they meet their operational goals and continuously improve their processes. Rapid Innovation employs AI-driven analytics to provide real-time insights, allowing clients to make data-informed decisions that enhance operational efficiency and drive profitability.
12.5. System Updates
System updates are vital for maintaining the security, functionality, and performance of software and hardware systems. Regular updates help protect against vulnerabilities and ensure that systems operate smoothly.
- Schedule regular updates: Establish a routine for checking and applying updates, whether they are for operating systems, applications, or firmware. This can be done monthly, quarterly, or as needed.
- Prioritize critical updates: Focus on applying security patches and updates that address known vulnerabilities first. This helps mitigate risks associated with cyber threats.
- Test updates before deployment: Implement a testing phase to ensure that updates do not disrupt existing systems or processes. This can involve using a staging environment to evaluate the impact of updates.
- Communicate with users: Inform users about upcoming updates, including any expected downtime or changes in functionality. Clear communication helps manage expectations and reduces frustration.
- Monitor post-update performance: After applying updates, monitor the system to ensure that it is functioning as intended. Address any issues that arise promptly to minimize disruption.
By implementing a structured approach to system updates, organizations can enhance their security posture and ensure that their systems remain efficient and effective. Rapid Innovation assists clients in automating the update process, ensuring that they are always equipped with the latest security features and performance enhancements, ultimately leading to improved ROI and reduced operational risks.
13. Troubleshooting and Support
In any system or product, troubleshooting and support are essential components that ensure smooth operation and user satisfaction. Understanding common issues and knowing how to access technical support can significantly enhance the user experience.
13.1. Common Issues
Users often encounter a variety of common issues that can disrupt their experience. Recognizing these problems can help in quickly resolving them. Some of the most frequent issues include:
- Connectivity Problems: Many users face issues with internet connectivity, which can stem from router malfunctions, incorrect settings, or service outages.
- Software Bugs: Software applications may have bugs that lead to crashes or unexpected behavior. Regular updates can help mitigate these issues.
- Performance Slowdowns: Devices may slow down due to insufficient memory, background applications consuming resources, or malware infections.
- Compatibility Issues: New software or hardware may not be compatible with existing systems, leading to functionality problems.
- User Errors: Sometimes, the issue may arise from user error, such as incorrect settings or misuse of features.
Identifying these common issues can help users troubleshoot effectively. Many resources are available online, including forums and FAQs, which can provide guidance on resolving these problems.
13.2. Technical Support
When troubleshooting does not resolve the issue, accessing technical support becomes crucial. Technical support can provide expert assistance and solutions tailored to specific problems. Users can reach technical support through various methods:
- Contact Methods: Most companies offer multiple ways to reach technical support, including: Â
- Phone Support: for immediate assistance.
- Email Support: for detailed inquiries.
- Live Chat Options: for real-time help.
- Self-Service Resources: Many organizations provide self-service resources such as: Â
- Knowledge Bases: with articles and guides.
- Video Tutorials: that demonstrate solutions.
- Community Forums: where users can share experiences and solutions.
- Service Level Agreements (SLAs): Understanding the SLAs can help users know what to expect in terms of response times and support availability.
- Remote Assistance: Some technical support teams offer remote assistance, allowing them to access the user’s device to diagnose and fix issues directly.
- Escalation Procedures: If initial support does not resolve the issue, users should be aware of escalation procedures to reach higher-level support.
By utilizing these technical support options, users can effectively address their concerns and ensure their systems function optimally. At Rapid Innovation, we understand the importance of robust troubleshooting and support mechanisms. Our AI-driven solutions not only enhance user experience but also streamline support processes, ultimately leading to greater efficiency and ROI for your business.
13.3. System Maintenance
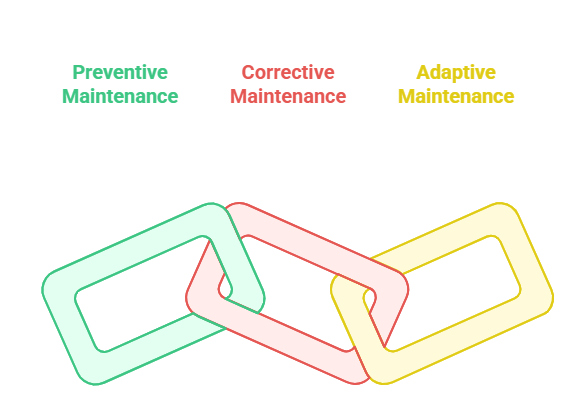
‍
System maintenance is a critical aspect of ensuring that software and hardware systems operate efficiently and effectively. Regular maintenance helps to prevent system failures, enhance performance, and extend the lifespan of technology assets.
- Types of Maintenance Â
- Preventive Maintenance: Scheduled activities aimed at preventing potential issues before they occur. This includes regular updates, patches, and system checks.
- Corrective Maintenance: Actions taken to fix defects or issues that have already occurred. This may involve troubleshooting and repairing hardware or software problems.
- Adaptive Maintenance: Modifications made to the system to adapt to changes in the environment or user requirements. This can include updates to comply with new regulations or to integrate new technologies.
- Importance of Regular Updates
- Keeping software up to date is crucial for security, as vulnerabilities in outdated software can be exploited by cybercriminals. Regular updates can also improve system performance and introduce new features that enhance user experience.
- Monitoring System Performance
- Implementing monitoring tools can help track system performance metrics, such as uptime, response time, and resource utilization. Proactive monitoring allows for early detection of potential issues, enabling timely intervention.
- Backup and Recovery Plans
- Regular backups are essential to protect data from loss due to hardware failure, cyberattacks, or natural disasters. A well-defined recovery plan ensures that systems can be restored quickly and efficiently in the event of a failure.
13.4. User Training
User training is vital for maximizing the effectiveness of any system. Proper training ensures that users are equipped with the knowledge and skills necessary to utilize the system efficiently.
- Types of Training Â
- Onboarding Training: Introduces new users to the system, covering basic functionalities and navigation.
- Advanced Training: Provides in-depth knowledge of specific features and tools for experienced users.
- Ongoing Training: Regular sessions to update users on new features, best practices, and system changes.
- Training Methods Â
- In-Person Workshops: Interactive sessions that allow users to ask questions and practice in real-time.
- Online Courses: Flexible training options that users can complete at their own pace.
- User Manuals and Guides: Written resources that users can refer to for step-by-step instructions.
- Benefits of User Training
- Â
- User training increases confidence and satisfaction, leading to higher productivity. It also reduces the number of support requests, as users become more self-sufficient, and ensures compliance with organizational policies and procedures.
- Measuring Training Effectiveness
- Â
- Surveys and feedback forms can help assess user satisfaction and knowledge retention. Tracking performance metrics before and after training can provide insights into its impact on productivity.
13.5. Documentation
Documentation is an essential component of system management, providing a comprehensive reference for users and administrators alike. Well-organized documentation enhances understanding and facilitates smoother operations.
- Types of Documentation Â
- User Documentation: Guides and manuals designed for end-users, detailing how to use the system effectively.
- Technical Documentation: Information intended for IT staff, including system architecture, configuration settings, and troubleshooting procedures.
- Process Documentation: Outlines workflows, procedures, and policies related to system use and maintenance.
- Importance of Clear Documentation
- Â
- Clear documentation reduces the learning curve for new users, enabling them to become productive more quickly. It serves as a reference point for troubleshooting and resolving issues, minimizing downtime, and ensures consistency in processes and procedures, which is crucial for compliance and quality control.
- Best Practices for Documentation Â
- Keep documentation up to date with regular reviews and revisions to reflect system changes.
- Use clear, concise language and avoid jargon to ensure accessibility for all users.
- Organize documentation logically, using headings, bullet points, and tables for easy navigation.
- Utilizing Technology for Documentation
- Â
- Employ documentation management systems to store, organize, and share documents efficiently. Consider using collaborative tools that allow multiple users to contribute to and edit documentation in real-time.
At Rapid Innovation, we understand that effective system maintenance training documentation, user training, and comprehensive documentation are essential for achieving your business goals. By leveraging our expertise in AI development, we can help you implement robust maintenance strategies, enhance user capabilities through tailored training programs, and create clear documentation that supports your operational needs. This holistic approach not only minimizes downtime but also maximizes your return on investment, ensuring that your technology assets work for you efficiently and effectively. Additionally, if you're looking for specialized services, consider our NFT wallet development services to enhance your digital asset management. For insights on how technology can improve sports training, check out our article on computer vision in sports training.
14. Future Developments
The future of technology is poised for significant transformations, particularly in the realms of artificial intelligence (AI) and market dynamics. As we look ahead, several key developments are expected to shape industries and consumer behavior.
14.1. AI Advancements
Artificial intelligence is rapidly evolving, with advancements that promise to revolutionize various sectors. The following trends are anticipated:
- Enhanced Machine Learning: Algorithms are becoming more sophisticated, allowing machines to learn from data with greater accuracy. This will lead to improved predictive analytics and decision-making capabilities, enabling businesses to optimize operations and enhance customer experiences.
- Natural Language Processing (NLP): AI's ability to understand and generate human language is improving. This will enhance customer service through chatbots and virtual assistants, making interactions more seamless and efficient, ultimately driving customer satisfaction and loyalty.
- AI in Healthcare: The integration of AI in healthcare is set to grow, with applications in diagnostics, personalized medicine, and patient management. AI can analyze vast amounts of medical data to identify patterns and suggest treatment options, leading to better patient outcomes and reduced costs.
- Autonomous Systems: The development of self-driving vehicles and drones is advancing. These technologies promise to transform transportation and logistics, reducing costs and increasing efficiency, which can significantly impact supply chain management.
- Ethical AI: As AI becomes more prevalent, discussions around ethical considerations are intensifying. Ensuring fairness, transparency, and accountability in AI systems will be crucial to gaining public trust, which is essential for widespread adoption.
- AI in Cybersecurity: With the rise of cyber threats, AI is being utilized to enhance security measures. Machine learning algorithms can detect anomalies and respond to threats in real-time, providing a robust defense against cyberattacks, thereby protecting sensitive business data.
- Latest Developments in AI: The field is witnessing a surge in new developments in AI, including the creation of the most advanced AI systems that can outperform previous models in various tasks.
14.2. Market Evolution
The market landscape is continuously changing, influenced by technological advancements, consumer preferences, and global events. Key aspects of market evolution include:
- Digital Transformation: Businesses are increasingly adopting digital technologies to enhance operations and customer experiences. This shift is driving the need for digital marketing strategies and e-commerce solutions, which can lead to increased market reach and revenue.
- Sustainability Trends: Consumers are becoming more environmentally conscious, prompting companies to adopt sustainable practices. This includes reducing carbon footprints, using eco-friendly materials, and promoting ethical sourcing, which can enhance brand reputation and customer loyalty.
- Remote Work Culture: The COVID-19 pandemic accelerated the shift towards remote work. Companies are now re-evaluating their workplace strategies, leading to a hybrid work model that combines in-office and remote work, which can improve employee satisfaction and productivity.
- E-commerce Growth: Online shopping continues to rise, with consumers preferring the convenience of purchasing goods and services from home. This trend is pushing businesses to enhance their online presence and optimize their supply chains, ultimately driving sales growth.
- Globalization and Localization: While globalization allows companies to reach broader markets, there is also a growing emphasis on localization. Businesses are tailoring their products and marketing strategies to meet the specific needs of local consumers, which can enhance market penetration.
- Data-Driven Decision Making: Companies are increasingly relying on data analytics to inform their strategies. By leveraging big data, businesses can gain insights into consumer behavior, market trends, and operational efficiencies, leading to more informed decision-making and improved ROI.
- Subscription Economy: The rise of subscription-based models is changing how consumers access products and services. This trend is prevalent in various industries, from software to entertainment, providing businesses with recurring revenue streams and greater financial stability.
As we move forward, the interplay between AI advancements, including the most advanced artificial intelligence and recent developments in AI, and market evolution will create new opportunities and challenges. Companies that adapt to these changes will be better positioned to thrive in an increasingly competitive landscape. At Rapid Innovation, we are committed to helping our clients navigate these developments, leveraging our expertise in AI to drive efficiency and maximize ROI.
14.3. Integration Possibilities
Integration possibilities refer to the various ways in which different systems, applications, and technologies can work together to enhance functionality and improve efficiency. In today's digital landscape, seamless integration is crucial for businesses to remain competitive.
- API Integration: Application Programming Interfaces (APIs) allow different software systems to communicate with each other. This enables businesses to connect their existing tools with new applications, such as intacct sage and salesforce crm, streamlining processes and improving data flow. At Rapid Innovation, we assist clients in developing custom APIs that facilitate smooth integration, ensuring that their technology ecosystem operates cohesively.
- Cloud Integration: With the rise of cloud computing, integrating cloud services with on-premises systems has become essential. This allows for greater flexibility, scalability, and access to real-time data from anywhere. Our team at Rapid Innovation specializes in cloud integration solutions that help businesses leverage the full potential of cloud technologies, including salesforce commerce cloud and shopify erp, while maintaining operational efficiency.
- Data Integration: Combining data from various sources into a unified view is vital for informed decision-making. Data integration tools can help businesses consolidate information from different databases, applications, and platforms, such as microsoft erp and netsuite integration. Rapid Innovation provides tailored data integration services that empower organizations to harness their data effectively, leading to improved insights and greater ROI.
- IoT Integration: The Internet of Things (IoT) is transforming industries by connecting devices and enabling data exchange. Integrating IoT solutions with existing systems can enhance operational efficiency and provide valuable insights. Rapid Innovation helps clients implement IoT strategies that optimize their operations and drive innovation.
- Cross-Platform Integration: Businesses often use multiple platforms for different functions. Cross-platform integration ensures that these systems work together, providing a seamless user experience and reducing data silos. Our expertise in cross-platform integration allows clients to create a unified operational framework that enhances productivity and collaboration, including solutions for salesforce erp integration and hubspot erp. For more information on AI platforms, check out the ultimate guide to AI platforms.
14.4. Emerging Technologies
Emerging technologies are innovations that are currently developing or will be developed over the next few years, significantly impacting various industries. Staying informed about these technologies is essential for businesses looking to leverage new opportunities.
- Artificial Intelligence (AI): AI is revolutionizing industries by automating processes, enhancing decision-making, and improving customer experiences. From chatbots to predictive analytics, AI applications are vast and varied. Rapid Innovation offers AI development and consulting services that help businesses implement AI solutions tailored to their specific needs, driving efficiency and increasing ROI.
- Blockchain: Originally developed for cryptocurrencies, blockchain technology is now being explored for various applications, including supply chain management, secure transactions, and data integrity. Its decentralized nature offers enhanced security and transparency. Rapid Innovation provides blockchain consulting services that enable organizations to explore and implement blockchain solutions effectively.
- Augmented Reality (AR) and Virtual Reality (VR): AR and VR technologies are transforming how businesses engage with customers. These immersive experiences can enhance training, marketing, and product demonstrations. Our team at Rapid Innovation develops AR and VR applications that create engaging customer experiences and improve training outcomes.
- 5G Technology: The rollout of 5G networks is set to revolutionize connectivity, enabling faster data transfer and supporting a higher number of connected devices. This will enhance IoT applications and improve overall user experiences. Rapid Innovation helps clients prepare for the 5G transition, ensuring their systems are ready to take advantage of this technology.
- Quantum Computing: Although still in its infancy, quantum computing has the potential to solve complex problems much faster than traditional computers. This could lead to breakthroughs in fields such as cryptography, drug discovery, and optimization. Rapid Innovation is at the forefront of exploring quantum computing applications, helping clients understand its potential impact on their industries.
14.5. Industry Trends
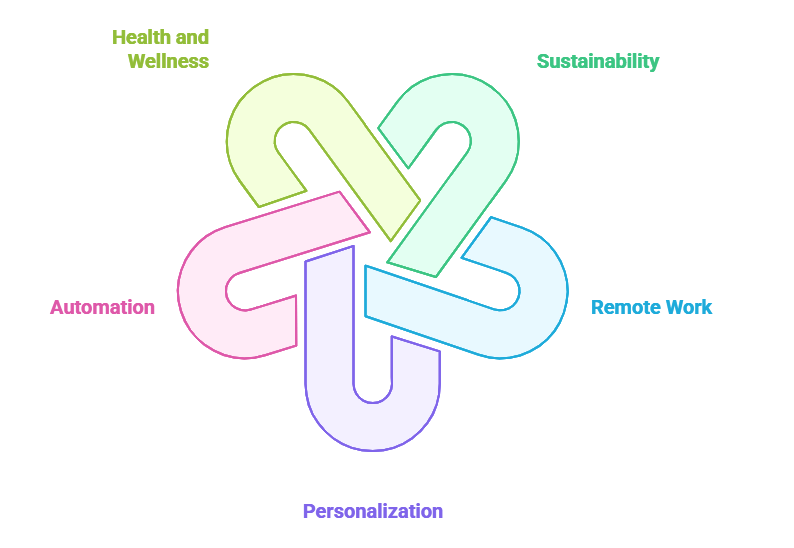
‍
Industry trends refer to the general direction in which a particular industry is moving. Understanding these trends is crucial for businesses to adapt and thrive in a competitive environment.
- Sustainability: There is a growing emphasis on sustainable practices across industries. Companies are adopting eco-friendly processes, reducing waste, and focusing on renewable resources to meet consumer demand for responsible business practices. Rapid Innovation supports clients in implementing sustainable technologies that align with their business goals.
- Remote Work: The COVID-19 pandemic accelerated the shift towards remote work. Many organizations are now adopting hybrid work models, allowing employees to work from home while maintaining some in-office presence. Our solutions at Rapid Innovation facilitate remote collaboration and productivity, ensuring businesses can thrive in this new work environment.
- Personalization: Consumers increasingly expect personalized experiences. Businesses are leveraging data analytics and AI to tailor products, services, and marketing efforts to individual preferences. Rapid Innovation helps clients harness data-driven insights to create personalized customer experiences that drive engagement and loyalty.
- Automation: Automation is becoming a key trend across various sectors. Businesses are implementing robotic process automation (RPA) and AI-driven solutions to streamline operations, reduce costs, and improve efficiency. Rapid Innovation provides automation solutions that help clients optimize their workflows and achieve significant cost savings.
- Health and Wellness: The focus on health and wellness has surged, influencing industries such as food, fitness, and technology. Companies are developing products and services that promote well-being and cater to health-conscious consumers. Rapid Innovation is committed to helping clients innovate in the health and wellness space, leveraging technology to meet evolving consumer demands, including integration solutions for platforms like sugarcrm crm and shopify erp integration.
‍