Table Of Contents
Category
Artificial Intelligence
Automation
1. Introduction to AI Equipment Analysis
Artificial Intelligence (AI) is revolutionizing various industries, and AI equipment analysis is no exception. AI equipment analysis involves using advanced algorithms and machine learning techniques to assess the performance, efficiency, and reliability of machinery and tools. This technology enables businesses to make data-driven decisions, optimize operations, and enhance productivity. By leveraging AI, organizations can gain insights into equipment health, predict failures, and streamline maintenance processes.
- AI can process vast amounts of data quickly and accurately.
- It helps in identifying patterns and anomalies in equipment performance.
- The integration of AI in equipment analysis leads to improved decision-making.
1.1. Role of AI in Equipment Performance
AI plays a crucial role in enhancing equipment performance through predictive maintenance, real-time monitoring, and data analytics. By utilizing sensors and IoT devices, AI systems can collect data on equipment usage and performance metrics.
Predictive maintenance involves AI algorithms analyzing historical data to predict when equipment is likely to fail, allowing for timely maintenance and reducing downtime. Real-time monitoring enables AI systems to continuously monitor equipment performance, providing instant alerts for any irregularities or potential issues. Additionally, data analytics tools can analyze complex datasets to identify trends and optimize equipment usage, leading to improved efficiency.
The implementation of AI in equipment performance not only minimizes operational risks but also extends the lifespan of machinery. Companies can achieve higher productivity levels and reduce costs associated with unexpected breakdowns.
1.2. Business Value and ROI
Investing in AI equipment analysis can yield significant business value and return on investment (ROI). Organizations that adopt AI technologies can experience various benefits that contribute to their bottom line.
- Cost Savings: By predicting equipment failures and optimizing maintenance schedules, businesses can save on repair costs and reduce unplanned downtime.
- Increased Efficiency: AI-driven insights enable companies to streamline operations, leading to enhanced productivity and resource utilization.
- Competitive Advantage: Companies leveraging AI for equipment analysis can stay ahead of competitors by improving their operational capabilities and responsiveness to market demands.
According to a report, businesses that implement AI technologies can see an ROI of up to 30% within the first year of adoption. This demonstrates the financial impact AI can have on equipment management and overall business performance.
At Rapid Innovation, we specialize in providing tailored AI solutions that empower organizations to harness the full potential of AI agents for equipment monitoring. Our expertise in developing and implementing AI-driven systems ensures that our clients can optimize their operations, reduce costs, and achieve significant ROI.
In conclusion, AI equipment analysis is a transformative approach that enhances equipment performance and delivers substantial business value. By integrating AI into their operations, organizations can optimize maintenance, reduce costs, and improve efficiency, ultimately leading to a stronger competitive position in the market.
Refer to the image for a visual representation of AI equipment analysis concepts:

1.3. Key Performance Indicators (KPIs)
Key Performance Indicators (KPIs) are essential metrics that help organizations measure their performance against strategic goals. They provide a clear framework for assessing progress and making informed decisions.
- KPIs can be categorized into different types: Â
- Financial KPIs: Measure profitability, revenue growth, and cost management.
- Customer KPIs: Assess customer satisfaction, retention rates, and acquisition costs.
- Operational KPIs: Evaluate efficiency, productivity, and process improvements.
- Employee KPIs: Track employee engagement, turnover rates, and training effectiveness.
- Effective KPIs should be: Â
- Specific: Clearly defined and focused on a particular area.
- Measurable: Quantifiable to track progress over time.
- Achievable: Realistic and attainable within a given timeframe.
- Relevant: Aligned with the organization's strategic objectives.
- Time-bound: Set within a specific period for evaluation.
- Examples of common KPIs include: Â
- Net Profit Margin: Indicates the percentage of revenue that remains as profit after all expenses.
- Customer Lifetime Value (CLV): Estimates the total revenue a business can expect from a single customer account.
- Employee Turnover Rate: Measures the rate at which employees leave the organization.
By regularly monitoring KPIs, organizations can identify trends, make data-driven decisions, and adjust strategies to improve overall performance. At Rapid Innovation, we leverage AI-driven analytics to help clients define, track, and optimize their KPIs, including key performance indicators examples, ensuring they achieve greater ROI through informed decision-making. For more information on how we can assist with fine-tuning language models to enhance your KPI tracking, visit our fine-tuning language model services and learn about the ROI of enterprise blockchain.
1.4. System Overview
A system overview provides a high-level understanding of how various components of a system interact to achieve specific objectives. It serves as a foundational reference for stakeholders, helping them grasp the system's purpose and functionality.
- Key elements of a system overview include: Â
- Purpose: Clearly defines the system's goals and objectives.
- Components: Outlines the main parts of the system, such as hardware, software, and network infrastructure.
- Interactions: Describes how different components communicate and work together.
- Users: Identifies the primary users of the system and their roles.
- Benefits of a comprehensive system overview: Â
- Enhances communication among stakeholders by providing a common understanding.
- Facilitates better planning and resource allocation.
- Aids in identifying potential risks and areas for improvement.
- Supports training and onboarding for new users.
- A well-structured system overview typically includes: Â
- Diagrams or flowcharts to visualize component interactions.
- Descriptions of key functionalities and features.
- Performance metrics to evaluate system effectiveness.
By providing a clear and concise system overview, organizations can ensure that all stakeholders are aligned and informed about the system's capabilities and objectives. Rapid Innovation assists clients in developing comprehensive system overviews that enhance clarity and drive project success.
2. Core System Architecture
Core system architecture refers to the fundamental structure of a system, encompassing its components, relationships, and principles that guide its design and development. Understanding the core architecture is crucial for ensuring scalability, reliability, and maintainability.
- Key components of core system architecture include: Â
- Hardware: Physical devices that support the system, such as servers, storage, and networking equipment.
- Software: Applications and services that run on the hardware, including operating systems, databases, and middleware.
- Network: The communication infrastructure that connects hardware and software components, enabling data exchange.
- Core architectural styles include: Â
- Monolithic Architecture: A single, unified system where all components are interconnected, making it easier to develop but harder to scale.
- Microservices Architecture: A modular approach where applications are broken down into smaller, independent services that can be developed and deployed separately.
- Serverless Architecture: A cloud-based model where the cloud provider manages the infrastructure, allowing developers to focus on writing code without worrying about server management.
- Considerations for designing core system architecture: Â
- Scalability: The ability to handle increased loads without compromising performance.
- Security: Implementing measures to protect data and ensure compliance with regulations.
- Performance: Optimizing system responsiveness and resource utilization.
- Maintainability: Ensuring that the system can be easily updated and modified as needed.
By carefully designing the core system architecture, organizations can create robust systems that meet current needs while being adaptable for future growth and changes. Rapid Innovation specializes in crafting scalable and efficient architectures that align with our clients' business objectives, ensuring they remain competitive in a rapidly evolving market.
Refer to the image for a visual representation of Key Performance Indicators (KPIs) and their categories.

2.1. Data Collection Systems
Data collection systems are essential for gathering, storing, and managing data from various sources. These systems ensure that organizations can access accurate and timely information for decision-making, ultimately driving business success.
- Types of Data Collection Systems: Â
- Surveys and Questionnaires: Used for gathering qualitative and quantitative data directly from users, enabling businesses to understand customer needs and preferences.
- Sensors and IoT Devices: Collect real-time data from physical environments, such as temperature, humidity, and movement, which can be crucial for industries like manufacturing and logistics. This includes automatic data collection and telemetry collection.
- Web Scraping Tools: Extract data from websites for market research and competitive analysis, allowing organizations to stay ahead of market trends.
- APIs: Allow for the integration of data from different software applications, enabling seamless data flow and enhancing operational efficiency.
- Importance of Data Quality: Â
- Ensures that the data collected is accurate, reliable, and relevant, which is vital for informed decision-making.
- Reduces the risk of making decisions based on faulty information, thereby minimizing potential losses.
- Enhances the overall effectiveness of data-driven strategies, leading to improved business outcomes.
- Challenges in Data Collection: Â
- Data Privacy: Compliance with regulations like GDPR and CCPA is crucial to protect customer information and maintain trust.
- Data Volume: Managing large datasets can be overwhelming without proper systems in place, potentially leading to inefficiencies. This is where automated data collection systems and data collection hardware come into play.
- Data Integration: Combining data from multiple sources can lead to inconsistencies, which can hinder analysis and decision-making.
2.2. AI Processing Engine
An AI processing engine is a core component of artificial intelligence systems, responsible for analyzing data and generating insights. This engine utilizes algorithms and machine learning models to process vast amounts of information efficiently, enabling organizations to leverage data for strategic advantage.
- Key Functions of an AI Processing Engine: Â
- Data Preprocessing: Cleans and prepares data for analysis, ensuring it is in a usable format, which is essential for accurate insights.
- Model Training: Uses historical data to train machine learning models, enabling them to make predictions or classifications that can inform business strategies.
- Real-time Processing: Analyzes data as it is collected, allowing for immediate insights and actions that can enhance operational responsiveness. This is particularly relevant for real-time data collection.
- Technologies Involved: Â
- Machine Learning: Algorithms that learn from data to improve their performance over time, driving continuous improvement in business processes.
- Natural Language Processing (NLP): Enables machines to understand and interpret human language, facilitating better customer interactions and support.
- Neural Networks: Mimic the human brain's structure to process complex data patterns, allowing for advanced analytics and insights.
- Benefits of an AI Processing Engine: Â
- Increased Efficiency: Automates data analysis, reducing the time required for manual processing and freeing up resources for strategic initiatives.
- Enhanced Decision-Making: Provides actionable insights that can lead to better business outcomes, ultimately increasing ROI.
- Scalability: Can handle growing amounts of data without a significant increase in resources, supporting business growth.
2.3. Analytics Framework
An analytics framework is a structured approach to analyzing data, providing organizations with the tools and methodologies needed to derive insights and make informed decisions that align with their business goals.
- Components of an Analytics Framework: Â
- Data Sources: Identifies where data will be collected from, including internal databases and external APIs, ensuring comprehensive data coverage. This may include data collection systems examples and electronic data collection systems.
- Analytical Tools: Software and applications used for data analysis, such as Tableau, Power BI, or R, which facilitate effective data visualization and interpretation.
- Reporting Mechanisms: Systems for presenting data insights, including dashboards and visualizations, that support clear communication of findings.
- Types of Analytics: Â
- Descriptive Analytics: Analyzes historical data to understand trends and patterns, providing a foundation for strategic planning.
- Predictive Analytics: Uses statistical models to forecast future outcomes based on historical data, enabling proactive decision-making.
- Prescriptive Analytics: Recommends actions based on data analysis to optimize outcomes, helping organizations achieve their objectives more effectively.
- Importance of an Analytics Framework: Â
- Facilitates Data-Driven Decision Making: Provides a clear process for analyzing data and deriving insights, ensuring that decisions are based on solid evidence.
- Improves Collaboration: Encourages teamwork by providing a common framework for data analysis, fostering a culture of data-driven innovation.
- Supports Continuous Improvement: Enables organizations to refine their strategies based on data insights over time, driving sustained business growth and success.
At Rapid Innovation, we specialize in developing and implementing these systems and frameworks, ensuring that our clients can harness the power of data and AI to achieve their business goals efficiently and effectively. By leveraging our expertise, organizations can enhance their ROI and stay competitive in an ever-evolving market. This includes solutions like mobile data collection platforms and portable data collection devices to streamline their operations.
Refer to the image for a visual representation of the data collection systems discussed above:

.
2.4. Reporting Interface
A reporting interface is a crucial component of any data management system, providing users with the ability to visualize and analyze data effectively. This interface serves as the bridge between raw data and actionable insights, allowing users to generate reports that can inform decision-making processes.
- User-Friendly Design: A well-designed reporting interface should be intuitive, enabling users to navigate easily without extensive training. This includes clear menus, drag-and-drop functionalities, and customizable dashboards, similar to the power bi desktop interface.
- Real-Time Data Access: Users should have access to real-time data updates, ensuring that reports reflect the most current information. This is particularly important in fast-paced environments where timely decisions are critical.
- Customizable Reporting Options: The ability to customize reports is essential. Users should be able to select specific data points, apply filters, and choose different visualization formats (charts, graphs, tables) to suit their needs, akin to the sas report writing interface.
- Exporting Capabilities: A robust reporting interface should allow users to export reports in various formats (PDF, Excel, CSV) for sharing and further analysis.
- Interactive Features: Incorporating interactive elements, such as drill-down capabilities, can enhance user engagement and provide deeper insights into the data.
- Security and Access Control: Ensuring that sensitive data is protected is vital. The reporting interface should include user authentication and role-based access controls to restrict data visibility based on user permissions.
2.5. Integration Points
Integration points refer to the various connections between different systems, applications, or data sources that allow for seamless data exchange and interoperability. Effective integration is essential for creating a cohesive data ecosystem.
- API Connectivity: Application Programming Interfaces (APIs) are critical for enabling integration between disparate systems. They allow for the automated transfer of data, reducing manual entry and the potential for errors.
- Data Synchronization: Integration points should facilitate real-time data synchronization across platforms. This ensures that all systems reflect the same information, which is crucial for maintaining data integrity.
- Third-Party Integrations: Many organizations rely on third-party applications for specific functions (e.g., CRM, ERP). Integration points should support these connections, allowing for a unified workflow and enhanced functionality.
- Middleware Solutions: Middleware can act as a bridge between different systems, enabling them to communicate effectively. This is particularly useful when integrating legacy systems with modern applications.
- Scalability: As organizations grow, their integration needs may change. Integration points should be designed to scale, accommodating new systems and data sources without significant reconfiguration.
- Monitoring and Maintenance: Regular monitoring of integration points is essential to ensure they function correctly. This includes tracking data flow, identifying bottlenecks, and performing necessary updates.
3. Data Collection and Sensors
Data collection is the process of gathering information from various sources, and sensors play a pivotal role in this process, especially in the context of the Internet of Things (IoT). Effective data collection strategies are essential for informed decision-making and operational efficiency.
- Types of Sensors: Various types of sensors are used for data collection, including temperature sensors, humidity sensors, motion detectors, pressure sensors, and light sensors.
- Real-Time Monitoring: Sensors enable real-time monitoring of environmental conditions, equipment status, and other critical parameters. This allows organizations to respond quickly to changes and potential issues.
- Data Accuracy: The accuracy of data collected by sensors is paramount. Regular calibration and maintenance of sensors are necessary to ensure reliable data.
- Data Transmission: Collected data must be transmitted to a central system for analysis. This can be achieved through wired connections, wireless networks, or cloud-based solutions.
- Data Storage: Once collected, data needs to be stored securely. Organizations should consider using cloud storage solutions that offer scalability and accessibility.
- Analytics and Insights: The ultimate goal of data collection is to derive insights that can drive decision-making. Advanced analytics tools can process the collected data, identifying trends and patterns that inform strategic initiatives.
- Compliance and Security: Organizations must ensure that their data collection practices comply with relevant regulations (e.g., GDPR, HIPAA). Additionally, data security measures should be in place to protect sensitive information from unauthorized access.
At Rapid Innovation, we leverage these principles to help our clients implement effective reporting interfaces, such as the power bi desktop interface and the sas report writing interface, and integration points, ensuring they can harness the power of their data to achieve greater ROI. By providing tailored solutions that enhance data accessibility and usability, we empower organizations to make informed decisions that drive business success.
Refer to the image for a visual representation of the reporting interface and its key components:

3.1. Sensor Types and Applications
Sensors are critical components in various industries, enabling the collection of data that drives decision-making and automation. Different types of sensors serve specific applications, enhancing efficiency and accuracy.
- Temperature Sensors: Used in HVAC systems, food storage, and manufacturing processes to monitor and control temperature. Rapid Innovation can help clients implement advanced temperature monitoring systems that optimize energy consumption and reduce operational costs.
- Pressure Sensors: Essential in automotive, aerospace, and industrial applications to measure pressure levels in gases and liquids. Our expertise in AI can enhance predictive analytics for pressure variations, leading to improved safety and maintenance schedules. This includes pressure sensor transducers and pressure sensors and transducers for more precise measurements.
- Proximity Sensors: Commonly found in smartphones and industrial automation, these sensors detect the presence of nearby objects without physical contact. We can assist in developing smart automation solutions that leverage proximity sensors for enhanced user interaction and operational efficiency.
- Light Sensors: Utilized in smart lighting systems and photography, they adjust brightness based on ambient light conditions. Rapid Innovation can create intelligent lighting systems that adapt to user preferences, improving energy efficiency and user satisfaction.
- Motion Sensors: Employed in security systems and smart home devices to detect movement and trigger alerts. Our AI-driven analytics can enhance the accuracy of motion detection, reducing false alarms and improving security measures. This includes the use of automotive radar for advanced motion detection capabilities.
- Humidity Sensors: Important in agriculture and climate control, these sensors monitor moisture levels in the air or soil. We can develop data-driven solutions that optimize irrigation and climate control, leading to better crop yields and resource management.
- Inertial Measurement Units: These sensors are crucial in navigation and motion tracking applications, providing data on acceleration and angular velocity. Our expertise in inertial measurement unit sensors can enhance the performance of various systems.
- Ultrasonic Sensors: Used in distance measurement and object detection, these sensors are vital in robotics and automation. We can integrate ultrasonic transducers for improved sensing capabilities.
- Image Sensors: Including cmos image sensors and ccd image sensors, these are essential in capturing visual data for applications in security, automotive, and consumer electronics. Our solutions can enhance image processing and analysis.
- MEMS Sensors: Such as mems accelerometers and mems gyroscopes, these sensors are compact and highly sensitive, making them ideal for a range of applications from smartphones to automotive systems. We can help clients leverage mems technology for innovative solutions.
Each sensor type has unique applications that contribute to improved operational efficiency, safety, and user experience across various sectors. For businesses looking to enhance their operations through automation, AI business automation solutions can provide the necessary tools and expertise. Additionally, insights on AI agents for IoT sensor integration can further enhance the effectiveness of these sensor applications.
3.2. IoT Integration

‍
The Internet of Things (IoT) refers to the interconnection of devices and systems that communicate and exchange data over the internet. IoT integration enhances the functionality of sensors and other devices, leading to smarter solutions.
- Data Collection: IoT devices gather data from sensors, enabling real-time monitoring and analysis. Rapid Innovation can help clients design and implement IoT frameworks that maximize data collection efficiency.
- Remote Monitoring: Users can access data from anywhere, allowing for proactive management of systems and processes. Our solutions empower businesses to monitor operations remotely, enhancing responsiveness and decision-making.
- Automation: IoT integration facilitates automated responses based on sensor data, improving efficiency and reducing human intervention. We can develop custom automation solutions that streamline operations and reduce costs.
- Predictive Maintenance: By analyzing data trends, organizations can predict equipment failures and schedule maintenance, minimizing downtime. Our AI capabilities can enhance predictive maintenance strategies, leading to significant cost savings and improved asset longevity.
- Smart Cities: IoT integration in urban infrastructure enhances traffic management, waste management, and energy efficiency. Rapid Innovation can assist municipalities in developing smart city solutions that improve quality of life and operational efficiency.
- Healthcare Applications: Wearable devices monitor patient health metrics, providing real-time data to healthcare providers. We can create advanced healthcare solutions that leverage IoT for better patient outcomes and operational efficiency.
The integration of IoT with sensors is transforming industries by enabling smarter, data-driven decision-making.
3.3. Real-time Data Streaming
Real-time data streaming refers to the continuous flow of data that is processed and analyzed as it is generated. This capability is crucial for applications that require immediate insights and actions.
- Instant Decision-Making: Organizations can respond to events as they happen, improving operational efficiency and customer satisfaction. Rapid Innovation can implement real-time data solutions that enhance decision-making processes across various sectors.
- Enhanced User Experience: Real-time data allows businesses to tailor services and products to meet customer needs instantly. Our expertise in AI can help clients create personalized experiences that drive customer loyalty.
- Monitoring and Alerts: Systems can trigger alerts based on specific data thresholds, enabling quick responses to potential issues. We can develop robust monitoring systems that ensure timely interventions and risk mitigation.
- Data-Driven Insights: Continuous data streaming provides a wealth of information that can be analyzed for trends and patterns, leading to informed decision-making. Our analytics solutions can transform raw data into actionable insights, driving strategic initiatives.
- Scalability: Real-time data streaming solutions can scale to handle increasing data volumes, making them suitable for growing businesses. Rapid Innovation can design scalable architectures that support business growth and adaptability.
- Applications in Various Industries: From finance to healthcare, real-time data streaming is used for fraud detection, patient monitoring, and supply chain management. Our tailored solutions can address specific industry challenges, enhancing operational effectiveness and ROI.
The ability to process and analyze data in real-time is revolutionizing how organizations operate, making them more agile and responsive to changes in their environment. Rapid Innovation is committed to helping clients leverage these technologies to achieve their business goals efficiently and effectively.
3.4. Data Quality Management
Data Quality Management (DQM) is a critical process that ensures the accuracy, consistency, and reliability of data throughout its lifecycle. Effective DQM helps organizations make informed decisions based on high-quality data, ultimately leading to enhanced business performance.
- Importance of DQM: Â
- Enhances decision-making by providing reliable data, which is essential for strategic planning and operational efficiency.
- Reduces operational costs associated with poor data quality, allowing organizations to allocate resources more effectively.
- Increases customer satisfaction through accurate information, fostering trust and loyalty.
- Key Components of DQM: Â
- Data Profiling: Analyzing data to understand its structure, content, and quality, enabling organizations to identify areas for improvement.
- Data Cleansing: Identifying and correcting errors or inconsistencies in data, ensuring that decision-makers have access to accurate information.
- Data Governance: Establishing policies and standards for data management, which is crucial for maintaining data integrity and compliance. This includes frameworks like data governance data quality and data quality governance.
- Techniques for Ensuring Data Quality: Â
- Regular audits to assess data quality, helping organizations stay proactive in addressing potential issues.
- Implementing automated data validation processes, which can significantly reduce human error and improve efficiency.
- Training staff on data entry best practices, ensuring that data is captured accurately from the outset. Utilizing data quality management tools can enhance these practices.
- Challenges in DQM: Â
- Managing large volumes of data from diverse sources, which can complicate the DQM process.
- Ensuring compliance with data regulations, which is increasingly important in today's data-driven landscape.
- Balancing data quality with operational efficiency, as organizations strive to maintain high standards without sacrificing productivity. The integration of data quality management in healthcare is an example of this challenge.
3.5. Calibration Requirements
Calibration is essential in various industries to ensure that instruments and systems provide accurate measurements. Calibration requirements vary based on the type of equipment and the industry standards, making it a vital aspect of operational excellence.
- Importance of Calibration: Â
- Ensures measurement accuracy, which is critical for quality control and maintaining product standards.
- Helps maintain compliance with industry regulations, reducing the risk of legal issues and penalties.
- Reduces the risk of errors that can lead to costly mistakes, ultimately protecting the organization's bottom line.
- Key Calibration Requirements: Â
- Frequency of Calibration: Determined by the type of equipment and its usage, ensuring that instruments remain reliable over time.
- Calibration Standards: Use of recognized standards to ensure accuracy, which is essential for maintaining credibility in the marketplace.
- Documentation: Keeping detailed records of calibration procedures and results, which is crucial for audits and compliance verification.
- Best Practices for Calibration: Â
- Establish a calibration schedule based on equipment usage, optimizing resource allocation and minimizing downtime.
- Use certified calibration services to ensure compliance, providing peace of mind and reliability.
- Train personnel on proper calibration techniques, fostering a culture of quality and precision.
- Challenges in Calibration: Â
- Keeping up with technological advancements, which can render existing equipment obsolete or less effective.
- Managing costs associated with regular calibration, as organizations must balance quality with budget constraints.
- Ensuring that all equipment is calibrated consistently, which is vital for maintaining operational integrity.
4. Performance Analysis Capabilities
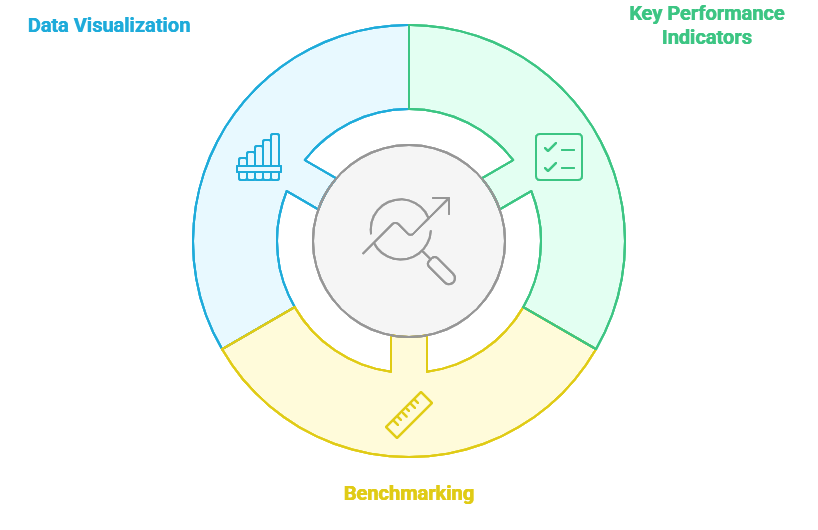
‍
Performance analysis capabilities are essential for organizations to evaluate their operational efficiency and effectiveness. These capabilities help identify areas for improvement and drive strategic decision-making, ultimately leading to greater ROI.
- Importance of Performance Analysis: Â
- Provides insights into operational performance, enabling organizations to make data-driven decisions that align with their strategic goals.
- Helps organizations meet their strategic goals by identifying performance gaps and opportunities for improvement.
- Facilitates data-driven decision-making, empowering leaders to act on insights derived from comprehensive analysis.
- Key Components of Performance Analysis: Â
- Key Performance Indicators (KPIs): Metrics used to measure success, providing a clear framework for evaluating performance.
- Benchmarking: Comparing performance against industry standards or competitors, which can highlight areas for growth and innovation.
- Data Visualization: Using charts and graphs to present performance data clearly, making it easier for stakeholders to understand and act on insights.
- Techniques for Effective Performance Analysis: Â
- Regularly review and update KPIs to align with business objectives, ensuring that performance metrics remain relevant and actionable.
- Utilize advanced analytics tools for deeper insights, enabling organizations to uncover trends and patterns that drive performance.
- Engage stakeholders in the analysis process to gather diverse perspectives, fostering collaboration and buy-in for strategic initiatives.
- Challenges in Performance Analysis: Â
- Data silos that hinder comprehensive analysis, making it difficult to obtain a holistic view of performance.
- Difficulty in interpreting complex data sets, which can lead to misinformed decisions if not addressed.
- Resistance to change from stakeholders when implementing new strategies, which can impede progress and innovation.
At Rapid Innovation, we leverage our expertise in Data Quality Management, including financial data quality management and data quality maturity model, Calibration Requirements, and Performance Analysis to help clients achieve their business goals efficiently and effectively. By implementing tailored solutions, we enable organizations to enhance data integrity, ensure measurement accuracy, and drive performance improvements, ultimately leading to greater ROI. For more information on our services, check out our MLOps consulting services.
4.1. Real-time Monitoring
Real-time monitoring refers to the continuous observation of systems, processes, or environments to ensure optimal performance and immediate response to any issues. This capability is crucial in various sectors, including IT, healthcare, finance, and manufacturing.
- Provides instant feedback on system performance. Â
- Enables quick identification of issues, reducing downtime. Â
- Facilitates proactive decision-making by offering live data. Â
- Enhances customer experience through immediate service adjustments. Â
- Supports compliance with regulatory requirements by maintaining constant oversight. Â
At Rapid Innovation, we leverage cutting-edge technologies such as IoT devices, cloud computing, and advanced analytics to enable real-time monitoring for our clients. For instance, in IT, our application performance monitoring (APM) solutions can track user interactions and system health in real-time, allowing for immediate troubleshooting and minimizing disruptions. In healthcare, our real-time monitoring solutions for patient vitals can lead to timely interventions, potentially saving lives and improving patient outcomes. Additionally, our real-time data management and monitoring systems ensure that organizations can maintain a comprehensive view of their operations, enhancing overall efficiency. For more information on our healthcare solutions, visit our AI healthcare management services and learn more about anomaly detection.
4.2. Predictive Analytics
Predictive analytics involves using statistical algorithms and machine learning techniques to identify the likelihood of future outcomes based on historical data. This approach is increasingly popular across various industries for its ability to forecast trends and behaviors.
- Helps organizations anticipate customer needs and preferences. Â
- Reduces risks by predicting potential failures or issues. Â
- Optimizes resource allocation by forecasting demand. Â
- Enhances marketing strategies through targeted campaigns. Â
- Improves operational efficiency by identifying bottlenecks. Â
At Rapid Innovation, we empower businesses to harness the power of predictive analytics to make informed decisions that drive growth and efficiency. For example, in retail, our predictive analytics solutions can help determine which products are likely to be in demand, allowing for better inventory management and reduced waste. In finance, we assist clients in assessing credit risk and detecting fraudulent activities, ultimately leading to improved financial performance. According to a report by McKinsey, companies that leverage predictive analytics can significantly enhance their decision-making processes.
4.3. Anomaly Detection
Anomaly detection is the process of identifying unusual patterns or outliers in data that do not conform to expected behavior. This technique is essential for maintaining the integrity of systems and ensuring security.
- Detects fraud by identifying transactions that deviate from normal patterns. Â
- Enhances cybersecurity by spotting unauthorized access or breaches. Â
- Improves quality control in manufacturing by identifying defects. Â
- Supports operational efficiency by flagging unusual system behavior. Â
- Aids in compliance by ensuring adherence to regulatory standards. Â
Rapid Innovation employs advanced anomaly detection methods, including statistical tests, machine learning algorithms, and data mining techniques, to help our clients maintain system integrity and security. For instance, in finance, our machine learning models can analyze transaction data to flag potentially fraudulent activities, while in IT, our anomaly detection tools monitor network traffic to identify unusual access patterns, helping to prevent data breaches. According to a study by Gartner, organizations that implement anomaly detection can significantly reduce the time to identify and respond to security threats, enhancing overall operational resilience.
4.4. Pattern Recognition
Pattern recognition is a critical aspect of machine learning and artificial intelligence, enabling systems to identify and classify data based on patterns and regularities. This process is essential in various applications, from image and speech recognition to fraud detection and medical diagnosis.
- Definition: Pattern recognition involves the classification of input data into categories based on the patterns it exhibits. This can be achieved through supervised or unsupervised learning techniques, including data mining and pattern recognition.
- Techniques: Common techniques include: Â
- Neural networks: Particularly convolutional neural networks (CNNs) for image data.
- Decision trees and random forests: For structured data.
- Support vector machines (SVM): For classification tasks.
- Feature selection for data and pattern recognition: To enhance model performance.
- Applications: Â
- Image recognition: Identifying objects, faces, or scenes in images.
- Speech recognition: Converting spoken language into text.
- Anomaly detection: Identifying unusual patterns that do not conform to expected behavior, useful in fraud detection.
- Machine learning and data mining in pattern recognition: To extract meaningful insights from large datasets.
- Challenges: Â
- Variability in data: Patterns can change over time, making it difficult for models to adapt.
- Noise in data: Irrelevant information can obscure true patterns, leading to inaccurate classifications.
- Future Trends: Â
- Increased use of deep learning techniques: To improve accuracy.
- Integration of pattern recognition with other AI technologies: For enhanced capabilities.
- Pattern recognition algorithms for data mining: To improve the efficiency of data analysis.
At Rapid Innovation, we leverage advanced pattern recognition techniques to help our clients enhance their operational efficiency and decision-making processes. For instance, in the healthcare sector, we have developed AI solutions that utilize image recognition to assist radiologists in diagnosing conditions more accurately and swiftly, ultimately leading to better patient outcomes and reduced costs. Our work also includes applying machine learning pattern matching and NLP pattern recognition to further enhance our solutions. Additionally, we offer specialized services in transformer model development to advance pattern recognition capabilities.
4.5. Efficiency Optimization
Efficiency optimization in machine learning focuses on improving the performance of algorithms and models while minimizing resource consumption. This is crucial for deploying machine learning solutions in real-world applications where computational resources may be limited.
- Importance: Â
- Reduces operational costs: By minimizing the computational power required.
- Enhances the speed of model training and inference: Leading to quicker decision-making.
- Techniques for Optimization: Â
- Algorithmic improvements: Using more efficient algorithms can significantly reduce processing time.
- Hyperparameter tuning: Adjusting model parameters to find the most efficient configuration.
- Data preprocessing: Cleaning and transforming data to reduce complexity and improve model performance.
- Tools and Frameworks: Â
- TensorFlow and PyTorch: Offer built-in functions for optimizing model performance.
- Libraries like Optuna and Hyperopt: Assist in hyperparameter optimization.
- Metrics for Efficiency: Â
- Time complexity: Measures how the runtime of an algorithm increases with the size of the input data.
- Space complexity: Evaluates the amount of memory an algorithm uses relative to the input size.
- Future Directions: Â
- Development of more efficient hardware: Such as TPUs (Tensor Processing Units), specifically designed for machine learning tasks.
- Research into energy-efficient algorithms: To reduce the carbon footprint of machine learning operations.
At Rapid Innovation, we prioritize efficiency optimization to ensure our clients achieve maximum ROI from their AI investments. By implementing tailored optimization strategies, we have helped businesses reduce their operational costs significantly while improving the speed and accuracy of their machine learning models.
5. Machine Learning Models
Machine learning models are mathematical representations that learn from data to make predictions or decisions without being explicitly programmed. These models are the backbone of machine learning applications and can be categorized into various types based on their learning approach.
- Types of Models: Â
- Supervised Learning Models: These models learn from labeled data, where the input-output pairs are known. Examples include: Â
- Linear regression: For predicting continuous values.
- Logistic regression: For binary classification.
- Decision trees: For both classification and regression tasks.
- Unsupervised Learning Models: These models work with unlabeled data to find hidden patterns. Examples include: Â
- K-means clustering: For grouping similar data points.
- Principal Component Analysis (PCA): For dimensionality reduction.
- Reinforcement Learning Models: These models learn by interacting with an environment and receiving feedback in the form of rewards or penalties. They are commonly used in robotics and game playing.
- Supervised Learning Models: These models learn from labeled data, where the input-output pairs are known. Examples include: Â
- Model Evaluation: Â
- Accuracy: Measures the proportion of correct predictions made by the model.
- Precision and Recall: Important metrics for evaluating classification models, especially in imbalanced datasets.
- F1 Score: A balance between precision and recall, providing a single metric for model performance.
- Deployment Considerations: Â
- Scalability: Ensuring the model can handle increased data loads as the application grows.
- Interpretability: Understanding how the model makes decisions, which is crucial for trust and compliance in many industries.
- Emerging Trends: Â
- Transfer learning: Utilizing pre-trained models to improve performance on new tasks with limited data.
- Automated machine learning (AutoML): Streamlining the process of model selection and hyperparameter tuning to make machine learning more accessible.
At Rapid Innovation, we specialize in developing and deploying a variety of machine learning models tailored to meet the unique needs of our clients. By focusing on model evaluation and deployment considerations, we ensure that our solutions are not only effective but also scalable and interpretable, providing our clients with the insights they need to drive their business forward.
5.1. Supervised Learning Applications
Supervised learning is a type of machine learning where a model is trained on labeled data. This means that the input data is paired with the correct output, allowing the model to learn the relationship between the two. Supervised learning is widely used across various industries due to its effectiveness in making predictions and classifications.
- Image Recognition: Supervised learning algorithms are extensively used in image classification tasks. For instance, convolutional neural networks (CNNs) can identify objects in images, which is crucial for applications like facial recognition and autonomous vehicles. At Rapid Innovation, we leverage these algorithms to help clients enhance their security systems and improve user experiences through advanced image processing, including machine learning applications in computer vision.
- Spam Detection: Email services utilize supervised learning to filter out spam. By training models on labeled emails (spam vs. not spam), these systems can accurately classify incoming messages. Our consulting services can assist businesses in implementing robust spam detection systems, ensuring better communication and productivity, applying machine learning for business intelligence.
- Medical Diagnosis: In healthcare, supervised learning helps in diagnosing diseases. Models can be trained on patient data to predict conditions based on symptoms and medical history, improving patient outcomes. Rapid Innovation collaborates with healthcare providers to develop predictive models that enhance diagnostic accuracy and patient care, including machine learning in drug discovery.
- Financial Forecasting: Financial institutions use supervised learning for credit scoring and risk assessment. By analyzing historical data, models can predict the likelihood of loan defaults. We support financial organizations in building sophisticated forecasting models that drive better investment decisions and risk management strategies, utilizing machine learning for systems.
- Natural Language Processing (NLP): Supervised learning is fundamental in NLP tasks such as sentiment analysis and language translation. Models are trained on labeled text data to understand context and meaning. Rapid Innovation helps businesses harness NLP technologies to improve customer interactions and automate responses, leading to increased efficiency and customer satisfaction, including applications of machine learning in business intelligence.
5.2. Unsupervised Learning Methods

‍
Unsupervised learning involves training models on data without labeled outputs. The goal is to identify patterns or groupings within the data. This approach is particularly useful for exploratory data analysis and can reveal insights that are not immediately apparent.
- Clustering: One of the primary methods in unsupervised learning is clustering, where algorithms like K-means or hierarchical clustering group similar data points. This is useful in market segmentation, where businesses can identify distinct customer groups. Rapid Innovation assists clients in utilizing clustering techniques to tailor marketing strategies and enhance customer engagement, including manifold learning.
- Dimensionality Reduction: Techniques such as Principal Component Analysis (PCA) reduce the number of features in a dataset while preserving its variance. This is beneficial for visualizing high-dimensional data and improving the performance of other algorithms. Our team can help organizations streamline their data analysis processes, making insights more accessible and actionable, including signal processing in machine learning.
- Anomaly Detection: Unsupervised learning is effective in identifying outliers in data. This is crucial in fraud detection, where unusual patterns can indicate fraudulent activity. Rapid Innovation provides solutions that implement anomaly detection systems, safeguarding businesses against potential threats.
- Association Rule Learning: This method uncovers relationships between variables in large datasets. It is commonly used in market basket analysis to determine which products are frequently purchased together. We help retailers leverage these insights to optimize inventory and enhance cross-selling strategies.
- Recommendation Systems: Unsupervised learning techniques can enhance recommendation systems by analyzing user behavior and preferences, leading to personalized content suggestions. Rapid Innovation develops tailored recommendation engines that improve user experiences and drive sales, including machine learning mobile applications.
5.3. Reinforcement Learning
Reinforcement learning (RL) is a type of machine learning where an agent learns to make decisions by taking actions in an environment to maximize cumulative rewards. Unlike supervised learning, RL does not rely on labeled data but instead learns from the consequences of its actions.
- Game Playing: RL has gained prominence in game development, with algorithms like Deep Q-Networks (DQN) achieving superhuman performance in games like Go and chess. These systems learn optimal strategies through trial and error. Rapid Innovation can apply similar principles to develop training simulations for various industries, enhancing decision-making processes.
- Robotics: In robotics, reinforcement learning is used to train robots to perform tasks such as walking or grasping objects. The robot learns from its interactions with the environment, improving its performance over time. We collaborate with manufacturers to integrate RL into robotic systems, optimizing their functionality and efficiency, including machine learning for embedded systems.
- Autonomous Vehicles: RL plays a crucial role in the development of self-driving cars. These vehicles learn to navigate complex environments by receiving feedback on their actions, allowing them to make real-time decisions. Rapid Innovation partners with automotive companies to advance their autonomous driving technologies, ensuring safety and reliability.
- Healthcare Treatment Planning: In healthcare, RL can optimize treatment plans for patients by learning from past treatment outcomes. This personalized approach can lead to better health results. Our expertise in RL enables healthcare providers to enhance patient care through data-driven treatment strategies, including machine learning in drug discovery.
- Finance and Trading: Reinforcement learning is applied in algorithmic trading, where models learn to make buy or sell decisions based on market conditions, aiming to maximize returns. Rapid Innovation assists financial institutions in developing RL-based trading algorithms that improve profitability and reduce risks.
Each of these learning methods—supervised, unsupervised, and reinforcement—offers unique advantages and applications, making them essential tools in the field of artificial intelligence and machine learning. At Rapid Innovation, we are committed to helping our clients achieve greater ROI through tailored AI solutions that drive efficiency and effectiveness in their operations, including designing machine learning systems and applying machine learning for signal processing.
5.4. Model Training and Validation
Model training and validation are critical steps in the machine learning lifecycle. They ensure that the model learns effectively from the data and can generalize well to unseen data.
- Training Phase: In this phase, the model learns from a training dataset, which is typically a subset of the entire dataset used to adjust the model's parameters. Various algorithms can be employed, such as supervised learning, unsupervised learning, or reinforcement learning, depending on the problem at hand. The training process involves feeding the model input data and corresponding output labels, allowing it to learn the relationship between them. At Rapid Innovation, we leverage advanced algorithms tailored to your specific business needs, ensuring that the model is optimized for maximum efficiency and effectiveness. This includes training AI models and training machine learning models to enhance performance.
- Validation Phase: After training, the model is validated using a separate validation dataset that is not used during the training phase, ensuring an unbiased evaluation. Validation helps in tuning hyperparameters and selecting the best model configuration. Techniques like k-fold cross-validation can be employed to ensure that the model's performance is consistent across different subsets of data. This rigorous validation process is crucial for our clients, as it directly impacts the return on investment (ROI) by minimizing the risk of deploying underperforming models. We also focus on training data in machine learning to ensure quality inputs.
- Overfitting and Underfitting: Overfitting occurs when the model learns the training data too well, capturing noise and outliers, which leads to poor performance on new data. Underfitting happens when the model is too simple to capture the underlying patterns in the data. Regularization techniques, such as L1 and L2 regularization, can help mitigate overfitting. At Rapid Innovation, we implement these techniques to ensure that our models maintain a balance between complexity and generalization, ultimately enhancing their predictive power. We also utilize transfer learning deep learning methods to improve model performance.
- Final Model Selection: After validation, the best-performing model is selected based on metrics like accuracy, precision, recall, and F1 score. This model is then tested on a separate test dataset to evaluate its performance in real-world scenarios. Our commitment to thorough testing ensures that our clients can confidently deploy models that deliver tangible business results. We emphasize the importance of machine learning model training and testing to achieve optimal results.
5.5. Continuous Learning Systems
Continuous learning systems are designed to adapt and improve over time as new data becomes available. This approach is essential in dynamic environments where data is constantly changing.
- Incremental Learning: Continuous learning allows models to update themselves incrementally without needing to retrain from scratch. This is particularly useful in applications like fraud detection, where new patterns emerge regularly. Rapid Innovation specializes in developing such systems, enabling our clients to stay ahead of emerging trends and threats. Online machine learning techniques are also employed to facilitate this process.
- Feedback Loops: Implementing feedback loops enables the model to learn from its predictions and user interactions. For instance, in recommendation systems, user feedback can refine the model's suggestions over time. By integrating feedback mechanisms, we help our clients enhance user engagement and satisfaction, leading to improved business outcomes. This is particularly relevant in online machine learning model training.
- Data Drift Management: Continuous learning systems must be equipped to handle data drift, which occurs when the statistical properties of the input data change over time. Techniques such as monitoring model performance and retraining with new data can help maintain accuracy. Our proactive approach to data drift management ensures that our clients' models remain relevant and effective in a rapidly changing landscape. We also focus on federated learning models to enhance data privacy and security.
- Scalability: These systems are designed to scale efficiently, allowing them to process large volumes of data continuously. Cloud-based solutions often support continuous learning by providing the necessary computational resources. Rapid Innovation leverages cloud technologies, such as AWS Sagemaker training, to ensure that our clients can scale their AI solutions seamlessly as their data needs grow.
- Real-World Applications: Continuous learning is applied in various fields, including finance, healthcare, and autonomous vehicles, where real-time data is crucial for decision-making. Our expertise in these domains allows us to deliver tailored solutions that drive significant ROI for our clients, including the use of deep learning train methods and machine learning training and testing strategies.
6. Performance Metrics and Analytics
Performance metrics and analytics are vital for evaluating the effectiveness of machine learning models. They provide insights into how well a model performs and guide improvements.
- Common Performance Metrics: Â
- Accuracy: The ratio of correctly predicted instances to the total instances.
- Precision: The ratio of true positive predictions to the total predicted positives, indicating the model's ability to avoid false positives.
- Recall: The ratio of true positive predictions to the total actual positives, reflecting the model's ability to identify all relevant instances.
- F1 Score: The harmonic mean of precision and recall, providing a balance between the two metrics. At Rapid Innovation, we emphasize the importance of these metrics in aligning model performance with business objectives, particularly in machine learning training and testing data.
- Confusion Matrix: A confusion matrix is a table that visualizes the performance of a classification model. It shows true positives, true negatives, false positives, and false negatives. This matrix helps in understanding the types of errors the model makes and can guide further improvements. Our analytical approach ensures that clients can make informed decisions based on comprehensive performance insights.
- ROC and AUC: The Receiver Operating Characteristic (ROC) curve illustrates the trade-off between true positive rate and false positive rate at various threshold settings. The Area Under the Curve (AUC) quantifies the overall ability of the model to discriminate between classes. We utilize these metrics to provide our clients with a clear understanding of their models' capabilities.
- Model Monitoring: Continuous monitoring of model performance is essential to ensure it remains effective over time. Metrics should be tracked in real-time to detect any degradation in performance due to data drift or other factors. Rapid Innovation implements robust monitoring solutions that empower our clients to maintain optimal model performance, including the use of analytics tools for better insights.
- Analytics Tools: Various analytics tools and platforms can help visualize and interpret performance metrics, making it easier to communicate findings to stakeholders. Tools like TensorBoard, MLflow, and various BI tools can provide dashboards for monitoring model performance. We assist our clients in selecting and integrating the right tools to enhance their data-driven decision-making processes.
- Iterative Improvement: Performance metrics should inform an iterative process of model improvement, guiding decisions on feature engineering, model selection, and hyperparameter tuning. Regularly revisiting and refining the model based on performance analytics ensures it remains relevant and effective. At Rapid Innovation, we foster a culture of continuous improvement, ensuring that our clients achieve sustained success through their AI initiatives, including the application of MLOps training methodologies.
6.1. Equipment Efficiency Metrics
Equipment efficiency metrics are essential for assessing the performance of machinery and tools in various industries. These metrics help organizations identify areas for improvement, optimize operations, and reduce costs. Key metrics include:
- Overall Equipment Effectiveness (OEE): This metric combines availability, performance, and quality to provide a comprehensive view of equipment efficiency. A high OEE score indicates that equipment is being used effectively.
- Overall Throughput Effectiveness: This metric evaluates the total output of a manufacturing line relative to its potential output, helping organizations understand the efficiency of their production processes.
- Utilization Rate: This measures the percentage of time equipment is in use compared to its total available time. A low utilization rate may suggest underuse or inefficiencies in scheduling.
- Performance Rate: This metric evaluates the speed at which equipment operates compared to its maximum potential. It helps identify bottlenecks in production processes.
- Quality Rate: This measures the percentage of products produced that meet quality standards. A low quality rate can indicate issues with equipment or processes that need addressing.
- Equipment Efficiency Metrics: These encompass various measurements that provide insights into how well equipment is performing in relation to its designed capabilities.
By regularly monitoring these metrics, organizations can make data-driven decisions to enhance productivity and reduce downtime. At Rapid Innovation, we leverage AI-driven analytics to provide real-time insights into these metrics, enabling our clients to optimize their operations and achieve greater ROI. Additionally, we explore the role of AI in predictive maintenance to further enhance equipment efficiency.
6.2. Reliability Analysis

‍
Reliability analysis focuses on the ability of equipment to perform its intended function without failure over a specified period. This analysis is crucial for ensuring operational continuity and minimizing unexpected breakdowns. Key components of reliability analysis include:
- Mean Time Between Failures (MTBF): This metric calculates the average time between equipment failures. A higher MTBF indicates greater reliability and less frequent downtime.
- Failure Rate: This measures the frequency of equipment failures over a specific time frame. Understanding failure rates helps organizations anticipate maintenance needs and improve reliability.
- Root Cause Analysis (RCA): This systematic approach identifies the underlying causes of equipment failures. By addressing root causes, organizations can implement corrective actions to prevent future issues.
- Reliability-Centered Maintenance (RCM): This strategy focuses on maintaining equipment based on its reliability and criticality. RCM helps prioritize maintenance efforts, ensuring that resources are allocated effectively.
Implementing reliability analysis can lead to improved equipment performance, reduced maintenance costs, and enhanced safety. Rapid Innovation employs advanced AI algorithms to predict equipment failures and optimize maintenance schedules, ultimately driving down costs and increasing operational efficiency for our clients.
6.3. Maintenance Indicators
Maintenance indicators are metrics that help organizations evaluate the effectiveness of their maintenance strategies. These indicators provide insights into maintenance performance and can guide improvements. Key maintenance indicators include:
- Mean Time To Repair (MTTR): This metric measures the average time taken to repair equipment after a failure. A lower MTTR indicates efficient repair processes and quicker return to operation.
- Maintenance Cost as a Percentage of Replacement Asset Value (RAV): This indicator assesses the cost-effectiveness of maintenance efforts. A lower percentage suggests that maintenance practices are efficient relative to the value of the assets.
- Scheduled vs. Unscheduled Maintenance: This comparison highlights the proportion of maintenance activities that are planned versus those that occur unexpectedly. A higher ratio of scheduled maintenance indicates better planning and resource allocation.
- Maintenance Backlog: This metric tracks the number of maintenance tasks that are overdue. A growing backlog can signal resource constraints or inefficiencies in the maintenance process.
By monitoring these maintenance indicators, organizations can enhance their maintenance strategies, reduce costs, and improve equipment reliability. At Rapid Innovation, we utilize AI-driven predictive maintenance solutions that help clients minimize downtime and optimize their maintenance operations, leading to significant cost savings and improved asset performance.
6.4. Energy Consumption
Energy consumption is a critical factor in the operational efficiency of any manufacturing or industrial process. Understanding and managing energy usage can lead to significant cost savings and environmental benefits. Energy efficiency measures can reduce operational costs by up to 30%. Monitoring energy consumption helps identify areas for improvement, and implementing energy-efficient technologies can lower greenhouse gas emissions.
At Rapid Innovation, we leverage advanced AI algorithms to analyze energy consumption patterns, enabling organizations to make data-driven decisions that enhance efficiency. Our AI-driven energy management systems (EMS) provide real-time insights, allowing clients to optimize their energy usage effectively. We also offer solutions such as building energy management systems and battery energy management systems to further enhance energy efficiency.
Key strategies for managing energy consumption include:
- Conducting energy audits to assess current usage and identify inefficiencies.
- Utilizing energy management systems (EMS) to monitor and control energy use in real-time.
- Investing in renewable energy sources, such as solar or wind, to reduce reliance on fossil fuels.
- Training employees on energy-saving practices to foster a culture of sustainability.
By focusing on energy consumption, organizations can not only improve their bottom line but also contribute to a more sustainable future. Implementing smart home energy management systems and energy monitoring systems for commercial buildings can also play a significant role in achieving these goals. For tailored solutions, consider our custom AI model development services to enhance your energy management strategies.
6.5. Production Quality Metrics
Production quality metrics are essential for evaluating the effectiveness of manufacturing processes. These metrics help organizations ensure that products meet specified standards and customer expectations. Key performance indicators (KPIs) for production quality include defect rates, yield rates, and customer complaints. Regularly tracking these metrics can lead to continuous improvement in production processes, and high-quality production can enhance customer satisfaction and brand loyalty.
At Rapid Innovation, we utilize AI-driven analytics to monitor production quality metrics in real-time, enabling organizations to identify defects early and reduce waste. Our solutions help clients achieve higher First Pass Yield (FPY) and Overall Equipment Effectiveness (OEE), ultimately leading to improved operational efficiency.
Common production quality metrics include:
- First Pass Yield (FPY): Measures the percentage of products manufactured correctly without rework.
- Overall Equipment Effectiveness (OEE): Assesses the efficiency of a manufacturing process by considering availability, performance, and quality.
- Scrap Rate: Indicates the percentage of materials wasted during production, highlighting areas for improvement.
By focusing on production quality metrics, organizations can enhance their operational efficiency and maintain a competitive edge in the market.
7. Predictive Maintenance
Predictive maintenance is a proactive approach to equipment maintenance that uses data analysis to predict when maintenance should be performed. This strategy helps prevent unexpected equipment failures and reduces downtime. Predictive maintenance can reduce maintenance costs by 10-30% compared to traditional methods. It relies on data from sensors and IoT devices to monitor equipment health in real-time, and implementing predictive maintenance can extend the lifespan of machinery and improve overall productivity.
At Rapid Innovation, we harness the power of AI and machine learning to develop predictive maintenance solutions that analyze historical and real-time data, allowing organizations to anticipate equipment failures before they occur.
Key components of predictive maintenance include:
- Condition monitoring: Using sensors to track the performance and condition of equipment.
- Data analytics: Analyzing historical and real-time data to identify patterns and predict failures.
- Maintenance scheduling: Planning maintenance activities based on predictive insights to minimize disruption.
By adopting predictive maintenance, organizations can optimize their maintenance strategies, reduce costs, and enhance operational efficiency. Rapid Innovation is committed to helping clients achieve greater ROI through innovative AI solutions tailored to their specific needs.
7.1. Failure Prediction
Failure prediction is a critical aspect of maintenance management, particularly in industries reliant on machinery and equipment. By anticipating potential failures, organizations can minimize downtime and reduce maintenance costs.
- Predictive analytics: Utilizing data from sensors and historical performance to forecast when a failure might occur.
- Machine learning algorithms: These can analyze vast amounts of data to identify patterns that precede equipment failures.
- Real-time monitoring: Continuous data collection allows for immediate detection of anomalies, enabling proactive maintenance actions.
- Benefits: Â
- Reduced unplanned downtime
- Increased equipment lifespan
- Enhanced safety for workers
- Tools and technologies: Â
- IoT devices for real-time data collection
- Software platforms for data analysis and visualization
- Industry applications: Â
- Manufacturing, where machinery failure can halt production
- Transportation, to ensure vehicle reliability and safety
At Rapid Innovation, we leverage advanced AI techniques to implement failure prediction maintenance systems tailored to your specific operational needs. By integrating predictive analytics and machine learning, we help clients achieve greater ROI through reduced maintenance costs and improved equipment reliability. For more information on our services, learn more about AI agents for maintenance tracking.
7.2. Maintenance Scheduling
Maintenance scheduling is essential for optimizing the performance and longevity of equipment. Effective scheduling ensures that maintenance tasks are performed at the right time, minimizing disruptions to operations.
- Types of maintenance: Â
- Preventive maintenance: Scheduled based on time or usage to prevent failures.
- Predictive maintenance: Based on data analysis to predict when maintenance should occur.
- Corrective maintenance: Reactive maintenance performed after a failure has occurred.
- Factors influencing scheduling: Â
- Equipment criticality: Prioritizing maintenance for essential machinery.
- Resource availability: Ensuring that skilled personnel and parts are available when needed.
- Production schedules: Aligning maintenance activities with production demands to minimize impact.
- Benefits: Â
- Improved equipment reliability
- Lower maintenance costs
- Enhanced operational efficiency
- Tools and technologies: Â
- Computerized Maintenance Management Systems (CMMS) for tracking and scheduling tasks
- Mobile applications for on-the-go access to maintenance schedules
- Industry applications: Â
- Energy sector, where equipment reliability is crucial for continuous operation
- Healthcare, to ensure medical equipment is always functional
Rapid Innovation employs AI-driven maintenance scheduling solutions that optimize resource allocation and minimize operational disruptions. Our expertise in data analytics ensures that your maintenance activities are aligned with production demands, leading to significant cost savings and enhanced efficiency.
7.3. Component Lifetime Analysis
Component lifetime analysis involves assessing the expected lifespan of various parts within machinery and equipment. Understanding component longevity helps organizations plan for replacements and avoid unexpected failures.
- Factors affecting component life: Â
- Material properties: Different materials have varying resistance to wear and tear.
- Operating conditions: Temperature, humidity, and load can significantly impact lifespan.
- Maintenance practices: Regular maintenance can extend the life of components.
- Methods of analysis: Â
- Statistical analysis: Using historical data to predict failure rates and lifespans.
- Accelerated life testing: Simulating conditions to determine how long components will last under stress.
- Reliability-centered maintenance (RCM): A structured approach to determine the most effective maintenance strategies based on component reliability.
- Benefits: Â
- Cost savings through timely replacements
- Enhanced safety by preventing catastrophic failures
- Improved planning for inventory and resource allocation
- Tools and technologies: Â
- Software for reliability analysis and modeling
- Data analytics platforms for tracking component performance over time
- Industry applications: Â
- Aerospace, where component reliability is critical for safety
- Automotive, to ensure vehicle parts meet safety and performance standards
At Rapid Innovation, we utilize advanced data analytics and AI methodologies to conduct comprehensive component lifetime analyses. This enables our clients to make informed decisions regarding maintenance and replacements, ultimately leading to improved safety and significant cost savings.
7.4. Cost Optimization
Cost optimization is a critical aspect of any business strategy, particularly in today's competitive landscape. It involves analyzing and reducing expenses while maintaining or improving the quality of products and services. Effective cost optimization can lead to increased profitability and sustainability.
- Identify unnecessary expenses: Regularly review your budget to pinpoint areas where costs can be cut without sacrificing quality. Rapid Innovation can assist in this process by utilizing AI-driven analytics to uncover hidden costs and inefficiencies.
- Leverage technology: Implementing automation and advanced software solutions can streamline operations and reduce labor costs. Our AI solutions can automate repetitive tasks, allowing your team to focus on higher-value activities, thus enhancing productivity. This is particularly relevant in the context of cloud cost optimization strategies.
- Negotiate with suppliers: Building strong relationships with suppliers can lead to better pricing and terms, ultimately lowering costs. Rapid Innovation can provide data-driven insights to support negotiations, ensuring you secure the best possible deals.
- Monitor key performance indicators (KPIs): Track metrics related to cost efficiency to identify trends and areas for improvement. Our AI tools can help you set up real-time monitoring systems that provide actionable insights, which is essential for effective cost management strategies.
- Embrace lean methodologies: Adopt lean principles to eliminate waste and enhance productivity across all departments. We can guide you in implementing AI solutions that identify wasteful processes and suggest improvements, aligning with cost optimization plans.
- Invest in employee training: Well-trained employees can perform tasks more efficiently, reducing errors and associated costs. Rapid Innovation offers tailored training programs that leverage AI to enhance learning outcomes and operational efficiency.
- Implement cloud cost management strategies: Utilizing cloud services can lead to significant savings, but it requires careful management. Our expertise in cloud optimization strategy can help you maximize your cloud investments while minimizing unnecessary expenses.
- Explore multi-cloud optimization strategy: For organizations using multiple cloud providers, a multi-cloud optimization strategy can help streamline costs and improve resource allocation across platforms. Additionally, for accurate budgeting and planning, consider our AI project estimation services and insights on artificial intelligence cost estimation.
7.5. Resource Planning

‍
Resource planning is essential for ensuring that an organization effectively utilizes its assets, including human resources, finances, and materials. Proper resource planning helps in aligning resources with business goals and optimizing their use.
- Assess current resources: Conduct a thorough inventory of available resources to understand strengths and weaknesses. Our AI tools can analyze resource utilization patterns to provide a comprehensive overview.
- Forecast future needs: Anticipate future resource requirements based on projected growth and market trends. Rapid Innovation employs predictive analytics to help you make informed decisions about resource allocation.
- Prioritize resource allocation: Allocate resources based on strategic priorities to maximize impact and efficiency. We can assist in developing algorithms that optimize resource distribution according to your business objectives.
- Utilize project management tools: Implement software solutions that facilitate resource tracking and management. Our custom AI solutions can integrate with existing project management tools to enhance their capabilities.
- Encourage cross-department collaboration: Foster communication between departments to ensure resources are shared effectively. Rapid Innovation can implement collaborative platforms that utilize AI to streamline communication and resource sharing.
- Regularly review and adjust plans: Continuously evaluate resource allocation and make adjustments as necessary to respond to changing conditions. Our AI-driven dashboards provide real-time insights, enabling agile decision-making.
8. User Interface and Visualization

‍
User interface (UI) and visualization are crucial components of software design and user experience. A well-designed UI enhances usability, while effective visualization helps users understand complex data.
- Focus on simplicity: A clean and intuitive interface allows users to navigate easily and find what they need without confusion. Rapid Innovation emphasizes user-centered design principles to create interfaces that resonate with users.
- Use consistent design elements: Consistency in colors, fonts, and layouts helps create a cohesive experience that users can quickly adapt to. Our design team ensures that your UI adheres to best practices for consistency and branding.
- Implement responsive design: Ensure that your UI works seamlessly across various devices and screen sizes, enhancing accessibility. We leverage AI to test and optimize UI responsiveness across platforms.
- Prioritize user feedback: Regularly gather user input to identify pain points and areas for improvement in the interface. Our AI tools can analyze user feedback to provide actionable insights for UI enhancements.
- Utilize data visualization techniques: Employ charts, graphs, and infographics to present data in a way that is easy to understand and interpret. Rapid Innovation specializes in creating dynamic visualizations that make complex data accessible.
- Test usability: Conduct usability testing to identify issues and refine the interface based on real user interactions. We utilize AI-driven testing methodologies to ensure your UI meets user expectations.
By focusing on cost optimization, including cost optimization strategies, resource planning, and user interface design, organizations can enhance their operational efficiency and improve overall user satisfaction. Rapid Innovation is committed to helping you achieve these goals through our tailored AI solutions and expertise.
8.1. Dashboard Design
A well-designed dashboard is crucial for effective data visualization and decision-making. It serves as the central hub for users to access key performance indicators (KPIs) and other relevant metrics, ultimately driving business efficiency and enhancing ROI. This includes various approaches such as dashboard design in Tableau and power bi dashboard design.
- User-Centric Layout: The dashboard should prioritize user experience, ensuring that information is easily accessible and understandable. This approach minimizes the learning curve and accelerates user adoption, particularly in dashboard ui and dashboard user interface design.
- Visual Hierarchy: Use size, color, and placement to highlight the most important data. This helps users quickly identify critical information, enabling faster decision-making. Good dashboard design practices can enhance this aspect.
- Customization Options: Allow users to personalize their dashboards to fit their specific needs and preferences. This can include rearranging widgets or selecting which metrics to display, ensuring that the dashboard aligns with individual business objectives. Customization is key in dashboard ui design and dashboard interface design.
- Data Integration: Ensure that the dashboard can pull data from various sources, providing a comprehensive view of performance across different areas. This holistic perspective allows organizations to make informed decisions based on real-time data, which is essential for power bi report design.
- Responsive Design: The dashboard should be optimized for various devices, including desktops, tablets, and smartphones, ensuring accessibility on the go. This flexibility supports a mobile workforce and enhances productivity, making it a great dashboard design.
- Interactive Elements: Incorporate features like drill-down capabilities, filters, and tooltips to allow users to explore data in more depth. This interactivity fosters a deeper understanding of the data, leading to more strategic insights, which is a hallmark of dashboard ux. For more insights on the future of knowledge management in AI, check out AI Knowledge Management in 2024.
8.2. Real-time Monitoring Screens
Real-time monitoring screens are essential for organizations that need to track performance and respond to changes instantly. These screens provide live updates on various metrics, enabling proactive decision-making and improving operational efficiency.
- Instant Data Updates: Real-time monitoring screens should refresh data automatically, ensuring that users have the most current information at their fingertips. This immediacy allows for timely interventions and adjustments.
- Key Metrics Display: Focus on displaying critical metrics that require immediate attention, such as system performance, user activity, or sales figures. Highlighting these metrics ensures that decision-makers can act swiftly when necessary.
- Visual Indicators: Use color coding and visual alerts to signify performance levels, making it easy to identify issues at a glance. This visual clarity aids in prioritizing responses to emerging challenges.
- Customizable Views: Allow users to tailor their monitoring screens to focus on the metrics that matter most to them, enhancing relevance and usability. This customization empowers users to align monitoring with their specific goals.
- Integration with Alerts: Real-time monitoring should be linked with alert management systems to notify users of significant changes or anomalies in the data. This integration ensures that users are always informed and can take immediate action.
8.3. Alert Management
Effective alert management is vital for ensuring that users are promptly informed of important events or changes in data. A robust alert system can help organizations respond quickly to potential issues, ultimately safeguarding business performance.
- Threshold Settings: Users should be able to set thresholds for alerts based on specific metrics. This allows for tailored notifications that align with organizational goals, ensuring that users are alerted only to relevant changes.
- Multi-Channel Notifications: Alerts should be sent through various channels, such as email, SMS, or in-app notifications, ensuring that users receive timely updates regardless of their location. This multi-channel approach enhances responsiveness.
- Prioritization of Alerts: Implement a system to categorize alerts by severity, helping users focus on the most critical issues first. This prioritization streamlines the response process and minimizes potential disruptions.
- Historical Data Analysis: Provide users with access to historical alert data, enabling them to identify patterns and trends over time. This analysis can inform future strategies and improve overall decision-making.
- User Feedback Mechanism: Incorporate a feedback system that allows users to report false positives or irrelevant alerts, helping to refine the alert management process. Continuous improvement in alert accuracy enhances user trust and engagement.
- Integration with Incident Management: Ensure that alerts are linked to incident management systems, allowing for a streamlined response process when issues arise. This integration fosters a cohesive approach to incident resolution, ultimately enhancing operational resilience.
By leveraging these advanced dashboard designs, including dashboard design examples, real-time monitoring screens, and effective alert management systems, Rapid Innovation empowers organizations to achieve their business goals efficiently and effectively, driving greater ROI through informed decision-making and proactive management. For more information on our services, check out our hybrid exchange development.
8.4. Report Generation
Report generation is a critical feature in any software system, providing users with the ability to extract meaningful insights from data. Effective report generation can enhance decision-making processes and improve operational efficiency. At Rapid Innovation, we leverage advanced AI algorithms to help clients create customizable reports tailored to their specific needs by selecting relevant data fields, filters, and formats. Many systems we develop offer automated report generation, allowing users to schedule reports to be created and sent at regular intervals, thus saving valuable time and resources. Our report generation software includes options for automated report generation, making it easier for users to manage their reporting needs. Reports often include charts, graphs, and tables to present data visually, making it easier to understand trends and patterns. Users can typically export reports in various formats, such as PDF, Excel, or CSV, facilitating easy sharing and further analysis. Our advanced systems provide real-time reporting capabilities, ensuring that users have the most current data at their fingertips, which is essential for making informed business decisions.
- Customizable Reports: Users can tailor reports to meet specific needs, selecting relevant data fields, filters, and formats.
- Automated Reporting: Many systems offer automated report generation tools, allowing users to schedule reports to be created and sent at regular intervals.
- Visual Data Representation: Reports often include charts, graphs, and tables to present data visually, making it easier to understand trends and patterns.
- Export Options: Users can typically export reports in various formats, such as PDF, Excel, or CSV, facilitating easy sharing and further analysis.
- Real-time Data Access: Advanced systems provide real-time reporting capabilities, ensuring that users have the most current data at their fingertips.
8.5. Mobile Integration
Mobile integration is increasingly essential in today’s fast-paced business environment. It allows users to access systems and data from mobile devices, enhancing flexibility and productivity. Rapid Innovation specializes in developing mobile applications that enable users to access critical information and perform tasks from anywhere, whether in the office or on the go. Our mobile applications are designed with intuitive interfaces, ensuring ease of use for all users, regardless of technical expertise. Users can receive real-time alerts and notifications on their mobile devices, keeping them informed of important updates and changes. Some mobile integrations allow users to access certain features and data offline, ensuring continuity even without internet connectivity. Additionally, mobile integration fosters better communication and collaboration among team members, as they can share information and updates instantly.
- Access Anywhere: Mobile integration enables users to access critical information and perform tasks from anywhere, whether in the office or on the go.
- User-Friendly Interfaces: Mobile applications are designed with intuitive interfaces, ensuring ease of use for all users, regardless of technical expertise.
- Push Notifications: Users can receive real-time alerts and notifications on their mobile devices, keeping them informed of important updates and changes.
- Offline Capabilities: Some mobile integrations allow users to access certain features and data offline, ensuring continuity even without internet connectivity.
- Enhanced Collaboration: Mobile integration fosters better communication and collaboration among team members, as they can share information and updates instantly.
9. System Integration

‍
System integration refers to the process of connecting different software applications and systems to work together seamlessly. This is crucial for organizations looking to streamline operations and improve data flow. Rapid Innovation excels in system integration, ensuring that data is consistent across all platforms, thereby reducing errors and discrepancies. By automating data transfer between systems, organizations can eliminate manual data entry, saving time and reducing the risk of human error. Our integrated systems allow for centralized data management, making it easier to access and analyze information from a single source. As businesses grow, system integration allows for the easy addition of new applications and tools without disrupting existing workflows. Integrated systems can provide a more cohesive experience for customers, as data flows seamlessly between different touchpoints, leading to improved service delivery.
- Improved Data Consistency: System integration ensures that data is consistent across all platforms, reducing errors and discrepancies.
- Enhanced Efficiency: By automating data transfer between systems, organizations can eliminate manual data entry, saving time and reducing the risk of human error.
- Centralized Data Management: Integrated systems allow for centralized data management, making it easier to access and analyze information from a single source.
- Scalability: As businesses grow, system integration allows for the easy addition of new applications and tools without disrupting existing workflows.
- Better Customer Experience: Integrated systems can provide a more cohesive experience for customers, as data flows seamlessly between different touchpoints, leading to improved service delivery.
Incorporating these features into a software system can significantly enhance its functionality and user experience, ultimately driving better business outcomes. At Rapid Innovation, we are committed to helping our clients achieve greater ROI through our tailored development and consulting solutions, including options for AI as a Service report generation software, report generation tools, and open-source report generation tools, as well as the advantages of OpenAI integrating in mobile app development..
9.1. ERP Integration
Enterprise Resource Planning (ERP) integration is crucial for businesses looking to streamline operations and enhance productivity. ERP systems consolidate various business processes into a single framework, allowing for better data management and decision-making. Rapid Innovation specializes in implementing ERP solutions, including microsoft erp and enterprise resource planning systems integrate, that align with your business objectives, ensuring you achieve greater ROI.
- Centralized Data Management: ERP integration ensures that all departments have access to the same data, reducing discrepancies and improving accuracy. This centralized approach allows for more informed decision-making across the organization.
- Enhanced Collaboration: With integrated systems, teams can collaborate more effectively, sharing information in real-time and reducing silos. Rapid Innovation's expertise in ERP integration fosters a culture of collaboration, driving innovation and efficiency, particularly through erp and crm integration.
- Improved Reporting: ERP systems provide comprehensive reporting tools that help businesses analyze performance metrics and make informed decisions. Our tailored reporting solutions enable you to track KPIs that matter most to your business, including those related to salesforce enterprise resource planning.
- Cost Efficiency: By automating processes and reducing manual data entry, ERP integration can lead to significant cost savings. Rapid Innovation helps identify areas where automation can be implemented, maximizing your return on investment through services like erp integration services.
- Scalability: As businesses grow, integrated ERP systems can easily adapt to new requirements, supporting expansion without major overhauls. Our solutions are designed with scalability in mind, ensuring your ERP system evolves alongside your business, including capabilities for erp and ecommerce integration. For more insights on how technology is shaping ERP systems, check out this article on how artificial intelligence is transforming ERP software.
9.2. SCADA Systems
Supervisory Control and Data Acquisition (SCADA) systems are essential for monitoring and controlling industrial processes. These systems provide real-time data and control capabilities, making them vital for industries such as manufacturing, energy, and water management. Rapid Innovation offers SCADA integration services that enhance operational efficiency and data-driven decision-making.
- Real-Time Monitoring: SCADA systems allow operators to monitor processes in real-time, enabling quick responses to any issues that arise. Our solutions ensure that you have the necessary tools to maintain operational continuity.
- Data Collection: These systems collect vast amounts of data from sensors and devices, which can be analyzed for performance optimization. Rapid Innovation leverages AI analytics to turn this data into actionable insights.
- Remote Control: SCADA enables remote management of operations, reducing the need for on-site personnel and improving safety. Our remote monitoring solutions enhance operational flexibility and safety protocols.
- Alarm Management: SCADA systems can trigger alarms for abnormal conditions, allowing for immediate corrective actions. Rapid Innovation ensures that your SCADA system is equipped with robust alarm management features.
- Integration with Other Systems: SCADA can be integrated with ERP and MES systems, providing a comprehensive view of operations and enhancing decision-making. Our integration services ensure seamless communication between systems, optimizing your operational landscape, including wms erp integration.
9.3. MES Integration
Manufacturing Execution Systems (MES) play a critical role in managing and monitoring production processes on the shop floor. Integrating MES with other systems like ERP and SCADA can significantly enhance operational efficiency. Rapid Innovation's MES integration services are designed to help you achieve optimal production outcomes.
- Real-Time Production Tracking: MES provides real-time visibility into production processes, helping to identify bottlenecks and optimize workflows. Our solutions enable you to respond swiftly to production challenges.
- Quality Control: MES integration allows for better quality management by tracking defects and ensuring compliance with standards. Rapid Innovation helps implement quality control measures that align with industry best practices.
- Resource Management: By integrating MES with ERP, businesses can optimize resource allocation, ensuring that materials and labor are used efficiently. Our expertise in resource management ensures that you maximize your operational capabilities, including through solutions like magento erp system integration.
- Data-Driven Decisions: MES systems collect data that can be analyzed to improve production strategies and reduce waste. Rapid Innovation employs advanced analytics to help you make informed decisions that drive efficiency.
- Enhanced Communication: Integration facilitates better communication between the shop floor and management, ensuring that everyone is aligned with production goals. Our solutions foster a collaborative environment that enhances overall productivity, including through crm integration with erp.
9.4. Cloud Services
Cloud services have revolutionized the way businesses operate by providing scalable, flexible, and cost-effective solutions. These services allow organizations to access computing resources over the internet, eliminating the need for extensive on-premises infrastructure.
- Types of Cloud Services: Â
- Infrastructure as a Service (IaaS): Provides virtualized computing resources over the internet. Examples include Amazon Web Services (AWS), googledrive, and Microsoft Azure.
- Platform as a Service (PaaS): Offers hardware and software tools over the internet, primarily for application development. Google App Engine and google cloud computing are notable examples.
- Software as a Service (SaaS): Delivers software applications over the internet on a subscription basis. Popular examples include Salesforce, Microsoft 365, and cloud based storage solutions.
- Benefits of Cloud Services: Â
- Cost Efficiency: Reduces the need for physical hardware and maintenance costs, allowing businesses to allocate resources more effectively.
- Scalability: Easily scale resources up or down based on demand, ensuring that businesses can adapt to changing market conditions without incurring unnecessary costs.
- Accessibility: Access services from anywhere with an internet connection, facilitating remote work and collaboration, such as with icloud storage plans and cloud storage.
- Disaster Recovery: Provides backup and recovery solutions to protect data, ensuring business continuity in the event of an incident.
- Security Considerations: Â
- Ensure data encryption both in transit and at rest to protect sensitive information.
- Regularly update and patch cloud services to protect against vulnerabilities, maintaining a secure environment.
- Implement strong access controls and authentication measures to safeguard against unauthorized access.
9.5. Third-party Applications
Third-party applications are software solutions developed by external vendors that can integrate with existing systems to enhance functionality. These applications can significantly improve productivity and streamline operations.
- Types of Third-party Applications: Â
- Productivity Tools: Applications like Slack and Trello that facilitate team collaboration and project management.
- CRM Systems: Customer Relationship Management tools such as HubSpot and Zoho that help manage customer interactions and data.
- E-commerce Platforms: Solutions like Shopify and WooCommerce that enable businesses to sell products online.
- Advantages of Using Third-party Applications: Â
- Specialization: Access to specialized features and functionalities that may not be available in-house, allowing businesses to leverage best-in-class solutions.
- Cost Savings: Often more affordable than developing custom solutions, enabling organizations to achieve greater ROI.
- Faster Implementation: Quick deployment and integration with existing systems, reducing time-to-market for new initiatives.
- Integration Challenges: Â
- Ensure compatibility with existing software and systems to avoid disruptions.
- Address data security and privacy concerns when sharing information with third-party applications, maintaining compliance with regulations.
- Monitor performance and reliability to avoid disruptions in service, ensuring a seamless user experience.
10. Implementation Guide
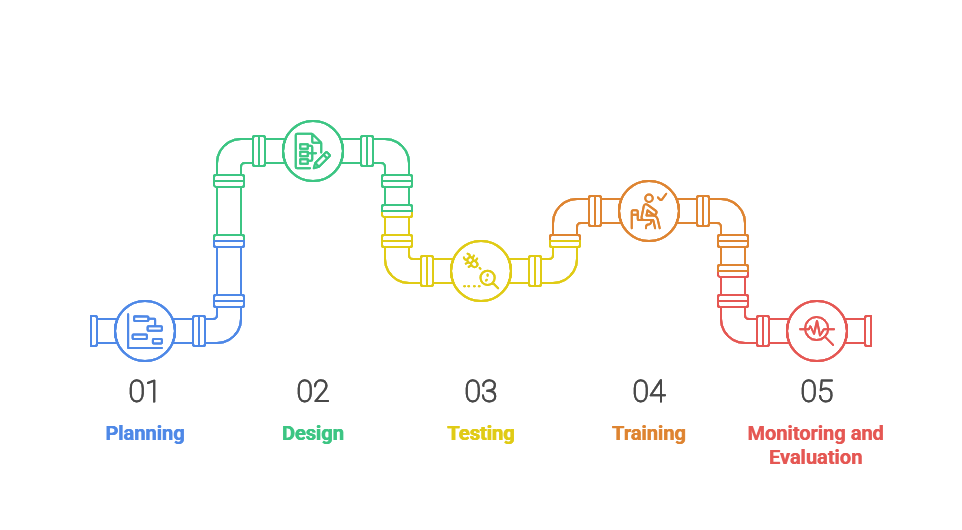
‍
An implementation guide is a comprehensive document that outlines the steps necessary to successfully deploy a new system or technology. It serves as a roadmap for organizations to follow during the implementation process.
- Key Components of an Implementation Guide: Â
- Project Scope: Clearly define the objectives, deliverables, and timeline for the project to align expectations.
- Stakeholder Engagement: Identify key stakeholders and their roles in the implementation process, ensuring buy-in and support.
- Resource Allocation: Determine the resources required, including personnel, technology, and budget, to facilitate a smooth implementation.
- Steps for Successful Implementation: Â
- Planning: Conduct a thorough analysis of current systems and processes to identify areas for improvement, setting the stage for effective change.
- Design: Create a detailed design of the new system, including architecture and user interfaces, to ensure alignment with business needs.
- Testing: Implement a testing phase to identify and resolve any issues before full deployment, minimizing risks.
- Training: Provide training for users to ensure they are comfortable with the new system, enhancing adoption and productivity.
- Monitoring and Evaluation: Continuously monitor the system's performance and gather feedback for future improvements, fostering a culture of continuous enhancement.
- Best Practices: Â
- Establish clear communication channels among all stakeholders to facilitate collaboration and transparency.
- Set realistic timelines and milestones to track progress, ensuring accountability.
- Be prepared to adapt the plan based on feedback and unforeseen challenges, maintaining flexibility in the implementation process.
At Rapid Innovation, we leverage our expertise in cloud services, including icloud, onedrive, and gcp google cloud, as well as third-party applications to help clients achieve their business goals efficiently and effectively, ultimately driving greater ROI. For more information on AI as a Service, check out this guide.
10.1. System Requirements
Before installing any software, it is crucial to understand the system requirements to ensure optimal performance. System requirements typically include hardware specifications, operating system compatibility, and additional software dependencies.
- Hardware Specifications: Â
- Processor: A minimum of a dual-core processor is recommended for efficient operation, particularly when running AI algorithms that require significant computational power.
- RAM: At least 4GB of RAM is necessary, though 8GB or more is ideal for better multitasking, especially when handling large datasets or complex models. For example, software like sql server 2012 and sql server 2014 have specific RAM requirements that should be considered.
- Storage: Sufficient disk space is required, usually around 10GB or more, depending on the software size and data storage needs. For AI applications, consider additional space for model training data. Ensure you have enough storage for applications like vmware workstation and oracle vm virtualbox.
- Graphics: A dedicated graphics card may be needed for applications that require high graphical performance, particularly in machine learning tasks that leverage GPU acceleration.
- Operating System Compatibility: Â
- Ensure the software is compatible with your operating system, whether it’s Windows, macOS, or Linux. Check for specific version requirements, as some software may not support older or newer OS versions. For instance, windows 11 need internet to install, and certain applications may require the windows c++ redistributable.
- Additional Software Dependencies: Â
- Some applications may require additional software, such as .NET Framework, Java Runtime Environment, or specific libraries. For example, virtualbox needs microsoft visual c++, and applications like adobe illustrator may have their own installation requirements. Always check the documentation for any prerequisites to ensure all components function correctly. For more information on software recommendations, you can refer to this comprehensive guide.
10.2. Installation Process
The installation process can vary depending on the software, but it generally follows a standard procedure. Understanding this process can help avoid common pitfalls and ensure a smooth installation.
- Download the Software: Â
- Obtain the software from the official website or a trusted source to avoid malware. Ensure you download the correct version for your operating system.
- Run the Installer: Â
- Locate the downloaded file and double-click to run the installer. You may need administrative privileges to proceed.
- Follow the Installation Wizard: Â
- Most installations will guide you through a series of prompts. Pay attention to:
- License agreements: Read and accept the terms.
- Installation location: Choose the default location or specify a custom folder.
- Component selection: Some installers allow you to choose which components to install, which can be crucial for optimizing AI software performance. For example, when installing software like whmcs or lansweeper, ensure you select the necessary components.
- Most installations will guide you through a series of prompts. Pay attention to:
- Complete the Installation: Â
- Once all prompts are completed, click the finish button. Some installations may require a system restart to finalize the process.
- Verify Installation: Â
- After installation, check if the software runs correctly. Look for any updates or patches that may need to be applied to ensure you are using the latest features and security enhancements.
10.3. Configuration Steps
After installation, configuring the software is essential to tailor it to your needs. Proper configuration can enhance functionality and improve user experience.
- Initial Setup: Â
- Launch the software and complete any initial setup wizards. This may include setting up user accounts, preferences, and default settings that align with your business objectives.
- Adjust Settings: Â
- Navigate to the settings or preferences menu to customize options such as:
- User interface: Change themes, layouts, or display settings to improve usability.
- Notifications: Set preferences for alerts and updates to stay informed about system performance and updates.
- Performance: Adjust settings for optimal performance based on your system capabilities, particularly for resource-intensive AI tasks.
- Navigate to the settings or preferences menu to customize options such as:
- Integrate with Other Tools: Â
- If the software supports integrations, connect it with other tools you use. This could include cloud storage services, productivity apps, or communication platforms, enhancing collaboration and data accessibility.
- Backup Configuration: Â
- Once configured, consider backing up your settings. This can save time if you need to reinstall or transfer the software to another system, ensuring continuity in your AI projects.
- Test Functionality: Â
- After configuration, test the software to ensure all features work as expected. This may involve running sample tasks or projects to verify performance, which is critical for achieving greater ROI in your AI initiatives.
By following these steps for system requirements, installation, and configuration, users can ensure a seamless experience with their software, ultimately leading to more efficient and effective business outcomes with the support of Rapid Innovation's expertise in AI development and consulting.
10.4. Testing Procedures
Testing procedures are critical in ensuring that a product or system meets its intended requirements and functions correctly. A well-structured testing process can help identify defects, improve quality, and enhance user satisfaction, ultimately leading to greater ROI for your business.
- Types of Testing: Â
- Unit Testing: Focuses on individual components to ensure they work as intended, allowing for early detection of issues.
- Integration Testing: Examines how different components interact with each other, ensuring seamless functionality across the system.
- System Testing: Validates the complete and integrated software product, confirming that it meets all specified requirements.
- User Acceptance Testing (UAT): Conducted by end-users to confirm the system meets their needs, ensuring that the final product aligns with user expectations.
- Testing Phases: Â
- Planning: Define the scope, objectives, and resources required for testing, which helps in aligning testing efforts with business goals.
- Design: Create test cases and scenarios based on requirements, ensuring comprehensive coverage of functionalities.
- Execution: Carry out the tests and document the results, providing valuable insights into product performance. This may include various medical tests such as city scan, cat scans, electrocardiogram test, and hida scan.
- Reporting: Analyze the outcomes and report any defects or issues, facilitating informed decision-making for further development.
- Best Practices: Â
- Automate repetitive tests to save time and reduce human error, allowing your team to focus on more complex testing scenarios.
- Involve stakeholders early in the testing process to gather feedback, ensuring that the product meets business and user requirements.
- Maintain clear documentation for all testing activities to ensure traceability, which is essential for compliance and future reference.
At Rapid Innovation, we specialize in Stable Diffusion Development to enhance your testing procedures and ensure your products meet the highest standards of quality and performance. For more insights on improving testing processes, check out our article on AI agents in software testing.
10.5. User Training
User training is essential for ensuring that end-users can effectively utilize a system or product. Proper training can lead to increased productivity, reduced errors, and higher user satisfaction, ultimately contributing to a better return on investment.
- Training Methods: Â
- Instructor-Led Training: Traditional classroom-style training led by an expert, providing personalized guidance.
- Online Training: E-learning modules that users can complete at their own pace, offering flexibility and accessibility.
- Hands-On Training: Practical sessions where users can practice using the system, reinforcing learning through experience.
- Training Content: Â
- System Overview: Introduction to the system's features and functionalities, ensuring users understand its capabilities.
- Step-by-Step Guides: Detailed instructions on how to perform specific tasks, facilitating ease of use. This may include guides on procedures like electrocardiogram ecg, transvaginal ultrasound, and bone density exam.
- FAQs and Troubleshooting: Common issues and their solutions to assist users, enhancing their confidence in using the system.
- Best Practices: Â
- Assess user needs to tailor training programs accordingly, ensuring relevance and effectiveness.
- Provide ongoing support and resources for users after initial training, fostering continuous improvement and user satisfaction.
- Gather feedback to continuously improve training materials and methods, aligning them with user experiences and expectations.
11. Data Security and Management
Data security and management are vital for protecting sensitive information and ensuring compliance with regulations. A robust data management strategy helps organizations safeguard their data while maximizing its value, which is crucial for maintaining trust and achieving business objectives.
- Key Components of Data Security: Â
- Access Control: Implementing user authentication and authorization to restrict data access, ensuring that only authorized personnel can view sensitive information.
- Encryption: Protecting data at rest and in transit to prevent unauthorized access, safeguarding your organization’s critical assets.
- Regular Audits: Conducting periodic reviews to identify vulnerabilities and ensure compliance, helping to mitigate risks.
- Data Management Practices: Â
- Data Classification: Categorizing data based on sensitivity and importance, allowing for tailored security measures.
- Backup and Recovery: Establishing regular backup procedures to prevent data loss, ensuring business continuity.
- Data Retention Policies: Defining how long data should be kept and when it should be disposed of, optimizing storage and compliance.
- Best Practices: Â
- Train employees on data security awareness to minimize human error, fostering a culture of security within the organization.
- Use strong passwords and multi-factor authentication to enhance security, protecting against unauthorized access.
- Stay updated on the latest security threats and technologies to protect data effectively, ensuring that your organization remains resilient against evolving risks.
At Rapid Innovation, we leverage our expertise in AI and data management to help clients implement these best practices, ensuring that their systems are robust, user-friendly, and secure, ultimately driving greater ROI and business success.
11.1. Data Protection
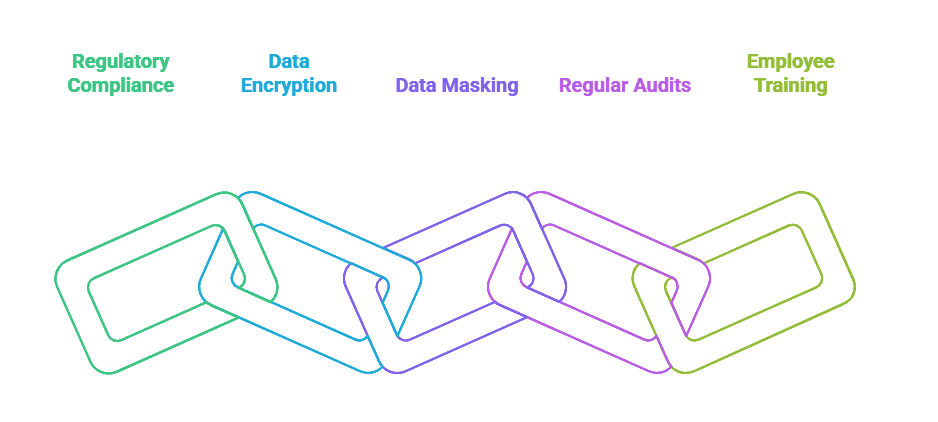
‍
Data protection is a critical aspect of information security that involves safeguarding sensitive data from unauthorized access, corruption, or loss. Organizations must implement robust data protection strategies, such as a data privacy strategy and a DLP strategy, to ensure compliance with regulations and maintain customer trust. Rapid Innovation can assist clients in achieving these goals through tailored AI-driven solutions. Key strategies include:
- Regulatory Compliance: Many industries are governed by strict data protection laws, such as GDPR in Europe and HIPAA in the United States. Compliance with these regulations is essential to avoid hefty fines and legal repercussions. Rapid Innovation can help automate compliance monitoring, ensuring that organizations stay up-to-date with regulatory changes, including GDPR compliance strategy.
- Data Encryption: Encrypting data both at rest and in transit is vital. This ensures that even if data is intercepted or accessed without authorization, it remains unreadable without the proper decryption keys. Our AI solutions can enhance encryption processes, making them more efficient and secure.
- Data Masking: This technique involves obscuring specific data within a database to protect it from unauthorized access while still allowing for data analysis and processing. Rapid Innovation can implement advanced data masking techniques that leverage AI to maintain data utility while ensuring privacy.
- Regular Audits: Conducting regular audits of data protection measures helps identify vulnerabilities and ensures that policies are being followed effectively. Our AI tools can streamline the audit process, providing real-time insights and reducing the time and resources required for compliance checks.
- Employee Training: Educating employees about data protection best practices is crucial. Human error is often a significant factor in data breaches, so training can mitigate risks. Rapid Innovation offers AI-driven training programs that adapt to individual learning styles, ensuring that employees are well-equipped to handle data responsibly. The data protection strategy of an organisation will ensure that employees understand their roles in safeguarding sensitive information.
11.2. Access Control
Access control is a security technique that regulates who can view or use resources in a computing environment. It is essential for protecting sensitive information and ensuring that only authorized personnel have access to critical systems. Effective access control measures include:
- Role-Based Access Control (RBAC): This method assigns permissions based on the user's role within the organization. It simplifies management and ensures that employees only have access to the information necessary for their job functions. Rapid Innovation can develop customized RBAC systems that integrate seamlessly with existing workflows.
- Multi-Factor Authentication (MFA): Implementing MFA adds an extra layer of security by requiring users to provide two or more verification factors to gain access. This significantly reduces the risk of unauthorized access. Our AI solutions can enhance MFA processes, making them more user-friendly while maintaining high security.
- Access Control Lists (ACLs): ACLs are used to define which users or system processes have permission to access specific resources. They help in managing permissions effectively. Rapid Innovation can automate the management of ACLs, ensuring that access rights are updated in real-time as roles change.
- Regular Reviews: Periodically reviewing access permissions ensures that only current employees have access to sensitive data and that permissions are adjusted as roles change. Our AI tools can facilitate these reviews, providing insights into access patterns and potential risks.
- Logging and Monitoring: Keeping detailed logs of access attempts and monitoring for unusual activity can help detect and respond to potential security breaches quickly. Rapid Innovation can implement AI-driven monitoring systems that analyze access logs in real-time, alerting organizations to suspicious activities.
11.3. Backup Systems
Backup systems are essential for data recovery and business continuity. They ensure that critical data is preserved and can be restored in the event of data loss due to hardware failure, cyberattacks, or natural disasters. Important practices for effective backup systems include:
- Regular Backups: Establishing a routine for regular backups is crucial. This can be daily, weekly, or monthly, depending on the volume of data and the organization’s needs. Rapid Innovation can automate backup schedules, ensuring that data is consistently protected without manual intervention.
- Offsite Storage: Storing backups in a secure offsite location protects data from local disasters. Cloud storage solutions are increasingly popular for this purpose. Our team can help clients implement secure cloud backup solutions that leverage AI for enhanced data integrity.
- Testing Backup Restores: Regularly testing the restore process ensures that backups are functional and that data can be recovered quickly when needed. Rapid Innovation can develop automated testing protocols that verify backup integrity without disrupting business operations.
- Incremental Backups: Instead of backing up all data every time, incremental backups only save changes made since the last backup. This approach saves time and storage space. Our AI solutions can optimize incremental backup processes, ensuring efficiency and reliability.
- Data Retention Policies: Establishing clear data retention policies helps determine how long backups should be kept and when they should be deleted, ensuring compliance with legal and regulatory requirements. Rapid Innovation can assist in creating and managing these policies, utilizing AI to ensure compliance and minimize risks, including strategies to comply with data handling legislation.
By leveraging Rapid Innovation's expertise in AI and data protection, organizations can achieve greater ROI through enhanced security, compliance, and operational efficiency, including an enterprise data protection strategy and a database security strategy.
11.4. Compliance Requirements
Compliance requirements are essential for organizations to ensure they adhere to laws, regulations, and standards relevant to their industry. These requirements can vary significantly based on the sector, geographical location, and specific operational practices.
- Regulatory frameworks: Organizations must understand the regulatory frameworks that govern their operations, such as GDPR for data protection in Europe or HIPAA for healthcare in the United States. Additionally, compliance with regulations like Sarbanes-Oxley (SOX) and ITAR is crucial for organizations in finance and defense sectors. Rapid Innovation can assist clients in navigating these complex regulations by implementing AI-driven compliance solutions that automate monitoring and reporting processes, thereby reducing the risk of non-compliance and associated penalties.
- Industry standards: Compliance with industry standards, such as ISO 27001 for information security management, is crucial for maintaining credibility and trust with clients and stakeholders. Organizations must also consider compliance regulations by industry, including PCI data security standards and GLBA for financial institutions. Our expertise in AI can help organizations streamline their compliance efforts, ensuring they meet these standards efficiently and effectively.
- Internal policies: Companies should develop internal policies that align with external compliance requirements, ensuring that all employees are aware of their responsibilities. This includes understanding section 508 compliance for accessibility and ADA requirements. Rapid Innovation can support the creation of tailored internal policies that leverage AI insights to enhance compliance culture within the organization.
- Regular training: Ongoing training programs for employees help ensure that everyone understands compliance requirements and the importance of adhering to them. We offer AI-powered training solutions that personalize learning experiences, making compliance training more engaging and effective. This is particularly important for compliance certification related to SOX and ITAR compliance.
- Documentation: Maintaining accurate and up-to-date documentation is vital for demonstrating compliance during audits and inspections. This includes documentation for Sarbanes-Oxley audits and 21 CFR Part 11 compliance in the pharmaceutical industry. Our AI solutions can automate documentation processes, ensuring that records are consistently updated and easily accessible.
- Risk assessments: Conducting regular risk assessments helps identify potential compliance gaps and allows organizations to take corrective actions proactively. Rapid Innovation employs advanced analytics to enhance risk assessment processes, enabling organizations to anticipate and mitigate compliance risks effectively, including those related to contractor compliance. For organizations in the insurance sector, our tailored AI insurance solutions can further enhance compliance efforts. Additionally, our insights on AI and machine learning for regulatory compliance can provide organizations with innovative strategies to meet their compliance needs.
11.5. Audit Trails
Audit trails are systematic records that provide a chronological sequence of events related to a specific process or transaction. They are crucial for ensuring accountability and transparency within an organization.
- Data integrity: Audit trails help maintain data integrity by tracking changes made to data, including who made the changes and when. Our AI solutions can enhance data tracking capabilities, ensuring that organizations have a clear view of data modifications.
- Compliance verification: Organizations can use audit trails to demonstrate compliance with regulatory requirements during audits, showcasing their adherence to established protocols. This is particularly relevant for compliance with Sarbanes-Oxley and ITAR regulations. Rapid Innovation's AI tools can automate the generation of compliance reports, simplifying the verification process.
- Incident investigation: In the event of a security breach or data loss, audit trails provide valuable information for investigating the incident and determining the root cause. Our AI-driven analytics can assist in quickly identifying anomalies and potential vulnerabilities.
- User activity monitoring: By tracking user activities, organizations can identify unauthorized access or suspicious behavior, enhancing overall security. Rapid Innovation offers AI solutions that provide real-time monitoring and alerts for unusual user activities.
- System performance: Audit trails can also help monitor system performance and identify areas for improvement, ensuring that processes run efficiently. Our AI analytics can provide insights into system performance trends, enabling organizations to optimize their operations.
- Retention policies: Establishing clear retention policies for audit trails is essential to balance compliance needs with storage costs. We can help organizations develop AI-driven retention strategies that ensure compliance while managing data storage effectively.
12. Industry Applications
Industry applications refer to the specific ways in which technologies, processes, or methodologies are utilized across various sectors. Understanding these applications can help organizations leverage best practices and improve operational efficiency.
- Healthcare: In the healthcare sector, electronic health records (EHR) systems streamline patient data management, enhance communication among providers, and improve patient care outcomes. Rapid Innovation can implement AI solutions that enhance EHR systems, improving data accuracy and accessibility.
- Finance: Financial institutions use advanced analytics and machine learning algorithms to detect fraudulent transactions, manage risk, and comply with regulatory requirements. Our expertise in AI can help financial organizations enhance their fraud detection capabilities and streamline compliance processes, including adherence to GLBA and SOX.
- Manufacturing: The manufacturing industry employs automation and IoT technologies to optimize production processes, reduce waste, and improve supply chain management. Rapid Innovation can integrate AI solutions that enhance predictive maintenance and operational efficiency.
- Retail: Retailers utilize customer relationship management (CRM) systems to analyze consumer behavior, personalize marketing efforts, and enhance customer experiences. Our AI-driven analytics can provide deeper insights into customer preferences, enabling more effective marketing strategies.
- Education: Educational institutions implement learning management systems (LMS) to facilitate online learning, track student progress, and improve engagement. Rapid Innovation can enhance LMS platforms with AI features that personalize learning experiences for students.
- Transportation: The transportation sector leverages GPS and route optimization software to enhance logistics, reduce fuel consumption, and improve delivery times. Our AI solutions can optimize routing and scheduling, leading to significant cost savings and improved service delivery.
By understanding compliance requirements, maintaining robust audit trails, and exploring industry applications, organizations can enhance their operational effectiveness and ensure they meet the demands of their respective sectors. Rapid Innovation is committed to helping clients achieve these goals through tailored AI solutions that drive efficiency and effectiveness.
12.1. Manufacturing
Manufacturing is a critical sector that encompasses the production of goods through the transformation of raw materials into finished products. This sector plays a vital role in the economy, contributing significantly to GDP and employment.
- Diverse Industries: Manufacturing includes various industries such as automotive, electronics, textiles, and food processing. Technologies like computer aided machining and cnc machining are integral to these industries, enhancing precision and efficiency.
- Technological Advancements: The integration of technology, such as automation and robotics, has revolutionized manufacturing processes, increasing efficiency and reducing costs. At Rapid Innovation, we leverage AI-driven solutions to optimize production lines, enabling manufacturers to achieve higher throughput and lower operational costs. Techniques such as metal 3d printing and selective laser melting are examples of advancements that are reshaping the landscape of manufacturing.
- Supply Chain Management: Effective supply chain management is essential for timely production and distribution, ensuring that materials are available when needed. Our AI algorithms can analyze supply chain data in real-time, predicting disruptions and optimizing inventory levels, which leads to significant cost savings and improved service levels. The use of demand flow technology can further enhance these processes.
- Sustainability: Many manufacturers are adopting sustainable practices, focusing on reducing waste and energy consumption to minimize their environmental impact. Rapid Innovation assists clients in implementing AI solutions that monitor energy usage and waste generation, helping them achieve sustainability goals while enhancing profitability. Additive manufacturing techniques, including laminated object manufacturing and electron beam melting, contribute to more sustainable production methods.
- Global Competition: Manufacturers face competition not only locally but also globally, necessitating innovation and quality improvements to maintain market share. Our consulting services guide manufacturers in adopting cutting-edge AI technologies that foster innovation, ensuring they remain competitive in a rapidly evolving market. The integration of manufacturing technology and manufacturing medical devices is crucial in this competitive landscape. Additionally, our expertise in computer vision software development can further enhance manufacturing processes by enabling advanced quality control and automation. Furthermore, the use of AI agents for grid management can optimize energy consumption in manufacturing facilities, contributing to overall efficiency and sustainability.
12.2. Energy Sector
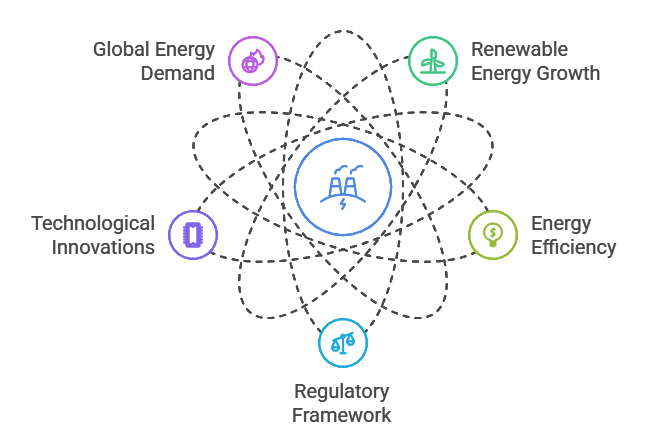
‍
The energy sector is a crucial component of the global economy, responsible for the production and distribution of energy resources. This sector includes various forms of energy, such as fossil fuels, renewable energy, and nuclear power.
- Renewable Energy Growth: The shift towards renewable energy sources, such as solar, wind, and hydroelectric power, is gaining momentum as countries aim to reduce carbon emissions. Rapid Innovation supports energy companies in deploying AI models that optimize energy production and consumption, enhancing the efficiency of renewable energy systems.
- Energy Efficiency: Improving energy efficiency in buildings, transportation, and industrial processes is essential for reducing overall energy consumption and costs. Our AI solutions analyze energy usage patterns, providing actionable insights that help organizations reduce waste and lower energy bills.
- Regulatory Framework: The energy sector is heavily regulated, with policies and regulations impacting production, distribution, and pricing. We help clients navigate these complexities by developing AI-driven compliance solutions that ensure adherence to regulatory requirements while minimizing operational risks.
- Technological Innovations: Advances in technology, such as smart grids and energy storage solutions, are transforming how energy is produced and consumed. Rapid Innovation's expertise in AI allows us to create predictive maintenance systems for energy infrastructure, reducing downtime and maintenance costs.
- Global Energy Demand: As the global population grows, so does the demand for energy, leading to increased exploration and investment in various energy sources. Our consulting services assist energy companies in identifying new market opportunities and optimizing their resource allocation through data-driven strategies.
12.3. Transportation
Transportation is a vital sector that facilitates the movement of goods and people. It encompasses various modes, including road, rail, air, and maritime transport.
- Infrastructure Development: Investment in transportation infrastructure, such as roads, bridges, and airports, is essential for efficient movement and economic growth. Rapid Innovation provides AI solutions that analyze traffic patterns and infrastructure usage, helping governments and organizations make informed investment decisions.
- Logistics and Supply Chain: Transportation plays a key role in logistics, ensuring that products reach consumers in a timely manner. Our AI-driven logistics platforms optimize routing and scheduling, reducing delivery times and costs while enhancing customer satisfaction.
- Environmental Impact: The transportation sector is a significant contributor to greenhouse gas emissions, prompting a shift towards more sustainable practices, such as electric vehicles and public transit systems. We assist clients in implementing AI technologies that monitor emissions and improve fuel efficiency, contributing to a greener future.
- Technological Integration: Innovations like autonomous vehicles and ride-sharing apps are reshaping the transportation landscape, improving efficiency and convenience. Rapid Innovation is at the forefront of developing AI solutions that enhance the safety and efficiency of autonomous systems, paving the way for smarter transportation networks.
- Global Trade: Transportation is crucial for international trade, enabling the exchange of goods across borders and contributing to economic development. Our AI analytics tools help businesses optimize their global supply chains, ensuring they remain competitive in the international market. The integration of technologies such as flex pcb in transportation systems can further enhance efficiency and reliability.
12.4. Process Industries
Process industries encompass a wide range of sectors that transform raw materials into finished products through chemical, physical, or biological processes. These industries include chemicals, petrochemicals, pharmaceuticals, food and beverages, and more.
- Characteristics of Process Industries: Â
- Continuous production: Unlike discrete manufacturing, process industries often operate continuously, producing large volumes of products.
- Complex processes: The production processes are typically intricate, involving multiple stages and requiring precise control.
- Safety and compliance: Due to the nature of the materials handled, safety regulations and compliance with environmental standards are critical.
- Key Challenges: Â
- Equipment reliability: Downtime can lead to significant losses, making maintenance and reliability crucial.
- Quality control: Maintaining consistent product quality is essential, often requiring advanced monitoring and control systems.
- Supply chain management: Fluctuations in raw material availability can impact production schedules and costs.
- Process industries challenges: These challenges include managing the complexity of operations, ensuring safety in handling hazardous materials, and adapting to regulatory changes.
- Technological Advancements: Â
- Automation: The use of automation technologies enhances efficiency and reduces human error. Rapid Innovation can assist in implementing AI-driven automation solutions that optimize production processes, leading to reduced operational costs and increased output.
- Data analytics: Advanced analytics help in optimizing processes and improving decision-making. By leveraging AI and machine learning, we enable clients to gain insights from their data, enhancing their ability to predict maintenance needs and improve quality control.
- Sustainability: Many process industries are adopting greener practices to minimize environmental impact. Rapid Innovation supports clients in integrating AI solutions that monitor and reduce energy consumption, contributing to sustainability goals. Additionally, our generative AI consulting services can further enhance innovation and efficiency in these sectors.
12.5. Utilities
Utilities refer to essential services that provide water, electricity, gas, and telecommunications to consumers and businesses. These services are critical for daily life and economic activities.
- Types of Utilities: Â
- Water utilities: Responsible for the supply and treatment of water for residential and industrial use.
- Electric utilities: Generate, transmit, and distribute electricity to consumers.
- Gas utilities: Provide natural gas for heating, cooking, and industrial processes.
- Challenges Faced by Utilities: Â
- Aging infrastructure: Many utility companies are dealing with outdated systems that require significant investment for upgrades. Rapid Innovation can help modernize these systems through AI-driven predictive maintenance solutions, reducing downtime and extending asset life.
- Regulatory pressures: Utilities must comply with various regulations, which can impact operational flexibility and costs. Our consulting services can guide clients in navigating these complexities while optimizing their operations.
- Demand management: Balancing supply and demand is crucial, especially during peak usage times. AI algorithms developed by Rapid Innovation can forecast demand patterns, enabling utilities to optimize resource allocation and reduce costs.
- Innovations in Utilities: Â
- Smart grids: The implementation of smart grid technology allows for better monitoring and management of electricity distribution. Rapid Innovation specializes in developing AI solutions that enhance grid reliability and efficiency.
- Renewable energy: Utilities are increasingly integrating renewable energy sources, such as solar and wind, into their energy mix. We assist clients in optimizing their renewable energy strategies through advanced analytics and AI modeling.
- Water conservation technologies: Advanced metering and treatment technologies help in reducing water waste and improving efficiency. Our AI solutions can analyze consumption patterns, leading to more effective conservation strategies.
13. Best Practices
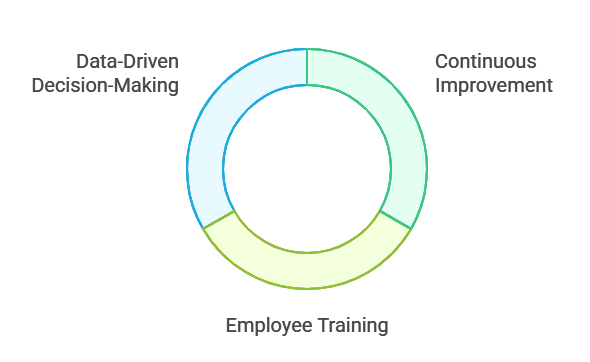
‍
Implementing best practices in any industry is essential for enhancing efficiency, ensuring safety, and achieving sustainability. Best practices are proven methods that lead to superior performance.
- Key Best Practices: Â
- Continuous improvement: Regularly assess processes and seek opportunities for enhancement. Rapid Innovation advocates for a culture of continuous improvement, leveraging AI to identify inefficiencies and recommend solutions.
- Employee training: Invest in training programs to ensure that employees are skilled and knowledgeable about the latest technologies and safety protocols. We offer tailored training solutions that empower teams to effectively utilize AI tools.
- Data-driven decision-making: Utilize data analytics to inform decisions and optimize operations. Our expertise in AI analytics enables clients to make informed decisions that drive profitability.
- Safety and Compliance: Â
- Regular audits: Conduct safety audits to identify potential hazards and ensure compliance with regulations. Rapid Innovation can implement AI-driven audit systems that streamline compliance processes.
- Incident reporting: Establish a culture of transparency where employees can report safety concerns without fear of repercussions.
- Sustainability Initiatives: Â
- Waste reduction: Implement strategies to minimize waste generation and promote recycling. Our AI solutions can track waste metrics and suggest improvements.
- Energy efficiency: Adopt energy-efficient technologies and practices to reduce consumption and lower costs. We help clients identify energy-saving opportunities through data analysis.
- Collaboration and Communication: Â
- Cross-functional teams: Encourage collaboration between departments to foster innovation and problem-solving. Rapid Innovation promotes the use of AI tools that facilitate communication and collaboration across teams.
- Stakeholder engagement: Maintain open lines of communication with stakeholders, including employees, customers, and regulatory bodies.
By focusing on these areas, industries can enhance their operational efficiency, ensure compliance, and contribute to a sustainable future. Rapid Innovation is committed to helping clients achieve these goals through our AI-driven solutions and consulting expertise.
13.1. Implementation Strategies
Effective implementation strategies are crucial for the success of any project, particularly in technology and data-driven initiatives. At Rapid Innovation, we understand that these strategies ensure that projects are executed efficiently and meet their objectives, ultimately leading to greater ROI for our clients.
- Define clear goals: We help clients establish specific, measurable, achievable, relevant, and time-bound (SMART) goals to guide the implementation process, ensuring alignment with their business objectives.
- Stakeholder engagement: Involving all relevant stakeholders early in the process is essential. We facilitate workshops and discussions to gather input and foster buy-in from team members, management, and end-users, which enhances project success.
- Resource allocation: Our team assists in identifying and allocating the necessary resources, including budget, personnel, and technology, to support the implementation, ensuring that clients have what they need to succeed.
- Develop a timeline: We create detailed project timelines that outline key milestones and deadlines, helping clients keep their projects on track and within budget.
- Training and support: Rapid Innovation provides comprehensive training and support for team members, ensuring they are equipped to use new systems or processes effectively, which minimizes downtime and accelerates adoption.
- Monitor progress: We regularly assess the implementation process against established goals and timelines, making adjustments as needed to keep projects aligned with client expectations.
- Risk management: Our experts identify potential risks and develop proactive mitigation strategies, ensuring that clients are prepared for any challenges that may arise during implementation.
- Implementation strategy example: We provide clients with examples of successful implementation strategies that have been tailored to similar projects, helping them visualize the path forward.
- Change implementation strategies: Our approach includes developing change implementation strategies that facilitate smooth transitions and minimize resistance from stakeholders.
- Business strategy implementation: We align our implementation strategies with the overall business strategy implementation to ensure coherence and maximize impact. For more insights on effective strategies, check out our best practices for transformer model development.
13.2. Data Management
Data management is a critical component of any organization, as it involves the collection, storage, and utilization of data to drive decision-making and improve operations. At Rapid Innovation, we implement effective data management practices that enhance data quality, security, and accessibility, ultimately leading to improved business outcomes.
- Data governance: We establish a framework for data governance that defines roles, responsibilities, and policies for data management within the organization, ensuring accountability and compliance.
- Data quality: Our team implements processes to ensure data accuracy, consistency, and completeness. Regular audits and validation checks help maintain high data quality, which is essential for informed decision-making.
- Data storage: We assist clients in choosing appropriate storage solutions, such as cloud storage or on-premises databases, based on their needs and budget, optimizing data accessibility and cost-effectiveness.
- Data integration: Utilizing advanced tools and techniques, we integrate data from various sources, ensuring a unified view of information across the organization, which enhances analytical capabilities.
- Data security: Rapid Innovation implements robust security measures to protect sensitive data from unauthorized access and breaches, including encryption, access controls, and regular security audits.
- Data lifecycle management: We develop policies for managing data throughout its lifecycle, from creation and storage to archiving and deletion, ensuring compliance and efficiency.
- Compliance: Our experts stay informed about relevant data protection regulations and ensure that data management practices comply with legal requirements, reducing the risk of penalties.
13.3. Model Optimization
Model optimization is the process of improving the performance of predictive models to achieve better accuracy and efficiency. At Rapid Innovation, we focus on enhancing model performance, which is particularly important in fields such as machine learning and data analytics, to drive better business results.
- Feature selection: We help clients identify and select the most relevant features that contribute to the model's predictive power, reducing complexity and improving performance.
- Hyperparameter tuning: Our team adjusts the model's hyperparameters to find the optimal settings that enhance performance, employing techniques such as grid search or random search.
- Cross-validation: We utilize cross-validation techniques to assess the model's performance on different subsets of data, ensuring that it generalizes well to unseen data, which is critical for reliable predictions.
- Ensemble methods: Rapid Innovation combines multiple models to improve overall performance. Techniques like bagging, boosting, and stacking lead to more robust predictions, enhancing decision-making capabilities.
- Regularization: We apply regularization techniques to prevent overfitting, ensuring that the model performs well on both training and test datasets, which is essential for maintaining accuracy.
- Performance metrics: Our experts utilize appropriate performance metrics, such as accuracy, precision, recall, and F1 score, to evaluate the model's effectiveness, providing clients with clear insights into model performance.
- Continuous improvement: We regularly review and update models based on new data and changing conditions, ensuring they remain relevant and accurate, which ultimately drives greater ROI for our clients.
- Formulating and implementing strategy: Our approach includes formulating and implementing strategy that aligns with the overall business objectives, ensuring that model optimization efforts contribute to broader goals.
13.4. System Maintenance
System maintenance is crucial for ensuring the longevity and efficiency of any software or hardware system. Regular maintenance helps in identifying potential issues before they escalate into significant problems. Key aspects of system maintenance include:
- Regular Updates: Keeping software up to date is essential, as updates often include security patches, bug fixes, and new features that enhance performance. Rapid Innovation employs automated update management systems to ensure that clients' software remains secure and efficient, thereby reducing vulnerabilities and enhancing user experience.
- Performance Monitoring: Regularly monitoring system performance can help identify bottlenecks or inefficiencies. Tools like performance dashboards can provide insights into system health. Our AI-driven analytics solutions enable real-time performance monitoring, allowing clients to proactively address issues and optimize resource allocation for greater ROI.
- Data Backup: Regular backups are vital to prevent data loss. Implementing automated backup solutions can ensure that data is consistently saved without manual intervention. Rapid Innovation offers tailored backup strategies that align with clients' operational needs, ensuring data integrity and availability.
- Hardware Checks: Periodic checks on hardware components can prevent failures. This includes inspecting hard drives, memory, and other critical components for wear and tear. Our consulting services include hardware assessment and recommendations for upgrades, ensuring that clients' systems are always running at peak performance. This is similar to hvac maintenance, where regular checks on heating systems repair and ac unit maintenance are essential.
- Documentation: Maintaining clear documentation of system configurations, updates, and maintenance activities can help in troubleshooting and future upgrades. We assist clients in developing comprehensive documentation practices that streamline maintenance processes and facilitate smoother transitions during upgrades. For instance, insights from computer vision in sports training can be integrated into documentation practices.
13.5. User Training
User training is essential for maximizing the effectiveness of any system. Proper training ensures that users are familiar with the system's features and can utilize them efficiently. Important elements of user training include:
- Initial Training Sessions: Conducting comprehensive training sessions for new users can help them understand the system's functionalities from the start. Rapid Innovation provides customized training programs that cater to the specific needs of each client, ensuring that users are equipped to leverage the system effectively.
- Ongoing Training: As systems evolve, ongoing training sessions can keep users updated on new features and best practices. Our continuous learning modules ensure that users remain proficient and can adapt to changes seamlessly, enhancing overall productivity.
- User Manuals and Resources: Providing user manuals, FAQs, and online resources can empower users to troubleshoot minor issues independently. We develop user-friendly documentation and resources that facilitate self-service support, reducing dependency on IT teams.
- Feedback Mechanism: Establishing a feedback mechanism allows users to report difficulties they encounter, which can inform future training sessions. Rapid Innovation implements feedback loops that help refine training content and address user concerns promptly.
- Hands-On Practice: Encouraging hands-on practice during training can enhance user confidence and competence in using the system. Our training sessions incorporate practical exercises that simulate real-world scenarios, ensuring users are well-prepared to handle their tasks.
14. Troubleshooting
Troubleshooting is a systematic approach to diagnosing and resolving problems within a system. Effective troubleshooting can minimize downtime and improve user satisfaction. The troubleshooting process involves several steps:
- Identify the Problem: Clearly define the issue. Gathering detailed information from users can help pinpoint the problem more accurately. Our AI tools assist in quickly identifying issues by analyzing user reports and system logs.
- Check for Common Issues: Many problems have common causes. Reviewing a checklist of frequent issues can expedite the troubleshooting process. Rapid Innovation provides clients with a curated list of common issues and solutions, streamlining the troubleshooting process. This is akin to checking for common hvac servicing near me issues.
- Use Diagnostic Tools: Employing diagnostic tools can help identify system errors or performance issues. These tools can provide valuable insights into the root cause of problems. We leverage advanced diagnostic tools that utilize machine learning to predict and identify potential failures before they impact operations.
- Consult Documentation: Referring to system documentation can provide guidance on resolving specific issues. This includes user manuals, troubleshooting guides, and online resources. Our comprehensive documentation practices ensure that clients have access to the information they need for effective troubleshooting.
- Implement Solutions: Once the problem is identified, implement the appropriate solution. This may involve applying patches, reconfiguring settings, or replacing faulty hardware. Rapid Innovation supports clients in executing solutions efficiently, minimizing disruption to their operations. This may also involve hvac system repair or ac unit repair.
- Test the Solution: After implementing a solution, testing is crucial to ensure that the issue is resolved and that no new problems have arisen. We emphasize rigorous testing protocols to validate solutions and ensure system stability.
- Document the Process: Keeping a record of the troubleshooting process can help in future incidents. Documenting what worked and what didn’t can serve as a valuable reference for similar issues. Our approach includes creating detailed logs of troubleshooting efforts, which can enhance future incident response and knowledge sharing. This is similar to maintaining records for furnace maintenance or air conditioning unit maintenance.
14.1. Common Issues
In any system or process, common issues can arise that hinder performance and efficiency. Identifying these issues early can save time and resources. Here are some prevalent problems:
- Software Bugs: Glitches in the code can lead to unexpected behavior, crashes, or data loss, impacting user experience and operational efficiency.
- Hardware Failures: Components like hard drives, RAM, or power supplies can fail, causing system downtime and disrupting business continuity. This is particularly relevant for systems like Dell, where users may experience slow performance due to hardware issues.
- Network Connectivity Problems: Issues with routers, switches, or internet service can disrupt communication and access to resources, affecting productivity. For instance, Citrix network latency can significantly impact user experience.
- User Errors: Mistakes made by users, such as incorrect data entry or misconfiguration, can lead to significant operational challenges and data integrity issues.
- Compatibility Issues: Software or hardware may not work well together, leading to performance degradation or failures that can stall projects. This is often seen in environments running Windows Server performance monitor tools.
- Security Vulnerabilities: Weaknesses in the system can be exploited by malicious actors, leading to data breaches or system compromise, which can have severe financial and reputational repercussions.
Understanding these common issues is crucial for maintaining system integrity and performance, especially in contexts like Windows Server 2016 slow performance or Windows Server 2019 performance issues. Rapid Innovation is here to help you navigate these challenges effectively.
14.2. Diagnostic Tools

‍
To effectively address issues, diagnostic tools are essential. These tools help identify, analyze, and troubleshoot problems within systems. Here are some widely used diagnostic tools:
- Network Analyzers: Tools like
Wireshark
allow users to capture and analyze network traffic, helping to identify connectivity issues that could disrupt operations. - System Monitors: Applications such as
Task Manager
orResource Monitor
provide insights into CPU, memory, and disk usage, helping to pinpoint performance bottlenecks that could affect productivity. This is particularly useful for diagnosing Windows Server performance issues. - Error Log Analyzers: Tools that review system logs can help identify recurring errors or warnings that may indicate underlying issues, allowing for proactive maintenance.
- Hardware Diagnostic Tools: Utilities like
MemTest86
orCrystalDiskInfo
can assess the health of RAM and hard drives, ensuring that hardware failures are addressed before they impact operations. This is crucial for systems experiencing slow performance, such as Sage 100 or Epicor. - Performance Profilers: Tools like
VisualVM
orJProfiler
help developers analyze application performance, identifying slow methods or memory leaks that could hinder efficiency.
Using these diagnostic tools can streamline the troubleshooting process and lead to quicker resolutions, ultimately enhancing your return on investment (ROI).
14.3. Resolution Procedures
Once issues are identified, having a structured resolution procedure is vital for effective problem-solving. Here are steps to follow in resolution procedures:
- Identify the Problem: Clearly define the issue based on symptoms and diagnostic results to ensure accurate resolution. For example, identifying whether the issue is related to Windows Server performance troubleshooting or specific applications like Epicor.
- Gather Information: Collect relevant data, including error messages, logs, and user reports, to understand the context of the problem and its potential impact on business operations.
- Develop a Plan: Create a step-by-step approach to address the issue, considering potential impacts and necessary resources to minimize disruption. This may include addressing system performance issues or slow performance in Windows Server 2019.
- Implement the Solution: Execute the plan, making necessary changes or repairs to resolve the issue efficiently.
- Test the Solution: Verify that the implemented solution effectively resolves the problem without introducing new issues, ensuring system stability.
- Document the Process: Record the problem, resolution steps, and outcomes for future reference and to aid in similar issues, fostering a culture of continuous improvement.
- Monitor the System: After resolution, keep an eye on the system to ensure stability and performance, allowing for quick adjustments if needed. This is especially important for systems that have previously experienced slow performance.
Following these resolution procedures can enhance efficiency and minimize downtime, leading to a more robust system overall. At Rapid Innovation, we leverage our expertise to help clients implement these best practices, ensuring that your systems operate at peak performance and contribute to your business goals effectively.
14.4. Support Resources
Support resources are essential for ensuring that users can effectively utilize a system or service. These resources provide assistance, guidance, and troubleshooting to enhance user experience and system performance.
- Documentation: Comprehensive user manuals, FAQs, and online help guides are crucial. They should be easily accessible and cover common issues, installation procedures, and troubleshooting steps.
- Customer Support: A dedicated support team can assist users with technical issues. This can include: Â
- Live chat options for immediate assistance
- Email support for detailed inquiries
- Phone support for urgent problems
- Community Forums: User forums can be a valuable resource for sharing experiences and solutions. They allow users to: Â
- Post questions and receive answers from other users
- Share tips and best practices
- Discuss updates and features
- Training and Tutorials: Offering training sessions, webinars, and video tutorials can help users understand the system better. This can include: Â
- Step-by-step guides on using features
- Best practices for maximizing system efficiency
- Knowledge Base: A searchable database of articles and resources can help users find solutions quickly. This should include: Â
- Troubleshooting guides
- System updates and patch notes
- Tips for optimizing performance including the use of AI agents for technical troubleshooting.
14.5. System Recovery
System recovery is a critical aspect of IT management, ensuring that systems can be restored to operational status after a failure or disaster. Effective recovery strategies minimize downtime and data loss.
- Backup Solutions: Regular backups are essential for data integrity. This can involve: Â
- Automated backups to cloud storage
- Local backups on external drives
- Incremental backups to save only changes made since the last backup
- Disaster Recovery Plans: A well-defined disaster recovery plan outlines steps to take in case of a system failure. Key components include: Â
- Identification of critical systems and data
- Recovery time objectives (RTO) and recovery point objectives (RPO)
- Roles and responsibilities for recovery tasks
- Testing Recovery Procedures: Regularly testing recovery procedures ensures they work as intended. This can involve: Â
- Simulating disaster scenarios
- Conducting drills to practice recovery steps
- Updating the recovery plan based on test results
- Redundancy and Failover Systems: Implementing redundancy can prevent system failures. This includes: Â
- Using multiple servers to handle load
- Setting up failover systems that automatically switch to backup resources
- Documentation of Recovery Processes: Clear documentation of recovery processes is vital. This should include: Â
- Step-by-step instructions for restoring systems
- Contact information for support teams
- Logs of previous recovery attempts for reference
15. Case Studies
Case studies provide real-world examples of how systems and strategies are implemented, showcasing successes and lessons learned. They serve as valuable resources for understanding best practices and potential pitfalls.
- Industry-Specific Examples: Case studies can highlight how different industries utilize systems. For instance: Â
- Healthcare organizations may focus on patient data management and compliance.
- Retail businesses might emphasize inventory management and customer engagement.
- Success Stories: Highlighting successful implementations can inspire confidence. Key elements include: Â
- Overview of the challenges faced
- Description of the solutions implemented
- Measurable outcomes, such as increased efficiency or reduced costs
- Lessons Learned: Analyzing failures or challenges faced during implementation can provide insights. This can involve: Â
- Identifying what went wrong and why
- Discussing adjustments made to improve future implementations
- Sharing strategies for overcoming similar challenges
- Comparative Analysis: Comparing different approaches within case studies can reveal best practices. This can include: Â
- Evaluating the effectiveness of various support resources
- Analyzing recovery strategies across different organizations
- Discussing the impact of user training on system adoption
- Future Trends: Case studies can also explore emerging trends and technologies. This may involve: Â
- Adoption of AI and machine learning in support resources
- Innovations in backup and recovery solutions
- Evolving user needs and expectations in system design.
At Rapid Innovation, we leverage these support resources and recovery strategies to ensure our clients achieve their business goals efficiently and effectively. By implementing tailored AI solutions, we help organizations enhance their operational capabilities, leading to greater ROI and sustained growth.
15.1. Manufacturing Implementation
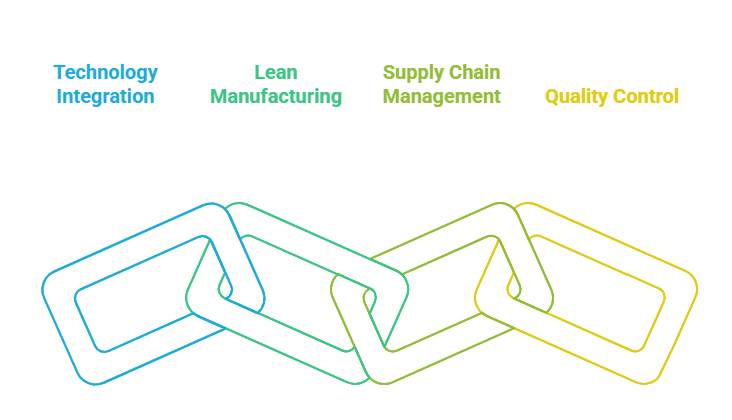
‍
Manufacturing implementation refers to the process of integrating new technologies, systems, and methodologies into existing manufacturing operations. This can involve the adoption of advanced manufacturing techniques, automation, and data analytics to enhance productivity and efficiency.
- Key components of manufacturing implementation include: Â
- Technology Integration: Incorporating robotics, IoT devices, and AI to streamline production processes. Rapid Innovation specializes in deploying AI-driven solutions that optimize machine learning algorithms for predictive maintenance, significantly reducing downtime and enhancing productivity.
- Lean Manufacturing: Applying lean principles to minimize waste and optimize resource utilization. Our consulting services guide clients in implementing lean methodologies, including implementing lean manufacturing, implementation of lean, and lean management implementation, resulting in substantial cost savings and improved operational workflows.
- Supply Chain Management: Enhancing coordination and communication across the supply chain to improve delivery times and reduce costs. By leveraging AI analytics, we help clients forecast demand more accurately, leading to better inventory management and reduced excess stock.
- Quality Control: Implementing rigorous quality assurance processes to ensure product consistency and reliability. Our AI solutions enable real-time monitoring of production quality, allowing for immediate adjustments and minimizing defects.
- Benefits of effective manufacturing implementation: Â
- Increased operational efficiency
- Reduced production costs
- Enhanced product quality
- Greater flexibility to adapt to market changes
Successful manufacturing implementation often requires a cultural shift within the organization, emphasizing continuous improvement and employee engagement. Companies like Siemens and General Electric have successfully integrated advanced manufacturing technologies, showcasing the potential for increased competitiveness in the global market. Rapid Innovation is here to support your journey towards achieving these goals through tailored AI solutions, including implementing 5s in manufacturing and 5s implementation in manufacturing industry, as well as real-time energy demand balancing.
15.2. Energy Sector Applications
The energy sector is undergoing a significant transformation driven by technological advancements and the need for sustainable practices. Applications in this sector focus on improving efficiency, reducing emissions, and integrating renewable energy sources.
- Key applications in the energy sector include: Â
- Smart Grids: Utilizing digital technology to monitor and manage energy flows, enhancing reliability and efficiency. Rapid Innovation can assist in developing AI algorithms that optimize energy distribution and consumption patterns.
- Renewable Energy Integration: Incorporating solar, wind, and other renewable sources into the energy mix to reduce dependence on fossil fuels. Our expertise in AI can help clients analyze and predict energy generation from renewable sources, facilitating better integration into existing grids.
- Energy Storage Solutions: Developing advanced battery technologies to store excess energy for use during peak demand periods. We provide consulting on AI-driven energy management systems that optimize storage and usage.
- Demand Response Programs: Implementing strategies to adjust consumer energy usage during peak times, helping to balance supply and demand. Our AI solutions can analyze consumption data to create effective demand response strategies.
- Benefits of energy sector applications: Â
- Reduced greenhouse gas emissions
- Improved energy efficiency
- Enhanced energy security
- Lower energy costs for consumers
Companies like Tesla and NextEra Energy are leading the way in energy sector applications, demonstrating how innovation can drive sustainability and economic growth. The global shift towards cleaner energy sources is expected to create new opportunities and challenges in the coming years, and Rapid Innovation is positioned to help clients navigate this landscape effectively.
15.3. Process Industry Examples
The process industry encompasses a wide range of sectors, including chemicals, pharmaceuticals, food and beverage, and oil and gas. These industries rely on continuous processes to convert raw materials into finished products, making efficiency and safety paramount.
- Examples of process industry applications include: Â
- Chemical Manufacturing: Utilizing advanced process control systems to optimize chemical reactions and improve yield. Rapid Innovation offers AI solutions that enhance process control, leading to higher yields and lower operational costs.
- Pharmaceutical Production: Implementing stringent quality control measures to ensure product safety and efficacy. Our AI-driven analytics can monitor production processes in real-time, ensuring compliance with regulatory standards.
- Food Processing: Adopting automation and robotics to enhance production speed and maintain hygiene standards. We provide consulting on integrating AI technologies that improve food safety and operational efficiency, including implementation of six sigma in manufacturing industry.
- Oil and Gas Refining: Employing advanced analytics to monitor equipment performance and predict maintenance needs. Our predictive maintenance solutions help clients reduce downtime and extend equipment life.
- Benefits of innovations in the process industry: Â
- Enhanced operational efficiency
- Improved product quality and safety
- Reduced environmental impact
- Increased compliance with regulatory standards
Companies like BASF and Dow Chemical are examples of leaders in the process industry, leveraging technology to drive innovation and sustainability. The ongoing evolution of the process industry is critical for meeting global demands while addressing environmental concerns, and Rapid Innovation is committed to providing the AI expertise necessary to achieve these objectives, including kaizen implementation in manufacturing and six sigma implementation in manufacturing industry.
15.4. ROI Analysis
Return on Investment (ROI) analysis is a critical component for evaluating the effectiveness of any business initiative. It helps organizations determine the profitability of their investments and make informed decisions about future projects.
- Definition: ROI is a financial metric that compares the gain or loss from an investment relative to its cost.
- Calculation: The basic formula for ROI is:
language="language-plaintext"``` -a1b2c3- Â ROI = (Net Profit / Cost of Investment) x 100
- Importance:
- Helps in assessing the financial viability of projects.
- Aids in comparing different investment opportunities.
- Provides insights into resource allocation and budgeting.
At Rapid Innovation, we leverage advanced AI analytics to enhance the accuracy of ROI assessments. For instance, by utilizing predictive modeling, we can help clients forecast potential returns based on historical data and market trends, leading to more informed investment decisions.
To conduct a thorough ROI analysis, consider the following steps:
- Identify the investment costs, including direct and indirect expenses.
- Estimate the expected returns, which can include increased revenue, cost savings, or enhanced productivity.
- Analyze the time frame for realizing these returns, as timing can significantly impact ROI.
- Use historical data and market trends to validate assumptions and projections.
A well-executed ROI analysis can lead to better strategic planning and improved financial performance. This includes conducting a detailed roi analysis marketing to understand the impact of marketing campaigns on overall profitability. Additionally, understanding the roi analysis meaning is crucial for stakeholders to grasp the significance of the findings.
Examples such as a return on investment analysis example can provide practical insights into how ROI is calculated and interpreted. Furthermore, utilizing roi data analytics and roi data analysis can enhance the depth of the analysis, allowing for more nuanced decision-making.
The Dupont formula roi and the dupont roi model are also valuable tools that can help break down the components of ROI, providing a clearer picture of financial performance. By incorporating these methodologies, organizations can refine their financial roi analysis and ensure that their investments yield the desired returns. For those interested in enhancing their financial strategies, our AI banking solutions can provide significant insights and support. Additionally, exploring the benefits and advantages of multi-agent AI developments can further enhance decision-making processes.
15.5. Success Metrics
Success metrics are quantifiable measures used to evaluate the performance of a project, initiative, or business strategy. They provide a framework for assessing whether objectives are being met and help guide decision-making.
- Definition: Success metrics are specific indicators that reflect the effectiveness of a strategy or project.
- Types of Success Metrics: Â
- Financial Metrics: Revenue growth, profit margins, and cost reductions.
- Operational Metrics: Efficiency ratios, production output, and turnaround times.
- Customer Metrics: Customer satisfaction scores, retention rates, and net promoter scores (NPS).
At Rapid Innovation, we assist clients in defining and tracking success metrics tailored to their unique business objectives. By integrating AI-driven insights, we enable organizations to monitor performance in real-time, ensuring they remain agile and responsive to market changes.
When establishing success metrics, consider the following:
- Align metrics with business goals to ensure relevance.
- Use both leading and lagging indicators to get a comprehensive view of performance.
- Regularly review and adjust metrics based on changing business conditions and objectives.
By focusing on the right success metrics, organizations can drive continuous improvement and achieve long-term success.
16. Future Developments
Future developments refer to the anticipated changes and advancements that can impact a business or industry. Staying ahead of these trends is crucial for maintaining competitiveness and ensuring sustainable growth.
- Importance of Future Developments:
- Helps organizations adapt to changing market conditions.
- Encourages innovation and the adoption of new technologies.
- Provides insights into potential risks and opportunities.
Key areas to watch for future developments include:
- Technological Advancements: Emerging technologies such as artificial intelligence, machine learning, and blockchain are reshaping industries. Rapid Innovation is at the forefront of these advancements, helping clients integrate AI solutions that drive efficiency and innovation.
- Market Trends: Shifts in consumer behavior, preferences, and demographics can influence product offerings and marketing strategies.
- Regulatory Changes: New laws and regulations can impact operational practices and compliance requirements.
To prepare for future developments, organizations should:
- Invest in research and development to foster innovation.
- Monitor industry trends and competitor activities.
- Engage in scenario planning to anticipate potential challenges and opportunities.
By proactively addressing future developments, businesses can position themselves for success in an ever-evolving landscape. Rapid Innovation is committed to guiding clients through these changes, ensuring they leverage the latest technologies to achieve their business goals.
16.1. Technology Trends
Technology trends are constantly evolving, shaping the way businesses operate and interact with customers. Staying updated with these trends is crucial for organizations aiming to maintain a competitive edge. Some key technology trends include:
- Artificial Intelligence (AI) and Machine Learning (ML): AI and ML are transforming industries by automating processes, enhancing decision-making, and personalizing customer experiences. At Rapid Innovation, we help businesses leverage AI for predictive analytics, chatbots, and customer service automation, ultimately driving greater ROI through improved efficiency and customer satisfaction. This includes exploring AI trends and artificial intelligence trends that are emerging in various sectors. For more insights, you can read about learning from real-world AI implementations.
- Cloud Computing: The shift to cloud-based solutions allows organizations to scale operations efficiently, reduce costs, and improve collaboration. Rapid Innovation assists clients in adopting cloud services that provide flexibility and accessibility, enabling remote work and real-time data sharing, which can lead to significant cost savings and operational improvements. This aligns with the latest technology trends in cloud computing.
- Internet of Things (IoT): IoT connects devices and systems, allowing for smarter operations and data collection. Industries like manufacturing and healthcare are utilizing IoT for real-time monitoring and predictive maintenance. Rapid Innovation supports clients in implementing IoT solutions that enhance operational efficiency and reduce downtime, resulting in higher productivity and ROI. This is part of the broader technology trends in healthcare technology trends and emerging technologies in the healthcare industry.
- Blockchain Technology: Beyond cryptocurrencies, blockchain is being adopted for secure transactions, supply chain transparency, and data integrity. Rapid Innovation guides organizations in leveraging blockchain's decentralized nature to enhance security and trust among stakeholders, ultimately leading to more reliable business operations. This technology is also part of the latest tech trends.
- 5G Technology: The rollout of 5G networks is set to revolutionize connectivity, enabling faster data transfer and supporting the growth of IoT devices. Rapid Innovation helps clients harness the potential of 5G technology to enhance mobile experiences and facilitate innovations across various sectors, driving growth and competitive advantage. This is a significant aspect of current trends and technologies.
16.2. Enhanced Analytics
Enhanced analytics refers to the advanced techniques and tools used to analyze data more effectively, providing deeper insights and driving better business decisions. Key aspects of enhanced analytics include:
- Predictive Analytics: This technique uses historical data and statistical algorithms to forecast future outcomes. Rapid Innovation empowers businesses to anticipate customer behavior, optimize inventory, and improve marketing strategies, leading to increased sales and customer retention. This is particularly relevant in the context of trends of AI.
- Real-Time Analytics: The ability to analyze data as it is generated allows organizations to make immediate decisions. Rapid Innovation enables clients in industries like finance and e-commerce to leverage real-time analytics, where timely insights can lead to competitive advantages and improved operational performance.
- Self-Service Analytics: Empowering non-technical users to access and analyze data without relying on IT teams enhances agility. Rapid Innovation provides self-service tools that enable employees to generate reports and insights, fostering a data-driven culture that enhances decision-making across the organization.
- Data Visualization: Effective data visualization tools help in presenting complex data in an understandable format. Rapid Innovation assists clients in implementing visualization solutions, such as dashboards and charts, making it easier for stakeholders to grasp insights quickly and make informed decisions.
- Natural Language Processing (NLP): NLP enables users to interact with data using natural language queries. Rapid Innovation simplifies data analysis for clients by integrating NLP technologies, allowing users to ask questions and receive insights without needing advanced technical skills, thus democratizing data access.
16.3. Integration Capabilities
Integration capabilities refer to the ability of different systems, applications, and data sources to work together seamlessly. Effective integration is essential for optimizing workflows and enhancing productivity. Key points regarding integration capabilities include:
- API Integration: Application Programming Interfaces (APIs) allow different software applications to communicate with each other. Rapid Innovation helps businesses connect various tools and platforms through API integration, streamlining processes and improving data flow, which enhances overall efficiency.
- Data Integration: Combining data from multiple sources into a unified view is crucial for accurate analysis. Rapid Innovation provides data integration tools that help organizations consolidate information, ensuring that decision-makers have access to comprehensive insights for better strategic planning.
- Cloud Integration: As businesses increasingly adopt cloud solutions, integrating on-premises systems with cloud applications becomes vital. Rapid Innovation ensures that data is synchronized across platforms through cloud integration, enhancing collaboration and operational efficiency.
- Enterprise Resource Planning (ERP) Integration: Integrating ERP systems with other business applications allows for better resource management and operational efficiency. Rapid Innovation facilitates this integration, providing a holistic view of business processes that supports informed decision-making.
- Workflow Automation: Integration capabilities enable the automation of repetitive tasks across different systems. Rapid Innovation helps clients implement workflow automation solutions that reduce manual effort, minimize errors, and allow employees to focus on higher-value activities, ultimately driving productivity and ROI. This is increasingly important in the context of technology industry 4.0 and upcoming IT technologies.
16.4. AI Advancements
Artificial Intelligence (AI) has made significant strides in recent years, transforming various sectors and enhancing operational efficiencies. The advancements in AI, including the latest developments in AI and recent developments in artificial intelligence, can be categorized into several key areas:
- Machine Learning (ML): This subset of AI focuses on algorithms that allow computers to learn from and make predictions based on data. ML is widely used in applications such as recommendation systems, fraud detection, and predictive analytics. At Rapid Innovation, we leverage ML to help clients optimize their operations, leading to improved decision-making and increased ROI. The most advanced AI techniques in ML are being utilized to drive these improvements.
- Natural Language Processing (NLP): NLP enables machines to understand and interpret human language. This technology powers virtual assistants, chatbots, and translation services, making human-computer interaction more intuitive. Our expertise in NLP allows us to develop solutions that enhance customer engagement and streamline communication processes for our clients, reflecting the latest advancements in AI technology.
- Computer Vision: AI advancements in computer vision allow machines to interpret and process visual information. This technology is crucial in areas like autonomous vehicles, facial recognition, and medical imaging. Rapid Innovation utilizes computer vision to create innovative applications that improve accuracy and efficiency in various industries, showcasing the most advanced AI capabilities.
- Robotics: AI-driven robotics has revolutionized manufacturing and logistics. Robots equipped with AI can perform complex tasks, adapt to new environments, and work alongside humans safely. We assist clients in integrating AI robotics into their operations, resulting in significant cost savings and productivity gains. The most advanced artificial intelligence robots are now capable of performing tasks that were once thought to be exclusive to humans.
- Generative AI: This emerging field focuses on creating new content, such as images, music, and text, based on learned patterns. Generative AI has applications in creative industries, gaming, and content creation. Rapid Innovation helps clients harness the power of generative AI to enhance their creative processes and deliver unique offerings to their customers, reflecting the latest advancements in artificial intelligence.
- AI Ethics and Governance: As AI technology evolves, so does the need for ethical considerations. Organizations are increasingly focusing on responsible AI practices, ensuring transparency, fairness, and accountability in AI systems. At Rapid Innovation, we guide clients in implementing ethical AI frameworks that align with their business values and regulatory requirements, addressing the recent AI advancements in governance. For more on this topic, you can read about leveraging generative AI and ethical frameworks for sustainable innovation.
The impact of these advancements is profound, leading to increased productivity, enhanced customer experiences, and the creation of new business models. According to a report by McKinsey, AI could potentially add $13 trillion to the global economy by 2030. By partnering with Rapid Innovation, businesses can effectively leverage these advancements, including the most advanced AI in the world, to achieve greater ROI and stay ahead in a competitive landscape.
16.5. Industry Evolution
The evolution of various industries is closely tied to technological advancements, particularly in AI and digital transformation. This evolution can be observed through several trends:
- Digital Transformation: Companies are increasingly adopting digital technologies to improve operations, enhance customer engagement, and drive innovation. This shift is evident in sectors like retail, where e-commerce has surged. Rapid Innovation supports clients in navigating their digital transformation journeys, ensuring they remain competitive and responsive to market demands, especially with the latest developments in AI.
- Data-Driven Decision Making: Organizations are leveraging big data analytics to inform strategic decisions. By analyzing consumer behavior and market trends, businesses can tailor their offerings and improve customer satisfaction. Our data analytics solutions empower clients to make informed decisions that drive growth and profitability, reflecting the recent advances in AI.
- Remote Work and Collaboration: The rise of remote work has transformed workplace dynamics. Tools like video conferencing, project management software, and cloud services have become essential for collaboration and productivity. Rapid Innovation provides clients with AI-driven collaboration tools that enhance team productivity and streamline workflows, showcasing the latest advancements in AI technology.
- Sustainability Initiatives: Industries are evolving to prioritize sustainability. Companies are adopting eco-friendly practices, reducing waste, and investing in renewable energy sources to meet consumer demand for responsible business practices. We help clients implement AI solutions that optimize resource usage and promote sustainable practices, reflecting the recent AI innovations in this area.
- Personalization: Businesses are increasingly focusing on personalized experiences. By utilizing AI and data analytics, companies can offer tailored products and services, enhancing customer loyalty and engagement. Rapid Innovation assists clients in developing personalized marketing strategies that resonate with their target audiences, leveraging the most advanced AI techniques.
- Agile Business Models: The need for adaptability has led to the rise of agile business models. Companies are restructuring their operations to respond quickly to market changes and consumer demands. Our consulting services guide clients in adopting agile methodologies that foster innovation and responsiveness, reflecting the latest advancements in AI.
The evolution of industries is not just about technology; it also involves cultural shifts and changing consumer expectations. As industries continue to adapt, the integration of AI and other technologies will play a crucial role in shaping the future landscape. According to a report by Deloitte, 70% of organizations are prioritizing digital transformation initiatives to stay competitive in the evolving market. Rapid Innovation is committed to helping clients navigate this transformation effectively, ensuring they achieve their business goals efficiently and effectively, utilizing the most advanced AI solutions available.
‍